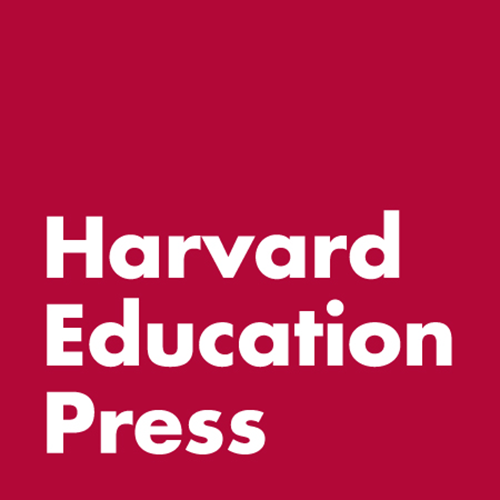
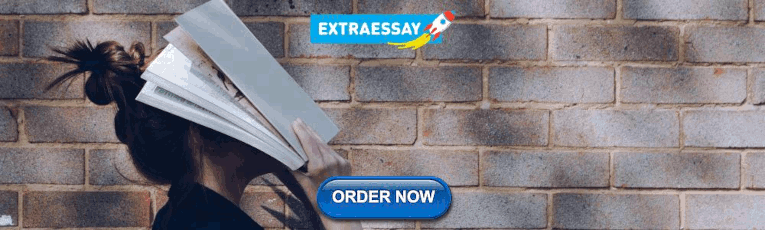
On The Site
Harvard educational review.
Edited by Maya Alkateb-Chami, Jane Choi, Jeannette Garcia Coppersmith, Ron Grady, Phoebe A. Grant-Robinson, Pennie M. Gregory, Jennifer Ha, Woohee Kim, Catherine E. Pitcher, Elizabeth Salinas, Caroline Tucker, Kemeyawi Q. Wahpepah
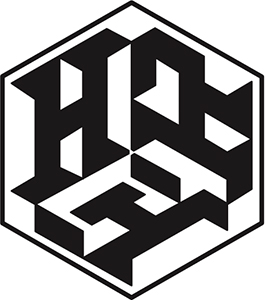
Individuals
Institutions.
- Read the journal here
Journal Information
- ISSN: 0017-8055
- eISSN: 1943-5045
- Keywords: scholarly journal, education research
- First Issue: 1930
- Frequency: Quarterly
Description
The Harvard Educational Review (HER) is a scholarly journal of opinion and research in education. The Editorial Board aims to publish pieces from interdisciplinary and wide-ranging fields that advance our understanding of educational theory, equity, and practice. HER encourages submissions from established and emerging scholars, as well as from practitioners working in the field of education. Since its founding in 1930, HER has been central to elevating pieces and debates that tackle various dimensions of educational justice, with circulation to researchers, policymakers, teachers, and administrators.
Our Editorial Board is composed entirely of doctoral students from the Harvard Graduate School of Education who review all manuscripts considered for publication. For more information on the current Editorial Board, please see here.
A subscription to the Review includes access to the full-text electronic archives at our Subscribers-Only-Website .
Editorial Board
2023-2024 Harvard Educational Review Editorial Board Members
Maya Alkateb-Chami Development and Partnerships Editor, 2023-2024 Editor, 2022-2024 [email protected]
Maya Alkateb-Chami is a PhD student at the Harvard Graduate School of Education. Her research focuses on the role of schooling in fostering just futures—specifically in relation to language of instruction policies in multilingual contexts and with a focus on epistemic injustice. Prior to starting doctoral studies, she was the Managing Director of Columbia University’s Human Rights Institute, where she supported and co-led a team of lawyers working to advance human rights through research, education, and advocacy. Prior to that, she was the Executive Director of Jusoor, a nonprofit organization that helps conflict-affected Syrian youth and children pursue their education in four countries. Alkateb-Chami is a Fulbright Scholar and UNESCO cultural heritage expert. She holds an MEd in Language and Literacy from Harvard University; an MSc in Education from Indiana University, Bloomington; and a BA in Political Science from Damascus University, and her research on arts-based youth empowerment won the annual Master’s Thesis Award of the U.S. Society for Education Through Art.
Jane Choi Editor, 2023-2025
Jane Choi is a second-year PhD student in Sociology with broad interests in culture, education, and inequality. Her research examines intra-racial and interracial boundaries in US educational contexts. She has researched legacy and first-generation students at Ivy League colleges, families served by Head Start and Early Head Start programs, and parents of pre-K and kindergarten-age children in the New York City School District. Previously, Jane worked as a Research Assistant in the Family Well-Being and Children’s Development policy area at MDRC and received a BA in Sociology from Columbia University.
Jeannette Garcia Coppersmith Content Editor, 2023-2024 Editor, 2022-2024 [email protected]
Jeannette Garcia Coppersmith is a fourth-year Education PhD student in the Human Development, Learning and Teaching concentration at the Harvard Graduate School of Education. A former public middle and high school mathematics teacher and department chair, she is interested in understanding the mechanisms that contribute to disparities in secondary mathematics education, particularly how teacher beliefs and biases intersect with the social-psychological processes and pedagogical choices involved in math teaching. Jeannette holds an EdM in Learning and Teaching from the Harvard Graduate School of Education where she studied as an Urban Scholar and a BA in Environmental Sciences from the University of California, Berkeley.
Ron Grady Editor, 2023-2025
Ron Grady is a second-year doctoral student in the Human Development, Learning, and Teaching concentration at the Harvard Graduate School of Education. His central curiosities involve the social worlds and peer cultures of young children, wondering how lived experience is both constructed within and revealed throughout play, the creation of art and narrative, and through interaction with/production of visual artifacts such as photography and film. Ron also works extensively with educators interested in developing and deepening practices rooted in reflection on, inquiry into, and translation of the social, emotional, and aesthetic aspects of their classroom ecosystems. Prior to his doctoral studies, Ron worked as a preschool teacher in New Orleans. He holds a MS in Early Childhood Education from the Erikson Institute and a BA in Psychology with Honors in Education from Stanford University.
Phoebe A. Grant-Robinson Editor, 2023-2024
Phoebe A. Grant-Robinson is a first year student in the Doctor of Education Leadership(EdLD) program at the Harvard Graduate School of Education. Her ultimate quest is to position all students as drivers of their destiny. Phoebe is passionate about early learning and literacy. She is committed to ensuring that districts and school leaders, have the necessary tools to create equitable learning organizations that facilitate the academic and social well-being of all students. Phoebe is particularly interested in the intersection of homeless students and literacy. Prior to her doctoral studies, Phoebe was a Special Education Instructional Specialist. Supporting a portfolio of more than thirty schools, she facilitated the rollout of New York City’s Special Education Reform. Phoebe also served as an elementary school principal. She holds a BS in Inclusive Education from Syracuse University, and an MS in Curriculum and Instruction from Pace University.
Pennie M. Gregory Editor, 2023-2024
Pennie M. Gregory is a second-year student in the Doctor of Education Leadership (EdLD) program at the Harvard Graduate School of Education. Pennie was born in Incheon, South Korea and raised in Gary, Indiana. She has decades of experience leading efforts to improve outcomes for students with disabilities first as a special education teacher and then as a school district special education administrator. Prior to her doctoral studies, Pennie helped to create Indiana’s first Aspiring Special Education Leadership Institute (ASELI) and served as its Director. She was also the Capacity Events Director for MelanatED Leaders, an organization created to support educational leaders of color in Indianapolis. Pennie has a unique perspective, having worked with members of the school community, with advocacy organizations, and supporting state special education leaders. Pennie holds an EdM in Education Leadership from Marian University.
Jennifer Ha Editor, 2023-2025
Jen Ha is a second-year PhD student in the Culture, Institutions, and Society concentration at the Harvard Graduate School of Education. Her research explores how high school students learn to write personal narratives for school applications, scholarships, and professional opportunities amidst changing landscapes in college access and admissions. Prior to doctoral studies, Jen served as the Coordinator of Public Humanities at Bard Graduate Center and worked in several roles organizing academic enrichment opportunities and supporting postsecondary planning for students in New Haven and New York City. Jen holds a BA in Humanities from Yale University, where she was an Education Studies Scholar.
Woohee Kim Editor, 2023-2025
Woohee Kim is a PhD student studying youth activists’ civic and pedagogical practices. She is a scholar-activist dedicated to creating spaces for pedagogies of resistance and transformative possibilities. Shaped by her activism and research across South Korea, the US, and the UK, Woohee seeks to interrogate how educational spaces are shaped as cultural and political sites and reshaped by activists as sites of struggle. She hopes to continue exploring the intersections of education, knowledge, power, and resistance.
Catherine E. Pitcher Editor, 2023-2025
Catherine is a second-year doctoral student at Harvard Graduate School of Education in the Culture, Institutions, and Society program. She has over 10 years of experience in education in the US in roles that range from special education teacher to instructional coach to department head to educational game designer. She started working in Palestine in 2017, first teaching, and then designing and implementing educational programming. Currently, she is working on research to understand how Palestinian youth think about and build their futures and continues to lead programming in the West Bank, Gaza, and East Jerusalem. She holds an EdM from Harvard in International Education Policy.
Elizabeth Salinas Editor, 2023-2025
Elizabeth Salinas is a doctoral student in the Education Policy and Program Evaluation concentration at HGSE. She is interested in the intersection of higher education and the social safety net and hopes to examine policies that address basic needs insecurity among college students. Before her doctoral studies, Liz was a research director at a public policy consulting firm. There, she supported government, education, and philanthropy leaders by conducting and translating research into clear and actionable information. Previously, Liz served as a high school physics teacher in her hometown in Texas and as a STEM outreach program director at her alma mater. She currently sits on the Board of Directors at Leadership Enterprise for a Diverse America, a nonprofit organization working to diversify the leadership pipeline in the United States. Liz holds a bachelor’s degree in civil engineering from the Massachusetts Institute of Technology and a master’s degree in higher education from the Harvard Graduate School of Education.
Caroline Tucker Co-Chair, 2023-2024 Editor, 2022-2024 [email protected]
Caroline Tucker is a fourth-year doctoral student in the Culture, Institutions, and Society concentration at the Harvard Graduate School of Education. Her research focuses on the history and organizational dynamics of women’s colleges as women gained entry into the professions and coeducation took root in the United States. She is also a research assistant for the Harvard and the Legacy of Slavery Initiative’s Subcommittee on Curriculum and the editorial assistant for Into Practice, the pedagogy newsletter distributed by Harvard University’s Office of the Vice Provost for Advances in Learning. Prior to her doctoral studies, Caroline served as an American politics and English teaching fellow in London and worked in college advising. Caroline holds a BA in History from Princeton University, an MA in the Social Sciences from the University of Chicago, and an EdM in Higher Education from the Harvard Graduate School of Education.
Kemeyawi Q. Wahpepah Co-Chair, 2023-2024 Editor, 2022-2024 [email protected]
Kemeyawi Q. Wahpepah (Kickapoo, Sac & Fox) is a fourth-year doctoral student in the Culture, Institutions, and Society concentration at the Harvard Graduate School of Education. Their research explores how settler colonialism is addressed in K-12 history and social studies classrooms in the United States. Prior to their doctoral studies, Kemeyawi taught middle and high school English and history for eleven years in Boston and New York City. They hold an MS in Middle Childhood Education from Hunter College and an AB in Social Studies from Harvard University.
Submission Information
Click here to view submission guidelines .
Contact Information
Click here to view contact information for the editorial board and customer service .
Subscriber Support
Individual subscriptions must have an individual name in the given address for shipment. Individual copies are not for multiple readers or libraries. Individual accounts come with a personal username and password for access to online archives. Online access instructions will be attached to your order confirmation e-mail.
Institutional rates apply to libraries and organizations with multiple readers. Institutions receive digital access to content on Meridian from IP addresses via theIPregistry.org (by sending HER your PSI Org ID).
Online access instructions will be attached to your order confirmation e-mail. If you have questions about using theIPregistry.org you may find the answers in their FAQs. Otherwise please let us know at [email protected] .
How to Subscribe
To order online via credit card, please use the subscribe button at the top of this page.
To order by phone, please call 888-437-1437.
Checks can be mailed to Harvard Educational Review C/O Fulco, 30 Broad Street, Suite 6, Denville, NJ 07834. (Please include reference to your subscriber number if you are renewing. Institutions must include their PSI Org ID or follow up with this information via email to [email protected] .)
Permissions
Click here to view permissions information.
Article Submission FAQ
Closing the open call, question: “i have already submitted an article to her and i am awaiting a decision, what can i expect”.
Answer: First, any manuscripts already submitted through the open call and acknowledged by HER, as well as all invited manuscripts, R&R’d manuscripts, and manuscripts currently in production are NOT affected in any way by our pause in open calls. Editors are working to move through all current submissions and you can expect to receive any updates or decisions as we move through each step of our production process. If you have any questions, please contact the Co-Chairs, Caroline Tucker and Kemeyawi Wahpepah at [email protected] .
Question: “Can you share more about why you are closing the open call?”
Answer: As a graduate student run journal, we perform our editorial tasks in addition to our daily lives as doctoral students. We have been (and continue to be) incredibly grateful for the authors who share their work with us. In closing the open call, we hope to give ourselves time to review each manuscript in the best manner possible.
Submissions
Question: “what manuscripts are a good fit for her ”.
Answer: As a generalist scholarly journal, HER publishes on a wide range of topics within the field of education and related disciplines. We receive many articles that deserve publication, but due to the restrictions of print publication, we are only able to publish very few in the journal. The originality and import of the findings, as well as the accessibility of a piece to HER’s interdisciplinary, international audience which includes education practitioners, are key criteria in determining if an article will be selected for publication.
We strongly recommend that prospective authors review the current and past issues of HER to see the types of articles we have published recently. If you are unsure whether your manuscript is a good fit, please reach out to the Content Editor at [email protected] .
Question: “What makes HER a developmental journal?”
Answer: Supporting the development of high-quality education research is a key tenet of HER’s mission. HER promotes this development through offering comprehensive feedback to authors. All manuscripts that pass the first stage of our review process (see below) receive detailed feedback. For accepted manuscripts, HER also has a unique feedback process called casting whereby two editors carefully read a manuscript and offer overarching suggestions to strengthen and clarify the argument.
Question: “What is a Voices piece and how does it differ from an essay?”
Answer: Voices pieces are first-person reflections about an education-related topic rather than empirical or theoretical essays. Our strongest pieces have often come from educators and policy makers who draw on their personal experiences in the education field. Although they may not present data or generate theory, Voices pieces should still advance a cogent argument, drawing on appropriate literature to support any claims asserted. For examples of Voices pieces, please see Alvarez et al. (2021) and Snow (2021).
Question: “Does HER accept Book Note or book review submissions?”
Answer: No, all Book Notes are written internally by members of the Editorial Board.
Question: “If I want to submit a book for review consideration, who do I contact?”
Answer: Please send details about your book to the Content Editor at [email protected].
Manuscript Formatting
Question: “the submission guidelines state that manuscripts should be a maximum of 9,000 words – including abstract, appendices, and references. is this applicable only for research articles, or should the word count limit be followed for other manuscripts, such as essays”.
Answer: The 9,000-word limit is the same for all categories of manuscripts.
Question: “We are trying to figure out the best way to mask our names in the references. Is it OK if we do not cite any of our references in the reference list? Our names have been removed in the in-text citations. We just cite Author (date).”
Answer: Any references that identify the author/s in the text must be masked or made anonymous (e.g., instead of citing “Field & Bloom, 2007,” cite “Author/s, 2007”). For the reference list, place the citations alphabetically as “Author/s. (2007)” You can also indicate that details are omitted for blind review. Articles can also be blinded effectively by use of the third person in the manuscript. For example, rather than “in an earlier article, we showed that” substitute something like “as has been shown in Field & Bloom, 2007.” In this case, there is no need to mask the reference in the list. Please do not submit a title page as part of your manuscript. We will capture the contact information and any author statement about the fit and scope of the work in the submission form. Finally, please save the uploaded manuscript as the title of the manuscript and do not include the author/s name/s.
Invitations
Question: “can i be invited to submit a manuscript how”.
Answer: If you think your manuscript is a strong fit for HER, we welcome your request for invitation. Invited manuscripts receive one round of feedback from Editors before the piece enters the formal review process. To submit information about your manuscript for the Board to consider for invitation, please fill out the Invitation Request Form. Please provide as many details as possible. Whether we could invite your manuscript depends on the interest and availability of the current Board. Once you submit the form, we will give you an update in about 2–3 weeks on whether there are Editors who are interested in inviting your manuscript.
Review Timeline
Question: “who reviews manuscripts”.
Answer: All manuscripts are reviewed by the Editorial Board composed of doctoral students at Harvard University.
Question: “What is the HER evaluation process as a student-run journal?”
Answer: HER does not utilize the traditional external peer review process and instead has an internal, two-stage review procedure.
Upon submission, every manuscript receives a preliminary assessment by the Content Editor to confirm that the formatting requirements have been carefully followed in preparation of the manuscript, and that the manuscript is in accord with the scope and aim of the journal. The manuscript then formally enters the review process.
In the first stage of review, all manuscripts are read by a minimum of two Editorial Board members. During the second stage of review, manuscripts are read by the full Editorial Board at a weekly meeting.
Question: “How long after submission can I expect a decision on my manuscript?”
Answer: It usually takes 6 to 10 weeks for a manuscript to complete the first stage of review and an additional 12 weeks for a manuscript to complete the second stage. Due to time constraints and the large volume of manuscripts received, HER only provides detailed comments on manuscripts that complete the second stage of review.
Question: “How soon are accepted pieces published?”
Answer: The date of publication depends entirely on how many manuscripts are already in the queue for an issue. Typically, however, it takes about 6 months post-acceptance for a piece to be published.
Submission Process
Question: “how do i submit a manuscript for publication in her”.
Answer: Manuscripts are submitted through HER’s Submittable platform, accessible here. All first-time submitters must create an account to access the platform. You can find details on our submission guidelines on our Submissions page.
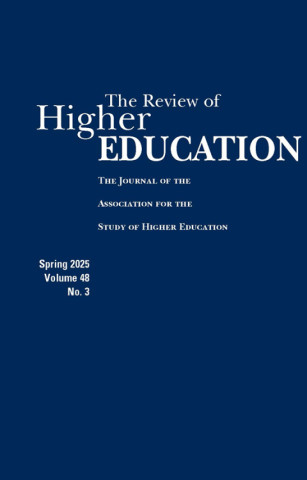
The Review of Higher Education
Penny A. Pasque, The Ohio State University; Thomas F. Nelson Laird, Indiana University, Bloomington
Journal Details
The Review of Higher Education is interested in empirical research studies, empirically-based historical and theoretical articles, and scholarly reviews and essays that move the study of colleges and universities forward. The most central aspect of RHE is the saliency of the subject matter to other scholars in the field as well as its usefulness to academic leaders and public policymakers. Manuscripts submitted for RHE need to extend the literature in the field of higher education and may connect across fields and disciplines when relevant. Selection of articles for publication is based solely on the merits of the manuscripts with regards to conceptual or theoretical frameworks, methodological accurateness and suitability, and/or the clarity of ideas and gathered facts presented. Additionally, our publications center around issues within US Higher Education and any manuscript that we send for review must have clear implications for US Higher Education.
Guidelines for Contributors
Manuscripts should be typed, serif or san serif text as recommended by APA 7th edition (e.g., 11-point Calibri, 11-point Arial, and 10-point Lucida Sans Unicode, 12-point Times New Roman, 11-point Georgia, 10-point Computer Modern) double-spaced throughout, including block quotes and references. Each page should be numbered on the top right side of the page consecutively and include a running head. Please supply the title of your submission, an abstract of 100 or fewer words, and keywords as the first page of your manuscript submission (this page does not count towards your page limit). The names, institutional affiliations, addresses, phone numbers, email addresses and a short biography of authors should appear on a separate cover page to aid proper masking during the review process. Initial and revised submissions should not run more than 32 pages (excluding abstract, keywords, and references; including tables, figures and appendices). Authors should follow instructions in the 7th edition Publication Manual of the American Psychological Association; any manuscripts not following all APA guidelines will not be reviewed. Please do not change fonts, spacing, or margins or use style formatting features at any point in the manuscript except for tables. All tables should be submitted in a mutable format (i.e. not a fixed image). Please upload your manuscript as a word document. All supporting materials (i.e., tables, figures, appendices) should be editable in the manuscript or a separate word document (i.e., do not embedded tables or figures). For a fixed image, please upload a separate high-resolution JPEG.
Authors should use their best judgment when masking citations. Masking some or all citations that include an author’s name can help prevent reviewers from knowing the identities of the authors. However, in certain circumstances masking citations is unnecessary or could itself reveal the identities of manuscript authors. Because authors are in the best position to know when masking citations will be effective, the editorial team will generally defer to them for these decisions.
Manuscripts are to be submitted in Word online at mc.manuscriptcentral.com/rhe . (If you have not previously registered on this website, click on the “Register here” link to create a new account.) Once you log on, click on the “Author Center” link and then follow the printed instructions to submit your manuscript.
The term “conflict of interest” means any financial or other interest which conflicts with the work of the individual because it (1) could significantly impair the individual’s objectivity or (2) could create an unfair advantage for any person or organization. We recommend all authors review and adhere to the ASHE Conflict of Interest Policy before submitting any and all work. Please refer to the policy at ashe.ws/ashe_coi
Please note that The Review of Higher Education does not require potential contributors to pay an article submission fee in order to be considered for publication. Any other website that purports to be affiliated with the Journal and that requires you to pay an article submission fee is fraudulent. Do not provide payment information. Instead, we ask that you contact the RHE editorial office at [email protected] or William Breichner the Journals Publisher at the Johns Hopkins University Press [email protected] .
Author Checklist for New Submissions
Page Limit. Manuscripts should not go over 32 pages (excluding abstract, keywords, and references; including tables, figures and appendices.)
Masked Review. All author information (i.e., name, affiliation, email, phone number, address) should appear on a separate cover page of the manuscript. The manuscript should have no indication of authorship. Any indication of authorship will result in your manuscript being unsubmitted.
Formatting. Manuscripts should be typed, serif or san serif text as recommended by APA 7th edition (e.g., 11-point Calibri, 11-point Arial, and 10-point Lucida Sans Unicode, 12-point Times New Roman, 11-point Georgia, 10-point Computer Modern), double-spaced throughout, including block quotes and references, and each page should be numbered on the top right side of the page consecutively. Authors should follow instructions in the 7th edition Publication Manual of the American Psychological Association; this includes running heads, heading levels, spacing, margins, etc.. Any manuscripts not following APA 7th edition will be unsubmitted. [Please note, the RHE editorial team recommends 12-pt Times New Roman font to ensure proper format conversion within the ScholarOne system.]
Abstract. All manuscripts must include an abstract of 100 words or fewer, and keywords as the first page of your manuscript submission (this page does not count towards your page limit).
Author Note. An Author’s note may include Land Acknowledgments, Disclosure Statement (i.e., funding sources), or other acknowledgments. This should appear on your title page (not in the masked manuscript).
Tables. All tables should be editable. Tables may be uploaded in the manuscript itself or in a separate word document. All tables must be interpretable by readers without the reference to the manuscript. Do not duplicate information from the manuscript into tables. Tables must present additional information from what has already been stated in the manuscript.
Figures. Figures should be editable in the manuscript or a separate word document (i.e., no embedded tables). For fixed images, please upload high-resolution JPEGs separately.
References. The reference page should follow 7th edition APA guidelines and be double spaced throughout (reference pages do not count toward your page limit).
Appendices. Appendices should generally run no more than 3 manuscript pages.
Additional Checklist for Revised Submissions
Revised manuscripts should follow the checklist above, with the following additional notes:
Page Limit. Revised manuscripts should stay within the page limit for new submissions (32 pages). However, we do realize that this is not always possible, and we may allow for a couple of extra pages for your revisions. Extensions to your page length will be subject to editor approval upon resubmission, but may not exceed 35 pages (excluding abstract, keywords, and references).
- Author Response to Reviewer Comments. At the beginning of your revised manuscript file, please include a separate masked statement that indicates fully [1] all changes that have been made in response to the reviewer and editor suggestions and the pages on which those changes may be found in the revised manuscript and [2] those reviewer and editor suggestions that are not addressed in the revised manuscript and a rationale for why you think such revisions are not necessary. This can be in the form of a table or text paragraphs and must appear at the front of your revised manuscript document. Your response to reviewer and editor comments will not count toward your manuscript page limit. Please note that, because you will be adding your response to the reviewer and editor feedback to the beginning of your submission, this may change the page numbers of your document unless you change the pagination and start your manuscript itself on page 1. The choice is yours but either way, please ensure that you reference the appropriate page numbers within your manuscript in these responses. Additionally, when you submit your revised manuscript, there will be a submission box labeled “Author Response to Decision Letter”. You are not required to duplicate information already provided in the manuscript, but instead may use this to send a note to the reviewer team (e.g., an anonymous cover letter or note of appreciation for feedback). Please maintain anonymity throughout the review process by NOT including your name or by masking any potentially identifying information when providing your response to the reviewer's feedback (both in documents and the ScholarOne system).
Editorial Correspondence
Please address all correspondence about submitting articles (no subscriptions, please) to one or both of the following editors:
Dr. Penny A. Pasque, PhD Editor, Review of Higher Education 341 C Ramseyer Hall 29 W. Woodruff Avenue The Ohio State University Columbus, OH 43210 email: [email protected]
Dr. Thomas F. Nelson Laird, PhD Editor, Review of Higher Education 201 North Rose Avenue Indiana University School of Education Bloomington, IN 47405-100 email: [email protected]
Submission Policy
RHE publishes original works that are not available elsewhere. We ask that all manuscripts submitted to our journal for review are not published, in press or submitted to other journals while under our review. Additionally, reprints and translations of previously published articles will not be accepted.
Type of Preliminary Review
RHE utilizes a collaborative review process that requires several members of the editorial team to ensure that submitted manuscripts are suitable before being sent out for masked peer-review. Members of this team include a Editor, Associate Editor and Managing Editors. Managing Editors complete an initial review of manuscripts to ensure authors meet RHE ’s Author Guidelines and work with submitting authors to address preliminary issues and concerns (i.e., APA formatting). Editors and Associate Editors work together to decide whether it should be sent out for review and select appropriate reviewers for the manuscript.
Type of Review
When a manuscript is determined as suitable for review by the collaborative decision of the editorial team, Editors and/or Associate Editors will assign reviewers. Both the authors’ and reviewers’ are masked throughout the review and decision process.
Criteria for Review
Criteria for review include, but are not limited to, the significance of the topic to higher education, completeness of the literature review, appropriateness of the research methods or historical analysis, and the quality of the discussion concerning the implications of the findings for theory, research, and practice. In addition, we look for the congruence of thought and approach throughout the manuscript components.
Type of Revisions Process
Some authors will receive a “Major Revision” or “Minor Revision” decision. Authors who receive such decisions are encouraged to carefully attend to reviewer’s comments and recommendations and resubmit their revised manuscripts for another round of reviews. When submitting their revised manuscripts, authors are asked to include a response letter and indicate how they have responded to reviewer comments and recommendations. In some instances, authors may be asked to revise and resubmit a manuscript more than once.
Review Process Once Revised
Revised manuscripts are sent to the reviewers who originally made comments and recommendations regarding the manuscript, whenever possible. We rely on our editorial board and ad-hoc reviewers who volunteer their time and we give those reviewers a month to provide thorough feedback. Please see attached pdf for a visual representation of the RHE workflow .
Timetable (approx.)
- Managing Editor Technical Checks – 1-3 days
- Editor reviews and assigns manuscript to Associate Editors – 3-5 days
- Associate Editor reviews and invites reviewers – 3-5 days
- Reviewer comments due – 30 days provided for reviews
- Associate Editor makes a recommendation – 5-7 days
- Editor makes decision – 5-7 days
- If R&R, authors revise and resubmit manuscript – 90 days provided for revisions
- Repeat process above until manuscript is accepted or rejected -
Type of review for book reviews
Book reviews are the responsibility of the associate editor of book reviews. Decisions about acceptance of a book review are made by that associate editor.
The Hopkins Press Journals Ethics and Malpractice Statement can be found at the ethics-and-malpractice page.
The Review of Higher Education expects all authors to review and adhere to ASHE’s Conflict of Interest Policy before submitting any and all work. The term “conflict of interest” means any financial or other interest which conflicts with the work of the individual because it (1) could significantly impair the individual’s objectivity or (2) could create an unfair advantage for any person or organization. Please refer to the policy at ashe.ws/ashe_coi .
Guidelines for Book Reviews
RHE publishes book reviews of original research, summaries of research, or scholarly thinking in book form. We do not publish reviews of books or media that would be described as expert opinion or advice for practitioners.
The journal publishes reviews of current books, meaning books published no more than 12 months prior to submission to the associate editor in charge of book reviews.
If you want to know whether the RHE would consider a book review before writing it, you may email the associate editor responsible for book reviews with the citation for the book.
Reviewers should have scholarly expertise in the higher education research area they are reviewing.
Graduate students are welcome to co-author book reviews, but with faculty or seasoned research professionals as first authors.
Please email the review to the associate editor in charge of book reviews (Timothy Reese Cain, [email protected] ), who will work through necessary revisions with you if your submission is accepted for publishing.
In general, follow the APA Publication Manual, 7th edition.
Provide a brief but clear description and summary of the contents so that the reader has a good idea of the scope and organization of the book. This is especially important when reviewing anthologies that include multiple sections with multiple authors.
Provide an evaluation of the book, both positive and negative points. What has been done well? Not so well? For example the following are some questions that you can address (not exclusively), as appropriate:
What are the important contributions that this book makes?
What contributions could have been made, but were not made?
What arguments or claims were problematic, weak, etc.?
How is the book related to, how does it supplement, or how does it complicate current work on the topic?
To which audience(s) will this book be most helpful?
How well has the author achieved their stated goals?
Use quotations efficiently to provide a flavor of the writing style and/or statements that are particularly helpful in illustrating the author(s) points.
If you cite any other published work, please provide a complete reference.
Please include a brief biographical statement immediately after your name, usually title and institution. Follow the same format for co authored reviews. The first author is the contact author.
Please follow this example for the headnote of the book(s) you are reviewing: Stefan M. Bradley. Upending the Ivory Tower: Civil Rights, Black Power, and the Ivy League. New York: New York University Press, 2018. 465 pp. $35. ISBN 97814798739999.
Our preferred length is 2,000–2,500 words in order for authors to provide a complete, analytical, review. Reviews of shorter books may not need to be of that length.
The term “conflict of interest” means any financial or other interest which conflicts with the work of the individual because it (1) could significantly impair the individual’s objectivity or (2) could create an unfair advantage for any person or organization. We recommend all book reviewers read and adhere to the ASHE Conflict of Interest Policy before submitting any and all work. Please refer to the policy at ashe.ws/ashe_coi
NOTE: If the Editor has sent a book to an author for review, but the author is unable to complete the review within a reasonable timeframe, we would appreciate the return of the book as soon as possible; thanks for your understanding.
Please send book review copies to the contact above. Review copies received by the Johns Hopkins University Press office will be discarded.
Penny A. Pasque, The Ohio State University
Thomas F. Nelson Laird, Indiana University-Bloomington
Associate Editors
Angela Boatman, Boston College
Timothy Reese Cain (including Book Reviews), University of Georgia
Milagros Castillo-Montoya, University of Connecticut
Tania D. Mitchell, University of Minnesota
Chrystal George Mwangi George Mason University
Federick Ngo, University of Nevada, Las Vegas
Managing Editors
Stephanie Nguyen, Indiana University Bloomington
Monica Quezada Barrera, The Ohio State University
Editorial Board
Sonja Ardoin, Clemson University
Peter Riley Bahr, University of Michigan
Vicki Baker, Albion College
Allison BrckaLorenz, Indiana University Bloomington
Nolan L. Cabrera, The University of Arizona
Brendan Cantwell, Michigan State University
Rozana Carducci, Elon University
Deborah Faye Carter, Claremont Graduate University
Ashley Clayton, Louisiana State University
Regina Deil-Amen, The University of Arizona
Jennifer A. Delaney, University of Illinois Urbana Champaign
Erin E. Doran, Iowa State University
Antonio Duran, Arizona State University
Michelle M. Espino, University of Maryland
Claudia García-Louis, University of Texas, San Antonio
Deryl Hatch-Tocaimaza, University of Nebraska-Lincoln
Nicholas Hillman, University of Wisconsin-Madison
Cindy Ann Kilgo, Indiana University-Bloomington
Judy Marquez Kiyama, University of Arizona
Román Liera, Montclair State University
Angela Locks, California State University, Long Beach
Demetri L. Morgan, Loyola University Chicago
Rebecca Natow, Hofstra University
Z Nicolazzo, The University of Arizona
Elizabeth Niehaus, University of Nebraska-Lincoln
Robert T. Palmer, Howard University
Rosemary Perez, University of Michigan
OiYan Poon, Spencer Foundation
Kelly Rosinger, The Pennsylvania State University
Vanessa Sansone, The University of Texas at San Antonio
Tricia Seifert, Montana State University
Barrett Taylor, University of North Texas
Annemarie Vaccaro, University of Rhode Island
Xueli Wang, University of Wisconsin-Madison
Stephanie Waterman, University of Toronto
Rachelle Winkle-Wagner, University of Wisconsin-Madison
Association for the Study of Higher Education Board of Directors
The Review of Higher Education is the journal of Association for the Study Higher Education (ASHE) and follows the ASHE Bylaws and Statement on Diversity.
ASHE Board of Directors
Abstracting & Indexing Databases
- Current Contents
- Web of Science
- Dietrich's Index Philosophicus
- IBZ - Internationale Bibliographie der Geistes- und Sozialwissenschaftlichen Zeitschriftenliteratur
- Internationale Bibliographie der Rezensionen Geistes- und Sozialwissenschaftlicher Literatur
- Academic Search Alumni Edition, 9/1/2003-
- Academic Search Complete, 9/1/2003-
- Academic Search Elite, 9/1/2003-
- Academic Search Premier, 9/1/2003-
- Current Abstracts, 9/1/2003-
- Education Research Complete, 3/1/1997-
- Education Research Index, Sep.2003-
- Education Source, 3/1/1997-
- Educational Administration Abstracts, 3/1/1991-
- ERIC (Education Resources Information Center), 1977-
- MLA International Bibliography (Modern Language Association)
- Poetry & Short Story Reference Center, 3/1/1997-
- PsycINFO, 2001-, dropped
- Russian Academy of Sciences Bibliographies
- TOC Premier (Table of Contents), 9/1/2003-
- Scopus, 1996-
- Gale Academic OneFile
- Gale OneFile: Educator's Reference Complete, 12/2001-
- Higher Education Abstracts (Online)
- ArticleFirst, vol.15, no.3, 1992-vol.35, no.2, 2011
- Electronic Collections Online, vol.20, no.1, 1996-vol.35, no.2, 2011
- Periodical Abstracts, v.26, n.4, 2003-v.33, n.3, 2010
- PsycFIRST, vol.24, no.3, 2001-vol.33, no.1, 2009
- Personal Alert (E-mail)
- Education Collection, 7/1/2003-
- Education Database, 7/1/2003-
- Health Research Premium Collection, 7/1/2003-
- Hospital Premium Collection, 7/1/2003-
- Periodicals Index Online, 1/1/1981-7/1/2000
- Professional ProQuest Central, 07/01/2003-
- ProQuest 5000, 07/01/2003-
- ProQuest 5000 International, 07/01/2003-
- ProQuest Central, 07/01/2003-
- Psychology Database, 7/1/2003-
- Research Library, 07/01/2003-
- Social Science Premium Collection, 07/01/2003-
- Educational Research Abstracts Online
- Research into Higher Education Abstracts (Online)
- Studies on Women and Gender Abstracts (Online)
Abstracting & Indexing Sources
- Contents Pages in Education (Ceased) (Print)
- Family Index (Ceased) (Print)
- Psychological Abstracts (Ceased) (Print)
Source: Ulrichsweb Global Serials Directory.
1.8 (2022) 3.2 (Five-Year Impact Factor) 0.00195 (Eigenfactor™ Score) Rank in Category (by Journal Impact Factor): 185 of 269 journals, in “Education & Educational Research”
© Clarivate Analytics 2023
Published quarterly
Readers include: Scholars, academic leaders, administrators, public policy makers involved in higher education, and all members of the Association for the Study of Higher Education (ASHE)
Print circulation: 761
Print Advertising Rates
Full Page: (4.75 x 7.5") - $450.00
Half Page: (4.75 x 3.5") - $338.00
2 Page Spread - $675.00
Print Advertising Deadlines
September Issue – July 15
December Issue – October 15
March Issue – January 15
June Issue – April 15
Online Advertising Rates (per month)
Promotion (400x200 pixels) – $338.00
Online Advertising Deadline
Online advertising reservations are placed on a month-to-month basis.
All online ads are due on the 20th of the month prior to the reservation.
General Advertising Info
For more information on advertising or to place an ad, please visit the Advertising page.
eTOC (Electronic Table of Contents) alerts can be delivered to your inbox when this or any Hopkins Press journal is published via your ProjectMUSE MyMUSE account. Visit the eTOC instructions page for detailed instructions on setting up your MyMUSE account and alerts.
Also of Interest
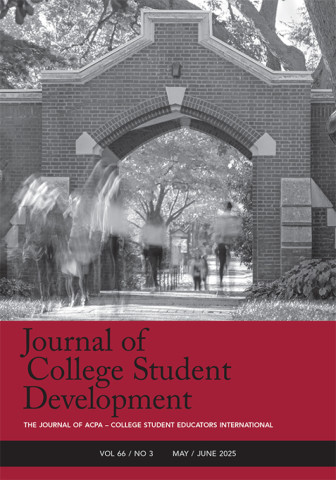
Vasti Torres, Indiana University
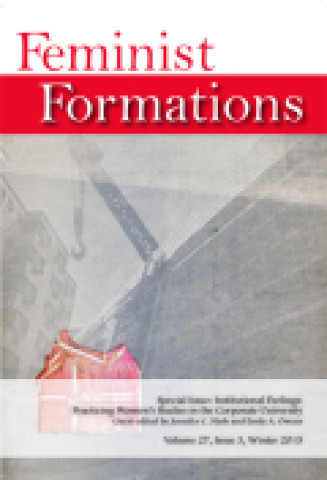
Patti Duncan, Oregon State University
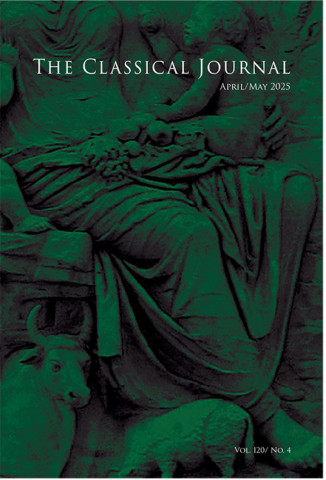
Georgia L. Irby, College of William & Mary
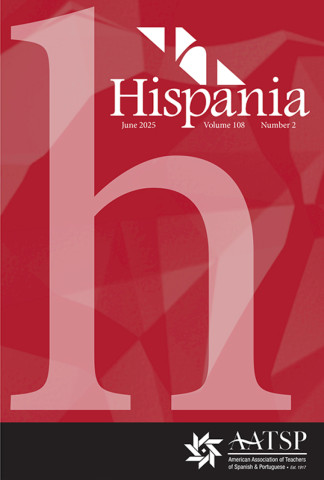
Benjamin Fraser, The University of Arizona
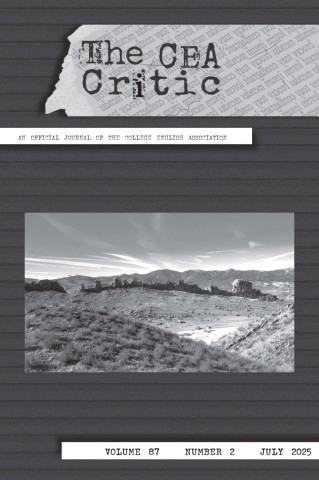
Jeraldine Kraver, University of Northern Colorado; Peter Kratzke, University of Colorado, Boulder
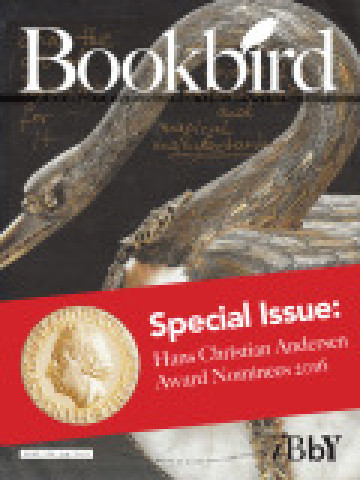
Chrysogonus Siddha Malilang, Malmö University, Sweden
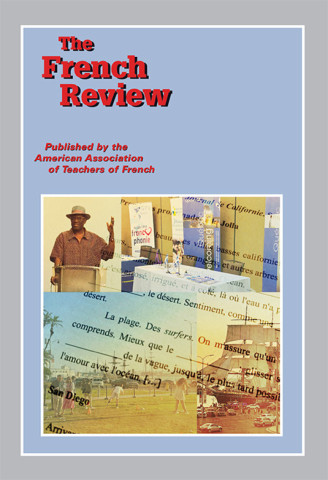
Carine Bourget, University of Arizona
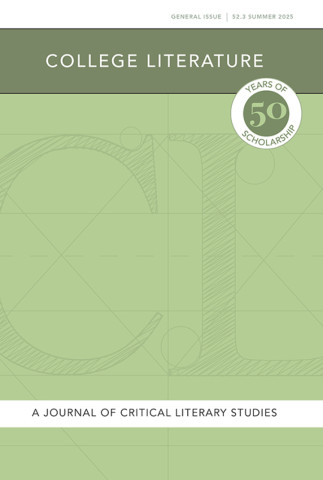
Megan Corbin, West Chester University
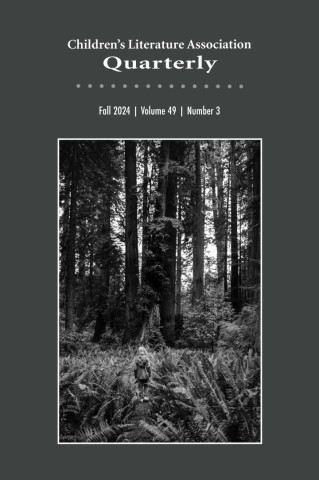
Joseph Michael Sommers, Central Michigan University
Hopkins Press Journals
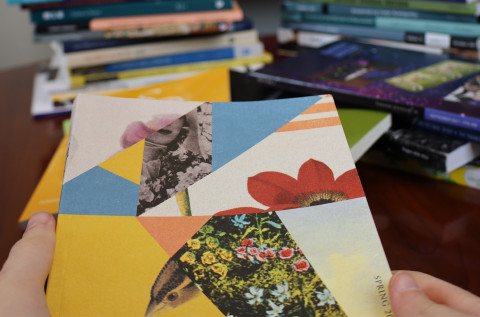
Click through the PLOS taxonomy to find articles in your field.
For more information about PLOS Subject Areas, click here .
Loading metrics
Open Access
Peer-reviewed
Research Article
Student engagement and wellbeing over time at a higher education institution
Roles Conceptualization, Formal analysis, Investigation, Methodology, Visualization, Writing – original draft
* E-mail: [email protected]
Affiliation Computer Science, University of Exeter, Exeter, United Kingdom
Roles Data curation, Methodology, Software
Affiliation School of Psychology, University of Exeter, Exeter, United Kingdom

Roles Conceptualization, Data curation, Investigation, Methodology, Writing – review & editing
Roles Conceptualization, Data curation, Formal analysis, Funding acquisition, Methodology, Supervision, Writing – review & editing
Roles Conceptualization, Funding acquisition, Methodology, Supervision, Writing – original draft, Writing – review & editing
- Chris A. Boulton,
- Emily Hughes,
- Carmel Kent,
- Joanne R. Smith,
- Hywel T. P. Williams
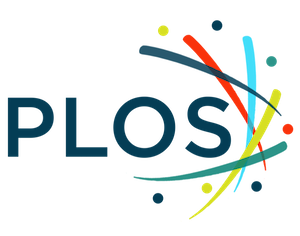
- Published: November 27, 2019
- https://doi.org/10.1371/journal.pone.0225770
- Reader Comments
Student engagement is an important factor for learning outcomes in higher education. Engagement with learning at campus-based higher education institutions is difficult to quantify due to the variety of forms that engagement might take (e.g. lecture attendance, self-study, usage of online/digital systems). Meanwhile, there are increasing concerns about student wellbeing within higher education, but the relationship between engagement and wellbeing is not well understood. Here we analyse results from a longitudinal survey of undergraduate students at a campus-based university in the UK, aiming to understand how engagement and wellbeing vary dynamically during an academic term. The survey included multiple dimensions of student engagement and wellbeing, with a deliberate focus on self-report measures to capture students’ subjective experience. The results show a wide range of engagement with different systems and study activities, giving a broad view of student learning behaviour over time. Engagement and wellbeing vary during the term, with clear behavioural changes caused by assessments. Results indicate a positive interaction between engagement and happiness, with an unexpected negative relationship between engagement and academic outcomes. This study provides important insights into subjective aspects of the student experience and provides a contrast to the increasing focus on analysing educational processes using digital records.
Citation: Boulton CA, Hughes E, Kent C, Smith JR, Williams HTP (2019) Student engagement and wellbeing over time at a higher education institution. PLoS ONE 14(11): e0225770. https://doi.org/10.1371/journal.pone.0225770
Editor: Marina Della Giusta, University of Reading, UNITED KINGDOM
Received: April 23, 2019; Accepted: November 12, 2019; Published: November 27, 2019
Copyright: © 2019 Boulton et al. This is an open access article distributed under the terms of the Creative Commons Attribution License , which permits unrestricted use, distribution, and reproduction in any medium, provided the original author and source are credited.
Data Availability: Survey response data can be found at 10.5281/zenodo.3480070.
Funding: This research was supported by the Effective Learning Analytics project at the University of Exeter. The funder had no role in study design, data collection and analysis, decision to publish, or preparation of the manuscript.
Competing interests: The authors have declared that no competing interests exist.
Introduction
Engagement with learning is believed to be an important factor in student success in higher education. Engagement has been defined in different ways in the literature [ 1 ], but is considered here to refer to the active commitment and purposeful effort expended by students towards all aspects of their learning, including both formal and informal activities [ 2 ]. Student engagement has been shown to be related to success in both online learning [ 3 – 5 ] and more traditional campus-based higher education settings [ 6 – 8 ]. However, engagement can be difficult to measure. In most studies of online-only education (e.g. [ 9 – 13 ]), student engagement is measured from the interactions a student has within a virtual learning environment (VLE). This may be a reasonable approach for digital-only contexts where a large proportion of learning activities occur through this channel. In contrast, in a traditional, face-to-face learning, university environment, VLE usage only captures one dimension of student learning activity and full engagement with learning is much harder to measure. The numerous and varied interactions students have with their learning programmes, including lectures, seminars, peer group discussions and ad hoc interactions with teaching staff, as well as other aspects of campus life such as participation in sports and student societies, are harder to record, requiring innovative methods for their capture [ 14 , 15 ].
Exploration of the relationship between student engagement and success raises the important question of how “success” is defined. Most obviously, success relates to academic performance, such as final grades (e.g. [ 6 – 8 , 16 ]), but success is also often discussed in terms of retention and completion of a course of learning (e.g. [ 7 , 10 , 13 , 17 – 19 ]). It is important to consider that students may have different motivations for attending university, including, for example, social or sporting aims alongside conventional academic goals. Thus, in seeking to link engagement to success, there is value in adopting a more holistic view of student motivations and appropriate measures of outcomes. Furthermore, it is important to note that engagement and success, however measured, are dynamic and should be expected to vary within and between individuals over the duration of academic study.
There is increasing interest in learning analytics [ 20 – 25 ], which may use either static attributes of students (e.g. demographics, socioeconomic indicators, previous attainment) or dynamic attributes based on digital traces of learning behaviour to understand many aspects of the student experience, including student engagement. Traditionally, such studies have primarily made use of “found” data from institutional databases and “by-product” data from digital learning platforms. This kind of data, which is not collected for the purpose of pedagogical research, has limitations. The records that are collected institutionally tend to relate to either the administration of higher education (e.g., demographic data, recruitment/retention statistics) or to the core components of academic performance (e.g., grades, progression, completion). Data collected as the by-product of student learning activities on digital platforms such as VLEs (e.g. [ 8 – 10 ] only offers a partial view of a complex whole. For example, previous work that examined the relationship between academic performance and engagement at a traditional University found that VLE usage alone is a relatively poor predictor of academic performance in this context [ 8 ], while another study showed that VLE usage was a useful predictor of outcomes for online learning but not significant for face-to-face learning [ 9 ].
Dispositional learning analytics (see [ 26 ]), on the other hand, seeks to combine digital trace data (e.g., those generated by engagement in online learning activities) with learner data (e.g., dispositions, attitudes, and values assessed via self-report surveys). By doing so, recent research has found that learning dispositions (e.g., motivation, emotion, self-regulation) strongly and dynamically influence engagement and academic performance over time (e.g., [ 27 – 29 ]). In addition, this research suggests that the predictive value added by consideration of learner data might be time-dependent: learner data seems to play a critical role up until the point that feedback from assessment or online activities becomes available. This raises the possibility that whether incorporating learner dispositions into learning analytics models is useful depends on learning context (i.e., online only versus campus-based institutions).
Another limitation of learning analytics based solely on digital traces, is that these sources often cannot capture subjective aspects of student life, such as wellbeing and satisfaction, which are rarely routinely measured. Relationships between student engagement and wellbeing, or between wellbeing and success, have consequently been less well studied for higher education than that between engagement and success (but see [ 30 , 31 ]). One project that has moved beyond by-product data and used deliberate collection of digital records to measure student behaviour and wellbeing is the StudentLife study at Dartmouth College in the USA [ 14 ]. This project supplied mobile phones to student participants in a term-long study that attempted to capture a multi-dimensional and longitudinal view of student behaviour. Findings used aspects of student life that had previously been inaccessible to researchers, including social interactions and physical activity patterns, to predict academic performance [ 16 ] and also to diagnose wellbeing issues [ 14 , 32 ]. While the StudentLife study showed that deliberate data collection using digital methods can access important aspects of the subjective student experience, it does not address the difficulty of doing so using the kinds of by-product digital records and institutional data that are routinely collected and used as input into learning analytics.
The importance of student wellbeing for academic outcomes, and the relationships between wellbeing and engagement, remain open research questions for higher education. Wellbeing is a loosely defined concept that may include a number of different dimensions, including satisfaction, positive affect (e.g. enjoyment, gratitude, contentment) and negative affect (e.g. anger, sadness, worry) [ 33 , 34 ]. Many studies have explored the relationship between wellbeing and academic performance, commonly finding a positive association, e.g. in US college undergraduates [ 35 , 36 ] and among high school students [ 37 ]. The relationship between engagement and wellbeing is less well studied in higher education, but a positive association has been found in other working environments [ 34 ]. A recent government report on student mental health and wellbeing in UK universities found increasing incidence of mental illness, mental distress and low wellbeing [ 38 ]. The same study found that these negative wellbeing factors had a substantial harmful impact on student performance and course completion; by extension, students with positive wellbeing are likely to perform better and complete their studies. Another study by the UK Higher Education Academy focused on methods for promoting wellbeing in higher education, as well as identifying several pedagogical benefits [ 39 ].
Here we report on a longitudinal survey of student learning behaviours at a traditional campus-based university in the United Kingdom. Our survey was designed to capture multiple dimensions of student engagement and wellbeing over time, deliberately using self-report to look beyond digital traces and institutional records. An initial questionnaire included questions to characterise individual students on different dimensions including learning style and motivations for study. Subsequent waves captured student learning behaviours and engagement with a wide variety of learning systems (both offline and online) and activities, as well as their subjective feelings of satisfaction and wellbeing. The survey ran in 10 waves spanning a teaching semester, vacation and exam period, allowing observation of changes over time.
This study aims to complement the growing body of work that uses digital trace data to measure engagement, with a more subjective offline approach that captures a fuller representation of the student experience. Our research goals are to understand how engagement and wellbeing vary over time, as well as to determine a multidimensional view of student learning behaviours and patterns. Addressing these questions will make an important contribution to the academic study of student engagement and will help to identify other learning dispositions (e.g., engagement) that might be of value to combine with digital trace data in learning analytic models. Findings may also offer instrumental benefit by helping to guide institutional decision-making around interventions and student support.
The cohort for the survey consisted of 1st year and 2nd year undergraduate students at a research-intensive campus-based university in the United Kingdom. Students were invited to participate via emails containing a link to survey registration. In addition, recruitment booths were set up at the university’s main campus and researchers approached students to invite them to participate. Students were incentivised by entry into a prize draw to win gift vouchers for a well-known online retailer, with 10 prizes available in each wave. There were 10 waves in all. To incentivise continued participation, there was an additional final prize draw with larger prizes available to students who had completed 80% of surveys. Every participant explicitly gave their consent to their data being analysed for research purposes.
The survey ran from February to June 2017. Of the 10 waves, Waves 1–7 were released weekly during the Spring term, followed by a break for the Easter vacation period. Waves 8–10 were released fortnightly during the Summer term, which at this institution was mostly taken up with revision and examinations. Responses were received asynchronously, so although the survey was released in waves, we analyse the data over a continuous time interval spanning 19 weeks.
Our longitudinal survey consisted of a series of questions that students completed in every wave. To measure engagement with learning, we asked respondents to report their participation in each of 17 different learning activities (see Table 1 ), measured as the number of days in the past 7 days they had performed that activity. These activities were selected to represent the variety of online and offline activities, as well as social and academic activities, available to students at the university. To give context, we also asked respondents to report whether they had an assessment due in the past 7 days.
- PPT PowerPoint slide
- PNG larger image
- TIFF original image
https://doi.org/10.1371/journal.pone.0225770.t001
Effort over the preceding week was assessed with two items assessed on a 5-point Likert scale (specifically, “How engaged were you with your studies?”; “How much effort did you put into your studies?”, 1 = not at all, 5 = very much). The mean response from each student was used to form a reliable scale (Pearson’s r = 0.78, p < .001). Well-being over the last week was assessed with four items that asked about happiness in general (e.g., “How happy did you feel about your life in general?”) and in relation to their programme of study (e.g., “How well do you feel you are doing in your course?”, 1 = not at all, 5 = very much). Responses were averaged to form a reliable scale (Cronbach’s α = 0.69).
In addition to the longitudinal survey questions, we also asked further questions in Wave 1 to determine their self-reported learning engagement style and motivation for attending university.
Engagement with learning was assessed with 10 items adapted from the Student Engagement in Schools Questionnaire (SESQ; [ 40 ]). Participants indicated the extent of their agreement with the statements on a 5-point Likert scale (1 = strongly disagree, 5 = strongly agree). Principal components analysis with varimax rotation extracted two factors, accounting for 53% of the variance. The first factor was characterised by the items assessing cognitive engagement (e.g., “When I study, I try to understand the material better by relating it to things I already know”), and items were averaged to form a cognitive engagement scale (α = 0.73). The second factor was characterised by the items assessing behavioural engagement (e.g., “In my modules, I work as hard as I can”), and items were averaged to form a behavioural engagement scale (α = 0.75).
Participants indicated their agreement with six different reasons for attending university (1 = not at all, 5 = very much). Principal components analysis with varimax rotation extracted two factors, accounting for 57% of the variance. The first factor was characterised by the items assessing social motivations (e.g., “To socialise with friends”), and items were averaged to form a social motivations scale (α = 0.62). The second factor was characterised by the items assessing academic motivations (e.g., “To get good grades”), and items were averaged to form an academic motivations scale (α = 0.48). The original survey is shown in Supplementary Information ( S1 File ).
The survey and following analysis were undertaken in accordance with the guidelines of the British Psychological Society. All participants provided informed consent prior to participation and were free to withdraw at any time without penalty. The survey and analysis received ethical approval from the University of Exeter Psychology Ethics Committee prior to commencement of data collection.
Our analysis is based on both static and dynamic variables from the survey responses for each student. Static variables include the motivation and engagement style measurements that were calculated from Wave 1. An additional static variable was also used to measure student academic performance across the term in which the survey was conducted, using grade data from the university database; for this metric, a student grade variable was calculated as their credit-weighted average grade from all the modules they took during the term in which the survey was conducted. Dynamic variables include the engagement and wellbeing measurements recorded in every wave. To allow comparison between static variables and dynamic variables, we take the mean value for the dynamic variable (e.g., the mean number of days per week that a student participated in a learning activity, or their mean effort scale score). Correlations between variables are measured using the Pearson correlation coefficient and measure correlations between both the static and dynamic variables. In both cases, all data is used in the correlation measurement, such that there is one record per student who answered in Wave 1, and all the responses are used to calculate the correlation between the dynamic variables.
Dynamic variables were used to analyse trends in behaviour over time, such as trends in engagement and wellbeing. To allow analysis of trends across the whole cohort, we created time series for engagement and wellbeing variables using a moving average across all responses with a 7-day window size. To ensure robustness, we made sure there were at least 10 responses in each window for which a mean was calculated. Since counts were lower during vacation and examination periods, we restricted our trend analysis to term-time only. Trends in these time series were calculated using the Kendall rank correlation coefficient, which counts the proportion of concordant pairs (both x i >x j and y i >y j or x i <x j and y i <y j ). Using time as one of the variables, this gives a measure of tendency in the range [– 1 , 1 ], with a score of -1 if the time series is always decreasing, a score of +1 if the time series is always increasing, and a score of 0 if there is no overall trend.
Our analysis involved looking for differences in behaviour between sub-populations within our respondent cohort (e.g. splitting the cohort into those who did or did not have an assessment due each week). We present differences in the mean values between the two distributions and then use a Mann-Whitney U-test to determine if the distributions are significantly different. We use these non-parametric tests since the distributions of values are typically non-normal and vary in shape between different variables. We also have a small sample size once the distributions have been split. However, we still present the difference in mean values, rather than the difference in median values, since the discrete nature of our data (e.g., integer values in range 0–7, which for some variables have an inter-quartile range of 0 to 1) means that medians are sometimes too coarse-grained to show differences even where the distributions are significantly different.
Survey response
Overall, we had responses from 175 unique students, 174 of which answered the Wave 1 survey including questions to determine engagement style and motivations. We had 1050 responses overall, giving an average of exactly 6 responses per student.
Fig 1 shows the number of responses received over time during the 19-week period that the survey was active. There is an expected decline in the number of responses over time as participants lose interest or for other reasons drop out of the cohort. Despite this, we still have a reasonably steady and high response rate during the Spring term (left of the grey shaded area). There is a significant drop off in survey participation during the Easter break (grey shaded area), before the response rate recovers during the Summer term, although not to the levels seen previously (right of great shaded area). The Summer term in our survey is dominated by revision and exams, which suggests we might see different student behaviour.
Grey shaded region refers to the Easter break between semesters. Spring Term is to the left of the grey region, Summer Term to the right. Vertical dotted lines indicate the weeks in which a survey email was sent and a responder lottery was held to incentivise participation. Note that students could answer a survey wave in the following week, hence a lower amount of first-week responses is observed when compared to the 174 students that answered the first wave of the survey.
https://doi.org/10.1371/journal.pone.0225770.g001
Table 2 shows some demographics of our survey respondents (n = 175), compared to the entire student population (n = 15646). We find that our survey respondents are slightly biased towards being female and in their first year of study. The students who took the survey also have slightly higher marks than the student population. The number of students in the Life and Environmental Sciences college is greater than expected, with less representation of students from the Social Sciences and International Studies college and the Medical School. The low numbers from the Medical School reflect the fact that this School is based on a different campus to where physical recruitment of participants occurred.
https://doi.org/10.1371/journal.pone.0225770.t002
Respondent characteristics
The Wave 1 survey included one-time questions intended to allow construction of engagement style and motivation scores for each individual student (see Methods ). The distributions of these scores are shown in Fig 2 . Due to the nature of these measurements, and the fact that they are only measured once, they make up part of our ‘static’ data and can be thought of as measuring a student’s underlying dispositions. They suggest that generally students reported slightly higher levels of behavioural engagement than cognitive engagement, although there was a bigger spread in behavioural engagement scores. Most of the students who responded to our survey reported higher academic motivation than social motivation for attending university.
Students were asked a one-time set of questions to determine their engagement type and motivations (see Methods ) and as such this is a static measurement. Dotted lines show the minimum and maximum scores, solid lines show the interquartile range, and points show the medians.
https://doi.org/10.1371/journal.pone.0225770.g002
Relationships among student characteristics, average engagement and performance
Fig 3 shows the distributions of values from the longitudinal survey questions used to measure dynamic variables related to engagement with different learning activities and levels of student wellbeing. The plots show all responses from all students aggregated together, with the various learning activities ordered according to their mean usage level. The distributions suggest that activities that are most directly associated with learning (e.g. using the VLE, using the info app, using the Internet for learning, attending a teaching session) are used much more frequently than those that are not (e.g. using sports facilities, talking to a year representative, using SU facilities). This is consistent with the finding above that most students in the sample had stronger academic than social motivations for attending university. Distributions of scores on the “effort” and “happy” scales derived from the wellbeing questions asked each week (see Methods ) show that both metrics have a broad absolute range but a relatively narrow interquartile range. These metrics cannot be usefully compared.
The underlying survey questions were asked in all waves and as such these are dynamic variables. Plot shows minimum and maximum scores (dotted lines), the interquartile range (solid lines) and median values (points). For this analysis all student responses were pooled.
https://doi.org/10.1371/journal.pone.0225770.g003
Next, we related the various static variables to each other and to the mean values for the various dynamic variables for each student in our cohort. Table 3 shows (Spearman’s) correlations between static variables across the cohort for: engagement style, motivation, grades, wellbeing, and engagement levels. Statistical significance is indicated in Table 3 ; henceforth we only discuss correlations with statistical significance at level p <0.05, unless stated explicitly. For the dynamic variables, we use the mean reported level across all responses for each student. Grades are analysed using the average credit-weighted module grade from the term in which the survey was carried out (see Methods ).
https://doi.org/10.1371/journal.pone.0225770.t003
We find relatively strong positive correlation (ρ = 0.36) between levels of the two engagement styles (behavioural and cognitive). Behavioural engagement is correlated positively with academic motivation for attending university (ρ = 0.15) but correlated negatively with social motivation (ρ = -0.22). Behavioural engagement is very strongly positively correlated with effort (ρ = 0.55) and positively correlated with grades (ρ = 0.24). Cognitive engagement, on the other hand, is not correlated with grades (ρ = 0.02) but is positively correlated with happiness (ρ = 0.30). Cognitive engagement is also often positively correlated with participation in the various learning activities, with several positive correlations: seeing a lecturer (ρ = 0.32); going to the library (ρ = 0.28); using social media for learning (ρ = 0.18); and using the Internet for learning (ρ = 0.24). Cognitive engagement is negatively correlated with viewing lecture recordings (ρ = -0.16). Interestingly, behavioural engagement was typically uncorrelated with participation in learning activities except negatively with attending scheduled teaching sessions (ρ = -0.16); and viewing lecture recordings (ρ = -0.17).
The two types of motivation (academic and social) are not significantly correlated with each other (ρ = 0.14), but social motivation is correlated negatively with grades (ρ = -0.25). Academic motivation is significantly correlated with wellbeing scales for both effort (ρ = 0.28) and happiness (ρ = 0.29), whereas social motivation is not. Regarding participation in learning activities, the pattern of correlations makes intuitive sense. Academic motivation is weakly positively correlated with two academic activities: info app usage (ρ = 0.22); and VLE usage (ρ = 0.23). Social motivation is positively correlated with one core academic activity, attending a teaching session (ρ = 0.26), but is also positively correlated with several activities that are less directly academic and have a social aspect: working with friends (ρ = 0.19), using sports facilities (ρ = 0.46), using retail facilities (ρ = 0.23), using catering facilities (ρ = 0.23), using social media for learning (ρ = 0.21), and attending clubs or societies (ρ = 0.36).
It is interesting to note that the only significant correlations between student academic performance (measured by average grades) and levels of participation in learning activities are negative. Perhaps less surprising are negative correlations between grades and participation in “social” activities: using retail facilities (ρ = -0.22); and using catering facilities (ρ = -0.32). It is hard to explain the negative correlations between grades and attending a teaching session (ρ = -0.17). We return to this topic in the Discussion.
The wellbeing scales (effort and happiness) are positively correlated with each other (ρ = 0.30): students who put in more effort report greater happiness. Effort is positively correlated with several non-compulsory learning activities: using the VLE (ρ = 0.27); going to the library (ρ = 0.31); using career services (ρ = 0.30); using social media for learning (ρ = 0.36); and using the Internet for learning (ρ = 0.50). Effort is also positively correlated with using retail facilities (ρ = 0.27), perhaps suggesting more time spent on campus. Happiness is uncorrelated with core learning activities but is positively correlated with more social activities: using SU facilities (ρ = 0.28); and going to clubs or societies (ρ = 0.36).
Table 3 shows many positive correlations between levels of participation in various learning activities. Without listing all the pairwise relationships here, we find that 50% of activity pairs are significantly positively correlated, with no activity pairs negatively correlated. This suggests that students who engage more with learning do so in a holistic manner, with raised participation across a variety of learning activities.
Temporal trends and correlations
Next, we consider trends or changes in behaviour during the Spring term ( Fig 4 ), looking first at time series of reported participation levels for each learning activity (see Methods ). Since we use a moving average to give robust values, and since survey response rate falls outside term time, we restrict our analysis to the period within the Spring term (Waves 1–7, prior to the grey shaded area in Fig 1 ). We use a moving average equal to one week (7 days) and when doing this, the lowest number of responses in any window is 17 (on the last day of term), suggesting the plotted values are reliable. Apart from the final two days of term, all the windows have 38 or more responses within them. Trends are calculated using Kendall’s tau correlation coefficient (see Methods ). For ease of viewing, we have split the learning activities into ‘Online’ learning activities ( Fig 4a ), ‘Offline’ learning activities ( Fig 4b ) and ‘Other’ activities ( Fig 4c ). We also plot time series for wellbeing variables ( Fig 4d ).
Time series are calculated as a moving average using data from all students. Trends and significance are calculated using Kendall’s tau correlation coefficient.
https://doi.org/10.1371/journal.pone.0225770.g004
There is a general downward trend in participation with learning activities over the Spring term. Of the ‘Online’ systems ( Fig 4a ), all of them have a significantly downward trend as the term goes on: using the VLE (τ = -0.72); using the info app (τ = -0.65); using the Internet for learning (τ = -0.85); using social media for learning (τ = -0.67); and accessing lecture recordings (τ = -0.47). Three of the ‘Offline’ systems also decrease over the term ( Fig 4b ): attending teaching sessions (τ = -0.91); accessing the library (τ = -0.20); viewing past exams (τ = -0.56). Since teaching activities are scheduled with a roughly uniform density throughout the term, the downward trend in engagement with learning activities is notable. A similar trend is seen for many of the ‘Other’ activities ( Fig 4c ): going to clubs or societies (τ = -0.70); using the sports facilities (τ = -0.32); using retail facilities (τ = -0.83); using catering facilities (τ = -0.63); talking to a year rep (τ = -0.49); using SU facilities (τ = -0.68). There are no learning activities that show an increase in participation over the term.
Looking at trends in the wellbeing variables over the term, we see that effort increases slightly but not significantly (τ = 0.10). However, happiness increases significantly (τ = 0.36), suggesting that students report greater happiness as the term progresses. We cannot say whether this increase in self-reported happiness is related to the concurrent decrease in engagement, though it is tempting to speculate.
Table 4 shows correlations between the dynamic variables measuring participation in learning activities and wellbeing. This analysis shows whether there are temporal associations between levels of participation in different activities (e.g., if a student does more of one activity, does this correspond to more engagement in other activities). The striking observation in this analysis is that nearly all pairwise relationships between dynamic variables show significant positive correlations, with a small number of exceptions. This indicates a pattern whereby student learning activity varies holistically; students may be more or less active, but when they are active, they are active across a wide range of activities and behaviours. Again, the two wellbeing scales are correlated with each other (ρ = 0.37). Overall, 83% of the pairwise relationships between learning activities show a positive correlation over time (compared to 50% for the averaged data shown in Table 3 ). We find two significant negative correlations: between viewing past exam papers and visiting a lecturer (ρ = -0.08) and attending a teaching session (ρ = -0.13). This is most likely because Table 4 uses time-resolved information and is affected by the switch between attendance at scheduled teaching sessions during the Spring term and using past exams to revise for upcoming exams during the Summer term.
https://doi.org/10.1371/journal.pone.0225770.t004
Impact of assessments on engagement and wellbeing
To determine the impact of assessments (e.g., coursework, class tests, final exams, etc.) on student engagement and wellbeing, we split our dataset into “assessment week” responses (those responses where the student answered that there was an assessment due in the 7-day reporting period) and “non-assessment week” responses (where no assessments were due). Note that “assessment weeks” are temporally heterogeneous and specific to the individual; that is, the assessment/non-assessment weeks are not temporally correlated across the cohort. This rules out effects from globally correlated hidden variables such as, for example, campus wide events, external media stories, etc. For each set of responses, we create distributions for each dynamic variable and then measure the differences between these distributions using the difference in means and Mann-Whitney U-tests (see Methods ). Results are shown in Fig 5 . The bars in Fig 5 plot the difference in mean values for each distribution, with positive differences referring to increased participation in assessment weeks. Bar colours indicate whether the difference between the distributions is statistically significant according to the Mann-Whitney U-test.
Bars show the difference in mean values for reported score distributions for (upper panel) participation in each learning activity measured in days, or (lower panel) levels of effort and happiness on scale 1–5. Positive values indicate an increase in assessment weeks. Bar colours indicate statistical significance for the difference between distributions calculated from a Mann-Whitney U-test (blue—significant positive difference, red—significant negative difference, white—not significant).
https://doi.org/10.1371/journal.pone.0225770.g005
Fig 5 (upper panel) shows the mean difference for assessment weeks and non-assessment weeks in the reported number of days of participation in each learning activity. We find increased participation in all learning activities during assessment weeks, except using career services, which had significantly less usage when an assessment was due. Of the activities with increased participation, 9 of the 15 increases were significant. Interestingly, increased participation in assessment weeks extends across a mix of activity types; for example, there is greater attendance at clubs and societies when assessments are due. Overall, the analysis suggests there is higher engagement with most learning activities when assessments are due.
We also look for differences in the wellbeing variables of effort and happiness between assessment weeks and non-assessment weeks ( Fig 5 , lower panel). We find that there is a significant increase in the effort levels students report when an assessment is due. There is also, perhaps surprisingly, a slight increase in happiness, although this is not significant.
Relationships between behaviour and wellbeing
To explore the relationship between engagement with learning activities and reported wellbeing, we again split our dataset, this time into sets of responses where the student reported high/low levels of effort and high/low levels of happiness for that week. Since both variables are measured on an integer scale from 1 (low) to 5 (high), we use a threshold of 3 to split the cohort in each case, creating datasets for those who responded below 3 and those who reported 3 or above. This gives comparator sets for students who report “high effort” or “low effort” and students who report “happy” or “not happy”. Results are shown in Fig 6 .
Bars show the difference in mean scores (in days) from the distributions of participation levels for different learning activities. Positive values indicate higher participation by the (left) high effort and right (happy) students. Bar colour indicates significant differences between the distributions according to a Mann-Whitney U-test (blue—significant positive difference, red—significant negative difference, white—not significant).
https://doi.org/10.1371/journal.pone.0225770.g006
As expected, we find that 16 of the 17 learning activities show higher mean participation levels by high effort students, and for 10 of these the difference between the distributions is significant ( Fig 6 , left panel). Happy students have higher mean participation levels in all activities that students who are not happy ( Fig 6 , right panel). However, these differences are generally smaller than those for high vs low effort groups. When comparing the left and right panels in Fig 6 , there is a significant increase in going to the Sports Park and using catering facilities for happier students, whereas rates of viewing past exams are only significantly increased for high effort students.
In planning this research, we expected to find different patterns of engagement among students, such as individuals showing more engagement with certain systems and less with others. This might be driven by students’ personal preferences (e.g., [ 27 , 28 ]) or by the teaching activities prescribed and/or preferred by different disciplines and programmes (see e.g. [ 8 , 41 ]). Instead we find that students who are engaged with learning tend to be engaged with all learning activities and systems; engagement appears to be a holistic phenomenon (Tables 3 and 4 ). The only exception to this pattern is a negative correlation between attending scheduled teaching sessions and viewing past exam papers. This might be explained by the separation (for most students) of learning and revision, with exam papers used for revision after scheduled teaching has finished. The strong correlation between all forms of engagement with learning has possible instrumental value for the design of systems to monitor student engagement, since it suggests that engagement could be effectively tracked using only a subset of engagement metrics as indicators. Monitoring of engagement might be used to identify anomalies or changes in behaviour of individuals, for example, to assist tutors in providing support and pastoral care. Indeed, the predictive analytics project at Nottingham Trent University (NTU Student Dashboard), which calculates engagement scores based on five online resources (VLE access, library usage, attendance, assignment submissions, and card swipes), has identified a positive relationship between student engagement and both progression and attainment. Moreover, this information, when communicated to students and staff, has been used to provide more targeted support to students from pastoral tutors (see [ 42 ]).
A feature of our survey design is the ability to measure variables at a campus-based university that would otherwise be difficult to access. Of the 17 learning activities recorded by our survey, only four could be tracked digitally with current methods (VLE, info app, past exam views and recorded lecture viewing), with the rest not routinely measured. Furthermore, this study provides temporally resolved data on student wellbeing, giving the opportunity to explore relationships between engagement and wellbeing.
Engagement and wellbeing are shown in this study to be positively related. Looking longitudinally across the survey ( Table 4 ), we find 13 forms of engagement were positively (and significantly) correlated with at least one of the wellbeing variables, either effort or happiness. Reasonably, one could suggest a possible feedback loop where increasing engagement increases academic performance, which in turn increases wellbeing (happiness and grades are correlated; Table 4 ), which then increases engagement. Alternatively, students with greater background levels of wellbeing may be more likely to engage with learning (see also [ 30 , 31 ]). This study cannot separate these potential mechanisms, since it only shows correlation and cannot assign causality.
The responses to our survey show a broad sample of student engagement at the university where the study was based. The survey was widely advertised and contains responses from students across all disciplines. However, in common with most survey studies, it relies on voluntary participation and we had no control over who would participate (see also [ 43 ]). This may introduce bias into our results. For example, we find that the students who responded scored much higher on academic motivation than on social motivation ( Fig 2 ), but this may be an artefact of self-selection bias in the sample of survey respondents, such that academically motivated students who are engaged with learning were more likely to participate (see also [ 43 , 44 ]). Indeed, analysis of the demographic data of respondents suggests that certain disciplines were over-sampled. This might limit the generalizability of our findings to the whole cohort, given that there are likely to be disciplinary differences in the extent to which students are expected to engage with various learning systems (see [ 8 ]). Furthermore, since this study was based at a single university in the UK, it may not represent students at other universities in the UK or worldwide. We encourage other researchers to repeat our study at other institutions in order to consolidate our findings. We make our survey design available in the Supplementary Information ( S1 File ) to facilitate this.
Another caveat to our results is that differences between student workloads associated with different learning activities are not considered. In previous work, we have shown that the amount of observed VLE usage differs between different disciplines [ 8 ], explained by the differing requirements of different disciplines, programmes and modules. For example, a humanities student is likely to have a balance of learning activities that differs from an engineering student, with resulting variation in the time they spend on the VLE. In addition, the number of scheduled lectures and other contact hours will differ between disciplines, with students taking STEM subjects typically having more contact hours than those taking arts or humanities subjects which require more self-study. It is possible that these differences might affect some of our findings. For example, the correlation between attending scheduled teaching sessions and student happiness might be influenced by the fraction of sessions attended, rather than the absolute number; a student who attends 100% of 4 scheduled sessions might be happier than a student who attends 50% of 8 scheduled sessions, even though the number of attended sessions remains the same. This kind of difference might mask or confound some relationships, so it is possible that a study sample stratified on discipline or programme would give a more nuanced picture of the relationships between engagement and wellbeing. With a larger sample size, we would have been able to create disciplinary subsets of students to explore this aspect, but our sample size did not permit this here.
One interesting dimension of student engagement that we are yet to explore within our survey is how well students predict their own usage of various learning systems; that is, do they accurately report their usage of digital tools? Results given here are based on student self-report rather than documented usage of different systems. In general, students might mis-report their behaviour either by mistake or deliberately, for whatever reason. If self-reported data in the current survey are inaccurate, it might raise the interesting question of whether some students systematically under- or over-report their levels of engagement with learning, and whether students who misreport perform better or worse academically (see [ 45 , 46 ]). We will return to this question in future work. If self-report and documented data (where available) do not agree, it raises the question of which sources show a more accurate picture of student behaviour and which are more important in relation to student wellbeing.
We can only speculate why there is an observed decrease in engagement during the academic term. It could be because students like to get ahead at the start of term and work harder or engage more to do this. The larger drop off in engagement at the end of term may be due to students having assessments that are not due until after the break and therefore not needing to work as much as they do during the middle of term. The rise in reported effort during the term (although not statistically significant) is interesting in relation to the decrease in reported engagement. The observed increase in happiness towards the end of term seems to be robust but is hard to explain; we speculate that perhaps students become happier as they start to receive assessment outcomes, or maybe they are simply looking forward to the end of term. This may be at odds with the correlations between engagement and wellbeing discussed previously. However, we believe that the correlations are picking out individual student behaviours, whereas these trends reflect the whole population.
Our research identified strong differences in behaviour between students who have an assessment due and those who do not. This gives us confidence that our survey can identify meaningful results, despite the limited sample size. We also find strong differences in behaviour between those students who feel engaged as well as happy. Finding that students who are happy are engaging more is an important result for our understanding of student wellbeing. Coupled with mechanisms to routinely measure engagement, it could assist tutors to identify students who are suffering with poor wellbeing and might benefit from intervention or greater support.
Supporting information
S1 file. questions used in survey completed by participants..
The original survey was completed using survey software Qualtrics.
https://doi.org/10.1371/journal.pone.0225770.s001
Acknowledgments
This project aims to make effect use of data to help students reach their full academic potential while studying at the University of Exeter.
- View Article
- Google Scholar
- 12. Na KS, Tasir Z, editors. Identifying at-risk students in online learning by analysing learning behaviour: A systematic review. 2017 IEEE Conference on Big Data and Analytics (ICBDA); 2017 16–17 Nov. 2017.
- 14. Wang R, Chen F, Chen Z, Li T, Harari G, Tignor S, et al. StudentLife: assessing mental health, academic performance and behavioral trends of college students using smartphones. Proceedings of the 2014 ACM International Joint Conference on Pervasive and Ubiquitous Computing; Seattle, Washington. 2632054: ACM; 2014. p. 3–14.
- 15. Kent C, Boulton CA, Williams HTP. Towards Measurement of the Relationship between Student Engagement and Learning Outcomes at a Bricks-and-Mortar University. Sixth Multimodal Learning Analytics (MMLA) Workshop and the Second Cross-LAK Workshop co-located with 7th International Learning Analytics and Knowledge Conference (LAK 2017); Vancouver, Canada2017.
- 16. Wang R, Harari G, Hao P, Zhou X, Campbell AT. SmartGPA: how smartphones can assess and predict academic performance of college students. Proceedings of the 2015 ACM International Joint Conference on Pervasive and Ubiquitous Computing; Osaka, Japan. 2804251: ACM; 2015. p. 295–306.
- 19. Dekker GW, Pechenizkiy M, Vleeshouwers JM. Predicting Students Drop Out: A Case Study. 2nd Educational Data Mining; Cordoba, Spain: ERIC; 2009.
- 23. Sclater N, Peasgood A, Mullan J. Learning Analytics in Higher Education: A review of UK and international practice. Joint Information of Systems Committee (JISC). CC by 4.0 Licence: UK; 2016.
- 24. Sclater N, Mullan J. Learning analytics and student success: Assessing the evidence. Joint Information of Systems Committee (JISC). CC by 4.0 License: UK; 2016.
- 26. Shum SB, Crick RD, editors. Learning dispositions and transferable competencies: pedagogy, modelling and learning analytics. Proceedings of the 2nd international conference on learning analytics and knowledge; 2012: ACM.
- PubMed/NCBI
- 38. Thorley C. Not By Degrees: Not by degrees: Improving student mental health in the UK’s universities. IPPR; 2017.
- 40. Lam SF, Jimerson SR. Exploring student engagement in schools internationally: Consultation paper. Chicago, IL: International School Psychologist Association; 2008.
- 42. Lawther S, Foster E, Mutton J, Kerrigan M. Can the Use of Learning Analytics Encourage Positive Student Behaviours? In: Janes G, Nutt D, Taylor P, editors. Student Behaviour and Positive Learning Cultures: SEDA; 2016. p. 13–21.
Advertisement
First-year university students’ academic success: the importance of academic adjustment
- Open access
- Published: 04 November 2017
- Volume 33 , pages 749–767, ( 2018 )
Cite this article
You have full access to this open access article
- Els C. M. van Rooij 1 ,
- Ellen P. W. A. Jansen 1 &
- Wim J. C. M. van de Grift 1
53k Accesses
102 Citations
11 Altmetric
Explore all metrics
A Correction to this article was published on 08 January 2018
This article has been updated
Considering the pivotal role of academic adjustment for student success, it is important to gain insight into how several motivational and behavioural factors affect academic adjustment and the extent to which academic adjustment influences student success. This empirical study investigated how intrinsic motivation, academic self-efficacy, self-regulated study behaviour and satisfaction with the chosen degree programme influenced academic adjustment in university and how these variables and adjustment affected three important indicators of student success: grade point average (GPA), attained number of credits (ECTS) and intention to persist. The sample consisted of 243 first-year university students in the Netherlands. Structural equation modelling showed that academic adjustment was influenced by intrinsic motivation, self-regulated study behaviour and degree programme satisfaction, which together explained 72% of the variance in adjustment. Motivational and behavioural variables did not influence GPA and credits directly but through academic adjustment. Furthermore, only satisfaction with the degree programme predicted intention to persist. These results point to the importance of academic adjustment in predicting university GPA and credits and the pivotal role of satisfaction with the degree programme in predicting intention to persist. Universities could integrate the development of self-regulated study skills—the biggest contributor to academic adjustment—in the first-year programme. Moreover, looking at the importance of students’ satisfaction with the programme, communication and collaboration between secondary schools and universities should be enhanced in order to help students to choose a university degree programme that matches their abilities, interests and values.
Similar content being viewed by others
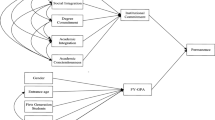
Effects of pre-college variables and first-year engineering students’ experiences on academic achievement and retention: a structural model
Rafael García-Ros, Francisco Pérez-González, … José M. Tomás
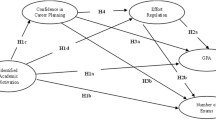
Motivation is not enough: how career planning and effort regulation predict academic achievement
Marcella Floris, Giulia Paganin, … Greta Mazzetti
Student Motivation and ‘Dropout’ Rates in Brazil
Avoid common mistakes on your manuscript.
Introduction
Drop-out rates in the first year of university are high worldwide. In the Netherlands, where this study was conducted, 33% of first-year university students do not continue to the second year of the programme they initially started (Inspectie van het Onderwijs 2016 ). A smooth transition from secondary school to university increases the chances of student success, in terms of achievement and persistence (Lowe and Cook 2003 ; Rienties et al. 2012 ). Therefore, it is important for university educators to understand how to improve this transition for students. An effective measure of how well a student has transitioned to university is the level of academic adjustment to this new environment. In this study, we draw on traditional theories of student success [e.g. Tinto ( 1993 ) and Astin ( 1999 )] and earlier research on academic adjustment (Baker and Siryk 1989 ) and conceptualise academic adjustment as the ability to have successful interactions with the new academic environment and to cope with its academic demands. In other words, it revolves around the fit between the student and the university environment (Ramsay et al. 2007 ). To make the concept of academic adjustment more explicit, we follow Baker and Siryk’s ( 1984 ) categorisation of four aspects of academic adjustment, which are motivation to learn and having clear academic goals, applying oneself to academic work, exerting effort to meet academic demands and being satisfied with the academic environment. Previous research consistently showed that academic adjustment influences academic achievement (Bailey and Phillips 2016 ; Rienties et al. 2012 ).
This study has two goals. First, we aim to determine which motivational and behavioural variables measured in the first year of university affect students’ academic adjustment and success, i.e. grade point average (GPA), number of attained study credits [European Credit Transfer and Accumulation System (ECTS)] and intention to persist after 3 months of study. An important question here is which variables influence student success, either directly or indirectly through adjustment. Second, we investigate the magnitude of the influence of academic adjustment on the three outcome variables. Most research only uses one outcome measure, even though the specific outcome measure chosen may affect the results. Robbins et al. ( 2004 ) showed, for example, that the impact of predictive factors differs for achievement and persistence. Moreover, these outcome measures in themselves differ. A student’s GPA reflects how well a student performs, whereas the number of credits merely shows whether a student is passing courses. Persistence is yet another distinct measure of success, in that students with a high GPA and many credits may drop out, whereas students with low GPAs or few credits may choose to persist. The differences in measures of success makes it important to include all of them and investigate whether academic adjustment affects them differently.
The motivational and behavioural input variables on which we focus appear in prior literature as important correlates of student success and academic adjustment in university and will be discussed below.
Theoretical framework
Academic adjustment influencing student success.
Research on student success in higher education has a rich history. The traditional theories of Tinto ( 1993 ) and Astin ( 1999 ) focus on the interaction between the student and the institution, where Tinto’s theory of student attrition includes academic, social and institutional integration and goal commitment and Astin’s student development theory revolves around student involvement, which he defines as the energy that a student devotes to the academic experience (Astin 1999 ). The common ground lies therein that a student enters higher education with certain personal characteristics, e.g. personality, motivation, study skills, which change and may even be challenged in interaction with the new educational environment. Successful interaction with this new environment, such as having positive interactions with lecturers and fellow students and being able to handle the increased complexity and quantity of the learning content, then determines whether or not a student is satisfied with the first-year experience and whether he or she obtains good grades, passes his or her courses and persists to the second year (Astin 1999 ; Pascarella and Terenzini 2005 ; Sevinç and Gizir 2014 ). Successful interaction between a first-year student and the academic characteristics and demands of the university environment can be summarised by the construct of academic adjustment. Prior literature consistently showed the pivotal role of academic adjustment in predicting achievement (Aspelmeier et al. 2012 ; Rienties et al. 2012 ; Wintre et al. 2011 ) and persistence (Kennedy et al. 2000 ; Kuh et al. 2006 ) in higher education. Some studies even reported that the effects of background variables on achievement were indirect, with adjustment as a mediator (Kamphorst et al. 2012 ; Petersen et al. 2009 ). Moreover, academic adjustment explained variance in achievement beyond secondary school GPA (McKenzie and Schweitzer 2001 ). Lowe and Cook ( 2003 ) found that 20 to 30% of university students experienced considerable difficulty adjusting to higher education, leading a significant number to drop out or underperform. These factors make academic adjustment an important concept when investigating student success.
Correlates of student success and academic adjustment
Because of the aforementioned importance of academic adjustment as a correlate of first-year success, it is useful to know which variables influence adjustment. Robbins et al. ( 2004 ) emphasised the importance of combining motivational factors and study skills when explaining academic achievement, and Kennedy et al. ( 2000 ) warned against using too narrow a range of variables. We followed this line of thought to explain adjustment and included different motivational and behavioural factors in our model to obtain a more integrative view of adjustment and achievement.
Motivational correlates of success and adjustment
Intrinsic motivation.
Meta-analyses on academic achievement showed a consistent relationship between motivation and achievement (Richardson et al. 2012 ; Robbins et al. 2004 ). Other studies investigated the link between motivational factors and adjustment. For example, Lynch ( 2006 ) and Petersen et al. ( 2009 ) reported a positive link between intrinsic motivation and adjustment. Baker and Siryk ( 1984 ) showed that achievement motivation was correlated with academic adjustment. Moreover, Baker ( 2004 ) showed that lack of motivation is related to poorer adjustment to university. Following these findings and the expectation that students who are intrinsically motivated to study a certain topic will find it easier to adjust to an educational environment where they get the opportunity to study this topic, we expected intrinsic motivation to have a direct and an indirect effect on achievement through adjustment.
Academic self-efficacy
According to Robbins et al.’s ( 2004 ) meta-analysis, academic self-efficacy is the strongest non-cognitive correlate of GPA. Self-efficacy is a person’s perception of the ability to perform adequately in a given situation (Bandura 1997 ). Academic self-efficacy in the university context thus refers to a student’s confidence that he or she can perform adequately in the university environment. Besides being an important correlate of achievement, academic self-efficacy relates to effort and perseverance in learning, self-regulation, less stress in demanding situations and better adjustment to new learning situations (Chemers et al. 2001 ). McKenzie and Schweitzer ( 2001 ) found that the prediction of GPA improved by 12% when academic integration and self-efficacy were added to a model with university entry score as a predictor. De Clercq et al. ( 2013 ), who used an inclusive approach that took into account several predictors, also reported that self-efficacy was one of the most powerful predictors of GPA at the end of the first year in university. When investigating persistence as an outcome measure, Kennedy et al. ( 2000 ) found no differences in self-efficacy between students who continued their studies after 1 year and those who did not. Still, there is some evidence that self-efficacy could affect persistence, because Willcoxson et al. ( 2011 ) found that the opposite of academic self-efficacy, lack of academic confidence, caused students to give up their studies. Examining the relationship between academic self-efficacy and adjustment, several studies showed that self-efficacy, or the comparable concept of academic self-confidence, positively affected adjustment (Chemers et al. 2001 ; Martin et al. 1999 ). This finding can be explained by Bandura’s ( 1997 ) self-efficacy theory, which states that people high in efficacy show more persistence in the face of challenges. The transition from secondary education to university is such a challenge. Moreover, Aspelmeier et al. ( 2012 ), who found that self-esteem and internal locus of control had a positive effect on first-year students’ academic adjustment, suggested that academic self-efficacy is an important factor to consider in future research on adjustment. We thus hypothesised that academic self-efficacy influences achievement both directly and via adjustment.
- Degree programme satisfaction
Although models explaining university success included degree programme satisfaction less often than motivation and self-efficacy, it may be crucial for predicting persistence (Suhre et al. 2007 ; Yorke and Longden 2007 ), especially in the Netherlands and many other European countries such as Germany and Belgium, where students entering university immediately start in a specific major. Not being satisfied with the programme is one of the most important determinants of dropping out (De Buck 2009 ; Wartenbergh and Van den Broek 2008 ). Moreover, satisfaction relates to achievement; Suhre et al. ( 2007 ) showed that students who were more satisfied obtained more credits. We know of no research that investigates the relationship between degree programme satisfaction and academic adjustment, but we expect that students who are satisfied can better cope with academic demands. In the first few weeks of the programme, where students immediately start with several courses specific to the degree programme they chose, students already get a good view of what the programme entails and they can judge the extent to which the programme meets their expectations and the extent to which they are satisfied with the programme. Adjustment to the whole first-year experience, which includes among other things adjusting to a new way of learning, to more independency, and to a faster learning pace, however, is a process that takes longer. The rationale here is that when students are satisfied with the programme they chose, the process of adjusting academically may be easier. On the contrary, students who are having doubts regarding whether this specific degree programme matches their interests may be preoccupied with the dilemma of whether or not to proceed with this programme, which may also have a negative effect on their process of adjusting to university. Their doubts about the programme may even transfer into doubts about belonging in university altogether. Thus, we expected degree programme satisfaction to be related to academic adjustment, achievement and persistence.
Behavioural correlate of success and adjustment
Self-regulated study behaviour.
Motivation is an important but insufficient condition to perform well in university. As Robbins et al. ( 2004 ) concluded, it is important to include study skills, along with psychosocial variables, in models predicting achievement. Self-regulation is a specifically important skill in the university environment, where students must regulate their own study behaviour. Moreover, students who live independently may have many personal and social demands that compete with academic demands. At this point, behaviour regulation becomes crucial. According to Pintrich ( 2004 ), behaviour regulation is part of self-regulation, referring to individual attempts to control one’s own behaviour. Important behaviour regulation activities in the academic environment—or self-regulated study behaviour—are effort regulation, time management and environment management. Effort regulation refers to the ability to control the allocation and intensity of effort, with the goal of doing well in a course; time management involves activities such as making schedules for studying and allocating time for different activities; and environment management pertains to finding the optimal physical conditions for a learning environment, such as avoiding distractors (e.g. social media [Jacobsen and Forste 2011 ]) or people (Pintrich 2004 ). Effort, time and environment regulation are among the study skills often connected to achievement (Burlison et al. 2009 ; Lynch 2006 ). A meta-analysis of the Motivated Strategies for Learning Questionnaire (MSLQ) even showed that of all learning strategies included in the MSLQ, effort regulation and time and study environment management had the highest observed validities for predicting GPA (Credé and Phillips 2011 ). In addition, Wintre et al. ( 2011 ) reported that first-year students who maintained their secondary school GPA in the first year of higher education had better time management skills than those whose GPA would drop and Hurtado et al. ( 2007 ) found that students’ time management skills was a significant predictor of academic adjustment. In contrast with university lecturers’ expectations, first-year students often do not possess the self-regulatory skills that the university environment demands, because they are accustomed to the structured and supervised situation in secondary education (Cook and Leckey 1999 ). This lack of regulatory skill could cause adjustment problems in university; Abott-Chapman et al. ( 1992 ) showed that students with insufficient study skills were at risk of academic adjustment problems. We therefore expected self-regulated study behaviour to influence adjustment and achievement.
Previous achievement as a predictor of success and adjustment
Much research indicated that past achievement is a predictor of university achievement (Bowles et al. 2014 ; McKenzie and Schweitzer 2001 ; Richardson et al. 2012 ; Robbins et al. 2004 ; Suhre et al. 2007 ). However, it is equivocal whether past achievement (i.e. secondary school GPA) also influences academic adjustment at university. It seems reasonable to expect that students with higher scores in secondary education will be better equipped to cope with academic demands and thus adjust to university more easily (Baker and Siryk 1989 ; Kaczmarek et al. 1990 ). However, Wouters et al. ( 2011 ) found no relationship between achievement in secondary education and academic adjustment in higher education, so we questioned whether to expect a pathway from secondary school GPA to adjustment in university.
The conceptual model
Figure 1 presents a schematic representation of the conceptual model of motivational and behavioural factors influencing academic adjustment and the three measures of student success. We expected intrinsic motivation, academic self-efficacy, self-regulated study behaviour and satisfaction with degree programme choice to relate to academic adjustment, as well as to the measures of student success, GPA, credits and intention to persist.
Conceptual model of motivational and behavioural factors impacting academic adjustment and student success outcomes
Educational context
There are two characteristics of the Dutch secondary and higher education system that are relevant in this study. First, in the Netherlands, as in many other European countries, the secondary school system is differentiated: from grade 7 onwards, students attend a level of secondary education that matches their capabilities. Pre-university education is the highest of the three existing levels and graduating from pre-university grants access to a degree programme at a research university. For some programmes, additional requirements are at play, such as specific subject uptake in pre-university education, but in general, the application process is not as intense as in for example the USA. Second, also quite common in Europe, students entering university choose the degree programme they major in before they start.
The total sample was a convenience sample that consisted of 243 first-year university students from different research-intensive universities in the Netherlands, who completed the questionnaire approximately 3 months after the start of their programme. Many different degree programmes were represented in the sample, but a large majority of the students were pursuing a social sciences degree (77%), e.g. Spatial Sciences, Sociology and Law, and a smaller number of students were in the humanities (4%) and natural sciences (19%). Women were overrepresented in this study (60%, as opposed to 53% in the population of first-year university students in the Netherlands; Sociaal en Cultureel Planbureau [SCP] 2014 ). Most students started university after graduating from pre-university education (82%); 14% came from higher vocational education, and the other 4% had switched from another programme at university. Students’ average age was 19.13 years (SD 1.57), ranging from 17 to 28 years, hence the sample can be seen as a sample of traditional students. This makes the sample representative, as in the Netherlands 80% of all pre-university students directly continue to university education (Centraal Bureau voor de Statistiek [CBS] 2016 ). Furthermore, 24% of students can be classified as first-generation university students, students of whom neither of their parents had attended higher education. Among all first-year university students in the Netherlands, the percentage of first-generation students is 33% (Van den Broek et al. 2014 ).
Student success outcomes
Students indicated the average grade they obtained for the courses they had taken in the first quarter of the study year. In the Dutch education system, grades range from 1 to 10, and a 5.5 or higher is required to pass. The students’ grades in this sample ranged from 4 to 9, with an average grade of 6.90 (SD = 0.98).
In addition to their GPA, students reported the number of credits they had obtained in the first quarter of the year.
Intention to persist
We measured students’ intention to persist with one question: ‘Do you intend to finish this degree programme (i.e. the 3-year university bachelor’s program)?’
- Academic adjustment
We measured students’ academic adjustment with the academic adjustment subscale of the Student Adaptation to College Questionnaire (SACQ) by Baker and Siryk ( 1984 ). This subscale consists of 24 questions that involve coping with the academic demands of the university experience. In line with Baker and Siryk’s internal consistency measures for the scale, which range from α = .82 to .87, and with more recent studies who used the academic adjustment subscale of the SACQ [e.g. Jones et al. ( 2015 ) and Rodríguez-González et al. ( 2012 )], the alpha of this scale in our study was good: .85.
Motivational factors
We used a measure of intrinsic motivation specifically focused on the university environment, i.e. a desire to gain academic knowledge in one’s field of interest and to conduct research because one finds it inherently interesting or enjoyable (based on Ryan and Deci 2000 ). The 13 items were based on the Scientific Attitude Inventory II (SAI II; Moore and Foy 1997 ).
To measure academic self-efficacy for the university environment, we used 16 of the 33 items of the College Academic Self-Efficacy Scale (CASES; Owen and Froman 1988 ). Previous research has shown that these items were sufficient to obtain a reliable measure of academic self-efficacy (Van Rooij et al. 2017 ). The 16 items were typical behaviours that students need to demonstrate at university, such as being able to understand difficult passages in textbooks and attending class consistently even in a dull course.
We measured the extent to which the university students were satisfied with the degree programme they had chosen by averaging the score on two items: ‘I am satisfied with the programme I chose’ and ‘Looking back, I wish I had chosen a different degree programme’ (reverse coded).
Behavioural factor: self-regulated study behaviour
The self-regulated study behaviour scale consisted of the effort regulation and the time and study environment management subscales of Part B of the Motivated Strategies for Learning Questionnaire (MSLQ) (Pintrich et al. 1993 ). The effort regulation scale had four items, and the time and study environment management subscale consisted of eight items. Credé and Phillips’s ( 2011 ) meta-analyses of the MSLQ showed that the scales of time and study environment management and effort regulation were so strongly correlated that they may assess the same construct. Correspondingly, the internal consistency of the complete self-regulated study behaviour scale was good (α = .87). Table 1 summarises measurement characteristics.
Previous achievement
We determined academic achievement in secondary school with an item that asked for average secondary school diploma grade. Scores ranged from 6 (satisfactory) to 9 (very good), with an average grade of 7.07 (SD = .72).
The Ethics Committee of the university had given approval of the study. All participants received an e-mail invitation to participate in the study. Seventy-one percent of participants received this e-mail, composed by the researchers, via a coordinator of their programme who was interested in having the first-year students of his or her programme participate in the study and the other 29% received the invitation directly from the researchers. This latter group participated in a previous study a year earlier, when they were still in secondary school, and had given consent to be contacted again for a follow-up study. The e-mail invitation explained the research purpose and asked the student to complete an online questionnaire; participation was voluntary. Incentives were allotted among participants who had completed the questionnaire. The response rate was 52%.
Due to 11 students having missing values on multiple variables, we based our structural equation model tests on 232 first-year university students. We used Mplus, Version 7, to perform the analyses. First, we inspected the descriptive statistics and correlational matrix to conclude whether certain variables were significantly and substantially related to each other. Second, using these results, we decided which variables to include in the first model, based on the conceptual model with both direct and indirect links from the motivational and behavioural factors to the student success outcomes. Third, we tested this first model and evaluated its goodness of fit based on agreed-upon criteria (e.g. Kline 2005 ). Fourth, if the model fit was insufficient, we adapted the model, according to the reported modification indices and theoretical considerations, after which we tested the new model.
Descriptive results
Table 2 presents the descriptive statistics of all factors used in the model. The mean scores on all factors, as well as on secondary school and university GPA, were relatively high and the variances, especially those of academic adjustment and academic self-efficacy, were quite low. There were no significant differences in factor and outcome means between first-generation students and continuing generation students. We also did not find any significant differences between students who came from pre-university, from higher vocational education and from another university degree programme.
Table 3 shows the correlations between all factors used in the model. The motivational and behavioural variables had higher correlations with academic adjustment than with the three measures of student success, with the exception of degree programme satisfaction, which correlated equally strongly with intention to persist and academic adjustment.
Path analysis
As can be deduced from Fig. 1 , our conceptual model consisted of many links, between each of the motivational and behavioural variables and academic adjustment and each of the student success outcomes. However, because correlations lower than .25 would likely have resulted in insignificant links in the model, we excluded the hypothesised pathways between intrinsic motivation and GPA, ECT and intention to persist, and those between academic self-efficacy and ECT and intention to persist. We then tested this model in Mplus. Goodness-of-fit statistics showed that this model had good fit ( Χ 2 (11) = 14.91, p = .07, Χ 2 /df = 1.36, RMSEA = .04 [confidence interval = .00–.08], CFI = .99, TLI = .98, SRMR = .06). However, many of the pathways were insignificant: secondary school GPA and academic self-efficacy were not significantly related to academic adjustment; self-regulated study behaviour and satisfaction with the choice of degree programme were not significantly related to GPA; secondary school GPA, self-regulated study behaviour and satisfaction with the programme were not significantly related to credits; and academic adjustment was not significantly related to intention to persist.
These results implied that many links from the motivational and behavioural variables affected university success outcomes not directly but through adjustment. Therefore, we tested a second model in which we removed all insignificant pathways from the first model. We present this model in Fig. 2 . This model achieved good fit: Χ 2 (19) = 20.55, p = .36, Χ 2 /df = 1.08, RMSEA = .02 [CI = .00–.06], CFI = .99, TLI = .99, SRMR = .06. All hypothesised links were significant, except the link from academic self-efficacy to university GPA (β = .13 (SE = .06), p = .06). Moreover, university GPA and intention to persist were not significantly related to each other (β = .12 (SE = .07), p = .12) and neither were self-regulated study behaviour and intrinsic motivation (β = .14 (SE = .08), p = .10). The model showed that the motivational and behavioural variables affected two university success outcomes, GPA and credits, through academic adjustment. Self-regulated study behaviour (β = .61), intrinsic motivation (β = .14) and satisfaction with the choice of degree programme (β = .36) had impacts on academic adjustment. Academic self-efficacy was not significantly related to university GPA, but did correlate highly with self-regulated study behaviour (β = .63), thereby indirectly influencing adjustment and subsequent achievement. In total, 72% of the variance in academic adjustment was explained by the aforementioned variables. Academic adjustment influenced both GPA (β = .38) and the number of attained credits (β = .50) in university. Secondary school GPA only had impact on university GPA (β = .28). Respectively, 29 and 25% of the variance in GPA and credits were explained. The intention to persist was largely influenced by students’ satisfaction with the degree programme they had chosen (β = .60).
Fitted model of motivational and behavioural factors impacting academic adjustment and student success outcomes. Note: dotted lines represent insignificant pathways
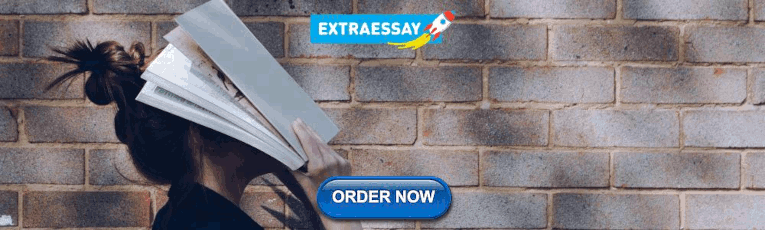
Conclusions and discussion of the main findings
We investigated which motivational and behavioural variables measured in the beginning of the first year of university affected students’ academic adjustment and success (GPA, credits and intention to persist) and the influence of academic adjustment in predicting these three outcomes. Students who were more intrinsically motivated to gain academic knowledge and to do research, who could effectively regulate their study behaviour and who were more satisfied with their chosen degree programme had better academic adjustment (i.e. had more successful interactions with the academic experience and were better able to cope with the academic demands of the university environment). Furthermore, students with better academic adjustment and who had a higher GPA in secondary education had a higher university GPA. In addition, better academic adjustment led to more credits in the first half of the first semester of university. However, whether these students actually intended to persist was a different question: it depended less on the level of academic adjustment and secondary school GPA than on their satisfaction with their chosen degree programme. Our results thus confirmed the importance of academic adjustment as a measure of how successfully the student transitioned from secondary school to higher education in predicting study results in the first year of university. In addition, academic adjustment was substantially more important in predicting the number of attained credits and university GPA than secondary school GPA. Thus, it is again confirmed that first-year students’ experiences, more specifically how they interact with the learning environment, have more impact on their success than their previous results (Kuh et al. 2006 ). Motivational and behavioural factors did not influence GPA and credits directly but only through academic adjustment. Thus, effectively regulating study behaviour (e.g. maintaining study schedules, turning off social media when studying), being intrinsically motivated to gain academic knowledge and being satisfied with chosen degree programme did not necessarily mean students would achieve high grades and obtain all credits. It did, however, increase their chances of being well-adjusted (i.e. able to cope with the academic demands of the new learning environment). Subsequently, this academic adjustment led to a better GPA and more credits. Studies that tested the effects of these motivational and behavioural factors as having only direct effects on achievement may underemphasise the pivotal role of adjustment.
Another important finding was that self-regulated study behaviour exerted the largest influence on academic adjustment of all measured variables. This means that in order to experience a smooth transition, it is very important that students are capable of regulating their study behaviour and less important that they are intrinsically motivated and satisfied with the degree programme. The high degree of self-regulation that university demands is one of the largest differences with secondary school; therefore, students who are good self-regulators will adjust more easily. Another possible explanation is that behavioural factors are more important in explaining adjustment than motivational ones. In this regard, Astin’s claim about student involvement can be applied to academic adjustment as well: “It is not so much what the individual thinks or feels, but what the individual does, how he or she behaves” (Astin 1999 , p. 519). Furthermore, the differences in magnitude of influence on adjustment could be attributable to smaller differences between students in intrinsic motivation than in self-regulated study behaviour.
A surprising result was that academic self-efficacy, widely accepted as a very important correlate of student success (e.g. Robbins et al. 2004 ), did not affect any of the student success outcomes of our study, nor did it affect academic adjustment. Its only role in the model was as an important correlate of self-regulated study behaviour, consistent with previous research indicating high correlations between self-regulation and self-efficacy (Bouffard-Bouchard et al. 1991 ; Fenollar et al. 2007 ). Again, a possible explanation is that behavioural factors are more important in influencing adjustment than motivational factors such as self-efficacy and that the differences between students in self-efficacy were rather small. Two other explanations are provided by De Clercq et al. ( 2017 ), who found a relationship between self-efficacy and achievement that was less strong than expected in their person-centred study on first-year achievement. As they explained, global self-efficacy, such as the general measure of academic self-efficacy that we used in this study, is not as good a predictor as domain-specific self-efficacy, e.g. self-efficacy in a specific subject or a specific skill, and self-efficacy beliefs are not good predictors of achievement in new learning contexts, such as the first year at university (De Clercq et al. 2017 ). However, we did find that students who were more confident in their academic skills tended to regulate their effort and manage their study time and environment more effectively than students lower in self-efficacy. Because self-regulated study behaviour is very important in university—where instructors provide little control or structure and more autonomy and responsibility is demanded of students (Pintrich 2004 )—self-efficacy is still an important factor in the transition from secondary to university education due to its influence on behaviour regulation.
Contrary to our expectation, only one variable influenced students’ intention to persist, namely, the level of satisfaction with their chosen degree programme. Although this satisfaction also influenced academic adjustment, academic adjustment did not have any influence on intention to persist. Thus, whether a student planned to continue his or her studies after the first year was not related to how well the student could cope with the demands of the academic environment in general, but rather how well he or she fitted within the specific study programme. The outcome variable, intention to persist, thus measured a different entity than the outcome variables GPA and credits, which did not directly relate to a specific degree programme. If we had measured intention to persist as a students’ intention to stay in university altogether or drop out completely, academic adjustment may have played a role.
Implications
The results indicated the crucial role of academic adjustment in predicting achievement in university. Self-regulated study behaviour, satisfaction with degree programme choice and, to a lesser extent, intrinsic motivation influenced students’ academic adjustment. All these factors can be influenced, both before and after the transition. For example, secondary education could emphasise the development of self-regulated study behaviour. Jansen and Suhre ( 2010 ) showed that study skills preparation in secondary school, regarding time management and learning skills, positively influenced university students’ study behaviour. We also found a connection between academic self-efficacy and self-regulated study behaviour (e.g. Bouffard-Bouchard et al. 1991 ). Schunk and Ermter ( 2000 ) stated that when either of these aspects is low or lacking, the other aspect cannot fully develop, because they influence each other reciprocally. Therefore, they recommended addressing self-efficacy and self-regulatory competence together: interventions that teach self-regulation skills should contain components that increase students’ confidence in their academic skills (Schunk and Ermter 2000 ).
University staff should temper their expectations of first-year students’ self-regulation skills. Previous studies showed that many first-year lecturers believe students already possess these skills (Cook and Leckey 1999 ), and therefore, they do not emphasise (further) development of these skills, even though they are crucial to student success. Paying attention to study skill development, however, may produce positive effects. Interventions focused on the development of academic skills led to gains in academic achievement (Evans and Burck 1992 ). Promoting good study behaviour alone may not be sufficient, in that intrinsic motivation also influenced adjustment. Moreover, many researchers emphasised the importance of combining study skills factors and motivational factors to boost students’ achievement (Eccles and Wigfield 2002 ; Pintrich et al. 1993 ; Robbins et al. 2004 ). Of Zepke and Leach’s ( 2010 ) ten proposed actions to enhance higher education students’ engagement, the first two focus on increasing motivation: enhancing students’ self-belief and enabling students to work autonomously, enjoy learning relationships with others and feel they are able to reach their own goals. These actions could be a meaningful starting point to increase motivation.
Whereas self-regulation skills and motivation can be positively influenced when the student already is in university, this is to a lesser extent the case with students’ satisfaction with their chosen programme. There is not much that can be done when the student has simply chosen a programme that is not what he or she expected it to be and thus does not match his or her abilities, interests and values. Switching programmes then is a good solution. Because of this large influence we found of satisfaction with the programme on persistence [which is in line with a study by Jansen and Suhre ( 2010 )], it is worthwhile to help prospective students make a good programme choice. Both secondary schools and universities play important roles in this regard. Secondary schools could provide students with the opportunity to get to know the programmes in which they are interested—for example, by having them write a comparative essay of three study programmes, which would encourage them to go in depth to investigate the study programmes and the extent to which they fit the students’ individual strengths, interests, values and learner characteristics. Universities could provide information for prospective students in such a way that their expectations of a programme will be realistic. Information should be transparent about crucial characteristics of the study, such as the curriculum, the degree of difficulty, the level of guidance and availability of staff, the available facilities of the university and so on. Last, since both universities and secondary schools are important parties in the transition, it would be beneficial if they would communicate and collaborate more.
Limitations and directions for future research
A first limitation of the current study was that we only accounted for academic adjustment, not for other types of adjustment. Although it is the most consistent correlate of achievement compared with other types (Rienties et al. 2012 ), measuring social, personal-emotional and institutional adjustment in addition could be valuable. Second, there were some limitations regarding the sample: it was a convenience sample that consisted of students from several universities and degree programmes without taking these differences into account. Although interesting for future research, investigating differences between fields or programmes was not the intention of this study and the sample was not sufficiently large to do so. Looking at the relatively high GPAs, and the relatively small variance of measures as self-efficacy and academic adjustment that we found, it seems likely that the sample was biased towards the better performing students. We know from research on response bias that it is a familiar problem that higher-achieving students are more inclined to complete surveys than their lower-achieving peers (Sax et al. 2003 ). Therefore, it is important to validate these results with a larger and more diverse sample. In this regard, especially the absence of a link between self-efficacy and academic adjustment would be worthwhile to re-investigate. If the variance between both factors would be increased the model may behave differently. Third, we measured all variables at one point in time, which makes it impossible to detect causal relationships and to map processes. Many of the proposed linkages in the conceptual model could arguably be turned around, e.g. academic adjustment could influence self-efficacy. To determine causal relationships, it would be worthwhile to conduct longitudinal research that starts measuring motivational and behavioural variables in secondary school and investigates how they relate to adjustment and student success outcomes later in university. Relatedly, research should investigate whether secondary school teachers are giving adequate attention to preparing students for university. If so, how, and are their practices in line with what students need to be well prepared? In this study, for example, self-regulated study behaviour is very important for first-year university students. Are secondary schools preparing their students to manage their time, environment, and effort efficiently? Moreover, study choice is crucial; students who are dissatisfied with their chosen programme are at a high risk to quit. Researchers could provide a clear image of what teachers and advisors in secondary school currently are doing to help their students make suitable choices and how they could improve those choice processes.
Change history
08 january 2018.
The article “First-year university students’ academic success: the importance of academic adjustment,” written by Els C. M. van Rooij, Ellen P. W. A. Jansen, and Wim J. C. M. van de Grift, was originally published electronically on the publisher’s internet portal (currently SpringerLink) on 4 November 2017 without open access.
Abott-Chapman, J. A., Hughes, P. W., & Wyld, C. (1992). Monitoring student progress: A framework for improving student performance and reducing attrition in higher education . Hobart: National Clearinghouse for Youth Studies.
Google Scholar
Aspelmeier, J. E., Love, M. M., McGill, L. A., Elliott, A. N., & Pierce, T. W. (2012). Self-esteem, locus of control, college adjustment, and GPA among first- and continuing-generation students: a moderator model of generational status. Research in Higher Education, 53 (7), 755–781.
Article Google Scholar
Astin, A. (1999). Student involvement: a developmental theory for higher education. Journal of College Student Development, 40 (5), 518–529.
Bailey, T. H., & Phillips, L. J. (2016). The influence of motivation and adaptation on students’ subjective well-being, meaning in life and academic performance. Higher Education Research and Development, 35 (2), 201–216.
Baker, R. W., & Siryk, B. (1984). Measuring adjustment to college. Journal of Counseling Psychology, 31 (2), 179–189.
Baker, R. W., & Siryk, B. (1989). SACQ: student adaptation to college questionnaire manual . Los Angeles: Western Psychological Services.
Baker, S. R. (2004). Intrinsic, extrinsic, and amotivational orientations: their role in university adjustment, stress, well-being, and subsequent academic performance. Current Psychology, 23 (3), 189–202.
Bandura, A. (1997). Self-efficacy: The exercise of control . New York: W. H. Freeman.
Bouffard-Bouchard, T., Parent, S., & Larivée, S. (1991). Influence of self-efficacy on self-regulation and performance among junior and senior high-school age students. International Journal of Behavioral Development, 14 (2), 153–164.
Bowles, A., Fisher, R., McPhail, R., Rosenstreich, D., & Dobson, A. (2014). Staying the distance: students’ perceptions of enables of transition to higher education. Higher Education Research and Development, 33 (2), 212–225.
Burlison, J. D., Murphy, C. S., & Dwyer, W. O. (2009). Evaluation of the motivated strategies for learning questionnaire for predicting academic performance in college students of varying scholastic aptitude. College Student Journal, 43 (4), 1313–1323.
CBS (Centraal Bureau voor de Statistiek [Statistics Netherlands]) (2016). Meer vwo’ers direct naar de universiteit [More pre-university students directly to university]. Retrieved from https://www.cbs.nl/nl-nl/nieuws/2016/02/meer-vwo-ers-direct-naar-de-universiteit .
Chemers, M. M., Hu, L., & Garcia, B. F. (2001). Academic self-efficacy and first-year college student performance and adjustment. Journal of Educational Psychology, 93 (1), 55–64.
Cook, A., & Leckey, J. (1999). Do expectations meet reality? A survey of changes in first-year student opinion. Journal of Further and Higher Education, 23 (2), 157–171.
Credé, M., & Phillips, L. A. (2011). A meta-analytic review of the motivated strategies for learning questionnaire. Learning and Individual Differences, 21 (4), 337–346.
De Buck, W. (2009). Studiekeuze, informatiegebruik en studie-uitval in het hoger onderwijs [study choice, information use and drop-out in higher education]. Tijdschrift voor Hoger Onderwijs, 27 (3), 147–156.
De Clercq, M., Galand, B., Dupont, S., & Frenay, M. (2013). Achievement among first-year university students: an integrated and contextualized approach. European Journal of Psychology of Education, 28 (3), 641–662.
De Clercq, M., Galand, B., & Frenay, M. (2017). Transition from high school to university: a person-centered approach to academic achievement. European Journal of Psychology of Education, 32 , 39–59.
Eccles, J. S., & Wigfield, A. (2002). Motivational beliefs, values, and goals. Annual Review of Psychology, 53 (1), 109–132.
Evans, J. H., & Burck, H. D. (1992). The effects of career education interventions on academic achievement: a meta-analysis. Journal of Counseling & Development, 71 (1), 63–68.
Fenollar, P., Román, S., & Cuestas, P. J. (2007). University students’ academic performance: an integrative conceptual framework and empirical analysis. British Journal of Educational Psychology, 77 (4), 873–891.
Hurtado, S., Han, J. C., Sáenz, V. B., Espinosa, L. L., Cabrera, N. L., & Cerna, O. S. (2007). Predicting transition and adjustment to college: biomedial and behavioral science aspirants’ and minority students’ first year of college. Research in Higher Education, 48 (7), 841–887.
Inspectie van het Onderwijs [Inspectorate of Education] (2016). De staat van het onderwijs: Onderwijsverslag 2014/2015 [The current state of education. Educational report 2014/2015]. Retrieved from http://www.onderwijsinspectie.nl/binaries/content/assets/Onderwijsverslagen/2016/de-staat-van-het-onderwijs-2014-2015.pdf .
Jacobsen, W. C., & Forste, R. (2011). The wired generation: academic and social outcomes of electronic media use among university students. Cyberpsychology, Behavior and Social Networking, 14 (5), 275–280.
Jansen, E. P. W. A., & Suhre, C. J. M. (2010). The effect of secondary school study skills preparation on first-year university achievement. Educational Studies, 36 (5), 569–580.
Jones, H. A., Rabinovitch, A. E., & Hubbard, R. H. (2015). ADHD symptoms and academic adjustment to college: the role of parenting style. Journal of Attention Disorders, 19 (3), 251–259.
Kaczmarek, P. G., Matlock, C. G., & Franco, J. N. (1990). Assessment of college adjustment in three freshman groups. Psychological Reports, 66 (3), 1195–1202.
Kamphorst, J. C., Hofman, W. H. A., Jansen, E. P. W. A., & Terlouw, C. (2012). Een algemene benadering werkt niet. Disciplinaire verschillen als verklaring van studievoortgang in het hoger onderwijs [A general approach does not work. Disciplinary differences as an explanation of study progress in higher education]. Pedagogische Studiën, 89 (1), 20–38.
Kennedy, P. W., Sheckley, B. G., & Kehrhahn, M. T. (2000). The dynamic nature of student persistence: influence of interactions between student attachment, academic adaptation, and social adaptation . Paper presented at the Annual Meeting of the Association for International Research, Cincinnati, May 21–24.
Kline, R. B. (2005). Principles and practice of structural equation modeling . New York: The Guilford Press.
Kuh, G. D., Kinzie, J., Buckley, J. A., Bridge, B. K., & Hayek, J. C. (2006). What matters to student success: a review of the literature. Commissioned report for the National Symposium on postsecondary student success: spearheading a dialog on student success . Washington, DC: National Postsecondary Education Cooperative.
Lowe, H., & Cook, A. (2003). Mind the gap: are students prepared for higher education? Journal of Further and Higher Education, 27 (1), 53–76.
Lynch, D. J. (2006). Motivational factors, learning strategies and resource management as predictors of course grades. College Student Journal, 40 (2), 423–428.
Martin, W. E., Swartz-Kulstad, J. L., & Madson, M. (1999). Psychosocial factors that predict the college adjustment of first-year undergraduate students: implications for college counselors. Journal of College Counseling, 2 (2), 121–133.
McKenzie, K., & Schweitzer, R. (2001). Who succeeds at university? Factors predicting academic performance in first year Australian university students. Higher Education Research and Development, 20 (1), 121–133.
Moore, R. W., & Foy, R. L. H. (1997). The scientific attitude inventory: a revision (SAI II). Journal of Research in Science Teaching, 34 (4), 327–336.
Owen, S., & Froman, R. (1988). Development of an academic self-efficacy scale . Paper presented at the annual meeting of the National Council on Measurement in Education, New Orleans, LA, April 6–8.
Pascarella, E., & Terenzini, P. T. (2005). How college affects students. A third decade of research . San Francisco: Jossey-Bass.
Petersen, I., Louw, J., & Dumont, K. (2009). Adjustment to university and academic performance among disadvantaged students in South-Africa. Educational Psychology, 29 (1), 99–115.
Pintrich, P. R. (2004). A conceptual framework for assessing motivation and self-regulated learning in college students. Educational Psychology Review, 16 (4), 385–407.
Pintrich, P. R., Smith, D. A. R., Garcia, T., & McKeachie, W. J. (1993). Reliability and predictive validity of the motivated strategies for learning questionnaire (MSLQ). Educational and Psychological Measurement, 53 (3), 801–813.
Ramsay, S., Jones, E., & Barker, M. (2007). Relationship between adjustment and support types: young and mature-aged local and international first year university students. Higher Education, 54 (2), 247–265.
Richardson, M., Abraham, C., & Bond, R. (2012). Psychological correlates of university students’ academic performance: a systematic review and meta-analysis. Psychological Bulletin, 138 (2), 353–387.
Rienties, B., Beausaert, S., Grohnert, T., Niemantsverdriet, S., & Kommers, P. (2012). Understanding academic performance of international students: the role of ethnicity, academic and social integration. Higher Education, 63 (6), 685–700.
Robbins, S. B., Lauver, K., Le, H., Davis, D., Langley, R., & Carlstrom, A. (2004). Do psychological and study skills factors predict college outcomes? A meta-analysis. Psychological Bulletin, 130 (2), 261–288.
Rodríguez-González, M. S., Tinajero-Vacas, C., Guisande-Couñago, M. A., & Páramo-Fernández, M. F. (2012). The Student Adaptation to College questionnaire (SACQ) for use with Spanish students. Psychological Reports: Measures & Statistics, 111 (2), 624–640.
Ryan, R. M., & Deci, E. L. (2000). Intrinsic and extrinsic motivations: classic definitions and new directions. Contemporary Educational Psychology, 25 (1), 54–67.
Sax, L. J., Gilmartin, S. K., & Bryant, A. N. (2003). Assessing response rates and nonresponse bias in web and paper surveys. Research in Higher Education, 44 , 409–432.
Schunk, D. H., & Ertmer, P. A. (2000). Self-regulation and academic learning. Self-efficacy enhancing interventions. In M. Zeidner, P. R. Pintrich, & M. Boekaerts (Eds.), Handbook of self-regulation (pp. 631–649). Burlington: Academic Press.
Chapter Google Scholar
SCP (Sociaal Cultureel Planbureau [The Netherlands Institute for Social Research]). (2014). Emancipatiemonitor 2014 . Den Haag: Sociaal Cultureel Planbureau.
Sevinç, S., & Gizir, C. A. (2014). Factors negatively affecting university adjustment from the views of first-year university students: the case of Mersin University. Educational Sciences: Theory & Practice, 14 (4), 1301–1308.
Suhre, C. J. M., Jansen, E. P. W. A., & Harskamp, E. G. (2007). Impact of degree program satisfaction on the persistence of college students. Higher Education, 54 (2), 207–226.
Tinto, V. (1993). Leaving college: rethinking the causes and cures of student attrition (2nd ed.). Chicago: The University of Chicago Press.
Van den Broek, A., Tholen, R., Wartenbergh, F., Bendig-Jacobs, J., Brink, M., & Braam, C. (2014). Monitor Beleidsmaatregelen 2014. Studiekeuze, studiegedrag en leengedrag in relatie tot beleidsmaatregelen in het hoger onderwijs [monitor policy measures 2014. Degree programme choice, study behaviour, and student loans related to policy measures in higher education] . Nijmegen: ResearchNed.
Van Rooij, E. C. M., Jansen, E. P. W. A., & Van de Grift, W. J. C. M. (2017). Secondary school students' engagement profiles and their relationship with academic adjustment and achievement in university. Learning and Individual Differences, 54 , 9–19.
Wartenbergh, F., & Van den Broek, A. (2008). Studieuitval in het hoger onderwijs: Achtergrond en oorzaken [drop-out in higher education: background and causes] . Nijmegen: ResearchNed.
Willcoxson, L., Cotter, J., & Joy, S. (2011). Beyond the first-year experience: the impact on attrition of student experiences throughout undergraduate degree studies in six diverse universities. Studies in Higher Education, 36 (3), 331–352.
Wintre, M. G., Dilouya, B., Pancer, S. M., Pratt, M. W., Birnie-Lefcovitch, S., Polivy, J., & Adams, G. (2011). Higher Education, 62 (4), 467–481.
Wouters, S., Germeijs, V., Colpin, H., & Verschueren, K. (2011). Academic self-concept in high school: predictors and effects on adjustment in higher education. Scandinavian Journal of Psychology, 52 (6), 586–594.
Yorke, M., & Longden, B. (2007). The first-year experience in higher education in the UK. Report on phase 1 of a project funded by the Higher Education Academy . Bristol: Higher Education Academy.
Zepke, N., & Leach, L. (2010). Improving student engagement: ten proposals for action. Active Learning in Higher Education, 11 (3), 167–177.
Download references
Author information
Authors and affiliations.
Department of Teacher Education, University of Groningen, Grote Kruisstraat 2/1, 9712 TS, Groningen, The Netherlands
Els C. M. van Rooij, Ellen P. W. A. Jansen & Wim J. C. M. van de Grift
You can also search for this author in PubMed Google Scholar
Corresponding author
Correspondence to Els C. M. van Rooij .
Additional information
Els C. M. van Rooij (corresponding author). Department of Teacher Education, University of Groningen, Grote Kruisstraat 2/1, 9712 TS Groningen, The Netherlands. Telephone: +31 50 363 31 99. E-mail address: [email protected].
Current themes of research
University preparation in secondary education; the transition from secondary education to university; first-year university students’ achievement.
Relevant publication
Van Rooij, E., Jansen, E. & Van de Grift, W. (2017). Secondary school students’ engagement profiles and their relationship with academic adjustment and achievement in university. Learning and Individual Differences, 54, 9–19.
Ellen P. W. A. Jansen . Department of Teacher Education, University of Groningen, Grote Kruisstraat 2/1, 9712 TS Groningen, The Netherlands. Telephone: +31 50 363 3644. E-mail address: [email protected].
The transition from secondary education to university; assessment and self-selection for teacher education; learning communities in higher education; international students and the international classroom; excellence in higher education.
Relevant publications
Jansen, E. P. W. A., Andre, S., & Suhre, C. J. M. (2013). Readiness and expectations questionnaire: a cross-cultural measurement instrument for first-year university students . Educational Assessment Evaluation and Accountability , 25 (2), 115–130.
Jansen, E. P. W. A., & Suhre, C. J. M. (2010). The effect of secondary school study skills preparation on first-year university achievement . Educational Studies , 36 (5), 569–580.
Jansen, E. P. W. A., Suhre, C. J. M., & André, S. (2016). Transition to an international degree programme:
Preparedness, first-year experiences and study success of students from different nationalities. In E. Kyndt, V. Donche, K. Trigwell, & S. Lindblom-Ylänne (Eds.), Higher Education Transitions: Theory and Research (New perspectives on learning and instruction). Routledge.
Jansen, E. P. W. A., & Van der Meer, J. (2012). Ready for university? A cross national study on students’ perceived preparedness for university . Australian Educational Researcher , 39 (1), 1–16.
Wim J. C. M. van de Grift . Department of Teacher Education, University of Groningen, Grote Kruisstraat 2/1, 9712 TS Groningen, The Netherlands. Telephone: +31 50 363 8518. E-mail address: [email protected].
Evidence-based education; teacher development; school didactics.
Huijgen, T., van de Grift, W., van Boxtel, C., & Holthuis, P. (2016). Teaching Historical Contextualization: The Construction of a Reliable Observation Instrument . European Journal of Psychology of Education , 1–23.
Maulana, R., Helms-Lorenz, M., & van de Grift, W. (2016). The role of autonomous motivation for academic engagement of Indonesian secondary school students: A multilevel modelling. In R. B. King, & A. B. I. Bernardo (Eds.), The psychology of Asian learners: A festschrift in honor of David Watkins (pp. 237–251). Singapore: Springer.
Van de Grift, W., Helms-Lorenz, M., & Maulana, R. (2014). Teaching skills of student teachers: Calibration of an evaluation instrument and its value in predicting student academic engagement . Studies in Educational Evaluation , 43 , 150–159.
Rights and permissions
Open Access This article is distributed under the terms of the Creative Commons Attribution 4.0 International License (http://creativecommons.org/licenses/by/4.0/), which permits use, duplication, adaptation, distribution and reproduction in any medium or format, as long as you give appropriate credit to the original author(s) and the source, provide a link to the Creative Commons license, and indicate if changes were made.
Reprints and permissions
About this article
van Rooij, E.C.M., Jansen, E.P.W.A. & van de Grift, W.J.C.M. First-year university students’ academic success: the importance of academic adjustment. Eur J Psychol Educ 33 , 749–767 (2018). https://doi.org/10.1007/s10212-017-0347-8
Download citation
Received : 15 February 2017
Revised : 04 July 2017
Accepted : 04 August 2017
Published : 04 November 2017
Issue Date : October 2018
DOI : https://doi.org/10.1007/s10212-017-0347-8
Share this article
Anyone you share the following link with will be able to read this content:
Sorry, a shareable link is not currently available for this article.
Provided by the Springer Nature SharedIt content-sharing initiative
- Achievement
- Persistence
- Self-regulation
- Find a journal
- Publish with us
- Track your research
- Open supplemental data
- Reference Manager
- Simple TEXT file
People also looked at
Review article, how to promote diversity and inclusion in educational settings: behavior change, climate surveys, and effective pro-diversity initiatives.
- Department of Psychology, University of Wisconsin-Madison, Madison, WI, United States
We review recent developments in the literature on diversity and inclusion in higher education settings. Diversity interventions increasingly focus on changing behaviors rather than mental constructs such as bias or attitudes. Additionally, there is now a greater emphasis on the evaluation of initiatives aimed at creating an inclusive climate. When trying to design an intervention to change behavior, it is advised to focus on a segment of the population (the “target audience”), to try to get people to adopt a small number of specific new behaviors (the “target behaviors”), and to address in the intervention the factors that affect the likelihood that members of the target audience will engage in the new target behaviors (the “barriers and benefits”). We report our recent work developing a climate survey that allows researchers and practitioners to identify these elements in a particular department or college. We then describe recent inclusion initiatives that have been shown to be effective in rigorous empirical studies. Taken together this paper shows that by implementing techniques based on research in the behavioral sciences it is possible to increase the sense of belonging, the success, and the graduation rate of minority students in STEM.
Introduction
Women, people of color, members of the LGBTQ + community, and members of other marginalized groups continue to be underrepresented in STEM fields ( National Science Foundation, 2020 ). Students from these groups are the target of both subtle and overt acts of discrimination, face negative stereotypes about their abilities, and experience disrespect and lack of inclusion by their instructors and peers ( Spencer and Castano, 2007 ; Wiggan, 2007 ; Cheryan et al., 2009 ). For example, students from marginalized groups are often assumed to be less intelligent and competent ( Moss-Racusin et al., 2014 ) and are often excluded when students form study groups or gather outside of class ( Slavin, 1990 ). Students from marginalized groups receive less challenging materials, worse feedback, and less time to respond to questions in class than their peers ( Beaman et al., 2006 ; Sadker et al., 2009 ). Additionally, the cultural mismatch between university norms and the cultural norms that students from marginalized groups were socialized in frequently leads to increased stress and negative emotions for these students ( Stephens et al., 2012 ).
Not surprisingly, students from marginalized groups are far more likely than high-status group members (e.g., White people, men) to report feeling as though they do not belong at universities ( Walton and Cohen, 2011 ). This is particularly problematic given that social belonging has been shown to be a key predictor of educational outcomes ( Dortch and Patel, 2017 ; Wolf et al., 2017 ; Murphy et al., 2020 ). Students who feel a greater sense of belonging are more likely to persist to graduation ( Strayhorn, 2012 ). Additionally, increased concerns about belonging can lead students to view common challenges—such as struggling to make friends or failing a test—as signs that they do not belong, promoting psychological disengagement and poorer educational outcomes ( Walton and Cohen, 2007 ). These challenges are exacerbated in STEM fields, which are typically dominated by members of high-status groups ( Rainey et al., 2018 ). Students from marginalized groups are particularly vulnerable to dropping out of STEM programs and the lack of a sense of community greatly contributes to this vulnerability ( O’Keefe, 2013 ).
It is clear then that the key to promoting academic success and retention of students from marginalized groups in STEM is creating an inclusive climate. In this article we will review recent developments within the diversity and inclusion literature about how to best promote inclusive behaviors and create an inclusive climate at colleges and universities. We will start out by describing recent shifts in the literature emphasizing the importance of changing behaviors rather than attitudes and the necessity to systematically evaluate diversity interventions. We will then review the key elements to designing effective interventions to promote diversity and inclusion. We will also talk about the use of focus groups and climate surveys to acquire the relevant background knowledge needed to design effective interventions. In the final section, we present recent initiatives that have successfully promoted diversity and inclusion in a variety of ways.
Recent Developments in Research on Diversity and Inclusion
A shift from reducing bias to promoting inclusive behavior.
Even though prejudice is communicated through behavior ( Carr et al., 2012 ), the traditional approach to prejudice reduction was to change explicit and implicit bias. The focus on bias was based on the assumption that changes in attitudes will subsequently lead to changes in behavior ( Dovidio et al., 2002 ). The universal acceptance of this assumption is surprising given the weak evidence for a link between attitudes and behavior. Explicit biases and attitudes more generally have been shown to predict behavior only weakly ( Wicker, 1969 ; Ajzen and Sheikh, 2013 ). Similarly, there is little to no connection between implicit bias and behavior ( Kurdi et al., 2019 ; Clayton et al., 2020 ). Implicit bias scores explain, at most, a very small proportion of the variability in intergroup behavior measured in lab settings, and this proportion is likely to be even smaller in more complex, real-world situations ( Oswald et al., 2013 ). Further, a change in implicit bias is not associated with a change in intergroup behavior. Lai et al. (2013) and Forscher et al. (2019) showed that while a variety of methods have been developed to change implicit bias, these methods produce trivial or nonexistent changes in intergroup behavior, and if they do, none of them last longer than 24 hours.
A growing body of research suggests that it is possible–and likely more effective–to focus on promoting inclusive behavior rather than improving individuals’ attitudes toward outgroup members. For example, Mousa (2020) randomly assigned Iraqi Christians displaced by the Islamic State of Iraq and Syria (ISIS) either to an all-Christian soccer team or to a team mixed with Muslims. Christians with Muslim teammates were more likely to vote for a Muslim from another team to receive a sportsmanship award, register for a mixed faith team next season, and train with other Muslim soccer players six months after the intervention. However, attitudes toward Muslims more broadly did not change. Similarly, Scacco and Warren (2018) examined if sustained intergroup contact in an educational setting between Christian and Muslim men in Kaduna, Nigeria led to increased harmony and reduced discrimination between the two groups. After the intervention, there were no reported changes in prejudicial attitudes for either groups, but Christians and Muslims who had high levels of intergroup contact engaged in fewer discriminatory behaviors than peers who had low levels of intergroup contact. These findings demonstrate that while promoting both positive intergroup attitudes and inclusive behavior is ideal, it is necessary to target inclusive behaviors directly rather than trying to change people’s biased attitudes with the assumption that such change will translate into a subsequent behavior change.
Greater Emphasis on Evaluation
Since the Civil Rights Act of 1964, researchers and practitioners have developed a variety of initiatives to combat racial prejudice in the United States (for reviews see Murrar et al., 2017 ; Paluck and Green, 2009 ; Paluck et al., 2021 ). Although these initiatives have been tested in individual studies, primarily in the lab, many of them have not undergone the rigorous scientific testing that is required to be able to conclude that they are effective in real-world settings ( Paluck and Green, 2009 ). Further, the evaluation studies frequently examined only the effects on self-report attitudes and not behavioral outcomes, which is problematic for reasons outlined in the previous paragraphs. In light of this deficit, there has been a recent shift in this field of research which now emphasizes the need for systemic evaluation of the effectiveness of diversity initiatives in the field ( Moss-Racusin et al., 2014 ).
Recent work examining the effectiveness of diversity initiatives has found mixed evidence for the idea that existing strategies reduce discrimination, create more inclusive environments, or increase the representation of marginalized groups ( Noon, 2018 ; FitzGerald et al., 2019 ; Dover et al., 2020 ). Most diversity training or implicit bias training workshops have been shown to be ineffective ( Bezrukova et al., 2016 ; Chang et al., 2019 ). Some interventions meant to promote diversity and inclusion actually achieve the opposite effect ( Dobbin and Kalev, 2018 ). For example, Dobbin et al., (2007) found that diversity training workshops had little to no effect on improving workplace diversity and some actually led to a decline in the number of Black women in management positions at companies. Similarly, Kulik et al. (2007) found that employees often respond to mandatory diversity training with anger and resistance and some report increased animosity toward members of marginalized groups afterward.
Designing Successful Behavioral Interventions
Behavior change interventions tend to be more effective if they involve a systematic, focused approach which consists of identifying and targeting specific behaviors, catering the intervention to a particular audience, and incorporating in the intervention relevant information about factors that affect how members of the target audience appraise the target behavior ( Campbell and Brauer, 2020 ). Below, we have outlined several methodological and theoretical considerations for practitioners whose goal is to develop a behavioral intervention to promote diversity and inclusion (see Figure 1 ).
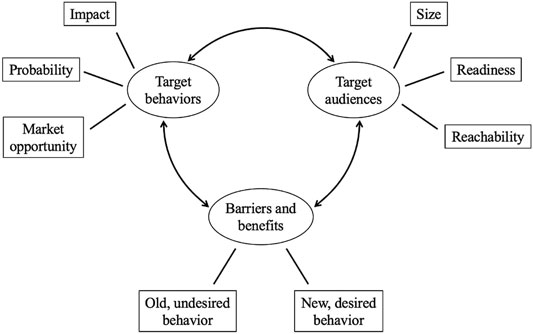
FIGURE 1 . Key elements to consider designing a behavior change intervention (adapted from Campbell and Brauer, 2020 ).
Selecting a Target Behavior
Once a broad issue has been identified (e.g., promoting diversity and inclusion at a university department), it must be distilled into a measurable, actionable goal ( Smith, 2006 ). For example, one might focus on an outcome such as reducing the racial achievement gap. It is critical that the desired outcome is quantifiable, as that will allow one to determine whether a behavioral intervention has been a success.
The next step is to identify and select a desired behavior to be adopted (i.e., the target behavior). The goal is to choose a target behavior that will lead to the desired outcome if people actually perform it ( Lee and Kotler, 2019 ). Continuing with the previous example, a behavioral intervention with the goal of reducing the racial achievement gap may target behaviors such as encouraging White students to include students of color in their study groups and social events or motivate instructors to highlight to a greater extent the contributions of female scientists. Sometimes it is possible to promote multiple similar target behaviors in the same intervention.
To identify potential target behaviors it is usually advised to conduct background research (see next section of this paper). This research may involve semi-structured interviews or focus groups with members of marginalized groups. Climate surveys with closed and open-ended questions can be equally informative. The goal of the background research is to determine the behaviors that affect members of marginalized groups the most. It is crucial to know what behaviors they find offensive and disrespectful and thereby decrease their sense of belonging, and what behaviors make them feel included, welcomed, and cared for. Examples of target behaviors to promote inclusion are attending diversity-outreach events or consciously forming diverse work groups.
Once a list of potential target behaviors has been established, it is advised to choose one of them for the intervention. The choice can be guided by evaluating each potential target behavior along a number of relevant dimensions ( McKenzie-Mohr, 2011 ). One may consider, for example, the extent to which the effect of changing from the old behavior to the new target behavior will have a large effect (“impact”), how likely people are to adopt the target behavior (“probability”), and how many people currently do not yet engage in the target behavior (“market opportunity”). For instance, an intervention seeking to reduce discriminatory behaviors toward members of the LGBTQ + community in STEM contexts might consider focusing on encouraging students to learn what terms hurt the feelings of queer people and then abstain from using them, get the students to avoid gendered language, or promote joining a queer-straight alliance at their university. While a large number students joining a queer-straight alliance would have a big effect on the sense of belonging of members of the LGBTQ + community (high impact), it is unlikely many students will adopt this behavior if they are not already predisposed to do so (low probability). Similarly, it may be easy to get students to switch to gender neutral language (high probability), but if most students are already using this language then promoting this behavior will lead to only minor improvements (low market opportunity).
Ultimately the goal is to choose a single behavior (or a small set of interrelated behaviors) that will make the biggest difference for members of marginalized groups and then design an intervention that specifically encourages the adoption of this behavior ( Wymer, 2011 ).
Selecting a Target Audience
One of the most vital considerations when designing a behavioral intervention is the selection of a specific target audience ( Kotler et al., 2001 ). Different segments of the population are receptive to different messages, possess different motivations, and have different reasons for engaging or not engaging in the desirable behavior ( Walsh et al., 2010 ). Although all individuals in a specific setting are usually exposed to a given pro-diversity initiative (e.g., everyone in a specific department or college), the initiative is more likely to be effective if it is designed with a specific subset of the population in mind ( French et al., 2010 ).
The first step in determining a target audience is to segment the population into various groups along either demographic criteria (e.g., Whites, men), occupation (e.g., students, teaching assistants, faculty, staff), or psychological dimensions (e.g., highly egalitarian individuals, individuals with racist attitudes, folks in the middle). The background research described in the next section will help practitioners identify the groups that have the most negative impact on the climate in a department or college. One can find out from members of marginalized groups, for example, which groups treat them in the most offensive way or which kind of people have the most negative impact on their sense of belonging.
Although multiple groups may emerge as potential target audiences, it is generally advised to choose only one as the focus of the intervention. Similar to the process of selecting a target behavior, the choice of the target audience can be guided by considering a number of relevant dimensions: How large is the segment, and what percentage of the members of this segment currently do not yet engage in the target behavior (“size”)? To what extent are members of this segment able, willing, and ready to change their behavior (“readiness”)? How easy it is to identify the members of this segment and are there known distribution channels for persuasive messages (“reachability”)? Teaching assistants may be a group that can easily be instructed to adopt certain behaviors (high reachability), individuals with hostile feelings toward certain social groups may not be willing to behave inclusively (low readiness), and academic advisors may be a group that is too small and that students from marginalized backgrounds interact with too infrequently to be chosen as the target audience (small size).
Most effective behavior change interventions are designed with a single target audience in mind. That is, the communications and campaign materials are designed so that they are appealing and persuasive for the members of the chosen target audience. The objective should thus be to choose a single target audience that can be persuaded to adopt the target behavior and has a big impact on how included members of marginalized groups feel in the department or college.
Barriers and Benefits
It is critical to consider the factors that influence the likelihood that members of the target audience will engage in the desired target behavior, the so-called “barriers” and “benefits” ( Lefebvre, 2011 ). Barriers refer to anything that prevents an individual from engaging in a given behavior. Benefits are the positive outcomes an individual anticipates receiving as a result of engaging in the behavior. The ultimate goal is to design an intervention that makes salient the target audience’s perceived benefits of the new, desired target behavior and the perceived barriers toward engaging in the current, undesired behavior ( McKenzie-Mohr and Schultz, 2014 ).
Practitioners likely want to conduct background research to learn about the target audience’s motivations to engage in various behaviors. This can again be done with interviews, focus groups, or climate surveys, but this time the responses of members of the target audience, rather than the responses of members of marginalized groups, are most relevant. One should find out why members of the target audience currently do not perform the target behavior. Are there any logistic barriers (e.g., lack of opportunity) or psychological barriers (i.e., discomfort experienced around certain groups)? Are there any incorrect beliefs that underly the current behavior? The background research should also identify the positive consequences members of the target audience value and expect to experience when performing the target behavior. These consequences can then be highlighted in the intervention.
Both barriers and benefits can be abstract or concrete, internal or external, and real or perceived. For example, if an intervention seeks to encourage students from different backgrounds to be friendly to one another in the classroom members of the target audience may be apprehensive when interacting with outgroup members due to fear of saying something offensive (a barrier) but would interact more frequently with outgroup members if they believed that it would provide them an opportunity to make new friends (benefits). A well-designed behavioral intervention would then use this information to craft persuasive messages that directly address the target audience’s barriers and benefits. In this specific example, the intervention might involve providing people with tools to avoid offensive language and emphasize the potential to make new friends.
Elements That Increase the Persistence of a Behavioral Change
Sometimes people adopt a new behavior but then switch back to the old, undesired behavior after a few days or weeks. What can be done to increase the persistence of behavior change? One strategy that has proven to be particularly effective is to change the assumptions that people make about themselves and their environments ( Frey and Rogers, 2014 ; Walton and Wilson, 2018 ). For example, believing that one is not culturally competent will lead to interpreting difficult interactions with outgroup members as proof of this assumption. The more entrenched these beliefs become, the more difficult behaviors are to change. However, the human tendency to “make meaning” of oneself and one’s social situations can be harnessed for positive behavioral change. By altering the assumptions that lead to undesirable behaviors, it is possible to set in motion recursive cycles where a person’s new behavior leads to positive reactions in the environment, which in turn reinforces the self-representation that they are “the kind of person” who cares about this issue (e.g., diversity) and engages in these behaviors (e.g., inclusive behaviors). Consider an example from a different domain: Fostering a growth mindset where students start to believe they can improve through practice will change how they interpret successes and failures, thereby disrupting the negative feedback cycle that leads to poorer performance in school (see Yeager et al., 2019 ).
In addition, interventions that foster habit formation are more likely to increase the persistence of new behaviors ( Wood and Rünger, 2016 ). Interventions can promote habit formation by increasing the perceived difficulty of performing an undesirable behavior or by decreasing the perceived difficulty of doing the new target behavior. People will most often engage in behaviors that they perceive as being easy to do, regardless of whether or not the difference in difficulty is minimal. Additionally, providing easy to understand, recurring cues that encourage desirable behaviors and disrupt old, undesirable behaviors can help facilitate habit formation.
How to Conduct Relevant Background Research
There are a variety of ways how members of higher education institutions can identify the diversity-related issues that should be addressed in their department or college. The most frequently used methods are focus groups and climate surveys. We will discuss each of these methods below.
Focus groups are effective because a group member’s comment may cause other members to remember issues that they would not have thought of otherwise. It is easy to recruit students from marginalized groups by appealing to their departmental citizenship or by promising attractive prizes (e.g., two $100 gift certificates that will be given out to two randomly selected members of the focus group). It is generally advised to form groups of individuals sharing some social identity (i.e., African Americans, Latinxs, women in technical fields). Most individuals feel more comfortable voicing their concerns if the focus group facilitator also shares their social identity. Many universities have skilled focus group facilitators, but if necessary, it is possible to train research assistants by directing them to appropriate resources ( Krueger, 1994 ; https://fyi.extension.wisc.edu/programdevelopment/files/2016/04/Tipsheet5.pdf ).
Focus group members should be encouraged to talk about the situations in which they felt excluded, disrespected, or discriminated against. For example, focus group members might be asked questions such as “What exactly did the other person do or say? Where did the situation occur (in the classroom, during office hours)? Who was the other person (peer, instructor, staff)?” Focus group members should then be asked about the situations in which they felt included, respected, and cared for. Again, the goal should be to obtain precise information about the exact nature of the behaviors, the place in which they occurred, and person who engaged in the behaviors. It is useful to ask about the relative impact of these negative and positive behaviors. For example, one might ask “If you could eliminate one behavior here in this department which one would it be?” and “Among all the inclusive and respectful behaviors you just mentioned which one would increase your sense of belonging the most?”.
To assess the barriers and benefits of the potential target behaviors it can be useful to conduct focus groups with individuals who a priori do not come from any of the marginalized groups mentioned above. The facilitator can describe the negative behaviors (without labeling them as discriminatory) and ask whether the focus group members sometimes engage in them and if they do, why. One might ask about potential pathways to eliminate these undesired behaviors, e.g., “What would have to be different for you–or your peers–to no longer behave like that?”. The next step is to have a similar discussion about the positive target behavior: What prevents focus group members currently from engaging in this behavior? What could someone say or show to them so that they would engage in this behavior? If some members of the focus groups have recently started to do the positive behavior, what got them to change in the first place?
Focus groups are also useful to determine how able, willing, and ready to change their behavior members of different potential target audiences are. Several factors contribute to individuals’ “readiness” to change their behavior. These factors include openness to acting more inclusively ( Brauer et al., in press ), internal motivation to respond without prejudice ( Plant and Devine, 1998 ), lack of discomfort interacting with members of different social groups ( Stephan, 2014 ), and general enthusiasm for diversity ( Pittinsky et al., 2011 ). Facilitators can get at these factors by asking the members of the focus group about their motivation and perceived ability to engage in the target behavior.
Climate surveys are effective because they usually provide data from a larger and thus more representative sample in a given department or college. Various techniques exist to increase the response rate of respondents (e.g., Dykema et al., 2013 ). The exact content and length of a climate survey depend on the participant population and the frequency with which the survey is administered. The online supplemental material contains two examples developed by the Wisconsin Louis Stokes Alliance for Minority Participation (WiscAMP), one for graduate students of a university department and one for all undergraduate students on a campus. Other climate surveys used in higher education and numerous relevant references can be downloaded from this web address: http://psych.wisc.edu/Brauer/BrauerLab/index.php/campaign-materials/information-resources/
All climate surveys should measure demographic information, but in smaller units, anonymity may be an issue. Once gender identity is crossed with racial/ethnic identity and occupation (e.g., postdoc vs. assistant professor vs. full professor) it may no longer be possible to protect all respondents’ anonymity. The solution is to form a small number of relatively large categories such that it is unlikely that there will be fewer than five respondents when all these categories are crossed with each other. If the analyses reveal that certain groups of respondents are too small, then the presentation of the results should be adjusted. For example, the means can be broken down once by gender identity and once by race/ethnicity, but not by gender identity and race/ethnicity.
To address the anonymity issue, we recently conducted a climate survey in which we only asked two demographic questions: “Do you identify as a man, yes or no?” and “Do you identify as a member of a marginalized group (unrelated to gender identity), yes or no?” We justified the use of these questions in the survey by explaining that the gender identity question was asked in this way because research shows that individuals who identify as men are less often the target of sexual assault than those who do not identify as men. We also provided a brief definition of “marginalized groups.”
Climate surveys have two goals. They should provide an accurate reading of respondents’ perception of the social climate and they should suggest concrete action steps about initiatives to be implemented (see Table 1 for a list of constructs that are frequently measured in climate surveys). To achieve the first goal the climate survey should contain at least one question about the overall climate and several questions about specific feelings related to the social climate. In addition, the survey should assess sense of belonging, as well as mental and physical health. Most climate surveys also include items about respondents’ experiences of discrimination and their intention to remain in the institution (sometimes referred to as “persistence”). Finally, the climate survey may assess a variety of other constructs such as respondents’ perception of the institution’s commitment to diversity, their personal values related to diversity, their level of discomfort being around people from other social groups (sometimes referred to as “intergroup anxiety”) and self-reported inclusive behaviors.
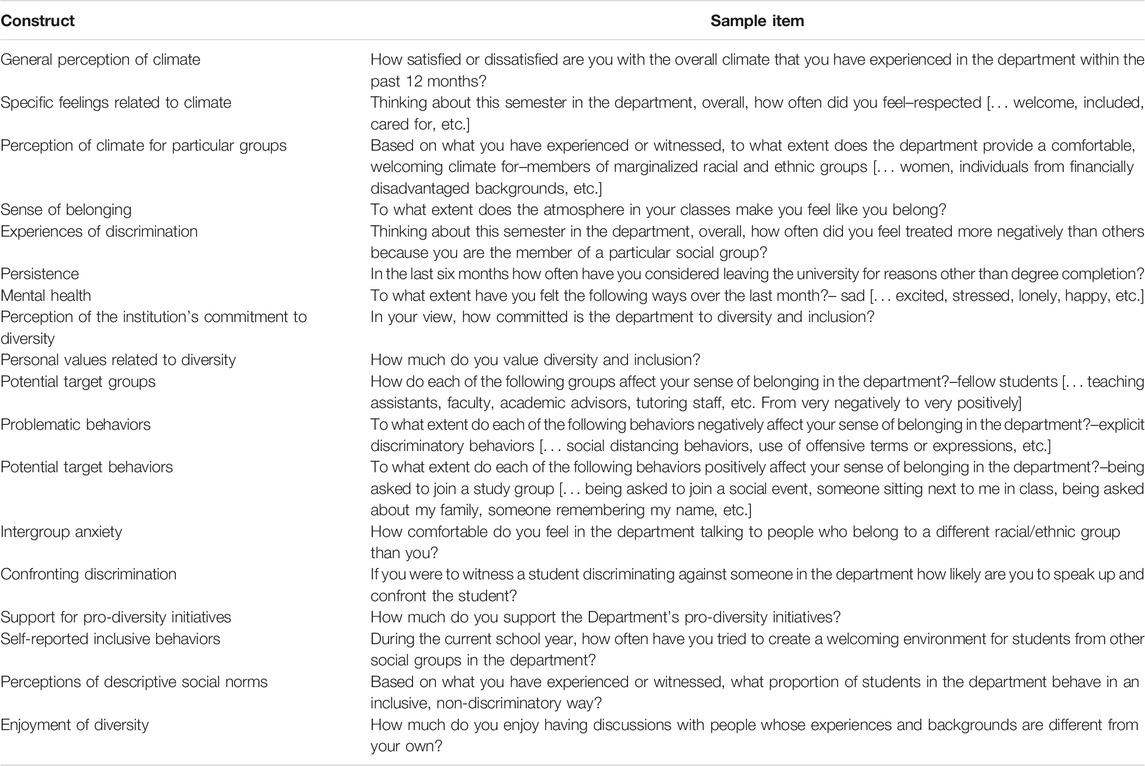
TABLE 1 . List of constructs that are frequently measured in climate surveys.
To achieve the second goal–identification of concrete action steps about initiatives to be implemented–the climate survey needs to contain questions that help identify potential target behaviors, potential target audiences, and the barriers and benefits. It is helpful to ask respondents about the groups of individuals that have the most negative impact on their experience in the Department. It is further important to get information about the behaviors that should be discouraged (behaviors that negatively affect the well-being of individuals belonging to marginalized groups) and behaviors that should be promoted in the future (behaviors that make members of marginalized groups feel welcome and included). Once these behaviors have been identified, which will likely be the case after the climate survey has been implemented once or twice in a given Department, it is even possible to include items that measure the barriers and benefits for these behaviors.
As will be described in the next section, one of the most effective ways to promote an inclusive climate is to make salient that inclusion is a social norm. People’s perceptions of social norms are determined in part by what their peers think and do, and it is thus important for a climate survey to assess how common inclusive beliefs and behaviors are (the so-called “descriptive norms”). The above-mentioned items measuring personal values related to diversity partially achieve this purpose. In addition, consider including in the climate survey items that measure respondents’ support for their department’s pro-diversity initiatives, their enjoyment of diversity, their self-reported inclusive behaviors, and their perceptions of the proportion of peers who behave in an inclusive, non-discriminatory way. The survey shown in the online Supplemental Material contains additional items that assess respondent’s perceptions of the extent to which it is “descriptively normative” to be inclusive. It can be highly effective to create persuasive messages in which the average response to these items is reported. For example, if respondents from marginalized groups answered that a numerical majority of their peers engage in inclusive behaviors and abstain from engaging in discriminatory behaviors, then obviously inclusion is a social norm. As will be explained in more detail in the next section, such “social norms messages” have been shown to promote the occurrence of inclusive behaviors and to promote a welcoming social climate, as long as is it acknowledged that acts of bigotry and exclusion still occur and it is communicated that the department or college will continue its diversity efforts until members of marginalized groups feel just as welcome and included as members of nonmarginalized groups.
Overview of Recently Developed Initiatives to Promote Inclusion
A few new approaches to promoting inclusion stand out among the rest. Rather than taking a traditional approach of reducing biased attitudes or raising awareness about persistent prejudice, many of these new initiatives focus on changing behavior. We will discuss in detail two types of interventions, one involving social norms messaging and the other promoting intergroup contact. We will also briefly describe the “pride and prejudice” approach to inclusion in academia. While only some of these initiatives have been specifically tested as ways to improve inclusion in STEM settings, all of them can easily be applied in these settings as they show promise for increasing inclusion in academic contexts.
Social Norms Messaging
Social norms influence behavior in a way that is consistent with desirable normative behavior ( McDonald and Crandall, 2015 ). Social norms messaging–persuasive messages about social norms–has recently emerged as a promising method for promoting inclusion ( Murrar et al., 2020 ). There are two main types of social norms, descriptive (i.e., what behaviors are common among a group of people) and injunctive (i.e., what is approved of among a group of people; Cialdini et al., 1990 ). Interventions that utilize messages about descriptive social norms have been used for many years and have been proven successful in a variety of areas (e.g., energy conservation, binge drinking among college students; Frey and Rogers, 2014 ; Lewis and Neighbors, 2006 ; Miller and Prentice, 2016 ). Such interventions influence behavior by changing or correcting individuals’ perceptions of their peers’ behavior, which is particularly powerful because people rely on each other and their environment for guidance on how to behave ( Rhodes et al., 2020 ).
Prejudice is often blamed on conformity to social norms ( Crandall et al., 2002 ). However, researchers have started to employ social norms messaging as a way to improve intergroup outcomes. For example, Murrar and colleagues (2020) developed two interventions that targeted peoples’ perceptions of their peers’ pro-diversity attitudes and inclusive behaviors (i.e., descriptive norms) and tested them within college classrooms. One intervention involved placing posters inside classrooms that communicated that most students at the university embrace diversity and welcome people from all backgrounds into the campus community. The other intervention consisted of a short video that portrayed interviews with students who expressed pro-diversity attitudes and intentions to behave inclusively. The video also showed interviews with diversity and inclusion experts who reported that the blatant acts of discrimination, which undoubtedly occur on campus and affect the well-being of students from marginalized groups, are perpetrated by a numerical minority of students. The interventions led to an increase in inclusive behaviors in all students, an enhanced sense of belonging among students from marginalized groups, and a reduction in the achievement gap (see Figure 2 ). Note that Murrar and colleagues’ Experiment 6 specifically examined the effectiveness of the intervention in STEM courses.
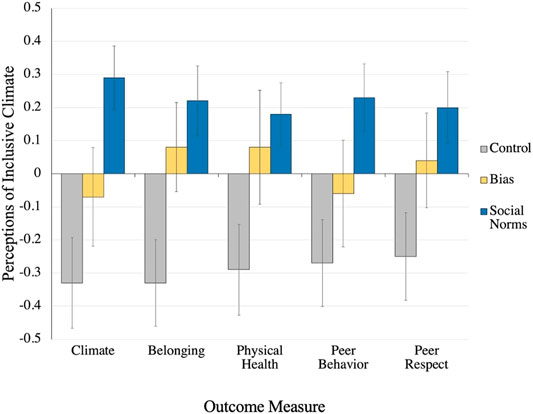
FIGURE 2 . Effect of condition on outcomes of interest for students from marginalized groups in experiment 5 of Murrar et al., 2020 . Note: The authors compared their social norms intervention to a no-exposure control group and an intervention highlighting bias.
Another intervention strategy that successfully utilized social norms messaging and improved the well-being of college students from marginalized groups was developed and tested by Brauer et al. (in press) . Using the steps to designing successful behavior interventions described earlier, these authors identified the target behavior (inclusive classroom behavior), target audience (White university students), barriers (perceptions of peer inclusive behaviors and lack of motivation to behave inclusively) and benefits (importance of working and communicating well with a diverse group of people for others and oneself) to design a theoretically informed intervention strategy: a one-page document to be included in course syllabi. The document included not only social norms messaging about students’ inclusive behaviors (descriptive norms), but also statements by the university leadership endorsing diversity (highlighting injunctive norms, Rhodes et al., 2020 ), a short text about the benefits of learning to behave inclusively (inspired by utility value interventions; Harackiewicz et al., 2016 ) and concrete behavioral recommendations (inspired by SMART goals; Wade, 2009 ). This approach of applying multiple theories in an intervention creates “theoretical synergy,” which refers to the situation where the elements of a multifaceted intervention mutually reinforce each other and thus become particularly effective ( Paluck et al., 2021 ).
Posters, videos, and syllabi documents are just a few ways through which social norms messaging can be implemented in classrooms to promote inclusive behaviors and improve the classroom climate for students belonging to marginalized groups. Social norms messaging can also be considered a cheap, easy, and flexible way for instructors to shape students’ norm perceptions of a classroom early on and establish expectations for inclusive behavior. When inclusive norms are established early, students are more likely to abide by them.
Intergroup Contact
The intergroup contact hypothesis, first proposed by Allport (1954) , has been the basis for many prejudice reduction strategies. The theory suggests that contact between members of different groups can cause prejudice reduction if there is equal status between the groups and they are in pursuit of common goals. Intergroup contact has rarely been tested as a means to promote inclusion in STEM settings, but some recent experiments involving interventions that utilize intergroup contact have shown promise in their ability to promote inclusion and reduce the occurrence of discriminatory behavior.
Described earlier in this paper, Mousa (2020) , Scacco and Warren (2018) are examples for how intergroup contact can promote inclusion in academic and non-academic settings. Similarly, Lowe (2021) randomly assigned men from different castes in India to be cricket teammates and compete against other teams. Lowe examined one to three weeks after the end of the cricket league whether intergroup contact experienced through being on a mixed-caste sports team and having opponents from different castes would affect willingness to interact with people from other castes, ingroup favoritism, and efficiency and trust in trading goods that had monetary value. Whereas collaborative contact improved the three outcomes, adversarial contact (i.e., contact through being opponents to different caste members) resulted in the opposite effects.
Lowe (2021) , Mousa (2020) , Scacco and Warren (2018) intergroup contact interventions show the importance of providing long-term intergroup interactions when trying to reduce discriminatory behavior and promote inclusive behavior. In particular, if the interactions involve being on the same teams and sharing common goals, engagement in inclusive behaviors and decision-making will be a likely outcome. Note that none of these interventions altered people’s attitudes. Attitude change is not a precondition for behavior change to occur. Classroom instructors in STEM can leverage insights from the research on intergroup contact by incorporating numerous opportunities for intergroup interaction in the classroom as well as in assignments and projects throughout the course. One easy way to achieve this goal is to form project groups randomly rather than allowing students to form groups themselves.
Pride and Prejudice
A new strategy for promoting inclusion in academia is the “Pride and Prejudice” approach, which has been created to address the complexity of marginalized identities ( Brannon and Lin, 2020 ). “Pride” refers to the acknowledgment of the history and culture of students from marginalized groups (e.g., classes, groups, and spaces dedicated to marginalized groups), whereas “prejudice” refers to initiatives that address the discrimination experienced by students from these groups. The key idea of this approach is that identity is a source for both pride and prejudice for those belonging to marginalized groups. Both supporting marginalized groups and addressing instances of prejudice are pathways to inclusion in academic settings.
Support for the “Pride and Prejudice” approach comes from Brannon and Lin (2020) analysis of demands made by students from 80 United States colleges and universities compiled in 2016 (see thedemands.org ) following a series of racial discrimination protests regarding what changes they wanted to see on their campuses ( Hartocollis and Bidgood, 2015 ). Their analysis revealed that most demands referenced pride experiences and prejudice experiences. Brannon and Lin also analyzed longitudinal data to assess for pride and prejudice experiences among college students in 27 colleges and universities and the relationships of these experiences with several intergroup outcomes. The results showed that pride and prejudice experiences impact students’ sense of belonging via ingroup and outgroup closeness. The findings suggest that to promote inclusion in academia, it may be best to create settings that support and celebrate the cultures of marginalized groups in addition to having practices in place to mitigate prejudice and discrimination toward marginalized groups.
A variety of strategies have been developed to reduce the achievement gap (e.g., self-affirmation interventions, promoting growth-mindsets, etc…). However, many of these strategies are meant to help students from marginalized students succeed in an environment that is not inclusive. Instead of placing the burden on students from marginalized groups (i.e., teaching them how to deal with the exclusion and discrimination), researchers and practitioners should shift their focus to creating inclusive academic environments. The research discussed in this article provides a framework for developing successful interventions to promote diversity and inclusion. Such an approach may hold the key to improving the experiences of individuals from marginalized groups by targeting the behaviors that can make them feel more recognized, respected, welcomed, and valued. In the long run this will be the most effective way to raise the success and graduation of students from marginalized groups in STEM.
Author Contributions
GM, NI, and MB participated in the writing and revision of the paper. MB approved the paper for submission.
Conflict of Interest
The authors declare that the research was conducted in the absence of any commercial or financial relationships that could be construed as a potential conflict of interest.
Supplementary Material
The Supplementary Material for this article can be found online at: https://www.frontiersin.org/articles/10.3389/feduc.2021.668250/full#supplementary-material
Ajzen, I., and Sheikh, S. (2013). Action versus Inaction: Anticipated Affect in the Theory of Planned Behavior. J. Appl. Soc. Psychol. 43 (1), 155–162. doi:10.1111/j.1559-1816.2012.00989.x
CrossRef Full Text | Google Scholar
Allport, G. (1954). The Nature of Prejudice . Boston, MA: Addison-Wesley.
Beaman, R., Wheldall, K., and Kemp, C. (2006). Differential Teacher Attention to Boys and Girls in the Classroom. Educ. Rev. 58, 339–366. doi:10.1080/00131910600748406
Bezrukova, K., Spell, C. S., Perry, J. L., and Jehn, K. A. (2016). A Meta-Analytical Integration of over 40 Years of Research on Diversity Training Evaluation. Psychol. Bull. 142 (11), 1227–1274. doi:10.1037/bul0000067
PubMed Abstract | CrossRef Full Text | Google Scholar
Brannon, T. N., and Lin, A. (2020). “Pride and Prejudice” Pathways to Belonging: Implications for Inclusive Diversity Practices within Mainstream Institutions. Am. Psychol. 76, 488–501. doi:10.1037/amp0000643
Brauer, M., Dumesnil, A., and Campbell, M. R. (in press). Using a Social Marketing Approach to Develop a Pro-diversity Intervention. J. Soc. Marketing.
Google Scholar
Campbell, M. R., and Brauer, M. (2020). Incorporating Social-Marketing Insights into Prejudice Research: Advancing Theory and Demonstrating Real-World Applications. Perspect. Psychol. Sci. 15, 608–629. doi:10.1177/1745691619896622
Carr, P. B., Dweck, C. S., and Pauker, K. (2012). “Prejudiced” Behavior without Prejudice? Beliefs about the Malleability of Prejudice Affect Interracial Interactions. J. Personal. Soc. Psychol. 103 (3), 452–471. doi:10.1037/a0028849
Chang, E. H., Milkman, K. L., Gromet, D. M., Rebele, R. W., Massey, C., Duckworth, A. L., et al. (2019). The Mixed Effects of Online Diversity Training. Proc. Natl. Acad. Sci. USA. 116 (16), 7778–7783. doi:10.1073/pnas.1816076116
Cheryan, S., Plaut, V. C., Davies, P. G., and Steele, C. M. (2009). Ambient Belonging: How Stereotypical Cues Impact Gender Participation in Computer Science. J. Personal. Soc. Psychol. 97 (6), 1045–1060. doi:10.1037/a0016239
Cialdini, R. B., Reno, R. R., and Kallgren, C. A. (1990). A Focus Theory of Normative Conduct: Recycling the Concept of Norms to Reduce Littering in Public Places. J. Personal. Soc. Psychol. 58, 1015–1026. doi:10.1037/0022-3514.58.6.1015
Clayton, K., Horrillo, J., and Sniderman, P. M. (2020). The Validity of the IAT and the AMP as Measures of Racial Prejudice. Available at SSRN . doi:10.2139/ssrn.3744338
Crandall, C. S., Eshleman, A., and O'Brien, L. (2002). Social Norms and the Expression and Suppression of Prejudice: The Struggle for Internalization. J. Personal. Soc. Psychol. 82, 359–378. doi:10.1037/0022-3514.82.3.359
Dobbin, F., Kalev, A., and Kelly, E. (2007). Diversity Management in Corporate America. Contexts . 6 (4), 21–27. doi:10.1525/ctx.2007.6.4.21
Dobbin, F., and Kalev, A. (2018). Why Doesn't Diversity Training Work? the Challenge for Industry and Academia. Anthropol. Now . 10 (2), 48–55. doi:10.1080/19428200.2018.1493182
Dortch, D., and Patel, C. (2017). Black Undergraduate Women and Their Sense of Belonging in STEM at Predominantly White Institutions. NASPA J. About Women Higher Education . 10 (2), 202–215. doi:10.1080/19407882.2017.1331854
Dover, T. L., Kaiser, C. R., and Major, B. (2020). Mixed Signals: The Unintended Effects of Diversity Initiatives. Soc. Issues Pol. Rev. 14 (1), 152–181. doi:10.1111/sipr.12059
Dovidio, J. F., Kawakami, K., and Gaertner, S. L. (2002). Implicit and Explicit Prejudice and Interracial Interaction. J. Personal. Soc. Psychol. 82 (1), 62–68. doi:10.1037/0022-3514.82.1.62
Dykema, J., Stevenson, J., Klein, L., Kim, Y., and Day, B. (2013). Effects of E-Mailed versus Mailed Invitations and Incentives on Response Rates, Data Quality, and Costs in a Web Survey of university Faculty. Soc. Sci. Computer Rev. 31 (3), 359–370. doi:10.1177/0894439312465254
FitzGerald, C., Martin, A., Berner, D., and Hurst, S. (2019). Interventions Designed to Reduce Implicit Prejudices and Implicit Stereotypes in Real World Contexts: A Systematic Review. BMC Psychol. 7 (1), 1–12. doi:10.1186/s40359-019-0299-7
Forscher, P. S., Lai, C. K., Axt, J. R., Ebersole, C. R., Herman, M., Devine, P. G., et al. (2019). A Meta-Analysis of Procedures to Change Implicit Measures. J. Personal. Soc. Psychol. 117 (3), 522–559. doi:10.1037/pspa0000160
French, J., Blair-Stevens, C., McVey, D., and Merritt, R. (2010). Social Marketing and Public Health: Theory and Practice . Oxford University Press
Frey, E., and Rogers, T. (2014). Persistence. Pol. Insights Behav. Brain Sci. 1 (1), 172–179. doi:10.1177/2372732214550405
Harackiewicz, J. M., Canning, E. A., Tibbetts, Y., Priniski, S. J., and Hyde, J. S. (2016). Closing Achievement Gaps with a Utility-Value Intervention: Disentangling Race and Social Class. J. Personal. Soc. Psychol. 111 (5), 745–765. doi:10.1037/pspp0000075
Hartocollis, A., and Bidgood, J. (2015). Racial Discrimination Demonstrations Spread at Universities across the U.S. The New York Times . Retrieved from https://www.nytimes.com/2015/11/12/us/racial-discrimination-protests-ignite-at-colleges-across-the-us.html .
Kotler, P., and Armstrong, G. (2001). Principles of Marketing . 9th edition. Upper Saddle River, N.J.: Prentice-Hall.
Krueger, R. (1994). Focus Groups: A Practical Guide for Applied Research . Thousand Oaks, CA: Sage Publications.
Kulik, C. T., Pepper, M. B., Roberson, L., and Parker, S. K. (2007). The Rich Get Richer: Predicting Participation in Voluntary Diversity Training. J. Organiz. Behav. 28 (6), 753–769. doi:10.1002/job.444
Kurdi, B., Seitchik, A. E., Axt, J. R., Carroll, T. J., Karapetyan, A., Kaushik, N., et al. (2019). Relationship between the Implicit Association Test and Intergroup Behavior: A Meta-Analysis. Am. Psychol. 74 (5), 569–586. doi:10.1037/amp0000364
Lai, C. K., Hoffman, K. M., and Nosek, B. A. (2013). Reducing Implicit Prejudice. Social Personal. Psychol. Compass . 7 (5), 315–330. doi:10.1111/spc3.12023
Lee, N. R., and Kotler, P. (2019). Social Marketing: Changing Behaviors for Good . Sage Publications .
Lefebvre, R. C. (2011). An Integrative Model for Social Marketing. J. Soc. Marketing . 1 (1), 54–72. doi:10.1108/20426761111104437
Lewis, M. A., and Neighbors, C. (2006). Social Norms Approaches Using Descriptive Drinking Norms Education: A Review of the Research on Personalized Normative Feedback. J. Am. Coll. Health . 54, 213–218. doi:10.3200/jach.54.4.213-218
Lowe, M. (2021). Types of Contact: A Field experiment on Collaborative and Adversarial Caste Integration. Am. Econ. Rev. 111 (6), 1807–1844. doi:10.1287/e8861e18-1b80-4134-918b-824c477abe4f
McDonald, R. I., and Crandall, C. S. (2015). Social Norms and Social Influence. Curr. Opin. Behav. Sci. 3, 147–151. doi:10.1016/j.cobeha.2015.04.006
McKenzie-Mohr, D. (2011). Fostering Sustainable Behavior: An Introduction to Community-Based Social Marketing . New society publishers .
McKenzie-Mohr, D., and Schultz, P. W. (2014). Choosing Effective Behavior Change Tools. Soc. Marketing Q. 20 (1), 35–46. doi:10.1177/1524500413519257
Miller, D. T., and Prentice, D. A. (2016). Changing Norms to Change Behavior. Annu. Rev. Psychol. 67, 339–361. doi:10.1146/annurev-psych-010814-015013
Moss-Racusin, C. A., van der Toorn, J., Dovidio, J. F., Brescoll, V. L., Graham, M. J., and Handelsman, J. (2014). Scientific Diversity Interventions. Science . 343 (6171), 615–616. doi:10.1126/science.1245936
Mousa, S. (2020). Building Social Cohesion between Christians and Muslims through Soccer in post-ISIS Iraq. Science . 369 (6505), 866–870. doi:10.1126/science.abb3153
Murphy, M. C., Gopalan, M., Carter, E. R., Emerson, K. T., Bottoms, B. L., and Walton, G. M. (2020). A Customized Belonging Intervention Improves Retention of Socially Disadvantaged Students at a Broad-Access university. Sci. Adv. 6 (29), eaba4677. doi:10.1126/sciadv.aba4677
Murrar, S., Campbell, M. R., and Brauer, M. (2020). Exposure to Peers' Pro-diversity Attitudes Increases Inclusion and Reduces the Achievement gap. Nat. Hum. Behav. 4 (9), 889–897. doi:10.1038/s41562-020-0899-5
Murrar, S., Gavac, S., and Brauer, M. (2017). Reducing Prejudice. Social Psychol. How other People influence our thoughts actions , 361–384.
National Science Foundation (2020). The State of U.S. Science and Engineering 2020. Retreived from https://ncses.nsf.gov/pubs/nsb20201 .
Noon, M. (2018). Pointless Diversity Training: Unconscious Bias, New Racism and agency. Work, Employment Soc. 32 (1), 198–209. doi:10.1177/0950017017719841
O'Keeffe, P. (2013). A Sense of Belonging: Improving Student Retention. Coll. Student J. 47 (4), 605–613.
Oswald, F. L., Mitchell, G., Blanton, H., Jaccard, J., and Tetlock, P. E. (2013). Predicting Ethnic and Racial Discrimination: a Meta-Analysis of IAT Criterion Studies. J. Personal. Soc. Psychol. 105 (2), 171–192. doi:10.1037/a0032734
Paluck, E. L., and Green, D. P. (2009). Prejudice Reduction: What Works? A Review and Assessment of Research and Practice. Annu. Rev. Psychol. 60, 339–367. doi:10.1146/annurev.psych.60.110707.163607
Paluck, E. L., Porat, R., Clark, C. S., and Green, D. P. (2021). Prejudice Reduction: Progress and Challenges. Annu. Rev. Psychol. 72. doi:10.1146/annurev-psych-071620-030619
Pittinsky, T. L., Rosenthal, S. A., and Montoya, R. M. (2011). “Measuring Positive Attitudes toward Outgroups: Development and Validation of the Allophilia Scale,” in Moving beyond prejudice reduction: Pathways to positive intergroup relations. . Editors L. R. Tropp, and R. K. Mallett American Psychological Association , 41–60. doi:10.1037/12319-002
Plant, E. A., and Devine, P. G. (1998). Internal and External Motivation to Respond without Prejudice. J. Personal. Soc. Psychol. 75 (3), 811–832. doi:10.1037/0022-3514.75.3.811
Rainey, K., Dancy, M., Mickelson, R., Stearns, E., and Moller, S. (2018). Race and Gender Differences in How Sense of Belonging Influences Decisions to Major in STEM. Int. J. STEM Education . 5 (1), 10. doi:10.1186/s40594-018-0115-6
Rhodes, N., Shulman, H. C., and McClaran, N. (2020). Changing Norms: A Meta-Analytic Integration of Research on Social Norms Appeals. Hum. Commun. Res. 46, 161–191. doi:10.1093/hcr/hqz023
Sadker, D., Sadker, M., and Zittleman, K. R. (2009). Still Failing at Fairness: How Gender Bias Cheats Girls and Boys and what We Can Do about it . Revised edition. New York: Charles Scribner.
Scacco, A., and Warren, S. S. (2018). Can Social Contact Reduce Prejudice and Discrimination? Evidence from a Field experiment in Nigeria. Am. Polit. Sci. Rev. 112 (3), 654–677. doi:10.1017/s0003055418000151
Slavin, R. E. (1990). Research on Cooperative Learning: Consensus and Controversy. Educ. Leadersh. 47 (4), 52–54.
Smith, W. A. (2006). Social Marketing: an Overview of Approach and Effects. Inj. Prev. 12 (Suppl. 1), i38–i43. doi:10.1136/ip.2006.012864
Spencer, B., and Castano, E. (2007). Social Class Is Dead. Long Live Social Class! Stereotype Threat Among Low Socioeconomic Status Individuals. Soc. Just Res. 20 (4), 418–432. doi:10.1007/s11211-007-0047-7
Stephan, W. G. (2014). Intergroup Anxiety. Pers Soc. Psychol. Rev. 18 (3), 239–255. doi:10.1177/1088868314530518
Stephens, N. M., Townsend, S. S. M., Markus, H. R., and Phillips, L. T. (2012). A Cultural Mismatch: Independent Cultural Norms Produce Greater Increases in Cortisol and More Negative Emotions Among First-Generation College Students. J. Exp. Soc. Psychol. 48 (6), 1389–1393. doi:10.1016/j.jesp.2012.07.008
Strayhorn, T. L. (2012). College Students’ Sense of Belonging: A Key to Educational success for All Students . Routledge . doi:10.4324/9780203118924
CrossRef Full Text
Wade, D. T. (2009). Goal Setting in Rehabilitation: An Overview of what, Why and How. Clin. Rehabil. 23, 291–295. doi:10.1177/0269215509103551
Walsh, G., Hassan, L. M., Shiu, E., Andrews, J. C., and Hastings, G. (2010). Segmentation in Social Marketing. Eur. J. Marketing 44 (7), 1140–1164. doi:10.1108/03090561011047562
Walton, G. M., and Cohen, G. L. (2011). A Brief Social-Belonging Intervention Improves Academic and Health Outcomes of Minority Students. Science . 331 (6023), 1447–1451. doi:10.1126/science.1198364
Walton, G. M., and Cohen, G. L. (2007). A Question of Belonging: Race, Social Fit, and Achievement. J. Personal. Soc. Psychol. 92 (1), 82–96. doi:10.1037/0022-3514.92.1.82
Walton, G. M., and Wilson, T. D. (2018). Wise Interventions: Psychological Remedies for Social and Personal Problems. Psychol. Rev. 125 (5), 617–655. doi:10.1037/rev0000115
Wicker, A. W. (1969). Attitudes versus Actions: The Relationship of Verbal and Overt Behavioral Responses to Attitude Objects. J. Soc. Issues 25 (4), 41–78. doi:10.1111/j.1540-4560.1969.tb00619.x
Wiggan, G. (2007). Race, School Achievement, and Educational Inequality: Toward a Student-Based Inquiry Perspective. Rev. Educ. Res. 77 (3), 310–333. doi:10.3102/003465430303947
Wolf, D. A. P. S., Perkins, J., Butler-Barnes, S. T., and Walker, T. A. (2017). Social Belonging and College Retention: Results from a Quasi-Experimental Pilot Study. J. Coll. Student Development . 58 (5), 777–782. doi:10.1353/csd.2017.0060
Wood, W., and Rünger, D. (2016). Psychology of Habit. Annu. Rev. Psychol. 67, 289–314. doi:10.1146/annurev-psych-122414-033417
Wymer, W. (2011). Developing More Effective Social Marketing Strategies. J. Soc. Marketing . 1 (1), 17–31. doi:10.1108/20426761111104400
Yeager, D. S., Hanselman, P., Walton, G. M., Murray, J. S., Crosnoe, R., Muller, C., et al. (2019). A National experiment Reveals where a Growth Mindset Improves Achievement. Nature . 573 (7774), 364–369. doi:10.1038/s41586-019-1466-y
Keywords: higher educaction, STEM–science technology engineering mathematics, diversity, inclusion, behavior change, intervention
Citation: Moreu G, Isenberg N and Brauer M (2021) How to Promote Diversity and Inclusion in Educational Settings: Behavior Change, Climate Surveys, and Effective Pro-Diversity Initiatives. Front. Educ. 6:668250. doi: 10.3389/feduc.2021.668250
Received: 15 February 2021; Accepted: 23 June 2021; Published: 08 July 2021.
Reviewed by:
Copyright © 2021 Moreu, Isenberg and Brauer. This is an open-access article distributed under the terms of the Creative Commons Attribution License (CC BY). The use, distribution or reproduction in other forums is permitted, provided the original author(s) and the copyright owner(s) are credited and that the original publication in this journal is cited, in accordance with accepted academic practice. No use, distribution or reproduction is permitted which does not comply with these terms.
*Correspondence: Markus Brauer, [email protected]
This article is part of the Research Topic
New Developments in Pathways Towards Diversity and Inclusion in STEM: A United States Perspective
Thank you for visiting nature.com. You are using a browser version with limited support for CSS. To obtain the best experience, we recommend you use a more up to date browser (or turn off compatibility mode in Internet Explorer). In the meantime, to ensure continued support, we are displaying the site without styles and JavaScript.
- View all journals
- Explore content
- About the journal
- Publish with us
- Sign up for alerts
- Published: 25 January 2021
Online education in the post-COVID era
- Barbara B. Lockee 1
Nature Electronics volume 4 , pages 5–6 ( 2021 ) Cite this article
137k Accesses
200 Citations
337 Altmetric
Metrics details
- Science, technology and society
The coronavirus pandemic has forced students and educators across all levels of education to rapidly adapt to online learning. The impact of this — and the developments required to make it work — could permanently change how education is delivered.
The COVID-19 pandemic has forced the world to engage in the ubiquitous use of virtual learning. And while online and distance learning has been used before to maintain continuity in education, such as in the aftermath of earthquakes 1 , the scale of the current crisis is unprecedented. Speculation has now also begun about what the lasting effects of this will be and what education may look like in the post-COVID era. For some, an immediate retreat to the traditions of the physical classroom is required. But for others, the forced shift to online education is a moment of change and a time to reimagine how education could be delivered 2 .
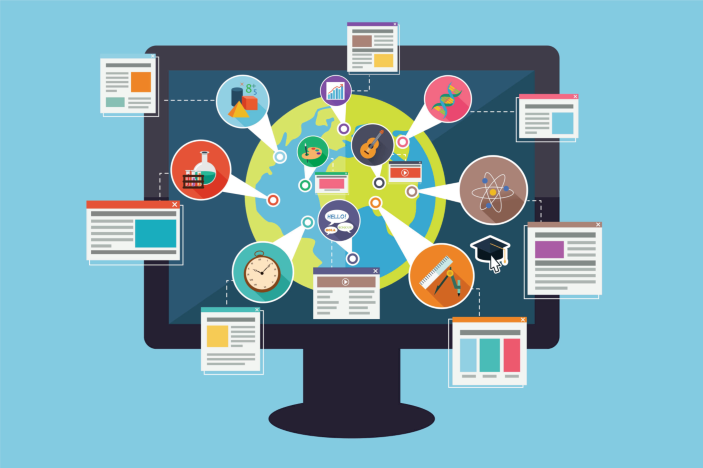
Looking back
Online education has traditionally been viewed as an alternative pathway, one that is particularly well suited to adult learners seeking higher education opportunities. However, the emergence of the COVID-19 pandemic has required educators and students across all levels of education to adapt quickly to virtual courses. (The term ‘emergency remote teaching’ was coined in the early stages of the pandemic to describe the temporary nature of this transition 3 .) In some cases, instruction shifted online, then returned to the physical classroom, and then shifted back online due to further surges in the rate of infection. In other cases, instruction was offered using a combination of remote delivery and face-to-face: that is, students can attend online or in person (referred to as the HyFlex model 4 ). In either case, instructors just had to figure out how to make it work, considering the affordances and constraints of the specific learning environment to create learning experiences that were feasible and effective.
The use of varied delivery modes does, in fact, have a long history in education. Mechanical (and then later electronic) teaching machines have provided individualized learning programmes since the 1950s and the work of B. F. Skinner 5 , who proposed using technology to walk individual learners through carefully designed sequences of instruction with immediate feedback indicating the accuracy of their response. Skinner’s notions formed the first formalized representations of programmed learning, or ‘designed’ learning experiences. Then, in the 1960s, Fred Keller developed a personalized system of instruction 6 , in which students first read assigned course materials on their own, followed by one-on-one assessment sessions with a tutor, gaining permission to move ahead only after demonstrating mastery of the instructional material. Occasional class meetings were held to discuss concepts, answer questions and provide opportunities for social interaction. A personalized system of instruction was designed on the premise that initial engagement with content could be done independently, then discussed and applied in the social context of a classroom.
These predecessors to contemporary online education leveraged key principles of instructional design — the systematic process of applying psychological principles of human learning to the creation of effective instructional solutions — to consider which methods (and their corresponding learning environments) would effectively engage students to attain the targeted learning outcomes. In other words, they considered what choices about the planning and implementation of the learning experience can lead to student success. Such early educational innovations laid the groundwork for contemporary virtual learning, which itself incorporates a variety of instructional approaches and combinations of delivery modes.
Online learning and the pandemic
Fast forward to 2020, and various further educational innovations have occurred to make the universal adoption of remote learning a possibility. One key challenge is access. Here, extensive problems remain, including the lack of Internet connectivity in some locations, especially rural ones, and the competing needs among family members for the use of home technology. However, creative solutions have emerged to provide students and families with the facilities and resources needed to engage in and successfully complete coursework 7 . For example, school buses have been used to provide mobile hotspots, and class packets have been sent by mail and instructional presentations aired on local public broadcasting stations. The year 2020 has also seen increased availability and adoption of electronic resources and activities that can now be integrated into online learning experiences. Synchronous online conferencing systems, such as Zoom and Google Meet, have allowed experts from anywhere in the world to join online classrooms 8 and have allowed presentations to be recorded for individual learners to watch at a time most convenient for them. Furthermore, the importance of hands-on, experiential learning has led to innovations such as virtual field trips and virtual labs 9 . A capacity to serve learners of all ages has thus now been effectively established, and the next generation of online education can move from an enterprise that largely serves adult learners and higher education to one that increasingly serves younger learners, in primary and secondary education and from ages 5 to 18.
The COVID-19 pandemic is also likely to have a lasting effect on lesson design. The constraints of the pandemic provided an opportunity for educators to consider new strategies to teach targeted concepts. Though rethinking of instructional approaches was forced and hurried, the experience has served as a rare chance to reconsider strategies that best facilitate learning within the affordances and constraints of the online context. In particular, greater variance in teaching and learning activities will continue to question the importance of ‘seat time’ as the standard on which educational credits are based 10 — lengthy Zoom sessions are seldom instructionally necessary and are not aligned with the psychological principles of how humans learn. Interaction is important for learning but forced interactions among students for the sake of interaction is neither motivating nor beneficial.
While the blurring of the lines between traditional and distance education has been noted for several decades 11 , the pandemic has quickly advanced the erasure of these boundaries. Less single mode, more multi-mode (and thus more educator choices) is becoming the norm due to enhanced infrastructure and developed skill sets that allow people to move across different delivery systems 12 . The well-established best practices of hybrid or blended teaching and learning 13 have served as a guide for new combinations of instructional delivery that have developed in response to the shift to virtual learning. The use of multiple delivery modes is likely to remain, and will be a feature employed with learners of all ages 14 , 15 . Future iterations of online education will no longer be bound to the traditions of single teaching modes, as educators can support pedagogical approaches from a menu of instructional delivery options, a mix that has been supported by previous generations of online educators 16 .
Also significant are the changes to how learning outcomes are determined in online settings. Many educators have altered the ways in which student achievement is measured, eliminating assignments and changing assessment strategies altogether 17 . Such alterations include determining learning through strategies that leverage the online delivery mode, such as interactive discussions, student-led teaching and the use of games to increase motivation and attention. Specific changes that are likely to continue include flexible or extended deadlines for assignment completion 18 , more student choice regarding measures of learning, and more authentic experiences that involve the meaningful application of newly learned skills and knowledge 19 , for example, team-based projects that involve multiple creative and social media tools in support of collaborative problem solving.
In response to the COVID-19 pandemic, technological and administrative systems for implementing online learning, and the infrastructure that supports its access and delivery, had to adapt quickly. While access remains a significant issue for many, extensive resources have been allocated and processes developed to connect learners with course activities and materials, to facilitate communication between instructors and students, and to manage the administration of online learning. Paths for greater access and opportunities to online education have now been forged, and there is a clear route for the next generation of adopters of online education.
Before the pandemic, the primary purpose of distance and online education was providing access to instruction for those otherwise unable to participate in a traditional, place-based academic programme. As its purpose has shifted to supporting continuity of instruction, its audience, as well as the wider learning ecosystem, has changed. It will be interesting to see which aspects of emergency remote teaching remain in the next generation of education, when the threat of COVID-19 is no longer a factor. But online education will undoubtedly find new audiences. And the flexibility and learning possibilities that have emerged from necessity are likely to shift the expectations of students and educators, diminishing further the line between classroom-based instruction and virtual learning.
Mackey, J., Gilmore, F., Dabner, N., Breeze, D. & Buckley, P. J. Online Learn. Teach. 8 , 35–48 (2012).
Google Scholar
Sands, T. & Shushok, F. The COVID-19 higher education shove. Educause Review https://go.nature.com/3o2vHbX (16 October 2020).
Hodges, C., Moore, S., Lockee, B., Trust, T. & Bond, M. A. The difference between emergency remote teaching and online learning. Educause Review https://go.nature.com/38084Lh (27 March 2020).
Beatty, B. J. (ed.) Hybrid-Flexible Course Design Ch. 1.4 https://go.nature.com/3o6Sjb2 (EdTech Books, 2019).
Skinner, B. F. Science 128 , 969–977 (1958).
Article Google Scholar
Keller, F. S. J. Appl. Behav. Anal. 1 , 79–89 (1968).
Darling-Hammond, L. et al. Restarting and Reinventing School: Learning in the Time of COVID and Beyond (Learning Policy Institute, 2020).
Fulton, C. Information Learn. Sci . 121 , 579–585 (2020).
Pennisi, E. Science 369 , 239–240 (2020).
Silva, E. & White, T. Change The Magazine Higher Learn. 47 , 68–72 (2015).
McIsaac, M. S. & Gunawardena, C. N. in Handbook of Research for Educational Communications and Technology (ed. Jonassen, D. H.) Ch. 13 (Simon & Schuster Macmillan, 1996).
Irvine, V. The landscape of merging modalities. Educause Review https://go.nature.com/2MjiBc9 (26 October 2020).
Stein, J. & Graham, C. Essentials for Blended Learning Ch. 1 (Routledge, 2020).
Maloy, R. W., Trust, T. & Edwards, S. A. Variety is the spice of remote learning. Medium https://go.nature.com/34Y1NxI (24 August 2020).
Lockee, B. J. Appl. Instructional Des . https://go.nature.com/3b0ddoC (2020).
Dunlap, J. & Lowenthal, P. Open Praxis 10 , 79–89 (2018).
Johnson, N., Veletsianos, G. & Seaman, J. Online Learn. 24 , 6–21 (2020).
Vaughan, N. D., Cleveland-Innes, M. & Garrison, D. R. Assessment in Teaching in Blended Learning Environments: Creating and Sustaining Communities of Inquiry (Athabasca Univ. Press, 2013).
Conrad, D. & Openo, J. Assessment Strategies for Online Learning: Engagement and Authenticity (Athabasca Univ. Press, 2018).
Download references
Author information
Authors and affiliations.
School of Education, Virginia Tech, Blacksburg, VA, USA
Barbara B. Lockee
You can also search for this author in PubMed Google Scholar
Corresponding author
Correspondence to Barbara B. Lockee .
Ethics declarations
Competing interests.
The author declares no competing interests.
Rights and permissions
Reprints and permissions
About this article
Cite this article.
Lockee, B.B. Online education in the post-COVID era. Nat Electron 4 , 5–6 (2021). https://doi.org/10.1038/s41928-020-00534-0
Download citation
Published : 25 January 2021
Issue Date : January 2021
DOI : https://doi.org/10.1038/s41928-020-00534-0
Share this article
Anyone you share the following link with will be able to read this content:
Sorry, a shareable link is not currently available for this article.
Provided by the Springer Nature SharedIt content-sharing initiative
This article is cited by
A comparative study on the effectiveness of online and in-class team-based learning on student performance and perceptions in virtual simulation experiments.
BMC Medical Education (2024)
Leveraging privacy profiles to empower users in the digital society
- Davide Di Ruscio
- Paola Inverardi
- Phuong T. Nguyen
Automated Software Engineering (2024)
Growth mindset and social comparison effects in a peer virtual learning environment
- Pamela Sheffler
- Cecilia S. Cheung
Social Psychology of Education (2024)
Nursing students’ learning flow, self-efficacy and satisfaction in virtual clinical simulation and clinical case seminar
- Sunghee H. Tak
BMC Nursing (2023)
Online learning for WHO priority diseases with pandemic potential: evidence from existing courses and preparing for Disease X
- Heini Utunen
- Corentin Piroux
Archives of Public Health (2023)
Quick links
- Explore articles by subject
- Guide to authors
- Editorial policies
Sign up for the Nature Briefing newsletter — what matters in science, free to your inbox daily.


An official website of the United States government
The .gov means it’s official. Federal government websites often end in .gov or .mil. Before sharing sensitive information, make sure you’re on a federal government site.
The site is secure. The https:// ensures that you are connecting to the official website and that any information you provide is encrypted and transmitted securely.
- Publications
- Account settings
Preview improvements coming to the PMC website in October 2024. Learn More or Try it out now .
- Advanced Search
- Journal List
- J Adv Med Educ Prof
- v.4(4); 2016 Oct
Effective Teaching Methods in Higher Education: Requirements and Barriers
Nahid shirani bidabadi.
1 Psychology and Educational Sciences School, University of Isfahan, Isfahan, Iran;
AHMMADREZA NASR ISFAHANI
Amir rouhollahi.
2 Department of English, Management and Information School, Isfahan University of Medical Science, Isfahan, Iran;
ROYA KHALILI
3 Quality Improvement in Clinical Education Research Center, Education Development Center, Shiraz University of Medical Sciences, Shiraz, Iran
Introduction:
Teaching is one of the main components in educational planning which is a key factor in conducting educational plans. Despite the importance of good teaching, the outcomes are far from ideal. The present qualitative study aimed to investigate effective teaching in higher education in Iran based on the experiences of best professors in the country and the best local professors of Isfahan University of Technology.
This qualitative content analysis study was conducted through purposeful sampling. Semi-structured interviews were conducted with ten faculty members (3 of them from the best professors in the country and 7 from the best local professors). Content analysis was performed by MAXQDA software. The codes, categories and themes were explored through an inductive process that began from semantic units or direct quotations to general themes.
According to the results of this study, the best teaching approach is the mixed method (student-centered together with teacher-centered) plus educational planning and previous readiness. But whenever the teachers can teach using this method confront with some barriers and requirements; some of these requirements are prerequisite in professors' behavior and some of these are prerequisite in professors’ outlook. Also, there are some major barriers, some of which are associated with the professors’ operation and others are related to laws and regulations. Implications of these findings for teachers’ preparation in education are discussed.
Conclusion:
In the present study, it was illustrated that a good teaching method helps the students to question their preconceptions, and motivates them to learn, by putting them in a situation in which they come to see themselves as the authors of answers, as the agents of responsibility for change. But training through this method has some barriers and requirements. To have an effective teaching; the faculty members of the universities should be awarded of these barriers and requirements as a way to improve teaching quality. The nationally and locally recognized professors are good leaders in providing ideas, insight, and the best strategies to educators who are passionate for effective teaching in the higher education. Finally, it is supposed that there is an important role for nationally and locally recognized professors in higher education to become more involved in the regulation of teaching rules.
Introduction
Rapid changes of modern world have caused the Higher Education System to face a great variety of challenges. Therefore, training more eager, thoughtful individuals in interdisciplinary fields is required ( 1 ). Thus, research and exploration to figure out useful and effective teaching and learning methods are one of the most important necessities of educational systems ( 2 ); Professors have a determining role in training such people in the mentioned field ( 3 ). A university is a place where new ideas germinate; roots strike and grow tall and sturdy. It is a unique space, which covers the entire universe of knowledge. It is a place where creative minds converge, interact with each other and construct visions of new realities. Established notions of truth are challenged in the pursuit of knowledge. To be able to do all this, getting help from experienced teachers can be very useful and effective.
Given the education quality, attention to students’ education as a main product that is expected from education quality system is of much greater demand in comparison to the past. There has always been emphasis on equal attention to research and teaching quality and establishing a bond between these two before making any decision; however, studies show that the already given attention to research in universities does not meet the educational quality requirements.
Attention to this task in higher education is considered as a major one, so in their instruction, educators must pay attention to learners and learning approach; along with these two factors, the educators should move forward to attain new teaching approaches. In the traditional system, instruction was teacher-centered and the students’ needs and interests were not considered. This is when students’ instruction must change into a method in which their needs are considered and as a result of the mentioned method active behavior change occurs in them ( 4 ). Moreover, a large number of graduated students especially bachelor holders do not feel ready enough to work in their related fields ( 5 ). Being dissatisfied with the status quo at any academic institution and then making decision to improve it require much research and assistance from the experts and pioneers of that institute. Giving the aforementioned are necessary, especially in present community of Iran; it seems that no qualitative study has ever been carried out in this area drawing on in-depth reports of recognized university faculties; therefore, in the present study the new global student-centered methods are firstly studied and to explore the ideas of experienced university faculties, some class observations and interviews were done. Then, efficient teaching method and its barriers and requirements were investigated because the faculty ideas about teaching method could be itemized just through a qualitative study.
The study was conducted with a qualitative method using content analysis approach. The design is appropriate for this study because it allows the participants to describe their experiences focusing on factors that may improve the quality of teaching in their own words. Key participants in purposeful sampling consist of three nationally recognized professors introduced based on the criteria of Ministry of Science, Research and Technology (based on education, research, executive and cultural qualifications) and seven other locally recognized professors according to Isfahan University of Technology standards and students votes. The purposive sampling continued until the saturation was reached, i.e. no further information was obtained for the given concept. All the participants had a teaching experience of above 10 years ( Table 1 ). They were first identified and after making appointments, they were briefed about the purpose of the study and they expressed their consent for the interview to be performed. The lack of female nationally recognized professors among respondents (due to lack of them) are restrictions of this research.
The participants’ characteristics
The data were collected using semi-structured in-depth interviews. Interviews began with general topics, such as “Talk about your experiences in effective teaching” and then the participants were asked to describe their perceptions of their expertise. Probing questions were also used to deeply explore conditions, processes, and other factors that the participants recognized as significant. The interview process was largely dependent on the questions that arose in the interaction between the interviewer and interviewees.
In the process of the study, informed consent was obtained from all the participants and they were ensured of the anonymity of their responses and that the audio files will be removed after use; then, after obtaining permission from the participants, the interview was recorded and transcribed verbatim immediately. The interviews were conducted in a private and quiet place and in convenient time. Then, verification of documents and coordination for subsequent interviews were done. The interviews lasted for one hour on average and each interview was conducted in one session with the interviewer’s notes or memos and field notes. Another method of data collection in this study was an unstructured observation in the educational setting. The investigator observed the method of interactions among faculty members and students. The interviews were conducted from November 2014 to April 2015. Each participant was interviewed for one or two sessions. The mean duration of the interviews was 60 minutes. To analyze the data, we used MAXQDA software (version 10, package series) for indexing and charting. Also, we used qualitative content analysis with a conventional approach to analyze the data. The data of the study were directly collected from the experiences of the study participants. The codes, categories and themes were explored through an inductive process, in which the researchers moved from specific to general. The consequently formulated concepts or categories were representative of the participants’ experiences. In content analysis at first, semantic units should be specified, and then the related codes should be extracted and categorized based on their similarities. Finally, in the case of having a high degree of abstraction, the themes can be determined. In the conventional approach, the use of predetermined classes is avoided and classes and their names are allowed to directly come out of the data. To do so, we read the manuscripts and listened to the recorded data for several times until an overall sense was attained. Then, the manuscript was read word by word and the codes were extracted. At the same time, the interviews were continued with other participants and coding of the texts was continued and sub-codes were categorized within the general topics. Then, the codes were classified in categories based on their similarities ( 6 ). Finally, by providing a comprehensive description about the topics, participants, data collection and analysis procedures and limitations of the study, we intend to create transferability so that other researchers clearly follow the research process taken by the researchers.
To improve the accuracy and the rigor of the findings, Lincoln and Cuba’s criteria, including credibility, dependability, conformability, and transferability, were used ( 7 ). To ensure the accuracy of the data, peer review, the researchers’ acceptability, and the long and continuing evaluation through in-depth, prolonged, and repeated interviews and the colleague’s comments must be used ( 8 ). In addition, the findings were repeatedly assessed and checked by supervisors (expert checking) ( 9 ). In this research, the researcher tried to increase the credibility of the data by keeping prolonged engagement in the process of data collection. Then, the accuracy of data analysis was confirmed by one specialist in the field of qualitative research and original codes were checked by some participants to compare the findings with the participants’ experiences. To increase the dependability and conformability of data, maximum variation was observed in the sampling. In addition, to increase the power of data transferability, adequate description of the data was provided in the study for critical review of the findings by other researchers.
Ethical considerations
The aim of the research and interview method was explained to the participants and in the process of the study, informed consent was obtained from all the participants and they were ensured of the anonymity of their responses and that audio files were removed after use. Informed consent for interview and its recording was obtained.
The mean age of faculty members in this study was 54.8 years and all of them were married. According to the results of the study, the best teaching approach was the mixed method one (student-centered with teacher-centered) plus educational planning and previous readiness. Meaning units expressed by professors were divided into 19 codes, 4 categories and 2 themes. In the present study, regarding the Effective Teaching Method in Higher Education, Requirements and Barriers, the experiences and perceptions of general practitioners were explored. As presented in Table 2 , according to data analysis, two themes containing several major categories and codes were extracted. Each code and category is described in more details below.
Examples of extracting codes, categories and themes from raw data
New teaching methods and barriers to the use of these methods
Teachers participating in this study believed that teaching and learning in higher education is a shared process, with responsibilities on both student and teacher to contribute to their success. Within this shared process, higher education must engage the students in questioning their preconceived ideas and their models of how the world works, so that they can reach a higher level of understanding. But students are not always equipped with this challenge, nor are all of them driven by a desire to understand and apply knowledge, but all too often aspire merely to survive the course, or to learn only procedurally in order to get the highest possible marks before rapidly moving on to the next subject. The best teaching helps the students to question their preconceptions, and motivates them to learn, by putting them in a situation in which their existing model does not work and in which they come to see themselves as authors of answers, as agents of responsibility for change. That means, the students need to be faced with problems which they think are important. Also, they believed that most of the developed countries are attempting to use new teaching methods, such as student-centered active methods, problem-based and project-based approaches in education. For example, the faculty number 3 said:
“In a project called EPS (European Project Semester), students come together and work on interdisciplinary issues in international teams. It is a very interesting technique to arouse interest, motivate students, and enhance their skills (Faculty member No. 3).”
The faculty number 8 noted another project-based teaching method that is used nowadays especially to promote education in software engineering and informatics is FLOSS (Free/Liber Open Source Software). In recent years, this project was used to empower the students. They will be allowed to accept the roles in a project and, therefore, deeply engage in the process of software development.
In Iran, many studies have been conducted about new teaching methods. For example, studies by Momeni Danaie ( 10 ), Noroozi ( 11 ), and Zarshenas ( 12 ), have shown various required methods of teaching. They have also concluded that pure lecture, regardless of any feedback ensuring the students learning, have lost their effectiveness. The problem-oriented approach in addition to improving communication skills among students not only increased development of critical thinking but also promoted study skills and an interest in their learning ( 12 ).
In this study, the professors noted that there are some barriers to effective teaching that are mentioned below:
As to the use of new methods of training such as problem-based methods or project-based approach, faculty members No. 4 and 9 remarked that "The need for student-centered teaching is obvious but for some reasons, such as the requirement in the teaching curriculum and the large volume of materials and resources, using these methods is not feasible completely" (Faculty member No. 9).
"If at least in the form of teacher evaluation, some questions were allocated to the use of project-based and problem-based approaches, teachers would try to use them further" (Faculty member No. 2).
The faculty members No. 6 and 7 believed that the lack of motivation in students and the lack of access to educational assistants are considered the reasons for neglecting these methods.
"I think one of the ways that can make student-centered education possible is employing educational assistants (Faculty member No. 6).”
"If each professor could attend crowded classes with two or three assistants, they could divide the class into some groups and assign more practical teamwork while they were carefully supervised (Faculty member No. 7).”
Requirements related to faculty outlook in an effective teaching
Having a successful and effective teaching that creates long-term learning on the part of the students will require certain feelings and attitudes of the teachers. These attitudes and emotions strongly influence their behavior and teaching. In this section, the attitudes of successful teachers are discussed.
Coordination with the overall organizational strategies will allow the educational system to move toward special opportunities for innovation based on the guidelines ( 13 ). The participants, 4, 3, 5 and 8 know that teaching effectively makes sense if the efforts of the professors are aligned with the goals of university.
"If faculty members know themselves as an inseparable part of the university, and proud of their employment in the university and try to promote the aim of training educated people with a high level of scientific expertise of university, it will become their goal, too. Thus, they will try as much as possible to attain this goal" (Faculty member No.9).
When a person begins to learn, according to the value of hope theory, he must feel this is an important learning and believe that he will succeed. Since the feeling of being successful will encourage individuals to learn, you should know that teachers have an important role in this sense ( 14 ). The interviewees’ number 1, 2, 3 and 10 considered factors like interest in youth, trust in ability and respect, as motivating factors for students.
Masters 7 and 8 signified that a master had a holistic and systematic view, determined the position of the teaching subject in a field or in the entire course, know general application of issues and determines them for students, and try to teach interdisciplinary topics. Interviewee No. 5 believed that: "Masters should be aware of the fact that these students are the future of the country and in addition to knowledge, they should provide them with the right attitude and vision" (Faculty member No.5).
Participants No. 2, 4 and 8 considered the faculty members’ passion to teach a lesson as responsible and believed that: "If the a teacher is interested in his field, he/she devotes more time to study the scriptures of his field and regularly updates his information; this awareness in his teaching and its influence on students is also very effective" (Faculty member No. 8).
Requirements related to the behavior and performance of faculty members in effective teaching
Teachers have to focus on mental differences, interest, and sense of belonging, emotional stability, practical experience and scientific level of students in training. Class curriculum planning includes preparation, effective transition of content, and the use of learning and evaluating teaching ( 15 ).
Given the current study subjects’ ideas, the following functional requirements for successful teaching in higher education can be proposed.
According to Choi and Pucker, the most important role of teachers is planning and controlling the educational process for students to be able to achieve a comprehensive learning ( 16 ).
"The fact that many teachers don’t have a predetermined plan on how to teach, and just collect what they should teach in a meeting is one reason for the lack of creativity in teaching" Faculty member No.4).
Klug and colleagues in an article entitled “teaching and learning in education” raise some questions and want the faculty members to ask themselves these questions regularly.
1- How to increase the students' motivation.
2- How to help students feel confident in solving problems.
3- How to teach students to plan their learning activities.
4- How to help them to carry out self-assessment at the end of each lesson.
5- How to encourage the students to motivate them for future work.
6- How I can give feedback to the students and inform them about their individual learning ( 14 ).
Every five faculty members who were interviewed cited the need to explain the lessons in plain language, give feedback to students, and explain the causes and reasons of issues.
"I always pay attention to my role as a model with regular self-assessment; I'm trying to teach this main issue to my students" (Faculty member No. 9).
Improving the quality of learning through the promotion of education, using pre-organizers and conceptual map, emphasizing the student-centered learning and developing the skills needed for employment are the strategies outlined in lifelong learning, particularly in higher education ( 17 ).
"I always give a five to ten-minute summary of the last topic to students at first; if possible, I build up the new lesson upon the previous one" (Faculty member No. 4).
The belief that creative talent is universal and it will be strengthened with appropriate programs is a piece of evidence to prove that innovative features of the programs should be attended to continually ( 18 ). Certainly, in addition to the enumerated powers, appropriate fields should be provided to design new ideas with confidence and purposeful orientation. Otherwise, in the absence of favorable conditions and lack of proper motivations, it will be difficult to apply new ideas ( 19 ). Teacher’s No. 3, 5 and 7 emphasized encouraging the students for creativity: "I always encourage the students to be creative when I teach a topic; for example, after teaching, I express some vague hints and undiscovered issues and ask them what the second move is to improve that process" (Faculty member No.3).
Senior instructors try to engage in self-management and consultation, tracking their usage of classroom management skills and developing action plans to modify their practices based on data. Through consultation, instructors work with their colleagues to collect and implement data to gauge the students’ strengths and weaknesses, and then use protocols to turn the weaknesses into strengths. The most effective teachers monitor progress and assess how their changed practices have impacted the students’ outcomes ( 20 ).
"It is important that what is taught be relevant to the students' career; however, in the future with the same information they have learned in university, they want to work in the industry of their country" (Faculty member No.1).
Skills in documenting the results of the process of teaching-learning cannot only facilitate management in terms of studying the records, but also provides easier access to up to date information ( 21 ). Faculty members No. 7 and 3 stressed the need for documenting learning experiences by faculty.
"I have a notebook in my office that I usually refer to after each class. Then, I write down every successful strategy that was highly regarded by students that day" (Faculty member No.3).
Developing a satisfactory interaction with students
To connect with students and impact their lives personally and professionally, teachers must be student-centered and demonstrate respect for their background, ideologies, beliefs, and learning styles. The best instructors use differentiated instruction, display cultural sensitivity, accentuate open communication, offer positive feedback on the students’ academic performance ( 20 ), and foster student growth by allowing them to resubmit assignments prior to assigning a grade ( 22 ).
"I pay attention to every single student in my class and every time when I see a student in class is not focused on a few consecutive sessions, I ask about his lack of focus and I help him solve his problem" (Faculty member No. 5).
The limitation in this research was little access to other nationally recognized university faculty members; also their tight schedule was among other limitations in this study that kept us several times from interviewing such faculties. To overcome such a problem, they were briefed about the importance of this study and then some appointments were set with them.
This study revealed the effective teaching methods, requirements and barriers in Iranian Higher Education. Teachers participating in this study believed that teaching and learning in higher education is a shared process, with responsibilities on both student and teacher to contribute to their success. Within this shared process, higher education must engage the students in questioning their preconceived ideas and their models of how the world works, so that they can reach a higher level of understanding. They believed that to grow successful people to deal with the challenges in evolving the society, most developed countries are attempting to use new teaching methods in higher education. All these methods are student-centered and are the result of pivotal projects. Research conducted by Momeni Danaei and colleagues also showed that using a combination of various teaching methods together will lead to more effective learning while implementing just one teaching model cannot effectively promote learning ( 10 ). However, based on the faculty member’s experiences, effective teaching methods in higher education have some requirements and barriers.
In this study, barriers according to codes were divided two major categories: professor-related barriers and regulation-related ones; for these reasons, the complete use of these methods is not possible. However, teachers who are aware of the necessity of engaging the student for a better understanding of their content try to use this method as a combination that is class speech presentation and involving students in teaching and learning. This result is consistent with the research findings of Momeni Danaei and colleagues ( 10 ), Zarshenas et al. ( 12 ) and Noroozi ( 11 ).
Using student-centered methods in higher education needs some requirements that according to faculty members who were interviewed, and according to the codes, such requirements for effective teaching can be divided into two categories: First, things to exist in the outlook of faculties about the students and faculties' responsibility towards them, to guide them towards effective teaching methods, the most important of which are adaptation to the organizational strategies, interest in the students and trust in their abilities, systemic approach in higher education, and interest in their discipline.
Second, the necessary requirements should exist in the faculties’ behavior to make their teaching methods more effective. This category emerged from some codes, including having lesson plan; using appropriate educational strategies and metacognition training and self-assessment of students during teaching; using concept and pre-organizer maps in training, knowledge; and explaining how to resolve problems in professional career through teaching discussion, documenting of experience and having satisfactory interaction with the students. This result is consistent with the findings of Klug et al., Byun et al., and Khanyfr et al. ( 14 , 17 , 18 ).
In addition and according to the results, we can conclude that a major challenge for universities, especially at a time of resource constraints, is to organize teaching so as to maximize learning effectiveness. As mentioned earlier, a major barrier to change is the fact that most faculty members are not trained for their teaching role and are largely ignorant of the research literature on effective pedagogy. These findings are in agreement with the research of Knapper, indicating that the best ideas for effective teaching include: Teaching methods that focus on the students’ activity and task performance rather than just acquisition of facts; Opportunities for meaningful personal interaction between the students and teachers; Opportunities for collaborative team learning; More authentic methods of assessment that stress task performance in naturalistic situations, preferably including elements of peer and self-assessment; Making learning processes more explicit, and encouraging the students to reflect on the way they learn; Learning tasks that encourage integration of information and skills from different fields ( 23 ).
In the present study, it was illustrated that a good teaching method helps the students to question their preconceptions, and motivates them to learn, by putting them in a situation in which they come to see themselves as the authors of answers and the agents of responsibility for change. But whenever the teachers can teach by this method, they are faced with some barriers and requirements. Some of these requirements are prerequisite of the professors' behavior and some of these are prerequisite of the professors’ outlook. Also, there are some major barriers some of which are associated with the professors’ behavior and others are related to laws and regulations. Therefore, to have an effective teaching, the faculty members of universities should be aware of these barriers and requirements as a way to improve the teaching quality.
Effective teaching also requires structural changes that can only be brought about by academic leaders. These changes include hiring practices reward structures that recognize the importance of teaching expertise, quality assurance approaches that measure learning processes, outcomes in a much more sophisticated way than routine methods, and changing the way of attaining university accreditation.
The nationally and locally recognized professors are good leaders in providing ideas, insight, and the best strategies to educators who are passionate for effective teaching in the higher education. Finally, it is supposed that there is an important role for nationally and locally recognized professors in higher education to become more involved in the regulation of teaching rules. This will help other university teachers to be familiar with effective teaching and learning procedures. Therefore, curriculum planners and faculty members can improve their teaching methods.
Acknowledgement
The authors would like to thank all research participants of Isfahan University of Technology (faculties) who contributed to this study and spent their time to share their experiences through interviews.
Conflict of Interest: None declared.
- Research article
- Open access
- Published: 24 April 2023
Artificial intelligence in higher education: the state of the field
- Helen Crompton ORCID: orcid.org/0000-0002-1775-8219 1 , 3 &
- Diane Burke 2
International Journal of Educational Technology in Higher Education volume 20 , Article number: 22 ( 2023 ) Cite this article
67k Accesses
59 Citations
59 Altmetric
Metrics details
This systematic review provides unique findings with an up-to-date examination of artificial intelligence (AI) in higher education (HE) from 2016 to 2022. Using PRISMA principles and protocol, 138 articles were identified for a full examination. Using a priori, and grounded coding, the data from the 138 articles were extracted, analyzed, and coded. The findings of this study show that in 2021 and 2022, publications rose nearly two to three times the number of previous years. With this rapid rise in the number of AIEd HE publications, new trends have emerged. The findings show that research was conducted in six of the seven continents of the world. The trend has shifted from the US to China leading in the number of publications. Another new trend is in the researcher affiliation as prior studies showed a lack of researchers from departments of education. This has now changed to be the most dominant department. Undergraduate students were the most studied students at 72%. Similar to the findings of other studies, language learning was the most common subject domain. This included writing, reading, and vocabulary acquisition. In examination of who the AIEd was intended for 72% of the studies focused on students, 17% instructors, and 11% managers. In answering the overarching question of how AIEd was used in HE, grounded coding was used. Five usage codes emerged from the data: (1) Assessment/Evaluation, (2) Predicting, (3) AI Assistant, (4) Intelligent Tutoring System (ITS), and (5) Managing Student Learning. This systematic review revealed gaps in the literature to be used as a springboard for future researchers, including new tools, such as Chat GPT.
A systematic review examining AIEd in higher education (HE) up to the end of 2022.
Unique findings in the switch from US to China in the most studies published.
A two to threefold increase in studies published in 2021 and 2022 to prior years.
AIEd was used for: Assessment/Evaluation, Predicting, AI Assistant, Intelligent Tutoring System, and Managing Student Learning.
Introduction
The use of artificial intelligence (AI) in higher education (HE) has risen quickly in the last 5 years (Chu et al., 2022 ), with a concomitant proliferation of new AI tools available. Scholars (viz., Chen et al., 2020 ; Crompton et al., 2020 , 2021 ) report on the affordances of AI to both instructors and students in HE. These benefits include the use of AI in HE to adapt instruction to the needs of different types of learners (Verdú et al., 2017 ), in providing customized prompt feedback (Dever et al., 2020 ), in developing assessments (Baykasoğlu et al., 2018 ), and predict academic success (Çağataylı & Çelebi, 2022 ). These studies help to inform educators about how artificial intelligence in education (AIEd) can be used in higher education.
Nonetheless, a gap has been highlighted by scholars (viz., Hrastinski et al., 2019 ; Zawacki-Richter et al., 2019 ) regarding an understanding of the collective affordances provided through the use of AI in HE. Therefore, the purpose of this study is to examine extant research from 2016 to 2022 to provide an up-to-date systematic review of how AI is being used in the HE context.
Artificial intelligence has become pervasive in the lives of twenty-first century citizens and is being proclaimed as a tool that can be used to enhance and advance all sectors of our lives (Górriz et al., 2020 ). The application of AI has attracted great interest in HE which is highly influenced by the development of information and communication technologies (Alajmi et al., 2020 ). AI is a tool used across subject disciplines, including language education (Liang et al., 2021 ), engineering education (Shukla et al., 2019 ), mathematics education (Hwang & Tu, 2021 ) and medical education (Winkler-Schwartz et al., 2019 ),
Artificial intelligence
The term artificial intelligence is not new. It was coined in 1956 by McCarthy (Cristianini, 2016 ) who followed up on the work of Turing (e.g., Turing, 1937 , 1950 ). Turing described the existence of intelligent reasoning and thinking that could go into intelligent machines. The definition of AI has grown and changed since 1956, as there has been significant advancements in AI capabilities. A current definition of AI is “computing systems that are able to engage in human-like processes such as learning, adapting, synthesizing, self-correction and the use of data for complex processing tasks” (Popenici et al., 2017 , p. 2). The interdisciplinary interest from scholars from linguistics, psychology, education, and neuroscience who connect AI to nomenclature, perceptions and knowledge in their own disciplines could create a challenge when defining AI. This has created the need to create categories of AI within specific disciplinary areas. This paper focuses on the category of AI in Education (AIEd) and how AI is specifically used in higher educational contexts.
As the field of AIEd is growing and changing rapidly, there is a need to increase the academic understanding of AIEd. Scholars (viz., Hrastinski et al., 2019 ; Zawacki-Richter et al., 2019 ) have drawn attention to the need to increase the understanding of the power of AIEd in educational contexts. The following section provides a summary of the previous research regarding AIEd.
Extant systematic reviews
This growing interest in AIEd has led scholars to investigate the research on the use of artificial intelligence in education. Some scholars have conducted systematic reviews to focus on a specific subject domain. For example, Liang et. al. ( 2021 ) conducted a systematic review and bibliographic analysis the roles and research foci of AI in language education. Shukla et. al. ( 2019 ) focused their longitudinal bibliometric analysis on 30 years of using AI in Engineering. Hwang and Tu ( 2021 ) conducted a bibliometric mapping analysis on the roles and trends in the use of AI in mathematics education, and Winkler-Schwartz et. al. ( 2019 ) specifically examined the use of AI in medical education in looking for best practices in the use of machine learning to assess surgical expertise. These studies provide a specific focus on the use of AIEd in HE but do not provide an understanding of AI across HE.
On a broader view of AIEd in HE, Ouyang et. al. ( 2022 ) conducted a systematic review of AIEd in online higher education and investigated the literature regarding the use of AI from 2011 to 2020. The findings show that performance prediction, resource recommendation, automatic assessment, and improvement of learning experiences are the four main functions of AI applications in online higher education. Salas-Pilco and Yang ( 2022 ) focused on AI applications in Latin American higher education. The results revealed that the main AI applications in higher education in Latin America are: (1) predictive modeling, (2) intelligent analytics, (3) assistive technology, (4) automatic content analysis, and (5) image analytics. These studies provide valuable information for the online and Latin American context but not an overarching examination of AIEd in HE.
Studies have been conducted to examine HE. Hinojo-Lucena et. al. ( 2019 ) conducted a bibliometric study on the impact of AIEd in HE. They analyzed the scientific production of AIEd HE publications indexed in Web of Science and Scopus databases from 2007 to 2017. This study revealed that most of the published document types were proceedings papers. The United States had the highest number of publications, and the most cited articles were about implementing virtual tutoring to improve learning. Chu et. al. ( 2022 ) reviewed the top 50 most cited articles on AI in HE from 1996 to 2020, revealing that predictions of students’ learning status were most frequently discussed. AI technology was most frequently applied in engineering courses, and AI technologies most often had a role in profiling and prediction. Finally, Zawacki-Richter et. al. ( 2019 ) analyzed AIEd in HE from 2007 to 2018 to reveal four primary uses of AIEd: (1) profiling and prediction, (2) assessment and evaluation, (3) adaptive systems and personalization, and (4) intelligent tutoring systems. There do not appear to be any studies examining the last 2 years of AIEd in HE, and these authors describe the rapid speed of both AI development and the use of AIEd in HE and call for further research in this area.
Purpose of the study
The purpose of this study is in response to the appeal from scholars (viz., Chu et al., 2022 ; Hinojo-Lucena et al., 2019 ; Zawacki-Richter et al., 2019 ) to research to investigate the benefits and challenges of AIEd within HE settings. As the academic knowledge of AIEd HE finished with studies examining up to 2020, this study provides the most up-to-date analysis examining research through to the end of 2022.
The overarching question for this study is: what are the trends in HE research regarding the use of AIEd? The first two questions provide contextual information, such as where the studies occurred and the disciplines AI was used in. These contextual details are important for presenting the main findings of the third question of how AI is being used in HE.
In what geographical location was the AIEd research conducted, and how has the trend in the number of publications evolved across the years?
What departments were the first authors affiliated with, and what were the academic levels and subject domains in which AIEd research was being conducted?
Who are the intended users of the AI technologies and what are the applications of AI in higher education?
A PRISMA systematic review methodology was used to answer three questions guiding this study. PRISMA principles (Page et al., 2021 ) were used throughout the study. The PRISMA extension Preferred Reporting Items for Systematic Reviews and Meta-Analysis for Protocols (PRISMA-P; Moher et al., 2015 ) were utilized in this study to provide an a priori roadmap to conduct a rigorous systematic review. Furthermore, the Preferred Reporting Items for Systematic Reviews and Meta-Analysis (PRISMA principles; Page et al., 2021 ) were used to search, identify, and select articles to be included in the research were used for searching, identifying, and selecting articles, then in how to read, extract, and manage the secondary data gathered from those studies (Moher et al., 2015 , PRISMA Statement, 2021 ). This systematic review approach supports an unbiased synthesis of the data in an impartial way (Hemingway & Brereton, 2009 ). Within the systematic review methodology, extracted data were aggregated and presented as whole numbers and percentages. A qualitative deductive and inductive coding methodology was also used to analyze extant data and generate new theories on the use of AI in HE (Gough et al., 2017 ).
The research begins with the search for the research articles to be included in the study. Based on the research question, the study parameters are defined including the search years, quality and types of publications to be included. Next, databases and journals are selected. A Boolean search is created and used for the search of those databases and journals. Once a set of publications are located from those searches, they are then examined against an inclusion and exclusion criteria to determine which studies will be included in the final study. The relevant data to match the research questions is then extracted from the final set of studies and coded. This method section is organized to describe each of these methods with full details to ensure transparency.
Search strategy
Only peer-reviewed journal articles were selected for examination in this systematic review. This ensured a level of confidence in the quality of the studies selected (Gough et al., 2017 ). The search parameters narrowed the search focus to include studies published in 2016 to 2022. This timeframe was selected to ensure the research was up to date, which is especially important with the rapid change in technology and AIEd.
The data retrieval protocol employed an electronic and a hand search. The electronic search included educational databases within EBSCOhost. Then an additional electronic search was conducted of Wiley Online Library, JSTOR, Science Direct, and Web of Science. Within each of these databases a full text search was conducted. Aligned to the research topic and questions, the Boolean search included terms related to AI, higher education, and learning. The Boolean search is listed in Table 1 . In the initial test search, the terms “machine learning” OR “intelligent support” OR “intelligent virtual reality” OR “chatbot” OR “automated tutor” OR “intelligent agent” OR “expert system” OR “neural network” OR “natural language processing” were used. These were removed as they were subcategories of terms found in Part 1 of the search. Furthermore, inclusion of these specific AI terms resulted in a large number of computer science courses that were focused on learning about AI and not the use of AI in learning.
Part 2 of the search ensured that articles involved formal university education. The terms higher education and tertiary were both used to recognize the different terms used in different countries. The final Boolean search was “Artificial intelligence” OR AI OR “smart technologies” OR “intelligent technologies” AND “higher education” OR tertiary OR graduate OR undergraduate. Scholars (viz., Ouyang et al., 2022 ) who conducted a systematic review on AIEd in HE up to 2020 noted that they missed relevant articles from their study, and other relevant journals should intentionally be examined. Therefore, a hand search was also conducted to include an examination of other journals relevant to AIEd that may not be included in the databases. This is important as the field of AIEd is still relatively new, and journals focused on this field may not yet be indexed in databases. The hand search included: The International Journal of Learning Analytics and Artificial Intelligence in Education, the International Journal of Artificial Intelligence in Education, and Computers & Education: Artificial Intelligence.
Electronic and hand searches resulted in 371 articles for possible inclusion. The search parameters within the electronic database search narrowed the search to articles published from 2016 to 2022, per-reviewed journal articles, and duplicates. Further screening was conducted manually, as each of the 138 articles were reviewed in full by two researchers to examine a match against the inclusion and exclusion criteria found in Table 2 .
The inter-rater reliability was calculated by percentage agreement (Belur et al., 2018 ). The researchers reached a 95% agreement for the coding. Further discussion of misaligned articles resulted in a 100% agreement. This screening process against inclusion and exclusion criteria resulted in the exclusion of 237 articles. This included the duplicates and those removed as part of the inclusion and exclusion criteria, see Fig. 1 . Leaving 138 articles for inclusion in this systematic review.
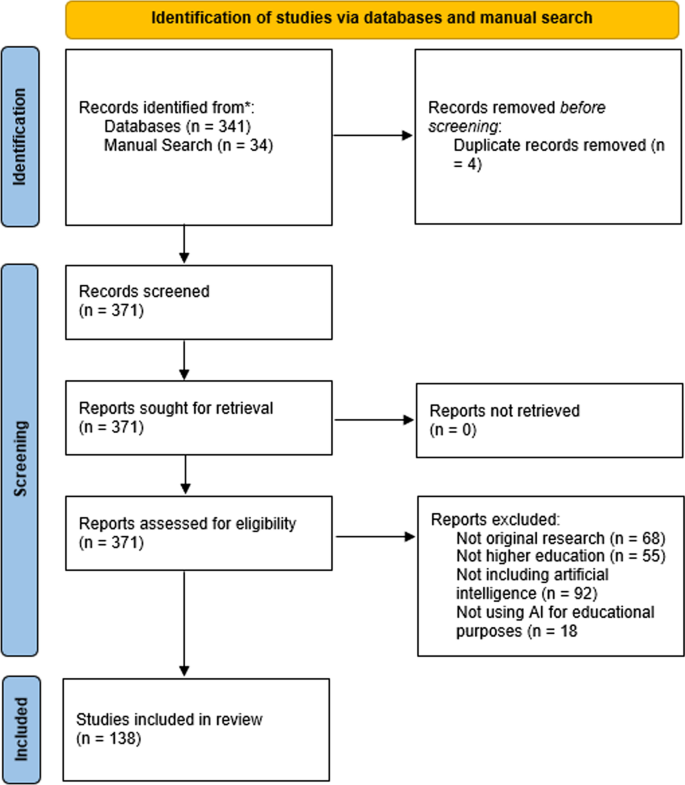
(From: Page et al., 2021 )
PRISMA flow chart of article identification and screening
The 138 articles were then coded to answer each of the research questions using deductive and inductive coding methods. Deductive coding involves examining data using a priori codes. A priori are pre-determined criteria and this process was used to code the countries, years, author affiliations, academic levels, and domains in the respective groups. Author affiliations were coded using the academic department of the first author of the study. First authors were chosen as that person is the primary researcher of the study and this follows past research practice (e.g., Zawacki-Richter et al., 2019 ). Who the AI was intended for was also coded using the a priori codes of Student, Instructor, Manager or Others. The Manager code was used for those who are involved in organizational tasks, e.g., tracking enrollment. Others was used for those not fitting the other three categories.
Inductive coding was used for the overarching question of this study in examining how the AI was being used in HE. Researchers of extant systematic reviews on AIEd in HE (viz., Chu et al., 2022 ; Zawacki-Richter et al., 2019 ) often used an a priori framework as researchers matched the use of AI to pre-existing frameworks. A grounded coding methodology (Strauss & Corbin, 1995 ) was selected for this study to allow findings of the trends on AIEd in HE to emerge from the data. This is important as it allows a direct understanding of how AI is being used rather than how researchers may think it is being used and fitting the data to pre-existing ideas.
Grounded coding process involved extracting how the AI was being used in HE from the articles. “In vivo” (Saldana, 2015 ) coding was also used alongside grounded coding. In vivo codes are when codes use language directly from the article to capture the primary authors’ language and ensure consistency with their findings. The grounded coding design used a constant comparative method. Researchers identified important text from articles related to the use of AI, and through an iterative process, initial codes led to axial codes with a constant comparison of uses of AI with uses of AI, then of uses of AI with codes, and codes with codes. Codes were deemed theoretically saturated when the majority of the data fit with one of the codes. For both the a priori and the grounded coding, two researchers coded and reached an inter-rater percentage agreement of 96%. After discussing misaligned articles, a 100% agreement was achieved.
Findings and discussion
The findings and discussion section are organized by the three questions guiding this study. The first two questions provide contextual information on the AIEd research, and the final question provides a rigorous investigation into how AI is being used in HE.
RQ1. In what geographical location was the AIEd research conducted, and how has the trend in the number of publications evolved across the years?
The 138 studies took place across 31 countries in six of seven continents of the world. Nonetheless, that distribution was not equal across continents. Asia had the largest number of AIEd studies in HE at 41%. Of the seven countries represented in Asia, 42 of the 58 studies were conducted in Taiwan and China. Europe, at 30%, was the second largest continent and had 15 countries ranging from one to eight studies a piece. North America, at 21% of the studies was the continent with the third largest number of studies, with the USA producing 21 of the 29 studies in that continent. The 21 studies from the USA places it second behind China. Only 1% of studies were conducted in South America and 2% in Africa. See Fig. 2 for a visual representation of study distribution across countries. Those continents with high numbers of studies are from high income countries and those with low numbers have a paucity of publications in low-income countries.
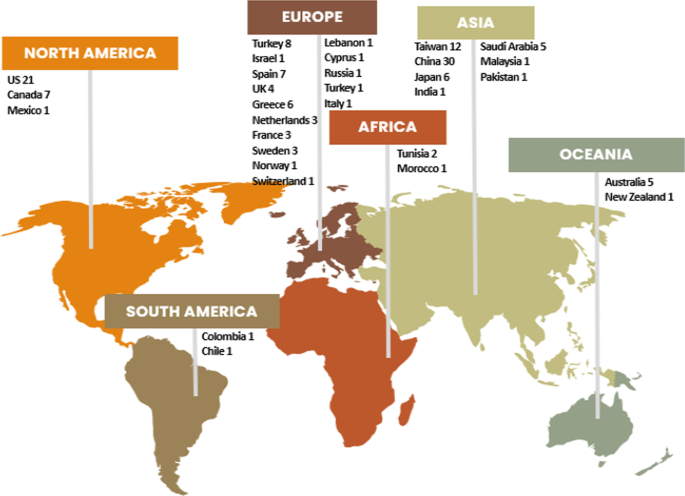
Geographical distribution of the AIEd HE studies
Data from Zawacki-Richter et. al.’s ( 2019 ) 2007–2018 systematic review examining countries found that the USA conducted the most studies across the globe at 43 out of 146, and China had the second largest at eleven of the 146 papers. Researchers have noted a rapid trend in Chinese researchers publishing more papers on AI and securing more patents than their US counterparts in a field that was originally led by the US (viz., Li et al., 2021 ). The data from this study corroborate this trend in China leading in the number of AIEd publications.
With the accelerated use of AI in society, gathering data to examine the use of AIEd in HE is useful in providing the scholarly community with specific information on that growth and if it is as prolific as anticipated by scholars (e.g., Chu et al., 2022 ). The analysis of data of the 138 studies shows that the trend towards the use of AIEd in HE has greatly increased. There is a drop in 2019, but then a great rise in 2021 and 2022; see Fig. 3 .
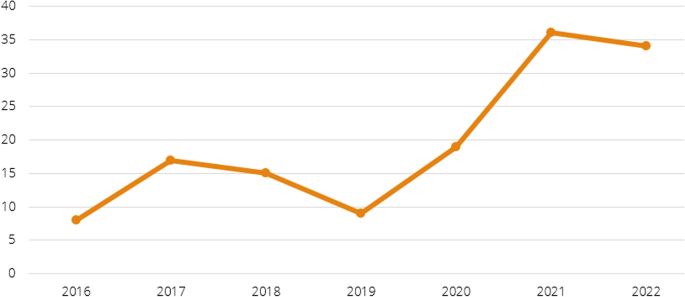
Chronological trend in AIEd in HE
Data on the rise in AIEd in HE is similar to the findings of Chu et. al. ( 2022 ) who noted an increase from 1996 to 2010 and 2011–2020. Nonetheless Chu’s parameters are across decades, and the rise is to be anticipated with a relatively new technology across a longitudinal review. Data from this study show a dramatic rise since 2020 with a 150% increase from the prior 2 years 2020–2019. The rise in 2021 and 2022 in HE could have been caused by the vast increase in HE faculty having to teach with technology during the pandemic lockdown. Faculty worldwide were using technologies, including AI, to explore how they could continue teaching and learning that was often face-to-face prior to lockdown. The disadvantage of this rapid adoption of technology is that there was little time to explore the possibilities of AI to transform learning, and AI may have been used to replicate past teaching practices, without considering new strategies previously inconceivable with the affordances of AI.
However, in a further examination of the research from 2021 to 2022, it appears that there are new strategies being considered. For example, Liu et. al.’s, 2022 study used AIEd to provide information on students’ interactions in an online environment and examine their cognitive effort. In Yao’s study in 2022, he examined the use of AI to determine student emotions while learning.
RQ2. What departments were the first authors affiliated with, and what were the academic levels and subject domains in which AIEd research was being conducted?
Department affiliations
Data from the AIEd HE studies show that of the first authors were most frequently from colleges of education (28%), followed by computer science (20%). Figure 4 presents the 15 academic affiliations of the authors found in the studies. The wide variety of affiliations demonstrate the variety of ways AI can be used in various educational disciplines, and how faculty in diverse areas, including tourism, music, and public affairs were interested in how AI can be used for educational purposes.
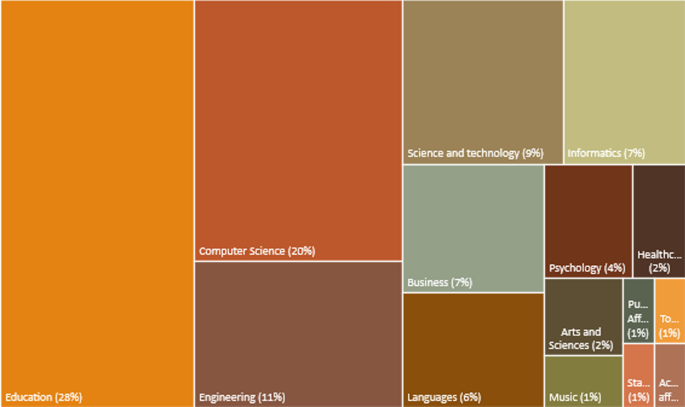
Research affiliations
In an extant AIED HE systematic review, Zawacki-Richter et. al.’s ( 2019 ) named their study Systematic review of research on artificial intelligence applications in higher education—where are the educators? In this study, the authors were keen to highlight that of the AIEd studies in HE, only six percent were written by researchers directly connected to the field of education, (i.e., from a college of education). The researchers found a great lack in pedagogical and ethical implications of implementing AI in HE and that there was a need for more educational perspectives on AI developments from educators conducting this work. It appears from our data that educators are now showing greater interest in leading these research endeavors, with the highest affiliated group belonging to education. This may again be due to the pandemic and those in the field of education needing to support faculty in other disciplines, and/or that they themselves needed to explore technologies for their own teaching during the lockdown. This may also be due to uptake in professors in education becoming familiar with AI tools also driven by a societal increased attention. As the focus of much research by education faculty is on teaching and learning, they are in an important position to be able to share their research with faculty in other disciplines regarding the potential affordances of AIEd.
Academic levels
The a priori coding of academic levels show that the majority of studies involved undergraduate students with 99 of the 138 (72%) focused on these students. This was in comparison to the 12 of 138 (9%) for graduate students. Some of the studies used AI for both academic levels: see Fig. 5
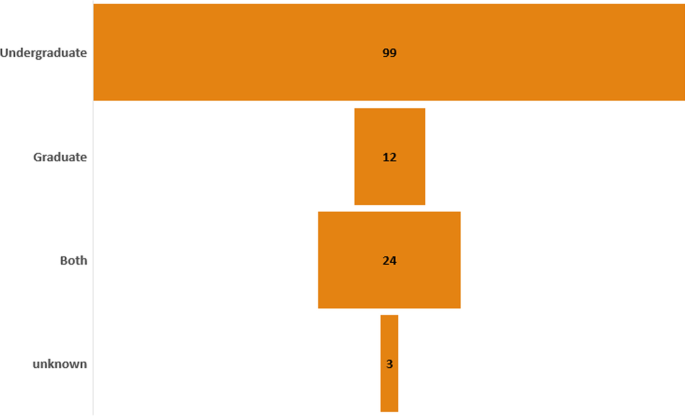
Academic level distribution by number of articles
This high percentage of studies focused on the undergraduate population was congruent with an earlier AIED HE systematic review (viz., Zawacki-Richter et al., 2019 ) who also reported student academic levels. This focus on undergraduate students may be due to the variety of affordances offered by AIEd, such as predictive analytics on dropouts and academic performance. These uses of AI may be less required for graduate students who already have a record of performance from their undergraduate years. Another reason for this demographic focus can also be convenience sampling, as researchers in HE typically has a much larger and accessible undergraduate population than graduates. This disparity between undergraduates and graduate populations is a concern, as AIEd has the potential to be valuable in both settings.
Subject domains
The studies were coded into 14 areas in HE; with 13 in a subject domain and one category of AIEd used in HE management of students; See Fig. 6 . There is not a wide difference in the percentages of top subject domains, with language learning at 17%, computer science at 16%, and engineering at 12%. The management of students category appeared third on the list at 14%. Prior studies have also found AIEd often used for language learning (viz., Crompton et al., 2021 ; Zawacki-Richter et al., 2019 ). These results are different, however, from Chu et. al.’s ( 2022 ) findings that show engineering dramatically leading with 20 of the 50 studies, with other subjects, such as language learning, appearing once or twice. This study appears to be an outlier that while the searches were conducted in similar databases, the studies only included 50 studies from 1996 to 2020.
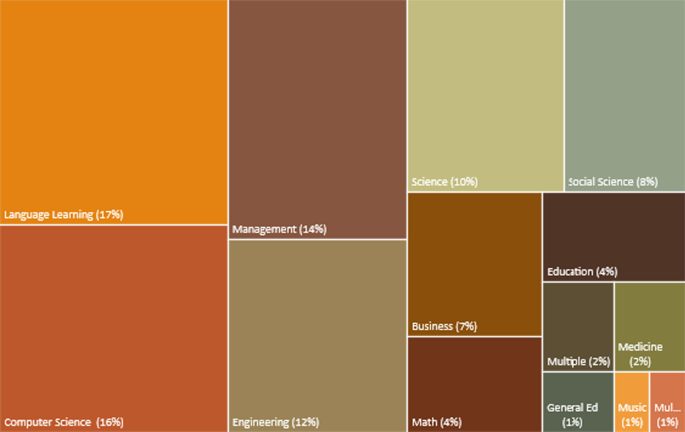
Subject domains of AIEd in HE
Previous scholars primarily focusing on language learning using AI for writing, reading, and vocabulary acquisition used the affordances of natural language processing and intelligent tutoring systems (e.g., Liang et al., 2021 ). This is similar to the findings in studies with AI used for automated feedback of writing in a foreign language (Ayse et al., 2022 ), and AI translation support (Al-Tuwayrish, 2016 ). The large use of AI for managerial activities in this systematic review focused on making predictions (12 studies) and then admissions (three studies). This is positive to see this use of AI to look across multiple databases to see trends emerging from data that may not have been anticipated and cross referenced before (Crompton et al., 2022 ). For example, to examine dropouts, researchers may consider examining class attendance, and may not examine other factors that appear unrelated. AI analysis can examine all factors and may find that dropping out is due to factors beyond class attendance.
RQ3. Who are the intended users of the AI technologies and what are the applications of AI in higher education?
Intended user of AI
Of the 138 articles, the a priori coding shows that 72% of the studies focused on Students, followed by a focus on Instructors at 17%, and Managers at 11%, see Fig. 7 . The studies provided examples of AI being used to provide support to students, such as access to learning materials for inclusive learning (Gupta & Chen, 2022 ), provide immediate answers to student questions, self-testing opportunities (Yao, 2022 ), and instant personalized feedback (Mousavi et al., 2020 ).
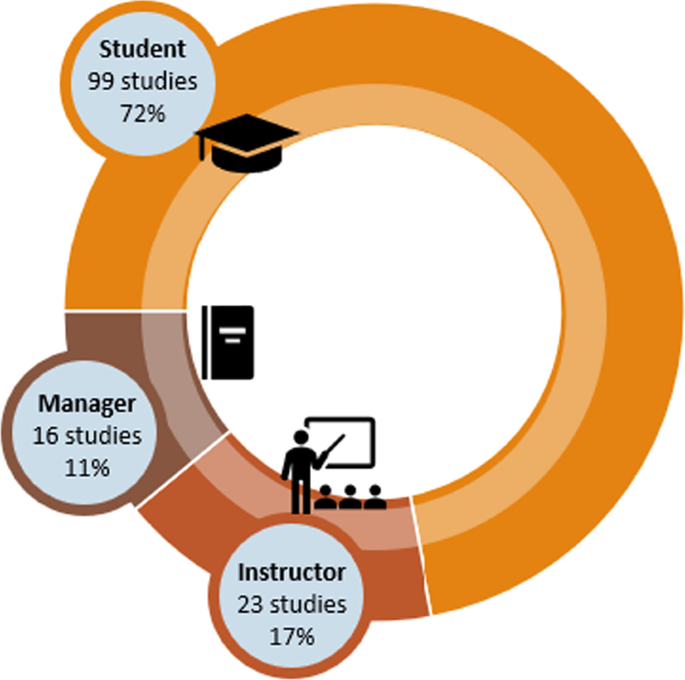
Intended user
The data revealed a large emphasis on students in the use of AIEd in HE. This user focus is different from a recent systematic review on AIEd in K-12 that found that AIEd studies in K-12 settings prioritized teachers (Crompton et al., 2022 ). This may appear that HE uses AI to focus more on students than in K-12. However, this large number of student studies in HE may be due to the student population being more easily accessibility to HE researchers who may study their own students. The ethical review process is also typically much shorter in HE than in K-12. Therefore, the data on the intended focus should be reviewed while keeping in mind these other explanations. It was interesting that Managers were the lowest focus in K-12 and also in this study in HE. AI has great potential to collect, cross reference and examine data across large datasets that can allow data to be used for actionable insight. More focus on the use of AI by managers would tap into this potential.
How is AI used in HE
Using grounded coding, the use of AIEd from each of the 138 articles was examined and six major codes emerged from the data. These codes provide insight into how AI was used in HE. The five codes are: (1) Assessment/Evaluation, (2) Predicting, (3) AI Assistant, (4) Intelligent Tutoring System (ITS), and (5) Managing Student Learning. For each of these codes there are also axial codes, which are secondary codes as subcategories from the main category. Each code is delineated below with a figure of the codes with further descriptive information and examples.
Assessment/evaluation
Assessment and Evaluation was the most common use of AIEd in HE. Within this code there were six axial codes broken down into further codes; see Fig. 8 . Automatic assessment was most common, seen in 26 of the studies. It was interesting to see that this involved assessment of academic achievement, but also other factors, such as affect.
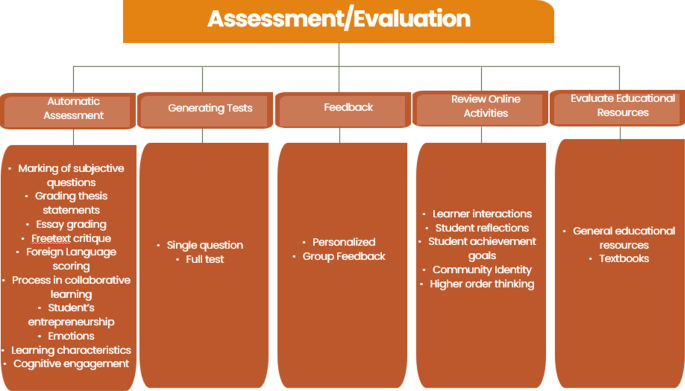
Codes and axial codes for assessment and evaluation
Automatic assessment was used to support a variety of learners in HE. As well as reducing the time it takes for instructors to grade (Rutner & Scott, 2022 ), automatic grading showed positive use for a variety of students with diverse needs. For example, Zhang and Xu ( 2022 ) used automatic assessment to improve academic writing skills of Uyghur ethnic minority students living in China. Writing has a variety of cultural nuances and in this study the students were shown to engage with the automatic assessment system behaviorally, cognitively, and affectively. This allowed the students to engage in self-regulated learning while improving their writing.
Feedback was a description often used in the studies, as students were given text and/or images as feedback as a formative evaluation. Mousavi et. al. ( 2020 ) developed a system to provide first year biology students with an automated personalized feedback system tailored to the students’ specific demographics, attributes, and academic status. With the unique feature of AIEd being able to analyze multiple data sets involving a variety of different students, AI was used to assess and provide feedback on students’ group work (viz., Ouatik et al., 2021 ).
AI also supports instructors in generating questions and creating multiple question tests (Yang et al., 2021 ). For example, (Lu et al., 2021 ) used natural language processing to create a system that automatically created tests. Following a Turing type test, researchers found that AI technologies can generate highly realistic short-answer questions. The ability for AI to develop multiple questions is a highly valuable affordance as tests can take a great deal of time to make. However, it would be important for instructors to always confirm questions provided by the AI to ensure they are correct and that they match the learning objectives for the class, especially in high value summative assessments.
The axial code within assessment and evaluation revealed that AI was used to review activities in the online space. This included evaluating student’s reflections, achievement goals, community identity, and higher order thinking (viz., Huang et al., 2021 ). Three studies used AIEd to evaluate educational materials. This included general resources and textbooks (viz., Koć‑Januchta et al., 2022 ). It is interesting to see the use of AI for the assessment of educational products, rather than educational artifacts developed by students. While this process may be very similar in nature, this shows researchers thinking beyond the traditional use of AI for assessment to provide other affordances.
Predicting was a common use of AIEd in HE with 21 studies focused specifically on the use of AI for forecasting trends in data. Ten axial codes emerged on the way AI was used to predict different topics, with nine focused on predictions regarding students and the other on predicting the future of higher education. See Fig. 9 .
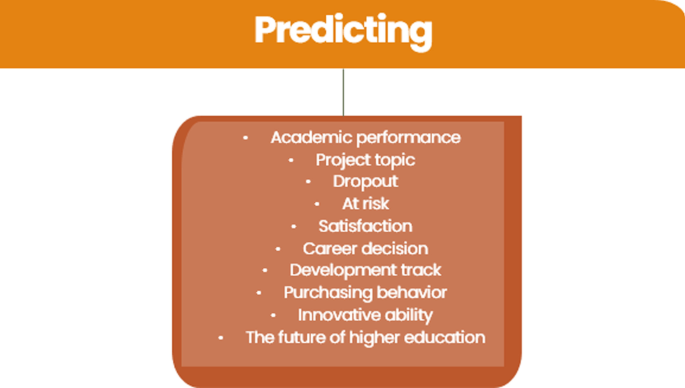
Predicting axial codes
Extant systematic reviews on HE highlighted the use of AIEd for prediction (viz., Chu et al., 2022 ; Hinojo-Lucena et al., 2019 ; Ouyang et al., 2022 ; Zawacki-Richter et al., 2019 ). Ten of the articles in this study used AI for predicting academic performance. Many of the axial codes were often overlapping, such as predicting at risk students, and predicting dropouts; however, each provided distinct affordances. An example of this is the study by Qian et. al. ( 2021 ). These researchers examined students taking a MOOC course. MOOCs can be challenging environments to determine information on individual students with the vast number of students taking the course (Krause & Lowe, 2014 ). However, Qian et al., used AIEd to predict students’ future grades by inputting 17 different learning features, including past grades, into an artificial neural network. The findings were able to predict students’ grades and highlight students at risk of dropping out of the course.
In a systematic review on AIEd within the K-12 context (viz., Crompton et al., 2022 ), prediction was less pronounced in the findings. In the K-12 setting, there was a brief mention of the use of AI in predicting student academic performance. One of the studies mentioned students at risk of dropping out, but this was immediately followed by questions about privacy concerns and describing this as “sensitive”. The use of prediction from the data in this HE systematic review cover a wide range of AI predictive affordances. students Sensitivity is still important in a HE setting, but it is positive to see the valuable insight it provides that can be used to avoid students failing in their goals.
AI assistant
The studies evaluated in this review indicated that the AI Assistant used to support learners had a variety of different names. This code included nomenclature such as, virtual assistant, virtual agent, intelligent agent, intelligent tutor, and intelligent helper. Crompton et. al. ( 2022 ), described the difference in the terms to delineate the way that the AI appeared to the user. For example, if there was an anthropomorphic presence to the AI, such as an avatar, or if the AI appeared to support via other means, such as text prompt. The findings of this systematic review align to Crompton et. al.’s ( 2022 ) descriptive differences of the AI Assistant. Furthermore, this code included studies that provide assistance to students, but may not have specifically used the word assistance. These include the use of chatbots for student outreach, answering questions, and providing other assistance. See Fig. 10 for the axial codes for AI Assistant.
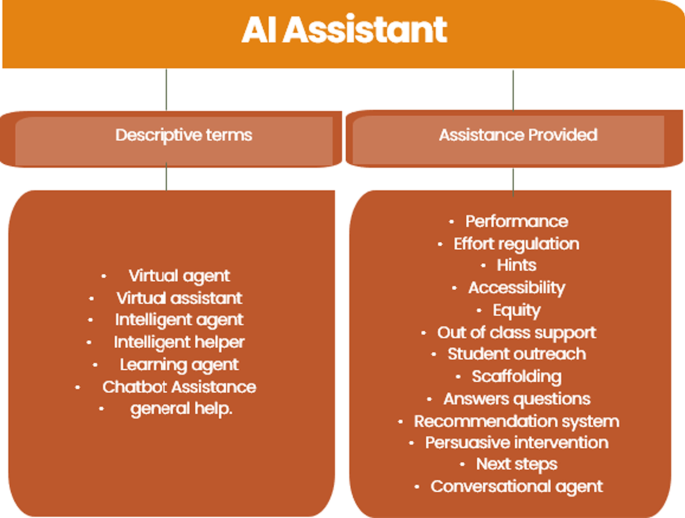
AI assistant axial codes
Many of these assistants offered multiple supports to students, such as Alex , the AI described as a virtual change agent in Kim and Bennekin’s ( 2016 ) study. Alex interacted with students in a college mathematics course by asking diagnostic questions and gave support depending on student needs. Alex’s support was organized into four stages: (1) goal initiation (“Want it”), (2) goal formation (“Plan for it”), (3) action control (“Do it”), and (4) emotion control (“Finish it”). Alex provided responses depending on which of these four areas students needed help. These messages supported students with the aim of encouraging persistence in pursuing their studies and degree programs and improving performance.
The role of AI in providing assistance connects back to the seminal work of Vygotsky ( 1978 ) and the Zone of Proximal Development (ZPD). ZPD highlights the degree to which students can rapidly develop when assisted. Vygotsky described this assistance often in the form of a person. However, with technological advancements, the use of AI assistants in these studies are providing that support for students. The affordances of AI can also ensure that the support is timely without waiting for a person to be available. Also, assistance can consider aspects on students’ academic ability, preferences, and best strategies for supporting. These features were evident in Kim and Bennekin’s ( 2016 ) study using Alex.
Intelligent tutoring system
The use of Intelligent Tutoring Systems (ITS) was revealed in the grounded coding. ITS systems are adaptive instructional systems that involve the use of AI techniques and educational methods. An ITS system customizes educational activities and strategies based on student’s characteristics and needs (Mousavinasab et al., 2021 ). While ITS may be an anticipated finding in AIED HE systematic reviews, it was interesting that extant reviews similar to this study did not always describe their use in HE. For example, Ouyang et. al. ( 2022 ), included “intelligent tutoring system” in search terms describing it as a common technique, yet ITS was not mentioned again in the paper. Zawacki-Richter et. al. ( 2019 ) on the other hand noted that ITS was in the four overarching findings of the use of AIEd in HE. Chu et. al. ( 2022 ) then used Zawacki-Richter’s four uses of AIEd for their recent systematic review.
In this systematic review, 18 studies specifically mentioned that they were using an ITS. The ITS code did not necessitate axial codes as they were performing the same type of function in HE, namely, in providing adaptive instruction to the students. For example, de Chiusole et. al. ( 2020 ) developed Stat-Knowlab, an ITS that provides the level of competence and best learning path for each student. Thus Stat-Knowlab personalizes students’ learning and provides only educational activities that the student is ready to learn. This ITS is able to monitor the evolution of the learning process as the student interacts with the system. In another study, Khalfallah and Slama ( 2018 ) built an ITS called LabTutor for engineering students. LabTutor served as an experienced instructor in enabling students to access and perform experiments on laboratory equipment while adapting to the profile of each student.
The student population in university classes can go into the hundreds and with the advent of MOOCS, class sizes can even go into the thousands. Even in small classes of 20 students, the instructor cannot physically provide immediate unique personalize questions to each student. Instructors need time to read and check answers and then take further time to provide feedback before determining what the next question should be. Working with the instructor, AIEd can provide that immediate instruction, guidance, feedback, and following questioning without delay or becoming tired. This appears to be an effective use of AIEd, especially within the HE context.
Managing student learning
Another code that emerged in the grounded coding was focused on the use of AI for managing student learning. AI is accessed to manage student learning by the administrator or instructor to provide information, organization, and data analysis. The axial codes reveal the trends in the use of AI in managing student learning; see Fig. 11 .
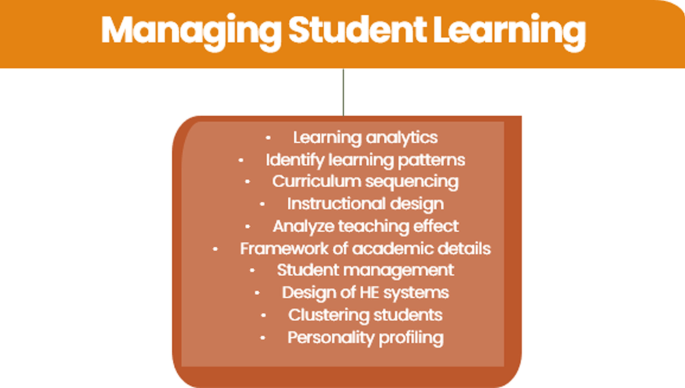
Learning analytics was an a priori term often found in studies which describes “the measurement, collection, analysis and reporting of data about learners and their contexts, for purposes of understanding and optimizing learning and the environments in which it occurs” (Long & Siemens, 2011 , p. 34). The studies investigated in this systematic review were across grades and subject areas and provided administrators and instructors different types of information to guide their work. One of those studies was conducted by Mavrikis et. al. ( 2019 ) who described learning analytics as teacher assistance tools. In their study, learning analytics were used in an exploratory learning environment with targeted visualizations supporting classroom orchestration. These visualizations, displayed as screenshots in the study, provided information such as the interactions between the students, goals achievements etc. These appear similar to infographics that are brightly colored and draw the eye quickly to pertinent information. AI is also used for other tasks, such as organizing the sequence of curriculum in pacing guides for future groups of students and also designing instruction. Zhang ( 2022 ) described how designing an AI teaching system of talent cultivation and using the digital affordances to establish a quality assurance system for practical teaching, provides new mechanisms for the design of university education systems. In developing such a system, Zhang found that the stability of the instructional design, overcame the drawbacks of traditional manual subjectivity in the instructional design.
Another trend that emerged from the studies was the use of AI to manage student big data to support learning. Ullah and Hafiz ( 2022 ) lament that using traditional methods, including non-AI digital techniques, asking the instructor to pay attention to every student’s learning progress is very difficult and that big data analysis techniques are needed. The ability to look across and within large data sets to inform instruction is a valuable affordance of AIEd in HE. While the use of AIEd to manage student learning emerged from the data, this study uncovered only 19 studies in 7 years (2016–2022) that focused on the use of AIEd to manage student data. This lack of the use was also noted in a recent study in the K-12 space (Crompton et al., 2022 ). In Chu et. al.’s ( 2022 ) study examining the top 50 most cited AIEd articles, they did not report the use of AIEd for managing student data in the top uses of AIEd HE. It would appear that more research should be conducted in this area to fully explore the possibilities of AI.
Gaps and future research
From this systematic review, six gaps emerged in the data providing opportunities for future studies to investigate and provide a fuller understanding of how AIEd can used in HE. (1) The majority of the research was conducted in high income countries revealing a paucity of research in developing countries. More research should be conducted in these developing countries to expand the level of understanding about how AI can enhance learning in under-resourced communities. (2) Almost 50% of the studies were conducted in the areas of language learning, computer science and engineering. Research conducted by members from multiple, different academic departments would help to advance the knowledge of the use of AI in more disciplines. (3) This study revealed that faculty affiliated with schools of education are taking an increasing role in researching the use of AIEd in HE. As this body of knowledge grows, faculty in Schools of Education should share their research regarding the pedagogical affordances of AI so that this knowledge can be applied by faculty across disciplines. (4) The vast majority of the research was conducted at the undergraduate level. More research needs to be done at the graduate student level, as AI provides many opportunities in this environment. (5) Little study was done regarding how AIEd can assist both instructors and managers in their roles in HE. The power of AI to assist both groups further research. (6) Finally, much of the research investigated in this systematic review revealed the use of AIEd in traditional ways that enhance or make more efficient current practices. More research needs to focus on the unexplored affordances of AIEd. As AI becomes more advanced and sophisticated, new opportunities will arise for AIEd. Researchers need to be on the forefront of these possible innovations.
In addition, empirical exploration is needed for new tools, such as ChatGPT that was available for public use at the end of 2022. With the time it takes for a peer review journal article to be published, ChatGPT did not appear in the articles for this study. What is interesting is that it could fit with a variety of the use codes found in this study, with students getting support in writing papers and instructors using Chat GPT to assess students work and with help writing emails or descriptions for students. It would be pertinent for researchers to explore Chat GPT.
Limitations
The findings of this study show a rapid increase in the number of AIEd studies published in HE. However, to ensure a level of credibility, this study only included peer review journal articles. These articles take months to publish. Therefore, conference proceedings and gray literature such as blogs and summaries may reveal further findings not explored in this study. In addition, the articles in this study were all published in English which excluded findings from research published in other languages.
In response to the call by Hinojo-Lucena et. al. ( 2019 ), Chu et. al. ( 2022 ), and Zawacki-Richter et. al. ( 2019 ), this study provides unique findings with an up-to-date examination of the use of AIEd in HE from 2016 to 2022. Past systematic reviews examined the research up to 2020. The findings of this study show that in 2021 and 2022, publications rose nearly two to three times the number of previous years. With this rapid rise in the number of AIEd HE publications, new trends have emerged.
The findings show that of the 138 studies examined, research was conducted in six of the seven continents of the world. In extant systematic reviews showed that the US led by a large margin in the number of studies published. This trend has now shifted to China. Another shift in AIEd HE is that while extant studies lamented the lack of focus on professors of education leading these studies, this systematic review found education to be the most common department affiliation with 28% and computer science coming in second at 20%. Undergraduate students were the most studied students at 72%. Similar to the findings of other studies, language learning was the most common subject domain. This included writing, reading, and vocabulary acquisition. In examination of who the AIEd was intended for, 72% of the studies focused on students, 17% instructors, and 11% managers.
Grounded coding was used to answer the overarching question of how AIEd was used in HE. Five usage codes emerged from the data: (1) Assessment/Evaluation, (2) Predicting, (3) AI Assistant, (4) Intelligent Tutoring System (ITS), and (5) Managing Student Learning. Assessment and evaluation had a wide variety of purposes, including assessing academic progress and student emotions towards learning, individual and group evaluations, and class based online community assessments. Predicting emerged as a code with ten axial codes, as AIEd predicted dropouts and at-risk students, innovative ability, and career decisions. AI Assistants were specific to supporting students in HE. These assistants included those with an anthropomorphic presence, such as virtual agents and persuasive intervention through digital programs. ITS systems were not always noted in extant systematic reviews but were specifically mentioned in 18 of the studies in this review. ITS systems in this study provided customized strategies and approaches to student’s characteristics and needs. The final code in this study highlighted the use of AI in managing student learning, including learning analytics, curriculum sequencing, instructional design, and clustering of students.
The findings of this study provide a springboard for future academics, practitioners, computer scientists, policymakers, and funders in understanding the state of the field in AIEd HE, how AI is used. It also provides actionable items to ameliorate gaps in the current understanding. As the use AIEd will only continue to grow this study can serve as a baseline for further research studies in the use of AIEd in HE.
Availability of data and materials
The datasets used and/or analysed during the current study are available from the corresponding author on reasonable request.
Alajmi, Q., Al-Sharafi, M. A., & Abuali, A. (2020). Smart learning gateways for Omani HEIs towards educational technology: Benefits, challenges and solutions. International Journal of Information Technology and Language Studies, 4 (1), 12–17.
Google Scholar
Al-Tuwayrish, R. K. (2016). An evaluative study of machine translation in the EFL scenario of Saudi Arabia. Advances in Language and Literary Studies, 7 (1), 5–10.
Ayse, T., & Nil, G. (2022). Automated feedback and teacher feedback: Writing achievement in learning English as a foreign language at a distance. The Turkish Online Journal of Distance Education, 23 (2), 120–139. https://doi.org/10.7575/aiac.alls.v.7n.1p.5
Article Google Scholar
Baykasoğlu, A., Özbel, B. K., Dudaklı, N., Subulan, K., & Şenol, M. E. (2018). Process mining based approach to performance evaluation in computer-aided examinations. Computer Applications in Engineering Education, 26 (5), 1841–1861. https://doi.org/10.1002/cae.21971
Belur, J., Tompson, L., Thornton, A., & Simon, M. (2018). Interrater reliability in systematic review methodology: Exploring variation in coder decision-making. Sociological Methods & Research, 13 (3), 004912411887999. https://doi.org/10.1177/0049124118799372
Çağataylı, M., & Çelebi, E. (2022). Estimating academic success in higher education using big five personality traits, a machine learning approach. Arab Journal Scientific Engineering, 47 , 1289–1298. https://doi.org/10.1007/s13369-021-05873-4
Chen, L., Chen, P., & Lin, Z. (2020). Artificial intelligence in education: A review. IEEE Access, 8 , 75264–75278. https://doi.org/10.1109/ACCESS.2020.2988510
Chu, H., Tu, Y., & Yang, K. (2022). Roles and research trends of artificial intelligence in higher education: A systematic review of the top 50 most-cited articles. Australasian Journal of Educational Technology, 38 (3), 22–42. https://doi.org/10.14742/ajet.7526
Cristianini, N. (2016). Intelligence reinvented. New Scientist, 232 (3097), 37–41. https://doi.org/10.1016/S0262-4079(16)31992-3
Crompton, H., Bernacki, M. L., & Greene, J. (2020). Psychological foundations of emerging technologies for teaching and learning in higher education. Current Opinion in Psychology, 36 , 101–105. https://doi.org/10.1016/j.copsyc.2020.04.011
Crompton, H., & Burke, D. (2022). Artificial intelligence in K-12 education. SN Social Sciences, 2 , 113. https://doi.org/10.1007/s43545-022-00425-5
Crompton, H., Jones, M., & Burke, D. (2022). Affordances and challenges of artificial intelligence in K-12 education: A systematic review. Journal of Research on Technology in Education . https://doi.org/10.1080/15391523.2022.2121344
Crompton, H., & Song, D. (2021). The potential of artificial intelligence in higher education. Revista Virtual Universidad Católica Del Norte, 62 , 1–4. https://doi.org/10.35575/rvuen.n62a1
de Chiusole, D., Stefanutti, L., Anselmi, P., & Robusto, E. (2020). Stat-Knowlab. Assessment and learning of statistics with competence-based knowledge space theory. International Journal of Artificial Intelligence in Education, 30 , 668–700. https://doi.org/10.1007/s40593-020-00223-1
Dever, D. A., Azevedo, R., Cloude, E. B., & Wiedbusch, M. (2020). The impact of autonomy and types of informational text presentations in game-based environments on learning: Converging multi-channel processes data and learning outcomes. International Journal of Artificial Intelligence in Education, 30 (4), 581–615. https://doi.org/10.1007/s40593-020-00215-1
Górriz, J. M., Ramírez, J., Ortíz, A., Martínez-Murcia, F. J., Segovia, F., Suckling, J., Leming, M., Zhang, Y. D., Álvarez-Sánchez, J. R., Bologna, G., Bonomini, P., Casado, F. E., Charte, D., Charte, F., Contreras, R., Cuesta-Infante, A., Duro, R. J., Fernández-Caballero, A., Fernández-Jover, E., … Ferrández, J. M. (2020). Artificial intelligence within the interplay between natural and artificial computation: Advances in data science, trends and applications. Neurocomputing, 410 , 237–270. https://doi.org/10.1016/j.neucom.2020.05.078
Gough, D., Oliver, S., & Thomas, J. (2017). An introduction to systematic reviews (2nd ed.). Sage.
Gupta, S., & Chen, Y. (2022). Supporting inclusive learning using chatbots? A chatbot-led interview study. Journal of Information Systems Education, 33 (1), 98–108.
Hemingway, P. & Brereton, N. (2009). In Hayward Medical Group (Ed.). What is a systematic review? Retrieved from http://www.medicine.ox.ac.uk/bandolier/painres/download/whatis/syst-review.pdf
Hinojo-Lucena, F., Arnaz-Diaz, I., Caceres-Reche, M., & Romero-Rodriguez, J. (2019). A bibliometric study on its impact the scientific literature. Education Science . https://doi.org/10.3390/educsci9010051
Hrastinski, S., Olofsson, A. D., Arkenback, C., Ekström, S., Ericsson, E., Fransson, G., Jaldemark, J., Ryberg, T., Öberg, L.-M., Fuentes, A., Gustafsson, U., Humble, N., Mozelius, P., Sundgren, M., & Utterberg, M. (2019). Critical imaginaries and reflections on artificial intelligence and robots in postdigital K-12 education. Postdigital Science and Education, 1 (2), 427–445. https://doi.org/10.1007/s42438-019-00046-x
Huang, C., Wu, X., Wang, X., He, T., Jiang, F., & Yu, J. (2021). Exploring the relationships between achievement goals, community identification and online collaborative reflection. Educational Technology & Society, 24 (3), 210–223.
Hwang, G. J., & Tu, Y. F. (2021). Roles and research trends of artificial intelligence in mathematics education: A bibliometric mapping analysis and systematic review. Mathematics, 9 (6), 584. https://doi.org/10.3390/math9060584
Khalfallah, J., & Slama, J. B. H. (2018). The effect of emotional analysis on the improvement of experimental e-learning systems. Computer Applications in Engineering Education, 27 (2), 303–318. https://doi.org/10.1002/cae.22075
Kim, C., & Bennekin, K. N. (2016). The effectiveness of volition support (VoS) in promoting students’ effort regulation and performance in an online mathematics course. Instructional Science, 44 , 359–377. https://doi.org/10.1007/s11251-015-9366-5
Koć-Januchta, M. M., Schönborn, K. J., Roehrig, C., Chaudhri, V. K., Tibell, L. A. E., & Heller, C. (2022). “Connecting concepts helps put main ideas together”: Cognitive load and usability in learning biology with an AI-enriched textbook. International Journal of Educational Technology in Higher Education, 19 (11), 11. https://doi.org/10.1186/s41239-021-00317-3
Krause, S. D., & Lowe, C. (2014). Invasion of the MOOCs: The promise and perils of massive open online courses . Parlor Press.
Li, D., Tong, T. W., & Xiao, Y. (2021). Is China emerging as the global leader in AI? Harvard Business Review. https://hbr.org/2021/02/is-china-emerging-as-the-global-leader-in-ai
Liang, J. C., Hwang, G. J., Chen, M. R. A., & Darmawansah, D. (2021). Roles and research foci of artificial intelligence in language education: An integrated bibliographic analysis and systematic review approach. Interactive Learning Environments . https://doi.org/10.1080/10494820.2021.1958348
Liu, S., Hu, T., Chai, H., Su, Z., & Peng, X. (2022). Learners’ interaction patterns in asynchronous online discussions: An integration of the social and cognitive interactions. British Journal of Educational Technology, 53 (1), 23–40. https://doi.org/10.1111/bjet.13147
Long, P., & Siemens, G. (2011). Penetrating the fog: Analytics in learning and education. Educause Review, 46 (5), 31–40.
Lu, O. H. T., Huang, A. Y. Q., Tsai, D. C. L., & Yang, S. J. H. (2021). Expert-authored and machine-generated short-answer questions for assessing students learning performance. Educational Technology & Society, 24 (3), 159–173.
Mavrikis, M., Geraniou, E., Santos, S. G., & Poulovassilis, A. (2019). Intelligent analysis and data visualization for teacher assistance tools: The case of exploratory learning. British Journal of Educational Technology, 50 (6), 2920–2942. https://doi.org/10.1111/bjet.12876
Moher, D., Shamseer, L., Clarke, M., Ghersi, D., Liberati, A., Petticrew, M., Shekelle, P., & Stewart, L. (2015). Preferred reporting items for systematic review and meta-analysis protocols (PRISMA-P) 2015 statement. Systematic Reviews, 4 (1), 1–9. https://doi.org/10.1186/2046-4053-4-1
Mousavi, A., Schmidt, M., Squires, V., & Wilson, K. (2020). Assessing the effectiveness of student advice recommender agent (SARA): The case of automated personalized feedback. International Journal of Artificial Intelligence in Education, 31 (2), 603–621. https://doi.org/10.1007/s40593-020-00210-6
Mousavinasab, E., Zarifsanaiey, N., Kalhori, S. R. N., Rakhshan, M., Keikha, L., & Saeedi, M. G. (2021). Intelligent tutoring systems: A systematic review of characteristics, applications, and evaluation methods. Interactive Learning Environments, 29 (1), 142–163. https://doi.org/10.1080/10494820.2018.1558257
Ouatik, F., Ouatikb, F., Fadlic, H., Elgoraria, A., Mohadabb, M. E. L., Raoufia, M., et al. (2021). E-Learning & decision making system for automate students assessment using remote laboratory and machine learning. Journal of E-Learning and Knowledge Society, 17 (1), 90–100. https://doi.org/10.20368/1971-8829/1135285
Ouyang, F., Zheng, L., & Jiao, P. (2022). Artificial intelligence in online higher education: A systematic review of empirical research from 2011–2020. Education and Information Technologies, 27 , 7893–7925. https://doi.org/10.1007/s10639-022-10925-9
Page, M. J., McKenzie, J. E., Bossuyt, P. M., Boutron, I., Hoffmann, T., Mulrow, C., Shamseer, L., Tetzlaff, J. M., Akl, E. A., Brennan, S. E., Chou, R., Glanville, J., Grimshaw, J. M., Hróbjartsson, A., Lalu, M. M., Li, T., Loder, E. W., Mayo-Wilson, E., McDonald, S., … Moher, D. (2021). The PRISMA 2020 statement: An updated guideline for reporting systematic reviews. British Medical Journal . https://doi.org/10.1136/bmj.n71
Popenici, S. A. D., & Kerr, S. (2017). Exploring the impact of artificial intelligence on teaching and learning in higher education. Research and Practice in Technology Enhanced Learning, 12 (22), 1–13. https://doi.org/10.1186/s41039-017-0062-8
PRISMA Statement. (2021). PRISMA endorsers. PRISMA statement website. http://www.prisma-statement.org/Endorsement/PRISMAEndorsers
Qian, Y., Li, C.-X., Zou, X.-G., Feng, X.-B., Xiao, M.-H., & Ding, Y.-Q. (2022). Research on predicting learning achievement in a flipped classroom based on MOOCs by big data analysis. Computer Applied Applications in Engineering Education, 30 , 222–234. https://doi.org/10.1002/cae.22452
Rutner, S. M., & Scott, R. A. (2022). Use of artificial intelligence to grade student discussion boards: An exploratory study. Information Systems Education Journal, 20 (4), 4–18.
Salas-Pilco, S., & Yang, Y. (2022). Artificial Intelligence application in Latin America higher education: A systematic review. International Journal of Educational Technology in Higher Education, 19 (21), 1–20. https://doi.org/10.1186/S41239-022-00326-w
Saldana, J. (2015). The coding manual for qualitative researchers (3rd ed.). Sage.
Shukla, A. K., Janmaijaya, M., Abraham, A., & Muhuri, P. K. (2019). Engineering applications of artificial intelligence: A bibliometric analysis of 30 years (1988–2018). Engineering Applications of Artificial Intelligence, 85 , 517–532. https://doi.org/10.1016/j.engappai.2019.06.010
Strauss, A., & Corbin, J. (1995). Grounded theory methodology: An overview. In N. K. Denzin & Y. S. Lincoln (Eds.), Handbook of qualitative research (pp. 273–285). Sage.
Turing, A. M. (1937). On computable numbers, with an application to the Entscheidungs problem. Proceedings of the London Mathematical Society, 2 (1), 230–265.
Turing, A. M. (1950). Computing machinery and intelligence. Mind, 59 , 443–460.
MathSciNet Google Scholar
Ullah, H., & Hafiz, M. A. (2022). Exploring effective classroom management strategies in secondary schools of Punjab. Journal of the Research Society of Pakistan, 59 (1), 76.
Verdú, E., Regueras, L. M., Gal, E., et al. (2017). Integration of an intelligent tutoring system in a course of computer network design. Educational Technology Research and Development, 65 , 653–677. https://doi.org/10.1007/s11423-016-9503-0
Vygotsky, L. S. (1978). Mind and society: The development of higher psychological processes . Harvard University Press.
Winkler-Schwartz, A., Bissonnette, V., Mirchi, N., Ponnudurai, N., Yilmaz, R., Ledwos, N., Siyar, S., Azarnoush, H., Karlik, B., & Del Maestro, R. F. (2019). Artificial intelligence in medical education: Best practices using machine learning to assess surgical expertise in virtual reality simulation. Journal of Surgical Education, 76 (6), 1681–1690. https://doi.org/10.1016/j.jsurg.2019.05.015
Yang, A. C. M., Chen, I. Y. L., Flanagan, B., & Ogata, H. (2021). Automatic generation of cloze items for repeated testing to improve reading comprehension. Educational Technology & Society, 24 (3), 147–158.
Yao, X. (2022). Design and research of artificial intelligence in multimedia intelligent question answering system and self-test system. Advances in Multimedia . https://doi.org/10.1155/2022/2156111
Zawacki-Richter, O., Marín, V. I., Bond, M., & Gouverneur, F. (2019). Systematic review of research on artificial intelligence applications in higher education—Where are the educators? International Journal of Educational Technology in Higher Education, 16 (1), 1–27. https://doi.org/10.1186/s41239-019-0171-0
Zhang, F. (2022). Design and application of artificial intelligence technology-driven education and teaching system in universities. Computational and Mathematical Methods in Medicine . https://doi.org/10.1155/2022/8503239
Zhang, Z., & Xu, L. (2022). Student engagement with automated feedback on academic writing: A study on Uyghur ethnic minority students in China. Journal of Multilingual and Multicultural Development . https://doi.org/10.1080/01434632.2022.2102175
Download references
Acknowledgements
The authors would like to thank Mildred Jones, Katherina Nako, Yaser Sendi, and Ricardo Randall for data gathering and organization.
Author information
Authors and affiliations.
Department of Teaching and Learning, Old Dominion University, Norfolk, USA
Helen Crompton
ODUGlobal, Norfolk, USA
Diane Burke
RIDIL, ODUGlobal, Norfolk, USA
You can also search for this author in PubMed Google Scholar
Contributions
HC: Conceptualization; Data curation; Project administration; Formal analysis; Methodology; Project administration; original draft; and review & editing. DB: Conceptualization; Data curation; Project administration; Formal analysis; Methodology; Project administration; original draft; and review & editing. Both authors read and approved this manuscript.
Corresponding author
Correspondence to Helen Crompton .
Ethics declarations
Competing interests.
The authors declare that they have no competing interests.
Additional information
Publisher's note.
Springer Nature remains neutral with regard to jurisdictional claims in published maps and institutional affiliations.
Rights and permissions
Open Access This article is licensed under a Creative Commons Attribution 4.0 International License, which permits use, sharing, adaptation, distribution and reproduction in any medium or format, as long as you give appropriate credit to the original author(s) and the source, provide a link to the Creative Commons licence, and indicate if changes were made. The images or other third party material in this article are included in the article's Creative Commons licence, unless indicated otherwise in a credit line to the material. If material is not included in the article's Creative Commons licence and your intended use is not permitted by statutory regulation or exceeds the permitted use, you will need to obtain permission directly from the copyright holder. To view a copy of this licence, visit http://creativecommons.org/licenses/by/4.0/ .
Reprints and permissions
About this article
Cite this article.
Crompton, H., Burke, D. Artificial intelligence in higher education: the state of the field. Int J Educ Technol High Educ 20 , 22 (2023). https://doi.org/10.1186/s41239-023-00392-8
Download citation
Received : 30 January 2023
Accepted : 23 March 2023
Published : 24 April 2023
DOI : https://doi.org/10.1186/s41239-023-00392-8
Share this article
Anyone you share the following link with will be able to read this content:
Sorry, a shareable link is not currently available for this article.
Provided by the Springer Nature SharedIt content-sharing initiative
- Artificial Intelligence
- Systematic review
- Higher education
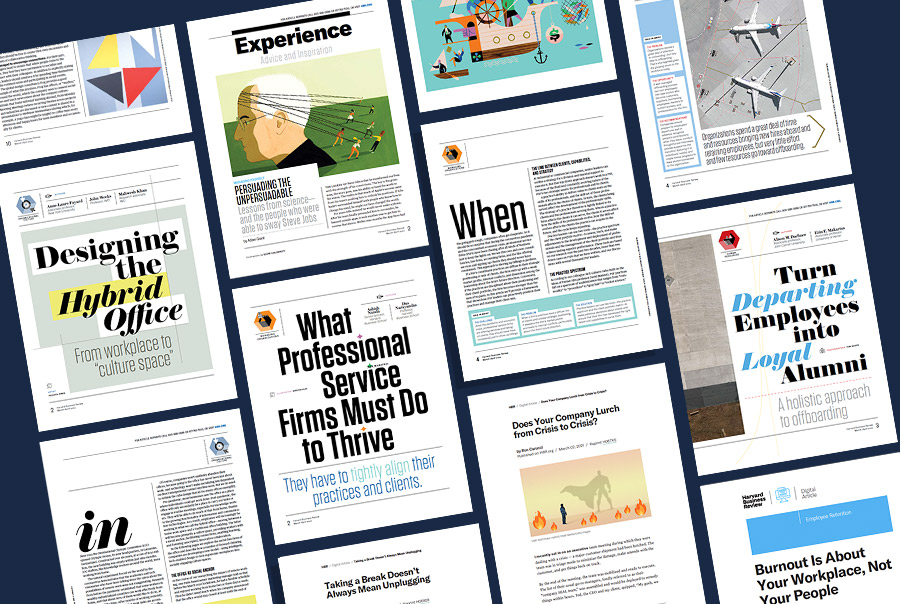
Introduce, explain, and elaborate on essential business topics with cutting-edge management articles.
Get timely and topical insights
New articles that touch upon pressing issues are added to the catalog daily.
Sourced from leading publications
Articles in our catalog come from diverse and global sources and authors.
Cover a variety of topics
Highlight leading insights and trends in the areas of strategy, leadership, innovation, and beyond.
Search Articles in Your Discipline
Select a discipline and start browsing available articles
- Business & Government Relations
- Business Ethics
- Entrepreneurship
- General Management
- Human Resource Management
- Information Technology
- International Business
- Negotiation
- Operations Management
- Organizational Behavior
- Service Management
- Social Enterprise
Discover New Articles
Stay up to date on the latest articles.
2974 word count
1080 word count
3274 word count

3261 word count
1135 word count
3742 word count
3547 word count
1145 word count
2061 word count
3259 word count
4607 word count
3011 word count
Many of my students specifically mentioned this article as a ‘key takeaway’ from the class. Review of "Leading Change: Why Transformation Efforts Fail"
Best article that clarifies how sustainability can be embedded in strategy. Review of "Creating Shared Value"
This article offers a complete perspective on persuasion that is not explored in other material. Review of "Harnessing the Science of Persuasion"
We use cookies to understand how you use our site and to improve your experience, including personalizing content. Learn More . By continuing to use our site, you accept our use of cookies and revised Privacy Policy .
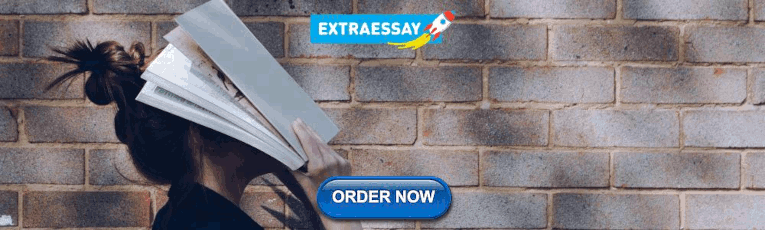
IMAGES
COMMENTS
0195-6744. 1549-6511. 2.5. Ranked #123 out of 269 "Education & Educational Research" journals. 3.3. Ranked #414 out of 1,469 "Education" journals. The American Journal of Education seeks to bridge and integrate the intellectual, methodological, and substantive diversity of educational scholarship and to encourage a vigorous dialogue ...
Description. The Harvard Educational Review (HER) is a scholarly journal of opinion and research in education. The Editorial Board aims to publish pieces from interdisciplinary and wide-ranging fields that advance our understanding of educational theory, equity, and practice. HER encourages submissions from established and emerging scholars, as ...
The Review of Higher Education (RHE) is considered one of the leading research journals in the field as it keeps scholars, academic leaders, and public policymakers abreast of critical issues facing higher education today.RHE advances the study of college and university issues by publishing peer-reviewed empirical research studies, empirically based historical and theoretical articles, and ...
'public' and 'private' in higher education from the perspective of (1) education providers and (2) undergraduate students. A comprehensive search of the literature selected 60 peer-reviewed journal articles and twenty-five books published between 2000 and 2016. Nine
Bo W (2009) Education as both Inculcation and emancipation [Online] Institute of Social Economy and Culture 1-6, Peking University Education Review. ... Journal of the Knowledge Economy (2024)
Universities and Gentrification: The Effects of Anchor Institution Initiatives on Rates of Neighborhood Change. Paul M. Garton. OriginalPaper 22 March 2023 Pages: 987 - 1010. 1. 2. …. 45. Next. Research in Higher Education is a journal that publishes empirical research on postsecondary education.
Research in Education provides a space for fully peer-reviewed, critical, trans-disciplinary, debates on theory, policy and practice in relation to Education. International in scope, we publish challenging, well-written and theoretically innovative contributions that question and explore the concept, practice and institution of Education as an object of study.
Introduction. Neoliberal policies typically understand universities as key drivers for developing infrastructures for the knowledge economy. Indeed, higher education (HE) is often deemed 'an input-output system which can be reduced to an economic production function' (Olssen & Peters, Citation 2005, p. 324).Such assumptions have serious implications for teaching and learning practices as ...
In this article, I examine the educational purposes of higher education in terms of the societal outcomes of educating students through higher education. Based on an analysis of the first 80 volume of Higher Education, published from 1972 to 2020, I argue that discussions of societal educational purposes were dominated by authors from the Anglophone, global North and these authors were more ...
Journal of Education. The oldest educational publication in the country, the Journal of Education's mission is to disseminate knowledge that informs practice in PK-12, higher, and professional education. A refereed publication, the Journal offers … | View full journal description. This journal is a member of the Committee on Publication ...
Academics from one Australian university were invited to participate in an academic development program focused on quality teaching during the teaching terms of Semester 2 2019 and Semester 1 2020. The program was promoted to academic staff via university-wide e-newsletters, staff meetings, and messaging from Heads of School.
Background. Concerns over student mental health have received increasing attention from within and beyond the Higher Education (HE) sector in recent years (OfS Citation 2019; OnS Citation 2018); and have led to a range of cross-sector policy developments (Universities UK Citation 2020) and a University Mental Health Charter in the United Kingdom (Hughes and Spanner Citation 2019).
This search tool was completed with the filters: articles in journals, university level education, peer review, complete text available, English language and time range from 2012 to 2021. ... both in the area of university education and in the pedagogic and didactic research of teaching methods—this was obtained via Google Scholar and ...
Student engagement is an important factor for learning outcomes in higher education. Engagement with learning at campus-based higher education institutions is difficult to quantify due to the variety of forms that engagement might take (e.g. lecture attendance, self-study, usage of online/digital systems). Meanwhile, there are increasing concerns about student wellbeing within higher education ...
European Journal of Psychology of Education, 28(3), 641-662. Article Google Scholar De Clercq, M., Galand, B., & Frenay, M. (2017). Transition from high school to university: a person-centered approach to academic achievement. European Journal of Psychology of Education, 32, 39-59.
Welcome to the Journal of Education. As the oldest educational publication in the country, the Journal has served many purposes in its long history. Our current mission is to disseminate knowledge that informs practice in PK-12, higher, and professional education. A refereed publication, the Journal offers three issues each calendar year.
An additional step was followed to analyze the articles: (1) mapping the factors based on access or equity and categorizing the factors as either having a positive or negative influence on access and equity in HE; (2) categorizing the factors in terms of organizational level (national, university, education before university, and student); (3 ...
Johanna E. Nieuwoudt, PhD, is an experienced lecturer at Southern Cross University, where she helps students from diverse backgrounds and experiences gain skills and confidence to be successful in their future university studies.She specialises in the curriculum design and delivery of higher education pathway courses for students in high school (Year 12), pre-award programs, and diplomas.
We review recent developments in the literature on diversity and inclusion in higher education settings. Diversity interventions increasingly focus on changing behaviors rather than mental constructs such as bias or attitudes. Additionally, there is now a greater emphasis on the evaluation of initiatives aimed at creating an inclusive climate. When trying to design an intervention to change ...
Frequency: 2 issues/year. ISSN: 1550-1175. E-ISSN: 2153-0327. Schools: Studies in Education provides a forum for classroom educators to describe and meditate on the complex experiences of school life. The journal publishes scholarly articles, reflective essays, and stories that convey how human relationships, thoughts, and emotions shape the ...
A comprehensive search of the literature selected 60 peer-reviewed journal articles and twenty-five books published between 2000 and 2016. ... college degree is highly relevant for higher ...
Metrics. The coronavirus pandemic has forced students and educators across all levels of education to rapidly adapt to online learning. The impact of this — and the developments required to make ...
In the present study, regarding the Effective Teaching Method in Higher Education, Requirements and Barriers, the experiences and perceptions of general practitioners were explored. As presented in Table 2, according to data analysis, two themes containing several major categories and codes were extracted.
This systematic review provides unique findings with an up-to-date examination of artificial intelligence (AI) in higher education (HE) from 2016 to 2022. Using PRISMA principles and protocol, 138 articles were identified for a full examination. Using a priori, and grounded coding, the data from the 138 articles were extracted, analyzed, and coded. The findings of this study show that in 2021 ...
Articles in our catalog come from diverse and global sources and authors. Cover a variety of topics Highlight leading insights and trends in the areas of strategy, leadership, innovation, and beyond.
Dr Wong Lung Hsiang, Senior Education Research Scientist and the co-Programme Director of the Learning Sciences and Innovation Research Programme at the Centre of Research in Pedagogy and Practice (CRPP), co-wrote a journal article titled "From Hype to Insight: Exploring ChatGPT's Early Footprint in Education via Altmetrics and Bibliometrics" in the Journal of Computer Assisted Learning ...
Research institutions are pushing back against proposed changes to misconduct and plagiarism investigations.
Michigan Tech has come out on top and Harvard at the bottom in the largest-ever survey looking into the state of free speech on America's college campuses. Most elite colleges, including Penn and ...
U.S. Education News Harvard University Applications Fall by 5% University had about 3,000 fewer applications than last year amid rising tensions on campus over the Israel-Hamas War
Most other universities similar in size to UW-Madison offer paid parental leave to their faculty, staff and graduate student workers. A committee that studied the feasibility of paid parental leave in 2022 found that among UW-Madison's peers, 90% of them provided paid parental leave to faculty and staff who become parents through birth or adoption.