College of Computing
Ph.d. in machine learning, about the curriculum.
The central goal of the Ph.D. program is to train students to perform original, independent research. The most important part of the curriculum is the successful defense of a Ph.D. dissertation, which demonstrates this research ability.
The curriculum is designed with the following principal educational goals:
• Students will develop a solid understanding of fundamental principles across a range of core areas in the machine learning discipline. • Students will develop a deep understanding and set of skills and expertise in a specific theoretical aspect or application area of the machine learning discipline. • The students will be able to apply and integrate the knowledge and skills they have developed and demonstrate their expertise and proficiency in an application area of practical importance. • Students will be able to engage in multidisciplinary activities by being able to communicate complex ideas in their area of expertise to individuals in other fields, be able to understand complex ideas and concepts from other disciplines, and be able to incorporate these concepts into their own work. The curriculum for the Ph.D. in Machine Learning is truly multidisciplinary, containing courses taught in eight schools across three colleges at Georgia Tech: • Computer Science (Computing) • Computational Science and Engineering (Computing) • Interactive Computing (Computing) – see Computer Science • Aerospace Engineering (Engineering) • Biomedical Engineering (Engineering) • Electrical and Computer Engineering (Engineering) • Industrial Systems Engineering (Engineering) • Mathematics (Sciences) Students must complete four core courses, five electives, a qualifying exam, and a doctoral dissertation defense. All doctorate students are advised by ML Ph.D. Program Faculty . All coursework must be completed before the Ph.D. proposal. An overall GPA of 3.0 is required for the Ph.D. coursework.
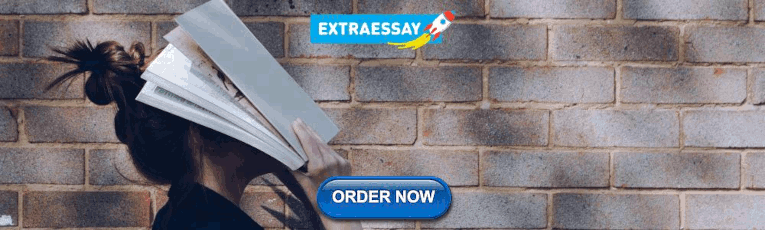
Research Opportunities
Our faculty comes from all six colleges across Georgia Tech’s campus, creating many interdisciplinary research opportunities for our students. Our labs focus on research areas such as artificial intelligence, data science, computer vision, natural language processing, optimization, machine learning theory, forecasting, robotics, computational biology, fintech, and more.
External applications are only accepted for the Fall semester each year. The application deadline varies by home school.
The Machine Learning Ph.D. admissions process works bottom-up through the home schools. Admissions decisions are made by the home school, and then submitted to the Machine Learning Faculty Advisory Committee (FAC) for final approval. Support for incoming students (including guarantees of teaching assistantships and/or fellowships) is determined by the home schools.
After the admissions have been approved by the FAC, the home school will communicate the acceptance to the prospective student. The home school will also communicate all rejections.
Get to Know Current ML@GT Students
Learn more about our current students, their interests inside and outside of the lab, favorite study spots, and more.
Career Outlook
The machine learning doctorate degree prepares students for a variety of positions in industry, government, and academia. These positions include research, development, product managers, and entrepreneurs.
Graduates are well prepared for position in industry in areas such as internet companies, robotic and manufacturing companies and financial engineering, to mention a few. Positions in government and with government contractors in software and systems are also possible career paths for program graduates. Graduates are also well-suited for positions in academia involving research and education in departments concerned with the development and application of data-driven models in engineering, the sciences, and computing.
Frequently Asked Questions
For additional questions regarding the ML Ph.D. program, please take a look at our frequently asked questions.
You can also view the ML Handbook which has detailed information on the program and requirements.
From the Catalog:
Skip to content
Georgia Institute of Technology
Search form.
- You are here:
- PhD Program
Program FAQ
Frequently asked questions.
The ML Ph.D. Handbook provides a detailed overview of the program and how it operates. Please see below for answers to our most commonly asked questions. If your question is not answered below or in the handbook, please contact [email protected]
General Program and Application Questions
When can I apply to the ML Ph.D. program?
External applications are only accepted for the Fall semester each year. The application deadline varies by home school with the earliest deadline of December 1. Most home schools have a final deadline of December 15. Check with home schools for more specific details.
Where can I apply for the ML Ph.D. program?
All application forms for graduate work at Georgia Tech are accepted through the admissions process which can be found here.
Is it possible to enroll in the program part-time?
No, doctoral students are required to spend at least two full-time semesters in residence at Georgia Tech and should complete research for their dissertation while in residence and be registered for a full course load of dissertation hours each semester. Additionally, Ph.D. students are generally funded with graduate teaching or research assistantships and include tuition waivers and stipends, which require full-time enrollment. Note international students on an F1 visa are required to be full-time status to maintain lawful status in the US.
Do you have to be on campus to enroll in the program? Can Distance Learning students enroll in the program?
The Ph.D. program is only offered on-campus. You must apply to the Ph.D. program through one of our home units
Can any of the courses be done online?
Currently, none of the courses for the ML Ph.D. are offered online.
Do you have to have a master's degree to enroll in the program?
No master's degree is required. Most of our applicants are applying straight from their undergrad programs.
Can I get any prerequisite classes waived as my previous degrees were in?
There are no pre-requisite courses to apply to the program. Credit for individual classes towards the Ph.D. program is handled on a case-by-case basis by the ML academic advisor after a student has matriculated in the program.
What does it mean that the Machine Learning Ph.D. Program is a multidisciplinary program?
The Machine Learning Ph.D. Program is a collaboration of nine participating schools at Georgia Tech. Incoming Ph.D. students are admitted to the ML Ph.D. program through one of these home schools.
How is my application processed for the Machine Learning Ph.D. Program?
Your application is first processed in the home school. Application deadlines, minimum GRE/TOEFL scores, and other requirements are all determined by the home school. Applications that satisfy all of the requirements are then forwarded to the Machine Learning Faculty Advisory Committee (ML FAC) for review. Decisions for admissions are made jointly between the home unit and the ML faculty.
Does the ML Ph.D. program offer support in the form of teaching assistantships, research assistantship, or fellowships?
Not directly. Teaching assistantships and fellowships are determined through the home schools. Research assistantships are typically funded through your thesis advisor but are subject to the rules imposed by the student's home school.
In addition, a student's home school may have extracurricular requirements (including a minimum number of semesters grading or serving as a TA). ML Ph.D. students are also subject to the extra-curricular requirements of their home schools.
Home Unit Questions
What home schools participate in the ML Ph.D. program?
Currently, there are 9 participating schools across 3 colleges:
College of Engineering
- Aerospace Engineering
- Biomedical Engineering
- Chemical and Biomolecular Engineering
- Electrical and Computer Engineering
- Industrial and Systems Engineering
College of Computing
- Computational Science and Engineering
- Computer Science
- Interactive Computing
College of Sciences
- Mathematics
Does it matter which home school I choose?
Yes. Home school may have different admissions requirements and deadlines. Additionally, for enrolled students, some home units may have GTA requirements, annual reviews, additional courses or seminars, and there may be differences in financial support. Please check with home schools for further details. The ML curriculum, qualifying exam, and thesis proposal, and defense requirements are the same for all ML students and can be found in the ML Handbook . Students are responsible for understanding and following both the ML program and their home schools’ policies.
How is a home unit selected on the application?
You will be asked to indicate a Program of Study on your application. Among the options are:
Ph.D. in Machine Learning (Aerospace Engineering), Ph.D. in Machine Learning (Biomedical Engineering), Ph.D. in Machine Learning (Chemical and Biomolecular Engineering), Ph.D. in Machine Learning (Electrical and Computer Engineering) Ph.D. in Machine Learning (Industrial and Systems Engineering) Ph.D. in Machine Learning (Mathematics), Ph.D. in Machine Learning (School of Interactive Computing) Ph.D. in Machine Learning (School of Computational Science and Engineering) Ph.D. in Machine Learning (School of Computer Science)
Can an advisor from outside my home school serve as my thesis advisor?
Yes. Any faculty member on this list can serve as your thesis advisor.
Are the curricular requirements different for the ML Ph.D. than for the Ph.D. program in the home school?
Yes. ML Ph.D. students have different course requirements and a different qualifying exam than the home school.
Detailed information about the ML Ph.D. curriculum can be found here.
Transfer Student Questions
I am currently a graduate student at Georgia Tech enrolled in a different degree program. Can I transfer into the ML PhD program?
Yes. Transfer applications are reviewed on a rolling basis. Potential transfer students will need to have found a thesis advisor who is willing to support them on a research assistantship. For more information, please email [email protected]
Georgia Tech Resources
- Offices & Departments
- News Center
- Campus Calendar
- Special Events
- Institute Communications
Visitor Resources
- Campus Visits
- Directions to Campus
- Visitor Parking Information
- GTvisitor Wireless Network Information
- Georgia Tech Global Learning Center
- Georgia Tech Hotel & Conference Center
- Barnes & Noble at Georgia Tech
- Ferst Center for the Arts
- Robert C. Williams Paper Museum
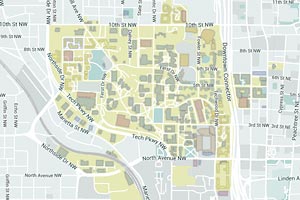
Georgia Institute of Technology North Avenue, Atlanta, GA 30332 Phone: 404-894-2000
School of Interactive Computing
College of computing, artificial intelligence & machine learning.
At Georgia Tech, artificial intelligence (AI) and machine learning (ML) focuses on core research problems in intelligence involving fundamental advances in artificial intelligence, machine learning, and deep learning, as well as challenges in computer vision, natural language processing, and other application areas. We also study the implications of AI and ML in explainable AI, computational creativity, and fairness in the context of ML models. Finally, our faculty work where AI and ML intersect with other areas and fields such as robotics, human computer-interaction, cognitive science, and computer graphics. We advise Ph.D. and MS students in AI/ML through graduate programs in CS and ML, and we offer a broad set of undergraduate and graduate courses.
At the undergraduate level, AI and ML are mainly found in three threads : Intelligence, People, and Devices. Popular courses include Introduction to Artificial Intelligence, Machine Learning, Computer Vision, Natural Language Understanding, Deep Learning, Knowledge-based AI, Game AI, and Cognitive Science. Graduate offerings of these courses are also available, as are specialized seminars on a range of topics in vision, language, deep learning, cognition and their intersections (e.g., Machine Learning with Limited Supervision). In addition, several courses in robotics and HCI also discuss new methods in AI or ML.
AI and ML touch multiple areas and schools within the College of Computing, representing a large swath of faculty and research interests across the different schools. In addition to the Ph.D. program in Computer Science, Faculty in the School of Interactive Computing also participate in focused Ph.D. programs in Robotics and Machine Learning. Our faculty also lead the Institute for Robotics and Intelligent Machines (IRIM) as well as two NSF-funded National AI centers, AI-ALOE and AI-CARING .
Recent Stories
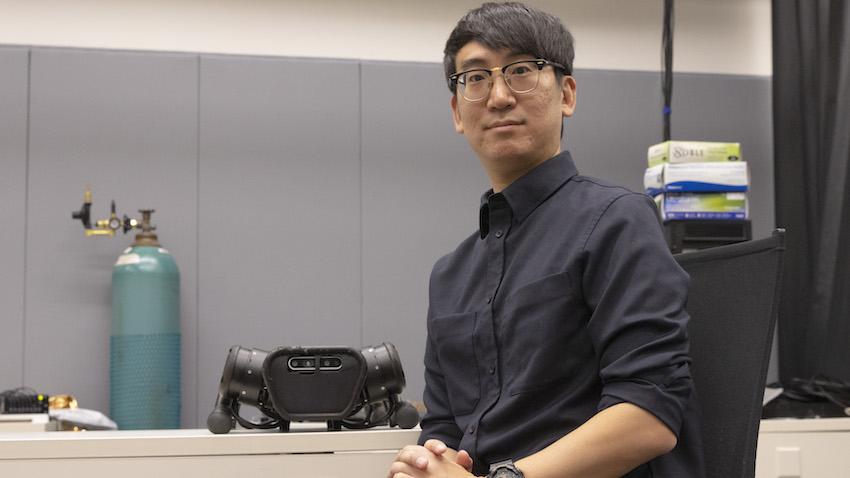
Two-World Training Approach Earns Roboticist NSF…
Thursday, April 4, 2024
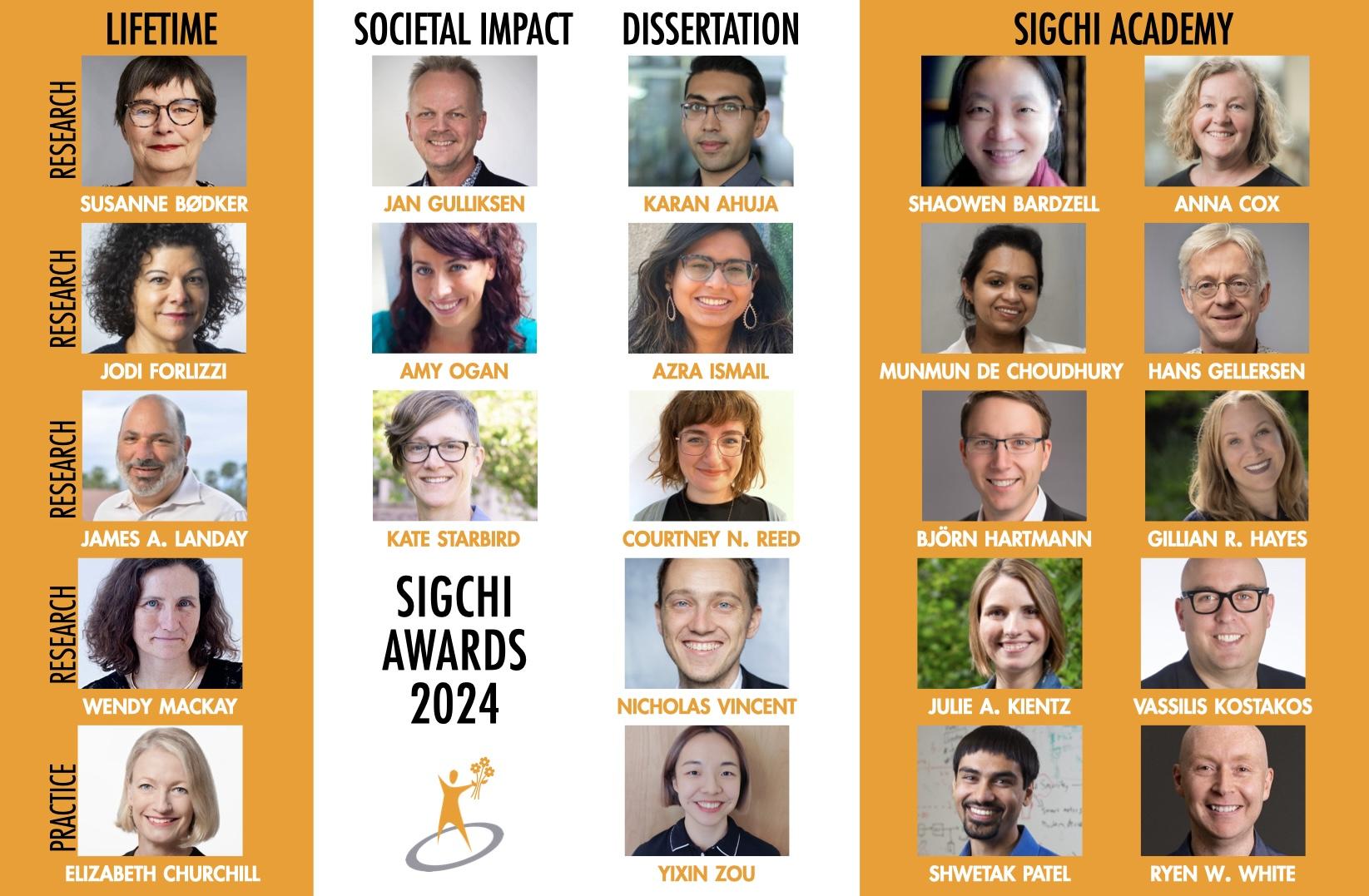
Two IC Faculty Inducted into SIGCHI Academy
Thursday, March 14, 2024
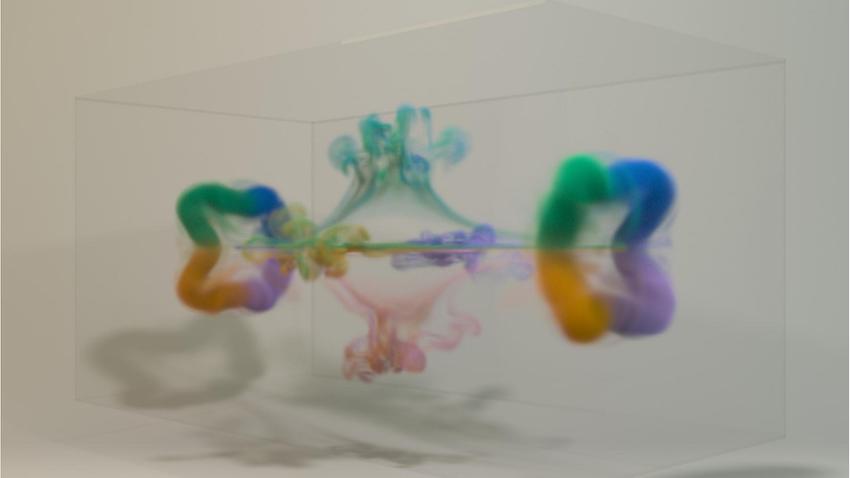
Researchers Reach New AI Benchmark for Computer Graphics…
Wednesday, February 28, 2024
Affiliated Labs & Institutes
Machine Learning at Georgia Tech
Institute of Robotics & Intelligent Machines
Computational Behavior Analysis Lab
Computer Vision Lab
CORE Robotics Lab
Design & Intelligence Lab
Entertainment & Intelligence Lab
Hoffman Lab
Machine Learning and Perception Lab
Natural Language Processing Lab
Dhruv Batra
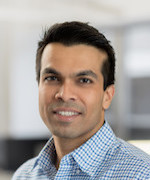
Associate Professor
AI, ML, CV, Robotics
Sonia Chernova
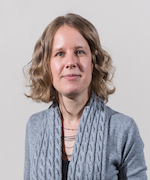
AI, Robotics
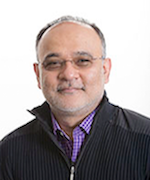
Distinguished Professor
Animesh garg.
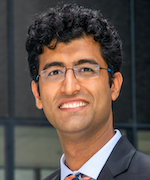
Assistant Professor
ML, CV, Robotics
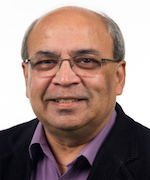
Kartik Goyal
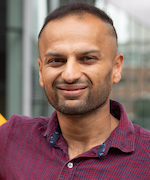
AI, ML, Robotics
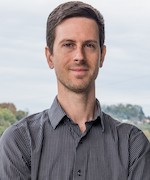
AI, ML, NLP
Judy Hoffman
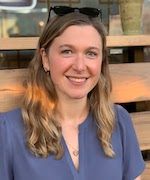
Matthew Gombolay
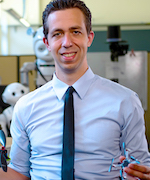
Christopher MacLellan
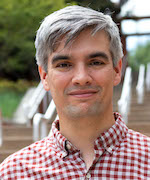
Keith McGreggor
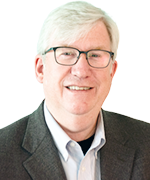
Professor of the Practice
Devi parikh.
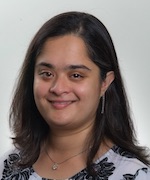
Thomas Ploetz
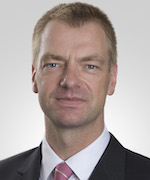
Harish Ravichandar
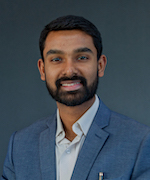
ML, Robotics
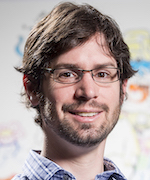
Alan Ritter
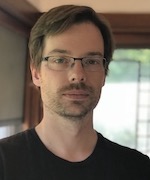
Humphrey Shi
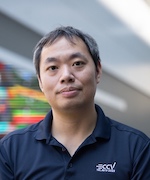
Thad Starner
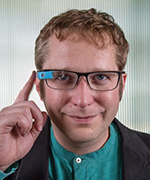
AI, ML, CV, NLP
Sashank Varma
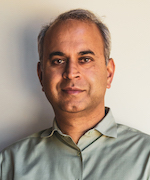
School of Computational Science and Engineering
College of computing, ph.d. programs.
For those students looking to build a career in computing research, the School of Computational Science and Engineering offers a range of Ph.D. programs that allow students to work side-by-side with some of the most brilliant researchers and computer scientists in the world. For those looking to join the ranks of academia, we regularly place our doctorate graduates in tenure-track positions in top programs. The School maintains strong, research relationships with companies from Fortune 500 to the latest startups that allow graduates to continue their research in jobs at some of the world’s hottest private sector employers.
Ph.D. in Computational Science and Engineering Interdisciplinary program devoted to the creation, study, and application of computer-based models of natural and engineered systems.
Ph.D. in Computer Science As a research-oriented degree, the Ph.D. in Computer Science prepares exceptional students for careers at the cutting edge of academia, industry and government.
Ph.D. in Machine Learning Machine learning builds and learns from both algorithm and theory to understand the world around us and create the tools we need and want.
Ph.D. in Bioinformatics An elite multidisciplinary program sponsored by the College of Computing, the H. Milton Stewart School of Industrial and Systems Engineering, and the School of Mathematics.
Ph.D. in Bioengineering Be a leader through interdisciplinary graduate education and research in areas that improve health and the environment.
Skip to content
Georgia Institute of Technology College of Sciences
Search form.
- You are here:
- Graduate Programs
Doctoral Programs
The School of Math offers or participates in six different multidisciplinary PhD programs, each with their own curriculum and set of requirements as described below. In the first year or two of the program students focus on the coursework required to pass the comprehensive exams. By the third year of study, students should have selected a major field and a research advisor. All students must satisfy a "minor" requirement of nine additional credit hours of graduate or advanced undergraduate coursework outside the School or away from their area of specialization, with a GPA of at least 3.0. The completion of the program, which culminates in writing a dissertation, takes about 5 years. All Doctoral students should also be aware of the general Institute wide Requirements for the Doctoral Degree described in the GT Catalog .
PhD in Mathematics
The PhD program in Math is de signed to train academic mathematicians, in a wide range of subdisciplines , and mathematical research scientists working in government or the private sector. By far, most of the PhD students in the School are enrolled in this program. PhD students in Math may work with any faculty member in the School.
PhD in Algorithms, Combinatorics, and Optimization (ACO)
The PhD in ACO is a multidisciplinary program sponsored jointly by the College of Computing, the H. Milton Stewart School of Industrial and Systems Engineering, and the School of Math. A ny affiliated faculty member can supervise the research of any ACO student regardless of departmental affiliation. The director of the ACO program is P rasad Tetali .
PhD in Computational Sciences and Engineering (CSE)
The PhD in CSE is a highly interdisciplinary program designed to provide students with practical skills and theoretical understandings needed to become leaders in the field of computational science and engineering. This is a joint program between the Colleges of Sciences, Computing, and Engineering. CSE applicants who choose Math as their home school are expected to have a strong background in Math. The School's lead advisor and coordinator of the CSE program is Sung Ha Kang .
PhD in Bioinformatics (BINF)
The mission of the Bioinformatics PhD Program is to educate and prepare graduate students to reach the forefront of leadership in the field of bioinformatics and computational biology; and to integrate research and education on the use of information technologies in biology and medicine. The School's lead advisor and coordinator of the Bioinformatics program is Leonid Bunimovich .
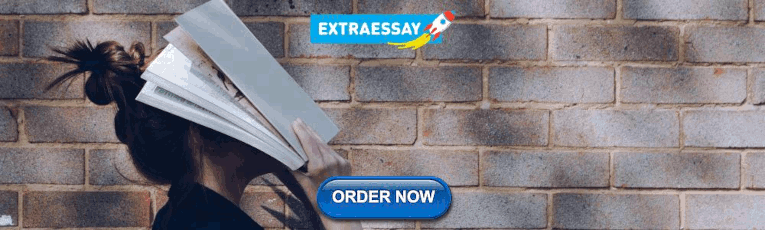
PhD in Quantitative Biosciences (QBios)
The mission of QBios is to educate students and advance research in quantitative biosciences, enabling the discovery of scientific principles underlying the dynamics, structure, and function of living systems. The School's lead advisor and coordinator of the QBioS program is Hannah Choi .
PhD in Machine Learning (ML)
Machine learning aims to produce machines that can learn from their experiences and make predictions based on those experiences and other data they have analyzed. This field crosses a wide variety of disciplines that use data to find patterns in the ways both living systems, such as the human body and artificial systems, such as robots, are constructed and perform. The School's lead advisor and coordinator of the Machine Learning program is Vladimir Koltchinskii
Prospective Students
Students with a bachelor's degree in Mathematics, or related fields, and good preparation for graduate study may apply for admission directly into the doctoral program. Completion of the master's degree is not a prerequisite. Other requirements include the GRE tests, and the TOEFL exam for international students. Virtually all students admitted to our PhD programs are offered full financial support including tuition waivers and stipends in the forms of teaching or research assistantships.
The list of all PhD alumni of the School of Math and their first employment after graduation, going back to 1965.
College of Computing
Ph.d. cs machine learning body of knowledge.
Prepared by : The Machine Learning Faculty, November, 2017
The exam will be divided to four areas: core, statistical methods and models, learning theory, and decision processes. There will be three questions in each area. Each student has to answer two out of the three questions in the core area. In addition, each student has to select two out of the remaining three areas where he or she will answer two out of the three questions.
Reading List:
KP Murphy, Machine learning: a probabilistic perspective, 2012, MIT press
Chapters 2-8, 10-11, 16, 17
Mohri, Mehryar, Afshin Rostamizadeh, and Ameet Talwalkar. Foundations of machine learning. MIT press, 2012.
Chapters 1-6, 8, 10
- Goodfellow, Ian, Yoshua Bengio, and Aaron Courville. Deep learning. MIT press, 2016.
- Chapters 2, 6-9
Statistical Models and Methods
- Daphne Koller and Nir Friedman. Probabilistic Graphical Models: Principles and Techniques, MIT Press, 2009.
Chapters 1-4, 8-11
Friedman, Jerome, Trevor Hastie, and Robert Tibshirani. The elements of statistical learning. Second edition, Springer, 2009.
Chapters 7, 8, 14
Learning Theory
- M. Kearns and U. Vazirani. An Introduction to Computational Learning Theory. The MIT Press 1994.
Chapters 1, 2, 3, 4, 5, 7
Decision Processes
- R. Sutton and A. Barto. Reinforcement Learning: An Introduction. 2nd edition The MIT Press 2017. Chapters 1-9, 12-13 ( http://incompleteideas.net/sutton/book/bookdraft2017nov5.pdf )
Dean's Messages
Gt computing helping stories.

Search form
Machine learning.
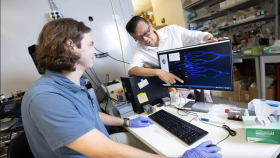
Eva Dyer at the forefront of surge in computational neuroscience research at Georgia Tech
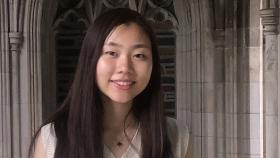
Velda Wang is a co-lead author on the paper in Brain Sciences
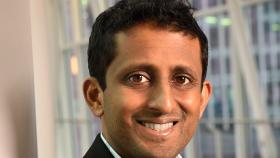
Kemp lab uses genome-scale modeling to understand tumor metabolism and predict tumors’ responses to radiation therapy
Lab Members
Phd students.
Vinam Arora
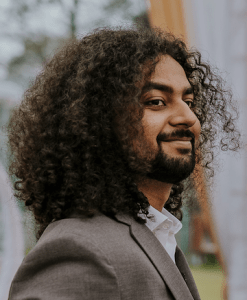
Mehdi Azabou
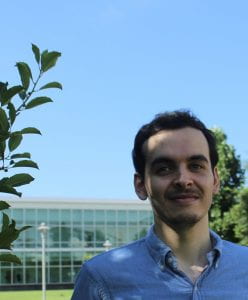
Email: [email protected] Program: PhD in Machine Learning Bio: Mehdi is a fourth-year PhD student in Machine Learning at Georgia Tech. He received his MS in Engineering from CentraleSupelec, France in 2019 and a MS in Computer Science from Georgia Tech in 2020. His interests lie in deep learning, computational neuroscience and representation learning on graphs and manifolds. He is excited about finding applications of machine learning that can improve our understanding of the brain and is an enthusiast for dynamic and interactive visualizations that can provide insights into complex data and enable new ways of thinking Webpage: http://www.mehai.dev/
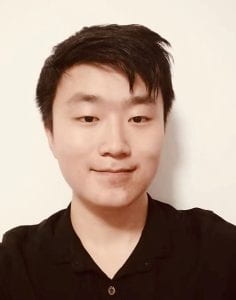
Email: [email protected] Program: PhD in Machine Learning Bio: Zihao Chen is a first year PhD student in Machine Learning at Georgia Tech. He received his bachelor’s degree in Biology from Wuhan University and Master of Engineering in Biomedical Engineering from Fudan University in 2023. Zihao is interested in the confluence of machine learning, stochastic dynamics, and optimization theory. His research is currently focused on representation learning and optimization theory. Besides, he applies machine learning and reinforcement learning techniques to solve real-world problems, such as mining factors in the stock market. He leads an active lifestyle, full of jogging, swimming, and fitness.
Divyansha Lachi
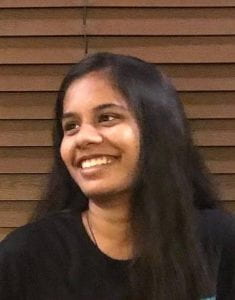
Email: [email protected] Program: PhD in Machine Learning Bio: Divyansha Lachi will be joining the lab as a Ph.D. student in Machine Learning in Fall 2023. She received her B.Tech in Computer Science and Engineering from the National Institute of Technology, Silchar, India. She is broadly interested in understanding biological intelligence to build better artificial intelligence. She wants to understand how the brain can robustly learn from just a handful of examples and develop machine-learning models capable of the same. To this end, some of her current interests include incorporating modularity in artificial neural networks (ANN) for functional specialization and improving Graph Neural Networks (GNN) by incorporating topological features of the brain (like long-range transmission). In her free time, Divyansha enjoys playing tennis and binging TV shows. Webpage: https://divyansha1115.github.io/
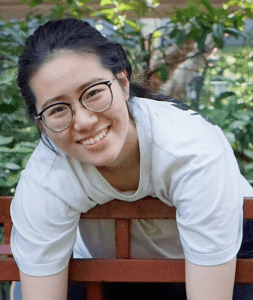
Email: [email protected] Program: PhD in Machine Learning Bio: Ran is a Machine Learning PhD student at Georgia Tech. Originally from mainland China, Ran received her bachelor’s degree in Physics from Fudan University with a lot of extra courses in data science, mathematics, and social science. Ran is broadly interested in machine learning and deep learning, and is particularly passionate about problems that utilize advanced machine learning algorithms on challenging practical problems in the field of health. Outside of research, Ran enjoys observing online communities and playing with her super adorable cat whose Instagram is @tiggerisasmalltiger. Webpage: https://ranliu98.github.io/
Jingyun Xiao
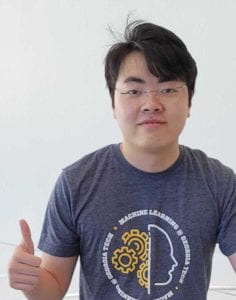
Masters Students
Venkataramana (Venky) Ganesh
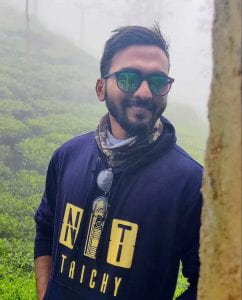
Amrit Khera
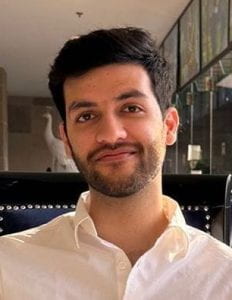
Keerthan Ramnath
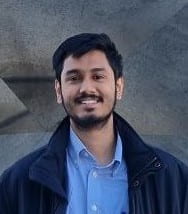
Undergraduate Students
Michael Mendelson
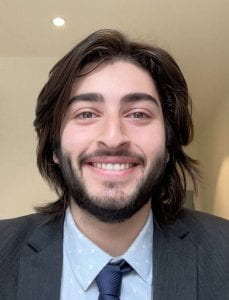
Email: [email protected] Program: BS in Biomedical Engineering
Bio: Michael is a third-year Biomedical Engineering student at Georgia Tech. His academic interests are broad, but he is particularly interested in the intersection between computation and biological processes, especially with regards to neuroscience. Michael is also a budding jazz musician and music producer. He is excited to be able to incorporate many of his interests into the lab, and looks forward to gaining a deeper understanding of the field.
Santosh Nachimuthu
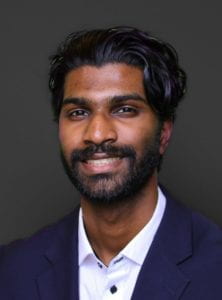
Bio: Santosh is a 3rd year Biomedical Engineering student at Georgia Tech who is also minoring in Computer Science. He is very interested in better understanding and modeling the brain using computer science. Outside of academics, he enjoys sports, good food, and new music. He wants to contribute to the neurotech field and learn as much as possible from the lab to better understand the brain.
Research Affiliates
Aidan Schneider
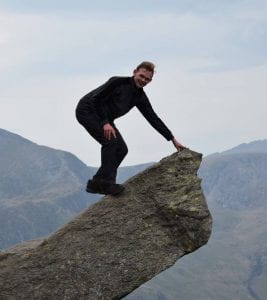
Bio: Aidan is a PhD student in Computational & Systems Biology at Washington University in St. Louis in the Hengen Lab and works in close collaboration with the NerDS Lab. He received a B.S. in Molecular BioSciences & Biotechnology and also a B.S. in Finance from Arizona State University in 2018. Aidan applies deep learning to characterize the influence of behavior, genetics, and anatomy on neuronal computations throughout the brain. He aims to develop models which generalize and transfer successfully across diverse contexts. He also hopes deep learning can facilitate insight from data with minimal pre-processing. Outside the lab, Aidan enjoys hiking and developing tabletop role-playing games.
Mohammad Gheshlaghi Azar
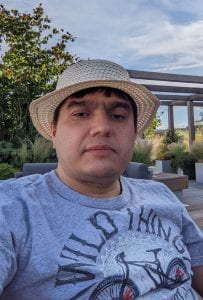
Bio: Mohammad Azar is a staff research scientist at DeepMind labs in London and an Affiliate Researcher in the Coulter Department of Biomedical Engineering at the Georgia Institute of Technology. Dr. Azar’s research encompasses a variety of topics in the field of AI and machine learning ranging from self-supervised learning to exploration, Deep RL, graph representation learning and RL theory. Mohammad obtained his master degree from university of Tehran, Iran in Electrical Engineering. Then he obtained his Ph.D. from University of Nijmegen, Netherlands in Biophysics. Dr. Azar then joined the CS department at CMU as a postdoctoral researcher under the supervision of Professor Emma Brunskill. Afterwards he joined the Kording lab at Northwestern university as a postdoctoral researcher in Computational Neuroscience.
- Pablo Vempala, High School Student, Current
- Katha Patel, High School Student, Current
- Geethika Atmakuri, High School Student, Current
- Farahnaz Soleimani , 2017-2019 -> Assistant Professor at Oregon State University
Ph.D. students:
- Chi-Heng Lin , 2018-2022; TRIAD Research Fellowship (2020); PhD Thesis: “ Understanding Data Manipulation and How to Leverage it to Improve Generalization” -> Now at Samsung Research
Graduate students:
- Aishwarya Balwani , 2018-2021 (PhD) -> Now in Hannah Choi’s lab @ Georgia Tech
- Kyle Milligan, 2018-2020 (PhD) -> Now at Northrup Grumman
- TJ LaGrow, 2017-2019 (PhD); NSF GRFP Honorable Mention -> Now in Shella Keilholz’s lab at Emory
Selected undergraduates and interns:
- Matthew Jin, 2022-2023 (Undergrad) -> Masters @ Stanford
- Carolina Urzay, 2021-2022 (Undergrad) -> Masters @ TU Delft
- Joy M. Jackson , 2021-2022 (Undergrad); REU SURE (2021) – Best Research in the Biomedical Category -> PhD Student at Georgia Tech w/ Alex Abramson
- Zijing Wu , 2020-2021 (Undergrad) -> Anqi Wu’s lab at Georgia Tech
- Max Dabagia , 2018-2020 (Undergrad); NSF GRFP (2020), Presidential Undergraduate Research Award (PURA) (2019) -> Ph.D. at Georgia Tech w/ Santosh Vempala
- Amaal Abdi, 2020-2021 (High School Intern) -> Undergrad at Wharton, University of Pennsylvania
- Joseph Miano, 2018-2020 (Undergrad); PURA (2019) -> MS in CS, AI & ML Associate at JPMorgan Chase
- Alexis Webber, 2019-2019 (Undergrad); Charles Hancock Award for Neuroengineering Research -> Alpha Omega
- Skip to content
GT Machine Learning Graduates
May 04 2022
Machine Learning PhD Graduates | Spring 2022

Spring semester is officially a wrap, and on May 6 and 7, members of the Graduating Class of Spring 2022 will take part in commencement ceremonies . Congratulations to all the graduates who are being conferred degrees and a special congratulations to the Machine Learning PhD graduates. You will break new ground in the field as you start your careers. You’ve been prepared well and we wish you the best of luck. Please stay connected with the Machine Learning Center and visit us when you’re back in the area.
Meet our three new alumni below and see where they are headed next.
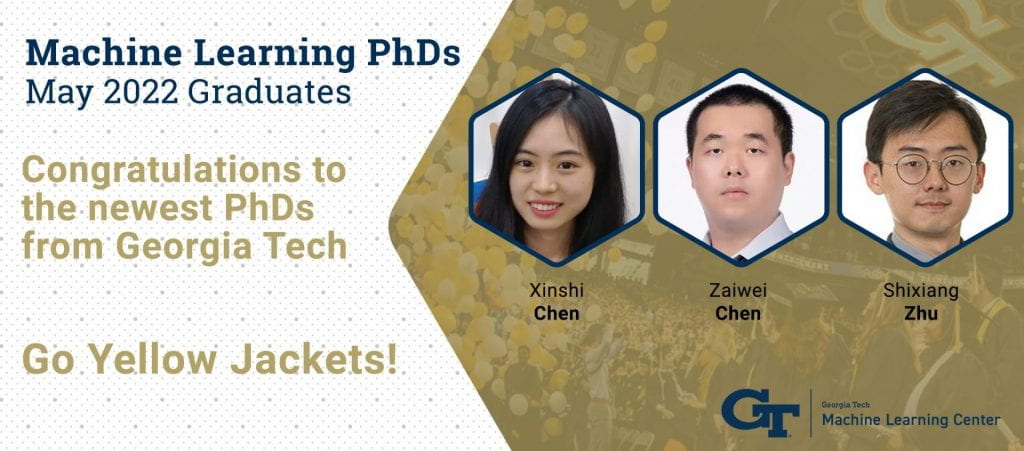
Xinshi Chen
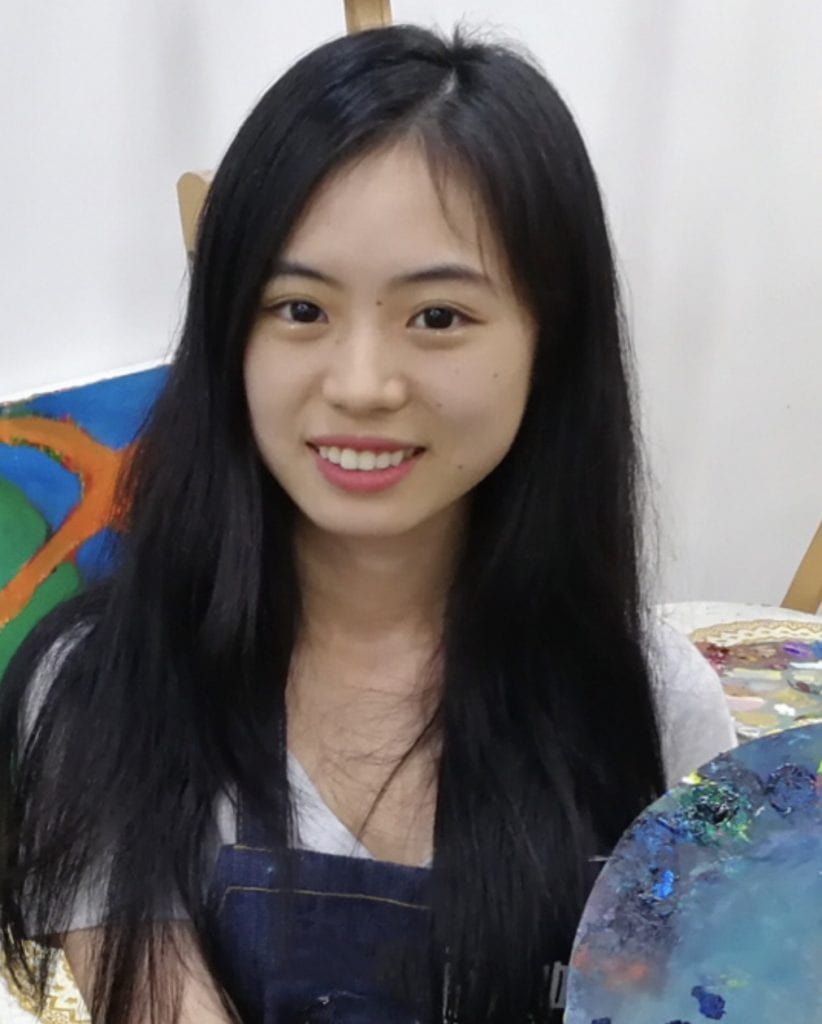
I am a PhD Graduation Candidate in Machine Learning at Georgia Tech , advised by Prof. Le Song . I am currently pursuing a job in the tech sector in China.
I am broadly interested in principled machine learning. My current research focuses on learning based algorithm design (more theory-oriented), deep learning on structured data (more application-oriented), and their intersections. Besides, I am interested in applications in the area of structural and computational biology. My research is generously supported by Google PhD Fellowship in Machine Learning .
I received my B.S. and M.Phil in Mathematics at the Chinese University of Hong Kong under the supervision of Prof. Eric Chung . I have also spent time at Oak Ridge National Laboratory , Ant Financial , Facebook AI , and MBZUAI as a Research Intern or Research Assistant.
Zaiwei Chen
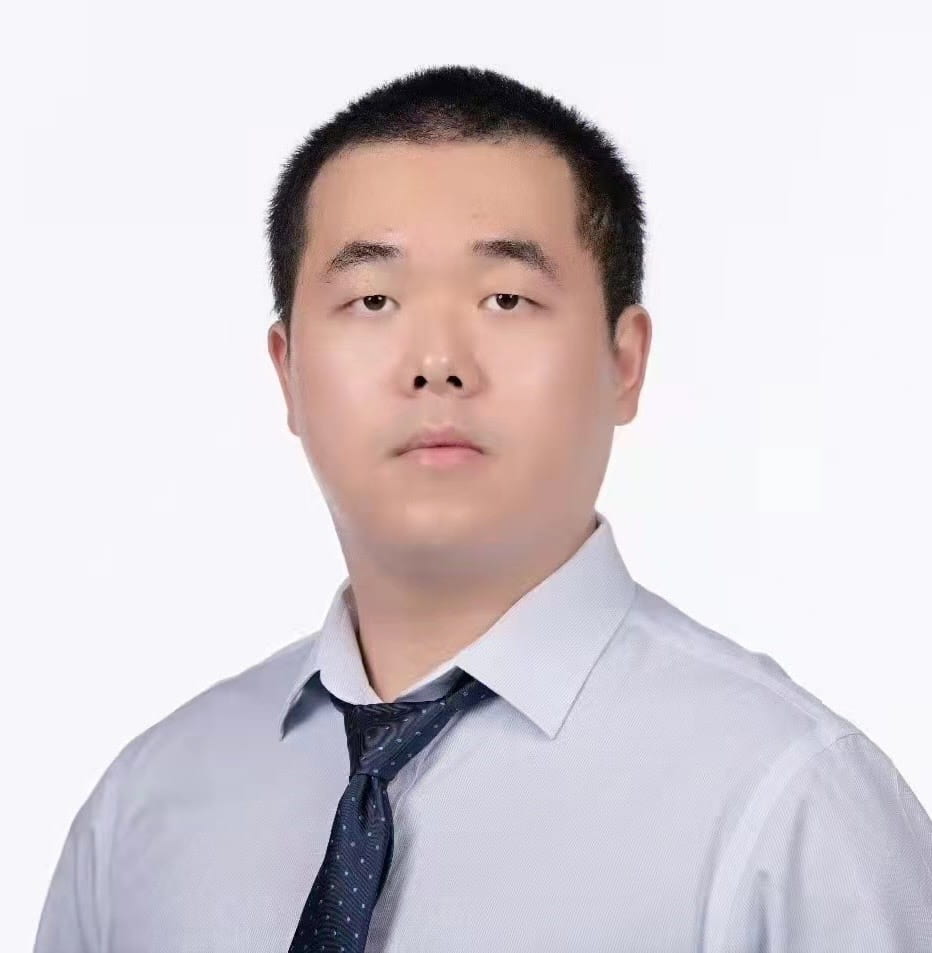
I will be joining the CMS Department of California Institute of Technology in summer 2022 under the CMI postdoctoral fellowship, working with Prof. Adam Wierman and Prof. Eric Mazumdar. I obtained a Ph.D. degree in Machine Learning – ISyE, an M.S. degree in Mathematics, and an M.S. degree in Operations Research from Georgia Institute of Technology, advised by Professor Siva Theja Maguluri and Professor John-Paul Clarke . Before that, I obtained my B.Eng. degree in Electrical Engineering at Zhejiang University, Chu Kochen Honors College.
SNAPP Seminar: I currently serve as the webmaster for the Stochastic Networks, Applied Probability, and Performance (SNAPP) seminar. The seminar series is broadly focused on theoretical topics spanning the areas of applied probability. Please visit our website for more details.
Shixiang (Woody) Zhu

I will join Heinz College , Carnegie Mellon University as an Assistant Professor starting August 2022. I obtained my Ph.D. in Machine Learning at H. Milton Stewart School of Industrial and Systems Engineering , Georgia Institute of Technology . I am advised by Yao Xie , Associate Professor in ISyE.
My research lies in the broad area of machine learning, data science, and optimization, with a particular interest in developing models for spatio-temporal data and dynamic networks and decision making under uncertainty . Much of my work aims to develop new methodologies for Social Good and address high-impact problems in a wide array of applications.
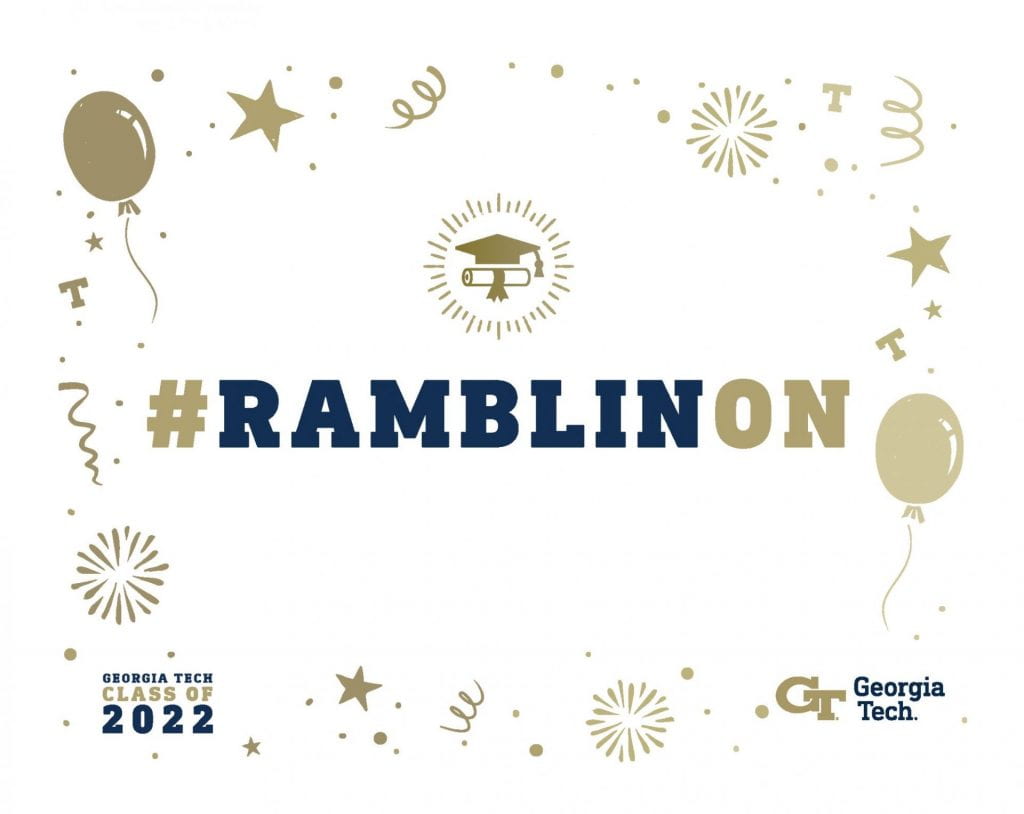
Graduate Education
Office of graduate and postdoctoral education, phd defense by hantian zhang, april 24, 2024.
Data-Centric Bias Mitigation in Machine Learning
Hantian Zhang
Ph.D. Candidate in Computer Science
School of Computer Science
Georgia Institute of Technology
Date/Time: April 24th, 2023, 8:00 AM to 10:00AM Eastern Time (US and Canada)
Location: join with zoom via https://gatech.zoom.us/j/95067902080?pwd=Tkp6cjFGUVhaaERTSzFVY3lQUm1PQT09
Join our Cloud HD Video Meeting
Zoom is the leader in modern enterprise video communications, with an easy, reliable cloud platform for video and audio conferencing, chat, and webinars across mobile, desktop, and room systems. Zoom Rooms is the original software-based conference room solution used around the world in board, conference, huddle, and training rooms, as well as executive offices and classrooms. Founded in 2011, Zoom helps businesses and organizations bring their teams together in a frictionless environment to get more done. Zoom is a publicly traded company headquartered in San Jose, CA.
gatech.zoom.us
Meeting ID: 950 6790 2080
Passcode: 692342
Dr. Xu Chu(co-advisor), School of Computer Science, Georgia Institute of Technology
Dr. Kexin Rong(co-advisor), School of Computer Science, Georgia Institute of Technology
Dr. Joy Arulraj, School of Computer Science, Georgia Institute of Technology
Dr. Shamkant Navathe, School of Computer Science, Georgia Institute of Technology
Dr. Steven Whang, School of Electrical Engineering, KAIST
As Machine Learning (ML) becomes increasingly central to decision-making processes in our society, it is crucial to acknowledge the potential of these ML models to inadvertently perpetuate biases, disproportionately impacting certain demographic groups and individuals. For instance, some ML models used in judicial systems have shown biases against African Americans when predicting recidivism rates. Therefore, addressing the inherent biases and ensuring fairness in ML models is imperative. While enhancements in fairness can be implemented by changing the ML models directly, I argue that a more foundational solution lies in correcting the data as biased data is often the root cause of unfairness.
In my proposed thesis, I aim to systematically understand and mitigate biases in ML models in the full ML life-cycle, from data preparation (pre-processing), to model training (in-processing) and model validation (post-processing). First, I develop a pioneering system, iFlipper, that optimizes for individual fairness in ML. iFlipper enhances training data during data preparation by adjusting the labels, thus mitigating inconsistencies that arise when similar individuals receive varying outcomes. Subsequently, I introduce a declarative system OmniFair that aims at bolstering group fairness in ML. OmniFair allows users to define specific group fairness constraints and change the weight of each training sample during the training process to achieve given group fairness constraints. Finally, I present a method to discover and explain semantically coherent subsets (slices) of unstructured data where the ML models underperform after the models are trained. To be specific, I introduce a new perspective for quantifying explainability in unstructured data slices by borrowing the concept of separability from machine learning literature. I find that separability, which captures how well a slice can be differentiated from the rest of the dataset, complements the coherence measure that focuses on the commonalities of all instances within a slice. With a good understanding of where the ML models are doing poorly, we can improve the ML models by augmenting the dataset and more examples for that specific slice.
Accessibility Information
Download Microsoft Products > Download Adobe Reader >
News Center
Recent phishing scheme at georgia tech.
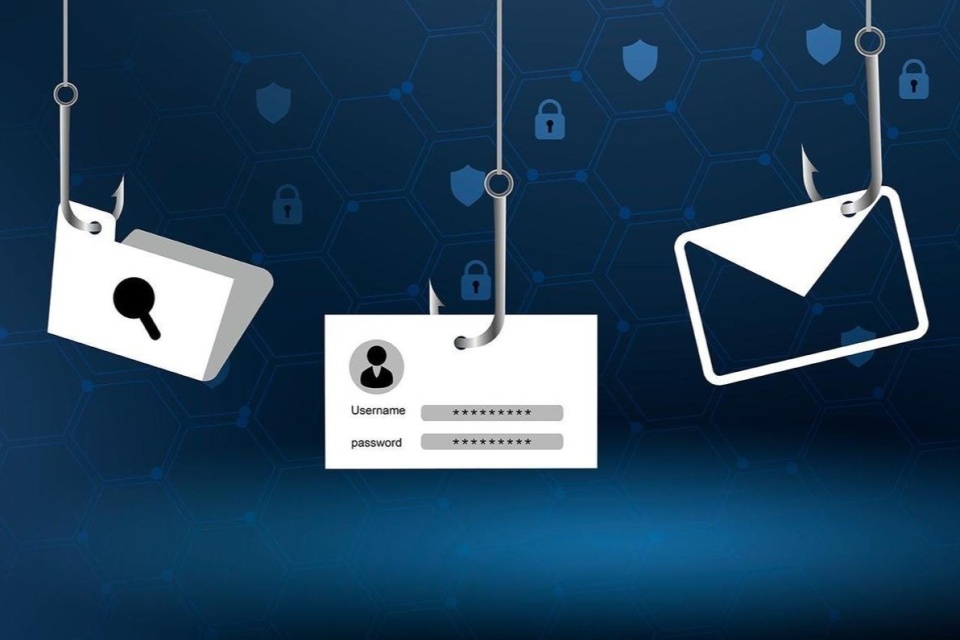
Phishing attempts often involve malicious emails to trick a recipient into revealing passwords and other sensitive information. “Bad actors” may attempt to make the email look legitimate by using an organization’s logo and even its email domain, such as “@gatech.edu.”
The Office of Information Technology (OIT) recently discovered an email phishing scheme that was sent to some members of our campus community. These emails appeared to come from legitimate Georgia Tech email addresses. OIT’s Cyber Security office quickly took measures to prevent these accounts from sending further emails.
OIT believes these emails were sent beginning April 12, and have the following characteristics:
- The subject may begin with, “IT-Desk: Mailbox Is Full,” and may contain an “incident number.”
- The subject may also include a disclaimer stating, “New Messages May Return.”
- The email may state the recipient has exceeded their inbox “quota limit,” and direct them to an attachment to “avoid restrictions and lose incoming messages.”
- The email or attachment may direct users to what appears to be the Georgia Tech Single-Sign-On (SSO) page.
- The email may appear to be a job advertisement.
- The email signature may contain the interlocking GT logo.
- The email may be signed, “Office of Information Technology, Georgia Institute of Technology, © 2024."
If you have received a similar email, or if you ever receive an email that you find suspicious, forward it immediately to [email protected] . It is important that you do not reply to the sender. Do not visit any links within the email and do not forward the email to others.
If you ever believe that your Georgia Tech accounts may have been compromised, contact OIT’s Security Operations Center immediately at [email protected] .
Related links
- Office of Information Technology
School Continues Award-Winning Trend in 2023-2024 Academic Year
The College of Computing’s countdown to commencement began on April 11 when students, faculty, and staff converged at the 33rd Annual Awards Celebration.
The banquet celebrated the college community for an exemplary academic year and recognized the most distinguished individuals of 2023-2024. For Alex Orso, the reception was a high-water mark in his role as interim dean.
“I always say that the best part about my job is to brag about the achievements and accolades of my colleagues,” said Orso.
“It is my distinct honor and privilege to recognize these award winners and the collective success of the College of Computing.”
Orso’s colleagues from the School of Computational Science and Engineering (CSE) were among the celebration’s honorees. School of CSE students, faculty, and alumni earning awards this year include:
- Grace Driskill, M.S. CSE student - The Donald V. Jackson Fellowship
- Harshvardhan Baldwa, M.S. CSE student - The Marshal D. Williamson Fellowship
- Mansi Phute, M.S. CS student- The Marshal D. Williamson Fellowship
- Assistant Professor Chao Zhang- Outstanding Junior Faculty Research Award
- Nazanin Tabatbaei, teaching assistant in Associate Professor Polo Chau’s CSE 6242 Data & Visual Analytics course- Outstanding Instructional Associate Teaching Award
- Rodrigo Borela (Ph.D. CSE-CEE 2021), School of Computing Instruction Lecturer and CSE program alumnus - William D. "Bill" Leahy Jr. Outstanding Instructor Award
- Pratham Metha, undergraduate student in Chau’s research group- Outstanding Legacy Leadership Award
- Alexander Rodriguez (Ph.D. CS 2023), School of CSE alumnus - Outstanding Doctoral Dissertation Award
At the Institute level, Georgia Tech recognized Driskill, Baldwa, and Phute for their awards on April 10 at the annual Student Honors Celebration .
Driskill’s classroom achievement earned her a spot on the 2024 All-ACC Indoor Track and Field Academic Team . This follows her selection for the 2023 All-ACC Academic Team for cross country .
Georgia Tech’s Center for Teaching and Learning released in summer 2023 the Class of 1934 Honor Roll for spring semester courses . School of CSE awardees included Assistant Professor Srijan Kumar (CSE 6240: Web Search & Text Mining), Lecturer Max Mahdi Roozbahani (CS 4641: Machine Learning), and alumnus Mengmeng Liu (CSE 6242: Data & Visual Analytics).
Accolades and recognition of School of CSE researchers for 2023-2024 expounded off campus as well.
School of CSE researchers received awards off campus throughout the year, a testament to the reach and impact of their work.
School of CSE Ph.D. student Gaurav Verma kicked off the year by receiving the J.P. Morgan Chase AI Research Ph.D. Fellowship . Verma was one of only 13 awardees from around the world selected for the 2023 class.
Along with seeing many of his students receive awards this year, Polo Chau attained a 2023 Google Award for Inclusion Research . Later in the year, the Institute promoted Chau to professor , which takes effect in the 2024-2025 academic year.
Schmidt Sciences selected School of CSE Assistant Professor Kai Wang as an AI2050 Early Career Fellow to advance artificial intelligence research for social good. By being part of the fellowship’s second cohort, Wang is the first ever Georgia Tech faculty to receive the award.
School of CSE Assistant Professor Yunan Luo received two significant awards to advance his work in computational biology. First, Luo received the Maximizing Investigator’s Research Award (MIRA) from the National Institutes of Health, which provides $1.8 million in funding for five years. Next, he received the 2023 Molecule Make Lab Institute (MMLI) seed grant .
Regents’ Professor Surya Kalidindi, jointly appointed with the George W. Woodruff School of Mechanical Engineering and School of CSE, was named a fellow to the 2023 class of the Department of Defense’s Laboratory-University Collaboration Initiative (LUCI) .
2023-2024 was a monumental year for Assistant Professor Elizabeth Qian, jointly appointed with the Daniel Guggenheim School of Aerospace Engineering and the School of CSE.
The Air Force Office of Scientific Research selected Qian for the 2024 class of their Young Investigator Program . Earlier in the year, she received a grant under the Department of Energy’s Energy Earthshots Initiative .
Qian began the year by joining 81 other early-career engineers at the National Academy of Engineering’s Grainger Foundation Frontiers of Engineering 2023 Symposium . She also received the Hans Fischer Fellowship from the Institute for Advance Study at the Technical University of Munich.
It was a big academic year for Associate Professor Elizabeth Cherry. Cherry was reelected to a three-year term as a council member-at-large of the Society of Industrial and Applied Mathematics (SIAM) . Cherry is also co-chair of the SIAM organizing committee for next year’s Conference on Computational Science and Engineering (CSE25).
Cherry continues to serve as the School of CSE’s associate chair for academic affairs. These leadership contributions led to her being named to the 2024 ACC Academic Leaders Network (ACC ALN) Fellows program .
School of CSE Professor and Associate Chair Edmond Chow was co-author of a paper that received the Test of Time Award at Supercomputing 2023 (SC23). Right before SC23, Chow’s Ph.D. student Hua Huang was selected as an honorable mention for the 2023 ACM-IEEE CS George Michael Memorial HPC Fellowship .
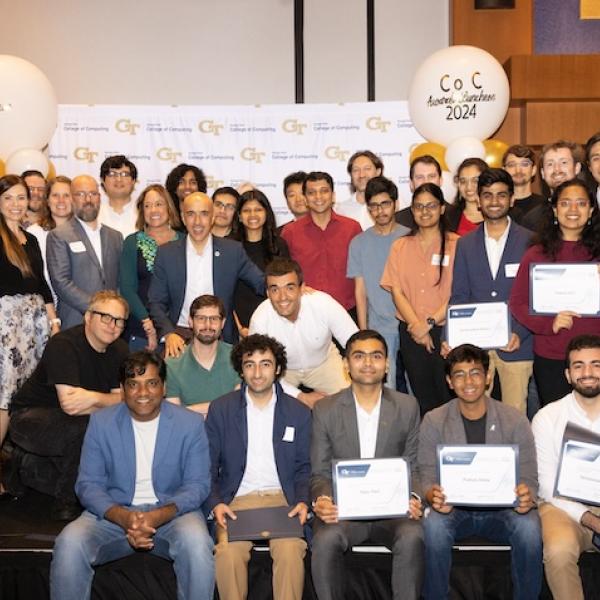
Bryant Wine, Communications Officer [email protected]
Related links
Suggestions or feedback?
MIT News | Massachusetts Institute of Technology
- Machine learning
- Social justice
- Black holes
- Classes and programs
Departments
- Aeronautics and Astronautics
- Brain and Cognitive Sciences
- Architecture
- Political Science
- Mechanical Engineering
Centers, Labs, & Programs
- Abdul Latif Jameel Poverty Action Lab (J-PAL)
- Picower Institute for Learning and Memory
- Lincoln Laboratory
- School of Architecture + Planning
- School of Engineering
- School of Humanities, Arts, and Social Sciences
- Sloan School of Management
- School of Science
- MIT Schwarzman College of Computing
How light can vaporize water without the need for heat
Press contact :, media download.
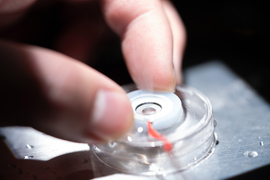
*Terms of Use:
Images for download on the MIT News office website are made available to non-commercial entities, press and the general public under a Creative Commons Attribution Non-Commercial No Derivatives license . You may not alter the images provided, other than to crop them to size. A credit line must be used when reproducing images; if one is not provided below, credit the images to "MIT."
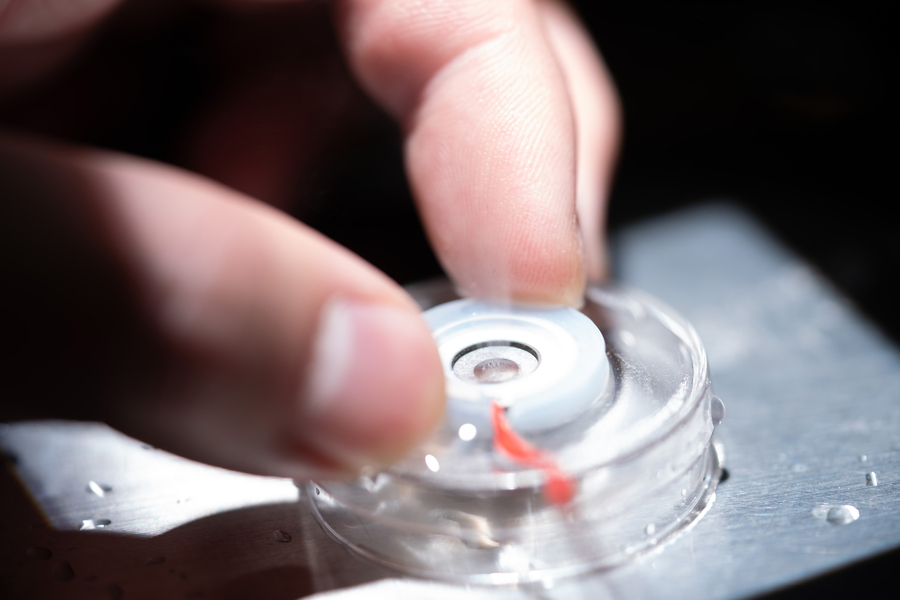
Previous image Next image
It’s the most fundamental of processes — the evaporation of water from the surfaces of oceans and lakes, the burning off of fog in the morning sun, and the drying of briny ponds that leaves solid salt behind. Evaporation is all around us, and humans have been observing it and making use of it for as long as we have existed.
And yet, it turns out, we’ve been missing a major part of the picture all along.
In a series of painstakingly precise experiments, a team of researchers at MIT has demonstrated that heat isn’t alone in causing water to evaporate. Light, striking the water’s surface where air and water meet, can break water molecules away and float them into the air, causing evaporation in the absence of any source of heat.
The astonishing new discovery could have a wide range of significant implications. It could help explain mysterious measurements over the years of how sunlight affects clouds, and therefore affect calculations of the effects of climate change on cloud cover and precipitation. It could also lead to new ways of designing industrial processes such as solar-powered desalination or drying of materials.
The findings, and the many different lines of evidence that demonstrate the reality of the phenomenon and the details of how it works, are described today in the journal PNAS, in a paper by Carl Richard Soderberg Professor of Power Engineering Gang Chen, postdocs Guangxin Lv and Yaodong Tu, and graduate student James Zhang.
The authors say their study suggests that the effect should happen widely in nature— everywhere from clouds to fogs to the surfaces of oceans, soils, and plants — and that it could also lead to new practical applications, including in energy and clean water production. “I think this has a lot of applications,” Chen says. “We’re exploring all these different directions. And of course, it also affects the basic science, like the effects of clouds on climate, because clouds are the most uncertain aspect of climate models.”
A newfound phenomenon
The new work builds on research reported last year , which described this new “photomolecular effect” but only under very specialized conditions: on the surface of specially prepared hydrogels soaked with water. In the new study, the researchers demonstrate that the hydrogel is not necessary for the process; it occurs at any water surface exposed to light, whether it’s a flat surface like a body of water or a curved surface like a droplet of cloud vapor.
Because the effect was so unexpected, the team worked to prove its existence with as many different lines of evidence as possible. In this study, they report 14 different kinds of tests and measurements they carried out to establish that water was indeed evaporating — that is, molecules of water were being knocked loose from the water’s surface and wafted into the air — due to the light alone, not by heat, which was long assumed to be the only mechanism involved.
One key indicator, which showed up consistently in four different kinds of experiments under different conditions, was that as the water began to evaporate from a test container under visible light, the air temperature measured above the water’s surface cooled down and then leveled off, showing that thermal energy was not the driving force behind the effect.
Other key indicators that showed up included the way the evaporation effect varied depending on the angle of the light, the exact color of the light, and its polarization. None of these varying characteristics should happen because at these wavelengths, water hardly absorbs light at all — and yet the researchers observed them.
The effect is strongest when light hits the water surface at an angle of 45 degrees. It is also strongest with a certain type of polarization, called transverse magnetic polarization. And it peaks in green light — which, oddly, is the color for which water is most transparent and thus interacts the least.
Chen and his co-researchers have proposed a physical mechanism that can explain the angle and polarization dependence of the effect, showing that the photons of light can impart a net force on water molecules at the water surface that is sufficient to knock them loose from the body of water. But they cannot yet account for the color dependence, which they say will require further study.
They have named this the photomolecular effect, by analogy with the photoelectric effect that was discovered by Heinrich Hertz in 1887 and finally explained by Albert Einstein in 1905. That effect was one of the first demonstrations that light also has particle characteristics, which had major implications in physics and led to a wide variety of applications, including LEDs. Just as the photoelectric effect liberates electrons from atoms in a material in response to being hit by a photon of light, the photomolecular effect shows that photons can liberate entire molecules from a liquid surface, the researchers say.
“The finding of evaporation caused by light instead of heat provides new disruptive knowledge of light-water interaction,” says Xiulin Ruan, professor of mechanical engineering at Purdue University, who was not involved in the study. “It could help us gain new understanding of how sunlight interacts with cloud, fog, oceans, and other natural water bodies to affect weather and climate. It has significant potential practical applications such as high-performance water desalination driven by solar energy. This research is among the rare group of truly revolutionary discoveries which are not widely accepted by the community right away but take time, sometimes a long time, to be confirmed.”
Solving a cloud conundrum
The finding may solve an 80-year-old mystery in climate science. Measurements of how clouds absorb sunlight have often shown that they are absorbing more sunlight than conventional physics dictates possible. The additional evaporation caused by this effect could account for the longstanding discrepancy, which has been a subject of dispute since such measurements are difficult to make.
“Those experiments are based on satellite data and flight data,“ Chen explains. “They fly an airplane on top of and below the clouds, and there are also data based on the ocean temperature and radiation balance. And they all conclude that there is more absorption by clouds than theory could calculate. However, due to the complexity of clouds and the difficulties of making such measurements, researchers have been debating whether such discrepancies are real or not. And what we discovered suggests that hey, there’s another mechanism for cloud absorption, which was not accounted for, and this mechanism might explain the discrepancies.”
Chen says he recently spoke about the phenomenon at an American Physical Society conference, and one physicist there who studies clouds and climate said they had never thought about this possibility, which could affect calculations of the complex effects of clouds on climate. The team conducted experiments using LEDs shining on an artificial cloud chamber, and they observed heating of the fog, which was not supposed to happen since water does not absorb in the visible spectrum. “Such heating can be explained based on the photomolecular effect more easily,” he says.
Lv says that of the many lines of evidence, “the flat region in the air-side temperature distribution above hot water will be the easiest for people to reproduce.” That temperature profile “is a signature” that demonstrates the effect clearly, he says.
Zhang adds: “It is quite hard to explain how this kind of flat temperature profile comes about without invoking some other mechanism” beyond the accepted theories of thermal evaporation. “It ties together what a whole lot of people are reporting in their solar desalination devices,” which again show evaporation rates that cannot be explained by the thermal input.
The effect can be substantial. Under the optimum conditions of color, angle, and polarization, Lv says, “the evaporation rate is four times the thermal limit.”
Already, since publication of the first paper, the team has been approached by companies that hope to harness the effect, Chen says, including for evaporating syrup and drying paper in a paper mill. The likeliest first applications will come in the areas of solar desalinization systems or other industrial drying processes, he says. “Drying consumes 20 percent of all industrial energy usage,” he points out.
Because the effect is so new and unexpected, Chen says, “This phenomenon should be very general, and our experiment is really just the beginning.” The experiments needed to demonstrate and quantify the effect are very time-consuming. “There are many variables, from understanding water itself, to extending to other materials, other liquids and even solids,” he says.
“The observations in the manuscript points to a new physical mechanism that foundationally alters our thinking on the kinetics of evaporation,” says Shannon Yee, an associate professor of mechanical engineering at Georgia Tech, who was not associated with this work. He adds, “Who would have thought that we are still learning about something as quotidian as water evaporating?”
“I think this work is very significant scientifically because it presents a new mechanism,” says University of Alberta Distinguished Professor Janet A.W. Elliott, who also was not associated with this work. “It may also turn out to be practically important for technology and our understanding of nature, because evaporation of water is ubiquitous and the effect appears to deliver significantly higher evaporation rates than the known thermal mechanism. … My overall impression is this work is outstanding. It appears to be carefully done with many precise experiments lending support for one another.”
The work was partly supported by an MIT Bose Award. The authors are currently working on ways to make use of this effect for water desalination, in a project funded by the Abdul Latif Jameel Water and Food Systems Lab and the MIT-UMRP program.
Share this news article on:
Related links.
- NanoEngineering Group
- Department of Mechanical Engineering
Related Topics
- Desalination
- Mechanical engineering
- Nanoscience and nanotechnology
Related Articles
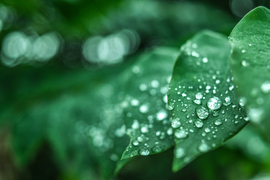
In a surprising finding, light can make water evaporate without heat
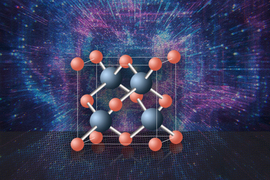
The best semiconductor of them all?
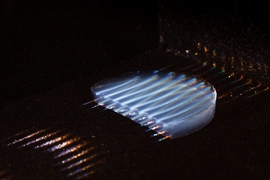
Getting more heat out of sunlight
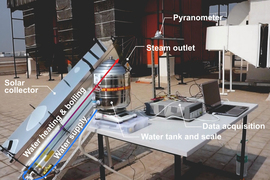
System can sterilize medical tools using solar heat
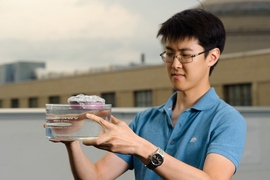
Sponge creates steam using ambient sunlight
Previous item Next item
More MIT News
Study demonstrates efficacy of MIT-led Brave Behind Bars program
Read full story →
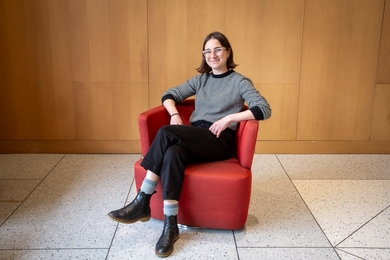
Bringing an investigator’s eye to complex social challenges
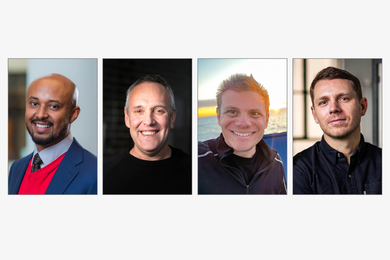
MIT announces 2024 Bose Grants
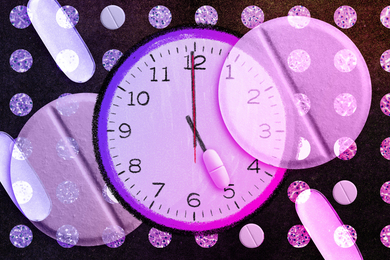
Circadian rhythms can influence drugs’ effectiveness
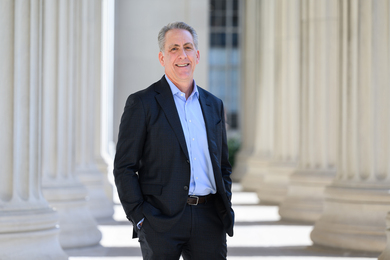
Ian Waitz named vice president for research
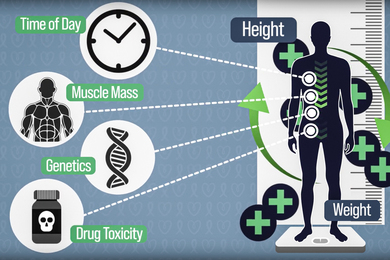
A closed-loop drug-delivery system could improve chemotherapy
- More news on MIT News homepage →
Massachusetts Institute of Technology 77 Massachusetts Avenue, Cambridge, MA, USA
- Map (opens in new window)
- Events (opens in new window)
- People (opens in new window)
- Careers (opens in new window)
- Accessibility
- Social Media Hub
- MIT on Facebook
- MIT on YouTube
- MIT on Instagram
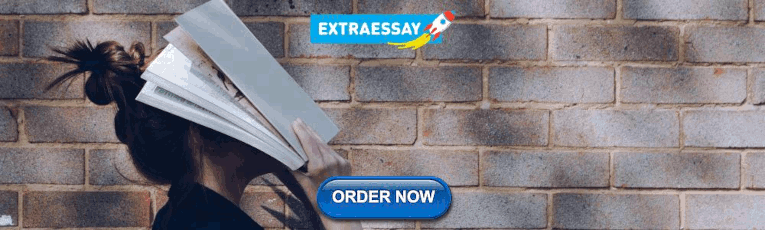
IMAGES
VIDEO
COMMENTS
PhD Program. The machine learning (ML) Ph.D. program is a collaborative venture between Georgia Tech's colleges of Computing, Engineering, and Sciences. Approximately 25-30 students enter the program each year through nine different academic units.
Ph.D. in Machine Learning. The machine learning (ML) Ph.D. program is a collaborative venture between Georgia Tech's colleges of Computing, Engineering, and Sciences and is housed in the Machine Learning Center (ML@GT.) The lifeblood of the program are the ML Ph.D. students, and the ML Ph.D. Program Faculty who advise, mentor, and conduct ...
The machine learning (ML) Ph.D. program is a collaborative venture between Georgia Tech's colleges of Computing, Engineering, and Sciences and is housed in the Machine Learning Center (ML@GT.) The lifeblood of the program are the ML Ph.D. students, and the ML Ph.D. Program Faculty who advise, mentor, and conduct research with these students.
The Machine Learning Ph.D. is an interdisciplinary doctoral program spanning three colleges (Computing, Engineering, Sciences). Students are admitted through one of eight participating home schools: Admission to the ML PhD program is contingent on meeting the requirement for admission into one of these schools.
Core curriculum (4 courses, 12 hours). Machine Learning PhD students will be required to complete courses in four different areas: Mathematical Foundations, Probabilistic and Statistical Methods in Machine Learning, ML Theory and Methods, and Optimization. Area electives (5 courses, 15 hours). Responsible Conduct of Research (RCR) (1 course, 1 ...
Application deadlines, minimum GRE/TOEFL scores, and other requirements are all determined by the home school. Applications that satisfy all of the requirements are then forwarded to the Machine Learning Faculty Advisory Committee (ML FAC) for review. Decisions for admissions are made jointly between the home unit and the ML faculty.
Ph.D. Programs. For those students looking to build a career in computing research, the School of Interactive Computing offers a range of Ph.D. programs that allow students to work side-by-side with some of the most brilliant researchers and computer scientists in the world. For those looking to join the ranks of academia, we regularly place ...
Graduate offerings of these courses are also available, as are specialized seminars on a range of topics in vision, language, deep learning, cognition and their intersections (e.g., Machine Learning with Limited Supervision). In addition, several courses in robotics and HCI also discuss new methods in AI or ML.
Interdisciplinary program devoted to the creation, study, and application of computer-based models of natural and engineered systems. Ph.D. in Computer Science. As a research-oriented degree, the Ph.D. in Computer Science prepares exceptional students for careers at the cutting edge of academia, industry and government. Ph.D. in Machine Learning.
PhD in Machine Learning (ML) Machine learning aims to produce machines that can learn from their experiences and make predictions based on those experiences and other data they have analyzed. This field crosses a wide variety of disciplines that use data to find patterns in the ways both living systems, such as the human body and artificial ...
Prepared by: The Machine Learning Faculty, November, 2017. The exam will be divided to four areas: core, statistical methods and models, learning theory, and decision processes. There will be three questions in each area. Each student has to answer two out of the three questions in the core area. In addition, each student has to select two out ...
Giving to the Coulter Department of Biomedical Engineering. Private support gives the Coulter Department the resources to take the lead in new initiatives, to weather cyclical changes in support from government, and to make long-term investments in constantly changing technology, often before needs or opportunities are recognized by others.
Fall 2023. The purpose of this course is to provide first year PhD students in engineering and computing with a solid mathematical background for two of the pillars of modern machine learning, data science, and artificial intelligence: linear algebra and applied probability. Instructor: Justin Romberg. Download the syllabus.
Bio: Ran is a Machine Learning PhD student at Georgia Tech. Originally from mainland China, Ran received her bachelor's degree in Physics from Fudan University with a lot of extra courses in data science, mathematics, and social science. Ran is broadly interested in machine learning and deep learning, and is particularly passionate about ...
My current research focuses on learning based algorithm design (more theory-oriented), deep learning on structured data (more application-oriented), and their intersections. Besides, I am interested in applications in the area of structural and computational biology. My research is generously supported by Google PhD Fellowship in Machine Learning.
To be specific, I introduce a new perspective for quantifying explainability in unstructured data slices by borrowing the concept of separability from machine learning literature. I find that separability, which captures how well a slice can be differentiated from the rest of the dataset, complements the coherence measure that focuses on the ...
The Office of Information Technology (OIT) recently discovered an email phishing scheme that was sent to some members of our campus community. These emails appeared to come from legitimate Georgia Tech email addresses. OIT's Cyber Security office quickly took measures to prevent these accounts from sending further emails.
The College of Computing's countdown to commencement began on April 11 when students, faculty, and staff converged at the 33rd Annual Awards Celebration. The banquet celebrated the college community for an exemplary academic year and recognized the most distinguished individuals of 2023-2024. For Alex Orso, the reception was a high-water mark in his role as interim dean. "I always say that ...
Machine learning; Social justice; Startups; ... and graduate student James Zhang. The authors say their study suggests that the effect should happen widely in nature— everywhere from clouds to fogs to the surfaces of oceans, soils, and plants — and that it could also lead to new practical applications, including in energy and clean water ...