Factor investing and asset allocation strategies: a comparison of factor versus sector optimization
- Original Article
- Open access
- Published: 29 May 2021
- Volume 22 , pages 488–506, ( 2021 )
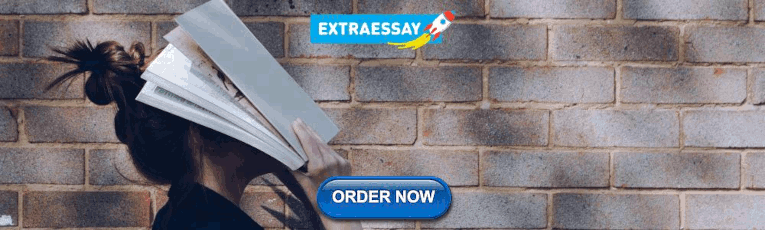
Cite this article
You have full access to this open access article
- Wolfgang Bessler 1 ,
- Georgi Taushanov 2 &
- Dominik Wolff 3
12k Accesses
10 Citations
23 Altmetric
Explore all metrics
Given the tremendous growth of factor allocation strategies in active and passive fund management, we investigate whether factor or sector asset allocation strategies provide investors with a superior performance. Our focus is on comparing factor versus sector allocations as some recent empirical evidence indicates the dominance of sector over country portfolios. We analyze the performance and performance differences of sector and factor portfolios for various weighting and portfolio optimization approaches, including “equal-weighting” (1/N), “risk parity,” minimum-variance, mean-variance, Bayes–Stein and Black–Litterman. We employ a sample-based approach in which the sample moments are the input parameters for the allocation model. For the period from May 2007 to November 2020, our results clearly reveal that, over longer investment horizons, factor portfolios provide relative superior performances. For shorter periods, however, we observe time-varying and alternating performance dominances as the relative advantage of one over the other strategy depends on the economic cycle. One important insight is that during “normal” times factor portfolios clearly dominate sector portfolios, whereas during crisis periods sector portfolios are superior offering better diversification opportunities.
Similar content being viewed by others
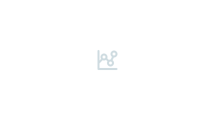
Diversification and portfolio theory: a review
Gilles Boevi Koumou
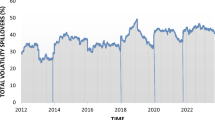
The dynamic connectedness between collateralized loan obligations and major asset classes: a TVP-VAR approach and portfolio hedging strategies for investors
Spyros Papathanasiou, Dimitris Kenourgios, … Georgios Pergeris
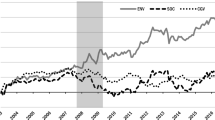
Integrating sustainability risks in asset management: the role of ESG exposures and ESG ratings
Benjamin Hübel & Hendrik Scholz
Avoid common mistakes on your manuscript.
Introduction
For many decades, favored asset allocation strategies focused on country or sector portfolios. However, more recently factor investing emerged as a popular new approach. The idea of factor investing is to diversify a portfolio among the underlying characteristic risk factors, introduced in the literature for explaining stock returns. The main objective of this research is to investigate whether factor-based portfolios perform superiorly relative to sector-based portfolios. Moreover, we are interested in the question whether factor timing adds value and examine whether dynamic factor investment strategies outperform a static multifactor benchmark. We extent the earlier research of Briere and Szafarz ( 2021 ) by focusing on investable factor and sector indices and by analyzing a variety of different out-of-sample investment strategies.
Extending the single-factor Capital Asset Pricing Model (Sharpe 1964 ) to multifactor models has a long tradition. Following a decade of research in arbitrage pricing theories (APT), Chen et al. (1986) introduced a multifactor model employing pre-specified macroeconomic variables. Fama and French ( 1993 ) extended and shifted the multifactor analysis toward characteristic factors, dividing the systematic risk spectrum initially into three core components. This offered researchers and investors the opportunity to better differentiate between the various sources of risk and risk premium in capital markets. Carhart ( 1997 ) extended this to a four-factor model by including a momentum factor, and Fama and French ( 2015 , 2018 ) added two additional factors to their own model resulting in a five-factor model or six-factor model when adding the momentum factor. Other often-employed factors are “dividends” (Fama and French 1988 , 1993 ), “liquidity” (Pástor and Stambaugh 2003 ), “betting-against-beta” (Frazzini and Pedersen 2014 ) and “quality-minus-junk” (Asness, et al. 2017 ).
The inroad of factors as asset classes into asset management, however, occurred through a government-mandated report for the Norwegian government pension fund conducted by Ang et al. (2010). The analysis suggested that systematic risk factors could explain a substantial part of the returns of actively managed funds. Consequently, asset management should employ factors not only to measure fund performance but also to construct portfolios. This research suggested that the asset allocation strategies should shift from the classical diversification across sectors or countries toward a factor-based approach. Subsequently, factor allocation strategies experienced a rising popularity among portfolio managers (Amenc et al. 2016 ).
In this study, we analyze different asset allocation models and compare their portfolio performance. As assets, we employ factor and sector indices, which are investable at low cost via exchange-traded funds (ETFs). We compare sector and factor allocations rather than comparing country and factor allocations as Bessler et al. ( 2021 ) provided recent evidence that sectors dominate country allocations. We measure the portfolio performance of both strategies first by analyzing the risk and return profiles and Sharpe ratios, and second, by comparing the alphas based on multifactor regressions. We also analyze whether factor-based optimized portfolios generate higher returns and higher multifactor alphas compared to a buy-and-hold factor investment. Third, we split the full period into sub-periods and compare the time variation of the sector and factor performance for each period. For longer investment horizons, we find that factor are superior to sector allocations. For shorter periods, the results are less consistent.
The rest of this research we structure as follows. In Sect. 2 , we review the literature and outline our general ideas. The optimization methodology is detailed in Sect. 3 , and the data are given in Sect. 4 . In Sect. 5 , we present the empirical results of the optimization processes as well as some further analyses. Chapter 6 concludes.
Literature review
Asness et al. ( 2013 ) were among the first to document the diversification potential of factor-based portfolios. Footnote 1 They studied the relationship between value and momentum characteristics and find strong, predominantly negative correlations between the underlying factors. Therefore, portfolios exposed to value or momentum characteristics should offer diversification benefits, substantially reducing risk. Both studies further report considerable value and momentum premium across different asset classes and markets, suggesting that a factor-based asset allocation approach provides higher diversification, return and performance potential relative to the “classic” strategies such as country or sector allocations.
Bender et al. ( 2010 ) extend the ideas of Asness et al. ( 2013 ) by increasing the number of factors in the portfolio allocation process. Their findings support the ideas that combining different factors in an investment strategy results in a superior performance relative to traditional asset allocation approaches. While an equally weighted portfolio of different factors and a traditional equity–bond portfolio yield similar average returns, factor portfolios contain much lower risk (less than one third of the risk of the traditional equity–bond portfolio), hence offering a higher performance (Sharpe ratio). In a similar study, Blitz ( 2011 ) uses the long components of four different factors (size, value, momentum and low volatility) as portfolio constituents. When extending the equally weighted optimized portfolios by including return predictions, the results confirm that factor-based allocation strategies provide significantly higher returns compared to a classical allocation strategy.
Hjalmarsson ( 2011 ) also extents the approach of Asness et al. ( 2013 ) by adding additional factors to the allocation process. Equal-weighted portfolios of seven or more factors generate higher Sharpe Ratios than any of the observed single-factor portfolios or the Asness et al. ( 2013 ) two-factor portfolios. This indicates that the classical diversification effect (decreasing risk when increasing the number of assets) might also apply to factor portfolios. In another study, Melas et al. ( 2011 ) simulated different fund strategies and organizational structures. Measuring the performance improvements of factor-based portfolios, the authors conclude that the focus of institutional investment funds should shift away from active asset allocations toward allocation between different sources of systematic risk such as factors. Consequently, the allocation of large portfolios should predominantly focus on beta, with a smaller attention to alpha management, which is in accordance with the ideas of Ang et al. ( 2010 ).
In fact, Ang ( 2010 ) describes the interaction between factors and returns as fundamental and equivalent to the relationship between “nutrients and food.” Following this concept, Ang and Kjaer ( 2012 ) argue that professional portfolio management should organize their allocation strategies based on the beta (“the nutrients”) instead of seeking the highest alpha. Consequently, the best strategy contains a diversification approach across factors, because “factors go beyond assets.” Footnote 2 Ilmanen and Kizer ( 2012 ) extend this view of Ang and Kjaer ( 2012 ) by comparing the effectiveness of “asset class” and “dynamic factor” diversifications. They report that factor-based portfolios contain much lower overall correlations than sector-based portfolios. Their performance comparison of equally weighted factor and “traditional asset” portfolios supports this view with factor portfolios yielding noticeably larger Sharpe ratios. However, the analysis of Ilmanen and Kizer ( 2012 ) is limited only to equally weighted portfolios and only for the period up to 2010. We extent the literature by comparing sector and factor portfolios for various dynamic asset allocation strategies and different portfolio optimization techniques up to the end of 2020.
As the factor portfolios’ popularity increased, Kahn and Lemmon ( 2016 ) predict substantial changes in active portfolio management. They believe that the smart beta and factor investment might replace a large part of the typical alpha-focused active management. Briere and Szafarz ( 2021 ) compare factor- and sector-based allocations and conclude that sector investing helps to reduce risks during crisis periods, while factor investing can boost returns during expansion periods. Dichtl et al. ( 2020 ) provide further evidence supporting the diversification effects of factors by developing a “factor completion” framework by incorporating the benefits of factors in a standardized allocation process.
The analysis of Briere and Szafarz ( 2021 ) builds on Fama–French factors, which are not directly investable. However, implementing portfolio strategies based on these factors involves large portfolio turnover and transaction costs, because not only Fama–French factor portfolios require updating on a monthly basis but also factor portfolio weights need adjusting over time. Therefore, we analyze factor versus sector strategies from a practical perspective and build our analysis on factor and sector indices, which are investable at low cost via ETFs. We also explicitly account for transactions costs. Moreover, relative to Briere and Szafarz ( 2021 ), we test a variety of out-of-sample allocation strategies to analyze the benefits of factor versus sector investing for different investor types and investment styles.
The growing academic interest in factor returns and asset allocation strategies, however, also resulted in an opposite and critical perspective. Arnott et al. ( 2016 ) distinguish between structural and situational sources of returns where the structural “value adds”—in contrast to the situational—are stable in time and therefore a reliable source of future returns. Footnote 3 The authors argue that in the recent years the prices of factor portfolios have grown too high due to the excessive demand by performance seeking portfolio managers. Consequently, the probability of generating persistently high returns in the future has declined, which also cause the situational character of the factor returns. Dimson et al. ( 2017 ) point out that the core meaning of factors lies in the co-movement of the group of stocks sharing specific attributes. They interpret the counter-movement in some of the observed groups as an essential driver for portfolio risk reduction. The authors, however, do not find evidence for a sustainable return premium in the observed factors.
A critical question is whether factor timing or factor tilting add value compared to a static factor allocation. In general, “factor tilting” describes the relative over- or underweighting of a factor compared to other factors. The benefit of factor timing critically depends on the predictability of factor returns. Dichtl et al. ( 2019 ) investigate the benefits of parametric portfolio strategies for factor timing and tilting, indicating that this might significantly improve the factor portfolio performance. However, benefits in the practical portfolio management deteriorate after including transaction costs.
Methodology
In this section, we present a variety of asset allocation models and out-of-sample estimation procedures that we implement in our study. We follow the results of DeMiguel et al. ( 2009 ) that no portfolio construction technique is persistently superior to 1/N and use this approach as our benchmark. We then employ a variety of weighting and optimization techniques, analyze their relative performance and evaluate whether they outperform the 1/N strategy. Our assets are sector and factor indices, and therefore, our research objective is to compare the benefits of various factor- and sector-based allocation strategies. These strategies include the naive “equally weighted” (1/N) portfolio as benchmark, the risk-based asset allocation rules “risk parity” (RP) as well as four portfolio optimization approaches: minimum variance (MinVar), mean–variance (MV), Bayes–Stein (BS) and Black–Litterman (BL).
We structure our optimization process as follows: The weights for each portfolio are determined at the last trading day of each month, based on all previous information. We calculate the portfolio returns for the following month by multiplying the calculated weights with the component returns of the corresponding month. We include 20 basis points of transaction costs, which is reasonable, given that we implement the sector and factor allocations with exchange-traded funds (ETFs). We repeat this process monthly by moving the sample period one month forward and by computing the optimized weights for the next month. Taking the forecast and optimization calibration periods into account, we compare all asset allocation strategies for these two asset classes, sectors and factors, for the out-of-sample period from May 2007 to November 2020.
A critical aspect in optimal portfolio allocation decisions besides the optimization model is the input data, for which we use historical average returns. As a robustness check, we employ different window lengths ranging from 12 to 60 months. We also employ the cumulated average (CA) strategy, which includes all observations from the beginning of the sample period up to the current observation. Further, to test the robustness of our results, we use different investment constraints such as long-only portfolios and portfolios with short positions. We also investigate the effects of constraints on performance by relaxing the constraints in the range between −35% (50%) (short positions) and +35% (50%) (maximum weight of a constituent in our portfolio). Next, we discuss the different asset allocation approaches for our investment strategies.
Asset allocation models
Both academia and the asset management industry analyzed and implemented a large variety of different asset allocation approaches. These models range from naive to sophisticated models. Our description begins with the rather simple rule-based weighting methods such as “equal-weighting” or “risk parity” and continues in the next section with the optimization-based methods: minimum variance, the classical mean–variance, the Bayes–Stein, as well as the Black–Litterman optimization approaches.
Naive diversification 1/N
Being one of the most prominent allocation methods among private investors according to Benartzi and Thaler ( 2001 ), we implement the 1/N wealth distribution approach, which allocates equal weights to every asset. Therefore, given the number of constituents available for portfolio construction (6 factor and 10 sector portfolios), 1/N always allocates 16.67% and 10% of the portfolio to every factor or sector index, respectively. As for all other allocation strategies, we rebalance the portfolios monthly.
Risk parity
The risk parity (RP) approach is another prominent weighting method, widely adopted by both academia and professional portfolio management. With the growing interest in the so-called smart beta strategies, a large number of mutual funds, index providers, pension funds, endowments and other long-term investors have adopted this strategy. Footnote 4 The basic idea of the risk parity weighting is that each portfolio component contributes equally to portfolio risk, neglecting correlations between asset returns. Therefore, assets are weighted counter-proportional to their sample variance \({{\widehat{\sigma }}_{i}}^{2}\) .
Anderson et al. ( 2012 ) report that risk parity strategies perform well and usually even outperform 1/N, value-weighted or 60/40 portfolios. The risk parity approach exploits the low-volatility anomaly, according to which low-volatility assets usually earn a higher premium per unit of volatility than high-volatility assets (Baker et al. 2011 ; Frazzini and Pedersen 2014 ). It therefore underweights high-volatility assets and overweight low-volatility assets. We next turn from the simple to the optimization-based asset allocation approaches.
Minimum variance
A simple and robust optimization-based strategy is the minimum variance approach (MinVar), which is increasingly popular among institutional investors such as quantitative mutual funds or exchange-traded funds. Footnote 5 The objective of the MinVar strategy is to minimize portfolio volatility. The minimization problem is:
where ω is the vector of portfolio weights and Σ is the covariance matrix. The main advantage of the minimum variance approach is that it does not require any return estimates, which are highly vulnerable to estimation errors and therefore the main source of sub-optimal allocation decisions. As risk parity, this concept utilizes the observation that low-risk assets often generate a higher return premium per unit of volatility (low-volatility anomaly). The core difference between the MinVar and the RP strategy is, however, that the MinVar strategy is a portfolio optimization-based approach that also takes the correlation of asset returns into account.
Mean–variance
Mean–variance (MV)—in contrast to minimum variance—also includes return predictions for optimizing the risk–return trade-off (Markowitz 1952 ). The mean–variance optimization problem is:
where U is the investor’s utility, μ is the vector of expected return estimates and δ is the risk aversion coefficient. Footnote 6 To implement the mean–variance strategy, we use the sample mean \(\widehat{\mu }\) and the sample covariance matrix \(\widehat{\Sigma }\) as described above. The optimization is subject to two restrictions. First, we include a budget restriction, ensuring that portfolio weights sum to one and second, we prohibit short positions in our base case. The risk aversion coefficient is set to five (for a discussion of parameter settings see Bessler et al. 2017 ).
Bayes–Stein
Developed by Jorion ( 1985 ), the Bayes–Stein (BS) model extends the MV strategy attempting to reduce estimation errors in the return and volatility forecasts by relying on a Bayesian estimation approach (Stein 1956 ; James and Stein 1956 ). The optimization procedure itself is identical as in the MV approach presented in equation ( 3 ).
The sample means as expected returns \(\widehat{\mu }\) are shrunk toward the expected returns of the minimum variance portfolio \({\widehat{\mu }}^{min}\) given the shrinkage factor \(\widehat{\gamma }:\)
The covariance matrix, is also adjusted following the Jorion ( 1986 ) methodology and calculated as follows:
where \(\overrightarrow{1}\) is a vector of ones, \(\widehat{\Sigma }\) denotes the unbiased sample covariance matrix, N the number of assets and T is the number of observations (Jorion 1986 ). Subsequently, we apply the same optimization procedure, constraints and estimation windows as for the MV approach.
Black–Litterman
Another approach to mitigate the noise problems in portfolio optimization was proposed by Black and Litterman ( 1992 ). Similarly to the MV and BS, the Black–Litterman (BL) model is popular among quantitative asset managers (Satchell and Scowcroft 2000 ; Jones et al. 2007 ). BL combines “implied” returns calculated from a benchmark portfolio via reverse optimization with explicit return forecasts or “views” taking the correlation structure between the assets into account (Black and Litterman 1992 ; Lee 2000 ). The major advantage of the BL model is that it integrates the reliability of the forecasts into the allocation process. The vector of the return forecasts according to Black and Litterman ( 1992 ) is estimated as follows:
where П is the vector of “implied” returns, Σ is the covariance matrix and Q is the vector of the investor’s return estimates. The reliability of the return predictions is contained in the diagonal matrix Ω and can be measured as the variance of the realized prediction errors in the estimation window . P denotes an identity matrix and τ a scalar that calibrates the tracking error to the benchmark portfolio. Footnote 7 The BL model also adjusts the covariance matrix. Following Satchell and Scowcroft ( 2000 ), the covariance matrix is computed as:
After computing expected returns (equation 7 ) and the covariance matrix (equation 8 ), the portfolio weights are calculated in the same fashion as for the MV and the BS methods—via traditional risk–return optimization, maximizing the investor’s utility as presented in equation ( 3 ). The rest of the relevant parameters such as risk aversion coefficient, optimization procedure and constraints remain the same across all risk–return strategies. Following Bessler and Wolff ( 2015 ), we implement a sample-based version of the BL model. Footnote 8
Performance measures
To evaluate the performance of factor- versus sector-based portfolios, we employ several performance measures. We compute the portfolio’s average out-of-sample return and volatility as well as the out-of-sample Sharpe ratio. Following Opdyke ( 2006 ), we test whether the difference in Sharpe ratios of two portfolios is significant. This test is applicable under very general conditions—stationary and ergodic returns. Most importantly for our analysis, the test permits autocorrelation and non-normal distribution of returns and allows for a likely high correlation between the portfolio returns. To provide further evidence and to make our study better comparable to the recent literature, we implement different factor models for computing multifactor alphas as risk-adjusted performance measures. The first factor model contains the six Fama–French (2018) long–short factors from Kenneth French’s website: “Market,” Value (“HML”), Size (“SMB”), Quality (“RMW”), Investment (“CMA”) and Momentum (“MOM”) augmented by the betting-against-beta factor (“BAB”) proposed by Frazzini and Pedersen ( 2014 ). This analysis allows us to determine whether the performance of our factor or sector portfolios is fully attributable to the known risk factors or whether they provide significant multifactor alphas.
The second factor model we employ to compute multifactor alphas includes the same six MSCI factors, which we employ as asset classes in the factor portfolios. This analysis allows us to investigate whether dynamically adjusting factor allocations adds value over a buy-and-hold factor portfolio. Finally, we compare the periodical performance of the two strategies depending on the state of the economy.
Our two investment strategies include 10 sector and 6 factor indices, which are investable with ETFs. Both sector- and factor-based portfolios contain only US stocks. The 16 total return indices are downloaded from Bloomberg on a monthly basis for the longest jointly available period from January 1999 to November 2020. The dataset includes 262 monthly return observations for each index. For implementing the portfolio optimization strategies, we need up to five years (60 months) for estimating the optimization inputs (average returns and the covariance matrix). Three more years of data are required in the Black–Litterman optimization for determining the reliability of return estimates. Therefore, our out-of-sample evaluation period ranges from 2007 to 2020 and includes 166 months.
The sector indices, as presented in Table 1 Panel A, are based on the constituents of the S&P 500 index. The indices (Factset) include the following sectors: “Industrials,” “Consumer Discretionary,” “Utilities,” “Energy,” “Consumer Staples,” “Information Technology,” “Communication Services,” “Health Care,” “Financials,” “Materials.”
From the large number of factors discussed in the literature, only a few factors are investable at low cost, for instance, through ETFs. Our factor portfolios, as presented in Table 1 Panel B, consist of the following 6 investable MSCI US long-only factor indices with a sufficient data history. The momentum factor (Carhart 1997 ), the size factor (Fama and French 1993 ), the value factor (Fama and French 1993 ), the quality factor (Fama and French 1993 ), the carry factor (based on “dividend yield” as proposed by Fama and French 1988 , 1993 ) and the low volatility/low beta factor (Frazzini and Pedersen 2014 ).
Tables 2 and 3 display the statistical properties of the sector and factor indices, respectively. In general, the mean returns for the factor and the sector indices are similar. For the factor indices, the returns range from 0.45% (“Value”) to 0.77% per month (“Momentum”). For the sector indices, the minimum and the maximum values are, 0.19% for the “Consumer Services” sector and 0.72% for the “Consumer Discretionary” per month. The standard deviations of returns reveal that, on average, the factor indices have lower risk with 4.42% per month compared to sector indices with 5.50% per month. Comparing the minimum and maximum values between both index groups, we observe the lowest risk for the “Consumer Staples” sector (3.46%) compared to the low volatility factor (3.79%). We measure the highest risk for the factor “Size” with 5.1%, whereas the highest risk for the sector group is 7.25% (“IT”).
One of the most important statistical aspects directly affecting the risk and therefore the performance of an optimized portfolio is the correlation structure among the assets. In general, the group of assets with the lower correlation offers better diversification opportunities, which, however, does not necessarily mean higher performance. For the sector indices, the highest correlated pairs are the following: “Industrials”—“Consumer Discretionary” (0.85), “Industrials”—“Materials” (0.84), as well as “Industrials”—“Financials” (0.82). The pairs with the lowest correlation in the sector group are “Utilities”—“IT” (0.20), “Consumer Staples”—“IT” (0.27) as well as “Utilities”—“Consumer Discretionary” (0.30).
Both, maximal and minimal correlation coefficients in the factor indices are higher than for the sector group. The highest correlations we observe for factors are for the following pairs: “Quality”—“Value” (0.95), as well as between “Carry”—“MinVol” and “Carry”—“Value” (both 0.93). The lowest correlations in the factor group are the following: “Carry”—“Momentum” (0.67) followed by “Value”—“Momentum” (0.77).
With respect to the optimization process, our insights from the descriptive statistical analysis are twofold. First, the correlations between factor indices are noticeably higher than between sectors, offering lower diversification opportunities. Second, average monthly returns were slightly higher for factor indices than for sectors, indicating higher portfolio returns. Therefore, it is essential to employ the different portfolio optimization algorithms to provide empirical evidence whether sectors or factors provide the better trade-off between risk and return and therefore are the superior building blocks for an optimal investment strategy.
Empirical results
We structure this section as follows. Section 5.1 reports the portfolio performance for the full period, Section 5.2 contains additional risk analyses and Section 5.3 shows the sub-period results.
Portfolio performance full period
In this section, we report the full period results by providing the following performance results: (a) full period analysis of Sharpe ratios, (b) sensitivity analysis of changing constraints, (c) risk and return analysis, (d) multifactor performance analysis and (e) multifactor alpha differences between both strategies.
Full period analysis of Sharpe ratios (Table 4 ).
In Table 4 , we report and compare the pairwise Sharpe ratios of the sector- and factor-optimized portfolios. We analyze six different asset allocation frameworks, which consist of equal weighting (1/N), two risk-based allocations (RP, MinVar) and the three risk–return optimizations (BL, MV, BS) approaches. For the risk–return optimization approaches, we employ four different estimation window lengths as model inputs. The portfolio constraint for our base case is a 35% allocation to one single asset (index) and no short positions. The second framework allows for a 35% long or 35% short position in any single index, whereas in the third framework we extend both limits to 50% for long and 50% for short position in a single index, allowing maximum flexibility for risk exposure management. This optimization framework (weighting or optimization algorithm, return forecast and constraints) we apply to both factor- and sector-based portfolios.
The initial results presented in Table 4 reveal that for all reported pair results, which are the differences between sector and factor portfolios, the factor allocations generate higher Sharpe ratios. Due to the relatively short history of the indices, however, the Sharpe ratio differences are statistically significant only for some portfolio pairs (according to the Opdyke ( 2006 ) test). Still, the Sharpe ratio differences are economically relevant and the information that factor portfolios dominate sector portfolios in all analyzed cases is a very strong outcome in favor of factor allocations.
Sensitivity analysis of changing constraints
We next focus on the effects of different weight constraints (columns (1) to (6) in Table 4 , respectively). We observe for the long–short allocations (maximum short and long positions between −35% and +35%, and between −50% and +50%) that the performance differences between the two allocation strategies become larger relative to the long-only case. The factor performance is relatively stable for different optimization constraints, whereas the performance of the sector allocations tends to deteriorate with more relaxed optimization restrictions.
We observe the highest performance difference for the mean–variance algorithm with the most relaxed portfolio restrictions (−50% to +50%). In contrast, the lowest performance difference occurs for the minimum variance approach, although factor portfolios outperform sector allocations. This is a rather surprising result, given that the average correlations between sectors were lower than between factor indices. The performance difference for the minimum variance approach gets even smaller when we further relax the optimization restrictions. The analysis of the risk and return structure of the portfolios in the next section provides further insights for explaining these results.
Risk and return analysis
Separating Sharpe ratios into their two basic components return and risk offers some additional insights into the source of the performance differences between sector and factor allocations. We present the annualized mean returns as well as the risk (standard deviation, volatility) of the optimized portfolios in Table 5 . The benefits of the factor allocation originate not only from larger returns (Table 5 , Panel A) but also from lower portfolio volatility (Table 5 , Panel B). In all cases, regardless of the allocation environment (optimization algorithm, window length for optimization inputs and weight constraints), the factor portfolios reveal lower or equal risk as the sector portfolios. These outcomes are in accordance with the observation in Bender, et al. ( 2010 ) that factor-based diversification lowers portfolio risk. There is only one exception, in which the sector allocation provides a marginal lower volatility: the minimum variance portfolio with narrow weight restrictions. For the properties of the optimization methods, we identify some interesting details for the factor allocations. Similar to the Sharpe ratios, the risk profile of the factor portfolios closely relates to the constraints. The highest differences in risk of all optimization–forecast combinations occurs in the case with the most relaxed weight constraints (−50% and 50%; column 5 and 6 in Table 5 ).
Widening the level of restrictions (columns 3 to 6) substantially increases the volatility of the sector portfolios, whereas the volatility of the factor portfolios remains at low levels. Accordingly, the largest risk differences occur among the least constrained portfolios with short and long positions between −50% and 50% (column 5 and 6 in Table 5 Panel B), respectively.
Comparing the mean returns presented in Table 5 Panel A suggests quite similar results relative to the Sharpe ratio and the risk results. In all reported cases, the factor-based portfolios yield larger or equal mean returns compared to the sector-based portfolios. The relationship between the returns and the allocation constraints is similar to the one we observed when assessing the Sharpe ratio differences for both strategies. Extending the level of the allocation freedom increases the return differences between factor and sector portfolios.
Multifactor performance analysis: Fama–French factors (Table 6 )
Next, we next analyze the performance of the optimized portfolios within a multifactor model framework. We regress the returns of our portfolios on the six Fama–French US equity long–short factors: “Market,” Value (“HML”), Size (“SMB”), Quality (“RMW”), Investment (“CMA”) and Momentum (“MOM”) augmented by the betting-against-beta factor (“BAB,” Frazzini and Pedersen 2014 ). Table 6 Panel A depicts the multifactor alphas of the factor and sector portfolios along with the significance levels for the null hypothesis that alphas are different from zero. Table 6 Panel B contains the differences between alphas of factor and sector portfolios.
In Table 6 , we observe positive multifactor alphas for all optimized portfolios. For the long-only case, all portfolios have positive alphas with the vast majority being significantly larger than zero. More specifically, all factor portfolios have statistically significant positive multifactor alphas, while for sector portfolios many alphas are not significantly larger than zero. Moreover, for all risk–return optimization models we find that alphas are larger for factor portfolios compared to sector allocations. In contrast, for risk-based allocations (risk parity and minimum variance) factor portfolios reveal lower multifactor alphas than sector portfolios.
This finding is in line with our earlier evidence that sectors are less correlated than factors. It also supports the conclusion of Briere and Szafarz ( 2021 ) that sector investing helps to reduce risks during crisis periods, while factor investing can boost returns during expansion periods. The highest alpha (1.22% per month) we obtain with the factor-based Black–Litterman optimization method with 60-month estimation window and with short sales allowed (weights between −50% and 50%). In line with Bessler et al. ( 2017 ), we find the largest multifactor alphas for BL portfolios compared to all other allocation strategies. Overall, the multifactor analysis confirms our finding that factor portfolios dominate sector portfolios at least for risk–return optimization models.
Multifactor performance analysis: difference between both strategies
In Table 6 Panel B, we provide additional insights into the performance difference between sector and factor portfolios. In contrast to the results in Table 6 Panel A, where we reported the significance levels for the null hypothesis that alphas are different from zero, we now test for the differences in the portfolio’s factor exposures using dummy regressions. Panel B provides the difference between the alpha quotients of both allocation strategies. The positive alpha differences suggest that factor portfolios have larger multifactor alphas than sector portfolios. Comparing the three columns in Panel B, we observe that the alpha differences between the sector and factor strategies tend to become larger with wider investment restrictions, illustrating that the advantages of factor portfolios increase when relaxing investment constraints. However, due to our relatively short evaluation period, the differences in multifactor alphas between factor and sector portfolios are mostly statistically insignificant, yet economically relevant.
Multifactor performance analysis: MSCI factors (Table 7 )
Next, we assess the potential outperformance of a dynamic factor timing strategy compared to a buy-and-hold factor portfolio. For this, we compute another set of multifactor alphas by regressing the returns of the optimized factor portfolios on its own components (the single MSCI factors). If the optimized portfolio was a buy-and-hold portfolio of any initial weighting, the estimated alpha would be zero and the underlying assets would fully explain the portfolio where the regression coefficients would equal the respective factor weights. In this case, the more passive the management of the underlying constituents, the closer to zero is the estimated alpha. In contrast, since the portfolios consist entirely of the same factors applied in the multifactor regression, the source of the alpha in the factor portfolios stems uniquely from the dynamic factor allocation.
While the effect of the “right bet at the right time’ might still be essential in the case of the industry allocation, here, the reported alphas contain another effect: the performance contribution of the diversification of the single sector component as well as the diversification from combining different sectors in a global portfolio. In summary, the comparison of the factor and sector alphas covers the difference between the two risk narratives described in Section 1.
In Table 7 , we present the results (alpha) from the multifactor performance analysis. For most of the optimized portfolios, we observe positive multifactor alphas. For the long-only portfolios, all portfolios show positive alphas with the vast majority being significantly larger than zero. This supports our conjecture that combining the factors in an optimized portfolio results in higher returns than the return of a static buy-and-hold factor portfolio. Simply combining factors in an equally weighted portfolio (1/N) with monthly rebalancing back to the 1/N weights already provides a low but statistically significant outperformance compared to a buy-and-hold portfolio. Again, the factor-based Black–Litterman optimization method with short sales allowed (12-month estimation window and weights between −50% and 50%) provides the highest alpha (1.43% per month).
In contrast to the Fama–French factor analysis in section e), we find sector portfolios yielding higher multifactor alphas. This might appear surprising, as it seems to contradict our earlier results. However, it is very reasonable to expect that the same underlying factors explain very well factor portfolios that consist of these factors. Particularly, factors can better explain returns of factor-optimized portfolios, based on the same underlying factors, rather than portfolios consisting of sectors.
(g) Portfolio turnover (Table 8 )
Next, we analyze portfolio turnover of factor and sector allocations. Higher portfolio turnover is associated with larger transaction costs. Our analyses so far included 20 basis points of transaction costs, which is reasonable, given that we implement the sector and factor allocations with exchange-traded funds (ETFs). In this section, we analyze whether our assumption on transaction costs affects our results. Table 8 Panel A presents the portfolio turnover for all factor and sector portfolio. We find that for almost all allocation strategies, portfolio turnover is lower for factor compared to sector portfolio. Therefore, the relative advantage of factor compared to sector allocations increases with larger transaction costs.
Risk analysis
An important aspect of the performance analysis is an in-depth risk analysis. For this, we analyze whether the performance difference between factor and sector portfolios is attributable to different levels of tail risk, such as maximum drawdown, or to the skewness and kurtosis of the return distribution.
Maximum drawdown
The first part of the risk analysis covers the comparison of the maximum drawdowns (MDD) that occurred during the full investment period in factor and sector portfolios. The maximum drawdown represents the absolute losses between the highest peak and the subsequent lowest trough of the portfolio. Table 8 Panel B presents the maximum drawdowns for all strategies over the full evaluation period. Analyzing the results, we do not find a strong relationship between the MDD and the portfolio allocation strategy. The factor-based allocations have even lower drawdowns in most of the analyzed cases. For 1/N and the risk-based allocation frameworks (RP and MinVar), MDD is lower for sector portfolios than for factor portfolios. In contrast, for the risk–return optimization strategies (BL, MV, BS) MDD is lower for factor portfolios than for sector portfolios in the majority of the cases.
Skewness analysis
Next, we analyze the skewness of portfolio returns. In general, investors seek positive skewness since it translates in a higher likelihood of positive returns or positive outliers. The negative skewness, in contrast, represents in general the higher likelihood of occurrences on the negative side of the return distribution (losses). For brevity, the results for the skewness analysis is available in the online appendix. Our results suggest that all of our optimized portfolios reveal negative skewness, meaning that tail risks on the negative side (left) is higher than normally distributed returns. In general, there is no clear relationship between skewness and the allocation framework. On the one hand, for 1/N and the risk-based allocation frameworks (RP and MinVar), portfolio skewness is higher for sector portfolios than for factor portfolios. On the other hand, for the risk–return optimization strategies (BL, MV, BS), the skewness is higher for factor portfolios than for sector portfolios in the vast majority of the cases. Overall, a higher skew risk cannot explain the higher returns of the factor portfolio as the skewness is even lower for most factor portfolios.
Kurtosis analysis
Kurtosis characterizes the second key property of the return distributions. In general, kurtosis represents the “tailedness” of the respected distributions. Our results for the kurtosis analysis are available on the online appendix. In general, we find that all observed portfolios show leptokurtic distributions, meaning that the extreme return observations occur more frequently than expected for normally distributed returns. Similar to the skewness analysis, the results do not reveal a clear pattern how the skewness relates to factors or sectors. However, on average, and in most analyzed cases, the sector portfolios have a slightly lower excess kurtosis than factor portfolios. However, as the excess kurtosis captures both, extreme positive and negative returns, the higher kurtosis of most factor portfolios might be due to periods of high factor returns.
Our conclusion from the MDD and the overall risk analysis is that the higher returns and Sharpe ratios of the factor portfolios are not explainable with the higher tail risks of these portfolios. Moreover, we do not find a clear difference between factor and sector portfolios with regard to tail risk measures.
Sub-period analysis
Following the analysis of the full period, we now investigate the performance for different optimization strategies and for different sub-periods. For this, we split the time series of the optimized portfolios in several sub-periods based on the state of the economy, where we distinguish between two different states: economic expansion and economic recession. As an indicator for the different states of the economy, we use the NBER recession dummy published by the US National Bureau of Economic Research.
Based on NBER recession dummies, we divide the full sample into three sub-periods. The first sub-period spans the period from May 2007 to July 2009 and includes the global financial crisis. The second sub-period contains the subsequent recovery of the global economy and financial markets. The last sub-period begins in 03/2020 due to the Corona crisis. The results of the sub-period analysis, expressed as the difference between the Sharpe ratios of the factor- and the sector-based portfolios, we present in Table 9 . We structure the results again in the same way as we did for the Sharpe ratios in Table 4 . In each Panel of Table 9 , we present the results for one restriction, moving from strictest to moderate to lowest restrictions.
Strictest restrictions
In Table 9 Panel A, we present the results for the portfolios with portfolio weight restrictions between 0% and +35%. The results suggest that this separates the dominance of one over the other strategy into two groups: During both crisis periods, the global financial crisis and the Covid-19 crisis, sector portfolios outperformed factors portfolios, indicating that sector portfolios offer higher diversification potential particularly during crisis periods relative to factor portfolios. This finding is in line with Briere and Szafarz ( 2021 ) who report that sector investing helps to reduce risks during crisis periods, while factor investing can boost returns during expansion periods. Consequently, during the long expansion period from August 2009 to February 2020, factor allocation clearly outperformed sector allocations. While the sector allocations dominate in two out of three sub-periods, it is important to recognize that the two sub-periods in which sectors outperform are relatively short, spanning only 11 and 9 months. In contrast, the second sub-period in which factor portfolios dominate stretches over more than 10 years. Therefore, for the full period we find factor portfolios clearly outperforming sector portfolios.
Moderate restrictions
The results for the moderately constrained portfolios (restrictions between −35% and +35%) we report in Table 9 Panel B. Compared with the long-only case (Panel A of Table 9 ), we find similar results for the second and third sub-periods with factor portfolios dominating during the long second sub-period and sector portfolios outperforming during the short Covid-19 crisis period. However, for the first sub-period (Global Financial Crisis), relaxing the weight restrictions inverses the results. While for long-only portfolios, sector portfolios dominated during the Global Financial Crisis, for portfolios with short positions, the factor portfolios also dominate during this period.
Lowest restrictions
The results for portfolios with the lowest restrictions we report in Table 9 Panel C. In general, the structure of the performance differences remains the same as for the moderate restrictions (Panel B) with only minor differences. As in the case with moderate restrictions, factor portfolios dominate in the first and second sub-period. Only in the third sub-period (Covid-19 crisis), sector allocations achieved larger Sharpe ratios.
One conclusion from the sub-period analyses is the observation that the performance differences between the sector- and factor-based allocations are not stable over time. The relative performance of sector and factor portfolios seems to relate to the state of the economy. While overall and for the full period factor portfolios dominate sector portfolios, the latter seem to be beneficial during crisis periods. However, the available history of factor indices is still too short to allow robust conclusions. Adjusting the relative size of short and long positions, optimization algorithms and different estimation window length in the optimization process, affects the comparative results, but do not fundamentally change the pattern of the relative performance.
Conclusions
In this study, we compare the performance of two different low-cost asset allocation strategies, one building on investable factors and the other one on investable sectors, both via ETFs. We extent the earlier research of Briere and Szafarz ( 2021 ) in different directions. While Briere and Szafarz ( 2021 ) build on Fama–French factors that are not directly investable, we focus on investable factor and sector indices and in addition analyze a variety of different out-of-sample investment strategies.
For the entire investment period between May 2007 and November 2020, we find that factor portfolios provide a superior performance relative to sector portfolios. Even though the differences are not always statistically significant, which might be due to the relatively short evaluation period, the results are economically relevant with substantial Sharpe ratio differences. The results are consistent among all analyzed asset allocation strategies and estimation window length for the input parameters. The reason for the results become more evident when we analyze the performance differences in more detail. The factor portfolios generate higher average returns with lower risk (volatility). Our analysis of tail risk measures such as skewness or kurtosis of returns or the maximum drawdown reveals that factor portfolios do not exhibit larger levels of tail risk. Therefore, higher risk cannot explain the superior performance of factor portfolios. Moreover, we find that for almost all allocation strategies, portfolio turnover is lower for factor compared to sector portfolios. Hence, for larger transaction costs the relative benefits of factor compared to sector allocations become even more pronounced.
Based on multifactor regressions, we observe that both factor and sector allocations yield positive multifactor alphas when including the six Fama–French long–short factors (“Market,” Value (“HML”), Size (“SMB”), Quality (“RMW”), Investment (“CMA”) and Momentum (“MOM”)) augmented by the betting-against-beta (BAB) factor (Frazzini and Pedersen 2014 ). Consistent with the Sharpe ratio results, we find for all risk–return optimization models that factor portfolios provide higher multifactor alphas compared to sector portfolios. Only for risk-based allocations, sector portfolios partially provide larger multifactor alphas than factor portfolios. This result is in line with our finding that sectors reveal a lower correlation structure and hence a higher diversification potential than long-only factors.
To analyze the potential benefits of factor timing, we regress factor portfolios on the same six MSCI long-only factors, which we employ as assets in the factor portfolios. We find that risk–return optimization as well as risk-based allocations add value compared to a buy-and-hold factor strategy. Finally, our sub-period analysis indicates that during “normal” times factor portfolios clearly dominate sector portfolios, whereas during crisis periods sector portfolios are superior offering better diversification opportunities. This finding is in line with Briere and Szafarz ( 2021 ) who reported that sector investing reduces risks during crisis periods, while factor investing can boost returns during expansion periods.
However, for the full sample, we find a clear outperformance of factor portfolios. Overall, factor indices offer an attractive investment universe and are already investable for instance via ETFs. For further research, it might be interesting to investigate whether combining sectors and factors in a single portfolio adds additional value. Briere and Szafarz ( 2020 ) propose blended portfolios, which combine the diversification benefits of sector investing particularly during crisis periods with the risk premiums of factor investing. Analyzing the benefits of blended portfolios, we leave for further research.
Kim et al. ( 2017 ) provide an extensive literature review on the topic of factor allocation. They conclude that despite the ambiguity between factors and assets, incorporating the factor models in the investment process might improve the investment strategy.
Ang et al. (2009) and Ang ( 2014 ) support the idea of factors representing different risk classes and, therefore, of independent return sources.
Critical views on the factor allocations approach have also been stated by Asness ( 2016 ), Asness et al. ( 2017 ) as well as Lee ( 2017 )
See Anderson et al. ( 2012 ) or Maillard et al. ( 2010 ), for a discussion of this class of models.
Coqueret ( 2015 ) summarizes the most important reasons for the higher attention. First, in contrast to the “classical” optimization methods, no return forecasting is necessary, which could have considerable effects on the allocation outcome (Kondor et al. 2007 ). Second, in crisis-periods investors tend to buy lower-risk products. Finally, since Black ( 1972 ) as well as Haugen and Heins (1972) introduced the so-called low-volatility paradox, a large body of literature concludes that lower-risk assets do not necessarily perform worse than their higher-risk counterparts do.
Mean–variance relies on the critical assumption that asset returns are normally distributed. Although this requirement is often identified as one of the main weaknesses in academia, Landsman and Nešlehová ( 2008 ) demonstrate that it is sufficient that returns are elliptically symmetrically distributed so that all investor preferences are equivalent to mean–variance preferences. Therefore, it is sensible to apply the mean–variance framework for portfolio optimization even if asset returns are non-normal, as long as they are symmetric.
For a detailed exposition see Bessler et al. ( 2017 ) and Bessler and Wolff ( 2015 ).
For an application, see Bessler et al. ( 2017 , 2021 ), Bessler and Wolff ( 2015 ). In the sample-based version of the BL model “views” are the sample means of the respective asset returns. The reliability of “views” is measured as the variance of the historical forecast errors ε i during the sample period “Implied” returns are computed based on the 1/N portfolio. The parameter τ is set to 0.05. Earlier studies use similar values ranging from 0.025 to 0.3 (Black and Litterman 1992 ; He and Litterman 2002 ; Idzorek 2005 ).
Amenc, Noël., Frédéric. Ducoulombier, Felix Goltz, Ashish Lodh, and Sivagaminathan Sivasubramanian. 2016. Diversified or Concentrated Factor Tilts?. The Journal of Portfolio Management 42: 64–76.
Article Google Scholar
Anderson, Robert M., Stephen W. Bianchi, and Lisa R. Goldberg. 2012. Will My Risk Parity Strategy Outperform?. Financial Analysts Journal 68: 75–93.
Ang, Andrew, 2011, The Four Benchmarks of Sovereign Wealth Funds, Sovereign Wealth Funds and Long-Term Investing, Columbia University Press
Ang, Andrew. 2014. Asset Management: A Systematic Approach to Factor Investing . New York, USA: Oxford University Press.
Book Google Scholar
Ang, Andrew, William Goetzmann, and Stephen Schaefer, 2009, Evaluation of Active Management of the Norwegian GPFG, Norwegian Ministry of Finance .
Ang, Andrew, and Knut N. Kjaer, 2014, Investing for the Long Run, Asset Management: A Systematic Approach to Factor Investing, Oxford University Press.
Arnott, Robert D., Noah Beck, Vitali Kalesnik, and John West, 2016, How Can 'Smart Beta' Go Horribly Wrong? Working Paper.
Asness, Clifford S., Tobias J. Moskowitz, and Lasse H. Pedersen. 2013. Value and Momentum Everywhere. The Journal of Finance 68: 929–985.
Asness, Clifford S. 2016. The Siren Song of Factor Timing, aka ‘Smart Beta Timing’, aka ‘Style Timing’. Journal of Portfolio Management 42: 1–6.
Asness, Clifford S., Swati Chandra, Antti Ilmanen, and Ronen Israel. 2017. Contrarian Factor Timing Is Deceptively Difficult. Journal of Portfolio Management 43: 72–87.
Baker, Malcolm, Brendan Bradley, and Jeffrey Wurgler. 2011. Benchmarks as Limits to Arbitrage. Understanding the Low-Volatility Anomaly. Financial Analysts Journal 67: 40–54.
Benartzi, Shlomo, and Richard H. Thaler. 2001. Naive Diversification Strategies in Defined Contribution Saving Plans. The American Economic Review 91: 79–98.
Bender, Jennifer, Remy Briand, Frank Nielsen, and Dan Stefek. 2010. Portfolio of Risk Premia. A New Approach to Diversification. The Journal of Portfolio Management 36: 17–25.
Bessler, Wolfgang, Heiko Opfer, and Dominik Wolff. 2017. Multi-asset portfolio optimization and out-of-sample performance. An Evaluation of Black-Litterman, Mean-Variance, and Naïve Diversification Approaches. The European Journal of Finance 23 (1): 1–30.
Bessler, Wolfgang, Georgi Taushanov, and Dominik Wolff, 2021, Optimal Asset Allocation Strategies for International Equity Portfolios: A Comparison of Country versus Sector Optimization, Journal of International Financial Markets, Institutions and Money, 72, 101343–101362.
Bessler, Wolfgang, and Dominik Wolff. 2015. Do Commodities Add Value in Multi-Asset-Portfolios? An out-of-Sample Analysis for Different Commodity Groups. Journal of Banking and Finance 60: 1–20.
Black, Fischer. 1972. Capital market equilibrium with restricted borrowing. The Journal of Business 45 (3): 444–455.
Black, Fischer, and Robert Litterman. 1992. Global Portfolio Optimization. Financial Analysts Journal 48: 28–43.
Blitz, David, 2011, Strategic Allocation to Premiums in the Equity Market,Journal of Index Investing 2: 42–49.
Briere, Marie, and Ariane Szafarz. 2020. Good Diversification is Never Wasted: How to Tilt Factor Portfolios with Sectors. Finance Research Letters 33 (101197): 1–5.
Google Scholar
Briere, Marie, and Ariane Szafarz, 2021, When it Rains, it Pours: Multifactor Asset Management in Good and Bad Times, Journal of Financial Research , forthcoming.
Carhart, Mark M. 1997. On Persistence in Mutual Fund Performance. The Journal of Finance 52 (1): 57–82.
Coqueret, Guillaume. 2015. Diversified minimum-variance portfolios. Annals of Finance 11: 221–241.
DeMiguel, Victor, Lorenzo Garlappi, and Raman Uppal. 2009. Optimal versus naive diversification. How inefficient is the 1/N portfolio strategy?. The Review of Financial Studies 22: 1915–1953.
Dichtl, Hubert, Wolfgang Drobetz, Harald Lohre, Carsten Rother, and Patrick Vosskamp. 2019. Optimal Timing and Tilting of Equity Factors. The Financial Analysts Journal 75 (4): 84–102.
Dichtl, Hubert, Wolfgang Drobetz, Harald Lohre, and Carsten Rother, 2020, Active factor completion strategies. The Journal of Portfolio Management, 47 (2): 9–37
Dimson, Elroy, Paul Marsh, and Mike Staunton. 2017. Factor-Based Investing. The Long-Term Evidence. The Journal of Portfolio Management 43: 15–37.
Fama, Eugene F., and Kenneth R. French. 1988. Permanent and Temporary Components of Stock Prices. Journal of Political Economy 96 (2): 246–273.
Fama, Eugene F., and Kenneth R. French. 1993. Common Risk Factors in the Returns on Stocks and Bonds. Journal of Financial Economics 33: 3–56.
Fama, Eugene F., and Kenneth R. French. 2015. A Five-Factor Asset Pricing Model. Journal of Financial Economics 116 (1): 1–22.
Fama, Eugene F., and Kenneth R. French. 2018. Choosing Factors. Journal of Financial Economics 128 (2): 234–252.
Frazzini, Andrea, and Lasse H. Pedersen. 2014. Betting Against Beta. Journal of Financial Economics 111: 1–25.
Haugen, Robert A., and A. James Heins, 1972, On the Evidence Supporting the Existence of Risk Premiums in the Capital Markets, Working Paper .
He, Guangliang, and Robert Litterman, 2002, The Intuition Behind Black-Litterman Model Portfolios, Working Paper .
Hjalmarsson, Erik. 2011. Portfolio Diversification across Characteristics. The Journal of Investing 20: 84–88.
Idzorek, Thomas, 2005, A Step-by-Step Guide to the Black-Litterman Model, in: Forecasting Expected Returns in the Financial Markets (Elsevier).
Ilmanen, Antti, and Jared Kizer. 2012. The Death of Diversification Has Been Greatly Exaggerated. The Journal of Portfolio Management 38: 15–27.
James, W., and Charles Stein, 1956, Estimation with Quadratic Loss, in: Proceedings of the Third Berkeley Symposium on Mathematical Statistics and Probability, Volume 1: Contributions to the Theory of Statistics (The Regents of the University of California).
Jones, Robert C., Terence Lim, and Peter J. Zangari. 2007. The Black-Litterman Model for Structured Equity Portfolios. The Journal of Portfolio Management 33: 24–33.
Jorion, Philippe. 1985. International Portfolio Diversification with Estimation Risk. The Journal of Business 58: 259–278.
Jorion, Philippe. 1986. Bayes-Stein Estimation for Portfolio Analysis, . The Journal of Financial and Quantitative Analysis 21: 279.
Kahn, Ronald N., and Michael Lemmon. 2016. The Asset Manager’s Dilemma. How Smart Beta Is Disrupting the Investment Management Industry. Financial Analysts Journal 72: 15–20.
Kim, Jang Ho, Woo Chang Kim, and Frank J. Fabozzi. 2017. Robust Factor-Based Investing. The Journal of Portfolio Management 43: 157–164.
Kondor, Imre, Szilárd Pafka, and Gábor. Nagy. 2007. Noise Sensitivity of Portfolio Selection Under Various Risk Measures. Journal of Banking & Finance 31: 1545–1573.
Landsman, Zinoviy, and Johanna Nešlehová. 2008. Stein’s Lemma for Elliptical Random Vectors. Journal of Multivariate Analysis 99: 912–927.
Lee, Wai, 2000, Theory and methodology of tactical asset allocation (Frank J. Fabozzi Associates, New Hope Pa.).
Lee, Wai. 2017. Factors Timing Factors. Journal of Portfolio Management 43: 66–71.
Maillard, Sébastien., Thierry Roncalli, and Jérôme. Teïletche. 2010. The Properties of Equally Weighted Risk Contribution Portfolios. The Journal of Portfolio Management 36: 60–70.
Markowitz, Harry. 1952. Portfolio Selection. Journal of Finance 7: 77.
Melas, Dimitris, Remy Briand, and Roger Urwin, 2011, Harvesting Risk Premia with Strategy Indices. From Today’s Alpha to Tomorrow’s Beta, MSCI Research Insight .
Opdyke, J.D. 2006. Comparing Sharpe Ratios: So Where are the p-Values?. Journal of Asset Management 8 (5): 308–336.
Pástor, Ľuboš, and Robert F. Stambaugh. 2003. Liquidity Risk and Expected Stock Returns. Journal of Political Economy 111: 642–685.
Satchell, S., and A. Scowcroft. 2000. A Demystification of the Black-Litterman Model: Managing Quantitative and Traditional Portfolio Construction. Journal of Asset Management 1: 2.
Stein, Charles, 1956, Inadmissibility of the Usual Estimator for the Mean of a Multivariate Normal Distribution, in: Proceedings of the Third Berkeley Symposium on Mathematical Statistics and Probability, Volume 1: Contributions to the Theory of Statistics (The Regents of the University of California).
Sharpe, William F. 1964. Capital Asset Prices: A Theory of Market Equilibrium Under Conditions of Risk. The Journal of Finance 19: 325–442.
Download references
Open Access funding enabled and organized by Projekt DEAL.
Author information
Authors and affiliations.
Deutsche Börse Senior Professor of Empirical Capital Market Research, University of Hamburg, Moorweidenstrase 18, 20148, Hamburg, Germany
Wolfgang Bessler
Gothaer Asset Management GmbH, Cologne, Germany
Georgi Taushanov
Technical University Darmstadt and Deka Investment GmbH, Frankfurt, Germany
Dominik Wolff
You can also search for this author in PubMed Google Scholar
Corresponding author
Correspondence to Wolfgang Bessler .
Additional information
Publisher's note.
Springer Nature remains neutral with regard to jurisdictional claims in published maps and institutional affiliations.
Supplementary Information
Below is the link to the electronic supplementary material.
Supplementary file1 (DOCX 15 kb)
Rights and permissions.
Open Access This article is licensed under a Creative Commons Attribution 4.0 International License, which permits use, sharing, adaptation, distribution and reproduction in any medium or format, as long as you give appropriate credit to the original author(s) and the source, provide a link to the Creative Commons licence, and indicate if changes were made. The images or other third party material in this article are included in the article's Creative Commons licence, unless indicated otherwise in a credit line to the material. If material is not included in the article's Creative Commons licence and your intended use is not permitted by statutory regulation or exceeds the permitted use, you will need to obtain permission directly from the copyright holder. To view a copy of this licence, visit http://creativecommons.org/licenses/by/4.0/ .
Reprints and permissions
About this article
Bessler, W., Taushanov, G. & Wolff, D. Factor investing and asset allocation strategies: a comparison of factor versus sector optimization. J Asset Manag 22 , 488–506 (2021). https://doi.org/10.1057/s41260-021-00225-1
Download citation
Revised : 06 May 2021
Accepted : 07 May 2021
Published : 29 May 2021
Issue Date : October 2021
DOI : https://doi.org/10.1057/s41260-021-00225-1
Share this article
Anyone you share the following link with will be able to read this content:
Sorry, a shareable link is not currently available for this article.
Provided by the Springer Nature SharedIt content-sharing initiative
- Asset allocation
- Portfolio optimization
- Factor investing
- Factor versus sector allocation
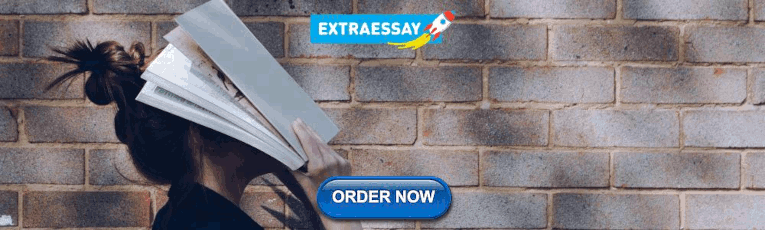
JEL Classification
- Find a journal
- Publish with us
- Track your research

An official website of the United States government
The .gov means it’s official. Federal government websites often end in .gov or .mil. Before sharing sensitive information, make sure you’re on a federal government site.
The site is secure. The https:// ensures that you are connecting to the official website and that any information you provide is encrypted and transmitted securely.
- Publications
- Account settings
Preview improvements coming to the PMC website in October 2024. Learn More or Try it out now .
- Advanced Search
- Journal List
- Springer Nature - PMC COVID-19 Collection

Fixed income mutual fund performance during and after a crisis: a Canadian case
Laleh samarbakhsh.
Ted Rogers School of Management, Ryerson University, 350 Victoria St, Toronto, ON M5B 2K3 Canada
Associated Data
Raw data were purchased from Fundata Inc. The authors are not authorized to share data.
This study investigates the performance of the fixed income mutual funds industry, focusing on Canadian fixed income funds before, during, and after the 2008 global financial crisis. After recessionary shocks, investors look for ways to diversify their systematic risks. We investigate Canadian fixed income funds’ excess returns and dollar fund flow and show that the funds significantly underperform bond markets throughout the study period after controlling for fund characteristics and macroeconomic factors. Consistent with previous studies, we find that this underperformance is more apparent during market downturns. Indicating a spiral relationship, these results suggest that institutional investors perform poorly during financial crises, which is also observable in the volatility patterns in dollar fund flow. We also find that the flow is positively related to the crisis period, indicating that the dollar fund flow in fixed income funds reported a positive value during the recession, signifying an inflow.
Introduction
Mutual funds are portfolios of various financial securities selected by professional fund managers. These funds ensure that the risk factor of their portfolios matches the risk preferences of the investors. A fixed income balanced fund must invest a minimum of 70% of total net assets in Canadian equity and fixed income securities. Of that 70%, fund managers must allocate no more than 40% of assets into equity holdings. As this portfolio combines both equity and debt securities, it is intended for investors with low-to-medium risk preferences. As all mutual funds aim to yield positive returns on investments, mutual fund managers try to use strategies that can outperform standard indexes over specific periods. Various equity funds include other index funds or a combination of small/large capitalization stock. By contrast, debt funds include corporate or government bonds as well as fixed income and gilt funds. 1 The strategies vary according to the individual risk preferences. Investors around the world have different beliefs about risk. Risk-seeking investors invest in riskier assets with a higher beta 2 than the market, whereas risk-averse investors may invest in safer securities with a lower but guaranteed rate of return (e.g., GICs). As part of a diversification strategy, managers often invest in different asset classes and different geographic locations to reduce the overall risk of the portfolio, often referred to as “systematic risk.” The literature has analyzed the contributions made by various parameters of international funds, such as asset and fund size, management expenses, strategies, investment performance, and fees (Ippolito 1989 ; Cumby and Glen 1990 ; Droms and Walker 1994 ). Many U.S. mutual funds that invest abroad deal extensively with equity funds in developed nations but also invest a lesser share in transitional economies because of their higher default risk (Kaminsky et al. 2001 ). Investing in international mutual funds offers a practical way to diversify and earn the highest risk-adjusted return (Droms and Walker 1994 ).
The mutual fund industry averaged positive returns during the 2008 financial crisis (Bello 2008 ). The crisis affected the lives of many individuals around the world due to the collapse of the stock market, the burst of the housing bubble, the increased unemployment rates, and the significant drop in interest rates (Helppie 2011 ). Researchers were led to conclude that specific predatory trading techniques contributed to the crisis. Nevertheless, investors still managed to invest their funds in different markets domestically as well as internationally to diversify their risk and boost portfolio returns. Research dating back to the inception of mutual funds has examined a variety of angles concerning the mutual fund industry, from those who manage the funds (Aggarwal and Jorion 2010 ) to fund performance with respect to market indexes (Cumby and Glen 1990 , Blake et al. 1993 , Leite and Armada 2017 ). While research on equity funds has been accumulating, there remains little practical or academic knowledge on debt funds, such as fixed income funds in Canada. Fixed income funds are investments that pay a fixed rate of return on government bonds, corporate bonds, and other grade bonds. Debt funds have been growing at a higher rate than equity funds. 3 Various global events have impacted financial markets and the lives of many, the most recent being the COVID-19 pandemic. Zenteno and Aquino ( 2020 ) claim that balanced and fixed-income funds suffered the most amongst all fund classes. Based on that observation, this study poses the following research question: How did the financial crisis impact the performance of Canadian fixed income funds? This question can help determine how fixed income funds respond to crisis periods. Institutional investors can use this research to focus on allocation strategies during moments of distress.
Determining how fund flow varies for fixed income funds throughout recessionary periods allows this study to offer a good indication of the economic environment and depict levels of variation in returns that provide an accurate snapshot of the debt funds in the industry. Having clearer insight into fixed income funds in crisis periods allows investors, practitioners, and fund managers to construct portfolios that minimize risk during economic downturns. Identifying performance variations in a specific segment of the mutual fund industry requires that we first understand the overall nature of the mutual fund industry, so that we can better assess how the recession impacted the funds under examination.
The government of Canada weathered the global financial crisis because it prevented top banks from engaging in risky behavior. Due to such policies and the creation of strict regulatory reforms, banks in Canada were spared a total market collapse. For example, in the United States, the collapse of the Lehman Brothers was due primarily to mortgage-backed securities depreciating below their book value, which dried up liquidity in financial markets. Unlike in the United States and Europe, the Canadian economy was not at risk of insolvency. Our research focuses on Canadian mutual funds—specifically, fixed-income mutual funds—which are presumably protected from endogenous crisis factors due to government measures designed to help banks endure the crisis, leading to the stability of the economy during the post-crisis period.
This study examines the impact of flow-induced mutual funds trading in the fixed income market to see how changes in fund size differ between the pre- and post-crisis periods. The rest of the paper is organized as follows. Section 2 reviews the related literature. Section 3 describes the methodology used to conduct the empirical analysis. Section 4 examines the study’s dataset and interprets the results of the empirical analysis. Finally, Section 5 concludes the paper by discussing the results and avenues for future research.
Literature review
Mutual funds are increasing every year. An ordinary investor with nominal capital can pool their money with other like-minded individuals in a managed, diversified basket of stocks and bonds. Few studies focus on debt funds, with equity funds receiving most of the interest in the literature. One type of study analyzes the performance of various categories of equity mutual funds (Aggressive Growth, Small Company, Growth, Growth and Income, Equity Income) during the recessions of 1990 and 2001. This type of study offers intuitive insights into the relationship between stock prices and the economic life cycle of a specific region. One theory, first proven by Moore ( 1983 ), posited that stock prices rose when an economy was in the expanding stages and declined when the economy entered a recession. Stock prices are a good economic predictor, but they can send misleading signals. With this in mind, investors shifted to common stocks from bonds when the economy was booming and did the opposite when the economy was suffering (Moore 1983 ). Mutual funds, or portfolios of funds, consist of small-capitalization stocks 4 during the growth of an economy because the lower rates allow small businesses to capitalize on the opportunity to grow their businesses, which in turn helps boost the economy. When the economy is going through a recession, investors prefer to divert their holdings toward mutual funds that contain mostly large-capitalization stocks. 5
Equity mutual funds have shown greater returns in post-recessionary periods than they did in the recessionary period of 1990. However, in 2001, both the S&P500 Index and mutual funds experienced negative returns during and after the recession. Mutual funds earned a higher return on funds that included a variety of small market cap stocks than did the market both during the recession and in the post-recession period (Bello 2008 ). This is a consistent finding in studies that have examined the relationship between stock prices and economic stages. The creation of a model that emphasizes the effects of equity funds in various economic states led to studies proving that equity funds typically perform better during recessions than during expansionary periods (Glode 2011 ). The question remains whether these patterns continued in the most recent financial crisis of 2008.
Mutual funds have the ability to diversify in various ways and still thrive in downturn economies. A risk-averse mutual fund investor would be more inclined to invest in a collection of low-risk securities such as bond funds. According to the Investment Company Institute ( 2019 ), bond mutual funds hold nearly 22% of total net assets, whereas equity mutual funds hold nearly 43% (Investment Company Institute 2019 ). Measuring the performance of bond-related mutual funds could prove helpful, as it would further our understanding of the nature of debt instruments in portfolios. However, bond funds can exceed investor expectations (Clare et al. 2019 ) or perform below the measured index (Blake et al. 1993 ).
The findings so far have reached no consensus. Thus, it is important to better understand the relationship between bond mutual funds and other similar factors. The relationship a bond mutual fund has with its fund characteristics (e.g., asset size, expense fees, trading strategies/objectives) is like that of equity funds. Like other funds, bond funds were also exposed to volatility during the global crisis, but this was the result of managers’ inability to act according to recessionary signs (Clare et al. 2019 ). A multi-factor model allowed the analysis of bond mutual funds leading up to the financial crisis (Clare et al. 2019 ). Removing error biases, the model led to the argument that bond funds experienced higher returns before the crisis than after. Granted that models are imperfect, but studies have shown strong evidence of performance skewness during hard economic times, when both bond and equity mutual funds were exposed to various risk factors (Clare et al. 2019 ).
Leite and Armada ( 2017 ) find that bond funds significantly underperformed the markets during the financial crisis in Europe. At the same time, however, Europe also experienced the euro sovereign debt crisis, which affected the performance of mutual funds. Bond mutual funds performed better than the market at various economic periods, leading to the possibility that the drastic fluctuations caused the skewness. Leite and Armada ( 2017 ) find that the performance of bond funds continued to improve during the euro sovereign debt crisis. The U.S. pattern was different from that in Europe: U.S. bond funds experienced greater alphas in the post-crisis period than in the pre-crisis period for all maturity sectors (Clare et al. 2019 ). Most debt-driven performance funds use the Capital Asset Pricing Model (CAPM) 6 to price bonds. The CAPM model can prove how low-grade 7 bond mutual funds match the performance of high-grade bond funds over a longer period (Cornell and Green 1991 ). European bond markets have also shown how the past performance of bond funds can repeat (Huij and Derwall 2008 ). This study offers further analysis to compare recessionary impacts on bond performance in various transitional periods.
International funds include a basket of securities from different asset and sector classes from around the world. They allow investors and mutual fund managers to diversify and reduce the systematic risk of their portfolios. Many international mutual funds are compared via specific international indexes like the EAFE 8 or domestic indexes like the S&P 500 (Droms and Walker 1994 ). A more complex and niche study might look at combinations of two different indexes, like the Morgan Stanley World Index and Euro currency deposits (Cumby and Glen 1990 ). The literature has analyzed such international fund parameters as asset and fund size, expenses, strategies, investment performance, and fees (Ippolito 1989 ; Droms and Walker 1994 ; Cumby and Glen 1990 ). Many U.S. mutual funds that invest abroad deal extensively with equity funds in developed nations but also invest a lesser share in transitional economies because of the higher default risk (Kaminsky et al. 2001 ). Investing in international mutual funds offers a practical way to diversify and earn the highest risk-adjusted return (Droms and Walker 1994 ). This is demonstrated by Grinblatt’s, Titman’s, and Jensen’s measurements (Cumby and Glen 1990 ) used to check international fund performance against different benchmark indexes. Grinblatt and Titman’s theories add a timing measure that allows the model to show how different asset classes perform with added information.
In theory, an investor who can foresee the future of the economy will invest in funds and earn a better return than an investor who cannot. Investors could buy equity funds if they expect stock prices to increase. The opposite is true when an increase in interest rates is observed, which leads investors to switch from equity to debt funds (Edwards and Zhang 1998 ). International funds performed better than the average in 1987 until October, when a sudden unexpected shock caused the stock market to crash. As a result, international funds suffered all around the world except in the Japanese market (Cumby and Glen 1990 ).
International mutual funds are used as a proxy in many studies to determine whether international fund indices make up an efficient market. 9 This shows that investors seeking to diversify their holdings would need to include international mutual funds to support a well-balanced portfolio of assets, regardless of the fund class. In the U.S. market, investors choose to invest in low-fee funds and not in actively managed funds 10 (Otten and Bams 2002 ). However, these findings contradict the market growth in Europe, which garnered much attention during the early 2000s, when researchers used that period to analyze the stock market of booming countries within Europe.
This study helps close the gap in the literature on debt funds, primarily that on fixed income funds. The literature is strongly focused on equity-based fund performance, whereas this study examines the recessionary impact on Canadian fixed income funds. As mentioned, the literature on equity funds describes the relationships affecting equity mutual funds on a macro-economic level. By contrast, the literature on bond mutual funds has grown but has failed to establish whether the financial crisis of 2008 affected the performance of Canadian fixed income funds. This study also investigates the size and performance of bond funds to decide if investors are, in fact, investing in bond funds to ensure more security in their investments.
Methodology
This study uses a thorough empirical method to analyze whether the financial crisis led to a significant change in fund flow within specific Canadian fixed income funds. The data consist of funds that have performed both well and poorly. Failure to use funds that were dropped by fund managers due to poor performance may result in survivorship bias, which affects the economic significance (Elton et al. 1996 ) of equity mutual funds. However, the performance bias has no effect on closed-end mutual funds relative to open-ended mutual funds (Bers and Madura 2000 ). Moreover, as new managers use funds during various periods, back-fill bias may skew the results at times. With the rise of new fixed income funds, managers can be provoked to give out information about funds that have shown better-than-average performance. However, Del Guercio and Tkac ( 2002 ) explain that neither of the biases affects flow or performance dramatically.
This study measures the performance of mutual funds as the average monthly return and total net assets. We use dollar fund flow to identify the relationship between fund performance and the inflow/outflow of funds within a fixed income fund group on a monthly basis. Previous studies focus on percentage fund flow (Cao et al. 2008 , Rakowski 2010 ), but this study uses the standard definition employed in the literature. Equation ( 1 ) denotes the change in total net assets minus appreciation (Del Guercio and Tkac 2008; Kolokolova et al. 2020 ). Using this allows for a concise analysis of monthly net flow in Canadian fixed income funds. This definition of fund flow assumes that the flow occurs at the end of the month. Dflow i , t is the monthly net dollar fund flow at time t to an individual mutual fund i :
where Ret i , t is the monthly return of a mutual fund at a point in time, and TNA i , t represents the corresponding total net assets. Therefore, flow is the difference between total net assets at time t and the relationship of a one-month lag of total net assets ( t-1) scaled with the monthly return of the fund at time t. The linear model will enable further insight into how well fixed income funds perform. Comparing the dollar fund flow to a bond index return like that of a bond index is helpful because it directs further analyses toward a benchmark while measuring the change in flow size. Total net assets is a key indicator for determining the change of overall fund size. The monthly return is another performance measure that allows investors to compare the returns of different assets across different time periods. This study’s analysis is broken down into three time periods: Q1.2000–Q2.2007 (pre-crisis), Q3.2007–Q2.2009 (during-crisis), and Q3.2009–2018 (after-crisis) (Bedendo and Bruno 2012 ). The goal is to determine if a significant change to fund flow occurred as a result of the 2008 financial crisis. The following hypothesis and null hypothesis are suggested:
- Ha: There is a higher dollar fund flow in fixed income funds after the 2008 financial crisis than during the crisis.
- Ho: The 2008 financial crisis did not have an impact on the dollar fund flow of fixed income funds.
The dataset comprises a collection of funds from 2000 to 2018 compartmentalized into three classes: Asset, Sector, and Geography. 11 These categories enable different analyses of funds intended to develop a better understanding of the nature of fixed income funds. This study focuses on Canadian fixed income funds. No study has yet provided evidence on the fixed income market in Canada. Thus, the study examines how mutual fund performance varied across periods using comparisons with bond indexes and other Canadian mutual funds. Using key statistical analysis is important for understanding and evaluating the recessionary effects on mutual funds. The study first uses summary and descriptive statistics to acquire a broader understanding of the mutual funds and macroeconomic variables (e.g., monthly return, fund flow, change in total net assets) and enable an evaluation of the funds on a month-to-month basis. Next, an OLS regression analysis determines the statistical significance of the monthly return and dollar fund flow with the corresponding mutual fund and macroeconomic variables. Various models are used to clearly determine the variability of monthly return and dollar fund flow. The study conducts a time series analysis to examine the trends of fixed income bonds compared to short- and long-term bond indexes in the three study periods (pre-recession, during the recession, and post-recession). The time series notes the difference in fund sizes and compares among the returns to evaluate the performance across economic stages. The data analysis merges the data based on a unique fund ID. The data are retrieved from Fundata Inc., Statistics Canada, and the Bank of Canada.
Data and empirical results
The dataset from Fundata Inc. is the primary source for the data used in the analysis on mutual funds, including monthly returns, management fees, fund allocation, and operating expenses for all fixed income funds from 2000 to 2018. Over the sample period, the number of bond funds increased significantly. Of the 30,470 unique funds, this study focuses on fixed income funds in Canada to determine the recession’s impact on performance during and after the 2008 financial crisis. The last sample contains 2493 unique funds as of September 2018. The funds are then divided into six categories: (i) Canadian fixed income, (ii) Canadian fixed income balanced (iii) Canadian short-term fixed income, (iv) Canadian corporate fixed income, (v) Canadian inflation-protected fixed income, and (vi) Canadian long-term fixed income. In addition to Canadian fixed income funds, we also compare the effects of the financial crisis on Canadian equity and global fixed income funds. 12 Investors used to be limited in their choices of investments in heavy-bond funds in Canada. Due to the recession, the industry changed dramatically. First, the need for diversification in the new issue bond market greatly increased the supply of bond-driven funds. Second, rising interest rates and several severe economic shocks sharply increased the number of “safe” securities. As a result, debt-driven bonds now account for 8.18% of the fixed income dataset.
Macroeconomic factors are used to assess fund performance with reference to a relative index. Three-month (short-term) and 10-year (long-term) yields (Bank of Canada 2019 ) are chosen to proxy for the Canadian bond index. The winsorization technique is implemented to ensure the statistical efficiency of the data. This method of eliminating spurious outliers allows us to readjust the mean and variance of the data. In the dataset, values associated with monthly return, total net assets, and management expense ratio (MER) account for a large quantity of extreme outliers. Therefore, following Kolokolova et al. ( 2020 ), we winsorize the lower and upper 1% to reduce the influence of any extreme values. Summary statistics for the fixed income mutual fund and bond yields are provided in Table Table1. 1 . Panel A reports the descriptive statistics of short- and long-term bond yields. The average return on a long-term bond is 1.7 times greater than that of a short-term government bond (3.40% vs. 2.00%). The standard deviation is lower for the long-term bonds than for the short-term bonds (1.42% vs. 1.53%). Bonds, like other trading instruments, carry some degree of risk. Long-term investments are associated with volatility (standard deviation), where short-term investments may be subject to purchasing power risk. With long-term investments, market variability can lead to uncertainty in investments, causing funds to lose value. For short-term investments, however, the return is valued less than the future value as a result of inflation, suggesting that returns lag the inflation rate.
Descriptive statistics
This table reports the descriptive statistics of the data from 2000 to 2018. Panel A reports the macroeconomic variables expressed as monthly values. The 3-month and 10-year Government Bonds are monthly returns, stated in percentages. TSX Index is an index that provides investible opportunities and serves as a benchmark. Panel B describes the characteristics of Fixed Income Fund samples; Total Net Assets (TNA), Fund return (Monthly Return), Management Expense Ratio (MER), and the Dollar Fund Flow (Dollar flow). Panel A reports the descriptive statistics of short and long-term bond yields. The average return on a long-term bond is 1.7 times larger than the short-term government bond (3.40% vs. 2.00%), where the standard deviation of 3 M bonds is 1.07 times greater than 10Y bonds (1.53% vs. 1.42%). When conducting summary statistics, outliers were present and affected the mean and variance of data. The winsorization technique is used to help deal with outliers as well as improve the statistical efficiency of the results. Panel B of Table Table1 1 presents the descriptive statistics of the key fund characteristic of the sample. The fixed income funds category shows a minor positive average of returns of 0.003% with a standard deviation of 0.011%. The average fund size is $300.22 M, with a standard deviation of $1034.07 M
The sample period covers 19 years, from January 2000 to September 2018. It also includes time-series data on monthly returns, dollar fund flow, MER, and TNA. Panel B of Table Table1 1 displays the descriptive statistics of the key variables in the sample. The fixed income funds category shows a minor positive average of returns of 0.003% with a standard deviation of 0.011%. The total net asset size of fixed income funds is $300.22 M, with a standard deviation of $1030.07 M. The standard deviation is extremely high for the funds in the assets because of the significant change in flow—specifically, the fund inflow and outflow throughout the period. On average, the MER is 1.34%, with a standard deviation of 0.64%. Figure Figure1 1 depicts the relationship between bond indexes, fixed income funds, and median fund flow by displaying the trend over time. There are two scale measures; the primary axis (left-hand side) is measured in percentages, while the secondary axis (right-hand side) measures the flow in millions of dollars. The fixed income return is much smaller than that of the government bond yield, but this allows for a visual representation of the variation in returns.

Flow and return 2000 to 2018. Rate of Monthly Returns compared to the ten year (10Y) bond, three month (3M) Bond indexes, and median Fund Flow. The figure plots the average rate of return for Fixed Income Funds and Bond yields from 2000 to 2018 against the primary axis (left-side). The blue line represents the return of Canadian Fixed Income Funds. The red line is the three month Government Canadian Bond Yields. And the yellow line represents the ten year Government Canadian Bond Yield. The bar chart represents the median Dollar Fund Flow of Fixed Income Funds from 2000 to 2018 plotted against the secondary axis in $MM (right-side). As the scales are different for yield and flow, it was necessary to plot the data differently for clarity and visual purposes. The average returns for the 10Y-Bond, 3M-Bond, and Fixed Income Fund are 3.40%, 2.00 and 0.003%, respectively. The average dollar fund flow is −35.31 $MM, representing an outflow. Source: Fundata Canada Inc.; Statistic Canada ( 2019 ); Bank of Canada ( 2019 )
The pattern indicates that the dollar fund flow displayed higher volatility leading up to the crisis period and eventually leveled out to a more stable flow. During the early period, flow fluctuations were abnormally higher than the later periods. During the crisis period, defined by Bedendo and Bruno ( 2012 ) as the period between Q3.2007–Q1.2009, a significant drop in both short-term and long-term bonds is clear; however, the returns did not fall below 0%. The short-term bonds experienced the greatest drop during the financial crisis because of the bonds’ maturity factor. Relative to the government bond indexes, fixed income funds show minimal returns and a large volatility spread during the crisis period. Long-term bond yields steadily declined after 2001, implying that the value of a bond suffers over time due to various uncontrollable macroeconomic factors. We further analyze the dollar fund flow figures within various categories of mutual funds to assess changes in behavior amongst them. Figure Figure2 2 displays the average monthly dollar fund flow of Canadian fixed income, Canadian equity, and global fixed income funds. Naturally, we see peaks and troughs in each category, reflecting market ups and downs.

Dollar fund flow comparison. This figure shows the average Dollar Fund Flow of all Canadian Fixed Income, Canadian Equity, and Global Fixed Income funds from January 2000–September 2018. The breakdown of each category can be found in the Appendix . The highlighted areas within the graphs indicate different crisis periods. The Dotcom bubble (Q2.2001 - Q4.2001), Global financial crisis (Q.42007; Bedendo and Bruno 2012 ), and Euro-Sovereign debt crisis (Q2.2011 - Q1.2013; Leite and Armada 2017 ). Relative to Canadian funds, we see Global Fixed Income funds experiencing a greater drop in fund flow during the financial crisis and the euro-sovereign debt crisis, but a greater increase during the dotcom bubble. Source: Fundata Canada Inc.
At the start of the financial crisis, we see an insignificant change in the flow of Canadian fixed income funds, but we see a greater outflow in Canadian equity and global fixed income funds. In the same figure, we see a slight impact of the “taper tantrum” on the flow of Canadian and global mutual funds. As the pace of bond purchases in the United States slowed, less money was being infused into the economy, resulting in less flow. The effects of the U.S. policy are seen to influence the behavior of Canadian mutual funds. Figure Figure2 2 shows the change in flow during different crisis periods from 2000 to 2018. We can see the fund flow variations for the subcategories of Global Fixed Income funds, Canadian Equity funds, and Canadian Fixed Income funds. We see instances of flow changing though economic distress periods such as Tech bubble aftermath, global financial crisis, and European debt crisis.
We filter the eligible fund flow data for Canadian fixed income, Canadian equity, and global fixed income funds. Then, we run a mean comparison t-test to determine the difference in flow between the two time periods (pre- and post-crisis). The results (see Table Table2) 2 ) suggest that there is a significant difference in fund flow between the two periods, as the p value shows a significance level of less than 1%. The greatest change is observed in Canadian equity funds, where the average flow is negative, signifying an outflow of funds after the financial crisis. Both fixed income categories saw positive flow (inflow) after the crisis, suggesting a change in behavioral patterns among institutional investors after financial distress. As bond prices fluctuate in volatile markets, high-quality bonds do not typically experience the price deviation one would expect to see in the stock market. In this study, most of the fixed income funds invest primarily in investment-grade fixed income securities, meaning that the credit quality of a healthy portfolio is BBB or higher.
Fund flow comparison between mutual fund categories
The table summarizes the average monthly Dollar Flow between Canadian Fixed Income, Canadian Equity, and Global Fixed Income mutual funds from January 2000 to December 2018. The breakdown of each category is available in the Appendix . The pre- and post-crisis periods are defined as Q1.2000–Q3.2007 and Q4.2007–Q4.2018, respectively (Bedendo and Bruno 2012 ). The use of t-tests is needed to measure the statistical significance of the monthly return and the size of each individual fund category. From this preliminary test, we see a significant decrease in fund flow for Canadian Equity Funds relative to other funds. T-statistics are reported in parentheses. ***, **, and * indicate significance at the 1%, 5%, and 10% levels, respectively
T-statistics reported in parentheses
* p < 0.1, ** p < 0.05, *** p < 0.01
Table Table3 3 reports the descriptive statistics of monthly dollar fund flow in yearly format to illustrate the change in flow from year to year. The flow represents the dollar value increase or decrease based on positions held. In general, the dollar fund flow measures the positions held in various categories. The inflow bound by a positive value corresponds to an increase in the position, which is a component for net flow. For instance, Coval and Stafford ( 2007 ) find that mutual funds rely on the flow to determine positions held by investors in equity portfolios. One limitation of the calculations for this sample is the data disparity in 2018. In 2018, the fixed income funds reported monthly total net assets and returns near the end of September 2018. This may not seem like an important issue, but this selection bias may skew the data when scaled in a yearly format. Fund inflow represents an increase in position, meaning that an investor is buying more into the funds, and a fund outflow represents the opposite (Kolokolova et al. 2020 ). The average dollar fund flow for the Canadian fixed income funds only is 112.50, implying that, on average, fixed income funds are increasing in size. The standard deviation is highest during the crisis period. The variation in fund flow indicates a change in holdings; the greater the standard deviation, the greater the change in fund size because of the constant inflow and outflow of funds. More institutional investors are adding fixed income funds in their portfolios to diversify some of the systematic risk they are exposed to through the equity funds.
Annual summary statistic for dollar flow
This table reports the monthly Dollar Fund Flow of Fixed Income Funds for the sample period of 2000–2018, scaled in a yearly format. A positive Flow value represents an inflow, and a negative Flow value represents an Outflow.Flow is calculated using a standard definition of the changes in total net assets minus appreciation (Del Guercio and Tkac 2008 ). The formula is as follows: DollarFlow = TNA i , t − (1 + MonthlyReturn i , t )( TNA i , t − 1) )
To further analyze the fund flows within this context, we break them down to see if we find similar statistically significant correlations with returns. Table Table4 4 reports the mean comparisons for fund flow and return for each fixed income fund during the two time periods (pre- and post-crisis, defined as Q1.2000–Q3.2007 and Q4.2007–Q4.2018 respectively; Bedendo and Bruno 2012 ). The Canadian fixed income subcategory is defined as follows: “Funds in the Canadian Fixed Income category must invest at least 90% of their fixed income holdings in Canadian dollars with an average duration greater than 3.5 years and less than 9.0 years” (Canadian Investment Funds Standards Committee 2017 ). These saw increased flow after the global financial crisis, but the difference in means is not statistically significant. The dollar fund flow for each subcategory of Canadian fixed income funds was positive after the crisis, except for Canadian inflation-protected fixed income (−81.10) and Canadian short-term fixed income funds (−0.236). The Canadian long-term fixed income fund saw the highest percentage increase in dollar fund flow after the financial crisis (111.114). For each subcategory, all fixed income funds experienced 0.13% lower monthly returns after the crisis. As fund flow for all fixed income funds increased, the monthly return decreased. The substantial increase in fund flow after the crisis is partly due to the crisis itself. Institutional investors are investing more in these mutual funds to help diversify some of the risks. Overall, we find evidence of significant differences among the Canadian fixed income fund categories. Over time, these funds experienced natural changes brought on by market conditions.
Pre- and post-crisis: flow and return of canadian fixed income funds
This table summarizes the average Monthly Dollar Fund Flow (Panel A) and Monthly Return (Panel B) of all Canadian Fixed Income Fund categories from January 2000 to December 2018. The pre- and post-crisis periods are defined as Q1.2000–Q3.2007 and Q4.2007–Q4.2018, respectively (Bedendo and Bruno 2012 ). Monthly return is winsorized at the 5% level at each tail to ensure statistical efficiency. The use of t-tests is needed to measure the statistical significance of monthly return and the size of each individual fund category. We see a convex relationship between flow and return on fixed income funds after the financial crisis. T-statistics are reported in parentheses. ***, **, and * indicate significance at the 1%, 5%, and 10% levels, respectively
Table Table5 5 reports a comparison of the monthly means of the Canadian fixed income fund return between the three time periods. We report a t-test of the two groups to determine statistical significance and fit. The full sample period is used as the base to compare the means. The mean return over time is positive, and we see a lower return during the post-crisis stage, when the spread is (0.257% vs 0.243%) 1.4 bps. The return is relatively close to the median (0.257% vs 0.247%), indicating that the returns are not affected by any outliers, and the data are marginally skewed to the right. The pre- and post-crisis periods are statistically significant at the 1% level, which is useful for developing the regression models.
Summary statistics on the returns of fixed income portfolio
This table summarizes the average monthly return of fixed income funds from 2000 to 2018 and provides a breakdown of the funds during three economic periods. The pre-, during-, and post-crisis periods are defined as Q1.2000–Q2.2007, Q3.2007–Q1.2009, and Q2.2009–Q4.2018, respectively (Bedendo and Bruno 2012 ). The winsorization technique is used to help deal with outliers and improve the statistical efficiency of the results. As the preliminary results indicate the anticipated negative returns during recessionary times, fixed income funds yield a greater return than during the pre- and post-stages. The use of t-tests is needed to measure the statistical significance of monthly returns in different periods. The full sample period is used as the base to compare each period separately. The first difference of means is between the entire period and the pre-crisis period, the second is between the entire period and the during-crisis period, and the last is between the entire period and the post-crisis period. T-values are reported in parentheses. ***, **, and * indicate significance at the 1%, 5%, and 10% levels, respectively
The greatest variation in return is observed during the crisis period (1.498%) because funds have much higher volatility during economic crisis periods than during expansionary periods (Leite and Armada 2017 ). The performance, as measured in terms of return, declines marginally over time. Regarding the timing indicators, the pre-crisis mean returns are 1.6 times greater than the returns in the post-crisis period. Investors may experience some gains but would also suffer an above-average market loss in the process. The observations during the post-crisis period account for 85% of the sample because of the number of funds that were created and filled after the crisis. The anticipated growth in fixed income funds following the crisis can be attributed to several factors, such as the capitalization of opportunity, which leads to macroeconomic uncertainty and the need for low-risk portfolios. The low-interest rates in Canada during the last 10 years ultimately led to the creation of new fixed income funds. As the interest rates decreased, the value of issued bonds increased. The increase in the rates of many debt funds induced investors and fund managers alike to take advantage of the opportunity.
The correlation analysis presented in Table Table6 6 reports the pairwise correlation coefficients between variables in order to identify the numeric strength of the macroeconomic factors and key control variables. P -values are reported in parentheses. The correlation coefficient is shown between the average monthly return, dollar fund flow, management fee, mutual fund age, government bond indexes, and the TSX index. The dollar fund flow forms a weak positive correlation with the 10Y and 3 M government bond indexes (0.001 vs. 0.006) and is deemed to be statistically significant, contrary to the findings of Kolokolova et al. ( 2020 ). Although the coefficients are minuscule and point to no correlation, this result suggests that, as dollar fund flow increases for fixed income mutual funds, long- and short-term government yields also increase. The positive relationship between dollar fund flow and return indicates that, as the aggregate flow of the fund increases, the return also increases. However, the coefficient between fund flow and return is reported as a negative correlation (−0.001) and is statistically insignificant. The significant correlations between the three macroeconomic variables ( 10Y Bond , 3 M Bond , and TSX Index ) reveal that bonds move in harmonious wavelengths with one another. As the TSX index increases, the correlation coefficients indicate a higher negative factor for long-term bonds as opposed to short-term bond yields (−0.583 and − 0.280). This may raise multicollinearity issues; however, they are not a concern because the index is used only in combination with all control variables when estimating the regression models.
Correlation analysis
This table reports the pairwise correlation coefficients over time for the 2000–2018 sample period between the variables used in this study. Dollar Flow is the growth size of a specific fund; Total Net Assets is the monthly average used to measure the total value of a fund; MER is the Management Expense Ratio, which represents an associated fee for a fund; Monthly Return is the log return on fixed income funds in the dataset; TSX Index denotes the monthly average index values of the TSX Market; Long Term Bond is the 10-Year Government Bond index; Short Term Bond is the 3-Month Government Bond index, and Dollar Flow is the monthly dollar fund flow of fixed income funds. P -values are reported in parentheses. ***, **, and * indicate significance at the 1%, 5%, and 10% levels, respectively
Table Table7 7 reports the estimation results for Eqs. ( 2 ), ( 3 ), and ( 4 ). As shown in Eq. ( 2 ), the dollar fund flow is regressed on the average monthly total net assets, monthly return, the monthly management expense ratio, and the age of the fund; the combination of macroeconomic short- and long-term bond yields and the market index is described in Eqs. ( 3 ) and ( 4 ):
Dollar fund flow regression
The table presents the results of the OLS regressions explaining the monthly dollar fund flow of unbalanced Fixed Income Mutual Funds from 2000 to 2018. Fixed Effect estimation is used to control for variables to avoid collinearity within the unbalanced sample data. Here, Dflow is the dependent variable of the monthly flow of fixed income funds; TNA is the monthly average of Total Net Assets; MFRet is the average monthly return of an individual fund; MER is the average monthly Management Expense ratio indicating an associated fee; 10Y bond is the monthly average yield of the Canadian 10-Year maturity bonds; 3 M bond is the monthly average yield of the Canadian 3-Month maturity bonds; and the TSX is the monthly index performance for the TSX Composite Index. Crisis is a dummy variable taking a value of 1 from Q3.2007–Q1.2009 (Bedendo and Bruno 2012 ) and 0 otherwise. The bond yield data are obtained from the Bank of Canada (Bank of Canada 2019 ) website, and the TSX index data are retrieved from the Government of Canada (Statistics Canada 2019 ). P-values are given in parentheses. *, **, and *** indicate significance at 1%, 5%, and 10% levels, respectively. The general baseline model is given by the equation below: Dflow i , t = β 1 ( TNA i , t ) + β 2 ( MFRet i , t ) + β 3 ( MER i , t ) + β 4 ( Age ) + ε i
where MFRet represents the average monthly return of fixed income funds during the sample period, TNA is the average monthly total net assets during the sample period, MER is the average monthly fee deducted from the portfolio (also known as the “Management Expense Ratio”), and Age is a mutual fund characteristic reflecting the age of a fund from its date of inception.
where 10 YBond and 3 MBond are monthly average long- and short-term bond yields, respectively. Models (3) and (4) include the average monthly index figure, denoted by TSX Index . Models (5) and (6) use a dummy variable to control for the effects of the financial crisis. Models (1) and (2) include the control variables used to model dollar fund flow. All regression models are treated with fixed effects to exploit the anomalies within a certain subset of groups. OLS regression models may omit certain variables that are not time-invariant, and this would cause biases. Fixed effects incorporate all the characteristics of funds that do not change over time and include the effects of time-invariant characteristics that are hard to measure. When macroeconomic interactions are included in models (3) to (6), the adjusted R 2 increases by 0.20%. Flow is positively related to total net assets and age when only fund characteristics are estimated. The coefficients from the OLS regression, followed by fixed effects in parentheses, of 0.99(1.01) and − 0.01(−0.01) are statistically significant at the 1% level. The interactions between the combination of age and flow, or of fees and flow, in model 3 are not statistically significant. However, they include market factors that are significant at the 1% and 5% levels. An additional increase of 50 bps in long-term bonds results in an increase of 25.48 bps in fund flow. An increase of 50 bps in short-term bonds results in a decrease in fund flow of 0.11 bps. The economic significance suggests that both government bond yields reflect the different nature of bond funds. To verify the robustness of the results, we examine the TSX index to evaluate the impact of the dollar fund flow on fixed income funds. The relationship between equity and bond markets has been noted in theory; the regression models suggest that, when the price of equity goes up, bond yields go down, forming an inverse relationship. With the increase in the TSX index, a proxy for equity markets, we observe an outflow of funds, indicating that the allocation of assets is being repositioned. This illustrates the foundational theory’s suggestion that, as investors see a rise in equity markets, bond markets lose value, thus creating an outflow. Fund flows can reflect the risk preferences of investors and also provide context for the influence of investment decisions within the market (Kamstra et al. 2017 ).
We compare the regression estimates from Table Table7 7 to those in Table Table8 8 to measure the impact of the crisis on fixed income and equity funds. We find that the size of Canadian and global fixed income funds increased after the crisis to a much greater extent than Canadian equity funds, indicating a change in investing behavior. As mentioned, institutional investors invest more capital into fixed income mutual funds, thus strengthening the hypothesis that fund flow increases after the financial crisis. Inversely proportional in both periods, as the flow increases, monthly returns for Canadian fixed income funds decrease by 9.20%, which is statistically significant at the 1% level. The opposite is true for Canadian equity funds, where a one standard deviation increase in flow increases returns by 39.58%.
Categorical dollar fund flow regression
The table presents the estimations of regression models explaining the monthly dollar flow of each Canadian Fixed Income, Canadian Equity, and Global Fixed Income mutual fund from 2000 to 2018. Fixed Effect estimation is used to control for variables to avoid collinearity within the unbalanced sample data. Panel A reports the estimates of the regression for funds during the pre-crisis period, whereas panel B reports the regression results for funds during the post-crisis period. The Crisis dummy variable takes the value of 1 from Q3.2007–Q4.2018 (Bedendo and Bruno 2012 ) and 0 otherwise. The bond yield data are obtained from the Bank of Canada website, and the TSX index data are retrieved from the Government of Canada (StatsCan). T-statistics are in parentheses. *, **, and *** indicate significance at the 1%, 5%, and 10% levels, respectively
*p < 0.1, **p < 0.05, ***p < 0.01
Table Table9 9 shows the results for each sub-period to depict the impact of the variables on dollar fund flow. Most estimations are statistically insignificant, but we see the relationships between certain variables in specific periods. The results show that, during the post-crisis era, there are linkages between long-term bond yields and fund flow. The coefficient of 59.02 is statistically significant at the 1% level. Economically, as bond yields increase, estimates of the flow of funds also increase, implying a positive flow. This further proves that flow has a positive relationship with long-term bonds. In the first two stages (pre-crisis and during), the R 2 increases by nearly 2% from 46.71% to 48.56%, respectively, for the models that include mutual fund characteristics only. The same is observed when the macroeconomic variables are incorporated. This indicates that, during the recession, most portfolios and stocks experienced a loss in investments, despite the diversification strategies used (Helppie 2011 ). These findings are also consistent with those of earlier studies that have investigated recessionary impacts and have found that bond funds earn marginally higher returns during pre-crisis periods than they do afterwards (Clare et al. 2019 ). Not only did size increase after the crisis, but returns were marginally greater than zero, indicating gains on the holdings. However, there are several limitations to the study. One important limitation that may impact the findings on flow and return are the macroeconomic variables, which might account for the full impact of economic activity. For instance, including variables such as inflation or price of commodities as a proxy could enable a better adjustment for those outside factors in this model. Overall, there are strategies in place to diversify funds in different categories within fixed income funds.
Sub-period dollar fund flow regression
This Table presents the results of the OLS regressions and Fixed Effect modelling to explain the monthly dollar fund flows of unbalanced Fixed Income Mutual Funds from 2000 to 2018 in the three periods. The pre-crisis, during-crisis, and post-crisis periods are defined as Q1.2000–Q2.2007, Q3.2007–Q1.2009, and Q2.2009–2018, respectively (Bedendo and Bruno 2012 ). Here, Dflow is the dependent variable of the monthly flow of fixed income funds; TNA is the monthly average of Total Net Assets; MFRet is the average monthly return of individual funds. MER is the average monthly Management Expense ratio indicating an associated fee; Age is the age of the mutual fund, 10Y bond is the monthly average yield of the Canadian 10-Year maturity bonds; 3 M bond is the monthly average yield of the Canadian 3-Month maturity bonds, and TSX is the monthly index performance for the TSX Composite Index. The bond yield data were obtained from the Bank of Canada (Bank of Canada 2019 ) website, and the TSX index data were retrieved from the Government of Canada (Statistics Canada 2019 ). T-statistics are given in parentheses. ***, **, and * indicate significance at the 1%, 5%, and 10% levels, respectively
Future studies on this topic could seek to determine the allocation breakdown within fixed income funds during the 2008 financial crisis. Incorporating seasonality studies of fund categories, like that of Kamstra et al. ( 2017 ), can help determine the changes in the asset allocation of Canadian fixed income funds. This would allow us to understand the composition of funds, and it would also enable further testing to examine investor sentiment. Further research on the flows of long- and short-term bonds could also provide an indication of how fixed income mutual funds impact government bonds and other debentures.
Our results show that, for the overall sample period, Canadian fixed income funds underperformed the bond markets. This underperformance is more apparent during recessionary periods than during economic expansion periods, but the bond fund returns are lower than the market average during both periods (Leite and Armada 2017 ). When evaluating the different stages, we find significant variation in fund flow leading up to the financial crisis as well as during the crisis. This suggests that institutional investors such as mutual funds act differently in periods of distress, which leads to patterns of volatility in fund flow. These findings are in line with Rakowski and Wang ( 2009 ), who suggest that variation in behavioral patterns are observed through fund flow. The change in Canadian fixed income funds indicates that investors are transitioning their holdings to other sectors or assets, which is observed through total net assets and fund flow.
In this study, DollarFlow is used to measure the interactions between mutual fund characteristics and macroeconomic variables during various states of the economy. Prior studies have focused on equity-driven funds, which outperformed more during the recession than they did during post-recession periods (Glode 2011 ). In contrast to debt-driven funds, these funds have been shown to experience higher returns before the crisis than after Clare et al. ( 2019 ). This study reinforces the finding that returns were higher in the pre-crisis period but also finds a significant change in the total size of the holdings of Canadian fixed income funds. These results strengthen the view that flows were higher in the post-crisis period than during the crisis period, but do not explain the correlation between changes in the asset allocation of fixed income funds during the three periods. Dollar fund flow is positively related to the crisis period, indicating that, during the recession, the dollar fund flow in fixed income funds had a positive value, signifying an inflow. The monthly return follows the same path taken by flow. Institutional investors can use these findings to build an allocation strategy during periods of distress. By showing how the flow size varied for fixed income funds during recessionary times, this study presents a clear picture of the economic environment and depicts levels of variation in returns, offering an accurate snapshot of the debt funds in the industry.
Code availability
STATA code available upon editor’s request.
Dr. Samarbakhsh acknowledges funding from the Social Sciences and Humanities Research Council of Canada (SSHRC).
Data availability
Declarations.
Not Applicable.
1 Long-term debt trading funds that have an above-average risk profile.
2 Risk of a stock based on the overall market. The greater the risk, the higher the beta.
3 Investment Company Institute. 2019 . “Annual Report for Members”. Accessed November 15, 2019. https://www.ici.org/pubs/annuals .
4 Small businesses that have a market cap between $300 M and $2B, measured by the stock price and any outstanding shares.
5 Medium-to-large businesses that have a market cap of over $5B.
6 This model is used to price assets given their current risk level. It measures the expected return on an asset given the market risk-free rate and the market risk premium.
7 Low-grade mutual funds must have most of their portfolio invested in corporate bonds rated BAA or lower by Moody’s or by Standard & Poor’s Index. In this case, bonds classified as low-grade have higher yields because the risk of default is higher. (The opposite is true for high-grade rated bonds.)
8 Index used to compare performance outside of North America. EAFE stands for “Europe, Australasia, Far East.”
9 Market efficiency is reached when the market has the knowledge necessary to evaluate the prices of stocks. This would mean that stocks are neither undervalued nor overvalued.
10 Active managed funds are those in which a manager or team of managers makes regular decisions to invest funds for their clients.
11 See Exhibit A in the Appendix for a breakdown of categories.
12 See Exhibit B in the Appendix for a breakdown of Canadian equity and global fixed income funds.
Publisher’s note
Springer Nature remains neutral with regard to jurisdictional claims in published maps and institutional affiliations.
Contributor Information
Laleh Samarbakhsh, Email: ac.nosreyr@hshkabramasl .
Meet Shah, Email: [email protected] .
- Aggarwal RK, Jorion P. The performance of emerging hedge funds and managers. J Financ Econ. 2010; 96 (2):238–256. doi: 10.1016/j.jfineco.2009.12.010. [ CrossRef ] [ Google Scholar ]
- Bank of Canada. (2019) Selected Bond Yields . Accessed December 1, 2019. https://www.bankofcanada.ca/rates/interest-rates/canadian-bonds/
- Bedendo M, Bruno B. Credit risk transfer in U.S. commercial banks: What changed during the 2007–2009 crisis? Journal of Banking & Finance 36. 2012; 12 :3260–3273. doi: 10.1016/j.jbankfin.2012.07.011. [ CrossRef ] [ Google Scholar ]
- Bello, Zakri Y (2008) The performance of U.S. domestic equity mutual funds during recent recessions. Global Journal of Finance and Banking. Issues 3, no. 2
- Bers MK, Madura J. The performance persistence of closed-end funds. Financ Rev. 2000; 35 (3):33–52. doi: 10.1111/j.1540-6288.2000.tb01420.x. [ CrossRef ] [ Google Scholar ]
- Blake CR, Elton EJ, Gruber MJ. The performance of bond mutual funds. J Bus. 1993; 66 :371–403. doi: 10.1086/296609. [ CrossRef ] [ Google Scholar ]
- Canadian Investment Funds Standards Committee (2017) Mutual funds. Accessed August 10, 2020. http://www.cifsc.org/
- Cao C, Chang EC, Wang Y. An empirical analysis of the dynamic relationship between mutual fund flow and market return volatility. J Bank Financ. 2008; 32 (10):2111–2123. doi: 10.1016/j.jbankfin.2007.12.035. [ CrossRef ] [ Google Scholar ]
- Clare A, O'Sullivan N, Sherman M, Zhu S. The performance of U.S. bond mutual funds. Int Rev Financ Anal. 2019; 61 :1–8. doi: 10.1016/j.irfa.2018.12.001. [ CrossRef ] [ Google Scholar ]
- Cornell B, Green K. The investment performance of low-grade bond funds. J Financ. 1991; 46 (1):29–48. doi: 10.1111/j.1540-6261.1991.tb03744.x. [ CrossRef ] [ Google Scholar ]
- Coval J, Stafford E. Asset fire sales (and purchases) in equity markets. J Financ Econ. 2007; 86 (2):479–512. doi: 10.1016/j.jfineco.2006.09.007. [ CrossRef ] [ Google Scholar ]
- Cumby RE, Glen JD. Evaluating the performance of international mutual funds. J Financ. 1990; 45 (2):497–521. doi: 10.1111/j.1540-6261.1990.tb03700.x. [ CrossRef ] [ Google Scholar ]
- Del Guercio D, Tkac PA. The determinants of the flow of funds of managed portfolios: Mutual funds vs. pension funds. Journal of Financial and Quantitative Analysis. 2002; 37 (4):523–557. doi: 10.2307/3595011. [ CrossRef ] [ Google Scholar ]
- Del Guercio D, Tkac PA. Star power: the effect of monrningstar ratings on mutual fund flow. J Financ Quant Anal. 2008; 43 (4):907–936. doi: 10.1017/S0022109000014393. [ CrossRef ] [ Google Scholar ]
- Droms WG, Walker DA. Investment performance of international mutual funds. J Financ Res. 1994; 17 (1):1–14. doi: 10.1111/j.1475-6803.1994.tb00170.x. [ CrossRef ] [ Google Scholar ]
- Edwards FR, Zhang X. Mutual funds and stock and bond market stability. J Financ Serv Res. 1998; 13 (3):257–282. doi: 10.1023/A:1008084311260. [ CrossRef ] [ Google Scholar ]
- Elton EJ, Gruber MJ, Blake CR. Survivor bias and mutual fund performance. Rev Financ Stud. 1996; 9 (4):1097–1120. doi: 10.1093/rfs/9.4.1097. [ CrossRef ] [ Google Scholar ]
- Fundata Canada Inc. (2019) Canadian mutual fund, ETF and stock data provider | Fundata Canada Inc. Fundata Canada Inc. Accessed September 10, 2019. http://www.fundata.com/
- Glode V. Why mutual funds "underperform". J Financ Econ. 2011; 99 (3):546–559. doi: 10.1016/j.jfineco.2010.10.008. [ CrossRef ] [ Google Scholar ]
- Helppie McFall, B. (2011). Crash and wait? The impact of the Great Recession on the retirement plans of older Americans. American Economic Review, 101(3), 40–44 [ PMC free article ] [ PubMed ]
- Huij J, Derwall J. "hot hands" in bond funds. J Bank Financ. 2008; 32 (4):559–572. doi: 10.1016/j.jbankfin.2007.04.023. [ CrossRef ] [ Google Scholar ]
- Investment Company Institute. (2019). Annual report for members. Accessed November 15, 2019. https://www.ici.org/pubs/annuals
- Ippolito RA. Efficiency with costly information: A study of mutual fund performance, 1965–1984. The Quarterly Journal of Economics. 1989; 104 (1):1–23. doi: 10.2307/2937832. [ CrossRef ] [ Google Scholar ]
- Kaminsky G, Lyons R, Schmukler S. Mutual fund investment in emerging markets: an overview. Boston, MA: International financial contagion. Springer; 2001. pp. 157–185. [ Google Scholar ]
- Kamstra MJ, Kramer LA, Levi MD, Wermers R. Seasonal asset allocation: evidence from mutual fund flows. J Financ Quant Anal. 2017; 52 (1):71–109. doi: 10.1017/S002210901600082X. [ CrossRef ] [ Google Scholar ]
- Kolokolova O, Lin M-T, Poon S-H. Too big to ignore? Hedge fund flows and bond yields. J Bank Financ. 2020; 112 :105271. doi: 10.1016/j.jbankfin.2017.12.009. [ CrossRef ] [ Google Scholar ]
- Leite P, Armada MR. Bond fund performance during recessions and expansions: Empirical evidence from a small market. International Review of Finance. 2017; 17 (1):163–170. doi: 10.1111/irfi.12098. [ CrossRef ] [ Google Scholar ]
- Moore GH (1983) Security markets and business cycles. n Business Cycles, Inflation, and Forecasting, 2nd edition, pp. 139–160. Ballinger
- Otten R, Bams D. European mutual fund performance. Eur Financ Manag. 2002; 8 (1):75–101. doi: 10.1111/1468-036X.00177. [ CrossRef ] [ Google Scholar ]
- Rakowski D. Fund flow volatility and performance. J Financ Quant Anal. 2010; 45 (1):223–237. doi: 10.1017/S0022109009990500. [ CrossRef ] [ Google Scholar ]
- Rakowski D, Wang X. The dynamics of short-term mutual fund flows and returns: a time-series and cross-sectional investigation. J Bank Financ. 2009; 33 (11):2102–2109. doi: 10.1016/j.jbankfin.2009.05.001. [ CrossRef ] [ Google Scholar ]
- Statistics Canada. (2019). Table 10-10-0122-01 Financial Market Statistics. Accessed November 30, 2019. https://www150.statcan.gc.ca/t1/tbl1/en/tv.action?pid=1010012201
- Zenteno, M. & Aquino, J. (2020, May 1). Canada mutual funds post record redemptions as COVID-19 worries grow. Retrieved August 13, 2020, from https://rsmcanada.com/our-insights/coronavirus-resource-center/canada-mutual-funds-post-record-redemptions-as-covid-19-worries-.html
Title: Predicting the net asset value of mutual fund: an extended literature review
Authors : Shikha Singla; Gaurav Gupta
Addresses : Department of Computer Science and Engineering, Punjabi University, Patiala (Pb), India ' Department of Computer Science and Engineering, Punjabi University, Patiala (Pb), India
Abstract : Mutual funds have emerged as the most dynamic segment of the Indian financial system. With its potential to provide higher return by investment in diversified securities, mutual funds emerge as one of the most promising investments in uncertain markets. With a variety of mutual funds competing in the present scenario market, it becomes a challenging decision for the investor to balance risk and return trade-off on the portfolio to maximise returns. Therefore, the important aspect for portfolio manager is to predict the net asset value (NAV) of mutual funds. Various methods and techniques in the field of economics and computer science have been used in the quest to gain insights into NAV prediction. The aim of the paper is to provide an extended literature review of different techniques in different areas of computer science in order to explore the future possibilities. This paper explores the past research work and proposes the future roadmap to predict the NAV of mutual funds by using different techniques with greater accuracy.
Keywords : net asset value; NAV; radial basis function; RBF; functional link artificial neural network; FLANN; mutual fund.
DOI : 10.1504/IJAIS.2021.117841
International Journal of Adaptive and Innovative Systems, 2021 Vol.3 No.1, pp.14 - 24
Received: 18 Mar 2019 Accepted: 07 Feb 2020 Published online: 04 Oct 2021 *
Keep up-to-date
- Our Newsletter ( subscribe for free )
- New issue alerts
- Inderscience is a member of publishing organisations including:
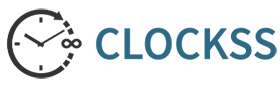
Predicting the net asset value of mutual fund: an extended literature review
- Related Documents
Thai Equity Mutual Fund Net Asset Value Return Prediction Using Internal Factors
Pengaruh inflasi, bi-7 day reverse repo rate, kurs, jumlah uang beredar dan indeks harga saham gabungan terhadap nilai aktiva bersih reksa dana saham.
The purpose of this study is to examine the effect of inflation, BI-7 day reverses repo rate, exchange rate, the money supply, and composite stock price index on the net asset value of stock mutual funds. The population of this study is the stock mutual fund which was listed on the financial services authority in 2017-2020. The results of this study indicate that simultaneously inflation, BI-7 day reverse repo rate, exchange rate, the money supply, and composite stock price index affect the net asset value of the stock mutual fund. Partially, this study show BI-7 day reverse repo rate has a positive and significant effect on the net asset value of a stock mutual fund. The exchange rate has a positive and significant effect on the net asset value of stock mutual funds. The composite stock price index has a positive and significant effect on the net asset value of stock mutual funds. The money supply has a negative and significant effect on the net asset value of a stock mutual fund while inflation has no significant effect on the net asset value of a stock mutual fund.
A Factorial Analysis of Information Ratio and Its Causal Effect on Yearly Return of Net Asset Value – A Study of Indian Equity Diversified Mutual Fund Schemes
Evaluation of performance of mutual fund schemes has gained a wide range of attention from both investors and academicians. The study aims at assessing the returns from equity mutual fund schemes in India by applying risk adjusted performance evaluation techniques. The study is based on secondary data collected for ten years for selected open ended equity diversified mutual funds. A comparative assessment of performance of public sector sponsored equity funds and non-government sponsored sector funds bring forth with an interesting inference. The present study also constitutes a modest attempt to assess the information ratio and its causal effect on the average yearly return of Net Asset Value (NAV). Based on the previous research findings, this paper puts an honest effort to identify twelve independent variables which affects significantly the performance of NAV. The evaluation relies on the Sharpe, Trenor and Jensen’s technique, which have been applied in conjunction with parametric and non-parametric statistical tools using. The result shows significant relationship exists between the NAV return and fund’s risk, information ratio, macro-economic variables such as inflation, interest rates, market index performance, foreign flow of funds and foreign exchange on the basis of regression analysis.
Neural Network Models for Forecasting Mutual Fund Net Asset Value
Time series models to predict the net asset value (nav) of an asset allocation mutual fund vwelx, indonesian mutual funds classification using clustering method.
Mutual funds have existed since 1990 as an alternative investment in Indonesia. The objective of this research is to examine the existing classification of mutual funds database. The data of mutual funds is taken from Bloomberg through Portal Reksadana 2013 which covered 690 mutual funds. The existing classification consists of mutual funds fixed income (reksadana pendapatan tetap), equity (reksadana saham), money market (reksadana pasar uang) and structured (reksadana campuran). The existing financial attributes consists of the net asset value, percentage annualized return the last 6 months, 1 year, 3 years, 5 years and year-to-date. This paper uses K-means clustering to propose new classification of Indonesian mutual funds. The result reveals that mutual funds in equity and fixed income belong to its group. However, mutual funds money market is belong to mutual fund fixed income and mutual funds structures are identified to mutual funds equity. Furthermore, we find that in average 43% of Indonesian mutual funds are misclassified in accordance with their attributes. Finally, it is suggested to re-group the mutual funds into smaller classification, which has lower rates of misclassified mutual funds and possibility to achieve better performances in terms of its percentage annualized return.
PENGARUH INFLASI, NILAI TUKAR RUPIAH, BI RATE TERHADAP NILAI AKTIVA BERSIH REKSA DANA TERPROTEKSI SYARIAH PERIODE 2014-2018 DI INDONESIA
The Research aims to determine the influence of inflation, currency exchange rate, interest rate that affect net asset value of protected Islamic mutual fund from 2014-2018 in Indonesia partially and simultaneously. The research also uses quantitative approach and multiple regression analysis technique. The sample used for this research is obtained from monthly statistic data of Bank Indonesia, Otoritas Jasa Keuangan, and Pusat data kontan which had been published online from January 2014 to Desember 2018, so there are 60 samples taken for this reserch. The variabels of this research include inflation (X1), currency exchange rate (X2), interest rate (X3), and net asset value of protected Islamic mutual fund (Y). The result of this research shows that partially, the inflation affect Net Asset Value of Protected Islamic Mutual Fund from 2014-2018 in Indonesia insignificantly, currency exchange rate affect Net Asset Value of Protected Islamic Mutual Fund from 2014-2018 in Indonesia significantly, and interest rate affect Net Asset Value of Protected Islamic Mutual Fund from 2014-2018 in Indonesia significantly. Simultaneously, all independent variables affect Net Asset Value of Protected Islamic Mutual Fund from 2014-2018 in Indonesia significantly.Keywords: Macroeconomic, Inflation, InterestRate, Currency Exchange Rate, Net Asset Value, Protected Islamic Mutual Fund
Exchange-traded funds as an alternative investment option
We conduct an analysis of Exchange-traded Funds (ETFs), Index and Equity mutual funds and their respective benchmark during the 2010-2015 period for the Portuguese fund industry. For the period 2010-2017, we test ETFs for price inefficiency (existence of deviations between prices and the Net Asset Value) and persistence. We find that the studied ETF does not always outperform index funds in replicating the variations of the PSI 20 index, despite exhibiting better tracking ability when facing downside deviations of the benchmark and a better capacity of smoothing tracking deviations. Regarding ETFs price efficiency and its persistence, the study reveals that the examined ETF is priced at a low average discount with evidence of deviations persistence of at least two days. The investment schemes with the highest ability to track the PSI 20 Index were PSI20 (ETF), BBVA PPA Índice PSI20, and the equity mutual fund BPI Portugal.
PENGARUH SERTIFIKAT BANK INDONESIA SYARIAH (SBIS), INDEKS HARGA SAHAM GABUNGAN (IHSG), DAN NILAI TUKAR RUPIAH TERHADAP NILAI AKTIVA BERSIH (NAB) DANAREKSA SYARIAH BERIMBANG
<p>The purpose of this study is to determine the factors that influence the growth of the Net Asset Value (NAV) of a mutual fund sharia, namely Sharia Bank Indonesia Certificates (SBIS), Composite Stock Price Index (CSPI) and Exchange Rate (USD). All of the factor are assumed have significant impact on the net asset value (NAV). Danareksa Syariah Berimbang of PT. Danareksa Management. The research method is done by multiple regression. The results showed there is negative influence significant between Sharia Bank Indonesia Certificates (SBIS) and exchange rate, there is positive effect between the stock price index (CSPI), and the Asset Value net (NAB) Danareksa Syariah Berimbang</p>
Export Citation Format
Share document.
Academia.edu no longer supports Internet Explorer.
To browse Academia.edu and the wider internet faster and more securely, please take a few seconds to upgrade your browser .
Enter the email address you signed up with and we'll email you a reset link.
- We're Hiring!
- Help Center
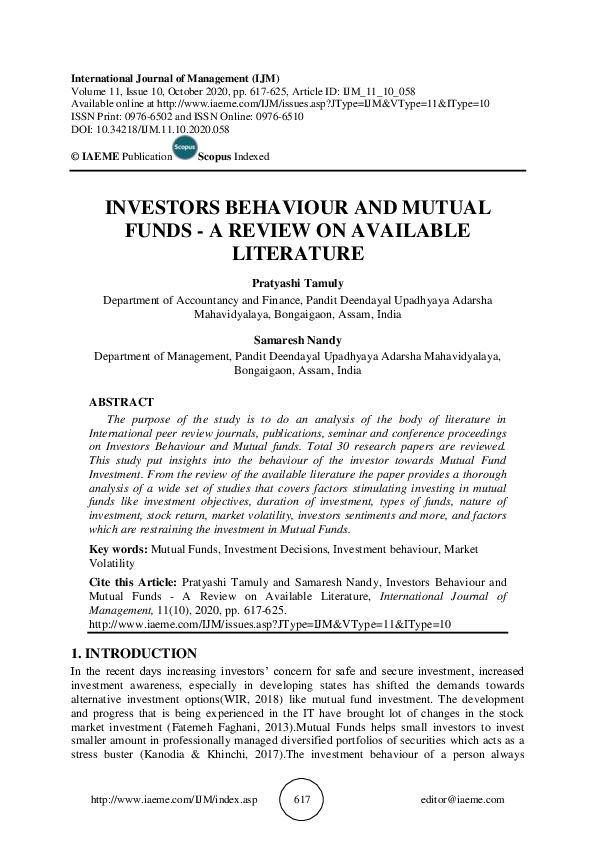
INVESTORS BEHAVIOUR AND MUTUAL FUNDS -A REVIEW ON AVAILABLE LITERATURE

2020, IAEME PUBLICATION
The purpose of the study is to do an analysis of the body of literature in International peer review journals, publications, seminar and conference proceedings on Investors Behaviour and Mutual funds. Total 30 research papers are reviewed. This study put insights into the behaviour of the investor towards Mutual Fund Investment. From the review of the available literature the paper provides a thorough analysis of a wide set of studies that covers factors stimulating investing in mutual funds like investment objectives, duration of investment, types of funds, nature of investment, stock return, market volatility, investors sentiments and more, and factors which are restraining the investment in Mutual Funds.
Related Papers
Nittan Arora
The ever-changing stock markets across the globe have always fuelled jitters among the investors, particularly the small and medium investors. Mutual Funds protect the interest of the small investors not only from the downside market risk through the diversification of risks, but also provide the benefits from the upward market returns. The study mainly focuses on the investor’s inclination towards mutual funds and the impact of demographic factors on their risk perception, investment pattern and risk taking ability. Study also focuses on the factors influencing Investors while investing in mutual funds. 200 respondents contacted from doaba region of Punjab. 113 respondents out of 200 are investing in mutual fund schemes. Returns on schemes have emerged to be the most important factor and entry & exit load least important that affects the choice of the investors in selecting Mutual fund schemes. Most of the demographic factors have no significant association with investment pattern,...
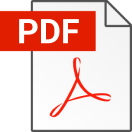
World Journal of Management and Economics
Dr. Venkateswararao Podile
India is one of the emerging global markets and is considered as an investment destination from all over the world. Nowadays many investment alternatives available to the investors like Post office savings, insurance, chits, mutual funds and shares etc. Amongst the available investment alternatives, mutual funds are the most popular investment opportunity to the investors. Mutual Funds provide a platform for a common investor to participate in the capital market with professional fund management irrespective of the amount invested. It works on the concept of "Small drops of water can make big ocean". Small investors can also invest in mutual fund and earn a fair rate of return with less risk compared to shares and other financial products. It also provides the benefits of specialized services, expert knowledge, tax benefits, liquidity, profitability, and transparency etc. However it has been observed that most of the investors are not aware of the benefits of investing in mutual funds. This study will analyze the perception of investors towards investment in mutual funds with appropriate descriptive and inferential statistics.
nadeem maqbool
Ramaswamy nandagopal
Rishi Manrai
Mutual funds act as a medium for retail investors to invest their savings in the professional funds management system, irrespective of the sum invested. It enables masses to enter the Indian Financial Market with much more ease. Indian Mutual Funds industry is growing rapidly which is reflected with the growth in assets under management under various AMC’s year on year. Investment in mutual funds is less risky when compared to investment in equities market. Less risk combined with moderate returns and professional management act as a magnet for the risk averse investors to invest their savings in the financial markets. This project aims at finding out the factors affecting investment decision on mutual funds and the impact of behavioral factors on an investor. This project also aims at finding about the factors that prevent the people to invest in mutual funds. The findings will help mutual fund companies to identify the areas required for improvement and can also improve their mark...
INTERNATIONAL JOURNAL OF ENGINEERING TECHNOLOGIES AND MANAGEMENT RESEARCH I J E T M R JOURNAL
Shubham Rohella
— As the concept of mutual fund is gaining more and more importance with a wide array of institutions vying to lure the investing public, a proper evaluation of performance of mutual funds, ability of the funds to diversify and time their investment is of practical importance for a valued judgment. Mutual Fund is one of the most preferred investment alternatives for the small investors as it offers an opportunity to invest in a diversified, professionally managed portfolio at a relatively low cost. The paradigm shift toward mutual funds assumed greater importance ever since the financial sector gained momentum under the globalized and liberalized environment. Each mutual fund has its own investment objective such as capital appreciation, high current income or money market income. A mutual fund generally states its own investment objectives and investors as a part of their own investment strategies choose the appropriate mutual fund for investment. The performance of the mutual funds products become more complex in context of accommodating both risk and return measurement while giving due importance to investment objectives.
Journal of Asian Business Strategy
Sumit Vishwakarma
Indian mutual fund has gained a lot of popularity from the past few years. Earlier only UTI enjoyed the monopoly in this industry but with the passage of time many new players entered the market, due to which the UTI monopoly breaks down and the industry faces a severe competition. As the time passes this industry has become a buzz word in the Indian financial system. So it is very important to know the investors' perception about this industry. The present study analyses the mutual fund investments in relation to investor's behavior. Investors' opinion and perception has been studied relating to various issues like type of mutual fund scheme, main objective behind investing in mutual fund scheme, role of financial advisors and brokers, investors' opinion relating to factors that attract them to invest in mutual funds, sources of information, deficiencies in the services provided by the mutual fund managers, challenges before the Indian mutual fund industry etc.
IAEME PUBLICATION
IAEME Publication
Purpose: India is experiencing a phase of savings and investment, especially with the growth spurt in its working class. The Indian mutual fund industry is growing rapidly and this is reflected in the increase in Assets under management of various fund houses. This research paper focused on number of factors that highlights investors’ behaviour towards mutual funds and preferences of investors. The impact of various demographic and behavioural factors on investors has been studied. Design/Methodology/Approach: The study is empirical and analytical based on survey approach. It is primary data based study. Data is collected through structured questionnaire. For this purpose, we have used frequency distribution, percentages, chi square test, ANOVA, Average Score analysis and Kruskal Wallis test to prove the hypotheses. Also, Cronbach’s Alpha (CFA) is applied to measure the internal consistency of the variables. Findings: The major findings of the study reveal that demographic factors have impact on the investment decisions taken by investors. Also, it is found the aged and educated people preferred mostly safe and high return on their investment. They avoid risk. The study will be beneficial for researchers & academicians for further research, brokers, dealers, investors and share market of icials. Research Applications/Limitations: this study is helpful to identify the behaviour of investors towards mutual funds investment. Despite at most care, there has been some limitation in this study which may have impact on findings of the study. As we used convenience sampling method and only 100 samples of respondents has been selected, it is dif icult to generalize the findings to the large population. We have selected a limited area i.e. only Delhi and NCR. Also, there may be errors in development of questionnaire and data feeding. Practical Implications: Contributes to the body of knowledge on investor’s behaviours, perception n their attitude towards investment in mutual funds and its implications for investors, agencies and government for formulating policies. Originality/Value: The paper identifies a framework of relevant values and facilities that will be of use to those interested in this fields.
RELATED PAPERS
Elvira Buelna Serrano
HALAVATH BALAJI
Moana Hafoka
2012 Design, Automation & Test in Europe Conference & Exhibition (DATE)
Nova Revista Amazônica
Nivia Costa
MAREK SZCZEPAŃSKI
Ecosains: Jurnal Ilmiah Ekonomi dan Pembangunan
Proceedings of the National Academy of Sciences
Lecture Notes in Mathematics
René Fourneau
Química Nova
mariana vieira
Cuiying Xiao
Becoming Citizens in a Changing World
VALERIA DAMIANI
José Santiago Andrade Zapata , Santiago Andrade
Revista Brasileira de Zootecnia
José Vilar da Silva
Science of The Total Environment
stefano polesello
International Journal of Environmental Research and Public Health
Raffaella Calati
Muh Murtadho
Leonard Lodish
International journal of gynaecology and obstetrics
Shuchita Mundle
Christian Brousseau
Gustavo Santana Jubells
Helle Samdal
Bivash Biswas
Macromolecules
Tien-yau Luh
Alfred Böcking
RELATED TOPICS
- We're Hiring!
- Help Center
- Find new research papers in:
- Health Sciences
- Earth Sciences
- Cognitive Science
- Mathematics
- Computer Science
- Academia ©2024
- Contributors
Low Carbon Mutual Funds

Marco Ceccarelli is an Assistant Professor at Vrije Universiteit, Stefano Ramelli is an Assistant Professor at the University of St. Gallen, and Alexander F. Wagner is a Professor of Finance at the University of Zurich. This post is based on their article forthcoming in the Review of Finance .
How should investors behave in the face of climate–related risks and the energy transition to a low carbon world? To answer this question, it is important to recognize that accounting for climate risks in investment decisions brings investors both benefits and costs.
On the one hand, shunning carbon–intensive, “brown” assets can reduce an investor’s exposure to climate risks. These risks have arguably yet to fully materialize, both in terms of physical consequences and societal reactions. On the other hand, in our not–yet–low carbon economy, excluding “brown” assets and investing only in those considered “green” requires investors to forego opportunities to diversify. This trade–off is particularly salient in asset management, where portfolio diversification, not only the features of individual securities, plays a crucial role in reducing overall investment risk.
In a recent paper , we study how investors and asset managers navigate this trade–off. We focus on the mutual fund industry, which represents an important share of global financial markets, and exploit a quasi–natural experiment involving a sudden increase in both the availability and salience of information on carbon risk (climate transition risk), i.e., the class of risk deriving from the transition to a lower carbon economy. On April 30, 2018, Morningstar, the most important data provider in the mutual fund industry, released a new Portfolio Carbon Risk Score derived from firm-level data provided by Sustainalytics, which Morningstar has controlled since 2017. The novelty of Morningstar’s Portfolio Carbon Risk Score is highlighted by the fact that it correlates only mildly with other portfolio metrics, based on previously available environmental scores from Sustainalytics, Refinitiv, and MSCI KLD. Based on its new carbon risk score, combined with relatively standard information on firms’ fossil fuel involvement, Morningstar also issued an eco–label for mutual funds – the Low Carbon Designation (LCD). We use a large sample of active European and US mutual funds to study investors’ and fund managers’ reactions to these information shocks produced by the publication of Morningstar’s Portfolio Carbon Risk Score and its associated LCD eco–label.
We use the following conceptual framework guiding our empirical analyses. The extant literature has focused on the risk properties of individual green securities. For example, some studies argue that firms with lower carbon emissions are expected to be less risky than other firms due to lower exposure to climate-related uncertainties (e.g., Bolton and Kacperczyk (2021, 2023)). Similarly, other studies argue that shareholders of firms polluting more require a risk premium (Hsu et al., 2023) and so do, at least recently, shareholders of firms with a more negative biodiversity footprint (Garel et al., 2024).
However, how the risk properties of individual green securities translate to the portfolio level is still largely unexplored and, as we show, not obvious. In particular, one may naively think that the risk properties of low carbon funds should mirror those of their low carbon holdings. Such, we find, is not the case. The investment risk of a portfolio depends not only on the variance of its individual holdings’ returns, but also on the covariance of these returns (Markowitz, 1952). Empirically, while low carbon funds have lower exposure to climate risks, their volatility is not lower than that of more conventional funds. In fact, we find that the mutual funds with the lowest carbon risk scores have higher volatility than those with median scores. The source of this result is the high degree of industry concentration (Kacperczyk et al., 2005) of low carbon funds. These funds overweight IT, retail, and healthcare fi while they underweight energy, materials, and utility firm. Beyond the industry concentration, the fact that low carbon funds hold fewer stocks does not significantly further explain their surprisingly high volatility. Overall, low carbon funds hold assets that, although individually less risky, have a high degree of covariance, limiting risk–sharing. (That said, low carbon investing can come in different shapes. For instance, Andersson et al. (2016) and Bolton et al. (2022) outline approaches to reducing carbon risk with small tracking errors and sector–weighted deviations.)
In light of this trade-off, how did mutual fund investors react to the April 2018 information shock? Funds receiving the “Low Carbon Designation” enjoyed a substantial increase in their monthly flows relative to other funds. The economic impact of the LCD label corresponds to an average increase in flows of approximately 36 basis points each month through the end of 2018; this increase is equal to about two–thirds of the effect on flows caused by a one–standard–deviation stronger monthly financial performance. Importantly, at the margin, investors should attempt to strike a balance between the risk benefits of portfolio diversification and those of low carbon investing. Of two otherwise-equal LCD-labeled funds, investors should prefer the one with higher perceived risk-adjusted returns. This is precisely what we find.
Next, we employ a dataset of monthly portfolio holdings to study the reactions of fund managers to the release of Morningstar’s portfolio and firm-level carbon risk information. We show that, after April 2018, fund managers actively rebalanced their portfolios to reduce their carbon risk. On average, relative to the period before the publication of Morningstar’s carbon risk metrics, mutual funds reduced their position in the average high carbon risk fi by about 0.17 basis points of their assets under management per month. This effect is economically meaningful, considering that the median monthly position change is zero for the whole sample and 2.8 basis points for non–zero position changes.
Importantly, funds with higher ex–ante industry concentration reacted more strongly to the release of the new carbon risk information. For these funds, shifting to lower carbon risk assets is less likely to decrease (and may even increase) their diversification. They are also likely to serve clients who are less interested in broad diversification in the first place. Also importantly, we find that when managers reduced their positions in stocks with a score of medium or high carbon risk, they did so more aggressively for those with a higher return covariance with the remainder of the portfolio, consistent with an attempt to preserve diversification.
Overall, our results indicate that both the cost and benefit sides of low carbon investing should (and do) shape investor responses. They confirm climate risks to be a key consideration in the mutual fund industry and provide new insights into how climate-related information can re-orient capital flows in a low carbon direction. By highlighting the existing tension—at least in the short run—between the management of climate risks and traditional mean–variance portfolio considerations, we hope to provide practical implications and stimulate further research into the behavior of investors and fund managers during the transition to a low carbon economy.
Post a Comment
Your email is never published nor shared. Required fields are marked *
You may use these HTML tags and attributes: <a href="" title=""> <abbr title=""> <acronym title=""> <b> <blockquote cite=""> <cite> <code> <del datetime=""> <em> <i> <q cite=""> <s> <strike> <strong>
Supported By:
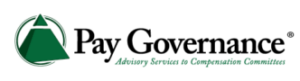
Subscribe or Follow
Program on corporate governance advisory board.
- William Ackman
- Peter Atkins
- Kerry E. Berchem
- Richard Brand
- Daniel Burch
- Arthur B. Crozier
- Renata J. Ferrari
- John Finley
- Carolyn Frantz
- Andrew Freedman
- Byron Georgiou
- Joseph Hall
- Jason M. Halper
- David Millstone
- Theodore Mirvis
- Maria Moats
- Erika Moore
- Morton Pierce
- Philip Richter
- Marc Trevino
- Steven J. Williams
- Daniel Wolf
HLS Faculty & Senior Fellows
- Lucian Bebchuk
- Robert Clark
- John Coates
- Stephen M. Davis
- Allen Ferrell
- Jesse Fried
- Oliver Hart
- Howell Jackson
- Kobi Kastiel
- Reinier Kraakman
- Mark Ramseyer
- Robert Sitkoff
- Holger Spamann
- Leo E. Strine, Jr.
- Guhan Subramanian
- Roberto Tallarita
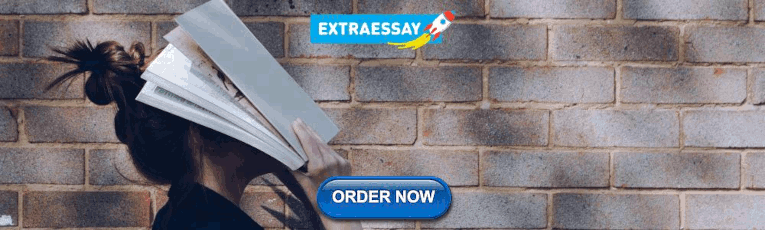
IMAGES
VIDEO
COMMENTS
This review divulges three research areas: a) holdings-based performance measures, b) risk approach of bond funds, and c) bond fund flows. Specifically, a key flow of research has emerged from the USA. However, strong collaborations from European countries are more apparent in the literature.
Delhi School of Economics, University of Delhi, New Delhi,India. ABSTRACT. A Mutual fund is a pure intermediary which performs a basic function of buying and. selling securities on behalf of its ...
Given the tremendous growth of factor allocation strategies in active and passive fund management, we investigate whether factor or sector asset allocation strategies provide investors with a superior performance. Our focus is on comparing factor versus sector allocations as some recent empirical evidence indicates the dominance of sector over country portfolios. We analyze the performance and ...
We review the major models of mutual fund performance: (1) using return data to evaluate equity funds—from single to multi-index models, (2) measuring passive portfolio performance, (3) using holdings-based performance measures, (4) measuring timing ability, and (5) measuring bond fund performance. We conclude with a discussion of issues ...
The size of the trade round the world has created the selection of funds analysis and ranking ways of key importance (Almeida et al., 2020; Fulkerson & Hong, 2021; Kutan, 2018).For example, mutual funds were of key importance to the USA capitalist and three-fourths people families closely held mutual funds (Elton & Gruber, 2020).However, investors are faced with difficulties in choosing a ...
from 2018 to 2019, the global long-term bond market has witnessed a spur in the issuance by. 19.7% to $21 trillion, while the issuance of global equity has decreased by 0.9% to $540.5 billion as ...
J Econ Finan. 2021; 45(4): 654-676. Published online 2021 Mar 4. ... Literature review. Mutual funds are increasing every year. An ordinary investor with nominal capital can pool their money with other like-minded individuals in a managed, diversified basket of stocks and bonds. Few studies focus on debt funds, with equity funds receiving ...
on 31st January 2021, there are 49 mutual fund companies with the AUMas₹ 30.50 Lakh ... why the Chronological Literature Review would give readers an idea of how the different
Abstract. Investors should focus on the performance of portfolios of active funds, not on the performance of individual active funds. Taking this portfolio approach with respect to active U.S. equity mutual funds, I build an optimized portfolio of funds that subsequently has low idiosyncratic volatility and a large, positive, statistically ...
mutual funds, as well as contagion effects in the fund industry. Regarding liquidity risks and the impact of sales from mutual funds, Zeng (2017) shows with a dynamic theoretical model that mutual funds are subject to bank-run -like risks. Following outflows from investors, mutual funds use first their cash buffers to meet redemptions. Then
1Office of the Investor Advocate, 100 F Street NE, Washington, DC 20549, USA 2NORC at the University of Chicago, 55 East Monroe, Chicago, IL 60602, USA. Abstract. We develop a measure of mutual fund investment knowledge that complements existing fi-nancial literacy measures. Our question battery was administered to 3,444 survey respondents.
Review of Financial Studies, forthcoming. 73 Pages Posted: 14 Mar 2019 Last revised: ... We show that mutual fund investors rely on simple signals and likely do not engage in sophisticated learning about managers' alpha as widely believed. Simplistic performance chasing best explains aggregate flows to the mutual fund space and flows across ...
Mutual funds have emerged as the most dynamic segment of the Indian financial system. With its potential to provide higher return by investment in diversified securities, mutual funds emerge as one of the most promising investments in uncertain markets. With a variety of mutual funds competing in the present scenario market, it becomes a challenging decision for the investor to balance risk ...
A. (2022). Performance of mutual funds In India: Comparative analysis of small-cap and mid-cap mutual funds. Academy of Marketing Studies Journal, 26(4), 1-10 . ... REVIEW OF LITERATURE Maheswari (2021) By comparing the corporate and national sectors. ... (2021) This research looks at equity mutual funds in India with varying fund earnings ...
International Journal of Adaptive and Innovative Systems; 2021 Vol.3 No.1; Title: Predicting the net asset value of mutual fund: an extended literature review Authors: Shikha Singla; Gaurav Gupta. Addresses: Department of Computer Science and Engineering, Punjabi University, Patiala (Pb), India ' Department of Computer Science and Engineering, Punjabi University, Patiala (Pb), India
LITERATURE REVIEW Many scholars have made studies on investors' behaviour, and many studies are going on. Also many researches on the performance of mutual fund schemes had been conducted. From the available studies from secondary sources the literature is reviewed here to have better clarity of investors' behaviour and the study ...
Abstract. GITAM Journal of Management; Vol. 19, No.2, Apr-Jun 2021; Pages: 78-98 Global Mutual Fund Management Industry was valued at $54.93 trillion in 2019 and is estimated to grow by 11.3% CAGR ...
Net Asset Value. The Research aims to determine the influence of inflation, currency exchange rate, interest rate that affect net asset value of protected Islamic mutual fund from 2014-2018 in Indonesia partially and simultaneously. The research also uses quantitative approach and multiple regression analysis technique.
Article History: Received: 11 January 2021; Accepted: 27 February 2021; Published online: 5 April 2021 Abstract: The Indian mutual fund's industry, which started its excursion with the foundation of the Unit Trust of India in 1964, ... Literature Review Abey (2017) - The paper contemplated the different elements impacting investment choice in ...
abstract. Mutual funds is playing a vital role in the development of Indian economy as it is acting as the gap between the supply and demand for funds in the market the AUM reached Rs.37.33 trillion as on October 31,2021. In the present study we have analyzed literature review about Mutual funds in India. This paper is an attempt to understand ...
Mutual funds is playing a vital role in the development of Indian economy as it is acting as the gap between the supply and demand for funds in the market the AUM reached Rs.37.33 trillion as on October 31,2021. In the present study we have analyzed literature review about Mutual funds in India. This paper is an attempt to understand the ...
Performance Evaluation of Mutual Funds in India: Literature Review. Account and Financial Management Journal , 898-908. [9] Kumar, V., & Bansal, P. (2014). A Study on Investors' Behaviour towards Mutual Funds in Rohrak Haryana. International Journal of Engineering and management Research, 224-228. ... 2021 • Markus Disse. Download Free PDF ...
Literature Review Year 1963 marks the beginning of Mutual fund . ... growth, types, advantages, and challenges. The study contributes to the existing body of literature on mutual funds in India and provides insights for future research and policy-making in the ... 1735 1495 0 500 1000 1500 2000 2500 3000 2015-16 2016-17 2017-18 2018-19 2019-20 ...
Funds receiving the "Low Carbon Designation" enjoyed a substantial increase in their monthly flows relative to other funds. The economic impact of the LCD label corresponds to an average increase in flows of approximately 36 basis points each month through the end of 2018; this increase is equal to about two-thirds of the effect on flows ...