Click through the PLOS taxonomy to find articles in your field.
For more information about PLOS Subject Areas, click here .
Loading metrics
Open Access
Peer-reviewed
Research Article
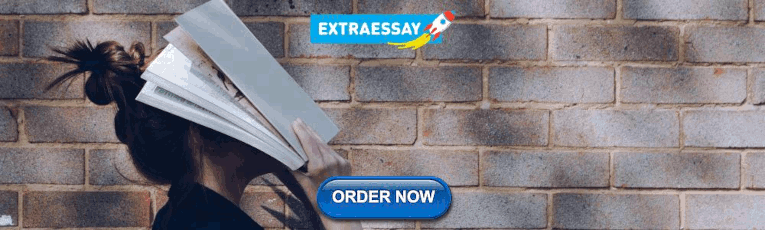
The Effectiveness of Teamwork Training on Teamwork Behaviors and Team Performance: A Systematic Review and Meta-Analysis of Controlled Interventions
* E-mail: [email protected]
Affiliation School of Kinesiology, The University of British Columbia, Vancouver, British Columbia, Canada
Affiliation Departments of Kinesiology/Physical Education and Psychology, Wilfrid Laurier University, Waterloo, Ontario, Canada
Affiliation Department of Educational and Counseling Psychology, Faculty of Education, University of British Columbia, Vancouver, British Columbia, Canada
- Desmond McEwan,
- Geralyn R. Ruissen,
- Mark A. Eys,
- Bruno D. Zumbo,
- Mark R. Beauchamp
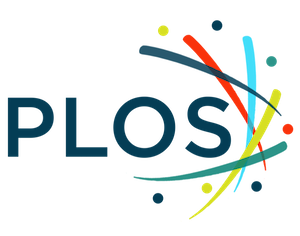
- Published: January 13, 2017
- https://doi.org/10.1371/journal.pone.0169604
- Reader Comments
The objective of this study was to conduct a systematic review and meta-analysis of teamwork interventions that were carried out with the purpose of improving teamwork and team performance, using controlled experimental designs. A literature search returned 16,849 unique articles. The meta-analysis was ultimately conducted on 51 articles, comprising 72 ( k ) unique interventions, 194 effect sizes, and 8439 participants, using a random effects model. Positive and significant medium-sized effects were found for teamwork interventions on both teamwork and team performance. Moderator analyses were also conducted, which generally revealed positive and significant effects with respect to several sample, intervention, and measurement characteristics. Implications for effective teamwork interventions as well as considerations for future research are discussed.
Citation: McEwan D, Ruissen GR, Eys MA, Zumbo BD, Beauchamp MR (2017) The Effectiveness of Teamwork Training on Teamwork Behaviors and Team Performance: A Systematic Review and Meta-Analysis of Controlled Interventions. PLoS ONE 12(1): e0169604. https://doi.org/10.1371/journal.pone.0169604
Editor: Nico W. Van Yperen, Rijksuniversiteit Groningen, NETHERLANDS
Received: September 15, 2016; Accepted: December 19, 2016; Published: January 13, 2017
Copyright: © 2017 McEwan et al. This is an open access article distributed under the terms of the Creative Commons Attribution License , which permits unrestricted use, distribution, and reproduction in any medium, provided the original author and source are credited.
Data Availability: All relevant data are within the paper and its Supporting Information files. Raw data (taken from the studies in our meta-analysis) are available upon request from the corresponding author.
Funding: The authors received no specific funding for this work.
Competing interests: The authors have declared that no competing interests exist.
Introduction
From road construction crews and professional soccer squads to political parties and special operations corps, teams have become a ubiquitous part of today’s world. Bringing a group of highly-skilled individuals together is not sufficient for teams to be effective. Rather, team members need to be able to work well together in order for the team to successfully achieve its purposes [ 1 , 2 ]. As a result, there has been a proliferation of research assessing whether, and how, teams can be improved through teamwork training. A wide range of studies have shown positive effects of teamwork interventions for improving team effectiveness across several contexts such as health care (e.g., [ 3 ]), military (e.g., [ 4 ]), aviation (e.g., [ 5 ]), and academic (e.g., [ 6 ]) settings. Similarly, improvements in teamwork have been observed as a result of training with a variety of team types including new teams (e.g., [ 7 ]), intact teams (e.g., [ 8 ]), and those created for laboratory-based experiments (e.g., [ 9 ]). In sum, the extant empirical evidence to date appears to suggest that teams can be improved via teamwork training.
What is Teamwork?
Within teams, members’ behaviors can be categorized in terms of both taskwork and teamwork processes [ 2 ]. Marks et al. [ 10 ] differentiated between the two by suggesting that “taskwork represents what it is that teams are doing, whereas teamwork describes how they are doing it with each other” (p. 357). Specifically, while taskwork involves the execution of core technical competencies within a given domain, teamwork refers to the range of interactive and interdependent behavioral processes among team members that convert team inputs (e.g., member characteristics, organizational funding, team member composition) into outcomes (e.g., team performance, team member satisfaction) [ 2 , 10 ]. Some examples of teamwork (and respective comparisons to taskwork) include: the seamless communication between a surgeon, nurse, and anaesthesiologist, rather than the technical competencies of these practitioners; the synergy between a quarterback and receiver to complete a passing play, rather than their respective skill sets related to throwing or catching a football; the collaborative adjustments a flight crew makes in response to adverse weather or system problems, rather than each individual’s aviation skills; and so forth. Research from an assortment of studies indicates that teamwork—the focus of the current paper—is positively related to important team effectiveness variables, including team performance, group cohesion, collective efficacy, and member satisfaction [ 1 ].
Teamwork has been conceptualized within several theoretical models. For example, in their review, Rousseau et al. [ 2 ] reported that 29 frameworks related to teamwork have been published. Although there is much overlap across these models, there are also some notable differences. These relate to the number of dimensions of teamwork being conceptualized as well as the specific labelling of these dimensions. One thing that is generally agreed upon, however, is that teamwork is comprised of multiple observable and measurable behaviors . For instance, two highly cited frameworks by Marks et al. [ 10 ] and Rousseau et al. [ 2 ] consist of 10 and 14 dimensions of teamwork, respectively. In general, teamwork models focus on behaviors that function to (a) regulate a team’s performance and/or (b) keep the team together. These two components coincide with the two respective processes that Kurt Lewin, the widely recognized father of group dynamics, originally proposed all groups to be involved in: locomotion and maintenance [ 11 ].
With regard to regulating team performance (i.e., locomotion), teamwork behaviors include those that occur (a) before/in preparation for team task performance, (b) during the execution of team performance, and (c) after completing the team task [ 2 ]. First, with regard to teamwork behaviors that occur before/in preparation for team task performance, these include the active process of defining the team’s overall purpose/mission, setting team goals, and formulating action plans/strategies for how goals and broader purposes will be achieved. These behaviors help ensure that all team members are clear in terms of what is required of them in order for the team to function effectively. Second, teamwork behaviors that occur during the execution of team tasks include actions that correspond to members’ communication, coordination, and cooperation with each other. At this stage, team members translate what they have previously planned (during the preparation phase) into action. Third, in terms of teamwork behaviors that occur after completing the team task (i.e., reflection), these include monitoring important situations and conducting post-task appraisals of the team’s performance and system variables (e.g., internal team resources, broader environmental conditions), solving problems that are precluding team goal attainment, making innovative adjustments to the team’s strategy, and providing/receiving verbal and behavioral assistance to/from teammates. Hence, team members determine whether their actions have moved them closer towards accomplishing the team goals and objectives, and whether any modifications are required in order to facilitate future success. In addition to these three dimensions concerned with the regulation of team performance, a fourth dimension of teamwork involves behaviors that function to keep the team together (i.e., maintenance). These behaviors focus on the team’s interpersonal dynamics , and include the management of interpersonal conflict between members and the provision of social support for members experiencing personal difficulties. Managing interpersonal dynamics is critical as it is theorized that teams cannot operate effectively when these issues are present [ 2 ].
How Can Teamwork Be Trained?
Teamwork interventions have utilized a number of training methods in order to target the regulation of team performance (i.e., preparation, execution, reflection) and management of team maintenance (i.e., interpersonal dynamics) dimensions. These intervention strategies generally fall under one of four categories. First, the most basic approach to training and developing teamwork involves providing didactic education to team members in a classroom-type setting, such as lecturing about the importance of providing social support within the team or promoting ways to manage interpersonal conflict among teammates. This type of training has been found to be useful for enhancing team effectiveness (e.g., [ 12 ]). A second category of team training involves utilizing a more interactive workshop-style format, wherein team members take part in various group activities, such as having discussions about the team’s purposes and goals (e.g., [ 13 ]) or working through case studies together (e.g. [ 14 ]). The third broad category of team training involves simulation training, wherein teams experientially enact various teamwork skills, such as interpersonal communication and coordination, in an environment that mimics upcoming team tasks (e.g., airline simulators or medical patient manikins). Although often used as a means of fostering taskwork competencies (e.g., teaching new surgeons how to perform the technical skills of a medical operation), simulation training has been found to be an efficacious approach to teamwork intervention (e.g., [ 15 ]). In addition to these three training approaches that occur outside of the team task environment (i.e., training within classroom and simulation settings), teamwork can also be fostered by incorporating team reviews in-situ (i.e., where the team actually performs its tasks), which allows teams to monitor/review their quality of teamwork on an ongoing basis. These team reviews involve some form of team briefs before (e.g., creating action plans), during (e.g., monitoring team members’ actions), and/or after (e.g., assessing the team’s performance) team task execution, and have also been shown to be efficacious in previous studies (e.g., [ 16 ]).
The effectiveness of teamwork interventions can be determined with an assortment of criteria, including team- and individually-based behaviors, cognitions, and affective states. Hackman and Katz 2010 [ 17 ] posit that team effectiveness can be determined by examining the extent to which the team has achieved its a priori objectives. Since the broad purpose of forming a team is to produce something of value, it is perhaps unsurprising that the most widely tested criterion of team effectiveness has been team performance [ 18 – 20 ]. Thus, although teams come from an array of settings and are idiosyncratic in their own ways, one question that essentially all teams address at some point during their tenure is whether they are performing well. For example, is that road construction crew fixing potholes adequately? Does the local soccer squad have a respectable winning percentage? Has an elected political party successfully completed the tasks for which they campaigned? Did a special operations corps achieve the mission it set out to accomplish? When taken in concert, questions related to team performance are often of central interest when characterizing a team’s effectiveness.
In addition to assessing the outcome variable of team performance, researchers have also been interested in whether teamwork training actually improves teamwork itself. The efficacy of these interventions can be determined with a number of objective (e.g., products produced by an industry team), self-report (e.g., questionnaires regarding perceived social support amongst team members), and third-party assessments (e.g., expert ratings of team behaviors). Both general/omnibus measures of teamwork (e.g., [ 21 ]) as well as those assessing specific dimensions of teamwork (e.g., communication [ 22 ]) have been operationalized to examine the effectiveness of these interventions. For example, do team goal setting activities actually result in members creating and pursuing effective team goals? Does simulation training improve the requisite coordination processes among aviation cockpit crews? Has a didactic lecture contributed to improved conflict management among team members? Answering these types of questions is important for determining whether an intervention is actually efficacious in changing the variable that is targeted for improvement (i.e., teamwork behaviors).
The Current Review
Prior to outlining the purposes of this systematic review, it is important to recognize that previous quantitative reviews have been conducted that addressed—to some degree—teamwork training. In preparation for this systematic review, we conducted a scoping review which revealed that eight previous meta-analyses have assessed teamwork intervention studies in some way. However, these reviews were delimited based on various sample and/or intervention characteristics. For example, some reviews included studies that were only conducted with certain team types (e.g., intact teams [ 23 ]) or within a particular context (e.g., sports [ 24 ]; medical teams [ 25 ]). Others were delimited to specific training programs/strategies that were restricted to a narrow range of teamwork strategies (e.g., [ 23 , 25 – 29 ]). Finally, studies that used a combination of teamwork and taskwork intervention components have been systematically reviewed [ 30 ]; however, these types of interventions result in a limited ability to determine the extent to which the resulting effects were due to teamwork training versus taskwork training.
It should also be noted that all but one [ 23 ] of these previous reviews pooled together studies that included a control condition (i.e., wherein teams do not receive any type of teamwork training) and those that did not (as mentioned above, that study only analyzed the effects of certain teamwork strategies). This is an important consideration, as it has been suggested that controlled and uncontrolled studies should not be combined into the same meta-analysis due to differences in study quality (which is a major source of heterogeneity) and since stronger conclusions can be derived from controlled interventions compared to uncontrolled interventions (e.g., [ 31 ]). Therefore, while previous systematic reviews have provided valuable contributions to the teamwork literature, a systematic review that assesses the effects of controlled teamwork interventions across a range of contexts, team types, and involving those that targeted diverse dimensions of teamwork appears warranted. In doing so, a more comprehensive assessment of the efficacy of these teamwork interventions is provided, while also having the capacity to look at the potential moderating effects of various sample, intervention, and measurement characteristics. Moreover, by including only controlled studies, one is able to make stronger conclusions regarding the observed effects.
The overall purpose of this study was to better understand the utility of teamwork training for enhancing team effectiveness. Specifically, a meta-analysis was conducted on controlled studies (i.e., comparing teams who have received teamwork training with those who have not) that have examined the effects of teamwork interventions on teamwork processes and/or team performance. To better disentangle the effectiveness of these studies, we also sought to assess potential moderators of these main effects; that is, to determine whether there are certain conditions under which the independent variable of teamwork training more strongly (or weakly) causally influences the dependent variables of teamwork behaviors or team performance [ 32 ]. The specific moderators that we assessed included: (a) the team context/field of study, (b) the type of teams that were trained, (c) the primary type of intervention method employed, (d) the dimensions of teamwork that were targeted in the intervention, (e) the number of dimensions targeted, (f) the types of measures used to quantify the training effects, and (g) in studies where teamwork was assessed as an outcome variable, the dimensions of teamwork that were measured. It was hypothesized that teamwork training would have a positive and significant effect on both teamwork and team performance and that these effects would be evident across a range of the aforementioned sample, intervention, and measurement characteristics/conditions.
Literature Search
Searches for potential articles were conducted in the following databases: PsycInfo , Medline , Cochrane Central Register of Controlled Trials , SportDiscus , and ProQuest Dissertations and Theses . Hand searches were also conducted across thirteen journals that typically publish articles on group dynamics (e.g., Group Dynamics : Theory , Research , and Practice ; Small Group Research , Journal of Applied Psychology ; Personnel Psychology , Human Factors ; Academy of Management Journal , Journal of Sport & Exercise Psychology ). In each database and journal search, the following combination of search terms were used: ( team OR interprofessional OR interdisciplinary ) AND ( intervention OR training OR building OR simulation ) AND ( teamwork OR mission analysis OR goal specification OR goal setting OR planning OR strategy OR coordination OR cooperation OR communication OR information exchange OR information sharing OR monitoring OR problem solving OR backing up OR coaching OR innovation OR adaptability OR feedback OR support OR conflict management OR situation awareness OR confidence building OR affect management ). These terms were based on various models of teamwork that exist within the literature (see Rousseau et al. [ 2 ] for an overview of these models). An additional search was conducted within these databases and journals using the search terms ( TeamSTEPPS OR Crew Resource Management OR SBAR [Situation-Background-Assessment-Recommendation]), as several articles in the initial search used these specific training programs. We also searched the reference sections of the articles from past teamwork training review papers as well as from articles that initially met inclusion criteria to determine if any additional articles could be retrieved. The searches were conducted in September 2015 and no time limits were placed on the search strategy. Each article was first subjected to title elimination, then abstract elimination, and finally full-text elimination.
Eligibility Criteria
To be included in the meta-analysis, a study needed to examine the effects of teamwork training by comparing teams in an experimental condition (i.e., those who received teamwork training) with those in a control condition (i.e., where teams did not receive teamwork training). Cross-sectional/non-experimental studies were excluded, as were intervention studies that did not include a control condition. As this review was only concerned with teamwork interventions, studies that focused on training taskwork—whether independent of, or in addition to, a teamwork intervention—were excluded. For example, as previously mentioned, simulation-based training (SBT) has been used as a means of training individuals to perform technical skills and also to enhance teamwork. In order for a SBT intervention to be included in this meta-analysis, it had to be clear that only teamwork (not technical skills) was being targeted during training. In order to address our primary research question, the study had to provide data on at least one teamwork dimension and/or team performance. The study also needed to provide sufficient statistics to compute an effect size. In cases of insufficient data, corresponding authors were contacted for this information. The articles were delimited to those published in the English language.
Data Analysis
Articles that met the aforementioned eligibility criteria were extracted for effect sizes and coded independently with respect to seven moderators by two of the authors (DM and GR). Interrater reliability for the coding of these moderators was over 90%, kappa (SE) = 0.80 (0.01). The moderators examined were based on a scoping review (the purpose of which included identifying pertinent characteristics that were commonly reported in previous teamwork intervention research), which was conducted in preparation for this systematic review. The moderators that were examined in this review included (1) the context within which an intervention was conducted ( health care , aviation , military , academia , industry , or laboratory experiment) , (2) the type of team targeted ( intact or new ), (3) the primary training method applied to conduct the intervention ( didactic education , workshop , simulation , or team reviews ), (4) the dimension(s) of teamwork ( preparation , execution , reflection , and/or interpersonal dynamics ) targeted in the intervention as well as (5) the number of dimensions targeted (between one and four), (6) the type of measure used to derive effect sizes ( self-report , third party , or objective measures ), and—when teamwork was assessed as the criterion variable—(7) the specific dimension(s) of teamwork that were measured ( general , preparation , execution , reflection , and interpersonal dynamics ).
Once coded, data were entered into the software Comprehensive Meta-Analysis , Version 2 [ 33 ] and analyzed as a random-effects model (DerSimonian and Laird approach). This type of model assumes that there is heterogeneity in the effect sizes across the included studies and is the appropriate model to use in social science research, as opposed to a fixed-effects model (which assumes that effect sizes do not vary from study to study) [ 34 , 35 ]. Where possible, effect sizes for each study were derived from means, standard deviations, and sample sizes at baseline and post-intervention [ 34 , 36 ]. If these statistics were not fully provided, they were supplemented with F -statistics, t scores, correlations, and p -values to compute the effect size. Each study was given a relative weight based on its precision, which is determined by the study’s sample size, standard error, and confidence interval (i.e., the more precise the data, the larger the relative study weight) [ 34 ].
In instances where a study provided data to calculate multiple effect sizes (such as when several measures of the criterion variable—teamwork or team performance—were examined), these effects were combined into one overall effect size statistic (i.e., a weighted average) for that study. This was done to ensure that those studies that had multiple measures of teamwork or team performance were not given greater weight compared to studies that only provided one effect size (i.e., only had one measure of performance or teamwork), which could potentially skew the overall results [ 34 ]. The exception to this was when articles reported the effects of more than one intervention (i.e., had multiple experimental conditions), each of which had a unique teamwork training protocol. In these cases, an effect size from each intervention was computed. Thus, these articles would contribute multiple effect sizes to the total number of comparisons within the meta-analysis. To correct for potential unit-of-analysis errors in these particular articles, the sample size of the control condition was divided by the number of within-study comparisons [ 31 ]. For example, if three different types of teamwork interventions were compared to one control condition (e.g., which had a sample size of 30 participants), the n of the control condition was divided by 3 (i.e., 30/3 = 10) when calculating the effect sizes of those interventions. Cohen’s d was used as the effect size metric to represent the standardized effect (i.e., the average magnitude of effectiveness) of teamwork interventions on teamwork and team performance [ 37 ]. Standard errors and 95% confidence intervals were computed to test for the accuracy of the standardized effects obtained.
To reduce heterogeneity and improve the interpretability of the results, we pooled studies into those that measured teamwork as its criterion variable and those that measured team performance. Pooling studies in this manner not only reduces heterogeneity but also allowed us to identify the extent to which teamwork interventions impact team performance and, separately, the extent to which they affect teamwork processes. Heterogeneity within the meta-analysis was also assessed by computing a Q value—which estimates the variability in the observed effect sizes across studies—and an I 2 statistic—which estimates the ratio of the true heterogeneity to the total observed variation across studies. High Q and I 2 statistics can be problematic for interpreting the results of a meta-analysis and can also indicate that the meta-analysis includes outlier studies. We also planned to identify and exclude outliers from subsequent moderator analyses in two ways. First, sensitivity analyses were carried out by removing a single intervention from the meta-analysis and noting the resulting effect size—this estimates the impact that each individual intervention has on the overall effect size of teamwork or team performance. If the resulting effect size with an intervention removed (i.e., K– 1) is substantially different than the effect size with that intervention present, this may suggest that it is an outlier and needs to be removed [ 34 ]. Second, we noted any studies that had abnormally high effect sizes and standardized residuals (above 3.0), especially when these values were accompanied by narrow confidence intervals. If heterogeneity ( Q and I 2 ) is substantially reduced upon removal of a study, this further confirms that the study is an outlier and should be omitted from subsequent subgroup/moderator analyses.
Once the two pools of studies were produced, bias within each pool was assessed. First, publication bias was examined by calculating a fail-safe N statistic, which estimates the number of unpublished studies with null findings that would have to exist to reduce the obtained effect size to zero [ 38 ]. If this number is sufficiently large—Rosenberg [ 39 ] recommends a critical value of 5 N +10—then the probability of such a number of studies existing is considered to be low. For example, if 20 studies were included in a meta-analysis, then the resulting fail-safe N should be larger than 110 (i.e., 5*20 + 10); if this value was not larger than 110, then publication bias is likely within this pool of studies. We also obtained two funnel plots (one for studies where teamwork was the outcome variable and one for team performance as the outcome) to provide a visual depiction of potential publication bias. We then conducted an Egger’s test as a measure of symmetry for these two funnel plots. If this test statistic is significant ( p < 0.05), this denotes that the distribution around the effect size is asymmetric and publication bias is likely present [ 34 ].
The literature search from the five databases returned 22,066 articles, while the hand searches of the 13 journals returned 3797 articles, vetting of studies from previous team training reviews returned 191 articles, and the ancestry search of reference lists returned 471 articles (see Fig 1 ). After removing duplicates, 16,849 articles were subject to title and abstract screening, where they were dichotomously coded as ‘potentially relevant’ or ‘clearly not relevant’. 1517 potentially relevant articles were then full-text reviewed and coded as meeting eligibility criteria or as ineligible for the following reasons: (1) not a teamwork intervention; (2) teamwork-plus-taskwork intervention; (3) insufficient statistics to compute an effect size; (4) not including a measure of teamwork or team performance; or (5) not including a control group. As a result of this eligibility coding, 51 articles were included in the meta-analysis. 13 of these studies reported results on two or more interventions, bringing the total number of comparisons ( k ) to 72 with 8439 participants (4966 experimental, 3473 control). See S1 Table for descriptions of each study with regard to study context, type of team and participants, targeted teamwork dimensions of the intervention, number of effect sizes, the criteria measured, and an overview of the intervention.
- PPT PowerPoint slide
- PNG larger image
- TIFF original image
https://doi.org/10.1371/journal.pone.0169604.g001
Summary Statistics
Results of the overall effect of teamwork interventions on teamwork processes along with summary statistics and sensitivity analyses (i.e., the final column marked ‘ES with study removed’) for this pool of studies are presented in Table 1 . This pool included a total of 39 interventions from 33 studies. The results revealed that teamwork interventions had a significant, medium-to-large effect on teamwork, d ( SE ) = 0.683 (0.13), 95% CI = 0.43–0.94, Z = 5.23, p < 0.001; Q ( df ) = 660.7 (38), I 2 = 94.2. The funnel plot for this pool of studies is shown in Fig 2 . The fail-safe N was 3598, which is sufficiently large, as it exceeds the critical value of 205 (5*39+10). The funnel plot for this pool of studies is presented in Fig 2 . Egger’s value for this funnel plot was not significant ( B = 0.364, SE = 1.30, 95% CI = -2.26–2.99, t = 0.28, p = 0.78), which also suggests that bias was not present. Two studies were identified as outliers within this pool of studies: Morey et al. [ 3 ] and Marshall et al. [ 22 ]. The resulting effect size when these studies were excluded was d (SE) = 0.550 (0.08), 95% CI = 0.39–0.71, Z = 6.73, p < 0.001; Q ( df ) = 187.53 (36), I 2 = 80.8. Subsequent moderator analyses were conducted with these two outlier studies being omitted.
Circles filled with black indicate outlier studies.
https://doi.org/10.1371/journal.pone.0169604.g002
https://doi.org/10.1371/journal.pone.0169604.t001
Results of the overall effect of teamwork interventions on team performance as well as summary statistics and sensitivity analyses (i.e., the final column marked ‘ES with intervention removed’) for this pool of studies are presented in Table 2 . This pool of studies included a total of 50 interventions from 32 studies. It was shown that teamwork interventions had a significant, large effect on team performance— d ( SE ) = 0.919 (0.14), 95% CI = 0.65–1.19, Z = 6.72, p < 0.001; Q ( df ) = 851.3 (49), I 2 = 94.2. The funnel plot for this pool of studies is shown in Fig 3 . The fail-safe N was 6692, which is sufficiently large, as it exceeds the critical value of 260 (5*50+10). The funnel plot for this pool of studies is presented in Fig 3 . Egger’s value for this funnel plot was not significant ( B = 0.131, SE = 1.19, 95% CI = -2.26–2.54, t = 0.11, p = 0.91), which also implies that bias was not present. There were five outlier interventions (from four studies) in this pool of studies that assessed team performance: Morey et al. [ 3 ], Smith-Jentsch et al. [ 4 ], one of the interventions from Buller and Bell [ 63 ]; teambuilding condition), and both interventions from Bushe and Coetzer [ 43 ]. When these outliers were removed, the resulting effect size was d ( SE ) = 0.582 (0.06), 95% CI = 0.47–0.69, Z = 10.30, p < 0.001; Q ( df ) = 101.1 (44), I 2 = 56.5. Subsequent moderator analyses were conducted with these five interventions omitted.
https://doi.org/10.1371/journal.pone.0169604.g003
https://doi.org/10.1371/journal.pone.0169604.t002
Moderator Analyses
The results of the moderator analyses are shown in Table 3 (for teamwork behaviors) and Table 4 (for team performance). With respect to sample characteristics, significant positive effects of teamwork interventions were found for enhancing teamwork across all contexts ( d s = 0.46–1.23) except for the single effect size from an industry setting ( d = 0.50). In terms of team performance, significant effects were evident across all settings ( d s = 0.40–1.76). In addition, interventions were effective for enhancing teamwork with intact teams ( d = 0.33) and newly-formed teams ( d = 0.67), with the effect size for new teams being significantly larger ( Q = 4.04, p = 0.004) than that for existing teams. Teamwork training was also effective at fostering team performance for both team types; however, in contrast to the findings on teamwork, the effect size for intact teams ( d = 0.99) was significantly larger ( Q = 6.04, p = 0.02) than that for new teams ( d = 0.54).
https://doi.org/10.1371/journal.pone.0169604.t003
https://doi.org/10.1371/journal.pone.0169604.t004
Three intervention characteristics were analyzed as potential moderators. First, with regard to the intervention method utilized, significant effects on teamwork were found for workshop training ( d = 0.50), simulation-based teamwork training ( d = 0.78), and team reviews ( d = 0.64) but not for didactic education ( d = 0.19). All training methods were effective for enhancing team performance ( d s = 0.41–0.69). Second, significant effects of training on teamwork were evident when two or more dimensions of teamwork were targeted ( d s = 0.65–0.98) but not when only one dimension was targeted ( d = 0.05). Team performance, however, improved significantly as a result of teamwork training regardless of the number of teamwork dimensions that were targeted ( d s = 0.46–0.67). Third, significant effects were shown regardless of which dimension (i.e., preparation, execution, reflection, interpersonal dynamics) was targeted for both teamwork ( d s = 0.64–0.75) and team performance ( d s = 0.52–0.60).
With regard to measurement characteristics, significant improvements on teamwork emerged when either third-party ( d = 0.80) or self-report ( d = 0.38) measures of teamwork were utilized; the effect size for third-party measures was significantly larger ( Q = 6.02, p = 0.014) than the effect size for self-report measures. For team performance outcomes, significant effects were shown for both objective ( d = 0.61) and third-party measures ( d = 0.56). Finally, significant effects on teamwork were found when general/omnibus measures of teamwork were taken ( d = 0.71), as well as when a specific dimension of teamwork was measured ( d s = 0.45–0.70).
The purpose of this systematic review and meta-analysis was to quantify the effects of the extant controlled experimental research of teamwork training interventions on teamwork and team performance. We found positive and significant medium-to-large sized effects for these interventions on teamwork and large effects on team performance. When outlier studies were removed, medium-sized effects were found for both criteria. Additional subgroup/moderator analyses also revealed several notable findings, each of which will be discussed in turn. The paper concludes with a discussion of the limitations associated with this meta-analysis as well as considerations for future teamwork training research.
Who Can Benefit From Teamwork Training?
With regard to sample characteristics, teamwork interventions were shown to be effective at enhancing both teamwork and team performance across a variety of team contexts, including laboratory settings as well as real-world contexts of health care, aviation, military, and academia. This highlights the efficacy of teamwork training as a means of improving teams; this is an important finding as effective teams (i.e., those that work well together and perform at a high level) are vital in many of the aforementioned contexts. For example, it has been estimated that approximately 70% of adverse events in medical settings are not due to individuals’ technical errors but, rather, as a result of breakdowns in teamwork [ 78 ]. Thus, there is a critical need to ensure that teams are effective across these settings, as these teams greatly impact (among other things) the welfare of others. The results of this meta-analysis suggest that teamwork training can indeed be a useful way of enhancing team effectiveness within these contexts.
We also examined whether there were differential effects of teamwork training for new teams compared to intact teams. It was shown that these interventions were effective for both team types. The effects of teamwork training on teamwork outcomes were significantly larger for new teams (who showed a medium-to-large effect size) compared to existing teams (who had a small-to-medium effect size). Interestingly, when we examined team performance as the criterion variable, the training effects were significantly larger for intact teams (who showed a large effect size) compared to newly-formed teams (who again showed a medium-to-large effect size). It should be noted that there were many more studies conducted with new teams compared to intact teams—thus, caution should be exercised in directly comparing these findings. Nonetheless, at this point, the existing research seems to suggest that teamwork interventions work particularly well at enhancing teamwork processes for newly established teams—and also work with existing teams—but not the same extent. It is possible that teamwork processes might be more malleable and display greater potential for improvement with new teams compared to more established teams whose teamwork processes may be more entrenched. On the other hand, it is notable that the effects of teamwork training on team performance were stronger for established teams. In line with this, it is plausible that, while intact teams may show less pronounced changes in teamwork, they might be better able to translate their teamwork training into improved team performance outcomes.
What Type of Training Works?
Three moderator variables were assessed with regard to intervention characteristics. First, with regard to the training method utilized, it was shown that all four training methods were effective for enhancing team performance. These included the provision of didactic lectures/presentations, workshops, simulation training, and review-type activities conducted in situ. Although significant effects were shown for the latter three training methods for teamwork outcomes, those interventions that targeted didactic instruction did not result in significant improvements in teamwork itself. This suggests that simply providing educational lectures wherein team members passively learn about teamwork is not an effective way of improving teamwork. When taken together these findings suggest that teamwork training should incorporate experiential activities that provide participants with more active ways of learning and practising teamwork. These may include various workshop-style exercises that involve all team members, such as working through case studies of how teams can improve teamwork, watching and critiquing video vignettes of teams displaying optimal versus suboptimal teamwork, discussing and setting teamwork-related goals and action plans, or other activities that help stimulate critical thinking and active learning of effective teamwork. Teams may also find it useful to conduct simulations of specific team tasks that the group is likely to encounter in-situ, such as aviation teams using an airplane simulator, surgical teams conducting mock-surgeries on medical manikins, military teams practising various field missions, and so on. Teamwork can be also fostered by having team members participate in team reviews/briefings before, during, and/or after the execution of team tasks that occur in-situ. In summary, simply lecturing about the importance of teamwork is not sufficient to create meaningful improvements in teamwork; rather, substantive positive effects can be derived by having team members engage in activities that require them to actively learn about and practise teamwork.
We also sought to assess how comprehensive an intervention should be—specifically, the number of teamwork dimensions that need to be targeted—in order to be effective. With regard to improving team performance, there were significant effects when one or more dimensions were targeted. However, in terms of improving teamwork behaviors, significant effects only emerged when two or more dimensions were targeted. From an applied perspective, individuals concerned with intervention (e.g., team consultants, coaches, managers, team leaders) can utilize these findings by targeting more than one dimension of teamwork within their training protocol. For instance, if the purpose of an intervention is to improve a health care team’s communication, greater effects may be derived by not merely targeting communication during the execution phase alone (e.g., with a structured communication tool), but by also incorporating strategies that target other dimensions of teamwork, such as setting goals and action plans for how communication will be improved (i.e., the preparation dimension of teamwork) as well as monitoring progress towards those goals, resolving any communication-related problems that arise, and making adjustments to action plans as necessary (i.e., the reflection dimension).
Relatedly, we sought to address whether there were differential effects of teamwork interventions on teamwork and team performance based on the dimensions of teamwork that were targeted. It was found that interventions had a significant effect on both teamwork behaviors and team performance when any dimension of teamwork was targeted. This is important as it means that if those concerned with intervention target any one of the four dimensions of teamwork, this will likely result in improvements in team functioning. While the preparation (i.e., behaviors occurring before team task performance such as setting goals and action plans), execution (i.e., intra-task behaviors such as communication and coordination), and reflection (i.e., behaviors occurring following task performance such as performance monitoring and problem solving) dimensions have each been theorized to be implicated in fostering team performance [ 2 , 79 ], is particularly noteworthy that interventions targeting the interpersonal dynamics of a team (i.e., managing interpersonal conflict and the provision of social support between members) also displayed significant effects in relation to team performance. Specifically, efforts to enhance interpersonal processes have generally been theorized to be related to supporting team maintenance more so than supporting team performance [ 2 , 79 ]. However, the results from the current review provide evidence that training teams with regard to social support and interpersonal conflict management processes may actually be a useful way to enhance team performance. While the exact reason for this effect is not immediately clear from this review, it may be that improving interpersonal dynamics has an indirect relationship with team performance. That is, teamwork training focused on improving social support and conflict management may improve the functioning of a team, which, in turn, improves the team’s performance. As Marks et al. [ 10 ] contend, these interpersonal processes “lay the foundation for the effectiveness of other processes” (p. 368). Relatedly, Rousseau et al. [ 2 ] suggest that problems related to social support and conflict management “may prevent team members from fully contributing to task accomplishment or from effectively regulating team performance” (p. 557). Further research examining this potential relationship is required as this would have implications in both research and applied teamwork settings.
Does It Matter How Criterion Variables Are Measured?
Two measurement characteristics were examined as moderators within this meta-analysis. First, significant, large- and small-to-medium sized effects were found for third party and self-report measures of teamwork, respectively. Significant medium effects were also evident for third party and objective measures of team performance. It is worth noting that significantly larger effect sizes emerged for third party assessments of teamwork compared to self-report measures. Taken together, these findings suggest that the positive effects that were found for teamwork interventions are not merely perceptive and/or due to individuals’ self-report biases (i.e., social desirability). Rather, these results indicate that the effects of these interventions on both teamwork and team performance are clearly observable with measures beyond self-report indices.
Finally, we sought to assess whether the effects of teamwork training varied based on which teamwork dimension(s) were measured. Medium-to-large effects emerged when general/omnibus measures of teamwork—that is, those that provided an overall score of teamwork as opposed to examining individual dimensions of teamwork—were taken. Measures that tapped into the specific dimensions of teamwork (e.g., those that provided individual scores on preparation, execution, reflection, and interpersonal dynamics) also yielded comparable effect sizes. Hence, teamwork interventions appear to have a somewhat similar effect on each of the components of teamwork. In summary, the results of the above two moderators (i.e., type of measure and dimension of teamwork examined) suggest that teamwork training has a positive impact on teamwork and team performance regardless of the way in which these variables are assessed.
Limitations
Despite the contributions of this meta-analytic review, it is not without limitations. First, there were additional variables that we had planned to analyze as moderators a priori including team size and length of/contact time within the intervention. However, there was an insufficient amount of reliable data across the studies on these variables to conduct these subgroup analyses appropriately. For instance, although many studies noted the total number of participants within an organization (e.g., a hospital) that took part in an intervention, information on the size of the teams within the organization (e.g., various units within the hospital) was often missing. Team composition variables such as this have been noted as important factors to take into account when examining teams (e.g., [ 30 , 80 ]). Similarly, although some studies were explicit about the total length of the intervention and the contact time between interventionists and participating teams, this information was not provided consistently. This too would have been a valuable feature to analyze in order to provide more specific recommendations about how teamwork training programs should be designed—that is, how long an intervention should last? Unfortunately, due to the paucity of information available in the included manuscripts, we were unable to determine whether these variables moderated the observed effects of teamwork training on teamwork and team performance in the current meta-analysis.
Furthermore, there was a considerable amount of variability within some of the moderator categories that were coded. For instance, with regard to intervention methods, ‘workshops’ consisted of many different types of activities including team charter sessions, strategy planning meetings, case study activities, and so on. Combining these activities into one category was done for the sake of being adequately powered to conduct moderator analyses (i.e., include a sufficient number of studies within each of the resulting categories). However, while the above examples are indeed activities that teams do together, they are of course each different in their own ways. Hence, although it is evident that workshop-type activities are effective overall, it is unclear if specific workshop activities are more effective than others. This example underscores the difficulty that can occur when trying to balance statistical power with accuracy for each moderator category when conducting subgroup analyses in a meta-analysis.
Relatedly, effect sizes were only computed with the statistics that were provided from baseline and post-intervention, even if studies provided additional data on teamwork and/or performance at some other point in between or at a follow-up point in time (although it is worth noting that relatively few studies actually did this). This was done in order to minimize heterogeneity within the meta-analysis and improve the interpretability of the results (i.e., determining the effects of teamwork training from pre- to post-intervention). However, by not taking these measurement time-points into consideration, two questions in particular are raised. First, do certain dimensions of teamwork and team performance evolve differently over time and, if so, how? For instance, do improvements in teamwork occur immediately in response to training and then plateau; or do they improve in a slower, more linear fashion from the onset of training? Second, what are the long-term implications of teamwork training? That is, does teamwork training result in sustained improvements in teamwork and team performance beyond the intervention period or do these effects eventually wane? Answers to these types of research questions would certainly be of interest to teamwork researchers and applied practitioners.
Future Directions
In addition to summarizing the previous research on teamwork interventions for improving teamwork and team performance, the findings from this systematic review also highlight several potential avenues of future research. First, with regard to sample characteristics, the majority of studies that examined the effects of teamwork interventions on team performance were conducted within laboratory settings, with relatively fewer controlled studies having been conducted in real-world settings. Thus, although significant effects on team performance (and teamwork) were found in health care, aviation, military, and academic settings, the extant literature would be strengthened by conducting further controlled intervention research within these contexts. It was also shown that teamwork training was less effective for improving teamwork for intact teams compared to new teams. Since many teams seeking teamwork training are likely to be intact, it is important that future research continue to test various training strategies that can be utilized with these types of teams. In addition, there are other contexts in which controlled interventions have not yet been conducted such as with police squads, firefighting crews, sports teams, political parties, and so on. Research in these areas is clearly ripe for future inquiry.
Further research on the ideal combination of teamwork dimensions (i.e., preparation and/or execution and/or reflection and/or interpersonal dynamics) targeted in an intervention would also enhance our current knowledge in terms of how to train teamwork most effectively and efficiently. We had originally planned to further assess this moderator by conducting a method co-occurrence analysis [ 81 ]. Specifically, since there would likely be a variety of combinations of dimensions that were targeted in the teamwork interventions (e.g., preparation only; preparation and execution; preparation, execution, reflection, and interpersonal dynamics; etc), we had hoped to examine if there would be differential effects of these combinations with regard to intervention effectiveness. Unfortunately, since there were such a large number of combinations of dimensions targeted in the included studies, there was an insufficient number of interventions that fell into each category. We were, therefore, unable to pursue this method co-occurrence analysis [ 81 ] of the various combinations of dimensions. Thus, although our findings suggest that interventions are more effective when two or more dimensions are targeted, further research that examines the effects of the ideal combinations of these dimensions would certainly enhance our current knowledge of teamwork training. For example, if the objective of teamwork training is to improve the coordination and cooperation of the team, should the training also target (in addition to targeting these execution behaviors) both the preparation and reflection dimensions of training (or simply one or the other)? Answering such complex questions will help to advance our understanding of what makes for an effective teamwork training program.
Balanced against the contributions and insights provided by the various moderator analyses conducted in this study, the overall take-home message is that teamwork training is an effective way to foster teamwork and team performance. These effects appear to be evident across a range of samples, utilizing numerous intervention methods, and when considering various measurement characteristics. Interventions appear to be particularly effective when they target multiple dimensions of teamwork and include experiential activities for team members to actively learn about, practise, and continually develop teamwork.
Supporting Information
S1 table. summaries of interventions..
Summaries of each study and intervention included in the meta-analysis is provided in the S1 Table.
https://doi.org/10.1371/journal.pone.0169604.s001
S1 File. PRISMA Checklist.
The Preferred Reporting Items for Systematic Reviews and Meta-Analyses (PRISMA) Checklist [ 82 ] for this review is presented in the S1 File.
https://doi.org/10.1371/journal.pone.0169604.s002
Author Contributions
- Conceptualization: DM ME BZ MB.
- Data curation: DM.
- Formal analysis: DM.
- Investigation: DM GR.
- Methodology: DM MB.
- Project administration: DM MB.
- Resources: DM MB.
- Supervision: MB.
- Validation: DM GR MB.
- Visualization: DM GR ME BZ MB.
- Writing – original draft: DM MB.
- Writing – review & editing: DM GR ME BZ MB.
- View Article
- Google Scholar
- PubMed/NCBI
- 6. Padmo Putri DA. The effect of communication strategy and planning intervention on the processes and performance of course material development teams [dissertation]. Tallahassee (FL): Florida State University; 2012.
- 7. Jankouskas TS. Crisis Resource Management training: Impact on team process and team effectiveness [dissertation]. State College (PA): Pennsylvania State University; 2012
- 9. Bjornberg NH. Mutual performance monitoring in virtual teams [dissertation]. Norfolk, VA: Old Dominion University; 2014
- 11. Lewin K. A dynamic theory of personality. New York, NY: McGraw-Hill; 1935.
- 17. Hackman JR, Katz N. Group behavior and performance. In: Fiske TE, Gilbert DT, Lindzey G, editors. Handbook of social psychology (Vol 2., 5th ed.). West Sussex, UK John Wiley & Sons; 2010. pp. 1251–1280
- 18. Argote L, McGrath JE. Group processes in organizations: Continuity and change. In Cooper CL, Robertson IT, editors. International review of industrial and organizational psychology. Chichester, UK: Wiley;1993. pp. 333–389
- 31. Higgins JP, Green S. Cochrane handbook for systematic reviews of interventions (Vol. 5.1.0). The Cochrane Collaboration. 2008. Available from www.cochrane-handbook.org .
- 33. Borenstein M, Hedges L, Higgins JPT, Rothstein HR. Comprehensive meta-analysis (2nd Ed.). Englewood, NJ: Biostat;2005.
- 34. Borenstein M, Hedges L, Higgins JPT, Rothstein HR. Introduction to meta-analysis. West Sussex, UK: John Wiley & Sons;2009
- 41. Beck-Jones JJ. The effect of cross-training and role assignment in cooperative learning groups on task performance, knowledge of accounting concepts, teamwork behavior, and acquisition of interpositional knowledge [dissertation]. Tallahassee (FL): Florida State University; 2004
- 47. Dibble R. Collaboration for the common good: An examination of internal and external adjustment [dissertation]. Irvine (CA): University of California; 2010.
- 49. Emmert MC. Pilot test of an innovative interprofessional education assessment strategy [doctoral dissertation]. Los Angeles (CA): University of California; 2011.
- 52. Green LR. The effectiveness of tactical adaptation and coordination training on team performance in tactical scenarios. Naval Postgraduate School Monterey CA; 1994 Jun.
- 53. Kim LY. The effects of simulation-based TeamSTEPPS interprofessional communication and teamwork training on patient and provider outcomes [dissertation]. Los Angeles (CA): University of California; 2014.
- 68. Ikomi PA, Boehm-Davis DA, Holt RW, Incalcaterra KA. Jump seat observations of advanced crew resource management (ACRM) effectiveness. In Proceedings of the 10th International Symposium on Aviation Psychology 1999 (Vol. 5, pp. 3–1999).
- 69. Jarrett S. The comparative effectiveness of after-action reviews in co-located and distributed team training environments [dissertation]. College Station (TX): Texas A&M University; 2012.
- 70. Kring JP. Communication modality and after action review performance in a distributed immersive virtual environment [dissertation]. Orlando (FL): University of Central Florida; 2005
- 72. Schurig IA. An investigation of the effect of after-action reviews on teams’ performance-efficacy relationships [dissertation]. College Station (TX): Texas A&M University; 2013.
- 78. The Joint Commission. Health care at the crossroads: Strategies for improving the medical liability system and preventing patient injury. 2005. Available from http://www.jointcommission.org/assets/1/18/Medical_Liability.pdf
SYSTEMATIC REVIEW article
Analyzing the impact of team-building interventions on team cohesion in sports teams: a meta-analysis study.
- Department of Physical Education, Yonsei University, Seoul, Republic of Korea
Introduction: Participation in team sports requires collaboration among multiple individuals over an extended period. Success in the game relies on more than just individual excellence; it necessitates effective teamwork. Team-building interventions have been shown to enhance team functioning, particularly in fostering cohesion among sports teams. This study aims to identify crucial factors in team-building interventions that contribute to improved team cohesion in sports teams.
Methods: A comprehensive meta-analysis of 15 articles was conducted to identify the crucial factors in team-building interventions that contribute to improved team cohesion in sports teams. The analysis focused on the age of participants, level of performance, and duration of interventions.
Results: The results of the analysis revealed that the positive impact of team-building was found to be most pronounced when the participants were between 15 and 20 years old, performed at collegiate teams, and engaged in interventions lasting more than 2 weeks. Among the four types of cohesion in sports teams, individual attraction to the group task (ATG-T) emerged as the aspect most influenced by team-building interventions.
Discussion: These findings provide valuable insights into the factors influencing the success of team-building interventions in enhancing team cohesion within sports teams.
1 Introduction
Psychological interventions in sports have proven effective in enhancing athletes’ skill development, team cohesion, and team performance. Among these interventions, team-building has emerged as a prominent strategy for promoting effective collaboration among team members, thereby strengthening cohesion and team performance in sports teams. This approach has been employed to optimize the functionality of sports teams, resulting in improved team performance.
This study aims to explore the impact of team-building interventions on cohesion within sports teams. While numerous investigations have reported favorable effects of team-building on team cohesion ( Cogan and Petrie, 1995 ; Prapavessis et al., 1996 ; Stevens and Bloom, 2003 ; Senécal et al., 2008 ; Kim and Kim, 2012 ; Durdubas and Koruc, 2023 ; Tassi et al., 2023 ), it remains challenging to assert that team-building interventions yield effective results. Some studies, such as those by Bloom and Stevens (2002) , Kilty (2000) , Kwon (2022) , Prapavessis et al. (1996) , and Rainey and Schweickert (1988) , did not report positive developments in group cohesion.
Moreover, improvements in cohesion achieved through team-building interventions were sometimes transient, with studies indicating that cohesion levels were not sustained throughout the season ( Cogan and Petrie, 1995 ; Stevens and Bloom, 2003 ). Drawing definitive findings about the effectiveness of team-building in sports is complicated by the diversity of methods and designs employed in these interventions, which yield unexpected results and necessitate an integrated examination of previous studies.
In the meta-analysis conducted by Carron et al. (2002) , the impact of team-building on four subgroups of cohesion – GI-T (group integration–task), GI-S (group integration–social), ATG-T (individual attractions to the group-task), and ATG-S (individual attractions to the group-social) – was examined, with reported effect sizes of 0.471, 0.349, 0.676, and 0.463. Martin et al. (2009) conducted a meta-analysis on team-building interventions within sports teams, reporting an effect size of 0.427. Their analysis revealed that team-building interventions had the most substantial impact on cognitions ( g = 0.799), with goal setting as the exclusive method coming in second ( g = 0.714). The effect sizes of task and social cohesion were 0.263 and 0.214.
While team-building is known to have a positive effect on team cohesion, in actual application, its implementation time is limited. Therefore, to ensure that the cohesion effect is evident in sports teams, understanding the factors that should be considered in team-building interventions is crucial. This study seeks to determine which moderator variables such as gender, age, athletes’ level, group size, and intervention duration, enhance the effect and which factors do not need to be considered.
2 Methodology
This methodology conforms to the relevant guidelines of the Preferred Reporting Items of Systematic Reviews and Meta-Analyses (PRISMA) Statement and ensures that the necessary scientific information is provided in the field ( Page et al., 2021 ).
2.1 Study selection and inclusion criteria
For this meta-analysis, literature selection focused on research studies examining the effectiveness of team-building interventions in interactive sport teams. The selection process followed rigorous and systematic procedures, incorporating keyword searches in computerized databases and employing a snowball sampling approach.
The computer-based search covered various databases, including PsychINFO, PsycARTICLES, SPORT Discus, and Google Scholar. This comprehensive search strategy involved using a range of keywords, such as “team-building in sport,” “team-building intervention in sport,” “team-building and cohesion,” and various combinations.
Two independent reviewers extracted the following data from each article: study design, total number of participants, gender, age, intervention duration, and athletes’ skill level. The accuracy of the extracted or calculated data was verified by comparing the data collection forms of the two investigators.
2.2 Dependent variables: cohesion
Team-building in sports teams can yield various outcomes, including enhanced cohesion. Carron and Spink (1993) developed a conceptual framework for team-building interventions in sports teams, designating group cohesiveness as the primary result of this process. Within this framework, four subgroups of cohesion, specifically GI-T, GI-S, ATG-T, and ATG-S, serve as dependent variables when assessing the impact of team-building interventions ( Eys and Kim, 2017 ).
2.3 Moderating factors
2.3.1 gender.
Within the studies under review, two distinct demographic cohorts were examined. Specifically, 5 studies with 38 cases focused on male participants, while 10 studies with 14 cases centered around female participants.
2.3.2 Age of participants
The participants’ ages were divided into three groups: under the age of 15, 15–20 years old, and over 20. Specifically, two studies with eight cases focused on participants under 15, while nine studies with 29 cases targeted the 15–20 age group, and four studies with 15 cases focused on participants over the age of 20.
2.3.3 Sample size
The sample size was categorized into three groups: under 20 participants, 20–30 participants, and over 30 participants. More specifically, five studies with 17 cases were centered on under 20 participants, while another five studies with 18 cases were aimed at the 20–30 participants group. Additionally, five studies with 17 cases were focused on participants comprising over 30 participants.
2.3.4 Skill level
The analysis covered a range of team proficiency levels. High school and collegiate teams were each represented in five studies with 16 effect sizes, whereas professional club teams were featured in five studies with 20 effect sizes.
2.3.5 Length of intervention
This study also investigated the duration of a team-building intervention as a potential moderator for their effectiveness. The intervention durations were classified into three groups: less than 2 weeks, 2 to 20 weeks, and 20 weeks or more. There were 8 studies with 32 cases that fell within the 2 to 20 weeks category, while 6 studies with 18 cases had intervention lasting over 20 weeks. Additionally, one study with two cases had an intervention duration of less than 2 weeks.
2.4 Coding methodology
Following established norms for meta-analytic research, we meticulously designed our coding procedure to thoughtfully capture and quantify crucial study characteristics and outcomes. Our comprehensive coding approach involved systematically extracting 11 essential pieces of information from each study. This included details such as authorship, year of publication, study setting, study design type, sport type, duration of intervention, athletes’ skill level, gender of participants, number of participants in experimental and control groups, means and standard deviations of intervention effectiveness at pretest and posttest, as well as effect size or measures of effectiveness.
2.5 Effect size calculations
The computation of effect sizes was conducted using R-4.3.2 for Windows. 1 This program provides various options for calculating effect sizes, and we chose Hedges g ( Hedges and Olkin, 2014 ), an effect size adjusted to consider differences in sample size and sample variance. In interpreting the magnitude of effect sizes, we followed Cohen’s (1988) guidelines. Specifically, a Hedges g of 0.80 was considered a large effect size, 0.50 signified a medium effect size, and 0.20 indicated a small effect size.
3.1 Study selection
Following a database search, a total of 1,928 documents were initially identified, with 35 documents found through snowballing methods. After removing duplicates, 1,752 articles remained. Subsequently, 525 articles were excluded based on title screening. Application of the inclusion criteria led to the exclusion of an additional 664 articles. This left us with 121 articles that underwent full-text screening, focusing on articles potentially relevant to the impact of team-building interventions on cohesion in sports teams. To ensure methodological rigor, studies lacking the necessary statistical information for calculating effect sizes were excluded from the meta-analysis. Following these criteria, a total of 15 studies, comprising 52 cases, were considered eligible for inclusion in the meta-analysis (refer to Figure 1 for details).
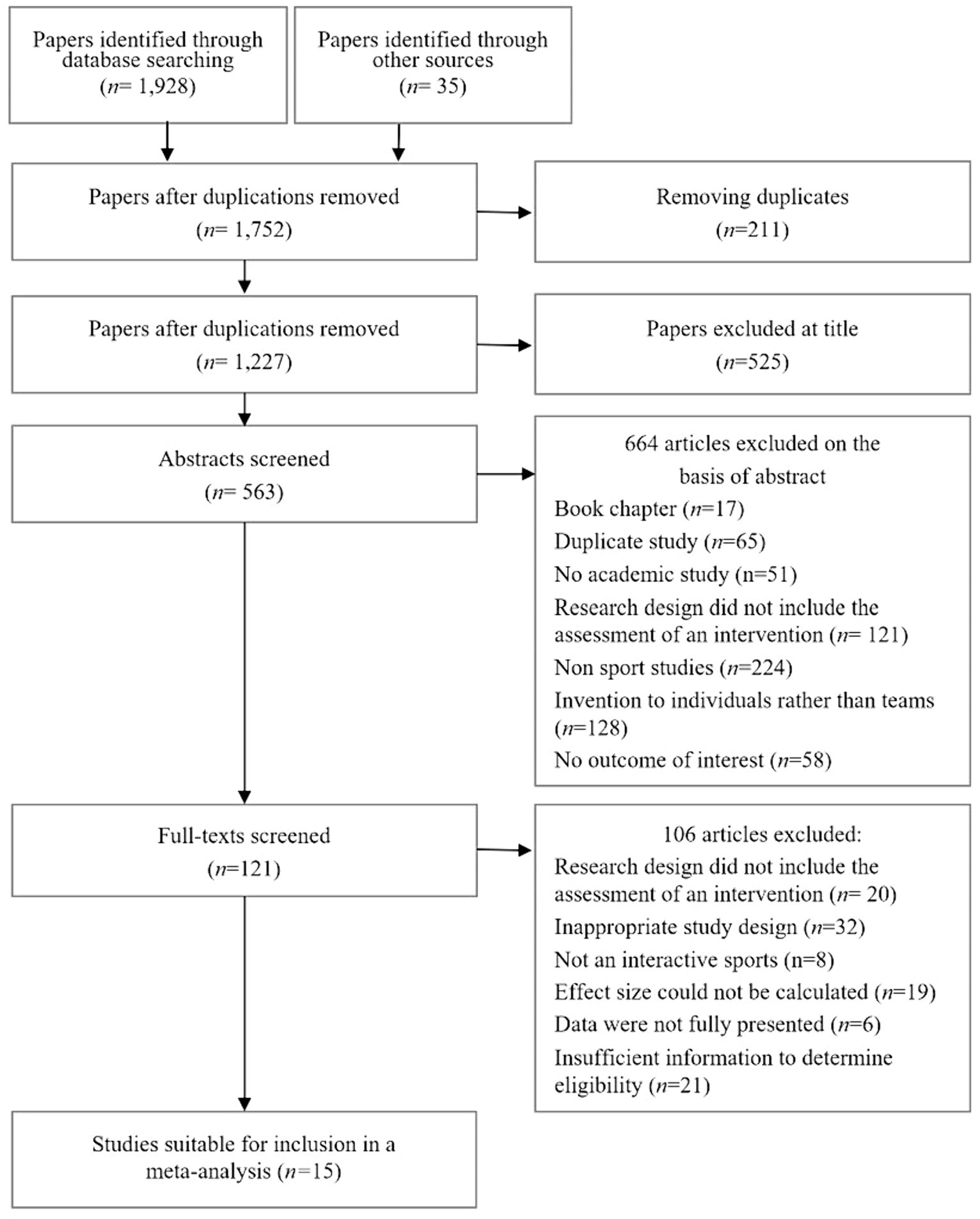
Figure 1 . Flowchart of the systematic review process according to the PRISMA protocol declarations.
3.2 Assessment of risk of bias
To assess the risk of bias in the included articles, we used the Cochrane Risk of Bias Tool ( Higgins and Altman, 2008 ). This tool assesses each article based on a checklist comprising five items: randomization process, deviation from the intended intervention, missing outcome data, measurement of the outcome, and selection of the reported result. We then categorized each article’s overall bias risk as low risk (indicating low risk across all items), some concerns, and high risk (indicating high risk of bias in at least one domain). Low risk indicates better methodological quality, while high risk suggests a high risk of bias.
Figure 2 provides a visual representation of risk of bias evaluations for each domain of the Cochrane Risk of Bias tool. Out of all included articles, 1 article (6.7%) had a low overall risk of bias, while 14 articles (93.3%) exhibited a high overall risk of bias. However, except for the randomization process domain, the other four checklist items showed low risk across all 15 articles.
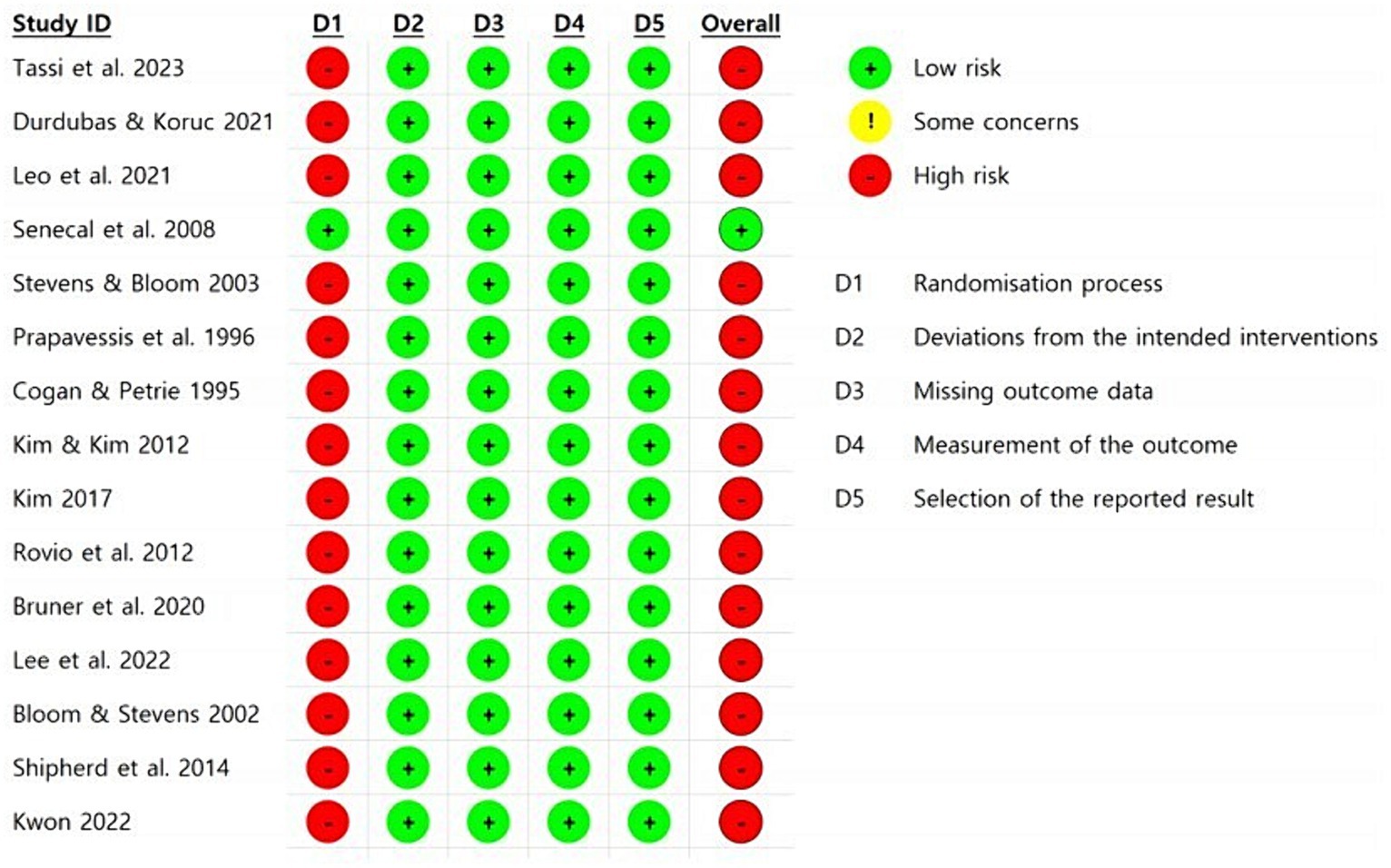
Figure 2 . Assessment of risk of bias in the included studies.
The high prevalence of ‘high’ risk is attributed to the inherent challenges in randomly selecting teams, particularly in studies involving interactive sports teams. This difficulty arises from the complexities associated with randomly assigning teams in research focused on sports team dynamics.
3.3 Overall analysis
3.3.1 overall effect size.
The meta-analysis results, drawn from 52 individual cases extracted from 15 papers, are presented in Table 1 . The table covers both the overall analysis and outcomes related to five moderating variables influencing cohesion. Additionally, Figure 3 illustrates a forest plot depicting effect sizes for the 52 individual cases. The overall analysis of these cases showed a significant moderate effect size (ES = 0.65, 95% CI = [0.40; 0.91]) of team-building intervention on cohesion. Additionally, the I 2 heterogeneity statistic indicated a significant level of heterogeneity at 96.9%.
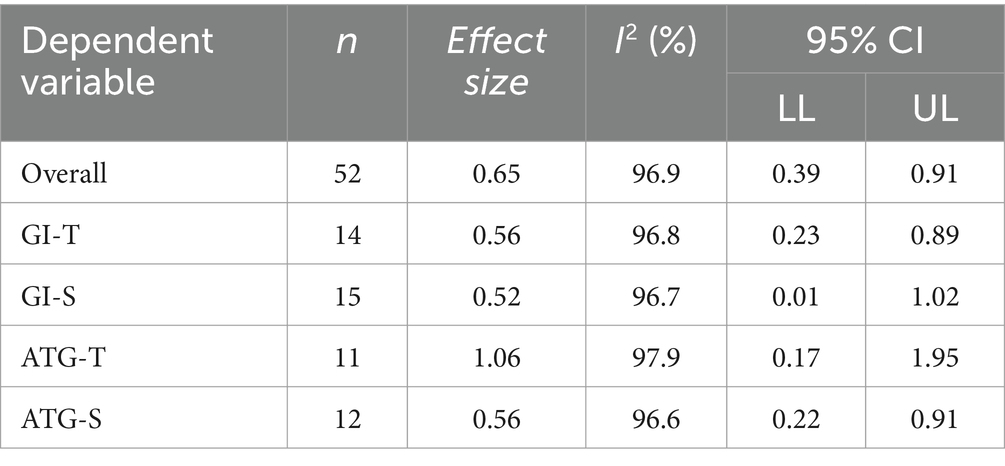
Table 1 . Effect sizes of dependent variables.
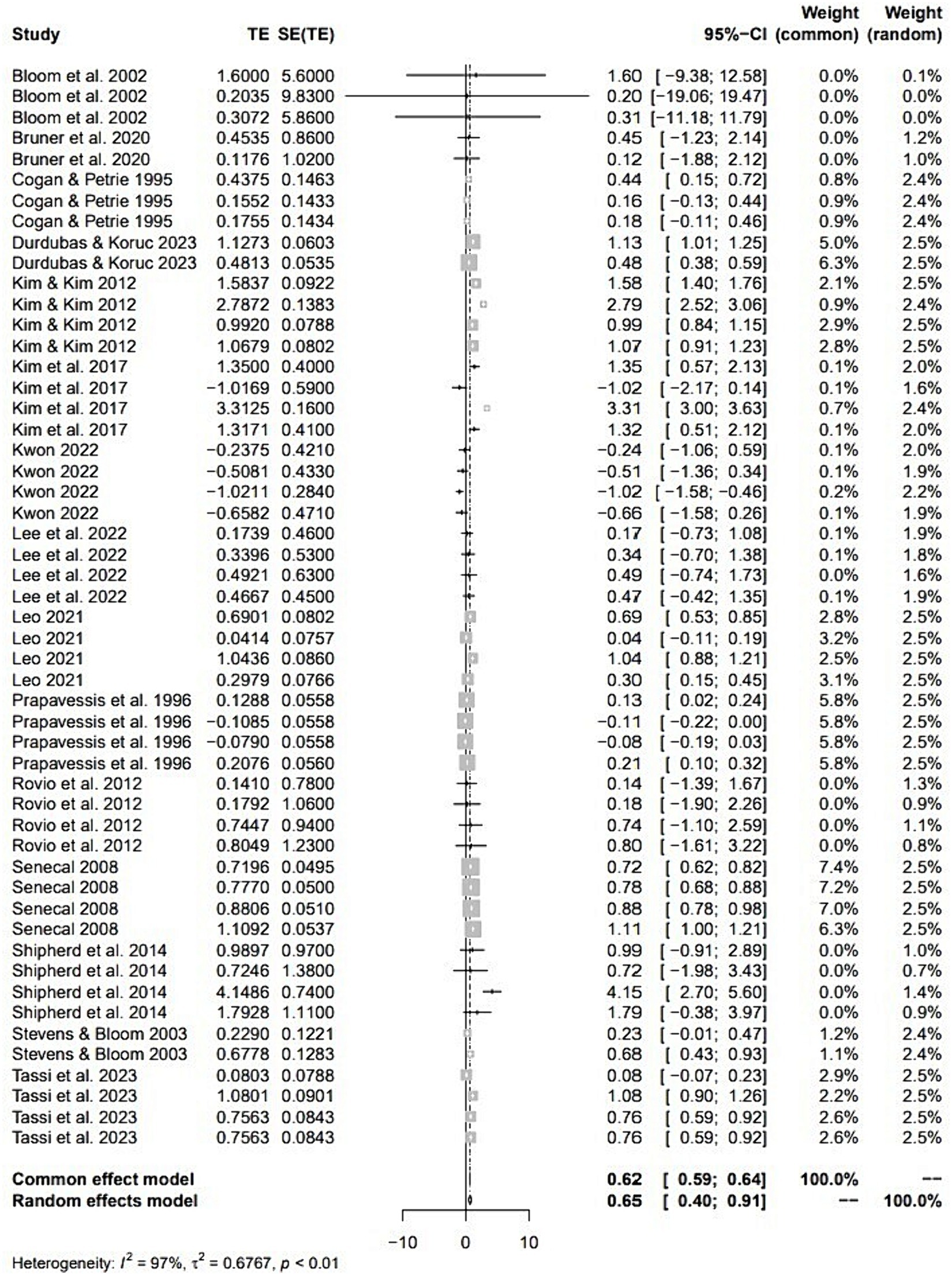
Figure 3 . Forest plot of meta-analysis for team building intervention on cohesion in sports teams. The individual effect sizes are identified as Hedges g with lower and upper limits of 95% CIs.
3.3.2 Publication bias
To assess the potential presence of publication bias in our meta-analysis of team-building intervention on cohesion, we utilized a funnel plot for visual examination, as illustrated in Figure 4 . In an ideal scenario without publication bias, data points (depicted as solid circles) from individual case studies would exhibit a symmetrical distribution. Any deviation from this symmetry suggests the potential presence of publication bias. As seen in Figure 4 , the distribution of effect sizes is slightly left–skewed.
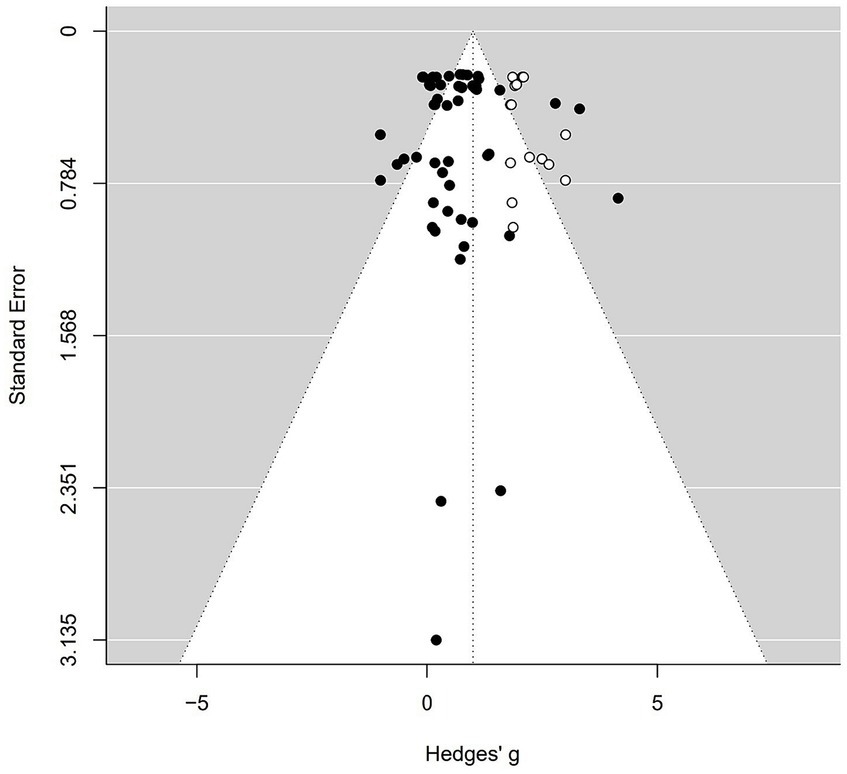
Figure 4 . Funnel plot of standard error by Hedges g.
Applying the trim-and-fill method by Duval and Tweedie (2000) reveals that 15 missing studies on the right side are required to achieve symmetry in the funnel plot. The required 15 additional cases are shown on the right as hollow circles in Figure 4 .
We also assessed publication bias using Rosenthal’s (1979) fail-safe N (N fs ) concept. When N fs exceeds 5 k + 10, where k represents the number of included case studies, it is unlikely to substantially impact the average effect size. In our specific study, with k equal to 52, the meta-analysis results remain stable as long as the N fs exceeds 270. Our N fs value is 2,570, well above the 270 threshold, emphasizing the robustness of the meta-analysis. In simpler terms, even if more than 2,570 studies with zero effect size were introduced, the overall results would remain largely unaltered.
According to the trim-and-fill method by Duval and Tweedie (2000) , an adjusted effect size of 1.00 (95% CI = [0.75; 1.25]), larger than the calculated effect size of 0.65, is presented.
3.4 Type of cohesion measure
Table 1 presents 52 effect sizes calculated for four cohesion types (GI-T, GI-S, ATG-T, and ATG-S). Notably, task cohesion exhibited a larger effect size than social cohesion. ATG-T showed a significant large effect size (ES = 1.06, 95% CI = [0.17; 1.95]). The other three cohesion types, GI-T (ES = 0.56, 95% CI = [0.23; 0.89]), ATG-S (ES = 0.56, 95% CI = [0.22; 0.91]), and GI-S (ES = 0.52, 95% CI = [0.01; 1.02]), showed a moderate effect size. According to meta-ANOVA, the differences between the four cohesion types were not statistically significant ( F (3, 48) = 1.312, p > 0.05).
3.5 Moderator variables
This study examined the effectiveness of team-building concerning five different moderators. These moderators encompassed the effectiveness of team-building on cohesion across gender, age, sample size, intervention duration, and athletes’ skill level. Notably, the only significant moderator identified was athletes’ skill level. No statistically significant differences were observed within the other four moderators (refer to Table 2 for details).
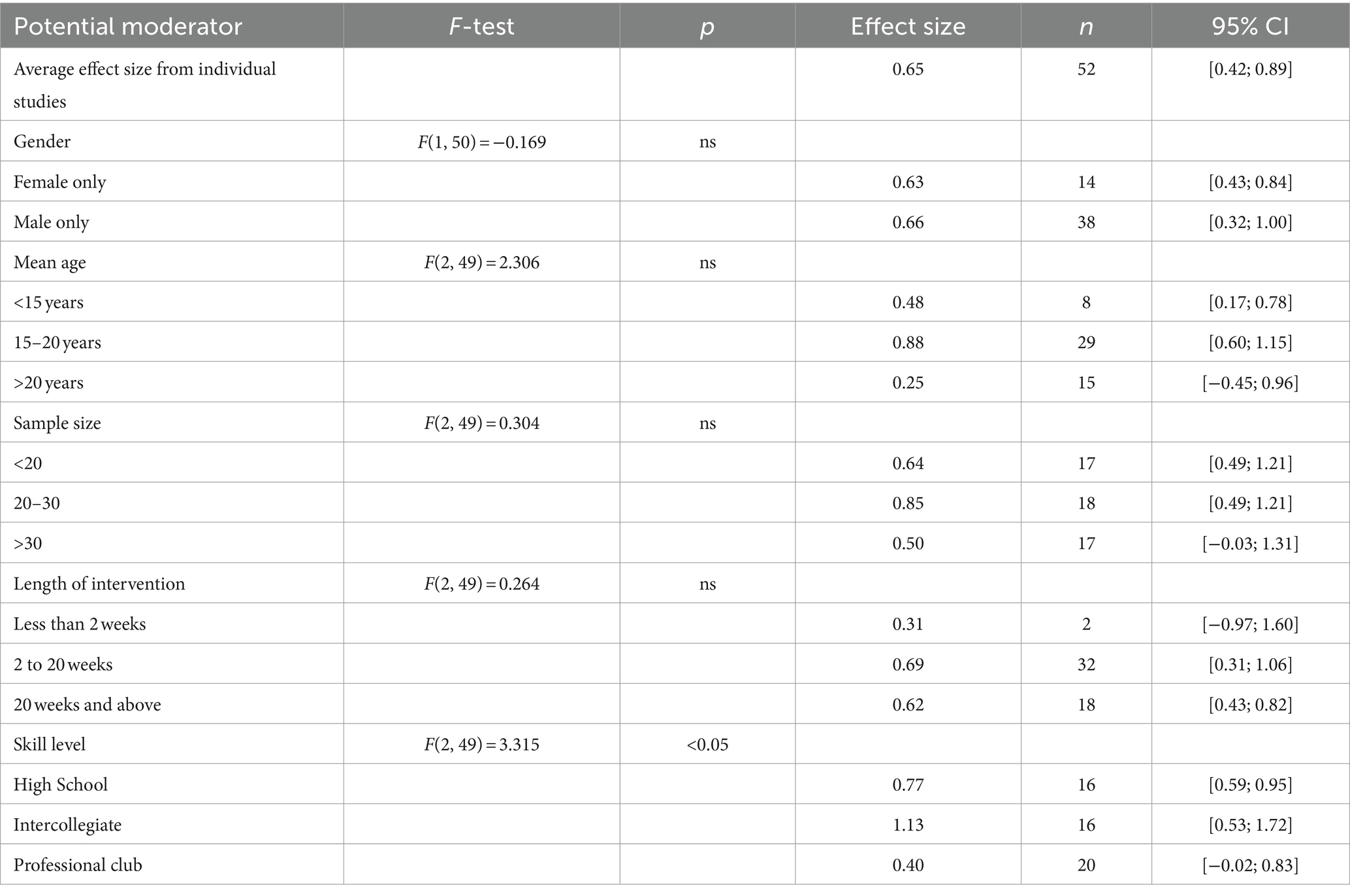
Table 2 . Moderator effects.
3.5.1 Gender
As indicated in Table 2 , there is a slightly larger effect size for male athlete teams (ES = 0.66, 95% CI = [0.32; 1.00]) compared to female athlete teams (ES = 0.63, 95% CI = [0.43; 0.84]). However, the difference is not statistically significant ( p > 0.05).
We categorized the ages of the participants into three groups. In the 15–20 years old category, we observed a significant large effect size (ES = 0.88, 95% CI = [0.60; 1.15]), while those under the age of 15 showed a significant moderate effect size (ES = 0.48, 95% CI = [0.17; 0.78]). However, the effect size (ES = 0.25, 95% CI = [−0.45; 0.96]) for those over the age of 20 was not statistically significant. The meta-ANOVA analysis indicated that the difference between these three categories was not statistically significant ( p > 0.05). Consequently, age was not identified as a significant moderator in this study.
3.5.3 Sample size
The sample size was divided into three groups. In the category with 20–30 participants, we observed a significant large effect size (ES = 0.85, 95% CI = [0.49; 1.21]). Additionally, the category with under 20 participants showed a significant moderate effect size (ES = 0.64, 95% CI = [0.49; 1.21]). However, the effect size (ES = 0.50, 95% CI = [−0.03; 1.31]) for those over 30 participants was not statistically significant. The meta-ANOVA analysis indicated that the difference between these three categories was not statistically significant ( p > 0.05). Consequently, the sample size was not identified as a significant moderator in this study.
3.5.4 Length of intervention
The team-building interventions in our study varied in duration, ranging from 1 day to the entire sports season. As shown in Table 2 , a significant moderate effect size (ES = 0.69, 95% CI = [0.31; 1.06]) was observed for interventions lasting between 2 and 20 weeks. Additionally, a significant moderate effect size was evident for interventions extending for 20 weeks or longer (ES = 0.62, 95% CI = [0.43; 0.82]). However, the effect size (ES = 0.31, 95% CI = [−0.97; 1.60]) for intervention durations less than 2 weeks was not statistically significant. The meta-ANOVA analysis indicated that the difference between these three categories was not statistically significant ( p > 0.05). Consequently, the length of intervention was not identified as a significant moderator in this study.
3.5.5 Skill level of the athletes
As outlined in Table 2 , we observed a significant large effect size (ES = 1.13, 95% CI = [0.53; 1.72]) in the category of collegiate teams, while we identified a significant moderate effect size (ES = 0.77, 95% CI = [0.59; 0.95]) in the category of high school teams. However, the effect size (ES = 0.40, 95% CI = [−0.02; 0.83]) was not statistically significant for professional teams.
According to the meta ANOVA and post-hoc test results, significant differences ( p < 0.05) in the effectiveness of team-building on cohesion were found between collegiate teams and professional teams. Consequently, athletes’ skill level can act as a moderator in the effectiveness of team-building intervention on cohesion.
3.6 Meta-regression analysis
We conducted meta-regression analyses to explore the association between three independent variables (age, sample size, and duration in weeks) and the effect size. The results of meta-regression analysis showed that the effect size tend to decrease with mean age, although this association did not reach statistical significance ( p > 0.05) (refer to Figure 5 and Table 3 ). Furthermore, the relationships between sample size and effect sizes, as well as the relationship between duration in weeks and effect sizes, did not show statistical significance ( p > 0.05) (refer to Table 3 ).
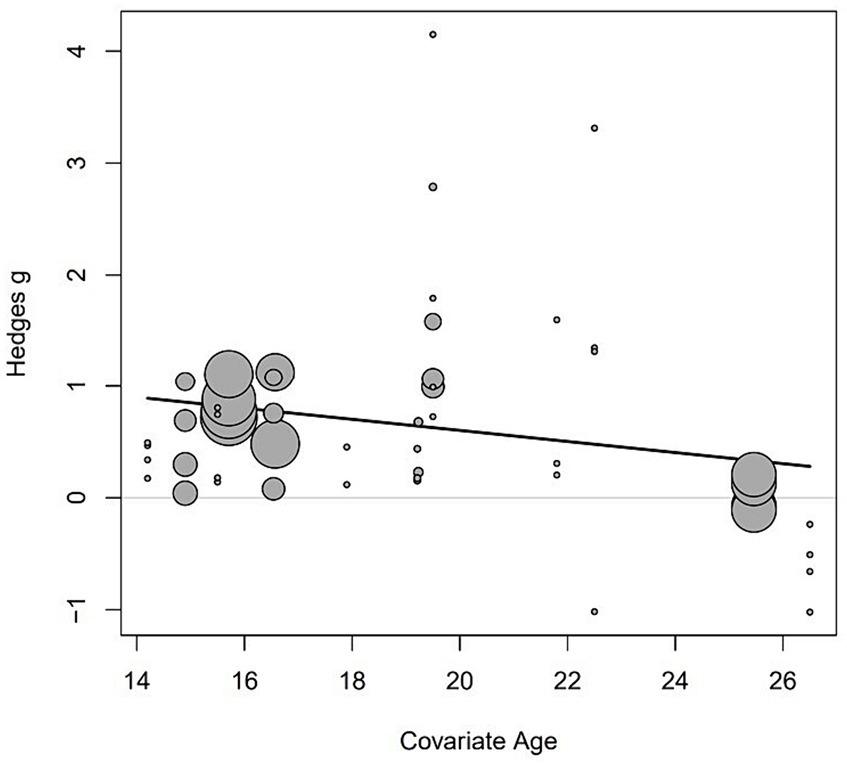
Figure 5 . Meta-regression analysis of the relationship between Hedges’ g and the mean age of participants.
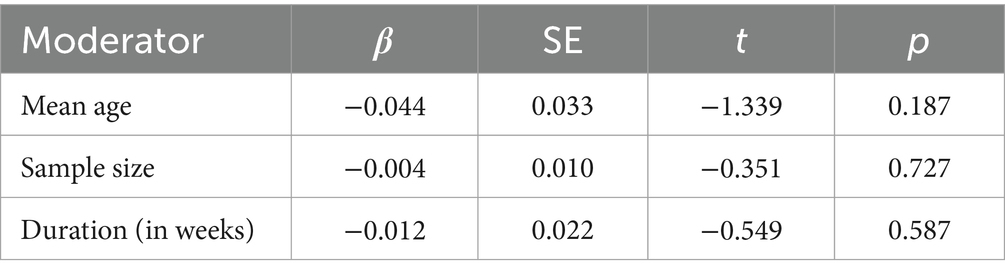
Table 3 . Univariate meta-regression analysis.
4 Discussion
The main goal of this meta-analysis is to assess the impact of team-building interventions on cohesion, a critical element in sports teams that plays a pivotal role in task execution and fostering social interactions ( Carron and Spink, 1993 ; Carron et al., 1997 ). If team-building interventions focused on fostering cohesion can establish a sense of unity among team members, they have the potential to serve as catalysts for enhancing overall team performance.
Our study’s key finding is that team-building activities indeed improve cohesion in sports teams. Among various measures of cohesion, we found that team-building interventions were most successful in enhancing ATG-T, followed by GI-T, GI-S, and ATG-S. Some team-building activities focus on social aspects, like team camping trips ( Cogan and Petrie, 1995 ), ropes and challenge courses (e.g., Meyer, 2000 ), and informal social gatherings (e.g., Yukelson, 1997 ). These activities are likely to enhance social cohesion within the team. On the other hand, other team-building activities concentrated on team goals and tasks, such as team goal-setting (e.g., Senécal et al., 2008 ; Kim et al., 2017 ; Durdubas and Koruc, 2023 ), tasks relevant to team performance (e.g., Leo et al., 2021 ), clarifying roles (e.g., Tassi et al., 2023 ), and adhering to team norms (e.g., Prapavessis et al., 1996 ). These activities are expected to primarily improve task cohesion within the team. Notably, our analysis revealed a stronger impact of team-building activities on task cohesion compared to social cohesion due to the predominant focus on tasks and objectives rather than social interactions in the studies examined.
Another aim of our study is to explore how various moderator variables affect the improvement of cohesion through team-building intervention. Several findings are associated with the influence of moderators. To begin with, we explored gender as a potential moderator. The findings indicate that team-building interventions are equally effective for teams composed solely of females as well as those with only males. In our meta-analysis, using gender served as a potential moderator, the results of the meta t -test showed no significant difference ( p > 0.05) in the effectiveness of team-building interventions applied to both men’s and women’s teams. This aligns with the results reported by Martin et al. (2009) .
In this study, the second potential modulator under scrutiny was the age of participants. We categorized subjects of individual study into three age groups, and then the effect size was calculated with age as a moderate variable. We found that the age category of 15–20 exhibited a large effect size, while the category under 15 years old showed a significant moderate effect size. However, there was no significant effect size observed for the category of those aged over 20 years. Consequently, we can conclude that team-building is most effective for sports teams with members between 15 and 20 years old, while it does not show effectiveness for sports teams with members aged over 20.
In our analysis, the third potential modulator we explored was sample size. We classified the sample size of each study into three groups, and then the effect size was calculated with sample size as a moderate variable. In the group with 20–30 participants, a significant large effect size was observed, while the category with under 20 participants showed a significant moderate effect size. However, there was no significant effect size observed for the category of those with over 30 participants. As a result, we can conclude that team-building is most effective for sports teams ranging from 20 to 30 members, while it does not show effectiveness for sports groups with over 30 members.
In our analysis, the fourth potential modulator we explored was athletes’ skill level, which turned out to be the only significant moderator in this study. Team-building interventions were most effective for collegiate teams, followed by high school teams, while the effectiveness in professional teams did not reach statistical significance ( p > 0.05). This discrepancy may be explained by a potential ceiling effect, given that professional athletes typically possess a strong understanding of cohesion. Consequently, while professional teams do benefit from team-building interventions, the extent of improvement may be comparatively modest due to their already robust cohesion and training. The meta-ANOVA indicated that the differences between the three groups were statistically significant ( p < 0.05), and the post-hoc test revealed that the effect size of the collegiate team was larger than that of the professional club team. Thus, it can be concluded that team-building is most effective for collegiate sports teams, while it does not show effectiveness for professional club teams.
Moving on to the fifth potential modulator, we explored intervention duration. The articles in this meta-analysis encompassed team-building interventions with durations ranging from a single day to an entire sports season. Notably, interventions lasting less than 2 weeks did not yield noticeable improvements in cohesion and were not statistically significant, aligning with the findings of Martin et al. (2009) . Conversely, Shipherd et al. (2014) conducted a single-day team-building intervention with a collegiate rugby team and observed a significant increase in team cohesion. These disparities in intervention duration underscore the need for meta-analytic investigations to gain a comprehensive understanding of the optimal duration required for team-building interventions to enhance cohesion in future studies.
Although numerous studies have demonstrated the positive effects of team-building interventions on cohesion, there are instances, as seen in some studies ( Prapavessis et al., 1996 ; Kwon, 2022 ), where significant improvements were not observed. The intervention period might have impacted why there wasn’t a significant change in group cohesion after the team-building program was implemented. Kwon (2022) and Prapavessis et al. (1996) conducted a team-building intervention over 8 weeks but did not find a clear improvement in group cohesion. This suggests that the intervention duration might have been too short to see significant differences in these studies. Group cohesion improves gradually through changing members’ perceptions and resolving conflicts that arise during interactions. Therefore, steady progress over a long enough time is important. However, conducting long-term team-building interventions can be challenging due to various environmental factors.
5 Conclusion
In conclusion, this study provides several key insights into the impact of team-building intervention on cohesion within sports teams. Firstly, team-building activities predominantly enhance task cohesion rather than social cohesion within sports teams. Different approaches to team-building, focusing on either social interactions or team goals and tasks, result in corresponding improvements in cohesion. Thus, social cohesion benefits from team-building activities emphasizing social interaction, while task cohesion improves when activities concentrate on team objectives.
Secondly, team-building interventions are most effective for individuals aged 15–20 and within collegiate sports teams. Conversely, the expected positive effects may not be noticeable when subjects are over 20 years old and belong to professional league teams.
Thirdly, interventions lasting longer than 2 weeks are crucial for enhancing team cohesion. Conversely, the expected positive effects may not be noticeable if the intervention period is less than 2 weeks. Based on our findings, an intervention period of at least 2 weeks is necessary to see the effects of a team-building intervention on group cohesion in sports teams. However, it is not necessarily the case that a longer intervention period will result in a greater intervention effect. Additionally, the time delay of the intervention was not investigated in this study. Therefore, the association between the team-building intervention period and group cohesion remains unclear. Further research is needed to determine the optimal intervention period that significantly affects group cohesion. It is also important to consider the time delay of intervention. Furthermore, there is possibility that a group cohesion may be influenced by multiple processes rather than just team-building alone. Therefore, claiming that team-building alone enhances group cohesion may not be reasonable. Therefore, decision-makers in sports teams should carefully consider the duration and realistic expectations of team-building interventions. In any case, to have an effective team-building intervention, it is necessary to implement the intervention for a long enough period. To address this, leaders should ensure interventions are implemented over a sufficient period to yield meaningful results.
In summary, team-building interventions can significantly enhance cohesion within sports teams, particularly when tailored to specific team dynamics and implemented over a sufficient duration.
Nevertheless, it’s important to note the limitations of this meta-analysis. First and foremost, the study focused exclusively on interactive sports, suggesting the need for future research to explore and compare the effectiveness of team-building interventions in both interactive and coactive sports settings. Secondly, the review concentrated solely on immediate post-intervention effects, emphasizing the necessity for longitudinal studies to gain a more profound understanding of the lasting benefits of team-building interventions for sports teams over an extended period.
Data availability statement
The raw data supporting the conclusions of this article will be made available by the author, without undue reservation.
Author contributions
SK: Data curation, Formal analysis, Investigation, Methodology, Validation, Visualization, Writing – original draft, Writing – review & editing.
The author(s) declare that no financial support was received for the research, authorship, and/or publication of this article.
Conflict of interest
The author declares that the research was conducted in the absence of any commercial or financial relationships that could be construed as a potential conflict of interest.
Publisher’s note
All claims expressed in this article are solely those of the authors and do not necessarily represent those of their affiliated organizations, or those of the publisher, the editors and the reviewers. Any product that may be evaluated in this article, or claim that may be made by its manufacturer, is not guaranteed or endorsed by the publisher.
1. ^ r-project.org
Note: References highlighted by an asterisk (*) were included in the meta-analysis.
Google Scholar
*Bloom, G. A., and Stevens, D. E. (2002). Case study: a team-building mental skills training program with an intercollegiate equestrian team. Athletic Insight J. Sport Psychol. 4, 1–16.
Carron, A. V., Colman, M. M., Wheeler, J., and Stevens, D. (2002). Cohesion and performance in sport: a meta-analysis. J. Sport Exerc. Psychol. 24, 168–188. doi: 10.1123/jsep.24.2.168
Crossref Full Text | Google Scholar
Carron, A. V., and Spink, K. S. (1993). Team building in an exercise setting. Sport Psychol. 7, 8–18. doi: 10.1123/tsp.7.1.8
Carron, A. V., Spink, K. S., and Prapavessis, H. (1997). Team building and cohesiveness in the sport and exercise setting: use of indirect interventions. J. Appl. Sport Psychol. 9, 61–72. doi: 10.1080/10413209708415384
*Cogan, K. D., and Petrie, T. A. (1995). Sport consultation: an evaluation of a season-long intervention with female collegiate gymnasts. Sport Psychol. 9, 282–296, doi: 10.1123/tsp.9.3.282
Cohen, J. (1988). Statistical power analysis for the behavioral sciences (2nd ed.). Hillsdale, NJ: Erlbaum.
*Durdubas, D., and Koruc, Z. (2023). Effects of a multifaceted team goal-setting intervention for youth volleyball teams. J. Appl. Sport Psychol. 35, 224–243, doi: 10.1080/10413200.2021.2021564
Duval, S., and Tweedie, R. (2000). Trim and fill: a simple funnel-plot–based method of testing and adjusting for publication bias in meta-analysis. Biometrics 56, 455–463. doi: 10.1111/j.0006-341X.2000.00455.x
PubMed Abstract | Crossref Full Text | Google Scholar
Eys, M., and Kim, J. (2017). Team building and group cohesion in the context of sport and performance psychology . Oxford Research Encyclopedia of Psychology. Oxford.
Hedges, L. V., and Olkin, I. (2014). Statistical methods for meta-analysis . San Diego, CA, USA: Academic Press.
Higgins, J. P., and Altman, D. G. (2008). Assessing risk of bias in included studies. In: Higgins, JPT , S Green , eds. Cochrane handbook for systematic reviews of interventions . Chichester, UK: Wiley, 187–241.
Kilty, K.M. (2000). A study of cohesion in women’s sports teams using adventure programming . Unpublished doctoral dissertation. Boston University, Boston, United States.
*Kim, Y. S., and Kim, B. J. (2012). The change of team cohesion in a university baseball team by applying team-building intervention. Korean J. Sports Sci. , 23, 334–342.
*Kim, Y., Park, S., and Kim, T. (2017). The development of a team building program for Korean curling team. Int. J. Appl. Sports Sci. 29, 155–168, doi: 10.24985/ijass.2017.29.2.155
*Kwon, S. H. (2022). A team building intervention case study of a male handball team. J. Coach. Dev. 24, 103–112, doi: 10.47684/jcd.2022.03.24.1.103
*Leo, F. M., Gonzalez-Ponce, I., Lopez-Gajardo, M. A., Pulido, J. J., and Garcia-Calvo, T. (2021). Team building intervention program and its relationship with group processes in young athletes. Int. J. Sport Psychol. 52, 120–136.
Martin, L. J., Carron, A. V., and Burke, S. M. (2009). Team building interventions in sport: a meta-analysis. Sport Exerc. Psychol. Rev. 5, 3–18. doi: 10.53841/bpssepr.2009.5.2.3
Meyer, B. B. (2000). The ropes and challenges course: a quasi-experimental examination. Percept. Mot. Skills 90, 1249–1257. doi: 10.2466/pms.2000.90.3c.1249
Page, M. J., McKenzie, J. E., Bossuyt, P. M., Boutron, I., Hoffmann, T. C., Mulrow, C. D., et al. (2021). The PRISMA 2020 statement: an updated guideline for reporting systematic reviews. Int. J. Surg. 88:105906. doi: 10.1016/j.ijsu.2021.105906
*Prapavessis, H., Carron, A. A., and Spink, K. S. (1996). Team building in sport. Int. J. Sport Psychol. , 27, 269–285.
Rainey, D. W., and Schweickert, G. J. (1988). An exploratory study of team cohesion before and after a spring trip. Sport Psychol. 2, 314–317. doi: 10.1123/tsp.2.4.314
Rosenthal, R. (1979). The file drawer problem and tolerance for null results. Psychol. Bull. 86, 638–641. doi: 10.1037/0033-2909.86.3.638
*Senécal, J., Loughead, T. M., and Bloom, G. A. (2008). A season-long team-building intervention: examining the effect of team goal setting on cohesion. J. Sport Exerc. Psychol. , 30, 186–199, doi: 10.1123/jsep.30.2.186
*Shipherd, A. M., Basevitch, I., Renner, K. B., and Siwatu, K. O. (2014). Development and evaluation of a team building intervention with a US collegiate Rugby team: a mixed methods approach. J. Multidiscip. Res. 6, 31–48.
*Stevens, D., and Bloom, G. (2003). The effect of team building on cohesion. Avante Ontario 9, 43–54.
*Tassi, J. M., López-Gajardo, M. A., Leo, F. M., Díaz-García, J., and García-Calvo, T. (2023). An intervention program based on team building during tactical training tasks to improve team functioning. Front. Psychol. , 14,:1065323, doi: 10.3389/fpsyg.2023.1065323
Yukelson, D. (1997). Principles of effective team building interventions in sport: a direct services approach at Penn State University. J. Appl. Sport Psychol. 9, 73–96. doi: 10.1080/10413209708415385
Keywords: group cohesion, group-based intervention, interactive sports, meta-analysis, teambuilding
Citation: Kwon SH (2024) Analyzing the impact of team-building interventions on team cohesion in sports teams: a meta-analysis study. Front. Psychol . 15:1353944. doi: 10.3389/fpsyg.2024.1353944
Received: 11 December 2023; Accepted: 04 March 2024; Published: 15 March 2024.
Reviewed by:
Copyright © 2024 Kwon. This is an open-access article distributed under the terms of the Creative Commons Attribution License (CC BY) . The use, distribution or reproduction in other forums is permitted, provided the original author(s) and the copyright owner(s) are credited and that the original publication in this journal is cited, in accordance with accepted academic practice. No use, distribution or reproduction is permitted which does not comply with these terms.
*Correspondence: Sang Hyun Kwon, [email protected]
Disclaimer: All claims expressed in this article are solely those of the authors and do not necessarily represent those of their affiliated organizations, or those of the publisher, the editors and the reviewers. Any product that may be evaluated in this article or claim that may be made by its manufacturer is not guaranteed or endorsed by the publisher.

An official website of the United States government
The .gov means it’s official. Federal government websites often end in .gov or .mil. Before sharing sensitive information, make sure you’re on a federal government site.
The site is secure. The https:// ensures that you are connecting to the official website and that any information you provide is encrypted and transmitted securely.
- Publications
- Account settings
Preview improvements coming to the PMC website in October 2024. Learn More or Try it out now .
- Advanced Search
- Journal List
- J Clin Transl Sci
- v.5(1); 2021

Creating effective academic research teams: Two tools borrowed from business practice
Holly h. brower.
1 Clinical and Translational Science Institute, Wake Forest University, Winston-Salem, NC, USA
Barbara J. Nicklas
2 Clinical and Translational Science Institute (CTSI), Wake Forest School of Medicine, Winston-Salem, NC, USA
Michael A. Nader
Lindsay m. trost, david p. miller, associated data.
For supplementary material accompanying this paper visit https://doi.org/10.1017/cts.2020.553.
Academic Medical Centers strive to create multidisciplinary research teams to produce impactful science. However, few faculty researchers receive training in “team science,” a well-established concept in business research and practice. Responding to demand for assistance developing effective research teams, the Collaboration and Team Science Program of the Clinical and Translational Science Institute (CTSI) at Wake Forest School of Medicine (WFSM) partnered with faculty from the Wake Forest University (WFU) School of Business with expertise in leadership, management, and team building. We initiated a needs assessment, including a written survey from a diverse set of 42 research scientists as well as semi-structured interviews with 8 researchers. In response to identified needs, we developed training sessions and consultations to teach teams to implement two tools known to enhance team dynamics: (1) Team charter, a document that defines the team’s purpose, goals, roles, and strategies; and (2) Responsible, Accountable, Consulted, Informed (RACI) matrix, a table or spreadsheet that clarifies tasks and accountability. Since 2018, 10 teams and over 100 individuals have attended training sessions and 6 teams received personalized team consults. We describe these tools, present a formal analysis of quantitative results, and highlight the next steps being taken in response to these findings.
Introduction
Complex work of various types, including the design and conduct of high-impact scientific research, hinges on the ability to collaboratively solve problems [ 1 ]. This reality has become increasingly evident in medicine and science. Notably, the American Association of Medical Colleges names the ability to “collaborate as a member of an interprofessional team” as one of its 13 core entrustable professional activities for entering residency training [ 2 ]. In biomedical research, multidisciplinary teams consistently outperform less diverse research teams [ 3 – 5 ]. If the Clinical and Translational Science Awards (CTSAs) are to meaningfully impact health, they must foster highly productive, diverse clinical and research teams [ 6 ]. However, the mere existence of an interdisciplinary team does not guarantee that positive effects will be realized. Best practices for effective collaboration among team members (often referred to as “team science”) must be discovered and implemented within medical care and research teams to foster their success.
The NIH Field Guide on Collaboration and Team Science [ 7 ], originally published by the National Cancer Institute in 2010 and updated in 2018, provides resources and tools for effective team operation. Other tools designed to enhance team effectiveness [ 8 , 9 ] and training curricula [ 10 – 12 ] have also been developed and evaluated. Despite the existence of these tools, few medical school faculties are aware of them or have used them.
Medical schools and graduate research programs have lagged behind in teaching the principles of effective team science [ 13 ]. A review of interprofessional primary care teams in health care affirmed that “a general understanding of optimal team design is not available (p. 550)” [ 14 ]. Though rigorously trained in science and the conduct of research, few research scientists understand how to effectively develop and work in teams. On the other hand, business school faculty have been investigating and implementing features of highly effective teams for decades in the fields of organizational behavior, management, leadership, and organizational psychology. Recognizing a critical need to train medical school clinical and research faculty in the principles of team science, particularly with an emphasis on translational research, the Wake Forest School of Medicine (WFSM) Clinical and Translational Science Institute (CTSI) reached out to experts in organizational science at our School of Business to develop and implement a novel team science training program for faculty and research staff.
In 2017, representatives from the medical school’s CTSI leadership team met with faculty from the Wake Forest University (WFU) School of Business who had expertise in leadership, management, and team building to discuss team science and forge a working partnership. The discussions resulted in two WFU Business School faculty members joining the CTSI’s Collaboration and Team Science Program’s leadership, alongside clinical and basic science researchers, to strategize and guide the development of effective research teams. This paper reports on the development and ongoing work of a team science training program established by this group.
Needs Assessment
After speaking with CTSI leaders, the experts from the business school recommended several best practices that demonstrate evidence-based success in building high-performing teams across multiple disciplines and applied contexts. They also recommended a number of topics for training and development in areas critical to team formation, cohesion, and effectiveness.
We then sought to prioritize these topics by conducting a needs assessment involving a survey of 42 basic and clinical research faculty accompanied by 8 individual interviews in 2017. Surveyed faculty ranged from early career to established scientists across various disciplines (Table 1 ). On the survey, participants used a 5-point Likert scale to rate the perceived need for training in nine team science-related competencies identified as critical success factors in high-performing teams. The scale was anchored at 1 = “great need for this training” and 5 = “no need for this training.” The survey also asked respondents to prioritize the nine topics from “1” (highest priority) to “9” (lowest priority). To better understand the survey data and preliminary results, we conducted eight semi-structured interviews following an interview guide with researchers to further explore and understand the specific needs of their research teams. Furthermore, these interviews allowed for a better understanding of the issues that make research teams more cohesive and productive. Survey responses showed, and interviews confirmed, that teams were struggling with the following issues: setting clear expectations; holding each other accountable to both tasks and deadlines; gaining buy-in on goals and objectives from the whole team rather than only senior faculty; and running effective meetings.
Needs assessment survey results for team science core competencies
n = 42 research scholars in Medical Center.
The needs assessment results and additional input from faculty and CTSI leadership led the Collaboration and Team Science Program to clearly define its mission: With a goal of producing the most high-impact translational research, this team exists to make the most effective, integrated research teams, then disseminate and share what we ’ ve learned broadly to be thought leaders in the field of team science and contribute to others ’ excellence as a result.
Objectives of the WFSM-WFU Business School Collaboration and Team Science Program
In response to the needs assessment, we developed five primary objectives:
- Facilitate formation of teams within the medical school through promoting research studios and team building/formation of new teams;
- Encourage and facilitate interdisciplinary team science;
- Connect people who wouldn’t normally work together by identifying gaps and bringing people together, as well as facilitating team engagement;
- Cause people to think about their research in a different way;
- Be thought leaders in the field of team science and contribute to others’ excellence as a result.
The focus of this paper is encouraging and facilitating interdisciplinary team science using recommendations from well-researched and implemented resources (including the NIH Field Guide). To meet this particular objective, we developed workshops and tools to teach and facilitate effective team formation and norms within research teams. Specifically, these training tools focused on clearly defining a team’s purpose, objectives, roles, developing trust within teams, managing tasks effectively while communicating clear expectations, and delivering effective feedback and conflict management.
We combined the needs assessment results with research on effective teams to develop the training plan and tools that would add the most value to the research teams. The tools and training plan described below were in response to the needs and priorities expressed by respondents.
Two Specific Tools for Effective Teams
Scientific teams that are carefully formed, nurtured, and developed exhibit better performance [ 7 ]. Clarification of roles, clear goal setting, and team cognition are particularly impactful on team performance [ 1 , 15 ]. Team cognition is the emergent process where teams develop a shared understanding of their overarching purpose and process, as well as what standards of interaction and performance will be expected [ 4 ].
We adapted two distinct tools that are having a strong early positive impact on team cognition and resulting effectiveness among our research teams.
1. Team charter: We developed a team charter protocol and an accompanying training program for its use (see online Appendix A). A team charter is a document that clearly defines a team’s purpose, goals, strategies, and team members’ roles for holding each other accountable to mutual expectations. Initiated by the team leader, teams agree on clear practices for team processes such as responding to emails, attendance, and timeliness of meetings. Such a team process is an effective way of forming team cognition, “the manner in which knowledge important to team functioning is mentally organized, represented, and distributed within the team and allows team members to anticipate actions, define processes and hold members accountable (p. 33)” [ 16 ]. In fact, effective practice mandates that high-performing teams discuss and commit to their mission and purpose, specific roles, goals, and practices and to hold each other accountable [ 17 ]. Teams set a charter together with input from every member and affirm their commitment to the charter when it is completed.
Importantly, teams agree on accountability guidelines and expectations about how a conflict will be resolved before there are personalities and specific issues causing division. High-performing teams communicate well, even when there are disagreements because a foundation of trust between members has been established [ 18 ]. The process of clearly establishing expectations and procedures builds trust in early formation.
2. RACI matrix: A team charter clearly sets expectations and goals for success, but it often fails to clarify who is responsible for specific tasks, which can be problematic and frustrating for the successful implementation of the team’s goals. We encourage the use of a Responsible, Accountable, Consulted, Informed (RACI) matrix to clearly define tasks and responsibilities, allowing teams to effectively track progress and hold members accountable [ 19 ]. The RACI matrix is a project management tool (Table 2 ). It can be utilized to manage team process even without a team charter, but the combination of the two tools is most effective. The team charter sets expectations and roles early in the team formation process, whereas the RACI matrix is a fluid document that is changed and added to throughout the team’s functioning. It is a working document used to manage specific tasks and accountability.
Example of a RACI * matrix for a research team
Constructing a RACI matrix begins by first listing the team’s specific tasks, such as “administer surveys” or “create annual progress report.” Then, for each task, the team decides who is “Accountable” (the individual ultimately responsible to ensure that the task is completed). To avoid confusion, each task should have only one person labeled “Accountable.” Next, others are assigned tasks according to the roles of “Responsible” (someone who will help do the work, but is not ultimately accountable), “Consulted” (someone who provides input or key information), or “Informed” (someone who is notified of progress but does not contribute to the work) [ 19 ]. A RACI matrix clearly defines who “owns” a task (the person responsible for seeing the task through to completion), avoids role confusion, and ensures work is balanced across team members and tasks. Scanning down individual columns allows members to see if a team member is being stretched across too many tasks or being underutilized. If a team member is involved in too many tasks, roles can be downgraded, such as changing a “responsible” to a “consulted.” Scanning across task rows allows members to see if any task is under-resourced. It also provides a simple, clear visual to readily assess progress on each task. As described below, the early impact on teams using the RACI matrix has been remarkable.
Coaching Teams to use the Tools
Early career faculty: We pilot tested our training materials with the Wake Forest Translational Research Scholars Academy, an institutionally sponsored career development program for early career faculty researchers. Three different 75-minute seminars on team science were added to the curriculum beginning in the Fall of 2017. The seminars involved sharing information and team science best practices, working through case studies, and discussions. The first two seminars, “Establishing Clear Expectations and a Successful Work Plan,” and “Holding Teams Accountable and Addressing Conflict” revolved around developing and using a team charter. The third seminar, “Effective Team Meetings,” was on principles of effective meetings, including pre-work and follow-up after meetings. Examples of these effective meeting principles include sending an agenda prior to meetings, planning specific timing for discussion topics to manage time carefully, and sending meeting minutes/notes within 1 week after a meeting with highlighted action steps.
Broader research audiences: After testing the content with early career faculty, we next implemented a process to share the information more broadly. As a result, two “Lunch-and-Learn” seminars were offered to all faculty and staff within the Medical Center in 2018. These seminars, attended by approximately 105 individuals, focused on principles and practices of effective meetings and trust-building. In addition, we conducted two half-day workshops for research teams across the Medical Center to understand and develop team charters; three months later, a follow-up 2-hour workshop was held for the continued development of their team charters. These workshops were very practical and action-oriented. Participants attended with their specific research teams. Throughout the workshops, teams engaged in exercises and discussions to build the foundation of effective teams according to a model prescribed by Patrick Lencioni [ 18 ]. Participation in these workshops provided opportunities for teams to bond with each other, while being coached through the process of developing their team charters. The result was that teams left the workshops with a draft of their team charter.
In addition to seminars and workshops, our Collaboration and Team Science Program offered a consultation with WFU Business School faculty experts for research teams in the Medical Center. Of the six individual team consults held in 2018–2019, three involved assisting additional teams in developing their team charters and one involved an already chartered team in implementing a RACI matrix to effectively manage tasks and expectations. Two other consults involved leadership coaching and conflict resolution.
1. Team charters: To date, 10 teams consisting of 71 individuals have been trained to implement team charters through workshops. We received 32 evaluations from attendees of team charter training sessions. Both early career faculty ( n = 15) and senior faculty or executive administrative staff ( n = 17) rated the training sessions highly favorably. On a scale of 1 (poor) to 5 (excellent), the mean ratings of the sessions were 4.5 for early career faculty and 4.6 for senior faculty/staff. Similarly, both groups reported they would recommend the session to a colleague (mean rating 4.5 for early career faculty and 4.3 for senior faculty/staff). The early career faculty completed a lengthier evaluation with additional items, and 93% (14/15) agreed the content was relevant to working in research teams, 93% (14/15) reported they acquired new skills, and 87% (13/15) reported they were confident applying the new skills acquired.
2. RACI matrix: We collected qualitative feedback from two teams that are using the RACI matrix. Project managers reported that the tool has helped “balance workload among faculty and staff, … enabled us to determine where additional effort was needed …, and helped give the leadership peace of mind knowing that most, if not all, areas of responsibility were covered.” Additionally, the RACI matrix “prevents confusion in regards to responsibility and is a great onboarding tool that helps new members quickly understand roles on the team.”
Early results show that research teams are responding well to training and the use of tools for facilitating team effectiveness/organization. While our initial evaluation has relied on self-assessment, we recognize the limitations of this method and are implementing more performance-based evaluation plans. These include tracking of team performance before and after receiving a Team Science Consultation, creating a team charter, or implementing RACI. We are also tracking the institution-wide utilization of these tools. We are receiving increasing numbers of requests for consults and training in these tools and other skills related to team leadership.
Our Collaboration and Team Science Program aspires to have every team utilize the team charter process (as required in many business settings) whenever a project team is formed. The RACI matrix becomes the operating tool to implement the agreed-upon purpose, goals, roles, and expectations outlined in the team charter, so the combination of the two tools are particularly effective at producing high-impact teams. We believe the small number of teams using the RACI tool to date is primarily due to the early stage of our work in this area. We are promoting the use of RACI matrices during team consults, and we have anecdotal evidence that additional teams have adopted this practice, as well. We expect the use to become a common practice as our number of team consults increases and trained team members spread across additional teams. An important future metric will be whether these training tools enhance multidisciplinary, translational research success as reflected in funding and high-impact publications.
We also aim to train team leaders to effectively facilitate team process excellence so they are more equipped to independently lead and manage successful teams. To that end, we launched a Leadership Academy early in 2020 for research leaders. This leadership development program is comprised of five 2-hour interactive training sessions on trust building, conflict resolution and feedback, delegation and team management, decision-making, and self-awareness and development. Due to COVID-19 social distancing mandates, we finished our first Leadership Academy via video conference, which was well received. Applications for the second iteration of the Leadership Academy will be received late in Fall 2020.
Finally, part of the mission of the Wake Forest School of Medicine CTSI is to spread the use of these effective tools to other Academic Medical Centers. The research literature and our initial results provide clear evidence that these practices enhance team outcomes, including productivity, communication, satisfaction, and longevity. Therefore, we plan to continue to carefully evaluate and disseminate our findings in future manuscripts and conferences.
Acknowledgments
We wish to thank Amy Wallis, PhD who was instrumental in shaping this work in its early stages and continues to partner with us as a consultant in this influential work.
The authors gratefully acknowledge the use of the services and facilities of the CTSI Team Science program, funded by the National Center for Advancing Translational Sciences (NCATS), National Institutes of Health, through Grant Award Number UL1TR001420.
Supplementary material
Disclosures.
The authors have no conflicts of interest to declare.
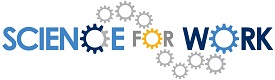
Team Building: How to Get Real Results from Team Building Activities
- and Training and Development
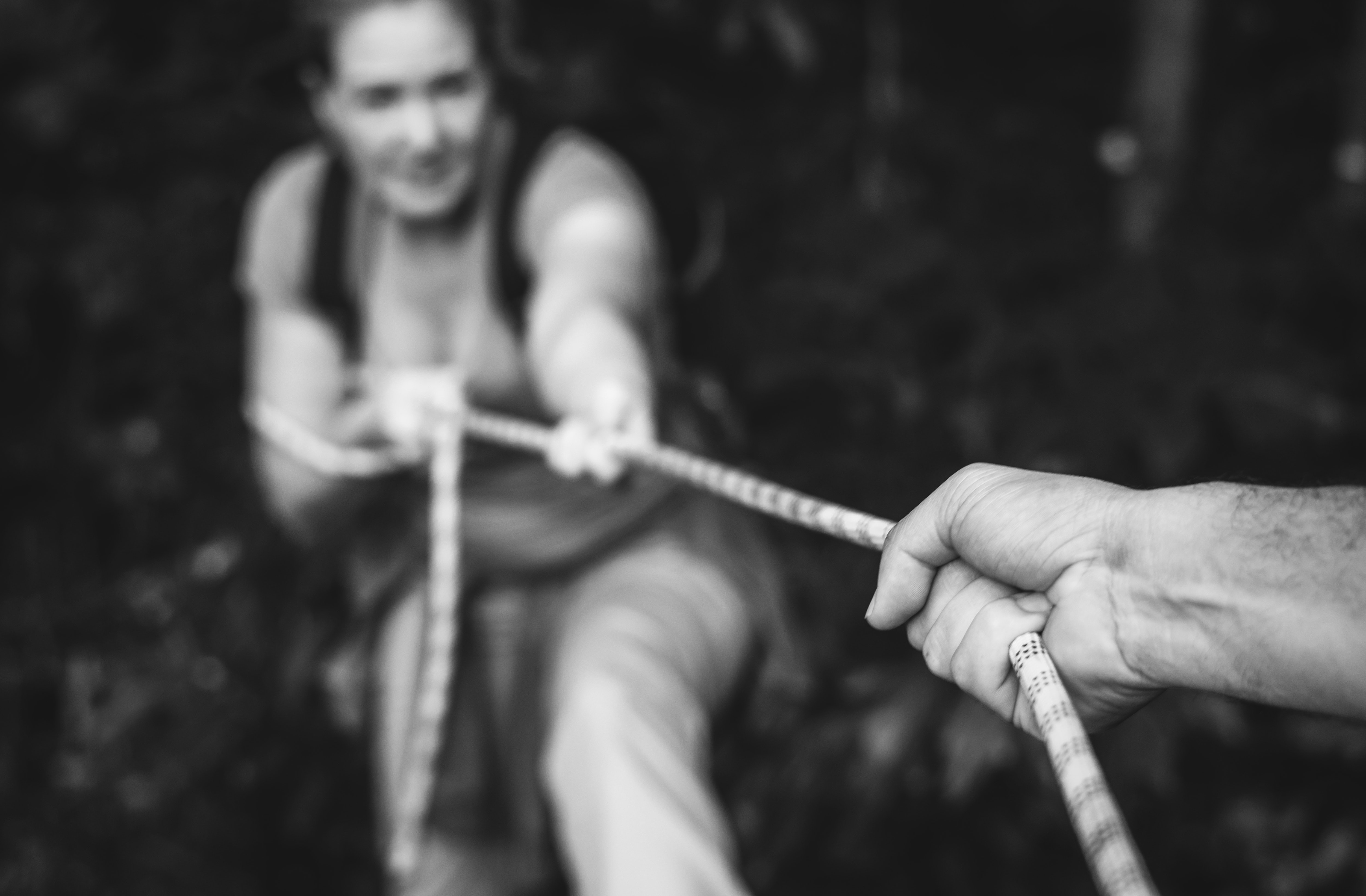
- Team building interventions have three essential characteristics which clarify the confusion around the term and help differentiate it from other interventions aimed at teams;
- Team building interventions improve team members’ feelings and team interactions, but don’t have a considerable effect on team performance;
- Effective team building activities are not only fun, but must also be aligned with the objective of the session.
Being a manager isn’t easy. Along with ensuring the team delivers, you are also expected to make sure that team members get along well and are happy as part of the team. Team building activities are often recommended as a solution to keep the team’s satisfaction high. Since everyone seems to be partaking in team building activities, and teams expect them as par for the course, you might see this as a potential option for your team. But before investing in team building, there are a few important questions that we will try to answer in this summary: Do team building activities have any impact on the team? What’s the difference between an effective team building activity and one that’s just a waste of time?
What is team building?
While everyone talks about team building, what it means isn’t always clear, and that makes it difficult to study its effectiveness. Researchers who study the topic show that there is no such thing as one definition of “team building”. Instead, the term refers to a category of interventions aimed at teams. What these interventions have in common is:
- Objective: improving a team’s functioning in general, by developing interpersonal relations, clarifying roles, or solving existent problems
- Method: mostly informal team building activities like games and physical exercises, as opposed to sitting in a classroom and discussing a topic
- Location: usually a different one to where the team works (e.g. in nature, in an events hall).
Team building interventions might sound similar to teamwork training, another type of intervention aimed at helping teams work better together. However, the two are different; teamwork training is:
- focused on building specific skills (e.g. teaching team members how to formulate an action plan)
- more formal and systematic (e.g. the trainer develops specific objectives for each session and evaluates if they were achieved)
- usually held in the same location where the team works (e.g. a conference room in the office).
For more information on teamwork training, including benefits it has and how to make it more effective, check out our previous evidence summary .
Which of these two is right for your team? If you know the specific need of your team and you want to provide focused support to meet that need, then teamwork training may be best. However, if you’re looking for an intervention to improve the general functioning of the team and to raise team members’ satisfaction, then team building might be better.
What’s the evidence for team building?
So now that we know what team building is, let’s understand the evidence. In 2009, researchers from the USA published a meta-analysis based on data from over 1500 teams. The meta-analysis investigated the effects of team building on different aspects of the team:
- team members’ feelings about the team and their colleagues
- team interactions that help it solve its task (e.g. how it communicates, collaborates or manages conflicts)
- team performance (how the team’s achievements compare to the expected outputs).
What is team building good for?
The meta-analysis showed that people who participate in team building feel more positively about their team. This positive feeling is manifested in certain ways such as more trust for their fellow team members, more satisfaction that they’re part of the team, and more confidence that the team will achieve its objectives.
Furthermore, teams that go through team building sessions have better interactions: they communicate, coordinate and manage conflicts better. These processes are key in accomplishing the team’s task.
By now, you might think “team building is great!”. However, team building didn’t significantly improve team performance. Despite better communication and more trust being associated with enhanced team performance, team building itself doesn’t bring any considerable direct benefits in terms of performance. The reason for this might be that performance depends on other factors besides how the team interacts, such as team members’ competence or managerial support.

When does a team building session work best?
Within team building interventions, some are more effective than others. What seems to make a difference is the aim of the session: team building activities focused on setting goals or clarifying roles bring the most benefits. Setting goals refers to defining both individual and team objectives, and planning what actions to take to turn the objectives into reality. Clarifying roles means team members discuss what is and isn’t within their own responsibility and they understand their duties better.
On the other hand, team building activities focused on improving interpersonal relations or solving existing problems bring slightly smaller, but still relevant benefits. Improving interpersonal relations sees team members work on their trust in each other and building connections on a more personal level. Solving problems means identifying what doesn’t work in the team and planning on what to do to improve the situation.

Besides the focus of the team building exercise, the team size can also have an impact on how effective such interventions are. When looking at large teams with over 10 members, the researchers found that these teams benefit much more from team building than smaller teams (with less than 10 members). The reason for this is that bigger teams might have more challenges to face than smaller teams. This leaves more room for improvement in the way they work, and team building activities supply some of the help that’s needed to function well together.
Takeaways for your practice
Team building is an effective intervention if you’re looking to improve the general functioning of your team. It is an activity that’s less formal and more fun than teamwork training, but if it follows an objective, it makes team members feel better and interact more effectively with each other. To gain the most benefit from team building, here are a few ideas:
- Identify what the team needs: does it lack a specific skill linked to their work together (e.g. formulating an action plan) or are you looking for more general improvement? While the first case might be a better fit with teamwork training, the latter rather requires team building as a solution
- Even if the benefits are more general, think of what the team building exercise needs to achieve to be effective. Especially in a new team, setting goals or clarifying roles might work best, but if your team’s situation requires r improving interpersonal relations or solving an existing problem, then focusing on one of these will likely work better. Don’t forget that people probably expect to have fun in a team building exercise, so find enjoyable activities that work towards these goals. Searching online for team building activities to do is fine as long as you compare them with the objectives and adapt them to fit the purpose. Asking an experienced facilitator for help to keep things engaging can also be a good idea
- Explain the benefits of team building to the team and why, even though it’s fun, you still need to have some purposeful activities linked to a general objective. By setting their expectations, you will win over the sceptics, but also you won’t disappoint the enthusiasts.
Trustworthiness score
We critically evaluated the strength and quality of the study we used to inform this Evidence Summary. We found that the study design – a meta-analysis of studies with before-and-after measurement – is highly appropriate to demonstrate a causal relationship. Therefore we can conclude that it is shown that team building interventions have positive effects on team processes and team member’s affective outcomes.
Learn how we critically appraise studies to assign them a Trustworthiness Score .
ScienceForWork is an independent, non-profit foundation of evidence-based practitioners who want to #MakeWorkBetter.
Our mission is to provide leaders and decision-makers with trustworthy and actionable insights from behavioural science..
Did you like this Evidence Summary? Share it with your network by clicking on the buttons below!
Follow us on LinkedIn and Twitter to receive the most trustworthy scientific research summarized in less than 1000 words!
Reference :
Klein, C., DiazGranados, D., Salas, E., Le, H., Burke, C. S., Lyons, R., & Goodwin, G. F. (2009). Does team building work? . Small Group Research , 40 (2), 181-222. DOI: 10.1177/1046496408328821

Iulia Alina Cioca
Iulia graduated in WOP psychology from the University of Valencia, after an educational journey that started in Romania, and continued through Austria, Italy and Canada. She has worked as a talent management consultant in Milan, Italy. Currently she is a trainer for a multinational company. Her professional interests are organizational change, teamwork, psychometrics and training. Her passions are reading, taking long walks anywhere in cities or nature, and languages, especially teaching them to children.
Other Evidence Summaries keep reading! NAME NEWEST
- Employee Turnover
- and Featured
- and Leadership
- and Performance Management
- and Work Motivation

Turnover: Decoding It’s Effects on Performance
- and Health and Safety
- and Myth-Busting

Effective Coaching Methods: Not all coaching is created equal or is it?
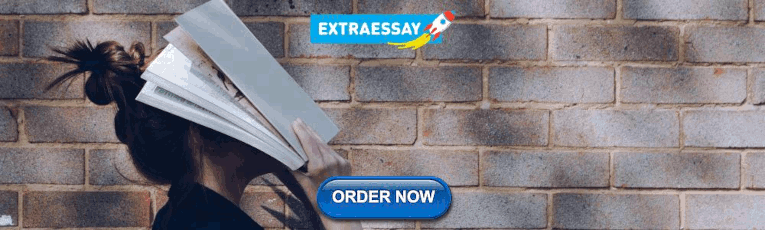
Liz Pavese, Ph.D.

Workplace Coaching: What Does the Evidence Say and Does it Work?
- and Organizational Development

The Quality of Quality Management: a workout recipe for firm performance
Stefano Gravina
Leave a reply cancel reply.
This site uses Akismet to reduce spam. Learn how your comment data is processed .
Jennifer Miller
I just got hired on at a company to help with one of their new projects. They signed me a few other members from the team for a team building exercise as an ice breaker. I think it was a great idea to get to know everyone before I started working. There was myself, 2 new guys and a few of the senior members. They went through escape room rank and found one that had to do with stopping a hacker from setting off a bomb, to keep with the theme or our tech company (kinda corny, but hey it was neat) We all went through an escape room together and it was great. We spent an hour trying to solve puzzles to get out of a room and I have to say I loved doing this to get to know my new coworkers. I told my family and friends and can’t wait to go again!
If team building does not improve team performance, there is no reason to do it.
Pingback: Team Building: How to Get Real Results from Team Building Activities • ScienceForWork - The Admin Division
teambuildingactivitiesmelbourne
Thank you for sharing your thoughts about team building. It’s good to know that group activities Melbourne helps members to work together. It also develops productivity and communication.
Privacy Overview
Necessary cookies are absolutely essential for the website to function properly. This category only includes cookies that ensures basic functionalities and security features of the website. These cookies do not store any personal information.
Any cookies that may not be particularly necessary for the website to function and is used specifically to collect user personal data via analytics, ads, other embedded contents are termed as non-necessary cookies. It is mandatory to procure user consent prior to running these cookies on your website.
Kellogg School of Management at Northwestern University
What science can tell us about
Building great teams.
Assembling and managing successful teams is a core leadership skill, whether you are convening a temporary task force, managing a full department, or running a school fundraiser.
But how well do you understand what makes a great team?
If you think it’s simply assembling a group of highly talented people and letting them do their thing, then you’re in good company. Research shows that’s what people tend to believe. But, unfortunately, you’d also be wrong.
Teams are more than the sum of their parts. In fact, sometimes having lots of top talent on a team actually hurts performance. We’ll get back to that in a minute.
First, let’s look at why it’s become increasingly important to know how to lead, and be part of, teams.
Teams are more important than they used to be because there’s so much more to know in a given field.
You’ve likely been taught the importance of teamwork since you were in kindergarten. Today, those lessons are more important than ever, as teams have become a mainstay in organizations. The world of scientific research offers a telling example: a study of 19.9 million scientific papers and 2.1 million patents generated over 5 decades shows that teams are increasingly producing more work than individuals are. And, those teams are getting bigger over time. Teams also generally produce research and patents that are more frequently cited—a measure of the paper’s or patent’s impact within its field—than research by individuals. That trend has also increased over time. ( Wuchty, Jones, and Uzzi, Science , 2007 .)
Here’s one way to explain the trend toward teams: As technology advances, the quantity of knowledge in the world grows. With more and more to know, it becomes harder and harder for any one person to have a deep general knowledge in a field. Instead, people are joining forces to combine their specific knowledge and cover more of a field. Another study on 2.9 million patents issued from 1963 to 1999 shows that team-size increases are bigger in fields with a larger “depth of knowledge,” such as biotech. ( Jones, Review of Economic Studies , 2009 .)
Teams have their own level of intelligence, which is separate from the combined intelligence of their team members.
A well-assembled team can be more than the sum of its parts. Research shows that teams have their own level of intelligence, dubbed “collective intelligence,” which is a measure of the group’s ability to perform a wide variety of tasks. And, importantly, having a lot of individually intelligent team members does not mean the team will have a high collective intelligence. Lab experiments in one study had teams spend several hours on a variety of exercises, from brainstorming to solving visual puzzles. The results point to three ways to increase a team’s collective intelligence:
- Have more members who are socially sensitive, meaning they’re perceptive of their teammates’ views and feelings.
- Have more women on the team (in large part because women tend to be more socially perceptive).
- Make sure team members take turns talking instead of being dominated by just a few voices.
( Woolley, Chabris, Pentland, Hashmi, and Malone, Science , 2010 .)
Sometimes building a team with lots of really talented people actually hurts the team’s performance.
Packing your team with top talent boosts success—but only up to a point. Research from the world of professional athletes shows that in sports that require a lot of interdependence, there’s a point at which lots of talent starts hurting performance.
This happens because top talents don’t always coordinate effectively, perhaps because superstars are angling to be top dog instead of focusing on winning. (The researchers note similar findings in the animal kingdom, citing a study of chickens. Having too many high-producing egg layers in a colony reduces overall egg production “as a result of intense conflicts.”)
The human-based study found that professional soccer and basketball teams suffer the diminishing returns of top talent, but Major League Baseball teams do not, likely because the nature of baseball means players can coordinate less and still win games. ( Swaab, Schaerer, Anicich, Ronay, and Galinsky, Psychological Science . 2014 .)
By definition, those who are highly skilled at something have, well, lots of skills. But sometimes those top performers share the same skills as their high-performing peers. And this is another reason that overemphasizing top talent can hurt teams.
One study looked at the relative merit of having a team made up of the very best problem-solvers versus a team whose members were accomplished problem-solvers but had a diverse set of approaches for tackling those problems. The results showed that the diverse teams outperformed the teams made up of the very best individuals. ( Hong and Page, PNAS, 2004 . )
Diversity is often an asset for teams, but can also lead to common pitfalls. So be thoughtful and deliberate in how you lead diverse teams.
As the importance of teams has grown, so has the diversity of the American workforce. Yet, there’s no simple prescription for how to effectively lead diverse teams. Sorry, even researchers disagree on the nitty-gritty pros and cons. However, research does point to a benefit in having a diversity of thinking on teams that tackle complex tasks. Meaning, teams of people who approach problems differently (often, but certainly not always, as a result of gender, cultural, or educational diversity) are usually better at solving those problems. But that diversity brings a set of potential pitfalls as well. Scholars generally agree that these include the following:
- Team members may splinter into subgroups of similar people.
- These teams can have higher turnover.
- Teams tend to lose their diversity over time as members start thinking similarly.
But researchers have also identified ways to prevent these pitfalls. Here are some tips for leading diverse teams in ways that boost their performance.
It’s unlikely to solve all of diversity’s conundrums, but here’s one easy tip: explain to your team that a diversity of thinking can help them succeed. This was the takeaway from a study of four-person teams of university students, comprised of two men and two women. When teams were told ahead of time that gender diversity helps with team decision-making and group processes, they performed better on a complex decision-making task. ( Homan, Knippenberg, Van Kleef, and De Dreu, Journal of Applied Psychology , 2007. )
Another study found that diversity enhances group performance when teammates have a mutual understanding of each other’s skills. Meaning, if Luisa considers herself creative and hard-working, then her teammate Larry also sees these traits in her.
Roughly 400 MBA students were grouped into small, highly diverse teams. The members of each team assessed themselves and one another on 11 attributes, such as leadership ability and competitiveness. The groups then worked together on creative tasks for four months.
The researchers found that diversity improved team performance in groups with a high level of mutual understanding. Yet diversity hindered teams at the opposite end of the spectrum. ( Polzer, Milton, and Swann, Administrative Science Quarterly , 2002.)
A study of NBA teams points to a beneficial way of structuring diversity for organizations that have two clear tiers of workers—think partners and associates, or physicians and residents. On the court, these two tiers are the starters and subs.
Researchers analyzed 23 years of league data, using players’ college conferences as a measure of diversity, since different conferences tend to have different playing styles. The results show a benefit to having a diversity of playing styles among starters that is duplicated among bench players. Teams with this sort of overlapping diversity won 10.7 percent more games per season.
The researchers point out that without this redundancy of diversity, people might forget about the skills that make them unique. ( Smith and Hou, Organization Science, 2014 .)
Don’t always opt for a generalist. Sometimes you need a specialist.
The allure of recruiting a jack- or jill-of-all-trades is strong. So strong, it seems, that managers are biased against recruiting and rewarding specialists—even when a specialist is exactly what their organization needs. This was the finding in a set of experiments, where participants were tasked with assembling a fictional team. They were more likely to favor generalists, even when a specialist would have a bigger impact. Why? We have a natural tendency to compare people against one another, the researchers say. And when you compare specialists who excel in a single area to generalists who are high-achieving in multiple areas, those specialists don’t look as impressive. The lesson: evaluate job candidates on their own merits, as opposed to in contrast to another candidate, when you know a specialist is what your team needs. ( Wang and Murnighan, Organizational Behavior and Human Decision Processes , 2013. )
Simply bringing a specialist onboard, though, may not be enough to make your team hum efficiently. Team members should talk through how to use their expert before embarking on a group task.
This was demonstrated in an experiment with four-person teams that had to crack a hypothetical terrorist plot. To do so, the teams needed experts in facial recognition to analyze security footage and experts in verbal memory to analyze email evidence. Teams with these experts performed better than those without them only when they started with 10 minutes of collaborative planning before diving into the challenge. ( Woolley, Gerbasi, Chabris, Kosslyn, and Hackman, Small Group Research , 2008. )
Team members’ history together , especially if it’s been successful, can help a lot .
There’s a euphoria to being part of a winning team, whether you claimed the championship title or nailed the product launch. And, research shows, winning together also predicts future success—over and above simply having highly skilled people on your team. In looking at four professional sports leagues and a multiplayer online game, researchers found that the level of talent helped to predict whether a team would win. But taking into account a history of shared successes also helped predict wins—above and beyond the effect of talent. ( Mukherjee, Huang, Neidhardt, Uzzi, and Contractor, Nature Human Behaviour, 2018 .)
Having a history of collaboration matters for scientific teams, too. A different study looked at researchers in cell biology and physics and found that some groups of scientists had collaborated frequently enough to be dubbed “super ties.” Those super ties were a significant boon to a scientist’s career, leading to more published papers and a higher rate of citations on those papers, which is an indicator that the papers were considered higher impact. ( Petersen, PNAS , 2015. )
Relying on super ties alone would be a mistake. You want some inflow of new people and ideas. But how much?
One study looked at the six-person teams that create Broadway musicals. (These creative teams tend to be made up of a composer, lyricist, librettist, choreographer, director, and producer.) The researchers wanted to explore the best way to assemble teams within tight-knit fields where the same people often collaborate with each other or with people who have one degree of separation from previous collaborators.
They found that both critical acclaim and financial success peak when there is a medium level of these tight-knit connections: Too much, and new ideas don’t flow in. Too little, and there aren’t enough of the common bonds that allow teams to establish trust and enable members to vouch for each other’s innovative instincts. ( Uzzi and Spiro, American Journal of Sociology, 2005 .)
Another study looked specifically at the benefit of bringing new people into team brainstorming exercises. In several experiments with three-person teams, groups that retained their same makeup generated fewer total ideas and fewer different kinds of ideas than did teams that had one of their three members swapped out.
And it wasn’t simply that the newcomers came in with their creative guns blazing. The study showed that having a productive new person join a team increased the productivity of the old-timers, as well. ( Choi and Thompson, Organizational Behavior and Human Decision Processes , 2005. )
So Broadway musicals and brainstormers benefit from some turnover. But how about the very different world of private equity? Indeed, investors often seek out team stability among private equity managers with the assumption that this cohesion boosts profits.
But a study of 138 private equity managers over 20 years shows that this is not the case. Some degree of team turnover actually improved fund performance over both the short and long term. This is likely because turnover helps get rid of poor performers while also bringing in team members with new skills, which helps private equity companies adapt to a changing environment. ( Cornelli, Simintzi, and Vig, Working paper, 2019. )
A study of nearly 18 million research articles over five decades showed that the highest-impact papers were ones that combined a little bit of novelty with otherwise conventional prior ideas. ( Uzzi, Mukherjee, Stringer, and Jones, Science , 2013. )
How big of a team should you convene? It depends on what your ultimate goal is.
How big of a team should you assemble? It depends whether you are trying to advance the field by building off prior discoveries—in which case a large team is best—or whether you're trying to disrupt your field by going in a totally new direction—in which case you might want a small, nimble team. That’s the finding from an analysis of 65 million scientific papers, patents, and software products from 1954 to 2014. This may be because large teams need to secure significant and ongoing funding, so they are less likely to pursue something risky and novel—whereas smaller teams or solo authors are able to take more risk, as they have less to lose. ( Wu, Wang, and Evans, Nature, 2019. )
Delegation is nothing new. But it has become increasingly easy, as advances in technology allow highly skilled professionals to offload more of their work to subordinates in order to focus on the tasks that require the knowledge that they, alone, possess. So managers within these “knowledge hierarchies” should ensure that their teams are big enough to accommodate sufficient delegation.
Take lawyers, for example. Once upon a time, law partners were the exclusive fonts of knowledge in their firm, holding much of the institution’s information and expertise in their brains. This made it difficult to delegate to associates. But new technology, like the legal search engine Lexis, changed that by collecting much of that knowledge in a place that associates can easily access. One study of all U.S. law firms found that these and other changes helped increase the productivity boost that partners got from delegating to associates by 30 percent between 1977 and 1992. ( Garicano and Hubbard, Journal of Law, Economics, & Organization . 2018. )
Teams are more important than they used to be because there’s so much more to know in a given field.
Teams have their own level of intelligence, which is separate from the combined intelligence of their team members.
Sometimes building a team with lots of really talented people actually hurts the team’s performance.
Diversity is often an asset for teams, but can also lead to common pitfalls. So be thoughtful and deliberate in how you lead diverse teams.
Don’t always opt for a generalist. Sometimes you need a specialist.
How big of a team should you convene? It depends on what your ultimate goal is.
Emily Stone
Jessica Love
Michael Meier
Aaron Geller
Dashun Wang
Wuchty, Stefan, Benjamin F. Jones, and Brian Uzzi. 2007. “The Increasing Dominance of Teams in the Production of Knowledge.” Science . 316(5827): 1036–1039.
Jones, F. Benjamin. 2009. “The Burden of Knowledge and the ‘Death of the Renaissance Man’: Is Innovation Getting Harder?” Review of Economic Studies . 76(1): 283–317.
Woolley, Anita W., Christopher F. Chabris, Alex Pentland, Nada Hashmi, and Thomas W. Malone. 2010. “Evidence for a Collective Intelligence Factor in the Performance of Human Groups.” Science . 330(6004): 686–688.
Woolley, Anita. W., Ishani Aggarwal, and Thomas W. Malone. 2015. “Collective Intelligence and Group Performance.” Current Directions in Psychological Science . 24(6): 420–424.
Swaab, Roderick. I., Michael Schaerer, Eric M. Anicich, Richard Ronay, and Adam D. Galinsky. 2014. “The Too-Much-Talent Effect: Team Interdependence Determines When More Talent Is Too Much or Not Enough.” Psychological Science . 25(8): 1581–1591.
Hong, Lu, and Scott E. Page. 2004. “Groups of Diverse Problem Solvers Can Outperform Groups of High-Ability Problem Solvers.” PNAS. 101(46): 16385–16389.
Homan, Astrid C., Daan van Knippenberg, Gerben A. Van Kleef, and Carsten K.W. De Dreu. 2007. “Bridging Faultlines by Valuing Diversity: Diversity Beliefs, Information Elaboration, and Performance in Diverse Work Groups.” Journal of Applied Psychology .92(5): 1189–1199.
Polzer, Jeffrey T., Laurie P. Milton, and William B. Swann Jr. 2002. “Capitalizing on Diversity: Interpersonal Congruence in Small Work Groups.” Administrative Science Quarterly . 47(2): 296–324.
Smith, Edward (Ned), and Yuan Hou. 2015. “Redundant Heterogeneity and Group Performance.” Organization Science . 26(1): 37–51.
Wang, Long, and J. Keith Murnighan. 2013. “The Generalist Bias.” Organizational Behavior and Human Decision Processes . 120(1): 47–61.
Woolley, Anita W., Margaret E. Gerbasi, Christopher F. Chabris, Stephen M. Kosslyn, and J. Richard Hackman. 2008. “Bringing in the Experts: How Team Composition and Collaborative Planning Jointly Shape Analytic Effectiveness.” Small Group Research . 39(3): 352–371.
Mukherjee, Satyam, Yun Huang, Julia Neidhardt, Brian Uzzi and Noshir Contractor. 2018. “Prior Shared Success Predicts Victory in Team Competitions.” Nature Human Behaviour . 3: 74–81.
Petersen, Alexander Michael. 2015. “Quantifying the Impact of Weak, Strong, and Super Ties in Scientific Careers.” PNAS . 112(34): E4671–E4680.
Uzzi, Brian, and Jarrett Spiro. 2005. "Collaboration and Creativity: The Small World Problem." American Journal of Sociology. 111(2): 447–504.
Choi, Hoon-Seok, and Leigh Thompson. 2005. “Old Wine in a New Bottle: Impact of Membership Change on Group Creativity.” Organizational Behavior and Human Decision Processes . 98(2): 121-132.
Cornelli, Francesca, Elena Simintzi, and Vikrant Vig. 2019. “Team Stability and Performance: Evidence from Private Equity.” Working paper.
Uzzi, Brian, Satyam Mukherjee, Michael Stringer, and Ben Jones. 2013. “Atypical Combinations and Scientific Impact.” Science . 342(6157): 468–472.
Wu, Lingfei, Dashun Wang, and James A. Evans. 2019. "Large Teams Develop and Small Teams Disrupt Science and Technology." Nature. 566(7742): 378–382.
Garicano, Luis, and Thomas N. Hubbard. 2018. "Earnings Inequality and Coordination Costs: Evidence from U.S. Law Firms." Journal of Law, Economics, & Organization . 34.
Thank you for visiting nature.com. You are using a browser version with limited support for CSS. To obtain the best experience, we recommend you use a more up to date browser (or turn off compatibility mode in Internet Explorer). In the meantime, to ensure continued support, we are displaying the site without styles and JavaScript.
- View all journals
- My Account Login
- Explore content
- About the journal
- Publish with us
- Sign up for alerts
- Open access
- Published: 18 April 2024
A method for identifying different types of university research teams
- Zhe Cheng ORCID: orcid.org/0009-0002-5120-6124 1 ,
- Yihuan Zou 1 &
- Yueyang Zheng ORCID: orcid.org/0000-0001-7751-2619 2
Humanities and Social Sciences Communications volume 11 , Article number: 523 ( 2024 ) Cite this article
Metrics details
Identifying research teams constitutes a fundamental step in team science research, and universities harbor diverse types of such teams. This study introduces a method and proposes algorithms for team identification, encompassing the project-based research team (Pbrt), the individual-based research team (Ibrt), the backbone-based research group (Bbrg), and the representative research group (Rrg), scrutinizing aspects such as project, contribution, collaboration, and similarity. Drawing on two top universities in Materials Science and Engineering as case studies, this research reveals that university research teams predominantly manifest as backbone-based research groups. The distribution of members within these groups adheres to Price’s Law, indicating a concentration of research funding among a minority of research groups. Furthermore, the representative research groups in universities exhibit interdisciplinary characteristics. Notably, significant differences exist in collaboration mode and member structures among high-level backbone-based research groups across diverse cultural backgrounds.
Similar content being viewed by others
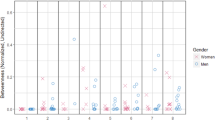
Towards understanding the characteristics of successful and unsuccessful collaborations: a case-based team science study
Hannah B. Love, Bailey K. Fosdick, … Ellen R. Fisher
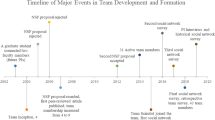
Interpersonal relationships drive successful team science: an exemplary case-based study
Hannah B. Love, Jennifer E. Cross, … Ellen R. Fisher
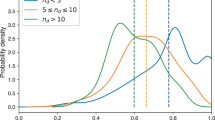
Mixing Patterns in Interdisciplinary Co-Authorship Networks at Multiple Scales
Shihui Feng & Alec Kirkley
Introduction
Team science has emerged as a burgeoning field of inquiry, attracting the attention of numerous scholars (e.g., Stokols et al., 2008 ; Bozeman & Youtie, 2018 ; Coles et al., 2022 ; Deng et al., 2022 ; Forscher et al., 2023 ), who endeavor to explore and try to summarize strategies for fostering effective research teams. Conducting team science research would help improve team efficacy. The National Institutes of Health in the USA pointed out that team science is a new interdisciplinary field that empirically examines the processes by which scientific teams, research centers, and institutes, both large and small, are structured (National Research Council, 2015 ). In accordance with this conceptualization, research teams can be delineated into various types based on their size and organizational form. Existing research also takes diverse teams as focal points when probing issues such as team construction and team performance. For example, Wu et al. ( 2019 ) and Abramo et al. ( 2017 ) regard the co-authors of a single paper as a team, discussing issues of research team innovation and benefits. Meanwhile, Zhao et al. ( 2014 ) and Lungeanu et al. ( 2014 ) consider the project members as a research team, exploring issues such as internal interest distribution and team performance. Boardman and Ponomariov ( 2014 ), Lee et al. ( 2008 ), and Okamoto and Centers for Population Health and Health Disparities Evaluation Working Group ( 2015 ) view the university’s research center as a research group, investigating themes about member collaboration, management, and knowledge management portals.
Regarding the definition of research teams, some researchers believe that a research team is a collection of people who work together to achieve a common goal and discover new phenomena through research by sharing information, resources, and professional expertise (Liu et al., 2020 ). Conversely, others argue that groups operating across distinct temporal and spatial contexts, such as virtual teams, do not meet the criteria for teams, as they engage solely in collaborative activities between teams. According to this perspective, Research teams should be individuals collaborating over an extended period (typically exceeding six months) (Barjak & Robinson, 2008 ). Contemporary discourse on team science tends to embrace a broad conceptualization wherein research teams include both small-scale teams comprising 2–10 individuals and larger groups consisting of more than 10 members (National Research Council, 2015 ). These research teams are typically formed to conduct a project or finish research papers, while research groups are formed to solve complex problems, drawing members from diverse departments or geographical locations.
Obviously, different research inquiries are linked to different types of research teams. Micro-level investigations, such as those probing the impact of international collaboration on citations, often regard co-authors of research papers as research teams. Conversely, meso-level inquiries, including those exploring factors impacting team organization and management, often view center-based researchers as research groups. Although various approaches can be adopted to identify research teams, such as retrieving names from research centers’ websites or obtaining lists of project-funded members, when the study involves a large sample size and requires more data to measure the performance of research teams, it becomes necessary to use bibliometric methods for team identification.
Existing literature on team identification uses social network analysis (Zhang et al., 2019 ), cohesive subgroup (Dino et al., 2020 ), faction algorithm (Imran et al., 2018 ), FP algorithm (Liao, 2018 ), etc. However, these identification methods often target a singular type of research team or fail to categorize the identified research teams. Moreover, existing studies mostly explore the evolution of specific disciplines (Wang et al., 2017 ), with limited attention devoted to identifying university research teams and the influencing factors of team effectiveness. Therefore, this study tries to develop algorithms to identify diverse university research teams, drawing insights from two universities characterized by different cultural backgrounds. It aims to address two research questions:
How can we identify different types of university research teams?
What are the characteristics of research groups within universities?
Literature review
Why is it necessary to identify research teams? The research focuses on scientific research teams, mostly first identifying the members of research teams through their names on the list of funding projects or institutions’ websites and then conducting research through questionnaires or interviews. However, this methodology may compromise research validity for several reasons. Firstly, the mere inclusion of individuals on funding project lists does not guarantee genuine research team membership or substantive collaboration among members. Secondly, the institutional website generally announces important research team members, potentially overlooking auxiliary personnel or important members from external institutions. Thirdly, reliance solely on lists of research team members fails to capture nuanced information about the team, such as their research ability or communication intensity, thus hindering the exploration of team science-related issues.
Consequently, researchers have turned to co-authorship and citation to identify research teams using established software tools and customized algorithms. For example, Li and Tan ( 2012 ) applied UCINET and social network analysis to identify university research teams, while Hu et al. ( 2019 ) used Citespace to analyze research communities of four disciplines in China, the UK, and the US. Similarly, some researchers also identify the members and leaders of research teams by using and optimizing existing algorithms. For example, Liao ( 2018 ) applied the Fast-Unfolding algorithm to identify research teams in the field of solar cells, while Yu et al. ( 2020 ) and Li et al. ( 2017 ) employed the Louvain community discovery algorithm to identify research teams in artificial intelligence. Lv et al. ( 2016 ) applied the FP-GROWTH algorithm to identify core R&D teams. Yu et al. ( 2018 ) used the faction algorithm to identify research teams in intelligence. Dino et al. ( 2020 ) developed the CL-leader algorithm to confirm research teams and their leaders. Boyack and Klavans ( 2014 ) regard researchers engaged in the same research topic as research teams based on citation information. Notably, these community detection algorithms complement each other, offering versatile tools for identifying research teams.
Despite the utility of these identification methods, they are not without limitations. For example, fixed software algorithms are constrained by predefined rules, posing challenges for researchers seeking to customize identification criteria. Moreover, for developed algorithms, although algorithms based on computer programming languages have high accuracy, they overemphasize the connection relationship between members and do not consider the definition of research teams. In addition, research based on co-authorship networks and community identification algorithms faces inherent problems: (1) Ensuring temporal consistency in co-authorship networks is challenging due to variations in publication timelines, potentially undermining the temporal alignment of team member collaborations; (2) The lack of stability in team identification result means that different identification standards would produce different outcomes; (3) Team members only belong to one research team, but in the actual process, researchers often participate in multiple research teams with different identities, or the same members conduct research in different team combinations.
In summary, research teams in a specific field can be identified using co-authorship information, designing or introducing identification algorithms. However, achieving more accurate identification necessitates consideration of the nuanced definition of research teams. Therefore, this study focuses on university research teams, addressing temporal and spatial collaboration issues among team members by incorporating project information and first-author information. Furthermore, it tackles the issue of classifying research team members by introducing Price’s Law and Everett’s Rule. Additionally, it tackles the issue of team members’ multiple affiliations through the Jaccard Similarity Coefficient and the Louvain Algorithm. Ultimately, this study aims to achieve the classification recognition of university research teams.
Team identification method
An effective team identification method requires both consideration of the definition of research teams and the ability to transform this definition into operable programming languages. University research teams, by definition, comprise researchers collaborating towards a shared objective. As a typical form of the output of a research team, the co-authorship of a scientific research paper implies information exchange and interaction among team members. Thus, this study uses co-authorship relationships within papers to reflect the collaborative relationships among research team members. In this section, novel algorithms for identifying research teams are proposed to address deficiencies observed in prior research.
Classification of research team members
A researcher might be part of multiple research teams, with varying roles within each. Members of the research team can be categorized according to how the research team is defined.
The original idea of team member classification
The prevailing notion of teams underscores the collaborative efforts between individual team members and their contributions toward achieving research objectives. This study similarly classifies team members based on these dual dimensions.
In terms of overall contributions, members who make substantial contributions are typically seen as pivotal figures within the research team, providing the primary impetus for the team’s productivity. Conversely, those with lesser input only contribute to specific facets of the team’s goals and engage in limited research activities, thus being regarded as standard team members.
In terms of collaboration, it is essential to recognize that high levels of contribution do not inherently denote a core position within a team. The collaboration among team members serves as an important indicator of their identity characteristics within the research team. Based on the collaboration between members, this study believes that researchers who have high contributions and collaborate with many high-contribution team members assume the core members of the research team. Conversely, members who have high contributions but only collaborate with a limited number of high-contribution team members are identified as backbone members. Similarly, members displaying low levels of contributions but collaborating widely with high contributors are categorized as ordinary members. Conversely, those with low contributions and limited collaboration with high-contributing team members are regarded as marginal members of the research team.
Establishment of team member classification criteria
This study introduces Price’s Law and Everett’s Rule to realize the idea of team member classification.
In terms of overall contribution, the well-known bibliometrics Price, drawing from Lotka’s Law, deduced that the number of papers published by prolific scientists is 0.749 times the square root of the number of papers published by the most prolific scientist in a group. Existing research also used this law when analyzing prolific authors of an organization. This study believes that prolific authors who conform to Price’s Law are important members who contribute more to the research team.
In terms of collaboration, existing research mostly employs the concept of factions. Factions refer to a relationship where members reciprocate and cannot readily join new groups without altering the reciprocal nature of their factional ties. However, in real-world settings, relationships with overtly reciprocal characteristics are uncommon. Therefore, to ensure the applicability and stability of the faction, Seidman and Foster ( 1978 ) proposed the concept of K-plex, pointing out that in a group of size n, when the number of direct connections of any point in the group is not less than n-k, this group is called k-plex. For k-plex, as the number k increases, the stability of the entire faction will decrease. Addressing this concern, renowned sociologist Martin Everett ( 2002 ), based on the empirical rule of research, proposed specific values for k and corresponding minimum group sizes, stipulating that the overall team size should not fall below 2k-1 (Scott, 2017 ). The expression is:
In other words, for a K-plex, the most acceptable definition to qualify as a faction is when each member of the team is directly connected to at least ( n − 1)/2 members of the team. Applied to research teams, this empirical guideline necessitates that team members maintain collaborative ties with at least half or more of the team.
Based on Price’s Law and Everett’s Empirical Rule, this study gives the criteria for distinguishing prolific authors, core members, backbone members, ordinary members, and marginal members of research teams. The specifics are shown in the following Table 1 .
Classification of research teams
Within universities, a diverse array of research teams exists, categorized by their scale, the characteristics of funded projects, and the platforms they rely upon. This study proposes the identification algorithms for project-based teams, individual-based teams, backbone-based groups, and representative groups.
Project-based research teams: identification based on research projects
Traditional methods for identifying research teams attribute co-authorship to collaboration among multiple authors without considering the time scope. However, in practice, collaborations vary in content and duration. Therefore, in the identification process, it is necessary to introduce appropriate standards to distinguish varying degrees of collaboration and content among scholars.
Research projects serve as evidence of researchers engaging in the same research topic, thereby indicating that the paper’s authors belong to the same research team. Upon formal acceptance of a research paper, authors typically append funding information to the paper. Therefore, papers sharing the same funding information can be aggregated into paper clusters to identify the research team members who completed the fund project. The specific steps proposed for identifying a single research project fund are as follows.
Firstly, extract the funding number and regard all papers attached with the same funding number as a paper cluster. Secondly, construct a co-authorship network based on the paper cluster. Thirdly, identify the research team using the team member classification criteria.
Individual-based research teams: team identification based on the first author
For research papers lacking project numbers, clustering can be performed based on the contribution and research experience of the authors. Each co-author of the research paper contributes differently to the paper’s content. In 2014, the Consortia Advancing Standards in Research Administration Information (CASRAI) proposed classification standards for paper contributions, including 14 types such as conceptualization, data processing, formal analysis, funding acquisition, investigation, methods, project management, resources, software, supervision, validation, visualization, paper writing, review, and editing.
In this study, the primary author of a paper lacking project funding is considered the initiator, while other authors are seen as contributors who advance and finalize the research. For papers not affiliated with any project, the first author and all their published papers form a paper group for team identification purposes. The procedure entails the following steps: Initially, gather the first author and all papers authored by them within the identification period to constitute a paper group. Subsequently, a co-authorship network will be constructed using the papers within the group. Lastly, the research team will be identified based on the criteria for classifying team members.
Backbone-based research group: merging based on project-based and individual-based research teams
Research teams can be identified either by a single project number or by individual researchers. Upon identification, it becomes evident that many research teams share similar members. This is because a research team may engage in multiple projects, and some members collaborate without funding support. While identification algorithms are suitable for evaluating the quality of a research article or funding, they may not suffice when assessing the research group, or they may not suffice when assessing the key factors affecting their performance. To address this, it is necessary to merge highly similar individual-based or project-based research teams according to specific criteria. The merged one should be termed a group, as it encompasses multiple project-based and individual-based research teams.
In the pursuit of building world-class universities, governments worldwide often emphasize the necessity of fostering research teams led by discipline backbones. In this vein, this study further develops a backbone-based research group identification algorithm, which considers project-based and individual-based research teams.
Identification of university discipline backbone members
Previous studies have summarized the characteristics of the university discipline backbones, revealing that these individuals often excel in indicators such as degree centrality, eigenvector centrality, and betweenness centrality. Each centrality indicator demonstrates a strong positive correlation with the author’s output volume, indicating that high-productive researchers with more collaborators are more inclined to be university discipline backbones. Based on these characteristics, Price’s law is applied, defining discipline backbone members as researchers whose publications count exceeds 0.749 times the square root of the highest publication count within the discipline.
Team identification with discipline backbone members as the Core
Following the identification of discipline backbones, this study consolidates paper groups wherein the discipline backbone serves as the core member of either individual-based or project-based research teams. Subsequently, backbone-based research groups are formed.
Merging based on similarity perspective
It should be noted that different discipline backbones may simultaneously participate as core members in the same individual-based or project-based research teams. Consequently, distinct backbone-based research groups may encompass duplicate project-based and individual-based research teams, necessitating the merging of backbone-based research groups.
To address this redundancy issue, this study introduces the concept of similarity in community identification. In the community identification process, existing algorithms often assess whether to incorporate members into the community based on their level of similarity. Among various algorithms for calculating similarity, the Jaccard coefficient is deemed to possess superior validity and robustness in merging nodes within network communities (Wang et al., 2020 ). Its calculation formula is as follows.
N i denotes the nodes within subset i , while N j represents the nodes within subset j ; N i ∩ N j signifies the nodes present in both subsets, whereas N i ∪ N j encompasses all nodes in subsets i and j . Existing research shows that when the Jaccard coefficient equals or exceeds 0.5 (Guo et al., 2022 ), the community identification algorithm achieves optimal precision.
In the context of this study, N i represents the core and backbone members of research group i , while N j denotes the core and backbone members of research group j . If these two groups exhibit significant overlap in core and backbone members, the papers from both research groups are merged into a new set of papers to identify the research team.
Given the efficacy of the Jaccard similarity measure in identifying community networks and merging, this study employs this principle to merge backbone-based research groups. Specifically, groups are merged if the Jaccard similarity coefficient between their core and backbone members equals or exceeds 0.5. Subsequently, new research groups are formed based on the merged set of papers.
It’s important to note that during the merging process, certain research teams within a backbone-based group may be utilized multiple times. Initially, the merging occurs based on the core and backbone members of the backbone-based research group, adhering to the Jaccard coefficient criterion. However, since project or individual-based research teams within a backbone-based research group may be reused, resulting in the similarity of research papers across different groups, the study further tested the team duplication of the merged papers of various groups. During the research process, it was found that the research papers within groups often exhibit similarity due to their association with multiple funding projects. Therefore, a principle of “if connected, then merged” was adopted among groups with highly similar research papers to ensure the heterogeneity of papers within the final merged research groups.
The generation process of the backbone-based research groups is illustrated in Fig. 1 below. Initially, university discipline backbones α, β, γ, θ, δ, and ε are each designated as core members within project-based or individual-based research teams A, B, C, D, E, and F, among which αβγ, γθ, θδ, δε ‘s core and backbone members’ Jaccard coefficient meet the merging standard and generate lines. After the first merging, the Jaccard coefficient of the papers of the αβγ, γθ, θδ, δε are calculated, and the lines are generated because of a high duplicated papers between γθ, θδ, and θδ, δε. Finally, αβγ and γθδε are retained based on the rule.
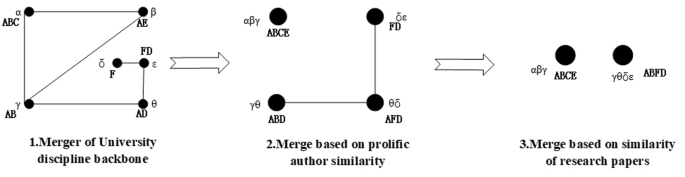
The α, β, γ, θ, δ, and ε are core members within project-based or individual-based research teams. The A, B, C, D, E, and F are project-based or individual-based research teams. From step 1 to step 2, research groups are merged according to the Jaccard coefficient between research team members. From step 2 to step 3, research groups are merged according to the Jaccard coefficient between research group papers.
In summary, the process of identifying a backbone-based research group involves the following steps: (1) Identify prolific authors within the university’s discipline by analyzing all papers published in the field, considering them as the discipline’s backbones members; (2) Merge the project-based and individual-based research teams wherein university discipline backbones are core member, thereby forming backbone-based research groups; (3) Merge the backbone-based research group identified in step (2) based on the Jaccard coefficient between their core and backbone members; (4) Calculate the Jaccard coefficient of the papers of the merged groups in step (3), merge the groups with significant paper overlap, and generate new backbone-based research groups.
The research groups identified through the above steps offer two advantages: Firstly, they integrate similar project-based and individual-based research teams, avoiding redundancy in team identification outcomes. Secondly, the same member may participate in different research teams, assuming distinct roles within each, thus better reflecting the complexity of scientific research practices.
Representative team: consolidation via backbone-based research group
When universities introduce their research groups to external parties, they typically highlight the most significant research members within the institution. Although the backbone-based research group has condensed the project-based and individual-based research teams, there may still be some overlap among members from different backbone-based research groups.
In order to create condensed and representative research groups that accurately reflect the development of the university’s discipline, this study extracts the core and backbone members identified in the backbone-based research group. It then identifies the representative group using the widely utilized Louvain algorithm (Blondel et al., 2008 ) commonly employed in research group identification. This algorithm facilitates the integration of important members from different backbone-based research groups while ensuring there is no redundancy among group members. The merging process is shown in Fig. 2 .
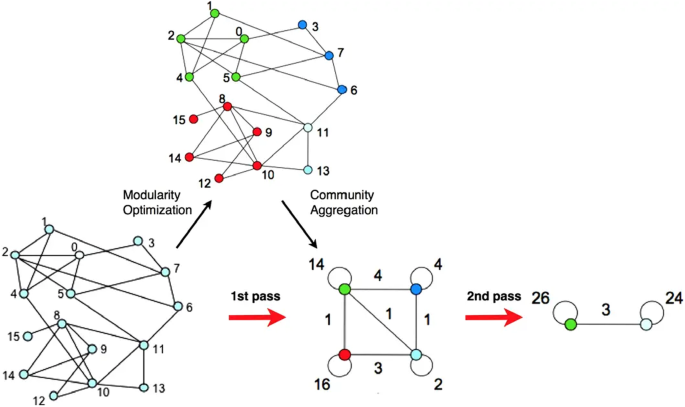
Each pass is made of two phases: one where modularity is optimized by allowing only local changes of communities, and one where the communities found are aggregated in order to build a new network of communities. The passes are repeated iteratively until no increase in modularity is possible.
Research team identification process and its pros and cons
Overall, the method of identifying university research teams proposed in this research encompasses four stages: Initially, research teams are categorized into project-based research teams and individual-based research teams based on information provided with research papers, distinguishing between those supported by funding projects and those not. Subsequently, the prolific authors of universities are identified to combine individual-based and project-based research teams, and backbone-based research groups are generated. Finally, representative research groups are established utilizing the Louvain algorithm and the interrelations among members within the backbone-based research groups. The entire process is depicted in Fig. 3 below.
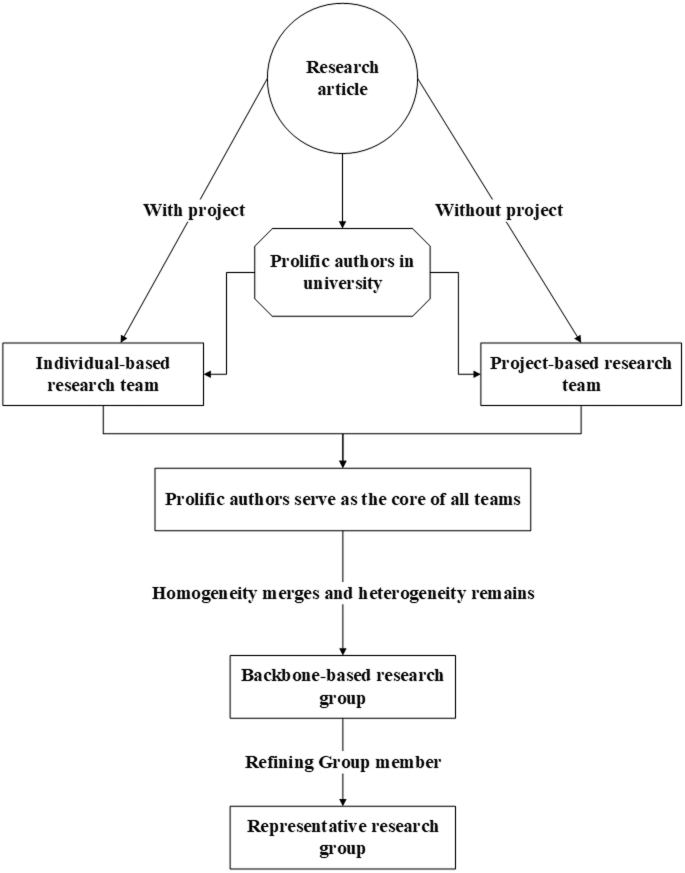
Different university research teams are identified at different stage.
Each type of research team or group has its advantages and disadvantages, as shown in Table 2 below.
Validation of identification results
In order to verify the accuracy of the identification results, the method proposed by Boyack and Klavans ( 2014 ), which relies on citation analysis, is utilized. This method calculates the level of consistency regarding the main research areas of the core and backbone members, thereby verifying the validity of the identification method.
In the SCIVAL database, all research papers are clustered into relevant topic groups, providing insights into the research area of individual authors. By examining the research topic clusters of team papers in the SCIVAL database, the predominant research areas of prolific authors can be determined. Authors sharing common research areas within a university are regarded as constituting a research team. Given that authors often conduct research in various research areas, this study focuses solely on the top three research areas for each author.
As demonstrated in Table 3 below, for the prolific authors A, B, C, D, and E of the research team, their top three research areas collectively span five distinct fields. By calculating the highest value of the consistency among these research areas, it can be judged whether these researchers can be classified as members of the same research group. As depicted in Table 3 , the main research areas of all prolific authors include Research Area 3, indicating that this field is one of the three most important research areas for all prolific authors. This consistency validates that the main research areas of the five authors align, affirming their classification within the same research team.
Data collection and preprocessing
In order to present the distinct characteristics of various types of scientific research teams as intuitively as possible, this study focuses on the field of material science, with Tsinghua University and Nanyang Technological University selected for analysis. The selection of these two institutions is driven by several considerations: (1) both universities boast exceptional performance in the field of material science on a global scale, consistently ranking within the top 10 worldwide for numerous years; (2) The scientific research systems in the respective countries where these universities are situated differ significantly. China’s scientific research system operates under a government-led funding model, whereas Singapore’s system involves a multi-party funding approach with contributions from the government, enterprises, and societies. By examining universities from these distinct scientific research cultures, this study aims to validate the proposed methods and highlight disparities in the characteristics of their scientific research teams. (3) Material science is inherently interdisciplinary, with contributions from researchers across various domains. Although the selected papers focus on material science, they may also intersect with other disciplines. Therefore, investigating research teams in material science could somewhat represent the interdisciplinary research teams.
The data utilized in this study is sourced from the Clarivate Analytics database, which categorizes scientific research papers based on the subject classification catalogs. In order to ensure the consistency and reliability of scientific research paper identification, this study focuses on the papers published in the field of material science by the two selected universities between 2017 and 2021. Additionally, considering the duration of funded projects, papers associated with projects that have appeared in 2017–2021 within ten years (2011–2022) are also included for analysis to enhance the precision of identification. In order to ensure the affiliation of a research team with the respective universities, this study exclusively considers papers authored by the first author or the corresponding author affiliated with the university as the subject of analysis.
Throughout this process, it should be noted that the name problem in identifying scientific research. Abbreviations, orders, and other name-related information are cleaned and verified. Given that this study exports data utilizing the Author’s Full name and restricts it to specific universities and disciplines, the cleaning process targets the rectification of identification discrepancies arising from a minority of abbreviations and similar names. The specific cleaning procedures entail the following steps.
First, all occurrences of “-” are replaced with null values, and names are standardized by capitalization. Second, the Python dedupe module is employed to mitigate ambiguity in author names, facilitating the differentiation or unification of authors sharing the same surname, name, and initials. List and output all personnel names of each university in this discipline and observe in ascending order. Third, a comparison of names and abbreviations is conducted in reverse order, alongside their respective affiliations and replacements in the identification data. For example, names such as “LONG, W.H” “LONG, WEN, HUI” and “LONG, WENHUI” are uniformly replaced with “LONG, WENHUI.” Fourth, identify and compare similar names in both abbreviations and full forms and confirm whether they are consistent by scrutinizing their affiliations and collaborators. Names exhibiting consistency are replaced accordingly, while those lacking uniformity remain unchanged. For example, “LI, W.D” and “LI, WEIDE” lacking common affiliations and collaborators, are not considered the same person and thus remain distinct.
The publication of the two universities in the field of Materials Science and Engineering across two distinct time periods is shown in Table 4 below.
Based on the publication count of papers authored by the first author or corresponding author from both universities, Tsinghua University demonstrates a significantly higher publication output than Nanyang Technological University, indicating a substantial disparity between the two institutions.
Subsequent to data preprocessing, this study uses the Python tool to develop algorithms in accordance with the proposed principles, thereby facilitating the identification of research teams and groups.
This study has identified several research teams through the sorting and analysis of original data. In order to provide a comprehensive overview of the identification results, this study begins by outlining the characteristics of the identification results and then analyzes the research teams affiliated with both universities, focusing on three aspects: scale, structure, and output.
Identification results of university research teams
The results reveal that both Tsinghua University and Nanyang Technological University boast a considerable number of Pbrts, indicating that most of the researchers from both universities have received funding support. Additionally, a small number of teams have not received funding support, although their overall proportion is relatively low. The Bbrgs predominantly encompass the majority of the Ibrts and Pbrts, underscoring the significant influence of the discipline backbone members within both universities. Notably, the total count of Rrg across the two universities stands at 39, reflecting that many research groups are supporting the construction of material disciplines in the two universities (Table 5 ).
In order to validate the accuracy of the developed method, this study verifies the effectiveness of the identification algorithm. Given that the method emphasizes the main research area of its members, it is appropriate to apply it to the verification of the Bbrgs, which encompass the majority of the individual-based and project-based teams.
The analysis reveals that the consistency level of the most concentrated research area within the identified Bbrgs is 0.93. This signifies that within a Bbrg comprising 10 core or backbone members, a minimum of 9.3 individuals share the same main research area. Moreover, across Bbrgs of varying sizes, the average consistency level of the most concentrated research area also reached 0.90, indicating that the algorithm proposed in this study is valid (Table 6 ).
Analysis of the characteristics of Bbrg in universities
The findings of the analysis show that the Bbrgs encompass the vast majority of Pbrts and Ibrts within universities. Consequently, this study further analyzes the scale, structure, and output of the Bbrgs to present the characteristics of university research teams.
Group scale
Upon scrutinizing the distribution of Bbrgs across the two universities, it is observed that the number of core members is similar. Bbrg with a core member scale of 6–10 individuals are the most prevalent, followed by those with a scale of 0–5 members. Additionally, there are Bbrgs comprising 11–15 members, with relatively fewer Bbrgs consisting of 15 members or more. On average, the number of core members in Bbrgs stands at 7.08. Tsinghua University has more Bbrgs than Nanyang Technological University, while the average number of core members is relatively less. Notably, the proportion of core and backbone members amounts to nearly 12%, ranging from 11.22% to 13.88% (Table 7 ).
Group structure
The structural attributes of the research groups could be assessed through network density among core members, core and backbone members, and all team members. Additionally, departmental distribution can be depicted based on the identification of core members and their organizational affiliations. The formula for network density calculation is as follows:
Note : R is the number of relationships, and N is the number of members.
Overall, the network density characteristics exhibit consistency across both universities. Specifically, the network density among research group members tends to decrease as the group size expands. The network density among core members is the highest, while that among all members records the lowest. Comparatively, the average amount of various types of network density at Tsinghua University is relatively lower than that at Nanyang Technological University, indicating a lesser degree of connectivity among members within Tsinghua University’s research group. However, the network density levels among core members and core and backbone members of research teams in both institutions remain relatively high. Notably, the network density of backbone-based research groups exceeds 0.5, indicating a close collaboration among the core and backbone members of these university research groups (Table 8 ).
The T-test analysis reveals no significant difference in the network density among core members between Tsinghua University and Nanyang Technological University. This suggests that core members of research groups from universities with high-level discipline often maintain close communication. However, concerning the network density among core and backbone members and all members, the average amount of Tsinghua University’s research groups is significantly lower than those of Nanyang Technological University. This implies less direct collaboration among prolific authors at Tsinghua University, with backbone members relying more on different core members of the group to carry out research.
To present the cooperative relationship among the core and backbone members of the Bbrgs, the prolific authors associated with the backbone-based research groups are extracted. Subsequently, the representative research groups affiliated with Nanyang Technological University and Tsinghua University are identified using the fast-unfolding algorithm. The resultant collaboration network diagram among prolific authors is depicted in Fig. 4 , wherein each node color corresponds to different representative research groups of the respective universities.
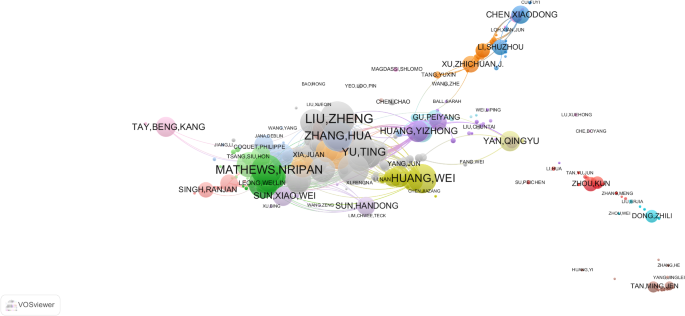
Nodes (author) and links (relation between different authors) with the same color could be seen as the same representative research group.
The network connection diagram of Nanyang Technological University illustrates the presence of 39 Rrgs, including Rrgs from the School of Materials Science and Engineering and the Singapore Centre for 3D Printing. Owing to the inherently interdisciplinary characteristics of the materials discipline, its research groups are not only distributed in the School of Materials Science and Engineering; other academic units also have research groups engaged in materials science research.
Further insights into the distribution of research groups can be gleaned by examining the departments to which the primary members belong. Counting the departmental affiliations of the members with the highest centrality in each representative team reveals that, among the 39 Rrgs, the School of Materials Science and Engineering and the College of Engineering boast the highest number of affiliations, with nine core members of the research groups coming from these two departments, Following closely is the School of Physical and Mathematical Sciences. Notably, entities external to the university, such as the National Institute of Education and the Singapore Institute of Manufacturing Technology, also host important representative groups, underscoring the interdisciplinarity nature of material science. The distribution of Rrgs affiliations is delineated in Table 9 .
Similar to Nanyang Technological University, Tsinghua University also exhibits tightly woven connections within its backbone-based research group in Materials Science and Engineering, comprising a total of 39 Rrgs. Compared with Nanyang Technological University, Tsinghua University boasts a larger cohort of core and backbone members. The collaboration network diagram of representative groups is shown below (Fig. 5 ).
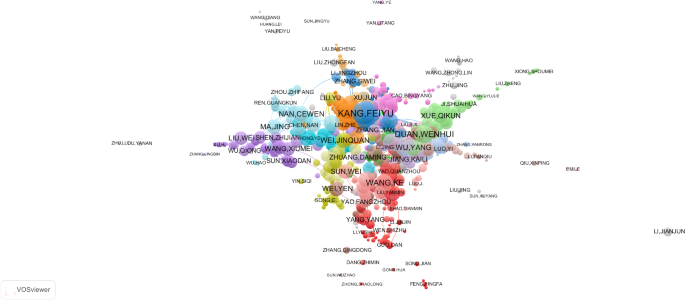
Similar to Nanyang Technological University, representative research groups at Tsinghua University are distributed in different schools within the institution, with the School of Materials being the directly related department. In addition, the School of Medicine and the Center for Brain-like Computing also conduct research related to materials science (Table 10 ).
By summarizing the departmental affiliations of the research groups, it becomes evident that the Rrgs in Materials Science and Engineering at these universities span various academic departments, reflecting the interdisciplinary characteristics of the field. The network density of the research groups is also calculated, with Nanyang Technological University exhibiting a higher density (0.028) compared to Tsinghua University (0.022), indicating tighter connections within the representative research groups at Nanyang Technological University.
Group output
In order to control the impact of scale, this study compares several metrics, including publication, publication per capita of core and backbone members, capita of the most prolific author within the groups, field-weighted citation impact, and citations per publication of Bbrgs at these two top universities.
Regarding publications, the average number and the T-test results show that Tsinghua University significantly outperforms Nanyang Technological University, suggesting that the Bbrgs and prolific authors affiliated with Tsinghua University are more productive in terms of research output.
However, in terms of field-weighted citation impact and citations per publication of the Bbrgs, the average number and the T-test results show that Tsinghua University is significantly lower than that of Nanyang Technological University, which indicates the research papers originating from the Bbrgs at Nanyang Technological University have a greater academic influence (see Table 11 ).
Typical cases
To intuitively present the research groups identified, this study has selected the two Bbrgs with the highest number of published papers at Tsinghua University and Nanyang Technological University for analysis, aiming to offer insights for constructing research teams.
Basic Information of the Bbrgs
Examining the basic information of the Bbrgs reveals that although Kang Feiyu’s group at Tsinghua University comprises fewer researchers than Liu Zheng’s group at Nanyang Technological University, Kang Feiyu’s group has a higher total number of published papers. In order to measure the performance of the research results of these two Bbrgs, the field-weighted citation impact of their research papers was queried using SCIVAL. The results showed that the field-weighted citation impact of Kang Feiyu’s group at Tsinghua University was higher, indicating a greater influence in the field of Materials Science and Engineering. Furthermore, the identity information of the two group leaders was compared. It was found that Kang Feiyu, in addition to being a professor at Tsinghua University, holds administrative positions as the dean of the Shenzhen Graduate School of Tsinghua University. Meanwhile, LIU, Zheng, mainly serves as the chairman of the Singapore Materials Society alongside his role as a professor (see Table 12 ).
Characteristics of team member network structure
In order to reflect the collaboration characteristics of research groups, this study calculates the network density of the two groups and utilizes VOSviewer to present the collaboration network diagrams of their members.
In terms of network density, both groups exhibit a density of 1 among core members, indicating that the collaboration between core members is tight. However, regarding the network density of core and backbone members, as well as all members, Liu Zheng’s group at Nanyang Technological University demonstrates a higher density. This indicates a stronger interconnectedness between the backbone and other members within the group (refer to Table 13 ).
For the co-authorship network diagram of group members, distinctive characteristics are observed between the two Bbrgs. In Kang Feiyu’s team, the core members exhibit prominence, with sub-team structures under evident each team member (Fig. 6 ). Conversely, while Liu Zheng’s team also features different core members, the centrality within each member is not obvious (Fig. 7 ).
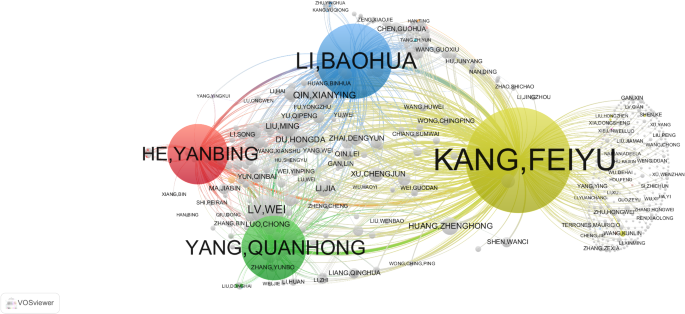
Nodes (author) and links (relation between different authors) with the same color could be seen as the same sub-team.
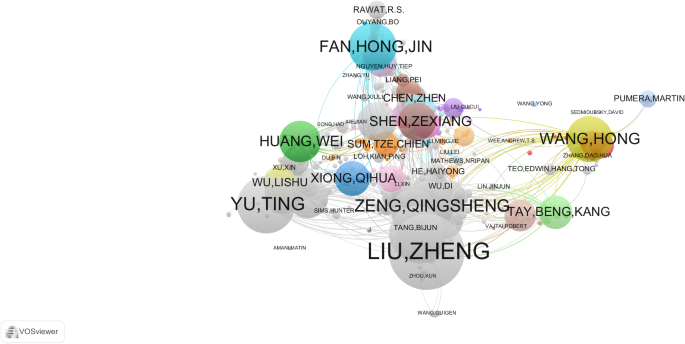
Discussion and conclusion
Distinguishing different research teams constitutes the foundational stage in conducting team science research. In this study, we employ Price’s Law, Everett’s Rule, Jaccard Similarity Coefficient, and Louvain Algorithm to identify different research teams and groups in two world-leading universities specializing in Materials Science and Engineering. Through this exploration, we aim to explore the characteristics of research teams. The main findings are discussed as follows.
First, based on the co-authorship and project data from scholarly articles, this study develops a methodology for identifying research teams that distinguishes between different types of research teams or groups. In contrast to the prior identification method, our algorithms could identify different types of research teams and realize the member classification within research teams. This affords greater clarity regarding collaboration time and content among team members. The validation of identification results, conducted using the methodology proposed by Boyack and Klavans ( 2014 ), demonstrates the consistency of the main research areas among identified research group members. This validation shows the accuracy and efficacy of the research team identification methodology proposed in this study.
Second, universities have different types of research teams or groups, encompassing both project-based research teams and individual-based research teams lacking project support. Among these, most research teams rely on projects to conduct research (Bloch & Sørensen, 2015 ). Concurrently, this research finds that university research groups predominantly coalesce around eminent scholars, with backbone-based research groups comprising the majority of both project-based and individual-based research teams. This phenomenon shows the concentration of research resources within a select few research groups and institutions, a concept previously highlighted by Mongeon et al. ( 2016 ), who pointed out that research funding tends to be concentrated among a minority of researchers. In this research, we not only corroborate this assertion but also observe that researchers with abundant funding collaborate to form research groups, thereby mutually supporting each other. In addition, based on the structures of research groups at Nanyang Technological University and Tsinghua University, one could posit that these institutions resemble what might be termed a “rich club” (Ma et al., 2015 ). However, despite the heightened productivity of relatively concentrated research groups at Tsinghua University in terms of research output, their academic influence pales compared to that of Nanyang Technological University. To enhance research influence, it seems that the funding agency should curtail funding allocations to these “rich” research groups and instead allocate resources to support more financially challenged research teams. This approach would serve to alleviate the trend of concentration in research project funding, as suggested by Aagaard et al. ( 2020 ).
Thirdly, research groups in Material Science and Engineering exhibit obvious interdisciplinary characteristics. Despite all research papers being classified under the Material Science and Engineering discipline, the distribution of research groups across various academic departments suggests a pervasive interdisciplinary nature. This phenomenon underscores the interconnectedness of Materials Science and Engineering with other disciplines and serves as evidence that members from diverse departments within high-caliber universities actively engage in collaborative efforts. Previous research conducted in the United Kingdom has revealed that interdisciplinary researchers from arts and humanities, biology, economics, engineering and physics, medicine, environmental sciences, and astronomy occupy a pivotal position in academic collaboration and can obtain more funding (Sun et al., 2021 ). In this research, similar conclusions are also found in Material Science and Engineering.
Fourth, the personnel structure distribution in university research groups adheres to Price’s Law, wherein prolific authors are a small part of the group members, with approximately 20% of individuals contributing to 80% of the work. Backbone-based research groups, comprising predominantly project-based and individual-based research teams in universities, typically exhibit a core and backbone members ratio of approximately 10%–15%, aligning with Price’s Law. Peterson ( 2018 ) also pointed out that Price’s Law is almost universally present in all creative work. Scientific research relies more on innovative thinking and collaboration among researchers, and the phenomenon was first confirmed within university research groups. Besides, systematic research activities require many researchers to participate, but few people make important intellectual support and contributions. In practical research endeavors, principal researchers, such as professors and associate professors, often exhibit higher levels of innovation and stability, while graduate students and external support staff tend to be more transient, engaging in foundational research tasks.
Fifth, regarding the research group with the highest publication count of the two universities, Tsinghua University has more core members, highlighting the research model centered around a single scholar, while Nanyang Technological University exhibits a more dispersed distribution of researchers. This discrepancy may be attributed to differences in the university’s system. In China, valuable scientific research often unfolds under the leadership of authoritative scholars, typically holding multiple administrative roles, thus exhibiting hierarchical centralization within the group. This hierarchical structure aligns with Merton’s Sociology of Science ( 1973 ), positing that the higher the position of scientists, the higher their status in the hierarchy, facilitating increased funding acquisition and research impact. Conversely, Singapore’s research system is more like that of developed countries such as the UK and the US, fostering a more democratic culture where communication among members is more open. This relatively flat team culture is conducive to generating high-level research outcomes (Xu et al., 2022 ). However, concerning the field-weighted citation impact of research group papers, the Chinese backbone-based research group outperforms in both publication volume and academic influence, suggesting that this organizational characteristic is more suitable for China and is more conducive to doing research with stronger academic influence.
The research teams and groups in these top two universities offer insights for constructing science teams: Firstly, the university should prioritize individual-based research teams to enhance the academic influence of their research. Secondly, intra-university research teams should foster collaboration across different departments to promote interdisciplinary research, contributing to the advancement of the discipline. Thirdly, emphasis should be placed on supporting core and backbone members who often generate innovative ideas and contribute more to the academic community. Fourth, the research team should cultivate a suitable research atmosphere according to their cultural background, whether centralized or democratic, to harness researchers’ strengths effectively.
This research proposes a method for identifying university research teams and analyzing the characteristics of such teams at the top two universities. In the future, further exploration into the role of different team members and the development of more effective research team construction strategies are warranted.
Data availability
The datasets generated during and/or analyzed during the current study are available from the corresponding author upon reasonable request. The data about the information of research papers authored by the two universities and the identification results of the members of university research teams are shared.
Aagaard K, Kladakis A, Nielsen MW (2020) Concentration or dispersal of research funding? Quant Sci Stud 1(1):117–149. https://doi.org/10.1162/qss_a_00002
Article Google Scholar
Abramo G, D’Angelo CA, Di Costa F (2017) Do interdisciplinary research teams deliver higher gains to science? Scientometrics 111:317–336. https://doi.org/10.1007/s11192-017-2253-x
Barjak F, Robinson S (2008) International collaboration, mobility and team diversity in the life sciences: impact on research performance. Soc Geogr 3(1):23–36. https://doi.org/10.5194/sg-3-23-2008
Boardman C, Ponomariov B (2014) Management knowledge and the organization of team science in university research centers. J Technol Transf 39:75–92. https://doi.org/10.1007/s10961-012-9271-x
Boyack KW, Klavans R (2014) 12 Identifying and Quantifying Research Strengths Using Market Segmentation. In: Beyond bibliometrics: Harnessing multidimensional indicators of scholarly impact, 225, MIT Press, Cambridge
Bozeman B, Youtie J (2018) The strength in numbers: The new science of team science. Princeton University Press. https://doi.org/10.1515/9781400888610
Bloch C, Sørensen MP (2015) The size of research funding: Trends and implications. Sci Public Policy 42(1):30–43. https://doi.org/10.1093/scipol/scu019
Blondel VD, Guillaume JL, Lambiotte R, Lefebvre E (2008) Fast unfolding of communities in large networks. J Stat Mech Theory Exp 2008(10):P10008. https://doi.org/10.1088/1742-5468/2008/10/P10008
Coles NA, Hamlin JK, Sullivan LL, Parker TH, Altschul D (2022) Build up big-team science. Nature 601(7894):505–507. https://doi.org/10.1038/d41586-022-00150-2
Article ADS CAS PubMed Google Scholar
Dino H, Yu S, Wan L, Wang M, Zhang K, Guo H, Hussain I (2020) Detecting leaders and key members of scientific teams in co-authorship networks. Comput Electr Eng 85:106703. https://doi.org/10.1016/j.compeleceng.2020.106703
Deng H, Breunig H, Apte J, Qin Y (2022) An early career perspective on the opportunities and challenges of team science. Environ Sci Technol 56(3):1478–1481. https://doi.org/10.1021/acs.est.1c08322
Everett M (2002) Social network analysis. In: Textbook at Essex Summer School in SSDA, 102, Essex Summer School in Social Science Data Analysis, United Kingdom
Forscher PS, Wagenmakers EJ, Coles NA, Silan MA, Dutra N, Basnight-Brown D, IJzerman H (2023) The benefits, barriers, and risks of big-team science. Perspect Psychological Sci 18(3):607–623. https://doi.org/10.1177/17456916221082970
Guo K, Huang X, Wu L, Chen Y (2022) Local community detection algorithm based on local modularity density. Appl Intell 52(2):1238–1253. https://doi.org/10.1007/s10489-020-02052-0
Hu Z, Lin A, Willett P (2019) Identification of research communities in cited and uncited publications using a co-authorship network. Scientometrics 118:1–19. https://doi.org/10.1007/s11192-018-2954-9
Imran F, Abbasi RA, Sindhu MA, Khattak AS, Daud A, Amjad T (2018) Finding research areas of academicians using clique percolation. In 2018 14th International Conference on Emerging Technologies (ICET). IEEE, pp 1–6. https://doi.org/10.1109/ICET.2018.8603549
Lee HJ, Kim JW, Koh J, Lee Y (2008) Relative Importance of Knowledge Portal Functionalities: A Contingent Approach on Knowledge Portal Design for R&D Teams. In Proceedings of the 41st Annual Hawaii International Conference on System Sciences (HICSS 2008). IEEE, pp 331–331, https://doi.org/10.1109/HICSS.2008.373
Liao Q (2018) Research Team Identification and Influence Factors Analysis of Team Performance. M. A. Thesis. Beijing Institute of Technology, Beijing
Google Scholar
Li Y, Tan S (2012) Research on identification and network analysis of university research team. Sci Technol Prog policy 29(11):147–150
Li G, Liu M, Wu Q, Mao J (2017) A Research of Characters and Identifications of Roles Among Research Groups Based on the Bow-Tie Model. Libr Inf Serv 61(5):87–94
Liu Y, Wu Y, Rousseau S, Rousseau R (2020) Reflections on and a short review of the science of team science. Scientometrics 125:937–950. https://doi.org/10.1007/s11192-020-03513-6
Lungeanu A, Huang Y, Contractor NS (2014) Understanding the assembly of interdisciplinary teams and its impact on performance. J Informetr 8(1):59–70. https://doi.org/10.1016/j.joi.2013.10.006
Article PubMed PubMed Central Google Scholar
Lv L, Zhao Y, Wang X, Zhao P (2016) Core R&D Team Recognition Method Based on Association Rules Mining. Sci Technol Manag Res 36(17):148–152
Ma A, Mondragón RJ, Latora V (2015) Anatomy of funded research in science. Proc Natl Acad Sci 112(48):14760–14765. https://doi.org/10.1073/pnas.1513651112
Article ADS CAS PubMed PubMed Central Google Scholar
Merton RK (1973) The sociology of science: Theoretical and empirical investigations. University of Chicago Press, Chicago
Mongeon P, Brodeur C, Beaudry C, Larivière V (2016) Concentration of research funding leads to decreasing marginal returns. Res Eval 25(4):396–404. https://doi.org/10.1093/reseval/rvw007
National Research Council (2015) Enhancing the effectiveness of team science. The National Academies Press, Washington, DC
Okamoto J, Centers for Population Health and Health Disparities Evaluation Working Group (2015) Scientific collaboration and team science: a social network analysis of the centers for population health and health disparities. Transl Behav Med 5(1):12–23. https://doi.org/10.1007/s13142-014-0280-1
Article PubMed Google Scholar
Peterson JB (2018) 12 rules for life: An antidote to chaos. Random House, Canada
Scott J (2017) Social network analysis. Sage Publications Ltd, London
Seidman SB, Foster BL (1978) A graph‐theoretic generalization of the clique concept. J Math Sociol 6(1):139–154. https://doi.org/10.1080/0022250X.1978.9989883
Article MathSciNet Google Scholar
Sun Y, Livan G, Ma A, Latora V (2021) Interdisciplinary researchers attain better long-term funding performance. Commun Phys 4(1):263. https://doi.org/10.1038/s42005-021-00769-z
Stokols D, Hall KL, Taylor BK, Moser RP (2008) The science of team science: overview of the field and introduction to the supplement. Am J Prev Med 35(2):S77–S89. https://doi.org/10.1016/j.amepre.2008.05.002
Wang C, Cheng Z, Huang Z (2017) Analysis on the co-authoring in the field of management in China: based on social network analysis. Int J Emerg Technol Learn 12(6):149. https://doi.org/10.3991/ijet.v12i06.7091
Wang T, Chen S, Wang X, Wang J (2020) Label propagation algorithm based on node importance. Phys A Stat Mech Appl. 551:124137. https://doi.org/10.1016/j.physa.2020.124137
Wu L, Wang D, Evans JA (2019) Large teams develop and small teams disrupt science and technology. Nature 566(7744):378–382. https://doi.org/10.1038/s41586-019-0941-9
Xu F, Wu L, Evans J (2022) Flat teams drive scientific innovation. Proc. Natl Acad. Sci 119(23):e2200927119. https://doi.org/10.1073/pnas.2200927119
Article CAS PubMed PubMed Central Google Scholar
Yu H, Bai K, Zou B, Wang Y (2020) Identification and Extraction of Research Team in the Artificial Intelligence Field. Libr Inf Serv 64(20):4–13
Yu Y, Dong C, Han H, Li Z (2018) The Method of Research Teams Identification Based on Social Network Analysis:Identifying Research Team Leaders Based on Iterative Betweenness Centrality Rank Method. Inf Stud Theory Appl 41(7):105–110
Zhao L, Zhang Q, Wang L (2014) Benefit distribution mechanism in the team members’ scientific research collaboration network. Scientometrics 100:363–389. https://doi.org/10.1007/s11192-014-1322-7
Zhang M, Jia Y, Wang N, Ge S (2019) Using Relative Tie Strength to Identify Core Teams of Scientific Research. Int J Emerg Technol Learn 14(23):33–54. https://www.learntechlib.org/p/217243/
Download references
Author information
Authors and affiliations.
School of Education, Central China Normal University, Wuhan, PR China
Zhe Cheng & Yihuan Zou
Faculty of Education, The Chinese University of Hong Kong, Hong Kong SAR, PR China
Yueyang Zheng
You can also search for this author in PubMed Google Scholar
Contributions
Zhe Cheng contributed to the study conception, research design, data collection, and data analysis. Zhe Cheng wrote the first draft of the manuscript. Yihuan Zou made the last revisions. Yihuan Zou and Yueyang Zheng supervised, proofread, and commented on previous versions of this manuscript. All authors read and approved the final manuscript.
Corresponding author
Correspondence to Yueyang Zheng .
Ethics declarations
Competing interests.
The authors declare no competing interests.
Ethical approval
This article does not contain any studies with human participants performed by the authors.
Informed consent
This article does not contain any studies with human participants performed by any of the authors.
Additional information
Publisher’s note Springer Nature remains neutral with regard to jurisdictional claims in published maps and institutional affiliations.
Rights and permissions
Open Access This article is licensed under a Creative Commons Attribution 4.0 International License, which permits use, sharing, adaptation, distribution and reproduction in any medium or format, as long as you give appropriate credit to the original author(s) and the source, provide a link to the Creative Commons licence, and indicate if changes were made. The images or other third party material in this article are included in the article’s Creative Commons licence, unless indicated otherwise in a credit line to the material. If material is not included in the article’s Creative Commons licence and your intended use is not permitted by statutory regulation or exceeds the permitted use, you will need to obtain permission directly from the copyright holder. To view a copy of this licence, visit http://creativecommons.org/licenses/by/4.0/ .
Reprints and permissions
About this article
Cite this article.
Cheng, Z., Zou, Y. & Zheng, Y. A method for identifying different types of university research teams. Humanit Soc Sci Commun 11 , 523 (2024). https://doi.org/10.1057/s41599-024-03014-4
Download citation
Received : 03 August 2023
Accepted : 28 March 2024
Published : 18 April 2024
DOI : https://doi.org/10.1057/s41599-024-03014-4
Share this article
Anyone you share the following link with will be able to read this content:
Sorry, a shareable link is not currently available for this article.
Provided by the Springer Nature SharedIt content-sharing initiative
Quick links
- Explore articles by subject
- Guide to authors
- Editorial policies

Google consolidates its DeepMind and Research teams amid AI push
- Medium Text
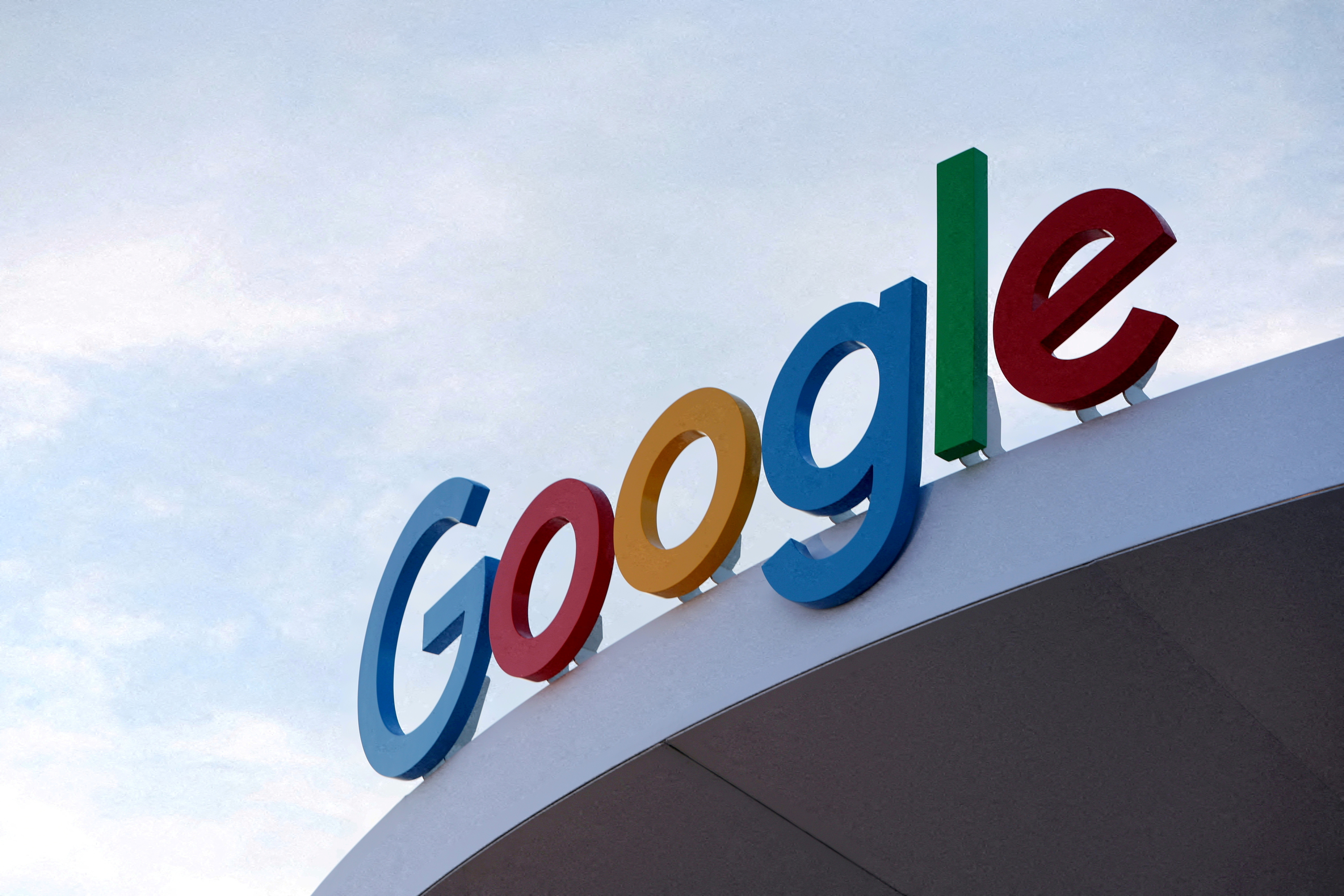
The Technology Roundup newsletter brings the latest news and trends straight to your inbox. Sign up here.
Reporting by Zaheer Kachwala in Bengaluru; Editing by Tasim Zahid
Our Standards: The Thomson Reuters Trust Principles. New Tab , opens new tab
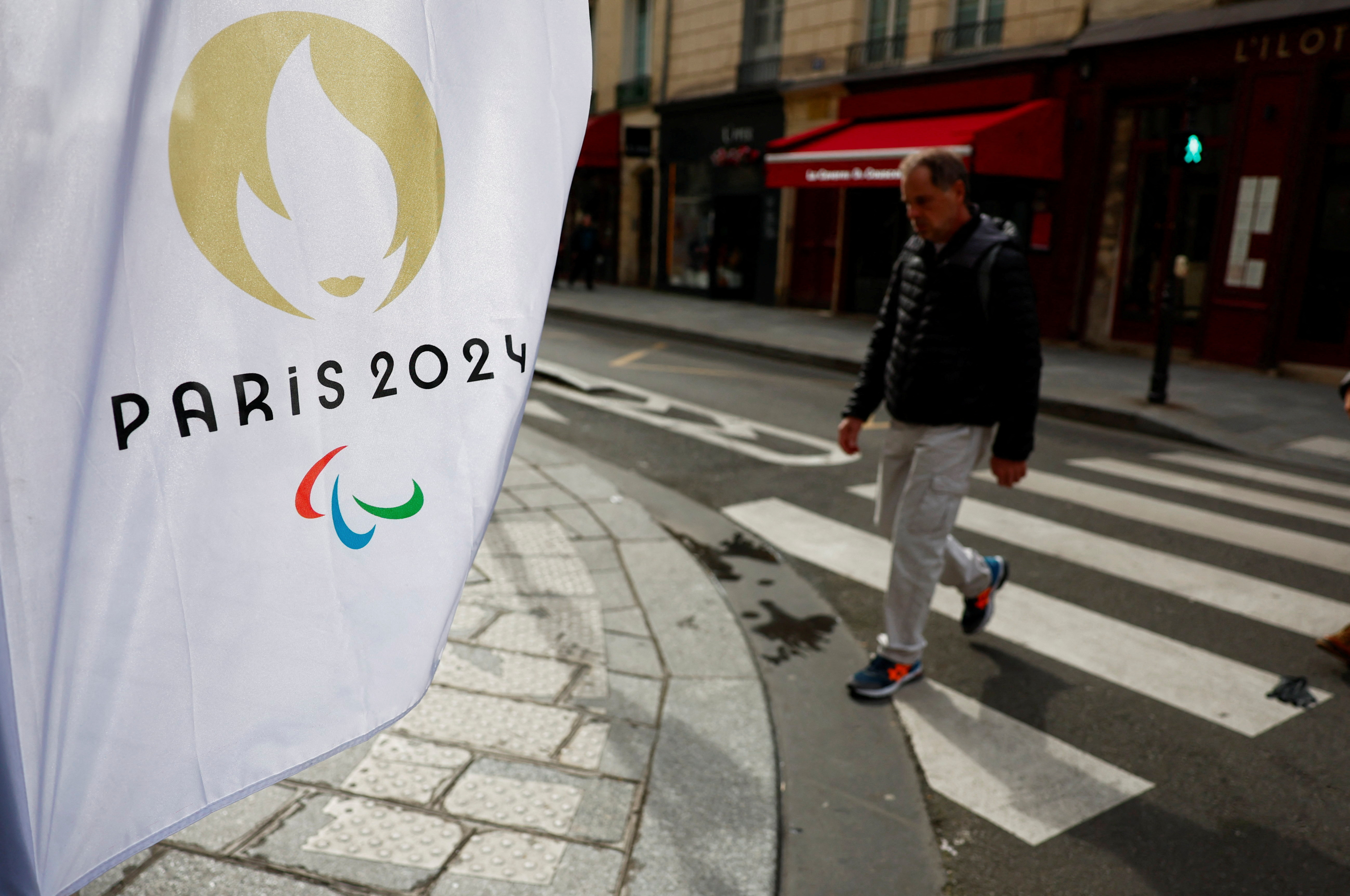
Technology Chevron
Games' broadcaster embracing ai but remains wary of deepfakes.
As part of a push by the International Olympic Committee to embrace artificial intelligence (AI) in Paris, the Games' broadcaster is stepping up its use of the technology -- but not without concerns about the risk of "deepfakes".
Shares in SoftwareOne rose on Friday after a boardroom shake-up made the prospect of a bid for the Swiss IT service provider more likely, investors and analysts said.
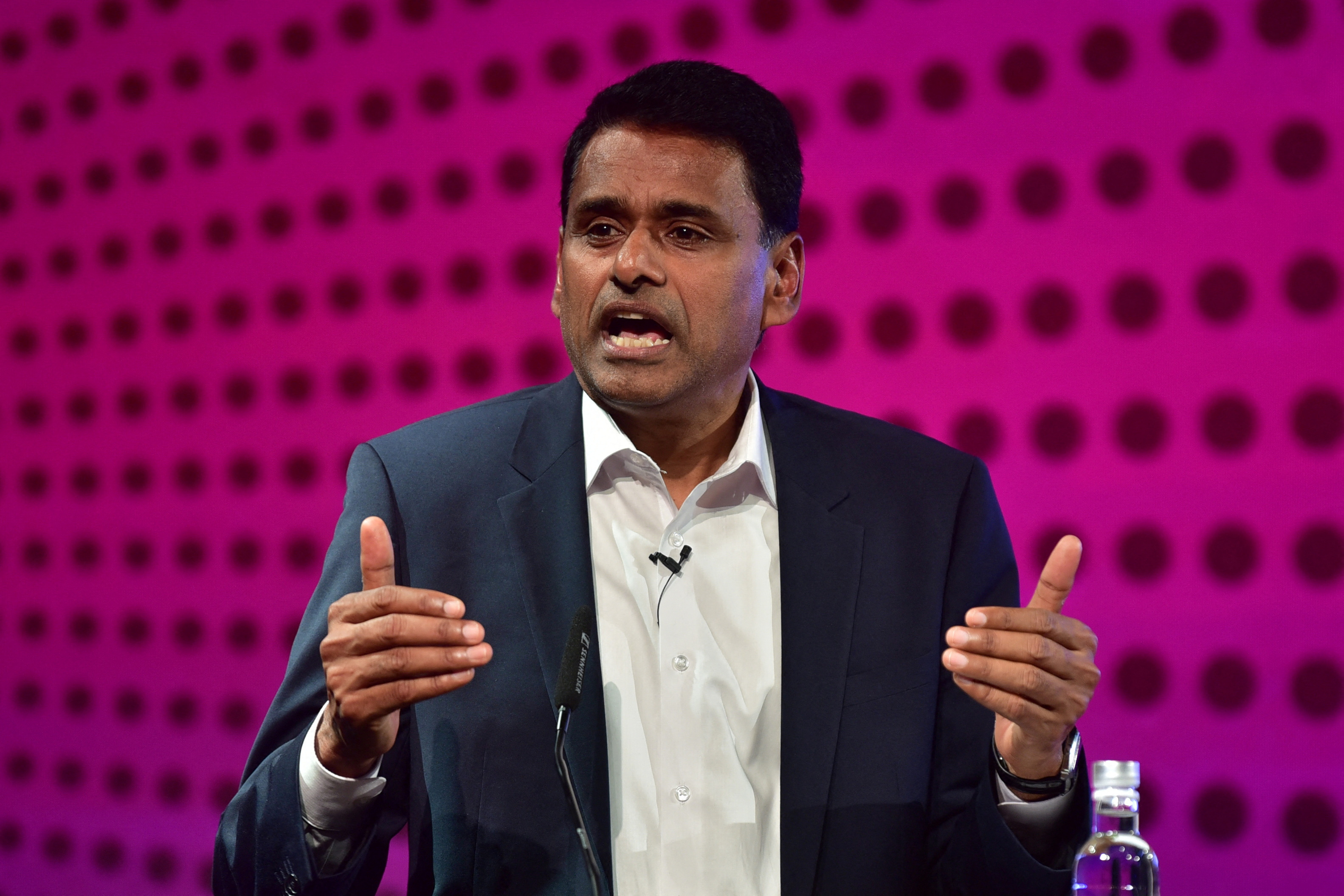

- SUGGESTED TOPICS
- The Magazine
- Newsletters
- Managing Yourself
- Managing Teams
- Work-life Balance
- The Big Idea
- Data & Visuals
- Reading Lists
- Case Selections
- HBR Learning
- Topic Feeds
- Account Settings
- Email Preferences
Stop Wasting Money on Team Building
- Carlos Valdes-Dapena

There are better ways to learn collaboration.
Many companies, when they decide to invest in team building, decide to do offsite events like bowling nights or ropes courses. Some spend thousands on special events, hoping to improve collaboration. These efforts often fail, so Mars Inc. took a different approach. HR leaders decided to interview and survey 125 teams. They used this data to develop a team-building approach that focused on the work itself. In an intensive two-day workshop, they asked a pilot group to discuss two questions: Why is their collaboration essential to achieving their business results? And second: What work, which specific tasks, would require collaboration to deliver those results? The results from these discussions were so good that Mars rolled out this approach to the whole company.
Most corporate team building is a waste of time and money. I say this based on my 25+ years of research and practice in the field of team effectiveness. Seventeen of those years were with Mars Inc., a family-owned $35 billion global business with a commitment to collaboration.

- CV Carlos Valdes-Dapena is the CEO of Corporate Collaboration Resources and the author of Lessons from Mars: How One Global Company Cracked the Code on High Performance Collaboration and Teamwork .
Partner Center
Study of modified VVER and typical PWR fuel in the HBWR reactor (Norway)
- Published: 21 December 2012
- Volume 113 , pages 171–178, ( 2013 )
Cite this article
- B. Yu. Volkov 1 ,
- W. Wiesenack 1 ,
- V. V. Yakovlev 2 ,
- E. P. Ryazantsev 2 ,
- A. K. Panyushkin 3 ,
- A. V. Ivanov 3 ,
- O. V. Kryukov 3 ,
- P. I. Lavrenyuk 4 &
- Yu. V. Pimenov 4
126 Accesses
3 Citations
Explore all metrics
Two experiments studying the standard and modified VVER fuel fabricated at the Machine-Building Plant (in Elektrostal) and PWR fuel produced according to the typical specifications were performed on the HBWR research reactor (Halden, Norway) from 1995 to 2005. The objective of these experiments was to study the effect of the structural-technological parameters on the behavior of VVER fuel in comparison with the typical PWR fuel. These studies made it possible to expand the in-reactor data base on the behavior of VVER uranium oxide fuel as well as to develop recommendations for improving the technology of its production in order to increase fuel stability under irradiation.
This is a preview of subscription content, log in via an institution to check access.
Access this article
Price includes VAT (Russian Federation)
Instant access to the full article PDF.
Rent this article via DeepDyve
Institutional subscriptions
Similar content being viewed by others
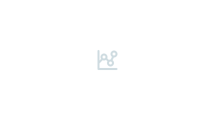
Fuel for VVER and PWR: Current Status and Prospects
D. L. Zverev, O. B. Samoilov, … S. A. Zotov
Techno-Economic Prognosis for the Manufacture of Fuel Assemblies with Mixed Uranium-Plutonium Fuel for the VVER-SKD Power Reactor
M. V. Kormilitsyn, L. A. Kormilitsyna, … T. D. Shchepetina
PWR Fuel Cycle Increased Enrichment, Combination of Burnable Absorbers
B. Yu. Volkov, E. P. Ryazantsev, V. V. Yakovlev, et al., “Studies of the behavior of VVER and PWR irradiated in the HBWR reactor (Halden, Norway),” At. Énerg. , 111 , No. 6, 342–348 (2011).
Google Scholar
B. Volkov, E. Ryazantzev, and V. Yakovlev, The Thermal and Mechanical Behaviour of Modified WWER Fuel Compared with PWR Specification Fuel in IFA-503.2 , HWR-637, December 2000.
B. Volkov and T. Tverberg, “Irradiation performance of modified WWER fuel compared with typical PWR fuel in the Halden Reactor Test,” in: 4th Int. Conf. on WWER Fuel Performance, Modelling, and Experimental Support , Bulgaria, Varna, Oct. 15, 2001, pp. 186–196.
B. Volkov and E. Kolstad, “Review of WWER fuel and material tests in the Halden reactor,” in: 6th Int. Conf. on WWER Fuel Performance, Modelling and Experimental Support , Bulgaria, Albena, Sept. 19–23, 2005, pp. 214–221.
K. Vinjamuru and D. Owen, “Helium fill gas absorption in pressurized UO2 fuel rods during irradiation,” Nucl. Technol ., 47 , No. 1, 119–124 (1980).
G. Small, “Densification of uranium dioxide at low burnup,” J. Nucl. Mater. , 148 , No. 3, 302–315 (1987).
Article MathSciNet ADS Google Scholar
M. Freshley et al., “Irradiation-induced densification of UO2 pellet fuel,” ibid ., 62 , No. 2, 138–166 (1976).
W. Wiesenack and T. Tverberg, “Thermal performance of high burnup fuel – in-pile temperature data and analysis,” in: Int. Topical Meeting on LWR Fuel Performance , Utah, USA, April 10–3, 2000, pp. 626–633.
Download references
Author information
Authors and affiliations.
Halden Reactor Project, Halden, Norway
B. Yu. Volkov & W. Wiesenack
National Research Center Kurchatov Institute, Moscow, Russia
V. V. Yakovlev & E. P. Ryazantsev
Machine-Building Plant, Elektrostal, Moscow Oblast, Russia
A. K. Panyushkin, A. V. Ivanov & O. V. Kryukov
TVEL Company, Moscow, Russia
P. I. Lavrenyuk & Yu. V. Pimenov
You can also search for this author in PubMed Google Scholar
Additional information
Translated from Atomnaya Énergiya, Vol. 113, No. 3, pp. 140–145, September, 2012.
Rights and permissions
Reprints and permissions
About this article
Volkov, B.Y., Wiesenack, W., Yakovlev, V.V. et al. Study of modified VVER and typical PWR fuel in the HBWR reactor (Norway). At Energy 113 , 171–178 (2013). https://doi.org/10.1007/s10512-012-9613-7
Download citation
Received : 14 February 2012
Published : 21 December 2012
Issue Date : January 2013
DOI : https://doi.org/10.1007/s10512-012-9613-7
Share this article
Anyone you share the following link with will be able to read this content:
Sorry, a shareable link is not currently available for this article.
Provided by the Springer Nature SharedIt content-sharing initiative
- Fuel Element
- Uranium Dioxide
- Fuel Kernel
- Experimental Fuel
- Find a journal
- Publish with us
- Track your research
Sustainability
Sustainability Staff at Neuroscience Research Building

The newly occupied Jeffrey T. Fort Neuroscience Research Building (NRB) is a state-of-the-art facility for WashU School of Medicine researchers, with many amenities and a team of dedicated staff. As of late March, Alicia Hubert has joined the NRB team as the newly appointed program manager and NRB operations liaison to research labs and green lab manager.
Alicia began her career at WashU as a research technician in the biology department before becoming the first sustainability coordinator at the School of Medicine. Through the COVID-19 pandemic, her position shifted to include equipment planning for the NRB, in addition to leading the sustainability program at WUSM. Shifting titles again into the Program Manager and NRB Operations Liaison, Alicia will continue equipment planning and updating, facilitate the replacement of inefficient lab equipment, and serve as a liaison between labs and WashU School of Medicine Operations & Facilities Management Department (OFMD) leadership.
David Lott, executive director of research facilities for the Fort Neuroscience Research Building states, “I am thrilled to welcome Alicia to my team. She played a crucial role in our recent move-in to Fort Labs, so it was an obvious choice to bring her on board in this new role. Alicia is highly organized, has extensive experience with all the labs, and has developed strong relationships with the researchers here. We will be relying on her expertise to conduct audits of lab space utilization, address lab-specific concerns, and assist department leadership with their recruitment space needs.”
Under her new title, Alicia will be providing a more hands-on approach to incorporating sustainability into the culture and daily operations of the newly occupied NRB labs, and supporting lab members through operational challenges. Alicia hopes that the Green Lab program in the NRB will become a model for other buildings on campus. Lott states, “Fort Labs researchers will directly benefit from the continuity of our staff from planning through occupancy. It will allow them to have greater focus on their research, knowing that Alicia will be there to support them with her expertise.”
DNA nanotube rings: Research team develops important building block for artificial cells
D uring cell division, a ring forms around the cell equator, which contracts to divide the cell into two daughter cells. Together with researchers from Heidelberg, Dresden, Tübingen and Harvard, Professor Jan Kierfeld and Lukas Weise from the Department of Physics at TU Dortmund University have succeeded for the first time in synthesizing such a contractile ring with the help of DNA nanotechnology and uncovering its contraction mechanism. The results have been published in Nature Communications .
In synthetic biology, researchers try to recreate crucial mechanisms of life in vitro, such as cell division. The aim is to be able to synthesize minimal cells. The research team led by Professor Kerstin Göpfrich from Heidelberg University has now synthetically reproduced contractile rings for cell division using polymer rings composed of DNA nanotubes.
The formation of a ring that constricts and separates dividing cells is an important step in natural cell division. In nature, this is achieved by a machinery of proteins: motor proteins powered by chemical energy from ATP hydrolysis pull together a ring of filaments of the protein actin. Adenosine triphosphate, or ATP, is a molecule that occurs in all living cells and supplies the energy for numerous cellular processes.
The contraction mechanism of the DNA rings developed by the researchers no longer relies on motor proteins powered by ATP hydrolysis. Instead, molecular attraction between ring segments can trigger the contraction of the polymer rings.
This molecular attraction can be induced in two ways: either by crosslinking molecules with two "sticky" ends that can connect two polymer segments, or by means of the depletion interaction, where the polymers are surrounded by "crowder" molecules that press the segments together. This mechanism consumes no chemical energy, meaning that no energy source needs to be incorporated in the synthetic cell for the mechanism to function.
Professor Jan Kierfeld, Professor of Theoretical Physics, and doctoral researcher Lukas Weise are working in the field of biological physics. As part of their research work, they have developed a theoretical description and a molecular dynamics simulation of the contraction mechanism, which match the experimental results of their research partners.
To this end, they devised special methods for simulating the DNA rings on a realistic scale. Theory and the simulation make it possible to explain quantitatively how the polymer rings form and contract.
"This means we are able not only to predict that an increased concentration of 'crowder' molecules will make the ring smaller but also by how much smaller," says Professor Kierfeld. In this way, it is possible to determine how the diameter of the DNA ring can be precisely controlled, which is highly significant for future applications of contractile rings in synthetic biology.
Mechanisms for cell division are an important step towards an artificial cell, the construction of which facilitates a better understanding of the functional mechanisms of natural cells and, thus, of the foundations of life.
More information: Maja Illig et al, Triggered contraction of self-assembled micron-scale DNA nanotube rings, Nature Communications (2024). DOI: 10.1038/s41467-024-46339-z
Provided by TU Dortmund University
- History of cooperation
- Areas of cooperation
- Procurement policy
- Useful links
- Becoming a supplier
- Procurement
- Rosatom newsletter
© 2008–2024Valtiollinen Rosatom-ydinvoimakonserni

- Rosatom Global presence
- Rosatom in region
- For suppliers
- Preventing corruption
- Press centre
Rosatom Starts Life Tests of Third-Generation VVER-440 Nuclear Fuel
- 16 June, 2020 / 13:00
This site uses cookies. By continuing your navigation, you accept the use of cookies. For more information, or to manage or to change the cookies parameters on your computer, read our Cookies Policy. Learn more
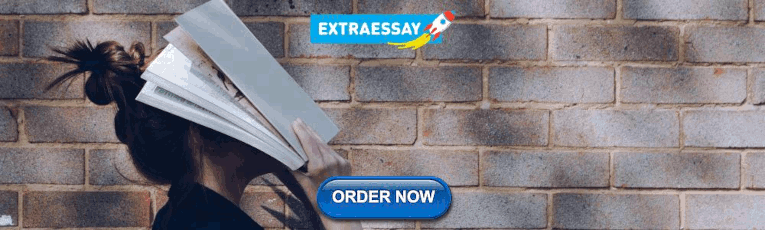
IMAGES
VIDEO
COMMENTS
The science of teamwork has been extensively studied, 1 and with good reason. Successful teams improve business outcomes, including revenue and performance. 2 Many organizations are intentionally fostering a collaborative team-based culture, 2 and feeling like a part of a team is a primary driver of employee engagement. 3 Prior to the pandemic, organizational shifts had resulted in teams that ...
Research on team science has found that collaborating across organizational and geographic boundaries increases productivity and scientific impact. And cross-disciplinary teams produce more academic publications and publish in more diverse outlets, Hall and her colleagues report. ... Team-building interventions, meanwhile, aim to better teams ...
The objective of this study was to conduct a systematic review and meta-analysis of teamwork interventions that were carried out with the purpose of improving teamwork and team performance, using controlled experimental designs. A literature search returned 16,849 unique articles. The meta-analysis was ultimately conducted on 51 articles, comprising 72 (k) unique interventions, 194 effect ...
An empirical analysis of team-building research. Group & Organization Studies, 6 (3): 357-378. [Google Scholar] DeRue DS, Hollenbeck JR, Johnson MD, Ilgen DR, & Jundt DK 2008. How different team downsizing approaches influence team-level adaptation and performance. Academy of Management Journal, 51 (1): 182-196. [Google Scholar] Devine DJ 1999.
Serendipitously, this interest in teams as focal units for research coincided with rising interest in multilevel theory (MLT; Kozlowski & Klein, 2000), which is focused on connecting the levels (often defined by disciplinary boundaries) that separate the science of organizations into micro- (individual), meso- (group or team), and macro- (organizational) levels of analysis.
Led by Sandy Pentland, researchers at MIT's Human Dynamics Laboratory set out to solve that puzzle. Hoping to decode the "It factor" that made groups click, they equipped teams from a broad ...
To understand how the best teams build trust among themselves, researchers interviewed 1,000 U.S.-based office workers and identified five key behaviors that set these teams apart: 1) They don't ...
In this paper we review the extant literature on team building in sport, and address a range of conceptual, methodological, and applied considerations that have the potential to advance theory, research, and applied intervention initiatives within the field. This involves expanding the scope of team building strategies that have, to date ...
Despite the essential need for such interdisciplinary interactions, little research has examined the impact of scientific team support measures like training, facilitation, team building, and ...
Here is our list of team building research studies. Team building research studies are academic papers that present experiments and observations on the science of team building. These scholarly articles have been published in reputable journals and are cited often by experts. These studies help highlight team building statistics, the elements ...
Research on team building has revealed 4 fundamental components of team-building interventions: goal setting, interpersonal relations, problem solving, and role clarification [39-42]. These components are not necessarily activated equally in every team-building intervention. A team-building intervention might include one, multiple, or all these ...
For this meta-analysis, literature selection focused on research studies examining the effectiveness of team-building interventions in interactive sport teams. The selection process followed rigorous and systematic procedures, incorporating keyword searches in computerized databases and employing a snowball sampling approach.
Martine Haas. and. Mark Mortensen. From the Magazine (June 2016) RW13 (Fair Game), oil on canvas, Museum of Fine Arts, Boston, 2010 Jeff Perrott. Summary. Over the years, as teams have grown more ...
E mail: [email protected]. Abstract. Team building involves a wide range of activities, designed for improving team performance. Its aim is to bring out the. best in a team t o ensure self ...
Abstract. Academic Medical Centers strive to create multidisciplinary research teams to produce impactful science. However, few faculty researchers receive training in "team science," a well-established concept in business research and practice. Responding to demand for assistance developing effective research teams, the Collaboration and ...
Objective: improving a team's functioning in general, by developing interpersonal relations, clarifying roles, or solving existent problems. Method: mostly informal team building activities like games and physical exercises, as opposed to sitting in a classroom and discussing a topic. Location: usually a different one to where the team works ...
And, importantly, having a lot of individually intelligent team members does not mean the team will have a high collective intelligence. Lab experiments in one study had teams spend several hours on a variety of exercises, from brainstorming to solving visual puzzles. The results point to three ways to increase a team's collective intelligence:
Identifying research teams constitutes a fundamental step in team science research, and universities harbor diverse types of such teams. This study introduces a method and proposes algorithms for ...
Alphabet-owned Google said on Thursday it would consolidate teams that focus on building artificial intelligence models across its Research and DeepMind divisions in its latest push to develop its ...
Lifeway Research. Enlightening today's church with relevant research and insights. Research. State of Ministry to Women; Insights; Resource Library; Fast Facts; Search; Newsletter; building a healthy team. 4 Habits of A Healthy Team. ... Apr 19, 2024. Commit to creating a healthy culture for your team that supports, encourages, and propels ...
Team Building and Networking Guided Tour of Traveling Stonewall Exhibit. Event Host Westphal College of Media Arts and Design Who Should Attend Everyone ... Research Development 3250 Chestnut Street, Suite 3010 Philadelphia, PA 19104 View on Map. Hours: Monday - Friday, 8:00am-5:00pm ...
Most corporate team building is a waste of time and money. I say this based on my 25+ years of research and practice in the field of team effectiveness.
A team led by neuroscientists at The Herbert Wertheim UF Scripps Institute for Biomedical Innovation & Technology has discovered a key molecular player in fear conditioning. ... the study's corresponding author, said the research also adds an important piece to the enormously complex puzzle of how and why neurons deliver such RNAs long ...
Two experiments studying the standard and modified VVER fuel fabricated at the Machine-Building Plant (in Elektrostal) and PWR fuel produced according to the typical specifications were performed on the HBWR research reactor (Halden, Norway) from 1995 to 2005. The objective of these experiments was to study the effect of the structural-technological parameters on the behavior of VVER fuel in ...
The newly occupied Jeffrey T. Fort Neuroscience Research Building (NRB) is a state-of-the-art facility for WashU School of Medicine researchers, with many amenities and a team of dedicated staff. As of late March, Alicia Hubert has joined the NRB team as the newly appointed program manager and NRB operations liaison to research labs and green […]
The research team led by Professor Kerstin Göpfrich from Heidelberg University has now synthetically reproduced contractile rings for cell division using polymer rings composed of DNA nanotubes.
The index for the Burevestnik space complex is 14K168. The idea that "293" is a satellite launch vehicle is corroborated by the fact that the index for one of its stages (14S47) is similar to that of some upper stages of space launch vehicles. Moreover, plans to use the MiG-31 as a satellite launch platform are not new.
16 June, 2020 / 13:00. 10 704. OKB Gidropress research and experiment facility, an enterprise of Rosatom machinery division Atomenergomash, has started life tests of a mock-up of the third-generation nuclear fuel RK3+ for VVER-440 reactors. The work is carried out within the contract between TVEL Fuel Company of Rosatom and Czech power company ...
Find company research, competitor information, contact details & financial data for AVANGARD, OOO of Elektrostal, Moscow region. Get the latest business insights from Dun & Bradstreet. ... Lessors of Real Estate Residential Building Construction Utility System Construction Building Equipment Contractors. Printer Friendly View Address: d. 010 ...