Loading metrics
Open Access
Peer-reviewed
Meta-Research Article
Meta-Research Articles feature data-driven examinations of the methods, reporting, verification, and evaluation of scientific research.
See Journal Information »
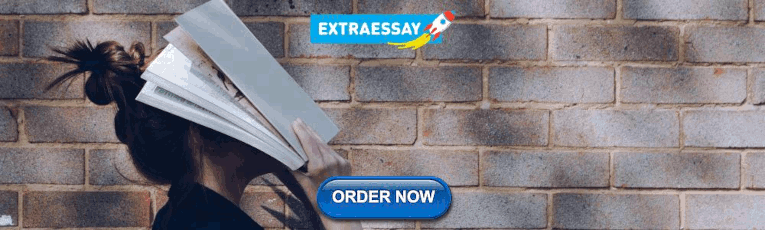
The evolving role of preprints in the dissemination of COVID-19 research and their impact on the science communication landscape
Contributed equally to this work with: Nicholas Fraser, Liam Brierley
Roles Conceptualization, Data curation, Formal analysis, Investigation, Methodology, Software, Validation, Visualization, Writing – original draft, Writing – review & editing
Affiliation Leibniz Information Centre for Economics, Kiel, Germany

Affiliation Department of Health Data Science, University of Liverpool, Liverpool, United Kingdom
Roles Conceptualization, Investigation, Writing – original draft, Writing – review & editing
Affiliations MRC Lab for Molecular Cell Biology, UCL, London, United Kingdom, Cell Biology and Biophysics Unit, European Molecular Biology Laboratory, Heidelberg, Germany
Roles Conceptualization, Investigation, Resources, Writing – original draft, Writing – review & editing
Affiliation ASAPbio, San Francisco, California, United States of America
Affiliation The Company of Biologists, Cambridge, United Kingdom
Roles Writing – review & editing
Affiliation The Alan Turing Institute, London, United Kingdom
Roles Conceptualization, Data curation, Formal analysis, Investigation, Methodology, Project administration, Resources, Supervision, Validation, Writing – original draft, Writing – review & editing
* E-mail: [email protected]
Affiliations Hughes Hall College, University of Cambridge, Cambridge, United Kingdom, William Harvey Research Institute, Charterhouse Square Barts and the London School of Medicine and Dentistry Queen Mary University of London, London, United Kingdom
- Nicholas Fraser,
- Liam Brierley,
- Gautam Dey,
- Jessica K. Polka,
- Máté Pálfy,
- Federico Nanni,
- Jonathon Alexis Coates
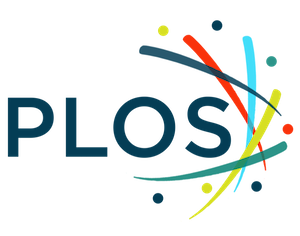
- Published: April 2, 2021
- https://doi.org/10.1371/journal.pbio.3000959
- Peer Review
- Reader Comments
The world continues to face a life-threatening viral pandemic. The virus underlying the Coronavirus Disease 2019 (COVID-19), Severe Acute Respiratory Syndrome Coronavirus 2 (SARS-CoV-2), has caused over 98 million confirmed cases and 2.2 million deaths since January 2020. Although the most recent respiratory viral pandemic swept the globe only a decade ago, the way science operates and responds to current events has experienced a cultural shift in the interim. The scientific community has responded rapidly to the COVID-19 pandemic, releasing over 125,000 COVID-19–related scientific articles within 10 months of the first confirmed case, of which more than 30,000 were hosted by preprint servers. We focused our analysis on bioRxiv and medRxiv, 2 growing preprint servers for biomedical research, investigating the attributes of COVID-19 preprints, their access and usage rates, as well as characteristics of their propagation on online platforms. Our data provide evidence for increased scientific and public engagement with preprints related to COVID-19 (COVID-19 preprints are accessed more, cited more, and shared more on various online platforms than non-COVID-19 preprints), as well as changes in the use of preprints by journalists and policymakers. We also find evidence for changes in preprinting and publishing behaviour: COVID-19 preprints are shorter and reviewed faster. Our results highlight the unprecedented role of preprints and preprint servers in the dissemination of COVID-19 science and the impact of the pandemic on the scientific communication landscape.
Citation: Fraser N, Brierley L, Dey G, Polka JK, Pálfy M, Nanni F, et al. (2021) The evolving role of preprints in the dissemination of COVID-19 research and their impact on the science communication landscape. PLoS Biol 19(4): e3000959. https://doi.org/10.1371/journal.pbio.3000959
Academic Editor: Ulrich Dirnagl, Charite Universitatsmedizin Berlin, GERMANY
Received: October 8, 2020; Accepted: March 8, 2021; Published: April 2, 2021
Copyright: © 2021 Fraser et al. This is an open access article distributed under the terms of the Creative Commons Attribution License , which permits unrestricted use, distribution, and reproduction in any medium, provided the original author and source are credited.
Data Availability: All data and code used in this study are available on GitHub ( https://github.com/preprinting-a-pandemic/pandemic_preprints ) and Zenodo (DOI: 10.5281/zenodo.4501924 ).
Funding: NF acknowledges funding from the German Federal Ministry for Education and Research, grant numbers 01PU17005B (OASE) and 01PU17011D (QuaMedFo). LB acknowledges funding from a Medical Research Council Skills Development Fellowship award, grant number MR/T027355/1. The funders had no role in study design, data collection and analysis, decision to publish, or preparation of the manuscript.
Competing interests: I have read the journal’s policy and the authors of this manuscript have the following competing interests: JP is the executive director of ASAPbio, a non-profit organization promoting the productive use of preprints in the life sciences. GD is a bioRxiv Affiliate, part of a volunteer group of scientists that screen preprints deposited on the bioRxiv server. MP is the community manager for preLights, a non-profit preprint highlighting service. GD and JAC are contributors to preLights and ASAPBio fellows.
Abbreviations: AAAS, American Association for the Advancement of Science; ACE2, angiotensin converting enzyme 2; API, Application Programming Interface; COVID-19, Coronavirus Disease 2019; CSHL, Cold Spring Harbor Laboratory; ECDC, European Centre for Disease Prevention and Control; HSD, honest significant difference; MERS, Middle East Respiratory Syndrome; ROR, Research Organisation Registry; SARS-CoV-2, Severe Acute Respiratory Syndrome Coronavirus 2; UK POST, United Kingdom Parliamentary Office of Science and Technology; WHO SB, World Health Organization Scientific Briefs
Introduction
Since January 2020, the world has been gripped by the Coronavirus Disease 2019 (COVID-19) outbreak, which has escalated to pandemic status, and caused over 98 million cases and 2.1 million deaths (43 million cases and 1.1 million deaths within 10 months of the first reported case) [ 1 – 3 ]. The causative pathogen was rapidly identified as a novel virus within the family Coronaviridae and was named Severe Acute Respiratory Syndrome Coronavirus 2 (SARS-CoV-2) [ 4 ]. Although multiple coronaviruses are ubiquitous among humans and cause only mild disease, epidemics of newly emerging coronaviruses were previously observed in SARS in 2002 [ 5 ] and Middle East Respiratory Syndrome (MERS) in 2012 [ 6 ]. The unprecedented extent and rate of spread of COVID-19 has created a critical global health emergency, and academic communities have raced to respond through research developments.
New scholarly research has traditionally been communicated via published journal articles or conference presentations. The traditional journal publishing process involves the submission of manuscripts by authors to an individual journal, which then organises peer review, the process in which other scientists (“peers”) are invited to scrutinise the manuscript and determine its suitability for publication. Authors often conduct additional experiments or analyses to address the reviewers’ concerns in 1 or more revisions. Even after this lengthy process is concluded, almost half of submissions are rejected and require resubmission to a different journal [ 7 ]. The entire publishing timeline from submission to acceptance is estimated to take approximately 6 months in the life sciences [ 8 , 9 ]; the median time between the date a preprint is posted and the date on which the first DOI of a journal article is registered is 166 days in the life sciences [ 8 ].
Preprints are publicly accessible scholarly manuscripts that have not yet been certified by peer review and have been used in some disciplines, such as physics, for communicating scientific results for over 30 years [ 10 ]. In 2013, 2 new preprint initiatives for the biological sciences launched: PeerJ Preprints, from the publisher PeerJ, and bioRxiv, from Cold Spring Harbor Laboratory (CSHL). The latter established partnerships with journals that enabled simultaneous preprint posting at the time of submission [ 11 ]. More recently, CSHL, in collaboration with Yale and BMJ, launched medRxiv, a preprint server for the medical sciences [ 12 ]. Preprint platforms serving the life sciences have subsequently flourished, and preprints submissions continue to grow year on year; two-thirds of these preprints are eventually published in peer-reviewed journals [ 8 ].
While funders and institutions explicitly encouraged prepublication data sharing in the context of the recent Zika and Ebola virus disease outbreaks [ 13 ], usage of preprints remained modest through these epidemics [ 14 ]. The COVID-19 crisis represents the first time that preprints have been widely used outside of specific communities to communicate during an epidemic.
We assessed the role of preprints in the communication of COVID-19 research in the first 10 months of the pandemic, between January 1 and October 31, 2020. We found that preprint servers hosted almost 25% of COVID-19–related science, that these COVID-19 preprints were being accessed and downloaded in far greater volume than other preprints on the same servers, and that these were widely shared across multiple online platforms. Moreover, we determined that COVID-19 preprints are shorter and are published in journals with a shorter delay following posting than their non-COVID-19 counterparts. Taken together, our data demonstrate the importance of rapidly and openly sharing science in the context of a global pandemic and the essential role of preprints in this endeavour.
COVID-19 preprints were posted early in the pandemic and represent a significant proportion of the COVID-19 literature
The COVID-19 pandemic has rapidly spread across the globe, from 3 patients in the city of Wuhan on the December 27, 2019 to over 46.1 million confirmed cases worldwide by the end of October 2020 ( Fig 1A ). The scientific community responded rapidly as soon as COVID-19 emerged as a serious threat, with publications appearing within weeks of the first reported cases ( Fig 1B ). By the end of April 2020, over 19,000 scientific publications had appeared, published both in scientific journals (12,679; approximately 65%) and on preprint servers (6,710; approximately 35%) ( Fig 1B )—in some cases, preprints had already been published in journals during this time period and thus contribute to the counts of both sources. Over the following months, the total number of COVID-19–related publications increased approximately linearly, although the proportion of these which were preprints fell: By the end of October, over 125,000 publications on COVID-19 had appeared (30,260 preprints; approximately 25%). Given an output of approximately 5 million journal articles and preprints in the entirety of 2020 (according to data from Dimensions; https://dimensions.ai ), the publication response to COVID-19 represented >2.5% of outputs during our analysis period. In comparison to other recent outbreaks of global significance caused by emerging RNA viruses, the preprint response to COVID-19 has been much larger; 10,232 COVID-19–related preprints were posted to bioRxiv and medRxiv in the first 10 months of the pandemic; in comparison, only 78 Zika virus–related and 10 Ebola virus–related preprints were posted to bioRxiv during the entire duration of the respective Zika virus epidemic (2015 to 2016) and Western African Ebola virus epidemic (2014 to 2016) ( S1A Fig ). This surge in COVID-19 preprints is not explained by general increases in preprint server usage; considering counts of outbreak-related and non-outbreak–related preprints for each outbreak (COVID-19, Ebola or Zika virus), preprint type was significantly associated with outbreak (chi-squared, χ 2 = 2559.2, p < 0.001), with the proportion of outbreak-related preprints being greatest for COVID-19.
- PPT PowerPoint slide
- PNG larger image
- TIFF original image
(A) Number of COVID-19 confirmed cases and reported deaths. Data are sourced from https://github.com/datasets/covid-19/ , based on case and death data aggregated by the Johns Hopkins University Center for Systems Science and Engineering ( https://systems.jhu.edu/ ). Vertical lines labelled (i) and (ii) refer to the date on which the WHO declared COVID-19 outbreak a Public Health Emergency of International Concern, and the date on which the WHO declared the COVID-19 outbreak to be a pandemic, respectively. (B) Cumulative growth of journal articles and preprints containing COVID-19–related search terms. (C) Cumulative growth of preprints containing COVID-19–related search terms, categorised by individual preprint servers. Journal article data in (B) are based upon data extracted from Dimensions ( https://www.dimensions.ai ; see Methods section for further details), and preprint data in (B) and (C) are based upon data gathered by Fraser and Kramer (2020). The data underlying this figure may be found in https://github.com/preprinting-a-pandemic/pandemic_preprints and https://zenodo.org/record/4587214#.YEN22Hmnx9A . COVID-19, Coronavirus Disease 2019; WHO, World Health Organization.
https://doi.org/10.1371/journal.pbio.3000959.g001
The 30,260 manuscripts posted as preprints were hosted on a range of preprint servers covering diverse subject areas not limited to biomedical research ( Fig 1C , data from [ 15 ]). It is important to note that this number includes preprints that may have been posted on multiple preprint servers simultaneously; however, by considering only preprints with unique titles (case insensitive), it appears that this only applies to a small proportion of preprint records (<5%). The total number is preprints is nevertheless likely an underestimation of the true volume of preprints posted, as a number of preprint servers and other repositories (e.g., institutional repositories) that could be expected to host COVID-19 research are not included [ 15 ]. Despite being one of the newest preprint servers, medRxiv hosted the largest number of preprints (7,882); the next largest were SSRN (4,180), Research Square (4,089), RePEc (2,774), arXiv (2,592), bioRxiv (2,328), JMIR (1,218), and Preprints.org (1,020); all other preprint servers were found to host <1,000 preprints ( Fig 1C ).
One of the most frequently cited benefits of preprints is that they allow free access to research findings [ 16 ], while a large proportion of journal articles often remain behind subscription paywalls. In response to the pandemic, a number of journal publishers began to alter their open-access policies in relation to COVID-19 manuscripts. One such change was to make COVID-19 literature temporarily open access (at least for the duration of the pandemic), with over 80,000 papers in our dataset being open access ( S1B Fig ).
Attributes of COVID-19 preprints posted between January and October 2020
To explore the attributes of COVID-19 preprints in greater detail, we focused our following investigation on two of the most popular preprint servers in the biomedical sciences: bioRxiv and medRxiv. We compared attributes of COVID-19–related preprints posted within our analysis period between January 1 and October 31, 2020 against non-COVID-19–related preprints posted in the same time frame. In total, 44,503 preprints were deposited to bioRxiv and medRxiv in this period, of which the majority (34,271, 77.0%) were non-COVID-19–related preprints ( Fig 2A , S1 Table ). During the early phase of the pandemic, the posted monthly volumes of non-COVID-19 preprints was relatively constant, while the monthly volume of COVID-19 preprints increased, peaking at 1,967 in May, and subsequently decreased month by month. These patterns persisted when the 2 preprint servers were considered independently ( S2A Fig ). Moreover, COVID-19 preprints have represented the majority of preprints posted to medRxiv each month after February 2020.
(A) Number of new preprints deposited per month. (B) Preprint screening time in days. (C) License type chosen by authors. (D) Number of versions per preprint. (E) Boxplot of preprint word counts, binned by posting month. (F) Boxplot of preprint reference counts, binned by posting month. Boxplot horizontal lines denote lower quartile, median, upper quartile, with whiskers extending to 1.5*IQR. All boxplots additionally show raw data values for individual preprints with added horizontal jitter for visibility. The data underlying this figure may be found in https://github.com/preprinting-a-pandemic/pandemic_preprints and https://zenodo.org/record/4587214#.YEN22Hmnx9A . COVID-19, Coronavirus Disease 2019.
https://doi.org/10.1371/journal.pbio.3000959.g002
The increase in the rate of preprint posting poses challenges for their timely screening. A minor but detectable difference was observed between screening time for COVID-19 and non-COVID-19 preprints ( Fig 2B ), although this difference appeared to vary with server (2-way ANOVA, interaction term; F 1,83333 = 19.22, p < 0.001). Specifically, screening was marginally slower for COVID-19 preprints than for non-COVID-19 preprints deposited to medRxiv (mean difference = 0.16 days; Tukey honest significant difference [HSD] test, p < 0.001), but not to bioRxiv ( p = 0.981). The slower screening time for COVID-19 preprints was a result of more of these preprints being hosted on medRxiv, which had slightly longer screening times overall; bioRxiv screened preprints approximately 2 days quicker than medRxiv independent of COVID-19 status (both p < 0.001; S2B Fig , S1 Table ).
Preprint servers offer authors the opportunity to post updated versions of a preprint, enabling them to incorporate feedback, correct mistakes, or add additional data and analysis. The majority of preprints existed as only a single version for both COVID-19 and non-COVID-19 works, with very few preprints existing in more than 2 versions ( Fig 2C ). This may somewhat reflect the relatively short time span of our analysis period. Although distributions were similar, COVID-19 preprints appeared to have a slightly greater number of versions, 1 [IQR 1] versus 1 [IQR 0]; Mann–Whitney test, p < 0.001). The choice of preprint server did not appear to impact on the number of versions ( S2C Fig , S1 Table ).
bioRxiv and medRxiv allow authors to select from a number of different Creative Commons ( https://creativecommons.org/ ) license types when depositing their work: CC0 (No Rights Reserved), CC-BY (Attribution), CC BY-NC (Attribution, Noncommercial), CC-BY-ND (Attribution, No Derivatives), and CC-BY-NC-ND (Attribution, Noncommercial, No Derivatives). Authors may also select to post their work without a license (i.e., All Rights Reserved) that allows text and data mining. A previous analysis has found that bioRxiv authors tend to post preprints under the more restrictive license types [ 17 ], although there appears to be some confusion among authors as to the precise implications of each license type [ 18 ]. License choice was significantly associated with preprint category (chi-squared, χ 2 = 336.0, df = 5, p < 0.001); authors of COVID-19 preprints were more likely to choose the more restrictive CC-BY-NC-ND or CC-BY-ND than those of non-COVID-19 preprints and less likely to choose CC-BY ( Fig 2D ). Again, the choice of preprint server did not appear to impact on the type of license selected by the authors ( S2D Fig ).
Given the novelty of the COVID-19 research field and rapid speed at which preprints are being posted, we hypothesised that researchers may be posting preprints in a less mature state, or based on a smaller literature base than for non-COVID preprints. To investigate this, we compared the word counts and reference counts of COVID-19 preprints and non-COVID-19 preprints from bioRxiv (at the time of data extraction, HTML full texts from which word and reference counts were derived were not available for medRxiv) ( Fig 2E ). We found that COVID-19 preprints are on average 32% shorter in length than non-COVID-19 preprints (median, 3,965 [IQR 2,433] versus 5,427 [IQR 2,790]; Mann–Whitney test, p < 0.001) ( S1 Table ). Although the length of preprints gradually increased over the analysis period, COVID-19 preprints remained shorter than non-COVID-19 preprints with a similar difference in word count, even when adjusted for factors such as authorship team size and bioRxiv subject categorisation ( S1 Model , S2 and S3 Tables). COVID-19 preprints also contain fewer references than non-COVID-19 preprints ( Fig 2F ), although not fewer than expected relative to overall preprint length, as little difference was detected in reference:word count ratios (median, 1:103 versus 1:101; p = 0.052). As word counts increased over time, the reference counts per preprint also steadily increased.
Scientists turned to preprints for the first time to share COVID-19 science
The number of authors per preprint may give an additional indication as to the amount of work, resources used, and the extent of collaboration in a manuscript. Although little difference was seen in number of authors between preprint servers ( S1 Table ), COVID-19 preprints had a marginally higher number of authors than non-COVID-19 preprints on average (median, 7 [IQR 8] versus 6 [IQR 5]; p < 0.001), due to the greater likelihood of large (11+) authorship team sizes ( Fig 3A ). However, single-author preprints were approximately 2.6 times more common for COVID-19 (6.1% of preprints) than non-COVID-19 preprints (2.3% of preprints) ( Fig 3A ).
(A) Proportion of preprints with N authors. (B) Proportion of preprints deposited by country of corresponding author (top 15 countries by total preprint volume are shown). (C) Proportions of COVID-19 and non-COVID-19 corresponding authors from each of the top 15 countries shown in (B) that had previously posted a preprint (darker bar) or were posting a preprint for the first time (lighter bar). (D) Correlation between date of the first preprint originating from a country (according to the affiliation of the corresponding author) and the date of the first confirmed case from the same country for COVID-19 preprints. (E) Change in bioRxiv/medRxiv preprint posting category for COVID-19 preprint authors compared to their previous preprint (COVID-19 or non-COVID-19), for category combinations with n > = 5 authors. For all panels containing country information, labels refer to ISO 3166 character codes. The data underlying this figure may be found in https://github.com/preprinting-a-pandemic/pandemic_preprints and https://zenodo.org/record/4587214#.YEN22Hmnx9A . COVID-19, Coronavirus Disease 2019.
https://doi.org/10.1371/journal.pbio.3000959.g003
The largest proportion of preprints in our dataset were from corresponding authors in the United States, followed by significant proportions from the United Kingdom and China ( Fig 3B ). It is notable that China is overrepresented in terms of COVID-19 preprints compared to its non-COVID-19 preprint output: 39% of preprints from Chinese corresponding authors were COVID-19 related, compared to 16.5% of the US output and 20.1% of the UK output. We also found a significant association for corresponding authors between preprint type (COVID-19 or non-COVID-19) and whether this was the author’s first bioRxiv or medRxiv preprint (chi-squared, χ 2 = 840.4, df = 1, p < 0.001). Among COVID-19 corresponding authors, 85% were posting a preprint for the first time, compared to 69% of non-COVID-19 corresponding authors in the same period. To further understand which authors have been drawn to begin using preprints since the pandemic began, we stratified these groups by country ( S4 Table ) and found significant associations for the US, UK, Germany, India (Bonferroni adjusted p < 0.001), France, Canada, Italy ( p < 0.01), and China ( p < 0.05). In all cases, a higher proportion were posting a preprint for the first time among COVID-19 corresponding authors than non-COVID-19 corresponding authors. Moreover, we found that most countries posted their first COVID-19 preprint close to the time of their first confirmed COVID-19 case ( Fig 3D ), with weak positive correlation considering calendar days of both events (Spearman rank; ρ = 0.54, p < 0.001). Countries posting a COVID-19 preprint in advance of their first confirmed case were mostly higher-income countries (e.g., US, UK, New Zealand, and Switzerland). COVID-19 preprints were deposited from over 100 countries, highlighting the global response to the pandemic.
There has been much discussion regarding the appropriateness of researchers switching to COVID-19 research from other fields [ 19 ]. To quantify whether this phenomenon was detectable within the preprint literature, we compared the bioRxiv or medRxiv category of each COVID-19 preprint to the most recent previous non-COVID-19 preprint (if any) from the same corresponding author. Most corresponding authors were not drastically changing fields, with category differences generally spanning reasonably related areas. For example, some authors that previously posted preprints in evolutionary biology have posted COVID-19 preprints in microbiology ( Fig 3E ). This suggests that—at least within the life sciences—principal investigators are utilising their labs’ skills and resources in an expected manner in their contributions to COVID-19 research.
COVID-19 preprints were published quicker than non-COVID-19 preprints
Critics have previously raised concerns that by forgoing the traditional peer-review process, preprint servers could be flooded by poor-quality research [ 20 , 21 ]. Nonetheless, earlier analyses have shown that a large proportion of preprints (approximately 70%) in the biomedical sciences are eventually published in peer-reviewed scientific journals [ 8 ]. We assessed differences in publication outcomes for COVID-19 versus non-COVID-19 preprints during our analysis period, which may be partially related to differences in preprint quality. Published status (published/unpublished) was significantly associated with preprint type (chi-squared, χ 2 = 186.2, df = 1, p < 0.001); within our time frame, 21.1% of COVID-19 preprints were published in total by the end of October, compared to 15.4% of non-COVID preprints. As expected, greater proportions published were seen among preprints posted earlier, with over 40% of COVID-19 preprints submitted in January published by the end of October and less than 10% for those published in August or later ( Fig 4A ). Published COVID-19 preprints were distributed across many journals, with clinical or multidisciplinary journals tending to publish the most COVID-19 preprints ( Fig 4B ). To determine how publishers were prioritising COVID-19 research, we compared the time from preprint posting to publication in a journal. The time interval from posting to subsequent publication was significantly reduced for COVID-19 preprints by a difference in medians of 48 days compared to non-COVID-19 preprints posted in the same time period (68 days [IQR 69] versus 116 days [IQR 90]; Mann–Whitney test, p < 0.001). This did not appear to be driven by any temporal changes in publishing practices, as the distribution of publication times for non-COVID-19 preprints was similar to our control time frame of January to December 2019 ( Fig 4C ). This acceleration additionally varied between publishers (2-way ANOVA, interaction term preprint type*publisher; F 9,5273 = 6.58, p < 0.001) and was greatest for the American Association for the Advancement of Science (AAAS) at an average difference of 102 days (Tukey HSD; p < 0.001) ( Fig 4D ).
(A) Percentage of COVID-19 versus non-COVID-19 preprints published in peer-reviewed journals, by preprint posting month. (B) Destination journals for COVID-19 preprints that were published within our analysis period. Shown are the top 10 journals by publication volume. (C) Distribution of the number of days between posting a preprint and subsequent journal publication for COVID-19 preprints (red), non-COVID-19 preprints posted during the same period (January to October 2020) (green), and non-COVID-19 preprints posted between January and December 2019 (grey). (D) Time from posting on bioRxiv or medRxiv to publication categorised by publisher. Shown are the top 10 publishers by publication volume. Boxplot horizontal lines denote lower quartile, median, upper quartile, with whiskers extending to 1.5*IQR. All boxplots additionally show raw data values for individual preprints with added horizontal jitter for visibility. The data underlying this figure may be found in https://github.com/preprinting-a-pandemic/pandemic_preprints and https://zenodo.org/record/4587214#.YEN22Hmnx9A . COVID-19, Coronavirus Disease 2019.
https://doi.org/10.1371/journal.pbio.3000959.g004
Extensive access of preprint servers for COVID-19 research
At the start of our time window, COVID-19 preprints received abstract views at a rate over 18 times that of non-COVID-19 preprints ( Fig 5A ) (time-adjusted negative binomial regression; rate ratio = 18.2, z = 125.0, p < 0.001) and downloads at a rate of almost 30 times ( Fig 5B ) (rate ratio = 27.1, z = 124.2, p < 0.001). Preprints posted later displayed lower usage rates, in part due to the reduced length of time they were online and able to accrue views and downloads. However, decreases in both views and downloads by posting date was stronger for COVID-19 preprints versus non-COVID-19 preprints (preprint type*calendar day interaction terms, both p < 0.001); each additional calendar month in posting date resulted in an estimated 24.3%/7.4% reduction in rate of views and an estimated 28.5%/12.0% reduction in rate of downloads for COVID-19/non-COVID-19 preprints, respectively. Similar trends of decrease were observed when restricting view and download data to the first respective month of each preprint, with highest rates of usage for those posted in January ( S3A and S3B Fig ). The disparity between COVID-19 and non-COVID-19 preprints suggests that either COVID-19 preprints continued to slowly accumulate total usage well beyond their first month online ( Fig 5 ) and/or they received a more diluted share of relative initial interest as larger volumes of preprints (and publications) were available by later months ( Fig 1B ).
(A) Boxplots of abstract views, binned by preprint posting month. (B) Boxplots of PDF downloads, binned by preprint posting month. Boxplot horizontal lines denote lower quartile, median, upper quartile, with whiskers extending to 1.5*IQR. All boxplots additionally show raw data values for individual preprints with added horizontal jitter for visibility. The data underlying this figure may be found in https://github.com/preprinting-a-pandemic/pandemic_preprints and https://zenodo.org/record/4587214#.YEN22Hmnx9A . COVID-19, Coronavirus Disease 2019.
https://doi.org/10.1371/journal.pbio.3000959.g005
To confirm that usage of COVID-19 and non-COVID-19 preprints was not an artefact of differing preprint server reliance during the pandemic, we compared usage rates during the pandemic period with those from the previous year (January to December 2019), as a non-pandemic control period. Beyond the expected effect of fewer views/downloads of preprints that have been uploaded for a shorter time, the usage data did not differ from that prior to the pandemic ( S3C and S3D Fig ).
Secondly, we investigated usage across additional preprint servers (data kindly provided by each of the server operators). We found that COVID-19 preprints were consistently downloaded more than non-COVID-19 preprints during our time frame, regardless of which preprint server hosted the manuscript ( S3E Fig ), although the gap in downloads varied between server (2-way ANOVA, interaction term; F 3,89990 = 126.6, p < 0.001). Server usage differences were more pronounced for COVID-19 preprints; multiple post hoc comparisons confirmed that bioRxiv and medRxiv received significantly higher usage per COVID-19 preprint than all other servers for which data were available (Tukey HSD; all p values < 0.001). However, for non-COVID-19 preprints, the only observed pairwise differences between servers indicated greater bioRxiv and medRxiv usage than Research Square (Tukey HSD; p < 0.001). This suggests that specific attention has been given disproportionately to bioRxiv and medRxiv as repositories for COVID-19 research.
COVID-19 preprints were shared and cited more widely than non-COVID-19 preprints
We quantified the citation and online sharing behaviour of COVID-19 preprints using citation count data from Dimensions ( https://dimensions.ai ) and counts of various altmetric indicators using data from Altmetric ( https://altmetric.com ) ( Fig 6 ; further details on data sources in Methods section). In terms of citations, we found higher proportions overall of COVID-19 preprints that received at least a single citation (57.9%) than non-COVID-19 preprints (21.5%) during our study period of January 1 to October 31, 2020, although the citation coverage expectedly decreased for both groups for newer posted preprints ( Fig 6A ). COVID-19 preprints also have greater total citation counts than non-COVID-19 preprints (time-adjusted negative binomial regression; rate ratio = 13.7, z = 116.3, p < 0.001). The highest cited COVID-19 preprint had 652 citations, with the 10th most cited COVID-19 preprint receiving 277 citations ( Table 1 ); many of the highest cited preprints focussed on the viral cell receptor, angiotensin converting enzyme 2 (ACE2), or the epidemiology of COVID-19.
Panels (A)–(F) show the proportion of preprints receiving at least 1 citation or mention in a given source, with the exception of panel (B) which shows the proportion of preprints receiving at least 2 tweets (to account for the fact that each preprint is tweeted once automatically by the official bioRxiv/medRxiv Twitter accounts). The inset in each panel shows a boxplot comparing citations/mentions for all COVID-19 and non-COVID-19 preprints posted within our analysis period. Boxplot horizontal lines denote lower quartile, median, upper quartile, with whiskers extending to 1.5*IQR. All boxplots additionally show raw data values for individual preprints with added horizontal jitter for visibility. Data are plotted on a log-scale with +1 added to each count for visualisation. (G) Proportion of preprints included in reference lists of policy documents from 3 sources: the ECDC, UK POST, and WHO SB. (H) Spearman correlation matrix between indicators shown in panels (A)–(F), as well as abstract views and PDF downloads for COVID-19 preprints. (I) Spearman correlation matrix between indicators shown in panels (A)–(F), in addition to abstract views and PDF downloads for non-COVID-19 preprints. The data underlying this figure may be found in https://github.com/preprinting-a-pandemic/pandemic_preprints and https://zenodo.org/record/4587214#.YEN22Hmnx9A . COVID-19, Coronavirus Disease 2019; ECDC, European Centre for Disease Prevention and Control; UK POST, United Kingdom Parliamentary Office of Science and Technology; WHO SB, World Health Organization Scientific Briefs.
https://doi.org/10.1371/journal.pbio.3000959.g006
https://doi.org/10.1371/journal.pbio.3000959.t001
Sharing of preprints on Twitter may provide an indicator of the exposure of wider public audiences to preprints. COVID-19 preprints received greater Twitter coverage (98.9% received >1 tweet) than non-COVID-19 preprints (90.7%) (note that the threshold for Twitter coverage was set at 1 rather than 0, to account for automated tweets by the official bioRxiv and medRxiv Twitter accounts) and were tweeted at an overall greater rate than non-COVID-19 preprints (rate ratio = 7.6, z = 135.7, p < 0.001) ( Fig 6B ). The most tweeted non-COVID-19 preprint received 1,656 tweets, whereas 8 of the top 10 tweeted COVID-19 preprints were tweeted over 10,500 times each ( Table 2 ). Many of the top 10 tweeted COVID-19 preprints were related to transmission, reinfection, or seroprevalence. The most tweeted COVID-19 preprint (26,763 tweets) was a study investigating antibody seroprevalence in California [ 22 ]. The fourth most tweeted COVID-19 preprint was a widely criticised (and later withdrawn) study linking the SARS-CoV-2 spike protein to HIV-1 glycoproteins [ 23 ].
https://doi.org/10.1371/journal.pbio.3000959.t002
To better understand the discussion topics associated with highly tweeted preprints, we analysed the hashtags used in original tweets (i.e., excluding retweets) mentioning the top 100 most tweeted COVID-19 preprints ( S4A Fig ). In total, we collected 30,213 original tweets containing 11,789 hashtags; we filtered these hashtags for those occurring more than 5 times and removed a selection of generic or overused hashtags directly referring to the virus (e.g., “#coronavirus” and “#covid-19”), leaving a final set of 2,981 unique hashtags. While many of the top-used hashtags were direct, neutral references to the disease outbreak such as “#coronavirusoutbreak” and “#wuhan,” we also found a large proportion of politicised tweets using hashtags associated with conspirational ideologies (e.g., “#qanon,” “#wwg1wga,” an abbreviation of “Where We Go One, We Go All” a tag commonly used by QAnon supporters), xenophobia (e.g., “#chinazi”), or US-specific right-wing populism (e.g., “#maga”). Other hashtags also referred to topics directly associated with controversial preprints, e.g., “#hydroxychloroquine” and “#hiv,” both of which were major controversial topics associated with several of the top 10 most tweeted preprints.
As well as featuring heavily on social media, COVID-19 research has also pervaded print and online news media. In terms of coverage, 28.7% of COVID-19 preprints were featured in at least a single news article, compared to 1.0% of non-COVID-19 preprints ( Fig 6C ), and were used overall in news articles at a rate almost 100 times that of non-COVID-19 preprints (rate ratio = 92.8, z = 83.3, p < 0.001). The top non-COVID-19 preprint was reported in 113 news articles, whereas the top COVID-19 preprints were reported in over 400 news articles ( Table 3 ). Similarly, COVID-19 preprints were also used more in blogs (coverage COVID-19/non-COVID-19 preprints = 14.3%/9.1%, rate ratio = 3.73, z = 37.3, p < 0.001) and Wikipedia articles (coverage COVID-19/non-COVID-19 preprints = 0.7%/0.2%, rate ratio = 4.47, z = 7.893, p < 0.001) at significantly greater rates than non-COVID-19 preprints ( Fig 6D and 6E , Table 4 ). We noted that several of the most widely disseminated preprints that we classified as being non-COVID-19 related featured topics nonetheless relevant to generalised infectious disease research, such as human respiratory physiology and personal protective equipment.
https://doi.org/10.1371/journal.pbio.3000959.t003
https://doi.org/10.1371/journal.pbio.3000959.t004
A potential benefit of preprints is that they allow authors to receive an incorporate feedback from the wider community prior to journal publication. To investigate feedback and engagement with preprints, we quantified the number of comments received by preprints directly via the commenting system on the bioRxiv and medRxiv platforms. We found that non-COVID-19 preprints were commented upon less frequently compared to COVID-19 preprints (coverage COVID-19/non-COVID-19 preprints = 15.9%/3.1%, time-adjusted negative binomial regression; rate ratio = 11.0, z = 46.5, p < 0.001) ( Fig 6F ); the most commented non-COVID-19 preprint received only 68 comments, whereas the most commented COVID-19 preprint had over 580 comments ( Table 5 ). One preprint, which had 129 comments, was retracted within 3 days of being posted following intense public scrutiny ( Table 4 , doi: 10.1101/2020.01.30.927871 ). As the pandemic has progressed, fewer preprints were commented upon. Collectively, these data suggest that the most discussed or controversial COVID-19 preprints are rapidly and publicly scrutinised, with commenting systems being used for direct feedback and discussion of preprints.
https://doi.org/10.1371/journal.pbio.3000959.t005
Within a set of 81 COVID-19 policy documents (which were manually retrieved from the European Centre for Disease Prevention and Control (ECDC), United Kingdom Parliamentary Office of Science and Technology (UK POST), and World Health Organization Scientific Briefs (WHO SB)), 52 documents cited preprints ( Fig 6G ). However, these citations occurred at a relatively low frequency, typically constituting less than 20% of the total citations in these 52 documents. Among 255 instances of citation to a preprint, medRxiv was the dominant server cited ( n = 209, 82%), with bioRxiv receiving a small number of citations ( n = 21) and 5 other servers receiving ≤10 citations each (arXiv, OSF, preprints.org , Research Square, and SSRN). In comparison, only 16 instances of citations to preprints were observed among 38 manually collected non-COVID-19 policy documents from the same sources.
To understand how different usage and sharing indicators may represent the behaviour of different user groups, we calculated the Spearman correlation between the indicators presented above (citations, tweets, news articles, blog mentions, Wikipedia citations, and comment counts) as well as with abstract views and download counts as previously presented ( Fig 6H and 6I ). Overall, we found stronger correlations between all indicators for COVID-19 preprints compared to non-COVID-19 preprints. For COVID-19 preprints, we found expectedly strong correlation between abstract views and PDF downloads (Spearman ρ = 0.91, p < 0.001), weak to moderate correlation between the numbers of citations and Twitter shares (Spearman ρ = 0.48, p < 0.001), and the numbers of citations and news articles (Spearman ρ = 0.33, p < 0.001) suggesting that the preprints cited extensively within the scientific literature did not necessarily correlate with those that were mostly shared by the wider public on online platforms. There was a slightly stronger correlation between COVID-19 preprints that were most blogged and those receiving the most attention in the news (Spearman ρ = 0.54, p < 0.001) and moderate correlation between COVID-19 preprints that were most tweeted and those receiving the most attention in the news (Spearman ρ = 0.51, p < 0.001), suggesting similarity between preprints shared on social media and in news media. Finally, there was a weak correlation between the number of tweets and number of comments received by COVID-19 preprints (Spearman ρ = 0.36, p < 0.001). Taking the top 10 COVID-19 preprints by each indicator, there was substantial overlap between all indicators except citations ( S4B Fig ).
In summary, our data reveal that COVID-19 preprints received a significant amount of attention from scientists, news organizations, the general public, and policy-making bodies, representing a departure for how preprints are normally shared (considering observed patterns for non-COVID-19 preprints).
The usage of preprint servers within the biological sciences has been rising since the inception of bioRxiv and other platforms [ 10 , 25 ]. The urgent threat of a global pandemic has catapulted the use of preprint servers as a means of quickly disseminating scientific findings into the public sphere, supported by funding bodies encouraging preprinting for COVID-19 research [ 26 , 27 ]. Our results show that preprints have been widely adopted for the dissemination and communication of COVID-19 research, and in turn, the pandemic has greatly impacted the preprint and science publishing landscape [ 28 ].
Changing attitudes and acceptance within the life sciences to preprint servers may be one reason why COVID-19 research is being shared more readily as preprints compared to previous epidemics. In addition, the need to rapidly communicate findings prior to a lengthy review process might be responsible for this observation ( Fig 3 ). A recent study involving qualitative interviews of multiple research stakeholders found “early and rapid dissemination” to be among the most often cited benefits of preprints [ 16 ]. These findings were echoed in a survey of approximately 4,200 bioRxiv users [ 10 ] and are underscored by the 6-month median lag between posting of a preprint and subsequent journal publication [ 8 , 16 ]. Such timelines for disseminating findings are clearly incompatible with the lightning-quick progression of a pandemic. An analysis of publication timelines for 14 medical journals has shown that some publishers have taken steps to accelerate their publishing processes for COVID-19 research, reducing the time for the peer-review stage (submission to acceptance) on average by 45 days and the editing stage (acceptance to publication) by 14 days [ 29 ], yet this still falls some way short of the approximately 1 to 3 days screening time for bioRxiv and medRxiv preprints ( Fig 2B ). This advantage may influence the dynamics of preprint uptake: As researchers in a given field begin to preprint, their colleagues may feel pressure to also preprint in order to avoid being scooped. Further studies on understanding the motivations behind posting preprints, for example, through quantitative and qualitative author surveys, may help funders and other stakeholders that support the usage of preprints to address some of the social barriers for their uptake [ 30 ].
One of the primary concerns among authors around posting preprints is premature media coverage [ 16 , 31 ]. Many preprint servers created highly visible collections of COVID-19 work, potentially amplifying its visibility. From mid-March 2020, bioRxiv and medRxiv included a banner to explain that preprints should not be regarded as conclusive and not reported on in the news media as established information [ 32 ]. Despite this warning message, COVID-19 preprints have received unprecedented coverage on online media platforms ( Fig 6 ). Indeed, even before this warning message was posted, preprints were receiving significant amounts of attention. Twitter has been a particularly notable outlet for communication of preprints, a finding echoed by a recent study on the spread of the wider (i.e., not limited to preprints) COVID-19 research field on Twitter, which found that COVID-19 research was being widely disseminated and driven largely by academic Twitter users [ 33 , 34 ]. Nonetheless, the relatively weak correlation found between citations and other indicators of online sharing ( Fig 6H ) suggests that the interests of scientists versus the broader public differ significantly: Of the articles in the top 10 most shared on Twitter, in news articles or on blogs, only one is ranked among the top 10 most cited articles ( S4B Fig ). Hashtags associated with individual, highly tweeted preprints reveal some emergent themes that suggest communication of certain preprints can also extend well beyond scientific audiences ( S4A Fig ) [ 34 ]. These range from good public health practice (“#washyourhands”) to right-wing philosophies (#chinalies), conspiracy theories (“#fakenews” and “#endthelockdown”), and xenophobia (“#chinazi”). Many of the negative hashtags have been perpetuated by public figures such as the former President of America and the right-wing media [ 35 , 36 ]. Following President Trump’s diagnosis of COVID-19, one investigation found a wave of anti-Asian sentiment and conspiracy theories across Twitter [ 37 ]. This type of misinformation is common to new diseases, and social media platforms have recently released a statement outlining their plans to combat this issue [ 38 ]. An even greater adoption of open science principles has recently been suggested as one method to counter the misuse of preprints and peer-reviewed articles [ 24 ]; this remains an increasingly important discourse.
The fact that news outlets are reporting extensively on COVID-19 preprints ( Fig 6C and 6D ) represents a marked change in journalistic practice: Pre-pandemic, bioRxiv preprints received very little coverage in comparison to journal articles [ 25 ]. This cultural shift provides an unprecedented opportunity to bridge the scientific and media communities to create a consensus on the reporting of preprints [ 21 , 39 ]. Another marked change was observed in the use of preprints in policy documents ( Fig 6G ). Preprints were remarkably underrepresented in non-COVID-19 policy documents yet present, albeit at relatively low levels, in COVID-19 policy documents. In a larger dataset, two of the top 10 journals which are being cited in policy documents were found to be preprint servers (medRxiv and SSRN in fifth and eighth position, respectively) [ 40 ]. This suggests that preprints are being used to directly influence policymakers and decision-making. We only investigated a limited set of policy documents, largely restricted to Europe; whether this extends more globally remains to be explored [ 41 ]. In the near future, we aim to examine the use of preprints in policy in more detail to address these questions.
As most COVID-19-preprints were not yet published, concerns regarding quality will persist [ 20 ]. This is partially addressed by prominent scientists using social media platforms such as Twitter to publicly share concerns about poor-quality COVID-19 preprints or to amplify high-quality preprints [ 42 ]. The use of Twitter to “peer-review” preprints provides additional public scrutiny of manuscripts that can complement the more opaque and slower traditional peer-review process. In addition to Twitter, the comments section of preprint servers can be used as a public forum for discussion and review. However, an analysis of all bioRxiv comments from September 2019 found a very limited number of peer-review style comments [ 43 ]. Despite increased publicity for established preprint review services (such as PREreview [ 44 , 45 ]), there has been a limited use of these platforms [ 46 ]. However, independent preprint review projects have arisen whereby reviews are posted in the comments section of preprint servers or hosted on independent websites [ 47 , 48 ]. These more formal projects partly account for the increased commenting on the most high-profile COVID-19 preprints ( Fig 4 ). Although these new review platforms partially combat poor-quality preprints, it is clear that there is a dire need to better understand the general quality and trustworthiness of preprints compared to peer-reviewed articles. Recent studies have suggested that the quality of reporting in preprints differs little from their later peer-reviewed articles [ 49 ], and we ourselves are currently undertaking a more detailed analysis. However, the problem of poor-quality science is not unique to preprints and ultimately, a multipronged approach is required to solve some of these issues. For example, scientists must engage more responsibly with journalists and the public, in addition to upholding high standards when sharing research. More significant consequences for academic misconduct and the swift removal of problematic articles will be essential in aiding this. Moreover, the politicisation of public health research has become a polarising issue, and more must be done to combat this; scientific advice should be objective and supported by robust evidence. Media outlets and politicians should not use falsehoods or poor-quality science to further a personal agenda. Thirdly, transparency within the scientific process is essential in improving the understanding of its internal dynamics and providing accountability.
Our data demonstrate the indispensable role that preprints, and preprint servers, are playing during a global pandemic. By communicating science through preprints, we are sharing research at a faster rate and with greater transparency than allowed by the current journal infrastructure. Furthermore, we provide evidence for important future discussions around scientific publishing and the use of preprint servers.
Preprint metadata for bioRxiv and medRxiv
We retrieved basic preprint metadata (DOIs, titles, abstracts, author names, corresponding author name and institution, dates, versions, licenses, categories, and published article links) for bioRxiv and medRxiv preprints via the bioRxiv Application Programming Interface (API; https://api.biorxiv.org ). The API accepts a “server” parameter to enable retrieval of records for both bioRxiv and medRxiv. We initially collected metadata for all preprints posted from the time of the server’s launch, corresponding to November 2013 for bioRxiv and June 2019 for medRxiv, until the end of our analysis period on October 31, 2020 ( N = 114,214). Preprint metadata, and metadata related to their linked published articles, were collected in the first week of December 2020. Note that where multiple preprint versions existed, we included only the earliest version and recorded the total number of following revisions. Preprints were classified as “COVID-19 preprints” or “non-COVID-19 preprints” on the basis of the following terms contained within their titles or abstracts (case insensitive): “coronavirus,” “covid-19,” “sars-cov,” “ncov-2019,” “2019-ncov,” “hcov-19,” “sars-2.” For comparison of preprint behaviour between the COVID-19 outbreak and previous viral epidemics, namely Western Africa Ebola virus and Zika virus ( S1 Fig ), the same procedure was applied using the keywords “ebola” or “zebov” and “zika” or “zikv,” respectively.
For a subset of preprints posted between September 1, 2019 and April 30, 2020 ( N = 25,883), we enhanced the basic preprint metadata with data from a number of other sources, as outlined below. Note that this time period was chosen to encapsulate a 10-month analysis period from January 1 to October 31, 2020, in which we make comparative analysis between COVID-19 and non-COVID-19–related preprints, ( N = 44,503), as well as the preceding year from January 1 to December 31, 2019 ( N = 30,094), to use as a pre-COVID-19 control group. Of the preprints contained in the 10-month analysis period, 10,232 (23.0%) contained COVID-19–related keywords in their titles or abstracts.
For all preprints contained in the subset, disambiguated author affiliation and country data for corresponding authors were retrieved by querying raw affiliation strings against the Research Organisation Registry (ROR) API ( https://github.com/ror-community/ror-api ). The API provides a service for matching affiliation strings against institutions contained in the registry, on the basis of multiple matching types (named “phrase,” “common terms,” “fuzzy,” “heuristics,” and “acronyms”). The service returns a list of potential matched institutions and their country, as well as the matching type used, a confidence score with values between 0 and 1, and a binary “chosen” indicator relating to the most confidently matched institution. A small number (approximately 500) of raw affiliation strings returned from the bioRxiv API were truncated at 160 characters; for these records, we conducted web scraping using the rvest package for R [ 50 ] to retrieve the full affiliation strings of corresponding authors from the bioRxiv public web pages, prior to matching. For the purposes of our study, we aimed for higher precision than recall, and thus only included matched institutions where the API returned a confidence score of 1. A manual check of a sample of returned results also suggested higher precision for results returned using the “phrase” matching type, and thus we only retained results using this matching type. In a final step, we applied manual corrections to the country information for a small subset of records where false positives would be most likely to influence our results by (a) iteratively examining the chronologically first preprint associated with each country following affiliation matching and applying manual rules to correct mismatched institutions until no further errors were detected ( n = 8 institutions); and (b) examining the top 50 most common raw affiliation strings and applying manual rules to correct any mismatched or unmatched institutions ( n = 2 institutions). In total, we matched 54,289 preprints to a country (72.8%); for COVID-19 preprints alone, 6,692 preprints (65.4%) were matched to a country. Note that a similar, albeit more sophisticated method of matching bioRxiv affiliation information with the ROR API service was recently documented by Abdill and colleagues [ 51 ].
Word counts and reference counts for each preprint were also added to the basic preprint metadata via scraping of the bioRxiv public web pages (medRxiv currently does not display full HTML texts, and so calculating word and reference counts was limited to bioRxiv preprints). Web scraping was conducted using the rvest package for R [ 50 ]. Word counts refer to words contained only in the main body text, after removing the abstract, figure captions, table captions, acknowledgements, and references. In a small number of cases, word counts could not be retrieved because no full text existed; this occurs as we targeted only the first version of a preprint, but in cases where a second version was uploaded very shortly (i.e., within a few days) after the first version, the full-text article was generated only for the second version. Word and reference counts were retrieved for 61,397 of 61,866 bioRxiv preprints (99.2%); for COVID-19 preprints alone, word and reference counts were retrieved for 2,314 of 2,333 preprints (99.2%). Word counts ranged from 408 to 49,064 words, while reference counts ranged from 1 to 566 references.
Our basic preprint metadata retrieved from the bioRxiv API also contained DOI links to published versions (i.e., a peer-reviewed journal article) of preprints, where available. In total, 22,151 records in our preprint subset (29.7%) contained links to published articles, although of COVID-19 preprints, only 2,164 preprints contained such links (21.1%). It should be noted that COVID-19 articles are heavily weighted towards the most recent months of the dataset and have thus had less time to progress through the journal publication process. Links to published articles are likely an underestimate of the total proportion of articles that have been subsequently published in journals—both as a result of the delay between articles being published in a journal and being detected by bioRxiv and bioRxiv missing some links to published articles when, e.g., titles change significantly between the preprint and published version [ 25 ]. Published article metadata (titles, abstracts, publication dates, journal, and publisher name) were retrieved by querying each DOI against the Crossref API ( https://api.crossref.org ), using the rcrossref package for R [ 52 ]. With respect to publication dates, we use the Crossref “created” field which represent the date on which metadata was first deposited and has been suggested as a good proxy of the first online availability of an article [ 53 , 54 ]. When calculating delay from preprint posting to publication dates, erroneous negative values (i.e., preprints posted after published versions) were ignored. We also retrieved data regarding the open access status of each article by querying each DOI against the Unpaywall API ( https://unpaywall.org/products/api ) via the roadoi package for R [ 55 ].
Usage, altmetrics, and citation data
For investigating the rates at which preprints are used, shared, and cited, we collected detailed usage, altmetrics, and citation data for all bioRxiv and medRxiv preprints posted between January 1, 2019 and October 31, 2020 (i.e., for every preprint where we collected detailed metadata, as described in the previous section). All usage, altmetrics, and citation data were collected in the first week of December 2020.
Usage data (abstract views and PDF downloads) were scraped from the bioRxiv and medRxiv public web pages using the rvest package for R [ 50 ]. bioRxiv and medRxiv web pages display abstract views and PDF downloads on a calendar month basis; for subsequent analysis (e.g., Fig 4 ), these were summed to generate total abstract views and downloads since the time of preprint posting. In total, usage data were recorded for 74,461 preprints (99.8%)—a small number were not recorded, possibly due to server issues during the web scraping process. Note that bioRxiv web pages also display counts of full-text views, although we did not include these data in our final analysis. This was partially to ensure consistency with medRxiv, which currently does not provide display full HTML texts, and partially due to ambiguities in the timeline of full-text publishing—the full text of a preprint is added several days after the preprint is first available, but the exact delay appears to vary from preprint to preprint. We also compared rates of PDF downloads for bioRxiv and medRxiv preprints with other preprint servers (SSRN and Research Square) ( S3C Fig )—these data were provided directly by representatives of each of the respective preprint servers.
Counts of multiple altmetric indicators (mentions in tweets, blogs, and news articles) were retrieved via Altmetric ( https://www.altmetric.com ), a service that monitors and aggregates mentions to scientific articles on various online platforms. Altmetric provide a free API ( https://api.altmetric.com ) against which we queried each preprint DOI in our analysis set. Importantly, Altmetric only contains records where an article has been mentioned in at least one of the sources tracked; thus, if our query returned an invalid response, we recorded counts for all indicators as 0. Coverage of each indicator (i.e., the proportion of preprints receiving at least a single mention in a particular source) for preprints were 99.3%, 10.3%, 7.4%, and 0.33 for mentions in tweets, blogs news, and Wikipedia articles, respectively. The high coverage on Twitter is likely driven, at least in part, by automated tweeting of preprints by the official bioRxiv and medRxiv Twitter accounts. For COVID-19 preprints, coverage was found to be 99.99%, 14.3%, 28.7%, and 0.76% for mentions in tweets, blogs, news, and Wikipedia articles, respectively.
To quantitatively capture how high-usage preprints were being received by Twitter users, we retrieved all tweets linking to the top 10 most-tweeted preprints. Tweet IDs were retrieved via the Altmetric API service and then queried against the Twitter API using the rtweet package [ 56 ] for R, to retrieve full tweet content.
Citations counts for each preprint were retrieved from the scholarly indexing database Dimensions ( https://dimensions.ai ). An advantage of using Dimensions in comparison to more traditional citation databases (e.g., Scopus, Web of Science) is that Dimensions also includes preprints from several sources within their database (including from bioRxiv and medRxiv), as well as their respective citation counts. When a preprint was not found, we recorded its citation counts as 0. Of all preprints, 13,298 (29.9%) recorded at least a single citation in Dimensions. For COVID-19 preprints, 5,294 preprints (57.9%) recorded at least a single citation.
bioRxiv and medRxiv html pages feature a Disqus ( https://disqus.com ) comment platform to allow readers to post text comments. Comment counts for each bioRxiv and medRxiv preprint were retrieved via the Disqus API service ( https://disqus.com/api/docs/ ). Where multiple preprint versions existed, comments were aggregated over all versions. Text content of comments for COVID-19 preprints were provided directly by the bioRxiv development team.
Screening time for bioRxiv and medRxiv
To calculate screening time, we followed the method outlined by Steve Royle [ 57 ]. In short, we calculate the screening time as the difference in days between the preprint posting date and the date stamp of submission approval contained within bioRxiv and medRxiv DOIs (only available for preprints posted after December 11, 2019). bioRxiv and medRxiv preprints were filtered to preprints posted between January 1 and October 31, 2020, accounting for the first version of a posted preprint.
Policy documents
To describe the level of reliance upon preprints in policy documents, a set of policy documents were manually collected from the following institutional sources: the ECDC (including rapid reviews and technical reports), UK POST, and WHO SB ( n = 81 COVID-19–related policies, n = 38 non-COVID-19–related policies). COVID-19 policy documents were selected from January 1, 2020 to October 31, 2020. Due to the limited number of non-COVID-19 policy documents from the same time period, these documents were selected dating back to September 2018. Reference lists of each policy document were then text mined and manually verified to calculate the proportion of references that were preprints.
Journal article data
To compare posting rates of COVID-19 preprints against publication rates of articles published in scientific journals ( Fig 1B ), we extracted a dataset of COVID-19 journal articles from Dimensions ( https://www.dimensions.ai ), via the Dimensions Analytics API service. Journal articles were extracted based on presence of the following terms (case insensitive) in their titles or abstracts: “coronavirus,” “covid-19,” “sars-cov,” “ncov-2019,” “2019-ncov,” “hcov-19,” and “sars-2.” Data were extracted in the first week of December 2020 and covered the period January 1, 2020 to October 31, 2020. To ensure consistency of publication dates with our dataset of preprints, journal articles extracted from Dimensions were matched with records in Crossref on the basis of their DOIs (via the Crossref API using the rcrossref package for R [ 52 ]), and the Crossref “created” field was used as the publication date. The open access status of each article ( S1B Fig ) was subsequently determined by querying each DOI against the Unpaywall API via the roadoi package for R [ 55 ].
Statistical analyses
Preprint counts were compared across categories (e.g., COVID-19 or non-COVID-19) using chi-squared tests. Quantitative preprint metrics (e.g., word count and comment count) were compared across categories using Mann–Whitney tests and correlated with other quantitative metrics using Spearman rank tests for univariate comparisons.
For time-variant metrics (e.g., views, downloads, which may be expected to vary with length of preprint availability), we analysed the difference between COVID-19 and non-COVID-19 preprints using generalised linear regression models with calendar days since January 1, 2020 as an additional covariate and negative binomially distributed errors. This allowed estimates of time-adjusted rate ratios comparing COVID-19 and non-COVID-19 preprint metrics. Negative binomial regressions were constructed using the function “glm.nb” in R package MASS [ 58 ]. For multivariate categorical comparisons of preprint metrics (e.g., screening time between preprint type and preprint server or publication delay between preprint type and publisher for top 10 publishers), we constructed 2-way factorial ANOVAs, testing for interactions between both category variables in all cases. Pairwise post hoc comparisons of interest were tested using Tukey HSD while correcting for multiple testing, using function “glht” while setting multiple comparisons to “Tukey” in R package multcomp [ 53 ].
Parameters and limitations of this study
We acknowledge a number of limitations in our study. Firstly, to assign a preprint as COVID-19 or not, we used keyword matching to titles/abstracts on the preprint version at the time of our data extraction. This means we may have captured some early preprints, posted before the pandemic that had been subtly revised to include a keyword relating to COVID-19. Our data collection period was a tightly defined window (January to October 2020) which may impact upon the altmetric and usage data we collected as those preprints posted at the end of October would have had less time to accrue these metrics.
Supporting information
S1 fig. preprints represent a higher proportion of the pandemic-related literature for covid-19 than previous pandemics, and most articles are open access..
(A) Total number of preprints posted on bioRxiv and medRxiv during multiple epidemics: Western Africa Ebola virus, Zika virus, and COVID-19. The number of preprints posted that were related to the epidemic and the number that were posted but not related to the epidemic in the same time period are shown. Periods of data collection for Western Africa Ebola virus (January 24, 2014 to June 9, 2016) and Zika virus (March 2, 2015 to November 18, 2016) correspond to the periods between the first official medical report and WHO end of Public Health Emergency of International Concern declaration. The period of data collection for COVID-19 refers to the analysis period used in this study, January 1, 2020 to October 31, 2020. (B) Comparison of COVID-19 journal article accessibility (open versus closed access) according to data provided by Unpaywall ( https://unpaywall.org ). The data underlying this figure may be found in https://github.com/preprinting-a-pandemic/pandemic_preprints and https://zenodo.org/record/4587214#.YEN22Hmnx9A . COVID-19, Coronavirus Disease 2019; WHO, World Health Organization.
https://doi.org/10.1371/journal.pbio.3000959.s001
S2 Fig. Properties of COVID-19 and non-COVID-19 preprints categorised by preprint server.
(A) Number of new preprints posted to bioRxiv versus medRxiv per month. (B) Preprint screening time in days for bioRxiv versus medRxiv. (C) Number of preprint versions posted to bioRxiv versus medRxiv. (D) License type chosen by authors for bioRxiv versus medRxiv. The data underlying this figure may be found in https://github.com/preprinting-a-pandemic/pandemic_preprints and https://zenodo.org/record/4587214#.YEN22Hmnx9A . COVID-19, Coronavirus Disease 2019.
https://doi.org/10.1371/journal.pbio.3000959.s002
S3 Fig. Additional access statistics for bioRxiv and medRxiv preprints.
(A) Boxplots of abstracts views received by COVID-19 and non-COVID-19 preprints in the same calendar month in which they were posted, binned by preprint posting month. (B) Boxplots of PDF downloads received by COVID-19 and non-COVID-19 preprints in the same calendar month in which they were posted, binned by preprint posting month. (C) Boxplots of total abstract views for non-COVID preprints between January 2019 and October 2020, binned by preprint posting month (D) Boxplots of total PDF downloads for for non-COVID preprints between January 2019 and October 2020, binned by preprint posting month. (E) Comparison of PDF downloads for COVID-19 and non-COVID-19 preprints across multiple preprint servers. Red shaded areas in (C) and (D) represent our analysis time period, concurrent with the COVID-19 pandemic. Boxplot horizontal lines denote lower quartile, median, upper quartile, with whiskers extending to 1.5*IQR. All boxplots additionally show raw data values for individual preprints with added horizontal jitter for visibility. The data underlying this figure may be found in https://github.com/preprinting-a-pandemic/pandemic_preprints and https://zenodo.org/record/4587214#.YEN22Hmnx9A . COVID-19, Coronavirus Disease 2019.
https://doi.org/10.1371/journal.pbio.3000959.s003
S4 Fig. Additional COVID-19 preprint usage data.
(A) Wordcloud of hashtags for the 100 most tweeted COVID-19 preprints. The size of the word reflects the hashtag frequency (larger = more frequent). Only hashtags used in at least 5 original tweets (excluding retweets) were included. Some common terms relating directly to COVID-19 were removed for visualisation (“covid19,” “coronavirus,” “ncov2019,” “covid,” “covid2019,” “sarscov2,” “2019ncov,” “hcov19,” “19,” “novelcoronavirus,” “corona,” “coronaovirus,” “coronarovirus,” and “coronarvirus”). (B) Euler diagram showing overlap between the 10 most tweeted COVID-19 preprints, the 10 most covered COVID-19 preprints in the news, the 10 most blogged about preprints, the 10 most commented-upon preprints, and the 10 most cited COVID-19 preprints. The data underlying this figure may be found in https://github.com/preprinting-a-pandemic/pandemic_preprints and https://zenodo.org/record/4587214#.YEN22Hmnx9A . COVID-19, Coronavirus Disease 2019.
https://doi.org/10.1371/journal.pbio.3000959.s004
S1 Table. Descriptive statistics for COVID-19 and non-COVID-19 preprints broken down by server.
COVID-19, Coronavirus Disease 2019.
https://doi.org/10.1371/journal.pbio.3000959.s005
S2 Table. Outputs from mixed-effects regression predicting word count using all bioRxiv preprints.
https://doi.org/10.1371/journal.pbio.3000959.s006
S3 Table. Outputs from mixed-effects regression predicting word count using only published bioRxiv preprints.
https://doi.org/10.1371/journal.pbio.3000959.s007
S4 Table. Statistics for first time or previous posting of preprints by senior authors based on country.
https://doi.org/10.1371/journal.pbio.3000959.s008
S1 Model. Mixed-effects regression models to investigate alternative factors on length of preprints.
https://doi.org/10.1371/journal.pbio.3000959.s009
Acknowledgments
The authors would like to thank Ted Roeder, John Inglis, and Richard Sever from bioRxiv and medRxiv for providing information relating to comments on Coronavirus Disease 2019 (COVID-19) preprints. We would also like to thank Martyn Rittman ( preprints.org ), Shirley Decker-Lucke (SSRN), and Michele Avissar-Whiting (Research Square) for kindly providing usage data. Further thanks to Helena Brown and Sarah Bunn for conversations regarding media usage and government policy.
- 1. WHO. COVID-19 Weekly Epidemiological Update-11. 2020 Oct. Available from: https://www.who.int/docs/default-source/coronaviruse/situation-reports/weekly-epi-update-11.pdf .
- View Article
- PubMed/NCBI
- Google Scholar
- 3. WHO. Coronavirus Disease (COVID-19) Weekly Epidemiological Update—24. 2021 Jan. Available from: https://www.who.int/docs/default-source/coronaviruse/situation-reports/20210127_weekly_epi_update_24.pdf
- 5. Ksiazek TG, Erdman D, Goldsmith CS, Zaki SR, Peret T, Emery S, et al. A Novel Coronavirus Associated with Severe Acute Respiratory Syndrome. In: http://dx.doi.org/10.1056/NEJMoa030781 [Internet]. Massachusetts Medical Society; 7 Oct 2009 [cited 13 May 2020]. https://doi.org/10.1056/NEJMoa030781 pmid:12690092
- 11. Kaiser J, 2014, Am 12:00. BioRxiv at 1 year: A promising start. In: Science | AAAS [Internet]. 11 Nov 2014 [cited 13 May 2020]. Available from: https://www.sciencemag.org/news/2014/11/biorxiv-1-year-promising-start
- 13. Wellcome Trust. Sharing data during Zika and other global health emergencies | Wellcome. In: Wellcomeacuk [Internet]. 10 Feb 2016 [cited 13 May 2020]. Available from: https://wellcome.ac.uk/news/sharing-data-during-zika-and-other-global-health-emergencies
- 18. ASAPbio. asapbio/licensing. ASAPbio; 2018. Available from: https://github.com/asapbio/licensing
- 26. Wellcome Trust. Coronavirus (COVID-19): sharing research data | Wellcome. 31 Jan 2020 [cited 21 May 2020]. Available from: https://wellcome.ac.uk/coronavirus-covid-19/open-data
- 27. Wellcome Trust. Publishers make coronavirus (COVID-19) content freely available and reusable | Wellcome. 16 Mar 2020 [cited 21 May 2020]. Available from: https://wellcome.ac.uk/press-release/publishers-make-coronavirus-covid-19-content-freely-available-and-reusable
- 31. ASAPbio. Preprint authors optimistic about benefits: preliminary results from the #bioPreprints2020 survey. In: ASAPbio [Internet]. 27 Jul 2020 [cited 1 Feb 2021]. Available from: https://asapbio.org/biopreprints2020-survey-initial-results
- 32. Inglis J. We’ve just put an additional, cautionary note about the use of preprints on every @biorxivpreprint https://t.co/08eSXL4dDi . In: Twitter [Internet]. 1 Feb 2020 [cited 22 May 2020]. Available from: https://twitter.com/johnringlis/status/1223598414493077505
- 33. Fang Z, Costas R. Tracking the Twitter attention around the research efforts on the COVID-19 pandemic. ArXiv200605783 Cs. 2020 [cited 16 Sep 2020]. Available from: http://arxiv.org/abs/2006.05783
- 37. Anti-Defamation League. At the Extremes: The 2020 Election and American Extremism | Part 3. In: At the Extremes: The 2020 Election and American Extremism | Part 3 [Internet]. 10 Aug 2020 [cited 27 Jan 2021]. Available from: https://www.adl.org/blog/at-the-extremes-the-2020-election-and-american-extremism-part-3
- 38. Lally C, Christie L. COVID-19 misinformation UK Parliam POST. 2020 [cited 21 May 2020]. Available from: https://post.parliament.uk/analysis/covid-19-misinformation/ , https://post.parliament.uk/analysis/covid-19-misinformation/
- 42. Markus A, Oransky I, Retraction Watch. Eye for Manipulation: A Profile of Elisabeth Bik. In: The Scientist Magazine® [Internet]. 7 May 2019 [cited 21 May 2020]. Available from: https://www.the-scientist.com/news-opinion/eye-for-manipulation—a-profile-of-elisabeth-bik-65839
- 44. OASPA. COVID-19 Publishers Open Letter of Intent—Rapid Review. In: OASPA [Internet]. 27 May 2020 [cited 13 May 2020]. Available from: https://oaspa.org/covid-19-publishers-open-letter-of-intent-rapid-review/
- 48. MIT Press. The MIT Press and UC Berkeley launch Rapid Reviews: COVID-19. In: MIT News | Massachusetts Institute of Technology [Internet]. 29 Jun 2020 [cited 13 Sep 2020]. Available from: https://news.mit.edu/2020/mit-press-and-uc-berkeley-launch-rapid-reviews-covid-19-0629
- 50. Wickham H, RStudio. rvest: Easily Harvest (Scrape) Web Pages. 2019. Available from: https://CRAN.R-project.org/package=rvest
- 52. Chamberlain S, Zhu H, Jahn N, Boettiger C, Ram K. rcrossref: Client for Various “CrossRef” “APIs” 2020. Available from: https://CRAN.R-project.org/package=rcrossref
- 54. Haustein S, Bowman TD, Costas R. When is an article actually published? An analysis of online availability, publication, and indexation dates. ArXiv150500796 Cs. 2015 [cited 22 Jan 2021]. Available from: http://arxiv.org/abs/1505.00796
- 55. Jahn N, rOpenSci TS roadoi: Find Free Versions of Scholarly Publications via Unpaywall. 2019. Available from: https://CRAN.R-project.org/package=roadoi
- 57. Royle Steve. Screenager: screening times at bioRxiv. In: quantixed [Internet]. 30 Mar 2020 [cited 22 May 2020]. Available from: https://quantixed.org/2020/03/30/screenager-screening-times-at-biorxiv/
- 58. Venables WN, Ripley BD. Modern Applied Statistics with S. 4th ed. New York: Springer-Verlag; 2002. https://doi.org/10.1007/978-0-387-21706-2
We use cookies on this site to enhance your experience
By clicking any link on this page you are giving your consent for us to set cookies.
A link to reset your password has been sent to your email.
Back to login
We need additional information from you. Please complete your profile first before placing your order.
Thank you. payment completed., you will receive an email from us to confirm your registration, please click the link in the email to activate your account., there was error during payment, orcid profile found in public registry, download history, is it safe to publish preprints on researchgate.
- Charlesworth Author Services
- 25 May, 2021
ResearchGate is a professional networking site for research scientists that enables researchers to connect with one another professionally, and allows them to share and promote their work. In addition to facilitating communication between research scientists, this site also enable scientists to track their publication statistics and metrics and share publications by posting them to the site. ResearchGate not only allows users to post published works (which are not copyright-protected by the journal that originally published them), it also allows users to upload preprints , or author’s versions, much like a standard preprint server .
What are the advantages of publishing a preprint on ResearchGate?
Like other preprint servers, ResearchGate highlights the advantages of publishing a preprint prior to submitting your paper to a traditional journal for peer review, specifically citing early feedback, early citation of your work and the potential to attract a wider readership. By posting your work prior to peer review, you can receive advice and comments on your paper that may help you improve your paper before submission, potentially making the peer review process go smoother and more quickly. Having your work read and possibly cited prior to peer review can help increase the visibility of the research and establish your claim to a novel finding without the delay of the traditional review process. And promoting a preprint within your ResearchGate network can bring the paper to the attention of readers who might otherwise have overlooked it; indeed, publishing a preprint could even help build anticipation for the later, peer-reviewed version of the article.
What are the disadvantages of publishing a preprint on ResearchGate?
The concerns you may have about publishing a preprint on ResearchGate are likely to be the same that you have for other preprint server platforms.
A common fear shared by research scientists is that posting a preprint opens you up to the risk of being ‘ scooped ’ by another group, who could potentially repeat your study and then publish it in a peer-reviewed journal before you are able to do so. However, preprints are considered a formal and permanent part of the scientific record, and in almost all cases receive a Digital Object Identifier (DOI). This means that they can be formally identified and cited as authentic scientific publications, establishing your claim to the work and data prior to publication in peer-reviewed journal. Many preprint servers assign DOIs automatically, but in the case of ResearchGate it is your responsibility to request the generation of a DOI for a preprint when you upload it, so be sure to do this to protect your research once it has been posted.
Another common concern that researchers have regarding preprint publication is how to handle citations and publication records once the definitive version is published in a peer-reviewed journal. It is important to remember that a preprint is a formal publication in its own right, so even if the article is eventually published by a traditional journal, the preprint remains publicly available on the server it was uploaded to as part of the permanent scientific record. Best practice is to cite your own preprint in the definitive article submitted to (and ultimately published in) a peer-reviewed journal, for maximum clarity. You should also be sure to link to the preprint from the definitive version, and vice versa, to make it easy to find and compare each version of the paper. While some preprint servers handle this linking process automatically, ResearchGate does not, so it is your responsibility as the author to update the ResearchGate preprint page to add a link to the published version when it is available.
If you have identified your preprint with a DOI and have been transparent about publishing your research as a preprint when it is time to submit to a traditional journal, then publishing a preprint on ResearchGate is just as safe as any other preprint server. As we have already discussed above, preprints are visible and documented parts of the scientific record, and as such can and should be cited accordingly. This means that you can’t be ‘scooped’ by anyone who reads your preprint and attempts to replicate the work.
As with other preprint servers, you should check that your target journal publishes articles that have already been published as a preprint; this is typically the case, but not always. Once the definitive/final version of your paper is published, you can manually update the ResearchGate record to link to the most recent version.
However, there is a unique aspect to ResearchGate that can trip some authors up . As ResearchGate is primarily a networking site, copyright regulations can be somewhat complex for some authors to navigate when it comes to sharing the definitive version of a paper that was previously published as a preprint on the site. As we mentioned earlier, it is best practice to update your preprint to link to the definitive version of the paper once it is published in a peer-reviewed journal. However, many authors are tempted to simply upload a PDF version of the final paper to their ResearchGate account instead of providing a link to the journal website.
This can cause problems with copyright , depending on which journal you published in and what their open access policy is. If the journal is fully open access and you as the author retain the copyright, then you are free to distribute it in any way you choose, including by posting it publicly to ResearchGate. However, if the journal retains the copyright to your paper, then uploading a public copy to ResearchGate would be a violation of copyright law, and you will most likely be required to take it down. This gets a little murky when you consider that you are allowed to share the final version of your paper with friends and colleagues through the ResearchGate site, in much the same way that you would be allowed to pass out hard copies of the paper within your department or at a conference, even if it is protected by journal copyright . The important point here is to distinguish between whether you are making the paper publicly available or sharing it with a select group.
ResearchGate provides considerable guidance on this topic to help authors make the right decision, and you are encouraged to read through this guidance thoroughly before updating your ResearchGate preprint with the final version of your paper. If you are still unsure, you may wish to consult a site like www.howcanishareit.com, which can help you understand the copyright regulations surrounding a specific publication. The easiest way to do this is to search the DOI of your preprint and/or the final version of the paper to see what avenues of publication and sharing are open to you.
Charlesworth Author Services , a trusted brand supporting the world’s leading academic publishers, institutions and authors since 1928.
To know more about our services, visit: Our Services
Visit our new Researcher Education Portal that offers articles and webinars covering all aspects of your research to publication journey! And sign up for our newsletter on the Portal to stay updated on all essential researcher knowledge and information!
Register now: Researcher Education Portal
Maximise your publication success with Charlesworth Author Services .
Share with your colleagues
Related articles.
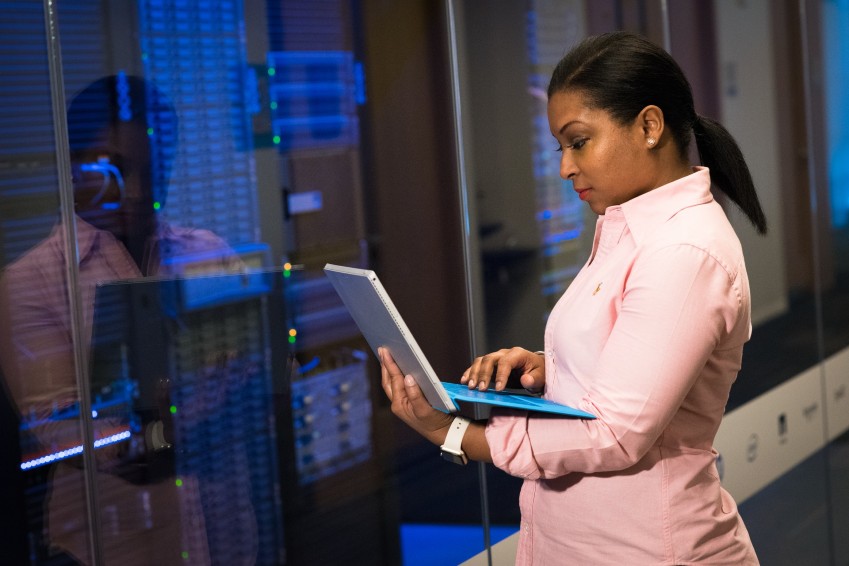
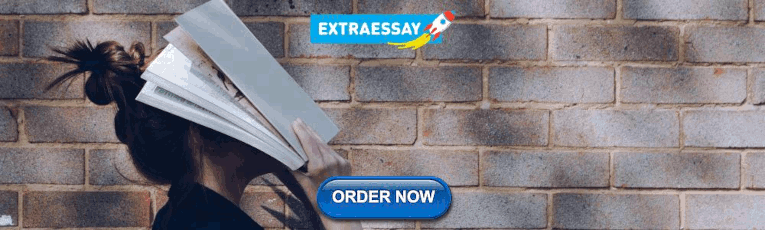
Introduction to Preprints
Charlesworth Author Services 28/01/2020 00:00:00
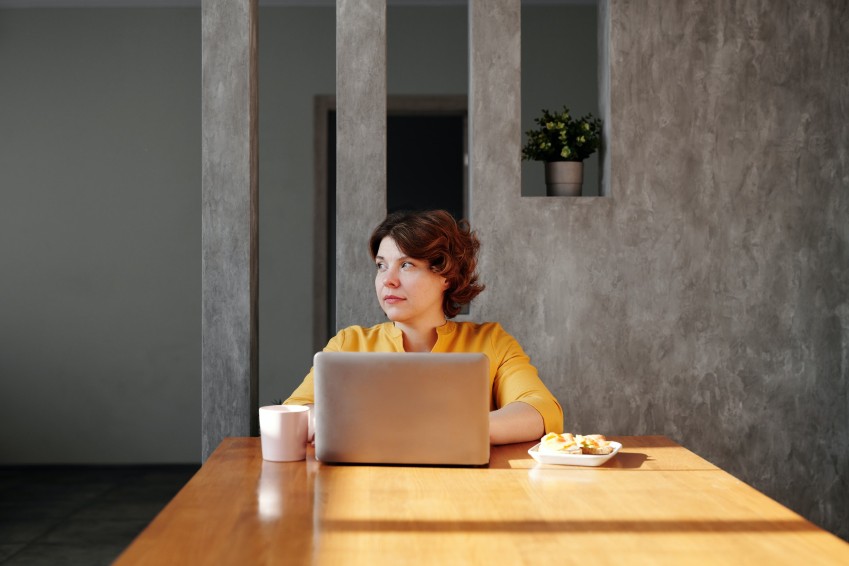
Advantages and disadvantages of releasing your paper as a preprint
Charlesworth Author Services 25/05/2021 00:00:00
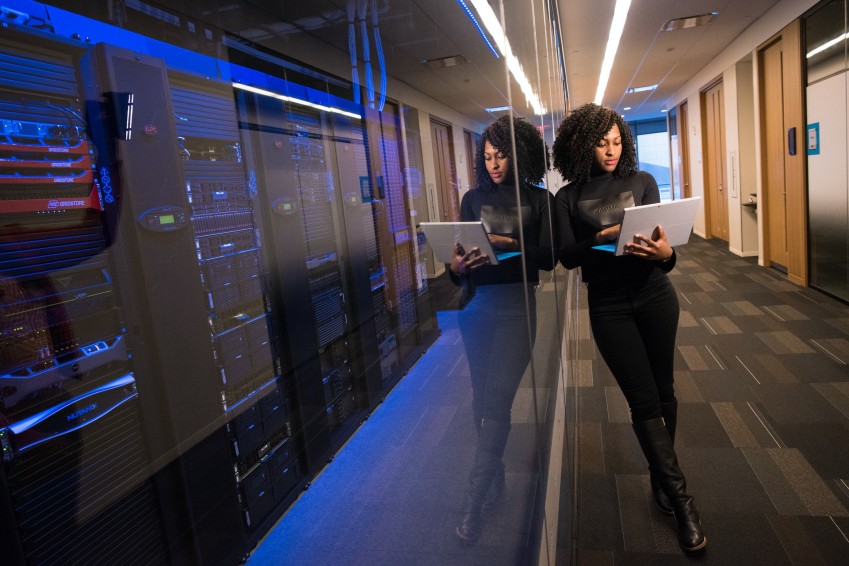
Does an article need to be removed from preprint if it is accepted by a journal?
Related webinars.

Bitesize Webinar: Understanding Open Access - Module 1 - Introduction to Open Access
Charlesworth Author Services 10/03/2021 00:00:00

Bitesize Webinar: Understanding Open Access - Module 2 - Open Access Publishing Models

Bitesize Webinar: Understanding Open Access - Module 3 - Benefits and Challenges of Open Access

Bitesize Webinar: Understanding Open Access - Module 4 - Copyright and Licensing under Open Access
Open access.
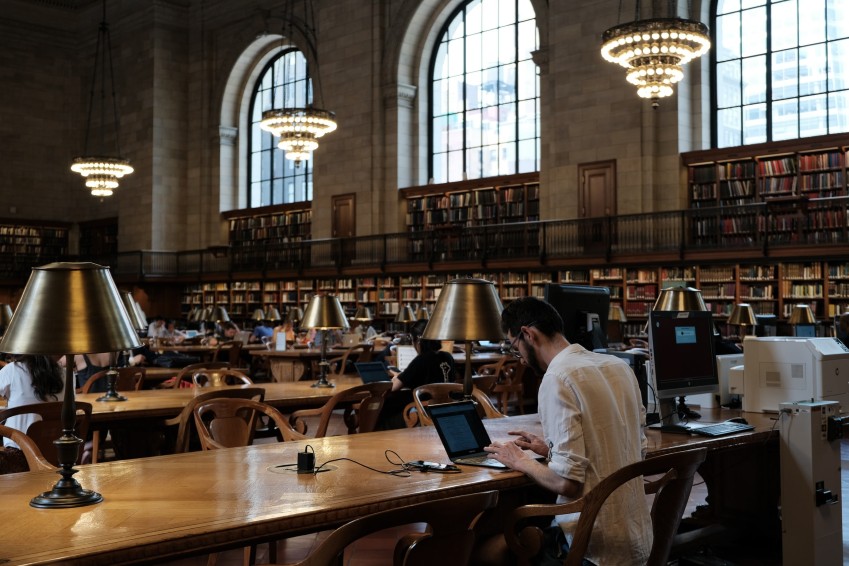
Introduction to Open Access journals
Charlesworth Author Services 07/02/2020 00:00:00
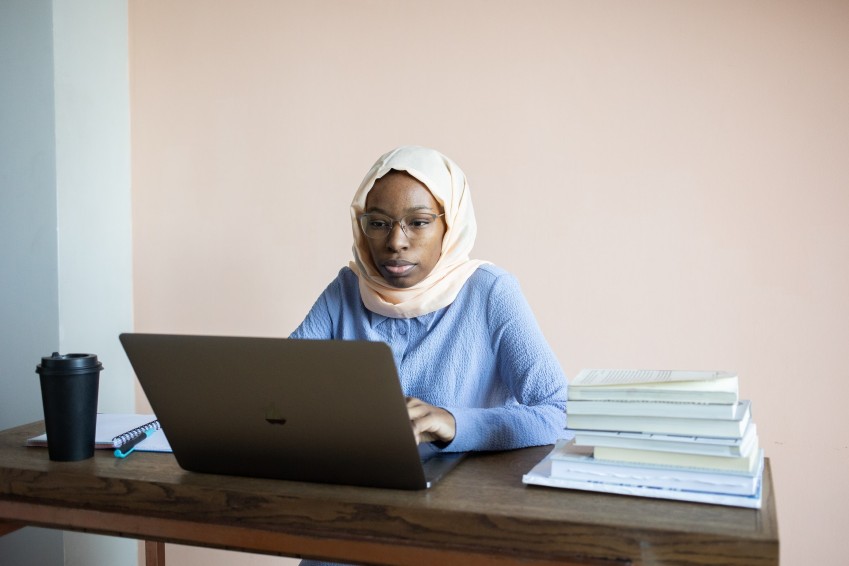
What to consider when choosing an open access outlet for your article
Charlesworth Author Services 20/10/2020 00:00:00
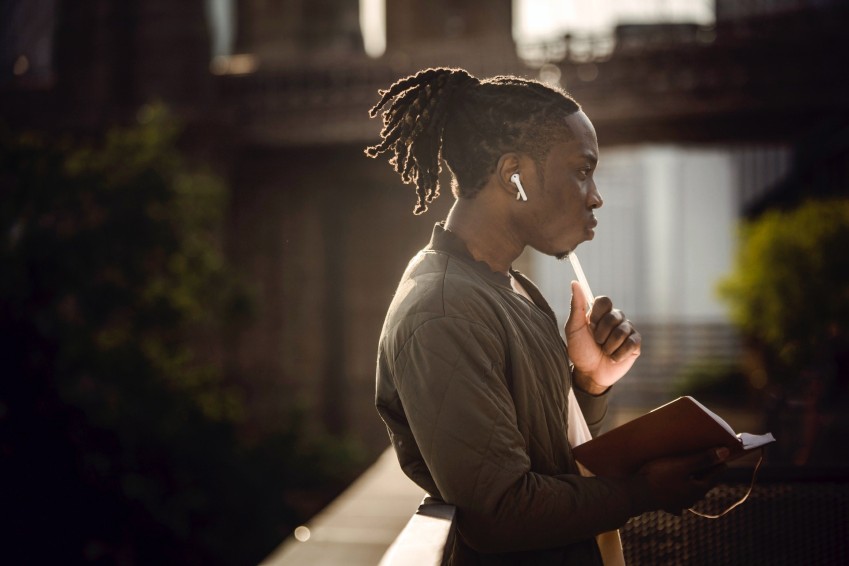
Deciding between the gold open access and the green open access publishing models
Charlesworth Author Services 24/10/2019 00:00:00

- Instructions for Authors
- Submit Log in/Register
The Multidisciplinary Preprint Platform
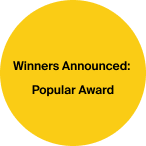
Preprints.org 2023 Most Popular Preprints Award Winner Collection
How it works, mdpi topics, friendly journals.
- Time saving
- Journal recommendations

- IEEE Xplore Digital Library
- IEEE Standards
- IEEE Spectrum

- Publications
RA-L Frequently Asked Questions
Is the page limit of 6 for the IEEE Robotics and Automation Letters (RA-L) strict?
Up to 2 extra pages beyond the 6-page limit are allowed for a fee of $175 per extra page. No appendices or other materials can extend beyond a total of 8 pages. Multimedia attachments cannot be used to circumvent the page limit; these are limited to materials described on the Information for Authors page .
Can a paper that was published in the Proceedings of an IEEE conference be resubmitted to RA-L?
Formally yes, if the paper follows the “evolutionary paradigm” of IEEE, i.e., if it incorporates substantial improvements and it discloses openly the source(s) and discusses the changes (these are the same conditions under which the same paper could be submitted to Transactions). However, RA-L are intended to publish novel results rapidly, whereas submission to Transactions of an evolved version of a previous conference paper is normal.
I submitted a paper to RA-L w/CO (with Conference Option) before the RAL w/CO submission deadline, and then a new version to the same Conference before the Conference submission deadline. Is that right?
No, this is unacceptable. When you submit to RA-L with a Conference option, you have already submitted to that Conference. A later submission to the Conference will void the previous submission, and stop the RA-L evaluation procedure.
I submitted a paper to RA-L without Conference option before, and then a new version to the Conference before the Conference submission deadline. Is that right?
No, this is unacceptable. In general, once you have submitted a manuscript to a journal (as RA-L is), you are not supposed to submit independently a version of the manuscript as a conference paper.
I have submitted a paper to RA-L with the Conference option. The paper has been accepted for presentation at Conference (ICRA or CASE), and accepted by RA-L. Where will my paper be published?
It will appear in RA-L on Xplore. It will not appear in the Conference Proceedings.
I have submitted a paper to RA-L with the Conference option. I have been requested a revision by the Letter's Editorial Board (LEB). Later on, the paper has been accepted for presentation at Conference (ICRA or CASE), but eventually rejected by LEB. Where will my paper be published?
It will only appear in the Conference Proceedings on Xplore.
I have a paper published in the Letters. Can I expand on it and submit to Transactions, the same way I used to do with Conference Proceedings papers?
Not “in the same way.” RA Letters are a journal, and IEEE does not accept to republish the same material on two journal publications. A new Transactions submission should have little overlap with a paper published in the Letters, and contain mainly novel material.
When submitting to a Conference, I am used to upload incrementally refined versions of my manuscript. Can I do the same with submissions to RA-L?
No. You should submit to RA-L only a stable final version of your manuscript, just like you do with other journals. Incremental updates are typically not allowed by journal manuscript management systems.
After appearing in IEEE Xplore, will RA-L issues be published also in print?
No, RA-L is an electronic-only publication.
Will the Letters be indexed in journal databases and will they have an Impact Factor?
Yes, RA-L will be indexed in the major journal databases, as for all IEEE publications. At this time, RA-L is indexed in Scopus and Clarivate's ESCI (Emerging Sources Citation Index). Since vol. 2, no. 1, 2017, RA-L has been indexed and abstracted in the following Clarivate Analytics services: Science Citation Index Expanded (also known as SciSearch), Journal Citation Reports/Science Edition, Current Contents®/Engineering Computing and Technology. RA-L received an impact factor in June 2020.
I would like my papers to be freely accessible to everybody. Can I do this with RA-L?
Yes. RA-Letters are Hybrid Open Access: authors can elect to make a paper freely accessible to everyone, if the author pays an open access fee. All RA-L papers are acessible free of charge to the authors and IEEE RAS members. Authors can also post pre-prints publicly, as explained in the FAQ "Can authors post their manuscript on a preprint server such as such as ArXiv or TechRxiv?".
Does a Revise and Resubmit decision 'restart' the 6 month clock or does the 6 month timeline include revisions for RR papers?
6 months is the sub to e-pub timing, i.e. include the time needed for a revision and resubmission. Whatever is not published in 6 months is rejected. Only submission of a new paper start the clock.
Will articles appear online in full html form, as is the case now for many IEEE journals?
Yes, as soon as IEEE provides the moderately edited version, this will replace the preprint camera-ready PDF.
Do RA-Letters have a minimum length of six pages or they could be less?
There is no minimum page length, such as there is no minimum page length in any RAS Journal. It is possible to submit very short Letters but - being in competition for publication with 6-8 pages long papers - it might be hard for such papers to get accepted.
Can authors post their manuscript on a preprint server such as such as ArXiv or TechRxiv?
Yes. The IEEE recognizes that many authors share their unpublished manuscripts on public sites. Authors are supposed to include a specific statement for preprints that this work has been submitted to IEEE. The statement “This work has been submitted to the IEEE for possible publication. Copyright may be transferred without notice, after which this version may no longer be accessible.” implies that this exact version has been submitted. Thus the submitted and arxiv version should be identical.
Once manuscripts have been accepted for publication by IEEE, an author is required to post an IEEE copyright notice on the preprint. Upon publication, the author must replace the preprint with either 1) the full citation to the IEEE work with Digital Object Identifiers (DOI) or a link to the paper’s abstract in IEEE Xplore, or 2) the accepted version only (not the IEEE-published version), including the IEEE copyright notice and full citation, with a link to the final, published paper in IEEE Xplore.
See details here: https://ieeeauthorcenter.ieee.org/publishing-ethics/guidelines-and-policies/policy-posting-your-article/
Similarly, videos and other multimedia attachments can be posted online e.g., through YouTube, in advance of publication. Once the video or multimedia material is published, the full citation to the IEEE materials must be provided along with the posted materials.
Is there a suggested format for citing papers that have been accepted for publication in both RAL and a Conference? How about citing them in an authors' CV?
All citations must go to the RA-L paper only: you want to avoid splitting your citations and, by so doing, hurt your h index etc. You will be able to download the exact citation from RA-L page on Xplore directly in most bibliography formats.
In your own CV, we recommend that you list a RA-L paper only among your journal papers. You may add a note to this citation such as e.g. "The contents of this paper were also selected by ICRA'16 Program Committee for presentation at the Conference". Alternatively, you may have an additional list of Oral Presentations at Conferences (distinct from the list of Papers in Conference Proceedings) where you list the talk as such, possibly adding details such as e.g. the acceptance rate of the Conference.
My paper was accepted to RA-L, but I want to wait to post the paper on IEEE Xplore until after I have patented the intellectual property contained in the paper. Will you wait to publish the paper online?
This situation should not occur. If there is intellectual property contained in the paper, authors should complete the patenting process either before they submit the paper or within two months of the initial submission. Given that RA-L guarantees online publication of accepted papers in 6 months, and many papers are published much sooner than that, authors should handle any intellectual property issues well ahead of time. Delaying publication of an accepted paper negatively affects RA-L's promised submission-to-publication timeline and results in additional work for our volunteers and staff.
How do I indicate equal contributions or co-first authorship for an RA-L paper?
Please see http://ieeeauthorcenter.ieee.org/wp-content/uploads/IEEE_Style_Manual.pdf, page 6, regarding the options for “Equally contributed authors” and “Co-first authors”.
I would like to include supplementary material with the publication of my RA-L paper becase the reviewers asked for more information than can fit into the 8-page limit. Is this allowed?
RA-L papers are limited to 6 pages, plus up to 2 additional pages with overlength page charges. RA-L is meant for relatively brief research papers, and if more space is needed, authors should consider submitting to a journal such as T-RO, T-ASE or RA-M, which publish longer papers. Even if the paper becomes longer in order to respond to reviewers' comments, authors are responsible for editing their paper such that it fits into 8 pages maximum. Multimedia materials (i.e. videos) are the only extra material allowed. Datasets can be posted online and cited by URL in the references. For more information on Datasets
What do I need to do in order to re-use all or part of an IEEE copyrighted paper (on which I am an author) in my thesis or dissertation?
The IEEE does not require individuals working on a thesis to obtain a formal reuse license. However, you must follow the citation requirements described here .
How do I volunteer to be an Associate Editor (AE) or Reviewer for RA-L?
What is the acceptance rate of RA-L?
The acceptance rate, calculated as the number of “Accept” decisions issued divided by the total number of decisions issued, was 41% in 2019 and 38% in 2021. Note: Because RA-L submissions are mostly associated with a conference option, where the RA-L deadline is earlier than the conference option and the RA-L reviews impact the conference decision as well, we believe authors tend to self-select to submit RA-L papers that are especially well-prepared and significant compared to the average conference submission.
What do I do if there are errors in my paper already published on IEEE Xplore?
If your manuscript is currently a pre-print, you can request corrections when you receive the galley proofs. If the proofs have already been approved and finalized, then the text of the article cannot be changed, but it is possible to publish an erratum if it is deemed appropriate after editorial review (see example here). Please reach out to the Editorial Assistant at [email protected].
- Subscription Information
- Video Submission Guidelines
- Information for Authors
- Submission Procedures
- Special Issues
- Final Submission Checklist
- Information for Reviewers
- Information for Associate Editors
- Information for Editors
- Information for Conference Organizers
- Editorial Board
- Steering Committee
- RA-L Paper Awards
- RA-L Distinguished Service
- List of Reviewers
- RA Magazine
- Tips for Making a Good Robot Video
- IEEE Author Center
- Plagiarism & Ethical Issues
- Young Reviewers Program
Students are future of robotics and automation.

IEEE International Conference on Automation Science and Engineering
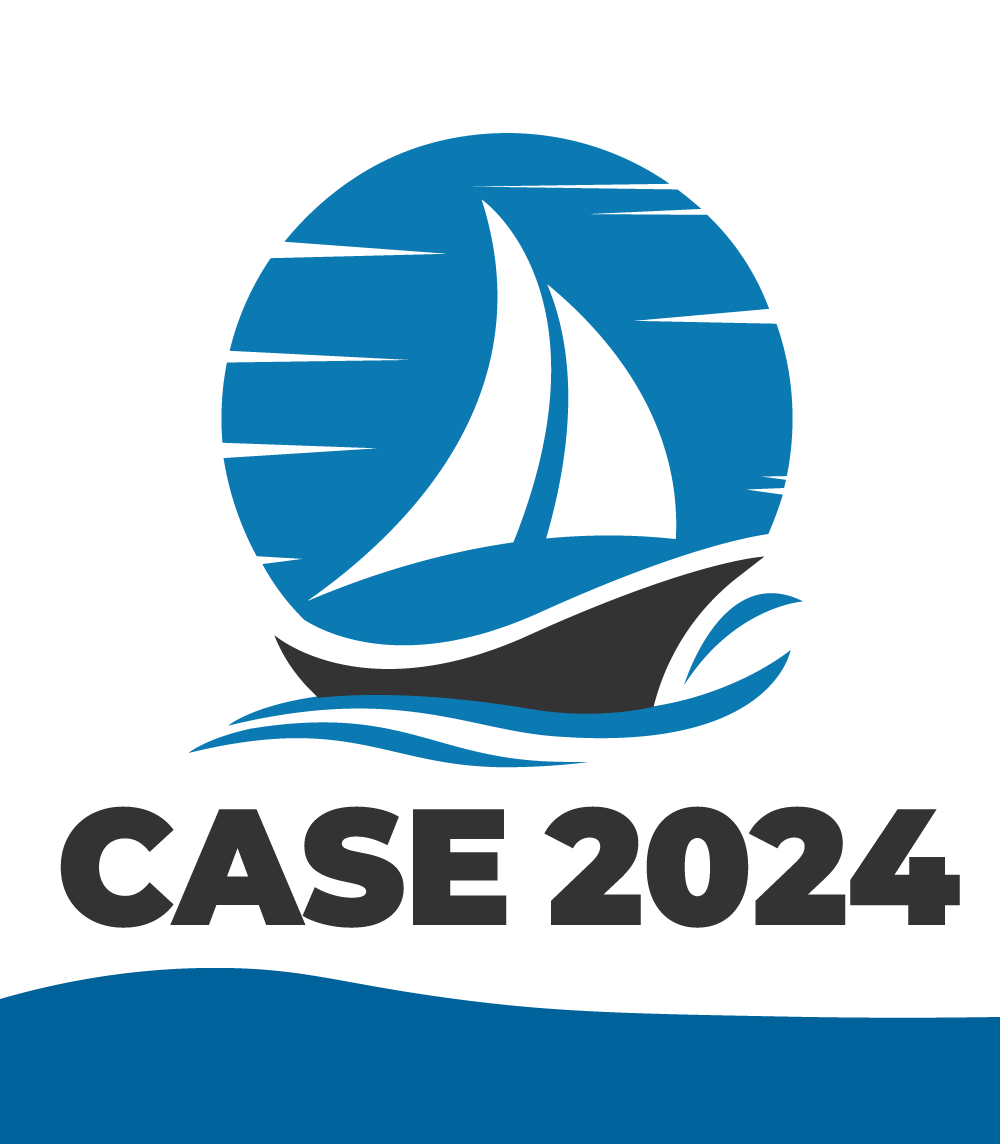
IEEE/RSJ International Conference on Intelligent Robots and Systems
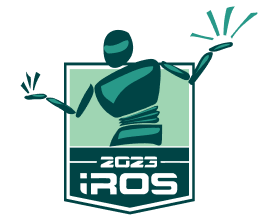
Special 40th anniversary celebration of RAS and ICRA
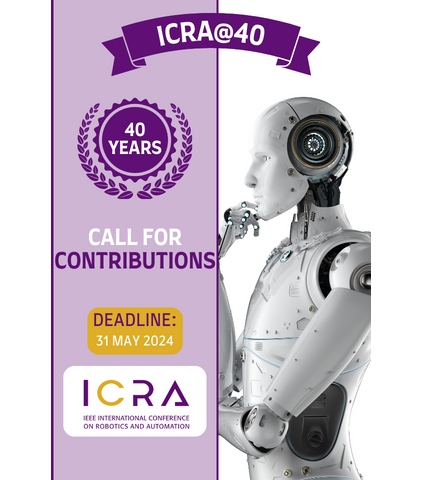
IEEE International conference on Robotics and Automation
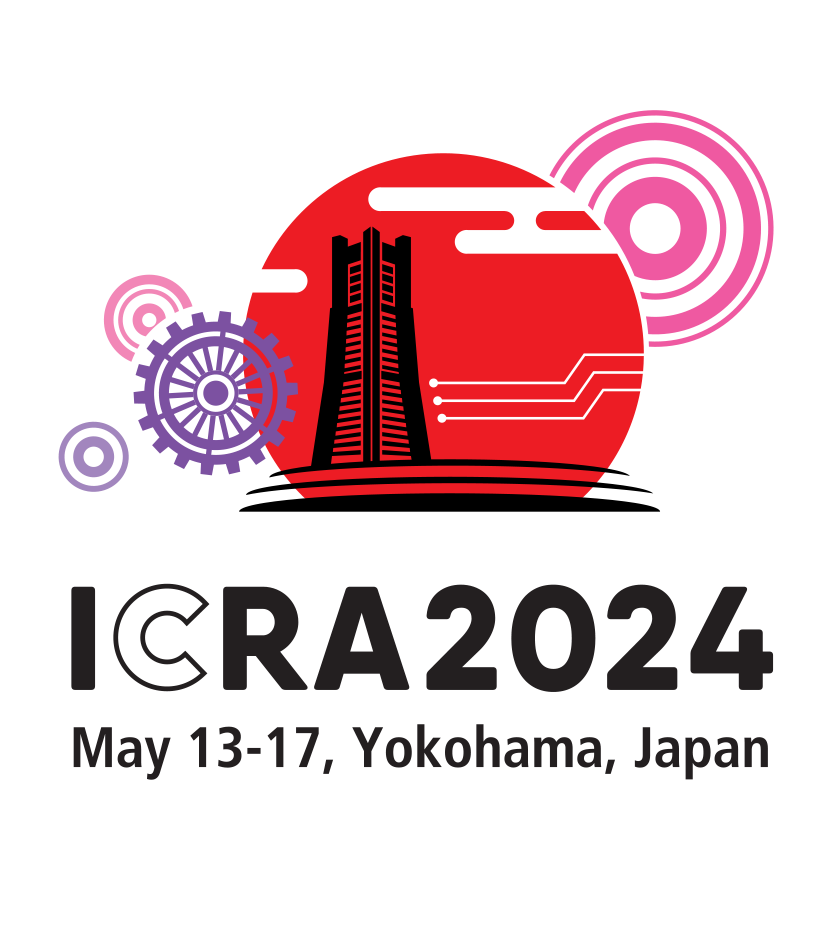
- Search Menu
- Advance articles
- AHFS First Release
- AJHP Voices
- AJHP Residents Edition
- Top Twenty-Five Articles
- ASHP National Surveys of Pharmacy Practice in Hospital Settings
- Medication Safety
- Pharmacy Technicians
- Specialty Pharmacy
- Emergency Preparedness and Clinician Well-being
- Author Guidelines
- Submission Site
- Open Access
- Information for Reviewers
- Self-Archiving Policy
- Author Instructions for Residents Edition
- Advertising and Corporate Services
- Advertising
- Reprints and ePrints
- Sponsored Supplements
- Editorial Board
- Permissions
- Journals on Oxford Academic
- Books on Oxford Academic
Article Contents
National trends in prescription drug expenditures and projections for 2024.
- Article contents
- Figures & tables
- Supplementary Data
Eric M Tichy, James M Hoffman, Mina Tadrous, Matthew H Rim, Sandra Cuellar, John S Clark, Mary Kate Newell, Glen T Schumock, National trends in prescription drug expenditures and projections for 2024, American Journal of Health-System Pharmacy , 2024;, zxae105, https://doi.org/10.1093/ajhp/zxae105
- Permissions Icon Permissions
In an effort to expedite the publication of articles, AJHP is posting manuscripts online as soon as possible after acceptance. Accepted manuscripts have been peer-reviewed and copyedited, but are posted online before technical formatting and author proofing. These manuscripts are not the final version of record and will be replaced with the final article (formatted per AJHP style and proofed by the authors) at a later time.
To report historical patterns of pharmaceutical expenditures, to identify factors that may influence future spending, and to predict growth in drug spending in 2024 in the United States, with a focus on the nonfederal hospital and clinic sectors.
Historical patterns were assessed by examining data on drug purchases from manufacturers using the IQVIA National Sales Perspectives database. Factors that may influence drug spending in hospitals and clinics in 2024 were reviewed—including new drug approvals, patent expirations, and potential new policies or legislation. Focused analyses were conducted for biosimilars, cancer drugs, endocrine drugs, generics, and specialty drugs. For nonfederal hospitals, clinics, and overall (all sectors), estimates of growth of pharmaceutical expenditures in 2024 were based on a combination of quantitative analyses and expert opinion.
In 2023, overall pharmaceutical expenditures in the US grew 13.6% compared to 2022, for a total of $722.5 billion. Utilization (a 6.5% increase), new drugs (a 4.2% increase) and price (a 2.9% increase) drove this increase. Semaglutide was the top drug in 2023, followed by adalimumab and apixaban. Drug expenditures were $37.1 billion (a 1.1% decrease) and $135.7 billion (a 15.0% increase) in nonfederal hospitals and clinics, respectively. In clinics, increased utilization drove growth, with a small impact from price and new products. In nonfederal hospitals, a drop in utilization led the decrease in expenditures, with price and new drugs modestly contributing to growth in spending. Several new drugs that will influence spending are expected to be approved in 2024. Specialty, endocrine, and cancer drugs will continue to drive expenditures.
For 2024, we expect overall prescription drug spending to rise by 10.0% to 12.0%, whereas in clinics and hospitals we anticipate an 11.0% to 13.0% increase and a 0% to 2.0% increase, respectively, compared to 2023. These national estimates of future pharmaceutical expenditure growth may not be representative of any health system because of the myriad of local factors that influence actual spending.
Supplementary data
Email alerts.
- Projected prescription drug expenditures in 2024: Looking ahead to the inflation reduction era
Citing articles via
- Recommend to Your Librarian
Affiliations
- Online ISSN 1535-2900
- Print ISSN 1079-2082
- Copyright © 2024 American Society of Health-System Pharmacists
- About Oxford Academic
- Publish journals with us
- University press partners
- What we publish
- New features
- Open access
- Institutional account management
- Rights and permissions
- Get help with access
- Accessibility
- Media enquiries
- Oxford University Press
- Oxford Languages
- University of Oxford
Oxford University Press is a department of the University of Oxford. It furthers the University's objective of excellence in research, scholarship, and education by publishing worldwide
- Copyright © 2024 Oxford University Press
- Cookie settings
- Cookie policy
- Privacy policy
- Legal notice
This Feature Is Available To Subscribers Only
Sign In or Create an Account
This PDF is available to Subscribers Only
For full access to this pdf, sign in to an existing account, or purchase an annual subscription.
Sintering parameters for uranium-gadolinium oxide fuel pellets
- Published: 11 August 2012
- Volume 112 , pages 303–306, ( 2012 )
Cite this article
- V. G. Baranov 1 ,
- R. S. Kuzmin 1 ,
- A. V. Tenishev 1 ,
- A. V. Khlunov 1 ,
- A. V. Ivanov 2 ,
- I. V. Petrov 2 &
- I. S. Timoshin 2
143 Accesses
2 Citations
Explore all metrics
This is a preview of subscription content, log in via an institution to check access.
Access this article
Price includes VAT (Russian Federation)
Instant access to the full article PDF.
Rent this article via DeepDyve
Institutional subscriptions
K. Kim, J. Yang, K. Kang, et al., “Measurement of Gd content in (U, Gd)O 2 using thermal gravimetric analysis,” J. Nucl. Mater ., 325 , 129–133 (2004).
Article ADS Google Scholar
V. V. Gorskii, “Uranium-gadolinium oxide fuel. Pt. 2. Thermophysical properties of UO 2 –Gd 2 O 3 and methods for monitoring them,” At. Tekhn. Rubezh. , No. 3, 6–15 (1989).
Google Scholar
R. Holzer, “Progress in the design of fuel assemblies for LWR,” in: Proc. Symp. on Improvements in Water Reactor Fuel Technology and Utilization , Stockholm, September 15–19, 1986, IAEA, Vienna (1987), pp. 43–56.
H. Assmann, M. Peehs, and H. Roepenack, “Survey of binary oxide fuel manufacturing and quality control,” J. Nucl. Mater ., 153 , 115–126 (1988).
L. Newman, Thermal and Physical Properties of Uranium-Gadolinia , Babcock and Wilcox Utility Power Generation, Rep. RAV-1759 (1984).
V. G. Baranov, R. S. Kuzmin, A. V. Tenishev, et al., “Sintering particulars of pelleted oxide nuclear fuel,” At. Énerg. , 110 , No. 3, 146–149 (2011).
Download references
Author information
Authors and affiliations.
National Nuclear Research University – Moscow Engineering-Physics Institute (NIYaU MIFI), Moscow, Russia
V. G. Baranov, R. S. Kuzmin, A. V. Tenishev & A. V. Khlunov
Machine Building Works, Elektrostal, Moscow Oblast, Russia
A. V. Ivanov, I. V. Petrov & I. S. Timoshin
You can also search for this author in PubMed Google Scholar
Additional information
Translated from Atomnaya Énergiya, Vol. 112, No. 4, pp. 245–248, April, 2012.
Rights and permissions
Reprints and permissions
About this article
Baranov, V.G., Kuzmin, R.S., Tenishev, A.V. et al. Sintering parameters for uranium-gadolinium oxide fuel pellets. At Energy 112 , 303–306 (2012). https://doi.org/10.1007/s10512-012-9561-2
Download citation
Received : 10 June 2011
Published : 11 August 2012
Issue Date : August 2012
DOI : https://doi.org/10.1007/s10512-012-9561-2
Share this article
Anyone you share the following link with will be able to read this content:
Sorry, a shareable link is not currently available for this article.
Provided by the Springer Nature SharedIt content-sharing initiative
- Fuel Assembly
- Shrinkage Rate
- Uranium Oxide
- Sintered Pellet
- Fuel Pellet
- Find a journal
- Publish with us
- Track your research
Help | Advanced Search
Computer Science > Artificial Intelligence
Title: chinchilla scaling: a replication attempt.
Abstract: Hoffmann et al. (2022) propose three methods for estimating a compute-optimal scaling law. We attempt to replicate their third estimation procedure, which involves fitting a parametric loss function to a reconstruction of data from their plots. We find that the reported estimates are inconsistent with their first two estimation methods, fail at fitting the extracted data, and report implausibly narrow confidence intervals--intervals this narrow would require over 600,000 experiments, while they likely only ran fewer than 500. In contrast, our rederivation of the scaling law using the third approach yields results that are compatible with the findings from the first two estimation procedures described by Hoffmann et al.
Submission history
Access paper:.
- HTML (experimental)
- Other Formats

References & Citations
- Google Scholar
- Semantic Scholar
BibTeX formatted citation

Bibliographic and Citation Tools
Code, data and media associated with this article, recommenders and search tools.
- Institution
arXivLabs: experimental projects with community collaborators
arXivLabs is a framework that allows collaborators to develop and share new arXiv features directly on our website.
Both individuals and organizations that work with arXivLabs have embraced and accepted our values of openness, community, excellence, and user data privacy. arXiv is committed to these values and only works with partners that adhere to them.
Have an idea for a project that will add value for arXiv's community? Learn more about arXivLabs .
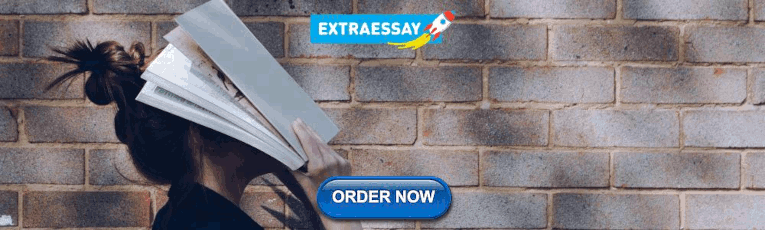
IMAGES
VIDEO
COMMENTS
On the left, select Preprints and locate your publication. Click Add published version under the preprint title. Select the published work you want to link to if it's already on ResearchGate, or create a new publication if it's not. Click Add published version. Your published work's page is now linked and accessible directly from your preprint.
Select a repository that assigns a DOI so that citations to the preprint get aggregated. When using a preprint repository that assigns a DOI, the same work may eventually have two sets of citations, one for the preprint and one for the published version. In repositories like PsyArXiv that are indexed by Google Scholar, both versions will be ...
Altmetric provide a free API (https://api.altmetric.com) against which we queried each preprint DOI in our analysis set. Importantly, Altmetric only contains records where an article has been mentioned in at least one of the sources tracked; thus, if our query returned an invalid response, we recorded counts for all indicators as 0. Coverage of ...
If you have identified your preprint with a DOI and have been transparent about publishing your research as a preprint when it is time to submit to a traditional journal, then publishing a preprint on ResearchGate is just as safe as any other preprint server. As we have already discussed above, preprints are visible and documented parts of the ...
Can other authors cite my ResearchGate preprints? For the manual addition of my paper, I have performed the following steps. For the manual addition of my paper at first I tried with Add articles option but I did not find it on scholar. Then I tried with Add articles manually but I did not find any preprint option there.,
However, please make sure only one page lists the DOI. This is how you can edit the items so that they merge automatically: Go to the publication's ResearchGate page by clicking on the title of the publication; Click the More button at the top right of the page and select Edit from the drop-down list; Make the necessary changes; Click Save.
Total preprints. 238,876. Unique authors. 22.49. Median hours to announcement. 16,141,467. Total page views. Preprints is a multidisciplinary preprint platform that accepts articles from all fields of science and technology, given that the preprint is scientifically sound and can be considered part of academic literature.
Show the journal that is considering your work on your preprint and track your manuscript through peer review. In Review is a free journal-integrated preprint service offered to authors at participating journals. Authors can opt to have their manuscript automatically posted online in the form of a preprint with a DOI. Learn more here.
Request PDF | On Jan 1, 2024, Mu Liu and others published Global Insect Herbivory and its Response to Climate Change | Find, read and cite all the research you need on ResearchGate
Focus is on both applied and theoretical issues in robotics and automation. Robotics is here defined to include intelligent machines and systems; whereas automation includes the use of automated methods in various applications to improve performance and productivity. The society sponsors a number of conferences, including the annual ...
DOI:10.1109/TPS.2018. ... Preprint of Keldysh Institute of Applied Mathematics of Russian Academy of Sciences, #51, 2015, 23 p. ... Most researchers use their institutional email address as their ...
In 2023, overall pharmaceutical expenditures in the US grew 13.6% compared to 2022, for a total of $722.5 billion. Utilization (a 6.5% increase), new drugs (a 4.2% increase) and price (a 2.9% increase) drove this increase.
Authors and Affiliations. National Nuclear Research University - Moscow Engineering-Physics Institute (NIYaU MIFI), Moscow, Russia. V. G. Baranov, R. S. Kuzmin, A ...
Sintering of oxide nuclear fuel pellets has been studied by dilatometric and thermogravimetric studies in. the medium Ar-8%H 2 at temperatures to 1600°C. Samples pr oduced under commercial ...
References Anumanchipalli,GopalaK.,JoshChartier,andEdwardF.Chang.2019."SpeechSynthesisfrom NeuralDecodingofSpokenSentences."Nature568(7753):493-98.
An experimental study of sintering parameters for uranium-gadolinium oxide fuel pellets was conducted. A DIL 402 C high-temperature dilatometer and a STA 409 CD synchronous thermal analyzer were used.
Hoffmann et al. (2022) propose three methods for estimating a compute-optimal scaling law. We attempt to replicate their third estimation procedure, which involves fitting a parametric loss function to a reconstruction of data from their plots. We find that the reported estimates are inconsistent with their first two estimation methods, fail at fitting the extracted data, and report ...