- Research article
- Open access
- Published: 04 June 2021
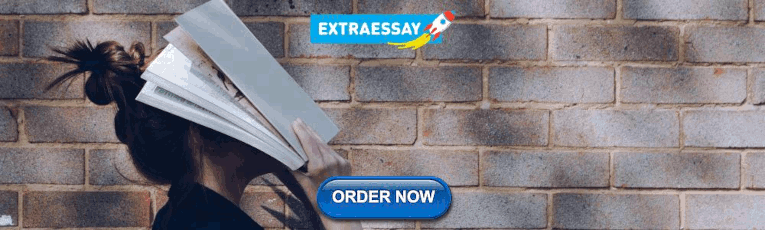
Coronavirus disease (COVID-19) pandemic: an overview of systematic reviews
- Israel Júnior Borges do Nascimento 1 , 2 ,
- Dónal P. O’Mathúna 3 , 4 ,
- Thilo Caspar von Groote 5 ,
- Hebatullah Mohamed Abdulazeem 6 ,
- Ishanka Weerasekara 7 , 8 ,
- Ana Marusic 9 ,
- Livia Puljak ORCID: orcid.org/0000-0002-8467-6061 10 ,
- Vinicius Tassoni Civile 11 ,
- Irena Zakarija-Grkovic 9 ,
- Tina Poklepovic Pericic 9 ,
- Alvaro Nagib Atallah 11 ,
- Santino Filoso 12 ,
- Nicola Luigi Bragazzi 13 &
- Milena Soriano Marcolino 1
On behalf of the International Network of Coronavirus Disease 2019 (InterNetCOVID-19)
BMC Infectious Diseases volume 21 , Article number: 525 ( 2021 ) Cite this article
16k Accesses
28 Citations
13 Altmetric
Metrics details
Navigating the rapidly growing body of scientific literature on the SARS-CoV-2 pandemic is challenging, and ongoing critical appraisal of this output is essential. We aimed to summarize and critically appraise systematic reviews of coronavirus disease (COVID-19) in humans that were available at the beginning of the pandemic.
Nine databases (Medline, EMBASE, Cochrane Library, CINAHL, Web of Sciences, PDQ-Evidence, WHO’s Global Research, LILACS, and Epistemonikos) were searched from December 1, 2019, to March 24, 2020. Systematic reviews analyzing primary studies of COVID-19 were included. Two authors independently undertook screening, selection, extraction (data on clinical symptoms, prevalence, pharmacological and non-pharmacological interventions, diagnostic test assessment, laboratory, and radiological findings), and quality assessment (AMSTAR 2). A meta-analysis was performed of the prevalence of clinical outcomes.
Eighteen systematic reviews were included; one was empty (did not identify any relevant study). Using AMSTAR 2, confidence in the results of all 18 reviews was rated as “critically low”. Identified symptoms of COVID-19 were (range values of point estimates): fever (82–95%), cough with or without sputum (58–72%), dyspnea (26–59%), myalgia or muscle fatigue (29–51%), sore throat (10–13%), headache (8–12%) and gastrointestinal complaints (5–9%). Severe symptoms were more common in men. Elevated C-reactive protein and lactate dehydrogenase, and slightly elevated aspartate and alanine aminotransferase, were commonly described. Thrombocytopenia and elevated levels of procalcitonin and cardiac troponin I were associated with severe disease. A frequent finding on chest imaging was uni- or bilateral multilobar ground-glass opacity. A single review investigated the impact of medication (chloroquine) but found no verifiable clinical data. All-cause mortality ranged from 0.3 to 13.9%.
Conclusions
In this overview of systematic reviews, we analyzed evidence from the first 18 systematic reviews that were published after the emergence of COVID-19. However, confidence in the results of all reviews was “critically low”. Thus, systematic reviews that were published early on in the pandemic were of questionable usefulness. Even during public health emergencies, studies and systematic reviews should adhere to established methodological standards.
Peer Review reports
The spread of the “Severe Acute Respiratory Coronavirus 2” (SARS-CoV-2), the causal agent of COVID-19, was characterized as a pandemic by the World Health Organization (WHO) in March 2020 and has triggered an international public health emergency [ 1 ]. The numbers of confirmed cases and deaths due to COVID-19 are rapidly escalating, counting in millions [ 2 ], causing massive economic strain, and escalating healthcare and public health expenses [ 3 , 4 ].
The research community has responded by publishing an impressive number of scientific reports related to COVID-19. The world was alerted to the new disease at the beginning of 2020 [ 1 ], and by mid-March 2020, more than 2000 articles had been published on COVID-19 in scholarly journals, with 25% of them containing original data [ 5 ]. The living map of COVID-19 evidence, curated by the Evidence for Policy and Practice Information and Co-ordinating Centre (EPPI-Centre), contained more than 40,000 records by February 2021 [ 6 ]. More than 100,000 records on PubMed were labeled as “SARS-CoV-2 literature, sequence, and clinical content” by February 2021 [ 7 ].
Due to publication speed, the research community has voiced concerns regarding the quality and reproducibility of evidence produced during the COVID-19 pandemic, warning of the potential damaging approach of “publish first, retract later” [ 8 ]. It appears that these concerns are not unfounded, as it has been reported that COVID-19 articles were overrepresented in the pool of retracted articles in 2020 [ 9 ]. These concerns about inadequate evidence are of major importance because they can lead to poor clinical practice and inappropriate policies [ 10 ].
Systematic reviews are a cornerstone of today’s evidence-informed decision-making. By synthesizing all relevant evidence regarding a particular topic, systematic reviews reflect the current scientific knowledge. Systematic reviews are considered to be at the highest level in the hierarchy of evidence and should be used to make informed decisions. However, with high numbers of systematic reviews of different scope and methodological quality being published, overviews of multiple systematic reviews that assess their methodological quality are essential [ 11 , 12 , 13 ]. An overview of systematic reviews helps identify and organize the literature and highlights areas of priority in decision-making.
In this overview of systematic reviews, we aimed to summarize and critically appraise systematic reviews of coronavirus disease (COVID-19) in humans that were available at the beginning of the pandemic.
Methodology
Research question.
This overview’s primary objective was to summarize and critically appraise systematic reviews that assessed any type of primary clinical data from patients infected with SARS-CoV-2. Our research question was purposefully broad because we wanted to analyze as many systematic reviews as possible that were available early following the COVID-19 outbreak.
Study design
We conducted an overview of systematic reviews. The idea for this overview originated in a protocol for a systematic review submitted to PROSPERO (CRD42020170623), which indicated a plan to conduct an overview.
Overviews of systematic reviews use explicit and systematic methods for searching and identifying multiple systematic reviews addressing related research questions in the same field to extract and analyze evidence across important outcomes. Overviews of systematic reviews are in principle similar to systematic reviews of interventions, but the unit of analysis is a systematic review [ 14 , 15 , 16 ].
We used the overview methodology instead of other evidence synthesis methods to allow us to collate and appraise multiple systematic reviews on this topic, and to extract and analyze their results across relevant topics [ 17 ]. The overview and meta-analysis of systematic reviews allowed us to investigate the methodological quality of included studies, summarize results, and identify specific areas of available or limited evidence, thereby strengthening the current understanding of this novel disease and guiding future research [ 13 ].
A reporting guideline for overviews of reviews is currently under development, i.e., Preferred Reporting Items for Overviews of Reviews (PRIOR) [ 18 ]. As the PRIOR checklist is still not published, this study was reported following the Preferred Reporting Items for Systematic Reviews and Meta-Analyses (PRISMA) 2009 statement [ 19 ]. The methodology used in this review was adapted from the Cochrane Handbook for Systematic Reviews of Interventions and also followed established methodological considerations for analyzing existing systematic reviews [ 14 ].
Approval of a research ethics committee was not necessary as the study analyzed only publicly available articles.
Eligibility criteria
Systematic reviews were included if they analyzed primary data from patients infected with SARS-CoV-2 as confirmed by RT-PCR or another pre-specified diagnostic technique. Eligible reviews covered all topics related to COVID-19 including, but not limited to, those that reported clinical symptoms, diagnostic methods, therapeutic interventions, laboratory findings, or radiological results. Both full manuscripts and abbreviated versions, such as letters, were eligible.
No restrictions were imposed on the design of the primary studies included within the systematic reviews, the last search date, whether the review included meta-analyses or language. Reviews related to SARS-CoV-2 and other coronaviruses were eligible, but from those reviews, we analyzed only data related to SARS-CoV-2.
No consensus definition exists for a systematic review [ 20 ], and debates continue about the defining characteristics of a systematic review [ 21 ]. Cochrane’s guidance for overviews of reviews recommends setting pre-established criteria for making decisions around inclusion [ 14 ]. That is supported by a recent scoping review about guidance for overviews of systematic reviews [ 22 ].
Thus, for this study, we defined a systematic review as a research report which searched for primary research studies on a specific topic using an explicit search strategy, had a detailed description of the methods with explicit inclusion criteria provided, and provided a summary of the included studies either in narrative or quantitative format (such as a meta-analysis). Cochrane and non-Cochrane systematic reviews were considered eligible for inclusion, with or without meta-analysis, and regardless of the study design, language restriction and methodology of the included primary studies. To be eligible for inclusion, reviews had to be clearly analyzing data related to SARS-CoV-2 (associated or not with other viruses). We excluded narrative reviews without those characteristics as these are less likely to be replicable and are more prone to bias.
Scoping reviews and rapid reviews were eligible for inclusion in this overview if they met our pre-defined inclusion criteria noted above. We included reviews that addressed SARS-CoV-2 and other coronaviruses if they reported separate data regarding SARS-CoV-2.
Information sources
Nine databases were searched for eligible records published between December 1, 2019, and March 24, 2020: Cochrane Database of Systematic Reviews via Cochrane Library, PubMed, EMBASE, CINAHL (Cumulative Index to Nursing and Allied Health Literature), Web of Sciences, LILACS (Latin American and Caribbean Health Sciences Literature), PDQ-Evidence, WHO’s Global Research on Coronavirus Disease (COVID-19), and Epistemonikos.
The comprehensive search strategy for each database is provided in Additional file 1 and was designed and conducted in collaboration with an information specialist. All retrieved records were primarily processed in EndNote, where duplicates were removed, and records were then imported into the Covidence platform [ 23 ]. In addition to database searches, we screened reference lists of reviews included after screening records retrieved via databases.
Study selection
All searches, screening of titles and abstracts, and record selection, were performed independently by two investigators using the Covidence platform [ 23 ]. Articles deemed potentially eligible were retrieved for full-text screening carried out independently by two investigators. Discrepancies at all stages were resolved by consensus. During the screening, records published in languages other than English were translated by a native/fluent speaker.
Data collection process
We custom designed a data extraction table for this study, which was piloted by two authors independently. Data extraction was performed independently by two authors. Conflicts were resolved by consensus or by consulting a third researcher.
We extracted the following data: article identification data (authors’ name and journal of publication), search period, number of databases searched, population or settings considered, main results and outcomes observed, and number of participants. From Web of Science (Clarivate Analytics, Philadelphia, PA, USA), we extracted journal rank (quartile) and Journal Impact Factor (JIF).
We categorized the following as primary outcomes: all-cause mortality, need for and length of mechanical ventilation, length of hospitalization (in days), admission to intensive care unit (yes/no), and length of stay in the intensive care unit.
The following outcomes were categorized as exploratory: diagnostic methods used for detection of the virus, male to female ratio, clinical symptoms, pharmacological and non-pharmacological interventions, laboratory findings (full blood count, liver enzymes, C-reactive protein, d-dimer, albumin, lipid profile, serum electrolytes, blood vitamin levels, glucose levels, and any other important biomarkers), and radiological findings (using radiography, computed tomography, magnetic resonance imaging or ultrasound).
We also collected data on reporting guidelines and requirements for the publication of systematic reviews and meta-analyses from journal websites where included reviews were published.
Quality assessment in individual reviews
Two researchers independently assessed the reviews’ quality using the “A MeaSurement Tool to Assess Systematic Reviews 2 (AMSTAR 2)”. We acknowledge that the AMSTAR 2 was created as “a critical appraisal tool for systematic reviews that include randomized or non-randomized studies of healthcare interventions, or both” [ 24 ]. However, since AMSTAR 2 was designed for systematic reviews of intervention trials, and we included additional types of systematic reviews, we adjusted some AMSTAR 2 ratings and reported these in Additional file 2 .
Adherence to each item was rated as follows: yes, partial yes, no, or not applicable (such as when a meta-analysis was not conducted). The overall confidence in the results of the review is rated as “critically low”, “low”, “moderate” or “high”, according to the AMSTAR 2 guidance based on seven critical domains, which are items 2, 4, 7, 9, 11, 13, 15 as defined by AMSTAR 2 authors [ 24 ]. We reported our adherence ratings for transparency of our decision with accompanying explanations, for each item, in each included review.
One of the included systematic reviews was conducted by some members of this author team [ 25 ]. This review was initially assessed independently by two authors who were not co-authors of that review to prevent the risk of bias in assessing this study.
Synthesis of results
For data synthesis, we prepared a table summarizing each systematic review. Graphs illustrating the mortality rate and clinical symptoms were created. We then prepared a narrative summary of the methods, findings, study strengths, and limitations.
For analysis of the prevalence of clinical outcomes, we extracted data on the number of events and the total number of patients to perform proportional meta-analysis using RStudio© software, with the “meta” package (version 4.9–6), using the “metaprop” function for reviews that did not perform a meta-analysis, excluding case studies because of the absence of variance. For reviews that did not perform a meta-analysis, we presented pooled results of proportions with their respective confidence intervals (95%) by the inverse variance method with a random-effects model, using the DerSimonian-Laird estimator for τ 2 . We adjusted data using Freeman-Tukey double arcosen transformation. Confidence intervals were calculated using the Clopper-Pearson method for individual studies. We created forest plots using the RStudio© software, with the “metafor” package (version 2.1–0) and “forest” function.
Managing overlapping systematic reviews
Some of the included systematic reviews that address the same or similar research questions may include the same primary studies in overviews. Including such overlapping reviews may introduce bias when outcome data from the same primary study are included in the analyses of an overview multiple times. Thus, in summaries of evidence, multiple-counting of the same outcome data will give data from some primary studies too much influence [ 14 ]. In this overview, we did not exclude overlapping systematic reviews because, according to Cochrane’s guidance, it may be appropriate to include all relevant reviews’ results if the purpose of the overview is to present and describe the current body of evidence on a topic [ 14 ]. To avoid any bias in summary estimates associated with overlapping reviews, we generated forest plots showing data from individual systematic reviews, but the results were not pooled because some primary studies were included in multiple reviews.
Our search retrieved 1063 publications, of which 175 were duplicates. Most publications were excluded after the title and abstract analysis ( n = 860). Among the 28 studies selected for full-text screening, 10 were excluded for the reasons described in Additional file 3 , and 18 were included in the final analysis (Fig. 1 ) [ 25 , 26 , 27 , 28 , 29 , 30 , 31 , 32 , 33 , 34 , 35 , 36 , 37 , 38 , 39 , 40 , 41 , 42 ]. Reference list screening did not retrieve any additional systematic reviews.
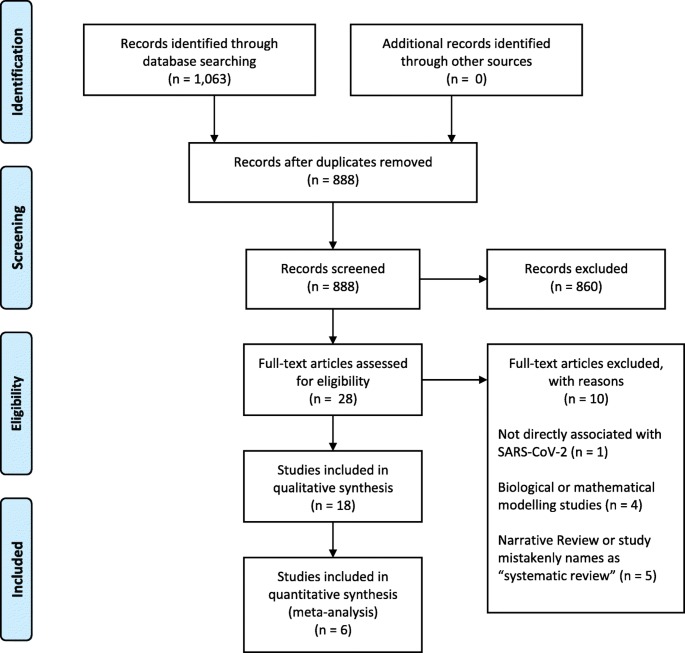
PRISMA flow diagram
Characteristics of included reviews
Summary features of 18 systematic reviews are presented in Table 1 . They were published in 14 different journals. Only four of these journals had specific requirements for systematic reviews (with or without meta-analysis): European Journal of Internal Medicine, Journal of Clinical Medicine, Ultrasound in Obstetrics and Gynecology, and Clinical Research in Cardiology . Two journals reported that they published only invited reviews ( Journal of Medical Virology and Clinica Chimica Acta ). Three systematic reviews in our study were published as letters; one was labeled as a scoping review and another as a rapid review (Table 2 ).
All reviews were published in English, in first quartile (Q1) journals, with JIF ranging from 1.692 to 6.062. One review was empty, meaning that its search did not identify any relevant studies; i.e., no primary studies were included [ 36 ]. The remaining 17 reviews included 269 unique studies; the majority ( N = 211; 78%) were included in only a single review included in our study (range: 1 to 12). Primary studies included in the reviews were published between December 2019 and March 18, 2020, and comprised case reports, case series, cohorts, and other observational studies. We found only one review that included randomized clinical trials [ 38 ]. In the included reviews, systematic literature searches were performed from 2019 (entire year) up to March 9, 2020. Ten systematic reviews included meta-analyses. The list of primary studies found in the included systematic reviews is shown in Additional file 4 , as well as the number of reviews in which each primary study was included.
Population and study designs
Most of the reviews analyzed data from patients with COVID-19 who developed pneumonia, acute respiratory distress syndrome (ARDS), or any other correlated complication. One review aimed to evaluate the effectiveness of using surgical masks on preventing transmission of the virus [ 36 ], one review was focused on pediatric patients [ 34 ], and one review investigated COVID-19 in pregnant women [ 37 ]. Most reviews assessed clinical symptoms, laboratory findings, or radiological results.
Systematic review findings
The summary of findings from individual reviews is shown in Table 2 . Overall, all-cause mortality ranged from 0.3 to 13.9% (Fig. 2 ).
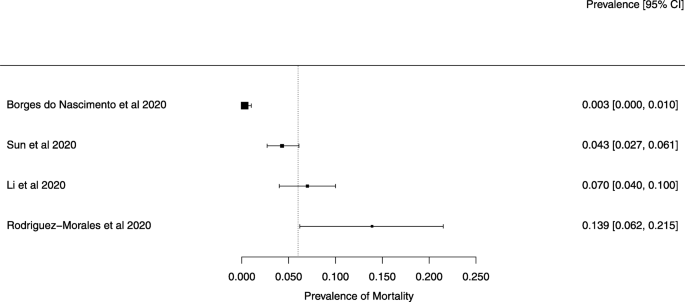
A meta-analysis of the prevalence of mortality
Clinical symptoms
Seven reviews described the main clinical manifestations of COVID-19 [ 26 , 28 , 29 , 34 , 35 , 39 , 41 ]. Three of them provided only a narrative discussion of symptoms [ 26 , 34 , 35 ]. In the reviews that performed a statistical analysis of the incidence of different clinical symptoms, symptoms in patients with COVID-19 were (range values of point estimates): fever (82–95%), cough with or without sputum (58–72%), dyspnea (26–59%), myalgia or muscle fatigue (29–51%), sore throat (10–13%), headache (8–12%), gastrointestinal disorders, such as diarrhea, nausea or vomiting (5.0–9.0%), and others (including, in one study only: dizziness 12.1%) (Figs. 3 , 4 , 5 , 6 , 7 , 8 and 9 ). Three reviews assessed cough with and without sputum together; only one review assessed sputum production itself (28.5%).
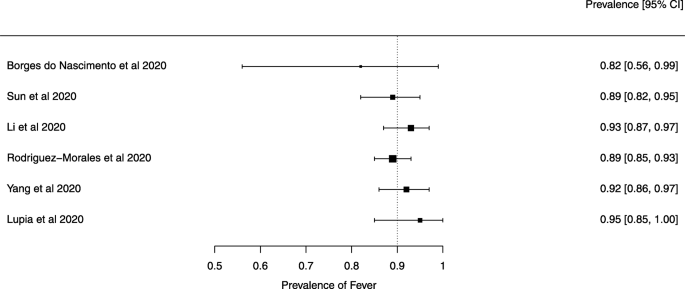
A meta-analysis of the prevalence of fever
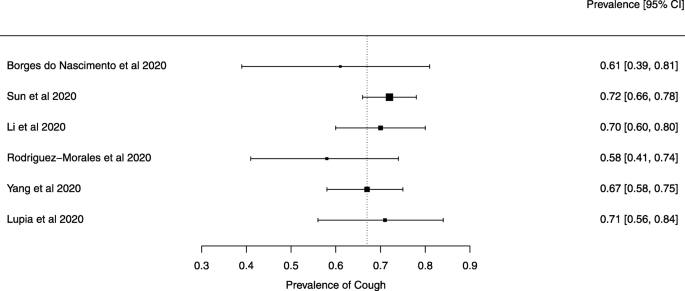
A meta-analysis of the prevalence of cough
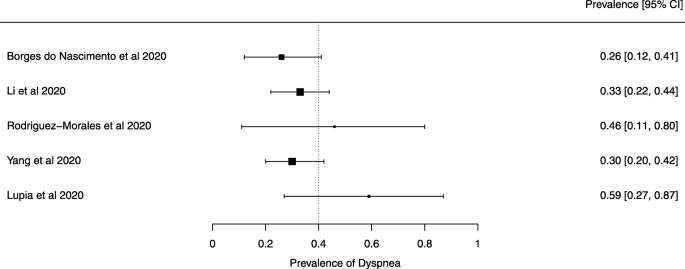
A meta-analysis of the prevalence of dyspnea
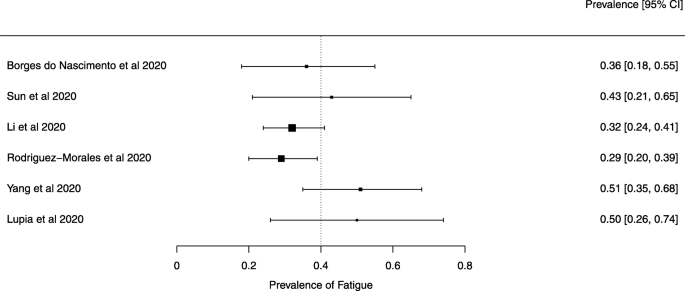
A meta-analysis of the prevalence of fatigue or myalgia
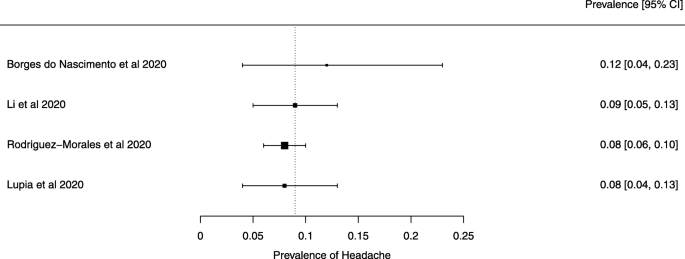
A meta-analysis of the prevalence of headache
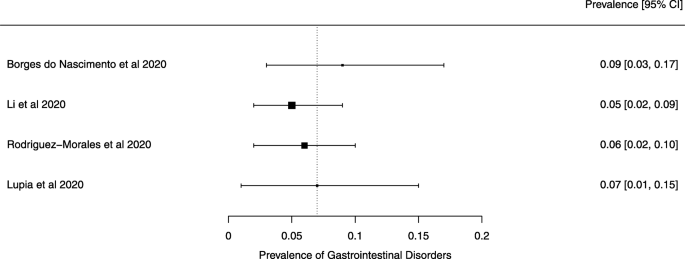
A meta-analysis of the prevalence of gastrointestinal disorders
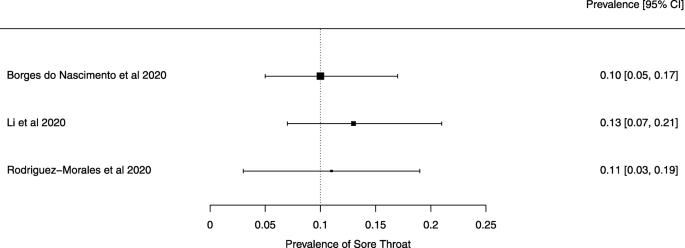
A meta-analysis of the prevalence of sore throat
Diagnostic aspects
Three reviews described methodologies, protocols, and tools used for establishing the diagnosis of COVID-19 [ 26 , 34 , 38 ]. The use of respiratory swabs (nasal or pharyngeal) or blood specimens to assess the presence of SARS-CoV-2 nucleic acid using RT-PCR assays was the most commonly used diagnostic method mentioned in the included studies. These diagnostic tests have been widely used, but their precise sensitivity and specificity remain unknown. One review included a Chinese study with clinical diagnosis with no confirmation of SARS-CoV-2 infection (patients were diagnosed with COVID-19 if they presented with at least two symptoms suggestive of COVID-19, together with laboratory and chest radiography abnormalities) [ 34 ].
Therapeutic possibilities
Pharmacological and non-pharmacological interventions (supportive therapies) used in treating patients with COVID-19 were reported in five reviews [ 25 , 27 , 34 , 35 , 38 ]. Antivirals used empirically for COVID-19 treatment were reported in seven reviews [ 25 , 27 , 34 , 35 , 37 , 38 , 41 ]; most commonly used were protease inhibitors (lopinavir, ritonavir, darunavir), nucleoside reverse transcriptase inhibitor (tenofovir), nucleotide analogs (remdesivir, galidesivir, ganciclovir), and neuraminidase inhibitors (oseltamivir). Umifenovir, a membrane fusion inhibitor, was investigated in two studies [ 25 , 35 ]. Possible supportive interventions analyzed were different types of oxygen supplementation and breathing support (invasive or non-invasive ventilation) [ 25 ]. The use of antibiotics, both empirically and to treat secondary pneumonia, was reported in six studies [ 25 , 26 , 27 , 34 , 35 , 38 ]. One review specifically assessed evidence on the efficacy and safety of the anti-malaria drug chloroquine [ 27 ]. It identified 23 ongoing trials investigating the potential of chloroquine as a therapeutic option for COVID-19, but no verifiable clinical outcomes data. The use of mesenchymal stem cells, antifungals, and glucocorticoids were described in four reviews [ 25 , 34 , 35 , 38 ].
Laboratory and radiological findings
Of the 18 reviews included in this overview, eight analyzed laboratory parameters in patients with COVID-19 [ 25 , 29 , 30 , 32 , 33 , 34 , 35 , 39 ]; elevated C-reactive protein levels, associated with lymphocytopenia, elevated lactate dehydrogenase, as well as slightly elevated aspartate and alanine aminotransferase (AST, ALT) were commonly described in those eight reviews. Lippi et al. assessed cardiac troponin I (cTnI) [ 25 ], procalcitonin [ 32 ], and platelet count [ 33 ] in COVID-19 patients. Elevated levels of procalcitonin [ 32 ] and cTnI [ 30 ] were more likely to be associated with a severe disease course (requiring intensive care unit admission and intubation). Furthermore, thrombocytopenia was frequently observed in patients with complicated COVID-19 infections [ 33 ].
Chest imaging (chest radiography and/or computed tomography) features were assessed in six reviews, all of which described a frequent pattern of local or bilateral multilobar ground-glass opacity [ 25 , 34 , 35 , 39 , 40 , 41 ]. Those six reviews showed that septal thickening, bronchiectasis, pleural and cardiac effusions, halo signs, and pneumothorax were observed in patients suffering from COVID-19.
Quality of evidence in individual systematic reviews
Table 3 shows the detailed results of the quality assessment of 18 systematic reviews, including the assessment of individual items and summary assessment. A detailed explanation for each decision in each review is available in Additional file 5 .
Using AMSTAR 2 criteria, confidence in the results of all 18 reviews was rated as “critically low” (Table 3 ). Common methodological drawbacks were: omission of prospective protocol submission or publication; use of inappropriate search strategy: lack of independent and dual literature screening and data-extraction (or methodology unclear); absence of an explanation for heterogeneity among the studies included; lack of reasons for study exclusion (or rationale unclear).
Risk of bias assessment, based on a reported methodological tool, and quality of evidence appraisal, in line with the Grading of Recommendations Assessment, Development, and Evaluation (GRADE) method, were reported only in one review [ 25 ]. Five reviews presented a table summarizing bias, using various risk of bias tools [ 25 , 29 , 39 , 40 , 41 ]. One review analyzed “study quality” [ 37 ]. One review mentioned the risk of bias assessment in the methodology but did not provide any related analysis [ 28 ].
This overview of systematic reviews analyzed the first 18 systematic reviews published after the onset of the COVID-19 pandemic, up to March 24, 2020, with primary studies involving more than 60,000 patients. Using AMSTAR-2, we judged that our confidence in all those reviews was “critically low”. Ten reviews included meta-analyses. The reviews presented data on clinical manifestations, laboratory and radiological findings, and interventions. We found no systematic reviews on the utility of diagnostic tests.
Symptoms were reported in seven reviews; most of the patients had a fever, cough, dyspnea, myalgia or muscle fatigue, and gastrointestinal disorders such as diarrhea, nausea, or vomiting. Olfactory dysfunction (anosmia or dysosmia) has been described in patients infected with COVID-19 [ 43 ]; however, this was not reported in any of the reviews included in this overview. During the SARS outbreak in 2002, there were reports of impairment of the sense of smell associated with the disease [ 44 , 45 ].
The reported mortality rates ranged from 0.3 to 14% in the included reviews. Mortality estimates are influenced by the transmissibility rate (basic reproduction number), availability of diagnostic tools, notification policies, asymptomatic presentations of the disease, resources for disease prevention and control, and treatment facilities; variability in the mortality rate fits the pattern of emerging infectious diseases [ 46 ]. Furthermore, the reported cases did not consider asymptomatic cases, mild cases where individuals have not sought medical treatment, and the fact that many countries had limited access to diagnostic tests or have implemented testing policies later than the others. Considering the lack of reviews assessing diagnostic testing (sensitivity, specificity, and predictive values of RT-PCT or immunoglobulin tests), and the preponderance of studies that assessed only symptomatic individuals, considerable imprecision around the calculated mortality rates existed in the early stage of the COVID-19 pandemic.
Few reviews included treatment data. Those reviews described studies considered to be at a very low level of evidence: usually small, retrospective studies with very heterogeneous populations. Seven reviews analyzed laboratory parameters; those reviews could have been useful for clinicians who attend patients suspected of COVID-19 in emergency services worldwide, such as assessing which patients need to be reassessed more frequently.
All systematic reviews scored poorly on the AMSTAR 2 critical appraisal tool for systematic reviews. Most of the original studies included in the reviews were case series and case reports, impacting the quality of evidence. Such evidence has major implications for clinical practice and the use of these reviews in evidence-based practice and policy. Clinicians, patients, and policymakers can only have the highest confidence in systematic review findings if high-quality systematic review methodologies are employed. The urgent need for information during a pandemic does not justify poor quality reporting.
We acknowledge that there are numerous challenges associated with analyzing COVID-19 data during a pandemic [ 47 ]. High-quality evidence syntheses are needed for decision-making, but each type of evidence syntheses is associated with its inherent challenges.
The creation of classic systematic reviews requires considerable time and effort; with massive research output, they quickly become outdated, and preparing updated versions also requires considerable time. A recent study showed that updates of non-Cochrane systematic reviews are published a median of 5 years after the publication of the previous version [ 48 ].
Authors may register a review and then abandon it [ 49 ], but the existence of a public record that is not updated may lead other authors to believe that the review is still ongoing. A quarter of Cochrane review protocols remains unpublished as completed systematic reviews 8 years after protocol publication [ 50 ].
Rapid reviews can be used to summarize the evidence, but they involve methodological sacrifices and simplifications to produce information promptly, with inconsistent methodological approaches [ 51 ]. However, rapid reviews are justified in times of public health emergencies, and even Cochrane has resorted to publishing rapid reviews in response to the COVID-19 crisis [ 52 ]. Rapid reviews were eligible for inclusion in this overview, but only one of the 18 reviews included in this study was labeled as a rapid review.
Ideally, COVID-19 evidence would be continually summarized in a series of high-quality living systematic reviews, types of evidence synthesis defined as “ a systematic review which is continually updated, incorporating relevant new evidence as it becomes available ” [ 53 ]. However, conducting living systematic reviews requires considerable resources, calling into question the sustainability of such evidence synthesis over long periods [ 54 ].
Research reports about COVID-19 will contribute to research waste if they are poorly designed, poorly reported, or simply not necessary. In principle, systematic reviews should help reduce research waste as they usually provide recommendations for further research that is needed or may advise that sufficient evidence exists on a particular topic [ 55 ]. However, systematic reviews can also contribute to growing research waste when they are not needed, or poorly conducted and reported. Our present study clearly shows that most of the systematic reviews that were published early on in the COVID-19 pandemic could be categorized as research waste, as our confidence in their results is critically low.
Our study has some limitations. One is that for AMSTAR 2 assessment we relied on information available in publications; we did not attempt to contact study authors for clarifications or additional data. In three reviews, the methodological quality appraisal was challenging because they were published as letters, or labeled as rapid communications. As a result, various details about their review process were not included, leading to AMSTAR 2 questions being answered as “not reported”, resulting in low confidence scores. Full manuscripts might have provided additional information that could have led to higher confidence in the results. In other words, low scores could reflect incomplete reporting, not necessarily low-quality review methods. To make their review available more rapidly and more concisely, the authors may have omitted methodological details. A general issue during a crisis is that speed and completeness must be balanced. However, maintaining high standards requires proper resourcing and commitment to ensure that the users of systematic reviews can have high confidence in the results.
Furthermore, we used adjusted AMSTAR 2 scoring, as the tool was designed for critical appraisal of reviews of interventions. Some reviews may have received lower scores than actually warranted in spite of these adjustments.
Another limitation of our study may be the inclusion of multiple overlapping reviews, as some included reviews included the same primary studies. According to the Cochrane Handbook, including overlapping reviews may be appropriate when the review’s aim is “ to present and describe the current body of systematic review evidence on a topic ” [ 12 ], which was our aim. To avoid bias with summarizing evidence from overlapping reviews, we presented the forest plots without summary estimates. The forest plots serve to inform readers about the effect sizes for outcomes that were reported in each review.
Several authors from this study have contributed to one of the reviews identified [ 25 ]. To reduce the risk of any bias, two authors who did not co-author the review in question initially assessed its quality and limitations.
Finally, we note that the systematic reviews included in our overview may have had issues that our analysis did not identify because we did not analyze their primary studies to verify the accuracy of the data and information they presented. We give two examples to substantiate this possibility. Lovato et al. wrote a commentary on the review of Sun et al. [ 41 ], in which they criticized the authors’ conclusion that sore throat is rare in COVID-19 patients [ 56 ]. Lovato et al. highlighted that multiple studies included in Sun et al. did not accurately describe participants’ clinical presentations, warning that only three studies clearly reported data on sore throat [ 56 ].
In another example, Leung [ 57 ] warned about the review of Li, L.Q. et al. [ 29 ]: “ it is possible that this statistic was computed using overlapped samples, therefore some patients were double counted ”. Li et al. responded to Leung that it is uncertain whether the data overlapped, as they used data from published articles and did not have access to the original data; they also reported that they requested original data and that they plan to re-do their analyses once they receive them; they also urged readers to treat the data with caution [ 58 ]. This points to the evolving nature of evidence during a crisis.
Our study’s strength is that this overview adds to the current knowledge by providing a comprehensive summary of all the evidence synthesis about COVID-19 available early after the onset of the pandemic. This overview followed strict methodological criteria, including a comprehensive and sensitive search strategy and a standard tool for methodological appraisal of systematic reviews.
In conclusion, in this overview of systematic reviews, we analyzed evidence from the first 18 systematic reviews that were published after the emergence of COVID-19. However, confidence in the results of all the reviews was “critically low”. Thus, systematic reviews that were published early on in the pandemic could be categorized as research waste. Even during public health emergencies, studies and systematic reviews should adhere to established methodological standards to provide patients, clinicians, and decision-makers trustworthy evidence.
Availability of data and materials
All data collected and analyzed within this study are available from the corresponding author on reasonable request.
World Health Organization. Timeline - COVID-19: Available at: https://www.who.int/news/item/29-06-2020-covidtimeline . Accessed 1 June 2021.
COVID-19 Dashboard by the Center for Systems Science and Engineering (CSSE) at Johns Hopkins University (JHU). Available at: https://coronavirus.jhu.edu/map.html . Accessed 1 June 2021.
Anzai A, Kobayashi T, Linton NM, Kinoshita R, Hayashi K, Suzuki A, et al. Assessing the Impact of Reduced Travel on Exportation Dynamics of Novel Coronavirus Infection (COVID-19). J Clin Med. 2020;9(2):601.
Chinazzi M, Davis JT, Ajelli M, Gioannini C, Litvinova M, Merler S, et al. The effect of travel restrictions on the spread of the 2019 novel coronavirus (COVID-19) outbreak. Science. 2020;368(6489):395–400. https://doi.org/10.1126/science.aba9757 .
Article CAS PubMed PubMed Central Google Scholar
Fidahic M, Nujic D, Runjic R, Civljak M, Markotic F, Lovric Makaric Z, et al. Research methodology and characteristics of journal articles with original data, preprint articles and registered clinical trial protocols about COVID-19. BMC Med Res Methodol. 2020;20(1):161. https://doi.org/10.1186/s12874-020-01047-2 .
EPPI Centre . COVID-19: a living systematic map of the evidence. Available at: http://eppi.ioe.ac.uk/cms/Projects/DepartmentofHealthandSocialCare/Publishedreviews/COVID-19Livingsystematicmapoftheevidence/tabid/3765/Default.aspx . Accessed 1 June 2021.
NCBI SARS-CoV-2 Resources. Available at: https://www.ncbi.nlm.nih.gov/sars-cov-2/ . Accessed 1 June 2021.
Gustot T. Quality and reproducibility during the COVID-19 pandemic. JHEP Rep. 2020;2(4):100141. https://doi.org/10.1016/j.jhepr.2020.100141 .
Article PubMed PubMed Central Google Scholar
Kodvanj, I., et al., Publishing of COVID-19 Preprints in Peer-reviewed Journals, Preprinting Trends, Public Discussion and Quality Issues. Preprint article. bioRxiv 2020.11.23.394577; doi: https://doi.org/10.1101/2020.11.23.394577 .
Dobler CC. Poor quality research and clinical practice during COVID-19. Breathe (Sheff). 2020;16(2):200112. https://doi.org/10.1183/20734735.0112-2020 .
Article Google Scholar
Bastian H, Glasziou P, Chalmers I. Seventy-five trials and eleven systematic reviews a day: how will we ever keep up? PLoS Med. 2010;7(9):e1000326. https://doi.org/10.1371/journal.pmed.1000326 .
Lunny C, Brennan SE, McDonald S, McKenzie JE. Toward a comprehensive evidence map of overview of systematic review methods: paper 1-purpose, eligibility, search and data extraction. Syst Rev. 2017;6(1):231. https://doi.org/10.1186/s13643-017-0617-1 .
Pollock M, Fernandes RM, Becker LA, Pieper D, Hartling L. Chapter V: Overviews of Reviews. In: Higgins JPT, Thomas J, Chandler J, Cumpston M, Li T, Page MJ, Welch VA (editors). Cochrane Handbook for Systematic Reviews of Interventions version 6.1 (updated September 2020). Cochrane. 2020. Available from www.training.cochrane.org/handbook .
Higgins JPT, Thomas J, Chandler J, Cumpston M, Li T, Page MJ, et al. Cochrane handbook for systematic reviews of interventions version 6.1 (updated September 2020). Cochrane. 2020; Available from www.training.cochrane.org/handbook .
Pollock M, Fernandes RM, Newton AS, Scott SD, Hartling L. The impact of different inclusion decisions on the comprehensiveness and complexity of overviews of reviews of healthcare interventions. Syst Rev. 2019;8(1):18. https://doi.org/10.1186/s13643-018-0914-3 .
Pollock M, Fernandes RM, Newton AS, Scott SD, Hartling L. A decision tool to help researchers make decisions about including systematic reviews in overviews of reviews of healthcare interventions. Syst Rev. 2019;8(1):29. https://doi.org/10.1186/s13643-018-0768-8 .
Hunt H, Pollock A, Campbell P, Estcourt L, Brunton G. An introduction to overviews of reviews: planning a relevant research question and objective for an overview. Syst Rev. 2018;7(1):39. https://doi.org/10.1186/s13643-018-0695-8 .
Pollock M, Fernandes RM, Pieper D, Tricco AC, Gates M, Gates A, et al. Preferred reporting items for overviews of reviews (PRIOR): a protocol for development of a reporting guideline for overviews of reviews of healthcare interventions. Syst Rev. 2019;8(1):335. https://doi.org/10.1186/s13643-019-1252-9 .
Moher D, Liberati A, Tetzlaff J, Altman DG, PRISMA Group. Preferred reporting items for systematic reviews and meta-analyses: the PRISMA statement. Open Med. 2009;3(3):e123–30.
Krnic Martinic M, Pieper D, Glatt A, Puljak L. Definition of a systematic review used in overviews of systematic reviews, meta-epidemiological studies and textbooks. BMC Med Res Methodol. 2019;19(1):203. https://doi.org/10.1186/s12874-019-0855-0 .
Puljak L. If there is only one author or only one database was searched, a study should not be called a systematic review. J Clin Epidemiol. 2017;91:4–5. https://doi.org/10.1016/j.jclinepi.2017.08.002 .
Article PubMed Google Scholar
Gates M, Gates A, Guitard S, Pollock M, Hartling L. Guidance for overviews of reviews continues to accumulate, but important challenges remain: a scoping review. Syst Rev. 2020;9(1):254. https://doi.org/10.1186/s13643-020-01509-0 .
Covidence - systematic review software. Available at: https://www.covidence.org/ . Accessed 1 June 2021.
Shea BJ, Reeves BC, Wells G, Thuku M, Hamel C, Moran J, et al. AMSTAR 2: a critical appraisal tool for systematic reviews that include randomised or non-randomised studies of healthcare interventions, or both. BMJ. 2017;358:j4008.
Borges do Nascimento IJ, et al. Novel Coronavirus Infection (COVID-19) in Humans: A Scoping Review and Meta-Analysis. J Clin Med. 2020;9(4):941.
Article PubMed Central Google Scholar
Adhikari SP, Meng S, Wu YJ, Mao YP, Ye RX, Wang QZ, et al. Epidemiology, causes, clinical manifestation and diagnosis, prevention and control of coronavirus disease (COVID-19) during the early outbreak period: a scoping review. Infect Dis Poverty. 2020;9(1):29. https://doi.org/10.1186/s40249-020-00646-x .
Cortegiani A, Ingoglia G, Ippolito M, Giarratano A, Einav S. A systematic review on the efficacy and safety of chloroquine for the treatment of COVID-19. J Crit Care. 2020;57:279–83. https://doi.org/10.1016/j.jcrc.2020.03.005 .
Li B, Yang J, Zhao F, Zhi L, Wang X, Liu L, et al. Prevalence and impact of cardiovascular metabolic diseases on COVID-19 in China. Clin Res Cardiol. 2020;109(5):531–8. https://doi.org/10.1007/s00392-020-01626-9 .
Article CAS PubMed Google Scholar
Li LQ, Huang T, Wang YQ, Wang ZP, Liang Y, Huang TB, et al. COVID-19 patients’ clinical characteristics, discharge rate, and fatality rate of meta-analysis. J Med Virol. 2020;92(6):577–83. https://doi.org/10.1002/jmv.25757 .
Lippi G, Lavie CJ, Sanchis-Gomar F. Cardiac troponin I in patients with coronavirus disease 2019 (COVID-19): evidence from a meta-analysis. Prog Cardiovasc Dis. 2020;63(3):390–1. https://doi.org/10.1016/j.pcad.2020.03.001 .
Lippi G, Henry BM. Active smoking is not associated with severity of coronavirus disease 2019 (COVID-19). Eur J Intern Med. 2020;75:107–8. https://doi.org/10.1016/j.ejim.2020.03.014 .
Lippi G, Plebani M. Procalcitonin in patients with severe coronavirus disease 2019 (COVID-19): a meta-analysis. Clin Chim Acta. 2020;505:190–1. https://doi.org/10.1016/j.cca.2020.03.004 .
Lippi G, Plebani M, Henry BM. Thrombocytopenia is associated with severe coronavirus disease 2019 (COVID-19) infections: a meta-analysis. Clin Chim Acta. 2020;506:145–8. https://doi.org/10.1016/j.cca.2020.03.022 .
Ludvigsson JF. Systematic review of COVID-19 in children shows milder cases and a better prognosis than adults. Acta Paediatr. 2020;109(6):1088–95. https://doi.org/10.1111/apa.15270 .
Lupia T, Scabini S, Mornese Pinna S, di Perri G, de Rosa FG, Corcione S. 2019 novel coronavirus (2019-nCoV) outbreak: a new challenge. J Glob Antimicrob Resist. 2020;21:22–7. https://doi.org/10.1016/j.jgar.2020.02.021 .
Marasinghe, K.M., A systematic review investigating the effectiveness of face mask use in limiting the spread of COVID-19 among medically not diagnosed individuals: shedding light on current recommendations provided to individuals not medically diagnosed with COVID-19. Research Square. Preprint article. doi : https://doi.org/10.21203/rs.3.rs-16701/v1 . 2020 .
Mullins E, Evans D, Viner RM, O’Brien P, Morris E. Coronavirus in pregnancy and delivery: rapid review. Ultrasound Obstet Gynecol. 2020;55(5):586–92. https://doi.org/10.1002/uog.22014 .
Pang J, Wang MX, Ang IYH, Tan SHX, Lewis RF, Chen JIP, et al. Potential Rapid Diagnostics, Vaccine and Therapeutics for 2019 Novel coronavirus (2019-nCoV): a systematic review. J Clin Med. 2020;9(3):623.
Rodriguez-Morales AJ, Cardona-Ospina JA, Gutiérrez-Ocampo E, Villamizar-Peña R, Holguin-Rivera Y, Escalera-Antezana JP, et al. Clinical, laboratory and imaging features of COVID-19: a systematic review and meta-analysis. Travel Med Infect Dis. 2020;34:101623. https://doi.org/10.1016/j.tmaid.2020.101623 .
Salehi S, Abedi A, Balakrishnan S, Gholamrezanezhad A. Coronavirus disease 2019 (COVID-19): a systematic review of imaging findings in 919 patients. AJR Am J Roentgenol. 2020;215(1):87–93. https://doi.org/10.2214/AJR.20.23034 .
Sun P, Qie S, Liu Z, Ren J, Li K, Xi J. Clinical characteristics of hospitalized patients with SARS-CoV-2 infection: a single arm meta-analysis. J Med Virol. 2020;92(6):612–7. https://doi.org/10.1002/jmv.25735 .
Yang J, Zheng Y, Gou X, Pu K, Chen Z, Guo Q, et al. Prevalence of comorbidities and its effects in patients infected with SARS-CoV-2: a systematic review and meta-analysis. Int J Infect Dis. 2020;94:91–5. https://doi.org/10.1016/j.ijid.2020.03.017 .
Bassetti M, Vena A, Giacobbe DR. The novel Chinese coronavirus (2019-nCoV) infections: challenges for fighting the storm. Eur J Clin Investig. 2020;50(3):e13209. https://doi.org/10.1111/eci.13209 .
Article CAS Google Scholar
Hwang CS. Olfactory neuropathy in severe acute respiratory syndrome: report of a case. Acta Neurol Taiwanica. 2006;15(1):26–8.
Google Scholar
Suzuki M, Saito K, Min WP, Vladau C, Toida K, Itoh H, et al. Identification of viruses in patients with postviral olfactory dysfunction. Laryngoscope. 2007;117(2):272–7. https://doi.org/10.1097/01.mlg.0000249922.37381.1e .
Rajgor DD, Lee MH, Archuleta S, Bagdasarian N, Quek SC. The many estimates of the COVID-19 case fatality rate. Lancet Infect Dis. 2020;20(7):776–7. https://doi.org/10.1016/S1473-3099(20)30244-9 .
Wolkewitz M, Puljak L. Methodological challenges of analysing COVID-19 data during the pandemic. BMC Med Res Methodol. 2020;20(1):81. https://doi.org/10.1186/s12874-020-00972-6 .
Rombey T, Lochner V, Puljak L, Könsgen N, Mathes T, Pieper D. Epidemiology and reporting characteristics of non-Cochrane updates of systematic reviews: a cross-sectional study. Res Synth Methods. 2020;11(3):471–83. https://doi.org/10.1002/jrsm.1409 .
Runjic E, Rombey T, Pieper D, Puljak L. Half of systematic reviews about pain registered in PROSPERO were not published and the majority had inaccurate status. J Clin Epidemiol. 2019;116:114–21. https://doi.org/10.1016/j.jclinepi.2019.08.010 .
Runjic E, Behmen D, Pieper D, Mathes T, Tricco AC, Moher D, et al. Following Cochrane review protocols to completion 10 years later: a retrospective cohort study and author survey. J Clin Epidemiol. 2019;111:41–8. https://doi.org/10.1016/j.jclinepi.2019.03.006 .
Tricco AC, Antony J, Zarin W, Strifler L, Ghassemi M, Ivory J, et al. A scoping review of rapid review methods. BMC Med. 2015;13(1):224. https://doi.org/10.1186/s12916-015-0465-6 .
COVID-19 Rapid Reviews: Cochrane’s response so far. Available at: https://training.cochrane.org/resource/covid-19-rapid-reviews-cochrane-response-so-far . Accessed 1 June 2021.
Cochrane. Living systematic reviews. Available at: https://community.cochrane.org/review-production/production-resources/living-systematic-reviews . Accessed 1 June 2021.
Millard T, Synnot A, Elliott J, Green S, McDonald S, Turner T. Feasibility and acceptability of living systematic reviews: results from a mixed-methods evaluation. Syst Rev. 2019;8(1):325. https://doi.org/10.1186/s13643-019-1248-5 .
Babic A, Poklepovic Pericic T, Pieper D, Puljak L. How to decide whether a systematic review is stable and not in need of updating: analysis of Cochrane reviews. Res Synth Methods. 2020;11(6):884–90. https://doi.org/10.1002/jrsm.1451 .
Lovato A, Rossettini G, de Filippis C. Sore throat in COVID-19: comment on “clinical characteristics of hospitalized patients with SARS-CoV-2 infection: a single arm meta-analysis”. J Med Virol. 2020;92(7):714–5. https://doi.org/10.1002/jmv.25815 .
Leung C. Comment on Li et al: COVID-19 patients’ clinical characteristics, discharge rate, and fatality rate of meta-analysis. J Med Virol. 2020;92(9):1431–2. https://doi.org/10.1002/jmv.25912 .
Li LQ, Huang T, Wang YQ, Wang ZP, Liang Y, Huang TB, et al. Response to Char’s comment: comment on Li et al: COVID-19 patients’ clinical characteristics, discharge rate, and fatality rate of meta-analysis. J Med Virol. 2020;92(9):1433. https://doi.org/10.1002/jmv.25924 .
Download references
Acknowledgments
We thank Catherine Henderson DPhil from Swanscoe Communications for pro bono medical writing and editing support. We acknowledge support from the Covidence Team, specifically Anneliese Arno. We thank the whole International Network of Coronavirus Disease 2019 (InterNetCOVID-19) for their commitment and involvement. Members of the InterNetCOVID-19 are listed in Additional file 6 . We thank Pavel Cerny and Roger Crosthwaite for guiding the team supervisor (IJBN) on human resources management.
This research received no external funding.
Author information
Authors and affiliations.
University Hospital and School of Medicine, Universidade Federal de Minas Gerais, Belo Horizonte, Minas Gerais, Brazil
Israel Júnior Borges do Nascimento & Milena Soriano Marcolino
Medical College of Wisconsin, Milwaukee, WI, USA
Israel Júnior Borges do Nascimento
Helene Fuld Health Trust National Institute for Evidence-based Practice in Nursing and Healthcare, College of Nursing, The Ohio State University, Columbus, OH, USA
Dónal P. O’Mathúna
School of Nursing, Psychotherapy and Community Health, Dublin City University, Dublin, Ireland
Department of Anesthesiology, Intensive Care and Pain Medicine, University of Münster, Münster, Germany
Thilo Caspar von Groote
Department of Sport and Health Science, Technische Universität München, Munich, Germany
Hebatullah Mohamed Abdulazeem
School of Health Sciences, Faculty of Health and Medicine, The University of Newcastle, Callaghan, Australia
Ishanka Weerasekara
Department of Physiotherapy, Faculty of Allied Health Sciences, University of Peradeniya, Peradeniya, Sri Lanka
Cochrane Croatia, University of Split, School of Medicine, Split, Croatia
Ana Marusic, Irena Zakarija-Grkovic & Tina Poklepovic Pericic
Center for Evidence-Based Medicine and Health Care, Catholic University of Croatia, Ilica 242, 10000, Zagreb, Croatia
Livia Puljak
Cochrane Brazil, Evidence-Based Health Program, Universidade Federal de São Paulo, São Paulo, Brazil
Vinicius Tassoni Civile & Alvaro Nagib Atallah
Yorkville University, Fredericton, New Brunswick, Canada
Santino Filoso
Laboratory for Industrial and Applied Mathematics (LIAM), Department of Mathematics and Statistics, York University, Toronto, Ontario, Canada
Nicola Luigi Bragazzi
You can also search for this author in PubMed Google Scholar
Contributions
IJBN conceived the research idea and worked as a project coordinator. DPOM, TCVG, HMA, IW, AM, LP, VTC, IZG, TPP, ANA, SF, NLB and MSM were involved in data curation, formal analysis, investigation, methodology, and initial draft writing. All authors revised the manuscript critically for the content. The author(s) read and approved the final manuscript.
Corresponding author
Correspondence to Livia Puljak .
Ethics declarations
Ethics approval and consent to participate.
Not required as data was based on published studies.
Consent for publication
Not applicable.
Competing interests
The authors declare no conflict of interest.
Additional information
Publisher’s note.
Springer Nature remains neutral with regard to jurisdictional claims in published maps and institutional affiliations.
Supplementary Information
Additional file 1: appendix 1..
Search strategies used in the study.
Additional file 2: Appendix 2.
Adjusted scoring of AMSTAR 2 used in this study for systematic reviews of studies that did not analyze interventions.
Additional file 3: Appendix 3.
List of excluded studies, with reasons.
Additional file 4: Appendix 4.
Table of overlapping studies, containing the list of primary studies included, their visual overlap in individual systematic reviews, and the number in how many reviews each primary study was included.
Additional file 5: Appendix 5.
A detailed explanation of AMSTAR scoring for each item in each review.
Additional file 6: Appendix 6.
List of members and affiliates of International Network of Coronavirus Disease 2019 (InterNetCOVID-19).
Rights and permissions
Open Access This article is licensed under a Creative Commons Attribution 4.0 International License, which permits use, sharing, adaptation, distribution and reproduction in any medium or format, as long as you give appropriate credit to the original author(s) and the source, provide a link to the Creative Commons licence, and indicate if changes were made. The images or other third party material in this article are included in the article's Creative Commons licence, unless indicated otherwise in a credit line to the material. If material is not included in the article's Creative Commons licence and your intended use is not permitted by statutory regulation or exceeds the permitted use, you will need to obtain permission directly from the copyright holder. To view a copy of this licence, visit http://creativecommons.org/licenses/by/4.0/ . The Creative Commons Public Domain Dedication waiver ( http://creativecommons.org/publicdomain/zero/1.0/ ) applies to the data made available in this article, unless otherwise stated in a credit line to the data.
Reprints and permissions
About this article
Cite this article.
Borges do Nascimento, I.J., O’Mathúna, D.P., von Groote, T.C. et al. Coronavirus disease (COVID-19) pandemic: an overview of systematic reviews. BMC Infect Dis 21 , 525 (2021). https://doi.org/10.1186/s12879-021-06214-4
Download citation
Received : 12 April 2020
Accepted : 19 May 2021
Published : 04 June 2021
DOI : https://doi.org/10.1186/s12879-021-06214-4
Share this article
Anyone you share the following link with will be able to read this content:
Sorry, a shareable link is not currently available for this article.
Provided by the Springer Nature SharedIt content-sharing initiative
- Coronavirus
- Evidence-based medicine
- Infectious diseases
BMC Infectious Diseases
ISSN: 1471-2334
- Submission enquiries: [email protected]
- General enquiries: [email protected]
Click through the PLOS taxonomy to find articles in your field.
For more information about PLOS Subject Areas, click here .
Loading metrics
Open Access
Peer-reviewed
Research Article
The impact of the COVID-19 pandemic on scientific research in the life sciences
Roles Conceptualization, Formal analysis, Methodology, Writing – original draft, Writing – review & editing
Affiliation AXES, IMT School for Advanced Studies Lucca, Lucca, Italy
Roles Conceptualization, Data curation, Formal analysis, Methodology, Software, Visualization, Writing – original draft, Writing – review & editing
* E-mail: [email protected]
Affiliation Chair of Systems Design D-MTEC, ETH Zürich, Zurich, Switzerland

- Massimo Riccaboni,
- Luca Verginer
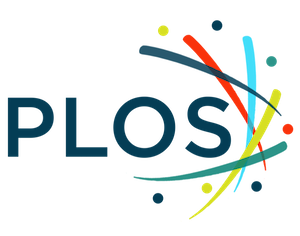
- Published: February 9, 2022
- https://doi.org/10.1371/journal.pone.0263001
- Reader Comments
The COVID-19 outbreak has posed an unprecedented challenge to humanity and science. On the one side, public and private incentives have been put in place to promptly allocate resources toward research areas strictly related to the COVID-19 emergency. However, research in many fields not directly related to the pandemic has been displaced. In this paper, we assess the impact of COVID-19 on world scientific production in the life sciences and find indications that the usage of medical subject headings (MeSH) has changed following the outbreak. We estimate through a difference-in-differences approach the impact of the start of the COVID-19 pandemic on scientific production using the PubMed database (3.6 Million research papers). We find that COVID-19-related MeSH terms have experienced a 6.5 fold increase in output on average, while publications on unrelated MeSH terms dropped by 10 to 12%. The publication weighted impact has an even more pronounced negative effect (-16% to -19%). Moreover, COVID-19 has displaced clinical trial publications (-24%) and diverted grants from research areas not closely related to COVID-19. Note that since COVID-19 publications may have been fast-tracked, the sudden surge in COVID-19 publications might be driven by editorial policy.
Citation: Riccaboni M, Verginer L (2022) The impact of the COVID-19 pandemic on scientific research in the life sciences. PLoS ONE 17(2): e0263001. https://doi.org/10.1371/journal.pone.0263001
Editor: Florian Naudet, University of Rennes 1, FRANCE
Received: April 28, 2021; Accepted: January 10, 2022; Published: February 9, 2022
Copyright: © 2022 Riccaboni, Verginer. This is an open access article distributed under the terms of the Creative Commons Attribution License , which permits unrestricted use, distribution, and reproduction in any medium, provided the original author and source are credited.
Data Availability: The processed data, instructions on how to process the raw PubMed dataset as well as all code are available via Zenodo at https://doi.org/10.5281/zenodo.5121216 .
Funding: The author(s) received no specific funding for this work.
Competing interests: The authors have declared that no competing interests exist.
Introduction
The COVID-19 pandemic has mobilized the world scientific community in 2020, especially in the life sciences [ 1 , 2 ]. In the first three months after the pandemic, the number of scientific papers about COVID-19 was fivefold the number of articles on H1N1 swine influenza [ 3 ]. Similarly, the number of clinical trials related to COVID-19 prophylaxis and treatments skyrocketed [ 4 ]. Thanks to the rapid mobilization of the world scientific community, COVID-19 vaccines have been developed in record time. Despite this undeniable success, there is a rising concern about the negative consequences of COVID-19 on clinical trial research, with many projects being postponed [ 5 – 7 ]. According to Evaluate Pharma, clinical trials were one of the pandemic’s first casualties, with a record number of 160 studies suspended for reasons related to COVID-19 in April 2020 [ 8 , 9 ] reporting a total of 1,200 trials suspended as of July 2020. As a consequence, clinical researchers have been impaired by reduced access to healthcare research infrastructures. Particularly, the COVID-19 outbreak took a tall on women and early-career scientists [ 10 – 13 ]. On a different ground, Shan and colleagues found that non-COVID-19-related articles decreased as COVID-19-related articles increased in top clinical research journals [ 14 ]. Fraser and coworker found that COVID-19 preprints received more attention and citations than non-COVID-19 preprints [ 1 ]. More recently, Hook and Porter have found some early evidence of ‘covidisation’ of academic research, with research grants and output diverted to COVID-19 research in 2020 [ 15 ]. How much should scientists switch their efforts toward SARS-CoV-2 prevention, treatment, or mitigation? There is a growing consensus that the current level of ‘covidisation’ of research can be wasteful [ 4 , 5 , 16 ].
Against this background, in this paper, we investigate if the COVID-19 pandemic has induced a shift in biomedical publications toward COVID-19-related scientific production. The objective of the study is to show that scientific articles listing covid-related Medical Subject Headings (MeSH) when compared against covid-unrelated MeSH have been partially displaced. Specifically, we look at several indicators of scientific production in the life sciences before and after the start of the COVID-19 pandemic: (1) number of papers published, (2) impact factor weighted number of papers, (3) opens access, (4) number of publications related to clinical trials, (5) number of papers listing grants, (6) number of papers listing grants existing before the pandemic. Through a natural experiment approach, we analyze the impact of the pandemic on scientific production in the life sciences. We consider COVID-19 an unexpected and unprecedented exogenous source of variation with heterogeneous effects across biomedical research fields (i.e., MeSH terms).
Based on the difference in difference results, we document the displacement effect that the pandemic has had on several aspects of scientific publishing. The overall picture that emerges from this analysis is that there has been a profound realignment of priorities and research efforts. This shift has displaced biomedical research in fields not related to COVID-19.
The rest of the paper is structured as follows. First, we describe the data and our measure of relatedness to COVID-19. Next, we illustrate the difference-in-differences specification we rely on to identify the impact of the pandemic on scientific output. In the results section, we present the results of the difference-in-differences and network analyses. We document the sudden shift in publications, grants and trials towards COVID-19-related MeSH terms. Finally, we discuss the findings and highlight several policy implications.
Materials and methods
The present analysis is based primarily on PubMed and the Medical Subject Headings (MeSH) terminology. This data is used to estimate the effect of the start of the COVID 19 pandemic via a difference in difference approach. This section is structured as follows. We first introduce the data and then the econometric methodology. This analysis is not based on a pre-registered protocol.
Selection of biomedical publications.
We rely on PubMed, a repository with more than 34 million biomedical citations, for the analysis. Specifically, we analyze the daily updated files up to 31/06/2021, extracting all publications of type ‘Journal Article’. For the principal analysis, we consider 3,638,584 papers published from January 2019 to December 2020. We also analyze 11,122,017 papers published from 2010 onwards to identify the earliest usage of a grant and infer if it was new in 2020. We use the SCImago journal ranking statistics to compute the impact factor weighted number (IFWN) of papers in a given field of research. To assign the publication date, we use the ‘electronically published’ dates and, if missing, the ‘print published’ dates.
Medical subject headings.
We rely on the Medical Subject Headings (MeSH) terminology to approximate narrowly defined biomedical research fields. This terminology is a curated medical vocabulary, which is manually added to papers in the PubMed corpus. The fact that MeSH terms are manually annotated makes this terminology ideal for classification purposes. However, there is a delay between publication and annotation, on the order of several months. To address this delay and have the most recent classification, we search for all 28 425 MeSH terms using PubMed’s ESearch utility and classify paper by the results. The specific API endpoint is https://eutils.ncbi.nlm.nih.gov/entrez/eutils/esearch.fcgi , the relevant scripts are available with the code. For example, we assign the term ‘Ageusia’ (MeSH ID D000370) to all papers listed in the results of the ESearch API. We apply this method to the whole period (January 2019—December 2020) and obtain a mapping from papers to the MeSH terms. For every MeSH term, we keep track of the year they have been established. For instance, COVID-19 terms were established in 2020 (see Table 1 ): in January 2020, the WHO recommended 2019-nCoV and 2019-nCoV acute respiratory disease as provisional names for the virus and disease. The WHO issued the official terms COVID-19 and SARS-CoV-2 at the beginning of February 2020. By manually annotating publications, all publications referring to COVID-19 and SARS-CoV-2 since January 2020 have been labelled with the related MeSH terms. Other MeSH terms related to COVID-19, such as coronavirus, for instance, have been established years before the pandemic (see Table 2 ). We proxy MeSH term usage via search terms using the PubMed EUtilities API; this means that we are not using the hand-labelled MeSH terms but rather the PubMed search results. This means that the accuracy of the MeSH term we assign to a given paper is not perfect. In practice, this means that we have assigned more MeSH terms to a given term than a human annotator would have.
- PPT PowerPoint slide
- PNG larger image
- TIFF original image
https://doi.org/10.1371/journal.pone.0263001.t001
The list contains only terms with at least 100 publications in 2020.
https://doi.org/10.1371/journal.pone.0263001.t002
Clinical trials and publication types.
We classify publications using PubMed’s ‘PublicationType’ field in the XML baseline files (There are 187 publication types, see https://www.nlm.nih.gov/mesh/pubtypes.html ). We consider a publication to be related to a clinical trial if it lists any of the following descriptors:
- D016430: Clinical Trial
- D017426: Clinical Trial, Phase I
- D017427: Clinical Trial, Phase II
- D017428: Clinical Trial, Phase III
- D017429: Clinical Trial, Phase IV
- D018848: Controlled Clinical Trial
- D065007: Pragmatic Clinical Trial
- D000076362: Adaptive Clinical Trial
- D000077522: Clinical Trial, Veterinary
In our analysis of the impact of COVID-19 on publications related to clinical trials, we only consider MeSH terms that are associated at least once with a clinical trial publication over the two years. We apply this restriction to filter out MeSH terms that are very unlikely to be relevant for clinical trial types of research.
Open access.
We proxy the availability of a journal article to the public, i.e., open access, if it is available from PubMed Central. PubMed Central archives full-text journal articles and provides free access to the public. Note that the copyright license may vary across participating publishers. However, the text of the paper is for all effects and purposes freely available without requiring subscriptions or special affiliation.
We infer if a publication has been funded by checking if it lists any grants. We classify grants as either ‘old’, i.e. existed before 2019, or ‘new’, i.e. first observed afterwards. To do so, we collect all grant IDs for 11,122,017 papers from 2010 on-wards and record their first appearance. This procedure is an indirect inference of the year the grant has been granted. The basic assumption is that if a grant number has not been listed in any publication since 2010, it is very likely a new grant. Specifically, an old grant is a grant listed since 2019 observed at least once from 2010 to 2018.
Note that this procedure is only approximate and has a few shortcomings. Mistyped grant numbers (e.g. ‘1234-M JPN’ and ‘1234-M-JPN’) could appear as new grants, even though they existed before, or new grants might be classified as old grants if they have a common ID (e.g. ‘Grant 1’). Unfortunately, there is no central repository of grant numbers and the associated metadata; however, there are plans to assign DOI numbers to grants to alleviate this problem (See https://gitlab.com/crossref/open_funder_registry for the project).
Impact factor weighted publication numbers (IFWN).
In our analysis, we consider two measures of scientific output. First, we simply count the number of publications by MeSH term. However, since journals vary considerably in terms of impact factor, we also weigh the number of publications by the impact factor of the venue (e.g., journal) where it was published. Specifically, we use the SCImago journal ranking statistics to weigh a paper by the impact factor of the journal it appears in. We use the ‘citation per document in the past two years’ for 45,230 ISSNs. Note that a journal may and often has more than one ISSN, i.e., one for the printed edition and one for the online edition. SCImago applies the same score for a venue across linked ISSNs.
For the impact factor weighted number (IFWN) of publication per MeSH terms, this means that all publications are replaced by the impact score of the journal they appear in and summed up.
COVID-19-relatedness.
To measure how closely related to COVID-19 is a MeSH term, we introduce an index of relatedness to COVID-19. First, we identify the focal COVID-19 terms, which appeared in the literature in 2020 (see Table 1 ). Next, for all other pre-existing MeSH terms, we measure how closely related to COVID-19 they end up being.
Our aim is to show that MeSH terms that existed before and are related have experienced a sudden increase in the number of (impact factor weighted) papers.
Intuitively we can read this measure as: what is the probability in 2020 that a COVID-19 MeSH term is present given that we chose a paper with MeSH term i ? For example, given that in 2020 we choose a paper dealing with “Ageusia” (i.e., Complete or severe loss of the subjective sense of taste), there is a 96% probability that this paper also lists COVID-19, see Table 1 .
Note that a paper listing a related MeSH term does not imply that that paper is doing COVID-19 research, but it implies that one of the MeSH terms listed is often used in COVID-19 research.
In sum, in our analysis, we use the following variables:
- Papers: Number of papers by MeSH term;
- Impact: Impact factor weighted number of papers by MeSH term;
- PMC: Papers listed in PubMed central by MeSH term, as a measure of Open Access publications;
- Trials: number of publications of type “Clinical Trial” by MeSH term;
- Grants: number of papers with at least one grant by MeSH term;
- Old Grants: number of papers listing a grant that has been observed between 2010 and 2018, by MeSH term;
Difference-in-differences
The difference-in-differences (DiD) method is an econometric technique to imitate an experimental research design from observation data, sometimes referred to as a quasi-experimental setup. In a randomized controlled trial, subjects are randomly assigned either to the treated or the control group. Analogously, in this natural experiment, we assume that medical subject headings (MeSH) have been randomly assigned to be either treated (related) or not treated (unrelated) by the pandemic crisis.
Before the COVID, for a future health crisis, the set of potentially impacted medical knowledge was not predictable since it depended on the specifics of the emergency. For instance, ageusia (loss of taste), a medical concept existing since 1991, became known to be a specific symptom of COVID-19 only after the pandemic.
Specifically, we exploit the COVID-19 as an unpredictable and exogenous shock that has deeply affected the publication priorities for biomedical scientific production, as compared to the situation before the pandemic. In this setting, COVID-19 is the treatment, and the identification of this new human coronavirus is the event. We claim that treated MeSH terms, i.e., MeSH terms related to COVID-19, have experienced a sudden increase in terms of scientific production and attention. In contrast, research on untreated MeSH terms, i.e., MeSH terms not related to COVID-19, has been displaced by COVID-19. Our analysis compares the scientific output of COVID-19 related and unrelated MeSH terms before and after January 2020.
In our case, some of the terms turn out to be related to COVID-19 in 2020, whereas most of the MeSH terms are not closely related to COVID-19.
Thus β 1 identifies the overall effect on the control group after the event, β 2 the difference across treated and control groups before the event (i.e. the first difference in DiD) and finally the effect on the treated group after the event, net of the first difference, β 3 . This last parameter identifies the treatment effect on the treated group netting out the pre-treatment difference.
For the DiD to have a causal interpretation, it must be noted that pre-event, the trends of the two groups should be parallel, i.e., the common trend assumption (CTA) must be satisfied. We will show that the CTA holds in the results section.
To specify the DiD model, we need to define a period before and after the event and assign a treatment status or level of exposure to each term.
Before and after.
The pre-treatment period is defined as January 2019 to December 2019. The post-treatment period is defined as the months from January 2020 to December 2020. We argue that the state of biomedical research was similar in those two years, apart from the effect of the pandemic.
Treatment status and exposure.
The treatment is determined by the COVID-19 relatedness index σ i introduced earlier. Specifically, this number indicates the likelihood that COVID-19 will be a listed MeSH term, given that we observe the focal MeSH term i . To show that the effect becomes even stronger the closer related the subject is, and for ease of interpretation, we also discretize the relatedness value into three levels of treatment. Namely, we group MeSH terms with a σ between, 0% to 20%, 20% to 80% and 80% to 100%. The choice of alternative grouping strategies does not significantly affect our results. Results for alternative thresholds of relatedness can be computed using the available source code. We complement the dichotomized analysis by using the treatment intensity (relatedness measure σ ) to show that the result persists.
Panel regression.
In this work, we estimate a random effects panel regression where the units of analysis are 28 318 biomedical research fields (i.e. MeSH terms) observed over time before and after the COVID-19 pandemic. The time resolution is at the monthly level, meaning that for each MeSH term, we have 24 observations from January 2019 to December 2020.
The outcome variable Y it identifies the outcome at time t (i.e., month), for MeSH term i . As before, P t identifies the period with P t = 0 if the month is before January 2020 and P t = 1 if it is on or after this date. In (3) , the treatment level is measure by the relatedness to COVID-19 ( σ i ), where again the γ 1 identifies pre-trend (constant) differences and δ 1 the overall effect.
In total, we estimate six coefficients. As before, the δ l coefficient identifies the DiD effect.
Verifying the Common Trend Assumption (CTA).
We show that the CTA holds for this model by comparing the pre-event trends of the control group to the treated groups (COVID-19 related MeSH terms). Namely, we show that the pre-event trends of the control group are the same as the pre-event trends of the treated group.
Co-occurrence analysis
To investigate if the pandemic has caused a reconfiguration of research priorities, we look at the MeSH term co-occurrence network. Precisely, we extract the co-occurrence network of all 28,318 MeSH terms as they appear in the 3.3 million papers. We considered the co-occurrence networks of 2018, 2019 and 2020. Each node represents a MeSH term in these networks, and a link between them indicates that they have been observed at least once together. The weight of the edge between the MeSH terms is given by the number of times those terms have been jointly observed in the same publications.
Medical language is hugely complicated, and this simple representation does not capture the intricacies, subtle nuances and, in fact, meaning of the terms. Therefore, we do not claim that we can identify how the actual usage of MeSH terms has changed from this object, but rather that it has. Nevertheless, the co-occurrence graph captures rudimentary relations between concepts. We argue that absent a shock to the system, their basic usage patterns, change in importance (within the network) would essentially be the same from year to year. However, if we find that the importance of terms changes more than expected in 2020, it stands to reason that there have been some significant changes.
To show that that MeSH usage has been affected, we compute for each term in the years 2018, 2019 and 2020 their PageRank centrality [ 17 ]. The PageRank centrality tells us how likely a random walker traversing a network would be found at a given node if she follows the weights of the empirical edges (i.e., co-usage probability). Specifically, for the case of the MeSH co-occurrence network, this number represents how often an annotator at the National Library of Medicine would assign that MeSH term following the observed general usage patterns. It is a simplistic measure to capture the complexities of biomedical research. Nevertheless, it captures far-reaching interdependence across MeSH terms as the measure uses the whole network to determine the centrality of every MeSH term. A sudden change in the rankings and thus the position of MeSH terms in this network suggests that a given research subject has risen as it is used more often with other important MeSH terms (or vice versa).
We then compare the growth for each MeSH i term in g i (2019), i.e. before the the COVID-19 pandemic, with the growth after the event ( g i (2020)).
Publication growth
Changes in output and COVID-19 relatedness
Before we show the regression results, we provide descriptive evidence that publications from 2019 to 2020 have drastically increased. By showing that this growth correlates strongly with a MeSH term’s COVID-19 relatedness ( σ ), we demonstrate that (1) σ captures an essential aspect of the growth dynamics and (2) highlight the meteoric rise of highly related terms.
We look at the year over year growth in the number of the impact weighted number of publications per MeSH term from 2018 to 2019 and 2019 to 2020 as defined in the methods section.
Fig 1 shows the yearly growth of the impact weighted number of publications per MeSH term. By comparing the growth of the number of publications from the years 2018, 2019 and 2020, we find that the impact factor weighted number of publications has increased by up to a factor of 100 compared to the previous year for Betacoronavirus, one of the most closely related to COVID-19 MeSH term.
Each dot represents, a MeSH term. The y axis (growth) is in symmetric log scale. The x axis shows the COVID-19 relatedness, σ . Note that the position of the dots on the x-axis is the same in the two plots. Below: MeSH term importance gain (PageRank) and their COVID-19 relatedness.
https://doi.org/10.1371/journal.pone.0263001.g001
Fig 1 , first row, reveals how strongly correlated the growth in the IFWN of publication is to the term’s COVID-19 relatedness. For instance, we see that the term ‘Betacoronavirus’ skyrocketed from 2019 to 2020, which is expected given that SARS-CoV-2 is a species of the genus. Conversely, the term ‘Alphacoronavirus’ has not experienced any growth given that it is twin a genus of the Coronaviridae family, but SARS-CoV-2 is not one of its species. Note also the fast growth in the number of publications dealing with ‘Quarantine’. Moreover, MeSH terms that grew significantly from 2018 to 2019 and were not closely related to COVID-19, like ‘Vaping’, slowed down in 2020. From the graph, the picture emerges that publication growth is correlated with COVID-19 relatedness σ and that the growth for less related terms slowed down.
To show that the usage pattern of MeSH terms has changed following the pandemic, we compute the PageRank centrality using graph-tool [ 18 ] as discussed in the Methods section.
Fig 1 , second row, shows the change in the PageRank centrality of the MeSH terms after the pandemic (2019 to 2020, right plot) and before (2018 to 2019, left plot). If there were no change in the general usage pattern, we would expect the variance in PageRank changes to be narrow across the two periods, see (left plot). However, PageRank scores changed significantly more from 2019 to 2020 than from 2018 to 2019, suggesting that there has been a reconfiguration of the network.
To further support this argument, we carry out a DiD regression analysis.
Common trends assumption
As discussed in the Methods section, we need to show that the CTA assumption holds for the DiD to be defined appropriately. We do this by estimating for each month the number of publications and comparing it across treatment groups. This exercise also serves the purpose of a placebo test. By assuming that each month could have potentially been the event’s timing (i.e., the outbreak), we show that January 2020 is the most likely timing of the event. The regression table, as noted earlier, contains over 70 estimated coefficients, hence for ease of reading, we will only show the predicted outcome per month by group (see Fig 2 ). The full regression table with all coefficients is available in the S1 Table .
The y axis is in log scale. The dashed vertical line identifies January 2020. The dashed horizontal line shows the publications in January 2019 for the 0–20% group before the event. This line highlights that the drop happens after the event. The bands around the lines indicate the 95% confidence interval of the predicted values. The results are the output of the Stata margins command.
https://doi.org/10.1371/journal.pone.0263001.g002
Fig 2 shows the predicted number per outcome variable obtained from the panel regression model. These predictions correspond to the predicted value per relatedness group using the regression parameters estimated via the linear panel regression. The bands around the curves are the 95% confidence intervals.
All outcome measures depict a similar trend per month. Before the event (i.e., January 2020), there is a common trend across all groups. In contrast, after the event, we observe a sudden rise for the outcomes of the COVID-19 related treated groups (green and red lines) and a decline in the outcomes for the unrelated group (blue line). Therefore, we can conclude that the CTA assumption holds.
Regression results
Table 3 shows the DiD regression results (see Eq (3) ) for the selected outcome measures: number of publications (Papers), impact factor weighted number of publications (Impact), open access (OA) publications, clinical trial related publications, and publications with existing grants.
https://doi.org/10.1371/journal.pone.0263001.t003
Table 3 shows results for the discrete treatment level version of the DiD model (see Eq (4) ).
Note that the outcome variable is in natural log scale; hence to get the effect of the independent variable, we need to exponentiate the coefficient. For values close to 0, the effect is well approximated by the percentage change of that magnitude.
In both specifications we see that the least related group, drops in the number of publications between 10% and 13%, respectively (first row of Tables 3 and 4 , exp(−0.102) ≈ 0.87). In line with our expectations, the increase in the number of papers published by MeSH term is positively affected by the relatedness to COVID-19. In the discrete model (row 2), we note that the number of documents with MeSH terms with a COVID-19 relatedness between 20 and 80% grows by 18% and highly related terms by a factor of approximately 6.6 (exp(1.88)). The same general pattern can be observed for the impact weighted publication number, i.e., Model (2). Note, however, that the drop in the impact factor weighted output is more significant, reaching -19% for COVID-19 unrelated publications, and related publications growing by a factor of 8.7. This difference suggests that there might be a bias to publish papers on COVID-19 related subjects in high impact factor journals.
https://doi.org/10.1371/journal.pone.0263001.t004
By looking at the number of open access publications (PMC), we note that the least related group has not been affected negatively by the pandemic. However, the number of COVID-19 related publications has drastically increased for the most COVID-19 related group by a factor of 6.2. Note that the substantial increase in the number of papers available through open access is in large part due to journal and editorial policies to make preferentially COVID research immediately available to the public.
Regarding the number of clinical trial publications, we note that the least related group has been affected negatively, with the number of publications on clinical trials dropping by a staggering 24%. At the same time, publications on clinical trials for COVID-19-related MeSH have increased by a factor of 2.1. Note, however, that the effect on clinical trials is not significant in the continuous regression. The discrepancy across Tables 3 and 4 highlights that, especially for trials, the effect is not linear, where only the publications on clinical trials closely related to COVID-19 experiencing a boost.
It has been reported [ 19 ] that while the number of clinical trials registered to treat or prevent COVID-19 has surged with 179 new registrations in the second week of April 2020 alone. Only a few of these have led to publishable results in the 12 months since [ 20 ]. On the other hand, we find that clinical trial publications, considering related MeSH (but not COVID-19 directly), have had significant growth from the beginning of the pandemic. These results are not contradictory. Indeed counting the number of clinical trial publications listing the exact COVID-19 MeSH term (D000086382), we find 212 publications. While this might seem like a small number, consider that in 2020 only 8,485 publications were classified as clinical trials; thus, targeted trials still made up 2.5% of all clinical trials in 2020 . So while one might doubt the effectiveness of these research efforts, it is still the case that by sheer number, they represent a significant proportion of all publications on clinical trials in 2020. Moreover, COVID-19 specific Clinical trial publications in 2020, being a delayed signal of the actual trials, are a lower bound estimate on the true number of such clinical trials being conducted. This is because COVID-19 studies could only have commenced in 2020, whereas other studies had a head start. Thus our reported estimates are conservative, meaning that the true effect on actual clinical trials is likely larger, not smaller.
Research funding, as proxied by the number of publications with grants, follows a similar pattern, but notably, COVID-19-related MeSH terms list the same proportion of grants established before 2019 as other unrelated MeSH terms, suggesting that grants which were not designated for COVID-19 research have been used to support COVID-19 related research. Overall, the number of publications listing a grant has dropped. Note that this should be because the number of publications overall in the unrelated group has dropped. However, we note that the drop in publications is 10% while the decline in publications with at least one grant is 15%. This difference suggests that publications listing grants, which should have more funding, are disproportionately COVID-19 related papers. To further investigate this aspect, we look at whether the grant was old (pre-2019) or appeared for the first time in or after 2019. It stands to reason that an old grant (pre-2019) would not have been granted for a project dealing with the pandemic. Hence we would expect that COVID-19 related MeSH terms to have a lower proportion of old grants than the unrelated group. In models (6) in Table 4 we show that the number of old grants for the unrelated group drops by 13%. At the same time, the number of papers listing old grants (i.e., pre-2019) among the most related group increased by a factor of 3.1. Overall, these results suggest that COVID-19 related research has been funded largely by pre-existing grants, even though a specific mandate tied to the grants for this use is unlikely.
The scientific community has swiftly reallocated research efforts to cope with the COVID-19 pandemic, mobilizing knowledge across disciplines to find innovative solutions in record time. We document this both in terms of changing trends in the biomedical scientific output and the usage of MeSH terms by the scientific community. The flip side of this sudden and energetic prioritization of effort to fight COVID-19 has been a sudden contraction of scientific production in other relevant research areas. All in all, we find strong support to the hypotheses that the COVID-19 crisis has induced a sudden increase of research output in COVID-19 related areas of biomedical research. Conversely, research in areas not related to COVID-19 has experienced a significant drop in overall publishing rates and funding.
Our paper contributes to the literature on the impact of COVID-19 on scientific research: we corroborate previous findings about the surge of COVID-19 related publications [ 1 – 3 ], partially displacing research in COVID-19 unrelated fields of research [ 4 , 14 ], particularly research related to clinical trials [ 5 – 7 ]. The drop in trial research might have severe consequences for patients affected by life-threatening diseases since it will delay access to new and better treatments. We also confirm the impact of COVID-19 on open access publication output [ 1 ]; also, this is milder than traditional outlets. On top of this, we provide more robust evidence on the impact weighted effect of COVID-19 and grant financed research, highlighting the strong displacement effect of COVID-19 on the allocation of financial resources [ 15 ]. We document a substantial change in the usage patterns of MeSH terms, suggesting that there has been a reconfiguration in the way research terms are being combined. MeSH terms highly related to COVID-19 were peripheral in the MeSH usage networks before the pandemic but have become central since 2020. We conclude that the usage patterns have changed, with COVID-19 related MeSH terms occupying a much more prominent role in 2020 than they did in the previous years.
We also contribute to the literature by estimating the effect of COVID-19 on biomedical research in a natural experiment framework, isolating the specific effects of the COVID-19 pandemic on the biomedical scientific landscape. This is crucial to identify areas of public intervention to sustain areas of biomedical research which have been neglected during the COVID-19 crisis. Moreover, the exploratory analysis on the changes in usage patterns of MeSH terms, points to an increase in the importance of covid-related topics in the broader biomedical research landscape.
Our results provide compelling evidence that research related to COVID-19 has indeed displaced scientific production in other biomedical fields of research not related to COVID-19, with a significant drop in (impact weighted) scientific output related to non-COVID-19 and a marked reduction of financial support for publications not related to COVID-19 [ 4 , 5 , 16 ]. The displacement effect is persistent to the end of 2020. As vaccination progresses, we highlight the urgent need for science policy to re-balance support for research activity that was put on pause because of the COVID-19 pandemic.
We find that COVID-19 dramatically impacted clinical research. Reactivation of clinical trials activities that have been postponed or suspended for reasons related to COVID-19 is a priority that should be considered in the national vaccination plans. Moreover, since grants have been diverted and financial incentives have been targeted to sustain COVID-19 research leading to an excessive entry in COVID-19-related clinical trials and the ‘covidisation’ of research, there is a need to reorient incentives to basic research and otherwise neglected or temporally abandoned areas of biomedical research. Without dedicated support in the recovery plans for neglected research of the COVID-19 era, there is a risk that more medical needs will be unmet in the future, possibly exacerbating the shortage of scientific research for orphan and neglected diseases, which do not belong to COVID-19-related research areas.
Limitations
Our empirical approach has some limits. First, we proxy MeSH term usage via search terms using the PubMed EUtilities API. This means that the accuracy of the MeSH term we assign to a given paper is not fully validated. More time is needed for the completion of manually annotated MeSH terms. Second, the timing of publication is not the moment the research has been carried out. There is a lead time between inception, analysis, write-up, review, revision, and final publication. This delay varies across disciplines. Nevertheless, given that the surge in publications happens around the alleged event date, January 2020, we are confident that the publication date is a reasonable yet imperfect estimate of the timing of the research. Third, several journals have publicly declared to fast-track COVID-19 research. This discrepancy in the speed of publication of COVID-19 related research and other research could affect our results. Specifically, a surge or displacement could be overestimated due to a lag in the publication of COVID-19 unrelated research. We alleviate this bias by estimating the effect considering a considerable time after the event (January 2020 to December 2020). Forth, on the one hand, clinical Trials may lead to multiple publications. Therefore we might overestimate the impact of COVID-19 on the number of clinical trials. On the other hand, COVID-19 publications on clinical trials lag behind, so the number of papers related COVID-19 trials is likely underestimated. Therefore, we note that the focus of this paper is scientific publications on clinical trials rather than on actual clinical trials. Fifth, regarding grants, unfortunately, there is no unique centralized repository mapping grant numbers to years, so we have to proxy old grants with grants that appeared in publications from 2010 to 2018. Besides, grant numbers are free-form entries, meaning that PubMed has no validation step to disambiguate or verify that the grant number has been entered correctly. This has the effect of classifying a grant as new even though it has appeared under a different name. We mitigate this problem by using a long period to collect grant numbers and catch many spellings of the same grant, thereby reducing the likelihood of miss-identifying a grant as new when it existed before. Still, unless unique identifiers are widely used, there is no way to verify this.
So far, there is no conclusive evidence on whether entry into COVID-19 has been excessive. However, there is a growing consensus that COVID-19 has displaced, at least temporally, scientific research in COVID-19 unrelated biomedical research areas. Even though it is certainly expected that more attention will be devoted to the emergency during a pandemic, the displacement of biomedical research in other fields is concerning. Future research is needed to investigate the long-run structural consequences of the COVID-19 crisis on biomedical research.
Supporting information
S1 table. common trend assumption (cta) regression table..
Full regression table with all controls and interactions.
https://doi.org/10.1371/journal.pone.0263001.s001
- View Article
- Google Scholar
- PubMed/NCBI
- 8. Brown A, Edwin E, Fagg J. Evaluate Pharma 2021 Preview; 2020. https://www.evaluate.com/thought-leadership/vantage/evaluate-vantage-2021-preview .
- 15. Hook D, Porter S. The COVID Brain Drain; 2020. https://www.natureindex.com/news-blog/covid-brain-drain-scientific-research .
- 17. Page L, Brin S, Motwani R, Winograd T. The PageRank citation ranking: Bringing order to the web. Stanford InfoLab; 1999.
- Frontiers in Psychology
- Personality and Social Psychology
- Research Topics
Coronavirus Disease (COVID-19): The Impact and Role of Mass Media During the Pandemic
Total Downloads
Total Views and Downloads
About this Research Topic
The outbreak of coronavirus disease 2019 (COVID-19) has created a global health crisis that has had a deep impact on the way we perceive our world and our everyday lives. Not only the rate of contagion and patterns of transmission threatens our sense of agency, but the safety measures put in place to contain ...
Keywords : COVID-19, coronavirus disease, mass media, health communication, prevention, intervention, social behavioral changes
Important Note : All contributions to this Research Topic must be within the scope of the section and journal to which they are submitted, as defined in their mission statements. Frontiers reserves the right to guide an out-of-scope manuscript to a more suitable section or journal at any stage of peer review.
Topic Editors
Topic coordinators, recent articles, submission deadlines.
Submission closed.
Participating Journals
Total views.
- Demographics
No records found
total views article views downloads topic views
Top countries
Top referring sites, about frontiers research topics.
With their unique mixes of varied contributions from Original Research to Review Articles, Research Topics unify the most influential researchers, the latest key findings and historical advances in a hot research area! Find out more on how to host your own Frontiers Research Topic or contribute to one as an author.
- All Publications
- Priorities Magazine Spring 2018
- The Next Plague and How Science Will Stop It
- Priorities Magazine Winter 2018
- Priorities Magazine Fall 2017
- Little Black Book of Junk Science
- Priorities Magazine Winter 2017
- Should You Worry About Artificial Flavors Or Colors?
- Should You Worry About Artificial Sweeteners?
- Summer Health and Safety Tips
- How Toxic Terrorists Scare You With Science Terms
- Adult Immunization: The Need for Enhanced Utilization
- Should You Worry About Salt?
- Priorities Magazine Spring 2016
- IARC Diesel Exhaust & Lung Cancer: An Analysis
- Teflon and Human Health: Do the Charges Stick?
- Helping Smokers Quit: The Science Behind Tobacco Harm Reduction
- Irradiated Foods
- Foods Are Not Cigarettes: Why Tobacco Lawsuits Are Not a Model for Obesity Lawsuits
- The Prevention and Treatment of Osteoporosis: A Review
- Are "Low Dose" Health Effects of Chemicals Real?
- The Effects of Nicotine on Human Health
- Traditional Holiday Dinner Replete with Natural Carcinogens - Even Organic Thanksgiving Dinners
- A Primer On Dental Care: Quality and Quackery
- Nuclear Energy and Health And the Benefits of Low-Dose Radiation Hormesis
- Priorities in Caring for Your Children: A Primer for Parents
- Endocrine Disrupters: A Scientific Perspective
- Good Stories, Bad Science: A Guide for Journalists to the Health Claims of "Consumer Activist" Groups
- A Comparison of the Health Effects of Alcohol Consumption and Tobacco Use in America
- Moderate Alcohol Consumption and Health
- Irradiated Foods Fifth Edition
- Media/Contact
- Write For Us
Our COVID-19 Research Summary - 2021
The published literature on COVID now exceeds 211,000 papers, books, and documents, which include: 22,866 observational studies, 19,591 reviews, 1496 meta-analyses and 781 randomized control trials. These publications comprise the backdrop for our research and writing. The project began in the spring of 2020 based on a limited source of cumulative COVID-19 data and has broadened considerably. Here is what we have learned.
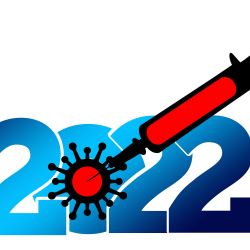
Our objectives remain to
- Describe trends and the geographic extent of the pandemic, including associated predictors
- Evaluate the effectiveness of vaccinations and exposure limitations.
- Provide public health perspectives.
“We know that people’s behavior, the mode of transmission, and the virus‘s characteristics all play a role but we don’t have a clear quantitative understanding of how all of these forces interact. With COVID, the biggest wild card has been human behavior.”
Dr. R. Rosenfeld , Head, Department of Machine Learning, Carnegie Mellon
Our findings along with other COVID studies are observational , focusing on numbers rather than people. Since the annual COVID mortality rate is about is only about 1 in 700, achieving reliable statistics by state would require a cohort approaching a million subjects, each of which would have to be tracked over time in order to estimate exposures. Observational studies are thus the only practical option and uncertainties about causality vs. association are inevitable.
COVID-19 trends continue to defy analysis in large part because of unpredictable variants, the latest of which is still unfolding. However, some aspects remain and will continue to dominate:
- Daily cases, deaths, and incidence of long-haul COVID can be reduced more than 10-fold by vaccination, notwithstanding deterioration over time that requires boosting.
- Acceptance of vaccination remains a personal choice - a choice that may be associated with personal characteristics including income, education, and political perspective.
- COVID-19 comprises a major cause of death in the United States and may continue to do so.
Findings In 2021 .
January . We showed that COVID-19 cases increased 10-fold, by 30% per month, during the 2020-21 winter; deaths generally followed suit, while case fatality rates (CFRs) decreased up to 10-fold. The Northeast region had shifted from worst to best, so that urban predictor variables like population density were no longer important. Regional rates coalesced in January.
February . The regional trend analyses showed declining cases but not deaths, with steady CFRs. We reported that “No plausible hypotheses have been advanced for the order-of-magnitude increases in cases and deaths since September”, now referred to as the “winter surge”. We compared urban and rural rates and noted the shift towards higher cases, deaths, and CFRs in rural areas.
March . We found that cases and deaths declined while CFRs increased 5-fold. Regional trends, which had ranged 4-fold for cases and deaths now coalesced. We analyzed short-term deaths and found a strong day-of-week effect, probably due to reporting error, but no evidence of important holiday surges.
April . We tried explaining the cyclical behavior of cases in terms of “susceptibles;” predicting an underlying trend of 5000 new cases per million per month. By contrast, the average case rate is now about 8000 cases per million per month - 53 million cases in total. However, after vaccinations got underway and prior to the Delta variant, new cases dropped to levels similar to those at the beginning of the pandemic. CFR’s ranged from about 0.015 in northern regions to 0.05 in the Southwest by April. We showed that Caucasian and mixed-race subjects had far lower COVID death rates than persons of color, and that COVID death rates increased with age at the same rate as non-COVID deaths.
May . COVID rates remained low in May. Comparing states, we reported significant relationships between COVID rates and political preference along with situational factors like household crowding. An increase in Republican voters of 60 percentage points, used as a marker of political perspective, was associated with a doubling of cumulative cases.
June . We revisited our previous consideration of airborne virus transmission, which had been espoused by CDC and the epidemiological community. We estimated ventilation rates and concluded that exposures in a small apartment were likely worse than in subways or aircraft. We also revisited urban-rural differences in more detail and showed that regional COVID rates had continued to coalesce.
July . We did a detailed analysis of vaccination rates and benefits. Daily vaccination rates peaked in April, at about 50% higher in the Northeast than elsewhere. We showed a strong significant decrease in daily state-level cases associated with full vaccination. We estimated unvaccinated case rates to be hundred-folds higher than with full vaccinations. We compared vaccination effects with education, and air pollution concluding that such personal characteristics could also be important. We also showed a negative state-level relationship between voting Republican and cumulative vaccination rates. Interestingly, vaccination rates correlated with COVID rates in 2020 before mass vaccinations began, and vaccinations at this time, also apparently, reduced mortality not associated with COVID. “ Could the decision to vaccinate have been more critical than the vaccination itself? ”
August . We reported that COVID case rates showed a sharp upturn, followed by death, likely due to the arrival of the Delta variant. Death rates and cases had decreased steadily until July to about 30 per million or 10,000 per day – a level the CDC considered as a “tolerable” endemic. We have not had these low levels since then. CFRs peaked in July, growing six-fold with substantial geographic variability.
September . We examined cyclical variations in daily infection rates and found substantial heterogeneity. State-level vaccination rates predicted both cases and death; and complete vaccination decreased case and death rates about 100-fold, even in the presence of the Delta variant.
October . We compared October’s COVID rates with those of the 2018-19 influenza to obtain a public health perspective. Total COVID-19 and influenza cases were similar at about 30 million and both were controlled by vaccination. Compare to influenza, COVID hospitalizations were 4-fold higher and deaths were 20-fold higher - COVID is clearly the more serious disease. We concluded that 178,000 lives may have been saved by COVID-19 vaccination.
November . We continued examining vaccine effects and found no difference in the real-world effectiveness of Pfizer or Moderna vaccines. We found that COVID vaccinations were associated with reduced non-COVID deaths by 3-fold. We built an empirical mathematical model of the temporal variation of cases that fit the existing data very well but grossly underestimated the current situation. We predicted that full vaccinations for the U.S. might reach 72% in the next year, but with a range of 50-90% among states.
December . Cases began a sharp upward trend at years end, with deaths lagging behind. Regional gradients shifted, with Northwest highest and Northeast lowest. Vaccination rates continued to increase slowly, led by the Northeast. Previous beneficial effects of vaccination had been overshadowed by the severity of the Delta variant. We reported that vaccine effectiveness appears to decrease substantially over time. Long-haul COVID, neglected by the epidemiology community, was inversely associated with vaccination rates and the socioeconomic factors underlying vaccine reluctance or refusal. We estimated trends and the contributions of immunity acquired from previous infection, which we found to be statistically modest.
View the discussion thread.
By Fred Lipfert, PhD
Latest from Fred Lipfert, PhD :
Greater Good Science Center • Magazine • In Action • In Education
Society Articles & More
11 questions to ask about covid-19 research, how can you tell if a scientific study about the pandemic is valid and useful we have some tips..
Debates have raged on social media, around dinner tables, on TV, and in Congress about the science of COVID-19. Is it really worse than the flu? How necessary are lockdowns? Do masks work to prevent infection? What kinds of masks work best? Is the new vaccine safe?
You might see friends, relatives, and coworkers offer competing answers, often brandishing studies or citing individual doctors and scientists to support their positions. With so much disagreement—and with such high stakes—how can we use science to make the best decisions?
Here at Greater Good , we cover research into social and emotional well-being, and we try to help people apply findings to their personal and professional lives. We are well aware that our business is a tricky one.
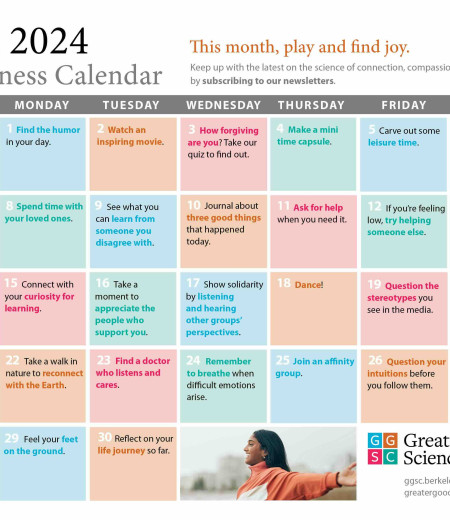
Summarizing scientific studies and distilling the key insights that people can apply to their lives isn’t just difficult for the obvious reasons, like understanding and then explaining formal science terms or rigorous empirical and analytic methods to non-specialists. It’s also the case that context gets lost when we translate findings into stories, tips, and tools, especially when we push it all through the nuance-squashing machine of the Internet. Many people rarely read past the headlines, which intrinsically aim to be relatable and provoke interest in as many people as possible. Because our articles can never be as comprehensive as the original studies, they almost always omit some crucial caveats, such as limitations acknowledged by the researchers. To get those, you need access to the studies themselves.
And it’s very common for findings and scientists to seem to contradict each other. For example, there were many contradictory findings and recommendations about the use of masks, especially at the beginning of the pandemic—though as we’ll discuss, it’s important to understand that a scientific consensus did emerge.
Given the complexities and ambiguities of the scientific endeavor, is it possible for a non-scientist to strike a balance between wholesale dismissal and uncritical belief? Are there red flags to look for when you read about a study on a site like Greater Good or hear about one on a Fox News program? If you do read an original source study, how should you, as a non-scientist, gauge its credibility?
Here are 11 questions you might ask when you read about the latest scientific findings about the pandemic, based on our own work here at Greater Good.
1. Did the study appear in a peer-reviewed journal?
In peer review, submitted articles are sent to other experts for detailed critical input that often must be addressed in a revision prior to being accepted and published. This remains one of the best ways we have for ascertaining the rigor of the study and rationale for its conclusions. Many scientists describe peer review as a truly humbling crucible. If a study didn’t go through this process, for whatever reason, it should be taken with a much bigger grain of salt.
“When thinking about the coronavirus studies, it is important to note that things were happening so fast that in the beginning people were releasing non-peer reviewed, observational studies,” says Dr. Leif Hass, a family medicine doctor and hospitalist at Sutter Health’s Alta Bates Summit Medical Center in Oakland, California. “This is what we typically do as hypothesis-generating but given the crisis, we started acting on them.”
In a confusing, time-pressed, fluid situation like the one COVID-19 presented, people without medical training have often been forced to simply defer to expertise in making individual and collective decisions, turning to culturally vetted institutions like the Centers for Disease Control (CDC). Is that wise? Read on.
2. Who conducted the study, and where did it appear?
“I try to listen to the opinion of people who are deep in the field being addressed and assess their response to the study at hand,” says Hass. “With the MRNA coronavirus vaccines, I heard Paul Offit from UPenn at a UCSF Grand Rounds talk about it. He literally wrote the book on vaccines. He reviewed what we know and gave the vaccine a big thumbs up. I was sold.”
From a scientific perspective, individual expertise and accomplishment matters—but so does institutional affiliation.
Why? Because institutions provide a framework for individual accountability as well as safety guidelines. At UC Berkeley, for example , research involving human subjects during COVID-19 must submit a Human Subjects Proposal Supplement Form , and follow a standard protocol and rigorous guidelines . Is this process perfect? No. It’s run by humans and humans are imperfect. However, the conclusions are far more reliable than opinions offered by someone’s favorite YouTuber .
Recommendations coming from institutions like the CDC should not be accepted uncritically. At the same time, however, all of us—including individuals sporting a “Ph.D.” or “M.D.” after their names—must be humble in the face of them. The CDC represents a formidable concentration of scientific talent and knowledge that dwarfs the perspective of any one individual. In a crisis like COVID-19, we need to defer to that expertise, at least conditionally.
“If we look at social media, things could look frightening,” says Hass. When hundreds of millions of people are vaccinated, millions of them will be afflicted anyway, in the course of life, by conditions like strokes, anaphylaxis, and Bell’s palsy. “We have to have faith that people collecting the data will let us know if we are seeing those things above the baseline rate.”
3. Who was studied, and where?
Animal experiments tell scientists a lot, but their applicability to our daily human lives will be limited. Similarly, if researchers only studied men, the conclusions might not be relevant to women, and vice versa.
Many psychology studies rely on WEIRD (Western, educated, industrialized, rich and democratic) participants, mainly college students, which creates an in-built bias in the discipline’s conclusions. Historically, biomedical studies also bias toward gathering measures from white male study participants, which again, limits generalizability of findings. Does that mean you should dismiss Western science? Of course not. It’s just the equivalent of a “Caution,” “Yield,” or “Roadwork Ahead” sign on the road to understanding.
This applies to the coronavirus vaccines now being distributed and administered around the world. The vaccines will have side effects; all medicines do. Those side effects will be worse for some people than others, depending on their genetic inheritance, medical status, age, upbringing, current living conditions, and other factors.
For Hass, it amounts to this question: Will those side effects be worse, on balance, than COVID-19, for most people?
“When I hear that four in 100,000 [of people in the vaccine trials] had Bell’s palsy, I know that it would have been a heck of a lot worse if 100,000 people had COVID. Three hundred people would have died and many others been stuck with chronic health problems.”
4. How big was the sample?
In general, the more participants in a study, the more valid its results. That said, a large sample is sometimes impossible or even undesirable for certain kinds of studies. During COVID-19, limited time has constrained the sample sizes.
However, that acknowledged, it’s still the case that some studies have been much larger than others—and the sample sizes of the vaccine trials can still provide us with enough information to make informed decisions. Doctors and nurses on the front lines of COVID-19—who are now the very first people being injected with the vaccine—think in terms of “biological plausibility,” as Hass says.
Did the admittedly rushed FDA approval of the Pfizer-BioNTech vaccine make sense, given what we already know? Tens of thousands of doctors who have been grappling with COVID-19 are voting with their arms, in effect volunteering to be a sample for their patients. If they didn’t think the vaccine was safe, you can bet they’d resist it. When the vaccine becomes available to ordinary people, we’ll know a lot more about its effects than we do today, thanks to health care providers paving the way.
5. Did the researchers control for key differences, and do those differences apply to you?
Diversity or gender balance aren’t necessarily virtues in experimental research, though ideally a study sample is as representative of the overall population as possible. However, many studies use intentionally homogenous groups, because this allows the researchers to limit the number of different factors that might affect the result.
While good researchers try to compare apples to apples, and control for as many differences as possible in their analyses, running a study always involves trade-offs between what can be accomplished as a function of study design, and how generalizable the findings can be.

The Science of Happiness
What does it take to live a happier life? Learn research-tested strategies that you can put into practice today. Hosted by award-winning psychologist Dacher Keltner. Co-produced by PRX and UC Berkeley’s Greater Good Science Center.
- Apple Podcasts
- Google Podcasts
You also need to ask if the specific population studied even applies to you. For example, when one study found that cloth masks didn’t work in “high-risk situations,” it was sometimes used as evidence against mask mandates.
However, a look beyond the headlines revealed that the study was of health care workers treating COVID-19 patients, which is a vastly more dangerous situation than, say, going to the grocery store. Doctors who must intubate patients can end up being splattered with saliva. In that circumstance, one cloth mask won’t cut it. They also need an N95, a face shield, two layers of gloves, and two layers of gown. For the rest of us in ordinary life, masks do greatly reduce community spread, if as many people as possible are wearing them.
6. Was there a control group?
One of the first things to look for in methodology is whether the population tested was randomly selected, whether there was a control group, and whether people were randomly assigned to either group without knowing which one they were in. This is especially important if a study aims to suggest that a certain experience or treatment might actually cause a specific outcome, rather than just reporting a correlation between two variables (see next point).
For example, were some people randomly assigned a specific meditation practice while others engaged in a comparable activity or exercise? If the sample is large enough, randomized trials can produce solid conclusions. But, sometimes, a study will not have a control group because it’s ethically impossible. We can’t, for example, let sick people go untreated just to see what would happen. Biomedical research often makes use of standard “treatment as usual” or placebos in control groups. They also follow careful ethical guidelines to protect patients from both maltreatment and being deprived necessary treatment. When you’re reading about studies of masks, social distancing, and treatments during the COVID-19, you can partially gauge the reliability and validity of the study by first checking if it had a control group. If it didn’t, the findings should be taken as preliminary.
7. Did the researchers establish causality, correlation, dependence, or some other kind of relationship?
We often hear “Correlation is not causation” shouted as a kind of battle cry, to try to discredit a study. But correlation—the degree to which two or more measurements seem connected—is important, and can be a step toward eventually finding causation—that is, establishing a change in one variable directly triggers a change in another. Until then, however, there is no way to ascertain the direction of a correlational relationship (does A change B, or does B change A), or to eliminate the possibility that a third, unmeasured factor is behind the pattern of both variables without further analysis.
In the end, the important thing is to accurately identify the relationship. This has been crucial in understanding steps to counter the spread of COVID-19 like shelter-in-place orders. Just showing that greater compliance with shelter-in-place mandates was associated with lower hospitalization rates is not as conclusive as showing that one community that enacted shelter-in-place mandates had lower hospitalization rates than a different community of similar size and population density that elected not to do so.
We are not the first people to face an infection without understanding the relationships between factors that would lead to more of it. During the bubonic plague, cities would order rodents killed to control infection. They were onto something: Fleas that lived on rodents were indeed responsible. But then human cases would skyrocket.
Why? Because the fleas would migrate off the rodent corpses onto humans, which would worsen infection. Rodent control only reduces bubonic plague if it’s done proactively; once the outbreak starts, killing rats can actually make it worse. Similarly, we can’t jump to conclusions during the COVID-19 pandemic when we see correlations.
8. Are journalists and politicians, or even scientists, overstating the result?
Language that suggests a fact is “proven” by one study or which promotes one solution for all people is most likely overstating the case. Sweeping generalizations of any kind often indicate a lack of humility that should be a red flag to readers. A study may very well “suggest” a certain conclusion but it rarely, if ever, “proves” it.
This is why we use a lot of cautious, hedging language in Greater Good , like “might” or “implies.” This applies to COVID-19 as well. In fact, this understanding could save your life.
When President Trump touted the advantages of hydroxychloroquine as a way to prevent and treat COVID-19, he was dramatically overstating the results of one observational study. Later studies with control groups showed that it did not work—and, in fact, it didn’t work as a preventative for President Trump and others in the White House who contracted COVID-19. Most survived that outbreak, but hydroxychloroquine was not one of the treatments that saved their lives. This example demonstrates how misleading and even harmful overstated results can be, in a global pandemic.
9. Is there any conflict of interest suggested by the funding or the researchers’ affiliations?
A 2015 study found that you could drink lots of sugary beverages without fear of getting fat, as long as you exercised. The funder? Coca Cola, which eagerly promoted the results. This doesn’t mean the results are wrong. But it does suggest you should seek a second opinion : Has anyone else studied the effects of sugary drinks on obesity? What did they find?
It’s possible to take this insight too far. Conspiracy theorists have suggested that “Big Pharma” invented COVID-19 for the purpose of selling vaccines. Thus, we should not trust their own trials showing that the vaccine is safe and effective.
But, in addition to the fact that there is no compelling investigative evidence that pharmaceutical companies created the virus, we need to bear in mind that their trials didn’t unfold in a vacuum. Clinical trials were rigorously monitored and independently reviewed by third-party entities like the World Health Organization and government organizations around the world, like the FDA in the United States.
Does that completely eliminate any risk? Absolutely not. It does mean, however, that conflicts of interest are being very closely monitored by many, many expert eyes. This greatly reduces the probability and potential corruptive influence of conflicts of interest.
10. Do the authors reference preceding findings and original sources?
The scientific method is based on iterative progress, and grounded in coordinating discoveries over time. Researchers study what others have done and use prior findings to guide their own study approaches; every study builds on generations of precedent, and every scientist expects their own discoveries to be usurped by more sophisticated future work. In the study you are reading, do the researchers adequately describe and acknowledge earlier findings, or other key contributions from other fields or disciplines that inform aspects of the research, or the way that they interpret their results?
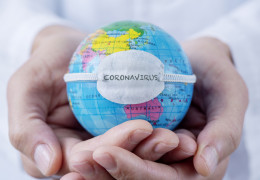
Greater Good’s Guide to Well-Being During Coronavirus
Practices, resources, and articles for individuals, parents, and educators facing COVID-19
This was crucial for the debates that have raged around mask mandates and social distancing. We already knew quite a bit about the efficacy of both in preventing infections, informed by centuries of practical experience and research.
When COVID-19 hit American shores, researchers and doctors did not question the necessity of masks in clinical settings. Here’s what we didn’t know: What kinds of masks would work best for the general public, who should wear them, when should we wear them, were there enough masks to go around, and could we get enough people to adopt best mask practices to make a difference in the specific context of COVID-19 ?
Over time, after a period of confusion and contradictory evidence, those questions have been answered . The very few studies that have suggested masks don’t work in stopping COVID-19 have almost all failed to account for other work on preventing the disease, and had results that simply didn’t hold up. Some were even retracted .
So, when someone shares a coronavirus study with you, it’s important to check the date. The implications of studies published early in the pandemic might be more limited and less conclusive than those published later, because the later studies could lean on and learn from previously published work. Which leads us to the next question you should ask in hearing about coronavirus research…
11. Do researchers, journalists, and politicians acknowledge limitations and entertain alternative explanations?
Is the study focused on only one side of the story or one interpretation of the data? Has it failed to consider or refute alternative explanations? Do they demonstrate awareness of which questions are answered and which aren’t by their methods? Do the journalists and politicians communicating the study know and understand these limitations?
When the Annals of Internal Medicine published a Danish study last month on the efficacy of cloth masks, some suggested that it showed masks “make no difference” against COVID-19.
The study was a good one by the standards spelled out in this article. The researchers and the journal were both credible, the study was randomized and controlled, and the sample size (4,862 people) was fairly large. Even better, the scientists went out of their way to acknowledge the limits of their work: “Inconclusive results, missing data, variable adherence, patient-reported findings on home tests, no blinding, and no assessment of whether masks could decrease disease transmission from mask wearers to others.”
Unfortunately, their scientific integrity was not reflected in the ways the study was used by some journalists, politicians, and people on social media. The study did not show that masks were useless. What it did show—and what it was designed to find out—was how much protection masks offered to the wearer under the conditions at the time in Denmark. In fact, the amount of protection for the wearer was not large, but that’s not the whole picture: We don’t wear masks mainly to protect ourselves, but to protect others from infection. Public-health recommendations have stressed that everyone needs to wear a mask to slow the spread of infection.
“We get vaccinated for the greater good, not just to protect ourselves ”
As the authors write in the paper, we need to look to other research to understand the context for their narrow results. In an editorial accompanying the paper in Annals of Internal Medicine , the editors argue that the results, together with existing data in support of masks, “should motivate widespread mask wearing to protect our communities and thereby ourselves.”
Something similar can be said of the new vaccine. “We get vaccinated for the greater good, not just to protect ourselves,” says Hass. “Being vaccinated prevents other people from getting sick. We get vaccinated for the more vulnerable in our community in addition for ourselves.”
Ultimately, the approach we should take to all new studies is a curious but skeptical one. We should take it all seriously and we should take it all with a grain of salt. You can judge a study against your experience, but you need to remember that your experience creates bias. You should try to cultivate humility, doubt, and patience. You might not always succeed; when you fail, try to admit fault and forgive yourself.
Above all, we need to try to remember that science is a process, and that conclusions always raise more questions for us to answer. That doesn’t mean we never have answers; we do. As the pandemic rages and the scientific process unfolds, we as individuals need to make the best decisions we can, with the information we have.
This article was revised and updated from a piece published by Greater Good in 2015, “ 10 Questions to Ask About Scientific Studies .”
About the Authors

Jeremy Adam Smith
Uc berkeley.
Jeremy Adam Smith edits the GGSC’s online magazine, Greater Good . He is also the author or coeditor of five books, including The Daddy Shift , Are We Born Racist? , and (most recently) The Gratitude Project: How the Science of Thankfulness Can Rewire Our Brains for Resilience, Optimism, and the Greater Good . Before joining the GGSC, Jeremy was a John S. Knight Journalism Fellow at Stanford University.

Emiliana R. Simon-Thomas
Emiliana R. Simon-Thomas, Ph.D. , is the science director of the Greater Good Science Center, where she directs the GGSC’s research fellowship program and serves as a co-instructor of its Science of Happiness and Science of Happiness at Work online courses.
You May Also Enjoy
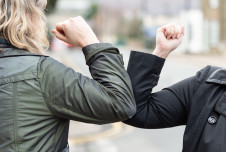
This article — and everything on this site — is funded by readers like you.
Become a subscribing member today. Help us continue to bring “the science of a meaningful life” to you and to millions around the globe.
- Research article
- Open access
- Published: 15 April 2024
What is quality in long covid care? Lessons from a national quality improvement collaborative and multi-site ethnography
- Trisha Greenhalgh ORCID: orcid.org/0000-0003-2369-8088 1 ,
- Julie L. Darbyshire 1 ,
- Cassie Lee 2 ,
- Emma Ladds 1 &
- Jenny Ceolta-Smith 3
BMC Medicine volume 22 , Article number: 159 ( 2024 ) Cite this article
1400 Accesses
64 Altmetric
Metrics details
Long covid (post covid-19 condition) is a complex condition with diverse manifestations, uncertain prognosis and wide variation in current approaches to management. There have been calls for formal quality standards to reduce a so-called “postcode lottery” of care. The original aim of this study—to examine the nature of quality in long covid care and reduce unwarranted variation in services—evolved to focus on examining the reasons why standardizing care was so challenging in this condition.
In 2021–2023, we ran a quality improvement collaborative across 10 UK sites. The dataset reported here was mostly but not entirely qualitative. It included data on the origins and current context of each clinic, interviews with staff and patients, and ethnographic observations at 13 clinics (50 consultations) and 45 multidisciplinary team (MDT) meetings (244 patient cases). Data collection and analysis were informed by relevant lenses from clinical care (e.g. evidence-based guidelines), improvement science (e.g. quality improvement cycles) and philosophy of knowledge.
Participating clinics made progress towards standardizing assessment and management in some topics; some variation remained but this could usually be explained. Clinics had different histories and path dependencies, occupied a different place in their healthcare ecosystem and served a varied caseload including a high proportion of patients with comorbidities. A key mechanism for achieving high-quality long covid care was when local MDTs deliberated on unusual, complex or challenging cases for which evidence-based guidelines provided no easy answers. In such cases, collective learning occurred through idiographic (case-based) reasoning , in which practitioners build lessons from the particular to the general. This contrasts with the nomothetic reasoning implicit in evidence-based guidelines, in which reasoning is assumed to go from the general (e.g. findings of clinical trials) to the particular (management of individual patients).
Not all variation in long covid services is unwarranted. Largely because long covid’s manifestations are so varied and comorbidities common, generic “evidence-based” standards require much individual adaptation. In this complex condition, quality improvement resources may be productively spent supporting MDTs to optimise their case-based learning through interdisciplinary discussion. Quality assessment of a long covid service should include review of a sample of individual cases to assess how guidelines have been interpreted and personalized to meet patients’ unique needs.
Study registration
NCT05057260, ISRCTN15022307.
Peer Review reports
The term “long covid” [ 1 ] means prolonged symptoms following SARS-CoV-2 infection not explained by an alternative diagnosis [ 2 ]. It embraces the US term “post-covid conditions” (symptoms beyond 4 weeks) [ 3 ], the UK terms “ongoing symptomatic covid-19” (symptoms lasting 4–12 weeks) and “post covid-19 syndrome” (symptoms beyond 12 weeks) [ 4 ] and the World Health Organization’s “post covid-19 condition” (symptoms occurring beyond 3 months and persisting for at least 2 months) [ 5 ]. Long covid thus defined is extremely common. In UK, for example, 1.8 million of a population of 67 million met the criteria for long covid in early 2023 and 41% of these had been unwell for more than 2 years [ 6 ].
Long covid is characterized by a constellation of symptoms which may include breathlessness, fatigue, muscle and joint pain, chest pain, memory loss and impaired concentration (“brain fog”), sleep disturbance, depression, anxiety, palpitations, dizziness, gastrointestinal problems such as diarrhea, skin rashes and allergy to food or drugs [ 2 ]. These lead to difficulties with essential daily activities such as washing and dressing, impaired exercise tolerance and ability to work, and reduced quality of life [ 2 , 7 , 8 ]. Symptoms typically cluster (e.g. in different patients, long covid may be dominated by fatigue, by breathlessness or by palpitations and dizziness) [ 9 , 10 ]. Long covid may follow a fairly constant course or a relapsing and remitting one, perhaps with specific triggers [ 11 ]. Overlaps between fatigue-dominant subtypes of long covid, myalgic encephalomyelitis and chronic fatigue syndrome have been hypothesized [ 12 ] but at the time of writing remain unproven.
Long covid has been a contested condition from the outset. Whilst long-term sequelae following other coronavirus (SARS and MERS) infections were already well-documented [ 13 ], SARS-CoV-2 was originally thought to cause a short-lived respiratory illness from which the patient either died or recovered [ 14 ]. Some clinicians dismissed protracted or relapsing symptoms as due to anxiety or deconditioning, especially if the patient had not had laboratory-confirmed covid-19. People with long covid got together in online groups and shared accounts of their symptoms and experiences of such “gaslighting” in their healthcare encounters [ 15 , 16 ]. Some groups conducted surveys on their members, documenting the wide range of symptoms listed in the previous paragraph and showing that whilst long covid is more commonly a sequel to severe acute covid-19, it can (rarely) follow a mild or even asymptomatic acute infection [ 17 ].
Early publications on long covid depicted a post-pneumonia syndrome which primarily affected patients who had been hospitalized (and sometimes ventilated) [ 18 , 19 ]. Later, covid-19 was recognized to be a multi-organ inflammatory condition (the pneumonia, for example, was reclassified as pneumonitis ) and its long-term sequelae attributed to a combination of viral persistence, dysregulated immune response (including auto-immunity), endothelial dysfunction and immuno-thrombosis, leading to damage to the lining of small blood vessels and (thence) interference with transfer of oxygen and nutrients to vital organs [ 20 , 21 , 22 , 23 , 24 ]. But most such studies were highly specialized, laboratory-based and written primarily for an audience of fellow laboratory researchers. Despite demonstrating mean differences in a number of metabolic variables, they failed to identify a reliable biomarker that could be used routinely in the clinic to rule a diagnosis of long covid in or out. Whilst the evidence base from laboratory studies grew rapidly, it had little influence on clinical management—partly because most long covid clinics had been set up with impressive speed by front-line clinical teams to address an immediate crisis, with little or no input from immunologists, virologists or metabolic specialists [ 25 ].
Studies of the patient experience revealed wide geographical variation in whether any long covid services were provided and (if they were) which patients were eligible for these and what tests and treatments were available [ 26 ]. An interim UK clinical guideline for long covid had been produced at speed and published in December 2020 [ 27 ], but it was uncertain about diagnostic criteria, investigations, treatments and prognosis. Early policy recommendations for long covid services in England, based on wide consultation across UK, had proposed a tiered service with “tier 1” being supported self-management, “tier 2” generalist assessment and management in primary care, “tier 3” specialist rehabilitation or respiratory follow-up with oversight from a consultant physician and “tier 4” tertiary care for patients with complications or complex needs [ 28 ]. In 2021, ring-fenced funding was allocated to establish 90 multidisciplinary long covid clinics in England [ 29 ]; some clinics were also set up with local funding in Scotland and Wales. These clinics varied widely in eligibility criteria, referral pathways, staffing mix (some had no doctors at all) and investigations and treatments offered. A further policy document on improving long covid services was published in 2022 [ 30 ]; it recommended that specialist long covid clinics should continue, though the long-term funding of these services remains uncertain [ 31 ]. To build the evidence base for delivering long covid services, major programs of publicly funded research were commenced in both UK [ 32 ] and USA [ 33 ].
In short, at the time this study began (late 2021), there appeared to be much scope for a program of quality improvement which would capture fast-emerging research findings, establish evidence-based standards and ensure these were rapidly disseminated and consistently adopted across both specialist long covid services and in primary care.
Quality improvement collaboratives
The quality improvement movement in healthcare was born in the early 1980s when clinicians and policymakers US and UK [ 34 , 35 , 36 , 37 ] began to draw on insights from outside the sector [ 38 , 39 , 40 ]. Adapting a total quality management approach that had previously transformed the Japanese car industry, they sought to improve efficiency, reduce waste, shift to treating the upstream causes of problems (hence preventing disease) and help all services approach the standards of excellence achieved by the best. They developed an approach based on (a) understanding healthcare as a complex system (especially its key interdependencies and workflows), (b) analysing and addressing variation within the system, (c) learning continuously from real-world data and (d) developing leaders who could motivate people and help them change structures and processes [ 41 , 42 , 43 , 44 ].
Quality improvement collaboratives (originally termed “breakthrough collaboratives” [ 45 ]), in which representatives from different healthcare organizations come together to address a common problem, identify best practice, set goals, share data and initiate and evaluate improvement efforts [ 46 ], are one model used to deliver system-wide quality improvement. It is widely assumed that these collaboratives work because—and to the extent that—they identify, interpret and implement high-quality evidence (e.g. from randomized controlled trials).
Research on why quality improvement collaboratives succeed or fail has produced the following list of critical success factors: taking a whole-system approach, selecting a topic and goal that fits with organizations’ priorities, fostering a culture of quality improvement (e.g. that quality is everyone’s job), engagement of everyone (including the multidisciplinary clinical team, managers, patients and families) in the improvement effort, clearly defining people’s roles and contribution, engaging people in preliminary groundwork, providing organizational-level support (e.g. chief executive endorsement, protected staff time, training and support for teams, resources, quality-focused human resource practices, external facilitation if needed), training in specific quality improvement techniques (e.g. plan-do-study-act cycle), attending to the human dimension (including cultivating trust and working to ensure shared vision and buy-in), continuously generating reliable data on both processes (e.g. current practice) and outcomes (clinical, satisfaction) and a “learning system” infrastructure in which knowledge that is generated feeds into individual, team and organizational learning [ 47 , 48 , 49 , 50 , 51 , 52 , 53 , 54 ].
The quality improvement collaborative approach has delivered many successes but it has been criticized at a theoretical level for over-simplifying the social science of human motivation and behaviour and for adopting a somewhat mechanical approach to the study of complex systems [ 55 , 56 ]. Adaptations of the original quality improvement methodology (e.g. from Sweden [ 57 , 58 ]) have placed greater emphasis on human values and meaning-making, on the grounds that reducing the complexities of a system-wide quality improvement effort to a set of abstract and generic “success factors” will miss unique aspects of the case such as historical path dependencies, personalities, framing and meaning-making and micropolitics [ 59 ].
Perhaps this explains why, when the abovementioned factors are met, a quality improvement collaborative’s success is more likely but is not guaranteed, as a systematic review demonstrated [ 60 ]. Some well-designed and well-resourced collaboratives addressing clear knowledge gaps produced few or no sustained changes in key outcome measures [ 49 , 53 , 60 , 61 , 62 ]. To identify why this might be, a detailed understanding of a service’s history, current challenges and contextual constraints is needed. This explains our decision, part-way through the study reported here, to collect rich contextual data on participating sites so as to better explain success or failure of our own collaborative.
Warranted and unwarranted variation in clinical practice
A generation ago, Wennberg described most variation in clinical practice as “unwarranted” (which he defined as variation in the utilization of health care services that cannot be explained by variation in patient illness or patient preferences) [ 63 ]. Others coined the term “postcode lottery” to depict how such variation allegedly impacted on health outcomes [ 64 ]. Wennberg and colleagues’ Atlas of Variation , introduced in 1999 [ 65 ], and its UK equivalent, introduced in 2010 [ 66 ], described wide regional differences in the rates of procedures from arthroscopy to hysterectomy, and were used to prompt services to identify and address examples of under-treatment, mis-treatment and over-treatment. Numerous similar initiatives, mostly based on hospital activity statistics, have been introduced around the world [ 66 , 67 , 68 , 69 ]. Sutherland and Levesque’s proposed framework for analysing variation, for example, has three domains: capacity (broadly, whether sufficient resources are allocated at organizational level and whether individuals have the time and headspace to get involved), evidence (the extent to which evidence-based guidelines exist and are followed), and agency (e.g. whether clinicians are engaged with the issue and the effect of patient choice) [ 70 ].
Whilst it is clearly a good idea to identify unwarranted variation in practice, it is also important to acknowledge that variation can be warranted . The very act of measuring and describing variation carries great rhetorical power, since revealing geographical variation in any chosen metric effectively frames this as a problem with a conceptually simple solution (reducing variation) that will appeal to both politicians and the public [ 71 ]. The temptation to expose variation (e.g. via visualizations such as maps) and address it in mechanistic ways should be resisted until we have fully understood the reasons why it exists, which may include perverse incentives, insufficient opportunities to discuss cases with colleagues, weak or absent feedback on practice, unclear decision processes, contested definitions of appropriate care and professional challenges to guidelines [ 72 ].
Research question, aims and objectives
Research question.
What is quality in long covid care and how can it best be achieved?
To identify best practice and reduce unwarranted variation in UK long covid services.
To explain aspects of variation in long covid services that are or may be warranted.
Our original objectives were to:
Establish a quality improvement collaborative for 10 long covid clinics across UK.
Use quality improvement methods in collaboration with patients and clinic staff to prioritize aspects of care to improve. For each priority topic, identify best (evidence-informed) clinical practice, measure performance in each clinic, compare performance with a best practice benchmark and improve performance.
Produce organizational case studies of participating long covid clinics to explain their origins, evolution, leadership, ethos, population served, patient pathways and place in the wider healthcare ecosystem.
Examine these case studies to explain variation in practice, especially in topics where the quality improvement cycle proves difficult to follow or has limited impact.
The LOCOMOTION study
LOCOMOTION (LOng COvid Multidisciplinary consortium Optimising Treatments and services across the NHS) was a 30-month multi-site case study of 10 long covid clinics (8 in England, 1 in Wales and 1 in Scotland), beginning in 2021, which sought to optimise long covid care. Each clinic offered multidisciplinary care to patients referred from primary or secondary care (and, in some cases, self-referred), and held regular multidisciplinary team (MDT) meetings, mostly online via Microsoft Teams, to discuss cases. A study protocol for LOCOMOTION, with details of ethical approvals, management, governance and patient involvement has been published [ 25 ]. The three main work packages addressed quality improvement, technology-supported patient self-management and phenotyping and symptom clustering. This paper reports on the first work package, focusing mainly on qualitative findings.
Setting up the quality improvement collaborative
We broadly followed standard methodology for “breakthrough” quality improvement collaboratives [ 44 , 45 ], with two exceptions. First, because of geographical distance, continuing pandemic precautions and developments in videoconferencing technology, meetings were held online. Second, unlike in the original breakthrough model, patients were included in the collaborative, reflecting the cultural change towards patient partnerships since the model was originally proposed 40 years ago.
Each site appointed a clinical research fellow (doctor, nurse or allied health professional) funded partly by the LOCOMOTION study and partly with clinical sessions; some were existing staff who were backfilled to take on a research role whilst others were new appointments. The quality improvement meetings were held approximately every 8 weeks on Microsoft Teams and lasted about 2 h; there was an agenda and a chair, and meetings were recorded with consent. The clinical research fellow from each clinic attended, sometimes joined by the clinical lead for that site. In the initial meeting, the group proposed and prioritized topics before merging their consensus with the list of priority topics generated separately by patients (there was much overlap but also some differences).
In subsequent meetings, participants attempted to reach consensus on how to define, measure and achieve quality for each priority topic in turn, implement this approach in their own clinic and monitor its impact. Clinical leads prepared illustrative clinical cases and summaries of the research evidence, which they presented using Microsoft Powerpoint; the group then worked towards consensus on the implications for practice through general discussion. Clinical research fellows assisted with literature searches, collected baseline data from their own clinic, prepared and presented anonymized case examples, and contributed to collaborative goal-setting for improvement. Progress on each topic was reviewed at a later meeting after an agreed interval.
An additional element of this work package was semi-structured interviews with 29 patients, recruited from 9 of the 10 participating sites, about their clinic experiences with a view to feeding into service improvement (in the other site, no patient volunteered).
Our patient advisory group initially met separately from the quality improvement collaborative. They designed a short survey of current practice and sent it to each clinic; the results of this informed a prioritization exercise for topics where they considered change was needed. The patient-generated list was tabled at the quality improvement collaborative discussions, but patients were understandably keen to join these discussions directly. After about 9 months, some patient advisory group members joined the regular collaborative meetings. This dynamic was not without its tensions, since sharing performance data requires trust and there were some concerns about confidentiality when real patient cases were discussed with other patients present.
How evidence-informed quality targets were set
At the time the study began, there were no published large-scale randomized controlled trials of any interventions for long covid. We therefore followed a model used successfully in other quality improvement efforts where research evidence was limited or absent or it did not translate unambiguously into models for current services. In such circumstances, the best evidence may be custom and practice in the best-performing units. The quality improvement effort becomes oriented to what one group of researchers called “potentially better practices”—that is, practices that are “developed through analysis of the processes of care, literature review, and site visits” (page 14) [ 73 ]. The idea was that facilitated discussion among clinical teams, drawing on published research where available but also incorporating clinical experience, established practice and systematic analysis of performance data across participating clinics would surface these “potentially better practices”—an approach which, though not formally tested in controlled trials, appears to be associated with improved outcomes [ 46 , 73 ].
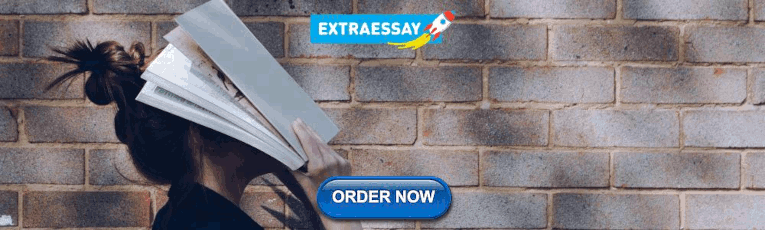
Adding an ethnographic component
Following limited progress made on some topics that had been designated high priority, we interviewed all 10 clinical research fellows (either individually or, in two cases, with a senior clinician present) and 18 other clinic staff (five individually plus two groups of 5 and 8), along with additional informal discussions, to explore the challenges of implementing the changes that had been agreed. These interviews were not audiotaped but detailed notes were made and typed up immediately afterwards. It became evident that some aspects of what the collaborative had deemed “evidence-informed” care were contested by front-line clinic staff, perceived as irrelevant to the service they were delivering, or considered impossible to implement. To unpack these issues further, the research protocol was amended to include an ethnographic component.
TG and EL (academic general practitioners) and JLD (a qualitative researcher with a PhD in the patient experience) attended a total of 45 MDT meetings in participating clinics (mostly online or hybrid). Staff were informed in advance that there would be an observer present; nobody objected. We noted brief demographic and clinical details of cases discussed (but no identifying data), dilemmas and uncertainties on which discussions focused, and how different staff members contributed.
TG made 13 in-person visits to participating long covid clinics. Staff were notified in advance; all were happy to be observed. Visits lasted between 5 and 8 h (54 h in total). We observed support staff booking patients in and processing requests and referrals, and shadowed different clinical staff in turn as they saw patients. Patients were informed of our presence and its purpose beforehand and given the opportunity to decline (three of 53 patients approached did). We discussed aspects of each case with the clinician after the patient left. When invited, we took breaks with staff and used these as an opportunity to ask them informally what it was like working in the clinic.
Ethnographic observation, analysis and reporting was geared to generating a rich interpretive account of the clinical, operational and interpersonal features of each clinic—what Van Maanen calls an “impressionist tales” [ 74 ]. Our work was also guided by the principles set out by Golden-Biddle and Locke, namely authenticity (spending time in the field and basing interpretations on these direct observations), plausibility (creating a plausible account through rich persuasive description) and criticality (e.g. reflexively examining our own assumptions) [ 75 ]. Our collection and analysis of qualitative data was informed by our own professional backgrounds (two general practitioners, one physical therapist, two non-clinicians).
In both MDTs and clinics, we took contemporaneous notes by hand and typed these up immediately afterwards.
Data management and analysis
Typed interview notes and field notes from clinics were collated in a set of Word documents, one for each clinic attended. They were analysed thematically [ 76 ] with attention to the literature on quality improvement and variation (see “ Background ”). Interim summaries were prepared on each clinic, setting out the narrative of how it had been established, its ethos and leadership, setting and staffing, population served and key links with other parts of the local healthcare ecosystem.
Minutes and field notes from the quality improvement collaborative meetings were summarized topic by topic, including initial data collected by the researchers-in-residence, improvement actions taken (or attempted) in that clinic, and any follow-up data shared. Progress or lack of it was interpreted in relation to the contextual case summary for that clinic.
Patient cases seen in clinic, and those discussed by MDTs, were summarized as brief case narratives in Word documents. Using the constant comparative method [ 77 ], we produced an initial synthesis of the clinical picture and principles of management based on the first 10 patient cases seen, and refined this as each additional case was added. Demographic and brief clinical and social details were also logged on Excel spreadsheets. When writing up clinical cases, we used the technique of composite case construction (in which we drew on several actual cases to generate a fictitious one, thereby protecting anonymity whilst preserving key empirical findings [ 78 ]); any names reported in this paper are pseudonyms.
Member checking
A summary was prepared for each clinic, including a narrative of the clinic’s own history and a summary of key quality issues raised across the ten clinics. These summaries included examples from real cases in our dataset. These were shared with the clinical research fellow and a senior clinician from the clinic, and amended in response to feedback. We also shared these summaries with representatives from the patient advisory group.
Overview of dataset
This study generated three complementary datasets. First, the video recordings, minutes, and field notes of 12 quality improvement collaborative meetings, along with the evidence summaries prepared for these meetings and clinic summaries (e.g. descriptions of current practice, audits) submitted by the clinical research fellows. This dataset illustrated wide variation in practice, and (in many topics) gaps or ambiguities in the evidence base.
Second, interviews with staff ( n = 30) and patients ( n = 29) from the clinics, along with ethnographic field notes (approximately 100 pages) from 13 in-person clinic visits (54 h), including notes on 50 patient consultations (40 face-to-face, 6 telephone, 4 video). This dataset illustrated the heterogeneity among the ten participating clinics.
Third, field notes (approximately 100 pages), including discussions on 244 clinical cases from the 45 MDT meetings (49 h) that we observed. This dataset revealed further similarities and contrasts among clinics in how patients were managed. In particular, it illustrated how, for the complex patients whose cases were presented at these meetings, teams made sense of, and planned for, each case through multidisciplinary dialogue. This dialogue typically began with one staff member presenting a detailed clinical history along with a narrative of how it had affected the patient’s life and what was at stake for them (e.g. job loss), after which professionals from various backgrounds (nursing, physical therapy, occupational therapy, psychology, dietetics, and different medical specialties) joined in a discussion about what to do.
The ten participating sites are summarized in Table 1 .
In the next two sections, we explore two issues—difficulty defining best practice and the heterogeneous nature of the clinics—that were key to explaining why quality, when pursued in a 10-site collaborative, proved elusive. We then briefly summarize patients’ accounts of their experience in the clinics and give three illustrative examples of the elusiveness of quality improvement using selected topics that were prioritized in our collaborative: outcome measures, investigation of palpitations and management of fatigue. In the final section of the results, we describe how MDT deliberations proved crucial for local quality improvement. Further detail on clinical priority topics will be presented in a separate paper.
“Best practice” in long covid: uncertainty and conflict
The study period (September 2021 to December 2023) corresponded with an exponential increase in published research on long covid. Despite this, the quality improvement collaborative found few unambiguous recommendations for practice. This gap between what the research literature offered and what clinical practice needed was partly ontological (relating what long covid is ). One major bone of contention between patients and clinicians (also evident in discussions with our patient advisory group), for example, was how far (and in whom) clinicians should look for and attempt to treat the various metabolic abnormalities that had been documented in laboratory research studies. The literature on this topic was extensive but conflicting [ 20 , 21 , 22 , 23 , 24 , 79 , 80 , 81 , 82 ]; it was heavy on biological detail but light on clinical application.
Patients were often aware of particular studies that appeared to offer plausible molecular or cellular explanations for symptom clusters along with a drug (often repurposed and off-label) whose mechanism of action appeared to be a good fit with the metabolic chain of causation. In one clinic, for example, we were shown an email exchange between a patient (not medically qualified) and a consultant, in which the patient asked them to reconsider their decision not to prescribe low-dose naltrexone, an opioid receptor antagonist with anti-inflammatory properties. The request included a copy of a peer-reviewed academic paper describing a small, uncontrolled pre-post study (i.e. a weak study design) in which this drug appeared to improve symptoms and functional performance in patients with long covid, as well as a mechanistic argument explaining why the patient felt this drug was a plausible choice in their own case.
This patient’s clinician, in common with most clinicians delivering front-line long covid services, considered that the evidence for such mechanism-based therapies was weak. Clinicians generally felt that this evidence, whilst promising, did not yet support routine measurement of clotting factors, antibodies, immune cells or other biomarkers or the prescription of mechanism-based therapies such as antivirals, anti-inflammatories or anticoagulants. Low-dose naltroxone, for example, is currently being tested in at least one randomized controlled trial (see National Clinical Trials Registry NCT05430152), which had not reported at the time of our observations.
Another challenge to defining best practice was the oft-repeated phrase that long covid is a “diagnosis by exclusion”, but the high prevalence of comorbidities meant that the “pure” long covid patient untainted by other potential explanations for their symptoms was a textbook ideal. In one MDT, for example, we observed a discussion about a patient who had had both swab-positive covid-19 and erythema migrans (a sign of Lyme disease) in the weeks before developing fatigue, yet local diagnostic criteria for each condition required the other to be excluded.
The logic of management in most participating clinics was pragmatic: prompt multidisciplinary assessment and treatment with an emphasis on obtaining a detailed clinical history (including premorbid health status), excluding serious complications (“red flags”), managing specific symptom clusters (for example, physical therapy for breathing pattern disorder), treating comorbidities (for example, anaemia, diabetes or menopause) and supporting whole-person rehabilitation [ 7 , 83 ]. The evidentiary questions raised in MDT discussions (which did not include patients) addressed the practicalities of the rehabilitation model (for example, whether cognitive therapy for neurocognitive complications is as effective when delivered online as it is when delivered in-person) rather than the molecular or cellular mechanisms of disease. For example, the question of whether patients with neurocognitive impairment should be tested for micro-clots or treated with anticoagulants never came up in the MDTs we observed, though we did visit a tertiary referral clinic (the tier 4 clinic in site H), whose lead clinician had a research interest in inflammatory coagulopathies and offered such tests to selected patients.
Because long covid typically produces dozens of symptoms that tend to be uniquely patterned in each patient, the uncertainties on which MDT discussions turned were rarely about general evidence of the kind that might be found in a guideline (e.g. how should fatigue be managed?). Rather they concerned particular case-based clinical decisions (e.g. how should this patient’s fatigue be managed, given the specifics of this case?). An example from our field notes illustrates this:
Physical therapist presents the case of a 39-year-old woman who works as a cleaner on an overnight ferry. Has had long covid for 2 years. Main symptoms are shortness of breath and possible anxiety attacks, especially when at work. She has had a course of physical therapy to teach diaphragmatic breathing but has found that focusing on her breathing makes her more anxious. Patient has to do a lot of bending in her job (e.g. cleaning toilets and under seats), which makes her dizzy, but Active Stand Test was normal. She also has very mild tricuspid incompetence [someone reads out a cardiology report—not hemodynamically significant].
Rehabilitation guidelines (e.g. WHO) recommend phased return to work (e.g. with reduced hours) and frequent breaks. “Tricky!” says someone. The job is intense and busy, and the patient can’t afford not to work. Discussion on whether all her symptoms can be attributed to tension and anxiety. Physical therapist who runs the breathing group says, “No, it’s long covid”, and describes severe initial covid-19 episode and results of serial chest X-rays which showed gradual clearing of ground glass shadows. Team discussion centers on how to negotiate reduced working hours in this particular job, given the overnight ferry shifts. --MDT discussion, Site D
This example raises important considerations about the nature of clinical knowledge in long covid. We return to it in the final section of the “ Results ” and in the “ Discussion ”.
Long covid clinics: a heterogeneous context for quality improvement
Most participating clinics had been established in mid-2020 to follow up patients who had been hospitalized (and perhaps ventilated) for severe acute covid-19. As mass vaccination reduced the severity of acute covid-19 for most people, the patient population in all clinics progressively shifted to include fewer “post-ICU [intensive care unit]” patients (in whom respiratory symptoms almost always dominated), and more people referred by their general practitioners or other secondary care specialties who had not been hospitalized for their acute covid-19 infection, and in whom fatigue, brain fog and palpitations were often the most troubling symptoms. Despite these similarities, the ten clinics had very different histories, geographical and material settings, staffing structures, patient pathways and case mix, as Table 1 illustrates. Below, we give more detail on three example sites.
Site C was established as a generalist “assessment-only” service by a general practitioner with an interest in infectious diseases. It is led jointly by that general practitioner and an occupational therapist, assisted by a wide range of other professionals including speech and language therapy, dietetics, clinical psychology and community-based physical therapy and occupational therapy. It has close links with a chronic fatigue service and a pain clinic that have been running in the locality for over 20 years. The clinic, which is entirely virtual (staff consult either from home or from a small side office in the community trust building), is physically located in a low-rise building on the industrial outskirts of a large town, sharing office space with various community-based health and social care services. Following a 1-h telephone consultation by one of the clinical leads, each patient is discussed at the MDT and then either discharged back to their general practitioner with a detailed management plan or referred on to one of the specialist services. This arrangement evolved to address a particular problem in this locality—that many patients with long covid were being referred by their general practitioner to multiple specialties (e.g. respiratory, neurology, fatigue), leading to a fragmented patient experience, unnecessary specialist assessments and wasteful duplication. The generalist assessment by telephone is oriented to documenting what is often a complex illness narrative (including pre-existing physical and mental comorbidities) and working with the patient to prioritize which symptoms or problems to pursue in which order.
Site E, in a well-regarded inner-city teaching hospital, had been set up in 2020 by a respiratory physician. Its initial ethos and rationale had been “respiratory follow-up”, with strong emphasis on monitoring lung damage via repeated imaging and lung function tests and in ensuring that patients received specialist physical therapy to “re-learn” efficient breathing techniques. Over time, this site has tried to accommodate a more multi-system assessment, with the introduction of a consultant-led infectious disease clinic for patients without a dominant respiratory component, reflecting the shift towards a more fatigue-predominant case mix. At the time of our fieldwork, each patient was seen in turn by a physician, psychologist, occupational therapist and respiratory physical therapist (half an hour each) before all four staff reconvened in a face-to-face MDT meeting to form a plan for each patient. But whilst a wide range of patients with diverse symptoms were discussed at these meetings, there remained a strong focus on respiratory pathology (e.g. tracking improvements in lung function and ensuring that coexisting asthma was optimally controlled).
Site F, one of the first long covid clinics in UK, was set up by a rehabilitation consultant who had been drafted to work on the ICU during the first wave of covid-19 in early 2020. He had a longstanding research interest in whole-patient rehabilitation, especially the assessment and management of chronic fatigue and pain. From the outset, clinic F was more oriented to rehabilitation, including vocational rehabilitation to help patients return to work. There was less emphasis on monitoring lung function or pursuing respiratory comorbidities. At the time of our fieldwork, clinic F offered both a community-based service (“tier 2”) led by an occupational therapist, supported by a respiratory physical therapist and psychologist, and a hospital-based service (“tier 3”) led by the rehabilitation consultant, supported by a wider MDT. Staff in both tiers emphasized that each patient needs a full physical and mental assessment and help to set and work towards achievable goals, whilst staying within safe limits so as to avoid post-exertional symptom exacerbation. Because of the research interest of the lead physician, clinic F adapted well to the growing numbers of patients with fatigue and quickly set up research studies on this cohort [ 84 ].
Details of the other seven sites are shown in Table 1 . Broadly speaking, sites B, E, G and H aligned with the “respiratory follow-up” model and sites F and I aligned with the “rehabilitation” model. Sites A and J had a high-volume, multi-tiered service whose community tier aligned with the “holistic GP assessment” model (site C above) and which also offered a hospital-based, rehabilitation-focused tier. The small service in Scotland (site D) had evolved from an initial respiratory focus to become part of the infectious diseases (ME/CFS) service; Lyme disease (another infectious disease whose sequelae include chronic fatigue) was also prevalent in this region.
The patient experience
Whilst the 10 participating clinics were very diverse in staffing, ethos and patient flows, the 29 patient interviews described remarkably consistent clinic experiences. Almost all identified the biggest problem to be the extended wait of several months before they were seen and the limited awareness (when initially referred) of what long covid clinics could provide. Some talked of how they cried with relief when they finally received an appointment. When the quality improvement collaborative was initially established, waiting times and bottlenecks were patients’ the top priority for quality improvement, and this ranking was shared by clinic staff, who were very aware of how much delays and uncertainties in assessment and treatment compounded patients’ suffering. This issue resolved to a large extent over the study period in all clinics as the referral backlog cleared and the incidence of new cases of long covid fell [ 85 ]; it will be covered in more detail in a separate publication.
Most patients in our sample were satisfied with the care they received when they were finally seen in clinic, especially how they finally felt “heard” after a clinician took a full history. They were relieved to receive affirmation of their experience, a diagnosis of what was wrong and reassurance that they were believed. They were grateful for the input of different members of the multidisciplinary teams and commented on the attentiveness, compassion and skill of allied professionals in particular (“she was wonderful, she got me breathing again”—patient BIR145 talking about a physical therapist). One or two patient participants expressed confusion about who exactly they had seen and what advice they had been given, and some did not realize that a telephone assessment had been an actual clinical consultation. A minority expressed disappointment that an expected investigation had not been ordered (one commented that they had not had any blood tests at all). Several had assumed that the help and advice from the long covid clinic would continue to be offered until they were better and were disappointed that they had been discharged after completing the various courses on offer (since their clinic had been set up as an “assessment only” service).
In the next sections, we give examples of topics raised in the quality improvement collaborative and how they were addressed.
Example quality topic 1: Outcome measures
The first topic considered by the quality improvement collaborative was how (that is, using which measures and metrics) to assess and monitor patients with long covid. In the absence of a validated biomarker, various symptom scores and quality of life scales—both generic and disease-specific—were mooted. Site F had already developed and validated a patient-reported outcome measure (PROM), the C19-YRS (Covid-19 Yorkshire Rehabilitation Scale) and used it for both research and clinical purposes [ 86 ]. It was quickly agreed that, for the purposes of generating comparative research findings across the ten clinics, the C19-YRS should be used at all sites and completed by patients three-monthly. A commercial partner produced an electronic version of this instrument and an app for patient smartphones. The quality improvement collaborative also agreed that patients should be asked to complete the EUROQOL EQ5D, a widely used generic health-related quality of life scale [ 87 ], in order to facilitate comparisons between long covid and other chronic conditions.
In retrospect, the discussions which led to the unopposed adoption of these two measures as a “quality” initiative in clinical care were somewhat aspirational. A review of progress at a subsequent quality improvement meeting revealed considerable variation among clinics, with a wide variety of measures used in different clinics to different degrees. Reasons for this variation were multiple. First, although our patient advisory group were keen that we should gather as much data as possible on the patient experience of this new condition, many clinic patients found the long questionnaires exhausting to complete due to cognitive impairment and fatigue. In addition, whilst patients were keen to answer questions on symptoms that troubled them, many had limited patience to fill out repeated surveys on symptoms that did not trouble them (“it almost felt as if I’ve not got long covid because I didn’t feel like I fit the criteria as they were laying it out”—patient SAL001). Staff assisted patients in completing the measures when needed, but this was time-consuming (up to 45 min per instrument) and burdensome for both staff and patients. In clinics where a high proportion of patients required assistance, staff time was the rate-limiting factor for how many instruments got completed. For some patients, one short instrument was the most that could be asked of them, and the clinician made a judgement on which one would be in their best interests on the day.
The second reason for variation was that the clinical diagnosis and management of particular features, complications and comorbidities of long covid required more nuance than was provided by these relatively generic instruments, and the level of detail sought varied with the specialist interest of the clinic (and the clinician). The modified C19-YRS [ 88 ], for example, contained 19 items, of which one asked about sleep quality. But if a patient had sleep difficulties, many clinicians felt that these needed to be documented in more detail—for example using the 8-item Epworth Sleepiness Scale, originally developed for conditions such as narcolepsy and obstructive sleep apnea [ 89 ]. The “Epworth score” was essential currency for referrals to some but not all specialist sleep services. Similarly, the C19-YRS had three items relating to anxiety, depression and post-traumatic stress disorder, but in clinics where there was a strong focus on mental health (e.g. when there was a resident psychologist), patients were usually invited to complete more specific tools (e.g. the Patient Health Questionnaire 9 [ 90 ], a 9-item questionnaire originally designed to assess severity of depression).
The third reason for variation was custom and practice. Ethnographic visits revealed that paper copies of certain instruments were routinely stacked on clinicians’ desks in outpatient departments and also (in some cases) handed out by administrative staff in waiting areas so that patients could complete them before seeing the clinician. These familiar clinic artefacts tended to be short (one-page) instruments that had a long tradition of use in clinical practice. They were not always fit for purpose. For example, the Nijmegen questionnaire was developed in the 1980s to assess hyperventilation; it was validated against a longer, “gold standard” instrument for that condition [ 91 ]. It subsequently became popular in respiratory clinics to diagnose or exclude breathing pattern disorder (a condition in which the normal physiological pattern of breathing becomes replaced with less efficient, shallower breathing [ 92 ]), so much so that the researchers who developed the instrument published a paper to warn fellow researchers that it had not been validated for this purpose [ 93 ]. Whilst a validated 17-item instrument for breathing pattern disorder (the Self-Evaluation of Breathing Questionnaire [ 94 ]) does exist, it is not in widespread clinical use. Most clinics in LOCOMOTION used Nijmegen either on all patients (e.g. as part of a comprehensive initial assessment, especially if the service had begun as a respiratory follow-up clinic) or when breathing pattern disorder was suspected.
In sum, the use of outcome measures in long covid clinics was a compromise between standardization and contingency. On the one hand, all clinics accepted the need to use “validated” instruments consistently. On the other hand, there were sometimes good reasons why they deviated from agreed practice, including mismatch between the clinic’s priorities as a research site, its priorities as a clinical service, and the particular clinical needs of a patient; the clinic’s—and the clinician’s—specialist focus; and long-held traditions of using particular instruments with which staff and patients were familiar.
Example quality topic 2: Postural orthostatic tachycardia syndrome (POTS)
Palpitations (common in long covid) and postural orthostatic tachycardia syndrome (POTS, a disproportionate acceleration in heart rate on standing, the assumed cause of palpitations in many long covid patients) was the top priority for quality improvement identified by our patient advisory group. Reflecting discussions and evidence (of various kinds) shared in online patient communities, the group were confident that POTS is common in long covid patients and that many cases remain undetected (perhaps misdiagnosed as anxiety). Their request that all long covid patients should be “screened” for POTS prompted a search for, and synthesis of, evidence (which we published in the BMJ [ 95 ]). In sum, that evidence was sparse and contested, but, combined with standard practice in specialist clinics, broadly supported the judicious use of the NASA Lean Test [ 96 ]. This test involves repeated measurements of pulse and blood pressure with the patient first lying and then standing (with shoulders resting against a wall).
The patient advisory group’s request that the NASA Lean Test should be conducted on all patients met with mixed responses from the clinics. In site F, the lead physician had an interest in autonomic dysfunction in chronic fatigue and was keen; he had already published a paper on how to adapt the NASA Lean Test for self-assessment at home [ 97 ]. Several other sites were initially opposed. Staff at site E, for example, offered various arguments:
The test is time-consuming, labor-intensive, and takes up space in the clinic which has an opportunity cost in terms of other potential uses;
The test is unvalidated and potentially misleading (there is a high incidence of both false negative and false positive results);
There is no proven treatment for POTS, so there is no point in testing for it;
It is a specialist test for a specialist condition, so it should be done in a specialist clinic where its benefits and limitations are better understood;
Objective testing does not change clinical management since what we treat is the patient’s symptoms (e.g. by a pragmatic trial of lifestyle measures and medication);
People with symptoms suggestive of dysautonomia have already been “triaged out” of this clinic (that is, identified in the initial telephone consultation and referred directly to neurology or cardiology);
POTS is a manifestation of the systemic nature of long covid; it does not need specific treatment but will improve spontaneously as the patient goes through standard interventions such as active pacing, respiratory physical therapy and sleep hygiene;
Testing everyone, even when asymptomatic, runs counter to the ethos of rehabilitation, which is to “de-medicalize” patients so as to better orient them to their recovery journey.
When clinics were invited to implement the NASA Lean Test on a consecutive sample of patients to resolve a dispute about the incidence of POTS (from “we’ve only seen a handful of people with it since the clinic began” to “POTS is common and often missed”), all but one site agreed to participate. The tertiary POTS centre linked to site H was already running the NASA Lean Test as standard on all patients. Site C, which operated entirely virtually, passed the work to the referring general practitioner by making this test a precondition for seeing the patient; site D, which was largely virtual, sent instructions for patients to self-administer the test at home.
The NASA Lean Test study has been published separately [ 98 ]. In sum, of 277 consecutive patients tested across the eight clinics, 20 (7%) had a positive NASA Lean Test for POTS and a further 28 (10%) a borderline result. Six of 20 patients who met the criteria for POTS on testing had no prior history of orthostatic intolerance. The question of whether this test should be used to “screen” all patients was not answered definitively. But the experience of participating in the study persuaded some sceptics that postural changes in heart rate could be severe in some long covid patients, did not appear to be fully explained by their previously held theories (e.g. “functional”, anxiety, deconditioning), and had likely been missed in some patients. The outcome of this particular quality improvement cycle was thus not a wholescale change in practice (for which the evidence base was weak) but a more subtle increase in clinical awareness, a greater willingness to consider testing for POTS and a greater commitment to contribute to research into this contested condition.
More generally, the POTS audit prompted some clinicians to recognize the value of quality improvement in novel clinical areas. One physician who had initially commented that POTS was not seen in their clinic, for example, reflected:
“ Our clinic population is changing. […] Overall there’s far fewer post-ICU patients with ECMO [extra-corporeal membrane oxygenation] issues and far more long covid from the community, and this is the bit our clinic isn’t doing so well on. We’re doing great on breathing pattern disorder; neuro[logists] are helping us with the brain fogs; our fatigue and occupational advice is ok but some of the dysautonomia symptoms that are more prevalent in the people who were not hospitalized – that’s where we need to improve .” -Respiratory physician, site G (from field visit 6.6.23)
Example quality topic 3: Management of fatigue
Fatigue was the commonest symptom overall and a high priority among both patients and clinicians for quality improvement. It often coexisted with the cluster of neurocognitive symptoms known as brain fog, with both conditions relapsing and remitting in step. Clinicians were keen to systematize fatigue management using a familiar clinical framework oriented around documenting a full clinical history, identifying associated symptoms, excluding or exploring comorbidities and alternative explanations (e.g. poor sleep patterns, depression, menopause, deconditioning), assessing how fatigue affects physical and mental function, implementing a program of physical and cognitive therapy that was sensitive to the patient’s condition and confidence level, and monitoring progress using validated patient-reported outcome measures and symptom diaries.
The underpinning logic of this approach, which broadly reflected World Health Organization guidance [ 99 ], was that fatigue and linked cognitive impairment could be a manifestation of many—perhaps interacting—conditions but that a whole-patient (body and mind) rehabilitation program was the cornerstone of management in most cases. Discussion in the quality improvement collaborative focused on issues such as whether fatigue was so severe that it produced safety concerns (e.g. in a person’s job or with childcare), the pros and cons of particular online courses such as yoga, relaxation and mindfulness (many were viewed positively, though the evidence base was considered weak), and the extent to which respiratory physical therapy had a crossover impact on fatigue (systematic reviews suggested that it may do, but these reviews also cautioned that primary studies were sparse, methodologically flawed, and heterogeneous [ 100 , 101 ]). They also debated the strengths and limitations of different fatigue-specific outcome measures, each of which had been developed and validated in a different condition, with varying emphasis on cognitive fatigue, physical fatigue, effect on daily life, and motivation. These instruments included the Modified Fatigue Impact Scale; Fatigue Severity Scale [ 102 ]; Fatigue Assessment Scale; Functional Assessment Chronic Illness Therapy—Fatigue (FACIT-F) [ 103 ]; Work and Social Adjustment Scale [ 104 ]; Chalder Fatigue Scale [ 105 ]; Visual Analogue Scale—Fatigue [ 106 ]; and the EQ5D [ 87 ]. In one clinic (site F), three of these scales were used in combination for reasons discussed below.
Some clinicians advocated melatonin or nutritional supplements (such as vitamin D or folic acid) for fatigue on the grounds that many patients found them helpful and formal placebo-controlled trials were unlikely ever to be conducted. But neurostimulants used in other fatigue-predominant conditions (e.g. brain injury, stroke), which also lacked clinical trial evidence in long covid, were viewed as inappropriate in most patients because of lack of evidence of clear benefit and hypothetical risk of harm (e.g. adverse drug reactions, polypharmacy).
Whilst the patient advisory group were broadly supportive of a whole-patient rehabilitative approach to fatigue, their primary concern was fatiguability , especially post-exertional symptom exacerbation (PESE, also known as “crashes”). In these, the patient becomes profoundly fatigued some hours or days after physical or mental exertion, and this state can last for days or even weeks [ 107 ]. Patients viewed PESE as a “red flag” symptom which they felt clinicians often missed and sometimes caused. They wanted the quality improvement effort to focus on ensuring that all clinicians were aware of the risks of PESE and acted accordingly. A discussion among patients and clinicians at a quality improvement collaborative meeting raised a new research hypothesis—that reducing the number of repeated episodes of PESE may improve the natural history of long covid.
These tensions around fatigue management played out differently in different clinics. In site C (the GP-led virtual clinic run from a community hub), fatigue was viewed as one manifestation of a whole-patient condition. The lead general practitioner used the metaphor of untangling a skein of wool: “you have to find the end and then gently pull it”. The underlying problem in a fatigued patient, for example, might be an undiagnosed physical condition such as anaemia, disturbed sleep, or inadequate pacing. These required (respectively) the chronic fatigue service (comprising an occupational therapist and specialist psychologist and oriented mainly to teaching the techniques of goal-setting and pacing), a “tiredness” work-up (e.g. to exclude anaemia or menopause), investigation of poor sleep (which, not uncommonly, was due to obstructive sleep apnea), and exploration of mental health issues.
In site G (a hospital clinic which had evolved from a respiratory service), patients with fatigue went through a fatigue management program led by the occupational therapist with emphasis on pacing, energy conservation, avoidance of PESE and sleep hygiene. Those without ongoing respiratory symptoms were often discharged back to their general practitioner once they had completed this; there was no consultant follow-up of unresolved fatigue.
In site F (a rehabilitation clinic which had a longstanding interest in chronic fatigue even before the pandemic), active interdisciplinary management of fatigue was commenced at or near the patient’s first visit, on the grounds that the earlier this began, the more successful it would be. In this clinic, patients were offered a more intensive package: a similar occupational therapy-led fatigue course as those in site G, plus input from a dietician to advise on regular balanced meals and caffeine avoidance and a group-based facilitated peer support program which centred on fatigue management. The dietician spoke enthusiastically about how improving diet in longstanding long covid patients often improved fatigue (e.g. because they had often lost muscle mass and tended to snack on convenience food rather than make meals from scratch), though she agreed there was no evidence base from trials to support this approach.
Pursuing local quality improvement through MDTs
Whilst some long covid patients had “textbook” symptoms and clinical findings, many cases were unique and some were fiendishly complex. One clinician commented that, somewhat paradoxically, “easy cases” were often the post-ICU follow-ups who had resolving chest complications; they tended to do well with a course of respiratory physical therapy and a return-to-work program. Such cases were rarely brought to MDT meetings. “Difficult cases” were patients who had not been hospitalized for their acute illness but presented with a months- or years-long history of multiple symptoms with fatigue typically predominant. Each one was different, as the following example (some details of which have been fictionalized to protect anonymity) illustrates.
The MDT is discussing Mrs Fermah, a 65-year-old homemaker who had covid-19 a year ago. She has had multiple symptoms since, including fluctuating fatigue, brain fog, breathlessness, retrosternal chest pain of burning character, dry cough, croaky voice, intermittent rashes (sometimes on eating), lips going blue, ankle swelling, orthopnoea, dizziness with the room spinning which can be triggered by stress, low back pain, aches and pains in the arms and legs and pins and needles in the fingertips, loss of taste and smell, palpitations and dizziness (unclear if postural, but clear association with nausea), headaches on waking, and dry mouth. She is somewhat overweight (body mass index 29) and admits to low mood. Functionally, she is mostly confined to the house and can no longer manage the stairs so has begun to sleep downstairs. She has stumbled once or twice but not fallen. Her social life has ceased and she rarely has the energy to see her grandchildren. Her 70-year-old husband is retired and generally supportive, though he spends most evenings at his club. Comorbidities include glaucoma which is well controlled and overseen by an ophthalmologist, mild club foot (congenital) and stage 1 breast cancer 20 years ago. Various tests, including a chest X-ray, resting and exercise oximetry and a blood panel, were normal except for borderline vitamin D level. Her breathing questionnaire score suggests she does not have breathing pattern disorder. ECG showed first-degree atrioventricular block and left axis deviation. No clinician has witnessed the blue lips. Her current treatment is online group respiratory physical therapy; a home visit is being arranged to assess her climbing stairs. She has declined a psychologist assessment. The consultant asks the nurse who assessed her: “Did you get a feel if this is a POTS-type dizziness or an ENT-type?” She sighs. “Honestly it was hard to tell, bless her.”—Site A MDT
This patient’s debilitating symptoms and functional impairments could all be due to long covid, yet “evidence-based” guidance for how to manage her complex suffering does not exist and likely never will exist. The question of which (if any) additional blood or imaging tests to do, in what order of priority, and what interventions to offer the patient will not be definitively answered by consulting clinical trials involving hundreds of patients, since (even if these existed) the decision involves weighing this patient’s history and the multiple factors and uncertainties that are relevant in her case. The knowledge that will help the MDT provide quality care to Mrs Fermah is case-based knowledge—accumulated clinical experience and wisdom from managing and deliberating on multiple similar cases. We consider case-based knowledge further in the “ Discussion ”.
Summary of key findings
This study has shown that a quality improvement collaborative of UK long covid clinics made some progress towards standardizing assessment and management in some topics, but some variation remained. This could be explained in part by the fact that different clinics had different histories and path dependencies, occupied a different place in the local healthcare ecosystem, served different populations, were differently staffed, and had different clinical interests. Our patient advisory group and clinicians in the quality improvement collaborative broadly prioritized the same topics for improvement but interpreted them somewhat differently. “Quality” long covid care had multiple dimensions, relating to (among other things) service set-up and accessibility, clinical provision appropriate to the patient’s need (including options for referral to other services locally), the human qualities of clinical and support staff, how knowledge was distributed across (and accessible within) the system, and the accumulated collective wisdom of local MDTs in dealing with complex cases (including multiple kinds of specialist expertise as well as relational knowledge of what was at stake for the patient). Whilst both staff and patients were keen to contribute to the quality improvement effort, the burden of measurement was evident: multiple outcome measures, used repeatedly, were resource-intensive for staff and exhausting for patients.
Strengths and limitations of this study
To our knowledge, we are the first to report both a quality improvement collaborative and an in-depth qualitative study of clinical work in long covid. Key strengths of this work include the diverse sampling frame (with sites from three UK jurisdictions and serving widely differing geographies and demographics); the use of documents, interviews and reflexive interpretive ethnography to produce meaningful accounts of how clinics emerged and how they were currently organized; the use of philosophical concepts to analyse data on how MDTs produced quality care on a patient-by-patient basis; and the close involvement of patient co-researchers and coauthors during the research and writing up.
Limitations of the study include its exclusive UK focus (the external validity of findings to other healthcare systems is unknown); the self-selecting nature of participants in a quality improvement collaborative (our patient advisory group suggested that the MDTs observed in this study may have represented the higher end of a quality spectrum, hence would be more likely than other MDTs to adhere to guidelines); and the particular perspective brought by the researchers (two GPs, a physical therapist and one non-clinical person) in ethnographic observations. Hospital specialists or organizational scholars, for example, may have noticed different things or framed what they observed differently.
Explaining variation in long covid care
Sutherland and Levesque’s framework mentioned in the “ Background ” section does not explain much of the variation found in our study [ 70 ]. In terms of capacity, at the time of this study most participating clinics benefited from ring-fenced resources. In terms of evidence, guidelines existed and were not greatly contested, but as illustrated by the case of Mrs Fermah above, many patients were exceptions to the guideline because of complex symptomatology and relevant comorbidities. In terms of agency, clinicians in most clinics were passionately engaged with long covid (they were pioneers who had set up their local clinic and successfully bid for national ring-fenced resources) and were generally keen to support patient choice (though not if the patient requested tests which were unavailable or deemed not indicated).
Astma et al.’s list of factors that may explain variation in practice (see “ Background ”) includes several that may be relevant to long covid, especially that the definition of appropriate care in this condition remains somewhat contested. But lack of opportunity to discuss cases was not a problem in the clinics in our sample. On the contrary, MDT meetings in each locality gave clinicians multiple opportunities to discuss cases with colleagues and reflect collectively on whether and how to apply particular guidelines.
The key problem was not that clinicians disputed the guidelines for managing long covid or were unaware of them; it was that the guidelines were not self-interpreting . Rather, MDTs had to deliberate on the balance of benefits and harms in different aspects of individual cases. In patients whose symptoms suggested a possible diagnosis of POTS (or who suspected themselves of having POTS), for example, these deliberations were sometimes lengthy and nuanced. Should a test result that is not technically in the abnormal range but close to it be treated as diagnostic, given that symptoms point to this diagnosis? If not, should the patient be told that the test excludes POTS or that it is equivocal? If a cardiology opinion has stated firmly that the patient does not have POTS but the cardiologist is not known for their interest in this condition, should a second specialist opinion be sought? If the gold standard “tilt test” [ 108 ] for POTS (usually available only in tertiary centres) is not available locally, does this patient merit a costly out-of-locality referral? Should the patient’s request for a trial of off-label medication, reflecting discussions in an online support group, be honoured? These are the kinds of questions on which MDTs deliberated at length.
The fact that many cases required extensive deliberation does not necessarily justify variation in practice among clinics. But taking into account the clinics’ very different histories, set-up, and local referral pathways, the variation begins to make sense. A patient who is being assessed in a clinic that functions as a specialist chronic fatigue centre and attracts referrals which reflect this interest (e.g. site F in our sample) will receive different management advice from one that functions as a telephone-only generalist assessment centre and refers on to other specialties (site C in our sample). The wide variation in case mix, coupled with the fact that a different proportion of these cases were highly complex in each clinic (and in different ways), suggests that variation in practice may reflect appropriate rather than inappropriate care.
Our patient advisory group affirmed that many of the findings reported here resonated with their own experience, but they raised several concerns. These included questions about patient groups who may have been missed in our sample because they were rarely discussed in MDTs. The decision to take a case to MDT discussion is taken largely by a clinician, and there was evidence from online support groups that some patients’ requests for their case to be taken to an MDT had been declined (though not, to our knowledge, in the clinics participating in the LOCOMOTION study).
We began this study by asking “what is quality in long covid care?”. We initially assumed that this question referred to a generalizable evidence base, which we felt we could identify, and we believed that we could then determine whether long covid clinics were following the evidence base through conventional audits of structure, process, and outcome. In retrospect, these assumptions were somewhat naïve. On the basis of our findings, we suggest that a better (and more individualized) research question might be “to what extent does each patient with long covid receive evidence-based care appropriate to their needs?”. This question would require individual case review on a sample of cases, tracking each patient longitudinally including cross-referrals, and also interviewing the patient.
Nomothetic versus idiographic knowledge
In a series of lectures first delivered in the 1950s and recently republished [ 109 ], psychiatrist Dr Maurice O’Connor Drury drew on the later philosophy of his friend and mentor Ludwig Wittgenstein to challenge what he felt was a concerning trend: that the nomothetic (generalizable, abstract) knowledge from randomized controlled trials (RCTs) was coming to over-ride the idiographic (personal, situated) knowledge about particular patients. Based on Wittgenstein’s writings on the importance of the particular, Drury predicted—presciently—that if implemented uncritically, RCTs would result in worse, not better, care for patients, since it would go hand-in-hand with a downgrading of experience, intuition, subjective judgement, personal reflection, and collective deliberation.
Much conventional quality improvement methodology is built on an assumption that nomothetic knowledge (for example, findings from RCTs and systematic reviews) is a higher form of knowing than idiographic knowledge. But idiographic, case-based reasoning—despite its position at the very bottom of evidence-based medicine’s hierarchy of evidence [ 110 ]—is a legitimate and important element of medical practice. Bioethicist Kathryn Montgomery, drawing on Aristotle’s notion of praxis , considers clinical practice to be an example of case-based reasoning [ 111 ]. Medicine is governed not by hard and fast laws but by competing maxims or rules of thumb ; the essence of judgement is deciding which (if any) rule should be applied in a particular circumstance. Clinical judgement incorporates science (especially the results of well-conducted research) and makes use of available tools and technologies (including guidelines and decision-support algorithms that incorporate research findings). But rather than being determined solely by these elements, clinical judgement is guided both by the scientific evidence and by the practical and ethical question “what is it best to do, for this individual, given these circumstances?”.
In this study, we observed clinical management of, and MDT deliberations on, hundreds of clinical cases. In the more straightforward ones (for example, recovering pneumonitis), guideline-driven care was not difficult to implement and such cases were rarely brought to the MDT. But cases like Mrs Fermah (see last section of “ Results ”) required much discussion on which aspects of which guideline were in the patient’s best interests to bring into play at any particular stage in their illness journey.
Conclusions
One systematic review on quality improvement collaboratives concluded that “ [those] reporting success generally addressed relatively straightforward aspects of care, had a strong evidence base and noted a clear evidence-practice gap in an accepted clinical pathway or guideline” (page 226) [ 60 ]. The findings from this study suggest that to the extent that such collaboratives address clinical cases that are not straightforward, conventional quality improvement methods may be less useful and even counterproductive.
The question “what is quality in long covid care?” is partly a philosophical one. Our findings support an approach that recognizes and values idiographic knowledge —including establishing and protecting a safe and supportive space for deliberation on individual cases to occur and to value and draw upon the collective learning that occurs in these spaces. It is through such deliberation that evidence-based guidelines can be appropriately interpreted and applied to the unique needs and circumstances of individual patients. We suggest that Drury’s warning about the limitations of nomothetic knowledge should prompt a reassessment of policies that rely too heavily on such knowledge, resulting in one-size-fits-all protocols. We also cautiously hypothesize that the need to centre the quality improvement effort on idiographic rather than nomothetic knowledge is unlikely to be unique to long covid. Indeed, such an approach may be particularly important in any condition that is complex, unpredictable, variable in presentation and clinical course, and associated with comorbidities.
Availability of data and materials
Selected qualitative data (ensuring no identifiable information) will be made available to formal research teams on reasonable request to Professor Greenhalgh at the University of Oxford, on condition that they have research ethics approval and relevant expertise. The quantitative data on NASA Lean Test have been published in full in a separate paper [ 98 ].
Abbreviations
Chronic fatigue syndrome
Intensive care unit
Jenny Ceolta-Smith
Julie Darbyshire
LOng COvid Multidisciplinary consortium Optimising Treatments and services across the NHS
Multidisciplinary team
Myalgic encephalomyelitis
Middle East Respiratory Syndrome
National Aeronautics and Space Association
Occupational therapy/ist
Post-exertional symptom exacerbation
Postural orthostatic tachycardia syndrome
Speech and language therapy
Severe Acute Respiratory Syndrome
Trisha Greenhalgh
United Kingdom
United States
World Health Organization
Perego E, Callard F, Stras L, Melville-JÛhannesson B, Pope R, Alwan N. Why the Patient-Made Term “Long Covid” is needed. Wellcome Open Res. 2020;5:224.
Article Google Scholar
Greenhalgh T, Sivan M, Delaney B, Evans R, Milne R: Long covid—an update for primary care. bmj 2022;378:e072117.
Centers for Disease Control and Prevention (US): Long COVID or Post-COVID Conditions (updated 16th December 2022). Atlanta: CDC. Accessed 2nd June 2023 at https://www.cdc.gov/coronavirus/2019-ncov/long-term-effects/index.html ; 2022.
National Institute for Health and Care Excellence (NICE) Scottish Intercollegiate Guidelines Network (SIGN) and Royal College of General Practitioners (RCGP): COVID-19 rapid guideline: managing the long-term effects of COVID-19, vol. Accessed 30th January 2022 at https://www.nice.org.uk/guidance/ng188/resources/covid19-rapid-guideline-managing-the-longterm-effects-of-covid19-pdf-51035515742 . London: NICE; 2022.
Organization WH: Post Covid-19 Condition (updated 7th December 2022), vol. Accessed 2nd June 2023 at https://www.who.int/europe/news-room/fact-sheets/item/post-covid-19-condition#:~:text=It%20is%20defined%20as%20the,months%20with%20no%20other%20explanation . Geneva: WHO; 2022.
Office for National Statistics: Prevalence of ongoing symptoms following coronavirus (COVID-19) infection in the UK: 31st March 2023. London: ONS. Accessed 30th May 2023 at https://www.ons.gov.uk/peoplepopulationandcommunity/healthandsocialcare/conditionsanddiseases/datasets/alldatarelatingtoprevalenceofongoingsymptomsfollowingcoronaviruscovid19infectionintheuk ; 2023.
Crook H, Raza S, Nowell J, Young M, Edison P: Long covid—mechanisms, risk factors, and management. bmj 2021;374.
Sudre CH, Murray B, Varsavsky T, Graham MS, Penfold RS, Bowyer RC, Pujol JC, Klaser K, Antonelli M, Canas LS. Attributes and predictors of long COVID. Nat Med. 2021;27(4):626–31.
Article CAS PubMed PubMed Central Google Scholar
Reese JT, Blau H, Casiraghi E, Bergquist T, Loomba JJ, Callahan TJ, Laraway B, Antonescu C, Coleman B, Gargano M: Generalisable long COVID subtypes: findings from the NIH N3C and RECOVER programmes. EBioMedicine 2023;87.
Thaweethai T, Jolley SE, Karlson EW, Levitan EB, Levy B, McComsey GA, McCorkell L, Nadkarni GN, Parthasarathy S, Singh U. Development of a definition of postacute sequelae of SARS-CoV-2 infection. JAMA. 2023;329(22):1934–46.
Brown DA, O’Brien KK. Conceptualising Long COVID as an episodic health condition. BMJ Glob Health. 2021;6(9): e007004.
Article PubMed Google Scholar
Tate WP, Walker MO, Peppercorn K, Blair AL, Edgar CD. Towards a Better Understanding of the Complexities of Myalgic Encephalomyelitis/Chronic Fatigue Syndrome and Long COVID. Int J Mol Sci. 2023;24(6):5124.
Ahmed H, Patel K, Greenwood DC, Halpin S, Lewthwaite P, Salawu A, Eyre L, Breen A, Connor RO, Jones A. Long-term clinical outcomes in survivors of severe acute respiratory syndrome (SARS) and Middle East respiratory syndrome coronavirus (MERS) outbreaks after hospitalisation or ICU admission: a systematic review and meta-analysis. J Rehabil Med. 2020;52(5):1–11.
Google Scholar
World Health Organisation: Clinical management of severe acute respiratory infection (SARI) when COVID-19 disease is suspected: Interim guidance (13th March 2020). Geneva: WHO. Accessed 3rd January 2023 at https://t.co/JpNdP8LcV8?amp=1 ; 2020.
Rushforth A, Ladds E, Wieringa S, Taylor S, Husain L, Greenhalgh T: Long Covid – the illness narratives. Under review for Sociology of Health and Illness 2021.
Russell D, Spence NJ. Chase J-AD, Schwartz T, Tumminello CM, Bouldin E: Support amid uncertainty: Long COVID illness experiences and the role of online communities. SSM-Qual Res Health. 2022;2: 100177.
Article PubMed PubMed Central Google Scholar
Ziauddeen N, Gurdasani D, O’Hara ME, Hastie C, Roderick P, Yao G, Alwan NA. Characteristics and impact of Long Covid: Findings from an online survey. PLoS ONE. 2022;17(3): e0264331.
Evans RA, McAuley H, Harrison EM, Shikotra A, Singapuri A, Sereno M, Elneima O, Docherty AB, Lone NI, Leavy OC. Physical, cognitive, and mental health impacts of COVID-19 after hospitalisation (PHOSP-COVID): a UK multicentre, prospective cohort study. Lancet Respir Med. 2021;9(11):1275–87.
Sykes DL, Holdsworth L, Jawad N, Gunasekera P, Morice AH, Crooks MG. Post-COVID-19 symptom burden: what is long-COVID and how should we manage it? Lung. 2021;199(2):113–9.
Altmann DM, Whettlock EM, Liu S, Arachchillage DJ, Boyton RJ: The immunology of long COVID. Nat Rev Immunol 2023:1–17.
Klein J, Wood J, Jaycox J, Dhodapkar RM, Lu P, Gehlhausen JR, Tabachnikova A, Greene K, Tabacof L, Malik AA et al : Distinguishing features of Long COVID identified through immune profiling. Nature 2023.
Chen B, Julg B, Mohandas S, Bradfute SB. Viral persistence, reactivation, and mechanisms of long COVID. Elife. 2023;12: e86015.
Wang C, Ramasamy A, Verduzco-Gutierrez M, Brode WM, Melamed E. Acute and post-acute sequelae of SARS-CoV-2 infection: a review of risk factors and social determinants. Virol J. 2023;20(1):124.
Cervia-Hasler C, Brüningk SC, Hoch T, Fan B, Muzio G, Thompson RC, Ceglarek L, Meledin R, Westermann P, Emmenegger M et al Persistent complement dysregulation with signs of thromboinflammation in active Long Covid Science 2024;383(6680):eadg7942.
Sivan M, Greenhalgh T, Darbyshire JL, Mir G, O’Connor RJ, Dawes H, Greenwood D, O’Connor D, Horton M, Petrou S. LOng COvid Multidisciplinary consortium Optimising Treatments and servIces acrOss the NHS (LOCOMOTION): protocol for a mixed-methods study in the UK. BMJ Open. 2022;12(5): e063505.
Rushforth A, Ladds E, Wieringa S, Taylor S, Husain L, Greenhalgh T. Long covid–the illness narratives. Soc Sci Med. 2021;286: 114326.
National Institute for Health and Care Excellence: COVID-19 rapid guideline: managing the long-term effects of COVID-19, vol. Accessed 4th October 2023 at https://www.nice.org.uk/guidance/ng188/resources/covid19-rapid-guideline-managing-the-longterm-effects-of-covid19-pdf-51035515742 . London: NICE 2020.
NHS England: Long COVID: the NHS plan for 2021/22. London: NHS England. Accessed 2nd August 2022 at https://www.england.nhs.uk/coronavirus/documents/long-covid-the-nhs-plan-for-2021-22/ ; 2021.
NHS England: NHS to offer ‘long covid’ sufferers help at specialist centres. London: NHS England. Accessed 10th October 2020 at https://www.england.nhs.uk/2020/10/nhs-to-offer-long-covid-help/ ; 2020 (7th October).
NHS England: The NHS plan for improving long COVID services, vol. Acessed 4th February 2024 at https://www.england.nhs.uk/publication/the-nhs-plan-for-improving-long-covid-services/ .London: Gov.uk; 2022.
NHS England: Commissioning guidance for post-COVID services for adults, children and young people, vol. Accessed 6th February 2024 at https://www.england.nhs.uk/long-read/commissioning-guidance-for-post-covid-services-for-adults-children-and-young-people/ . London: gov.uk; 2023.
National Institute for Health Research: Researching Long Covid: Adressing a new global health challenge, vol. Accessed 9.8.23 at https://evidence.nihr.ac.uk/collection/researching-long-covid-addressing-a-new-global-health-challenge/ . London: NIHR; 2022.
Subbaraman N. NIH will invest $1 billion to study long COVID. Nature. 2021;591(7850):356–356.
Article CAS PubMed Google Scholar
Donabedian A. The definition of quality and approaches to its assessment and monitoring. Ann Arbor: Michigan; 1980.
Laffel G, Blumenthal D. The case for using industrial quality management science in health care organizations. JAMA. 1989;262(20):2869–73.
Maxwell RJ. Quality assessment in health. BMJ. 1984;288(6428):1470.
Berwick DM, Godfrey BA, Roessner J. Curing health care: New strategies for quality improvement. The Journal for Healthcare Quality (JHQ). 1991;13(5):65–6.
Deming WE. Out of the Crisis. Cambridge, MA: MIT Press; 1986.
Argyris C: Increasing leadership effectiveness: New York: J. Wiley; 1976.
Juran JM: A history of managing for quality: The evolution, trends, and future directions of managing for quality: Asq Press; 1995.
Institute of Medicine (US): Crossing the Quality Chasm: A New Health System for the 21st Century. Washington, DC: National Academy Press; 2001.
McNab D, McKay J, Shorrock S, Luty S, Bowie P. Development and application of ‘systems thinking’ principles for quality improvement. BMJ Open Qual. 2020;9(1): e000714.
Sampath B, Rakover J, Baldoza K, Mate K, Lenoci-Edwards J, Barker P. Whole-System Quality: A Unified Approach to Building Responsive, Resilient Health Care Systems. Boston: Institute for Healthcare Immprovement; 2021.
Batalden PB, Davidoff F: What is “quality improvement” and how can it transform healthcare? In . , vol. 16: BMJ Publishing Group Ltd; 2007: 2–3.
Baker G. Collaborating for improvement: the Institute for Healthcare Improvement’s breakthrough series. New Med. 1997;1:5–8.
Plsek PE. Collaborating across organizational boundaries to improve the quality of care. Am J Infect Control. 1997;25(2):85–95.
Ayers LR, Beyea SC, Godfrey MM, Harper DC, Nelson EC, Batalden PB. Quality improvement learning collaboratives. Qual Manage Healthcare. 2005;14(4):234–47.
Brandrud AS, Schreiner A, Hjortdahl P, Helljesen GS, Nyen B, Nelson EC. Three success factors for continual improvement in healthcare: an analysis of the reports of improvement team members. BMJ Qual Saf. 2011;20(3):251–9.
Dückers ML, Spreeuwenberg P, Wagner C, Groenewegen PP. Exploring the black box of quality improvement collaboratives: modelling relations between conditions, applied changes and outcomes. Implement Sci. 2009;4(1):1–12.
Nadeem E, Olin SS, Hill LC, Hoagwood KE, Horwitz SM. Understanding the components of quality improvement collaboratives: a systematic literature review. Milbank Q. 2013;91(2):354–94.
Shortell SM, Marsteller JA, Lin M, Pearson ML, Wu S-Y, Mendel P, Cretin S, Rosen M: The role of perceived team effectiveness in improving chronic illness care. Medical Care 2004:1040–1048.
Wilson T, Berwick DM, Cleary PD. What do collaborative improvement projects do? Experience from seven countries. Joint Commission J Qual Safety. 2004;30:25–33.
Schouten LM, Hulscher ME, van Everdingen JJ, Huijsman R, Grol RP. Evidence for the impact of quality improvement collaboratives: systematic review. BMJ. 2008;336(7659):1491–4.
Hulscher ME, Schouten LM, Grol RP, Buchan H. Determinants of success of quality improvement collaboratives: what does the literature show? BMJ Qual Saf. 2013;22(1):19–31.
Dixon-Woods M, Bosk CL, Aveling EL, Goeschel CA, Pronovost PJ. Explaining Michigan: developing an ex post theory of a quality improvement program. Milbank Q. 2011;89(2):167–205.
Bate P, Mendel P, Robert G: Organizing for quality: the improvement journeys of leading hospitals in Europe and the United States: CRC Press; 2007.
Andersson-Gäre B, Neuhauser D. The health care quality journey of Jönköping County Council. Sweden Qual Manag Health Care. 2007;16(1):2–9.
Törnblom O, Stålne K, Kjellström S. Analyzing roles and leadership in organizations from cognitive complexity and meaning-making perspectives. Behav Dev. 2018;23(1):63.
Greenhalgh T, Russell J. Why Do Evaluations of eHealth Programs Fail? An Alternative Set of Guiding Principles. PLoS Med. 2010;7(11): e1000360.
Wells S, Tamir O, Gray J, Naidoo D, Bekhit M, Goldmann D. Are quality improvement collaboratives effective? A systematic review. BMJ Qual Saf. 2018;27(3):226–40.
Landon BE, Wilson IB, McInnes K, Landrum MB, Hirschhorn L, Marsden PV, Gustafson D, Cleary PD. Effects of a quality improvement collaborative on the outcome of care of patients with HIV infection: the EQHIV study. Ann Intern Med. 2004;140(11):887–96.
Mittman BS. Creating the evidence base for quality improvement collaboratives. Ann Intern Med. 2004;140(11):897–901.
Wennberg JE. Unwarranted variations in healthcare delivery: implications for academic medical centres. BMJ. 2002;325(7370):961–4.
Bungay H. Cancer and health policy: the postcode lottery of care. Soc Policy Admin. 2005;39(1):35–48.
Wennberg JE, Cooper MM: The Quality of Medical Care in the United States: A Report on the Medicare Program: The Dartmouth Atlas of Health Care 1999: The Center for the Evaluative Clinical Sciences [Internet]. 1999.
DaSilva P, Gray JM. English lessons: can publishing an atlas of variation stimulate the discussion on appropriateness of care? Med J Aust. 2016;205(S10):S5–7.
Gray WK, Day J, Briggs TW, Harrison S. Identifying unwarranted variation in clinical practice between healthcare providers in England: Analysis of administrative data over time for the Getting It Right First Time programme. J Eval Clin Pract. 2021;27(4):743–50.
Wabe N, Thomas J, Scowen C, Eigenstetter A, Lindeman R, Georgiou A. The NSW Pathology Atlas of Variation: Part I—Identifying Emergency Departments With Outlying Laboratory Test-Ordering Practices. Ann Emerg Med. 2021;78(1):150–62.
Jamal A, Babazono A, Li Y, Fujita T, Yoshida S, Kim SA. Elucidating variations in outcomes among older end-stage renal disease patients on hemodialysis in Fukuoka Prefecture, Japan. PLoS ONE. 2021;16(5): e0252196.
Sutherland K, Levesque JF. Unwarranted clinical variation in health care: definitions and proposal of an analytic framework. J Eval Clin Pract. 2020;26(3):687–96.
Tanenbaum SJ. Reducing variation in health care: The rhetorical politics of a policy idea. J Health Polit Policy Law. 2013;38(1):5–26.
Atsma F, Elwyn G, Westert G. Understanding unwarranted variation in clinical practice: a focus on network effects, reflective medicine and learning health systems. Int J Qual Health Care. 2020;32(4):271–4.
Horbar JD, Rogowski J, Plsek PE, Delmore P, Edwards WH, Hocker J, Kantak AD, Lewallen P, Lewis W, Lewit E. Collaborative quality improvement for neonatal intensive care. Pediatrics. 2001;107(1):14–22.
Van Maanen J: Tales of the field: On writing ethnography: University of Chicago Press; 2011.
Golden-Biddle K, Locke K. Appealing work: An investigation of how ethnographic texts convince. Organ Sci. 1993;4(4):595–616.
Braun V, Clarke V. Using thematic analysis in psychology. Qual Res Psychol. 2006;3(2):77–101.
Glaser BG. The constant comparative method of qualitative analysis. Soc Probl. 1965;12:436–45.
Willis R. The use of composite narratives to present interview findings. Qual Res. 2019;19(4):471–80.
Vojdani A, Vojdani E, Saidara E, Maes M. Persistent SARS-CoV-2 Infection, EBV, HHV-6 and other factors may contribute to inflammation and autoimmunity in long COVID. Viruses. 2023;15(2):400.
Choutka J, Jansari V, Hornig M, Iwasaki A. Unexplained post-acute infection syndromes. Nat Med. 2022;28(5):911–23.
Connors JM, Ariëns RAS. Uncertainties about the roles of anticoagulation and microclots in postacute sequelae of severe acute respiratory syndrome coronavirus 2 infection. J Thromb Haemost. 2023;21(10):2697–701.
Patel MA, Knauer MJ, Nicholson M, Daley M, Van Nynatten LR, Martin C, Patterson EK, Cepinskas G, Seney SL, Dobretzberger V. Elevated vascular transformation blood biomarkers in Long-COVID indicate angiogenesis as a key pathophysiological mechanism. Mol Med. 2022;28(1):122.
Greenhalgh T, Sivan M, Delaney B, Evans R, Milne R: Long covid—an update for primary care. bmj 2022, 378.
Parkin A, Davison J, Tarrant R, Ross D, Halpin S, Simms A, Salman R, Sivan M. A multidisciplinary NHS COVID-19 service to manage post-COVID-19 syndrome in the community. J Prim Care Commun Health. 2021;12:21501327211010990.
NHS England: COVID-19 Post-Covid Assessment Service, vol. Accessed 5th March 2024 at https://www.england.nhs.uk/statistics/statistical-work-areas/covid-19-post-covid-assessment-service/ . London: NHS England; 2024.
Sivan M, Halpin S, Gee J, Makower S, Parkin A, Ross D, Horton M, O'Connor R: The self-report version and digital format of the COVID-19 Yorkshire Rehabilitation Scale (C19-YRS) for Long Covid or Post-COVID syndrome assessment and monitoring. Adv Clin Neurosci Rehabil 2021;20(3).
The EuroQol Group. EuroQol-a new facility for the measurement of health-related quality of life. Health Policy. 1990;16(3):199–208.
Sivan M, Preston NJ, Parkin A, Makower S, Gee J, Ross D, Tarrant R, Davison J, Halpin S, O’Connor RJ, et al. The modified COVID-19 Yorkshire Rehabilitation Scale (C19-YRSm) patient-reported outcome measure for Long Covid or Post-COVID syndrome. J Med Virol. 2022;94(9):4253–64.
Johns MW. A new method for measuring daytime sleepiness: the Epworth sleepiness scale. Sleep. 1991;14(6):540–5.
Kroenke K, Spitzer RL, Williams JB. The PHQ-9: validity of a brief depression severity measure. J Gen Intern Med. 2001;16(9):606–13.
Van Dixhoorn J, Duivenvoorden H. Efficacy of Nijmegen Questionnaire in recognition of the hyperventilation syndrome. J Psychosom Res. 1985;29(2):199–206.
Evans R, Pick A, Lardner R, Masey V, Smith N, Greenhalgh T: Breathing difficulties after covid-19: a guide for primary care. BMJ 2023;381.
Van Dixhoorn J, Folgering H: The Nijmegen Questionnaire and dysfunctional breathing. In . , vol. 1: Eur Respiratory Soc; 2015.
Courtney R, Greenwood KM. Preliminary investigation of a measure of dysfunctional breathing symptoms: The Self Evaluation of Breathing Questionnaire (SEBQ). Int J Osteopathic Med. 2009;12(4):121–7.
Espinosa-Gonzalez A, Master H, Gall N, Halpin S, Rogers N, Greenhalgh T. Orthostatic tachycardia after covid-19. BMJ (Clinical Research ed). 2023;380:e073488–e073488.
PubMed Google Scholar
Bungo M, Charles J, Johnson P Jr. Cardiovascular deconditioning during space flight and the use of saline as a countermeasure to orthostatic intolerance. Aviat Space Environ Med. 1985;56(10):985–90.
CAS PubMed Google Scholar
Sivan M, Corrado J, Mathias C. The Adapted Autonomic Profile (Aap) Home-Based Test for the Evaluation of Neuro-Cardiovascular Autonomic Dysfunction. Adv Clin Neurosci Rehabil. 2022;3:10–13. https://doi.org/10.47795/QKBU46715 .
Lee C, Greenwood DC, Master H, Balasundaram K, Williams P, Scott JT, Wood C, Cooper R, Darbyshire JL, Gonzalez AE. Prevalence of orthostatic intolerance in long covid clinic patients and healthy volunteers: A multicenter study. J Med Virol. 2024;96(3): e29486.
World Health Organization: Clinical management of covid-19 - living guideline. Geneva: WHO. Accessed 4th October 2023 at https://www.who.int/publications/i/item/WHO-2019-nCoV-clinical-2021-2 ; 2023.
Ahmed I, Mustafaoglu R, Yeldan I, Yasaci Z, Erhan B: Effect of pulmonary rehabilitation approaches on dyspnea, exercise capacity, fatigue, lung functions and quality of life in patients with COVID-19: A Systematic Review and Meta-Analysis. Arch Phys Med Rehabil 2022.
Dillen H, Bekkering G, Gijsbers S, Vande Weygaerde Y, Van Herck M, Haesevoets S, Bos DAG, Li A, Janssens W, Gosselink R, et al. Clinical effectiveness of rehabilitation in ambulatory care for patients with persisting symptoms after COVID-19: a systematic review. BMC Infect Dis. 2023;23(1):419.
Learmonth Y, Dlugonski D, Pilutti L, Sandroff B, Klaren R, Motl R. Psychometric properties of the fatigue severity scale and the modified fatigue impact scale. J Neurol Sci. 2013;331(1–2):102–7.
Webster K, Cella D, Yost K. The Functional Assessment of Chronic Illness T herapy (FACIT) Measurement System: properties, applications, and interpretation. Health Qual Life Outcomes. 2003;1(1):1–7.
Mundt JC, Marks IM, Shear MK, Greist JM. The Work and Social Adjustment Scale: a simple measure of impairment in functioning. Br J Psychiatry. 2002;180(5):461–4.
Chalder T, Berelowitz G, Pawlikowska T, Watts L, Wessely S, Wright D, Wallace E. Development of a fatigue scale. J Psychosom Res. 1993;37(2):147–53.
Shahid A, Wilkinson K, Marcu S, Shapiro CM: Visual analogue scale to evaluate fatigue severity (VAS-F). In: STOP, THAT and one hundred other sleep scales . edn.: Springer; 2011:399–402.
Parker M, Sawant HB, Flannery T, Tarrant R, Shardha J, Bannister R, Ross D, Halpin S, Greenwood DC, Sivan M. Effect of using a structured pacing protocol on post-exertional symptom exacerbation and health status in a longitudinal cohort with the post-COVID-19 syndrome. J Med Virol. 2023;95(1): e28373.
Kenny RA, Bayliss J, Ingram A, Sutton R. Head-up tilt: a useful test for investigating unexplained syncope. The Lancet. 1986;327(8494):1352–5.
Drury MOC: Science and Psychology. In: The selected writings of Maurice O’Connor Drury: On Wittgenstein, philosophy, religion and psychiatry. edn.: Bloomsbury Publishing; 2017.
Concato J, Shah N, Horwitz RI. Randomized, controlled trials, observational studies, and the hierarchy of research designs. N Engl J Med. 2000;342(25):1887–92.
Mongtomery K: How doctors think: Clinical judgment and the practice of medicine: Oxford University Press; 2005.
Download references
Acknowledgements
We are grateful to clinic staff for allowing us to study their work and to patients for allowing us to sit in on their consultations. We also thank the funder of LOCOMOTION (National Institute for Health Research) and the patient advisory group for lived experience input.
This research is supported by National Institute for Health Research (NIHR) Long Covid Research Scheme grant (Ref COV-LT-0016).
Author information
Authors and affiliations.
Nuffield Department of Primary Care Health Sciences, University of Oxford, Woodstock Rd, Oxford, OX2 6GG, UK
Trisha Greenhalgh, Julie L. Darbyshire & Emma Ladds
Imperial College Healthcare NHS Trust, London, UK
LOCOMOTION Patient Advisory Group and Lived Experience Representative, London, UK
You can also search for this author in PubMed Google Scholar
Contributions
TG conceptualized the overall study, led the empirical work, supported the quality improvement meetings, conducted the ethnographic visits, led the data analysis, developed the theorization and wrote the first draft of the paper. JLD organized and led the quality improvement meetings, supported site-based researchers to collect and analyse data on their clinic, collated and summarized data on quality topics, and liaised with the patient advisory group. CL conceptualized and led the quality topic on POTS, including exploring reasons for some clinics’ reluctance to conduct testing and collating and analysing the NASA Lean Test data across all sites. EL assisted with ethnographic visits, data analysis, and theorization. JCS contributed lived experience of long covid and also clinical experience as an occupational therapist; she liaised with the wider patient advisory group, whose independent (patient-led) audit of long covid clinics informed the quality improvement prioritization exercise. All authors provided extensive feedback on drafts and contributed to discussions and refinements. All authors read and approved the final manuscript.
Corresponding author
Correspondence to Trisha Greenhalgh .
Ethics declarations
Ethics approval and consent to participate.
LOng COvid Multidisciplinary consortium Optimising Treatments and servIces acrOss the NHS study is sponsored by the University of Leeds and approved by Yorkshire & The Humber—Bradford Leeds Research Ethics Committee (ref: 21/YH/0276) and subsequent amendments.
Patient participants in clinic were approached by the clinician (without the researcher present) and gave verbal informed consent for a clinically qualified researcher to observe the consultation. If they consented, the researcher was then invited to sit in. A written record was made in field notes of this verbal consent. It was impractical to seek consent from patients whose cases were discussed (usually with very brief clinical details) in online MDTs. Therefore, clinical case examples from MDTs presented in the paper are fictionalized cases constructed from multiple real cases and with key clinical details changed (for example, comorbidities were replaced with different conditions which would produce similar symptoms). All fictionalized cases were checked by our patient advisory group to check that they were plausible to lived experience experts.
Consent for publication
No direct patient cases are reported in this manuscript. For details of how the fictionalized cases were constructed and validated, see “Consent to participate” above.
Competing interests
TG was a member of the UK National Long Covid Task Force 2021–2023 and on the Oversight Group for the NICE Guideline on Long Covid 2021–2022. She is a member of Independent SAGE.
Additional information
Publisher’s note.
Springer Nature remains neutral with regard to jurisdictional claims in published maps and institutional affiliations.
Rights and permissions
Open Access This article is licensed under a Creative Commons Attribution 4.0 International License, which permits use, sharing, adaptation, distribution and reproduction in any medium or format, as long as you give appropriate credit to the original author(s) and the source, provide a link to the Creative Commons licence, and indicate if changes were made. The images or other third party material in this article are included in the article's Creative Commons licence, unless indicated otherwise in a credit line to the material. If material is not included in the article's Creative Commons licence and your intended use is not permitted by statutory regulation or exceeds the permitted use, you will need to obtain permission directly from the copyright holder. To view a copy of this licence, visit http://creativecommons.org/licenses/by/4.0/ . The Creative Commons Public Domain Dedication waiver ( http://creativecommons.org/publicdomain/zero/1.0/ ) applies to the data made available in this article, unless otherwise stated in a credit line to the data.
Reprints and permissions
About this article
Cite this article.
Greenhalgh, T., Darbyshire, J.L., Lee, C. et al. What is quality in long covid care? Lessons from a national quality improvement collaborative and multi-site ethnography. BMC Med 22 , 159 (2024). https://doi.org/10.1186/s12916-024-03371-6
Download citation
Received : 04 December 2023
Accepted : 26 March 2024
Published : 15 April 2024
DOI : https://doi.org/10.1186/s12916-024-03371-6
Share this article
Anyone you share the following link with will be able to read this content:
Sorry, a shareable link is not currently available for this article.
Provided by the Springer Nature SharedIt content-sharing initiative
- Post-covid-19 syndrome
- Quality improvement
- Breakthrough collaboratives
- Warranted variation
- Unwarranted variation
- Improvement science
- Ethnography
- Idiographic reasoning
- Nomothetic reasoning
BMC Medicine
ISSN: 1741-7015
- Submission enquiries: [email protected]
- General enquiries: [email protected]
- Skip to main content
- Skip to FDA Search
- Skip to in this section menu
- Skip to footer links

The .gov means it’s official. Federal government websites often end in .gov or .mil. Before sharing sensitive information, make sure you're on a federal government site.
The site is secure. The https:// ensures that you are connecting to the official website and that any information you provide is encrypted and transmitted securely.
U.S. Food and Drug Administration
- Search
- Menu
- Science & Research
- Science and Research Special Topics
- Advancing Regulatory Science
Statistical methods to improve precision and reduce the required sample size in many phase 2 and 3 clinical trials, including COVID-19 trials, by covariate adjustment
CERSI Collaborators: Michael Rosenblum, PhD; Joshua Betz, MS; Kelly Van Lancker, PhD; Bingkai Wang, PhD
FDA Collaborators: Daniel Rubin, PhD, CDER; Greg Levin, PhD, CDER; Boguang Zhen, PhD, CBER; Gene Pennello, PhD, CDRH
CERSI Subcontractors: Weill Cornell Medicine Art Sedrakyan, MD, PhD; Jim C. Hu, MD, MPH; Jialin Mao, MD, MS; Miko Yu, MA; Sendong Zhao, PHD, Vahan Simonyan, PhD
Project Start Date: March 1, 2020
Regulatory Science Challenge
Investigators are addressing the following FDA research priority: “Developing methods and tools to improve and streamline clinical and post-market evaluation of FDA-regulated products.”
Project Description and Goals
Clinical trials are often conducted to learn whether new medical treatments are safe and effective. Data collected when participants first enter a trial are called baseline variables. Examples of baseline variables include age, sex, and disease severity. Sometimes, due to chance, there are imbalances in these variables between those assigned to the experimental treatment arm (or group) and those assigned to the control arm (or group). For example, in some trials participants in the treatment arm may have higher or lower baseline disease severity compared with participants in the control arm.
When baseline variables (e.g., older age as a risk factor for worse outcome among those with COVID-19 infection) are related to the outcome, (e.g., one intended to support effectiveness of a treatment), taking the baseline variables into account in the data analysis of a trial’s results can lead to more precise estimates of treatment effectiveness (i.e., smaller standard errors for the estimates). More precise estimation means that at the planning stage, trials could be conducted with fewer participants than when baseline variables are not considered in the data analysis. Unfortunately, in many clinical trials information in baseline variables is not considered, leading to a greater chance that the trial will fail due to greater uncertainty regarding conclusions that can be drawn, potentially wasting resources. At the planning stage, not accounting for baseline information may lead to the planning of a larger trial size and longer trial duration than is necessary.
A major barrier to using baseline variables (called covariate adjustment) is that for many common types of clinical trial outcomes, such as binary, ordinal, and time-to-event outcomes, confusion remains as to what statistical approach is appropriate. The results of this project will help to overcome this barrier by demonstrating how to appropriately adjust for baseline variables to improve precision of treatment effect estimates for outcomes of these types. The statistical adjustments will be demonstrated on case studies in several disease areas as examples of best practices for the use of baseline variables in clinical trial data analysis. Investigators plan to disseminate these case studies to the public through a free, online tutorial on the FDA public website.
Publications
Williams, N., Rosenblum, M. & Díaz, I. (2022) Optimising precision and power by machine learning in randomised trials with ordinal and time-to-event outcomes with an application to COVID-19. Journal of the Royal Statistical Society: Series A (Statistics in Society) , 1– 23. https://doi.org/10.1111/rssa.12915
Wang, B., Susukida, R., Mojtabai, R., Amin-Esmaeili, M., and Rosenblum, M. (2021) Model-Robust Inference for Clinical Trials that Improve Precision by Stratified Randomization and Adjustment for Additional Baseline Variables. Journal of the American Statistical Association, Theory and Methods Section. https://www.tandfonline.com/doi/full/10.1080/01621459.2021.1981338
Kelly Van Lancker, Joshua Betz, Michael Rosenblum. Combining Covariate Adjustment with Group Sequential, Information Adaptive Designs to Improve Randomized Trial Efficiency. Under review: https://arxiv.org/abs/2201.12921
- See us on facebook
- See us on twitter
- See us on youtube
- See us on linkedin
- See us on instagram
Stanford Medicine study flags unexpected cells in lung as suspected source of severe COVID
A previously overlooked type of immune cell allows SARS-CoV-2 to proliferate, Stanford Medicine scientists have found. The discovery has important implications for preventing severe COVID-19.
April 10, 2024 - By Bruce Goldman
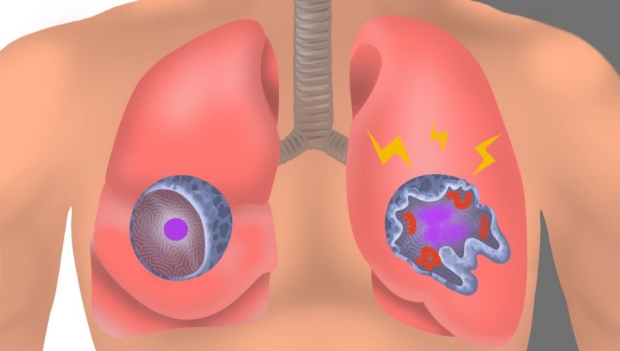
In an uninfected interstitial macrophage, the nucleus (purple) and outer cell membrane (blue) are intact. In an infected interstitial macrophage, the nucleus is shattered, copious newly made viral components (red) clump together, and the cell broadcasts inflammatory and scar-tissue-inducing chemical signals (yellow). Emily Moskal
The lung-cell type that’s most susceptible to infection by SARS-CoV-2, the virus that causes COVID-19, is not the one previously assumed to be most vulnerable. What’s more, the virus enters this susceptible cell via an unexpected route. The medical consequences may be significant.
Stanford Medicine investigators have implicated a type of immune cell known as an interstitial macrophage in the critical transition from a merely bothersome COVID-19 case to a potentially deadly one. Interstitial macrophages are situated deep in the lungs, ordinarily protecting that precious organby, among other things, engorging viruses, bacteria, fungi and dust particles that make their way down our airways. But it’s these very cells, the researchers have shown in a study published online April 10 in the Journal of Experimental Medicine , that of all known types of cells composing lung tissue are most susceptible to infection by SARS-CoV-2.
SARS-CoV-2-infected interstitial macrophages, the scientists have learned, morph into virus producersand squirt out inflammatory and scar-tissue-inducing chemical signals, potentially paving the road to pneumonia and damaging the lungs to the point where the virus, along with those potent secreted substances, can break out of the lungs and wreak havoc throughout the body.
The surprising findings point to new approaches in preventing a SARS-CoV-2 infection from becoming a life-threatening disease. Indeed, they may explain why monoclonal antibodies meant to combat severe COVID didn’t work well, if at all — and when they did work, it was only when they were administered early in the course of infection, when the virus was infecting cells in the upper airways leading to the lungs but hadn’t yet ensconced itself in lung tissue.
The virus surprises
“We’ve overturned a number of false assumptions about how the virus actually replicates in the human lung,” said Catherine Blish , MD, PhD, a professor of infectious diseases and of microbiology and immunology and the George E. and Lucy Becker Professor in Medicine and associate dean for basic and translational research.
Blish is the co-senior author of the study, along with Mark Krasnow , MD, PhD, the Paul and Mildred Berg Professor of biochemistry and the Executive Director of the Vera Moulton Wall Center for pulmonary vascular disease.
“The critical step, we think, is when the virus infects interstitial macrophages, triggering a massive inflammatory reaction that can flood the lungs and spread infection and inflammation to other organs,” Krasnow said. Blocking that step, he said, could prove to be a major therapeutic advance. But there’s a plot twist: The virus has an unusual way of getting inside these cells — a route drug developers have not yet learned how to block effectively — necessitating a new focus on that alternative mechanism, he added.
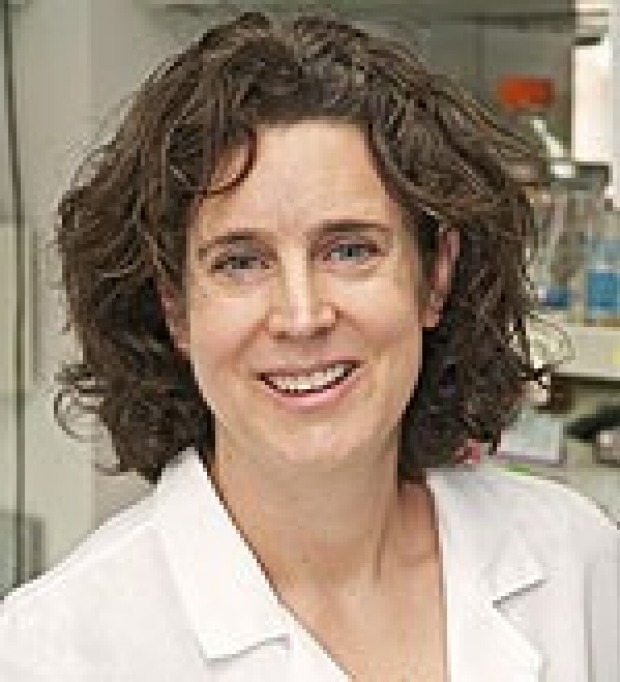
Catherine Blish
In a paper published in Nature in early 2020, Krasnow and his colleagues including then-graduate student Kyle Travaglini, PhD — who is also one of the new study’s co-lead authors along with MD-PhD student Timothy Wu — described a technique they’d worked out for isolating fresh human lungs; dissociating the cells from one another; and characterizing them, one by one, on the basis of which genes within each cell were active and how much so. Using that technique, the Krasnow lab and collaborators were able to discern more than 50 distinct cell types, assembling an atlas of healthy lung cells.
“We’d just compiled this atlas when the COVID-19 pandemic hit,” Krasnow said. Soon afterward, he learned that Blish and Arjun Rustagi , MD, PhD, instructor of infectious diseases and another lead co-author of the study, were building an ultra-safe facility where they could safely grow SARS-CoV-2 and infect cells with it.
A collaboration ensued. Krasnow and Blish and their associates obtained fresh healthy lung tissue excised from seven surgical patients and five deceased lung donors whose lungs were virus-free but for one reason or another not used in transplants. After infecting the lung tissue with SARS-CoV-2 and waiting one to three days for the infection to spread, they separated and typed the cells to generate an infected-lung-cell atlas, analogous to the one Krasnow’s team had created with healthy lung cells. They saw most of the cell types that Krasnow’s team had identified in healthy lung tissue.
Now the scientists could compare pristine versus SARS-CoV-2-infected lungs cells of the same cell type and see how they differed: They wanted to know which cells the virus infected, how easily SARS-CoV-2 replicated in infected cells, and which genes the infected cells cranked up or dialed down compared with their healthy counterparts’ activity levels. They were able to do this for each of the dozens of different cell types they’d identified in both healthy and infected lungs.
“It was a straightforward experiment, and the questions we were asking were obvious,” Krasnow said. “It was the answers we weren’t prepared for.”
It’s been assumed that the cells in the lungs that are most vulnerable to SARS-CoV-2 infection are those known as alveolar type 2 cells. That’s because the surfaces of these cells, along with those of numerous other cell types in the heart, gut and other organs, sport many copies of a molecule known as ACE2. SARS-CoV-2 has been shown to be able to grab onto ACE2 and manipulate it in a way that allows the virus to maneuver its way into cells.
Alveolar type 2 cells are somewhat vulnerable to SARS-CoV-2, the scientists found. But the cell types that were by far the most frequently infected turned out to be two varieties of a cell type called a macrophage.
Virus factories
The word “macrophage” comes from two Greek terms meaning, roughly, “big eater.” This name is not unearned. The air we inhale carries not only oxygen but, unfortunately, tiny airborne dirt particles, fungal spores, bacteria and viruses. A macrophage earns its keep by, among other things, gobbling up these foreign bodies.
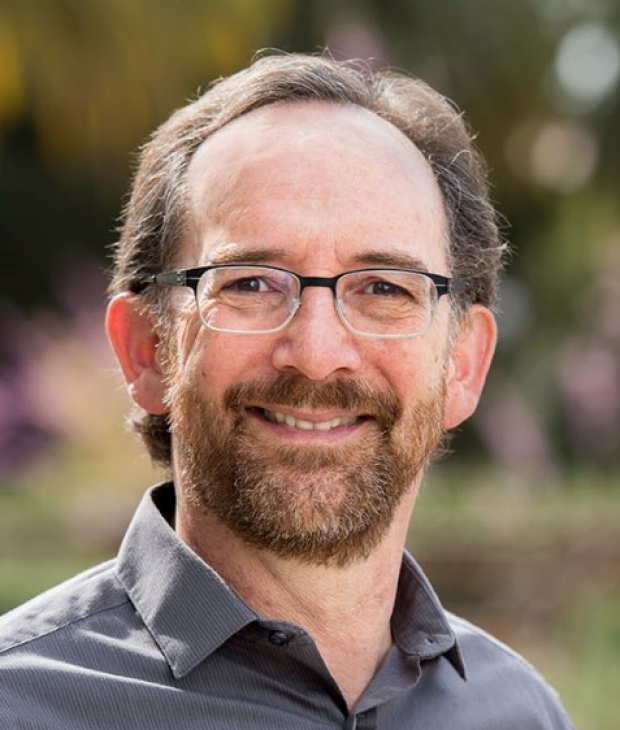
Mark Krasnow
The airways leading to our lungs culminate in myriad alveoli, minuscule one-cell-thick air sacs, whichare abutted by abundant capillaries. This interface, called the interstitium, is where oxygen in the air we breathe enters the bloodstream and is then distributed to the rest of the body by the circulatory system.
The two kinds of SARS-CoV-2-susceptible lung-associated macrophages are positioned in two different places. So-called alveolar macrophages hang out in the air spaces within the alveoli. Once infected, these cells smolder, producing and dribbling out some viral progeny at a casual pace but more or less keeping a stiff upper lip and maintaining their normal function. This behavior may allow them to feed SARS-CoV-2’s progression by incubating and generating a steady supply of new viral particles that escape by stealth and penetrate the layer of cells enclosing the alveoli.
Interstitial macrophages, the other cell type revealed to be easily and profoundly infected by SARS-CoV-2, patrol the far side of the alveoli, where the rubber of oxygen meets the road of red blood cells. If an invading viral particle or other microbe manages to evade alveolar macrophages’ vigilance, infect and punch through the layer of cells enclosing the alveoli, jeopardizing not only the lungs but the rest of the body, interstitial macrophages are ready to jump in and protect the neighborhood.
At least, usually. But when an interstitial macrophage meets SARS-CoV-2, it’s a different story. Rather than get eaten by the omnivorous immune cell, the virus infects it.
And an infected interstitial macrophage doesn’t just smolder; it catches on fire. All hell breaks loose as the virus literally seizes the controls and takes over, hijacking a cell’s protein- and nucleic-acid-making machinery. In the course of producing massive numbers of copies of itself, SARS-CoV-2 destroys the boundaries separating the cell nucleus from the rest of the cell like a spatula shattering and scattering the yolk of a raw egg. The viral progeny exit the spent macrophage and move on to infect other cells.
But that’s not all. In contrast to alveolar macrophages, infected interstitial macrophages pump out substances that signal other immune cells elsewhere in the body to head for the lungs. In a patient, Krasnow suggested, this would trigger an inflammatory influx of such cells. As the lungs fill with cells and fluid that comes with them, oxygen exchange becomes impossible. The barrier maintaining alveolar integrity grows progressively damaged. Leakage of infected fluids from damaged alveoli propels viral progeny into the bloodstream, blasting the infection and inflammation to distant organs.
Yet other substances released by SARS-CoV-2-infected interstitial macrophages stimulate the production of fibrous material in connective tissue, resulting in scarring of the lungs. In a living patient, the replacement of oxygen-permeable cells with scar tissue would further render the lungs incapable of executing oxygen exchange.
“We can’t say that a lung cell sitting in a dish is going to get COVID,” Blish said. “But we suspect this may be the point where, in an actual patient, the infection transitions from manageable to severe.”
Another point of entry
Compounding this unexpected finding is the discovery that SARS-CoV-2 uses a different route to infect interstitial macrophages than the one it uses to infect the other types.
Unlike alveolar type 2 cells and alveolar macrophages, to which the virus gains access by clinging to ACE2 on their surfaces, SARS-CoV-2 breaks into interstitial macrophages using a different receptor these cells display. In the study, blocking SARS-CoV-2’s binding to ACE2 protected the former cells but failed to dent the latter cells’ susceptibility to SARS-CoV-2 infection.
“SARS-CoV-2 was not using ACE2 to get into interstitial macrophages,” Krasnow said. “It enters via another receptor called CD209.”
That would seem to explain why monoclonal antibodies developed specifically to block SARS-CoV-2/ACE2 interaction failed to mitigate or prevent severe COVID-19 cases.
It’s time to find a whole new set of drugs that can impede SARS-CoV-2/CD209 binding. Now, Krasnow said.
The study was funded by the National Institutes of Health (grants K08AI163369, T32AI007502 and T32DK007217), the Bill & Melinda Gates Foundation, Chan Zuckerberg Biohub, the Burroughs Wellcome Fund, Stanford Chem-H, the Stanford Innovative Medicine Accelerator, and the Howard Hughes Medical Institute.

About Stanford Medicine
Stanford Medicine is an integrated academic health system comprising the Stanford School of Medicine and adult and pediatric health care delivery systems. Together, they harness the full potential of biomedicine through collaborative research, education and clinical care for patients. For more information, please visit med.stanford.edu .
Artificial intelligence
Exploring ways AI is applied to health care
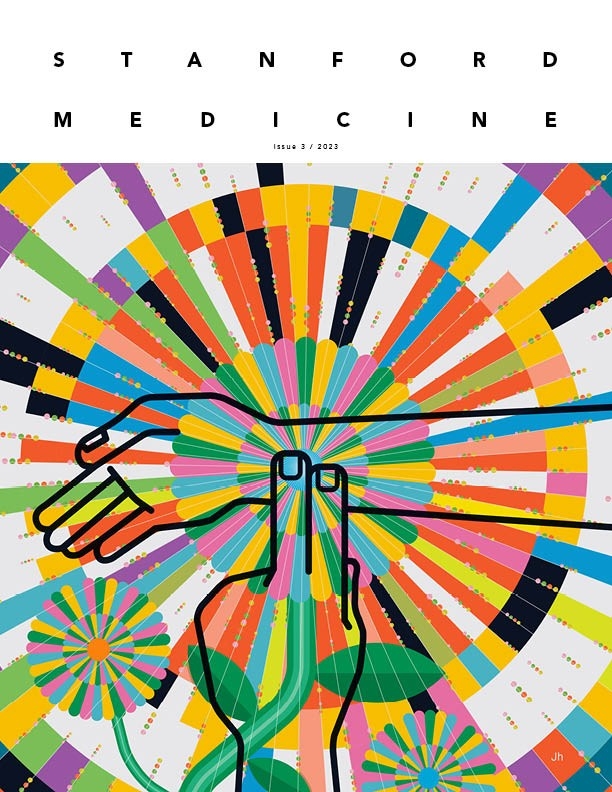
Thank you for visiting nature.com. You are using a browser version with limited support for CSS. To obtain the best experience, we recommend you use a more up to date browser (or turn off compatibility mode in Internet Explorer). In the meantime, to ensure continued support, we are displaying the site without styles and JavaScript.
- View all journals
- My Account Login
- Explore content
- About the journal
- Publish with us
- Sign up for alerts
- Open access
- Published: 28 September 2021
News media coverage of COVID-19 public health and policy information
- Katharine J. Mach 1 , 2 ,
- Raúl Salas Reyes ORCID: orcid.org/0000-0002-1683-8516 3 ,
- Brian Pentz ORCID: orcid.org/0000-0003-2713-6699 3 ,
- Jennifer Taylor ORCID: orcid.org/0000-0002-8301-3434 4 ,
- Clarissa A. Costa 3 ,
- Sandip G. Cruz 3 ,
- Kerronia E. Thomas 3 ,
- James C. Arnott ORCID: orcid.org/0000-0003-3989-6724 5 ,
- Rosalind Donald 1 ,
- Kripa Jagannathan ORCID: orcid.org/0000-0003-4584-8358 6 , 7 ,
- Christine J. Kirchhoff ORCID: orcid.org/0000-0002-2686-6764 8 ,
- Laura C. Rosella ORCID: orcid.org/0000-0003-4867-869X 9 &
- Nicole Klenk ORCID: orcid.org/0000-0001-8224-6992 3
Humanities and Social Sciences Communications volume 8 , Article number: 220 ( 2021 ) Cite this article
37k Accesses
55 Citations
72 Altmetric
Metrics details
- Cultural and media studies
- Science, technology and society
During a pandemic, news media play a crucial role in communicating public health and policy information. Traditional newspaper coverage is important amidst increasing disinformation, yet uncertainties make covering health risks and efforts to limit transmission difficult. This study assesses print and online newspaper coverage of the coronavirus disease COVID-19 for March 2020, when the global pandemic was declared, through August 2020 in three countries: Canada (with the lowest per-capita case and death rates during the study timeframe), the United Kingdom (with a pronounced early spike), and the United States (with persistently high rates). Tools previously validated for pandemic-related news records allow measurement of multiple indicators of scientific quality (i.e., reporting that reflects the state of scientific knowledge) and of sensationalism (i.e., strategies rendering news as more extraordinary than it really is). COVID-19 reporting had moderate scientific quality and low sensationalism across 1331 sampled articles in twelve newspapers spanning the political spectrums of the three countries. Newspapers oriented towards the populist-right had the lowest scientific quality in reporting, combined with very low sensationalism in some cases. Against a backdrop of world-leading disease rates, U.S. newspapers on the political left had more exposing coverage, e.g., focused on policy failures or misinformation, and more warning coverage, e.g., focused on the risks of the disease, compared to U.S. newspapers on the political right. Despite the generally assumed benefits of low sensationalism, pandemic-related coverage with low scientific quality that also failed to alert readers to public-health risks, misinformation, or policy failures may have exacerbated the public-health effects of the disease. Such complexities will likely remain central for both pandemic news media reporting and public-health strategies reliant upon it.
Similar content being viewed by others
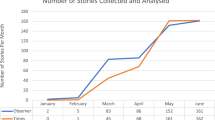
Newspapers’ coverage of the COVID-19 pandemic in Eswatini: from distanciated re/presentations to socio-health panics
Henri-Count Evans
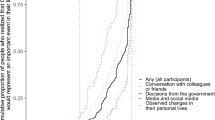
Spread of awareness of COVID-19 between December 2019 and March 2020 in France
Horace Blanc, Eliane Rothier Bautzer, … Viet-Thi Tran
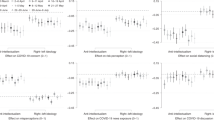
Anti-intellectualism and the mass public’s response to the COVID-19 pandemic
Eric Merkley & Peter John Loewen
Introduction
News media reporting is understood to play a central role during national security and health emergencies (Laing, 2011 ; Klemm et al., 2016 ; Pieri, 2019 ). News coverage communicates risks to readers and shapes public perceptions through the amount, content, and tone of reporting. It simultaneously frames ongoing public debates about policy responses, including conflicting priorities relevant to the timing or stringency of implemented policies (Laing, 2011 ; Pieri, 2019 ). Pandemic policy-making requires rapid, iterative responses under conditions of knowledge deficit, as well as the coordination of multi-level public-health agencies and sectors (e.g., hospitals, schools, and workplaces) (Laing, 2011 ; Rosella et al., 2013 ). In these complex circumstances, news media serve as a primary source of health information and uncertainties and connect health professionals, policymakers, and the public in critical ways (Laing, 2011 ; Hoffman and Justicz, 2016 ). The quality and balance of scientific coverage, such as through reporting that reflects the state of scientific knowledge and is not overstated, affect trust in science and accountability for decision-making (Laing, 2011 ; Klemm et al., 2016 ; Hoffman and Justicz, 2016 ).
Inadequate scientific quality in news coverage of past pandemics has posed risks and limited capacities to disseminate public-health guidance and coordinate responses (Hoffman and Justicz, 2016 ). Reporting on the state of scientific knowledge during a novel, evolving pandemic is challenging. Low-quality scientific reporting of pandemics may overstate or understate disease risks or the efficacy of protective measures for different individuals or fail to communicate the nature of the evidence. Such reporting may constrain the feasibility or effectiveness of options for policymakers directing government action, miss opportunities to inform individuals making health decisions, and increase the exposure of health professionals to disease. It can both exacerbate disease outcomes and generate unnecessary fear, in combination with other factors shaping perceptions among the public (Laing, 2011 ; Klemm et al., 2016 ; Hoffman and Justicz, 2016 ). For example, news media reporting may have overly emphasized the threat of the 2009 A/H1N1 influenza (H1N1) pandemic with insufficient indication of available protective measures, and in pairing trustworthy information from credible scientists with uninformed opinions, it may have promoted a “false balance” (Laing, 2011 ; Klemm et al., 2016 ; Hoffman and Justicz, 2016 ). Further, news coverage rapidly waned after the initial pandemic declaration even though public-health risks persisted (Klemm et al., 2016 ; Reintjes et al., 2016 ). Similar issues with media reporting occurred during the 2003 severe acute respiratory syndrome (SARS) outbreak and the 2014 Ebola outbreak (Hoffman and Justicz, 2016 ; Pieri, 2019 ).
During the COVID-19 pandemic, media representations of complex, rapidly evolving epidemiological science shape public understandings of the risks, measures to limit disease spread, and associated political and policy discourses. Traditional newspaper media coverage may have particular importance given simultaneous misinformation and disinformation, social fragmentation, political polarization, and failures of policy coordination, and national newspapers influence how other outlets cover the same subject across media platforms (Ball and Maxmen, 2020 ; Holtz et al., 2020 ; Thorp, 2020 ; Grossman et al., 2020 ). The COVID-19 pandemic creates an opportunity to assess the strengths and limitations of the media’s pandemic coverage and provide insights for future news media coverage. Such assessment also informs the communication strategies of public-health institutions and policymakers towards clear public-health guidance and coordinated responses across health systems (Laing, 2011 ; Hoffman and Justicz, 2016 ; Pieri, 2019 ).
Canada, the United Kingdom, and the United States, our countries of focus, differ in how they govern public health, including pandemic responses. In its constitutionally determined role, the Canadian federal government sets healthcare standards and administers funding to support the healthcare system spanning provinces and territories (Government of Canada, 2016 ). Pandemic health-related policies are set and implemented predominantly by provinces with federal guidance from Health Canada and the Public Health Agency of Canada (Adeel et al., 2020 ). The U.K. central government funds healthcare throughout the United Kingdom yet only sets policies for England. Northern Ireland, Scotland, and Wales each govern their own National Health Service systems. By contrast, the healthcare system in the United States is a complex mixture of public and private health insurance programs. The U.S. federal government generally adopts a leading role during national crises, although during the COVID-19 pandemic states and municipalities have led adoption and implementation of most policy measures to contain the spread of COVID-19 (Adeel et al., 2020 ). Prior to the COVID-19 pandemic, the 2019 Global Health Security Index ranked the United States first, United Kingdom second, and Canada fifth among 195 countries for preparedness to manage a serious disease outbreak (Cameron et al., 2019 ).
In this paper, we systematically quantify the amount, scientific quality, and sensationalism of newspaper media coverage of COVID-19 in Canada, the United Kingdom, and the United States. Newspapers studied span the political spectrum of each case-study country (Table 1 ) (Gentzkow and Shapiro, 2010 ; Puglisi and Snyder, 2015 ; Anderson and Coletto, 2017 ; Mitchell et al., 2018 ; Hönnige et al., 2020 ; Jurkowitz et al., 2020 ; Austen, 2020 ). Our analysis begins two weeks prior to COVID-19’s official recognition as a pandemic and follows its development over the subsequent five months (i.e., from 1 March 2020 to 15 August 2020). Given the volume of COVID-19 news media articles published over the timeframe of this study, we created a manageable corpus for analysis by randomly sampling one day of media coverage per week for six consecutive 4-week periods; we then randomly selected five eligible articles from each news outlet on each sampled day for the evaluation of scientific quality and sensationalism. In our evaluation, scientific quality refers to the alignment between reporting and the state of scientific evidence and its uncertainties, and sensationalism is a discursive strategy rendering news as more extraordinary, interesting, or relevant than it really is (Oxman et al., 1993 ; Molek-Kozakowska, 2013 ; Hoffman and Justicz, 2016 ). We apply previously validated survey tools developed to measure scientific quality and sensationalism of pandemic-related health news records in combination with broader methods from policy analyses of pandemic responses (SI Coding Tool) (Oxman et al., 1993 ; Rosella et al., 2013 ; Molek-Kozakowska, 2013 ; Reintjes et al., 2016 ; Hoffman and Justicz, 2016 ). We analyze (1) the COVID-19 public-health outcomes and policies in each country and (2) the amount, scientific quality, sensationalism, and topics of COVID-19 news media coverage across the political spectrum of each country.
Public health contextualization of news media analyses
To contextualize our news media analyses, we analyzed and visualized existing data sets on the number of COVID-19 cases, deaths, and tests in each country (e.g., Roser et al., 2020 ; CBC News, 2020 ; Public Health England and NHSX, 2020 ; CDC, 2020 ). We also recorded the key public-health declarations, policies, and guidance during the study time period (e.g., drawing from WHO, 2020a , 2020b ; see also SI Table S1 ). We tracked these decisions at international scales through to subnational scales in each country studied. Media analyses outlined below thereby were considered with respect to the reported number of cases and confirmed deaths and policy actions taken (Reintjes et al., 2016 ).
News media search strategy and inclusion criteria
Print and online news media records were retrieved from the Factiva database for news outlets across the political spectrum of Canada, the United Kingdom, and the United States (see Table 1 ) (Gentzkow and Shapiro, 2010 ; Puglisi and Snyder, 2015 ; Anderson and Coletto, 2017 ; Mitchell et al., 2018 ; Hönnige et al., 2020 ; Jurkowitz et al., 2020 ; Austen, 2020 ). Selected news media outlets have primary news products in print and online media, rather than television broadcasting or social media, and full article entries available in Factiva. Search terms included “coronavirus,” “COVID-19,” “epidemic,” “outbreak,” “pandemic,” or “SARS-CoV-2.” Individual English-language news articles were retrieved for sampled dates between 1 March 2020 and 15 August 2020. This period captures news media coverage prior to the declaration of the COVID-19 pandemic and over the subsequent five months.
Individual news records were screened to identify original news reporting (i.e., news reporting and news analysis articles) relevant to our study objectives. First, eligible articles must have a direct focus on the public-health implications of COVID-19 or on attempts to control its spread—in some or all of an article’s text. By excluding articles without this focus, we ensured all articles included in the study could contain scientific information on the public health effects or spread of COVID-19 and associated policies. Second, eligible articles must be focused on the newspaper’s country of publication (e.g., an article reporting on COVID-19 transmission or mitigation efforts in only New Zealand or China, without discussion of implications for the newspaper’s country of publication, would be excluded). We included this eligibility criterion to analyze science–policy interfaces and science–society interactions most proximate to the news outlets, although we acknowledge that articles about other countries may influence perceptions of readers even without direct discussion of implications for them. Third, eligible articles must be original news reporting or analysis, meaning we excluded opinion pieces, editorials, interview transcripts, microblogs, front-page snippets, news roundups, obituaries, advertisements, corrections memos, and letters to the editor; these excluded article types would have required distinct question framings beyond the scope of our codebook. This third criterion, therefore, ensured that coded responses could be compared coherently across articles for the different measures of scientific quality and sensationalism.
Sampling of news media articles
As the evaluation of scientific quality and sensationalism through manual coding is time intensive, and a very large number of COVID-19 news media articles were published during the timeframe of our study, we used a random sample of news media articles for analysis, prioritizing sampling during each week over the course of the study timeframe. The sample design enabled a manageable analysis of newspaper media coverage and potential changes over the timeframe of the study. First, the sample of news media articles was constructed by sampling one day of media coverage per week in consecutive four-week periods. These four days of the week were randomly sampled without replacement (Monday through Saturday only, not including Sunday in the sampling), given cyclic variation in news media coverage (Lacy et al., 2001 ; Riffe et al., 2016 ). The study timeframe was divided into six four-week periods of equal duration from 1 March to 15 August 2020.
Second, for each randomly sampled day, all available news records were retrieved from Factiva for the 12 news outlets (Table 1 ). Randomly selected articles were screened for eligibility, with the goal of identifying 5 eligible articles for each news outlet on each sampled day. In some cases, fewer than 5 eligible articles were published by a given outlet on a sampled day. In these cases, the full set of eligible articles was included in the study.
Analysis of scientific quality and sensationalism of news articles
The coding tool for measuring scientific quality and sensationalism of news article records was adapted from the final tool of Hoffman and Justicz, designed for evaluating pandemic-related health news records (Hoffman and Justicz, 2016 ). Scientific quality, as defined in that study, is “a measure of an article’s reliability and credibility on a given topic” (Hoffman and Justicz, 2016 ). Importantly, scientific quality is linked to the state of scientific understanding and its uncertainties at specific moments in time rather than being an absolute or objective characteristic. The codebook we applied for measuring scientific quality is therefore designed to be flexible and responsive to the inevitable shifts in scientific understanding that occur through time, most especially during a novel disease outbreak and evolving pandemic. Sensationalism, as defined in that study, is “a way of presenting articles to make them seem more interesting or extraordinary than they actually are” (Hoffman and Justicz, 2016 ).
Our coding tool (SI Coding Tool) included six questions for scientific quality (each evaluated on a scale from 1 to 5—5 corresponding to highest quality) and six questions for sensationalism (each evaluated on a scale from 1 to 5—5 corresponding to highest sensationalism). The question categories (SI Coding Tool) for assessing scientific quality were as follows: applicability, opinion versus facts, validity, precision, context, and global assessment (i.e., an overall assessment of the article’s scientific quality based on the five preceding specific measures). For sensationalism, the question categories (SI Coding Tool) included exposing, speculating, generalizing, warning, extolling, and global assessment (i.e., an overall assessment of the degree of sensationalism in the article based on the five preceding specific measures) (Oxman et al., 1993 ; Molek-Kozakowska, 2013 ; Hoffman and Justicz, 2016 ).
In addition, metadata collected for each article included the coder’s identity, the article title, the article’s sample date, the news outlet (including if the article was originally written by another outlet such as the Associated Press), the societal sector (up to 2 selected per article), and public-health measures discussed (SI Coding Tool).
Coder assignments, training, reliability assessment, and analysis
For each sampled day, two independent coders assessed all relevant news media records based on the scientific quality and sensationalism questions and article-attribute metadata. Coders recorded scores for each article through a Google-form version of the codebook (SI Coding Tool).
To ensure consistent application of the coding tool, substantial training and calibration occurred over a six-week period. First, the three coders in coordination with the project leadership team read national and international public-health agency descriptions of the coronavirus disease and associated public-health policies and measures. Second, the coders completed multiple rounds of individual coding of example news articles, followed by group discussions of application of the codebook. The group discussions considered difficult judgments and common versus unusual examples. The goal was to ensure consistent application of the coding tool across question categories and the range of article examples that arose. During the training and calibration phases of coding, we updated the codebook to include examples specific to news records on COVID-19 (SI Coding Tool), and we tracked illustrative examples (news articles and specific quotes) across the scale (1–3–5) for the scientific quality and sensationalism question categories. This process led to development of example answers particularly representative of low versus high scientific quality and low versus high sensationalism under each category of response. Additionally, we developed “decision rules” for the more unusual or challenging categories of examples to ensure consistency across coders, especially where disagreements arose in individually assigned responses.
Interrater reliability was assessed during the training and calibration stage and throughout the duration of the study. Where coders assigned scores for a given question that were 3 or 4 units apart on the 1–5 scale, a reconciliation discussion occurred; the small fraction of question responses in this category following the training stage enabled the coders and project team to continue developing and ensuring shared understanding of coding approaches for unusual or challenging applications. Weighted Cohen’s Kappa, with quadratic weighting, was applied given the high-inference codebook and ordinal data collected via a Likert scale, as previously done for related measures (Cohen, 1960 ; Fleiss and Cohen, 1973 ; Oxman et al., 1993 ; Antoine et al., 2014 ; Hoffman and Justicz, 2016 ; Tran et al., 2020 ). Coded data were analyzed with Kruskal–Wallis one-way analysis of variance and post-hoc multi-comparison pairwise tests (kruskal.test and kruskalmc in pgirmess package in R) (Giraudoux et al., 2018 ; R Core Team, 2020 ).
Public health and policy contexts
From March through August 2020, Canada, the United Kingdom, and the United States differed substantially in their public-health responses to COVID-19 and in health outcomes from the novel coronavirus disease (Fig. 1 ). Beginning in early March, all three countries implemented a combination of policy measures to contain the spread of COVID-19, including emergency laws, stay-at-home orders, mask mandates, school and business closures, border and travel restrictions, social distancing measures, and quarantines upon entry (SI Table S1 ). These restrictions were followed by gradual phases of reopening measures allowing restricted social and economic activities to occur. Across the three countries, the role of national versus subnational governments differed with respect to authority and actions on public-health guidance and care, resulting in differing timing and levels of coordination for both restrictions and reopening measures (SI Fig. S1 ). From March to August 2020, the United Kingdom experienced the highest death rate from COVID-19 (maximum 7-day average of 13.9 deaths per million people; Fig. 1 ), whereas the United States had the highest case rate of the three countries (maximum 7-day average of 203.5 cases per million), as well as the greatest cumulative number of cases and deaths globally (SI Figs. S2 - S3 ). Of the three countries, Canada had the most effective public-health outcomes as measured by per capita COVID-19 case or death rates (Fig. 1 ).
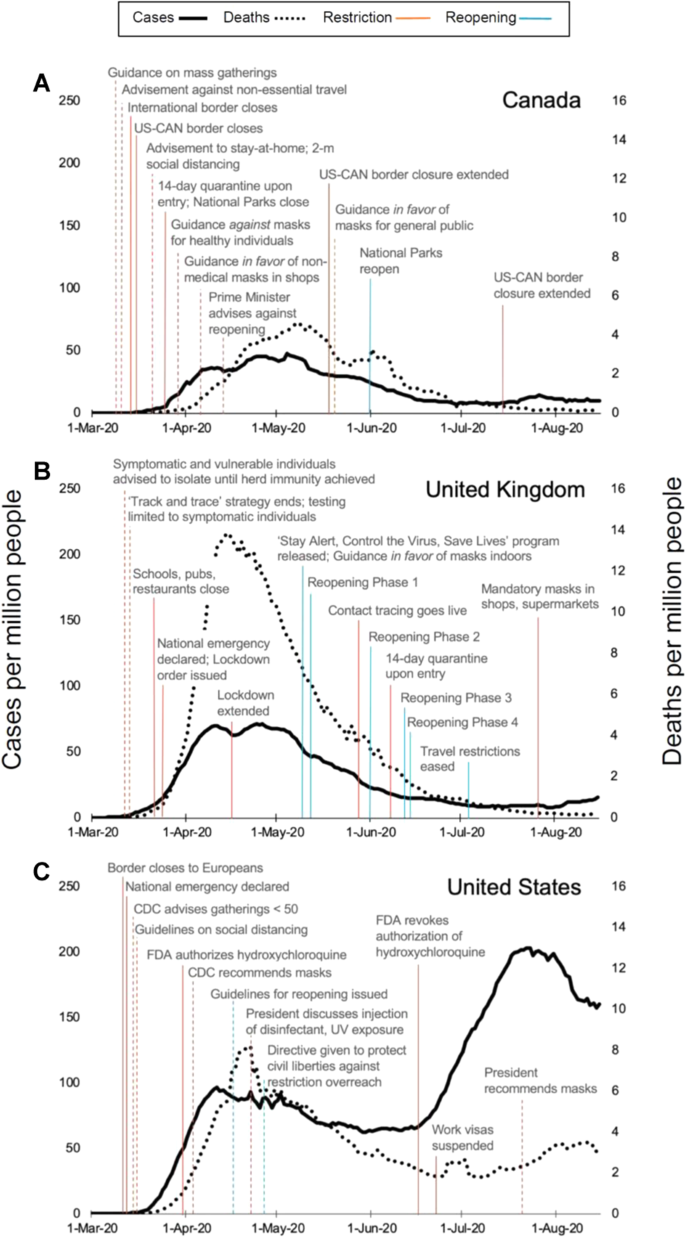
COVID-19 cases, deaths, and national-level policies are indicated for ( A ) Canada, ( B ) the United Kingdom, and ( C ) the United States. 7-day rolling averages of cases (left vertical axis, solid black line) and deaths (right vertical axis, dotted black line) per one million people are shown for the timeframe of this media study, 1 March through 15 August 2020 (Roser et al., 2020 ). The timeline for each country specifies national-level public-health policies and guidance, especially emergency declarations, school and non-essential business closures, travel and border restrictions, quarantines and social distancing, mask usage, and reopening phases. Implementation of enforceable policies (solid) and non-enforced guidance (dotted) is specified with vertical red lines, and corresponding reopening and relaxation of policies and guidance are specified with vertical blue lines. Detailed descriptions of national-level policies within each panel are provided in SI Table S1 .
The amount of pandemic media coverage
The studied news outlets differed in the amount of news media coverage related to COVID-19 from 1 March through 15 August 2020 (Fig. 2 ). The amount of coverage increased notably in March as case rates climbed in each country, subsequently decreasing gradually in May and June while case rates also declined. Across the 24 randomly sampled days, the 12 studied news outlets published 18,430 articles related to COVID-19. Of these, an estimated 4321 articles (23.4%) were eligible for inclusion in this study—that is, as news reporting or analysis relevant to the country of publication and containing a direct focus on COVID-19 public health or policy information (SI Figs. S4 - S5 ). Articles with a direct focus on COVID-19 public health or policy information (to a small or large extent) could be coded for the scientific quality of the reporting of this information and its sensationalism.
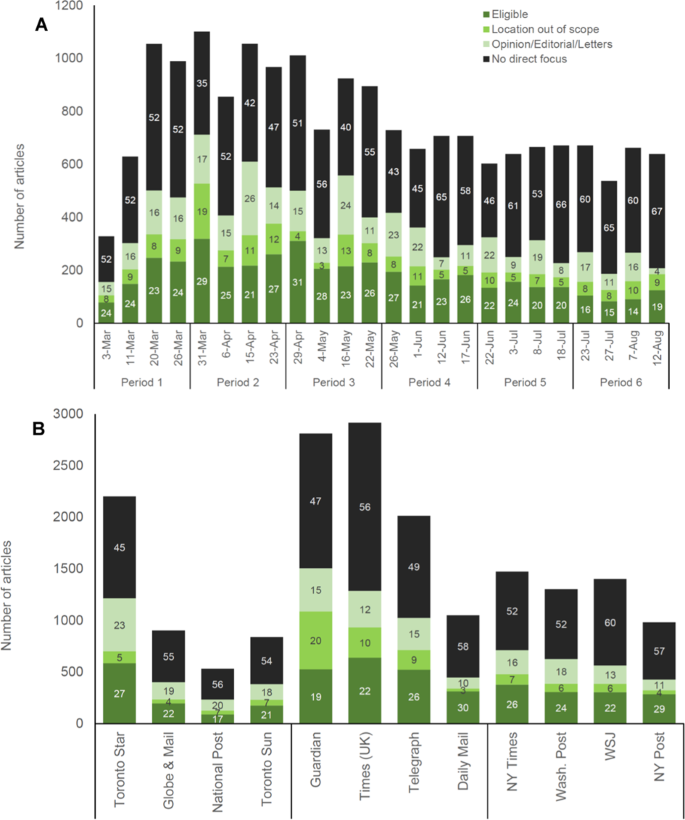
For each randomly sampled day ( A ) and each news outlet ( B ), the total number of individual news records is shown, based on Factiva database searches for articles related to COVID-19 public health and policy information (Methods). News articles are partitioned across the following categories: articles eligible for inclusion in our study (eligible), articles not focusing on the newspaper’s country of publication (location out of scope), articles that are not original news reporting or analysis (opinion/editorial/letters), and articles that include COVID-19-relevant search terms, but do not include any direct focus on COVID-19 public health or policy information (no direct focus). Estimated totals for these categories are calculated using (i) the total number of Factiva returns and (ii) the rates at which articles were assigned to these categories during the eligibility screening process for each outlet and randomly sampled day (SI Fig. S4 ). On the stacked bars, percentages of articles falling into each category are specified for each day ( A ) and news outlet ( B ).
Content analysis of pandemic media coverage
We collected a manageable, well-defined random sample of 1331 news media articles satisfying our eligibility criteria (SI Fig. S4 ) for coding of scientific quality and sensationalism (SI Coding Tool and Dataset S1 ). Six questions each for scientific quality and for sensationalism were evaluated on a scale from 1 to 5 (5 corresponding to highest scientific quality or sensationalism, 1 corresponding to lowest scientific quality or sensationalism). Question categories included for scientific quality: applicability, opinion versus facts, validity, precision, context, and global assessment (i.e., an overall assessment of the article’s scientific quality); and for sensationalism: exposing, speculating, generalizing, warning, extolling, and global assessment (i.e., an overall assessment of the degree of sensationalism in the article) (SI Coding Tool). For this content analysis, interrater reliability was moderate to substantial for the summative “global” assessment of scientific quality and sensationalism (SI Table S2 ). Reliability was similarly high for specific scientific quality and sensationalism measures, with the exception of questions for which coded scores displayed restriction of range or unbalanced distributions (e.g., “generalizing” scores of mostly 1 and 2, rather than ranging from 1 through 5 with balance around 3; SI Coding Tool and Dataset S1 ) (Hallgren, 2012 ; Tran et al., 2020 ).
The scientific quality of pandemic media coverage
The scientific quality of news media articles differed among news outlets across the political spectrums of the respective countries (Fig. 3 ). Within each country, the overall scientific quality of news reporting and analysis was lowest on the populist-right of the political spectrum (mean summative “global” scientific quality of 2.58, n = 106 articles, for Toronto Sun ; 2.67, n = 115, for Daily Mail ; and 2.28, n = 118, for New York Post ; p ≤ 0.001 for Kruskal–Wallis, p ≤ 0.05 for within-country pairwise comparisons except Daily Mail versus Times of London and Telegraph , SI Table S3 ). For these outlets, lower scientific quality was especially evident for validity, precision, and context as measures of scientific quality (e.g., articles reporting claims without fact checking, specificity, or background details) (Fig. 3 ).
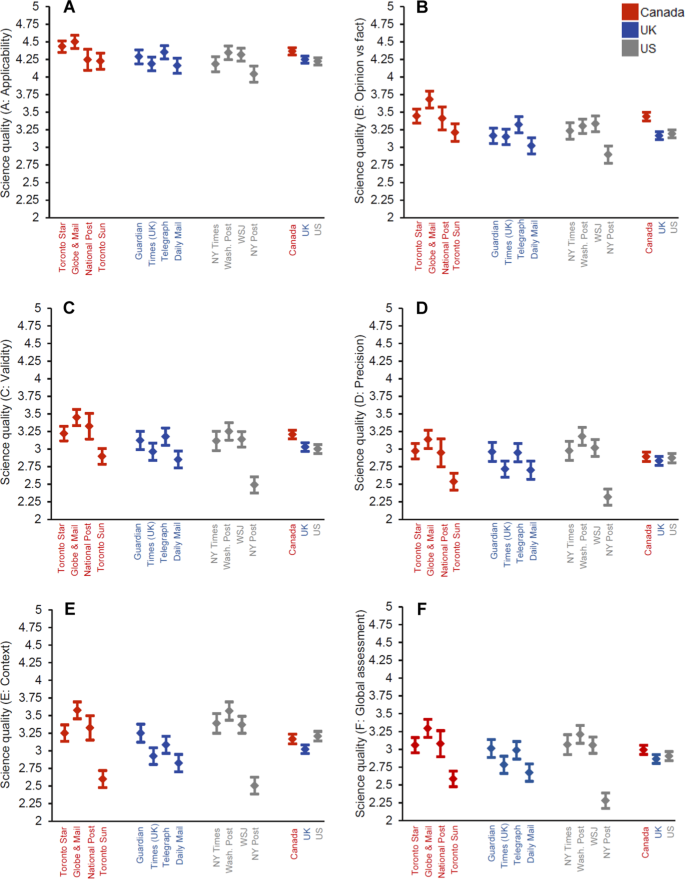
Scores for six scientific quality questions (SI Coding Tool) are shown (mean, 95% confidence interval) for articles ( n = 1331) communicating COVID-19 public health or policy information (Fig. 2 ): ( A ) applicability, ( B ) opinion versus facts, ( C ) validity, ( D ) precision, ( E ) context, and ( F ) global assessment (i.e., an overall assessment of the article’s scientific quality). Each question was evaluated on a scale from 1 to 5 (5 corresponding to highest scientific quality). Sampled articles were published between 1 March and 15 August 2020 (Methods). Kruskal–Wallis one-way analysis of variance and post-hoc multi-comparison test statistics are in SI Table S3 .
The sensationalism of pandemic media coverage
The sensationalism of news media articles was low overall for all news outlets, although somewhat greater for outlets on the left and middle of the political spectrum in Canada and the United States (Fig. 4F ). In both countries, news outlets at the populist-right combined low scientific quality with low sensationalism (Figs. 3 F and 4F ). In Canada, the overall sensationalism of news reporting and analysis was lowest for the Toronto Sun (mean summative “global” sensationalism of 1.77, n = 106 articles; p ≤ 0.001 for Kruskal–Wallis, p ≤ 0.05 for pairwise comparisons with Globe and Mail and National Post , SI Table S3 ). In the United States, overall sensationalism was lower in the Wall Street Journal (mean global sensationalism of 2.03, n = 118 articles) and New York Post (mean of 2.13, n = 118), as compared to the New York Times (mean of 2.40, n = 120) and Washington Post (mean of 2.38, n = 119; p ≤ 0.001 for Kruskal–Wallis, p ≤ 0.05 for pairwise comparisons, SI Table S3 ). For these outlets, lower sensationalism was especially observed for exposing, speculating, and warning as measures of sensationalism (Fig. 4 ). In the United Kingdom, overall sensationalism did not vary across news outlets ( p = 0.283 for Kruskal–Wallis, SI Table S3 ).
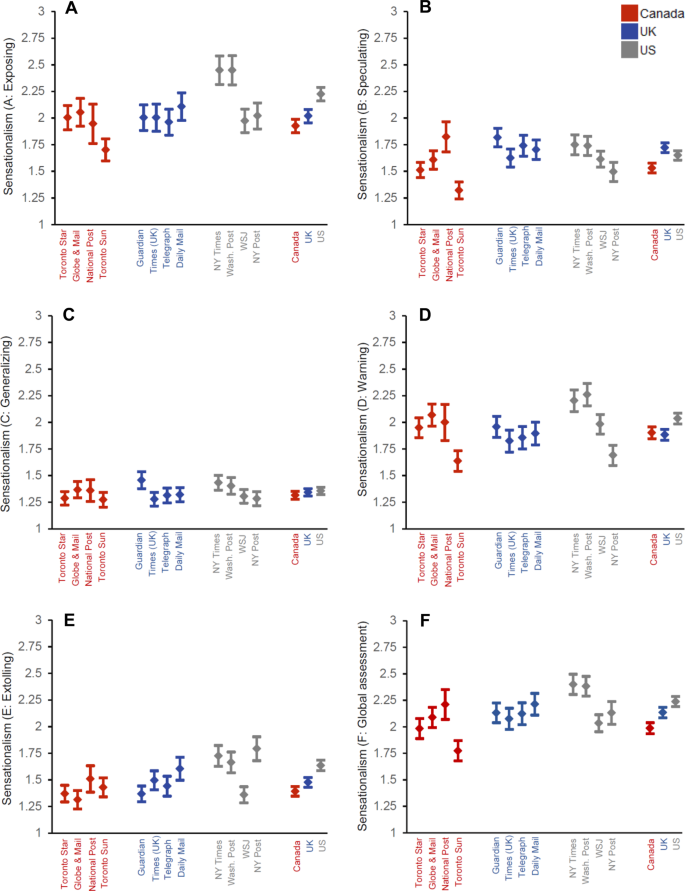
Scores for six sensationalism questions (SI Coding Tool) are shown (mean, 95% confidence interval) for articles ( n = 1331) communicating COVID-19 public health or policy information (Fig. 2 ): ( A ) exposing, ( B ) speculating, ( C ) generalizing, ( D ) warning, ( E ) extolling, and ( F ) global assessment (i.e., an overall assessment of the degree of sensationalism in the article). Each question was evaluated on a scale from 1 to 5 (5 corresponding to highest sensationalism). Sampled articles were published between 1 March and 15 August 2020 (Methods). Kruskal–Wallis one-way analysis of variance and post-hoc multi-comparison test statistics are in SI Table S3 .
Syndicated versus original reporting
Across all outlets, the scientific quality of original reporting (mean global scientific quality of 2.93, n = 1278 articles) was significantly higher than the scientific quality of syndicated articles (mean of 2.71, n = 54; p = 0.020, Kruskal–Wallis; SI Fig. S6 and Table S4 ). Additionally, the sensationalism of syndicated articles (mean global sensationalism of 1.82, n = 54 articles) was significantly lower than the sensationalism of original reporting (mean of 2.14, n = 1278; p ≤ 0.001, Kruskal–Wallis; SI Fig. S6 and Table S4 ). The Toronto Sun published the highest proportion of syndicated news articles by far, with 34% of the paper’s 106 coded articles originating from syndicated sources. Other news outlets with more than 1% of coded articles drawing from syndicated sources included the Toronto Star (6% of articles) and the National Post (11%).
Neither scientific quality nor sensationalism varied substantially through time, with the exception of lower scientific quality on 3 July 2020 resulting from limited coverage of the healthcare sector that day (Fig. 5 , SI Fig. S7 ).
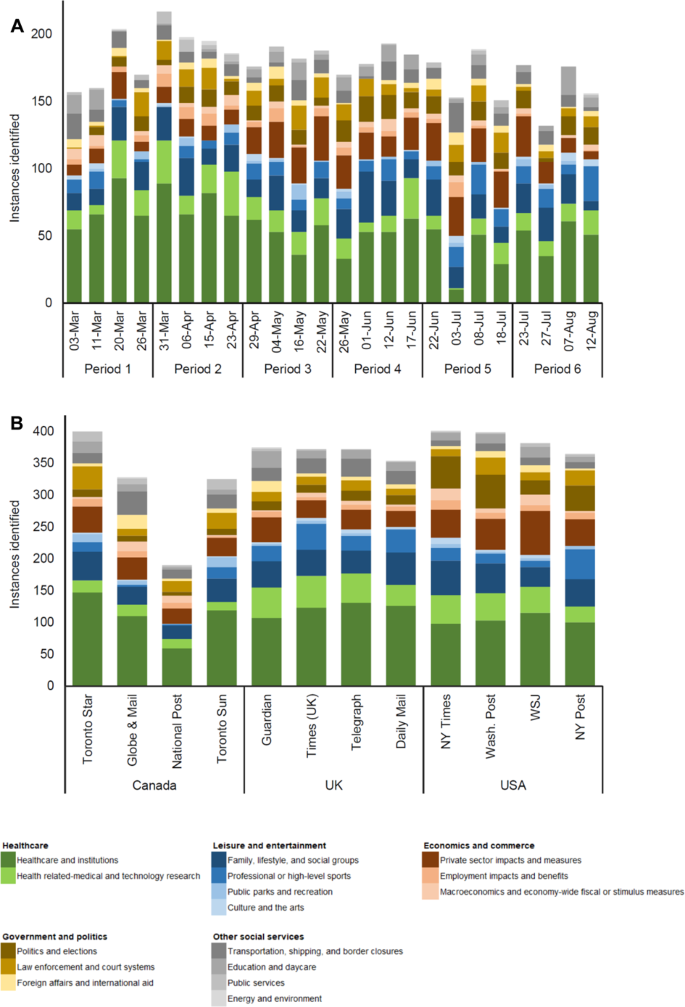
The topics of news media articles analyzed ( A ) over the timeframe of this study and ( B ) by news outlet are specified. Sampled articles were published on randomly sampled days between 1 March and 15 August 2020 (Methods). The topic of each article ( n = 1331) was categorized by societal sectors (up to 2 selected per article) related to healthcare, leisure and entertainment, economics and commerce, government and politics, and other social services.
The topics of pandemic media coverage
News media articles were categorized based on the societal sectors (up to 2 per article) that were the primary focus of each article (Fig. 5 ). The sectors, related to healthcare, leisure and entertainment, economics and commerce, government and politics, and other social services, are listed in full in Figs. 5 and 6 . Although all analyzed articles contained information on the public-health effects of COVID-19 or measures to limit its spread (SI Fig. S4 ), topics of focus differed widely, for example including recreation, the arts, transportation, or daycare, not just medical facilities or vaccine research.
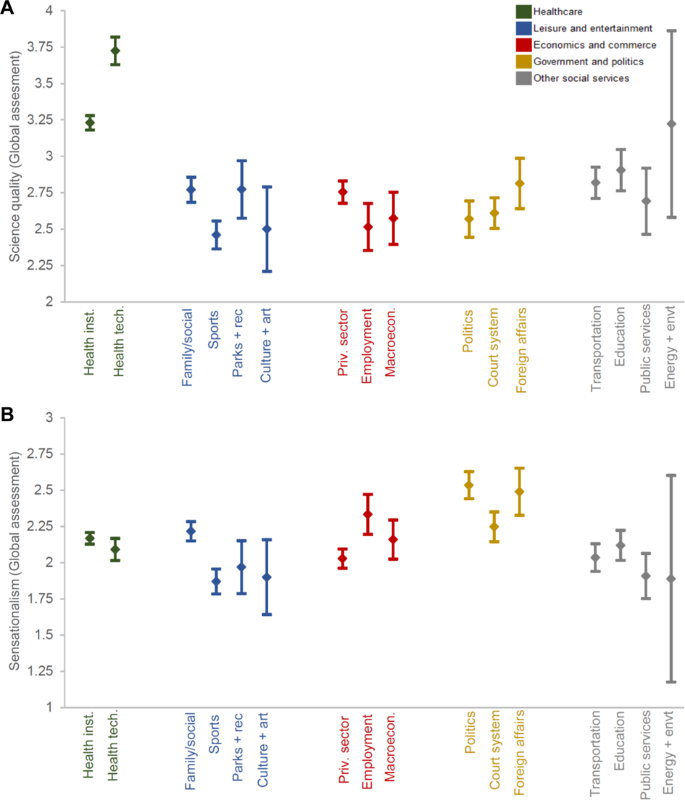
Scientific quality ( A ) and sensationalism ( B ) of news media articles are indicated by the topics of articles. Overall global assessment scores for scientific quality and sensationalism (SI Coding Tool) are shown (mean, 95% confidence interval) for articles communicating COVID-19 public health or policy information (Fig. 2 ). For each article, scientific quality and sensationalism were each evaluated on a scale from 1 to 5 (5 corresponding to highest scientific quality or to highest sensationalism). Sampled articles ( n = 1331) were published between 1 March and 15 August 2020 (Methods). The topic of each article was categorized across the following societal sectors (up to two selected per article): healthcare and institutions; health-related medical and technology research; family, lifestyle, and social groups; professional or high-level sports; public parks and recreation; culture and the arts; private sector impacts and measures; employment impacts and benefits; macroeconomics and economy-wide fiscal or stimulus measures; politics and elections; law enforcement and court systems; foreign affairs and international aid; transportation, shipping, and border closures; education and daycare; public services; and energy and the environment.
The topics of news media articles corresponded to scientific quality and sensationalism of news reporting and analysis to some degree (Fig. 6 ). News media articles related to healthcare, health institutions, and health-related research were most common (Fig. 5 ), and they had significantly greater scientific quality compared to articles on other topics (mean global scientific quality of 3.23 for healthcare and institutions and 3.72 for health-related research; p ≤ 0.001 for Kruskal–Wallis, p ≤ 0.05 for pairwise comparisons except with energy and the environment; Fig. 6A ). News media articles during the first four-week period studied, starting 1 March 2020, included the greatest focus (50.2% of coverage) on healthcare and related institutions and research (Fig. 5A ).
Sensationalism of articles related to politics and foreign affairs was greatest (mean global sensationalism of 2.53 for politics; and of 2.49 for foreign affairs; p < 0.001 for Kruskal–Wallis, p < 0.05 for pairwise comparisons of politics versus all sectors except foreign affairs, employment, and energy and the environment; Fig. 6B ). For example, sensational statements related to politics and foreign affairs could include exposing disinformation from political leaders or extolling political leaders for border closures as a pandemic or broader policy response. News outlets in the United States published the most articles related to politics and elections (63.8% of coverage across all outlets; Fig. 5B ).
Public-health policies consistently covered through time included measures related to social distancing, testing and tracing, and protective equipment and disinfection practices, while coverage of mask guidance and reopening policies increased over the course of the study (Fig. 7 ).
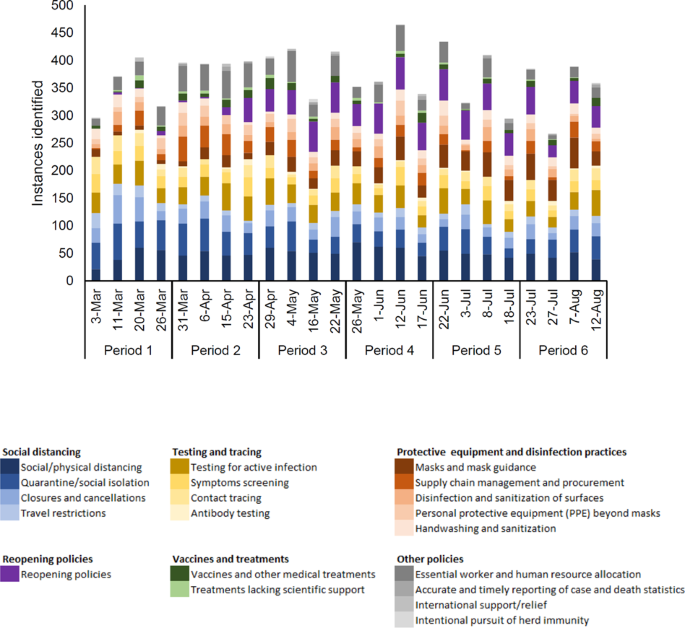
Sampled articles ( n = 1331) were published on randomly sampled days between 1 March and 15 August 2020 (Methods). Public-health policies and measures in each article were coded under specific categories related to social distancing, testing and tracing, protective equipment and disinfection practices, reopening policies, vaccines and treatments, and more (all relevant categories selected for each article).
Managing the public health and societal risks of a pandemic requires iterative, informed decision-making by governments, individuals, and the private sector. News media play a central role in communicating public health and policy information, establishing accountability for decision-making, and shaping public perceptions through the number of news reports, their content, and their tone (Klemm et al., 2016 ; Reintjes et al., 2016 ). For news outlets spanning the political spectrum of three countries with contrasting public-health outcomes and policy responses (Fig. 1 ), based on a random sample of days, coverage related to COVID-19 increased substantially in March 2020 and declined gradually thereafter in May and June (Fig. 2 ), not rebounding even during the dramatic increase in U.S. COVID-19 cases in June and July (SI Figure S5 ). Understanding this news media reporting in the early stages of COVID-19 response provides important lessons for ensuring the accessibility of information in support of public health and gauging its degree of effectiveness in creating accountability for policy decisions.
News media reporting grappled with complications of scientific understanding and its uncertainties during the timeframe of our study, as assessed through our measures of validity, precision, and overall scientific quality. For example, the mechanisms of disease transmission, especially airborne transmission, were slow to be recognized, leading to dynamic adjustments of public-health guidance (e.g., for mask usage by the general public) (Zhang et al., 2020 ). Despite such uncertainties and frequent knowledge updates over time, the scientific quality of reporting was highest for the healthcare sector, also the most commonly occurring article topic (Fig. 6 ). The scientific quality of reporting overall did not improve as the pandemic proceeded and knowledge of COVID-19 increased, which may be attributed to shifts from healthcare to other topics of news media reporting (Fig. 5 and SI Fig. S7 ).
We did, however, identify major differences in the degree to which newspaper reporting of COVID-19 presented high-quality scientific information about the public-health effects of the coronavirus disease and measures to limit its spread. News media articles generally had moderate scientific quality overall (Fig. 3F ). Outlets on the populist-right of the political spectrum of each country, though, had significantly lower scientific quality in reporting related to COVID-19 (Fig. 3F ). Scientific quality was low especially for validity, precision, and context as measures of scientific quality, as well as for the distinction between opinion versus facts in some cases (e.g., articles reporting claims without fact checking, specificity, background details, or sourcing) (Fig. 3 ). These findings pertain to news reporting and analysis, rather than opinion pieces, editorials, or letters, which were excluded from the scope of news media articles we evaluated. The differences across outlets suggest that, in reading news reporting and analysis in different newspapers, readers access reporting of varying scientific quality related to the health risks and effectiveness of available measures to limit disease transmission.
Further, patterns of U.S. media reporting were correlated with failures of national leadership under the Trump Administration, and they may have both reflected and contributed to politicization of COVID-19 in the United States. During this study’s timeframe, the United States led the world in cases and deaths despite its pre-pandemic ranking as the country best equipped to manage a pandemic such as COVID-19 (Cameron et al., 2019 ). These public-health outcomes occurred against a backdrop of disinformation and failures of national leadership (Evanega et al., 2020 ; Ball and Maxmen, 2020 ; Holtz et al., 2020 ; Lincoln, 2020 ; Thorp, 2020 ). Lack of national leadership was observed in the relative dearth of national-level public-health policies and guidance (Fig. 1 ) and the divergence of subnational policy responses, correlated with partisan politics (SI Fig. S1 and Table S1 ). Elites and incumbent governments have outsize influence on public opinion and media coverage, which likely contributed to polarization and politicization of pandemic media coverage (Green et al., 2020 ; Hart et al., 2020 ). Linked to these trends, we observed higher sensationalism related to politics and elections topics and greater coverage of these sectors among U.S. newspapers (Figs. 5 – 6 ). Additionally, news outlets on the political left in the United States (i.e., New York Times , Washington Post ) published articles with more exposing and warning coverage, for example discussing disinformation on the part of government leaders and the risks of disease (Fig. 4 ). Although most Americans believe the media are fulfilling key roles during the pandemic, the majority of these individuals identify as Democrats, and Democrats trust many more new sources than individuals identifying as Republican (Jurkowitz et al., 2020 ; Gottfried et al., 2020 ).
In both Canada and the United States, low scientific quality was paired with lower-than-average sensationalism in news outlets on the populist-right (Figs. 3 F and 4F ). Sensationalism was low overall for all news outlets, but within Canada and the United States, it was lowest for the Toronto Sun and New York Post , as well as the Wall Street Journal . Although low sensationalism is generally considered beneficial, very low sensationalism combined with low scientific quality may have failed to alert readers to public-health risks and policy failures in some cases (e.g., per the measures of exposing and warning coverage in Fig. 4 ). Such trends also resulted, in part, from higher reliance on syndicated articles, especially in Canada, potentially related to structural and economic changes in news media (SI Fig. S6 ). Across the political spectrum, our results demonstrate that existing ideological perspectives may influence how information is used in reporting (Rosella et al., 2013 ). For example, news outlets at the populist-right in the United Kingdom and the United States may tend towards support of populist-right governments, demonstrating preference for those governments’ interpretation of the science, implemented policies, and use of science to justify choices made (Bennett et al., 2008 ; Grundmann and Stehr, 2012 ).
The studied news media outlets—traditional, national-level print media—have disproportionate influence on the content of other media platforms and on how that content is covered (Project for Excellence in Journalism, 2010 ; Denham, 2014 ). A better understanding of the effects of news media—or lack thereof—on public-health decision-making and public sentiment in the early stages of this pandemic can, for future pandemics or other public-health crises, increase public-health officials’ capacity to adapt communication strategies in disseminating guidance and coordinating responses of health system stakeholders (Laing, 2011 ; Rosella et al., 2013 ; Klemm et al., 2016 ; Hoffman and Justicz, 2016 ; Pieri, 2019 ). Such understanding is crucial as the impacts of the policy actions themselves accumulate. The findings of this study point to complex interactions among scientific evidence on public-health risks and response measures, societal politicization of the science, and the scientific quality and sensationalism of media reporting. An inherent tension may exist: tendencies towards low sensationalism, especially combined with low scientific quality, may in some cases lead to characterization of public-health threats and policy failures as less extraordinary and relevant than they actually are.
Data availability
All data generated or analyzed during this study are included in this published article and its Supplementary Information .
Adeel AB, Catalano M, Catalano O, et al (2020) COVID-19 policy response and the rise of the sub-national governments. Can Public Policy. https://doi.org/10.3138/cpp.2020-101
Anderson B, Coletto D (2017) Canadian news media and “fake news” under a microscope. In: Abacus Data. https://abacusdata.ca/canadian-news-media-and-fake-news-under-a-microscope/ . Accessed 25 Aug 2020
Antoine J-Y, Villaneau J, Lefeuvre A (2014) Weighted Krippendorff’s alpha is a more reliable metrics for multi- coders ordinal annotations: experimental studies on emotion, opinion and coreference annotation. In: Proceedings of the 14th conference of the European chapter of the Association for Computational Linguistics, Association for Computational Linguistics, Gotenborg, Sweden, pp. 550–559
Austen I (2020) Canada’s largest newspaper changes hands amid vow to keep liberal voice. N. Y. Times
Ball P, Maxmen A (2020) The epic battle against coronavirus misinformation and conspiracy theories. Nature 581:371–374. https://doi.org/10.1038/d41586-020-01452-z
Article ADS CAS PubMed Google Scholar
Bennett WL, Lawrence RG, Livingston S (2008) When the press fails: political power and the news media from Iraq to Katrina. University of Chicago Press
Cameron EE, Nuzzo JB, Bell JA (2019) GHS index: global health security index: building collective action and accountability. NTI and Johns Hopkins Bloomberg School of Public Health
CBC News (2020) Tracking the spread of coronavirus in canada and around the world. https://newsinteractives.cbc.ca/coronavirustracker/ . Accessed 25 Aug 2020
Centers for Disease Control and Prevention (CDC) (2020) CDC COVID Data Tracker. https://covid.cdc.gov/covid-data-tracker . Accessed 30 Aug 2020
Cohen J (1960) A coefficient of agreement for nominal scales. Educ Psychol Meas 20:37–46. https://doi.org/10.1177/001316446002000104
Article Google Scholar
Denham BE (2014) Intermedia attribute agenda setting in the New York Times: the case of animal abuse in U.S. horse racing. Journal Mass Commun Q 91:17–37. https://doi.org/10.1177/1077699013514415
Evanega S, Lynas M, Adams J, Smolenyak K (2020) Coronavirus misinformation: quantifying sources and themes in the COVID-19 ‘infodemic.’ Cornell Alliance for Science
Fleiss JL, Cohen J (1973) The equivalence of weighted kappa and the intraclass correlation coefficient as measures of reliability. Educ Psychol Meas 33:613–619. https://doi.org/10.1177/001316447303300309
Gentzkow M, Shapiro JM (2010) What drives media slant? Evidence from U.S. daily newspapers. Econometrica 78:35–71. https://doi.org/10.3982/ECTA7195
Article MathSciNet Google Scholar
Giraudoux P, Antonietti J-P, Beale C, et al (2018) pgirmess: spatial analysis and data mining for field ecologists. R package version 1.6.9 https://CRAN.R-project.org/package=pgirmess
Gottfried J, Walker M, Mitchell A (2020) Americans’ views of the news media during the Coronavirus outbreak. Pew Research Center
Government of Canada (2016) Canada’s health care system. https://www.canada.ca/en/health-canada/services/canada-health-care-system.html . Accessed 18 Oct 2020
Green J, Edgerton J, Naftel D et al. (2020) Elusive consensus: polarization in elite communication on the COVID-19 pandemic. Sci Adv 6:eabc2717. https://doi.org/10.1126/sciadv.abc2717
Article ADS PubMed PubMed Central Google Scholar
Grossman G, Kim S, Rexer JM, Thirumurthy H (2020) Political partisanship influences behavioral responses to governors’ recommendations for COVID-19 prevention in the United States. Proc Natl Acad Sci USA 117:24144–24153. https://doi.org/10.1073/pnas.2007835117
Article CAS PubMed PubMed Central Google Scholar
Grundmann R, Stehr N (2012) The power of scientific knowledge: from research to public policy. Cambridge University Press, Cambridge
Book Google Scholar
Hallgren KA (2012) Computing inter-rater reliability for observational data: an overview and tutorial. Tutor Quant Methods Psychol 8:23–34. https://doi.org/10.20982/tqmp.08.1.p023
Article PubMed PubMed Central Google Scholar
Hart PS, Chinn S, Soroka S (2020) Politicization and polarization in COVID-19 news coverage. Sci Commun 42:679–697. https://doi.org/10.1177/1075547020950735
Article PubMed Central Google Scholar
Hoffman SJ, Justicz V (2016) Automatically quantifying the scientific quality and sensationalism of news records mentioning pandemics: validating a maximum entropy machine-learning model. J Clin Epidemiol 75:47–55. https://doi.org/10.1016/j.jclinepi.2015.12.010
Holtz D, Zhao M, Benzell SG et al. (2020) Interdependence and the cost of uncoordinated responses to COVID-19. Proc Natl Acad Sci USA 117:19837–19843. https://doi.org/10.1073/pnas.2009522117
Hönnige C, Nyhuis D, Meyer P et al. (2020) Dominating the debate: visibility bias and mentions of British MPs in newspaper reporting on Brexit. Polit Res Exch 2:1788955. https://doi.org/10.1080/2474736X.2020.1788955
Jurkowitz M, Mitchell A, Shearer E, Walker M (2020) U.S. media polarization and the 2020 election: a nation divided. Pew Research Center
Klemm C, Das E, Hartmann T (2016) Swine flu and hype: a systematic review of media dramatization of the H1N1 influenza pandemic. J Risk Res 19:1–20. https://doi.org/10.1080/13669877.2014.923029
Lacy S, Riffe D, Stoddard S et al. (2001) Sample size for newspaper content analysis in multi-year studies. J Mass Commun Q 78:836–45
Google Scholar
Laing A (2011) The H1N1 crisis: roles played by government communicators, the public and the media. J Prof Commun 1:123–149. https://doi.org/10.15173/jpc.v1i1.88
Article ADS Google Scholar
Lincoln M (2020) Study the role of hubris in nations’ COVID-19 response. Nature 585:325–325. https://doi.org/10.1038/d41586-020-02596-8
Mitchell A, Simmons K, Matsa KE, et al (2018) In Western Europe, public attitudes toward news media more divided by populist views than left-right ideology. Pew Research Center
Molek-Kozakowska K (2013) Towards a pragma-linguistic framework for the study of sensationalism in news headlines. Discourse Commun 7:173–197. https://doi.org/10.1177/1750481312471668
Oxman AD, Guyatt GH, Cook DJ et al. (1993) An index of scientific quality for health reports in the lay press. J Clin Epidemiol 46:987–1001. https://doi.org/10.1016/0895-4356(93)90166-x
Article CAS PubMed Google Scholar
Pieri E (2019) Media framing and the threat of global pandemics: the Ebola crisis in UK media and policy response. Sociol Res Online 24:73–92
Project for Excellence in Journalism (2010) How news happens: a study of the news ecosystem of one American city. Pew Research Center
Public Health England, NHSX (2020) Coronavirus (COVID-19) in the UK. https://coronavirus.data.gov.uk . Accessed 2 Oct 2020
Puglisi R, Snyder JM (2015) The balanced US press. J Eur Econ Assoc 13:240–264. https://doi.org/10.1111/jeea.12101
R Core Team (2020) R: a language and environment for statistical computing. R Foundation for Statistical Computing, Vienna, Austria
Reintjes R, Das E, Klemm C et al. (2016) “Pandemic public health paradox”: time series analysis of the 2009/10 influenza A / H1N1 epidemiology, media attention, risk perception and public reactions in 5 European countries. PLoS ONE 11:e0151258. https://doi.org/10.1371/journal.pone.0151258
Riffe D, Aust CF, Lacy SR (2016) The effectiveness of random, consecutive day and constructed week sampling in newspaper content analysis. Journal Q 70:133–139. https://doi.org/10.1177/107769909307000115
Rosella LC, Wilson K, Crowcroft NS et al. (2013) Pandemic H1N1 in Canada and the use of evidence in developing public health policies–A policy analysis. Soc Sci Med 83:1–9. https://doi.org/10.1016/j.socscimed.2013.02.009
Roser M, Ritchie H, Ortiz-Ospina E, Hasell J (2020) Coronavirus pandemic (COVID-19). Our World in Data. https://ourworldindata.org/coronavirus . Accessed 30 Aug 2020
Thorp HH (2020) Trump lied about science. Science 369:1409. https://doi.org/10.1126/science.abe7391
Tran D, Dolgun A, Demirhan H (2020) Weighted inter-rater agreement measures for ordinal outcomes. Commun Stat-Simul Comput 49:989–1003. https://doi.org/10.1080/03610918.2018.1490428
World Health Organization (WHO) (2020a) Coronavirus disease (COVID-19) pandemic. https://www.who.int/emergencies/diseases/novel-coronavirus-2019 . Accessed 7 Jun 2020
World Health Organization (WHO) (2020b) Timeline of WHO’s response to COVID-19. https://www.who.int/news/item/29-06-2020-covidtimeline . Accessed 5 Jun 2020
Zhang R, Li Y, Zhang AL et al. (2020) Identifying airborne transmission as the dominant route for the spread of COVID-19. Proc Natl Acad Sci USA 117:14857–14863. https://doi.org/10.1073/pnas.2009637117
Download references
Acknowledgements
S. Damouras provided advising on methods of statistical analysis, and J. Niemann formatted references. Funding for this work was provided by the University of Toronto Scarborough Department of Physical and Environmental Sciences and the University of Miami Rosenstiel School of Marine and Atmospheric Science.
Author information
Authors and affiliations.
Department of Environmental Science and Policy, Rosenstiel School of Marine and Atmospheric Science, University of Miami, Miami, FL, USA
Katharine J. Mach & Rosalind Donald
Leonard and Jayne Abess Center for Ecosystem Science and Policy, University of Miami, Coral Gables, FL, USA
Katharine J. Mach
Department of Physical and Environmental Sciences, University of Toronto, Toronto, ON, Canada
Raúl Salas Reyes, Brian Pentz, Clarissa A. Costa, Sandip G. Cruz, Kerronia E. Thomas & Nicole Klenk
Department of Geography and Planning, University of Toronto, Toronto, ON, Canada
Jennifer Taylor
Aspen Global Change Institute, Basalt, CO, USA
James C. Arnott
Earth and Environmental Sciences Area, Lawrence Berkeley National Laboratory, Berkeley, CA, USA
Kripa Jagannathan
School for Environment and Sustainability, University of Michigan, Ann Arbor, MI, USA
Department of Civil and Environmental Engineering, University of Connecticut, Storrs, CT, USA
Christine J. Kirchhoff
Dalla Lana School of Public Health, University of Toronto, Toronto, ON, Canada
Laura C. Rosella
You can also search for this author in PubMed Google Scholar
Contributions
All authors conceived the analysis. KJM, RSR, BP, JT, CAC, SGC, KET, and NK designed the methods of analysis with review by all authors. RSR, BP, JT, CAC, SGC, and KET collected data. KJM, RSR, BP, and JT performed analysis of data and developed visualizations of data. KJM, RSR, BP, JT, and NK drafted the manuscript with review and edits from all authors.
Corresponding author
Correspondence to Katharine J. Mach .
Ethics declarations
Competing interests.
The authors declare no competing interests.
Additional information
Publisher’s note Springer Nature remains neutral with regard to jurisdictional claims in published maps and institutional affiliations.
Supplementary information
Supplementary information, si appendix dataset s1, rights and permissions.
Open Access This article is licensed under a Creative Commons Attribution 4.0 International License, which permits use, sharing, adaptation, distribution and reproduction in any medium or format, as long as you give appropriate credit to the original author(s) and the source, provide a link to the Creative Commons license, and indicate if changes were made. The images or other third party material in this article are included in the article’s Creative Commons license, unless indicated otherwise in a credit line to the material. If material is not included in the article’s Creative Commons license and your intended use is not permitted by statutory regulation or exceeds the permitted use, you will need to obtain permission directly from the copyright holder. To view a copy of this license, visit http://creativecommons.org/licenses/by/4.0/ .
Reprints and permissions
About this article
Cite this article.
Mach, K.J., Salas Reyes, R., Pentz, B. et al. News media coverage of COVID-19 public health and policy information. Humanit Soc Sci Commun 8 , 220 (2021). https://doi.org/10.1057/s41599-021-00900-z
Download citation
Received : 06 January 2021
Accepted : 15 September 2021
Published : 28 September 2021
DOI : https://doi.org/10.1057/s41599-021-00900-z
Share this article
Anyone you share the following link with will be able to read this content:
Sorry, a shareable link is not currently available for this article.
Provided by the Springer Nature SharedIt content-sharing initiative
This article is cited by
Covid-19 pandemic and vaccination skepticism.
- Abdul Latif Anas
- Mashudu Salifu
- Hanan Lassen Zakaria
Human Arenas (2023)
Local TV News Coverage of Racial Disparities in COVID-19 During the First Wave of the Pandemic, March–June 2020
- Elizabeth K. Farkouh
- Jeff Niederdeppe
Race and Social Problems (2023)
Quick links
- Explore articles by subject
- Guide to authors
- Editorial policies


An official website of the United States government
The .gov means it’s official. Federal government websites often end in .gov or .mil. Before sharing sensitive information, make sure you’re on a federal government site.
The site is secure. The https:// ensures that you are connecting to the official website and that any information you provide is encrypted and transmitted securely.
- Publications
- Account settings
Preview improvements coming to the PMC website in October 2024. Learn More or Try it out now .
- Advanced Search
- Journal List
- Elsevier - PMC COVID-19 Collection

Coronavirus disease 2019 (COVID-19): A literature review
Harapan harapan.
a Medical Research Unit, School of Medicine, Universitas Syiah Kuala, Banda Aceh, Indonesia
b Tropical Disease Centre, School of Medicine, Universitas Syiah Kuala, Banda Aceh, Indonesia
c Department of Microbiology, School of Medicine, Universitas Syiah Kuala, Banda Aceh, Indonesia
d Division of Infectious Diseases, AichiCancer Center Hospital, Chikusa-ku Nagoya, Japan
Amanda Yufika
e Department of Family Medicine, School of Medicine, Universitas Syiah Kuala, Banda Aceh, Indonesia
Wira Winardi
f Department of Pulmonology and Respiratory Medicine, School of Medicine, Universitas Syiah Kuala, Banda Aceh, Indonesia
g School of Medicine, The University of Western Australia, Perth, Australia
Haypheng Te
h Siem Reap Provincial Health Department, Ministry of Health, Siem Reap, Cambodia
Dewi Megawati
i Department of Microbiology and Parasitology, Faculty of Medicine and Health Sciences, Warmadewa University, Denpasar, Indonesia
j Department of Medical Microbiology and Immunology, University of California, Davis, CA, USA
Zinatul Hayati
k Department of Clinical Microbiology, School of Medicine, Universitas Syiah Kuala, Banda Aceh, Indonesia
Abram L. Wagner
l Department of Epidemiology, University of Michigan, Ann Arbor, Michigan, MI 48109, USA
Mudatsir Mudatsir
In early December 2019, an outbreak of coronavirus disease 2019 (COVID-19), caused by a novel severe acute respiratory syndrome coronavirus 2 (SARS-CoV-2), occurred in Wuhan City, Hubei Province, China. On January 30, 2020 the World Health Organization declared the outbreak as a Public Health Emergency of International Concern. As of February 14, 2020, 49,053 laboratory-confirmed and 1,381 deaths have been reported globally. Perceived risk of acquiring disease has led many governments to institute a variety of control measures. We conducted a literature review of publicly available information to summarize knowledge about the pathogen and the current epidemic. In this literature review, the causative agent, pathogenesis and immune responses, epidemiology, diagnosis, treatment and management of the disease, control and preventions strategies are all reviewed.
On December 31, 2019, the China Health Authority alerted the World Health Organization (WHO) to several cases of pneumonia of unknown aetiology in Wuhan City in Hubei Province in central China. The cases had been reported since December 8, 2019, and many patients worked at or lived around the local Huanan Seafood Wholesale Market although other early cases had no exposure to this market [1] . On January 7, a novel coronavirus, originally abbreviated as 2019-nCoV by WHO, was identified from the throat swab sample of a patient [2] . This pathogen was later renamed as severe acute respiratory syndrome coronavirus 2 (SARS-CoV-2) by the Coronavirus Study Group [3] and the disease was named coronavirus disease 2019 (COVID-19) by the WHO. As of January 30, 7736 confirmed and 12,167 suspected cases had been reported in China and 82 confirmed cases had been detected in 18 other countries [4] . In the same day, WHO declared the SARS-CoV-2 outbreak as a Public Health Emergency of International Concern (PHEIC) [4] .
According to the National Health Commission of China, the mortality rate among confirmed cased in China was 2.1% as of February 4 [5] and the mortality rate was 0.2% among cases outside China [6] . Among patients admitted to hospitals, the mortality rate ranged between 11% and 15% [7] , [8] . COVID-19 is moderately infectious with a relatively high mortality rate, but the information available in public reports and published literature is rapidly increasing. The aim of this review is to summarize the current understanding of COVID-19 including causative agent, pathogenesis of the disease, diagnosis and treatment of the cases, as well as control and prevention strategies.
The virus: classification and origin
SARS-CoV-2 is a member of the family Coronaviridae and order Nidovirales. The family consists of two subfamilies, Coronavirinae and Torovirinae and members of the subfamily Coronavirinae are subdivided into four genera: (a) Alphacoronavirus contains the human coronavirus (HCoV)-229E and HCoV-NL63; (b) Betacoronavirus includes HCoV-OC43, Severe Acute Respiratory Syndrome human coronavirus (SARS-HCoV), HCoV-HKU1, and Middle Eastern respiratory syndrome coronavirus (MERS-CoV); (c) Gammacoronavirus includes viruses of whales and birds and; (d) Deltacoronavirus includes viruses isolated from pigs and birds [9] . SARS-CoV-2 belongs to Betacoronavirus together with two highly pathogenic viruses, SARS-CoV and MERS-CoV. SARS-CoV-2 is an enveloped and positive-sense single-stranded RNA (+ssRNA) virus [16] .
SARS-CoV-2 is considered a novel human-infecting Betacoronavirus [10] . Phylogenetic analysis of the SARS-CoV-2 genome indicates that the virus is closely related (with 88% identity) to two bat-derived SARS-like coronaviruses collected in 2018 in eastern China (bat-SL-CoVZC45 and bat-SL-CoVZXC21) and genetically distinct from SARS-CoV (with about 79% similarity) and MERS-CoV [10] . Using the genome sequences of SARS-CoV-2, RaTG13, and SARS-CoV [11] , a further study found that the virus is more related to BatCoV RaTG13, a bat coronavirus that was previously detected in Rhinolophus affinis from Yunnan Province, with 96.2% overall genome sequence identity [11] . A study found that no evidence of recombination events detected in the genome of SARS-CoV-2 from other viruses originating from bats such as BatCoV RaTG13, SARS-CoV and SARSr-CoVs [11] . Altogether, these findings suggest that bats might be the original host of this virus [10] , [11] .
However, a study is needed to elucidate whether any intermediate hosts have facilitated the transmission of the virus to humans. Bats are unlikely to be the animal that is directly responsible for transmission of the virus to humans for several reasons [10] : (1) there were various non-aquatic animals (including mammals) available for purchase in Huanan Seafood Wholesale Market but no bats were sold or found; (2) SARS-CoV-2 and its close relatives, bat-SL-CoVZC45 and bat-SL-CoVZXC21, have a relatively long branch (sequence identity of less than 90%), suggesting those viruses are not direct ancestors of SARS-CoV-2; and (3) in other coronaviruses where bat is the natural reservoir such as SARS-CoV and MERS-CoV, other animals have acted as the intermediate host (civets and possibly camels, respectively). Nevertheless, bats do not always need an intermediary host to transmit viruses to humans. For example, Nipah virus in Bangladesh is transmitted through bats shedding into raw date palm sap [12] .
Transmission
The role of the Huanan Seafood Wholesale Market in propagating disease is unclear. Many initial COVID-19 cases were linked to this market suggesting that SARS-CoV-2 was transmitted from animals to humans [13] . However, a genomic study has provided evidence that the virus was introduced from another, yet unknown location, into the market where it spread more rapidly, although human-to-human transmission may have occurred earlier [14] . Clusters of infected family members and medical workers have confirmed the presence of person-to-person transmission [15] . After January 1, less than 10% of patients had market exposure and more than 70% patients had no exposure to the market [13] . Person-to-person transmission is thought to occur among close contacts mainly via respiratory droplets produced when an infected person coughs or sneezes. Fomites may be a large source of transmission, as SARS-CoV has been found to persist on surfaces up to 96 h [16] and other coronaviruses for up to 9 days [17] .
Whether or not there is asymptomatic transmission of disease is controversial. One initial study published on January 30 reported asymptomatic transmission [18] , but later it was found that the researchers had not directly interviewed the patient, who did in fact have symptoms prior to transmitting disease [19] . A more recent study published on February 21 also purported asymptomatic transmission [20] , but any such study could be limited by errors in self-reported symptoms or contact with other cases and fomites.
Findings about disease characteristics are rapidly changing and subject to selection bias. A study indicated the mean incubation period was 5.2 days (95% confidence interval [95%CI]: 4.1–7.0) [13] . The incubation period has been found to be as long as 19 or 24 days [21] , [22] , although case definitions typically rely on a 14 day window [23] .
The basic reproductive number ( R 0 ) has been estimated with varying results and interpretations. R 0 measures the average number of infections that could result from one infected individual in a fully susceptible population [24] . Studies from previous outbreaks found R 0 to be 2.7 for SARS [25] and 2.4 for 2009 pandemic H1N1 influenza [26] . One study estimated that that basic reproductive number ( R 0 ) was 2.2 (95% CI: 1.4–3.9) [13] . However, later in a further analysis of 12 available studies found that R 0 was 3.28 [27] . Because R 0 represents an average value it is also important to consider the role of super spreaders, who may be hugely responsible for outbreaks within large clusters but who would not largely influence the value of R 0 [28] . During the acute phase of an outbreak or prepandemic, R 0 may be unstable [24] .
In pregnancy, a study of nine pregnancy women who developed COVID-19 in late pregnancy suggested COVID-19 did not lead to substantially worse symptoms than in nonpregnant persons and there is no evidence for intrauterine infection caused by vertical transmission [29] .
In hospital setting, a study involving 138 COVID-19 suggested that hospital-associated transmission of SARS-CoV-2 occurred in 41% of patients [30] . Moreover, another study on 425 patients found that the proportion of cases in health care workers gradually increased by time [13] . These cases likely reflect exposure to a higher concentration of virus from sustained contact in close quarters.
Outside China, as of February 12, 2020, there were 441 confirmed COVID-19 cases reported in 24 countries [6] of which the first imported case was reported in Thailand on January 13, 2020 [6] , [31] . Among those countries, 11 countries have reported local transmission with the highest number of cases reported in Singapore with 47 confirmed cases [6] .
Risk factors
The incidence of SARS-CoV-2 infection is seen most often in adult male patients with the median age of the patients was between 34 and 59 years [20] , [30] , [7] , [32] . SARS-CoV-2 is also more likely to infect people with chronic comorbidities such as cardiovascular and cerebrovascular diseases and diabetes [8] . The highest proportion of severe cases occurs in adults ≥60 years of age, and in those with certain underlying conditions, such as cardiovascular and cerebrovascular diseases and diabetes [20] , [30] . Severe manifestations maybe also associated with coinfections of bacteria and fungi [8] .
Fewer COVID-19 cases have been reported in children less than 15 years [20] , [30] , [7] , [32] . In a study of 425 COVID-19 patients in Wuhan, published on January 29, there were no cases in children under 15 years of age [13] , [33] . Nevertheless, 28 paediatric patients have been reported by January 2020 [34] . The clinical features of infected paediatric patients vary, but most have had mild symptoms with no fever or pneumonia, and have a good prognosis [34] . Another study found that although a child had radiological ground-glass lung opacities, the patient was asymptomatic [35] . In summary, children might be less likely to be infected or, if infected, present milder manifestations than adults; therefore, it is possible that their parents will not seek out treatment leading to underestimates of COVID-19 incidence in this age group.
Pathogenesis and immune response
Like most other members of the coronavirus family, Betacoronavirus exhibit high species specificity, but subtle genetic changes can significantly alter their tissue tropism, host range, and pathogenicity. A striking example of the adaptability of these viruses is the emergence of deadly zoonotic diseases in human history caused by SARS-CoV [36] and MERS-CoV [37] . In both viruses, bats served as the natural reservoir and humans were the terminal host, with the palm civet and dromedary camel the intermediary host for SARS-CoV and MERS-CoV, respectively [38] , [39] . Intermediate hosts clearly play a critical role in cross species transmission as they can facilitate increased contact between a virus and a new host and enable further adaptation necessary for an effective replication in the new host [40] . Because of the pandemic potential of SARS-CoV-2, careful surveillance is immensely important to monitor its future host adaptation, viral evolution, infectivity, transmissibility, and pathogenicity.
The host range of a virus is governed by multiple molecular interactions, including receptor interaction. The envelope spike (S) protein receptor binding domain of SARS-CoV-2 was shown structurally similar to that of SARS-CoV, despite amino acid variation at some key residues [10] . Further extensive structural analysis strongly suggests that SARS-CoV-2 may use host receptor angiotensin-converting enzyme 2 (ACE2) to enter the cells [41] , the same receptor facilitating SARS-CoV to infect the airway epithelium and alveolar type 2 (AT2) pneumocytes, pulmonary cells that synthesize pulmonary surfactant [42] . In general, the spike protein of coronavirus is divided into the S1 and S2 domain, in which S1 is responsible for receptor binding and S2 domain is responsible for cell membrane fusion [10] . The S1 domain of SARS-CoV and SARS-CoV-2 share around 50 conserved amino acids, whereas most of the bat-derived viruses showed more variation [10] . In addition, identification of several key residues (Gln493 and Asn501) that govern the binding of SARS-CoV-2 receptor binding domain with ACE2 further support that SARS-CoV-2 has acquired capacity for person-to-person transmission [41] . Although, the spike protein sequence of receptor binding SARS-CoV-2 is more similar to that of SARS-CoV, at the whole genome level SARS-CoV-2 is more closely related to bat-SL-CoVZC45 and bat-SL-CoVZXC21 [10] .
However, receptor recognition is not the only determinant of species specificity. Immediately after binding to their receptive receptor, SARS-CoV-2 enters host cells where they encounter the innate immune response. In order to productively infect the new host, SARS-CoV-2 must be able to inhibit or evade host innate immune signalling. However, it is largely unknown how SARS-CoV-2 manages to evade immune response and drive pathogenesis. Given that COVID-19 and SARS have similar clinical features [7] , SARS-CoV-2 may have a similar pathogenesis mechanism as SARS-CoV. In response to SARS-CoV infections, the type I interferon (IFN) system induces the expression of IFN-stimulated genes (ISGs) to inhibit viral replication. To overcome this antiviral activity, SARS-CoV encodes at least 8 viral antagonists that modulate induction of IFN and cytokines and evade ISG effector function [43] .
The host immune system response to viral infection by mediating inflammation and cellular antiviral activity is critical to inhibit viral replication and dissemination. However, excessive immune responses together with lytic effects of the virus on host cells will result in pathogenesis. Studies have shown patients suffering from severe pneumonia, with fever and dry cough as common symptoms at onset of illness [7] , [8] . Some patients progressed rapidly with Acute Respiratory Stress Syndrome (ARDS) and septic shock, which was eventually followed by multiple organ failure and about 10% of patients have died [8] . ARDS progression and extensive lung damage in COVID-19 are further indications that ACE2 might be a route of entry for the SARS-CoV-2 as ACE2 is known abundantly present on ciliated cells of the airway epithelium and alveolar type II (cells (pulmonary cells that synthesize pulmonary surfactant) in humans [44] .
Patients with SARS and COVID-19 have similar patterns of inflammatory damage. In serum from patients diagnosed with SARS, there is increased levels of proinflammatory cytokines (e.g. interleukin (IL)-1, IL6, IL12, interferon gamma (IFNγ), IFN-γ-induced protein 10 (IP10), macrophage inflammatory proteins 1A (MIP1A) and monocyte chemoattractant protein-1 (MCP1)), which are associated with pulmonary inflammation and severe lung damage [45] . Likewise, patients infected with SARS-CoV-2 are reported to have higher plasma levels of proinflammatory cytokines including IL1β, IL-2, IL7, TNF-α, GSCF, MCP1 than healthy adults [7] . Importantly, patients in the intensive care unit (ICU) have a significantly higher level of GSCF, IP10, MCP1, and TNF-α than those non-ICU patients, suggesting that a cytokine storm might be an underlying cause of disease severity [7] . Unexpectedly, anti-inflammatory cytokines such as IL10 and IL4 were also increased in those patients [7] , which was uncommon phenomenon for an acute phase viral infection. Another interesting finding, as explained before, was that SARS-CoV-2 has shown to preferentially infect older adult males with rare cases reported in children [7] , [8] . The same trend was observed in primate models of SARS-CoV where the virus was found more likely to infect aged Cynomolgus macaque than young adults [46] . Further studies are necessary to identify the virulence factors and the host genes of SARS-CoV-2 that allows the virus to cross the species-specific barrier and cause lethal disease in humans.
Clinical manifestations
Clinical manifestations of 2019-nCoV infection have similarities with SARS-CoV where the most common symptoms include fever, dry cough, dyspnoea, chest pain, fatigue and myalgia [7] , [30] , [47] . Less common symptoms include headache, dizziness, abdominal pain, diarrhoea, nausea, and vomiting [7] , [30] . Based on the report of the first 425 confirmed cases in Wuhan, the common symptoms include fever, dry cough, myalgia and fatigue with less common are sputum production, headache, haemoptysis, abdominal pain, and diarrhoea [13] . Approximately 75% patients had bilateral pneumonia [8] . Different from SARS-CoV and MERS-CoV infections, however, is that very few COVID-19 patients show prominent upper respiratory tract signs and symptoms such as rhinorrhoea, sneezing, or sore throat, suggesting that the virus might have greater preference for infecting the lower respiratory tract [7] . Pregnant and non-pregnant women have similar characteristics [48] . The common clinical presentation of 2019-nCoV infection are presented in Table 1 .
Clinical symptoms of patients with 2019-nCoV infection.
Severe complications such as hypoxaemia, acute ARDS, arrythmia, shock, acute cardiac injury, and acute kidney injury have been reported among COVID-19 patients [7] , [8] . A study among 99 patients found that approximately 17% patients developed ARDS and, among them, 11% died of multiple organ failure [8] . The median duration from first symptoms to ARDS was 8 days [30] .
Efforts to control spread of COVID-19, institute quarantine and isolation measures, and appropriately clinically manage patients all require useful screening and diagnostic tools. While SARS-CoV-2 is spreading, other respiratory infections may be more common in a local community. The WHO has released a guideline on case surveillance of COVID-19 on January 31, 2020 [23] . For a person who meets certain criteria, WHO recommends to first screen for more common causes of respiratory illness given the season and location. If a negative result is found, the sample should be sent to referral laboratory for SARS-CoV-2 detection.
Case definitions can vary by country and will evolve over time as the epidemiological circumstances change in a given location. In China, a confirmed case from January 15, 2020 required an epidemiological linkage to Wuhan within 2 weeks and clinical features such as fever, pneumonia, and low white blood cell count. On January 18, 2020 the epidemiological criterion was expanded to include contact with anyone who had been in Wuhan in the past 2 weeks [50] . Later, the case definitions removed the epidemiological linkage.
The WHO has put forward case definitions [23] . Suspected cases of COVID-19 are persons (a) with severe acute respiratory infections (history of fever and cough requiring admission to hospital) and with no other aetiology that fully explains the clinical presentation and a history of travel to or residence in China during the 14 days prior to symptom onset; or (b) a patient with any acute respiratory illness and at least one of the following during the 14 days prior to symptom onset: contact with a confirmed or probable case of SARS-CoV-2 infection or worked in or attended a health care facility where patients with confirmed or probable SARS-CoV-2 acute respiratory disease patients were being treated. Probable cases are those for whom testing for SARS-CoV-2 is inconclusive or who test positive using a pan-coronavirus assay and without laboratory evidence of other respiratory pathogens. A confirmed case is one with a laboratory confirmation of SARS-CoV-2 infection, irrespective of clinical signs and symptoms.
For patients who meet diagnostic criteria for SARS-CoV-2 testing, the CDC recommends collection of specimens from the upper respiratory tract (nasopharyngeal and oropharyngeal swab) and, if possible, the lower respiratory tract (sputum, tracheal aspirate, or bronchoalveolar lavage) [51] . In each country, the tests are performed by laboratories designated by the government.
Laboratory findings
Among COVID-19 patients, common laboratory abnormalities include lymphopenia [8] , [20] , [30] , prolonged prothrombin time, and elevated lactate dehydrogenase [30] . ICU-admitted patients had more laboratory abnormalities compared with non-ICU patients [30] , [7] . Some patients had elevated aspartate aminotransferase, creatine kinase, creatinine, and C-reactive protein [20] , [7] , [35] . Most patients have shown normal serum procalcitonin levels [20] , [30] , [7] .
COVID-19 patients have high level of IL1β, IFN-γ, IP10, and MCP1 [7] . ICU-admitted patients tend to have higher concentration of granulocyte-colony stimulating factor (GCSF), IP10, MCP1A, MIP1A, and TNF-α [7] .
Radiology findings
Radiology finding may vary with patients age, disease progression, immunity status, comorbidity, and initial medical intervention [52] . In a study describing 41 of the initial cases of 2019-nCoV infection, all 41 patients had pneumonia with abnormal findings on chest computed tomography (CT-scan) [7] . Abnormalities on chest CT-scan were also seen in another study of 6 cases, in which all of them showed multifocal patchy ground-glass opacities notably nearby the peripheral sections of the lungs [35] . Data from studies indicate that the typical of chest CT-scan findings are bilateral pulmonary parenchymal ground-glass and consolidative pulmonary opacities [7] , [8] , [20] , [30] , [32] , [53] . The consolidated lung lesions among patients five or more days from disease onset and those 50 years old or older compared to 4 or fewer days and those 50 years or younger, respectively [47] .
As the disease course continue, mild to moderate progression of disease were noted in some cases which manifested by extension and increasing density of lung opacities [49] . Bilateral multiple lobular and subsegmental areas of consolidation are typical findings on chest CT-scan of ICU-admitted patients [7] . A study among 99 patients, one patient had pneumothorax in an imaging examination [8] .
Similar to MERS-CoV and SARS-CoV, there is still no specific antiviral treatment for COVID-19 [54] . Isolation and supportive care including oxygen therapy, fluid management, and antibiotics treatment for secondary bacterial infections is recommended [55] . Some COVID-19 patients progressed rapidly to ARDS and septic shock, which was eventually followed by multiple organ failure [7] , [8] . Therefore, the effort on initial management of COVID-19 must be addressed to the early recognition of the suspect and contain the disease spread by immediate isolation and infection control measures [56] .
Currently, no vaccination is available, but even if one was available, uptake might be suboptimal. A study of intention to vaccinate during the H1N1 pandemic in the United States was around 50% at the start of the pandemic in May 2009 but had decreased to 16% by January 2010 [57] .
Neither is a treatment available. Therefore, the management of the disease has been mostly supportive referring to the disease severity which has been introduced by WHO. If sepsis is identified, empiric antibiotic should be administered based on clinical diagnosis and local epidemiology and susceptibility information. Routine glucocorticoids administration are not recommended to use unless there are another indication [58] . Clinical evidence also does not support corticosteroid treatment [59] . Use of intravenous immunoglobulin might help for severely ill patients [8] .
Drugs are being evaluated in line with past investigations into therapeutic treatments for SARS and MERS [60] . Overall, there is not robust evidence that these antivirals can significantly improve clinical outcomes A. Antiviral drugs such as oseltamivir combined with empirical antibiotic treatment have also been used to treat COVID-19 patients [7] . Remdesivir which was developed for Ebola virus, has been used to treat imported COVID-19 cases in US [61] . A brief report of treatment combination of Lopinavir/Ritonavir, Arbidol, and Shufeng Jiedu Capsule (SFJDC), a traditional Chinese medicine, showed a clinical benefit to three of four COVID-19 patients [62] . There is an ongoing clinical trial evaluating the safety and efficacy of lopinavir-ritonavir and interferon-α 2b in patients with COVID-19 [55] . Ramsedivir, a broad spectrum antivirus has demonstrated in vitro and in vivo efficacy against SARS-CoV-2 and has also initiated its clinical trial [63] , [64] . In addition, other potential drugs from existing antiviral agent have also been proposed [65] , [66] .
Control and prevention strategies
COVID-19 is clearly a serious disease of international concern. By some estimates it has a higher reproductive number than SARS [27] , and more people have been reported to have been infected or died from it than SARS [67] . Similar to SARS-CoV and MERS-CoV, disrupting the chain of transmission is considered key to stopping the spread of disease [68] . Different strategies should be implemented in health care settings and at the local and global levels.
Health care settings can unfortunately be an important source of viral transmission. As shown in the model for SARS, applying triage, following correct infection control measures, isolating the cases and contact tracing are key to limit the further spreading of the virus in clinics and hospitals [68] . Suspected cases presenting at healthcare facilities with symptoms of respiratory infections (e.g. runny nose, fever and cough) must wear a face mask to contain the virus and strictly adhere triage procedure. They should not be permitted to wait with other patients seeking medical care at the facilities. They should be placed in a separated, fully ventilated room and approximately 2 m away from other patients with convenient access to respiratory hygiene supplies [69] . In addition, if a confirmed COVID-19 case require hospitalization, they must be placed in a single patient room with negative air pressure – a minimum of six air changes per hour. Exhausted air has to be filtered through high efficiency particulate air (HEPA) and medical personnel entering the room should wear personal protective equipment (PPE) such as gloves, gown, disposable N95, and eye protection. Once the cases are recovered and discharged, the room should be decontaminated or disinfected and personnel entering the room need to wear PPE particularly facemask, gown, eye protection [69] .
In a community setting, isolating infected people are the primary measure to interrupt the transmission. For example, immediate actions taken by Chinese health authorities included isolating the infected people and quarantining of suspected people and their close contacts [70] . Also, as there are still conflicting assumptions regarding the animal origins of the virus (i.e. some studies linked the virus to bat [71] , [72] while others associated the virus with snake [73] ), contacts with these animal fluids or tissues or consumption of wild caught animal meet should be avoided. Moreover, educating the public to recognize unusual symptoms such as chronic cough or shortness of breath is essential therefore that they could seek medical care for early detection of the virus. If large-scale community transmission occurs, mitigating social gatherings, temporary school closure, home isolation, close monitoring of symptomatic individual, provision of life supports (e.g. oxygen supply, mechanical ventilator), personal hand hygiene, and wearing personal protective equipment such as facemask should also be enforced [74] .
In global setting, locking down Wuhan city was one of the immediate measure taken by Chinese authorities and hence had slowed the global spread of COVID-19 [74] . Air travel should be limited for the cases unless severe medical attentions are required. Setting up temperature check or scanning is mandatory at airport and border to identify the suspected cases. Continued research into the virus is critical to trace the source of the outbreak and provide evidence for future outbreak [74] .
Conclusions
The current COVID-19 pandemic is clearly an international public health problem. There have been rapid advances in what we know about the pathogen, how it infects cells and causes disease, and clinical characteristics of disease. Due to rapid transmission, countries around the world should increase attention into disease surveillance systems and scale up country readiness and response operations including establishing rapid response teams and improving the capacity of the national laboratory system.
Competing interests
The authors declare that they have no competing interests.
Ethical approval
Not required.
Numbers, Facts and Trends Shaping Your World
Read our research on:
Full Topic List
Regions & Countries
- Publications
- Our Methods
- Short Reads
- Tools & Resources
Read Our Research On:
Some gender disparities widened in the U.S. workforce during the pandemic
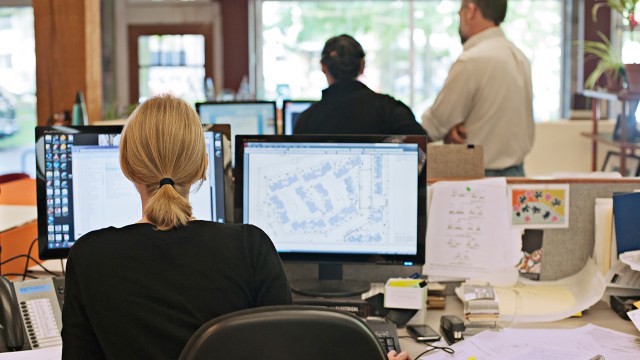
The COVID-19 recession resulted in a steep but transitory contraction in employment, with greater job losses among women than men. The recovery began in April 2020 and is not complete. As of the third quarter of 2021, the labor force ages 25 and older remains nearly 2 million below its level in the same quarter of 2019.
The pandemic is associated with an increase in some gender disparities in the labor market. Among adults 25 and older who have no education beyond high school, more women have left the labor force than men. Other disparities have stayed the same or even narrowed: The gender pay gap has remained steady, for example, and the difference in the average hours worked by men and women has slightly diminished.
The COVID-19 recession differed from prior recent recessions in that it disproportionately eliminated jobs employing women. Media accounts often referred to it as a “ shecession .” As the economy and labor market have been recovering for more than 21 months, Pew Research Center conducted this analysis to investigate if there are lingering impacts on the labor market opportunities of women.
Labor force estimates and average hours worked are derived from the monthly Current Population Survey (CPS), sponsored jointly by the U.S. Census Bureau and the U.S. Bureau of Labor Statistics. The CPS is the nation’s premier labor force survey and is the basis for the monthly national unemployment rate released on the first Friday of each month. The CPS is based on a sample survey of about 70,000 households . The estimates are not seasonally adjusted.
For a quarter of the sample each month, the CPS also records data on usual hourly earnings for workers paid by the hour and usual weekly earnings and hours worked for other workers.
The CPS microdata files analyzed were provided by the Integrated Public Use Microdata Series (IPUMS) at the University of Minnesota .
The COVID-19 outbreak has affected data collection efforts by the U.S. government in its surveys, especially limiting in-person data collection. This resulted in about an 8 percentage point dec rease in the response rate for the CPS in September 2021. It is possible that some measures of labor market activity and its demographic composition are affected by these changes in data collection.
Overall, the number of women ages 25 and older in the labor force has fallen 1.3% since the third quarter of 2019, similar to the 1.1% decline of men in the labor force.
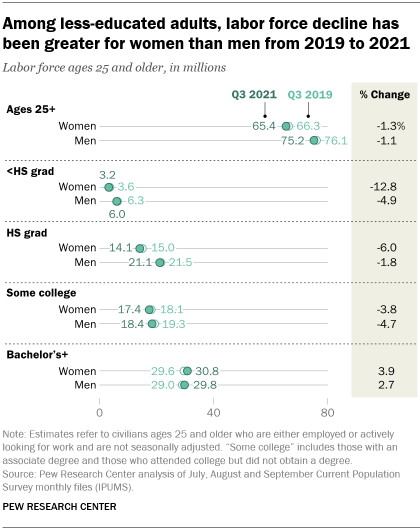
But this modest overall change obscures divergent outcomes for labor force members with different levels of education. Women who have no education beyond high school exited the labor force in greater numbers than similarly educated men. However, the pandemic has not interrupted the long-running gains of women among the college-educated labor force.
From the third quarter of 2019 to the same quarter of 2021, the number of women in the labor force who are not high school graduates decreased 12.8%, dwarfing the 4.9% contraction among comparably educated men. The pandemic also disproportionately affected women with a high school diploma. The ranks of women in the high-school-educated labor force have declined 6.0% since the third quarter of 2019. The labor force of similarly educated men has fallen only 1.8%.
Among the labor force with at least some amount of education beyond high school, women have fared at least as well as men. The number of men and women in the labor force who have some college experience but not a bachelor’s degree has contracted for both groups, with no strong disparities between the two. Both men and women with at least a bachelor’s degree saw positive gains in the labor force (2.7% and 3.9%, respectively) from 2019 to 2021.
What accounts for the larger labor force withdrawals among less-educated women than men during the pandemic? It is complex but there seems to be a consensus that it partly reflects how women are overrepresented in certain health care, food preparation and personal service occupations that were sharply curtailed at the start of the pandemic. Although women overall are more likely than men to be able to work remotely, they are disproportionately employed in occupations that require them to work on-site and in close proximity to others.
It is less clear whether women’s parental roles and limited child care and schooling options have played a large role in forcing them to exit the labor market. The number of mothers and fathers in the labor force has declined in similar fashion over the past two years.
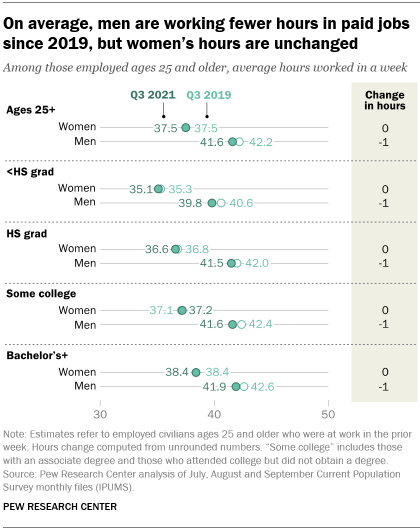
Turning to the number of hours employees work per week, on average, there have been small changes associated with the pandemic and they have occurred among men. In the third quarter of 2021, women ages 25 and older worked 37.5 hours on average in paid employment, unchanged from how much they worked two years earlier. Men ages 25 and older worked 41.6 hours on average in the third quarter of 2021. That is 0.7 fewer hours than they worked pre-pandemic (42.2). So, the disparity in hours of paid employment between women and men workers has somewhat narrowed.
The pandemic is also not associated with a widening of the gender pay gap . Among full- and part-time workers ages 25 and older, women earned 86% of what men earned based on median hourly earnings in the third quarter of 2021. Two years ago, the estimated gender pay gap was 85%.
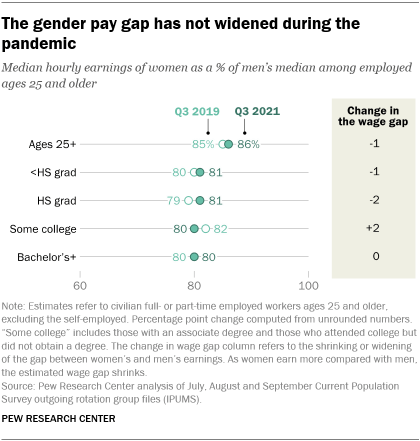
The overall pay gap partly reflects that employed women have higher levels of education than employed men. In 2021, 48% of women workers ages 25 and older had completed at least a bachelor’s degree compared with 40% of men. Workers with at least a bachelor’s degree tend to earn more and thus women’s earnings are boosted by their greater educational attainment. The gender pay gap is greater when you look at groups of women and men with equal levels of education. The gap depends on the education level, but in 2021 women ages 25 and older earned closer to 80 cents on the dollar compared with equally educated men.
- Coronavirus (COVID-19)
- COVID-19 & the Economy
- Economics, Work & Gender
- Gender & Work
- Gender Pay Gap
- Unemployment
How Americans View the Coronavirus, COVID-19 Vaccines Amid Declining Levels of Concern
Online religious services appeal to many americans, but going in person remains more popular, about a third of u.s. workers who can work from home now do so all the time, how the pandemic has affected attendance at u.s. religious services, mental health and the pandemic: what u.s. surveys have found, most popular.
1615 L St. NW, Suite 800 Washington, DC 20036 USA (+1) 202-419-4300 | Main (+1) 202-857-8562 | Fax (+1) 202-419-4372 | Media Inquiries
Research Topics
- Age & Generations
- Economy & Work
- Family & Relationships
- Gender & LGBTQ
- Immigration & Migration
- International Affairs
- Internet & Technology
- Methodological Research
- News Habits & Media
- Non-U.S. Governments
- Other Topics
- Politics & Policy
- Race & Ethnicity
- Email Newsletters
ABOUT PEW RESEARCH CENTER Pew Research Center is a nonpartisan fact tank that informs the public about the issues, attitudes and trends shaping the world. It conducts public opinion polling, demographic research, media content analysis and other empirical social science research. Pew Research Center does not take policy positions. It is a subsidiary of The Pew Charitable Trusts .
Copyright 2024 Pew Research Center
Terms & Conditions
Privacy Policy
Cookie Settings
Reprints, Permissions & Use Policy
Despite High Potential, 75 Vulnerable Economies Face ‘Historic Reversal’
In Half of IDA Countries, Income Gap with Wealthiest Economies is Widening
WASHINGTON, April 15, 2024 — Despite their high potential to advance global prosperity, one-half of the world’s 75 most vulnerable countries are facing a widening income gap with the wealthiest economies for the first time in this century, a new World Bank report has found . Taking full advantage of their younger populations, their rich natural resources, and their abundant solar-energy potential can help them overcome the setback.
The report, The Great Reversal: Prospects, Risks, and Policies in International Development Association Countries , offers the first comprehensive look at the opportunities and risks confronting the 75 countries eligible for grants and zero to low-interest loans from the World Bank’s International Development Association (IDA). These countries are home to a quarter of humanity—1.9 billion people. At a time when populations are aging nearly everywhere else, IDA countries will enjoy a growing share of young workers through 2070—a huge potential “demographic dividend.” These countries are also rich in natural resources, enjoy high potential for solar-energy generation, and boast a large reservoir of mineral deposits that could be crucial for the world’s transition to clean energy.
Yet a historic reversal is underway for them. Over 2020-24, average per capita incomes in half of IDA countries—the largest share since the start of this century—have been growing more slowly than those of wealthy economies. This is widening the income gap between these two groups of countries. One out of three IDA countries is poorer, on average, than it was on the eve of the COVID-19 pandemic . The extreme-poverty rate is more than eight times the average in the rest of the world: one in four people in IDA countries struggles on less than $2.15 a day. These countries now account for 90% of all people facing hunger or malnutrition. Half of these countries are either in debt distress or at high risk of it. Still, except for the World Bank Group and other multilateral development donors, foreign lenders—private as well as government creditors—have been backing away from them.
“The world cannot afford to turn its back on IDA countries,” said Indermit Gill, the World Bank Group’s Chief Economist and Senior Vice President . “The welfare of these countries has always been crucial to the long-term outlook for global prosperity. Three of the world’s economic powerhouses today—China, India, and South Korea—were all once IDA borrowers. All three prospered in ways that whittled down extreme poverty and raised living standards. With help from abroad, today’s batch of IDA countries has the potential to do the same.”
More than half of all IDA countries—39 in all—are in Sub-Saharan Africa. Fourteen of them—mainly small island states—are in East Asia, and eight are in Latin America and the Caribbean. In South Asia, all countries except for India are IDA countries. Thirty-one IDA countries have per capita incomes of less than $1,315 a year. Thirty-three are fragile and conflict-affected states.
IDA countries share similar opportunities. The “demographic dividend”—a deep and growing reserve of young workers—is one of them. Abundant natural resources is another. These countries account for about 20% of global production of tin, copper, and gold. In addition, some IDA countries possess critical mineral deposits essential for the global energy transition. Because of their abundant sunshine, most IDA countries are well situated to take advantage of solar energy. On average, their long-term daily solar-electricity generation potential is among the highest in the world.
This potential, however, comes with risks that must be managed. To reap the demographic dividend, IDA governments will need to undertake policies to improve education and health outcomes and make sure that jobs are available for the rising number of young people who will enter the workforce in the coming decades. To seize the full potential of their natural-resource wealth, IDA countries will need to improve policy frameworks and build stronger institutions capable of better economic management. All of this will require ambitious domestic policy reforms—and significant financial support from the international community.
“IDA countries have incredible potential to deliver strong, sustainable, and inclusive growth. Realizing this potential will require them to implement an ambitious set of policies centered on boosting investment,” said Ayhan Kose, the World Bank’s Deputy Chief Economist and Director of the Prospects Group . “ This means improving fiscal, monetary, and financial policy frameworks and advancing an array of structural reforms to strengthen institutions and enhance human capital."
IDA countries today have large investment needs. In the poorest of them, closing existing development and infrastructure gaps and building resilience to climate change will require investment that amounts to nearly 10% of GDP. The costs of climate disasters have doubled in IDA countries over the past decade: Economic losses from natural disasters average 1.3% of GDP a year—four times the average of other emerging market and developing economies. Such needs will require IDA countries to generate sustained investment booms—the type that boosts productivity and incomes and reduces poverty. Historically, such investment booms have often been sparked by a comprehensive package of policy measures—to bolster fiscal and monetary frameworks, ramp up cross-border trade and financial flows, and improve the quality of institutions. Such reforms are never easy, the report notes. They need careful sequencing and implementation. But previous IDA countries have shown they are possible.
IDA countries will need significant international financial support to make progress and lower the risk of “protracted stagnation,” the report notes . Stronger cooperation on global policy issues—including fighting climate change, facilitating more timely and effective debt restructurings, and supporting cross-border trade and investment—will also be crucial to help IDA countries avert a lost decade in development .
Website: https://www.worldbank.org/en/research/publication/prospects-risks-and-policies-in-IDA-countries
Facebook: http://www.facebook.com/worldbank
X (Twitter): http://www.twitter.com/worldbank
YouTube: http://www.youtube.com/worldbank
This site uses cookies to optimize functionality and give you the best possible experience. If you continue to navigate this website beyond this page, cookies will be placed on your browser. To learn more about cookies, click here .
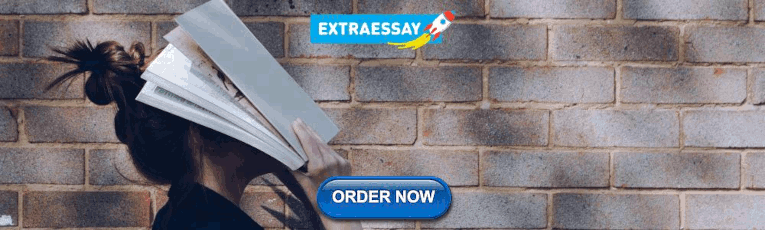
IMAGES
VIDEO
COMMENTS
Top 15 Covid-19 Research Topics and Areas. October 2020. DOI: 10.13140/RG.2.2.15159.75689. Authors: Joab O. Odhiambo. University of Nairobi. Jacob Oketch Okungu. Jomo Kenyatta University of ...
Scroll down the page to view all COVID-19 articles, stories, and resources from across NIH. You can also select a topic from the list to view resources on that topic. - Any -. Aging. Cancer. Children. Clinical Trials. Immune Responses. Long COVID.
Here the authors show that, in convalescent COVID-19 patients, memory T cell responses are detectable up to 317 days post-symptom onset, in which the presence of stem cell-like memory T cells ...
Covid-19 has affected humanity in a major way. An extremely dangerous virus, hitherto unknown to humanity, had to be studied and contained in order to overcome the pandemic. Research on Covid-19 had surged in the early days with an unprecedented surge in the publications on that specific topic.
The spread of the "Severe Acute Respiratory Coronavirus 2" (SARS-CoV-2), the causal agent of COVID-19, was characterized as a pandemic by the World Health Organization (WHO) in March 2020 and has triggered an international public health emergency [].The numbers of confirmed cases and deaths due to COVID-19 are rapidly escalating, counting in millions [], causing massive economic strain ...
The impact on research in progress prior to COVID-19 was rapid, dramatic, and no doubt will be long term. The pandemic curtailed most academic, industry, and government basic science and clinical ...
COVID-19 pandemic has severely impacted the crude, stock market, gold and metals and almost all areas of the global market [ 1 ]. Large research laboratories and corporate houses are working with a high speed to develop medicines and vaccines for the prevention and treatment of this dreaded disease. To deal with these current health management ...
In support of this notion, an analysis of 569,742 cases of COVID-19 collected from multiple sites in US indicated a death rate of 0.1% among vaccinated people infected with Delta variant of COVID-19 , which is much lower than those of 1.3% or 1.6% among unvaccinated people and comparable to that of seasonal influenza. Thus, even if the ...
Download MP3. In the final Coronapod of 2020, we dive into the scientific literature to reflect on the COVID-19 pandemic. Researchers have discovered so much about SARS-CoV-2 - information that ...
The WHO Covid-19 Research Database is a resource created in response to the Public Health Emergency of International Concern (PHEIC). Its content remains searchable and spans the time period March 2020 to June 2023. Since June 2023, manual updates to the database have been discontinued. The WHO evidence retrieval sub-group has begun ...
The COVID-19 outbreak has posed an unprecedented challenge to humanity and science. On the one side, public and private incentives have been put in place to promptly allocate resources toward research areas strictly related to the COVID-19 emergency. However, research in many fields not directly related to the pandemic has been displaced. In this paper, we assess the impact of COVID-19 on ...
This article is part of the Research Topic Coronavirus Disease (COVID-19): The Impact and Role of Mass Media During the Pandemic View all 16 articles. Editorial: Coronavirus Disease (COVID-19): The Impact and Role of Mass Media During the Pandemic ... Dhanani and Franz examined a sample of 1,141 adults (Mage = 44.66; 46.9% female, 74.7% White ...
The outbreak of coronavirus disease 2019 (COVID-19) has created a global health crisis that has had a deep impact on the way we perceive our world and our everyday lives. Not only the rate of contagion and patterns of transmission threatens our sense of agency, but the safety measures put in place to contain the spread of the virus also require social distancing by refraining from doing what ...
The published literature on COVID now exceeds 211,000 papers, books, and documents, which include: 22,866 observational studies, 19,591 reviews, 1496 meta-analyses and 781 randomized control trials. These publications comprise the backdrop for our research and writing. The project began in the spring of 2020 based on a limited source of cumulative COVID-19 data and has broadened considerably ...
The COVID-19 or the SARS-CoV-2 is rapidly unfurling from Wuhan in Hubei Province of China to worldwide [ 4 ]. Initial confirmation of the pandemic was carried out by conducting studies on 99 patients with COVID-19 pneumonia, from which 49% of patients exhibited a history of subjection to the Huanan seafood market.
determining students' COVID-19 experiences. For example, the expected probability of delaying graduation due to COVID-19 increases by approximately 25% if either a student's subjective probability of being late on a debt payment in the following 90 days (a measure of nancial fragility) or subjective probability of requiring
For example, when one study found that cloth masks didn't work in "high-risk situations," it was sometimes used as evidence against mask mandates. However, a look beyond the headlines revealed that the study was of health care workers treating COVID-19 patients, which is a vastly more dangerous situation than, say, going to the grocery store.
A systematic review of 194 studies with over 700,000 participants reported that on average, 45% of survivors of COVID-19 had at least one unresolved symptom at a mean follow-up of around 4 months ...
Just 20% of the public views the coronavirus as a major threat to the health of the U.S. population and only 10% are very concerned about getting a serious case themselves. In addition, a relatively small share of U.S. adults (28%) say they've received an updated COVID-19 vaccine since last fall. report | Mar 28, 2023.
The outbreak has dramatically changed Americans' lives and relationships over the past year. We asked people to tell us about their experiences - good and bad - in living through this moment in history. Pew Research Center has been asking survey questions over the past year about Americans' views and reactions to the COVID-19 pandemic.
Long covid (post covid-19 condition) is a complex condition with diverse manifestations, uncertain prognosis and wide variation in current approaches to management. There have been calls for formal quality standards to reduce a so-called "postcode lottery" of care. The original aim of this study—to examine the nature of quality in long covid care and reduce unwarranted variation in ...
When baseline variables (e.g., older age as a risk factor for worse outcome among those with COVID-19 infection) are related to the outcome, (e.g., one intended to support effectiveness of a ...
Research findings could explain why young kids rarely get very sick from COVID-19. Children's noses pack a punch that could help explain COVID-19's typically mild course in young kids. Researchers hope to parlay that 'nasal magic' into increased protections for adults.
Makam's research looks at the long-term effects of severe COVID-19 illness among the sickest of survivors early in the pandemic. Photo by Erin Lubin UC San Francisco researchers examined COVID-19 patients across the United States who survived some of the longest and most harrowing battles with the virus and found that about two-thirds still ...
The sixth question concerns how COVID-19 should be treated and what treatment options should be made available. COVID-19 is a self-limiting disease in more than 80% of patients. Severe pneumonia occurred in about 15% of cases as revealed in studies with large cohorts of patients. The gross case fatality is 3.4% worldwide as of February 25, 2020.
Many Republican governors reacted furiously after President Joe Biden said he would require employees at large businesses to either get vaccinated against COVID-19 or submit to weekly testing. But Republican- and Democratic-led states alike already require hundreds of thousands of their citizens - infants, toddlers and schoolchildren, mostly - to be vaccinated against a panoply of diseases.
Although all analyzed articles contained information on the public-health effects of COVID-19 or measures to limit its spread (SI Fig. S4), topics of focus differed widely, for example including ...
Transmission. The role of the Huanan Seafood Wholesale Market in propagating disease is unclear. Many initial COVID-19 cases were linked to this market suggesting that SARS-CoV-2 was transmitted from animals to humans .However, a genomic study has provided evidence that the virus was introduced from another, yet unknown location, into the market where it spread more rapidly, although human-to ...
The COVID-19 recession differed from prior recent recessions in that it disproportionately eliminated jobs employing women. Media accounts often referred to it as a "shecession."As the economy and labor market have been recovering for more than 21 months, Pew Research Center conducted this analysis to investigate if there are lingering impacts on the labor market opportunities of women.
Global data and statistics, research and publications, and topics in poverty and development. WORK WITH US. Jobs, procurement, training, and events. News; ... One out of three IDA countries is poorer, on average, than it was on the eve of the COVID-19 pandemic. The extreme-poverty rate is more than eight times the average in the rest of the ...