Editor's Choice: AI Could Mean Better Mental Health for All

- Original Investigation Ponatinib vs Imatinib in Frontline Philadelphia Chromosome–Positive Acute Lymphoblastic Leukemia: A Randomized Clinical Trial Elias Jabbour, MD; Hagop M. Kantarjian, MD; Ibrahim Aldoss, MD; et al Editorial PhALLCON Soars to New Heights—Faster, Stronger, but Better? Rebecca P. Bystrom, MD; Daniel J. DeAngelo, MD, PhD; Jacqueline S. Garcia, MD
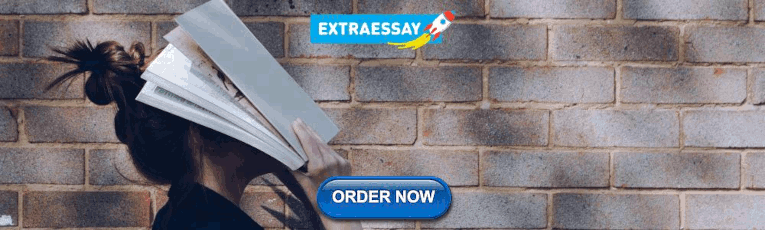
Just Published
- Ponatinib vs Imatinib in Frontline Philadelphia Chromosome–Positive ALL Elias Jabbour, MD; et al. Original Investigation online first Elias Jabbour, MD; et al. Editorial
- Cardiovascular-Kidney-Metabolic Syndrome Stages in US Adults Rahul Aggarwal, MD; et al. Research Letter online first Rahul Aggarwal, MD; et al.
- Associations of Milestone Ratings and Certification Examination Scores With Patient Outcomes Bradley M. Gray, PhD; et al. Original Investigation online first Bradley M. Gray, PhD; et al.
- Substances in Counterfeit Prescription Pills Seized by Law Enforcement, 2017-2022 Rachel S. Wightman, MD; et al. Research Letter online first Rachel S. Wightman, MD; et al.
- Youth Parental Death Due to Drug Poisoning and Firearm Violence in the US Benjamin-Samuel Schlüter, PhD; et al. Original Investigation online first Benjamin-Samuel Schlüter, PhD; et al.
- Meaning of Proposed Causal Inference Framework for the JAMA Network Annette Flanagin, RN, MA; et al. Editor's Note online first free access Annette Flanagin, RN, MA; et al.
- Best Versions of Ourselves Evelyn Blankenship-Lai, RN, MSN A Piece of My Mind online first Evelyn Blankenship-Lai, RN, MSN
- PhALLCON Soars to New Heights—Faster, Stronger, but Better? Rebecca P. Bystrom, MD; et al. Editorial online first Rebecca P. Bystrom, MD; et al.
- Safeguarding Research Using Federal Health Insurance Data William L. Schpero, PhD; et al. Viewpoint online first William L. Schpero, PhD; et al.
- Risks of Harm in Alzheimer Disease by Amyloid Lowering Madhav Thambisetty, MD, PhD; et al. Viewpoint online first Madhav Thambisetty, MD, PhD; et al.
- Causal Inference and Effects of Interventions From Observational Studies in Medical Journals Issa J. Dahabreh, MD, ScD; et al. Special Communication online first free access has active quiz Issa J. Dahabreh, MD, ScD; et al. Editor's Note
- A 3-Year-Old With Gingival Hemorrhage and Musculoskeletal Pain Khanh Trinh, DMD; et al. JAMA Clinical Challenge online first has active quiz Khanh Trinh, DMD; et al.
- The Women’s Health Initiative Randomized Trials and Clinical Practice JoAnn E. Manson, MD, DrPH; et al. Review online first has active quiz JoAnn E. Manson, MD, DrPH; et al.
- Lower Gastrointestinal Hemorrhage Hira Imran, MD; et al. JAMA Clinical Guidelines Synopsis online first has active quiz Hira Imran, MD; et al.
- Interstitial Lung Disease Toby M. Maher, MD, MSc, PhD Review online first has active quiz has multimedia Toby M. Maher, MD, MSc, PhD
Latest from the USPSTF
- USPSTF Recommendation: Screening for Breast Cancer
- USPSTF Recommendation: Primary Care Interventions to Prevent Child Maltreatment
- USPSTF Recommendation: Screening for Speech and Language Delay and Disorders
- 66,922 Views USPSTF Recommendation: Screening for Breast Cancer
- 28,406 Views Effect of Tirzepatide on Maintenance of Weight Reduction
- 26,957 Views Interstitial Lung Disease
- 26,806 Views Prostate-Specific Antigen Screening and 15-Year Prostate Cancer Mortality
- 26,082 Views The Women’s Health Initiative Randomized Trials and Clinical Practice
- 24,676 Views Good Enough
- 20,765 Views Aspirin vs Placebo as Adjuvant Therapy for Breast Cancer
- 20,643 Views Acetaminophen Use During Pregnancy and Children’s Risk of Autism, ADHD, and Intellectual Disability
- 20,124 Views Blockbuster Obesity Drugs Have Potential New Uses
- 20,087 Views Serious Bleeding in Patients With Atrial Fibrillation Using Diltiazem With Apixaban or Rivaroxaban
- 724 Citations Antibody Response to 2-Dose SARS-CoV-2 mRNA Vaccine Series in Solid Organ Transplant Recipients
- 705 Citations Strengthening the Reporting of Observational Studies in Epidemiology Using Mendelian Randomization
- 627 Citations Pancreatic Cancer
- 624 Citations USPSTF Recommendation: Screening for Colorectal Cancer
- 622 Citations Updated Guidance on the Reporting of Race and Ethnicity in Medical and Science Journals
- 522 Citations Effect of 2 Inactivated SARS-CoV-2 Vaccines on Symptomatic COVID-19 Infection in Adults
- 448 Citations Association Between IL-6 Antagonists and Mortality Among Patients Hospitalized for COVID-19
- 412 Citations Association Between 3 Doses of mRNA COVID-19 Vaccine and Symptomatic Infection Caused by Omicron and Delta Variants
- 383 Citations Cerebral Venous Sinus Thrombosis With Thrombocytopenia After Ad26.COV2.S Vaccination
- 381 Citations Association of COVID-19 mRNA Vaccination With Hospitalizations and Disease Severity
- Register for email alerts with links to free full-text articles
- Access PDFs of free articles
- Manage your interests
- Save searches and receive search alerts
When you choose to publish with PLOS, your research makes an impact. Make your work accessible to all, without restrictions, and accelerate scientific discovery with options like preprints and published peer review that make your work more Open.
- PLOS Biology
- PLOS Climate
- PLOS Complex Systems
- PLOS Computational Biology
- PLOS Digital Health
- PLOS Genetics
- PLOS Global Public Health
- PLOS Medicine
- PLOS Mental Health
- PLOS Neglected Tropical Diseases
- PLOS Pathogens
- PLOS Sustainability and Transformation
- PLOS Collections
- About This Blog
- Author Guidelines
- Official PLOS Blog
- EveryONE Blog
- Speaking of Medicine
- PLOS Biologue
- Absolutely Maybe
- DNA Science
- PLOS ECR Community
- All Models Are Wrong
- About PLOS Blogs
Explore the latest research making an impact in medicine and healthcare research

Our portfolio of medicine and healthcare journals continues to push the boundaries of research and research communication. Discover the latest papers making waves in your community, explore further insight into the contributions of PLOS journals to the field, and keep up-to-date with the latest research in 2024.
Selected most talked about so far in 2024*
1-year weight change after diabetes diagnosis and long-term incidence and sustainability of remission of type 2 diabetes in real-world settings in Hong Kong: An observational cohort study PLOS Medicine
Developing interconnectedness is critical in retaining rural general practitioners: A qualitative thematic analysis of recently recruited general practitioners to South East New South Wales, Australia mance of ChatGPT on USMLE: Potential for AI-assisted medical education using large language models PLOS Global Public Health
Impact on wine sales of removing the largest serving size by the glass: An A-B-A reversal trial in 21 pubs, bars, and restaurants in England PLOS Medicine
Unraveling the interplay of circadian rhythm and sleep deprivation on mood: A Real-World Study on first-year physicians PLOS Digital Health
Old tools, new applications: Use of environmental bacteriophages for typhoid surveillance and evaluating vaccine impact PLOS Neglected Tropical Diseases
Further reading and insights
PLOS publishes a broad range of research in medicine and health, spanning journals, disciplines, and regions.
Discover more research and insights in our curated research selections from across the PLOS portfolio.
Want to keep in the loop?
Discover the scope and submission guidelines for each of our medicine and healthcare journals, or simply sign up to receive the latest research direct to your inbox.
*data taken from Altmetric , January 2024 – May 2024.
Leave a Reply Cancel reply
Your email address will not be published. Required fields are marked *
Save my name and email for the next time I comment.
Alexander T. Limkakeng and Judd E Hollander discuss how the COVID-19 pandemic is changing the way clinical research is conducted and…
Michel Kazatchkine (Special Advisor to the Joint United Nations Program on AIDS (UNAIDS) for Eastern Europe and Central Asia), Jean-Louis Vincent…
A message from PLOS NTDs Managing Editor, Kendall McKenzie We hope you are safe and well during these turbulent times. We would…
Research articles
Ultra-processed food consumption and all cause and cause specific mortality, comparative effectiveness of second line oral antidiabetic treatments among people with type 2 diabetes mellitus, efficacy of psilocybin for treating symptoms of depression, reverse total shoulder replacement versus anatomical total shoulder replacement for osteoarthritis, effect of combination treatment with glp-1 receptor agonists and sglt-2 inhibitors on incidence of cardiovascular and serious renal events, prenatal opioid exposure and risk of neuropsychiatric disorders in children, temporal trends in lifetime risks of atrial fibrillation and its complications, antipsychotic use in people with dementia, predicting the risks of kidney failure and death in adults with moderate to severe chronic kidney disease, impact of large scale, multicomponent intervention to reduce proton pump inhibitor overuse, esketamine after childbirth for mothers with prenatal depression, glucagon-like peptide 1 receptor agonist use and risk of thyroid cancer, use of progestogens and the risk of intracranial meningioma, delirium and incident dementia in hospital patients, derivation and external validation of a simple risk score for predicting severe acute kidney injury after intravenous cisplatin, quality and safety of artificial intelligence generated health information, large language models and the generation of health disinformation, 25 year trends in cancer incidence and mortality among adults in the uk, cervical pessary versus vaginal progesterone in women with a singleton pregnancy, comparison of prior authorization across insurers, diagnostic accuracy of magnetically guided capsule endoscopy with a detachable string for detecting oesophagogastric varices in adults with cirrhosis, ultra-processed food exposure and adverse health outcomes, added benefit and revenues of oncology drugs approved by the ema, exposure to air pollution and hospital admission for cardiovascular diseases, short term exposure to low level ambient fine particulate matter and natural cause, cardiovascular, and respiratory morbidity, optimal timing of influenza vaccination in young children, effect of exercise for depression, association of non-alcoholic fatty liver disease with cardiovascular disease and all cause death in patients with type 2 diabetes, duration of cpr and outcomes for adults with in-hospital cardiac arrest, clinical effectiveness of an online physical and mental health rehabilitation programme for post-covid-19 condition, atypia detected during breast screening and subsequent development of cancer, publishers’ and journals’ instructions to authors on use of generative ai in academic and scientific publishing, effectiveness of glp-1 receptor agonists on glycaemic control, body weight, and lipid profile for type 2 diabetes, neurological development in children born moderately or late preterm, invasive breast cancer and breast cancer death after non-screen detected ductal carcinoma in situ, all cause and cause specific mortality in obsessive-compulsive disorder, acute rehabilitation following traumatic anterior shoulder dislocation, perinatal depression and risk of mortality, undisclosed financial conflicts of interest in dsm-5-tr, effect of risk mitigation guidance opioid and stimulant dispensations on mortality and acute care visits, update to living systematic review on sars-cov-2 positivity in offspring and timing of mother-to-child transmission, perinatal depression and its health impact, christmas 2023: common healthcare related instruments subjected to magnetic attraction study, using autoregressive integrated moving average models for time series analysis of observational data, demand for morning after pill following new year holiday, christmas 2023: christmas recipes from the great british bake off, effect of a doctor working during the festive period on population health: experiment using doctor who episodes, christmas 2023: analysis of barbie medical and science career dolls, christmas 2023: effect of chair placement on physicians’ behavior and patients’ satisfaction, management of chronic pain secondary to temporomandibular disorders, christmas 2023: projecting complete redaction of clinical trial protocols, christmas 2023: a drug target for erectile dysfunction to help improve fertility, sexual activity, and wellbeing, christmas 2023: efficacy of cola ingestion for oesophageal food bolus impaction, conservative management versus laparoscopic cholecystectomy in adults with gallstone disease, social media use and health risk behaviours in young people, untreated cervical intraepithelial neoplasia grade 2 and cervical cancer, air pollution deaths attributable to fossil fuels, implementation of a high sensitivity cardiac troponin i assay and risk of myocardial infarction or death at five years, covid-19 vaccine effectiveness against post-covid-19 condition, association between patient-surgeon gender concordance and mortality after surgery, intravascular imaging guided versus coronary angiography guided percutaneous coronary intervention, treatment of lower urinary tract symptoms in men in primary care using a conservative intervention, autism intervention meta-analysis of early childhood studies, effectiveness of the live zoster vaccine during the 10 years following vaccination, effects of a multimodal intervention in primary care to reduce second line antibiotic prescriptions for urinary tract infections in women, pyrotinib versus placebo in combination with trastuzumab and docetaxel in patients with her2 positive metastatic breast cancer, association of dcis size and margin status with risk of developing breast cancer post-treatment, racial differences in low value care among older patients in the us, pharmaceutical industry payments and delivery of low value cancer drugs, rosuvastatin versus atorvastatin in adults with coronary artery disease, clinical effectiveness of septoplasty versus medical management for nasal airways obstruction, ultrasound guided lavage with corticosteroid injection versus sham lavage with and without corticosteroid injection for calcific tendinopathy of shoulder, early versus delayed antihypertensive treatment in patients with acute ischaemic stroke, mortality risks associated with floods in 761 communities worldwide, interactive effects of ambient fine particulate matter and ozone on daily mortality in 372 cities, association between changes in carbohydrate intake and long term weight changes, future-case control crossover analysis for adjusting bias in case crossover studies, association between recently raised anticholinergic burden and risk of acute cardiovascular events, suboptimal gestational weight gain and neonatal outcomes in low and middle income countries: individual participant data meta-analysis, efficacy and safety of an inactivated virus-particle vaccine for sars-cov-2, effect of invitation letter in language of origin on screening attendance: randomised controlled trial in breastscreen norway, visits by nurse practitioners and physician assistants in the usa, non-erosive gastro-oesophageal reflux disease and oesophageal adenocarcinoma, venous thromboembolism with use of hormonal contraception and nsaids, food additive emulsifiers and risk of cardiovascular disease, balancing risks and benefits of cannabis use, promoting activity, independence, and stability in early dementia and mild cognitive impairment, effect of home cook interventions for salt reduction in china, cancer mortality after low dose exposure to ionising radiation, effect of a smartphone intervention among university students with unhealthy alcohol use, long term risk of death and readmission after hospital admission with covid-19 among older adults, mortality rates among patients successfully treated for hepatitis c, association between antenatal corticosteroids and risk of serious infection in children, the proportions of term or late preterm births after exposure to early antenatal corticosteroids, and outcomes, safety of ba.4-5 or ba.1 bivalent mrna booster vaccines, comparative effectiveness of booster vaccines among adults aged ≥50 years, third dose vaccine schedules against severe covid-19 during omicron predominance in nordic countries, private equity ownership and impacts on health outcomes, costs, and quality, healthcare disruption due to covid-19 and avoidable hospital admission, follow us on, content links.
- Collections
- Health in South Asia
- Women’s, children’s & adolescents’ health
- News and views
- BMJ Opinion
- Rapid responses
- Editorial staff
- BMJ in the USA
- BMJ in South Asia
- Submit your paper
- BMA members
- Subscribers
- Advertisers and sponsors
Explore BMJ
- Our company
- BMJ Careers
- BMJ Learning
- BMJ Masterclasses
- BMJ Journals
- BMJ Student
- Academic edition of The BMJ
- BMJ Best Practice
- The BMJ Awards
- Email alerts
- Activate subscription
Information
Medical image analysis based on deep learning approach
- Published: 06 April 2021
- Volume 80 , pages 24365–24398, ( 2021 )
Cite this article
- Muralikrishna Puttagunta 1 &
- S. Ravi ORCID: orcid.org/0000-0001-7267-9233 1
34k Accesses
112 Citations
9 Altmetric
Explore all metrics
Medical imaging plays a significant role in different clinical applications such as medical procedures used for early detection, monitoring, diagnosis, and treatment evaluation of various medical conditions. Basicsof the principles and implementations of artificial neural networks and deep learning are essential for understanding medical image analysis in computer vision. Deep Learning Approach (DLA) in medical image analysis emerges as a fast-growing research field. DLA has been widely used in medical imaging to detect the presence or absence of the disease. This paper presents the development of artificial neural networks, comprehensive analysis of DLA, which delivers promising medical imaging applications. Most of the DLA implementations concentrate on the X-ray images, computerized tomography, mammography images, and digital histopathology images. It provides a systematic review of the articles for classification, detection, and segmentation of medical images based on DLA. This review guides the researchers to think of appropriate changes in medical image analysis based on DLA.
Similar content being viewed by others
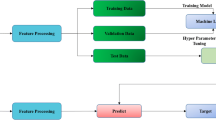
Machine learning and deep learning approach for medical image analysis: diagnosis to detection
UNet++: A Nested U-Net Architecture for Medical Image Segmentation
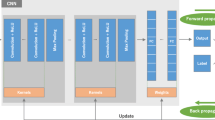
Convolutional neural networks: an overview and application in radiology
Avoid common mistakes on your manuscript.
1 Introduction
In the health care system, there has been a dramatic increase in demand for medical image services, e.g. Radiography, endoscopy, Computed Tomography (CT), Mammography Images (MG), Ultrasound images, Magnetic Resonance Imaging (MRI), Magnetic Resonance Angiography (MRA), Nuclear medicine imaging, Positron Emission Tomography (PET) and pathological tests. Besides, medical images can often be challenging to analyze and time-consuming process due to the shortage of radiologists.
Artificial Intelligence (AI) can address these problems. Machine Learning (ML) is an application of AI that can be able to function without being specifically programmed, that learn from data and make predictions or decisions based on past data. ML uses three learning approaches, namely, supervised learning, unsupervised learning, and semi-supervised learning. The ML techniques include the extraction of features and the selection of suitable features for a specific problem requires a domain expert. Deep learning (DL) techniques solve the problem of feature selection. DL is one part of ML, and DL can automatically extract essential features from raw input data [ 88 ]. The concept of DL algorithms was introduced from cognitive and information theories. In general, DL has two properties: (1) multiple processing layers that can learn distinct features of data through multiple levels of abstraction, and (2) unsupervised or supervised learning of feature presentations on each layer. A large number of recent review papers have highlighted the capabilities of advanced DLA in the medical field MRI [ 8 ], Radiology [ 96 ], Cardiology [ 11 ], and Neurology [ 155 ].
Different forms of DLA were borrowed from the field of computer vision and applied to specific medical image analysis. Recurrent Neural Networks (RNNs) and convolutional neural networks are examples of supervised DL algorithms. In medical image analysis, unsupervised learning algorithms have also been studied; These include Deep Belief Networks (DBNs), Restricted Boltzmann Machines (RBMs), Autoencoders, and Generative Adversarial Networks (GANs) [ 84 ]. DLA is generally applicable for detecting an abnormality and classify a specific type of disease. When DLA is applied to medical images, Convolutional Neural Networks (CNN) are ideally suited for classification, segmentation, object detection, registration, and other tasks [ 29 , 44 ]. CNN is an artificial visual neural network structure used for medical image pattern recognition based on convolution operation. Deep learning (DL) applications in medical images are visualized in Fig. 1 .
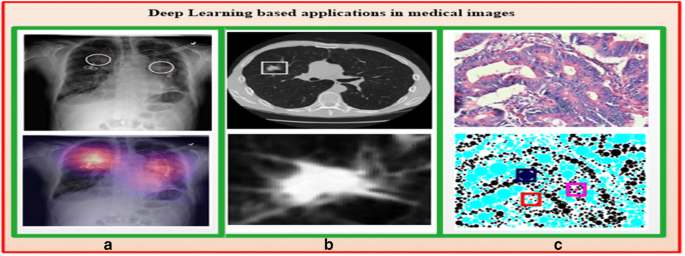
a X-ray image with pulmonary masses [ 121 ] b CT image with lung nodule [ 82 ] c Digitized histo pathological tissue image [ 132 ]
2 Neural networks
2.1 history of neural networks.
The study of artificial neural networks and deep learning derives from the ability to create a computer system that simulates the human brain [ 33 ]. A neurophysiologist, Warren McCulloch, and a mathematician Walter Pitts [ 97 ] developed a primitive neural network based on what has been known as a biological structure in the early 1940s. In 1949, a book titled “Organization of Behavior” [ 100 ] was the first to describe the process of upgrading synaptic weights which is now referred to as the Hebbian Learning Rule. In 1958, Frank Rosenblatt’s [ 127 ] landmark paper defined the structure of the neural network called the perceptron for the binary classification task.
In 1962, Windrow [ 172 ] introduced a device called the Adaptive Linear Neuron (ADALINE) by implementing their designs in hardware. The limitations of perceptions were emphasized by Minski and Papert (1969) [ 98 ]. The concept of the backward propagation of errors for purposes of training is discussed in Werbose1974 [ 171 ]. In 1979, Fukushima [ 38 ] designed artificial neural networks called Neocognitron, with multiple pooling and convolution layers. One of the most important breakthroughs in deep learning occurred in 2006, when Hinton et al. [ 9 ] implemented the Deep Belief Network, with several layers of Restricted Boltzmann Machines, greedily teaching one layer at a time in an unsupervised fashion. In 1989, Yann LeCun [ 71 ] combined CNN with backpropagation to effectively perform the automated recognition of handwritten digits. Figure 2 shows important advancements in the history of neural networks that led to a deep learning era.
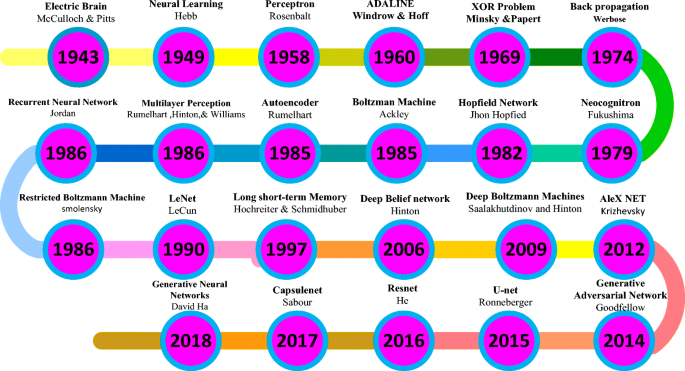
Demonstrations of significant developments in the history of neural networks [ 33 , 134 ]
2.2 Artificial neural networks
Artificial Neural Networks (ANN) form the basis for most of the DLA. ANN is a computational model structure that has some performance characteristics similar to biological neural networks. ANN comprises simple processing units called neurons or nodes that are interconnected by weighted links. A biological neuron can be described mathematically in Eq. ( 1 ). Figure 3 shows the simplest artificial neural model known as the perceptron.
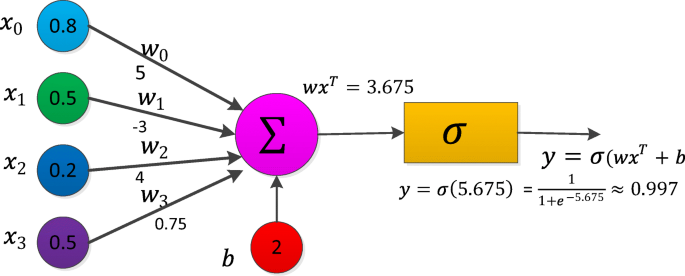
Perceptron [ 77 ]
2.3 Training a neural network with Backpropagation (BP)
In the neural networks, the learning process is modeled as an iterative process of optimization of the weights to minimize a loss function. Based on network performance, the weights are modified on a set of examples belonging to the training set. The necessary steps of the training procedure contain forward and backward phases. For Neural Network training, any of the activation functions in forwarding propagation is selected and BP training is used for changing weights. The BP algorithm helps multilayer FFNN to learn input-output mappings from training samples [ 16 ]. Forward propagation and backpropagation are explained with the one hidden layer deep neural networks in the following algorithm.
The backpropagation algorithm is as follows for one hidden layer neural network
Initialize all weights to small random values.
While the stopping condition is false, do steps 3 through10.
For each training pair (( x 1 , y 1 )…( x n , y n ) do steps 4 through 9.
Feed-forward propagation:
Each input unit ( X i , i = 1, 2, … n ) receives the input signal x i and send this signal to all hidden units in the above layer.
Each hidden unit ( Z j , j = 1. ., p ) compute output using the below equation, and it transmits to the output unit (i.e.) \( {z}_{j\_ in}={b}_j+{\sum}_{i=1}^n{w}_{ij}{x}_i \) applies to an activation function Z j = f ( Z j _ in ).
Compute the out signal for each output unit ( Y k , k = 1, …., m ).
\( {y}_{k\_ in}={b}_k+{\sum}_{j=1}^p{z}_j{w}_{jk} \) and calculate activation y k = f ( y k _ in )
Backpropagation
For input training pattern ( x 1 , x 2 …., x n ) corresponding output pattern ( y 1 , y 2 , …, y m ), let ( t 1 , t 2 , …. . t m ) be target pattern. For each output, the neuron computes network error δ k
At output-layer neurons δ k = ( t k − y k ) f ′ ( y k _ in )
For each hidden neuron, calculate its error information term δ j while doing so, use δ k of the output neurons as obtained in the previous step
At Hidden layer neurons \( {\delta}_j={f}^{\prime}\left({z}_{j\_ in}\right){\sum}_k^m{\delta}_k{w}_{jk} \)
Update weights and biases using the following formulas where η is learning rate
Each output layer ( Y k , k = 1, 2, …. m ) updates its weights ( J = 0, 1, … P ) and bias
w jk ( new ) = w jk ( old ) + ηδ k z j ; b k ( new ) = b k ( old ) + ηδ k
Each hidden layer ( Z J , J = 1, 2, … p ) updates its weights ( i = 0, 1, … n ) biases:
w ij ( new ) = w ij ( old ) + ηδ j x i ; b j ( old ) = b j ( old ) + ηδ j
Test stopping condition
2.4 Activation function
The activation function is the mechanism by which artificial neurons process and transfers information [ 42 ]. There are various types of activation functions which can be used in neural networks based on the characteristic of the application. The activation functions are non-linear and continuously differentiable. Differentiability property is important mainly when training a neural network using the gradient descent method. Some widely used activation functions are listed in Table 1 .
3 Deep learning
Deep learning is a subset of the machine learning field which deals with the development of deep neural networks inspired by biological neural networks in the human brain .
3.1 Autoencoder
Autoencoder (AE) [ 128 ] is one of the deep learning models which exemplifies the principle of unsupervised representation learning as depicted in Fig. 4a . AE is useful when the input data have more number of unlabelled data compared to labeled data. AE encodes the input x into a lower-dimensional space z. The encoded representation is again decoded to an approximated representation x ′ of the input x through one hidden layer z.
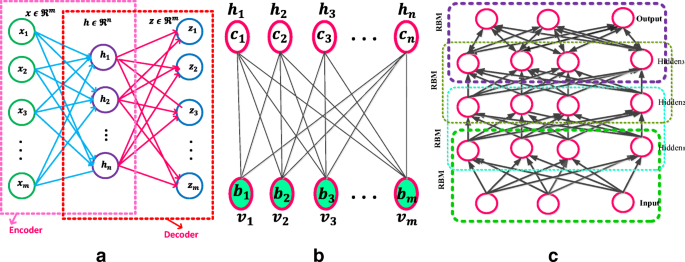
a Autoencoder [ 187 ] b Restricted Boltzmann Machine with n hidden and m visible units [ 88 ] c Deep Belief Networks [ 88 ]
Basic AE consists of three main steps:
Encode: Convert input vector \( x\ \epsilon\ {\mathbf{\mathfrak{R}}}^{\boldsymbol{m}} \) into \( h\ \epsilon\ {\mathbf{\mathfrak{R}}}^{\mathrm{n}} \) , the hidden layer by h = f ( wx + b )where \( w\ \epsilon\ {\mathbf{\mathfrak{R}}}^{\boldsymbol{m}\ast \boldsymbol{n}} \) and \( b\ \epsilon\ {\mathbf{\mathfrak{R}}}^{\boldsymbol{n}} \) . m and n are dimensions of the input vector and converted hidden state. The dimension of the hidden layer h is to be smaller than x . f is an activate function.
Decode: Based on the above h , reconstruct input vector z by equation z = f ′ ( w ′ h + b ′ ) where \( {w}^{\prime}\epsilon\ {\mathbf{\mathfrak{R}}}^{\boldsymbol{n}\ast \boldsymbol{m}} \) and \( {b}^{\prime}\boldsymbol{\epsilon} {\mathbf{\mathfrak{R}}}^{\boldsymbol{m}}. \) The f ′ is the same as the above activation function.
Calculate square error: L recons ( x , z) = ∥ x − z∥ 2 , which is the reconstruction error cost function. Reconstruct error minimization is achieved by optimizing the cost function (2)
Another unsupervised algorithm representation is known as Stacked Autoencoder (SAE). The SAE comprises stacks of autoencoder layers mounted on top of each other where the output of each layer was wired to the inputs of the next layer. A Denoising Autoencoder (DAE) was introduced by Vincent et al. [ 159 ]. The DAE is trained to reconstruct the input from random noise added input data. Variational autoencoder (VAE) [ 66 ] is modifying the encoder where the latent vector space is used to represent the images that follow a Gaussian distribution unit. There are two losses in this model; one is a mean squared error and the Kull back Leibler divergence loss that determines how close the latent variable matches the Gaussian distribution unit. Sparse autoencoder [ 106 ] and variational autoencoders have applications in unsupervised, semi-supervised learning, and segmentation.
3.2 Restricted Boltzmann machine
A Restricted Boltzmann machine [RBM] is a Markov Random Field (MRF) associated with the two-layer undirected probabilistic generative model, as shown in Fig. 4b . RBM contains visible units (input) v and hidden (output) units h . A significant feature of this model is that there is no direct contact between the two visible units or either of the two hidden units. In binary RBMs, the random variables ( v , h ) takes ( v , h ) ∈ {0, 1} m + n . Like the general Boltzmann machine [ 50 ], the RBM is an energy-based model. The energy of the state { v , h } is defined as (3)
where v j , h i are the binary states of visible unit j ∈ {1, 2, … m } and hidden unit i ∈ {1, 2, .. n }, b j , c i are their biases of visible and hidden units, w ij is the symmetric interaction term between the units v j and h i them. A joint probability of ( v , h ) is given by the Gibbs distribution in Eq. ( 4 )
Z is a “partition function” that can be given by summing over all possible pairs of visual v and hidden h (5).
A significant feature of the RBM model is that there is no direct contact between the two visible units or either of the two hidden units. In term of probability, conditional distributions p ( h | v ) and p ( v | h ) is computed as (6) \( p\left(h|v\right)={\prod}_{i=1}^np\left({h}_i|v\right) \)
For binary RBM condition distribution of visible and hidden are given by (7) and (8)
where σ( · ) is a sigmoid function
RBMs parameters ( w ij , b j , c i ) are efficiently calculated using the contrastive divergence learning method [ 150 ]. A batch version of k-step contrastive divergence learning (CD-k) can be discussed in the algorithm below [ 36 ]
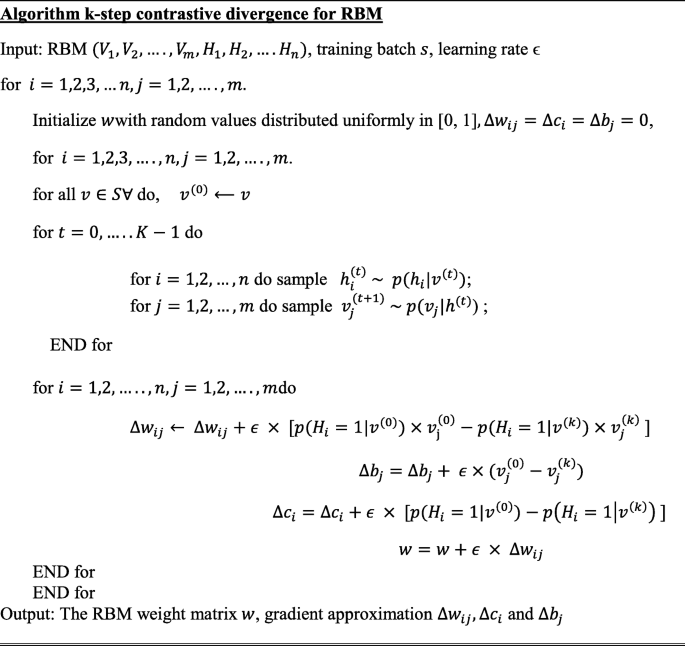
3.3 Deep belief networks
The Deep Belief Networks (DBN) proposed by Hinton et al. [ 51 ] is a non-convolution model that can extract features and learn a deep hierarchical representation of training data. DBNs are generative models constructed by stacking multiple RBMs. DBN is a hybrid model, the first two layers are like RBM, and the rest of the layers form a directed generative model. A DBN has one visible layer v and a series of hidden layers h (1) , h (2) , …, h ( l ) as shown in Fig. 4c . The DBN model joint distribution between the observed units v and the l hidden layers h k ( k = 1, … l ) as (9)
where v = h (0) , P ( h k | h k + 1 ) is a conditional distribution (10) for the layer k given the units of k + 1
A DBN has l weight matrices: W (1) , …. , W ( l ) and l + 1 bias vectors: b (0) , …, b ( l ) P ( h ( l ) , h ( l − 1) ) is the joint distribution of top-level RBM (11).
The probability distribution of DBN is given by Eq. ( 12 )
3.4 Convolutional neural networks (CNN)
In neural networks, CNN is a unique family of deep learning models. CNN is a major artificial visual network for the identification of medical image patterns. The family of CNN primarily emerges from the information of the animal visual cortex [ 55 , 116 ]. The major problem within a fully connected feed-forward neural network is that even for shallow architectures, the number of neurons may be very high, which makes them impractical to apply to image applications. The CNN is a method for reducing the number of parameters, allows a network to be deeper with fewer parameters.
CNN’s are designed based on three architectural ideas that are shared weights, local receptive fields, and spatial sub-sampling [ 70 ]. The essential element of CNN is the handling of unstructured data through the convolution operation. Convolution of the input signal x ( t ) with filter signal h ( t ) creates an output signal y ( t ) that may reveal more information than the input signal itself. 1D convolution of a discrete signals x ( t ) and h ( t ) is (13)
A digital image x ( n 1 , n 2 ) is a 2-D discrete signal. The convolution of images x ( n 1 , n 2 ) and h ( n 1 , n 2 ) is (14)
where 0 ≤ n 1 ≤ M − 1, 0 ≤ n 2 ≤ N − 1.
The function of the convolution layer is to detect local features x l from input feature maps x l − 1 using kernels k l by convolution operation (*) i.e. x l − 1 ∗ k l . This convolution operation is repeated for every convolutional layer subject to non-linear transform (15)
where \( {k}_{mn}^{(l)} \) represents weights between feature map m at layer l − 1 and feature map n at \( l.{x}_m^{\left(l-1\right)} \) represents the m feature map of the layer l − 1 and \( {x}_n^l \) is n feature map of the layer l . \( {b}_m^{(l)} \) is the bias parameter. f (.) is the non-linear activation function. M l − 1 denotes a set of feature maps. CNN significantly reduces the number of parameters compared with a fully connected neural network because of local connectivity and weight sharing. The depth, zero-padding, and stride are three hyperparameters for controlling the volume of the convolution layer output.
A pooling layer comes after the convolutional layer to subsample the feature maps. The goal of the pooling layers is to achieve spatial invariance by minimizing the spatial dimension of the feature maps for the next convolution layer. Max pooling and average pooling are commonly used two different polling operations to achieve downsampling. Let the size of the pooling region M and each element in the pooling region is given as x j = ( x 1 , x 2 , … x M × M ), the output after pooling is given as x i . Max pooling and average polling are described in the following Eqs. ( 16 ) and ( 17 ).
The max-pooling method chooses the most superior invariant feature in a pooling region. The average pooling method selects the average of all the features in the pooling area. Thus, the max-pooling method holds texture information that can lead to faster convergence, average pooling method is called Keep background information [ 133 ]. Spatial pyramid pooling [ 48 ], stochastic polling [ 175 ], Def-pooling [ 109 ], Multi activation pooling [ 189 ], and detailed preserving pooling [ 130 ] are different pooling techniques in the literature. A fully connected layer is used at the end of the CNN model. Fully connected layers perform like a traditional neural network [ 174 ]. The input to this layer is a vector of numbers (output of the pooling layer) and outputs an N-dimensional vector (N number of classes). After the pooling layers, the feature of previous layer maps is flattened and connected to fully connected layers.
The first successful seven-layered LeNet-5 CNN was developed by Yann LeCunn in 1990 for handwritten digit recognition successfully. Krizhevsky et al. [ 68 ] proposed AlexNet is a deep convolutional neural network composed of 5 convolutional and 3 fully-connected layers. In AlexNet changed the sigmoid activation function to a ReLU activation function to make model training easier.
K. Simonyan and A. Zisserman invented the VGG-16 [ 143 ] which has 13 convolutional and 3 fully connected layers. The Visual Geometric Group (VGG) research group released a series of CNN starting from VGG-11, VGG-13, VGG-16, and VGG-19. The main intention of the VGG group to understand how the depth of convolutional networks affects the accuracy of the models of image classification and recognition. Compared to the maximum VGG19, which has 16 convolutional layers and 3 fully connected layers, the minimum VGG11 has 8 convolutional layers and 3 fully connected layers. The last three fully connected layers are the same as the various variations of VGG.
Szegedy et al. [ 151 ] proposed an image classification network consisting of 22 different layers, which is GoogleNet. The main idea behind GoogleNet is the introduction of inception layers. Each inception layer convolves the input layers partially using different filter sizes. Kaiming He et al. [ 49 ] proposed the ResNet architecture, which has 33 convolutional layers and one fully-connected layer. Many models introduced the principle of using multiple hidden layers and extremely deep neural networks, but then it was realized that such models suffered from the issue of vanishing or exploding gradients problem. For eliminating vanishing gradients’ problem skip layers (shortcut connections) are introduced. DenseNet developed by Gao et al. [ 54 ] consists of several dense blocks and transition blocks, which are placed between two adjacent dense blocks. The dense block consists of three layers of batch normalization, followed by a ReLU and a 3 × 3 convolution operation. The transition blocks are made of Batch Normalization, 1 × 1 convolution, and average Pooling.
Compared to state-of-the-art handcrafted feature detectors, CNNs is an efficient technique for detecting features of an object and achieving good classification performance. There are drawbacks to CNNs, which are that unique relationships, size, perspective, and orientation of features are not taken into account. To overcome the loss of information in CNNs by pooling operation Capsule Networks (CapsNet) are used to obtain spatial information and most significant features [ 129 ]. The special type of neurons, called capsules, can detect efficiently distinct information. The capsule network consists of four main components that are matrix multiplication, Scalar weighting of the input, dynamic routing algorithm, and squashing function.
3.5 Recurrent neural networks (RNN)
RNN is a class of neural networks used for processing sequential information (deal with sequential data). The structure of the RNN shown in Fig. 5a is like an FFNN and the difference is that recurrent connections are introduced among hidden nodes. A generic RNN model at time t , the recurrent connection hidden unit h t receives input activation from the present data x t and the previous hidden state h t − 1 . The output y t is calculated given the hidden state h t . It can be represented using the mathematical Eqs. ( 18 ) and ( 19 ) as
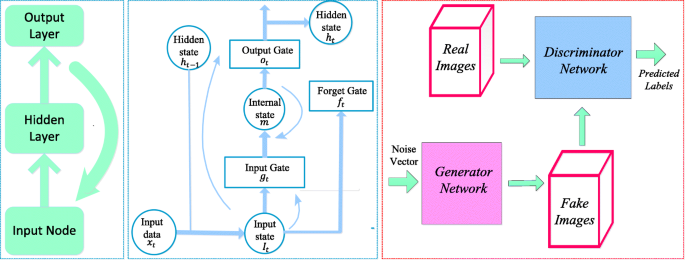
a Recurrent Neural Networks [ 163 ] b Long Short-Term Memory [ 163 ] c Generative Adversarial Networks [ 64 ]
Here f is a non-linear activation function, w hx is the weight matrix between the input and hidden layers, w hh is the matrix of recurrent weights between the hidden layers and itself w yh is the weight matrix between the hidden and output layer, and b h and b y are biases that allow each node to learn and offset. While the RNN is a simple and efficient model, in reality, it is, unfortunately, difficult to train properly. Real-Time Recurrent Learning (RTRL) algorithm [ 173 ] and Back Propagation Through Time (BPTT) [ 170 ] methods are used to train RNN. Training with these methods frequently fails because of vanishing (multiplication of many small values) or explode (multiplication of many large values) gradient problem [ 10 , 112 ]. Hochreiter and Schmidhuber (1997) designed a new RNN model named Long Short Term Memory (LSTM) that overcome error backflow problems with the aid of a specially designed memory cell [ 52 ]. Figure 5b shows an LSTM cell which is typically configured by three gates: input gate g t , forget gate f t and output gate o t , these gates add or remove information from the cell.
An LSTM can be represented with the following Eqs. ( 20 ) to ( 25 )
3.6 Generative adversarial networks (GAN)
In the field of deep learning, one of the deep generative models are Generative Adversarial Networks (GANs) introduced by Good Fellow in [ 43 ]. GANs are neural networks that can generate synthetic images that closely imitate the original images. In GAN shown in Fig. 5c , there are two neural networks, namely generator, and discriminator, which are trained simultaneously. The generator G generates counterfeit data samples which aim to “fool” the discriminator D , while the discriminator attempts to correctly distinguish the true and false samples. In mathematical terms, D and G play a two player minimax game with the cost function of (26) [ 64 ].
Where x represents the original image, z is a noise vector with random numbers. p data ( x ) and p z ( z ) are probability distributions of x and z , respectively. D ( x ) represents the probability that x comes from the actual data p data ( x ) rather than the generated data. 1 − D ( G (z)) is the probability that it can be generated from p z (z). The expectation of x from the real data distribution p data is expressed by \( {E}_{x\sim {p}_{data(x)}} \) and the expectation of z sampled from noise is \( {E}_{\mathrm{z}\sim {P}_{\mathrm{z}}\left(\mathrm{z}\right)}. \) The goal of the training is to maximize the loss function for the discriminator, while the training objective for the generator is to reduce the term log (1 − D ( G ( z ))).The most utilization of GAN in the field of medical image analysis is data augmentation (generating new data) and image to image translation [ 107 ]. Trustability of the Generated Data, Unstable Training, and evaluation of generated data are three major drawbacks of GAN that might hinder their acceptance in the medical community [ 183 ].
Ronneberger et al. [ 126 ] proposed CNN based U-Net architecture for segmentation in biomedical image data. The architecture consists of a contracting path (left side) to capture context and an expansive symmetric path (right side) that enables precise localization. U-Net is a generalized DLA used for quantification tasks such as cell detection and shape measurement in medical image data [ 34 ].
3.8 Software frameworks
There are several software frameworks available for implementing DLA which are regularly updated as new approaches and ideas are created. DLA encapsulates many levels of mathematical principles based on probability, linear algebra, calculus, and numerical computation. Several deep learning frameworks exist such as Theano, TensorFlow, Caffe, CNTK, Torch, Neon, pylearn, etc. [ 138 ]. Globally, Python is probably the most commonly used programming language for DL. PyTorch and Tensorflow are the most widely used libraries for research in 2019. Table 2 shows the analysis of various Deep Learning Frameworks based on the core language and supported interface language.
4 Use of deep learning in medical imaging
4.1 x-ray image.
Chest radiography is widely used in diagnosis to detect heart pathologies and lung diseases such as tuberculosis, atelectasis, consolidation, pleural effusion, pneumothorax, and hyper cardiac inflation. X-ray images are accessible, affordable, and less dose-effective compared to other imaging methods, and it is a powerful tool for mass screening [ 14 ]. Table 3 presents a description of the DL methods used for X-ray image analysis.
S. Hwang et al. [ 57 ] proposed the first deep CNN-based Tuberculosis screening system with a transfer learning technique. Rajaraman et al. [ 119 ] proposed modality-specific ensemble learning for the detection of abnormalities in chest X-rays (CXRs). These model predictions are combined using various ensemble techniques toward minimizing prediction variance. Class selective mapping of interest (CRM) is used for visualizing the abnormal regions in the CXR images. Loey et al. [ 90 ] proposed A GAN with deep transfer training for COVID-19 detection in CXR images. The GAN network was used to generate more CXR images due to the lack of the COVID-19 dataset. Waheed et al. [ 160 ] proposed a CovidGAN model based on the Auxiliary Classifier Generative Adversarial Network (ACGAN) to produce synthetic CXR images for COVID-19 detection. S. Rajaraman and S. Antani [ 120 ] introduced weakly labeled data augmentation for increasing training dataset to improve the COVID-19 detection performance in CXR images.
4.2 Computerized tomography (CT)
CT uses computers and rotary X-ray equipment to create cross-section images of the body. CT scans show the soft tissues, blood vessels, and bones in different parts of the body. CT is a high detection ability, reveals small lesions, and provides a more detailed assessment. CT examinations are frequently used for pulmonary nodule identification [ 93 ]. The detection of malignant pulmonary nodules is fundamental to the early diagnosis of lung cancer [ 102 , 142 ]. Table 4 summarizes the latest deep learning developments in the study of CT image analysis.
Li et al. 2016 [ 74 ] proposed deep CNN for the detection of three types of nodules that are semisolid, solid, and ground-glass opacity. Balagourouchetty et al. [ 5 ] proposed GoogLeNet based an ensemble FCNet classifier for The liver lesion classification. For feature extraction, basic Googlenet architecture is modified with three modifications. Masood et al. [ 95 ] proposed the multidimensional Region-based Fully Convolutional Network (mRFCN) for lung nodule detection/classification and achieved a classification accuracy of 97.91%. In lung nodule detection, the feature work is the detection of micronodules (less than 3 mm) without loss of sensitivity and accuracy. Zhao and Zeng 2019 [ 190 ] proposed DLA based on supervised MSS U-Net and 3DU-Net to automatically segment kidneys and kidney tumors from CT images. In the present pandemic situation, Fan et al. [ 35 ] and Li et al. [ 79 ] used deep learning-based techniques for COVID-19 detection from CT images.
4.3 Mammograph (MG)
Breast cancer is one of the world’s leading causes of death among women with cancer. MG is a reliable tool and the most common modality for early detection of breast cancer. MG is a low-dose x-ray imaging method used to visualize the breast structure for the detection of breast diseases [ 40 ]. Detection of breast cancer on mammography screening is a difficult task in image classification because the tumors constitute a small part of the actual breast image. For analyzing breast lesions from MG, three steps are involved that are detection, segmentation, and classification [ 139 ].
The automatic classification and detection of masses at an early stage in MG is still a hot subject of research. Over the past decade, DLA has shown some significant overcome in breast cancer detection and classification problem. Table 5 summarizes the latest DLA developments in the study of mammogram image analysis.
Fonseca et al. [ 37 ] proposed a breast composition classification according to the ACR standard based on CNN for feature extraction. Wang et al. [ 161 ] proposed twelve-layer CNN to detect Breast arterial calcifications (BACs) in mammograms image for risk assessment of coronary artery disease. Ribli et al. [ 124 ] developed a CAD system based on Faster R-CNN for detection and classification of benign and malignant lesions on a mammogram image without any human involvement. Wu et al. [ 176 ] present a deep CNN trained and evaluated on over 1,000,000 mammogram images for breast cancer screening exam classification. Conant et al. [ 26 ] developed a Deep CNN based AI system to detect calcified lesions and soft- tissue in digital breast tomosynthesis (DBT) images. Kang et al. [ 62 ] introduced Fuzzy completely connected layer (FFCL) architecture, which focused primarily on fused fuzzy rules with traditional CNN for semantic BI-RADS scoring. The proposed FFCL framework achieved superior results in BI-RADS scoring for both triple and multi-class classifications.
4.4 Histopathology
Histopathology is the field of study of human tissue in the sliding glass using a microscope to identify different diseases such as kidney cancer, lung cancer, breast cancer, and so on. The staining is used in histopathology for visualization and highlight a specific part of the tissue [ 45 ]. For example, Hematoxylin and Eosin (H&E) staining tissue gives a dark purple color to the nucleus and pink color to other structures. H&E stain plays a key role in the diagnosis of different pathologies, cancer diagnosis, and grading over the last century. The recent imaging modality is digital pathology
Deep learning is emerging as an effective method in the analysis of histopathology images, including nucleus detection, image classification, cell segmentation, tissue segmentation, etc. [ 178 ]. Tables 6 and 7 summarize the latest deep learning developments in pathology. In the study of digital pathology image analysis, the latest development is the introduction of whole slide imaging (WSI). WSI allows digitizing glass slides with stained tissue sections at high resolution. Dimitriou et al. [ 30 ] reviewed challenges for the analysis of multi-gigabyte WSI images for building deep learning models. A. Serag et al. [ 135 ] discuss different public “Grand Challenges” that have innovations using DLA in computational pathology.
4.5 Other images
Endoscopy is the insertion of a long nonsurgical solid tube directly into the body for the visual examination of an internal organ or tissue in detail. Endoscopy is beneficial in studying several systems inside the human body, such as the gastrointestinal tract, the respiratory tract, the urinary tract, and the female reproductive tract [ 60 , 101 ]. Du et al. [ 31 ] reviewed the Applications of Deep Learning in the Analysis of Gastrointestinal Endoscopy Images. A revolutionary device for direct, painless, and non-invasive inspection of the gastrointestinal (GI) tract for detecting and diagnosing GI diseases (ulcer, bleeding) is Wireless capsule endoscopy (WCE). Soffer et al. [ 145 ] performed a systematic analysis of the existing literature on the implementation of deep learning in the WCE. The first deep learning-based framework was proposed by He et al. [ 46 ] for the detection of hookworm in WCE images. Two CNN networks integrated (edge extraction and classification of hookworm) to detect hookworm. Since tubular structures are crucial elements for hookworm detection, the edge extraction network was used for tubular region detection. Yoon et al. [ 185 ] developed a CNN model for early gastric cancer (EGC) identification and prediction of invasion depth. The depth of tumor invasion in early gastric cancer (EGC) is a significant factor in deciding the method of treatment. For the classification of endoscopic images as EGC or non-EGC, the authors employed a VGG-16 model. Nakagawa et al. [ 105 ] applied DL technique based on CNN to enhance the diagnostic assessment of oesophageal wall invasion using endoscopy. J.choi et al. [ 22 ] express the feature aspects of DL in endoscopy.
Positron Emission Tomography (PET) is a nuclear imaging tool that is generally used by the injection of particular radioactive tracers to visualize molecular-level activities within tissues. T. Wang et al. [ 168 ] reviewed applications of machine learning in PET attenuation correction (PET AC) and low-count PET reconstruction. The authors discussed the advantages of deep learning over machine learning in the applications of PET images. AJ reader et al. [ 123 ] reviewed the reconstruction of PET images that can be used in deep learning either directly or as a part of traditional reconstruction methods.
5 Discussion
The primary purpose of this paper is to review numerous publications in the field of deep learning applications in medical images. Classification, detection, and segmentation are essential tasks in medical image processing [ 144 ]. For specific deep learning tasks in medical applications, the training of deep neural networks needs a lot of labeled data. But in the medical field, at least thousands of labeled data is not available. This issue is alleviated by a technique called transfer learning. Two transfer learning approaches are popular and widely applied that are fixed feature extractors and fine-tuning a pre-trained network. In the classification process, the deep learning models are used to classify images into two or more classes. In the detection process, Deep learning models have the function of identifying tumors and organs in medical images. In the segmentation task, deep learning models try to segment the region of interest in medical images for processing.
5.1 Segmentation
For medical image segmentation, deep learning has been widely used, and several articles have been published documenting the progress of deep learning in the area. Segmentation of breast tissue using deep learning alone has been successfully implemented [ 104 ]. Xing et al. [ 179 ] used CNN to acquire the initial shape of the nucleus and then isolate the actual nucleus using a deformable pattern. Qu et al. [ 118 ] suggested a deep learning approach that could segment the individual nucleus and classify it as a tumor, lymphocyte, and stroma nuclei. Pinckaers and Litjens [ 115 ] show on a colon gland segmentation dataset (GlaS) that these Neural Ordinary Differential Equations (NODE) can be used within the U-Net framework to get better segmentation results. Sun 2019 [ 149 ] developed a deep learning architecture for gastric cancer segmentation that shows the advantage of utilizing multi-scale modules and specific convolution operations together. Figure 6 shows U-Net is the most usually used network for segmentation (Fig. 6 ).
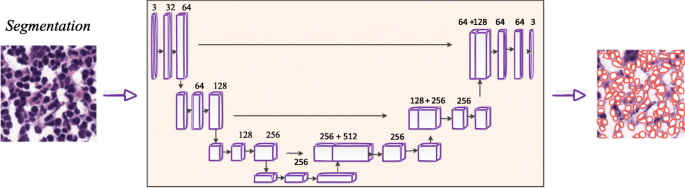
U-Net architecture for segmentation,comprising encoder (downsampling) and decoder (upsampling) sections [ 135 ]
5.2 Detection
The main challenge posed by methods of detection of lesions is that they can give rise to multiple false positives while lacking a good proportion of true positive ones . For tuberculosis detection using deep learning methods applied in [ 53 , 57 , 58 , 91 , 119 ]. Pulmonary nodule detection using deep learning has been successfully applied in [ 82 , 108 , 136 , 157 ].
Shin et al. [ 141 ] discussed the effect of CNN pre-trained architectures and transfer learning on the identification of enlarged thoracoabdominal lymph nodes and the diagnosis of interstitial lung disease on CT scans, and considered transfer learning to be helpful, given the fact that natural images vary from medical images. Litjens et al. [ 85 ] introduced CNN for the identification of Prostate cancer in biopsy specimens and breast cancer metastasis identification in sentinel lymph nodes. The CNN has four convolution layers for feature extraction and three classification layers. Riddle et al. [ 124 ] proposed the Faster R-CNN model for the detection of mammography lesions and classified these lesions into benign and malignant, which finished second in the Digital Mammography DREAM Challenge. Figure 7 shows VGG architecture for detection.
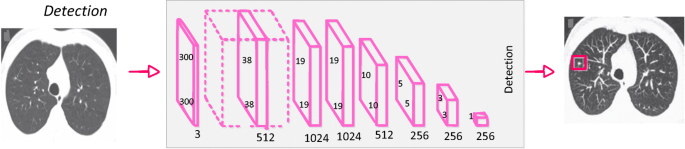
CNN architecture for detection [ 144 ]
An object detection framework named Clustering CNN (CLU-CNNs) was proposed by Z. Li et al. [ 76 ] for medical images. CLU-CNNs used Agglomerative Nesting Clustering Filtering (ANCF) and BN-IN Net to avoid much computation cost facing medical images. Image saliency detection aims at locating the most eye-catching regions in a given scene [ 21 , 78 ]. The goal of image saliency detection is to locate a given scene in the most eye-catching regions. In different applications, it also acts as a pre-processing tool including video saliency detection [ 17 , 18 ], object recognition, and object tracking [ 20 ]. Saliency maps are a commonly used tool for determining which areas are most important to the prediction of a trained CNN on the input image [ 92 ]. NT Arun et al. [ 4 ] evaluated the performance of several popular saliency methods on the RSNA Pneumonia Detection dataset and was found that GradCAM was sensitive to the model parameters and model architecture.
5.3 Classification
In classification tasks, deep learning techniques based on CNN have seen several advancements. The success of CNN in image classification has led researchers to investigate its usefulness as a diagnostic method for identifying and characterizing pulmonary nodules in CT images. The classification of lung nodules using deep learning [ 74 , 108 , 117 , 141 ] has also been successfully implemented.
Breast parenchymal density is an important indicator of the risk of breast cancer. The DL algorithms used for density assessment can significantly reduce the burden of the radiologist. Breast density classification using DL has been successfully implemented [ 37 , 59 , 72 , 177 ]. Ionescu et al. [ 59 ] introduced a CNN-based method to predict Visual Analog Score (VAS) for breast density estimation. Figure 8 shows AlexNet architecture for classification.
Alcoholism or alcohol use disorder (AUD) has effects on the brain. The structure of the brain was observed using the Neuroimaging approach. S.H.Wang et al. [ 162 ] proposed a 10-layer CNN for alcohol use disorder (AUD) problem using dropout, batch normalization, and PReLU techniques. The authors proposed a 10 layer CNN model that has obtained a sensitivity of 97.73, a specificity of 97.69, and an accuracy of 97.71. Cerebral micro-bleeding (CMB) are small chronic brain hemorrhages that can result in cognitive impairment, long-term disability, and neurologic dysfunction. Therefore, early-stage identification of CMBs for prompt treatment is essential. S. Wang et al. [ 164 ] proposed the transfer learning-based DenseNet to detect Cerebral micro-bleedings (CMBs). DenseNet based model attained an accuracy of 97.71% (Fig. 8 ).
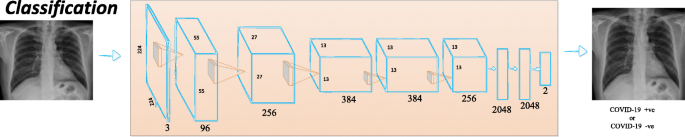
CNN architecture for classification [ 144 ]
5.4 Limitations and challenges
The application of deep learning algorithms to medical imaging is fascinating, but many challenges are pulling down the progress. One of the limitations to the adoption of DL in medical image analysis is the inconsistency in the data itself (resolution, contrast, signal-to-noise), typically caused by procedures in clinical practice [ 113 ]. The non-standardized acquisition of medical images is another limitation in medical image analysis. The need for comprehensive medical image annotations limits the applicability of deep learning in medical image analysis. The major challenge is limited data and compared to other datasets, the sharing of medical data is incredibly complicated. Medical data privacy is both a sociological and a technological issue that needs to be discussed from both viewpoints. For building DLA a large amount of annotated data is required. Annotating medical images is another major challenge. Labeling medical images require radiologists’ domain knowledge. Therefore, it is time-consuming to annotate adequate medical data. Semi-supervised learning could be implemented to make combined use of the existing labeled data and vast unlabelled data to alleviate the issue of “limited labeled data”. Another way to resolve the issue of “data scarcity” is to develop few-shot learning algorithms using a considerably smaller amount of data. Despite the successes of DL technology, there are many restrictions and obstacles in the medical field. Whether it is possible to reduce medical costs, increase medical efficiency, and improve the satisfaction of patients using DL in the medical field cannot be adequately checked. However, in clinical trials, it is necessary to demonstrate the efficacy of deep learning methods and to develop guidelines for the medical image analysis applications of deep learning.
6 Conclusion and future directions
Medical imaging is a place of origin of the information necessary for clinical decisions. This paper discusses the new algorithms and strategies in the area of deep learning. In this brief introduction to DLA in medical image analysis, there are two objectives. The first one is an introduction to the field of deep learning and the associated theory. The second is to provide a general overview of the medical image analysis using DLA. It began with the history of neural networks since 1940 and ended with breakthroughs in medical applications in recent DL algorithms. Several supervised and unsupervised DL algorithms are first discussed, including auto-encoders, recurrent, CNN, and restricted Boltzmann machines. Several optimization techniques and frameworks in this area include Caffe, TensorFlow, Theano, and PyTorch are discussed. After that, the most successful DL methods were reviewed in various medical image applications, including classification, detection, and segmentation. Applications of the RBM network is rarely published in the medical image analysis literature. In classification and detection, CNN-based models have achieved good results and are most commonly used. Several existing solutions to medical challenges are available. However, there are still several issues in medical image processing that need to be addressed with deep learning. Many of the current DL implementations are supervised algorithms, while deep learning is slowly moving to unsupervised and semi-supervised learning to manage real-world data without manual human labels.
DLA can support clinical decisions for next-generation radiologists. DLA can automate radiologist workflow and facilitate decision-making for inexperienced radiologists. DLA is intended to aid physicians by automatically identifying and classifying lesions to provide a more precise diagnosis. DLA can help physicians to minimize medical errors and increase medical efficiency in the processing of medical image analysis. DL-based automated diagnostic results using medical images for patient treatment are widely used in the next few decades. Therefore, physicians and scientists should seek the best ways to provide better care to the patient with the help of DLA. The potential future research for medical image analysis is the designing of deep neural network architectures using deep learning. The enhancement of the design of network structures has a direct impact on medical image analysis. Manual design of DL Model structure requires rich knowledge; hence Neural Network Search will probably replace the manual design [ 73 ]. A meaningful feature research direction is also the design of various activation functions. Radiation therapy is crucial for cancer treatment. Different medical imaging modalities are playing a critical role in treatment planning. Radiomics was defined as the extraction of high throughput features from medical images [ 28 ]. In the feature, Deep-learning analysis of radionics will be a promising tool in clinical research for clinical diagnosis, drug development, and treatment selection for cancer patients . Due to limited annotated medical data, unsupervised, weakly supervised, and reinforcement learning methods are the emerging research areas in DL for medical image analysis. Overall, deep learning, a new and fast-growing field, offers various obstacles as well as opportunities and solutions for a range of medical image applications.
Abadi M et al. (2016) TensorFlow: Large-Scale Machine Learning on Heterogeneous Distributed Systems, [Online]. Available: http://arxiv.org/abs/1603.04467 .
Abbas A, Abdelsamea MM, Gaber MM (2020) Classification of COVID-19 in chest X-ray images using DeTraC deep convolutional neural network, pp. 1–9, [Online]. Available: http://arxiv.org/abs/2003.13815 .
Apostolopoulos ID, Mpesiana TA (2020) Covid-19: automatic detection from X-ray images utilizing transfer learning with convolutional neural networks, Phys Eng Sci Med, no. 0123456789, pp. 1–6, DOI: https://doi.org/10.1007/s13246-020-00865-4 .
Arun NT et al. (2020) Assessing the validity of saliency maps for abnormality localization in medical imaging, pp. 1–5, [Online]. Available: http://arxiv.org/abs/2006.00063 .
L. Balagourouchetty, J. K. Pragatheeswaran, B. Pottakkat, and R. G, “GoogLeNet based ensemble FCNet classifier for focal liver lesion diagnosis,” IEEE J Biomed Heal Inf, vol. 2194, no. c, pp. 1–1, 2019, DOI: https://doi.org/10.1109/jbhi.2019.2942774 , 1694.
Bastien F et al. (2012) Theano: new features and speed improvements, pp. 1–10, [Online]. Available: http://arxiv.org/abs/1211.5590 .
Basu S, Mitra S, Saha N (2020) Deep Learning for Screening COVID-19 using Chest X-Ray Images, pp. 1–6, [Online]. Available: http://arxiv.org/abs/2004.10507 .
Bauer S, Wiest R, Nolte LP, Reyes M (2013) A survey of MRI-based medical image analysis for brain tumor studies. Phys Med Biol 58(13):1–44. https://doi.org/10.1088/0031-9155/58/13/R97
Article Google Scholar
Bengio Y, Lamblin P, Popovici D, Larochelle H (2006) Greedy layer-wise training of deep networks. In: The 19th International Conference on Neural Information Processing Systems(NIPS’06), pp 153–160. https://doi.org/10.5555/2976456.2976476
Chapter Google Scholar
Bengio Y, Simard P, Palo F (1994) Learning long -term dependencies with gradient descent is difficult. IEEE Trans Neural Netw 5(2):157–166
Bizopoulos P, Koutsouris D (2019) Deep learning in cardiology. IEEE Rev Biomed Eng 12(c):168–193. https://doi.org/10.1109/RBME.2018.2885714
Bulten W, Litjens G (2018) Unsupervised Prostate Cancer Detection on H&E using Convolutional Adversarial Autoencoders, [Online]. Available: http://arxiv.org/abs/1804.07098 .
Cai H et al. (2019) Breast Microcalcification Diagnosis Using Deep Convolutional Neural Network from Digital Mammograms, Comput Math Methods Med, vol. 2019, DOI: https://doi.org/10.1155/2019/2717454 .
Candemir S, Rajaraman S, Thoma G, Antani S (2018) Deep learning for grading cardiomegaly severity in chest x-rays : an investigation. In: 2018 IEEE Life Sciences Conference (LSC), pp 109–113. https://doi.org/10.1109/LSC.2018.8572113
Capizzi G, Lo Sciuto G, Napoli C, Połap D (2020) Small Lung Nodules Detection based on Fuzzy-Logic and Probabilistic Neural Network with Bio-inspired Reinforcement Learning, IEEE Trans Fuzzy Syst, vol. PP, no. XX, p. 1. https://doi.org/10.1109/TFUZZ.2019.2952831 .
Chen DS, Jain RC (1994) A robust back propagation learning algorithm for function approximation. IEEE Trans. Neural Networks 5(3):467–479. https://doi.org/10.1109/72.286917
Chen C, Li S, Qin H, Pan Z, Yang G (2018) Bilevel feature learning for video saliency detection. IEEE Trans Multimed 20(12):3324–3336. https://doi.org/10.1109/TMM.2018.2839523
Chen C, Li S, Wang Y, Qin H, Hao A (2017) Video saliency detection via spatial-temporal fusion and low-rank coherency diffusion. IEEE Trans Image Process 26(7):3156–3170. https://doi.org/10.1109/TIP.2017.2670143
Article MathSciNet MATH Google Scholar
Chen H, Qi X, Yu L, Dou Q, Qin J, Heng PA (2017) DCAN: deep contour-aware networks for object instance segmentation from histology images. Med Image Anal 36:135–146. https://doi.org/10.1016/j.media.2016.11.004
Chen C, Wang G, Peng C, Zhang X, Qin H (2020) Improved robust video saliency detection based on long-term spatial-temporal information. IEEE Trans Image Process 29:1090–1100. https://doi.org/10.1109/TIP.2019.2934350
Article MathSciNet Google Scholar
Chen C, Wei J, Peng C, Zhang W, Qin H (2020) Improved saliency detection in RGB-D images using two-phase depth estimation and selective deep fusion. IEEE Trans Image Process 29:4296–4307. https://doi.org/10.1109/TIP.2020.2968250
Choi J, Shin K, Jung J, Bae HJ, Kim DH, Byeon JS, Kim N (2020) Convolutional neural network technology in endoscopic imaging: artificial intelligence for endoscopy. Clin Endosc 53(2):117–126. https://doi.org/10.5946/ce.2020.054
Chougrad H, Zouaki H, Alheyane O (2018) Deep convolutional neural networks for breast cancer screening. Comput Methods Prog Biomed 157:19–30. https://doi.org/10.1016/j.cmpb.2018.01.011
Clevert DA, Unterthiner T, Hochreiter S (2016) Fast and accurate deep network learning by exponential linear units (ELUs). In: 4th International Conference on Learning Representations, ICLR 2016, pp 1–14
Google Scholar
Collobert R, Kavukcuoglu K, Farabet C (2011) Torch7: A matlab-like environment for machine learning, BigLearn, NIPS Work, pp. 1–6, [Online]. Available: http://infoscience.epfl.ch/record/192376/files/Collobert_NIPSWORKSHOP_2011.pdf .
Conant EF et al (2019) Improving Accuracy and Efficiency with Concurrent Use of Artificial Intelligence for Digital Breast Tomosynthesis. Radiol Artif Intell 1(4):e180096. https://doi.org/10.1148/ryai.2019180096
Coudray N, Ocampo PS, Sakellaropoulos T, Narula N, Snuderl M, Fenyö D, Moreira AL, Razavian N, Tsirigos A (2018) Classification and mutation prediction from non–small cell lung cancer histopathology images using deep learning. Nat Med 24(10):1559–1567. https://doi.org/10.1038/s41591-018-0177-5
Dercle L, Henry T, Carré A, Paragios N, Deutsch E, Robert C (2020) Reinventing radiation therapy with machine learning and imaging bio-markers (radiomics): State-of-the-art, challenges and perspectives, Methods, no. May, pp. 0–1, DOI: https://doi.org/10.1016/j.ymeth.2020.07.003 .
Dhillon A, Verma GK (2019) Convolutional neural network: a review of models, methodologies, and applications to object detection Prog Artif Intell, no. 0123456789, DOI: https://doi.org/10.1007/s13748-019-00203-0 .
Dimitriou N, Arandjelović O, Caie PD (2019) Deep Learning for Whole Slide Image Analysis: An Overview. Front Med 6(November):1–7. https://doi.org/10.3389/fmed.2019.00264
Du W et al (2019) Review on the applications of deep learning in the analysis of gastrointestinal endoscopy images. IEEE Access 7:142053–142069. https://doi.org/10.1109/ACCESS.2019.2944676
Dugas C, Bengio Y, Bélisle F, Nadeau C, Garcia R (2000) Incorporating second-order functional knowledge for better option pricing. In: 13th International Conference on Neural Information Processing Systems (NIPS’00), pp 451–457. https://doi.org/10.5555/3008751.3008817
Eberhart RC, Dobbins RW (1990) Early neural network development history: the age of Camelot. IEEE Eng Med Biol Mag 9(3):15–18. https://doi.org/10.1109/51.59207
Falk T, Mai D, Bensch R, Çiçek Ö, Abdulkadir A, Marrakchi Y, Böhm A, Deubner J, Jäckel Z, Seiwald K, Dovzhenko A, Tietz O, Dal Bosco C, Walsh S, Saltukoglu D, Tay TL, Prinz M, Palme K, Simons M, Diester I, Brox T, Ronneberger O (2019) U-net: deep learning for cell counting, detection, and morphometry. Nat Methods 16(1):67–70. https://doi.org/10.1038/s41592-018-0261-2
Fan D-P et al. (2020) Inf-Net: Automatic COVID-19 Lung Infection Segmentation from CT Scans, pp. 1–10, [Online]. Available: http://arxiv.org/abs/2004.14133 .
Fischer A, Igel C (2014) Training restricted Boltzmann machines: an introduction. Pattern Recogn 47(1):25–39. https://doi.org/10.1016/j.patcog.2013.05.025
Article MATH Google Scholar
Fonseca P et al (2015) Automatic breast density classification using a convolutional neural network architecture search procedure. Med Imaging 2015 Comput Diagnosis 9414(c):941428. https://doi.org/10.1117/12.2081576
Fukushima K (1980) Neocognitron: a self-organizing neural network model for a mechanism of pattern recognition unaffected by shift in position. Biol Cybern 36(4):193–202. https://doi.org/10.1007/BF00344251
Gadermayr M, Gupta L, Appel V, Boor P, Klinkhammer BM, Merhof D (2019) Generative adversarial networks for facilitating stain-independent supervised and unsupervised segmentation: a study on kidney histology. IEEE Trans Med Imaging 38(10):2293–2302. https://doi.org/10.1109/TMI.2019.2899364
Gardezi SJS, Elazab A, Lei B, Wang T (2019) Breast cancer detection and diagnosis using mammographic data: systematic review. J Med Internet Res 21(7):1–22. https://doi.org/10.2196/14464
Geras KJ et al. (2017) High-Resolution Breast Cancer Screening with Multi-View Deep Convolutional Neural Networks, pp. 1–9, [Online]. Available: http://arxiv.org/abs/1703.07047 .
Goodfellow I, Bengio Y, Courville A (2016) “Deep learning,” DOI: https://doi.org/10.1038/nmeth.3707
Goodfellow IJ et al (2014) Generative adversarial nets. Adv Neural Inf Process Syst 3(January):2672–2680
Greenspan H, Van Ginneken B, Summers RM (2016) Guest editorial deep learning in medical imaging: overview and future promise of an exciting new technique. IEEE Trans Med Imaging 35(5):1153–1159. https://doi.org/10.1109/TMI.2016.2553401
Gurcan MN, Boucheron LE, Can A, Madabhushi A, Rajpoot NM, Yener B (2009) Histopathological image analysis: a review. IEEE Rev Biomed Eng 2:147–171. https://doi.org/10.1109/RBME.2009.2034865
He JY, Wu X, Jiang YG, Peng Q, Jain R (2018) Hookworm detection in wireless capsule endoscopy images with deep learning. IEEE Trans Image Process 27(5):2379–2392. https://doi.org/10.1109/TIP.2018.2801119
He K, Zhang X, Ren S., Sun J (2015) Delving deep into rectifiers: Surpassing human-level performance on imagenet classification, Proc IEEE Int Conf Comput Vis, vol. 2015 Inter, pp 1026–1034, DOI: https://doi.org/10.1109/ICCV.2015.123 .
He K, Zhang X, Ren S, Sun J (2015) Spatial pyramid pooling in deep convolutional networks for visual recognition. IEEE Trans Pattern Anal Mach Intell 37(9):1904–1916
He K, Zhang X, Ren S, Sun J (2016) Deep residual learning for image recognition. Proc IEEE Comput Soc Conf Comput Vis Pattern Recognit 2016(Decem):770–778. https://doi.org/10.1109/CVPR.2016.90
Hinton G (2014) Boltzmann Machines, Encycl Mach Learn Data Min, no. 1, pp. 1–7, DOI: https://doi.org/10.1007/978-1-4899-7502-7_31-1 .
Hinton GE, Osindero S, Teh Y-W (2006) A fast learning algorithm for deep belief nets. Neural Comput 18:1527–1554. https://doi.org/10.1162/neco.2006.18.7.1527
Hochreiter S, Schmidhuber J (1997) Long short-term memory. Neural Comput 9(8):1735–1780. https://doi.org/10.1162/neco.1997.9.8.1735
Hooda R, Mittal A, Sofat S (2019) Automated TB classification using ensemble of deep architectures. Multimed Tools Appl 78(22):31515–31532. https://doi.org/10.1007/s11042-019-07984-5
Huang G, Liu Z, Van Der Maaten L, Weinberger KQ (2017) Densely connected convolutional networks. Proc - 30th IEEE Conf Comput Vis Pattern Recognition, CVPR 2017 2017(Janua):2261–2269. https://doi.org/10.1109/CVPR.2017.243
Hubel DH, Wiesel TN (1962) Receptive fields, binocular interaction and functional architecture in the cat’s visual cortex. J Physiol 160(1):106–154. https://doi.org/10.1113/jphysiol.1962.sp006837
Huynh BQ, Li H, Giger ML (2016) Digital mammographic tumor classification using transfer learning from deep convolutional neural networks. J Med Imaging 3(3):034501. https://doi.org/10.1117/1.jmi.3.3.034501
Hwang S, Kim H-E, Jeong J, Kim H-J (2016) A novel approach for tuberculosis screening based on deep convolutional neural networks. Med Imaging 2016 Comput Diagnosis 9785:97852W. https://doi.org/10.1117/12.2216198
Hwang EJ, Park S, Jin KN, Kim JI, Choi SY, Lee JH, Goo JM, Aum J, Yim JJ, Park CM, Deep Learning-Based Automatic Detection Algorithm Development and Evaluation Group, Kim DH, Woo W, Choi C, Hwang IP, Song YS, Lim L, Kim K, Wi JY, Oh SS, Kang MJ (2019) Development and validation of a deep learning–based automatic detection algorithm for active pulmonary tuberculosis on chest radiographs. Clin Infect Dis 69(5):739–747. https://doi.org/10.1093/cid/ciy967
Ionescu GV et al (2019) Prediction of reader estimates of mammographic density using convolutional neural networks. J Med Imaging 6(03):1. https://doi.org/10.1117/1.jmi.6.3.031405
Jani KK, Srivastava R (2019) A survey on medical image analysis in capsule endoscopy. Curr Med Imaging Rev 15(7):622–636. https://doi.org/10.2174/1573405614666181102152434
Jia Y et al. (2014) Caffe: Convolutional architecture for fast feature embedding,” MM 2014 – Proc 2014 ACM Conf Multimed , pp. 675–678, DOI: https://doi.org/10.1145/2647868.2654889 .
Kang C, Yu X, Wang SH, Guttery DS, Pandey HM, Tian Y, Zhang YD (2020) A heuristic neural network structure relying on fuzzy logic for images scoring. IEEE Trans Fuzzy Syst 6706(c):1–1. https://doi.org/10.1109/tfuzz.2020.2966163 45
S. Karthik, R. Srinivasa Perumal, and P. V. S. S. R. Chandra Mouli, “Breast cancer classification using deep neural networks,” Knowl Comput Its Appl Knowl Manip Process Tech Vol. 1, pp. 227–241, 2018, DOI: https://doi.org/10.1007/978-981-10-6680-1_12
Kazeminia S et al. (2020) GANs for Medical Image Analysis,” Artif Intell Med, p. 104262, DOI: https://doi.org/10.1016/j.jece.2020.104262 .
Kim EK, Kim HE, Han K, Kang BJ, Sohn YM, Woo OH, Lee CW (2018) Applying data-driven imaging biomarker in mammography for breast Cancer screening: preliminary study. Sci Rep 8(1):1–8. https://doi.org/10.1038/s41598-018-21215-1
Kingma DP, Welling M Auto-encoding variational bayes. In: 2nd International Conference on Learning, ICLR 2014, vol 2014, pp 1–14
Klambauer G, Unterthiner T, Mayr A, Hochreiter S (2017) Self-normalizing neural networks. Adv Neural Inf Process Syst 2017(Decem):972–981
Krizhevsky A, Sutskever I, Hinton GE (2012) ImageNet classification with deep convolutional neural networks. In: The 25th International Conference on Neural Information Processing Systems, pp 1097–1105. https://doi.org/10.1145/3065386
Kyono T, Gilbert FJ, van der Schaar M (2018) MAMMO: A Deep Learning Solution for Facilitating Radiologist-Machine Collaboration in Breast Cancer Diagnosis, pp. 1–18, [Online]. Available: http://arxiv.org/abs/1811.02661 .
LeCun Y, Bengio Y (1998) Convolutional networks for images, speech, and time-series. In: The handbook of brain theory and neural networks, pp 255–258
LeCun Y, Boser B, Denker JS, Henderson D, Howard RE, Hubbard W, Jackel LD (1989) Backpropagation applied to digit recognition. Neural Comput 1(4):541–551
Lehman CD, Yala A, Schuster T, Dontchos B, Bahl M, Swanson K, Barzilay R (2019) Mammographic breast density assessment using deep learning: clinical implementation. Radiology 290(1):52–58. https://doi.org/10.1148/radiol.2018180694
Lei T, Wang R, Wan Y, Du X, Meng H, Nandi AK (2020) Medical Image Segmentation Using Deep Learning: A survey, vol. 171, pp. 17–31, DOI: https://doi.org/10.1007/978-3-030-32606-7_2 .
Li W, Cao P, Zhao D, Wang J (2016) Pulmonary Nodule Classification with Deep Convolutional Neural Networks on Computed Tomography Images, Comput Math Methods Med, vol. 2016, DOI: https://doi.org/10.1155/2016/6215085 .
Li X, Chen H, Qi X, Dou Q, Fu CW, Heng PA (2018) H-DenseUNet: hybrid densely connected UNet for liver and tumor segmentation from CT volumes. IEEE Trans Med Imaging 37(12):2663–2674. https://doi.org/10.1109/TMI.2018.2845918
Li Z, Dong M, Wen S, Hu X, Zhou P, Zeng Z (2019) CLU-CNNs: Object detection for medical images. Neurocomputing 350(May):53–59. https://doi.org/10.1016/j.neucom.2019.04.028
Li Y, Huang C, Ding L, Li Z, Pan Y, Gao X (2019) Deep learning in bioinformatics: introduction, application, and perspective in the big data era. Methods 166:4–21. https://doi.org/10.1016/j.ymeth.2019.04.008
Li Y, Li S, Chen C, Hao A, Qin H (2020) A Plug-and-play Scheme to Adapt Image Saliency Deep Model for Video Data, IEEE Trans Circuits Syst Video Technol, no. Xx, pp. 1–1, DOI: https://doi.org/10.1109/tcsvt.2020.3023080 .
Li L, Qin L, Yin Y, Wang X et al (2019) Artificial Intelligence Distinguishes COVID-19 from Community Acquired Pneumonia on Chest CT. Radiology 2020:1–5. https://doi.org/10.1007/s10489-020-01714-3
Li C, Wang X, Liu W, Latecki LJ, Wang B, Huang J (2019) Weakly supervised mitosis detection in breast histopathology images using concentric loss. Med Image Anal 53:165–178. https://doi.org/10.1016/j.media.2019.01.013
Liang Q, Nan Y, Coppola G, Zou K, Sun W, Zhang D, Wang Y, Yu G (2019) Weakly supervised biomedical image segmentation by reiterative learning. IEEE J Biomed Heal Inf 23(3):1205–1214. https://doi.org/10.1109/JBHI.2018.2850040
Liao F, Liang M, Li Z, Hu X, Song S (2019) Evaluate the malignancy of pulmonary nodules using the 3-D deep leaky Noisy-OR network. IEEE Trans Neural Netw Learn Syst 30(11):3484–3495. https://doi.org/10.1109/TNNLS.2019.2892409
Lin H, Chen H, Graham S, Dou Q, Rajpoot N, Heng PA (2019) Fast ScanNet: fast and dense analysis of multi-Gigapixel whole-slide images for Cancer metastasis detection. IEEE Trans Med Imaging 38(8):1948–1958. https://doi.org/10.1109/TMI.2019.2891305
Litjens G, Kooi T, Bejnordi BE, Setio AAA, Ciompi F, Ghafoorian M, van der Laak JAWM, van Ginneken B, Sánchez CI (2017) A survey on deep learning in medical image analysis. Med Image Anal 42(1995):60–88. https://doi.org/10.1016/j.media.2017.07.005
Litjens G et al (2016) Deep learning as a tool for increased accuracy and efficiency of histopathological diagnosis. Sci Rep 6(January):1–11. https://doi.org/10.1038/srep26286
Little WA (1974) The existence of persistent states in the brain. Math Biosci 19(1–2):101–120. https://doi.org/10.1016/0025-5564(74)90031-5
Little WA, Shaw GL (1978) Analytic study of the memory storage capacity of a neural network. Math Biosci 39(3–4):281–290. https://doi.org/10.1016/0025-5564(78)90058-5
Liu W, Wang Z, Liu X, Zeng N, Liu Y, Alsaadi FE (2017) A survey of deep neural network architectures and their applications. Neurocomputing 234(November 2016):11–26. https://doi.org/10.1016/j.neucom.2016.12.038
Lo SLJLMFMCSMSC, Lo SCB, Lou SLA, Chien MV, Mun SK (1995) Artificial convolution neural network techniques and applications for lung nodule detection. IEEE Trans Med Imaging 14(4):711–718. https://doi.org/10.1109/42.476112
Loey M, Smarandache F, Khalifa NEM (2020) Within the lack of chest COVID-19 X-ray dataset: A novel detection model based on GAN and deep transfer learning, Symmetry (Basel)., vol. 12, no. 4, DOI: https://doi.org/10.3390/SYM12040651 .
Lopes UK, Valiati JF (2017) Pre-trained convolutional neural networks as feature extractors for tuberculosis detection. Comput Biol Med 89(August):135–143. https://doi.org/10.1016/j.compbiomed.2017.08.001
Ma G, Li S, Chen C, Hao A, Qin H (2020) Stage-wise salient object detection in 360 omnidirectional image via object-level Semantical saliency ranking. IEEE Trans Vis Comput Graph 26:3535–3545. https://doi.org/10.1109/tvcg.2020.3023636
Ma J, Song Y, Tian X, Hua Y, Zhang R, Wu J (2020) Survey on deep learning for pulmonary medical imaging. Front Med 14(4):450–469. https://doi.org/10.1007/s11684-019-0726-4
Maas AL, Hannun AY, Ng AY (2013) Rectifier nonlinearities improve neural network acoustic models. In: The 30th International Conference on Machine Learning, vol 30
Masood A, Sheng B, Yang P, Li P, Li H, Kim J, Feng DD (2020) Automated decision support system for lung Cancer detection and classification via enhanced RFCN with multilayer fusion RPN. IEEE Trans Ind Inf 3203(c):1–1. https://doi.org/10.1109/tii.2020.2972918 7801
Mazurowski MA, Buda M, Saha A, Bashir MR (2019) Deep learning in radiology: an overview of the concepts and a survey of the state of the art with a focus on MRI. J Magn Reson Imaging 49(4):939–954. https://doi.org/10.1002/jmri.26534
Mcculloch WS, Pitts W (1943) A logical calculus of the ideas immanent in nervous activity. Bull Math Biophys 5:115–133. https://doi.org/10.1007/BF02478259
Minsky M, Papert S (1969) Perceptrons: an introduction to computational geometry, vol 522. MIT Press, Cambridge MA, pp 20–522. https://doi.org/10.1016/S0019-9958(70)90409-2
Book MATH Google Scholar
Mittal A, Hooda R, Sofat S (2018) LF-SegNet : a fully convolutional encoder – decoder network for segmenting lung fields from chest, Wirel Pers Commun, DOI: https://doi.org/10.1007/s11277-018-5702-9
Morris RGM, Hebb DO (1949) The Organization of Behavior, Wiley: New York; 1949,” Brain Res Bull, vol. 50, no. 5–6, p. 437, DOI: https://doi.org/10.1016/S0361-9230(99)00182-3 .
Münzer B, Schoeffmann K, Böszörmenyi L (2018) Content-based processing and analysis of endoscopic images and videos: a survey. Multimed Tools Appl 77(1):1323–1362. https://doi.org/10.1007/s11042-016-4219-z
Murphy A, Skalski M, Gaillard F (2018) The utilisation of convolutional neural networks in detecting pulmonary nodules: a review. Br J Radiol 91(1090):1–6. https://doi.org/10.1259/bjr.20180028
Murphy K et al. (2019) Computer aided detection of tuberculosis on chest radiographs: An evaluation of the CAD4TB v6 system, pp. 1–11, [Online]. Available: http://arxiv.org/abs/1903.03349 .
Nair V, Hinton GE (2010) Rectified linear units improve restricted Boltzmann machines. Proc 27th Int Conf Mach Learn (ICML-10), 807–814 33(5):807–814
Nakagawa K, Ishihara R, Aoyama K, Ohmori M (2019) Classification for invasion depth of esophageal squamous cell carcinoma using a deep neural network compared with experienced endoscopists. Gastrointest Endosc 90(3):407–414. https://doi.org/10.1016/j.gie.2019.04.245
Ng A (2011) Sparse autoencoder. CS294A Lect. Notes 72:1–19
Nie D, Trullo R, Lian J, Wang L, Petitjean C, Ruan S, Wang Q, Shen D (2018) Medical image synthesis with deep convolutional adversarial networks. IEEE Trans Biomed Eng 65(12):2720–2730. https://doi.org/10.1109/TBME.2018.2814538
Onishi Y et al. (2019) Automated Pulmonary Nodule Classification in Computed Tomography Images Using a Deep Convolutional Neural Network Trained by Generative Adversarial Networks, Biomed Res Int, vol. 2019, DOI: https://doi.org/10.1155/2019/6051939 .
Ouyang W et al (2015) DeepID-Net: Deformable deep convolutional neural networks for object detection. Proc IEEE Comput Soc Conf Comput Vis Pattern Recognit 07–12(June):2403–2412. https://doi.org/10.1109/CVPR.2015.7298854
Ozturk T, Talo M, Yildirim EA, Baloglu UB, Yildirim O, Rajendra Acharya U (2020) Automated detection of COVID-19 cases using deep neural networks with X-ray images. Comput Biol Med 121(April):103792. https://doi.org/10.1016/j.compbiomed.2020.103792
Pang S, Zhang Y, Ding M, Wang X, Xie X (2020) A deep model for lung Cancer type identification by densely connected convolutional networks and adaptive boosting. IEEE Access 8:4799–4805. https://doi.org/10.1109/ACCESS.2019.2962862
Pascanu R, Mikolov T, Bengio Y (2013) On the difficulty of training recurrent neural networks. 30th Int Conf Mach Learn ICML 2013(PART 3):2347–2355
Perone CS, Cohen-Adad J (2019) Promises and limitations of deep learning for medical image segmentation. J Med Artif Intell 2:1–1. https://doi.org/10.21037/jmai.2019.01.01
Pezeshk A, Hamidian S, Petrick N, Sahiner B (2018) 3D convolutional neural networks for automatic detection of pulmonary nodules in chest CT. IEEE J Biomed Heal Inf PP(c):1. https://doi.org/10.1109/JBHI.2018.2879449
Pinckaers H, Litjens G (2019) Neural Ordinary Differential Equations for Semantic Segmentation of Individual Colon Glands, no. NeurIPS, [Online]. Available: http://arxiv.org/abs/1910.10470 .
Poggio T, Serre T (2013) Models of visual cortex. Scholarpedia 8(4):3516. https://doi.org/10.4249/scholarpedia.3516
Qiang Y, Ge L, Zhao X, Zhang X, Tang X (2017) Pulmonary nodule diagnosis using dual-modal supervised autoencoder based on extreme learning machine. Expert Syst 34(6):1–12. https://doi.org/10.1111/exsy.12224
Qu H et al (2019) Joint Segmentation and fine -grained classification of nuclei in histopathology images. In: 2019 IEEE 16th International Symposium on Biomedical Imaging (ISBI 2019), pp 900–904. https://doi.org/10.1109/ISBI.2019.8759457
Rajaraman S, Antani SK (2020) Modality-specific deep learning model ensembles toward improving TB detection in chest radiographs. IEEE Access 8:27318–27326. https://doi.org/10.1109/ACCESS.2020.2971257
Rajaraman S, Antani S (2020) Weakly labeled data augmentation for deep learning: a study on COVID-19 detection in chest X-rays. Diagnostics 10(6):1–17. https://doi.org/10.3390/diagnostics10060358
Rajpurkar P, Irvin J, Ball RL, Zhu K, Yang B, Mehta H, Duan T, Ding D, Bagul A, Langlotz CP, Patel BN, Yeom KW, Shpanskaya K, Blankenberg FG, Seekins J, Amrhein TJ, Mong DA, Halabi SS, Zucker EJ, Ng AY, Lungren MP (2018) Deep learning for chest radiograph diagnosis: a retrospective comparison of the CheXNeXt algorithm to practicing radiologists. PLoS Med 15(11):1–17. https://doi.org/10.1371/journal.pmed.1002686
Rajpurkar P et al. (2017) CheXNet: Radiologist-Level Pneumonia Detection on Chest X-Rays with Deep Learning, pp. 3–9, [Online]. Available: http://arxiv.org/abs/1711.05225 .
Reader AJ, Corda G, Mehranian A, da Costa-Luis C, Ellis S, Schnabel JA (2020) Deep learning for PET image reconstruction. IEEE Trans Radiat Plasma Med Sci 7311(1):1–1. https://doi.org/10.1109/trpms.2020.3014786 25
Ribli D, Horváth A, Unger Z, Pollner P, Csabai I (2018) Detecting and classifying lesions in mammograms with deep learning. Sci Rep 8(1):1–7. https://doi.org/10.1038/s41598-018-22437-z
Rodríguez-Ruiz A, Krupinski E, Mordang JJ, Schilling K, Heywang-Köbrunner SH, Sechopoulos I, Mann RM (2019) Detection of breast cancer with mammography: effect of an artificial intelligence support system. Radiology 290(3):1–10. https://doi.org/10.1148/radiol.2018181371
Ronneberger O, Fischer P, Brox T (2015) U-net: Convolutional networks for biomedical image segmentation. Lect Notes Comput Sci (including Subser Lect. Notes Artif Intell Lect Notes Bioinformatics) 9351:234–241. https://doi.org/10.1007/978-3-319-24574-4_28
Rosenblatt F (1958) The perceptron: a probabilistic model for information storage and organization in the brain. Psychol Rev 65(6):386–408. https://doi.org/10.1037/h0042519
Rumelhart DE, Hinton GE, Williams RJ (1986) Learning representations by back-propagating errors. Nature 323(9):533–536
Sabour S, Frosst N, Hinton GE (2017) Dynamic routing between capsules. Adv Neural Inf Process Syst 2017-Decem(Nips):3857–3867
Saeedan F, Weber N, Goesele M, Roth S (2018) Detail-Preserving Pooling in Deep Networks,” Proc IEEE Comput Soc Conf Comput Vis Pattern Recognit , no. June, pp. 9108–9116, DOI: https://doi.org/10.1109/CVPR.2018.00949 .
Sahiner B, Heang-Ping Chan, Petrick N, Datong Wei, Helvie MA, Adler DD, Goodsitt MM (1996) Classification of mass and normal breast tissue: a convolution neural network classifier with spatial domain and texture images. IEEE Trans Med Imaging 15(5):598–610. https://doi.org/10.1109/42.538937
Sari CT, Gunduz-Demir C (2019) Unsupervised feature extraction via deep learning for Histopathological classification of Colon tissue images. IEEE Trans Med Imaging 38(5):1139–1149. https://doi.org/10.1109/TMI.2018.2879369
Scherer D, Müller A, Behnke S (2010) Evaluation of pooling operations in convolutional architectures for object recognition. Lect Notes Comput Sci (including Subser Lect Notes Artif Intell Lect Notes Bioinformatics) 6354 LNCS(PART 3):92–101. https://doi.org/10.1007/978-3-642-15825-4_10
Schmidhuber J (2015) Deep learning in neural networks: an overview. Neural Netw 61:85–117. https://doi.org/10.1016/j.neunet.2014.09.003
Serag A et al (2019) Translational AI and Deep Learning in Diagnostic Pathology. Front Med 6(October):1–15. https://doi.org/10.3389/fmed.2019.00185
Setio AAA, Ciompi F, Litjens G, Gerke P, Jacobs C, van Riel SJ, Wille MMW, Naqibullah M, Sanchez CI, van Ginneken B (2016) Pulmonary nodule detection in CT images: false positive reduction using multi-view convolutional networks. IEEE Trans Med Imaging 35(5):1160–1169. https://doi.org/10.1109/TMI.2016.2536809
Shah A, Kadam E, Shah H, Shinde S, Shingade S (2016) Deep residual networks with exponential linear unit. ACM Int Conf Proceeding Ser 21–24(Sept):59–65. https://doi.org/10.1145/2983402.2983406
Shatnawi A, Al-Bdour G, Al-Qurran R, Al-Ayyoub M (2018) A comparative study of open source deep learning frameworks. 2018 9th Int Conf Inf Commun Syst ICICS 2018 2018-Janua:72–77. https://doi.org/10.1109/IACS.2018.8355444
Shen L, Margolies LR, Rothstein JH, Fluder E, McBride R, Sieh W (2019) Deep learning to improve breast Cancer detection on screening mammography. Sci Rep 9(1):1–13. https://doi.org/10.1038/s41598-019-48995-4
Shickel B, Tighe PJ, Bihorac A, Rashidi P (2017) Deep EHR : A Survey of Recent Advances in Deep Learning Techniques for Electronic Health Record, vol. 2194, no. c, pp. 1–17, DOI: https://doi.org/10.1109/JBHI.2017.2767063 .
Shin HC, Roth HR, Gao M, Lu L, Xu Z, Nogues I, Yao J, Mollura D, Summers RM (2016) Deep convolutional neural networks for computer-aided detection: CNN architectures, dataset characteristics and transfer learning. IEEE Trans Med Imaging 35(5):1285–1298. https://doi.org/10.1109/TMI.2016.2528162
Siegel RL, Miller KD, Jemal A (2019) Cancer statistics, 2019. CA Cancer J Clin 69(1):7–34. https://doi.org/10.3322/caac.21551
Simonyan K, Zisserman (2015) A Very deep convolutional networks for large-scale image recognition. In: 3rd International Conference on Learning Representations, ICLR 2015, pp 1–14
Soffer S, Ben-Cohen A, Shimon O, Amitai MM, Greenspan H, Klang E (2019) Convolutional neural networks for radiologic images: a Radiologist’s guide. Radiology 290(3):590–606. https://doi.org/10.1148/radiol.2018180547
Soffer S, Klang E, Shimon O, Nachmias N, Eliakim R (2020) Deep learning for wireless capsule endoscopy : a systematic review and meta-analysis. Gastrointest Endosc 92(4):831–839.e8. https://doi.org/10.1016/j.gie.2020.04.039
Song TH, Sanchez V, Eidaly H, Rajpoot NM (2019) Simultaneous cell detection and classification in bone marrow histology images. IEEE J Biomed Heal Inf 23(4):1469–1476. https://doi.org/10.1109/JBHI.2018.2878945
Song Y, Tan EL, Jiang X, Cheng JZ, Ni D, Chen S, Lei B, Wang T (2017) Accurate cervical cell segmentation from overlapping clumps in pap smear images. IEEE Trans Med Imaging 36(1):288–300. https://doi.org/10.1109/TMI.2016.2606380
Souza JC, Bandeira Diniz JO, Ferreira JL, França da Silva GL, Corrêa Silva A, de Paiva AC (2019) An automatic method for lung segmentation and reconstruction in chest X-ray using deep neural networks. Comput Methods Prog Biomed 177:285–296. https://doi.org/10.1016/j.cmpb.2019.06.005
Sun M, Zhang G, Dang H, Qi X, Zhou X, Chang Q (2019) Accurate gastric Cancer segmentation in digital pathology images using deformable convolution and multi-scale embedding networks. IEEE Access 7:75530–75541. https://doi.org/10.1109/ACCESS.2019.2918800
Swersky K, Chen B, Marlin B, de Freitas N (2010) A tutorial on stochastic approximation algorithms for training restricted Boltzmann machines and deep belief nets,” 2010 Inf Theory Appl Work ITA 2010, Conf Proc, pp. 80–89, DOI: https://doi.org/10.1109/ITA.2010.5454138 .
Szegedy C, Reed S, Sermanet P, Vanhoucke V, Rabinovich A (2015) Going deeper with convolutions. In: The IEEE conference on Computer Vision and Pattern Recognition (CVPR), pp 1–9. https://doi.org/10.1109/CVPR.2015.7298594
Tabibu S, Vinod PK, Jawahar CV (2019) Pan-renal cell carcinoma classification and survival prediction from histopathology images using deep learning. Sci Rep 9(1):1–9. https://doi.org/10.1038/s41598-019-46718-3
The Theano Development Team et al. (2016) Theano: A Python framework for fast computation of mathematical expressions, pp. 1–19, [Online]. Available: http://arxiv.org/abs/1605.02688 .
Valkonen M, Isola J, Ylinen O, Muhonen V, Saxlin A, Tolonen T, Nykter M, Ruusuvuori P (2020) Cytokeratin-supervised deep learning for automatic recognition of epithelial cells in breast cancers stained for ER, PR, and Ki-67. IEEE Trans Med Imaging 39(2):534–542. https://doi.org/10.1109/TMI.2019.2933656
Valliani AAA, Ranti D, Oermann EK (2019) Deep learning and neurology: a systematic review. Neurol Ther 8(2):351–365. https://doi.org/10.1007/s40120-019-00153-8
Van Eycke YR, Balsat C, Verset L, Debeir O, Salmon I, Decaestecker C (2018) Segmentation of glandular epithelium in colorectal tumours to automatically compartmentalise IHC biomarker quantification: a deep learning approach. Med Image Anal 49:35–45. https://doi.org/10.1016/j.media.2018.07.004
van Ginneken B, Setio AAA, Jacobs C, Ciompi F (2015) Off-the-shelf convolutional neural network features for pulmonary nodule detection in computed tomography scans. In: 2015 IEEE 12th International Symposium on Biomedical Imaging (ISBI), pp 286–289. https://doi.org/10.1109/ISBI.2015.7163869
Vedaldi A, Lenc K (2015) MatConvNet: Convolutional neural networks for MATLAB, MM 2015 – Proc 2015 ACM Multimed Conf, pp. 689–692, DOI: https://doi.org/10.1145/2733373.2807412 .
Vincent P, Larochelle H, Lajoie I, Bengio Y, Manzagol PA (2010) Stacked denoising autoencoders: learning useful representations in a deep network with a local Denoising criterion. J Mach Learn Res 11:3371–3408
MathSciNet MATH Google Scholar
Waheed A, Goyal M, Gupta D, Khanna A, Al-Turjman F, Pinheiro PR (2020) CovidGAN: data augmentation using auxiliary classifier GAN for improved Covid-19 detection. IEEE Access 8:91916–91923. https://doi.org/10.1109/ACCESS.2020.2994762
Wang J, Ding H, Bidgoli FA, Zhou B, Iribarren C, Molloi S, Baldi P (2017) Detecting cardiovascular disease from mammograms with deep learning. IEEE Trans Med Imaging 36(5):1172–1181. https://doi.org/10.1109/TMI.2017.2655486
Wang SH, Muhammad K, Hong J, Sangaiah AK, Zhang YD (2020) Alcoholism identification via convolutional neural network based on parametric ReLU, dropout, and batch normalization. Neural Comput & Applic 32(3):665–680. https://doi.org/10.1007/s00521-018-3924-0
Wang H, Raj B (2017) On the Origin of Deep Learning,” pp. 1–72, [Online]. Available: http://arxiv.org/abs/1702.07800 .
Wang S, Tang C, Sun J, Zhang Y (2019) Cerebral micro-bleeding detection based on densely connected neural network. Front Neurosci 13(MAY):1–11. https://doi.org/10.3389/fnins.2019.00422
Wang L, Wong A (2020) COVID-Net: A Tailored Deep Convolutional Neural Network Design for Detection of COVID-19 Cases from Chest X-Ray Images, pp. 1–12, [Online]. Available: http://arxiv.org/abs/2003.09871 .
Wang Y, Yan F, Lu X, Zheng G, Zhang X, Wang C, Zhou K, Zhang Y, Li H, Zhao Q, Zhu H, Chen F, Gao C, Qing Z, Ye J, Li A, Xin X, Li D, Wang H, Yu H, Cao L, Zhao C, Deng R, Tan L, Chen Y, Yuan L, Zhou Z, Yang W, Shao M, Dou X, Zhou N, Zhou F, Zhu Y, Lu G, Zhang B (2019) IILS: intelligent imaging layout system for automatic imaging report standardization and intra-interdisciplinary clinical workflow optimization. EBioMedicine 44:162–181. https://doi.org/10.1016/j.ebiom.2019.05.040
Wang X et al (2019) Weakly Supervised Deep Learning for Whole Slide Lung Cancer Image Analysis. IEEE Trans Cybern PP:1–13. https://doi.org/10.1109/tcyb.2019.2935141
Wang T et al (2020) Machine learning in quantitative PET: A review of attenuation correction and low-count image reconstruction methods. Phys Medica 76(March):294–306. https://doi.org/10.1016/j.ejmp.2020.07.028
Wei JW, Tafe LJ, Linnik YA, Vaickus LJ, Tomita N, Hassanpour S (2019) Pathologist-level classification of histologic patterns on resected lung adenocarcinoma slides with deep neural networks. Sci Rep 9(1):1–8. https://doi.org/10.1038/s41598-019-40041-7
Werbos PJ (1990) Backpropagation through time: what it does and how to do it. Proc IEEE 78(10):1550–1560. https://doi.org/10.1109/5.58337
Werbose J (1974) Beyond regression: new tools for prediction and analysis in the behavioral
Widrow B, Hoff ME (1962) Associative Storage and Retrieval of Digital Information in Networks of Adaptive ‘Neurons. Biol Prototypes Synth Syst:160–160. https://doi.org/10.1007/978-1-4684-1716-6_25
Williams RJ, David Z (1995) Gradient-based learning algorithms for recurrent networks and their computational complexity. In: Back-propagation: theory, architectures and applications. L. Erlbaum Associates Inc, pp 433–486
Wu J (2017) Convolutional Neural Networks. Med Imaging Inf Sci 34(2):109–111. https://doi.org/10.11318/mii.34.109
Wu H, Gu X (2015) Max-pooling dropout for regularization of convolutional neural networks. Lect Notes Comput Sci (including Subser Lect Notes Artif Intell Lect Notes Bioinformatics) 9489:46–54. https://doi.org/10.1007/978-3-319-26532-2_6
Wu N, Phang J, Park J, Shen Y, Huang Z, Zorin M, Jastrzebski S, Fevry T, Katsnelson J, Kim E, Wolfson S, Parikh U, Gaddam S, Lin LLY, Ho K, Weinstein JD, Reig B, Gao Y, Toth H, Pysarenko K, Lewin A, Lee J, Airola K, Mema E, Chung S, Hwang E, Samreen N, Kim SG, Heacock L, Moy L, Cho K, Geras KJ (2019) Deep neural networks improve radiologists’ performance in breast Cancer screening. IEEE Trans Med Imaging 39:1–1. https://doi.org/10.1109/tmi.2019.2945514 1194
Wu N et al (2018) Breast density classification with deep convolutional neural networks. ICASSP, IEEE Int Conf Acoust Speech Signal Process - Proc 2018-April:6682–6686. https://doi.org/10.1109/ICASSP.2018.8462671
Xing F, Xie Y, Su H, Liu F, Yang L (2018) Deep learning in microscopy image analysis: a survey. IEEE Trans Neural Netw Learn Syst 29(10):4550–4568. https://doi.org/10.1109/TNNLS.2017.2766168
Xing F, Xie Y, Yang L (2016) An automatic learning-based framework for robust nucleus segmentation. IEEE Trans Med Imaging 35(2):550–566. https://doi.org/10.1109/TMI.2015.2481436
Xu B, Wang N, Chen T, Li M (2015) Empirical Evaluation of Rectified Activations in Convolutional Network , [Online]. Available: http://arxiv.org/abs/1505.00853 .
Xu S, Wu H, Bie R (2019) CXNet-m1: anomaly detection on chest X-rays with image-based deep learning. IEEE Access 7(c):4466–4477. https://doi.org/10.1109/ACCESS.2018.2885997
Xu J, Xiang L, Liu Q, Gilmore H, Wu J, Tang J, Madabhushi A (2016) Stacked sparse autoencoder (SSAE) for nuclei detection on breast cancer histopathology images. IEEE Trans Med Imaging 35(1):119–130. https://doi.org/10.1109/TMI.2015.2458702
Yi X, Walia E, Babyn P (2019) Generative adversarial network in medical imaging: A review,” Med Image Anal, vol. 58, DOI: https://doi.org/10.1016/j.media.2019.101552 .
Yi F, Yang L, Wang S, Guo L, Huang C, Xie Y, Xiao G (2018) Microvessel prediction in H&E Stained Pathology Images using fully convolutional neural networks. BMC Bioinform 19(1):1–9. https://doi.org/10.1186/s12859-018-2055-z
Yoon HJ et al (2019) A Lesion-Based Convolutional Neural Network Improves Endoscopic Detection and Depth Prediction of Early Gastric Cancer. J Clin Med 8(9):1310. https://doi.org/10.3390/jcm8091310
Yu D et al (2014) An Introduction to Computational Networks and the Computational Network Toolkit. INTERSPEECH, Microsoft Research
Zhang S, Zhang S, Wang B, Habetler TG (2020) Deep learning algorithms for bearing fault diagnostics - a comprehensive review. IEEE Access 8:29857–29881. https://doi.org/10.1109/ACCESS.2020.2972859
Zhang X et al (2017) Whole mammogram image classification with convolutional neural networks. Proc - 2017 IEEE Int Conf Bioinforma Biomed BIBM 2017 2017-Janua(Cc):700–704. https://doi.org/10.1109/BIBM.2017.8217738
Zhao Q, Lyu S, Zhang B, Feng W (2018) Multiactivation pooling method in convolutional neural networks for image recognition. Wirel Commun Mob Comput 2018:1–16. https://doi.org/10.1155/2018/8196906
Zhao W, Zeng Z (2019) Multi Scale Supervised 3D U-Net for Kidney and Tumor Segmentation,, DOI: https://doi.org/10.24926/548719.007 .
Download references
Author information
Authors and affiliations.
Department of Computer Science, School of Engineering and Technology, Pondicherry University, Pondicherry, India
Muralikrishna Puttagunta & S. Ravi
You can also search for this author in PubMed Google Scholar
Corresponding author
Correspondence to S. Ravi .
Additional information
Publisher’s note.
Springer Nature remains neutral with regard to jurisdictional claims in published maps and institutional affiliations.
Rights and permissions
Reprints and permissions
About this article
Puttagunta, M., Ravi, S. Medical image analysis based on deep learning approach. Multimed Tools Appl 80 , 24365–24398 (2021). https://doi.org/10.1007/s11042-021-10707-4
Download citation
Received : 25 August 2020
Revised : 28 November 2020
Accepted : 10 February 2021
Published : 06 April 2021
Issue Date : July 2021
DOI : https://doi.org/10.1007/s11042-021-10707-4
Share this article
Anyone you share the following link with will be able to read this content:
Sorry, a shareable link is not currently available for this article.
Provided by the Springer Nature SharedIt content-sharing initiative
- Deep learning
- Convolutional neural networks
- Medical images
- Segmentation
- Classification
- Find a journal
- Publish with us
- Track your research
Thank you for visiting nature.com. You are using a browser version with limited support for CSS. To obtain the best experience, we recommend you use a more up to date browser (or turn off compatibility mode in Internet Explorer). In the meantime, to ensure continued support, we are displaying the site without styles and JavaScript.
- View all journals
Medical imaging articles from across Nature Portfolio
Medical imaging comprises different imaging modalities and processes to image human body for diagnostic and treatment purposes. It is also used to follow the course of a disease already diagnosed and/or treated.
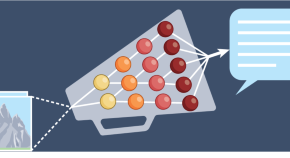
Adapting vision–language AI models to cardiology tasks
Vision–language models can be trained to read cardiac ultrasound images with implications for improving clinical workflows, but additional development and validation will be required before such models can replace humans.
- Rima Arnaout
Related Subjects
- Bone imaging
- Brain imaging
- Magnetic resonance imaging
- Molecular imaging
- Radiography
- Radionuclide imaging
- Three-dimensional imaging
- Ultrasonography
- Whole body imaging
Latest Research and Reviews
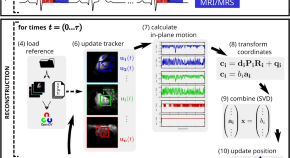
A modular motion compensation pipeline for prospective respiratory motion correction of multi-nuclear MR spectroscopy
- Stefan Wampl
- Tito Körner
- Albrecht Ingo Schmid
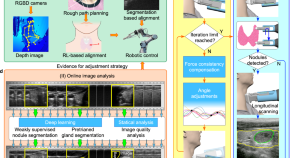
A fully autonomous robotic ultrasound system for thyroid scanning
Current thyroid ultrasounds rely heavily on the experience and skills of the sonographer and of the radiologist, and the process is physically and cognitively exhausting. Here, the authors show a fully autonomous robotic ultrasound system, which is able to scan thyroid regions without human assistance and identify malignant nodules.
- Jingwei Liu
- Peter Xiaoping Liu
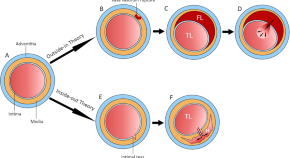
Spontaneous coronary artery dissection: a clinically oriented narrative review
- Sonya Burgess
- Sarah Zaman
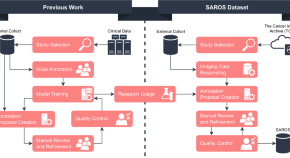
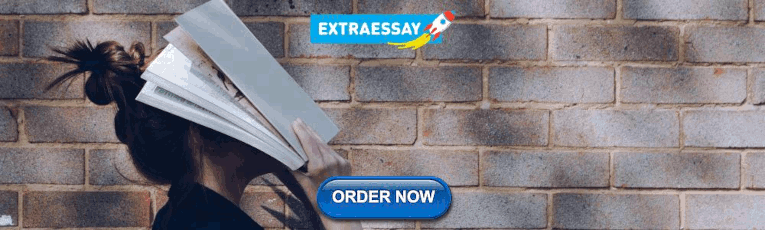
SAROS: A dataset for whole-body region and organ segmentation in CT imaging
- Sven Koitka
- Giulia Baldini
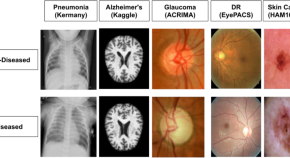
A comparative study of an on premise AutoML solution for medical image classification
- Kabilan Elangovan
- Gilbert Lim
- Daniel Ting
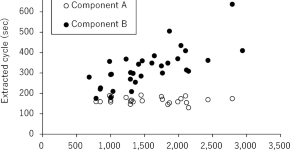
Continuous monitoring using thermography can capture the heat oscillations maintaining body temperature in neonates
- Aya Morimoto
- Shinji Nakamura
- Takashi Kusaka
News and Comment
Artificial intelligence chatbot interpretation of ophthalmic multimodal imaging cases.
- Andrew Mihalache
- Ryan S. Huang
- Rajeev H. Muni
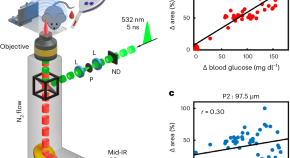
Blood glucose concentration measurement without finger pricking
A new sensor that detects optoacoustic signals generated by mid-infrared light enables measurement of glucose concentration from intracutaneous tissue rich in blood. This technology does not rely on glucose measurements in interstitial fluid or blood sampling and might yield the next generation of non-invasive glucose-sensing devices for improved diabetes management.
Enhancing diagnostic precision in liver lesion analysis using a deep learning-based system: opportunities and challenges
A recent study reported the development and validation of the Liver Artificial Intelligence Diagnosis System (LiAIDS), a fully automated system that integrates deep learning for the diagnosis of liver lesions on the basis of contrast-enhanced CT scans and clinical information. This tool improved diagnostic precision, surpassed the accuracy of junior radiologists (and equalled that of senior radiologists) and streamlined patient triage. These advances underscore the potential of artificial intelligence to enhance hepatology care, although challenges to widespread clinical implementation remain.
- Jeong Min Lee
- Jae Seok Bae
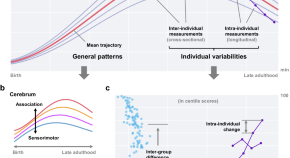
Population imaging cerebellar growth for personalized neuroscience
Growth chart studies of the human cerebellum, which is increasingly recognized as pivotal for cognitive development, are rare. Gaiser and colleagues utilized population-level neuroimaging to unveil cerebellar growth charts from childhood to adolescence, offering insights into brain development.
- Zi-Xuan Zhou
- Xi-Nian Zuo
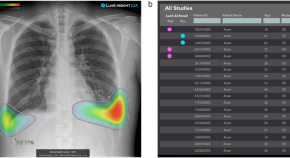
The lucent yet opaque challenge of regulating artificial intelligence in radiology
- James M. Hillis
- Jacob J. Visser
- Katherine P. Andriole
Quick links
- Explore articles by subject
- Guide to authors
- Editorial policies

77 interesting medical research topics for 2024
Last updated
25 November 2023
Reviewed by
Brittany Ferri, PhD, OTR/L
Medical research is the gateway to improved patient care and expanding our available treatment options. However, finding a relevant and compelling research topic can be challenging.
Use this article as a jumping-off point to select an interesting medical research topic for your next paper or clinical study.
- How to choose a medical research topic
When choosing a research topic , it’s essential to consider a couple of things. What topics interest you? What unanswered questions do you want to address?
During the decision-making and brainstorming process, here are a few helpful tips to help you pick the right medical research topic:
Focus on a particular field of study
The best medical research is specific to a particular area. Generalized studies are often too broad to produce meaningful results, so we advise picking a specific niche early in the process.
Maybe a certain topic interests you, or your industry knowledge reveals areas of need.
Look into commonly researched topics
Once you’ve chosen your research field, do some preliminary research. What have other academics done in their papers and projects?
From this list, you can focus on specific topics that interest you without accidentally creating a copycat project. This groundwork will also help you uncover any literature gaps—those may be beneficial areas for research.
Get curious and ask questions
Now you can get curious. Ask questions that start with why, how, or what. These questions are the starting point of your project design and will act as your guiding light throughout the process.
For example:
What impact does pollution have on children’s lung function in inner-city neighborhoods?
Why is pollution-based asthma on the rise?
How can we address pollution-induced asthma in young children?
- 77 medical research topics worth exploring in 2023
Need some research inspiration for your upcoming paper or clinical study? We’ve compiled a list of 77 topical and in-demand medical research ideas. Let’s take a look.
- Exciting new medical research topics
If you want to study cutting-edge topics, here are some exciting options:
COVID-19 and long COVID symptoms
Since 2020, COVID-19 has been a hot-button topic in medicine, along with the long-term symptoms in those with a history of COVID-19.
Examples of COVID-19-related research topics worth exploring include:
The long-term impact of COVID-19 on cardiac and respiratory health
COVID-19 vaccination rates
The evolution of COVID-19 symptoms over time
New variants and strains of the COVID-19 virus
Changes in social behavior and public health regulations amid COVID-19
Vaccinations
Finding ways to cure or reduce the disease burden of chronic infectious diseases is a crucial research area. Vaccination is a powerful option and a great topic to research.
Examples of vaccination-related research topics include:
mRNA vaccines for viral infections
Biomaterial vaccination capabilities
Vaccination rates based on location, ethnicity, or age
Public opinion about vaccination safety
Artificial tissues fabrication
With the need for donor organs increasing, finding ways to fabricate artificial bioactive tissues (and possibly organs) is a popular research area.
Examples of artificial tissue-related research topics you can study include:
The viability of artificially printed tissues
Tissue substrate and building block material studies
The ethics and efficacy of artificial tissue creation
- Medical research topics for medical students
For many medical students, research is a big driver for entering healthcare. If you’re a medical student looking for a research topic, here are some great ideas to work from:
Sleep disorders
Poor sleep quality is a growing problem, and it can significantly impact a person’s overall health.
Examples of sleep disorder-related research topics include:
How stress affects sleep quality
The prevalence and impact of insomnia on patients with mental health conditions
Possible triggers for sleep disorder development
The impact of poor sleep quality on psychological and physical health
How melatonin supplements impact sleep quality
Alzheimer’s and dementia
Cognitive conditions like dementia and Alzheimer’s disease are on the rise worldwide. They currently have no cure. As a result, research about these topics is in high demand.
Examples of dementia-related research topics you could explore include:
The prevalence of Alzheimer’s disease in a chosen population
Early onset symptoms of dementia
Possible triggers or causes of cognitive decline with age
Treatment options for dementia-like conditions
The mental and physical burden of caregiving for patients with dementia
- Lifestyle habits and public health
Modern lifestyles have profoundly impacted the average person’s daily habits, and plenty of interesting topics explore its effects.
Examples of lifestyle and public health-related research topics include:
The nutritional intake of college students
The impact of chronic work stress on overall health
The rise of upper back and neck pain from laptop use
Prevalence and cause of repetitive strain injuries (RSI)
- Controversial medical research paper topics
Medical research is a hotbed of controversial topics, content, and areas of study.
If you want to explore a more niche (and attention-grabbing) concept, here are some controversial medical research topics worth looking into:
The benefits and risks of medical cannabis
Depending on where you live, the legalization and use of cannabis for medical conditions is controversial for the general public and healthcare providers.
Examples of medical cannabis-related research topics that might grab your attention include:
The legalization process of medical cannabis
The impact of cannabis use on developmental milestones in youth users
Cannabis and mental health diagnoses
CBD’s impact on chronic pain
Prevalence of cannabis use in young people
The impact of maternal cannabis use on fetal development
Understanding how THC impacts cognitive function
Human genetics
The Human Genome Project identified, mapped, and sequenced all human DNA genes. Its completion in 2003 opened up a world of exciting and controversial studies in human genetics.
Examples of human genetics-related research topics worth delving into include:
Medical genetics and the incidence of genetic-based health disorders
Behavioral genetics differences between identical twins
Genetic risk factors for neurodegenerative disorders
Machine learning technologies for genetic research
Sexual health studies
Human sexuality and sexual health are important (yet often stigmatized) medical topics that need new research and analysis.
As a diverse field ranging from sexual orientation studies to sexual pathophysiology, examples of sexual health-related research topics include:
The incidence of sexually transmitted infections within a chosen population
Mental health conditions within the LGBTQIA+ community
The impact of untreated sexually transmitted infections
Access to safe sex resources (condoms, dental dams, etc.) in rural areas
- Health and wellness research topics
Human wellness and health are trendy topics in modern medicine as more people are interested in finding natural ways to live healthier lifestyles.
If this field of study interests you, here are some big topics in the wellness space:
Gluten sensitivity
Gluten allergies and intolerances have risen over the past few decades. If you’re interested in exploring this topic, your options range in severity from mild gastrointestinal symptoms to full-blown anaphylaxis.
Some examples of gluten sensitivity-related research topics include:
The pathophysiology and incidence of Celiac disease
Early onset symptoms of gluten intolerance
The prevalence of gluten allergies within a set population
Gluten allergies and the incidence of other gastrointestinal health conditions
Pollution and lung health
Living in large urban cities means regular exposure to high levels of pollutants.
As more people become interested in protecting their lung health, examples of impactful lung health and pollution-related research topics include:
The extent of pollution in densely packed urban areas
The prevalence of pollution-based asthma in a set population
Lung capacity and function in young people
The benefits and risks of steroid therapy for asthma
Pollution risks based on geographical location
Plant-based diets
Plant-based diets like vegan and paleo diets are emerging trends in healthcare due to their limited supporting research.
If you’re interested in learning more about the potential benefits or risks of holistic, diet-based medicine, examples of plant-based diet research topics to explore include:
Vegan and plant-based diets as part of disease management
Potential risks and benefits of specific plant-based diets
Plant-based diets and their impact on body mass index
The effect of diet and lifestyle on chronic disease management
Health supplements
Supplements are a multi-billion dollar industry. Many health-conscious people take supplements, including vitamins, minerals, herbal medicine, and more.
Examples of health supplement-related research topics worth investigating include:
Omega-3 fish oil safety and efficacy for cardiac patients
The benefits and risks of regular vitamin D supplementation
Health supplementation regulation and product quality
The impact of social influencer marketing on consumer supplement practices
Analyzing added ingredients in protein powders
- Healthcare research topics
Working within the healthcare industry means you have insider knowledge and opportunity. Maybe you’d like to research the overall system, administration, and inherent biases that disrupt access to quality care.
While these topics are essential to explore, it is important to note that these studies usually require approval and oversight from an Institutional Review Board (IRB). This ensures the study is ethical and does not harm any subjects.
For this reason, the IRB sets protocols that require additional planning, so consider this when mapping out your study’s timeline.
Here are some examples of trending healthcare research areas worth pursuing:
The pros and cons of electronic health records
The rise of electronic healthcare charting and records has forever changed how medical professionals and patients interact with their health data.
Examples of electronic health record-related research topics include:
The number of medication errors reported during a software switch
Nurse sentiment analysis of electronic charting practices
Ethical and legal studies into encrypting and storing personal health data
Inequities within healthcare access
Many barriers inhibit people from accessing the quality medical care they need. These issues result in health disparities and injustices.
Examples of research topics about health inequities include:
The impact of social determinants of health in a set population
Early and late-stage cancer stage diagnosis in urban vs. rural populations
Affordability of life-saving medications
Health insurance limitations and their impact on overall health
Diagnostic and treatment rates across ethnicities
People who belong to an ethnic minority are more likely to experience barriers and restrictions when trying to receive quality medical care. This is due to systemic healthcare racism and bias.
As a result, diagnostic and treatment rates in minority populations are a hot-button field of research. Examples of ethnicity-based research topics include:
Cancer biopsy rates in BIPOC women
The prevalence of diabetes in Indigenous communities
Access inequalities in women’s health preventative screenings
The prevalence of undiagnosed hypertension in Black populations
- Pharmaceutical research topics
Large pharmaceutical companies are incredibly interested in investing in research to learn more about potential cures and treatments for diseases.
If you’re interested in building a career in pharmaceutical research, here are a few examples of in-demand research topics:
Cancer treatment options
Clinical research is in high demand as pharmaceutical companies explore novel cancer treatment options outside of chemotherapy and radiation.
Examples of cancer treatment-related research topics include:
Stem cell therapy for cancer
Oncogenic gene dysregulation and its impact on disease
Cancer-causing viral agents and their risks
Treatment efficacy based on early vs. late-stage cancer diagnosis
Cancer vaccines and targeted therapies
Immunotherapy for cancer
Pain medication alternatives
Historically, opioid medications were the primary treatment for short- and long-term pain. But, with the opioid epidemic getting worse, the need for alternative pain medications has never been more urgent.
Examples of pain medication-related research topics include:
Opioid withdrawal symptoms and risks
Early signs of pain medication misuse
Anti-inflammatory medications for pain control
- Identify trends in your medical research with Dovetail
Are you interested in contributing life-changing research? Today’s medical research is part of the future of clinical patient care.
As your go-to resource for speedy and accurate data analysis , we are proud to partner with healthcare researchers to innovate and improve the future of healthcare.
Editor’s picks
Last updated: 11 January 2024
Last updated: 15 January 2024
Last updated: 25 November 2023
Last updated: 12 May 2023
Last updated: 30 April 2024
Last updated: 18 May 2023
Last updated: 10 April 2023
Latest articles
Related topics, .css-je19u9{-webkit-align-items:flex-end;-webkit-box-align:flex-end;-ms-flex-align:flex-end;align-items:flex-end;display:-webkit-box;display:-webkit-flex;display:-ms-flexbox;display:flex;-webkit-flex-direction:row;-ms-flex-direction:row;flex-direction:row;-webkit-box-flex-wrap:wrap;-webkit-flex-wrap:wrap;-ms-flex-wrap:wrap;flex-wrap:wrap;-webkit-box-pack:center;-ms-flex-pack:center;-webkit-justify-content:center;justify-content:center;row-gap:0;text-align:center;max-width:671px;}@media (max-width: 1079px){.css-je19u9{max-width:400px;}.css-je19u9>span{white-space:pre;}}@media (max-width: 799px){.css-je19u9{max-width:400px;}.css-je19u9>span{white-space:pre;}} decide what to .css-1kiodld{max-height:56px;display:-webkit-box;display:-webkit-flex;display:-ms-flexbox;display:flex;-webkit-align-items:center;-webkit-box-align:center;-ms-flex-align:center;align-items:center;}@media (max-width: 1079px){.css-1kiodld{display:none;}} build next, decide what to build next.

Users report unexpectedly high data usage, especially during streaming sessions.

Users find it hard to navigate from the home page to relevant playlists in the app.

It would be great to have a sleep timer feature, especially for bedtime listening.

I need better filters to find the songs or artists I’m looking for.
Log in or sign up
Get started for free
- PRO Courses Guides New Tech Help Pro Expert Videos About wikiHow Pro Upgrade Sign In
- EDIT Edit this Article
- EXPLORE Tech Help Pro About Us Random Article Quizzes Request a New Article Community Dashboard This Or That Game Popular Categories Arts and Entertainment Artwork Books Movies Computers and Electronics Computers Phone Skills Technology Hacks Health Men's Health Mental Health Women's Health Relationships Dating Love Relationship Issues Hobbies and Crafts Crafts Drawing Games Education & Communication Communication Skills Personal Development Studying Personal Care and Style Fashion Hair Care Personal Hygiene Youth Personal Care School Stuff Dating All Categories Arts and Entertainment Finance and Business Home and Garden Relationship Quizzes Cars & Other Vehicles Food and Entertaining Personal Care and Style Sports and Fitness Computers and Electronics Health Pets and Animals Travel Education & Communication Hobbies and Crafts Philosophy and Religion Work World Family Life Holidays and Traditions Relationships Youth
- Browse Articles
- Learn Something New
- Quizzes Hot
- This Or That Game
- Train Your Brain
- Explore More
- Support wikiHow
- About wikiHow
- Log in / Sign up
- Education and Communications
- College University and Postgraduate
- Academic Writing
- Research Papers
How to Write a Medical Research Paper
Last Updated: February 5, 2024 Approved
This article was co-authored by Chris M. Matsko, MD . Dr. Chris M. Matsko is a retired physician based in Pittsburgh, Pennsylvania. With over 25 years of medical research experience, Dr. Matsko was awarded the Pittsburgh Cornell University Leadership Award for Excellence. He holds a BS in Nutritional Science from Cornell University and an MD from the Temple University School of Medicine in 2007. Dr. Matsko earned a Research Writing Certification from the American Medical Writers Association (AMWA) in 2016 and a Medical Writing & Editing Certification from the University of Chicago in 2017. wikiHow marks an article as reader-approved once it receives enough positive feedback. In this case, 89% of readers who voted found the article helpful, earning it our reader-approved status. This article has been viewed 202,510 times.
Writing a medical research paper is similar to writing other research papers in that you want to use reliable sources, write in a clear and organized style, and offer a strong argument for all conclusions you present. In some cases the research you discuss will be data you have actually collected to answer your research questions. Understanding proper formatting, citations, and style will help you write and informative and respected paper.
Researching Your Paper

- Pick something that really interests you to make the research more fun.
- Choose a topic that has unanswered questions and propose solutions.

- Quantitative studies consist of original research performed by the writer. These research papers will need to include sections like Hypothesis (or Research Question), Previous Findings, Method, Limitations, Results, Discussion, and Application.
- Synthesis papers review the research already published and analyze it. They find weaknesses and strengths in the research, apply it to a specific situation, and then indicate a direction for future research.

- Keep track of your sources. Write down all publication information necessary for citation: author, title of article, title of book or journal, publisher, edition, date published, volume number, issue number, page number, and anything else pertaining to your source. A program like Endnote can help you keep track of your sources.
- Take detailed notes as you read. Paraphrase information in your own words or if you copy directly from the article or book, indicate that these are direct quotes by using quotation marks to prevent plagiarism.
- Be sure to keep all of your notes with the correct source.
- Your professor and librarians can also help you find good resources.

- Keep all of your notes in a physical folder or in a digitized form on the computer.
- Start to form the basic outline of your paper using the notes you have collected.
Writing Your Paper

- Start with bullet points and then add in notes you've taken from references that support your ideas. [1] X Trustworthy Source PubMed Central Journal archive from the U.S. National Institutes of Health Go to source
- A common way to format research papers is to follow the IMRAD format. This dictates the structure of your paper in the following order: I ntroduction, M ethods, R esults, a nd D iscussion. [2] X Research source
- The outline is just the basic structure of your paper. Don't worry if you have to rearrange a few times to get it right.
- Ask others to look over your outline and get feedback on the organization.
- Know the audience you are writing for and adjust your style accordingly. [3] X Research source

- Use a standard font type and size, such as Times New Roman 12 point font.
- Double-space your paper.
- If necessary, create a cover page. Most schools require a cover page of some sort. Include your main title, running title (often a shortened version of your main title), author's name, course name, and semester.

- Break up information into sections and subsections and address one main point per section.
- Include any figures or data tables that support your main ideas.
- For a quantitative study, state the methods used to obtain results.

- Clearly state and summarize the main points of your research paper.
- Discuss how this research contributes to the field and why it is important. [4] X Research source
- Highlight potential applications of the theory if appropriate.
- Propose future directions that build upon the research you have presented. [5] X Research source
- Keep the introduction and discussion short, and spend more time explaining the methods and results.

- State why the problem is important to address.
- Discuss what is currently known and what is lacking in the field.
- State the objective of your paper.
- Keep the introduction short.

- Highlight the purpose of the paper and the main conclusions.
- State why your conclusions are important.
- Be concise in your summary of the paper.
- Show that you have a solid study design and a high-quality data set.
- Abstracts are usually one paragraph and between 250 – 500 words.

- Unless otherwise directed, use the American Medical Association (AMA) style guide to properly format citations.
- Add citations at end of a sentence to indicate that you are using someone else's idea. Use these throughout your research paper as needed. They include the author's last name, year of publication, and page number.
- Compile your reference list and add it to the end of your paper.
- Use a citation program if you have access to one to simplify the process.

- Continually revise your paper to make sure it is structured in a logical way.
- Proofread your paper for spelling and grammatical errors.
- Make sure you are following the proper formatting guidelines provided for the paper.
- Have others read your paper to proofread and check for clarity. Revise as needed.
Expert Q&A

- Ask your professor for help if you are stuck or confused about any part of your research paper. They are familiar with the style and structure of papers and can provide you with more resources. Thanks Helpful 0 Not Helpful 0
- Refer to your professor's specific guidelines. Some instructors modify parts of a research paper to better fit their assignment. Others may request supplementary details, such as a synopsis for your research project . Thanks Helpful 0 Not Helpful 0
- Set aside blocks of time specifically for writing each day. Thanks Helpful 0 Not Helpful 0

- Do not plagiarize. Plagiarism is using someone else's work, words, or ideas and presenting them as your own. It is important to cite all sources in your research paper, both through internal citations and on your reference page. Thanks Helpful 4 Not Helpful 2
You Might Also Like

- ↑ http://www.ncbi.nlm.nih.gov/pmc/articles/PMC3178846/
- ↑ http://owl.excelsior.edu/research-and-citations/outlining/outlining-imrad/
- ↑ http://china.elsevier.com/ElsevierDNN/Portals/7/How%20to%20write%20a%20world-class%20paper.pdf
- ↑ http://intqhc.oxfordjournals.org/content/16/3/191
- ↑ http://www.ruf.rice.edu/~bioslabs/tools/report/reportform.html#form
About This Article

To write a medical research paper, research your topic thoroughly and compile your data. Next, organize your notes and create a strong outline that breaks up the information into sections and subsections, addressing one main point per section. Write the results and discussion sections first to go over your findings, then write the introduction to state your objective and provide background information. Finally, write the abstract, which concisely summarizes the article by highlighting the main points. For tips on formatting and using citations, read on! Did this summary help you? Yes No
- Send fan mail to authors
Reader Success Stories

Joshua Benibo
Jun 5, 2018
Did this article help you?

Dominic Cipriano
Aug 16, 2016

Obiajulu Echedom
Apr 2, 2017

Noura Ammar Alhossiny
Feb 14, 2017

Dawn Daniel
Apr 20, 2017

Featured Articles

Trending Articles

Watch Articles

- Terms of Use
- Privacy Policy
- Do Not Sell or Share My Info
- Not Selling Info
wikiHow Tech Help Pro:
Develop the tech skills you need for work and life
- Share full article
Advertisement
Supported by
Guest Essay
In Medicine, the Morally Unthinkable Too Easily Comes to Seem Normal

By Carl Elliott
Dr. Elliott teaches medical ethics at the University of Minnesota. He is the author of the forthcoming book “The Occasional Human Sacrifice: Medical Experimentation and the Price of Saying No,” from which this essay is adapted.
Here is the way I remember it: The year is 1985, and a few medical students are gathered around an operating table where an anesthetized woman has been prepared for surgery. The attending physician, a gynecologist, asks the group: “Has everyone felt a cervix? Here’s your chance.” One after another, we take turns inserting two gloved fingers into the unconscious woman’s vagina.
Had the woman consented to a pelvic exam? Did she understand that when the lights went dim she would be treated like a clinical practice dummy, her genitalia palpated by a succession of untrained hands? I don’t know. Like most medical students, I just did as I was told.
Last month the Department of Health and Human Services issued new guidance requiring written informed consent for pelvic exams and other intimate procedures performed under anesthesia. Much of the force behind the new requirement came from distressed medical students who saw these pelvic exams as wrong and summoned the courage to speak out.
Whether the guidance will actually change clinical practice I don’t know. Medical traditions are notoriously difficult to uproot, and academic medicine does not easily tolerate ethical dissent. I doubt the medical profession can be trusted to reform itself.
What is it that leads a rare individual to say no to practices that are deceptive, exploitative or harmful when everyone else thinks they are fine? For a long time I assumed that saying no was mainly an issue of moral courage. The relevant question was: If you are a witness to wrongdoing, will you be brave enough to speak out?
But then I started talking to insiders who had blown the whistle on abusive medical research. Soon I realized that I had overlooked the importance of moral perception. Before you decide to speak out about wrongdoing, you have to recognize it for what it is.
This is not as simple as it seems. Part of what makes medical training so unsettling is how often you are thrust into situations in which you don’t really know how to behave. Nothing in your life up to that point has prepared you to dissect a cadaver, perform a rectal exam or deliver a baby. Never before have you seen a psychotic patient involuntarily sedated and strapped to a bed or a brain-dead body wheeled out of a hospital room to have its organs harvested for transplantation. Your initial reaction is often a combination of revulsion, anxiety and self-consciousness.
To embark on a career in medicine is like moving to a foreign country where you do not understand the customs, rituals, manners or language. Your main concern on arrival is how to fit in and avoid causing offense. This is true even if the local customs seem backward or cruel. What’s more, this particular country has an authoritarian government and a rigid status hierarchy where dissent is not just discouraged but also punished. Living happily in this country requires convincing yourself that whatever discomfort you feel comes from your own ignorance and lack of experience. Over time, you learn how to assimilate. You may even come to laugh at how naïve you were when you first arrived.
A rare few people hang onto that discomfort and learn from it. When Michael Wilkins and William Bronston started working at the Willowbrook State School in Staten Island as young doctors in the early 1970s, they found thousands of mentally disabled children condemned to the most horrific conditions imaginable: naked children rocking and moaning on concrete floors in puddles of their own urine; an overpowering stench of illness and filth; a research unit where children were deliberately infected with hepatitis A and B.
“It was truly an American concentration camp,” Dr. Bronston told me. Yet when he and Dr. Wilkins tried to enlist Willowbrook doctors and nurses to reform the institution, they were met with indifference or hostility. It seemed as if no one else on the medical staff could see what they saw. It was only when Dr. Wilkins went to a reporter and showed the world what was happening behind the Willowbrook walls that anything began to change.
When I asked Dr. Bronston how it was possible for doctors and nurses to work at Willowbrook without seeing it as a crime scene, he told me it began with the way the institution was structured and organized. “Medically secured, medically managed, doctor-validated,” he said. Medical professionals just accommodated themselves to the status quo. “You get with the program because that’s what you’re being hired to do,” he said.
One of the great mysteries of human behavior is how institutions create social worlds where unthinkable practices come to seem normal. This is as true of academic medical centers as it is of prisons and military units. When we are told about a horrific medical research scandal, we assume that we would see it just as the whistle-blower Peter Buxtun saw the Tuskegee syphilis study : an abuse so shocking that only a sociopath could fail to perceive it.
Yet it rarely happens this way. It took Mr. Buxtun seven years to convince others to see the abuses for what they were. It has taken other whistle-blowers even longer. Even when the outside world condemns a practice, medical institutions typically insist that the outsiders don’t really understand.
According to Irving Janis, a Yale psychologist who popularized the notion of groupthink, the forces of social conformity are especially powerful in organizations that are driven by a deep sense of moral purpose. If the aims of the organization are righteous, its members feel, it is wrong to put barriers in the way.
This observation helps explain why academic medicine not only defends researchers accused of wrongdoing but also sometimes rewards them. Many of the researchers responsible for the most notorious abuses in recent medical history — the Tuskegee syphilis study, the Willowbrook hepatitis studies, the Cincinnati radiation studies , the Holmesburg prison studies — were celebrated with professional accolades even after the abuses were first called out.
The culture of medicine is notoriously resistant to change. During the 1970s, it was thought that the solution to medical misconduct was formal education in ethics. Major academic medical centers began establishing bioethics centers and programs throughout the 1980s and ’90s, and today virtually every medical school in the country requires ethics training.
Yet it is debatable whether that training has had any effect. Many of the most egregious ethical abuses in recent decades have taken place in medical centers with prominent bioethics programs, such as the University of Pennsylvania , Duke University , Columbia University and Johns Hopkins University , as well as my own institution, the University of Minnesota .
One could be forgiven for concluding that the only way the culture of medicine will change is if changes are forced on it from the outside — by oversight bodies, legislators or litigators. For example, many states have responded to the controversy over pelvic exams by passing laws banning the practice unless the patient has explicitly given consent.
You may find it hard to understand how pelvic exams on unconscious women without their consent could seem like anything but a terrible invasion. Yet a central aim of medical training is to transform your sensibility. You are taught to steel yourself against your natural emotional reactions to death and disfigurement; to set aside your customary views about privacy and shame; to see the human body as a thing to be examined, tested and studied.
One danger of this transformation is that you will see your colleagues and superiors do horrible things and be afraid to speak up. But the more subtle danger is that you will no longer see what they are doing as horrible. You will just think: This is the way it is done.
Carl Elliott ( @FearLoathingBTX ) teaches medical ethics at the University of Minnesota. He is the author of the forthcoming book “The Occasional Human Sacrifice: Medical Experimentation and the Price of Saying No,” from which this essay is adapted.
The Times is committed to publishing a diversity of letters to the editor. We’d like to hear what you think about this or any of our articles. Here are some tips . And here’s our email: [email protected] .
Follow the New York Times Opinion section on Facebook , Instagram , TikTok , WhatsApp , X and Threads .
- Teaching & Learning
- Student Concentration
- Assessment Hub
- Find CME Courses
- Plan CME Activity
- Medical Educator Series - YES!
- MEDG Discussion Group
- Session Recordings
- Submission Guidelines
- Poster Guidelines
- Criteria for Excellent Posters
- CME Accreditation
- Winning Posters
- Teaching Awards
- Education Scholar Fellowship
- Fellowship Alumni
- Center Team
INFORMATION FOR
- Residents & Fellows
- Researchers
Susan D. Boulware, MD
Contact information.
- Office 203.785.5809
- Appt 877.925.3637
- Clinic Fax 203.764.9149
Patient Care Location
- YNHH Specialty Clinic 1 Long Wharf Drive, Ste 2 New Haven, CT 06511 Appointments : 203.785.4081
Mailing Address
- Pediatric Endocrinology & Diabetes
1 Longwharf Drive
New Haven, CT 06511
United States
Patient Care
Research & publications, appointments.
Dr. Boulware has an interest in disorders of growth and development having published several papers looking at the metabolic actions of IGF-1, a hormone critical to childhood growth. She provides care to children with general disorders of the endocrine system (pituitary, thyroid, adrenal, testes or ovaries). She is the pediatric endocrinologist in the interdisciplinary Yale Clinic for Children with Differences in Sex Development and she is the Medical Director of the Interdisciplinary Yale Gender Program offering care to gender-expansive youth.
Education & Training
- Resident University of Texas Medical School (1987)
- MD University of Texas at San Antonio (1984)
- Fellow Yale University School of Medicine
Honors & Recognition
Departments & organizations.
- Dean's Advisory Council for LGBTQI Affairs
- Directories
- Pediatric Gender Program
- Research Interest
- Yale Medicine
This paper is in the following e-collection/theme issue:
Published on 10.5.2024 in Vol 26 (2024)
Spanish and Catalan Versions of the eHealth Literacy Questionnaire: Translation, Cross-Cultural Adaptation, and Validation Study
Authors of this article:

There are no citations yet available for this article according to Crossref .
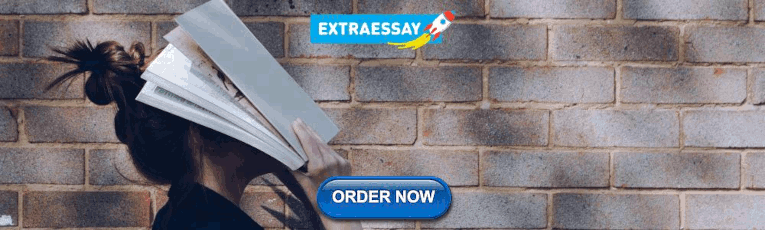
IMAGES
VIDEO
COMMENTS
The New England Journal of Medicine (NEJM) is a weekly general medical journal that publishes new medical research and review articles, and editorial opinion on a wide variety of topics of ...
Medical research involves research in a wide range of fields, such as biology, chemistry, pharmacology and toxicology with the goal of developing new medicines or medical procedures or improving ...
BMC Medical Ethics (2024) Artificial intelligence (AI) is poised to broadly reshape medicine, potentially improving the experiences of both clinicians and patients. We discuss key findings from a ...
Artificial intelligence in healthcare: transforming the practice of medicine is a review article that explores the current and potential applications of AI in various domains of medicine, such as diagnosis, treatment, research, and education. The article also discusses the challenges and ethical issues of implementing AI in healthcare, and provides some recommendations for future directions ...
Recent years have seen a revolution in the domain of medical science, with ground-breaking discoveries changing health care as we once knew it [].These advances have considerably improved disease diagnosis, treatment, and management, improving patient outcomes and quality of life [2-5].These innovations range from the creation of novel medications and treatments to the utilization of cutting ...
JAMA - The Latest Medical Research, Reviews, and Guidelines. Home New Online Issues For Authors. Editor's Choice: AI Could Mean Better Mental Health for All. Original Investigation Ponatinib vs Imatinib in Frontline Philadelphia Chromosome-Positive Acute Lymphoblastic Leukemia: A Randomized Clinical Trial Elias Jabbour, MD; Hagop M ...
Several papers on ML's application in the medical field have been widely published. Fig. 4 demonstrates published articles amount in the period between 2000 and December 2021. The materials are gathered depending on the keyword "machine learning in the medical field" First, published articles were collected from well-known publishers, including Springer, Elsevier, IEEE, and some other ...
May 9, 2024 PLOS General. Our portfolio of medicine and healthcare journals continues to push the boundaries of research and research communication. Discover the latest papers making waves in your community, explore further insight into the contributions of PLOS journals to the field, and keep up-to-date with the latest research in 2024.
Abstract. Artificially intelligent computer systems are used extensively in medical sciences. Common applications include diagnosing patients, end-to-end drug discovery and development, improving communication between physician and patient, transcribing medical documents, such as prescriptions, and remotely treating patients.
They also assess the future evolution of payment systems leading toward sustainable health, changes in provider roles, and the entrance of new nontraditional players. For more on this topic, watch Health Systems in 2030, a free NEJM Catalyst virtual event held on March 4, 2021.
Original research studies that can improve decision making in clinical medicine, public health, health care policy, medical education, or biomedical research.
The concept of ML and its versatile capabilities have been reported to serve the healthcare domain in various ways. Fig. 2 explores the different enablers and quality pillars for helping and caring for healthcare units. The outbreak prediction capability, medical imaging diagnosis, behavioural modifications, records of patient data, etc., are some of the majorly elaborated quality pillars of ...
Deep Learning Approach (DLA) in medical image analysis emerges as a fast-growing research field. DLA has been widely used in medical imaging to detect the presence or absence of the disease. This paper presents the development of artificial neural networks, comprehensive analysis of DLA, which delivers promising medical imaging applications.
Medical imaging comprises different imaging modalities and processes to image human body for diagnostic and treatment purposes. It is also used to follow the course of a disease already diagnosed ...
This paper covers the significant potential of biosensors in the medical field. Various applications can cover by the successful implementation of this technology. The major research objectives of this paper are as under: RO1: To study biosensors and their benefits for the medical field;
Interdisciplinary Medicine is an open access biomedicine journal publishing significant works related to any medical field with an interdisciplinary ... The wet membrane was then dried using filtration papers and ... which stabilizes the dipole direction even after the removal of the external magnetic field. In this research, the casted polymer ...
Summary. This review found that robots have played 10 main roles across a variety of clinical environments. The two predominant roles were surgical and rehabilitation and mobility. Although robots were mainly studied in the surgical theatre and rehabilitation unit, other settings ranged from the hospital ward to inpatient pharmacy.
Feature papers represent the most advanced research with significant potential for high impact in the field. A Feature Paper should be a substantial original Article that involves several techniques or approaches, provides an outlook for future research directions and describes possible research applications.
The image processing techniques were founded in the 1960s. Those techniques were used for different fields such as Space, clinical purposes, arts, and TV image improvement. In the 1970s with the ...
Since 2020, COVID-19 has been a hot-button topic in medicine, along with the long-term symptoms in those with a history of COVID-19. Examples of COVID-19-related research topics worth exploring include: The long-term impact of COVID-19 on cardiac and respiratory health. COVID-19 vaccination rates.
Include your main title, running title (often a shortened version of your main title), author's name, course name, and semester. 3. Compile your results. Divide the paper into logical sections determined by the type of paper you are writing.
For carrying out this study, relevant papers on Nanotechnology in the medical field from Scopus, Google scholar, ResearchGate, and other research platforms are identified and studied. The study discusses different types of Nanoparticles used in the medical field. This paper discusses nanotechnology applications in the medical field.
Summarize the research strengths, hotspots, and stages of scientific evolution in the field of motivation in PA by performing visualization analysis which used CiteSpace software on 2375 publications including from January 1st, 1980 to January 31st, 2024, in the Web of Science Core Collection (WOSCC).
Major academic medical centers began establishing bioethics centers and programs throughout the 1980s and '90s, and today virtually every medical school in the country requires ethics training.
Medical research is of high value to clinicians and society. ... limited to the human medical field (veterinary field, dentistry, or nursing were excluded). Only primary research articles with the above criteria were considered—all opinions, reviews, commentary, or editorial papers were excluded. ... They were also more likely to publish ...
Dr. Boulware has an interest in disorders of growth and development having published several papers looking at the metabolic actions of IGF-1, a hormone critical to childhood growth. She provides care to children with general disorders of the endocrine system (pituitary, thyroid, adrenal, testes or ovaries).
This paper is in the following e-collection/theme issue: eHealth Literacy / Digital Literacy (338) Research Instruments, Questionnaires, and Tools (527) Development and Evaluation of Research Methods, Instruments and Tools (388) Assessing and Building eHealth / Digital Literacy in Populations (22) Tools and Questionnaires in Human Factors Evaluation (42)
After studying the major research papers, the requirements of the medical field are classified into five major areas. 3D scanning technologies help to fulfil these medical field requirements. 3.2.1. Complexity. Traditional manufacturing technologies are not conformable in febricity complex and organic shapes of the outer part of the body.
In a randomized field experiment in Senegal, we assess the potential of three types of quality signaling to increase demand for high-quality solar lamps. We find no effect on demand when consumers are offered a money-back guarantee but increased demand with a third-party certification or warranty, consistent with the notion that consumers are ...
In principle, medical research is classified into primary and secondary research. While secondary research summarizes available studies in the form of reviews and meta-analyses, the actual studies are performed in primary research. Three main areas are distinguished: basic medical research, clinical research, and epidemiological research.