4.2 Sleep and Why We Sleep
Learning objectives.
By the end of this section, you will be able to:
- Describe areas of the brain involved in sleep
- Understand hormone secretions associated with sleep
- Describe several theories aimed at explaining the function of sleep
- Name and describe three theories about why we dream
We spend approximately one-third of our lives sleeping. Given the average life expectancy for U.S. citizens falls between 73 and 79 years old (Singh & Siahpush, 2006), we can expect to spend approximately 25 years of our lives sleeping. Some animals never sleep (e.g., some fish and amphibian species); other animals sleep very little without apparent negative consequences (e.g., giraffes); yet some animals (e.g., rats) die after two weeks of sleep deprivation (Siegel, 2008). Why do we devote so much time to sleeping? Is it absolutely essential that we sleep? This section will consider these questions and explore various explanations for why we sleep.
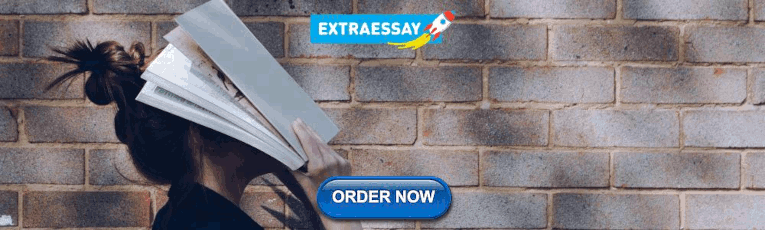
What is Sleep?
You have read that sleep is distinguished by low levels of physical activity and reduced sensory awareness. As discussed by Siegel (2008), a definition of sleep must also include mention of the interplay of the circadian and homeostatic mechanisms that regulate sleep. Homeostatic regulation of sleep is evidenced by sleep rebound following sleep deprivation. Sleep rebound refers to the fact that a sleep-deprived individual will fall asleep more quickly during subsequent opportunities for sleep. Sleep is characterized by certain patterns of activity of the brain that can be visualized using electroencephalography (EEG), and different phases of sleep can be differentiated using EEG as well.
Sleep-wake cycles seem to be controlled by multiple brain areas acting in conjunction with one another. Some of these areas include the thalamus, the hypothalamus, and the pons. As already mentioned, the hypothalamus contains the SCN—the biological clock of the body—in addition to other nuclei that, in conjunction with the thalamus, regulate slow-wave sleep. The pons is important for regulating rapid eye movement (REM) sleep (National Institutes of Health, n.d.).
Sleep is also associated with the secretion and regulation of a number of hormones from several endocrine glands including: melatonin, follicle stimulating hormone (FSH), luteinizing hormone (LH), and growth hormone (National Institutes of Health, n.d.). You have read that the pineal gland releases melatonin during sleep ( Figure 4.6 ). Melatonin is thought to be involved in the regulation of various biological rhythms and the immune system (Hardeland et al., 2006). During sleep, the pituitary gland secretes both FSH and LH which are important in regulating the reproductive system (Christensen et al., 2012; Sofikitis et al., 2008). The pituitary gland also secretes growth hormone, during sleep, which plays a role in physical growth and maturation as well as other metabolic processes (Bartke, Sun, & Longo, 2013).
Why Do We Sleep?
Given the central role that sleep plays in our lives and the number of adverse consequences that have been associated with sleep deprivation, one would think that we would have a clear understanding of why it is that we sleep. Unfortunately, this is not the case; however, several hypotheses have been proposed to explain the function of sleep.
Adaptive Function of Sleep
One popular hypothesis of sleep incorporates the perspective of evolutionary psychology. Evolutionary psychology is a discipline that studies how universal patterns of behavior and cognitive processes have evolved over time as a result of natural selection . Variations and adaptations in cognition and behavior make individuals more or less successful in reproducing and passing their genes to their offspring. One hypothesis from this perspective might argue that sleep is essential to restore resources that are expended during the day. Just as bears hibernate in the winter when resources are scarce, perhaps people sleep at night to reduce their energy expenditures. While this is an intuitive explanation of sleep, there is little research that supports this explanation. In fact, it has been suggested that there is no reason to think that energetic demands could not be addressed with periods of rest and inactivity (Frank, 2006; Rial et al., 2007), and some research has actually found a negative correlation between energetic demands and the amount of time spent sleeping (Capellini, Barton, McNamara, Preston, & Nunn, 2008).
Another evolutionary hypothesis of sleep holds that our sleep patterns evolved as an adaptive response to predatory risks, which increase in darkness. Thus we sleep in safe areas to reduce the chance of harm. Again, this is an intuitive and appealing explanation for why we sleep. Perhaps our ancestors spent extended periods of time asleep to reduce attention to themselves from potential predators. Comparative research indicates, however, that the relationship that exists between predatory risk and sleep is very complex and equivocal. Some research suggests that species that face higher predatory risks sleep fewer hours than other species (Capellini et al., 2008), while other researchers suggest there is no relationship between the amount of time a given species spends in deep sleep and its predation risk (Lesku, Roth, Amlaner, & Lima, 2006).
It is quite possible that sleep serves no single universally adaptive function, and different species have evolved different patterns of sleep in response to their unique evolutionary pressures. While we have discussed the negative outcomes associated with sleep deprivation, it should be pointed out that there are many benefits that are associated with adequate amounts of sleep. A few such benefits listed by the National Sleep Foundation (n.d.) include maintaining health, lowering stress levels, improving mood, and increasing motor coordination, as well as a number of benefits related to cognition and memory formation.
Cognitive Function of Sleep
Another theory regarding why we sleep involves sleep’s importance for cognitive function and memory formation (Rattenborg, Lesku, Martinez-Gonzalez, & Lima, 2007). Indeed, we know sleep deprivation results in disruptions in cognition and memory deficits (Brown, 2012), leading to impairments in our abilities to maintain attention, make decisions, and recall long-term memories. Moreover, these impairments become more severe as the amount of sleep deprivation increases (Alhola & Polo-Kantola, 2007). Furthermore, slow-wave sleep after learning a new task can improve resultant performance on that task (Huber, Ghilardi, Massimini, & Tononi, 2004) and seems essential for effective memory formation (Stickgold, 2005). Understanding the impact of sleep on cognitive function should help you understand that cramming all night for a test may not be effective and can even prove counterproductive.
Link to Learning
Watch this brief video that gives sleep tips for college students to learn more.
Getting the optimal amount of sleep has also been associated with other cognitive benefits. Research indicates that included among these possible benefits are increased capacities for creative thinking (Cai, Mednick, Harrison, Kanady, & Mednick, 2009; Wagner, Gais, Haider, Verleger, & Born, 2004), language learning (Fenn, Nusbaum, & Margoliash, 2003; Gómez, Bootzin, & Nadel, 2006), and inferential judgments (Ellenbogen, Hu, Payne, Titone, & Walker, 2007). It is possible that even the processing of emotional information is influenced by certain aspects of sleep (Walker, 2009).
Watch this brief video about the relationship between sleep and memory to learn more.
As an Amazon Associate we earn from qualifying purchases.
This book may not be used in the training of large language models or otherwise be ingested into large language models or generative AI offerings without OpenStax's permission.
Want to cite, share, or modify this book? This book uses the Creative Commons Attribution License and you must attribute OpenStax.
Access for free at https://openstax.org/books/psychology-2e/pages/1-introduction
- Authors: Rose M. Spielman, William J. Jenkins, Marilyn D. Lovett
- Publisher/website: OpenStax
- Book title: Psychology 2e
- Publication date: Apr 22, 2020
- Location: Houston, Texas
- Book URL: https://openstax.org/books/psychology-2e/pages/1-introduction
- Section URL: https://openstax.org/books/psychology-2e/pages/4-2-sleep-and-why-we-sleep
© Jan 6, 2024 OpenStax. Textbook content produced by OpenStax is licensed under a Creative Commons Attribution License . The OpenStax name, OpenStax logo, OpenStax book covers, OpenStax CNX name, and OpenStax CNX logo are not subject to the Creative Commons license and may not be reproduced without the prior and express written consent of Rice University.
Thank you for visiting nature.com. You are using a browser version with limited support for CSS. To obtain the best experience, we recommend you use a more up to date browser (or turn off compatibility mode in Internet Explorer). In the meantime, to ensure continued support, we are displaying the site without styles and JavaScript.
- View all journals
- My Account Login
- Explore content
- About the journal
- Publish with us
- Sign up for alerts
- Open access
- Published: 01 October 2019
Sleep quality, duration, and consistency are associated with better academic performance in college students
- Kana Okano 1 ,
- Jakub R. Kaczmarzyk 1 ,
- Neha Dave 2 ,
- John D. E. Gabrieli 1 &
- Jeffrey C. Grossman ORCID: orcid.org/0000-0003-1281-2359 3
npj Science of Learning volume 4 , Article number: 16 ( 2019 ) Cite this article
427k Accesses
119 Citations
1716 Altmetric
Metrics details
Although numerous survey studies have reported connections between sleep and cognitive function, there remains a lack of quantitative data using objective measures to directly assess the association between sleep and academic performance. In this study, wearable activity trackers were distributed to 100 students in an introductory college chemistry class (88 of whom completed the study), allowing for multiple sleep measures to be correlated with in-class performance on quizzes and midterm examinations. Overall, better quality, longer duration, and greater consistency of sleep correlated with better grades. However, there was no relation between sleep measures on the single night before a test and test performance; instead, sleep duration and quality for the month and the week before a test correlated with better grades. Sleep measures accounted for nearly 25% of the variance in academic performance. These findings provide quantitative, objective evidence that better quality, longer duration, and greater consistency of sleep are strongly associated with better academic performance in college. Gender differences are discussed.
Similar content being viewed by others
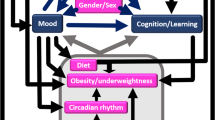
Effect of sleep and mood on academic performance—at interface of physiology, psychology, and education
Kosha J. Mehta
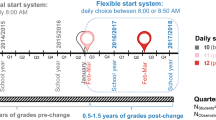
A 4-year longitudinal study investigating the relationship between flexible school starts and grades
Anna M. Biller, Carmen Molenda, … Eva C. Winnebeck
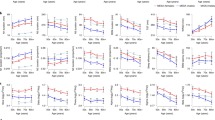
Macro and micro sleep architecture and cognitive performance in older adults
Ina Djonlagic, Sara Mariani, … Shaun M. Purcell
Introduction
The relationship between sleep and cognitive function has been a topic of interest for over a century. Well-controlled sleep studies conducted with healthy adults have shown that better sleep is associated with a myriad of superior cognitive functions, 1 , 2 , 3 , 4 , 5 , 6 including better learning and memory. 7 , 8 These effects have been found to extend beyond the laboratory setting such that self-reported sleep measures from students in the comfort of their own homes have also been found to be associated with academic performance. 9 , 10 , 11 , 12 , 13
Sleep is thought to play a crucial and specific role in memory consolidation. Although the exact mechanisms behind the relationship between sleep, memory, and neuro-plasticity are yet unknown, the general understanding is that specific synaptic connections that were active during awake-periods are strengthened during sleep, allowing for the consolidation of memory, and synaptic connections that were inactive are weakened. 5 , 14 , 15 Thus, sleep provides an essential function for memory consolidation (allowing us to remember what has been studied), which in turn is critical for successful academic performance.
Beyond the effects of sleep on memory consolidation, lack of sleep has been linked to poor attention and cognition. Well-controlled sleep deprivation studies have shown that lack of sleep not only increases fatigue and sleepiness but also worsens cognitive performance. 2 , 3 , 16 , 17 In fact, the cognitive performance of an individual who has been awake for 17 h is equivalent to that exhibited by one who has a blood alcohol concentration of 0.05%. 1 Outside of a laboratory setting, studies examining sleep in the comfort of peoples’ own homes via self-report surveys have found that persistently poor sleepers experience significantly more daytime difficulties in regards to fatigue, sleepiness, and poor cognition compared with persistently good sleepers. 18
Generally, sleep is associated with academic performance in school. Sleep deficit has been associated with lack of concentration and attention during class. 19 While a few studies report null effects, 20 , 21 most studies looking at the effects of sleep quality and duration on academic performance have linked longer and better-quality sleep with better academic performance such as school grades and study effort. 4 , 6 , 9 , 10 , 11 , 12 , 13 , 22 , 23 , 24 , 25 , 26 , 27 Similarly, sleep inconsistency plays a part in academic performance. Sleep inconsistency (sometimes called “social jet lag”) is defined by inconsistency in sleep schedule and/or duration from day to day. It is typically seen in the form of sleep debt during weekdays followed by oversleep on weekends. Sleep inconsistency tends to be greatest in adolescents and young adults who stay up late but are constrained by strict morning schedules. Adolescents who experience greater sleep inconsistency perform worse in school. 28 , 29 , 30 , 31
Although numerous studies have investigated the relationship between sleep and students’ academic performance, these studies utilized subjective measures of sleep duration and/or quality, typically in the form of self-report surveys; very few to date have used objective measures to quantify sleep duration and quality in students. One exception is a pair of linked studies that examined short-term benefits of sleep on academic performance in college. Students were incentivized with offers of extra credit if they averaged eight or more hours of sleep during final exams week in a psychology class 32 or five days leading up to the completion of a graphics studio final assignment. 33 Students who averaged eight or more hours of sleep, as measured by a wearable activity tracker, performed significantly better on their final psychology exams than students who chose not to participate or who slept less than eight hours. In contrast, for the graphics studio final assignments no difference was found in performance between students who averaged eight or more hours of sleep and those who did not get as much sleep, although sleep consistency in that case was found to be a factor.
Our aim in this study was to explore how sleep affects university students’ academic performance by objectively and ecologically tracking their sleep throughout an entire semester using Fitbit—a wearable activity tracker. Fitbit uses a combination of the wearer’s movement and heart-rate patterns to estimate the duration and quality of sleep. For instance, to determine sleep duration, the device measures the time in which the wearer has not moved, in combination with signature sleep movements such as rolling over. To determine sleep quality, the Fitbit device measures the wearer’s heart-rate variability which fluctuates during transitions between different stages of sleep. Although the specific algorithms that calculate these values are proprietary to Fitbit, they have been found to accurately estimate sleep duration and quality in normal adult sleepers without the use of research-grade sleep staging equipment. 34 By collecting quantitative sleep data over the course of the semester on nearly 100 students, we aimed to relate objective measures of sleep duration, quality, and consistency to academic performance from test to test and overall in the context of a real, large university college course.
A secondary aim was to understand gender differences in sleep and academic performance. Women outperform men in collegiate academic performance in most subjects 35 , 36 , 37 , 38 and even in online college courses. 39 Most of the research conducted to understand this female advantage in school grades has examined gender differences in self-discipline, 40 , 41 , 42 and none to date have considered gender differences in sleep as a mediating factor on school grades. There are inconsistencies in the literature on gender differences in sleep in young adults. While some studies report that females get more quantity 43 but worse quality sleep compared with males, 43 , 44 other studies report that females get better quality sleep. 45 , 46 In the current study, we aim to see whether we would observe a female advantage in grades and clarify how sleep contributes to gender differences.
Bedtime and wake-up times
On average, students went to bed at 1:54 a.m. (Median = 1:47 a.m., Standard Deviation (SD) of all bedtime samples = 2 h 11 min, SD of mean bedtime per participant = 1 h) and woke up at 9:17 a.m. (Median = 9:12 a.m., SD of all wake-up time samples = 2 h 2 min; SD of mean wake-up time per participant = 54 min). The data were confirmed to have Gaussian distribution using the Shapiro–Wilks normality test. We conducted an ANOVA with the overall score (sum of all grade-relevant quizzes and exams—see “Procedure”) as the dependent variable and bedtime (before or after median) and wake-up time (before or after median) as the independent variables. We found a main effect of bedtime ( F (1, 82) = 6.45, p = 0.01), such that participants who went to bed before median bedtime had significantly higher overall score ( X = 77.25%, SD = 13.71%) compared with participants who went to bed after median bedtime ( X = 70.68%, SD = 11.01%). We also found a main effect of wake-up time ( F (1, 82) = 6.43, p = 0.01), such that participants who woke up before median wake-up time had significantly higher overall score ( X = 78.28%, SD = 9.33%) compared with participants who woke up after median wake-up time ( X = 69.63%, SD = 14.38%), but found no interaction between bedtime and wake-up time ( F (1, 82) = 0.66, p = 0.42).
A Pearson’s product-moment correlation between average bedtime and overall score revealed a significant and negative correlation ( r (86) = −0.45, p < 0.0001), such that earlier average bedtime was associated with a higher overall score. There was a significant and negative correlation between average wake-up time and overall score ( r (86) = −0.35, p < 0.001), such that earlier average wake-up time was associated with a higher overall score. There was also a significant and positive correlation between average bedtime and average wake-up time (r (86) = 0.68, p < 0.0001), such that students who went to bed earlier tended to also wake up earlier.
Sleep duration, quality, and consistency in relation to academic performance
Overall, the mean duration of sleep for participants throughout the entire semester was 7 h 8 min (SD of all sleep samples = 1 h 48 min, SD of mean sleep duration per participant = 41 min). There was a significant positive correlation between mean sleep duration throughout the semester (sleep duration) and overall score ( r (86) = 0.38, p < 0.0005), indicating that a greater amount of sleep was associated with a higher overall score (Fig. 1a ). Similarly, there was a significant positive correlation between mean sleep quality throughout the semester (Sleep Quality) and Overall Score ( r (86) = 0.44, p < 0.00005). Sleep inconsistency was defined for each participant as the standard deviation of the participant’s daily sleep duration in minutes so that a larger standard deviation indicated greater sleep inconsistency. There was a significant negative correlation between sleep inconsistency and overall score ( r (86) = −0.36, p < 0.001), indicating that the greater inconsistency in sleep duration was associated with a lower overall score (Fig. 1b ).
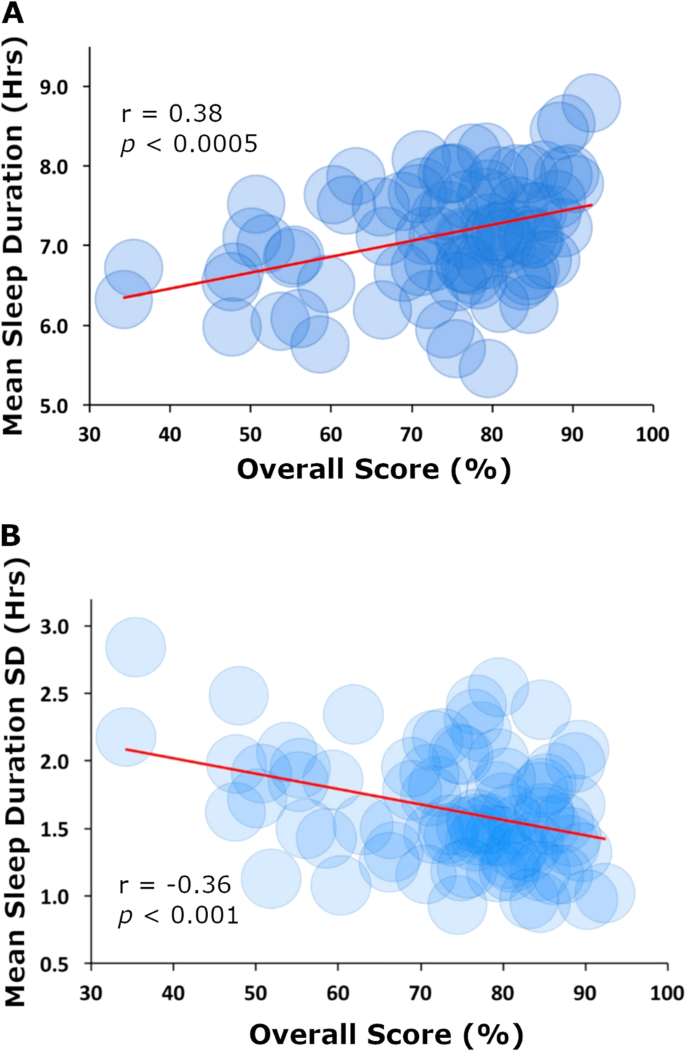
Correlations between sleep measures and overall score. a Average daily hours slept (sleep duration) vs. overall score for the semester. b Standard deviation of average daily hours of sleep (sleep inconsistency) vs. overall score in class
Timing of sleep and its relation to academic performance
To understand sleep and its potential role in memory consolidation, we examined the timing of sleep as it related to specific assessments. All Pearson correlations with three or more comparisons were corrected for multiple comparisons using false discovery rate. 47
Night before assessments
We conducted a correlation between sleep quality the night before a midterm and respective midterm scores as well as sleep duration the night before a midterm and respective scores. There were no significant correlations with sleep duration or sleep quality for all three midterms (all r s < 0.20, all p s > 0.05). Similar analyses for sleep duration and sleep quality the night before respective quizzes revealed no correlations ( r s from 0.01 to 0.26, all p s > 0.05).
Week and month leading up to assessments
To understand the effect of sleep across the time period while course content was learned for an assessment, we examined average sleep measures during the 1 month leading up to the midterms. We found a significant positive correlation between average sleep duration over the month leading up to scores on each midterm ( r s from 0.25 to 0.34, all p s < 0.02). Similar analyses for average sleep duration over one week leading up to respective quizzes were largely consistent with those of midterms, with significant correlations on 3 of 8 quizzes (rs from 0.3 to 0.4, all p s < 0.05) and marginal correlations on an additional 3 quizzes (rs from 0.25 to 0.27, all p s < 0.08).
There was a significant and positive correlation between sleep quality scores averaged over the month leading up to each midterm for all three midterms ( r s from 0.21 to 0.38, all p s < 0.05). Similar analyses for average Sleep Quality over one week leading up to respective quizzes revealed a significant correlation on 1 of 8 quizzes ( r (86) = 0.42, p < 0.005) and marginal correlations on 3 quizzes ( r s from 0.25 to 0.27, all p s < 0.08).
Variance of assessment performance accounted for by sleep measures
In order to calculate how much of the variance on assessment performance was accounted for by the sleep measures, we conducted a stepwise regression on overall score using three regressors: sleep duration, sleep quality, and sleep inconsistency. The relative importance of each variable was calculated using the relaimpo package in R 48 to understand individual regressor’s contribution to the model, which is not always clear from the breakdown of model R 2 when regressors are correlated. We found a significant regression ( F (3,84) = 8.95, p = .00003), with an R 2 of 0.24. Students’ predicted overall score was equal to 77.48 + 0.21 (sleep duration) + 19.59 (Sleep Quality) – 0.45 (sleep inconsistency). While sleep inconsistency was the only significant individual predictor of overall score ( p = 0.03) in this analysis, we found that 24.44% of variance was explained by the three regressors. The relative importance of these metrics were 7.16% sleep duration, 9.68% sleep quality, and 7.6% sleep inconsistency.
Gender differences
Females had better Sleep Quality ( t (88) = 2.63, p = 0.01), and less sleep inconsistency ( t (88) = 2.18, p = 0.03) throughout the semester compared with males, but the two groups experienced no significant difference in sleep duration ( t (88) = 1.03, p = 0.3). Sleep duration and sleep quality were significantly correlated in both males ( r (41) = 0.85, p < 0.00001) and females ( r (43) = 0.64, p < 0.00001), but this correlation was stronger in males ( Z = −2.25, p = 0.02) suggesting that it may be more important for males to get a long-duration sleep in order to get good quality sleep. In addition, sleep inconsistency and sleep quality were significantly negatively correlated in males ( r (41) = −0.51, p = 0.0005) but not in females ( r (43) = 0.29, p > 0.05), suggesting that it may be more important for males to stick to a regular daily sleep schedule in order to get good quality sleep.
Females scored higher on overall score compared with males ( t (88) = −2.48, p = 0.01), but a one-way analysis of covariance (ANCOVA) revealed that females and males did not perform significantly different on overall score when controlling for Sleep Quality, F (1, 85) = 2.22, p = 0.14. Sleep inconsistency and overall score were negatively correlated in males ( r (41) = −0.44, p = 0.003) but not in females ( r (43) = −0.13, p = 0.39), suggesting that it is important for males to stick to a regular sleep schedule in order to perform well in academic performance but less so for females. No other gender differences were detected between other sleep measures and overall score.
This study found that longer sleep duration, better sleep quality, and greater sleep consistency were associated with better academic performance. A multiple linear regression revealed that these three sleep measures accounted for 24.44% of the variance in overall grade performance. Thus, there was a substantial association between sleep and academic performance. The present results correlating overall sleep quality and duration with academic performance are well aligned with previous studies 6 , 11 , 12 , 24 , 25 on the role of sleep on cognitive performance. Similarly, this study compliments the two linked studies that found longer sleep duration during the week before final exams 47 and consistent sleep duration five days prior to a final assignment 48 enhanced students’ performance. The present study, however, significantly extends our understanding of the relation between sleep and academic performance by use of multiple objective measures of sleep throughout an entire semester and academic assessments completed along the way.
The present study also provides new insights about the timing of the relation between sleep and academic performance. Unlike a prior study, 23 we did not find that sleep duration the night before an exam was associated with better test performance. Instead we found that both longer sleep duration and better sleep quality over the full month before a midterm were more associated with better test performance. Rather than the night before a quiz or exam, it may be more important to sleep well for the duration of the time when the topics tested were taught. The implications of these findings are that, at least in the context of an academic assessment, the role of sleep is crucial during the time the content itself is learned, and simply getting good sleep the night before may not be as helpful. The outcome that better “content-relevant sleep” leads to improved performance is supported by previous controlled studies on the role of sleep in memory consolidation. 14 , 15
Consistent with some previous research 45 , 46 female students tended to experience better quality sleep and with more consistency than male students. In addition, we found that males required a longer and more regular daily sleep schedule in order to get good quality sleep. This female advantage in academic performance was eliminated once sleep patterns were statistically equated, suggesting that it may be especially important to encourage better sleep habits in male students (although such habits may be helpful for all students).
Several limitations of the present study may be noted. First, the sleep quality measures were made with proprietary algorithms. There is an evidence that the use of cardiac, respiratory, and movement information from Fitbit devices can accurately estimate sleep stages, 32 but there is no published evidence that Fitbit’s 1~10 sleep quality scores represent a valid assessment of sleep quality. Second, the relation between sleep and academic performance may be moderated by factors that can affect sleep, such as stress, anxiety, motivation, personality traits, and gender roles. Establishing a causal relation between sleep and academic performance will require experimental manipulations in randomized controlled trials, but these will be challenging to conduct in the context of real education in which students care about their grades. Third, these findings occurred for a particular student population at MIT enrolled in a particular course, and future studies will need to examine the generalizability of these findings to other types of student populations and other kinds of classes.
In sum, this study provides evidence for a strong relation between sleep and academic performance using a quantifiable and objective measures of sleep quality, duration, and consistency in the ecological context of a live classroom. Sleep quality, duration, and consistency together accounted for a substantial amount (about a quarter) of the overall variance in academic performance.
Participants
One hundred volunteers (47 females) were selected from a subset of students who volunteered among 370 students enrolled in Introduction to Solid State Chemistry at the Massachusetts Institute of Technology to participate in the study. Participants were informed of the study and gave written consent obtained in accordance with the guidelines of and approved by the MIT Committee on the Use of Humans as Experimental Subjects. Due to limitations in funding, we only had access to 100 Fitbit devices and could not enroll all students who volunteered; consequently, the first 100 participants to volunteer were selected. All participants were gifted a wearable activity tracker at the completion of the study in exchange for their participation. Seven participants were excluded from analysis because they failed to wear their activity tracker for more than 80% of the semester, three participants were excluded because they lost their wearable activity tracker, and another two participants were excluded because they completed less than 75% of the assessments in the class. Of the 88 participants who completed the study (45 females), 85 were freshmen, one was a junior and two were seniors (mean age = 18.19 years).
The Solid State Chemistry class is a single-semester class offered in the fall semester and geared toward freshmen students to satisfy MIT’s general chemistry requirement. The class consisted of weekly lectures by the professor and two weekly recitations led by 12 different teaching assistants (TAs). Each student was assigned to a specific recitation section that fit their schedule and was not allowed to attend other sections; therefore, each student had the same TA throughout the semester. Students took (1) weekly quizzes that tested knowledge on the content covered the week leading up to the quiz date, (2) three midterms that tested knowledge on the content covered in the 3–4 weeks leading up to the exam date, and (3) a final exam that tested content covered throughout the semester. Based on a one-way between subjects’ analysis of variance (ANOVA) to compare the effect of teaching assistants (TAs) on overall grade, we found no significant differences in overall grade across the TAs (F (10, 77) = 1.82, p = 0.07. (One TA was removed from the analysis because he only had one student who was participating in this study).
Participants were asked to wear an activity tracker for the entire duration of the semester without going below 80% usage each week. If 80% or more usage was not maintained, warning emails were sent at the end of that respective week. Participants were asked to return the device if they dipped below 80% usage more than three out of the 14 weeks of the semester. The average usage rate at the end of the semester for the 88 participants who completed the study was 89.4% (SD = 5.5%). The missing data appeared to be at random and were deleted prior to data analysis. As part of a separate research question, 22 of the 88 participants joined an intense cardiovascular exercise class for which they received separate physical education credit. These students performed similarly to the other 67 participants in terms of final class grade ( t (88) = 1.57, p = 0.12), exercise amount (total amount of moderately and very active minutes on the wearable device) (t (88) = 0.59, p = 0.56), sleep amount ( t (88) = 0.3, p = 0.77), and sleep quality ( t (88) = 0.14, p = 0.9), so they were included in all of the analyses.
Participants’ activities were tracked using a Fitbit Charge HR. Data from the device were recorded as follows: heart rate every 5 min; steps taken, distance traveled, floors climbed, calories burned and activity level measurements every 15 min; resting heart rate daily; and sleep duration and quality for every instance of sleep throughout the day. Sleep quality was determined using Fitbit’s proprietary algorithm that produces a value from 0 (poor quality) to 10 (good quality).
Assessments
Nine quizzes, three midterm examinations, and one final examination were administered throughout the 14-week class to assess the students’ academic achievement. The students’ cumulative class grade was made up of 25% for all nine quizzes (lowest quiz grade was dropped from the average), 15% for each midterm exam, and 30% for the final exam for a total of 100%.
At MIT, freshmen are graded on a Pass or No Record basis in all classes taken during their first semester. Therefore, all freshmen in this class needed a C- level or better (≥50%, no grading on a curve) to pass the class. A failing grade (a D or F grade) did not go on their academic record. All upperclassmen were given letter grades; A (≥85%), B (70–84%), C (50–69%), D (45–49%), F (≤44%). Because a large portion of the class had already effectively “passed” the class before taking Quiz 9 and the final exam, we excluded these two assessments from our analyses due to concerns about students’ motivation to perform their best. We calculated for each student an overall score defined as the sum of the eight quizzes and three midterms to summarize academic performance in the course.
Reporting summary
Further information on research design is available in the Nature Research Reporting Summary linked to this article.
Data availability
The data that support the findings of this study are available from the corresponding author upon reasonable request.
Code availability
No custom codes were used in the analysis of this study
Dawson, D. & Reid, K. Fatigue, alcohol and performance impairment. Nature 388 , 540–545 (1997).
Article Google Scholar
Lim, J. & Dinges, D. F. A meta-analysis of the impact of short-term sleep deprivation on cognitive variables. Psychol. Bull. 136 , 375–389 (2010).
Harrison, Y. & Horne, J. A. The impact of sleep deprivation on decision making: a review. J. Exp. Psychol. Appl. 6 , 236–249 (2000).
Article CAS Google Scholar
Wagner, U., Gais, S., Haider, H., Verleger, R. & Born, J. Sleep inspires insight. Nature 427 , 352–355 (2004).
Walker, M. P. & Stickgold, R. Sleep, memory, and plasticity. Annu. Rev. Psychol. 57 , 139–166 (2006).
Wong, M. L. et al. The interplay between sleep and mood in predicting academic functioning, physical health and psychological health: a longitudinal study. J. Psychosom. Res. 74 , 271–277 (2013).
Diekelmann, S., Wilhelm, I. & Born, J. The whats and whens of sleep-dependent memory consolidation. Sleep. Med. Rev. 13 , 309–321 (2009).
Fogel, S. M., Smith, C. T. & Cote, K. A. Dissociable learning-dependent changes in REM and non-REM sleep in declarative and procedural memory systems. Behav. Brain Res. 180 , 48–61 (2007).
Eliasson, A. H. & Lettieri, C. J. Early to bed, early to rise! Sleep habits and academic performance in college students. Sleep. Breath. 14 , 71–75 (2010).
Gaultney, J. F. The prevalence of sleep disorders in college students: Impact on academic performance. J. Am. Coll. Health 59 , 91–97 (2010).
Gilbert, S. P. & Weaver, C. C. Sleep quality and academic performance in university students: A wake-up call for college psychologists. J. Coll. Stud. Psychother. 24 , 295–306 (2010).
Gomes, A. A., Tavares, J. & de Azevedo, M. H. P. Sleep and academic performance in undergraduates: A multi-measure, multi-predictor approach. Chronobiol. Int. 28 , 786–801 (2011).
Lemma, S., Berhane, Y., Worku, A., Gelaye, B. & Williams, M. A. Good quality sleep is associated with better academic performance among university students in Ethiopia. Sleep. Breath. 18 , 257–263 (2014).
Gilestro, G. F., Tononi, G. & Cirelli, C. Widespread changes in synaptic markers as a function of sleep and wakefulness in drosophila. Science 324 , 109–112 (2009).
Rasch, B. & Born, J. About sleep’s role in memory. Physiol. Rev. 93 , 681–766 (2013).
Alhola, P. & Polo-Kantola, P. Sleep deprivation: Impact on cognitive performance. Neuropsychiatr. Dis. Treat. 3 , 553–567 (2007).
PubMed PubMed Central Google Scholar
Durmer, J. S. & Dinges, D. F. Neurocognitive consequences of sleep deprivation. Semin. Neurol. 25 , 117–129 (2005).
Alapin, I. et al. How is good and poor sleep in older adults and college students related to daytime sleepiness, fatigue, and ability to concentrate? J. Psychosom. Res. 49 , 381–390 (2000).
Orzech, K. M., Salafsky, D. B. & Hamilton, L. A. The state of sleep among college students at a large public university. J. Am. Coll. Health 59 , 612–619 (2011).
Eliasson, A., Eliasson, A., King, J., Gould, B. & Eliasson, A. Association of sleep and academic performance. Sleep. Breath. 6 , 45–48 (2002).
Johns, M. W., Dudley, H. A. & Masterton, J. P. The sleep habits, personality and academic performance of medical students. Med. Educ. 10 , 158–162 (1976).
Merikanto, I., Lahti, T., Puusniekka, R. & Partonen, T. Late bedtimes weaken school performance and predispose adolescents to health hazards. Sleep. Med. 14 , 1105–1111 (2013).
Zeek, M. L. et al. Sleep duration and academic performance among student pharmacists. Am. J. Pharm. Educ. 79 , 5–12 (2015).
Hartmann, M. E. & Prichard, J. R. Calculating the contribution of sleep problems to undergraduates’ academic success. Sleep. Health 4 , 463–471 (2018).
Mirghani, H. O., Mohammed, O. S., Almurtadha, Y. M. & Ahmed, M. S. Good sleep quality is associated with better academic performance among Sudanese medical students. BMC Res. Notes 8 , 706 (2015).
Onyper, S. V., Thacher, P. V., Gilbert, J. W. & Gradess, S. G. Class start times, Sleep, and academic performance in college: a path analysis. Chronobiol. Int. 29 , 318–335 (2012).
Ming, X. et al. Sleep insufficiency, sleep health problems and performance in high school students. Clin. Med. Insights Circ. Respir. Pulm. Med. 5 , 71–79 (2011).
Lee, Y. J., Park, J., Soohyun, K., Seong-jin, C. & Seog Ju, K. Academic performance among adolescents with behaviorally. J. Clin. Sleep. Med. 11 , 61–68 (2015).
Díaz-Morales, J. F. & Escribano, C. Social jetlag, academic achievement and cognitive performance: Understanding gender/sex differences. Chronobiol. Int. 32 , 822–831 (2015).
Raley, H., Naber, J., Cross, S. & Perlow, M. The impact of duration of sleep on academic performance in University students. Madr. J. Nurs. 1 , 11–18 (2016).
Haraszti, R. Á., Ella, K., Gyöngyösi, N., Roenneberg, T. & Káldi, K. Social jetlag negatively correlates with academic performance in undergraduates. Chronobiol. Int. 31 , 603–612 (2014).
Scullin, M. K. The eight hour sleep challenge during final exams week. Teach. Psychol. 46 , 55–63 (2018).
King, E., Mobley, C. & Scullin, M. K. The 8‐hour challenge: incentivizing sleep during end‐of‐term assessments. J. Inter. Des. 44 , 85–99 (2018).
PubMed Google Scholar
Beattie, Z. et al. Estimation of sleep stages using cardiac and accelerometer data from a wrist-worn device. Sleep 40 , A26–A26 (2017).
Clark, M. J. & Grandy, J. Sex differences in the academic performance of scholastic aptitude test takers. ETS Res. Rep. Ser. 2 , 1-27 (1984).
Google Scholar
Kimball, M. M. A new perspective on women’s math achievement. Psychol. Bull. 105 , 198–214 (1989).
Mau, W.-C. & Lynn, R. Gender differences on the scholastic aptitude test, the American college test and college grades. Educ. Psychol. 21 , 133–136 (2001).
Willingham, W. W. & Cole, N. S. Gender and fair assessment . (Mahwah, NJ, US, Lawrence Erlbaum Associates, 1997).
Volchok, E. Differences in the performance of male and female students in partially online courses at a community college. Community Coll . J . Res . Pract . 1–17, https://doi.org/10.1080/10668926.2018.1556134 (2018).
Duckworth, A. L. et al. Will not want: self-control rather than motivation explains the female advantage in report card grades. Learn. Individ. Differ. 39 , 13–23 (2015).
Carvalho, R. G. G. Gender differences in academic achievement: The mediating role of personality. Pers. Individ. Differ. 94 , 54–58 (2016).
Duckworth, A. L. & Seligman, M. E. P. Self-discipline gives girls the edge: gender in self-discipline, grades, and achievement test scores. J. Educ. Psychol. 98 , 198–208 (2006).
Tsai, L. L. & Li, S. P. Sleep patterns in college students: Gender and grade differences. J. Psychosom. Res. 56 , 231–237 (2004).
Becker, S. P. et al. Sleep in a large, multi-university sample of college students: sleep problem prevalence, sex differences, and mental health correlates. Sleep Health 4 , 174–181 (2018).
Bixler, E. O. et al. Women sleep objectively better than men and the sleep of young women is more resilient to external stressors: effects of age and menopause. J. Sleep. Res. 18 , 221–228 (2009).
Mallampalli, M. P. & Carter, C. L. Exploring sex and gender differences in sleep health: a Society for Women’s Health Research Report. J. Women’s. Health 23 , 553–562 (2014).
Benjamini, Y. & Hochberg, Y. Controlling the false discovery rate: A practical and powerful approach to multiple testing. J. R. Stat. Soc. Ser. B (Methodol.) 57 , 289–300 (1995).
Grömping, U. Relative importance for linear regression in R: The package relaimpo. J. Stat. Softw. 17 , 1–27 (2015).
Download references
Acknowledgements
This research was supported by a grant from the Horace A. Lubin Fund in the MIT Department of Materials Science and Engineering to J.C.G. and funding from MIT Integrated Learning Initiative to K.O. and J.R.K. The authors are grateful for many useful discussions with Carrie Moore and Matthew Breen at the Department of Athletics, Physical Education, and Recreation at MIT.
Author information
Authors and affiliations.
MIT Integrated Learning Initiative, Department of Brain and Cognitive Sciences, and McGovern Institute for Brain Research, Massachusetts Institute of Technology, Cambridge, MA, 02139, USA
Kana Okano, Jakub R. Kaczmarzyk & John D. E. Gabrieli
Harvard Business School, Boston, MA, 02163, USA
Department of Materials Science and Engineering Massachusetts Institute of Technology, Cambridge, MA, 02139, USA
Jeffrey C. Grossman
You can also search for this author in PubMed Google Scholar
Contributions
K.O. and J.C.G. conceived, designed, supervised, and analyzed the project. J.K. and N.D. helped analyze the data. The manuscript was written by K.O., J.D.E.G., and J.C.G.
Corresponding author
Correspondence to Jeffrey C. Grossman .
Ethics declarations
Competing interests.
The authors declare no competing interests.
Additional information
Publisher’s note: Springer Nature remains neutral with regard to jurisdictional claims in published maps and institutional affiliations.
Supplementary information
Reporting summary, rights and permissions.
Open Access This article is licensed under a Creative Commons Attribution 4.0 International License, which permits use, sharing, adaptation, distribution and reproduction in any medium or format, as long as you give appropriate credit to the original author(s) and the source, provide a link to the Creative Commons license, and indicate if changes were made. The images or other third party material in this article are included in the article’s Creative Commons license, unless indicated otherwise in a credit line to the material. If material is not included in the article’s Creative Commons license and your intended use is not permitted by statutory regulation or exceeds the permitted use, you will need to obtain permission directly from the copyright holder. To view a copy of this license, visit http://creativecommons.org/licenses/by/4.0/ .
Reprints and permissions
About this article
Cite this article.
Okano, K., Kaczmarzyk, J.R., Dave, N. et al. Sleep quality, duration, and consistency are associated with better academic performance in college students. npj Sci. Learn. 4 , 16 (2019). https://doi.org/10.1038/s41539-019-0055-z
Download citation
Received : 20 March 2019
Accepted : 17 July 2019
Published : 01 October 2019
DOI : https://doi.org/10.1038/s41539-019-0055-z
Share this article
Anyone you share the following link with will be able to read this content:
Sorry, a shareable link is not currently available for this article.
Provided by the Springer Nature SharedIt content-sharing initiative
This article is cited by
Social isolation consequences: lessons from covid-19 pandemic in a context of dynamic lock-down in chile.
- Alessandra Patrono
- Stefano Renzetti
- Roberto G. Lucchini
BMC Public Health (2024)
From good sleep to health and to quality of life – a path analysis of determinants of sleep quality of working adults in Abu Dhabi
- Masood Badri
- Mugheer Alkhaili
- Asma Alrashdi
Sleep Science and Practice (2023)
The association between academic performance indicators and lifestyle behaviors among Kuwaiti college students
- Ahmad R. Al-Haifi
- Balqees A. Al-Awadhi
- Hazzaa M. Al-Hazzaa
Journal of Health, Population and Nutrition (2023)
Screen time and sleep among medical students in Germany
- Lukas Liebig
- Antje Bergmann
- Henna Riemenschneider
Scientific Reports (2023)
Ontogeny and social context regulate the circadian activity patterns of Lake Malawi cichlids
- Aakriti Rastogi
- Alex C. Keene
Journal of Comparative Physiology B (2023)
Quick links
- Explore articles by subject
- Guide to authors
- Editorial policies
Sign up for the Nature Briefing newsletter — what matters in science, free to your inbox daily.

August 1, 2013
12 min read
New Hypothesis Explains Why We Sleep
During sleep, the brain weakens the connections among nerve cells, apparently conserving energy and, paradoxically, aiding memory
By Giulio Tononi & Chiara Cirelli
Every night, while we lie asleep, blind, dumb and almost paralyzed, our brains are hard at work. Neurons in the sleeping brain fire nearly as often as they do in a waking state, and they consume almost as much energy. What is the point of this unceasing activity at a time when we are supposedly resting? Why does the conscious mind disconnect so completely from the external environment while the brain keeps nattering on?
The brain's activity during rest likely serves some essential function. The evidence for this importance starts with sleep's ubiquity. All animals apparently sleep even though being unconscious and unresponsive greatly raises the risk of becoming another creature's lunch. Birds do it, bees do it, iguanas and cockroaches do it, even fruit flies do it, as we and others demonstrated more than a decade ago.
Furthermore, evolution has devised a few extraordinary adaptations to accommodate sleep: dolphins and some other marine mammals that must surface often to breathe, for example, sleep by alternately switching off one hemisphere of their brain while the other remains in a waking state.
On supporting science journalism
If you're enjoying this article, consider supporting our award-winning journalism by subscribing . By purchasing a subscription you are helping to ensure the future of impactful stories about the discoveries and ideas shaping our world today.
Like many scientists and nonscientists, the two of us have long wondered what benefit sleep provides that makes it so crucial to living creatures. More than 20 years ago, when we worked together at the Sant'Anna School of Advanced Studies in Pisa, Italy, we began to suspect that the brain's activity during slumber may somehow restore to a baseline state the billions of neural connections that get modified every day by the events of waking life. Sleep, in this telling, would preserve the ability of the brain's circuitry to form new memories continually over the course of an individual's lifetime without becoming oversaturated or obliterating older memories.
We also have an idea of why awareness of the external environment must be shut off during sleep. It seems to us that conscious experience of the here and now has to be interrupted for the brain to gain the chance to integrate new and old memories; sleep provides that respite.
Our hypothesis is somewhat controversial among our fellow neuroscientists who study sleep's role in learning and memory because we suggest that the return to baseline results from a weakening of the links among the neurons that fire during sleep. Conventional wisdom holds, instead, that brain activity during sleep strengthens the neural connections involved in storing newly formed memories. Yet years of research with organisms ranging from flies to people lend support to our notions.
School of Nod
Scientists first proposed the idea that sleep is important to memory nearly a century ago, and plenty of experiments since then have shown that after a night of sleep, and sometimes just a nap, newly formed memories “stick” better than they would if one had spent the same amount of time awake. This pattern holds for declarative memories, such as lists of words and associations between pictures and places, as well as for procedural memories, which underlie perceptual and motor skills, such as playing a musical instrument.
The evidence that sleep benefits memory led scientists to look for signs that the brain rehashes newly learned material at night. They found them: studies performed over the past 20 years, first in rodents and then in humans, show that patterns of neural activity during sleep sometimes do resemble those recorded while subjects are awake. For example, when a rat learns to navigate a maze, certain neurons in a part of the brain called the hippocampus fire in specific sequences. During subsequent sleep, rats “replay” these sequences more often than predicted by chance.
Because of such findings, many researchers came to assume that sleep “replay” consolidates memories by further reinforcing synapses—the contact points between neurons—that have been strengthened when an individual is awake. The idea is that, as linked neurons fire repeatedly, the synapses connecting them more readily convey signals from one neuron to another, helping neuronal circuits to encode memories in the brain. This process of selective strengthening is known as synaptic potentiation, and it is the favored mechanism by which the brain is thought to accomplish learning and remembering.
Yet while replay and potentiation are known to occur during waking activities, scientists have so far found no direct evidence that the synapses in replayed circuits get strengthened during sleep. This lack of evidence hardly surprises us. It is consistent with our suspicion that while the sleeper lies unaware, all that brain activity—the “replay” as well as other, seemingly random firings—might actually be weakening neural connections, not strengthening them.
The Price of Plasticity
There are many good reasons to propose that synapses must become weakened as well as strengthened for the brain to function properly. For one thing, strong synapses consume more energy than weak ones, and the brain does not have infinite stores of energy. In humans the brain accounts for almost 20 percent of the body's energy budget—more than any other organ by weight—and at least two thirds of that portion goes to supporting synaptic activity. Building and bolstering synapses is also a major source of cellular stress, requiring cells to synthesize and deliver components ranging from mitochondria (the cell's power plants), to synaptic vesicles (which ferry signaling molecules), to various proteins and lipids that are needed for communication across synapses.
It seems clear to us that this strain on resources is unsustainable. The brain cannot go on strengthening and maintaining revved-up synapses both day and night for the whole of an individual's lifetime. We do not doubt that learning occurs mainly through synaptic potentiation. We simply doubt that strengthening continues to happen during sleep.
In contrast, synaptic weakening during sleep would restore brain circuitry to a baseline level of strength, thereby avoiding excessive energy consumption and cellular stress. We refer to this baseline-restoring function of sleep as preserving synaptic homeostasis, and we call our overall hypothesis about the role of sleep the synaptic homeostasis hypothesis, or SHY. In principle, SHY explains the essential, universal purpose of sleep for all organisms that do it: sleep restores the brain to a state where it can learn and adapt when we are awake. The risk we take by becoming disconnected from the environment for hours at a time is the price we pay for this neural recalibration. Most generally, sleep is the price we pay for the brain's plasticity—its ability to modify its wiring in response to experience.
But how does SHY explain sleep's salutary effects on learning and memory? How can weakened synapses improve the overall retention of skills and facts? Consider that, over the course of a typical day, almost everything you experience leaves a neural trace in the brain and that the significant events, like meeting a new person or learning a piece of music on the guitar, make up just a trifling portion of that neural encoding. To improve memory, the sleeping brain must somehow distinguish the “noise” of irrelevant information from the “signal” of significant happenings.
We suggest that in sleep, the spontaneous firing of neurons in the brain activates many different circuits in many different combinations, encompassing both new memory traces and old networks of learned associations. (You get a glimpse of this neural free-for-all in dreams.) The spontaneous activity lets the brain try out which new memories fit better with stored memories of proved significance and weakens those synapses that do not fit well in the grand scheme of memory. We and other investigators are exploring possible mechanisms by which brain activity could selectively weaken synapses that encode the “noise” while preserving those that correspond to the “signal.”
While the brain tries out these imaginary scenarios and enacts weakening where appropriate, we had best be unaware of the surrounding environment and be incapable of acting in it; that is, we had best be asleep. Likewise, restoring synaptic homeostasis should not take place while we are awake because the events of the day would dominate the process, giving salience to them rather than to all the knowledge the brain has accumulated over a lifetime. The profound disconnection of sleep frees our brain from the tyranny of the present, creating an ideal circumstance for integrating and consolidating memories.
A Weak Connection
Our proposal that the brain uses neuronal firing during sleep to weaken rather than strengthen synapses is supported in part by close analyses of data from a standard workhorse of sleep research: the electroencephalogram, or EEG. EEGs record patterns of electrical activity in the cerebral cortex via electrodes attached to the scalp. Decades ago EEG recordings of the sleeping brain revealed two main categories of sleep, called rapid eye movement (REM) and non-REM (NREM), that alternate throughout the night. Each has distinctive brain-wave patterns. In addition to the jittering of eyeballs underneath closed lids that gives REM sleep its name, that stage is dominated by relatively fast oscillations—quick ups and downs in the curves of the EEG readout, resembling EEG recordings of the waking state. In contrast, slow oscillations—with frequencies of about one cycle per second—are the most prominent feature of NREM sleep.
A decade ago the late Mircea Steriade of Laval University in Quebec discovered that the slow oscillations of NREM sleep arise when groups of neurons fire together for a little while (so-called on periods), then fall silent for about a fraction of a second (off periods) and then resume their synchronized firing. This was one of the fundamental discoveries in sleep research. Since then, scientists have also discovered that in birds and mammals, the slow waves are large if preceded by a long period of wakefulness and become smaller as sleep goes on.
We reasoned that if synapses are strong, neurons will synchronize their firing more, producing larger slow waves. If synapses are weak, neurons will be less synchronized and the resulting slow waves will be smaller. Results of computer simulations and experiments in humans and animals led us to conclude that the big, steep slow waves early in the night indicate that synapses have been strengthened by prior wakefulness, whereas the small, shallow slow waves early in the morning indicate that synapses have become weaker during sleep.
Direct support for the idea that synapses become weaker during sleep, and may even be pruned away, comes from studies in animals. In fruit flies, for instance, we find that sleep reverses a progressive increase in the number and size of synapses that occurs during the day, especially when the flies are exposed to stimulating environments. Synaptic spines are specialized protrusions on a neuron's signal-detecting arm. When fruit flies spend the day interacting with other flies, neurons throughout their brain sprout more synaptic spines by evening than were present in the morning.
Just as remarkably, the number of spines goes back to the baseline level by the following morning if—and only if—the flies are allowed to sleep. We saw a similar phenomenon in the cerebral cortex of adolescent mice: the number of synaptic spines tended to rise when the animals were awake and to fall when they slept. In adult rodents, the upshot is the same, although it is not the number of synaptic spines that changes with wakefulness and sleep but rather the abundance of certain spine molecules, known as AMPA receptors, that determine the strength of a synapse. When we monitored these AMPA receptors, we found that their number per synapse increases after wakefulness and decreases after sleep. More receptors make for stronger synapses; fewer mean the synapses have weakened.
Synaptic strength can be gauged directly by using an electrical probe to stimulate neural fibers in the cortex. The neurons respond with an induced electrical discharge that is larger when synapses are strong and smaller when the connections are weak. We showed that in rats, stimulated neurons fire more strongly after a few hours of wakefulness and less strongly after sleep. Marcello Massimini of the University of Milan in Italy and Reto Huber, now at the University of Zurich, performed a similar experiment in humans. Instead of an electrical probe, they turned to transcranial magnetic stimulation—a short magnetic pulse applied to the scalp—to stimulate the underlying neurons. They then recorded the strength of the cortical responses with high-density EEG. The results were clear: the longer a subject was awake, the larger the EEG responses. It took a night of sleep for cortical responses to return to the baseline.
Less Is More
The common conclusion of these experiments, which we performed over two decades, is that spontaneous cortical activity in sleep does indeed weaken the synaptic connections in neural circuits, whether by damping their ability to send electrical impulses or by erasing them outright.
This process, which we call down selection, would ensure the survival of the circuits that are “fittest,” either because they were activated strongly and consistently during wakefulness (say, by playing the right notes on a guitar while trying to master a new piece) or because they were better integrated with previous, older memories (as would be the case for a new word encountered in a known language). Meanwhile synapses in circuits that were only mildly enhanced during wakefulness (such as fumbled notes on the guitar) or that fit less with old memories (such as a new word presented in an unknown language) would be depressed.
Down selection would ensure that insignificant events would leave no lasting trace in our neural circuitry, whereas memories of note would be preserved. As an additional bonus, down selection would also make room for another cycle of synaptic strengthening during wakefulness. Indeed, some findings imply that among its many other benefits for learning and memory, sleep aids the subsequent acquisition of new memories (material encountered before the next bout of sleep). Quite a few studies have shown that after a night of sleep, you can learn new material much better than you can after having been awake all day. (Students, take note.)
Although we have no direct evidence for a mechanism that would produce selective weakening of activated synapses as yet, we have a notion of how synaptic weakening could occur. We suspect the slow waves of mammalian NREM sleep somehow play a role. In lab studies of rat brain tissue, nerve cells became less effective at passing signals to one another when stimulated in ways that mimic the synchronized on/off cycles of slow-wave sleep.
The chemistry of the brain also changes in NREM sleep in a way that could lead to synaptic weakening. In the awake individual, a concentrated soup of signaling chemicals, or neuromodulators—including acetylcholine, norepinephrine, dopamine, serotonin, histamine and hypocretin—bathe the brain and bias synapses toward strengthening when signals pass through them. During sleep—especially NREM sleep—the soup becomes much less concentrated. This diluted milieu of neuromodulators may bias the neural circuitry so that synapses become weakened, rather than strengthened, when signals flow across them. The process might also involve a substance called brain-derived neurotrophic factor (BDNF), which is known to promote synaptic strengthening and to be involved in memory acquisition. BDNF levels are high in neurons during wakefulness and minimal during sleep.
Local Sleep
Regardless of specific mechanisms and selective processes, the evidence is strong in several species that overall synaptic strength goes up during wakefulness and down during sleep: the core prediction of SHY. We can test SHY further by examining some of its intriguing corollaries.
For example, if the hypothesis is correct, then the more plasticity a part of the brain undergoes during wakefulness, the more that part should need to sleep. “Sleep need” can, in turn, be indicated by an increase in the size and duration of NREM slow waves. To explore this prediction, we asked human subjects to learn a novel task: how to reach a target on a computer screen while the cursor (controlled by a mouse) is systematically rotated. The part of the brain that engages in this kind of learning is the right parietal cortex. Sure enough, when our subjects slept, the slow waves over their right parietal cortex were larger, relative to waves from the same area on the night before learning occurred. These large waves did flatten out in the course of the night, as such oscillations do. But those large, localized waves at the start of the night tell us that particular part of the brain had been exhausted by the task we assigned.
Many other experiments by the two of us and others have since confirmed that learning, and more generally the activation of synapses in circuits, produces a local increase in sleep need. Recently we have even found that prolonged or intense use of certain circuits can make local groups of neurons “fall asleep” even though the rest of the brain (and the organism itself) remains awake. Thus, if a rat stays awake longer than usual, some cortical neurons show brief periods of silence that are basically indistinguishable from the off periods observed during slow-wave sleep. Meanwhile the rat is running around, its eyes open, tending to its business, as any awake rat would do.
This phenomenon is called local sleep, and it is attracting scrutiny from other investigators. Our latest studies indicate that localized off periods also occur in the brains of sleep-deprived humans and that those periods become more frequent after intense learning. It seems that when we have been awake for too long or have overexerted certain circuits, small chunks of the brain may take quick naps without giving notice. One wonders how many errors of judgment, silly mistakes, irritable responses and foul moods result from local sleep in the brains of exhausted people who believe they are fully awake and in complete control.
SHY also predicts that sleep is especially important in childhood and adolescence, times of concentrated learning and of intense synaptic remodeling, as many studies have shown. In youth, synapses are formed, strengthened and pruned at an explosive rate never approached in adulthood. It makes sense that down selection during sleep would be crucial to minimize the energy costs of this frenzied synaptic remodeling and to favor the survival of adaptive neural circuits in these stages of life. One can only wonder what happens when sleep is disrupted or insufficient during critical periods in development. Might the deficit corrupt the proper refinement of neural circuits? In that case, the effect of sleep loss would not merely be occasional forgetfulness or misjudgment but a lasting change in the way the brain is wired.
We look forward to testing SHY's predictions and exploring its implications further. For example, we hope to discover whether sleep deprivation during neural development leads to changes in the organization of brain circuitry. We would also like to learn more about the effect of sleep on deep-brain areas, such as the thalamus, cerebellum, hypothalamus and brain stem, and about the role of REM sleep in synaptic homeostasis. Perhaps we would then learn if sleep is indeed the price of waking plasticity, a price that every brain and every neuron must pay.
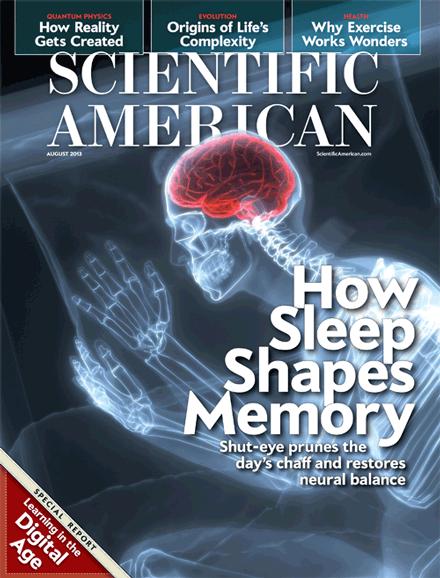

Directional Hypothesis: Definition and 10 Examples
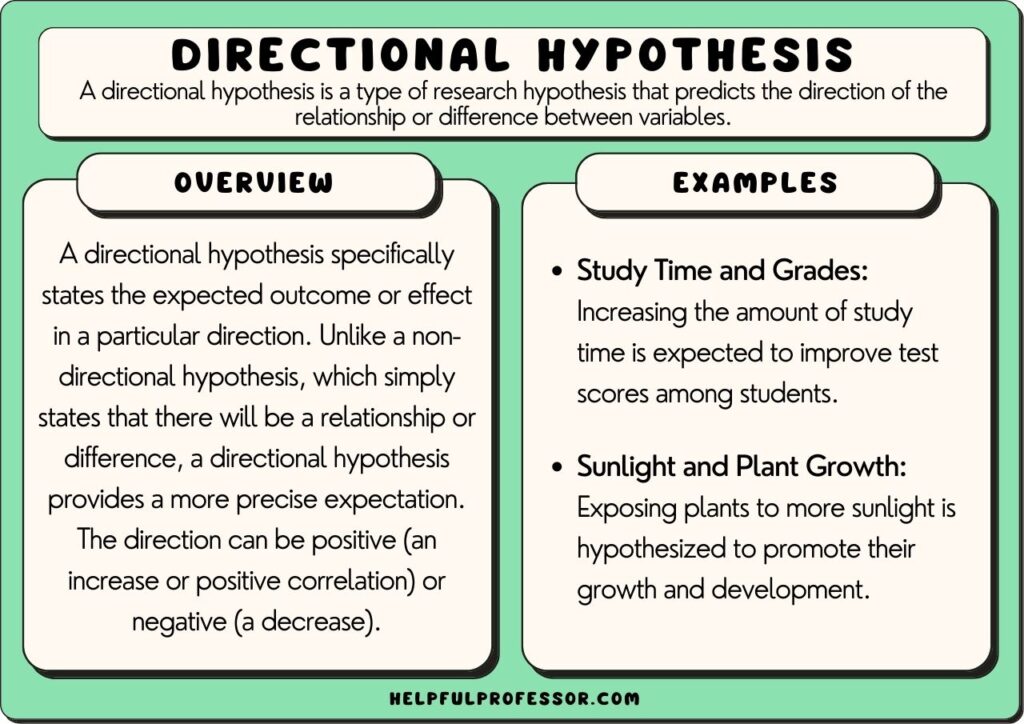
A directional hypothesis refers to a type of hypothesis used in statistical testing that predicts a particular direction of the expected relationship between two variables.
In simpler terms, a directional hypothesis is an educated, specific guess about the direction of an outcome—whether an increase, decrease, or a proclaimed difference in variable sets.
For example, in a study investigating the effects of sleep deprivation on cognitive performance, a directional hypothesis might state that as sleep deprivation (Independent Variable) increases, cognitive performance (Dependent Variable) decreases (Killgore, 2010). Such a hypothesis offers a clear, directional relationship whereby a specific increase or decrease is anticipated.
Global warming provides another notable example of a directional hypothesis. A researcher might hypothesize that as carbon dioxide (CO2) levels increase, global temperatures also increase (Thompson, 2010). In this instance, the hypothesis clearly articulates an upward trend for both variables.
In any given circumstance, it’s imperative that a directional hypothesis is grounded on solid evidence. For instance, the CO2 and global temperature relationship is based on substantial scientific evidence, and not on a random guess or mere speculation (Florides & Christodoulides, 2009).
Directional vs Non-Directional vs Null Hypotheses
A directional hypothesis is generally contrasted to a non-directional hypothesis. Here’s how they compare:
- Directional hypothesis: A directional hypothesis provides a perspective of the expected relationship between variables, predicting the direction of that relationship (either positive, negative, or a specific difference).
- Non-directional hypothesis: A non-directional hypothesis denotes the possibility of a relationship between two variables ( the independent and dependent variables ), although this hypothesis does not venture a prediction as to the direction of this relationship (Ali & Bhaskar, 2016). For example, a non-directional hypothesis might state that there exists a relationship between a person’s diet (independent variable) and their mood (dependent variable), without indicating whether improvement in diet enhances mood positively or negatively. Overall, the choice between a directional or non-directional hypothesis depends on the known or anticipated link between the variables under consideration in research studies.
Another very important type of hypothesis that we need to know about is a null hypothesis :
- Null hypothesis : The null hypothesis stands as a universality—the hypothesis that there is no observed effect in the population under study, meaning there is no association between variables (or that the differences are down to chance). For instance, a null hypothesis could be constructed around the idea that changing diet (independent variable) has no discernible effect on a person’s mood (dependent variable) (Yan & Su, 2016). This proposition is the one that we aim to disprove in an experiment.
While directional and non-directional hypotheses involve some integrated expectations about the outcomes (either distinct direction or a vague relationship), a null hypothesis operates on the premise of negating such relationships or effects.
The null hypotheses is typically proposed to be negated or disproved by statistical tests, paving way for the acceptance of an alternate hypothesis (either directional or non-directional).
Directional Hypothesis Examples
1. exercise and heart health.
Research suggests that as regular physical exercise (independent variable) increases, the risk of heart disease (dependent variable) decreases (Jakicic, Davis, Rogers, King, Marcus, Helsel, Rickman, Wahed, Belle, 2016). In this example, a directional hypothesis anticipates that the more individuals maintain routine workouts, the lesser would be their odds of developing heart-related disorders. This assumption is based on the underlying fact that routine exercise can help reduce harmful cholesterol levels, regulate blood pressure, and bring about overall health benefits. Thus, a direction – a decrease in heart disease – is expected in relation with an increase in exercise.
2. Screen Time and Sleep Quality
Another classic instance of a directional hypothesis can be seen in the relationship between the independent variable, screen time (especially before bed), and the dependent variable, sleep quality. This hypothesis predicts that as screen time before bed increases, sleep quality decreases (Chang, Aeschbach, Duffy, Czeisler, 2015). The reasoning behind this hypothesis is the disruptive effect of artificial light (especially blue light from screens) on melatonin production, a hormone needed to regulate sleep. As individuals spend more time exposed to screens before bed, it is predictably hypothesized that their sleep quality worsens.
3. Job Satisfaction and Employee Turnover
A typical scenario in organizational behavior research posits that as job satisfaction (independent variable) increases, the rate of employee turnover (dependent variable) decreases (Cheng, Jiang, & Riley, 2017). This directional hypothesis emphasizes that an increased level of job satisfaction would lead to a reduced rate of employees leaving the company. The theoretical basis for this hypothesis is that satisfied employees often tend to be more committed to the organization and are less likely to seek employment elsewhere, thus reducing turnover rates.
4. Healthy Eating and Body Weight
Healthy eating, as the independent variable, is commonly thought to influence body weight, the dependent variable, in a positive way. For example, the hypothesis might state that as consumption of healthy foods increases, an individual’s body weight decreases (Framson, Kristal, Schenk, Littman, Zeliadt, & Benitez, 2009). This projection is based on the premise that healthier foods, such as fruits and vegetables, are generally lower in calories than junk food, assisting in weight management.
5. Sun Exposure and Skin Health
The association between sun exposure (independent variable) and skin health (dependent variable) allows for a definitive hypothesis declaring that as sun exposure increases, the risk of skin damage or skin cancer increases (Whiteman, Whiteman, & Green, 2001). The premise aligns with the understanding that overexposure to the sun’s ultraviolet rays can deteriorate skin health, leading to conditions like sunburn or, in extreme cases, skin cancer.
6. Study Hours and Academic Performance
A regularly assessed relationship in academia suggests that as the number of study hours (independent variable) rises, so too does academic performance (dependent variable) (Nonis, Hudson, Logan, Ford, 2013). The hypothesis proposes a positive correlation , with an increase in study time expected to contribute to enhanced academic outcomes.
7. Screen Time and Eye Strain
It’s commonly hypothesized that as screen time (independent variable) increases, the likelihood of experiencing eye strain (dependent variable) also increases (Sheppard & Wolffsohn, 2018). This is based on the idea that prolonged engagement with digital screens—computers, tablets, or mobile phones—can cause discomfort or fatigue in the eyes, attributing to symptoms of eye strain.
8. Physical Activity and Stress Levels
In the sphere of mental health, it’s often proposed that as physical activity (independent variable) increases, levels of stress (dependent variable) decrease (Stonerock, Hoffman, Smith, Blumenthal, 2015). Regular exercise is known to stimulate the production of endorphins, the body’s natural mood elevators, helping to alleviate stress.
9. Water Consumption and Kidney Health
A common health-related hypothesis might predict that as water consumption (independent variable) increases, the risk of kidney stones (dependent variable) decreases (Curhan, Willett, Knight, & Stampfer, 2004). Here, an increase in water intake is inferred to reduce the risk of kidney stones by diluting the substances that lead to stone formation.
10. Traffic Noise and Sleep Quality
In urban planning research, it’s often supposed that as traffic noise (independent variable) increases, sleep quality (dependent variable) decreases (Muzet, 2007). Increased noise levels, particularly during the night, can result in sleep disruptions, thus, leading to poor sleep quality.
11. Sugar Consumption and Dental Health
In the field of dental health, an example might be stating as one’s sugar consumption (independent variable) increases, dental health (dependent variable) decreases (Sheiham, & James, 2014). This stems from the fact that sugar is a major factor in tooth decay, and increased consumption of sugary foods or drinks leads to a decline in dental health due to the high likelihood of cavities.
See 15 More Examples of Hypotheses Here
A directional hypothesis plays a critical role in research, paving the way for specific predicted outcomes based on the relationship between two variables. These hypotheses clearly illuminate the expected direction—the increase or decrease—of an effect. From predicting the impacts of healthy eating on body weight to forecasting the influence of screen time on sleep quality, directional hypotheses allow for targeted and strategic examination of phenomena. In essence, directional hypotheses provide the crucial path for inquiry, shaping the trajectory of research studies and ultimately aiding in the generation of insightful, relevant findings.
Ali, S., & Bhaskar, S. (2016). Basic statistical tools in research and data analysis. Indian Journal of Anaesthesia, 60 (9), 662-669. doi: https://doi.org/10.4103%2F0019-5049.190623
Chang, A. M., Aeschbach, D., Duffy, J. F., & Czeisler, C. A. (2015). Evening use of light-emitting eReaders negatively affects sleep, circadian timing, and next-morning alertness. Proceeding of the National Academy of Sciences, 112 (4), 1232-1237. doi: https://doi.org/10.1073/pnas.1418490112
Cheng, G. H. L., Jiang, D., & Riley, J. H. (2017). Organizational commitment and intrinsic motivation of regular and contractual primary school teachers in China. New Psychology, 19 (3), 316-326. Doi: https://doi.org/10.4103%2F2249-4863.184631
Curhan, G. C., Willett, W. C., Knight, E. L., & Stampfer, M. J. (2004). Dietary factors and the risk of incident kidney stones in younger women: Nurses’ Health Study II. Archives of Internal Medicine, 164 (8), 885–891.
Florides, G. A., & Christodoulides, P. (2009). Global warming and carbon dioxide through sciences. Environment international , 35 (2), 390-401. doi: https://doi.org/10.1016/j.envint.2008.07.007
Framson, C., Kristal, A. R., Schenk, J. M., Littman, A. J., Zeliadt, S., & Benitez, D. (2009). Development and validation of the mindful eating questionnaire. Journal of the American Dietetic Association, 109 (8), 1439-1444. doi: https://doi.org/10.1016/j.jada.2009.05.006
Jakicic, J. M., Davis, K. K., Rogers, R. J., King, W. C., Marcus, M. D., Helsel, D., … & Belle, S. H. (2016). Effect of wearable technology combined with a lifestyle intervention on long-term weight loss: The IDEA randomized clinical trial. JAMA, 316 (11), 1161-1171.
Khan, S., & Iqbal, N. (2013). Study of the relationship between study habits and academic achievement of students: A case of SPSS model. Higher Education Studies, 3 (1), 14-26.
Killgore, W. D. (2010). Effects of sleep deprivation on cognition. Progress in brain research , 185 , 105-129. doi: https://doi.org/10.1016/B978-0-444-53702-7.00007-5
Marczinski, C. A., & Fillmore, M. T. (2014). Dissociative antagonistic effects of caffeine on alcohol-induced impairment of behavioral control. Experimental and Clinical Psychopharmacology, 22 (4), 298–311. doi: https://psycnet.apa.org/doi/10.1037/1064-1297.11.3.228
Muzet, A. (2007). Environmental Noise, Sleep and Health. Sleep Medicine Reviews, 11 (2), 135-142. doi: https://doi.org/10.1016/j.smrv.2006.09.001
Nonis, S. A., Hudson, G. I., Logan, L. B., & Ford, C. W. (2013). Influence of perceived control over time on college students’ stress and stress-related outcomes. Research in Higher Education, 54 (5), 536-552. doi: https://doi.org/10.1023/A:1018753706925
Sheiham, A., & James, W. P. (2014). A new understanding of the relationship between sugars, dental caries and fluoride use: implications for limits on sugars consumption. Public health nutrition, 17 (10), 2176-2184. Doi: https://doi.org/10.1017/S136898001400113X
Sheppard, A. L., & Wolffsohn, J. S. (2018). Digital eye strain: prevalence, measurement and amelioration. BMJ open ophthalmology , 3 (1), e000146. doi: http://dx.doi.org/10.1136/bmjophth-2018-000146
Stonerock, G. L., Hoffman, B. M., Smith, P. J., & Blumenthal, J. A. (2015). Exercise as Treatment for Anxiety: Systematic Review and Analysis. Annals of Behavioral Medicine, 49 (4), 542–556. doi: https://doi.org/10.1007/s12160-014-9685-9
Thompson, L. G. (2010). Climate change: The evidence and our options. The Behavior Analyst , 33 , 153-170. Doi: https://doi.org/10.1007/BF03392211
Whiteman, D. C., Whiteman, C. A., & Green, A. C. (2001). Childhood sun exposure as a risk factor for melanoma: a systematic review of epidemiologic studies. Cancer Causes & Control, 12 (1), 69-82. doi: https://doi.org/10.1023/A:1008980919928
Yan, X., & Su, X. (2009). Linear regression analysis: theory and computing . New Jersey: World Scientific.
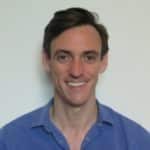
Chris Drew (PhD)
Dr. Chris Drew is the founder of the Helpful Professor. He holds a PhD in education and has published over 20 articles in scholarly journals. He is the former editor of the Journal of Learning Development in Higher Education. [Image Descriptor: Photo of Chris]
- Chris Drew (PhD) https://helpfulprofessor.com/author/chris-drew-phd/ 5 Top Tips for Succeeding at University
- Chris Drew (PhD) https://helpfulprofessor.com/author/chris-drew-phd/ 50 Durable Goods Examples
- Chris Drew (PhD) https://helpfulprofessor.com/author/chris-drew-phd/ 100 Consumer Goods Examples
- Chris Drew (PhD) https://helpfulprofessor.com/author/chris-drew-phd/ 30 Globalization Pros and Cons
Leave a Comment Cancel Reply
Your email address will not be published. Required fields are marked *
Sleep deprivation
Curator: David F. Dinges
Eugene M. Izhikevich
Nick Orbeck
Tobias Denninger
Julian Lim , University of Pennsylvania, Philadelphia, PA, USA
Dr. David F. Dinges , University of Pennsylvania School of Medicine, Philadelphia, PA
Sleep deprivation is the restriction of sleep below the level of basal sleep need; this can be both acute (a single period of extended wakefulness), or chronic (the accumulation of sleep debt over multiple nights of sleep restriction). The basal sleep need of an organism is its habitual sleep duration in the absence of pre-existing sleep debt, with sleep debt defined as the duration of sleep below which waking deficits can be observed. In human beings, these deficits can be seen across a spectrum of neurocognitive domains from basic attentional processes to high-level executive function.
Under unrestricted sleep conditions, endogenous circadian and homeostatic processes interact to promote periods of stable sleep and wakefulness, with relatively abrupt transitions from one state to the other. Sleep deprivation leads to a breakdown in this stability, beginning with transient and involuntary intrusions of sleep into periods of wakefulness. These intrusions can be observed through a number of neurobehavioral phenomena, including microsleeps, sleep attacks, slow eyelid closures, voluntary naps, and slow rolling eye movements .
Apart from these directly-observable phenomena, the effects of sleep deprivation on human beings fall into two main categories: objective (which can be physiological or cognitive), and subjective.
Objective effects
Physiological.
- Sleep propensity : The classic paradigms used to measure sleep propensity are the multiple sleep latency test (MSLT) (Carskadon and Dement, 1975) and the maintenance of wakefulness test (MWT) (Mitler et al., 1982). The MSLT measures time to sleep onset when subjects are instructed to fall asleep while lying supine in a sleep-conducive environment. In contrast, the MWT measures a subject’s ability to remain awake while sitting upright in a quiet, darkened room. Sleep latency on both of these tests has an inverse relationship with the number of hours a subject has been sleep deprived.
- Endocrine changes are regularly observed in experiments of total sleep deprivation, including a reduction in levels of growth hormone (Radomski et al., 1992; Seifritz et al., 1995) and in levels of leptin in both rodents (Everson and Crowley, 2004) and humans (Mullington et al., 2003) Increases have been observed in levels of thyroid axis hormones (Allan and Czeisler, 1994; Gary et al., 1996), prolactin, luteinizing hormone and estradiol during partial sleep deprivation (Baumgartner et al., 1993). Cortisol levels, which normally follow a circadian/ultradian cycle, are altered following periods of sleep deprivation. These changes are somewhat dependent on the time of measurement post-SD; there is a rebound increase of cortisol before sleep onset after a night without sleep, (Leproult et al. 1997; Mullington et al. 1996; Weitzman et al. 1983), but decreased levels directly after the deprivation period, or when integrated over the course of the subsequent night. (Akerstedt et al. 1980; Dinges et al. 1994; Vgontzas et al. 1999a; Frey et al. 2007). Certain investigators have also found no significant change in levels of this hormone. (Gary et al. 1996; Gonzalez-Ortiz et al. 2000; Haack et al. 2002).
- Immune system/inflammatory response : Sleep deprivation has effects on the immune system, including changes in natural killer cell activity (Irwin et al., 1994; Irwin et al., 1996), interleukin-6 (Redwine et al., 2000; Shearer et al., 2001), soluble tumor necrosis factor-alpha receptor 1 (Shearer et al., 2001) and cell adhesion molecules (Frey et al., 2007). Chronic sleep deprivation in shift workers and nurses is associated with increased cardiovascular risk, possibly mediated by inflammatory markers such as C-reactive protein (Ayas et al., 2003).
- Neurogenesis : There is evidence that sleep deprivation inhibits adult neurogenesis in the dentate gyrus of the hippocampus of rodents via the action of elevated levels of circulating glucocorticoids (Hairston et al., 2005; Mirescu et al., 2006).
- Electrophysiological markers : Sleep deprivation alters the signatures of brain electrophysiology. Increasing homeostatic sleep pressure is associated with increases in spectral power in the theta band (4 -8 Hz), and decreases in the alpha band (9.25 – 10 Hz) as measured by electroencephalography (EEG). EEG changes also manifest in recovery sleep after periods of sleep deprivation, with rebound increases in slow wave activity (0.5 – 4 Hz) reported during recovery nights (Brunner et al., 1993; Van Dongen et al., 2003). It has been suggested that this EEG slow wave activity rebound, which is assumed to involve the restorative aspects of sleep, may also reflect synaptic potentiation (Tononi and Cirelli, 2003).
- Functional magnetic resonance imaging (fMRI) markers : Subjects have been tested on a variety of tasks after sleep deprivation while undergoing functional magnetic resonance imaging (fMRI). Compared to baseline, blood oxygenation level dependent (BOLD) contrast typically reveals hypoactivation in inferior parietal regions after SD (Bell-McGinty et al., 2004; Chee and Choo, 2004; Chee et al., 2006). Given that this phenomenon has been observed across a wide range of cognitive tasks, it has been suggested that parietal hypoactivation after sleep deprivation is associated with a decrease in attentional resources. However, compensatory increases in cerebral activation during sleep deprivation have also been observed during task performance (Drummond et al., 2000; Drummond et al., 2005).
- Fundamental processes : Speed of processing, as measured by neuropsychological tests such as the Digit Symbol Substitution Task, decreases with increasing time awake (Leproult et al., 1997). Additionally, tonic, sustained attention becomes increasingly labile during periods of sleep deprivation, leading to increased variability in reaction times, as well as a greater number of performance lapses on the Psychomotor Vigilance Test (PVT) and other tests of attention (Dinges et al., 1997). The time-on-task effect in sustained attention tasks is also more pronounced in the sleep-deprived state.
- Higher cognitive functions : The effects of sleep deprivation on tasks of higher cognitive function are less well known, and experimental results have been mixed. Numerous studies (Linde et al., 1999; Harrison and Horne, 2000) have shown that subjects are able to recruit compensatory resources and temporarily perform at a level comparable to that of rested controls when performing relatively more complex and engaging tasks. This is more apparent in convergent tasks (e.g. tasks of critical thinking and rule-based deduction), and less true of divergent ones (e.g. assimilation of changing information, updating strategies based on new information, lateral thinking, risk assessment, insight and temporal memory skills). Sleep loss also adversely affects working memory function, defined as the ability to encode, manipulate and retrieve information, in one or more sensory modalities (Chee and Choo, 2004). One unifying hypothesis arising from these results is that sleep deprivation differentially affects prefrontal-cortex-related functions while leaving executive ability associated with other brain regions relatively intact.
- For a more complete review of the neurocognitive consequences of sleep deprivation, refer to Durmer and Dinges (2005).
Subjective effects
- Emotion and mood : In comparison to the effects of sleep deprivation on cognition and performance, relatively little is known about the effects of sleep deprivation on emotional processes, with the exception of subjective mood states (Dinges et al., 1997). Experimental data have shown that nearly all forms of sleep deprivation result in increased negative mood states, especially feelings of fatigue, loss of vigor, sleepiness, and confusion. These data are limited however in that they were obtained without systematic mood induction procedures or other experimental probes designed to influence mood. It is not yet known if there are systematic differences in how specific events influence mood in sleepy vs. rested subjects.
Sleep deprivation is also widely believed to be associated with impaired emotional functioning. Field studies of sleep deprivation in medical personnel (Asken and Raham, 1983; Orton and Gruzeller, 1989) have found that reduced sleep is associated with increased negative and decreased positive emotional responses to specific events, but the uncontrolled nature of these studies allows for alternative interpretations of these results. Other than simple subjective self-report measures of affect, there are few laboratory-based studies on affective reactions in sleep-deprived humans.
Mathematical modeling
Interest in understanding the patterns of sleep intrusions and the other detrimental effects of sleep deprivation have led to the use and modification of existing biomathematical models of sleep-wake regulation. In particular, the two-process model of sleep regulation, first proposed by Alexander Borbély (1982), is the leading paradigm used to predict the effects of sleep and sleep deprivation on human cognition and performance. This model consists of a circadian oscillator with a period of slightly over 24 hours, as well as a homeostatic process (reflecting sleep pressure) that builds exponentially during periods of wakefulness and dissipates exponentially during periods of sleep (Figure 1). These two processes interact to promote alertness in the day and sleep during the night. It has been suggested that waking cognitive function can be mathematically modeled as the difference between the quantitative states of the circadian and homeostatic processes (Mallis, 2004).
Numerous refinements have since been proposed to improve the predictive validity of the two-process model. Adjustments have been made for environmental variables (such as light levels, and location), sleep variables (such as length and quality), and the level of cognitive workload during waking periods. In particular, including sleep inertia as an input factor improves the predictive power of the two-process model significantly. Seven leading biomathematical models of human fatigue and performance have emerged from modifications to the two-process model; these are reviewed and discussed by Mallis et al. (2004) and Dinges (2004).
Lapse and wake-state instability hypotheses
Early attempts to understand the effects of sleep deprivation focused on the “lapse hypothesis” (Dinges and Kribbs, 1991). This theory suggested that baseline levels of functioning are identical in rested and sleep-deprived states, but that sleep-deprived individuals experience transient phases of low arousal during which sleep intrusions and performance lapses occur. Although the hypothesis has some measure of explanatory power, evidence from experiments of chronic sleep restriction (Dinges et al., 1997) suggests that it is insufficient to account for the changes in neurobehavioral functioning that occur over time. For example, the increasing variability of reaction times on the Psychomotor Vigilance Test as the number of hours of sleep loss increases would not be predicted by the lapse hypothesis alone.
An alternative hypothesis is the “wake-state instability” theory, which posits that waking-state function degrades after sleep deprivation both because of lapses in attention and a decrease in tonic aspects of functioning. These global deficits in cognitive functioning account for the increased variability in performance, as well as the observed slowing of fastest or optimal responses.
Biological basis
- A pathway, once active, will tend to stay active, because it both inhibits the opposing pathway as well as the other pathway’s inhibitory effect on itself.
- A small perturbation in the system may lead to a sudden change in the pathway whose activity is dominant.
- There is strong evidence that the orexin-hypocretins are responsible for both stabilizing the sleep switch and altering its equilibrium point (from promoting wakefulness to promoting sleep and vice versa). These molecules interact directly with the arousal system, but not the VLPO, suggesting that that their action inhibits unwanted lapses into sleep (Mignot, 2004).
For a more complete review of the VLPO and its function as a sleep switch, see Saper et al. (2005).
- Adenosine has been proposed as an endogenous marker of homeostatic sleep drive. As the number of hours awake increases, brain glycogen reserves and ATP levels are depleted, with ATP degraded into ADP, AMP, and finally adenosine, which accumulates in the basal forebrain (Porkka-Heiskanen et al., 2000). Adenosine A2a receptor agonists incite VLPO neurons to express Fos protein, and also promote sleep in rats; these, and other lines of evidence (Strecker et al., 2000) point to adenosine as the driver of the negative feedback loop of sleep propensity.
- The circadian sleep-wake cycle is temporally regulated at the molecular level by the suprachiasmatic nucleus (SCN). The regulation occurs via a cyclical, 24-hour transcriptional-translational feedback loop phase set by retinal inputs that carry information about environmental light, as well as melatonin secretion from the pineal gland.
Individual differences in response
Comparisons of inter- and intra-individual variability in vulnerability to sleep deprivation have demonstrated that the former are substantially larger than the latter. Van Dongen et al. (2004) measured the intra-class coefficients (ICC) of subjective sleepiness and cognitive performance of participants undergoing three 36-hour periods of sleep deprivation. ICCs were high, with between-subject variance an order of magnitude greater than within-subject variance. These findings indicate that behavioral response to sleep deprivation is trait-like and stable over time. Leproult et al. (2003) also found that subjective and objective (EEG) measures of vulnerability to sleep deprivation were consistent when measured during separate experimental sessions.
Causes and costs
Lack of sleep, both chronic and acute, is a prevalent problem in modern society. For example, of 1.1 million Americans surveyed, as many as 20% indicated that they sleep 6.5 hours or less each night. In its extreme form, pathological levels of sleep deprivation are classified by the International Classification of Diseases (ICD) as insufficient sleep syndrome (ICD #307.49-4). Sleep deprivation can result from physiological deficits (e.g. obstructive sleep apnea ), psychological problems (e.g. secondary insomnia ), or simply reflect a lifestyle choice. Regardless of the source, it remains an under-treated symptom compared to its dramatic and often overlooked costs. For example, the cost of accidents involving sleep-deprived operators has been estimated at $43-56 billion, with almost 20% of serious motor-vehicles crashes occurring because of drowsy drivers. Sleep deprivation has also been implicated with increased risk of on-the-job errors, personal conflicts, health complaints, poorer academic performance in adolescents and increased drug use, to name just a few of its multitudinous consequences. (IOM, 2006).
- Akerstedt T, Palmblad J, de la Torre B, Marana R, Gillberg M (1980) Adrenocortical and gonadal steroids during sleep deprivation. Sleep 3:23-30.
- Allan JS, Czeisler CA (1994) Persistence of the circadian thyrotropin rhythm under constant conditions and after light-induced shifts of circadian phase. J Clin Endocrinol Metab 79:508-512.
- Asken MJ, Raham DC (1983) Resident performance and sleep deprivation: a review. J Med Educ 58:382-388.
- Ayas NT, White DP, Manson JE, Stampfer MJ, Speizer FE, Malhotra A, Hu FB (2003) A prospective study of sleep duration and coronary heart disease in women. Arch Intern Med 163:205-209.
- Baumgartner A, Dietzel M, Saletu B, Wolf R, Campos-Barros A, Graf KJ, Kurten I, Mannsmann U (1993) Influence of partial sleep deprivation on the secretion of thyrotropin, thyroid hormones, growth hormone, prolactin, luteinizing hormone, follicle stimulating hormone, and estradiol in healthy young women. Psychiatry Res 48:153-178.
- Bell-McGinty S, Habeck C, Hilton HJ, Rakitin B, Scarmeas N, Zarahn E, Flynn J, DeLaPaz R, Basner R, Stern Y (2004) Identification and differential vulnerability of a neural network in sleep deprivation. Cereb Cortex 14:496-502.
- Borbely AA (1982) A two process model of sleep regulation. Hum Neurobiol 1:195-204.
- Brunner DP, Dijk DJ, Borbely AA (1993) Repeated partial sleep deprivation progressively changes in EEG during sleep and wakefulness. Sleep 16:100-113.
- Carskadon MA, Dement WC (1975) Sleep studies on a 90-minute day. Electroencephalogr Clin Neurophysiol 39:145-155.
- Chee MW, Choo WC (2004) Functional imaging of working memory after 24 hr of total sleep deprivation. J Neurosci 24:4560-4567.
- Chee MW, Chuah LY, Venkatraman V, Chan WY, Philip P, Dinges DF (2006) Functional imaging of working memory following normal sleep and after 24 and 35 h of sleep deprivation: Correlations of fronto-parietal activation with performance. Neuroimage 31:419-428.
- Dinges DF (2004) Critical research issues in development of biomathematical models of fatigue and performance. Aviat Space Environ Med 75:A181-191.
- Dinges DF, Kribbs NB (1991) Performing while sleepy: Effects of experimentally-induced sleepiness. In: Sleep, Sleepiness and Performance (Monk TH, ed), pp 97-128. Chister, England: Wiley.
- Dinges DF, Pack F, Williams K, Gillen KA, Powell JW, Ott GE, Aptowicz C, Pack AI (1997) Cumulative sleepiness, mood disturbance, and psychomotor vigilance performance decrements during a week of sleep restricted to 4-5 hours per night. Sleep 20:267-277.
- Durmer JS, Dinges DF (2005) Neurocognitive consequences of sleep deprivation. Semin Neurol 25:117-129.
- Everson CA, Crowley WR (2004) Reductions in circulating anabolic hormones induced by sustained sleep deprivation in rats. Amer J Physiol Endocrin Metab 286(6): E1060-E1070.
- Hairston IS, Little MT, Scanlon MD, Barakat MT, Palmer TD, Sapolsky RM, Heller HC (2005) Sleep restriction suppresses neurogenesis induced by hippocampus-dependent learning. J Neurophysiol 94:4224-4233.
- Harrison Y, Horne JA (2000) The impact of sleep deprivation on decision making: a review. J Exp Psychol Appl 6:236-249.
- IOM (2006) Sleep Disorders and Sleep Deprivation: An Unmet Public Health Problem. Washington DC: National Academies Press.
- Irwin M, Mascovich A, Gillin JC, Willoughby R, Pike J, Smith TL (1994) Partial sleep deprivation reduces natural killer cell activity in humans. Psychosom Med 56:493-498.
- Irwin M, McClintick J, Costlow C, Fortner M, White J, Gillin JC (1996) Partial night sleep deprivation reduces natural killer and cellular immune responses in humans. Faseb J 10:643-653.
- Leproult R, Van Reeth O, Byrne MM, Sturis J, Van Cauter E (1997) Sleepiness, performance, and neuroendocrine function during sleep deprivation: effects of exposure to bright light or exercise. J Biol Rhythms 12:245-258.
- Leproult R, Colecchia EF, Berardi AM, Stickgold R, Kosslyn SM, Van Cauter E (2003) Individual differences in subjective and objective alertness during sleep deprivation are stable and unrelated. Am J Physiol Regul Integr Comp Physiol 284:R280-290.
- Linde L, Edland A, Bergstrom M (1999) Auditory attention and multiattribute decision-making during a 33 h sleep-deprivation period: mean performance and between-subject dispersions. Ergonomics 42:696-713.
- Mallis MM, Mejdal S, Nguyen TT, Dinges DF (2004) Summary of the key features of seven biomathematical models of human fatigue and performance. Aviat Space Environ Med 75:A4-14.
- Mignot E (2004) Sleep, sleep disorders and hypocretin (orexin). Sleep Med 5 Suppl 1:S2-8.
- Mirescu C, Peters JD, Noiman L, Gould E (2006) Sleep deprivation inhibits adult neurogenesis in the hippocampus by elevating glucocorticoids. Proc Natl Acad Sci U S A 103:19170-19175.
- Mitler MM, Gujavarty KS, Browman CP (1982) Maintenance of wakefulness test: a polysomnographic technique for evaluation treatment efficacy in patients with excessive somnolence. Electroencephalogr Clin Neurophysiol 53:658-661.
- Mullington JM, Chan JL, Van Dongen HP, Szuba MP, Samaras J, Price NJ, Meier-Ewert HK, Dinges DF, Mantzoros CS (2003) Sleep loss reduces diurnal rhythm amplitude of leptin in healthy men. J Neuroendocrinol 15:851-854.
- Orton DI, Gruzeller JH (1989) Adverse changes in mood and cognitive performance of house officers after night duty. Br Med J 298:21-23.
- Porkka-Heiskanen T, Strecker RE, McCarley RW (2000) Brain site-specificity of extracellular adenosine concentration changes during sleep deprivation and spontaneous sleep: an in vivo microdialysis study. Neuroscience 99:507-517.
- Radomski MW, Hart LE, Goodman JM, Plyley MJ (1992) Aerobic fitness and hormonal responses to prolonged sleep deprivation and sustained mental work. Aviat Space Environ Med 63:101-106.
- Redwine L, Hauger RL, Gillin JC, Irwin M (2000) Effects of sleep and sleep deprivation on interleukin-6, growth hormone, cortisol, and melatonin levels in humans. J Clin Endocrinol Metab 85:3597-3603.
- Saper CB, Scammell TE, Lu J (2005) Hypothalamic regulation of sleep and circadian rhythms. Nature 437:1257-1263.
- Seifritz E, Hemmeter U, Trachsel L, Lauer CJ, Hatzinger M, Emrich HM, Holsboer F, Holsboer-Trachsler E (1995) Effects of flumazenil on recovery sleep and hormonal secretion after sleep deprivation in male controls. Psychopharmacology (Berl) 120:449-456.
- Shearer WT, Reuben JM, Mullington JM, Price NJ, Lee BN, Smith EO, Szuba MP, Van Dongen HP, Dinges DF (2001) Soluble TNF-alpha receptor 1 and IL-6 plasma levels in humans subjected to the sleep deprivation model of spaceflight. J Allergy Clin Immunol 107:165-170.
- Spiegel K, Leproult R, Van Cauter E (1999) Impact of sleep debt on metabolic and endocrine function. Lancet 354:1435-1439.
- Strecker RE, Morairty S, Thakkar MM, Porkka-Heiskanen T, Basheer R, Dauphin LJ, Rainnie DG, Portas CM, Greene RW, McCarley RW (2000) Adenosinergic modulation of basal forebrain and preoptic/anterior hypothalamic neuronal activity in the control of behavioral state. Behav Brain Res 115:183-204.
- Tononi G, Cirelli C (2003) Sleep and synaptic homeostasis: a hypothesis. Brain Res Bull 62:143-150.
- Van Dongen HP, Maislin G, Mullington JM, Dinges DF (2003) The cumulative cost of additional wakefulness: dose-response effects on neurobehavioral functions and sleep physiology from chronic sleep restriction and total sleep deprivation. Sleep 26:117-126.
- Van Dongen HP, Baynard MD, Maislin G, Dinges DF (2004) Systematic interindividual differences in neurobehavioral impairment from sleep loss: evidence of trait-like differential vulnerability. Sleep 27:423-433.
- Weitzman ED, Zimmerman JC, Czeisler CA, Ronda J (1983) Cortisol secretion is inhibited during sleep in normal man. J Clin Endocrinol Metab 56:352-358.
Internal references
- James B. Aimone, Sebastian Jessberger, Fred H. Gage (2007) Adult neurogenesis . Scholarpedia , 2(2):2100.
- Valentino Braitenberg (2007) Brain . Scholarpedia, 2(11):2918.
- Paul L. Nunez and Ramesh Srinivasan (2007) Electroencephalogram . Scholarpedia, 2(2):1348.
- Eugene M. Izhikevich (2007) Equilibrium . Scholarpedia, 2(10):2014.
- Keith Rayner and Monica Castelhano (2007) Eye movements . Scholarpedia, 2(10):3649.
- Seiji Ogawa and Yul-Wan Sung (2007) Functional magnetic resonance imaging . Scholarpedia, 2(10):3105.
- Jeff Moehlis, Kresimir Josic, Eric T. Shea-Brown (2006) Periodic orbit . Scholarpedia, 1(7):1358.
- John Dowling (2007) Retina . Scholarpedia, 2(12):3487.
- Philip Holmes and Eric T. Shea-Brown (2006) Stability . Scholarpedia, 1(10):1838.
- S. Murray Sherman (2006) Thalamus . Scholarpedia, 1(9):1583.
- Olivier Walusinski (2008) Yawn , Scholarpedia, 3(6):6463.
External links
- David F. Dinges's website
Insomnia , Sleep , Sleep Apnea
- Neuroscience
- Multiple Curators
Personal tools
- View source
- View history
- Propose a new article
- Instructions for Authors
- Random article
Focal areas
- Astrophysics
- Celestial mechanics
- Computational neuroscience
- Computational intelligence
- Dynamical systems
- More topics
- Recently published articles
- Recently sponsored articles
- Recent changes
- All articles
- List all Curators
- List all users
- Scholarpedia Journal
- What links here
- Related changes
- Special pages
- Printable version
- Permanent link

- "Sleep deprivation" by Julian Lim and David F. Dinges is licensed under a Creative Commons Attribution-NonCommercial-ShareAlike 3.0 Unported License . Permissions beyond the scope of this license are described in the Terms of Use
- Privacy policy
- About Scholarpedia
- Disclaimers
Sleep deprivation.
Productivity - Effect of sleep deprivation on.
HYPOTHESIS AND THEORY article
Conceptual framework for insomnia: a cognitive model in practice.
- 1 Neurocognitive Engineering Laboratory (NEL), Institute of Mathematics and Computer Science, University of São Paulo, São Carlos, Brazil
- 2 Department of Neuroscience and Behavioural Sciences, Ribeirão Preto Medical School, University of São Paulo, Ribeirão Preto, Brazil
- 3 Neuroscience Center, Instituto de Investigaciones Científicas Servicios de Alta Tecnología (INDICASAT AIP), Panama City, Panama
- 4 Department of Neuroscience, School of Advanced Medical Sciences and Technologies, Shiraz University of Medical Sciences, Shiraz, Iran
- 5 Dana Brain Health Institute, Iranian Neuroscience Society-Fars Chapter, Shiraz, Iran
- 6 Academy of Health, Senses Cultural Foundation, Sacramento, CA, United States
- 7 Department of Cognitive Neuroscience, Institute for Cognitive Science Studies (ICSS), Pardis, Iran
- 8 Reconfigurable Computing Laboratory, Institute of Mathematics and Computer Science, University of São Paulo, São Carlos, Brazil
- 9 Department of Ophthalmology, Otorhinolaryngology, Head and Neck Surgery, Ribeirão Preto Medical School, University of São Paulo, Ribeirão Preto, Brazil
Insomnia is a widespread neuropsychological sleep-related disorder known to result in various predicaments including cognitive impairments, emotional distress, negative thoughts, and perceived sleep insufficiency besides affecting the incidence and aggravation of other medical disorders. Despite the available insomnia-related theoretical cognitive models, clinical studies, and related guidelines, an evidence-based conceptual framework for a personalized approach to insomnia seems to be lacking. This study proposes a conceptual cognitive framework (CCF) providing insight into cognitive mechanisms involved in the predisposition, precipitation, and perpetuation of insomnia and consequent cognitive deficits. The current CCF for insomnia relies on evaluative conditional learning and appraisal which generates negative valence (emotional value) and arousal (cognitive value). Even with the limitations of this study, the suggested methodology is well-defined, reproducible, and accessible can help foster future high-quality clinical databases. During clinical insomnia but not the neutral one, negative mood (trait-anxiety) causes cognitive impairments only if mediating with a distorted perception of insomnia ( Ind-1 = 0.161, 95% CI 0.040–0.311). Further real-life testing of the CCF is intended to formulate a meticulous, decision-supporting platform for clinical interventions. Furthermore, the suggested methodology is expected to offer a reliable platform for CCF-development in other cognitive impairments and support the causal clinical data models. It may also improve our knowledge of psychological disturbances and complex comorbidities to help design rehabilitation interventions and comprehensive frameworks in line with the “preventive medicine” policies.
Introduction
Behavioral sleep disturbances are classified into various types of insomnia, excessive daytime somnolence (EDS), sleep phase disorders, and parasomnias. These are potentially rooted in psychophysiological, cognitive, emotional, and behavioral abnormalities resulting in impaired sleep efficacy, disintegrated sleep cycles, and/or arousal instability ( Cormier, 1990 ; Sateia, 2014 ). Insomnias are characterized by poor subjective sleep quality, difficulty in falling asleep and maintaining sleep at bed-time, wakes after sleep onset (WASO), or unprompted early morning awakening. The consequent diurnal symptoms may then present as inadequate cognitive functions, declined cognitive aptitude, fatigue, hampered productivity, depression or irritability, impaired decision-making, low motivation, and mood dysregulation ( Mai and Buysse, 2008 ; Nami, 2014 ).
Recently, cognitive-vulnerability models, which theoretically justify the interrelation between sleeplessness and mood dysregulation or cognitive insufficiencies, have drawn the attention of the research community. When insomnia becomes a chief complaint, the vicious cycle of insomnia-anxiety-insomnia starts to emerge. Undeniably, affective dysregulation, impulsivity, restlessness, EDS, disrupted vigilance, and cognitive decline are some consequences of long-term sleep insufficiency in many instances ( Nami, 2014 ).
Among the theoretical and cognitive-computational models related to insomnia, the cognitive vulnerability model for insomnia induced mood disturbances (CVMIMD), the sleep-specific cognitive vulnerability (SSCV), the behaviorally induced insufficient sleep syndrome with restricted and extended sleep opportunity (BIISS-RESO), and the global cognitive vulnerability to insomnia (GCVI) ( Bei et al., 2015 ) are the main highlights. These models are addressed subsequently.
Cognitive Vulnerability Model for Insomnia Induced Mood Disturbances
From the neurocognitive standpoint, the prefrontal cortex (PFC), which plays a pivotal role in affect-regulation and cognitive-control, develops intensely throughout the neurodevelopment phase and adolescence owing to neuroplasticity. When the hypnic tone is decreased either due to poor sleep hygiene or socio-behavioral and psychophysiological stressors, a proposed explanation is the activation of PFC’s maladaptive processes as a potential neurocognitive mechanism underlying the affective consequences of insomnia and inefficient sleep, in general ( Freeman et al., 2005 ).
The body of psycho-behavioral and neurocognitive empirical evidence describing the precise mechanisms that underlie the link between insomnia and negative mood is thin. However, subjective sleep insufficiencies and dysregulated mood observations exhibited more robust relationship as compared to objective findings from polysomnography or even full-setup sleep electroencephalography data. This points to the fact that psychological factors that hinder sleep efficiency might play significant roles in justifying the sleep-mood crosstalk. Yet some of these insomnia-related cognitive vulnerability factors are now acknowledged as erroneous beliefs, cognitive biases, and thought patterns that increase the likelihood of the predisposed individuals toward psychopathology ( Freeman et al., 2005 ).
Sleep Specific Cognitive Vulnerability
In some instances, the erroneous beliefs and attitudes represent exclusive sleep-related problems in which case, the distressing worries related to insomnia-continuation are usually evaluated using the dysfunctional beliefs and attitudes about sleep (DBAS) Scale. Harvey’s cognitive model ( Harvey, 2002 ) described the impact of the DBAS-related cognitive vulnerability on insomnia complaints. According to this model, insomniacs are generally worried about poor sleep and its daytime consequences, and such strong, negatively toned thoughts trigger selective attentional-emotional bias, wherein individuals over-monitor their sleep-related threat cues. Previous investigations proposed a strong connection between DBAS and poor sleepers, which happens to play a key role in DBAS-driven disturbances in sleep perception and sleep safety behaviors such as napping ( Harvey, 2002 ).
Behaviorally Induced Insufficient Sleep Syndrome With Restricted and Extended Sleep Opportunity
This condition refers to a typical complaint reported by the patients as “ at nights I cannot sleep, in the morning, I cannot wake up .” Habitual sleep episodes are usually shorter (confirmed by history, sleep log, or actigraphy) for patients experiencing initial or maintenance insomnia compared to the normative values from age-adjusted groups. Such patients also report sleep-inertia in the morning and complain about EDS for a minimum of 3 months before the interview. However, they tend to sleep considerably longer on weekends or during vacation. In general, the reported objective sleep efficiency as detected by polysomnography is below 80%, besides the mean initial nocturnal sleep latency which takes a longer time, more than 45 min. Also, these patients report repeated WASOs ( Bastien et al., 2008 ).
Global Cognitive Vulnerability to Insomnia
Cognitive vulnerability is defined as global when the dysfunctional beliefs and attitudes are general and not necessarily focused on a distinct behavioral or experiential area. According to Beck’s cognitive model, psycho-traumas in the early years of life combined with a complicated past can foster negative attitudes and biases concerning both self, world and the future. Such beliefs yield maladaptive schemas that may trigger cognitive vulnerabilities and negative tendencies based on depression later in life ( Beck, 2008 ). A few studies on GCVI suggest strong links between sleep predicaments (mainly insomnias) and negatively toned cognitive constructs. For instance, complaints of chronic insomnia in young adults were found to be associated with anxiety and depression-related cognitive factors ( Alfano et al., 2009 ). In the same vein, Sadler et al. (2013) claimed hopelessness, a global cognitive-vulnerability factor in older adults, can amplify the effects of insomnia on depressive symptoms ( Sadler et al., 2013 ).
The various types of insomnia (more than 10) require personalized treatment approaches. Some of the broadly described types include adjustment insomnia, drug or substance-induced insomnia, comorbid insomnia, onset insomnia, middle insomnia, late insomnia, conditioned, or psychophysiological insomnia, behavioral insomnia of childhood, idiopathic insomnia, paradoxical insomnia, and sleep hygiene insomnia ( Dzierzewski et al., 2018 ). Based on the severity of sleep insufficiency, we have categorized insomnia patients into Neutral (with a mild to moderate perception of sleep difficulties) and Clinical (with a severe perception of sleep difficulty) types.
Gross (1998) designed the modal model of emotion as a conceptual framework to illustrate how emotions can be generated and evolve over time. The emotion-generation process begins with internal or external goal-relevant situations that draw attention to specific features of the situation, appraisals emerge to make meaning of the situation resulting in multi-faceted emotional responses and feedback to modulate the current situation perpetually. Collectively, the modal model reflects the dynamic nature of the emotions and suggests possible emotion-regulation strategies comprising of situation selection, situation modification, attention deployment, cognitive change, and response modulation ( Gross, 2013 ).
To the best of our knowledge, the aforementioned studies have little mention of causality (mediation) relationships, which can easily mislead interpretations of the findings. Thus, necessitating the design of an approach to conceptualize these theories and hypotheses. A novel insomnia theoretical-conceptual framework would enable the drawing of data models for testing mediational relationships between independent variables and outcomes within retrospective studies. Besides, it may also help suggest research strategies and predictions designing prospective studies on insomnia. The present study aims to fill this void in the literature by proposing and validating a novel conceptual cognitive framework (CCF) for insomnia in light of the above-mentioned models. The CCF illustrates how cognitive processes and their interactions can generate annoyance-distress reactions, which in turn, lead to the development or maintenance of insomnia. The insomnia numerical model is also demonstrated through multi-mediatory (causality) modeling approaches.
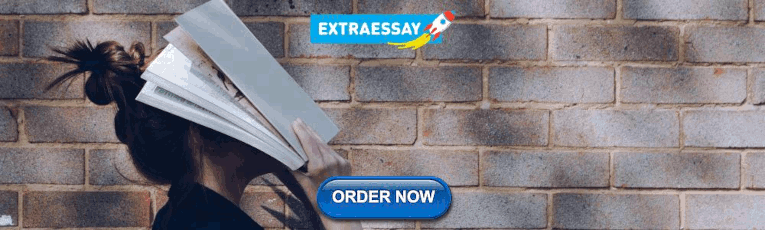
Proposed Conceptual Cognitive Framework
Fundamental ideas and postulations of the conceptual cognitive framework.
• Conceptual cognitive framework aims at illustrating the interaction between cognitive processes that cause annoyance-distress reactions in insomnia.
• Conceptual cognitive framework rests mainly on evaluative conditioning, assuming a conscious attended awareness perception (CAAP) to both unconditioned stimulus (US) and conditioned stimulus (CS), and their contingencies essential for attitude formation.
• Either or both, cognitive-value and emotional-value, can cause annoyance; however, they can also affect each other merely through annoyance. Furthermore, annoyance distorts the corresponding perception of sleep quality by affecting cognitive and emotional values.
• Lower levels of cognitive-emotional values such as those encountered in the Neutral stage might generate annoyance, yet not sufficient enough to trigger distress reactions. Consequently, annoyance and distress are considered two different concepts in the current framework.
• Cognitive processes for sleep-initiation and sleep-maintenance difficulties are presumed to occur analogously.
Hypothetically, CCF compartments include situation, attention bias, cognitive value (arousal), emotional value (valence), annoyance-distress reaction, and distorted perception. The proposed CCF aims at illustrating how the interaction among cognitive processes contributes to distress reactions.
This paper focuses on insomnia experienced before sleep, and the associated “situation” is restricted to the night-time silence period. According to the CCF, when insomnia-related stimuli capture attentional resources, either directly or through corresponding cognitive and emotional values, distress is triggered resulting in a distorted perception. Distress, in turn, feeds back and influences the situation. Similarly, distress reaction fuels back corresponding cognitive and emotional values. The proposed CCF is illustrated in Figure 1 .
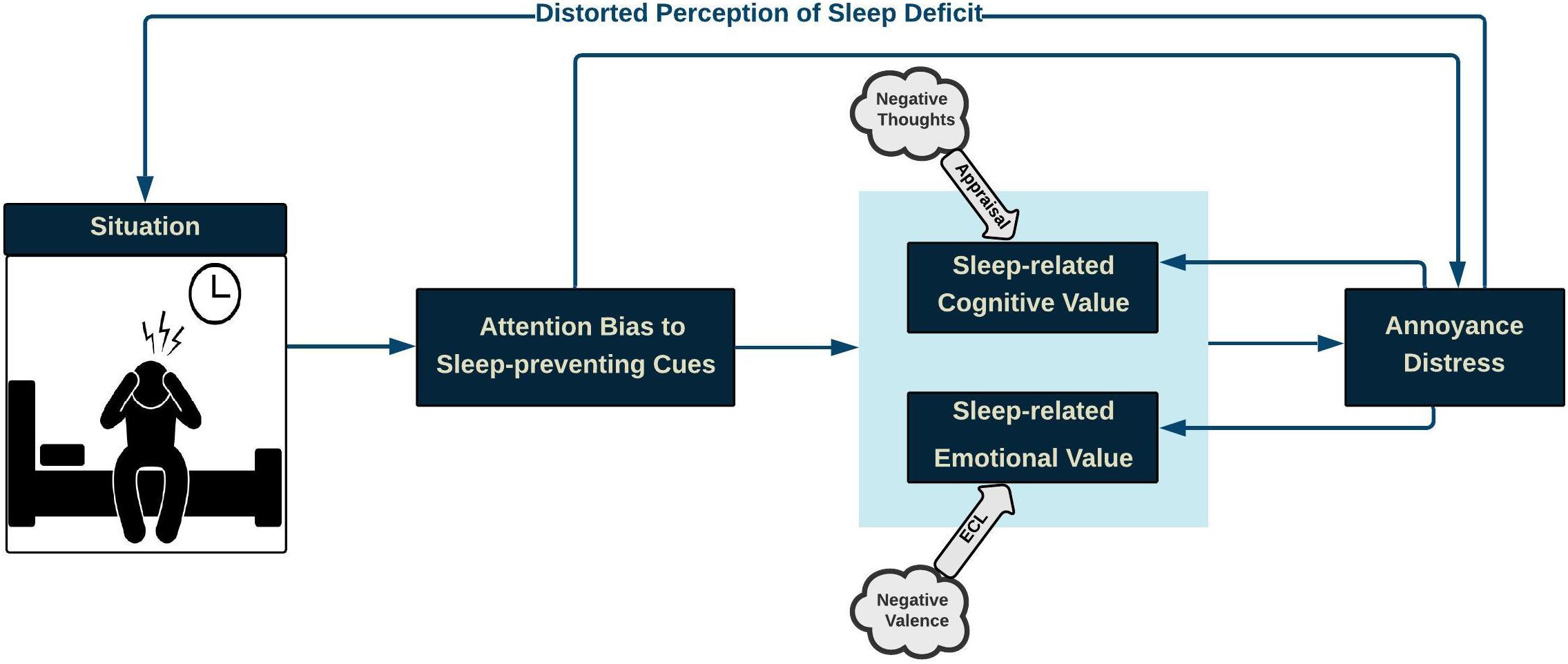
Figure 1. Conceptual Cognitive Framework of Insomnia: During the pre-sleep situation, when attentional resources are captured by insomnia-related stimuli, either directly or through insomnia-related cognitive and emotional values, distress is triggered, thus resulting in a distorted perception of sleep quality, which, in turn, worsens the sleep-initiation process. Likewise, insomnia distress strengthens the negative cognitive-emotional value of difficulty in sleep.
Toward providing a proof of concept for the proposed CCF, we primarily present some supporting evidence from the Insomnia literature.
Compartments and Cognitive Processes
Nighttime silence in the pre-sleep period can facilitate CAAP of internal (body sensation or thoughts) and external (environmental sounds, light, and heat) stimuli. Bootzin and Rider (1997) noted that “bedtime may often be the first quiet time during the day available to think about the day’s events and to worry and plan for the next day.” Therefore, bed and bedtime tend to be cues for arousal rather than for sleep.
Attention Bias
Consciously attended internal and external stimuli develop an individual’s predictions and expectations from the pre-sleep situation. Therefore, an attention bias takes place if any novelty or change occurs in the features of such stimuli ( Horstmann and Herwig, 2016 ). Similarly, Roberts et al. (2013) have supported the notion that “discrepancy between an expectation and upcoming stimuli can bias attention” ( Horstmann and Herwig, 2016 ). Additionally, emotion-laden or threat-related stimuli would be prioritized over other stimuli leading to an attentional bias. The same rationale applies to cognitive theories of anxiety disorders ( Beck and Clark, 1997 ), according to which prioritized attention-allocation to threat cues would trigger the development and maintenance of anxiety ( Dalgleish and Watts, 1990 ). Threat cues for patients with insomnia might be related to sleep quality (arising secondarily to bodily sensations such as palpitation, muscle tension, or attention bias toward noises outside and inside the house), which impairs the process of falling asleep.
One of the commonly used paradigms for experimental assessment of attentional bias is the Dot-Probe task. In this, a pair of stimuli (e.g., words or pictures) are presented simultaneously at different locations (up/down or top/bottom) on the screen. The stimuli pair disappear after a fixed time window and a probe appears in the location of emotional (congruent presentations) or neutral (incongruent presentations) stimuli. Subjects are asked to detect and respond to the location of the probe as fast as possible, and the attentional bias is measured through their reaction time in responding to the probe location. A faster probe detection for congruent trials is believed to indicate vigilance, and a slower probe detection for the incongruent trials is suggestive of difficulty in disengaging attention from emotional stimuli ( Koster et al., 2004 ).
Several studies have investigated the impact of the emotional-attentional bias on sleep-related threatening cues through different attentional paradigms, including Dot-probe ( MacMahon et al., 2006 ; Jansson-Fröjmark et al., 2012 ), flicker ( Jones et al., 2005 ), Posner ( Woods et al., 2009 ), emotional Stroop ( Barclay and Ellis, 2013 ), and eye-tracking ( Woods et al., 2013 ). Most of these studies have endorsed the notion that poor sleepers display attentional bias to sleep-related cues compared with controls. Jansson-Fröjmark et al. (2012) used a dot-probe task to demonstrate that individuals with primary insomnia had a considerably prolonged reaction-time when shifting attention away from insomnia-associated pictures paired with neutral pictures, in comparison to neutral-neutral paired picture presentations as control. Their findings suggest that insomniacs have more difficulty in shifting attention away from insomnia-related stimuli, but are not more vigilant to those stimuli than normal sleepers ( Jansson-Fröjmark et al., 2012 ). However, results reported by Spiegelhalder et al. (2010) yielded no statistically significant preferential attentional-allocation to sleep-related stimuli. Inconsistent results from studies on insomnia may have emerged due to confounding factors and possible bias, impeding their methodologies and study design.
Emotional Value
The emotional value gets shaped through the evaluative conditional learning (ECL) mechanism which plays a crucial role in liking and disliking stimuli ( Ghodratitoostani et al., 2016a , b ). Based on ECL, neutral stimuli (CS) can obtain either positive or negative valence after being repeatedly paired with emotion-laden stimuli (US) ( De Houwer et al., 2001 ). Valence represents emotional states varying along a spectrum, ranging from positive to negative feelings with a neutral center-point ( Bradley and Lang, 1994 ). Based on the CCF, CAAP of both CS and US, and their contingencies are required at the time of EC-learning formation. Additionally, evaluative conditioning is an accumulative procedure through which different valenced USs can add to CS valence after being repeatedly paired ( Stahl and Unkelbach, 2009 ). Therefore, EC-learning is resistant to extinction so that neither individual CS/US presence alone, nor pairing CS with different USs would cause the extinction of previously shaped evaluative conditioning ( De Houwer et al., 2001 ). Applying the CCF, the ECL mechanism suggests that the negative valence of other USs fuels a negative sleep-related emotional-value leading to annoyance or distress reaction. Different negative USs can also frequently get paired with internal (bodily sensations) and external (environmental sounds, light, or heat) sleep-preventing stimuli. Thereafter, attending to sleep-preventing cues alone might trigger distress reactions due to the learned USs’ valence.
Cognitive Value
The cognitive value related to internal and external stimuli is built through an appraisal process. This process initiates when the meaning of an object or event is evaluated in a particular situation according to pre-existing beliefs, desires, and intentions ( Scherer et al., 2001 ). However, not all information but that relevant to individuals’ concerns ( Frijda, 1987 ), can trigger a cognitively aroused state followed by the appraisal. Accordingly, attention bias to sleep-preventing cues (as concern-relevant stimuli) can trigger a cognitively aroused state with subsequent appraisals about insomnia, “ I am never going to get to sleep ,” “ I am not coping with the amount of sleep I get ,” and “ I am going to lose my job ” ( Harvey, 2002 ). Negative thoughts through this appraisal mechanism further fuel the negative sleep-related cognitive value, leading to annoyance or distress reaction.
Self-reported questionnaires are widely used for collecting patients’ thoughts and beliefs about events, situations, or objects that require conscious appraisals of conditions, and their corresponding consequences. Pre-Sleep Arousal Scale ( Nicassio et al., 1985 ), Sleep Disturbance Questionnaire ( Espie et al., 1989 ), and DBAS Scale ( Morin, 1993 ) are commonly applied for assessing thoughts and beliefs related to insomnia. The latter is greatly helpful in clinical practice since it distinguishes salient irrational, and often emotionally loaded thoughts that disturb sleep onset. Nicassio et al. (1985) and Lichstein and Rosenthal (1980) evaluated the intensity of cognitive and somatic arousal at bedtime through the Pre-Sleep Arousal Scale and reported cognitive arousal was more strongly associated with sleeping difficulty. Similarly, Espie et al. (1989) used the Sleep Disturbance Questionnaire and observed “ My mind keeps turning things over ” and “ I am unable to empty my mind ” were the most often endorsed statements among insomniacs ( Espie et al., 1989 ).
Several authors have assessed characteristics of pre-sleep thoughts in terms of content ( Harvey, 2000 ; Wicklow and Espie, 2000 ), frequency ( Barclay and Gregory, 2010 ), and valence ( Kuisk et al., 1989 ). For instance, Wicklow and Espie (2000) conducted an experimental study on people with clinically significant sleep difficulties using audiotape to record their pre-sleep thoughts and wrist-actigraphy to obtain sleep patterns. The authors indicated that the more frequent thoughts were related to “rehearsing, planning and problem-solving” and “sleep and its consequences,” which strongly correlated with unpleasant emotions and could predict objective sleep latency. Contrarily, Barclay and Gregory (2010) observed that the orientation of catastrophic thoughts in poor sleepers may not be necessarily sleep-specific, instead it was linked to a general tendency to be in an iterative manner regardless of the content or emotional valence. Sleepers were asked to catastrophize their thoughts into three topics namely sleep quality, current personal worries, and hypothetical positive topics. Poor sleepers exhibited greater catastrophic thoughts on every single topic in comparison with good ones, however, no difference was observed in occurrence of catastrophic worry about each topic among poor sleepers. Davey and Levy (1998) suggested that the tendency for repetitive thinking in insomniacs is similar to that of worriers who hold dysfunctional beliefs about the benefits of worrying. In other words, insomniacs believe the ongoing worry helps them find solutions and prevent adverse outcomes. Using DBAS, Morin et al. (1993) reported that not only excessive cognitive activity, but the valence of thoughts also plays a crucial role in provoking emotional reactions to sleep impairment.
Annoyance-Distress Reaction
Consistent with many cognitive-behavioral studies, the CCF suggests that negative appraisals of insomnia trigger the annoyance-distress reactions. According to the cognitive model of insomnia, excessively negative thinking in the pre-sleep time provokes autonomic arousal, and emotional distress ( Harvey, 2002 ). Tang and Harvey (2004a) have reported that the manipulation of psychological and physiological arousal produces adverse effects on the perception of sleep quality. For illustrative purposes, Baglioni et al. (2010) presented five blocks showing neutral, negative, positive, sleep-related negative and sleep-related positive pictures to evaluate the psychophysiological reactivity to emotional stimuli, both related and unrelated to sleep, in people with primary insomnia and normal sleepers. facial electromyography, heart rate, and cardiac vagal tone were recorded during the picture presentation. The insomnia group indicated an enhanced physiological “craving” response for positive sleep stimuli (e.g., picture of a person asleep in bed), prolonged physiological arousal in response to all stimuli, and increased subjective arousal for negative sleep stimuli (e.g., picture of a person lying awake in bed) when compared to normal sleepers ( Baglioni et al., 2010 ).
Distorted Perception
According to the CCF, valence and cognitive-arousal as two components of emotion can affect patients’ judgment about sleep quality perception. The following findings lend support to this proposal.
Yoo and Lee (2015) explored the effect of modulating arousal and valence on time-perception in subjects with social anxiety, comparing the time duration of the presented stimuli with the standard duration in training sessions. The perceived duration of negative-stimuli against positive-stimuli was longer with high arousal, but shorter with low arousal levels, suggesting that modifications in the type and magnitude of both valence and arousal modulate time-perception ( Yoo and Lee, 2015 ). This may also be analogous to the distortion in sleep quality-perception in insomniacs.
Using self-reported subjective sleep quality, Tang and Harvey (2004a) observed that experiencing anxious cognitive and physiological arousal in the pre-sleep period resulted in the perception of a longer sleep-onset latency and shorter total sleep time. Moreover, actigraphy results showed contradictions to the reported subjective sleep quality, thus corroborating distorted perception ( Tang and Harvey, 2004a ).
On the contrary, Herbert et al. (2017) inspected the psychophysiological predictors of subjective/objective sleep discrepancy in Total Sleep Time ( Manconi et al., 2010 ) and Sleep Onset Latency ( Herbert et al., 2017 ) indices among poor sleepers. They reported that excessive pre-sleep cognitive activity and lower mood at the awakening time of the following day are predictors of distortion in time estimation.
Hypotheses of Conceptual Cognitive Framework
The primary speculation was that the CAAP of internal and external sleep-preventing stimuli captures attentional resources preferentially and triggers the appraisal process, ending with annoyance and distress in the pre-sleep situation. A secondary hypothesis was that intermittent distress experienced in the Clinical stage leads to a misperception about sleep quality.
We applied the multi-mediation insomnia model based on clinical data toward putting the CCF into practice and provide supporting evidence for the proposed causality relationship between the cognitive processes in different stages of insomnia.
For the CCF assessment, data were collected from the participants of (1) a randomized crossover three-session double-blind study and (2) an observational prospective cohort study. Both studies were approved by the Ethics Committee for Analysis of Research Projects, Specialized Center of Otorhinolaryngology and Speech Therapy, Hospital das Clínicas de Ribeirão Preto, University of São Paulo, Brazil (HCRP no 55716616.1.1001.5440, and HCRP no 09813519.1.0000.5440; internationally registered with U1111-1236-5441). All participants gave written informed consent.
Two-hundred fifty-three participants (123 female, 130 male) aged 27–72 years (54.43 ± 10.31 years) were recruited for this study. Before the sessions in both studies, participants filled up a Portuguese version of a battery of questionnaires that included (a) a six-item state-trait anxiety inventory (STAI) ( Gorenstein and Andrade, 1996 ) for measuring the presence and severity of anxiety symptoms in the current moment (State anxiety) and a generalized predisposition to be anxious (Trait anxiety), and (b) a mini-sleep questionnaire (MSQ) ( Falavigna et al., 2011 ), i.e., a short screening for sleep disturbances in clinical populations for the assessment of insomnia and sleep difficulties ( Table 1 ). Table 1 shows the items selected from each questionnaire for the development of the insomnia Mediator-Causality model.
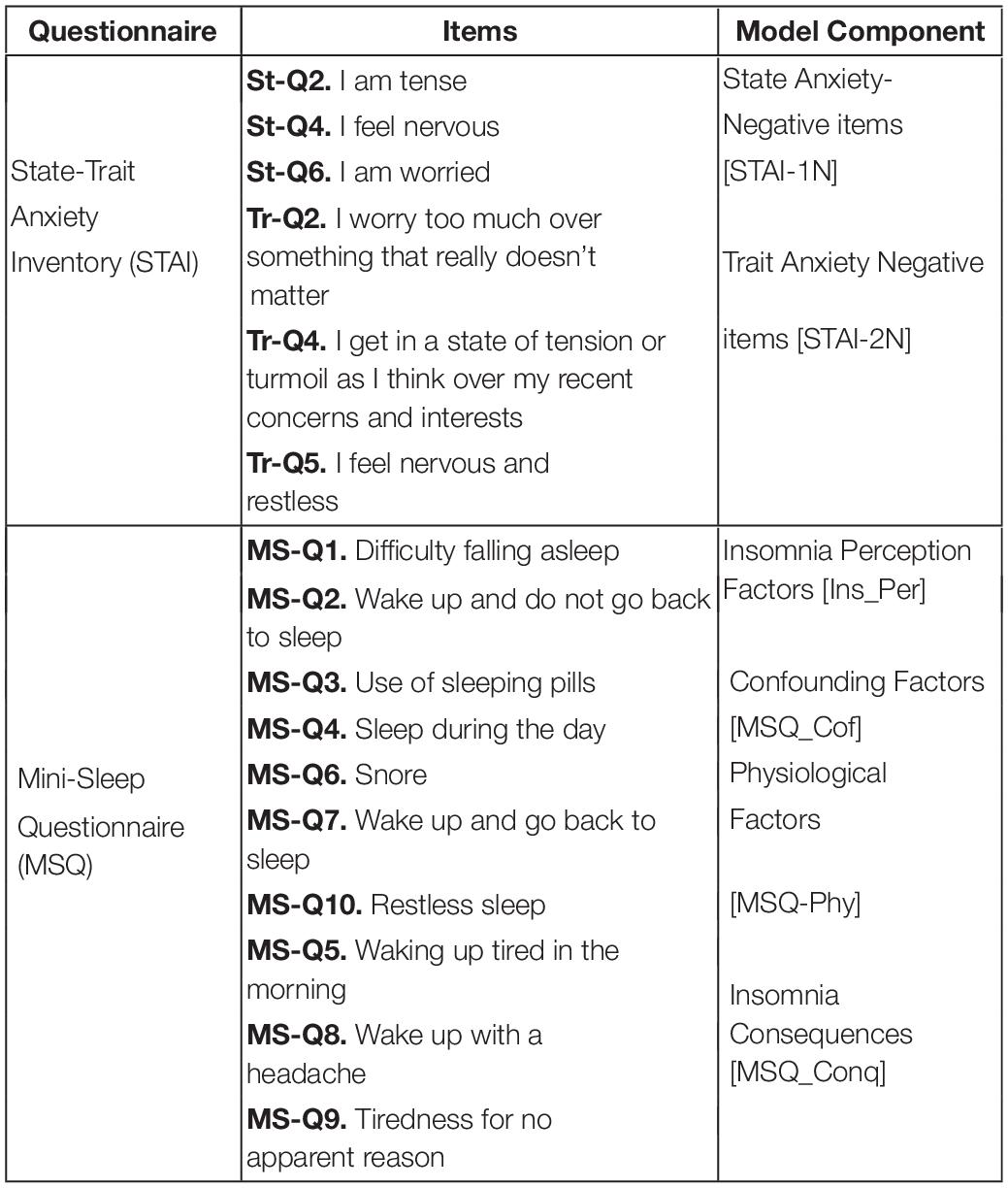
Table 1. List of selected questions from state-trait anxiety inventory ( Gorenstein and Andrade, 1996 ) and mini-sleep questionnaire ( Falavigna et al., 2011 ) for each model’s component.
Pre-processing of the Data
The data were anonymized to ensure blinding. Initially, those with missing values were omitted, which resulted in 112 and 134 session-wised questionnaires from the first and second studies, respectively. The datasets were then aggregated and segmented based on the insomnia severity stage. For insomnia, scores of the MSQ-questionnaire lower than 30 (MSQ-R < 30; mild to moderate sleep difficulties) were labeled as Neutral insomnia, and MSQ-R ≥ 30 (severe sleep difficulty) were denoted Clinical insomnia ( Natale et al., 2014 ). Such segmentations provided two sub-datasets (Neutral Insomnia, and Clinical Insomnia) for the statistical analysis. Figure 2 illustrates the correlation matrix of the variables in the mediator model.
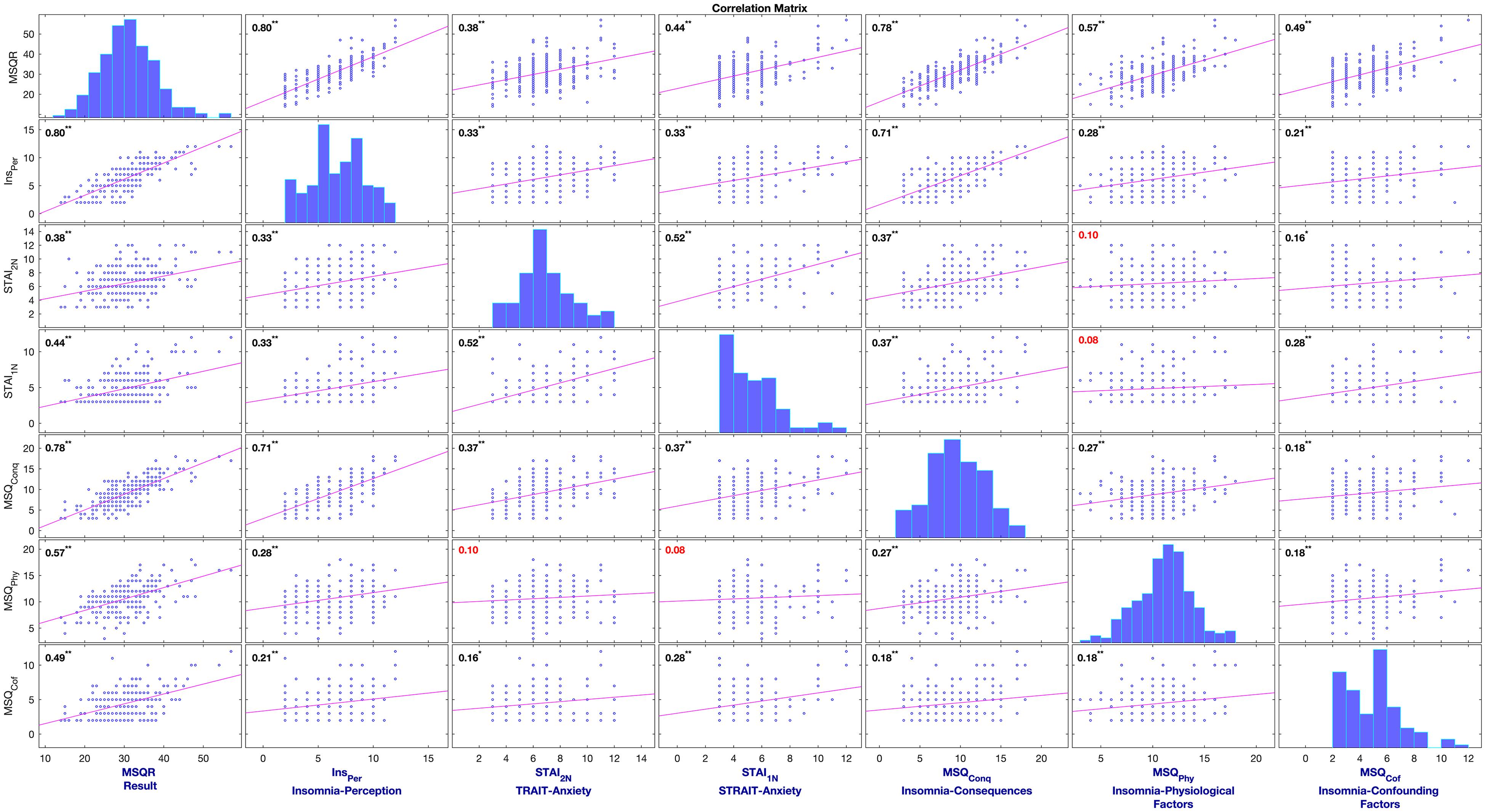
Figure 2. Correlation Matrix of variables used in the multi-mediation model of insomnia to support CCF of insomnia.
Statistical Analysis
Every segment of the dataset was tested for multicollinearity/autocorrelation by the Durbin-Watson test and showed independence in residuals in general. SPSS v.26 and PROCESS macro ( Hayes, 2017 ) were used for the data analysis. Within the macro, customized models and 5.000 bias-corrected bootstrap samples were set for all tests with the fixed random-seed (“ 12020 ”). A 95% confidence level was chosen, with significance at for P < 0.05 was set. A hierarchical regression analysis investigated the evidence for insomnia CCF within the data-segments, and multiple mediation models were constructed for determining the mediating effects of insomnia-related cognitive items and emotional factors for insomnia. PROCESS macro generated standard errors, P -values, and confidence intervals for direct effects, as well as bootstrap confidence intervals for conditional indirect effects.
Datasets and analyzed details are available on “Zenodo” repository with the doi: http://doi.org/10.5281/zenodo.4145224 .
Fundamental Ideas and Postulations for Mediator Models
• Insomnia-perception-factors (Ins_Per) variable contains difficulty in sleep initiation and maintenance.
• The employed dataset was unable to test hypotheses related to the distorted perception of sleep quality.
Proposed Mediator Model
The insomnia mediator model aims to illustrate that negative trait-anxiety can affect the perception of deficits in sleep quality. Concurrently, Insomnia-perception-factors can directly or through state-anxiety affect insomnia consequences . The insomnia model is depicted in Figure 3 .

Figure 3. Insomnia mediator model includes the direct effect of trait-anxiety on Insomnia consequences (I c’ ); Ind-1 [ I 01 → I 10 ]: Trait-anxiety → Insomnia perception factors → Insomnia consequences; Ind-2 [ I 01 → I 12 → I 20 ]: Trait-anxiety → Insomnia perception factors→ State-anxiety→ Insomnia consequences; Covariates: Confounding factors and Physiological problems.
Several studies introduced trait-anxiety as an important predisposing factor for both the development and maintenance of insomnia ( Sadigh et al., 2014 ; Bavafa et al., 2018 ; Lauriola et al., 2019 ). Harvey (2002) argued that anxious individuals tend to interpret ambiguous situations in a threat-related fashion which, in turn, promotes over-thinking about sleep-related threat cues. This process maintains individuals in a cognitively aroused state which is in contradiction to the relaxed state needed for getting to sleep ( Harvey, 2002 ; Lancee et al., 2017a ).
Multi-mediation regression analysis with the conventional least-squares method revealed that trait-anxiety can only indirectly influence the insomnia consequences. As shown in Figure 3 and Table 2 , in the full-dataset, trait-anxiety can lead to insomnia consequences through either insomnia perception, or cascade mediators from insomnia perception to state-anxiety. The 95% confidence interval of bootstrap results revealed “ Ind-1 ” [ I 01 × I 10 = 0.303] and “ Ind-2 ” [ I 01 × I 12 × I 20 = 0.025] were significantly different from zero (0.183–0.432) and (0.006–0.057), respectively, but there was not enough evidence for trait-anxiety ( I c ′ = 0.088, P = 0.32) that might directly lead to insomnia consequences.
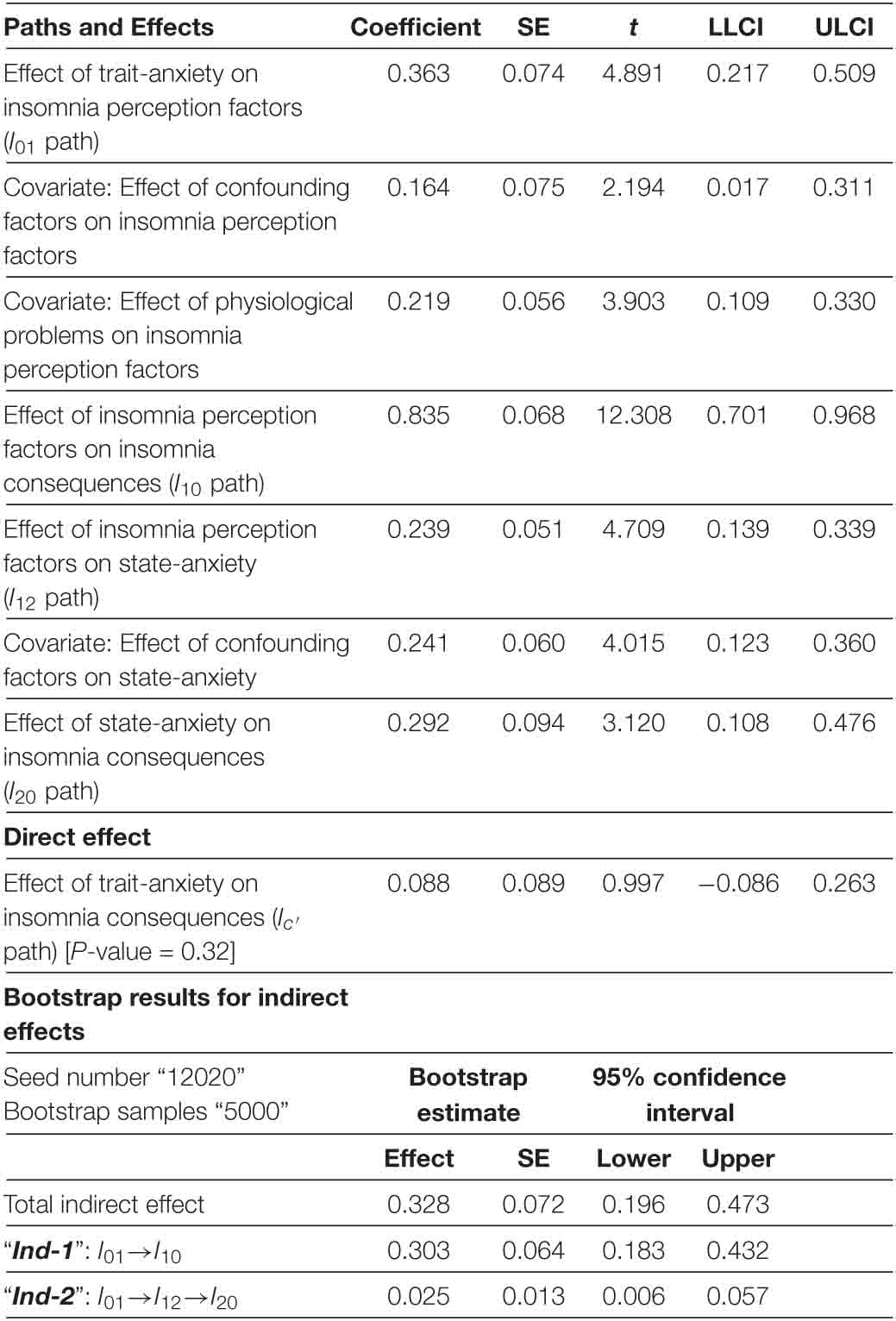
Table 2. Mediator model of insomnia in full-dataset.
According to Table 3 , trait-anxiety in the Clinical insomnia segment leads to insomnia consequences only through insomnia perception. The 95% confidence interval of bootstrap results revealed a significant difference in “ Ind-1 ” [ I 01 × I 10 = 0.161] different from zero (0.040–0.311), but not in “ Ind-2 .” Moreover, there was not enough evidence of trait-anxiety ( I c ′ = 0.2, P -value = 0.104) might directly lead to insomnia consequences.
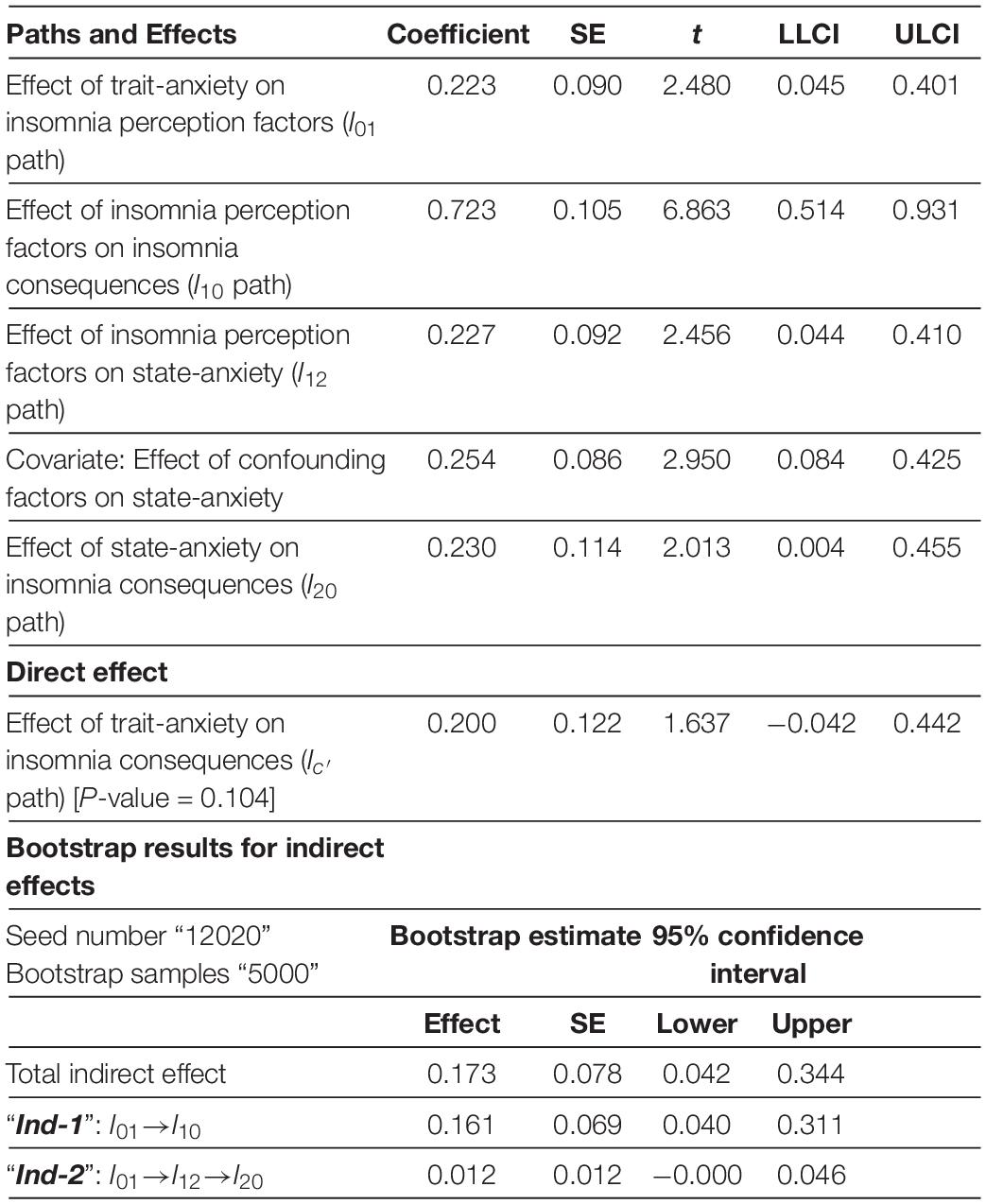
Table 3. Mediator model of insomnia in clinical insomnia segment.
Table 4 shows trait-anxiety neither directly, nor indirectly can lead to insomnia consequences within the Neutral insomnia segment.
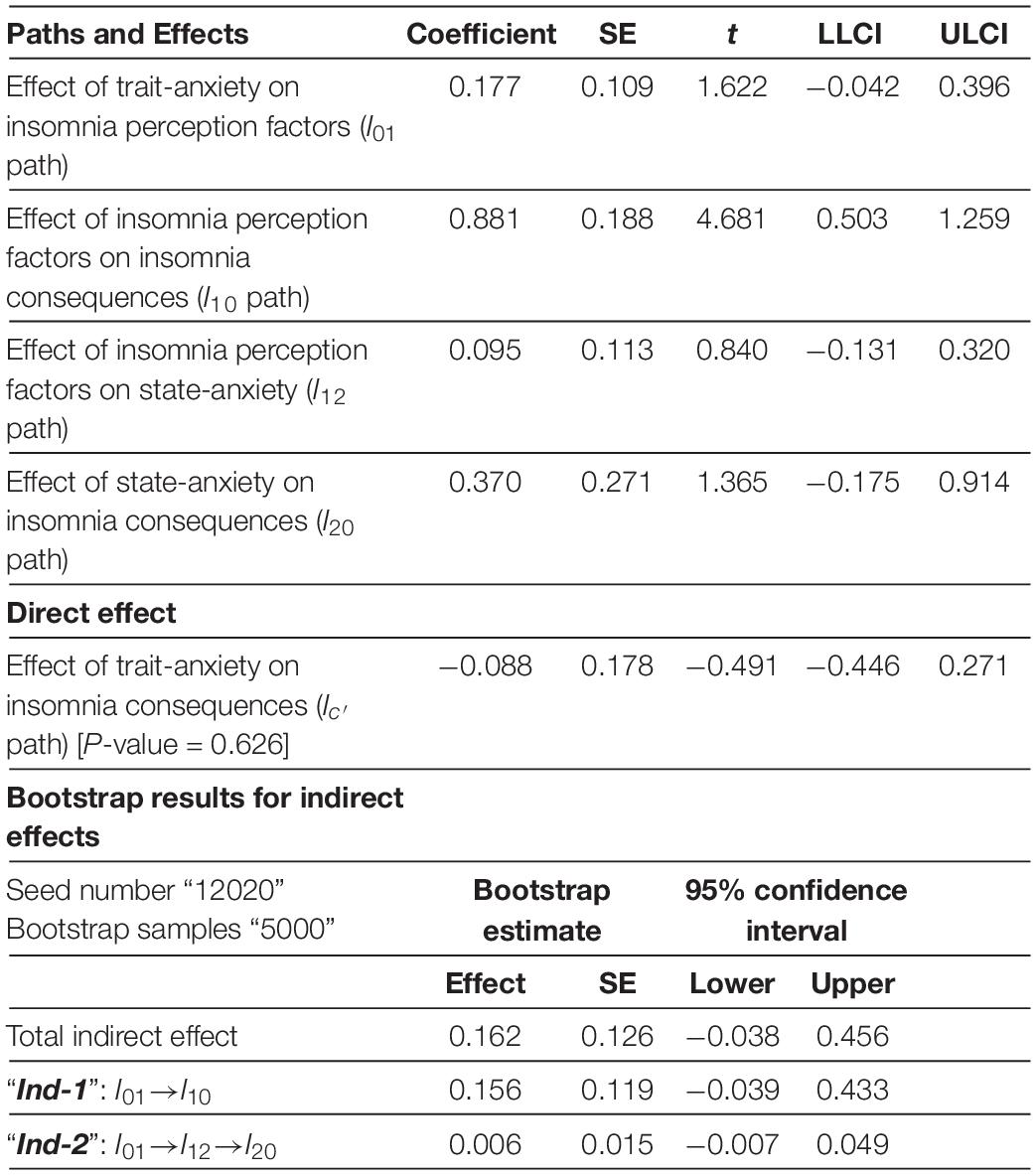
Table 4. Mediator model of insomnia in neutral insomnia segment.
Clinical Implications
Insomnia per se is a clinical issue with short-term and long-term consequences affecting both physiological and psychological systems. It has been associated (at least partly in terms of duration and severity) with increasing the incidence and worsening the state of many pre-existing clinical conditions ( Schutte-Rodin et al., 2008 ). Hence, the need for formulating a conceptual and concurrently pragmatic framework toward an individualized approach for people suffering from insomnia.
The proposed CCF, together with insomnia mediator models, explained the contribution of cognitive processes to the development and maintenance of clinical insomnia. The CCF proposes the following predictions and target-oriented clinical implications:
Decreasing Attentional Bias
Conceptual cognitive framework predicts that attentional bias modification (ABM) training can decrease the attentional bias to insomnia. In practice, the ABM treatment encourages the insomniacs to shift attention away from the negative sleep-related words toward a neutral one, thus reducing attention bias toward sleep-related threatening cue. Such a simple task enables patients to consciously and repeatedly select unbiased information over negative information, thereby progressively help to develop a tendency to not focus on negative information related to insomnia in their daily life ( Clarke et al., 2016 ).
Milkins et al. (2016) conducted a crossover study in which 18 insomniacs alternatively fulfilled an ABM task and a non-ABM control task before sleep across six successive nights. At nights on which the subjects performed the ABM task, they reported shorter sleep-onset latencies and lower pre-sleep worry, than the nights on which they performed the control task. Likewise, in a parallel design ( Clarke et al., 2016 ), 36 students with sleep problems underwent ABM or control training sessions across five nights. Compared with the control condition, subjects who underwent ABM training reported less pre-sleep arousal, fell asleep faster, woke less often during the night, reported better overall sleep quality, and had significant reductions in sleep-related anxiety ( Clarke et al., 2016 ). These findings support the above-mentioned proposition.
In contrast, the results of Lancee et al. (2017b) showed no added benefit of the ABM training over the placebo training on sleep-related indices and outcome measures. The authors believe it was probably due to the absence of attentional bias at baseline and hence no change could be deduced after training ( Lancee et al., 2017b ).
Employing Attention-Distraction Techniques Can Help Deviate Attention From Concerns-Relevant Topics to Neutral Ones
Addressing the issue of attentional bias toward relevant topics, Haynes et al. (1981) observed that engagement with a challenging mental arithmetic problem reduced subjective sleep latency among insomniacs ( Haynes et al., 1981 ). Similarly, practicing crossword puzzles, reading, and listening to audiobooks could provide sufficient distraction so that the patient would no longer attend to or think about their inability to sleep. Troxel et al. (2012) recommended patients should keep doing those activities until they feel sleepy enough to return to bed. And, if they cannot fall asleep after returning to bed, the process should be repeated ( Troxel et al., 2012 ).
Preventing Annoyance and Distress-Reaction
Conceptual cognitive framework draws attention to the crucial role of appraisal and ECL mechanisms in reducing negative cognitive and emotional value.
Cognitive-behavioral therapy (CBT) to reduce the negative cognitive-value related to insomnia
Sleep difficulties are commonly accompanied by dysfunctional beliefs, unrealistic expectations, and worries, which contribute to distress and maladaptive sleep habits producing an anxious state opposite to the relaxation required for sleeping. Therefore, patients’ beliefs regarding sleep and insomnia must be explored and attempts be made to change them eventually. Cognitive therapy aims at the identification of dysfunctional beliefs and attitudes related to sleep and their replacement with more adaptive substitutes. Cognitive therapies also address catastrophizing the consequences of poor sleep to help patients reconceptualize the realities of their beliefs, thereby reducing the upcoming distress and arousal that impedes sleeping ( Perlis et al., 2006 ). Through cognitive-behavioral therapy (CBT) a combination of cognitive reconstruction and behavioral techniques are delivered to encourage patients to develop more adaptive coping skills and stop self-criticizing ( Perlis et al., 2006 ). The European guideline for diagnosis and treatment of insomnia ( Riemann et al., 2017 ) recommends CBT for insomnia (CBT-I) as the first-line of treatment for chronic insomnia.
Furthermore, several systematic reviews and meta-analyses ( Taylor and Pruiksma, 2014 ; Mitchell et al., 2019 ) have reported strong empirical support for CBT-I on different subjective and objective sleep parameters. CBT-I’s common approaches for non-comorbid insomnia were cognitive therapy, stimulus control, sleep restriction, sleep hygiene, and relaxation. The results indicated that CBT-I improved sleep onset latency, wake after sleep onset, total sleep time, and sleep efficiency. The changes persisted over time alleviating the symptoms ( Wang et al., 2005 ; Trauer et al., 2015 ).
Mindfulness-based cognitive therapy (MBCT) for reducing the negative cognitive and emotional value related to insomnia
Mindfulness-based cognitive therapy (MBCT), as an emotion-regulation based psychotherapy, is a purposeful and unbiased form of therapy directing attention to the present moment as a way of self-regulation that promotes mind-body relaxation ( Ludwig and Kabat-Zinn, 2008 ). The approach educates people toward changing their relationship with their thoughts and negative emotions. Patients must be aware of their thoughts and are inspired to take a non-judgmental perspective on them rather than a negative, self-referential assessment that intensifies both negative thoughts and emotions ( Ludwig and Kabat-Zinn, 2008 ). In concordance with suggestions put forth by the CCF, Shallcross and Visvanathan (2016) have explained that experiential awareness, attentional control, and acceptance techniques used in MBCT interventions improve rumination, arousal, selective attention, and the distorted perception involved in the development and maintenance of insomnia ( Shallcross and Visvanathan, 2016 ).
The MBCT protocol tailored for insomniacs showed significant pre–post improvements in self-reported total sleep time and various thought-control domains, along with reductions in sleep-related monitoring and worry ( Heidenreich et al., 2006 ). MBCT was also effective for individuals with a history of depression or anxiety accompanied by sleep difficulty or insomnia ( Ree and Craigie, 2007 ; Yook et al., 2008 ; Britton et al., 2010 ). Ree and Craigie (2007) reported decreased scores of insomnia severity symptoms lasting for about 3 months with the MBCT. Similarly, MBCT protocol in older adults showed a 14.5% improvement in self-reported sleep problems ( Foulk et al., 2014 ). Self-regulation of attention and orientation to experience to achieve better sleep are the proposed mechanisms of actions for MBCT ( Larouche et al., 2014 ). A recent comprehensive meta-analysis reported significantly improved insomnia symptoms as measured by the Pittsburgh Sleep Quality Index ( Wang et al., 2020 ).
ECL mechanism for modifications in negative emotional-value related to insomnia
Positive emotion-induction techniques can reduce the negative valence of insomnia when paired with positively valenced and high arousal pictures, films ( Uhrig et al., 2016 ), audio ( Bergman et al., 2016 ), music, and video clips ( Lazar and Pearlman-Avnion, 2014 ; Siedlecka and Denson, 2019 ). Game-like design, app-based format, goggles of virtual reality, or a screen are different ways to present stimuli to provide cost-effective home-based individualized treatments.
Rectifying the Distorted Perception of the Quality of Sleep Deficit
Digital-technology approaches are believed to provide an online measurement of sleep duration and correct the distorted perception of sleep deficit. Since we have established that negative emotions might influence the perception of insomniacs about their sleep deficit, interventions aiming at emotion-regulation or modifications of dysfunctional beliefs may help prevent the formation of the distorted perception. Furthermore, Holter monitoring of rest/activity cycle of sleep, smartphone gadgets ( Izmailova et al., 2018 ), actigraphy, and sleep diary ( Tang and Harvey, 2004b ) might help insomniacs correct misperception. In contrast, parts of the literature studying the time-perception concept ( Thomas and Cantor, 1975 , 1976 ) have revealed that when more information is processed, time is perceived as longer. A high level of cognitive arousal and repetitive thought patterns distorts time perception for insomniacs, leading to an overestimation of sleep onset latency ( Tang and Harvey, 2004a ). Another implication is that during sleep onset, cognitive arousal maintains an enhanced sensory and memory processing level obscuring the distinction between sleep and wakefulness ( Perlis et al., 1997 ).
Future Trends
The clinical recommendations provided in this paper can be applied separately or in combination, to plan treatment for individuals with insomnia. The CCF builds upon a general assumption that patients should be consciously and actively involved in the rehabilitation process. Subsequently, new treatments can be developed aimed at encouraging patients to be consciously aware of their negative thoughts related to sleep-difficulty and contingencies for intervention. Moreover, the inclusion of surrogate measurements is recommended for guaranteeing the patient’s conscious attended-awareness. Collectively, the CCF can provide a decision-support platform for clinicians to deliver more targeted interventions, and eventually, the methodologies suggested can provide a reliable platform to build a CCF for other cognitive disorders and support the causal clinical data models. This novel approach can improve our knowledge of psychological disturbances and complex comorbidities toward the design of rehabilitation interventions and suggestions in line with the “preventive medicine” policies.
The CCF of insomnia, its predictions, and the corresponding suggested interventions do not include patients with organic sleep disorders, general cognitive distortion, and psychotic problems. MSQ was obtained from patients with complaints of tinnitus at the clinic during the day, and not before sleep. Despite their importance, daytime cognitive processes were not taken into account in the presented framework. Lastly, to achieve clinical endpoints, repeated measures and longitudinal studies are required to improve predictabilities.
Data Availability Statement
The datasets used and analyzed during this study are available from the corresponding author on reasonable request and filling out NEL-Consent redirecting to “Zenodo” with the doi: http://doi.org/10.5281/zenodo.4145224 .
Ethics Statement
The studies involving human participants were reviewed and approved by Ethics Committee for Analysis of Research Projects, Specialized Center of Otorhinolaryngology and Speech Therapy, Hospital das Clínicas de Ribeirão Preto, University of São Paulo, Brazil (HCRP no. 55716616.1.1001.5440, and HCRP no. 09813519.1.0000.5440; internationally registered with U1111-1236-5441). All subjects gave written informed consent prior to participation in the study.
Author Contributions
ZV: leading author responsible for manuscript development, concept and study design, conceptual modeling participation, and data acquisition in the clinic. MN: collaborating in manuscript development and concept. JL: supervising clinical data acquisition and conceptual development, and collaborating in manuscript development. AD: collaborating in manuscript development and supervising data mining and modeling. MH: monitoring clinical data acquisition, and collaborating in manuscript development. IG: manuscript development, concept and study design, analogy, numerical methodology design and implementation, and data acquisition, and data pre-processing. All authors read and approved the final manuscript.
This research is part of a Multidisciplinary Cognitive Rehabilitation (MCR) Platform and was supported (Grant number: 2013/07375-0) by Innovation and Diffusion of Mathematical Sciences Center Applied to Industry (CEPID-CeMEAI) of São Paulo Research Foundation (FAPESP). It also supported with Centro de Engenharia Aplicada a Saúde (CEAS) of the University of São Paulo, and the Coordenação de Aperfeiçoamento de Pessoal de Nível Superior (CAPES) for student’s scholarship.
Conflict of Interest
The authors declare that the research was conducted in the absence of any commercial or financial relationships that could be construed as a potential conflict of interest.
Acknowledgments
The authors would like to appreciate all efforts of employees and clinicians in CEOF who supported the current study voluntarily.
Abbreviations
ABM, attentional bias modification; BIISS-RESO, behaviorally induced insufficient sleep syndrome with restricted and extended sleep opportunity; CAAP, conscious attended awareness perception; CBT, cognitive-behavioral therapy; CBT-I, cognitive-behavioral therapy for insomnia; CCF, conceptual cognitive framework; CEOF, Centro Especializado de Otorrinolaringologia e Fonoaudiologia; CS, conditioned stimulus; CVMIMD, cognitive vulnerability model for insomnia induced mood disturbances; DBAS, dysfunctional beliefs and attitudes about sleep; ECL, evaluative conditional learning; EDS, excessive daytime somnolence; GCVI, global cognitive vulnerability to insomnia; Ind, Indirect; MBCT, mindfulness-based cognitive therapy; MSQ, mini-sleep questionnaire; MSQ-R, mini-sleep questionnaire result; PFC, prefrontal cortex; SSCV, sleep-specific cognitive vulnerability; STAI, state-trait anxiety inventory; US, unconditioned Stimulus; WASO, wakes after sleep onset.
Alfano, C. A., Zakem, A. H., Costa, N. M., Taylor, L. K., and Weems, C. F. (2009). Sleep problems and their relation to cognitive factors, anxiety, and depressive symptoms in children and adolescents. Depression Anxiety 26, 503–512. doi: 10.1002/da.20443
PubMed Abstract | CrossRef Full Text | Google Scholar
Baglioni, C., Lombardo, C., Bux, E., Hansen, S., Salveta, C., Biello, S., et al. (2010). Psychophysiological reactivity to sleep-related emotional stimuli in primary insomnia. Behav. Res. Ther. 48, 467–475. doi: 10.1016/j.brat.2010.01.008
Barclay, N. L., and Ellis, J. G. (2013). Sleep-related attentional bias in poor versus good sleepers is independent of affective valence. J. Sleep Res. 22, 414–421. doi: 10.1111/jsr.12035
Barclay, N. L., and Gregory, A. M. (2010). The presence of a perseverative iterative style in poor vs. good sleepers. J. Behav. Ther. Exp. Psychiatry 41, 18–23. doi: 10.1016/j.jbtep.2009.08.003
Bastien, C. H., St-Jean, G., Morin, C. M., Turcotte, I., and Carrier, J. (2008). Chronic psychophysiological insomnia: hyperarousal and/or inhibition deficits? An ERPs investigation. Sleep 31, 887–898.
Google Scholar
Bavafa, A., Akbar Foroughi, A., Khaledi-Paveh, B., Abbas Taheri, A., Fehrest, F., and Amiri, S. (2018). The comparison of effects of state and trait anxiety on the components of sleep quality. J. Sleep Sci. 3, 95–101.
Beck, A. T. (2008). The evolution of the cognitive model of depression and its neurobiological correlates. Am. J. Psychiatry 165, 969–977. doi: 10.1176/appi.ajp.2008.08050721
Beck, A. T., and Clark, D. A. (1997). An information processing model of anxiety: automatic and strategic processes. Behav. Res. Ther. 35, 49–58. doi: 10.1016/s0005-7967(96)00069-1
CrossRef Full Text | Google Scholar
Bei, B., Wiley, J. F., Allen, N. B., and Trinder, J. A. (2015). Cognitive vulnerability model of sleep and mood in adolescents under naturalistically restricted and extended sleep opportunities. Sleep 38, 453–461. doi: 10.5665/sleep.4508
Bergman, P., Vastfjall, D., Tajadura-Jimenez, A., and Asutay, E. (2016). Auditory-induced emotion mediates perceptual categorization of everyday sounds. Front. Psychol. 7:1565. doi: 10.3389/fpsyg.2016.01565
Bootzin, R. R., and Rider, S. P. (1997). “Behavioral techniques and biofeedback for insomnia,” in Understanding Sleep: The Evaluation and Treatment of Sleep Disorders , eds M. R. Pressman and C. William (Washington, DC: American Psychological Association), 315–338.
Bradley, M. M., and Lang, P. J. (1994). Measuring emotion: the self-assessment manikin and the semantic differential. J. Behav. Ther. Exp. Psychiatry 25, 49–59. doi: 10.1016/0005-7916(94)90063-9
Britton, W. B., Haynes, P. L., Fridel, K. W., and Bootzin, R. R. (2010). Polysomnographic and subjective profiles of sleep continuity before and after mindfulness-based cognitive therapy in partially remitted depression. Psych. Med. 72, 539–548. doi: 10.1097/PSY.0b013e3181dc1bad
Clarke, P. J., Bedford, K., Notebaert, L., Bucks, R. S., Rudaizky, D., Milkins, B. C., et al. (2016). Assessing the therapeutic potential of targeted attentional bias modification for insomnia using smartphone delivery. Psychother. Psych. 85, 187–189. doi: 10.1159/000442025
Cormier, R. E. (1990). “Sleep disturbances,” in Clinical Methods: The History, Physical, and Laboratory Examinations , eds H. K. Walker, W. D. Hall, and J. W. Hurst (Boston: Butterworths).
Dalgleish, T., and Watts, F. N. (1990). Biases of attention and memory in disorders of anxiety and depression. Clin. Psychol. Rev. 10, 589–604. doi: 10.1016/0272-7358(90)90098-U
Davey, G. C. L., and Levy, S. (1998). Catastrophic worrying: personal inadequacy and a perseverative iterative style as features of the catastrophizing process. J. Abnormal Psychol. 107, 576–586. doi: 10.1037/0021-843x.107.4.576
De Houwer, J., Thomas, S., and Baeyens, F. (2001). Associative learning of likes and dislikes: a review of 25 years of research on human evaluative conditioning. Psychol. Bull. 127, 853–869. doi: 10.1037/0033-2909.127.6.853
Dzierzewski, J. M., Dautovich, N., and Ravyts, S. (2018). Sleep and COGNITION IN OLDER ADULTS. Sleep Med. Clin. 13, 93–106. doi: 10.1016/j.jsmc.2017.09.009
Espie, C. A., Brooks, D. N., and Lindsay, W. R. (1989). An evaluation of tailored psychological treatment of insomnia. J. Behav. Ther. Exp. Psychiatry 20, 143–153. doi: 10.1016/0005-7916(89)90047-5
Falavigna, A., de Souza Bezerra, M. L., Teles, A. R., Kleber, F. D., Velho, M. C., da Silva, R. C., et al. (2011). Consistency and reliability of the Brazilian Portuguese version of the Mini-Sleep Questionnaire in undergraduate students. Sleep Breath 15, 351–355. doi: 10.1007/s11325-010-0392-x
Foulk, M. A., Ingersoll-Dayton, B., Kavanagh, J., Robinson, E., and Kales, H. C. (2014). Mindfulness-based cognitive therapy with older adults: an exploratory study. J. Gerontol. Soc. Work. 57, 498–520. doi: 10.1080/01634372.2013.869787
Freeman, A., Felgoise, S., Nezu, A., Nezu, C., and Reinecke, M. (2005). Encyclopedia of Cognitive Behavior Therapy. New York, NY: Springer Science+ Business Media.
Frijda, N. H. (1987). Comment on Oatley and Johnson-Laird’s “Towards a cognitive theory of emotions”. Cogn. Emotion 1, 51–58.
Ghodratitoostani, I., Delbem, A. C. B., Torabi-Nami, M., Makkiabadi, B., Jalilvand, H., and Sanchez, T. G. (2016a). Theoretical tinnitus multimodality framework: a neurofunctional model. J. Adv. Med. Sci. Appl. Technol. 2, 181–189.
Ghodratitoostani, I., Zana, Y., Delbem, A. C., Sani, S. S., Ekhtiari, H., and Sanchez, T. G. (2016b). Theoretical tinnitus framework: a neurofunctional model. Front. Neurosci. 10:370. doi: 10.3389/fnins.2016.00370
Gorenstein, C., and Andrade, L. (1996). Validation of a portuguese version of the beck depression inventory and the state-trait anxiety inventory in Brazilian subjects. Braz. J. Med. Biol. 29, 453–457.
Gross, J. J. (1998). The emerging field of emotion regulation: an integrative review. Rev. Gen. Psychol. 2:271.
Gross, J. J. (2013). Handbook of Emotion Regulation , Second Edn. New York, NY: Guilford Publications.
Harvey, A. G. (2000). Pre-sleep cognitive activity: a comparison of sleep-onset insomniacs and good sleepers. Br. J. Clin. Psychol. Br. Psychol. Soc. 39, 275–286. doi: 10.1348/014466500163284
Harvey, A. G. (2002). A cognitive model of insomnia. Behav. Res. Ther. 40, 869–893. doi: 10.1016/s0005-7967(01)00061-4
Hayes, A. F. (2017). Introduction to Mediation, Moderation, and Conditional Process Analysis: A Regression-Based Approach. New York, NY: Guilford publications.
Haynes, S. N., Adams, A., and Franzen, M. (1981). The effects of presleep stress on sleep-onset insomnia. J. Abnormal Psychol. 90, 601–606. doi: 10.1037//0021-843x.90.6.601
Heidenreich, T., Tuin, I., Pflug, B., Michal, M., and Michalak, J. (2006). Mindfulness-based cognitive therapy for persistent insomnia: a pilot study. Psychother. Psych. 75, 188–189. doi: 10.1159/000091778
Herbert, V., Pratt, D., Emsley, R., and Kyle, S. D. (2017). Predictors of nightly subjective-objective sleep discrepancy in poor sleepers over a seven-day period. Brain Sci. 7:29. doi: 10.3390/brainsci7030029
Horstmann, G., and Herwig, A. (2016). Novelty biases attention and gaze in a surprise trial. Atten. Percep. Psychophys. 78, 69–77. doi: 10.3758/s13414-015-0995-1
Izmailova, E. S., Wagner, J. A., and Perakslis, E. D. (2018). Wearable devices in clinical trials: hype and hypothesis. Clin. Pharmacol. Ther. 104, 42–52. doi: 10.1002/cpt.966
Jansson-Fröjmark, M., Bermås, M., and Kjellén, A. (2012). Attentional bias in insomnia: the dot-probe task with pictorial stimuli depicting daytime fatigue/malaise. Cogn. Ther. Res. 37, 534–546. doi: 10.1007/s10608-012-9486-z
Jones, B. T., Macphee, L. M., Broomfield, N. M., Jones, B. C., and Espie, C. A. (2005). Sleep-related attentional bias in good, moderate, and poor (primary insomnia) sleepers. J. Abnormal Psychol. 114, 249–258. doi: 10.1037/0021-843X.114.2.249
Koster, E. H., Crombez, G., Verschuere, B., and De Houwer, J. (2004). Selective attention to threat in the dot probe paradigm: differentiating vigilance and difficulty to disengage. Behav. Res. Ther. 42, 1183–1192. doi: 10.1016/j.brat.2003.08.001
Kuisk, L. A., Bertelson, A. D., and Walsh, J. K. (1989). Presleep cognitive hyperarousal and affect as factors in objective and subjective insomnia. Perceptual Motor Skills 69, 1219–1225. doi: 10.2466/pms.1989.69.3f.1219
Lancee, J., Eisma, M. C., van Zanten, K. B., and Topper, M. (2017a). When thinking impairs sleep: trait, daytime and nighttime repetitive thinking in insomnia. Behav. Sleep Med. 15, 53–69. doi: 10.1080/15402002.2015.1083022
Lancee, J., Yasiney, S. L., Brendel, R. S., Boffo, M., Clarke, P. J. F., and Salemink, E. (2017b). Attentional bias modification training for insomnia: a double-blind placebo controlled randomized trial. PLoS One 12:e0174531. doi: 10.1371/journal.pone.0174531
Larouche, M., Cote, G., Belisle, D., and Lorrain, D. (2014). Kind attention and non-judgment in mindfulness-based cognitive therapy applied to the treatment of insomnia: state of knowledge. Pathol. Biol. (Paris) 62, 284–291. doi: 10.1016/j.patbio.2014.07.002
Lauriola, M., Carleton, R. N., Tempesta, D., Calanna, P., Socci, V., Mosca, O., et al. (2019). A correlational analysis of the relationships among intolerance of uncertainty, anxiety sensitivity, subjective sleep quality, and insomnia symptoms. Int. J. Environ. Res. Public Health 16:3253. doi: 10.3390/ijerph16183253
Lazar, J. N., and Pearlman-Avnion, S. (2014). Effect of affect induction method on emotional valence and arousal. Psychology 5:595.
Lichstein, K. L., and Rosenthal, T. L. (1980). Insomniacs’ perceptions of cognitive versus somatic determinants of sleep disturbance. J. Abnormal Psychol. 89, 105–107. doi: 10.1037//0021-843x.89.1.105
Ludwig, D. S., and Kabat-Zinn, J. (2008). Mindfulness in medicine. Jama J. Am. Med. Assoc. 300, 1350–1352. doi: 10.1001/jama.300.11.1350
MacMahon, K. M., Broomfield, N. M., and Espie, C. A. (2006). Attention bias for sleep-related stimuli in primary insomnia and delayed sleep phase syndrome using the dot-probe task. Sleep 29, 1420–1427.
Mai, E., and Buysse, D. J. (2008). Insomnia: prevalence, impact, pathogenesis, differential diagnosis, and evaluation. Sleep Med. Clin. 3, 167–174. doi: 10.1016/j.jsmc.2008.02.001
Manconi, M., Ferri, R., Sagrada, C., Punjabi, N. M., Tettamanzi, E., Zucconi, M., et al. (2010). Measuring the error in sleep estimation in normal subjects and in patients with insomnia. J. Sleep Res. 19, 478–486. doi: 10.1111/j.1365-2869.2009.00801.x
Milkins, B., Notebaert, L., MacLeod, C., and Clarke, P. J. F. (2016). The potential benefits of targeted attentional bias modification on cognitive arousal and sleep quality in worry-related sleep disturbance. Clin. Psychol. Sci. 4, 1015–1027. doi: 10.1177/2167702615626898
Mitchell, L. J., Bisdounis, L., Ballesio, A., Omlin, X., and Kyle, S. D. (2019). The impact of cognitive behavioural therapy for insomnia on objective sleep parameters: a meta-analysis and systematic review. Sleep Med. Rev. 47, 90–102. doi: 10.1016/j.smrv.2019.06.002
Morin, C. M. (1993). Insomnia: Psychological Assessment and Management. New York, NY: Guilford press.
Morin, C. M., Stone, J., Trinkle, D., Mercer, J., and Remsberg, S. (1993). Dysfunctional beliefs and attitudes about sleep among older adults with and without insomnia complaints. Psychol. Aging 8, 463–467. doi: 10.1037/0882-7974.8.3.463
Nami, M. (2014). Chronic insomnia, pharmacotherapy and the cognitive behavioural approaches. J. Sleep Disord. Ther. 3:1000151.
Natale, V., Fabbri, M., Tonetti, L., and Martoni, M. (2014). Psychometric goodness of the Mini Sleep Questionnaire. Psychiatry Clin. Neurosci. 68, 568–573. doi: 10.1111/pcn.12161
Nicassio, P. M., Mendlowitz, D. R., Fussell, J. J., and Petras, L. (1985). The phenomenology of the pre-sleep state: the development of the pre-sleep arousal scale. Behav. Res. Ther. 23, 263–271. doi: 10.1016/0005-7967(85)90004-x
Perlis, M. L., Giles, D. E., Mendelson, W. B., Bootzin, R. R., and Wyatt, J. K. (1997). Psychophysiological insomnia: the behavioural model and a neurocognitive perspective. J. Sleep Res. 6, 179–188. doi: 10.1046/j.1365-2869.1997.00045.x
Perlis, M. L., Jungquist, C., Smith, M. T., and Posner, D. (2006). Cognitive Behavioral Treatment of Insomnia: A Session-By-Session Guide. Berlin: Springer Science & Business Media.
Ree, M. J., and Craigie, M. A. (2007). Outcomes following mindfulness-based cognitive therapy in a heterogeneous sample of adult outpatients. Behav. Change 24, 70–86. doi: 10.1375/bech.24.2.70
Riemann, D., Baglioni, C., Bassetti, C., Bjorvatn, B., Dolenc Groselj, L., Ellis, J. G., et al. (2017). European guideline for the diagnosis and treatment of insomnia. J. Sleep Res. 26, 675–700. doi: 10.1111/jsr.12594
Roberts, L. E., Husain, F. T., and Eggermont, J. J. (2013). Role of attention in the generation and modulation of tinnitus. Neurosci. Biobehav. Rev. 37, 1754–1773. doi: 10.1016/j.neubiorev.2013.07.007
Sadigh, M. R., Himmanen, S. A., and Scepansky, J. A. (2014). An investigation of the prevalence of insomnia in college students and its relationship to trait anxiety. College Student J. 48, 397–406.
Sadler, P., McLaren, S., and Jenkins, M. (2013). A psychological pathway from insomnia to depression among older adults. Int. Psychogeriatr. 25, 1375–1383. doi: 10.1017/S1041610213000616
Sateia, M. J. (2014). International classification of sleep disorders-third edition: highlights and modifications. Chest 146, 1387–1394. doi: 10.1378/chest.14-0970
Scherer, K. R., Schorr, A., and Johnstone, T. (2001). Appraisal Processes in Emotion: Theory, Methods, Research. Oxford: Oxford University Press.
Schutte-Rodin, S., Broch, L., Buysse, D., Dorsey, C., and Sateia, M. (2008). Clinical guideline for the evaluation and management of chronic insomnia in adults. J. Clin. Sleep Med. 4, 487–504.
Shallcross, A. J., and Visvanathan, P. D. (2016). “Mindfulness-based cognitive therapy for insomnia,” in Mindfulness-Based Cognitive Therapy , ed. S. J. Eisendrath (Berlin: Springer), 19–29.
Siedlecka, E., and Denson, T. F. (2019). Experimental methods for inducing basic emotions: a qualitative review. Emotion Rev. 11, 87–97. doi: 10.1177/1754073917749016
Spiegelhalder, K., Kyle, S. D., Feige, B., Prem, M., Nissen, C., Espie, C. A., et al. (2010). The impact of sleep-related attentional bias on polysomnographically measured sleep in primary insomnia. Sleep 33, 107–112. doi: 10.1093/sleep/33.1.107
Stahl, C., and Unkelbach, C. (2009). Evaluative learning with single versus multiple unconditioned stimuli: the role of contingency awareness. J. Exp. Psychol. Animal Behav. Proc. 35, 286–291. doi: 10.1037/a0013255
Tang, N. K., and Harvey, A. G. (2004b). Correcting distorted perception of sleep in insomnia: a novel behavioural experiment? Behav. Res. Ther. 42, 27–39. doi: 10.1016/s0005-7967(03)00068-8
Tang, N. K., and Harvey, A. G. (2004a). Effects of cognitive arousal and physiological arousal on sleep perception. Sleep 27, 69–78. doi: 10.1093/sleep/27.1.69
Taylor, D. J., and Pruiksma, K. E. (2014). Cognitive and behavioural therapy for insomnia (CBT-I) in psychiatric populations: a systematic review. Int. Rev. Psychiatry 26, 205–213. doi: 10.3109/09540261.2014.902808
Thomas, E. A., and Cantor, N. E. (1975). On the duality of simultaneous time and size perception. Percept. Psychophys. 18, 44–48.
Thomas, E. A. C., and Cantor, N. E. (1976). Simultaneous time and size perception. Percept. Psychophys. 19, 353–360. doi: 10.3758/Bf03204243
Trauer, J. M., Qian, M. Y., Doyle, J. S., Rajaratnam, S. M., and Cunnington, D. (2015). Cognitive behavioral therapy for chronic insomnia: a systematic review and meta-analysis. Ann. Intern. Med. 163, 191–204. doi: 10.7326/M14-2841
Troxel, W. M., Germain, A., and Buysse, D. J. (2012). Clinical management of insomnia with brief behavioral treatment (BBTI). Behav. Sleep Med. 10, 266–279. doi: 10.1080/15402002.2011.607200
Uhrig, M. K., Trautmann, N., Baumgartner, U., Treede, R. D., Henrich, F., Hiller, W., et al. (2016). Emotion elicitation: a comparison of pictures and films. Front. Psychol. 7:180. doi: 10.3389/fpsyg.2016.00180
Wang, M. Y., Wang, S. Y., and Tsai, P. S. (2005). Cognitive behavioural therapy for primary insomnia: a systematic review. J. Adv. Nursing 50, 553–564. doi: 10.1111/j.1365-2648.2005.03433.x
Wang, Y. Y., Wang, F., Zheng, W., Zhang, L., Ng, C. H., Ungvari, G. S., et al. (2020). Mindfulness-based interventions for insomnia: a meta-analysis of randomized controlled trials. Behav. Sleep Med. 18, 1–9. doi: 10.1080/15402002.2018.1518228
Wicklow, A., and Espie, C. A. (2000). Intrusive thoughts and their relationship to actigraphic measurement of sleep: towards a cognitive model of insomnia. Behav. Res. Ther. 38, 679–693. doi: 10.1016/S0005-7967(99)00136-9
Woods, H., Marchetti, L. M., Biello, S. M., and Espie, C. A. (2009). The clock as a focus of selective attention in those with primary insomnia: an experimental study using a modified Posner paradigm. Behav. Res. Ther. 47, 231–236. doi: 10.1016/j.brat.2008.12.009
Woods, H. C., Scheepers, C., Ross, K. A., Espie, C. A., and Biello, S. M. (2013). What are you looking at? moving toward an attentional timeline in insomnia: a novel semantic eye tracking study. Sleep 36, 1491–1499. doi: 10.5665/sleep.3042
Yoo, J. Y., and Lee, J. H. (2015). The effects of valence and arousal on time perception in individuals with social anxiety. Front. Psychol. 6:1208. doi: 10.3389/fpsyg.2015.01208
Yook, K., Lee, S. H., Ryu, M., Kim, K. H., Choi, T. K., Suh, S. Y., et al. (2008). Usefulness of mindfulness-based cognitive therapy for treating insomnia in patients with anxiety disorders: a pilot study. J. Nervous Mental Dis. 196, 501–503. doi: 10.1097/NMD.0b013e31817762ac
Keywords : cognitive model, insomnia, evaluative conditional learning, mediator model, distorted perception, appraisal, valence, conceptual cognitive framework
Citation: Vaziri Z, Nami M, Leite JP, Delbem ACB, Hyppolito MA and Ghodratitoostani I (2021) Conceptual Framework for Insomnia: A Cognitive Model in Practice. Front. Neurosci. 15:628836. doi: 10.3389/fnins.2021.628836
Received: 13 November 2020; Accepted: 03 June 2021; Published: 22 July 2021.
Reviewed by:
Copyright © 2021 Vaziri, Nami, Leite, Delbem, Hyppolito and Ghodratitoostani. This is an open-access article distributed under the terms of the Creative Commons Attribution License (CC BY) . The use, distribution or reproduction in other forums is permitted, provided the original author(s) and the copyright owner(s) are credited and that the original publication in this journal is cited, in accordance with accepted academic practice. No use, distribution or reproduction is permitted which does not comply with these terms.
*Correspondence: Iman Ghodratitoostani, [email protected]
Disclaimer: All claims expressed in this article are solely those of the authors and do not necessarily represent those of their affiliated organizations, or those of the publisher, the editors and the reviewers. Any product that may be evaluated in this article or claim that may be made by its manufacturer is not guaranteed or endorsed by the publisher.
- Type 2 Diabetes
- Heart Disease
- Digestive Health
- Multiple Sclerosis
- COVID-19 Vaccines
- Occupational Therapy
- Healthy Aging
- Health Insurance
- Public Health
- Patient Rights
- Caregivers & Loved Ones
- End of Life Concerns
- Health News
- Thyroid Test Analyzer
- Doctor Discussion Guides
- Hemoglobin A1c Test Analyzer
- Lipid Test Analyzer
- Complete Blood Count (CBC) Analyzer
- What to Buy
- Editorial Process
- Meet Our Medical Expert Board
Restorative Theory and More Ideas About Why We Sleep
Verywell / Hetal Rathod
Restorative Theory
Adaptive theory.
- Energy Conservation
- Brain Plasticity
Frequently Asked Questions
Even though it's something you have to do every day, why we sleep remains a mystery. Many sleep theories abound, yet scientists are far from universal agreement about how to answer the question, "Why do we sleep at night?" Only in the last few decades have they even begun to unravel sleep's true secrets. At least four common theories are in play, but it remains to be seen which—if any—are correct.
You may look at these theories and the scientific evidence that supports them and wonder why they all can't, at least in part, be right. They could be, but researchers are continuing to search for "the" core reason for sleep, and many subscribe to a belief expressed in a 1998 paper:
Allen Rechtschaffen
Sleep can be understood as fulfilling many different functions but intuition suggests there is one essential function. The discovery of this function will open an important door to the understanding of biological processes.
The sleep cycle involves several stages, but these theories generally focus on rapid-eye movement (REM) sleep —which is when you dream —and the other stages lumped together as non-REM sleep.
The restorative theory of sleep, first proposed in 2006, is among the more accepted explanations for why people need sleep. It suggests that the purpose of sleep is to store memory and restore our brains and bodies for the next day.
- Organizing and storing memories : Memories are believed to be converted from short-term to long-term storage, while information deemed unimportant is removed. This primarily occurs during REM sleep, which is when the brain cells most involved in memory, attention, and learning are least active.
- Clearing out and replenishing brain chemicals : Many brain chemicals build up while you're awake, including adenosine , which makes you sleepy when it accumulates. Meanwhile, during sleep, the brain "restocks" the chemicals it uses for sending signals and other purposes, so you have enough for the next day.
- Clearing waste toxins from the brain : Similar to brain chemicals, the waste products of energy metabolism build up during the day and are cleared out at night. (However, the primary evidence for this comes from studies of mice, not humans.)
- Repairing tissues throughout the body : During sleep, your body increases cellular division, growth hormone levels, and the production of proteins necessary for proper function. This is believed to be why strenuous physical activity during the day increases your time in REM sleep.
- Rest for the brain : The inactivity during non-REM sleep appears to provide a period of rest for the brain.
Some of the brain chemicals that accumulate during the day are associated with the plaques that characterize Alzheimer's disease , so it's theorized that the brain-clearing activities of sleep may protect you against Alzheimer's.
Also called the evolution theory or preservation theory, the original version of this early theory suggested that while humans evolved, hiding out overnight increased the ability to survive.
For our early ancestors, nighttime was dangerous—especially because the predators who hunt at night function better in the dark than humans do—so it made sense to seek a safe refuge. Also, because they couldn't be out finding food themselves, their bodies slowed down to conserve energy for when they could be active. People who avoided dangers in this way, the theory argued, lived longer and were more likely to reproduce.
Thus, sleep became an adaptive or evolutionary advantage and became part of the neurochemistry of the species. However, most experts reject this idea because sleeping leaves animals (including humans) vulnerable and defenseless, which doesn't support the idea that sleeping made our ancestors safer.
A flaw in this theory, some experts say, is that sleeping limits productivity, such as finding food and reproducing, so staying awake longer would be an evolutionary benefit. However, no species evolved without a need for sleep, which calls the adaptive benefit into question.
According to some sleep researchers, the daily need for sleep combined with the incentive not to be out in the dark caused us to adapt to function best during daylight, which prevented us from adapting to the dark.
Energy Conservation Theory
In a similar vein to aspects of other theories, some experts theorize the primary purpose of sleep is conserving energy. By sleeping, they say, you're able to spend part of your time functioning at a lower metabolism.
That lowers the number of calories you need to eat. For early humans, that extra food requirement could have been the difference between life and death, or survival of the species versus extinction. It was harder to gather food at night, so it made sense to stay hidden then. They also point to the brain's need to replenish its reserve of glycogen, which is an important fuel.
However, while it's true that metabolism slows during non-REM, the brain is extremely active during REM sleep, which some say is a strike against the energy conservation theory.
Brain Plasticity Theory
Among the more recent theories deals with brain plasticity (also called neuroplasticity), which is the brain's ability to change and adapt in response to experience. It can change both functional aspects (such as re-learning skills in a new area after damage) and structural aspects (such as forming new pathways due to learning).
The brain plasticity theory says that sleep is necessary for the brain to make structural changes. Support for this theory comes from many places.
As in restorative theory, this concept deals with information processing and memory formation. Research suggests that sleep loss leads to less structural plasticity, which can have a negative effect on alertness, cognition, and mood. Sleep deprivation also compromises memory formation, which is related to learning and plasticity.
It's believed that the plasticity theory explains why babies and young children require a lot of sleep—they're learning so much about the world that their brains need more time to process it. Researchers are even trying to promote less sleep interruption for preterm babies in neonatal intensive care, citing studies about sleep's long-term impact on brain development and plasticity.
Some researchers have even theorized that sleep is the price we pay for brain plasticity. That concept is based on the importance of the processes that occur during sleep to the brain's ability to adapt and change.
Declining Sleep Needs
Newborns need between 14 and 17 hours of sleep per day. The recommended amount of sleep declines throughout childhood, with teenagers needing between 8 and 10 hours a day.
Why do we close our eyes when we sleep?
There are several reasons why most people sleep with eyes closed, though some people do actually sleep with their eyes open. Closed eyes stay moist and are protected by the eyelids during sleep. Eyelids also block out light during sleep, and light signals to the brain that it is time to wake up.
At what stage of sleep can you experience sleep paralysis?
Sleep paralysis , or the feeling that you are conscious and yet unable to control your body, is very common and typically occurs during the transition from REM to being awake.
Why do we dream?
There are a lot of theories explaining why we dream, such as that dreaming aids in memory processing and that it allows us to process emotions. Sigmund Freud believed that dreams express our unconscious desires and deepest wishes.
A Word From Verywell
While it's a phenomenon we don't fully understand, sleep is critical to our daily health. Not only is it necessary for restoration and repair, learning and memory, growth and development, and brain plasticity, sleep also helps with problem-solving, a healthy metabolism, blood-sugar and hormone regulation, heart health, and strengthening immunity. With how crucial it is to our survival, it's no wonder many of us long for more of it.
Freiberg AS. Why we sleep: A hypothesis for an ultimate or evolutionary origin for sleep and other physiological rhythms . J Circadian Rhythms . 2020;18:2. Published 2020 Mar 30. doi:10.5334/jcr.189
Ezenwanne E. Current concepts in the neurophysiologic basis of sleep; a review . Ann Med Health Sci Res ; 1(2):173-179.
Xie L, Kang H, Xu Q, et al. Sleep drives metabolite clearance from the adult brain . Science . 2013;342(6156):373–377. doi:10.1126/science.1241224
Raven F, Van der Zee EA, Meerlo P, Havekes R. The role of sleep in regulating structural plasticity and synaptic strength: Implications for memory and cognitive function . Sleep Med Rev . 2018;39:3-11. doi:10.1016/j.smrv.2017.05.002
Park J. Sleep promotion for preterm infants in the NICU . Nurs Womens Health . 2020;24(1):24-35. doi:10.1016/j.nwh.2019.11.004
Tononi G, Cirelli C. Sleep and the price of plasticity: From synaptic and cellular homeostasis to memory consolidation and integration . Neuron . 2014;81(1):12-34. doi:10.1016/j.neuron.2013.12.025
U.S. Centers for Disease Control and Prevention. Sleep and sleep disorders: How much sleep do I need?
National Sleep Foundation. Can you really sleep with your eyes open ?
Rasch B, Born J. About sleep's role in memory . Physiol Rev . 2013;93(2):681-766. doi:10.1152/physrev.00032.2012
Zhang W, Guo B. Freud's dream interpretation: A different perspective based on the self-organization theory of dreaming . Front Psychol . 2018;9:1553. doi:10.3389/fpsyg.2018.01553
National Institutes of Health, National Heart, Lung, and Blood Institute. Sleep deprivation and deficiency .
By Brandon Peters, MD Dr. Peters is a board-certified neurologist and sleep medicine specialist and is a fellow of the American Academy of Sleep Medicine.
- Bipolar Disorder
- Therapy Center
- When To See a Therapist
- Types of Therapy
- Best Online Therapy
- Best Couples Therapy
- Best Family Therapy
- Managing Stress
- Sleep and Dreaming
- Understanding Emotions
- Self-Improvement
- Healthy Relationships
- Student Resources
- Personality Types
- Guided Meditations
- Verywell Mind Insights
- 2023 Verywell Mind 25
- Mental Health in the Classroom
- Editorial Process
- Meet Our Review Board
- Crisis Support
How to Write a Great Hypothesis
Hypothesis Definition, Format, Examples, and Tips
Kendra Cherry, MS, is a psychosocial rehabilitation specialist, psychology educator, and author of the "Everything Psychology Book."
:max_bytes(150000):strip_icc():format(webp)/IMG_9791-89504ab694d54b66bbd72cb84ffb860e.jpg)
Amy Morin, LCSW, is a psychotherapist and international bestselling author. Her books, including "13 Things Mentally Strong People Don't Do," have been translated into more than 40 languages. Her TEDx talk, "The Secret of Becoming Mentally Strong," is one of the most viewed talks of all time.
:max_bytes(150000):strip_icc():format(webp)/VW-MIND-Amy-2b338105f1ee493f94d7e333e410fa76.jpg)
Verywell / Alex Dos Diaz
- The Scientific Method
Hypothesis Format
Falsifiability of a hypothesis.
- Operationalization
Hypothesis Types
Hypotheses examples.
- Collecting Data
A hypothesis is a tentative statement about the relationship between two or more variables. It is a specific, testable prediction about what you expect to happen in a study. It is a preliminary answer to your question that helps guide the research process.
Consider a study designed to examine the relationship between sleep deprivation and test performance. The hypothesis might be: "This study is designed to assess the hypothesis that sleep-deprived people will perform worse on a test than individuals who are not sleep-deprived."
At a Glance
A hypothesis is crucial to scientific research because it offers a clear direction for what the researchers are looking to find. This allows them to design experiments to test their predictions and add to our scientific knowledge about the world. This article explores how a hypothesis is used in psychology research, how to write a good hypothesis, and the different types of hypotheses you might use.
The Hypothesis in the Scientific Method
In the scientific method , whether it involves research in psychology, biology, or some other area, a hypothesis represents what the researchers think will happen in an experiment. The scientific method involves the following steps:
- Forming a question
- Performing background research
- Creating a hypothesis
- Designing an experiment
- Collecting data
- Analyzing the results
- Drawing conclusions
- Communicating the results
The hypothesis is a prediction, but it involves more than a guess. Most of the time, the hypothesis begins with a question which is then explored through background research. At this point, researchers then begin to develop a testable hypothesis.
Unless you are creating an exploratory study, your hypothesis should always explain what you expect to happen.
In a study exploring the effects of a particular drug, the hypothesis might be that researchers expect the drug to have some type of effect on the symptoms of a specific illness. In psychology, the hypothesis might focus on how a certain aspect of the environment might influence a particular behavior.
Remember, a hypothesis does not have to be correct. While the hypothesis predicts what the researchers expect to see, the goal of the research is to determine whether this guess is right or wrong. When conducting an experiment, researchers might explore numerous factors to determine which ones might contribute to the ultimate outcome.
In many cases, researchers may find that the results of an experiment do not support the original hypothesis. When writing up these results, the researchers might suggest other options that should be explored in future studies.
In many cases, researchers might draw a hypothesis from a specific theory or build on previous research. For example, prior research has shown that stress can impact the immune system. So a researcher might hypothesize: "People with high-stress levels will be more likely to contract a common cold after being exposed to the virus than people who have low-stress levels."
In other instances, researchers might look at commonly held beliefs or folk wisdom. "Birds of a feather flock together" is one example of folk adage that a psychologist might try to investigate. The researcher might pose a specific hypothesis that "People tend to select romantic partners who are similar to them in interests and educational level."
Elements of a Good Hypothesis
So how do you write a good hypothesis? When trying to come up with a hypothesis for your research or experiments, ask yourself the following questions:
- Is your hypothesis based on your research on a topic?
- Can your hypothesis be tested?
- Does your hypothesis include independent and dependent variables?
Before you come up with a specific hypothesis, spend some time doing background research. Once you have completed a literature review, start thinking about potential questions you still have. Pay attention to the discussion section in the journal articles you read . Many authors will suggest questions that still need to be explored.
How to Formulate a Good Hypothesis
To form a hypothesis, you should take these steps:
- Collect as many observations about a topic or problem as you can.
- Evaluate these observations and look for possible causes of the problem.
- Create a list of possible explanations that you might want to explore.
- After you have developed some possible hypotheses, think of ways that you could confirm or disprove each hypothesis through experimentation. This is known as falsifiability.
In the scientific method , falsifiability is an important part of any valid hypothesis. In order to test a claim scientifically, it must be possible that the claim could be proven false.
Students sometimes confuse the idea of falsifiability with the idea that it means that something is false, which is not the case. What falsifiability means is that if something was false, then it is possible to demonstrate that it is false.
One of the hallmarks of pseudoscience is that it makes claims that cannot be refuted or proven false.
The Importance of Operational Definitions
A variable is a factor or element that can be changed and manipulated in ways that are observable and measurable. However, the researcher must also define how the variable will be manipulated and measured in the study.
Operational definitions are specific definitions for all relevant factors in a study. This process helps make vague or ambiguous concepts detailed and measurable.
For example, a researcher might operationally define the variable " test anxiety " as the results of a self-report measure of anxiety experienced during an exam. A "study habits" variable might be defined by the amount of studying that actually occurs as measured by time.
These precise descriptions are important because many things can be measured in various ways. Clearly defining these variables and how they are measured helps ensure that other researchers can replicate your results.
Replicability
One of the basic principles of any type of scientific research is that the results must be replicable.
Replication means repeating an experiment in the same way to produce the same results. By clearly detailing the specifics of how the variables were measured and manipulated, other researchers can better understand the results and repeat the study if needed.
Some variables are more difficult than others to define. For example, how would you operationally define a variable such as aggression ? For obvious ethical reasons, researchers cannot create a situation in which a person behaves aggressively toward others.
To measure this variable, the researcher must devise a measurement that assesses aggressive behavior without harming others. The researcher might utilize a simulated task to measure aggressiveness in this situation.
Hypothesis Checklist
- Does your hypothesis focus on something that you can actually test?
- Does your hypothesis include both an independent and dependent variable?
- Can you manipulate the variables?
- Can your hypothesis be tested without violating ethical standards?
The hypothesis you use will depend on what you are investigating and hoping to find. Some of the main types of hypotheses that you might use include:
- Simple hypothesis : This type of hypothesis suggests there is a relationship between one independent variable and one dependent variable.
- Complex hypothesis : This type suggests a relationship between three or more variables, such as two independent and dependent variables.
- Null hypothesis : This hypothesis suggests no relationship exists between two or more variables.
- Alternative hypothesis : This hypothesis states the opposite of the null hypothesis.
- Statistical hypothesis : This hypothesis uses statistical analysis to evaluate a representative population sample and then generalizes the findings to the larger group.
- Logical hypothesis : This hypothesis assumes a relationship between variables without collecting data or evidence.
A hypothesis often follows a basic format of "If {this happens} then {this will happen}." One way to structure your hypothesis is to describe what will happen to the dependent variable if you change the independent variable .
The basic format might be: "If {these changes are made to a certain independent variable}, then we will observe {a change in a specific dependent variable}."
A few examples of simple hypotheses:
- "Students who eat breakfast will perform better on a math exam than students who do not eat breakfast."
- "Students who experience test anxiety before an English exam will get lower scores than students who do not experience test anxiety."
- "Motorists who talk on the phone while driving will be more likely to make errors on a driving course than those who do not talk on the phone."
- "Children who receive a new reading intervention will have higher reading scores than students who do not receive the intervention."
Examples of a complex hypothesis include:
- "People with high-sugar diets and sedentary activity levels are more likely to develop depression."
- "Younger people who are regularly exposed to green, outdoor areas have better subjective well-being than older adults who have limited exposure to green spaces."
Examples of a null hypothesis include:
- "There is no difference in anxiety levels between people who take St. John's wort supplements and those who do not."
- "There is no difference in scores on a memory recall task between children and adults."
- "There is no difference in aggression levels between children who play first-person shooter games and those who do not."
Examples of an alternative hypothesis:
- "People who take St. John's wort supplements will have less anxiety than those who do not."
- "Adults will perform better on a memory task than children."
- "Children who play first-person shooter games will show higher levels of aggression than children who do not."
Collecting Data on Your Hypothesis
Once a researcher has formed a testable hypothesis, the next step is to select a research design and start collecting data. The research method depends largely on exactly what they are studying. There are two basic types of research methods: descriptive research and experimental research.
Descriptive Research Methods
Descriptive research such as case studies , naturalistic observations , and surveys are often used when conducting an experiment is difficult or impossible. These methods are best used to describe different aspects of a behavior or psychological phenomenon.
Once a researcher has collected data using descriptive methods, a correlational study can examine how the variables are related. This research method might be used to investigate a hypothesis that is difficult to test experimentally.
Experimental Research Methods
Experimental methods are used to demonstrate causal relationships between variables. In an experiment, the researcher systematically manipulates a variable of interest (known as the independent variable) and measures the effect on another variable (known as the dependent variable).
Unlike correlational studies, which can only be used to determine if there is a relationship between two variables, experimental methods can be used to determine the actual nature of the relationship—whether changes in one variable actually cause another to change.
The hypothesis is a critical part of any scientific exploration. It represents what researchers expect to find in a study or experiment. In situations where the hypothesis is unsupported by the research, the research still has value. Such research helps us better understand how different aspects of the natural world relate to one another. It also helps us develop new hypotheses that can then be tested in the future.
Thompson WH, Skau S. On the scope of scientific hypotheses . R Soc Open Sci . 2023;10(8):230607. doi:10.1098/rsos.230607
Taran S, Adhikari NKJ, Fan E. Falsifiability in medicine: what clinicians can learn from Karl Popper [published correction appears in Intensive Care Med. 2021 Jun 17;:]. Intensive Care Med . 2021;47(9):1054-1056. doi:10.1007/s00134-021-06432-z
Eyler AA. Research Methods for Public Health . 1st ed. Springer Publishing Company; 2020. doi:10.1891/9780826182067.0004
Nosek BA, Errington TM. What is replication ? PLoS Biol . 2020;18(3):e3000691. doi:10.1371/journal.pbio.3000691
Aggarwal R, Ranganathan P. Study designs: Part 2 - Descriptive studies . Perspect Clin Res . 2019;10(1):34-36. doi:10.4103/picr.PICR_154_18
Nevid J. Psychology: Concepts and Applications. Wadworth, 2013.
By Kendra Cherry, MSEd Kendra Cherry, MS, is a psychosocial rehabilitation specialist, psychology educator, and author of the "Everything Psychology Book."

An official website of the United States government
The .gov means it’s official. Federal government websites often end in .gov or .mil. Before sharing sensitive information, make sure you’re on a federal government site.
The site is secure. The https:// ensures that you are connecting to the official website and that any information you provide is encrypted and transmitted securely.
- Publications
- Account settings
Preview improvements coming to the PMC website in October 2024. Learn More or Try it out now .
- Advanced Search
- Journal List
- HHS Author Manuscripts

Theories, Models, and Frameworks Related to Sleep-Wake Disturbances in the Context of Cancer
The purpose of this article was to review theories, models, and frameworks of sleep disturbances referenced in the cancer literature. Sleep-wake disturbances in cancer are a significant problem that negatively affects quality of life. There is no previously published review of the theories, models, or frameworks used to study sleep-wake disturbances in the context of cancer. Describing existing theories or models and their application in cancer is important to advance knowledge in this area. Two theories and 9 models were identified for review. These have been used to further understand the problem of sleep-wake disturbances as a primary or secondary symptom within the cancer literature. Searches were conducted from January 1, 1970, to July 31, 2008, to find relevant articles using 4 electronic databases: MEDLINE, CINAHL, PubMed, and PsychINFO. On the basis of the search, 73 descriptive or intervention studies were identified and reviewed. Most research was atheoretical, with no identified theory, model, or framework. In studies that did use theory or models, few were applied in more than one study. Although several commonalities across models did emerge, a more comprehensive and widely used model could help guide nursing research to facilitate effective symptom management for this prominent problem in cancer.
Sleep-wake disturbances in the context of cancer are increasingly being recognized as a significant problem. 1 – 3 Sleep has been characterized as a psychobiological event that includes physiological, psychological, and behavioral mechanisms. 4 Sleep-wake disturbances have been defined as disruptions in nighttime sleep or wakefulness that can result in negative health outcomes. 4 In various American and Canadian samples, 18% to 90% of cancer patients and survivors reported subjective sleep-wake disturbances. 2 , 3 , 5 – 9 Subjectively reported sleep-wake disturbances can include a variety of diagnosable sleep-wake problems such as insomnia, restless leg syndrome, periodic limb movement disorder, obstructive sleep apnea, or daytime sleepiness. Of these, insomnia (difficulty falling asleep, staying asleep, or early awakening) is commonly reported among cancer patients and survivors. 10 Sleep-wake disturbances are important to address because, if left untreated, they can negatively effect quality of life. 9 , 11 – 13
A number of descriptive and intervention studies have targeted sleep-wake disturbances in various cancer populations, 2 , 14 , 15 and there are extensive published reviews of the physiology, measurement, and terminology of sleep-wake disturbances in cancer. 10 , 16 – 19 One common limitation noted within this literature has been the lack of conceptual clarity, in-part due to the inconsistent use or lack of disclosure of guiding theories, models, or frameworks. 17 , 19 To the best of our knowledge, a review article describing the theory, models, or frameworks used to guide research in this area has not been previously published.
The purpose of this article was to review theories, models, or frameworks applied to the problem of sleep-wake disturbances in the context of cancer. The main goals of this review were to (1) quantify, summarize, and compare the number of studies with and without explicitly described theories, models, or frameworks and (2) summarize and compare the theories, models, and frameworks that were used.
This review was important because theoretically grounded research advances science for evidence-based nursing research. One could question whether it is more important to base research on a theory, model, or framework or to have clear and consistent variable names, definitions, and measurement of sleep. We argue the former. Theories, models, or frameworks are important because they provide direction for research designs, assessment tools, and intervention development for effective symptom management. Typically, authors list the theories, models, or frameworks that specify interrelationships of critical attributes of concepts related to a problem. These critical attributes then link research findings to a body of knowledge that can be used by healthcare professionals for symptom management. 20 Therefore, it is through the use of the underlying theories, models, and frameworks that we better understand the definitions and measurement of certain research phenomena.
In nursing, the terms theory, model, and framework are often used interchangeably. By definition, a theory provides systematic explanations about relationships among phenomena, whereas a concept is based on observed characteristics or behaviors such as sleep. 20 A theory generally includes relational statements that are useful for explanation, description, prediction, control, or prescription based on definitions of the underlying concepts. 21 A model is a symbolic representation of relationships among variables or concepts. 20 A framework is often the illustration that describes the conceptual underpinnings of the research and can be based on a theory or, more specifically, on a concept (such as sleep-wake disturbances). 20 , 21
Data Sources
Searches were conducted to find relevant articles. Using MEDLINE, CINAHL, PubMed, and PsychINFO search engines (n = 4), citations from January 1, 1970, to July 31, 2008, were reviewed. Search terms used alone and in combination included the following: sleep, wake, disturbance, impaired sleep, insomnia, theoretical, conceptual, model, frame-work, adult, cancer , and survivor . We identified articles that included cancer patients undergoing treatment and cancer survivors (had completed treatment). Because these search terms resulted in more than 500 possible publications, articles were excluded from the review if they (1) were drug interventions, (2) focused on pediatric cancer or caregivers of cancer patients, (3) were instrument or questionnaire validation studies, (4) focused on statistical modeling of sleep-wake disturbances, (5) were letters to the editor or single case reports, or (6) reported sleep and wake as an incidental finding.
On the basis of the above, 73 studies were identified and reviewed. We quantified the number of studies with and without an explicitly stated a theory, model, or framework to clearly define these subsets of the studies and avoid assumptions of vague descriptions of theoretical underpinnings. We then summarized studies based on these criteria: (1) discipline of authors; (2) type of study; (3) sample information including ( a ) cancer diagnosis, ( b ) cancer stage, and ( c ) treatment status; (4) study variables; and (5) measures of sleep-wake. We then used χ 2 tests to compare these criteria between studies with and without a theory, model, or framework.
Next, we evaluated whether sleep was a primary or secondary variable within each theory, model, or framework. We developed a written summary of each theory, model, or framework and identified and compared their key attributes.
Quantification, Summary, and Comparison of Studies With and Without Explicitly Described Theories, Models, or Frameworks
Of the 73 studies, 51 (70%) did not contain a reported theory, model, or framework. 3 , 5 , 7 , 9 , 11 , 14 , 22 – 66 These studies were considered atheoretical because there was no explicit description of a theory, model, or framework that had guided the study. These 51 studies are listed alphabetically in Table 1 . The remaining 22 (30%) articles included a description of a theory, model, or framework that guided the study. 1 , 2 , 6 , 12 , 15 , 67 – 82 These 22 studies are listed alphabetically in Table 2 . Tables 1 and and2 2 provide a general summary of all studies based on our review criteria.
Summary of Studies Without a Theory, Model, or Framework
Abbreviations: NR, not reported; tx, treatment.
Summary of Studies With a Theory, Model, or Framework
Abbreviation: tx, treatment.
Comparisons between the 51 atheoretical and 22 theoretically based studies are provided in Table 3 . χ 2 Tests of differences indicated that studies that included a theory, model, or framework were significantly (1) more likely to include authors from the discipline of nursing and (2) less likely to have included wrist actigraphy as an outcome measure.
Comparisons Between Articles Without a Theory, Model, or Framework (n = 51) and Articles With a Theory, Model, or Framework (n = 22)
Significant differences are in bold.
Summary and Comparison of Theories, Models, and Frameworks
Table 4 contains a summary and comparison of theories, models, and frameworks. There were 2 nursing theories 83 , 84 and 9 different conceptual models. 67 , 71 , 72 , 85 – 93 Three conceptual models focused on sleep-wake disturbances as a primary variable of interest. Two theories and 6 additional models included sleep-wake disturbances as one of several variables. These 8 latter theories/models tended to include sleep-wake disturbances as a secondary variable or as one variable within a cluster of several symptoms (eg, pain, fatigue, and sleep-wake disturbances). A description of each theory and model follows, starting with ones that included sleep wake disturbances as a primary variable and concluding with those that included it as a secondary variable.
Abbreviation: SWD, sleep wake disturbances.
Models With Sleep-Wake Disturbances as a Primary Variable
Biobehavioral model of altered dysregulation in circadian systems.
The Biobehavioral Model of Altered Dysregulation in Circadian Systems proposes mechanisms that contribute to sleep-wake disturbances manifested as circadian dysregulation. 71 The model postulates that psychological functioning (stress, depression, mood states) have direct-reciprocal relationships with the endocrine system, autonomic nervous system, and sleep system. Dysregulation can be attributed to one or more of these biological systems. Dysregulation can be measured by sleep duration and sleep quality, as well as by cortisol, melatonin, and autonomic nervous system activity. The ultimate outcome of this dysregulation is poor quality of life, impaired well-being, poor treatment adherence, fatigue, disease progression, and poorer survival. 71 This model was included in one article. 71
SPIELMAN’S THREE-FACTOR INSOMNIA MODEL
Spielman’s Three-Factor Insomnia Model proposes interactions among predisposing, precipitating, and perpetuating factors of insomnia. 94 This cognitive-behavioral model is also known as the 3P model. The model includes both stress and behavioral factors to explain the evolution of insomnia and describe how individual differences cause initiation of acute disturbances in sleep that become chronic. The model proposes that people with insomnia have predisposing factors or traits that, when combined with life stress (precipitating factors), lead to chronic insomnia if there are maladaptive coping strategies (perpetuating factors). 86 When a person with predisposing factors of insomnia experiences one or more precipitating factors, the result can be acute episodes of insomnia. 94 Perpetuating factors are behaviors used to compensate for the loss of sleep. This model was included in 2 articles. 67 , 73
TWO-PROCESS MODEL OF SLEEP REGULATION
The Two-Process Model of Sleep Regulation provides an understanding of the physiological mechanisms that drive sleep and wakefulness. 88 The model has changed over time, as researchers were able to pinpoint mechanisms or processes of sleep in relation to the 2 major sleep cycles of non–rapid eye movement and rapid eye movement sleep. Visually, the model is a wavelike structure that shows the relationship between 2 physiological processes of sleep regulation, process S and process C. The homeostatic process (process S) increases during the awake state (drives the need for sleep) and decreases during sleep (decreases the need for sleep). Process S is considered the basic mechanism of sleep regulation. If there is a deficit of sleep, process S elicits a compensatory increase in the duration and intensity of sleep. This produces a sleepy feeling during wakefulness, thus driving the need or desire to correct this deficit by falling asleep. The circadian process (process C) determines alterations of high and low sleep propensity that are independent of prior sleeping-waking (the timing to sleep and wakefulness), which determines the onset and end of sleep. 88 Process C is directed by a clocklike mechanism that is not related to prior levels of sleep or the wake-like process S. Process C is the mechanism that helps the body stay asleep. It is driven by a pacemaker found in the brain called the hypothalamic suprachiasmatic nuclei, which works in conjunction with neurotransmitters that facilitate sleep and thermoregulatory processes. The interaction of process S and process C has been used to predict the timing and duration of sleep, structure of sleep, and changes in daytime wakefulness. 88 This model was adapted for use in one article. 1
Theories or Models With Sleep Disturbances as a Secondary or Clustered Variable
Model of quality of life.
The Model of Quality of Life identifies 4 domains that define an individual’s quality of life. Developed within the discipline of nursing, the model has been used to describe the quality of life in cancer patients. The 4 domains include physical well-being (eg, sleep), psychological well-being (eg, depression), social well-being (eg, family distress), and spiritual well-being (hopefulness). 90 – 92 , 95 , 96 Each domain is stated to act singly or in combination with the other domains and ultimately impact quality of life. The specific symptoms or factors within each quality of life domain have been further studied in various cancers such as breast cancer (see the study of Ferrell et al 90 ). For this model, sleep is depicted as a physical domain that could be singly or combined with other domain factors that impact quality of life. 90 This model was included in one article. 12
MODEL OF SYMPTOM MANAGEMENT
The Model of Symptom Management was a nursing model developed by nursing faculty from the University of California San Francisco’s Center for Symptom Management. 89 The conceptual model is based on the premise that a symptom is a subjective experience of a biophysical function, cognition, or sensation. Starting with the symptom experience, 3 inner relationships include the perception of the symptom, the evaluation of the symptom, and the response of the symptom, which is related back to the perception. The response then includes internal feelings, behaviors, or thoughts related to the perceived or actual presence of a health problem. Symptom management strategies are actions that overcome or stop the negative outcomes from the symptoms through medical or self-care interventions such as assessment and treatment. The outcome of the symptoms is a complex construct that includes 10 possible dimensions. 89 In this model, sleep-wake disturbances can be a symptom or an outcome. This model was included in 2 articles. 6 , 12
NEUROENDOCRINE-BASED REGULATORY FATIGUE MODEL
The Neuroendocrine-Based Regulatory Model was developed to describe mechanisms of fatigue in chronic illness. 93 Although thought to be a multidimensional concept, the model proposes that fatigue is strongly influenced by endocrine-based bio-chemicals such as tryptophan and melatonin. Any stress on the endocrine system creates biological effects that serve as markers for fatigue. 93 Antecedents of fatigue are stated to be either physiological (altered circadian rhythm of sleep/wake) or psychological (depression). The model proposes interventions that act as a mediator to reduce the impact of the antecedents on the endocrine system and improve fatigue. This model was used in 2 articles. 69 , 80
OREM’S SELF-CARE DEFICIT THEORY OF NURSING
Orem’s Self-care Deficit Theory of Nursing was developed in 1985 and focuses on the ability of an individual to perform self-care activities (termed self-care agency ) to maintain life, health, and well-being or the ability to care for others (termed dependent-care agency ). 20 , 84 The nursing role within the model is to assess the individual’s ability to perform self-care through a complete assessment of the current health state and symptoms being experienced by the individual. The model suggests that nurses provide 3 types of care: (1) total compensatory where the nurse provides total care when the individual cannot perform any self-care, (2) partial compensatory where the individual and nurse share self-care activities, and (3) supportive care or educational care where the nurse facilitates self-care through educating the individual with the tools to make decisions or learn skills needed for total self-care. 20 , 84 In this model, sleep-wake disturbances are symptoms that can result from cancer treatment that potentially impact self-care. This theory was used in 2 articles. 82 , 97
PIPER INTEGRATED FATIGUE MODEL
The Piper Integrated Fatigue Model has been used to study fatigue in relation to sleep disturbances in cancer patients. 15 The mid-range nursing model by Piper et al proposes a variety of factors as influencing subjective and objective manifestations of fatigue (see Piper et al 85 for the figure). Fatigue manifestations are divided into physiological, biochemical, and behavioral components. These components include physiological muscle fatigue, changes in behavioral work activity, and/or symptoms of depression, and they can occur in combination as fatigue becomes a chronic problem. 85 In this model, sleep-wake disturbances are one of several factors influencing fatigue. This model was included in 6 articles. 15 , 68 , 70 , 76 , 78 , 79
PSYCHOBIOLOGICAL HOT FLASH MODEL
This model depicts sleep disturbances as an outcome of meno-pausal hot flashes. It shows relationships among physiological hot flashes, perceived hot flashes, and the reporting of hot flash severity and bother, and it proposes that hot flashes contribute to a possible cluster of symptoms that include sleep-wake disturbances, mood disturbances, and changes in affect, which ultimately impact quality of life. This model was included in one article. 72
ROY ADAPTATION MODEL
Roy Adaptation Model is a complex nursing model postulating that human beings have the ability to adapt to changes in the environment. 87 Adaptation or coping with environmental change occurs through maintaining 4 subsystems: (1) physiologic needs, (2) self-concept, (3) interdependence, and (4) role function. For nursing, the goal is to use this model to guide health promotion and adaptation to illness. The model postulates that focal, contextual, or residual stimuli can be manipulated to promote adaptation based on stage of personal development, family development, and culture. 87 Adaptation responses can be effective or ineffective depending on the mode of adaptation. Effective adaptation responses are thought to promote integrity of the human system. 87 In this model, sleep can be conceptualized as a physiological mode of adaptation alone or in combination with other outcomes. 75 This model was included in one article. 75
THEORY OF UNPLEASANT SYMPTOMS
The Theory of Unpleasant Symptoms 83 is a mid-range nursing theory that has been used to describe sleep as it interacts with other symptoms. The theory has 3 interrelated components: (1) symptoms that the person experiences, (2) influencing factors that cause or affect the nature of the symptoms, and (3) consequences of the symptom experience. 83 Symptoms are the main component of the model and are measured in terms of intensity, quality, timing, and distress. The model posits that symptoms can occur alone or simultaneously with other symptoms and suggests that certain symptoms can catalyze other symptoms (see Figure 2 in the study of Lenz et al 83 ). Influencing factors are the physiological, psychological, and situational factors influenced by the symptoms. The third component is the consequence, defined as the impact of symptoms on an individual’s ability to perform functional or cognitive activities. Functional performance includes activities such as physical, daily living, social, interactive, and role performance (eg, work) activities. The interaction among the 3 major concepts of symptoms, influencing factors, and consequences is reciprocal and can change as the symptom experience changes. This suggests that symptoms can moderate or mediate the relationship between influencing factors and performance. 83 In this model, sleep-wake disturbances can be one of many symptoms or an influencing factor affecting other symptoms. This model was included in 2 articles. 2 , 74
The quantification of studies indicates that most research related to sleep-wake disturbances in cancer patients and survivors could be considered atheoretical. Most published studies that were reviewed here did not include an explicit description of a guiding theory, model, or framework. This is a significant limitation of existing research that may inhibit clarity of (1) the conceptual definition of sleep-wake disturbances, (2) the subsequent operationalization of sleep-wake disturbances, and (3) identification of contributing factors and consequences of sleep-wake disturbances.
The summary and comparison of studies indicated first that whether an article included a theory, model, or frame-work varied by discipline. Articles authored by nurses were significantly more likely to include an explicit description of a theory, model, or framework. It is well-known that non-nursing disciplines often do not disclose theoretical or conceptual models unless the purpose of the study is to further define the underlying concepts of the model. In addition, nonnursing journals may not allow space for the description of a theory or model. Therefore, one limitation of this review is that it is not known whether the studies were truly atheoretical or just lacking a written description of the theory, model, or framework.
The summary and comparison of studies also indicated that theoretically based articles were more likely to be based on subjective measurement rather than objective measures such as wrist actigraphy or polysomnography. Theories and models would seem to be equally relevant to subjective and objective measurement of sleep; however, exclusion of objective measures means that these theories and models are being tested only in the context of subjective sleep. In addition, the subjective measures that were used varied widely within both groups of studies (for details of sleep measures, see review 18 ). Although the Pittsburgh Sleep Quality Index was the most commonly used measure of subjective sleep, most studies used other types of questionnaires to measure sleep-wake disturbances. This is also reflected by the various terminology of sleep such as sleep-wake, insomnia, sleep disturbances, restless sleep , and tiredness . Because of these variations in terminology and measurement, it is difficult to ascertain if sleep-wake disturbances were conceptualized similarly across studies that did not include theories or models.
The summary and comparison of studies did not show significant differences by group in terms of type of study (descriptive or intervention) and sample characteristics. These criteria varied widely within both groups of studies. Varying sample characteristics may make comparisons across studies somewhat difficult.
This review revealed wide variation in the theories, models, and frameworks that are used to study sleep-wake disturbances in cancer. Several theories or models were only used in one study. The exceptions were Spielman’s Three-Factor Model, the Model of Symptom Management, Orem’s Self-care Deficit Theory, Piper’s Integrated Fatigue Model, the Neuroendocrine-Based Regulatory Fatigue Model, and the Theory of Unpleasant Symptoms. These were each used in more than one study. In addition, when an author produced multiple works, he/she tended to consistently use the same model. For example, Berger et al 15 , 68 – 70 , 78 , 79 used the Piper’s Integrated Fatigue Model and Payne 93 used the Neuroendocrine-Based Regulatory Fatigue Model in more than one study. On the other hand, Carpenter et al 2 , 6 , 72 used 3 different models for each of the studies reviewed. This illustrates that use of these theories may vary both within and between investigators and hypothesis driven.
Despite variation in the theories and models used, there were some commonalities. First, the theories and models overall are consistent with the idea that sleep disturbances are a combination of physiological, psychological, and behavioral phenomena. 71 , 86 , 88 Nine theories/models include physiological components, 71 , 72 , 84 , 85 , 88 – 90 , 93 , 94 eight included psychological components, 71 , 84 , 85 , 88 – 90 , 93 , 94 and six included behavioral components. 83 – 85 , 88 , 89 , 94 , 98
In addition, the theories/models suggest that other symptoms may be a combination of components and/or consequences of sleep-wake disturbances. For example, when the Theory of Unpleasant Symptoms 83 was applied, it included psychological and/or physical symptoms as components of sleep-wake disturbances. When the Model of Symptom Management was applied, the co-occurrence of sleep-wake and other symptoms was emphasized. 6 The Piper model 85 included sleep-wake disturbances as a cofactor of fatigue, suggesting that fatigue might be a consequence of sleep disturbances. Other consequences of sleep-wake disturbances included performance status in the Lenz model and impact on wakefulness in other models. 88 , 94
Finally, the models suggest that factors that contribute to sleep-wake disturbances are nonlinear; can change over time; and include predisposing, precipitating, and perpetuating factors. 94 Factors that initiate sleep disturbances (ie, predisposing or precipitating factors) may be different from those that lead to chronic sleep disturbances (ie, perpetuating factors). Thus, these models suggest that contributing factors should be reassessed over time.
Because of the high prevalence of sleep disturbances that contribute to poor quality of life in cancer patients, future research should focus on the development of a model or mid-range theory that is specific to the problem of sleep-wake disturbances in cancer. Such a model should include demographic, physiological, psychological, and behavioral factors of sleep disturbances and resulting health-related outcomes. In particular, cancer disease and treatment-related factors and other problems unique to this population should be included. For example, it is likely that women with breast cancer have unique health-related problems that might lead to sleep-wake disturbances (eg, premature menopause), 65 and these should be incorporated to increase model specificity. The model or theory should also account for symptoms as predisposing, co-occurring, or consequential phenomena. The theory/model also needs to clearly delineate the nonlinear nature of sleep disturbances. Finally, the model should consider the potentially broad impact of sleep and wake disturbances on physiological, psychological, behavioral, and social outcomes. Having a more specific conceptual model or theory for sleep-wake disturbances could help guide research by providing a mechanism that clearly defines and measures the concepts of sleep-wake disturbances in cancer. This would result in increased consistency among researchers by using similar types of measurement and similar variables increasing the ability to compare results across studies. Ultimately, a more specific model will provide researchers with a basis to facilitate symptom management through effective theory-based descriptive and interventions for this prominent problem in cancer.
In summary, although most existing research on sleep-wake disturbances in cancer does not include a description of a theory, model, or framework, several theories and models have been applied to this problem in this population at least once. The reviewed theories or models represent options that are available to guide research on sleep-wake disturbances in cancer populations. However, no one theory, model, or framework seems to provide an accurate picture of sleep-wake disturbances. Additional model or theory development is needed to enhance research that can be translated into improved clinical care in this area. It is recommended that authors should be encouraged to explicitly state the theory, model, or framework within the manuscript if one was used to guide the research. This could be accomplished through adding this type of reporting to publication guidelines to ensure that the readers can identify the conceptual underpinnings of the research.
ACKNOWLEDGMENT
The authors gratefully acknowledge Phyllis Dexter for the editorial support.
This work was supported by the following: National Institute of Nursing Research 1 F31 NR009890, American Cancer Society predoctoral fellowship (Doctoral Scholarship in Cancer Nursing 108142), Oncology Nursing Foundation predoctoral scholarship (2004), and Walther Cancer Institute predoctoral fellowship (2004–2008). National Cancer Institute R25 CA117865 post-doctoral fellowship.
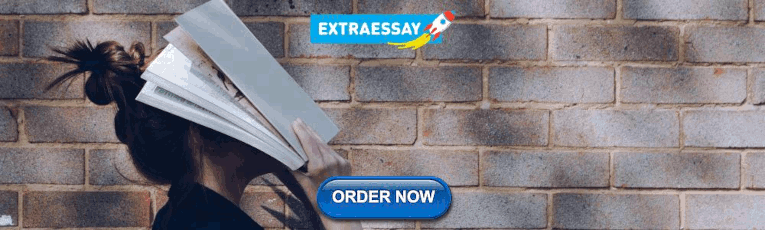
IMAGES
VIDEO
COMMENTS
Several other tasks have been used in the sleep deprivation studies (Table 1). For example, motor function, rhythm, ... However, although attention is needed to perform any task to some extent, the hypothesis that sleep deprivation can have an independent effect on other cognitive functions such as memory cannot be ruled out. Nevertheless, when ...
For example, sleep-deprived individuals are less accurate at rating facial expressions within the moderate range of emotional strength 93 and rate neutral ... Supporting this hypothesis, sleep-deprived individuals and habitually poor sleepers demonstrate impaired ... Sleep deprivation (SD) decreases encoding-related activity within the ...
There is a growing body of evidence suggesting a critical role of sleep in learning and memory (Diekelmann & Born, 2010).On the one hand, offline memory consolidation during sleep benefits both declarative and procedural memories acquired during preceding wake (Klinzing et al., 2019).On the other hand, memory encoding capacity has been argued to saturate gradually during wake, with sleep ...
Another theory regarding why we sleep involves sleep's importance for cognitive function and memory formation (Rattenborg, Lesku, Martinez-Gonzalez, & Lima, 2007). Indeed, we know sleep deprivation results in disruptions in cognition and memory deficits (Brown, 2012), leading to impairments in our abilities to maintain attention, make ...
The most common sleep health characteristics studied in students have been sleep duration and insomnia symptoms (i.e., the inability to fall or stay asleep) (Hershner, 2020).Sleep is considered a positive resource (Buysse, 2014), that promotes physical and mental well-being pivotal for academic achievement (José Sá, 2020).However, we lack clear guidance on how much sleep is sufficient for ...
Well-controlled sleep deprivation studies have shown that lack of sleep not only increases fatigue and sleepiness but also worsens cognitive performance. 2,3,16,17 In fact, the cognitive ...
During sleep, the brain weakens the connections among nerve cells, apparently conserving energy and, paradoxically, aiding memory. Every night, while we lie asleep, blind, dumb and almost ...
Chronic sleep deprivation (8-h, 6-h, 4-h — time in bed (TIB) per night for 14 nights) resulted in cumulative dose-dependent deficits in psychomotor vigilance performance, and daytime sleepiness showed an acute response but did not differentiate between the 6-h and 4-h conditions in Van Dongen's trial of 48 young adults (mean age 26 ± 3.6 y). ...
consequences of sleep deprivation are higher on tasks for simple versus complex order thinking. These studies have speculated that the repercussions of sleep deprivation are higher on memory and vigilance than complex higher order thinking (Philibert 2005); however, there are not enough specific tests conducted to support this theory.
As such, sleep health can include both the increased risk of chronic disease associated with long-term sleep deprivation, and acute effects of sleep deprivation such as stymied emotional regulation. ... For example, a theory-based intervention for changing sleep behaviors, must be able to demonstrate the causal connection between the constructs ...
For example, in a study investigating the effects of sleep deprivation on cognitive performance, a directional hypothesis might state that as sleep deprivation (Independent Variable) increases, cognitive performance (Dependent Variable) decreases (Killgore, 2010). ... Directional Hypothesis Examples 1. Exercise and Heart Health
For example, the increasing variability of reaction times on the Psychomotor Vigilance Test as the number of hours of sleep loss increases would not be predicted by the lapse hypothesis alone. ... Sleep deprivation has also been implicated with increased risk of on-the-job errors, personal conflicts, health complaints, poorer academic ...
All animals, for example need to retain the ability for arousal during sleep, but to different amounts and in different ways depending on their circumstances. Unihemispheric sleep is another example of how sleep has been adapted to specific needs [19,30,31,32]. Evolution has allowed for a variety of ways to spend the day and night, within which ...
Long-term total sleep deprivation: no sleep for more than 48 hours Nocturnal awakenings: waking up during a period of sleep Limitations As with all correlational studies, the findings from the current study cannot reveal causal relationships between sleep deprivation and personal productivity. Another key limitation has been identified.
Figure 1. Conceptual Cognitive Framework of Insomnia: During the pre-sleep situation, when attentional resources are captured by insomnia-related stimuli, either directly or through insomnia-related cognitive and emotional values, distress is triggered, thus resulting in a distorted perception of sleep quality, which, in turn, worsens the sleep-initiation process.
As in restorative theory, this concept deals with information processing and memory formation. Research suggests that sleep loss leads to less structural plasticity, which can have a negative effect on alertness, cognition, and mood. Sleep deprivation also compromises memory formation, which is related to learning and plasticity.
Abstract. Sleep is highly conserved across evolution, suggesting vital biological functions that are yet to be fully understood. Animals and humans experiencing partial sleep restriction usually exhibit detrimental physiological responses, while total and prolonged sleep loss could lead to death. The perturbation of sleep homeostasis is usually ...
Consider a study designed to examine the relationship between sleep deprivation and test performance. The hypothesis might be: "This study is designed to assess the hypothesis that sleep-deprived people will perform worse on a test than individuals who are not sleep-deprived." ... Examples of a null hypothesis include: "There is no difference ...
Sleep across the Life Span. One pressing question about the sleep-memory link concerns how it manifests over one's lifetime. Spencer and Riggins examined this link at the younger end of the age spectrum.They review evidence that naps in early childhood are essential for memory consolidation, presenting a fascinating new hypothesis connecting the psychological, physiological, and ...
Sleep deprivation and sleep insufficiency can stem from many factors. Sometimes they are the result of poor habits surrounding sleep. For example, an inconsistent sleep schedule, long daytime naps, the use of digital devices before bed, and a noisy or bright sleep environment can all interfere with a person's ability to get enough sleep.
Sleep patterning consists of (1) the temporal spacing of sleep and wakefulness within a 24-hour period, driven by the need for sleep (referred to as "homeostatic sleep pressure") and by circadian rhythm, and (2) the ordering of different sleep stages within a given sleep period, known as "ultradian" cycles.
One hypothesis is that sleep is necessary to replenish energy stores in the brain that are depleted during wakefulness. This theory posits that during waking, a relatively active metabolic period in the brain, energy stores become progressively diminished, thereby promoting sleep. During sleep, there is recovery of energy stores and thus ...
This review revealed wide variation in the theories, models, and frameworks that are used to study sleep-wake disturbances in cancer. Several theories or models were only used in one study. The exceptions were Spielman's Three-Factor Model, the Model of Symptom Management, Orem's Self-care Deficit Theory, Piper's Integrated Fatigue Model ...