- Open access
- Published: 09 September 2022
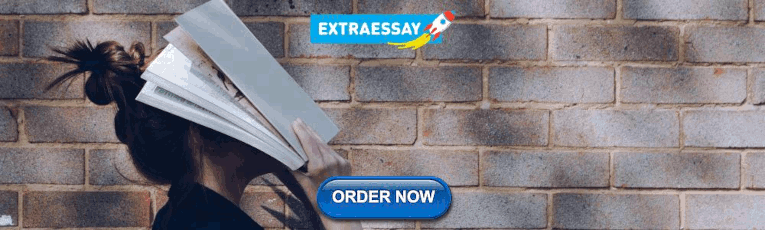
A review of landscape ecology experiments to understand ecological processes
- Yolanda F. Wiersma ORCID: orcid.org/0000-0003-4604-9240 1
Ecological Processes volume 11 , Article number: 57 ( 2022 ) Cite this article
5078 Accesses
4 Citations
1 Altmetric
Metrics details
One way in which we make inferences about ecological processes is via experimentation. Many ecological processes happen at landscape extents and it is at this extent that experimentation is more challenging. This review explores the intersection between experimentation, ecological processes and landscape ecology. Specifically, this review seeks to discover how scientists design experiments to understand ecological processes at landscape scales.
I found 87 papers where these three concepts intersected, and reviewed them in more depth to assess characteristics of scale (treatment and study area extent), replication, research question and experiment type.
Conclusions
The findings suggest that experimental approaches for understanding ecological processes are well established, and beginning to more readily accommodate spatial dimensions. However, there is room to integrate more spatially explicit, landscape-scale experiments into studies of ecological processes.
Introduction
A key tool for understanding mechanisms that shape patterns is via experimentation. This is true across scientific disciplines. Ecological processes, the focus of this journal, shape and influence ecological systems at all scales. Although research in this journal has traditionally examined ecological process at many different extents, ecological processes at large extents merit special consideration since these shape the systems that humans directly interact with, actively manage, and critically depend on. These include the agricultural and marine ecosystems that feed us, the forest ecosystems that provide timber and non-timber resources, and the myriad of ecosystems that provide carbon sequestration. These large extent systems of fields, forests and oceans are also the purview of the discipline of landscape ecology (Turner 2005 ; Turner and Gardner 2015 ). How to carry out experiments in landscapes to realize reliable inferences about the links between ecological patterns and ecological processes, and vice versa, is a key challenge for researchers, and is the focus of this review.
In a perspectives essay on experimental landscape ecology, Jenerette and Shen ( 2012 ) discussed different experimental approaches to identify how landscapes affect variation in ecological processes, and how landscape structure influences these processes. They highlighted the challenge of carrying out experiments at landscape extents (generally 10–100 s of kilometres), citing difficulty with replication, and the complexity of setting up experiments in spatially heterogeneous systems (Jenerette and Shen 2012 ). Many landscape-scale studies rely on observational data, and rely on correlations to infer processes, which may not capture the actually mechanisms at play.
Jenerette and Shen ( 2012 ) suggested four types of experiments that landscape ecologists could apply to help identify process variation within a landscape. These include distributed in situ experiments; ex situ experiments using samples collected throughout a landscape and brought back to the lab for analysis, translocation experiments and transport manipulations. Their group of experiment types to identify how processes responded to landscape structure echo many of the “classic” large-scale experiments, such as the experimental patches at the Savannah River Ecosystem, the Bowling Green fragmentation experiment, or systems such as Ecotrons (see an excellent summary of these types of experiments in Haddad 2012 ). Such experiments manipulate patch shape, connectivity, and fragmentation. Other experiments that can be used to infer how landscape structure affects process include manipulation of internal patch characteristics (e.g., via adding artificial structures, or adding nutrients), manipulation of landscape scale (e.g., mesocosms, microcosms, microlandscapes) or the construction of entire landscapes (Jenerette and Shen 2012 ). Wiersma ( 2022a , b ) summarized these approaches (large-scale manipulations, mesocosms, microcosms) along with in silico experiments (i.e., computer models) in more detail to show how researchers could harness these experimental tools to do spatially explicit experimentation (See Box 1 ). In this review, I pay particular attention to the experimental types (according to the taxonomy in Jenerette and Shen 2012 ) and tools used to study ecological processes at landscape extents.
Before discussing the literature review in more detail, a review of key terms is necessary. There has been much debate about whether we should consider observational studies to be “proper experiments” or not (Diamond 1983 ). In the mid-twentieth century, the increased reliance on sophisticated technology in the bench sciences, particularly molecular genetics, suggested to some ecologists that their field observational studies were too close to amateur natural history studies to be considered experimental and that manipulative experiments were the more reliable means for testing hypotheses (Kohler 2002 ). To clarify, by a manipulative experiment here, I mean an experiment whereby the researcher actively manipulates a factor of interest. This could be at a large extent in the field (e.g., via a controlled burn), in the field at smaller extent (e.g., via exclosures) or in a laboratory setting (e.g., experimental tanks or aquaria under controlled environments). Observational experiments (also called “natural experiments”) are those where natural processes have created the experimental treatment conditions. This treatment could be in the form of a disturbance, such as a flood or forest fire, or could be due to an underlying natural gradient (e.g., topography, soil moisture, light levels). If sampling is carried out as carefully as possible, so that experimental standards of control, replication, and randomization are applied, many suggest that observational experiments should be considered an equally credible approach as a manipulative one (Diamond 1983 ). Indeed, Diamond ( 2001 ) points out that heading to the field with a too narrowly focused experiment in mind can risk missing the chance to carry out an unplanned natural experiment. Laboratory/manipulative experiments have advantages of being easier to control for confounding effects, but being less realistic. Field manipulative experiments are more realistic, but can be logistically challenging to implement and have limited replication, and be influenced by stochastic events at the particular point in space and time they are implemented. Thus, it can be more challenging to meet the standards of experimental design in a manipulative field experiment (Diamond 1983 ; Wiersma 2022a ). Observational experiments are the most realistic, but the experimenter loses control over every aspect of the study except where and when they sample. For the purposes of this review, I am considering both observational (“natural”) and manipulative approaches in my consideration of what is an experiment. Moreover, this review is limited to experiments in ecological science. While studies of ecological processes and research in landscape ecology can certainly benefit from integration of methodology from the social sciences, an assessment of methodological approaches in social science is outside the scope of this review.
The experimental aspects of a control (a set of observations identical to the experiment minus the treatment factor), and randomization (ensuring experimental treatments and/or sampling are carried out without bias to underlying conditions) should be familiar to scientists. Conceptually, they are straightforward, but when working at large landscape extents, it can be difficult to implement these (Jenerette and Shen 2012 ; Wiersma 2022a , b ). The issue of replication can cause more confusion. Replication can happen at the experimental unit and at the sampling unit, and sometimes researcher can be confused as to what their sample size actually is. An experimental unit is defined by Krebs ( 1989 : 269) as “the smallest division of the experimental material such that any two units may receive different treatments”. A sampling unit, on the other hand, is the thing that the scientist measures to test the effect of the treatment. These can be the same thing; such as when plants are exposed to different light treatments in a greenhouse and the dried weight of the whole plant is taken to assess how light levels affect biomass. If the dark and light halves of the greenhouse had 200 plants each, then there are a total of 400 experimental units (200 × 2 treatment levels) and 400 sampling units. However, if four leaves from each of the plants were measured instead to assess the response, then there would still be 400 experimental units (200 per treatment), but 1600 sampling units. Confounding experimental units and sampling units incorrectly in the statistical analysis can lead to accusations of pseudoreplication (see chapter 4 in Wiersma ( 2022a , b ) for a detailed discussion of this issue as it pertains to landscape experiments).
In this review, I examine experiments designed to understand ecological processes, where space is either an implicit or an explicit component of the study design. Most happen at the ‘typical’ landscape extents of 1–100 km, but I did not limit the review to such studies, since what a small organism perceives as a landscape may be a very small area of just a few square metres or centimetres. My focus is to examine as wide a range of experiments about ecological processes as possible to deduce trends and best practices. There is value to taking landscapes/space into consideration when studying ecological processes. Although many papers published in this journal have examined spatial dimensions of ecological processes (e.g., Webb et al. 2012 ; Ahmed et al. 2016 ; Paca et al. 2019 ; Sieger and Hovestadt 2021 ; Barik et al. 2022 ; Bedane et al. 2022 ; Datta et al. 2022 ; John et al. 2022 ), few of these have been explicitly experimental. Thus, this review examines landscape experiments on ecological processes and experiments on ecological processes carried out with a landscape ecology focus.
Box 1 Six approaches to landscape experiments (from Wiersma 2022a )
Large-scale manipulative experiments—these refer to landscape experiments at extents of ~ 15 ha or greater. These can be observational or manipulative. Examples of long-term manipulative experiments of this type include the Savannah River Experiment (Brinkerhoff et al. 2005 ), the Biological Dynamics of Forest Fragmentation Project (Bierregaard et al. 1992 ) and the Stability of Altered Forest Ecosystems (SAFE) Experiment (Ewers et al. 2011 ).
Experimental model landscapes—these refer to landscape experiment that manipulate a smaller area (usually on the order of 1–15 ha), usually in a more anthropogenically manipulated landscape, such as an agricultural field. Examples include the Bowling Green Fragmentation Experiment (With and Pavuk 2011 ) and the Kansas University Fragmentation Experiment (Holt et al. 1995 ).
Mesocosms—these refer to experiments in artificial containers (e.g., tanks, aquaria), which are either assembled by the researcher (pots with investigator controlled plants grown in them), or are subsets of natural systems (e.g., aquaria with water from an adjacent pond). The experimental design places the mesocosms in situ in the natural environment for experimentation (Srivistava et al. 2004 ).
Microcosms—like mesocosms, microcosms are container experiments; the difference here is that microcosms are naturally occurring containers, or habitats/ecosystems with delineated boundaries, for example pitcher plants or tank bromeliads (Srivistava et al. 2004 ).
In silico landscapes—this refers to experiments involving computer models. These could include (but are not limited to) statistical models, mathematical models, cellular automata and agent-based models.
Novel landscapes—Wiersma ( 2022a ) highlighted how experiments in non-terrestrial landscapes such as seascapes (Pittman 2018 ) and riverscapes (Wiens 2002 ) create opportunities for different kinds of experiments. Similarly, experiments that take a landscape ecology lens to other disciplines such as acoustic ecology (“soundscapes”; Farina 2014) or medical science (“tumor-scapes”; Lloyd et al. 2015 ) or construct artificial landscapes in a laboratory setting (“microlandscapes”; Larsen and Hargreaves 2020 ) can offer new opportunities for experiments to address questions in landscape ecology.
I searched the journal database Scopus (which indexes 18,000 titles from over 5000 international publishers) on 30-May-2022 for papers that addressed ecological process experiments at landscape extents. The search string TITLE-ABS-KEY (“ecological process”) AND TITLE-ABS-KEY (landscape) AND TITLE-ABS-KEY (experiment*) yielded 177 papers. After removing duplicates and government reports and those where I could not access the document (see Fig. 1 for summary), I reviewed the abstracts of all papers, and excluded review/essay/op-ed papers (31), methods papers (8), those with no explicit experiment (24), and those that did not examine an ecological process (14). This yielded 87 papers (see Additional file 1 for full list). For each paper, I noted whether the experiment was observational or manipulative and whether the experimental design was spatially implicit or explicit. I also noted the spatial extent of the study area (if this was not reported, I attempted to infer it either via estimation from included maps, or by searching the internet for details on the study area), the spatial extent of the treatment units (to calculate the scope; Frazier 2022 ; Wiersma and Schneider In press), the degree of replication (of both experimental units and study landscapes) and the type of ecological process under assessment.
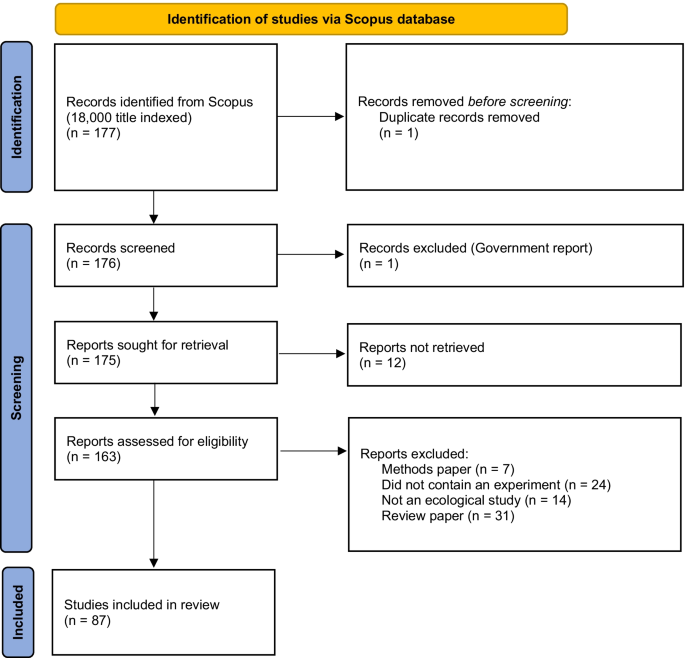
Schematic diagram of article identification and screening and sample size at each stage. Template for figure from: Page MJ, McKenzie JE, Bossuyt PM, Boutron I, Hoffmann TC, Mulrow CD, et al. The PRISMA 2020 statement: an updated guideline for reporting systematic reviews. BMJ 2021;372:n71. https://doi.org/10.1136/bmj.n71
Finally, I classified the experiment based on both type of question (following the taxonomy of Jenerette and Shen 2012 ) and by experimental method. For the latter, I used the six classes discussed in my book (Wiersma 2022a , b ) and summarized briefly in Wiersma ( 2022b ) and here in Box 1 . For clarity, definitions of meso- and microcosm here follow that in the book (Wiersma 2022a ), where mesocosms are artificial containers placed in the environment (e.g., tanks, aquaria) and microcosms are naturally occurring containers (e.g., pitcher plants, tank bromeliads). Microlandscapes refer to artificially constructed landscapes, which the experimenter manipulates under laboratory conditions (e.g., a dendritic network of pipes and petri dishes to assess ciliate movement). Because of the focus on ecological processes, I also noted a few other experiment types (e.g., food addition, seed addition, exclosures (to exclude predators/pollinators)) in addition to the categories in Wiersma ( 2022a , b ).
For the classification by experimental question, I tried to classify observational studies, even though Jenerette and Shen ( 2012 ) excluded such ‘natural experiments’ from their review. For example, I included observational studies that examined a natural disturbance as Type IV.13, even if the researcher did not actively manipulate the disturbance under study. Similarly, I classified observational studies that tested for differences in species distribution under different conditions as perception experiments (Type I.1), even if they were not explicitly manipulative. I did not classify the in silico experiments against Jenerette and Shen’s ( 2012 ) taxonomy, since their review focused on manipulative experiments.
Experiment types
The 87 papers reviewed represented a wide range of journals and disciplines (Table 1 ). Of these 87 papers, 15 were experiments in silico (discussed in further detail below). Of the remaining 72 papers, 17 were observational experiments, 45 were manipulative and 10 included a combination of an observational and manipulative experiment. Only one (Hess and Tschinkel 2017 ) used a full BACI (Before-After-Control-Impact) design. Three papers (Gornall et al. 2007 ; Lu et al. 2018 ; Menzies Pluer et al. 2020 ) had a lab component in addition to a field study, and one (Heggenes et al. 2017 ) transferred microcosms (lichen mats) from the field to the lab for the experimental treatment. The experimental methods are summarized in Table 2 . After in silico experiments, large-scale manipulative and large-scale observational experiments were most common (15 and 10 papers, respectively). As well, there were 11 experiments with some kind of addition, including food (6), artificial nests (1), seeds (2) and nutrients (2).
Research questions
The types of ecological processes addressed did not cover all the categories of Jenerette and Shen ( 2012 ); most common were manipulations of internal patch characteristics (Type III.7) and manipulation of disturbances (Type IV.13; although this count included natural disturbances; hence the number of observational studies in Table 3 does not match what is reported above.
Scale characteristics and replication
The spatial extent of the studies ranged from 78.5 m 2 to 20,300 km 2 and the size of the treatments from 4 cm 2 to ~ 500 km 2 for terrestrial studies, and 20 mL to 1000 L for aquatic/marine studies. The scope (ratio of extent to resolution/grain; Frazier 2022 ) ranged from 1.60 to 3.125 × 10 10 , with a mean of 4.17 × 10 8 . Variation in scope was narrowest for observational experiments and highest when studies combined both observational and manipulative experiments (Fig. 2 ). Replication of treatment units had a mean of 14.1 and median of 5 (range 1–320). Landscape replication was generally low, with 60 papers documenting an experiment in a single landscape, and only 7 papers documenting experiments in more than 2 landscapes (Marini 1997 ; Beckmann and Berger 2003 ; Cardoso et al. 2007 ; Hovel and Wahle 2010 ; Caballero-López et al. 2012 ; Bergerot et al. 2013 ; Augustine and Derner 2014 ; Giometto et al. 2014 ; Smith et al. 2014 ; Fronhofer and Altermatt 2015 ; Gillespie et al. 2017 ; Aristizábal and Metzger 2019 ; DiFiore et al. 2019 ; Menzies Pluer et al. 2020 ; Boone et al. 2022 ; Nunes and Byrne 2022 ).
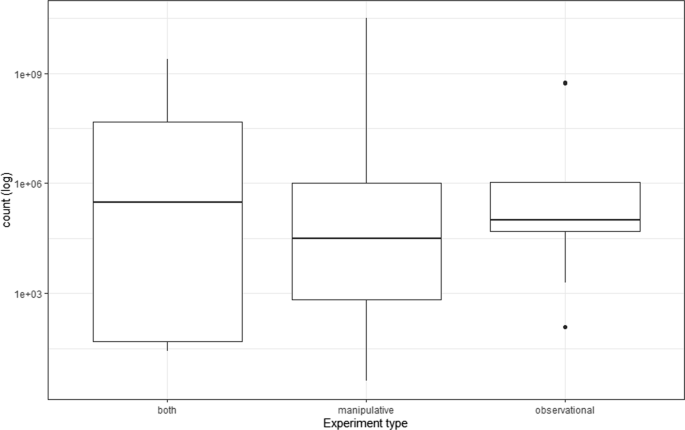
Scope (ratio of grain to extent) of 43 papers in this review. Note the log scale on the y -axis. Scope here is the minimum per paper; some papers had different grain sizes; only the smallest grain was used for the scope calculations. Note that data were not always available to calculate scope, and I only calculated scope for areal studies; studies incorporating treatments by volume or linear treatments (e.g., along streams, or soil depths) were excluded
Modelling tools
The in silico experiments used a range of modelling/computer tools, including cellular automata models (3), demographic models (1), agent-based models (1), process models (2), GIS/remote sensing (3), habitat models (2), scenario models (1) and mathematical models (2). Interestingly, the only paper in the collection obtained with the keyword search above to appear in this journal, was an in silico scenario model of the influence of ecological, economic and social drivers on future ecosystem goods and services (Huber et al. 2014 ). The majority of the in silico experiments modelled some kind of response to disturbance: either fire (Davies et al. 2021 ), grazing (King and Franz 2016 ; Verma et al. 2020 ), or climate change (Keane et al. 2017 ; Cui et al. 2021 ). Others modelled species movement (Samarasin et al. 2017 ; Baggio et al. 2019 ) or habitat use (Rowland et al. 2018 ; Muñoz et al. 2021 ) and still others modelled abiotic processes such as carbon (Güneralp et al. 2014 ; Xu et al. 2017 ), vegetation dynamics (Rango et al. 2002 ) or hydrology (Govind et al. 2011 ).
This review is an exploration of whether and how the themes of ecological processes, experiments and landscape ecology intersect. My findings suggests that experiments on ecological processes that have spatial dimensions occur in many kinds of ecological systems, including oceans (e.g., Cardoso et al. 2007 ), forests (e.g., Hylander 2005 ), urban areas (e.g., Visscher et al. 2018 ) and agricultural systems (e.g., Ouyang et al. 2020 ). Several of the experiments took place in long-term landscape-scale experimental sites, such as the Biological Dynamics of Forest Fragments Project (Laurance et al. 2002 ), the Savannah River Experiment (Levey et al. 2016 ), the Inner Mongolia Grassland Experiment (Yuan et al. 2015 ) and the Kansas Fragmentation Experiment (Alexander et al. 2012 ). Leveraging such long-term projects is a strategic approach to integrating landscape ecology perspectives into studies of ecological processes, since these sites have long-term data, as well as logistical resources and supports for researchers (Wiersma 2022a ).
The papers I reviewed examined a wide range of ecological processes, ranging from dispersal of organisms (e.g., Fronhofer and Altermatt 2015 ) or seeds (e.g., Miguel et al. 2018 ) to nutrient stocks and flows (e.g., Yuan et al. 2015 ). I also found papers carrying out experiments on species interactions such as pollination (e.g., Schmucki and De Blois 2009 ), predation (e.g., Gering and Blair 1999 ) and herbivory (e.g., DiFiore et al. 2019 ). A number of papers had an “applied” focus to restoration or management of ecological systems as evidenced by papers in the Journal of Applied Ecology (6), Restoration Ecology (2). Journal of Environmental Management (2), Forest Ecology and Management (2), Ecological Applications (1) and Ecological Management and Restoration (1) (Table 1 ).
Overall, it appears that many experiments concerned with ecological processes have taken a spatial/landscape approach, and at a range of extents and landscape types. This is not surprising, but what may be more surprising is the relatively low number (87 papers) of papers that are explicitly experimental. If we limit our characterization of an “experiment” to just manipulative experiments and exclude observational experiments, then this number drops to 55. Moreover, for a review focused on ecological processes, there was only a single paper from this journal (Huber et al. 2014 ); this documenting an in silico experiment. Although other papers in Ecological Processes are spatially explicit and borrow concepts and tools from landscape ecology, these did not appear in the keyword search, and were not presented by their authors as experiments. This is likely due to the challenges of doing robust experiments in landscape ecology (Jenerette and Shen 2012 ; Wiersma 2022a , b ).
Even where there are robust spatial experiments, as evidenced here, there can be challenges for researchers to meet the criteria of good experimental design. The majority of experiments occurred in a single landscape, thus making it difficult to assess if the inferences gained from one study would apply in a different landscape. This finding speaks, perhaps to the “case study” approach that characterized early work in landscape ecology (Opdam et al. 2002 ). Although case studies, whether qualitative or quantitative may not be fully replicable experiments, they certainly have a place in research; indeed in the medical and psychological fields, case studies are a major element of knowledge advancement (Stake 2008 ). Thus, researchers and reviewers should not dismiss case studies just because they may not be fully reproducible. Indeed, well-documented case studies can form the basis for valuable meta-analyses (Harrison 2011 ; Koricheva et al. 2013 ; Gerstner et al. 2017 ).
Where there was high replication (more than 2) of the experiment in different landscapes, this was often in anthropogenic systems, such as agricultural fields (Caballero-López et al. 2012 ; Augustine and Derner 2014 ), or when investigating dispersal of organisms that operate at smaller extents, such as butterflies (Bergerot et al. 2013 ) and ciliates (Giometto et al. 2014 ). A few were able to replicate landscapes across a broader extent, such as DiFiore et al. ( 2019 ), who examined two distinct coral reef system in the Caribbean. Experiments in this review generally had limited treatment replication; with 39 of the studies have 10 or fewer treatment replicates, and 25 having fewer than 5. All of the manipulative experiments had some kind of control; observational studies generally were comparisons in space and/or time and did not always have a strict control.
Overall, it appears that ecologists of all types and throughout the world are applying a great deal of creativity to experiments on ecological processes in landscapes. Most are meeting criteria of control and treatment replication; replication at landscape extents in more challenging, which is understandable. While many of the papers reviewed did not explicitly focus on landscape ecology, thinking about ecological process experiments in landscape context could yield useful insights. Experiments at smaller extents may be a strategic way to meet criteria of good experimental design, and with some effort, the inferences might be able to be scaled up to the extents at which human management happens. The experiment on soil organisms’ feeding activities by Joschko et al. ( 2008 ) is a good example of cross-scale work on ecological processes in a landscape. Since ecological processes are scaled in space and time, and landscape ecologists are familiar with scaling issues, considering how to extrapolate from small-scale process experiments to larger-extent landscapes is likely the next frontier to explore. A recent review by Wiersma and Schneider (In press) examined whether microlandscapes and sampling at small scales can usefully be extrapolated to make inferences at larger scales. Larsen and Hargreaves ( 2020 ) reviewed the broad array of microlandscape experiments, but did not examine scaling up in detail. Cross-scaling is facilitated when experiments are at different scales, but have the same scope; where scope is defined as the ratio of the extent to the grain (Frazier 2022 ). The scope of studies in this review varied several orders of magnitude (Fig. 2 ), making it difficult to compare across experiments. Ecologists considering experimental approaches as a means of understanding ecological processes in space would be wise to consider scale effects when designing the experiment. Whether experiments are manipulative or observational, researchers should make careful consideration of sampling design (including grain/extent, and hence scope), and degree of replication, randomization, experimental control and reproducibility in their studies. Although case studies have their place, experiments facilitate better understanding of the mechanisms influencing ecological processes, and thus should not be cast aside simply because they are difficult to do at landscape scales.
Availability of data and materials
The list of all papers reviewed is provided as an Excel spreadsheet in the additional material.
Ahmed F, Fakhruddin ANM, Imam MDT et al (2016) Spatial distribution and source identification of heavy metal pollution in roadside surface soil: a study of Dhaka Aricha highway, Bangladesh. Ecol Process 5:2. https://doi.org/10.1186/s13717-016-0045-5
Article Google Scholar
Alexander HM, Foster BL, Ballantyne F et al (2012) Metapopulations and metacommunities: combining spatial and temporal perspectives in plant ecology. J Ecol 100:88–103. https://doi.org/10.1111/j.1365-2745.2011.01917.x
Aristizábal N, Metzger JP (2019) Landscape structure regulates pest control provided by ants in sun coffee farms. J Appl Ecol 56:21–30. https://doi.org/10.1111/1365-2664.13283
Augustine DJ, Derner JD (2014) Controls over the strength and timing of fire-grazer interactions in a semi-arid rangeland. J Appl Ecol 51:242–250. https://doi.org/10.1111/1365-2664.12186
Baggio JA, Schoon ML, Valury S (2019) Managing networked landscapes: conservation in a fragmented, regionally connected world. Reg Environ Change 19:2551–2562. https://doi.org/10.1007/s10113-019-01567-8
Barik S, Saha GK, Mazumdar S (2022) Influence of land cover features on avian community and potential conservation priority areas for biodiversity at a Ramsar site in India. Ecol Process 11:1. https://doi.org/10.1186/s13717-022-00369-x
Beckmann JP, Berger J (2003) Using black bears to test ideal-free distribution models experimentally. J Mammal 84:594–606. https://doi.org/10.1644/1545-1542(2003)084%3c0594:UBBTTI%3e2.0.CO;2
Bedane GA, Feyisa GL, Senbeta F (2022) Spatial distribution of above ground carbon density in Harana Forest, Ethiopia. Ecol Process 11:4. https://doi.org/10.1186/s13717-021-00345-x
Bergerot B, Tournant P, Moussus J-P et al (2013) Coupling inter-patch movement models and landscape graph to assess functional connectivity. Popul Ecol 55:193–203. https://doi.org/10.1007/s10144-012-0349-y
Bierregaard RO, Lovejoy TE, Kapos V, Hutchings RW (1992) The biological dynamics of tropical rainforest fragments. Bioscience 42:859–866. https://doi.org/10.2307/1312085
Boone SR, Brehm AM, Mortelliti A (2022) Seed predation and dispersal by small mammals in a landscape of fear: effects of personality, predation risk and land-use change. Oikos. https://doi.org/10.1111/oik.08232
Brinkerhoff RJ, Haddad NM, Orrock JL (2005) Corridors and olfactory predator cues affect small mammal behavior. J Mammal 86:662–669. https://doi.org/10.1644/1545-1542(2005)086[0662:CAOPCA]2.0.CO;2
Caballero-López B, Bommarco R, Blanco-Moreno JM et al (2012) Aphids and their natural enemies are differently affected by habitat features at local and landscape scales. Biol Control 63:222–229. https://doi.org/10.1016/j.biocontrol.2012.03.012
Cardoso PG, Raffaelli D, Pardal MA (2007) Seagrass beds and intertidal invertebrates: an experimental test of the role of habitat structure. Hydrobiologia 575:221–230. https://doi.org/10.1007/s10750-006-0375-x
Cui F, Wang B, Zhang Q et al (2021) Climate change versus land-use change—what affects the ecosystem services more in the forest-steppe ecotone? Sci Total Environ 759:143525. https://doi.org/10.1016/j.scitotenv.2020.143525
Article CAS Google Scholar
Datta D, Bairagi M, Dey M et al (2022) Spatially explicit estimation of soil organic carbon stock of an estuarine mangrove wetland of eastern India using elemental analysis and very-fine resolution satellite data. Ecol Process 11:30. https://doi.org/10.1186/s13717-022-00370-4
Davies HF, Visintin C, Gillespie GR, Murphy BP (2021) Investigating the effects of fire management on savanna biodiversity with grid-based spatially explicit population simulations. J Appl Ecol 58:677–686. https://doi.org/10.1111/1365-2664.13801
Diamond JM (1983) Ecology: laboratory, field and natural experiments. Nature 304:586–587. https://doi.org/10.1038/304586a0
Diamond J (2001) Ecology: dammed experiments! Science 294:1847–1848. https://doi.org/10.1126/science.1067012
DiFiore BP, Queenborough SA, Madin EMP et al (2019) Grazing halos on coral reefs: predation risk, herbivore density, and habitat size influence grazing patterns that are visible from space. Mar Ecol Prog Ser 627:71–81. https://doi.org/10.3354/meps13074
Ewers RM, Didham RK, Fahrig L et al (2011) A large-scale forest fragmentation experiment: the stability of altered forest ecosystems project. Phil Trans Royal Soc B Biol Sci 366:3292–3302. https://doi.org/10.1098/rstb.2011.0049
Frazier AE (2022) Scope and its role in advancing a science of scaling in landscape ecology. Landsc Ecol. https://doi.org/10.1007/s10980-022-01403-1
Fronhofer EA, Altermatt F (2015) Eco-evolutionary feedbacks during experimental range expansions. Nat Commun 6:6844. https://doi.org/10.1038/ncomms7844
Gering JC, Blair RB (1999) Predation on artificial bird nests along an urban gradient: predatory risk or relaxation in urban environments? Ecography 22:532–541. https://doi.org/10.1111/j.1600-0587.1999.tb00542.x
Gerstner K, Moreno-Mateos D, Gurevitch J et al (2017) Will your paper be used in a meta-analysis? Make the reach of your research broader and longer lasting. Methods Ecol Evol 8:777–784. https://doi.org/10.1111/2041-210X.12758
Gillespie MAK, Baude M, Biesmeijer J et al (2017) A method for the objective selection of landscape-scale study regions and sites at the national level. Methods Ecol Evol 8:1468–1476. https://doi.org/10.1111/2041-210X.12779
Giometto A, Rinaldo A, Carrara F, Altermatt F (2014) Emerging predictable features of replicated biological invasion fronts. Proc Natl Acad Sci USA 111:297–301. https://doi.org/10.1073/pnas.1321167110
Gornall JL, Jónsdóttir IS, Woodin SJ, Van Der Wal R (2007) Arctic mosses govern below-ground environment and ecosystem processes. Oecologia 153:931–941. https://doi.org/10.1007/s00442-007-0785-0
Govind A, Chen JM, Mcdonnell J et al (2011) Effects of lateral hydrological processes on photosynthesis and evapotranspiration in a boreal ecosystem. Ecohydrology 4:394–410. https://doi.org/10.1002/eco.141
Güneralp I, Filippi AM, Randall J (2014) Estimation of floodplain aboveground biomass using multispectral remote sensing and nonparametric modeling. Int J Appl Earth Obs Geoinf 33:119–126. https://doi.org/10.1016/j.jag.2014.05.004
Haddad NM (2012) Connecting ecology and conservation through experiment. Nat Methods 9:794–795. https://doi.org/10.1038/nmeth.2107
Harrison F (2011) Getting started with meta-analysis. Methods Ecol Evol 2:1–10. https://doi.org/10.1111/j.2041-210X.2010.00056.x
Heggenes J, Odland A, Chevalier T et al (2017) Herbivore grazing—or trampling? Trampling effects by a large ungulate in cold high-latitude ecosystems. Ecol Evol 7:6423–6431. https://doi.org/10.1002/ece3.3130
Hess CA, Tschinkel WR (2017) Effect of thinning and clear-cuts on the transmission of fire in slash pine plantations during restoration to longleaf pine. Ecol Restor 35:33–40. https://doi.org/10.3368/er.35.1.33
Holt RD, Robinson GR, Gaines MS (1995) Vegetation dynamics in an experimentally fragmented landscape. Ecology 76:1610–1624. https://doi.org/10.2307/1938162
Hovel KA, Wahle RA (2010) Effects of habitat patchiness on American lobster movement across a gradient of predation risk and shelter competition. Ecology 91:1993–2002. https://doi.org/10.1890/09-0595.1
Huber R, Briner S, Bugmann H et al (2014) Inter- and transdisciplinary perspective on the integration of ecological processes into ecosystem services analysis in a mountain region. Ecol Process 3:9. https://doi.org/10.1186/2192-1709-3-9
Hylander K (2005) Aspect modifies the magnitude of edge effects on bryophyte growth in boreal forests. J Appl Ecol 42:518–525. https://doi.org/10.1111/j.1365-2664.2005.01033.x
Jenerette GD, Shen W (2012) Experimental landscape ecology. Landsc Ecol 27:1237–1248. https://doi.org/10.1007/s10980-012-9797-1
John K, Bouslihim Y, Isong IA et al (2022) Mapping soil nutrients via different covariates combinations: theory and an example from Morocco. Ecol Process 11:23. https://doi.org/10.1186/s13717-022-00368-y
Joschko M, Oehley J, Gebbers R et al (2008) A spatial approach to soil-ecological experimentation at landscape scale. J Plant Nutr Soil Sci 171:338–343. https://doi.org/10.1002/jpln.200700088
Keane RE, Holsinger LM, Mahalovich MF, Tomback DF (2017) Evaluating future success of whitebark pine ecosystem restoration under climate change using simulation modeling. Restor Ecol 25:220–233. https://doi.org/10.1111/rec.12419
King EG, Franz TE (2016) Combining ecohydrologic and transition probability-based modeling to simulate vegetation dynamics in a semi-arid rangeland. Ecol Model 329:41–63. https://doi.org/10.1016/j.ecolmodel.2016.02.019
Kohler RE (2002) Labscapes and landscapes: exploring the lab-field border in biology. University of Chicago Presss, Chicago and London
Book Google Scholar
Koricheva J, Gurevitch J, Mengersen K (eds) (2013) Handbook of meta-analysis in ecology and evolution. Princeton University Press, Princeton
Google Scholar
Krebs CJ (1989) Ecological methodology. Harper Collins, New York
Larsen C, Hargreaves A (2020) Miniaturizing landscapes to understand species distributions. Ecogrpahy 43:1625–1638. https://doi.org/10.1111/ecog.04959
Laurance WF, Lovejoy TE, Vasconcelos HL et al (2002) Ecosystem decay of Amazonian forest fragments: a 22-year investigation. Conserv Biol 16:605–618. https://doi.org/10.1046/j.1523-1739.2002.01025.x
Levey DJ, Caughlin TT, Brudvig LA et al (2016) Disentangling fragmentation effects on herbivory in understory plants of longleaf pine savanna. Ecology 97:2248–2258. https://doi.org/10.1002/ecy.1466
Lloyd MC, Rejniak KA, Brown JS et al (2015) Pathology to enhance precision medicine in oncology: lessons from landscape ecology. Adv Anat Pathol 22:267–272. https://doi.org/10.1097/PAP.0000000000000078
Lu W, Xiao J, Lei W et al (2018) Human activities accelerated the degradation of saline seepweed red beaches by amplifying top-down and bottom-up forces. Ecosphere 9:e02352. https://doi.org/10.1002/ecs2.2352
Marini MA (1997) Predation-mediated bird nest diversity: an experimental test. Can J Zool 75:317–323. https://doi.org/10.1139/z97-040
Menzies Pluer EG, Schneider RL, Morreale SJ et al (2020) Returning degraded soils to productivity: an examination of the potential of coarse woody amendments for improved water retention and nutrient holding capacity. Water Air Soil Pollut 231:15. https://doi.org/10.1007/s11270-019-4380-x
Miguel MF, Tabeni S, Cona MI, Campos CM (2018) Secondary seed dispersal by mammals between protected and grazed semiarid woodland. For Ecol Manage 422:41–48. https://doi.org/10.1016/j.foreco.2018.03.056
Muñoz P, García-Rodríguez A, Sandoval L (2021) Urbanization, habitat extension and spatial pattern, threaten a costa Rican endemic bird. Revista De Biologia Trop 69:170–180. https://doi.org/10.15517/RBT.V69I1.41742
Nunes AM, Byrne KM (2022) Drought and shrub cover differentially affect seed bank composition within two sagebrush steppe communities. J Arid Environ 202:104752. https://doi.org/10.1016/j.jaridenv.2022.104752
Opdam P, Foppen R, Vos C (2002) Bridging the gap between ecology and spatial planning in landscape ecology. Landsc Ecol 16:767–779
Ouyang F, Su W, Zhang Y et al (2020) Ecological control service of the predatory natural enemy and its maintaining mechanism in rotation-intercropping ecosystem via wheat-maize-cotton. Agr Ecosyst Environ 301:107024. https://doi.org/10.1016/j.agee.2020.107024
Paca VHM, Espinoza-Dávalos GE, Hessels TM et al (2019) The spatial variability of actual evapotranspiration across the Amazon River Basin based on remote sensing products validated with flux towers. Ecol Process 8:6. https://doi.org/10.1186/s13717-019-0158-8
Pittman SJ, Wiens JA, Wu J, Urban DL (2018) Landscape ecologists’ perspectives on seascape ecology. In: Pittman SJ (ed) Seascape ecology. Wiley Blackwell, Hoboken, pp 485–492
Rango A, Goslee S, Herrick J et al (2002) Remote sensing documentation of historic rangeland remediation treatments in southern New Mexico. J Arid Environ 50:549–572. https://doi.org/10.1006/jare.2001.0865
Rowland MM, Wisdom MJ, Nielson RM et al (2018) Modeling elk nutrition and habitat use in western Oregon and Washington. Wildl Monogr 199:1–6
Samarasin P, Shuter BJ, Wright SI, Rodd FH (2017) The problem of estimating recent genetic connectivity in a changing world. Conserv Biol 31:126–135. https://doi.org/10.1111/cobi.12765
Schmucki R, De Blois S (2009) Pollination and reproduction of a self-incompatible forest herb in hedgerow corridors and forest patches. Oecologia 160:721–733. https://doi.org/10.1007/s00442-009-1347-4
Sieger CS, Hovestadt T (2021) The effect of landscape structure on the evolution of two alternative dispersal strategies. Ecol Process 10:73. https://doi.org/10.1186/s13717-021-00343-z
Smith AL, Bull CM, Gardner MG, Driscoll DA (2014) Life history influences how fire affects genetic diversity in two lizard species. Mol Ecol 23:2428–2441. https://doi.org/10.1111/mec.12757
Srivastava DS, Kolasa J, Bengtsson J et al (2004) Are natural microcosms useful model systems for ecology? Trends Ecol Evol 19:379–384. https://doi.org/10.1016/j.tree.2004.04.010
Stake RE (2008) Qualitative case studies. Strategies of qualitative inquiry. Sage Publications, Thousand Oaks, pp 119–149
Turner MG (2005) Landscape ecology in North America: past, present, and future. Ecology 86:1967–1974. https://doi.org/10.1890/04-0890
Turner MG, Gardner RH (2015) Landscape ecology in theory and practice: pattern and process. Springer-Verlag, New York
Verma M, Schulte to Bühne H, Lopes M et al (2020) Can reindeer husbandry management slow down the shrubification of the Arctic? J Environ Manage 267:110636. https://doi.org/10.1016/j.jenvman.2020.110636
Visscher DR, Unger A, Grobbelaar H, Dewitt PD (2018) Bird foraging is influenced by both risk and connectivity in urban parks. J Urban Ecol 4:juy020. https://doi.org/10.1093/jue/juy0020
Webb SL, Olson CV, Dzialak MR et al (2012) Landscape features and weather influence nest survival of a ground-nesting bird of conservation concern, the greater sage-grouse, in human-altered environments. Ecol Process 1:4. https://doi.org/10.1186/2192-1709-1-4
Wiens JA (2002) Riverine landscapes: taking landscape ecology into the water. Freshw Biol 47:501–515. https://doi.org/10.1046/j.1365-2427.2002.00887.x
Wiersma YF (2022a) Experimental landscape ecology. Springer, New York
Wiersma YF (2022b) Carrying out experiments in landscape ecology. Landscape Ecol 37:1729–1732. https://doi.org/10.1007/s10980-022-01459-z
Wiersma YF, Schneider DC (In press) Microlandscape experiments: are they useful for scale, scaling, and cross-scale inference? Curr Landsc Ecol Rep
With KA, Pavuk DM (2011) Habitat area trumps fragmentation effects on arthropods in an experimental landscape system. Landscape Ecol 26:1035–1048. https://doi.org/10.1007/s10980-011-9627-x
Xu B, Pan Y, Plante AF et al (2017) Modeling forest carbon cycle using long-term carbon stock field measurement in the Delaware River Basin. Ecosphere 8:e01802. https://doi.org/10.1002/ecs2.1802
Yuan F, Wu J, Li A et al (2015) Spatial patterns of soil nutrients, plant diversity, and aboveground biomass in the Inner Mongolia grassland: before and after a biodiversity removal experiment. Landscape Ecol 30:1737–1750. https://doi.org/10.1007/s10980-015-0154-z
Download references
Acknowledgements
Thank you to Jiquan Chen for the invitation to submit this review paper.
There was no funding received from any funding sources for this publication.
Author information
Authors and affiliations.
Department of Biology, Memorial University, St. John’s, NL, A1C 5S7, Canada
Yolanda F. Wiersma
You can also search for this author in PubMed Google Scholar
Contributions
YFW collected and reviewed the articles, wrote and revised the paper. The author read and approved the final manuscript.
Corresponding author
Correspondence to Yolanda F. Wiersma .
Ethics declarations
Ethics approval and consent to participate.
Not applicable.
Consent for publication
Competing interests.
The author declares that they have no financial and non-financial competing interests.
Additional information
Publisher's note.
Springer Nature remains neutral with regard to jurisdictional claims in published maps and institutional affiliations.
Supplementary Information
Additional file 1..
The list of all papers reviewed is provided as an Excel spreadsheet.
Rights and permissions
Open Access This article is licensed under a Creative Commons Attribution 4.0 International License, which permits use, sharing, adaptation, distribution and reproduction in any medium or format, as long as you give appropriate credit to the original author(s) and the source, provide a link to the Creative Commons licence, and indicate if changes were made. The images or other third party material in this article are included in the article's Creative Commons licence, unless indicated otherwise in a credit line to the material. If material is not included in the article's Creative Commons licence and your intended use is not permitted by statutory regulation or exceeds the permitted use, you will need to obtain permission directly from the copyright holder. To view a copy of this licence, visit http://creativecommons.org/licenses/by/4.0/ .
Reprints and permissions
About this article
Cite this article.
Wiersma, Y.F. A review of landscape ecology experiments to understand ecological processes. Ecol Process 11 , 57 (2022). https://doi.org/10.1186/s13717-022-00401-0
Download citation
Received : 26 July 2022
Accepted : 26 August 2022
Published : 09 September 2022
DOI : https://doi.org/10.1186/s13717-022-00401-0
Share this article
Anyone you share the following link with will be able to read this content:
Sorry, a shareable link is not currently available for this article.
Provided by the Springer Nature SharedIt content-sharing initiative
- Landscape ecology
- Manipulative experiment
- Observational experiment
Restoring Tropical Forests: Lessons Learned from Case Studies on Three Continents
- First Online: 15 June 2023
Cite this chapter
- Nigel I. J. Tucker 5 , 6 ,
- Stephen Elliott 7 ,
- Karen D. Holl 8 &
- Rakan A. Zahawi 8 , 9 , 10
762 Accesses
Restoring the structural complexity and functional diversity of tropical rainforest is not possible in human time scales but knowledge of the process has significantly increased over the past three decades. Strategies to restore tropical forests must build on theories of community assembly and succession, as well as understanding of both the local ecological and human communities. In this chapter, we discuss three long-term tropical forest restoration case studies in Australia, Costa Rica, and Thailand, each using specific approaches tailored to overcome local ecological, cultural, and socioeconomic constraints. Differences are apparent in the intensity of restoration intervention adopted to manage ecological issues, and in the way local cultures, prevailing socioeconomic conditions, and therefore costs can influence outcomes. Based on the unifying threads identified, we detail key factors essential to recovering tropical biodiversity whilst protecting the livelihoods of landholders on whose land restoration is most likely to occur.
Lead authors of the case studies: Costa Rica: Karen D. Holl & Rakan A. Zahawi; Thailand: Stephen Elliott and Australia: Nigel I. J. Tucker.
This is a preview of subscription content, log in via an institution to check access.
Access this chapter
- Available as EPUB and PDF
- Read on any device
- Instant download
- Own it forever
- Durable hardcover edition
- Dispatched in 3 to 5 business days
- Free shipping worldwide - see info
Tax calculation will be finalised at checkout
Purchases are for personal use only
Institutional subscriptions
Policies and incentives, developed under the UN Framework Convention on Climate Change, to finance restoration and conservation of forests as carbon sinks.
Abram, N. K., MacMillan, D. C., Xofis, P., Ancrenaz, M., Tzanopoulos, J., Ong, R., Goossens, B., Koh, L. P., Del Valle, C., Peter, L., Morel, A. C., Lackman, I., Chung, R., Kler, H., Ambu, L., Baya, W., & Knight, A. T. (2016). Identifying where REDD+ financially out-competes oil palm in floodplain landscapes using a fine-scale approach. PLoS One, 11 , e0156481.
Article PubMed PubMed Central Google Scholar
Allen, M. F., Allen, E. B., & Gomez-Pompa, A. (2005). Effects of mycorrhizae and nontarget organisms on restoration of a seasonal tropical forest in Quintana Roo, Mexico: Factors limiting tree establishment. Restoration Ecology, 13 , 325–333.
Article Google Scholar
Armenteras, D., Dávalos, L. M., Barreto, J. S., Miranda, A., Hernández-Moreno, A., Zamorano-Elgueta, C., González-Delgado, T. M., Meza-Elizalde, M. C., & Retana, J. (2021). Fire-induced loss of the world’s most biodiverse forests in Latin America. Science Advances, 7 , eabd3357.
Bagong Pagasa Foundation. (2011). Cost comparison analysis ANR compared to conventional reforestation . FAO Regional Office for Asia and the Pacific.
Google Scholar
Blakesley, D., Elliott, S., Kuarak, C., Navakitbumrung, P., Zangkum, S., & Anusarnsunthorn, V. (2002). Propagating framework tree species to restore seasonally dry tropical forest: Implications of seasonal seed dispersal and dormancy. Forest Ecology and Management, 164 , 31–38.
Boissier, O., Feer, F., Henry, P.-Y., & Forget, P.-M. (2020). Modifications of the rain forest frugivore community are associated with reduced seed removal at the community level. Ecological Applications, 30 , e02086.
Article PubMed Google Scholar
Bradbury, R. B., Butchart, S. H. M., Fisher, B., Hughes, F. M. R., Ingwall-King, L., MacDonald, M. A., Merriman, J. C., Peh, K. S. H., Pellier, A.-S., Thomas, D. H. L., Trevelyan, R., & Balmford, A. (2021). The economic consequences of conserving or restoring sites for nature. Nature Sustainability, 4 , 602–608.
Brancalion, P. H. S., & Holl, K. D. (2020). Guidance for successful tree planting initiatives. Journal of Applied Ecology, 57 , 2349–2361.
Brancalion, P. H. S., Meli, P., Tymus, J. R. C., Lenti, F. E. B., Benini, R. M., Silva, A. P. M., Isernhagen, I., & Holl, K. D. (2019). What makes ecosystem restoration expensive? A systematic cost assessment of projects in Brazil. Biological Conservation, 240 , 108274.
Camargo, P. H. S. A., Pizo, M. A., Brancalion, P. H. S., & Carlo, T. A. (2020). Fruit traits of pioneer trees structure seed dispersal across distances on tropical deforested landscapes: Implications for restoration. Journal of Applied Ecology, 57 , 2329–2339.
Article CAS Google Scholar
Campbell, N. J. H. (1995). Mitochondrial control region variation in two genera of Australian rodents; Melomys and Uromys: Application to phylogenetics, phylogeography and conservation . Southern Cross University.
Carpenter, F. L., Mayorga, S. P., Quintero, E. G., & Schroeder, M. (2001). Land-use and erosion of a Costa Rican ultisol affect soil chemistry, mycorrhizal fungi and early regeneration. Forest Ecology and Management, 144 , 1–17.
Catterall, C. P., & Harrison, D. A. (2006). Rainforest restoration activities in Australia’s tropics and subtropics rainforest . Cooperative Research Centre for Tropical Rainforest Ecology and Management Rainforest CRC.
Chang, K., & Andersson, K. P. (2019). Contextual factors that enable forest users to engage in tree-planting for forest restoration. Land Use Policy, 104 , 104017.
Chawengkul, P. (2019). Effects of forest restoration age on species diversity of epiphytic bryophyte community . Naresuan University.
Chayaporn, P., Sasaki, N., Venkatappa, M., & Abe, I. (2021). Assessment of the overall carbon storage in a teak plantation in Kanchanaburi province, Thailand – Implications for carbon-based incentives. Cleaner Environmental Systems, 2 , 100023.
Chazdon, R. L. (2003). Tropical forest recovery: Legacies of human impact and natural disturbances. Perspectives in Plant Ecology Evolution and Systematics, 6 , 51–71.
Chazdon, R. L., & Guariguata, M. R. (2016). Natural regeneration as a tool for large-scale forest restoration in the tropics: Prospects and challenges. Biotropica, 48 , 716–730.
Cole, R. J., Holl, K. D., Zahawi, R. A., Wickey, P., & Townsend, A. R. (2016). Leaf litter arthropod responses to tropical forest restoration. Ecology and Evolution, 6 , 5158–5168.
Corbin, J. D., & Holl, K. D. (2012). Applied nucleation as a forest restoration strategy. Forest Ecology and Management, 265 , 37–46.
Cordell, S., Ostertag, R., Rowe, B., Sweinhart, L., Vasquez-Radonic, L., Michaud, J., Cole, T. C., & Schulten, J. R. (2009). Evaluating barriers to native seedling establishment in an invaded Hawaiian lowland wet forest. Biological Conservation, 142 , 2997–3004.
de Almeida, D. R. A., Stark, S. C., Valbuena, R., Broadbent, E. N., Silva, T. S. F., de Resende, A. F., Ferreira, M. P., Cardil, A., Silva, C. A., Amazonas, N., Zambrano, A. M. A., & Brancalion, P. H. S. (2020). A new era in forest restoration monitoring. Restoration Ecology, 28 , 8–11.
de Souza, S. E. X. F., Vidal, E., Chagas, G. d. F., Elgar, A. T., & Brancalion, P. H. S. (2016). Ecological outcomes and livelihood benefits of community-managed agroforests and second growth forests in Southeast Brazil. Biotropica, 48 , 868–881.
DellaSala, D. A., Martin, A., Spivak, R., Schulke, T., Bird, B., Criley, M., Van Daalen, C., Kreilick, J., Brown, R., & Aplet, G. (2003). A citizen’s call for ecological forest restoration: Forest restoration principles and criteria. Ecological Restoration, 21 , 14–23.
Di Sacco, A., Hardwick, K. A., Blakesley, D., Brancalion, P. H. S., Breman, E., Cecilio Rebola, L., Chomba, S., Dixon, K., Elliott, S., Ruyonga, G., Shaw, K., Smith, P., Smith, R. J., & Antonelli, A. (2021). Ten golden rules for reforestation to optimize carbon sequestration, biodiversity recovery and livelihood benefits. Global Change Biology, 27 , 1328–1348.
Duchelle, A. E., Seymour, F., Brockhaus, M., Angelsen, A., Moeliono, M., Wong, G. Y., Pham, T. T., & Martius, C. (2018). REDD+: Lessons from national and subnational implementation . World Resources Institute.
Elliott, S. (2018). The interface between forest science and policy—A review of the IUFRO International and Multidisciplinary Scientific Conference 4–7 October 2016: Forestry-related policy and governance: Analyses in the environmental social sciences. Natural History Bulletin of the Siam Society, 63 , 1–10.
Elliott, S., & Kuaraksa, C. (2008). Producing framework tree species for restoring forest ecosystems in northern Thailand. Small-Scale Forestry, 7 , 403–415.
Elliott, S., Navakitbumrung, P., Zangkum, S., Kuarak, C., Kerby, J., Blakesley, D., & Anusarnsunthorn, V. (2000). Performance of six native tree species, planted to restore degraded forestland in northern Thailand and their response to fertiliser. In S. Elliott, J. Kerby, D. Blakesley, K. Hardwick, K. Woods, & V. Anusarnsunthorn (Eds.), Thailand: Restoration for wildlife conservation: International tropical timber organization and the forest restoration research unit (pp. 244–255). Chiang Mai University.
Elliott, S., Kuarak, C., Navakitbumrung, P., Zangkum, S., Anusarnsunthorn, V., & Blakesley, D. (2002). Propagating framework trees to restore seasonally dry tropical forest in northern Thailand. New Forest, 23 , 63–70.
Elliott, S., Navakitbumrung, P., Kuarak, C., Zangkum, S., Anusarnsunthorn, V., & Blakesley, D. (2003). Selecting framework tree species for restoring seasonally dry tropical forests in northern Thailand based on field performance. Forest Ecology and Management, 184 , 177–191.
Elliott, S., Kuarak, C., Tunjai, P., Polchoo, T., Thongtao, J., & Maxwell, J. (2008). A technical strategy for restoring Krabi’s lowland tropical forest . Forest Restoration Research Unit, Chiang Mai University.
Elliott, S., Blakesley, D., & Hardwick, K. (2013). Restoring tropical forests: A practical guide . Royal Botanical Garden.
Elliott, S., Chairuangsri, S. Shannon, D. Nippanon, P., & Amphon, R. (2018). Where science meets communities: Developing forest restoration approaches for Northern Thailand. Natural History Bulletin of the Siam Society, 63 , 11–26.
Evans, K., Guariguata, M. R., & Brancalion, P. H. S. (2018). Participatory monitoring to connect local and global priorities for forest restoration. Conservation Biology, 32 , 525–534.
FAO. (2014). Documenting ANR impacts on biodiversity, water quality and carbon sequestration (Internal Report, Project: TCP/RAS/3307).
FAO. (2019). Restoring forest landscapes through assisted natural regeneration (ANR) – A practical manual . Food and Agriculture Organization.
Florentine, S. K., Pohlman, C. L., & Westbrooke, M. E. (2016). The effectiveness of different planting frameworks for recruitment of tropical rainforest species on ex-rainforest land. Restoration Ecology, 24 , 364–372.
Forest Restoration Research Unit. (2008). Research for restoring tropical forest ecosystems: A practical guide . Chiang Mai University.
FORRU-CMU. (2005). How to plant a forest: The principles and practice of restoring tropical forests . Chiang Mai University.
Fremout, T., Thomas, E., Taedoumg, H., Briers, S., Gutiérrez-Miranda, C. E., Alcázar-Caicedo, C., Lindau, A., Mounmemi Kpoumie, H., Vinceti, B., Kettle, C., Ekué, M., Atkinson, R., Jalonen, R., Gaisberger, H., Elliott, S., Brechbühler, E., Ceccarelli, V., Krishnan, S., Vacik, H., Wiederkehr-Guerra, G., Salgado-Negret, B., González, M. A., Ramírez, W., Moscoso-Higuita, L. G., Vásquez, Á., Cerrón, J., Maycock, C., & Muys, B. (2022). Diversity for Restoration (D4R): Guiding the selection of tree species and seed sources for climate-resilient restoration of tropical forest landscapes. Journal of Applied Ecology, 59 , 664–679.
Galetti, M., Guevara, R., Côrtes, M. C., Fadini, R., Von Matter, S., Leite, A. B., Labecca, F., Ribeiro, T., Carvalho, C. S., Collevatti, R. G., Pires, M. M., Guimarães, P. R., Brancalion, P. H., Ribeiro, M. C., & Jordano, P. (2013). Functional extinction of birds drives rapid evolutionary changes in seed size. Science, 340 , 1086–1090.
Article CAS PubMed Google Scholar
Gann, G. D., McDonald, T., Walder, B., Aronson, J., Nelson, C. R., Jonson, J., Hallett, J. G., Eisenberg, C., Guariguata, M. R., Liu, J., Hua, F., Echeverría, C., Gonzales, E., Shaw, N., Decleer, K., & Dixon, K. W. (2019). International principles and standards for the practice of ecological restoration (Restoration ecology) (Vol. 27, 2nd ed., pp. S1–S46).
Gilmour, D. (2007). Applying an adaptive management approach to FLR. In J. Reitbergen-McCraken, S. Maginnis, & A. Sarre (Eds.), The forest landscape restoration handbook (pp. 29–38). Earthscan.
González-Varo, J. P., Carvalho, C. S., Arroyo, J. M., & Jordano, P. (2017). Unravelling seed dispersal through fragmented landscapes: Frugivore species operate unevenly as mobile links. Molecular Ecology, 26 , 4309–4321.
Goosem, S., & Tucker, N. I. G. (2013). Repairing the rainforest (2nd ed.). Wet Tropics Management Authority and Biotropica Australia.
Gregorio, N., Herbohn, J., Tripoli, R., & Pasa, A. (2020). A local initiative to achieve global forest and landscape restoration challenge—Lessons learned from a community-based forest restoration project in Biliran Province, Philippines. Forests, 11 , 475.
Guariguata, M. R., & Brancalion, P. H. S. (2014). Current challenges and perspectives for governing forest restoration. Forests, 5 , 3022–3030.
Guariguata, M. R., Garcia-Fernandez, C., Sheil, D., Nasi, R., Herrero-Jauregui, C., Cronkleton, P., & Ingram, V. (2009). Compatibility of timber and non-timber forest product management in natural tropical forests: Perspectives, challenges, and opportunities. Forest Ecology and Management, 259 , 237–245.
Guevara, S., Purata, S. E., & Van der Maarel, E. (1986). The role of remnant forest trees in tropical secondary succession. Vegetatio, 66 , 77–84.
Hagazi, N., Gebrekirstos, A., Birhane, E., Bongers, F., Kelly, R., & Brauning, A. (2020). Land restoration requires a shift from quantity to quality: Lessons from Tigray, Ethiopia. ETFRN News , p. 60.
Hobbs, R. J., & Norton, D. A. (1996). Towards a conceptual framework for restoration ecology. Restoration Ecology, 4 , 93–110.
Holl, K. D. (2007). Oldfield vegetation succession in the Neotropics. In R. J. Hobbs & V. A. Cramer (Eds.), Old fields (pp. 93–117). Island Press.
Holl, K. D. (2012). Tropical forest restoration. In J. Van Andel & J. Aronson (Eds.), Restoration ecology (pp. 103–114). Blackwell Publishing.
Chapter Google Scholar
Holl, K. D. (2017). Research directions in tropical forest restoration. Annals of the Missouri Botanical Garden, 102 , 237–250.
Holl, K. D. (2020). Primer of ecological restoration . Island Press.
Holl, K. D., & Aide, T. M. (2011). When and where to actively restore ecosystems? Forest Ecology and Management, 261 , 1558–1563.
Holl, K. D., & Zahawi, R. A. (2014). Factors explaining variability in woody above-ground biomass accumulation in restored tropical forest. Forest Ecology and Management, 319 , 36–43.
Holl, K. D., Loik, M. E., Lin, E. H. V., & Samuels, I. A. (2000). Tropical montane forest restoration in Costa Rica: Overcoming barriers to dispersal and establishment. Restoration Ecology, 8 , 339–349
Holl, K. D., Zahawi, R. A., Cole, R. J., Ostertag, R., & Cordell, S. (2011). Planting seedlings in tree islands versus plantations as a large-scale tropical forest restoration strategy. Restoration Ecology, 19 , 470–479.
Holl, K. D., Stout, V. M., Reid, J. L., & Zahawi, R. A. (2013). Testing heterogeneity-diversity relationships in tropical forest restoration. Oecologia, 173 , 569–578.
Holl, K. D., Reid, J. L., Chaves-Fallas, J. M., Oviedo-Brenes, F., & Zahawi, R. A. (2017). Local tropical forest restoration strategies affect tree recruitment more strongly than does landscape forest cover. Journal of Applied Ecology, 54 , 1091–1099.
Holl, K. D., Reid, J. L., Oviedo-Brenes, F., Kulikowski, A. J., & Zahawi, R. A. (2018). Rules of thumb for predicting tropical forest recovery. Applied Vegetation Science, 21 , 669–677.
Holl, K. D., Reid, J. L., Cole, R. J., Oviedo-Brenes, F., Rosales, J. A., & Zahawi, R. A. (2020). Applied nucleation facilitates tropical forest recovery: Lessons learned from a 15-year study. Journal of Applied Ecology, 57 , 2316–2328.
Howe, H. F. (2016). Making dispersal syndromes and networks useful in tropical conservation and restoration. Global Ecology and Conservation, 6 , 152–178.
Howe, H. F., & Smallwood, J. (1982). Ecology of seed dispersal. Annual Review of Ecology and Systematics, 13 , 201–228.
Jansen, P. A., Hirsch, B. T., Emsens, W.-J., Zamora-Gutierrez, V., Wikelski, M., & Kays, R. (2012). Thieving rodents as substitute dispersers of megafaunal seeds. Proceedings of the National Academy of Sciences, 109 , 12610–12615.
Jantawong, K., Elliott, S., & Wangpakapattanawong, P. (2017). Above-ground carbon sequestration during restoration of upland evergreen forest in northern Thailand. Open Journal of Forestry, 7 , 157–171.
Jantawong, K., Kavinchan, N., Wangpakapattanawong, P., & Elliott, S. (2022). Financial analysis of potential carbon value over 14 years of forest restoration by the framework species method. Forests, 13 , 144.
Janzen, D. H. (2002). Tropical dry forest: Area de Conservación Guanacaste, northwestern Costa Rica. In M. R. Perrow & A. J. Davy (Eds.), Handbook of ecological restoration (pp. 559–583). Cambridge University Press.
Kavinchan, N., Wangpakapattanawong, P., Elliott, S., Chairuangsri, S., & Pinthong, J. (2015). Use of the framework species method to restore carbon flow via litterfall and decomposition in an evergreen tropical forest ecosystem, northern Thailand. Agriculture and Natural Resources, 49 , 639–650.
CAS Google Scholar
Kim, S. (2012). Identifying framework tree species for restoring forest ecosystems in Siem Reap Province . Royal University of Agriculture.
Kulikowski, A. J., Zahawi, R. A., Werden, L. K., Zhu, K., Holl Karen, D. (2023). Restoration interventions mediate tropical tree recruitment dynamics over time. Philosophical Transactions of the Royal Society B-Biological Sciences, 378, 20210077.
Lamb, D., Erskine, P. D., & Parrotta, J. D. (2005). Restoration of degraded tropical forest landscapes. Science, 310 , 1628–1632.
Lanuza, O., Casanoves, F., Zahawi, R. A., Celentano, D., Delgado, D., & Holl, K. D. (2018). Litterfall and nutrient dynamics shift in tropical forest restoration sites after a decade of recovery. Biotropica, 50 , 491–498.
Lees, A. M., Sejian, V., Wallage, A. L., Steel, C. C., Mader, T. L., Lees, J. C., & Gaughan, J. B. (2019). The impact of heat load on cattle. Animals, 9 , 322.
Letcher, S. G., & Chazdon, R. L. (2009). Rapid recovery of biomass, species richness, and species composition in a forest chronosequence in northeastern Costa Rica. Biotropica, 41 , 608–617.
Mansourian, S. (2020). Enabling factors to scale up forest landscape restoration: The roles of governance and economics . WWF/IUFRO.
Mansourian, S., & Vallauri, D. (2014). Restoring forest landscapes: Important lessons learnt. Environmental Management, 53 , 241–251.
Mappin, B., Ward, A., Hughes, L., Watson, J. E. M., Cosier, P., & Possingham, H. P. (2021). The costs and benefits of restoring a continent’s terrestrial ecosystems. Journal of Applied Ecology, 59 , 408–419.
Marín-Spiotta, E., Cusack, D. F., Ostertag, R., & Silver, W. L. (2008). Trends in above and belowground carbon with forest regrowth after agricultural abandonment in the neotropics. In R. Myster (Ed.), Post-agricultural succession in the neotropics (pp. 22–72). Springer.
Maxwell, J. F. (2001). Vegetation and vascular flora of Doi Sutep-Pui National park, northern Thailand . Biodiversity Research and Training Program.
McDonald, T., Jonson, J., & Dixon, K. W. (2016). National standards for the practice of ecological restoration in Australia. Restoration Ecology, 24 , S4–S32.
Meli, P., Martínez-Ramos, M., Rey-Benayas, J. M., & Carabias, J. (2014). Combining ecological, social and technical criteria to select species for forest restoration. Applied Vegetation Science, 17 , 744–753.
Mendenhall, C. D., Sekercioglu, C. H., Brenes, F. O., Ehrlich, P. R., & Daily, G. C. (2011). Predictive model for sustaining biodiversity in tropical countryside. Proceedings of the National Academy of Sciences of the United States of America, 108 , 16313–16316.
Article CAS PubMed PubMed Central Google Scholar
Moreno-Mateos, D., Barbier, E. B., Jones, P. C., Jones, H. P., Aronson, J., Lopez-Lopez, J. A., McCrackin, M. L., Meli, P., Montoya, D., & Benayas, J. M. R. (2017). Anthropogenic ecosystem disturbance and the recovery debt. Nature Communications, 8, 14163.
Nandakwang, P., Elliott, S., Youpensook, S., Dell, B., Teaumroon, N., & Lumyong, S. (2008). Arbuscular mycorrhizal status of indigenous tree species used to restore seasonally dry tropical forest in northern Thailand. Research Journal of Microbiology, 3 , 51–61.
Nepstad, D. C., Stickler, C. M., Soares, B., & Merry, F. (2008). Interactions among Amazon land use, forests and climate: Prospects for a near-term forest tipping point. Philosophical Transactions of the Royal Society B-Biological Sciences, 363 , 1737–1746.
Article PubMed Central Google Scholar
Norden, N., Angarita, H. A., Bongers, F., Martinez-Ramos, M., Granzow-de la Cerda, I., Breugel, M., Lebrija-Trejos, E., Meave, J. A., Vandermeer, J., Williamson, G. B., Finegan, B., Mesquita, R., & Chazdon, R. L. (2015). Successional dynamics in Neotropical forests are as uncertain as they are predictable. Proceedings of the National Academy of Sciences of the United States of America, 112 , 8013–8018.
Ong, R. (2011). Recent forest restoration initiatives in Sabah, Malaysia. In P. B. Durst, P. Sajise, & L. Leslie (Eds.), Forests beneath the grass (pp. 119–124). Food and Agriculture Organization, Regional Office for Asia and the Pacific.
Paetkau, D., Vazquez-Dominguez, E., Tucker, N. I. G., & Moritz, C. (2009). Monitoring movement into and through a newly planted rainforest corridor using genetic analysis of natal origin. Ecological Management & Restoration, 10 , 201–216.
Parrotta, J. A., Knowles, O. H., & Wunderle, J. M. (1997). Development of floristic diversity in 10-year-old restoration forests on a bauxite mined site in Amazonia. Forest Ecology and Management, 99 , 21–42.
Pedrini, S., & Dixon, K. W. (2020). International principles and standards for native seeds in ecological restoration. Restoration Ecology, 28 , S286–S303.
Phongchiewboon, A. (2006). Recovery of lichen diversity during forest restoration in northern Thailand . Chiang Mai University, M.Sc. thesis.
Pirard, R., de Buren, G., & Lapeyre, R. (2014). Do PES improve the governance of forest restoration? Forests, 5 , 404–424.
Porras, I., Grieg-Gran, M., & Neves, N. (2008). A review of payments for watershed services in developing countries (Natural resource issues no. 11). International Institute for Environment and Development.
Pothong, T., Elliott, S., Chairuangsri, S., Chanthorn, W., Shannon, D. P., & Wangpakapattanawong, P. (2021). New allometric equations for quantifying tree biomass and carbon sequestration in seasonally dry secondary forest in northern Thailand. New Forest, 53 , 17.
Powers, J. S., & Marín-Spiotta, E. (2017). Ecosystem processes and biogeochemical cycles in secondary tropical forest succession. Annual Review of Ecology, Evolution, and Systematics, 48 , 497–519.
Ramos, D. L., Pizo, M. A., Ribeiro, M. C., Cruz, R. S., Morales, J. M., & Ovaskainen, O. (2020). Forest and connectivity loss drive changes in movement behavior of bird species. Ecography, 43 , 1203–1214.
Raupp, P. P., Ferreira, M. C., Alves, M., Campos-Filho, E. M., Sartorelli, P. A. R., Consolaro, H. N., & Vieira, D. L. M. (2020). Direct seeding reduces the costs of tree planting for forest and savanna restoration. Ecological Engineering, 148 , 105788.
Reid, J. L., Mendenhall, C. D., Rosales, J. A., Zahawi, R. A., & Holl, K. D. (2014). Landscape context mediates avian habitat choice in tropical forest restoration. PLoS One, 9 , e90573.
Reid, J. L., Holl, K. D., & Zahawi, R. A. (2015a). Seed dispersal limitations shift over time in tropical forest restoration. Ecological Applications, 25 , 1072–1082.
Reid, J. L., Mendenhall, C. D., Zahawi, R. A., & Holl, K. D. (2015b). Scale-dependent effects of forest restoration on Neotropical fruit bats. Restoration Ecology, 23 , 681–689.
Reid, J. L., Chaves-Fallas, J. M., Holl, K. D., & Zahawi, R. A. (2016). Tropical forest restoration enriches vascular epiphyte recovery. Applied Vegetation Science, 19 , 508–517.
Reid, J. L., Fagan, M. E., Lucas, J., Slaughter, J., & Zahawi, R. A. (2019). The ephemerality of secondary forests in southern Costa Rica. Conservation Letters, 12 , e12607.
Reid, J. L., Korrman, U., Zarate-Charry, D., Holl, K. D., & Zahawi, R. A. (2021). Multi-scale habitat selection of key frugivores predicts large-seeded tree recruitment in tropical forest restoration. Ecosphere, 12, e03868.
Rodrigues, R. R., Lima, R. A. F., Gandolfi, S., & Nave, A. G. (2009). On the restoration of high diversity forests: 30 years of experience in the Brazilian Atlantic Forest. Biological Conservation, 142 , 1242–1251.
Sansupa, C., Purahong, W., Wubet, T., Tiansawat, P., Pathom-Aree, W., Teaumroong, N., Chantawannakul, P., Buscot, F., Elliott, S., & Disayathanoowat, T. (2021). Soil bacterial communities and their associated functions for forest restoration on a limestone mine in northern Thailand. PLoS One, 16 , e0248806.
Sangsupan, H. A., Hibbs, D. E., Withrow-Robinson, B. A., & Elliott, S. (2018). Seed and microsite limitations of large-seeded, zoochorous trees in tropical forest restoration plantations in northern Thailand. Forest Ecology and Management, 419–420, 91–100.
Sapanthuphong, A., Thampituk, S., & SukIn, A. (2011). Restoration of degraded forests in dry areas: Concepts and practices for forest restoration in the western region . Elephant Conservation Network.
Schwartz, N. B., Aide, T. M., Graesser, J., Grau, H. R., & Uriarte, M. (2020). Reversals of reforestation across Latin America limit climate mitigation potential of tropical forests. Frontiers in Forests and Global Change, 3 , 85.
Shaw, J. A., Roche, L. M., & Gornish, E. S. (2020). The use of spatially-patterned methods for vegetation restoration and management across systems. Restoration Ecology, 28 , 766–775.
Silver, W. L., Ostertag, R., & Lugo, A. E. (2000). The potential for carbon sequestration through reforestation of abandoned tropical agricultural and pasture lands. Restoration Ecology, 8 , 394–407.
Sinhaseni, K. (2008). Natural establishment of tree seedlings in forest restoration trials at Ban Mae Sa Mai, Chiang Mai Province . M.Sc. thesis, Chiang Mai University.
Sloan, S., Meyfroidt, P., Rudel, T. K., Bongers, F., & Chazdon, R. (2019). The forest transformation: Planted tree cover and regional dynamics of tree gains and losses. Global Environmental Change, 59 , 101988.
Swinfield, T., Afriandi, R., Antoni, F., & Harrison, R. D. (2016). Accelerating tropical forest restoration through the selective removal of pioneer species. Forest Ecology and Management, 381 , 209–216.
TEEB. (2009). TEEB climate issues update . http://www.teebweb.org/publication/climate-issues-update/
Toktang, T. (2005). The effects of forest restoration on the species diversity and composition of a bird community in Doi Suthep-Pui National Park Thailand from 2002–2003 . M.Sc. thesis, Chiang Mai University.
Tucker, N. I. J. (2000). Linkage restoration: Interpreting fragmentation theory for the design of a restored habitat linkage in tropical north-eastern Queensland. Ecological Management & Restoration, 1 , 35–42.
Tucker, N. I. J., & Murphy, T. M. (1997). The effects of ecological rehabilitation on vegetation recruitment: Some observations from the Wet Tropics of North Queensland. Forest Ecology and Management, 99 , 133–152.
Tucker, N. I. G., & Simmons, T. (2009). Restoring a rainforest habitat linkage in north Queensland: Donaghy’s Corridor. Ecological Management & Restoration, 10 , 98–112.
Vázquez-Yanes, C., & Orozco-Segovia, A. (1993). Patterns of seed longevity and germination in the tropical rainforest. Annual Review of Ecology and Systematics, 24 , 69–87.
Viani, R. A. G., Holl, K. D., Padovezi, A., Strassburg, B. B. N., Farah, F. T., Garcia, L. C., Chaves, R. B., Rodrigues, R. R., & Brancalion, P. H. S. (2017). Protocol for monitoring tropical forest restoration: Perspectives from the Atlantic Forest Restoration Pact in Brazil. Tropical Conservation Science, 10 , 1940082917697265.
Viani, R. A. G., Barreto, T. E., Farah, F. T., Rodrigues, R. R., & Brancalion, P. H. S. (2018). Monitoring young tropical forest restoration sites: How much to measure? Tropical Conservation Science, 11 , 1940082918780916.
Vieira, D. L. M., & Scariot, A. (2006). Principles of natural regeneration of tropical dry forests for restoration. Restoration Ecology, 14 , 11–20.
Wangpakapattanawong, P., Kavinchan, N., Vaidhayakarn, C., Schmidt-Vogt, D., & Elliott, S. (2010). Fallow to forest: Applying indigenous and scientific knowledge of swidden cultivation to tropical forest restoration. Forest Ecology and Management, 260 , 1399–1406.
Werden, L. K., Holl, K. D., Rosales, J. A., Sylvester, J. M., & Zahawi, R. A. (2020). Effects of dispersal- and niche-based factors on tree recruitment in tropical wet forest restoration. Ecological Applications, 30 , e02139.
Werden, L. K., Holl, K. D., Chaves-Fallas, J. M., Oviedo-Brenes, F., Rosales, J. A., & Zahawi, R. A. (2021). Degree of intervention affects interannual and within-plot heterogeneity of seed arrival in tropical forest restoration. Journal of Applied Ecology, 58 , 1693–1704.
Weyerhaeuser, H., & Kahrl, F. (2006). Planting trees on farms in southwest China. Mountain Research and Development, 26 , 205–208.
Wilson, S. J., Alexandre, N. S., Holl, K. D., Reid, J. L., Zahawi, R. A., Celentano, D., Sprenkle-Hyppolite, S. D., & Werden, L. K. (2021). Applied nucleation guide for tropical forests . Conservation International.
Zahawi, R. A., & Reid, J. L. (2018). Tropical secondary forest enrichment using giant stakes of keystone figs. Perspectives in Ecology and Conservation, 16 , 133–138.
Zahawi, R. A., Reid, J. L., & Holl, K. D. (2014). Hidden costs of passive restoration. Restoration Ecology, 22 , 284–287.
Zahawi, R. A., Werden, L. K., San-José, M., Rosales, J. A., Flores, J., & Holl, K. D. (2021). Proximity and abundance of mother trees affects recruitment patterns in a long-term tropical forest restoration study. Ecography, 44 , 1826–1837.
Zarin, D. J., Ducey, M. J., Tucker, J. M., & Salas, W. A. (2001). Potential biomass accumulation in Amazonian regrowth forests. Ecosystems, 4 , 658–668.
Zimmerman, J. K., Aide, T. M., & Lugo, A. E. (2007). Implications of land use history for natural forest regeneration and restoration strategies in Puerto Rico. In R. J. Hobbs & V. A. Cramer (Eds.), Old fields (pp. 51–74). Island Press.
Download references
Acknowledgements
Thanks are due to NSF for financial backing connected to the Islas Project (DEB 05-15577; DEB 09-18112; DEB 14-56520, DEB 20-16623), which provided support for the work of K.D.H. and R.A.Z. Also, thanks are recorded to Biotropica Australia Pty Ltd. for supporting NT’s study and to Chiang Mai University for supporting S.E.’s work on this chapter. We are grateful to the many field assistants and landowners who made the results reported here possible.
Author information
Authors and affiliations.
College of Science and Engineering, James Cook University, Cairns, Australia
Nigel I. J. Tucker
Biotropica Australia, Tarzali, QLD, Australia
Forest Restoration Research Unit, Department of Biology and Environmental Science Research Center, Faculty of Science, Chiang Mai University, Chiang Mai, Thailand
Stephen Elliott
Environmental Studies Department, University of California, Santa Cruz, CA, USA
Karen D. Holl & Rakan A. Zahawi
Lyon Arboretum and School of Life Sciences, University of Hawai’i at Mānoa, Honolulu, HI, USA
Rakan A. Zahawi
Charles Darwin Foundation, Puerto Ayora, Ecuador
You can also search for this author in PubMed Google Scholar
Corresponding author
Correspondence to Nigel I. J. Tucker .
Editor information
Editors and affiliations.
Future Regions Research Centre, Federation University Australia, Ballarat, VIC, Australia
Singarayer Florentine
Ecological Restoration Department, Kalbar Operations, Bairnsdale, VIC, Australia
Paul Gibson-Roy
School of Molecular and Life Sciences, ARC Centre for Mine Site Restoration, Curtin University, Bentley, WA, Australia
Kingsley Wayne Dixon
National Research Collections Australia, Centre for Australian National Biodiversity Research, CSIRO, Canberra, ACT, Australia
Linda Broadhurst
Rights and permissions
Reprints and permissions
Copyright information
© 2023 The Author(s), under exclusive license to Springer Nature Switzerland AG
About this chapter
Tucker, N.I.J., Elliott, S., Holl, K.D., Zahawi, R.A. (2023). Restoring Tropical Forests: Lessons Learned from Case Studies on Three Continents. In: Florentine, S., Gibson-Roy, P., Dixon, K.W., Broadhurst, L. (eds) Ecological Restoration. Springer, Cham. https://doi.org/10.1007/978-3-031-25412-3_3
Download citation
DOI : https://doi.org/10.1007/978-3-031-25412-3_3
Published : 15 June 2023
Publisher Name : Springer, Cham
Print ISBN : 978-3-031-25411-6
Online ISBN : 978-3-031-25412-3
eBook Packages : Biomedical and Life Sciences Biomedical and Life Sciences (R0)
Share this chapter
Anyone you share the following link with will be able to read this content:
Sorry, a shareable link is not currently available for this article.
Provided by the Springer Nature SharedIt content-sharing initiative
- Publish with us
Policies and ethics
- Find a journal
- Track your research

An official website of the United States government
The .gov means it’s official. Federal government websites often end in .gov or .mil. Before sharing sensitive information, make sure you’re on a federal government site.
The site is secure. The https:// ensures that you are connecting to the official website and that any information you provide is encrypted and transmitted securely.
- Publications
- Account settings
Preview improvements coming to the PMC website in October 2024. Learn More or Try it out now .
- Advanced Search
- Journal List

Experimental approach and initial forest response to a simulated ice storm experiment in a northern hardwood forest
Lindsey e. rustad.
1 USDA Forest Service, Northern Research Station, Durham, New Hampshire, United States of America
John L. Campbell
Charles t. driscoll.
2 Department of Civil and Environmental Engineering, Syracuse University, Syracuse, New York, United States of America
Timothy J. Fahey
3 Department of Natural Resources, Cornell University, Ithaca, New York, United States of America
Peter M. Groffman
4 Advanced Science Research Center at the Graduate Center, City University of New York, New York, New York, United States of America
5 Cary Institute of Ecosystem Studies, Millbrook, New York, United States of America
Paul G. Schaberg
6 USDA Forest Service, Northern Research Station, Burlington, Vermont, United States of America
Gary J. Hawley
7 Rubenstein School of Environment and Natural Resources, University of Vermont, Burlington, Vermont, United States of America
8 USDA Forest Service, Northern Research Station, North Woodstock, New Hampshire, United States of America
Frank Bowles
9 Research Designs, Lyme, New Hampshire, United States of America
Wendy Leuenberger
10 Department of Forestry and Natural Resources, University of Kentucky, Lexington, Kentucky, United States of America
Geoffrey Schwaner
11 Hubbard Brook Research Foundation, North Woodstock, New Hampshire, United States of America
Gabriel Winant
Brendan leonardi, associated data.
The data underlying the results presented in the study are available from Hubbard Brook Ecosystem Study at https://hubbardbrook.org/d/hubbard-brook-data-catalog .
Ice storms are a type of extreme winter weather event common to north temperate and boreal forests worldwide. Recent climate modelling studies suggest that these storms may become more frequent and severe under a changing climate. Compared to other types of storm events, relatively little is known about the direct and indirect impacts of these storms on forests, as naturally occurring ice storms are inherently difficult to study. Here we describe a novel experimental approach used to create a suite of ice storms in a mature hardwood forest in New Hampshire, USA. The experiment included five ice storm intensities (0, 6.4, 12.7 and 19.1 mm radial ice accretion) applied in a single year, and one ice storm intensity (12.7 mm) applied in two consecutive years. Results demonstrate the feasibility of this approach for creating experimental ice storms, quantify the increase in fine and coarse woody debris mass and nutrients transferred from the forest canopy to the soil under the different icing conditions, and show an increase in the damage to the forest canopy with increasing icing that evolves over time. In this forest, little damage occurred below 6.4 mm radial ice accretion, moderate damage occurred with up to 12.7 mm of accretion, and significant branch breakage and canopy damage occurred with 19.1 mm of ice. The icing in consecutive years demonstrated an interactive effect of ice storm frequency and severity such that some branches damaged in the first year of icing appeared to remain in the canopy and then fall to the ground in the second year of icing. These results have implications for National Weather Service ice storm warning levels, as they provide a quantitative assessment of ice-load related inputs of forest debris that will be useful to municipalities creating response plans for current and future ice storms.
Introduction
The increase in the frequency, intensity and severity of extreme weather events is emerging as a significant concern of climate change in the Anthropocene [ 1 , 2 ]. These types of episodic events can have a greater impact on natural and managed ecosystems than the more gradual changes in mean temperature and precipitation that are typically associated with climate change [ 3 , 4 ]. Despite the importance of these extreme weather events in shaping ecosystems, relatively little is known about their short- or long-term impacts. They are inherently difficult to study due to infrequent return intervals, high spatial variation in occurrence and intensity, difficulty to forecast reliably, and safety concerns [ 5 , 6 ]. Much of the literature on ecosystem response to extreme weather events is derived from post facto analyses following naturally occurring events such as ice storms, late spring frosts or microbursts [ 7 – 9 ]. These studies generally rely on serendipitous pre-event data, lack true controls and rarely have immediate post-storm impact assessments. Further, the historical understanding of ecosystem response to extreme events based on these post facto studies may not be useful in predicting responses to future events, as the underlying template of climate, nutrient reserves, or ecosystem health may have changed over the intervening years [ 10 ].
As an alternative to post facto analyses, a growing number of controlled experiments are emerging to help elucidate and model ecosystem responses to changes in the frequency, intensity and severity of extreme events [e.g., 11 , 12 ]. These experiments allow for investigation of cause and-effect relationships with rigorous statistical models. They typically include pre- and post-extreme event conditions as well as multiple levels and frequencies of disturbance that allow for determination of response thresholds (i.e., the level of disturbance when an ecosystem irreversibly shifts from one state to another) [ 4 , 6 , 13 ]. Experiments also have the advantage that they can be implemented under controlled conditions designed to improve safety associated with working in hazardous conditions.
Ice storms are a type of extreme winter weather event that are a major driver of forest disturbance in temperate and boreal ecosystems worldwide [ 14 – 18 ]. Climate modeling studies suggest that these types of winter storms will become more frequent and/or more intense under a changing climate [ 19 , 20 ]. Impacts of ice storms on forests depend on a variety of factors including amount of ice accretion, stand age, stand health and species composition [ 21 ]. Direct impacts range from twig and branch breakage with light-to-moderate icing, to loss of tree tops and uprooting of entire trees with severe icing [ 16 , 22 – 24 ]. Based on post facto studies, these changes can lead to a cascade of changes in forest ecosystem structure and function, including increased inputs of coarse and fine woody debris to the forest floor, increases in light below the forest canopy, reductions in photosynthetic capacity and stored plant carbohydrates, alterations of soil temperature and moisture, changes in belowground plant and microbial processes, and altered leaching and gaseous nutrient losses from the soil [ 5 , 8 , 25 – 27 ].
Despite this general understanding of the impacts of ice storms on forest ecosystems, manipulation of icing intensity and frequency followed by investigation of ecosystem effects have not been attempted to date. Given the widespread occurrence of these extreme weather events in north temperate and boreal regions, their immediate and lasting impacts, and projections for increased frequency and intensity under a changing climate, there is an urgent need to better understand the impacts of a range of ice loadings and return intervals on forest ecosystems.
The objectives of this paper are to (1) provide a detailed methodological approach for creating experimental ice storms, (2) discuss the efficacy of the design (i.e., our ability to create glaze ice on surfaces), (3) describe possible artifacts of the treatments, and (4) present results on the initial impact of these experimental storms on nutrient transfers in fine and coarse woody debris and tree canopy damage assessments. This work was conducted at the Hubbard Brook Experimental Forest (HBEF) in New Hampshire, builds on a feasibility study at the same site [ 17 ], and complements reporting by Fahey et al. [ 28 ] on the response of forest canopy structure to these experimental perturbations. Fahey et al. [ 28 ] detailed how experimental ice storms increased canopy openness, light transmission and complexity. Here, we describe the initial loss of wood and nutrients from the canopy that contributed to those changes in canopy structure. The detailed description of the methods and initial results on fine and coarse woody debris inputs and tree canopy damage will aid in the design of future studies on ice storm impacts specifically, and extreme weather events more generally, and inform utility companies, emergency management organizations, forest managers, municipalities and the public on how to better prepare for future ice storms.
Materials and methods
Site description.
The HBEF is a 3,160-ha valley in central New Hampshire, USA (43°56’46”N, 71°47’19”W) ( Fig 1A ). The climate is humid continental with mean monthly air temperatures ranging from -9 °C in January to 18°C in July [ 29 ]. Annual precipitation averages 1400 mm (30% as snow) and is distributed relatively evenly throughout the year. Much of the Hubbard Brook Valley was cut approximately 100 years ago, with subsequent impacts from a hurricane in 1938 and an ice storm in 1998.

(A) Experiment location within the Hubbard Brook Experimental Forest. (B) Ice plot location between the Hubbard Brook (top right) and the Hubbard Brook Experimental Forest access road (bottom). Plots 1–5 are in Block 1 and plots 6–10 are in Block 2. Asterisk indicates plots that were iced in both 2016 and 2017. (C) Individual ice plot design, showing the entire plot (20 x 30 m), the inner plot (10 x 20 m), and subplots (5x5 m) for destructive and non-destructive measurements.
The ice storm experiment was conducted in the central portion of the Hubbard Brook Valley (~520 m altitude; 43°56’09”N, 71°45’51”W; Fig 1a ). Total basal for all trees ≥ 5cm D.B.H. within the experimental plots was 37.9 m 2 /ha and was comprised of eight species: sugar maple ( Acer saccharum Marsh.) (34%), red maple ( Acer rubrum L.) (25%), American beech ( Fagus grandifolia Ehrh.) (17%), yellow birch ( Betula alleghaniensis Britton) (16%), red spruce ( Picea rubens Sarg.) (3%), bigtooth aspen ( Populus grandidentata (Michx . ) (2%), balsam fir ( Abies balsamea (L.) Mill.) (2%) and paper birch ( Betula paprifera Marsh.) (1%) ( Fig 2 ). Canopy height was ~20 m and stand age was estimated at 70–100 years in 2015, with the stand likely originating from the 1938 hurricane. No significant differences in basal area were observed across the treatment plots for any species at the start of the study ( Fig 2 ).

Treatments did not differ in mean basal area for any species ( P > 0.05). Vertical lines are ± 1 standard error.
Experimental design
Ten 20×30 m plots were established in May 2015 and were blocked by location, with plots 1 through 5 in one block on the eastern side of the study site, and plots 6 through 10 on the western side ( Fig 1B ). Within the block, plots were randomly assigned to one of five icing treatments, resulting in two replicate plots per treatment ( Fig 1B ). Treatments were based on target radial ice accretion, which is defined as the radius of ice added to a cylindrical structure such as a wire or branch [ 30 ]. Treatments (in mm radial ice accretion) included Control (0 mm), Low (6.4 mm), Mid (12.7 mm), High (19.1 mm), and Mid×2 (12.7 mm applied in two consecutive winters). The Low and Mid treatments were chosen to correspond to National Weather Service Ice Storm Warnings, which are 6.4 mm for mid-Atlantic states and 12.7 mm for New York and New England [ 31 ]. The High treatment was chosen to represent an extreme ice storm comparable to the 1998 storm [ 8 ].. The minimum distance between plots was 10 m. The rectangular plot shape maximized the ability to ice the interior of plots from outside the plots.
The entire 20×30-m plot was iced during treatments. All response measurements were made within an inner 10×20-m area, allowing for a 5-m buffer zone ( Fig 1C ). The inner 10×20-m plot was further subdivided into eight 5×5-m subplots ( Fig 1C ). Four of these subplots were used for non-destructive measurements repeated over time; three were used for destructive measurements; and one was undisturbed and reserved for future measurements.
Ice application
To create ice, stream water (~4°C) was taken from Hubbard Brook by two low pressure/high volume pumps and supplied to two track-equipped utility terrain vehicles (UTV) via moveable fire hoses. Each UTV was equipped with a BB4 ® centrifugal pump that fed high pressure water to a nozzle mounted next to the pump. Pump nozzles were pressurized to flow at approximately 300 liters per minute. All pumps were gasoline powered. One UTV operated along each of the two long sides of the plot, with each spraying water the full length and half the depth of the plot ( Fig 1C ; S1 Fig ). Water was sprayed up through gaps in the tree canopy, with spray nozzles adjusted so that the water would be deposited over the canopy as a fine mist, freezing on contact with vegetation surfaces. The two UTV teams worked simultaneously, spraying layers of ice on the canopy from opposite sides of the plot. The total amount of time of water application was recorded for each UTV ensemble, with approximately equal icing times on both sides. Icing continued until visual inspection of branches within the plots and physical measurements of ice accretion on twigs indicated that the target ice accretion had been achieved. Final measurements of ice accretion were made immediately following the icing treatments on passive ice collectors, as described below.
Weather conditions during icing
Air temperature was measured using two Campbell Scientific ® HMP 60 thermistors housed in gill solar radiation shields, with one located near plot 9 and one near plot 2. Wind speed was measured with a RM Young ® model 05103 anemometer at a weather station approximately 5 km east of the experimental plots (250 m altitude). Application dates were chosen to meet criteria assuring effective ice accumulation on canopy surfaces (air temperature < -4°C) with minimal drift of mist (i.e. wind speed < 4.5 m/s) as detailed by Rustad and Campbell [ 17 ]. Much of the applications took place at night when low temperature requirements were met.
Water volume and chemistry of application
Volume of water applied per plot was calculated as the product of application time and water flow. To construct chemical budgets for the treatments, a 60 ml aliquot of Hubbard Brook stream water was collected prior to each icing, and analyzed for sulfate (SO 4 ), nitrate (NO 3 ) and chloride (Cl) on a Metrohm ® Ion Chromatograph; calcium (Ca), magnesium (Mg), potassium (K), and sodium (Na) on an Agilent ® 730 ICP optical emission spectrometer; dissolved organic carbon (DOC) and total dissolved nitrogen (TDN) on a Shimadzu ® TOCV with a TNM-1 nitrogen (N) detector; and ammonium (NH 4 ) by colorimetry on a SEAL Analytical AQ2 discrete analyzer at the Louis C. Wyman Forest Sciences Laboratory, Durham, NH. Dissolved organic N (DON) was calculated as the difference between TDN and inorganic N (NO 3 -N + NH 4 -N). Total mass of elements applied to the canopy as iced ‘precipitation’ was calculated as the ‘precipitation’ chemistry from the stream aliquot multiplied by the ‘precipitation’ volume for each plot.
Ice accretion
Ice accretion was measured using passive collectors constructed from 2.54 cm diameter birch dowels, with six 30-cm long arms radiating out from a central six-way galvanized steel connector in six directions (0°, 90°, 180°, 270°, up and down; Fig 3 ). Four collectors were suspended in the forest canopy using parachute cord (to provide a low resistance surface to facilitate release after icing) at a height of ~15 m prior to the icing, with one collector in each of the four cardinal corners of the inner plots.

Radial ice accretion on collectors was measured with two methods. First, ice thickness was measured in the field immediately following icing with digital calipers at multiple points along the six dowel arms. Second, after ice thickness measurements were made in the field, each of the six arms of the collectors were cut at the base with a reciprocating saw, being careful to keep the ice on the dowel intact. Ice-coated dowels were placed individually in labeled zip lock bags and transported back to HBEF Headquarters where the ice was melted at room temperature and the volume of meltwater from each dowel measured. The radial ice accretion equivalent (Req) was calculated using the equation from [ 30 ]:
where D = diameter of the dowel (cm), L = length of the dowel (cm); V = volume of melted ice (mL), and ρ i = density of glaze ice (g cm -3 ).
Canopy throughfall volume and chemistry
Canopy throughfall volume was measured with 20 L (28.7 cm diameter) buckets placed on the ground in the center of each subplot (eight per plot). Two of these buckets were acid washed and designated for chemical analyses. After icing, buckets were retrieved, thawed, and the volume of canopy throughfall in each bucket was measured. Aliquots from the two acid-washed buckets per plot were poured off, refrigerated, and analyzed for SO 4 , NO 3 , Cl, Ca, Mg, K, Na, NH 4 , DOC, TDN and DON by the same methods as for the stream water aliquots. Total throughfall flux for each element in each plot was calculated as the mean plot chemistry multiplied by the mean plot volume.
Fine and coarse woody debris
Fine woody debris (i.e., woody material < 2 cm in diameter; hereafter FWD) and foliar litter were collected in plastic baskets (52L×37W×27H cm) that were placed in the center of each of seven subplots for all plots. Foliar litter was collected during the pre-treatment period 5 August, 2015 to 17 January, 2016. Fine woody debris was collected between two to four weeks after icing treatments in 2016 and ten weeks after icing treatments in 2017 to allow all the near-term broken branches to reach the ground. In instances where larger branches lay on the litter baskets, twigs less than 2 cm were clipped around the perimeter of the basket and were included as part of the sample. All foliar litter and FWD samples were oven dried at 60°C to constant weight, weighed, ground through a Wiley Mill ® #40 mesh sieve, and analyzed for total carbon (C) and nitrogen (N) on a Thermo Flash ® EA 1112 Series CN Analyzer ® and for total Ca, Mg, K, and phosphorus (P) by microwave digest and analysis on an ICP [ 32 ]at the Louis C. Wyman Forest Sciences Laboratory, Durham, NH. Total mass of all elements was calculated as total litter mass multiplied by element concentration.
Coarse woody debris (i.e., woody material ≥2 cm diameter; hereafter CWD) was collected on a 2m×2m quadrat located within each of the three subplots designated for destructive measurements on each of the main plots ( Fig 1C ). On November 16, 2015, prior to icing, all CWD was collected from these quadrats. Post-treatment CWD was collected in July and November of 2016 and 2017. Downed branches and tree boles that crossed quadrat boundaries were cut on the border with a hand saw. Coarse woody debris was sorted into five decay classes as described in Table 1 [after 33 ]. The wet weight of CWD for all categories was measured in the field for each quadrat. A subsample comprised of approximately twenty percent of the total wet weight of CWD for each category was brought back to the laboratory and oven-dried at 60°C to a constant weight to obtain oven dry mass and a moisture correction factor. Samples were ground and analyzed for nutrients by the same method as for FWD. Total mass of all elements was calculated as total litter mass multiplied by element concentration.
Tree canopy damage assessment
A qualitative tree canopy damage assessment was made pre- and post-treatment, following methods used after the 1998 ice storm and the HBEF pilot ice storm experiment [ 34 ]. In this method, all trees greater than 5.0 cm DBH were identified, tagged, measured (DBH, height), and mapped. Basal area was calculated from tree diameter by species. All tagged trees were assigned to an injury class value based on a visual inspection of the branches, using the following crown injury classes: 1 = no visible damage; 2 = 1–49%; 3 = 50–75%; 4 = >75%; 5 = dead. Damage assessments were made on May 12, 2015, August 9, 2016, August 16, 2017, August 7, 2018 and July 1, 2019.
Statistical analysis
Ice, throughfall and precipitation.
A series of linear mixed effects models were used to analyze throughfall ice accretion, water volumes and chemistry. Response variables included ice accretion (mm), water volume (mm depth m -2 ) and mass (mg m -2 ) of 10 analytes (Ca 2+ , Mg 2+ , K + , Na + , Cl - , SO 4 2- -S, NO 3 - -N, NH 4 + -N, DON, DOC); explanatory variables included treatment and a random effect for plot. The lme4 package in R was used to conduct mixed effects analyses [ 35 , 36 ]. As only one sample was collected per plot for precipitation, plot was not used as a random effect for these data. The emmeans package in R was used to conduct multiple comparisons testing and an α of 0.05 was used to assess significance for both throughfall and precipitation [ 37 ]. We calculated the amount of ice accretion retained in the canopy as the volume of precipitation minus the volume of throughfall.
Linear mixed effect models were used to analyze FWD and CWD total mass, C, N, P, Ca, Mg, and K mass. Response variables included FWD and CWD total mass and nutrients; explanatory variables included treatment (for FWD and CWD), sampling period (for CWD) and treatment × sampling period (for CWD). Plot was incorporated as a random effect. An α of 0.05 was used to compare treatments (for FWD and CWD) and sampling periods (for CWD). Cumulative link mixed models were used to assess decay class as an ordered, categorical variable [ 38 ]. CWD mass was used as weights in the decay class models. Differences among treatments were calculated in each sampling period, using treatment as a fixed effect and plot as a random effect. Decay classes from the July 2016 and 2017 samples were compared for Mid×2 plots. Weighted means of decay classes are presented as numbers to illustrate differences among treatments.
Cumulative link mixed models were used to assess damage as an ordered, categorical variable. Fixed effects were treatment × sampling period × species; and tree was used as a random effect. Only trees within the inner 10×20 m buffer were included in the analysis. Eight trees were excluded from analyses from one year of sampling ( n 2017 = 2, n 2018 = 6) because they were not found or were not assigned a damage class. Multiple comparisons testing with an α of 0.05 were used to compare treatments and sampling periods. We conducted three rounds of analyses: 1) all species including all treatments, species was not included as a fixed effect in this model; 2) American beech, sugar maple, and yellow birch including all treatments; and 3) American beech, red maple, sugar maple, and yellow birch, including the Control, Mid, Mid×2, and High treatments as there were no red maple trees in the Low treatment. Weighted means of damage classes are presented as numbers to illustrate differences among treatments, sampling periods and species.
Air temperature and wind speed during the spray applications were generally within the target range of < -4°C and < 4.5 m/s ( Table 2 ). Mean temperatures across the plots during spray applications ranged from -12.1 to -5.3°C, and mean wind speeds across the plots ranged from 0.23 to 1.2 m/s.
Air temperatures are from sensors at the plots; wind speeds are from Hubbard Brook Experimental Forest weather station 1, approximately 5 km from the treatment plots.
Water and chemical inputs
Because stream water used for the applications had greater concentrations of most chemical constituents than ambient precipitation at Hubbard Brook, inputs of many chemicals (especially base cations, Cl and SO 4 ) exceeded those of natural ice storms and comprised inputs comparable in magnitude to annual precipitation inputs at the site ( Table 3 ). Chemical inputs generally increased with increased treatment applications. Throughfall water volume and chemical constituent inputs were generally lower than those for precipitation water and chemical constituent inputs (reflecting water and chemical retention in the canopy as ice) and followed similar patterns to those described for precipitation volume and chemical constituents ( Table 3 ).
Different letters indicate significant differences among the treatments ( P < 0.05).
Canopy water retention and ice accretion
During the first winter of treatments (2016), 45 (Plot 3) to 68 (Plot 2) percent of the water sprayed onto plots was retained by the canopy as ice ( Table 1 ). During the second winter of treatments (2017), 9 (Plot 1) and 42 (Plot 8) percent of the water sprayed onto plots was retained by the canopy as ice ( Table 1 ).
The two methods for measuring ice accretion were in close agreement for smaller treatments (< 10 mm) with few icicles. Specifically, ice accretion measured by calipers was 111 and 99 percent of ice accretion measured by melted water volume for the Low and Mid treatments, respectively ( Fig 4 ). However, when estimated ice accretion was >10 mm, and icicles were more conspicuous, ice accretion measured by calipers was 75, 85 and 82 percent of ice accretion measured by melted water volume for Mid×2-yr1, Mid×2-yr2 and High treatments, respectively ( Fig 4 ).

Different letters denote significant differences among treatments ( P <0.05). Vertical lines are ±1 standard error.
In general, the Low, Mid×2-yr1 and Mid×2-yr2 treatments were close to target levels (89 to 99 percent, 87 to 115 percent, and 89 to 104 percent of target values based on caliper and volume estimates, respectively), whereas the Mid and the High treatments were below targets (66 to 67 percent and 70 to 86 percent of target values for caliper and volume estimates, respectively; Fig 4 ). Across all treatments, measured ice accretion showed a stepwise increase in values from Low- to Mid- to High ice accretion targets, resulting in a suite of experimental light to moderate to severe ice storms ( Fig 4 ).
Fine woody debris
Mean pre-treatment inputs of foliar litter across the treatments ranged from 1.67 to 310 g m -2 for total mass, 0.79 to 147 g m -2 for C, 0.01 to 4.61 g m -2 for N, 1.12 to 454 mg m -2 for P, 0.01 to 3.75 g m -2 for Ca, 1.49 to 659 mg m -2 for Mg and 5.20 to 1201 mg m -2 for K. There were no significant pre-treatment differences in foliar litter mass and nutrients among treatments.
In February 2016, the first ice applications resulted in a general stepwise increase in the FWD from the Control to the High treatment plots ( Fig 5 ). Statistically, the amounts of FWD deposited on the Mid and Mid×2 treatment plots were greater than the amount deposited on the Control treatment plots; and the amount of FWD deposited on the High treatment plots was greater than the amount deposited on the Low and Control treatment plots ( Fig 5 ). In 2017, following the second ice applications on the Mid×2 treatment plots, significantly greater FWD was deposited on the Mid×2 treatment plots compared to all other treatments ( Fig 5 ). Notably, the FWD deposited on the Mid×2 treatment plots was 2.1 times greater after the 2017 treatment compared to the 2016 treatment ( P < 0.01).

Different letters denote significant differences among treatments ( P <0.05) within a sampling period. Vertical lines are 95% confidence intervals.
The mass of FWD nutrients generally followed the same patterns as for total mass for both years. ( Table 4 ).
Letters signify differences among treatments within a sampling period using P < 0.05.
Coarse woody debris
Total mass and element content of CWD removed from the plots in November 2015 was highly variable, reflecting the localized history of inputs. Across all treatment plots, mean CWD mass ranged from 475.08 to 1564.05 g m -2 , mean C mass ranged from 225.79 to 750.79 g m -2 , mean N mass ranged from 1.48 to 2.72 g m -2 , mean P mass ranged from 0.07 to 0.12 g m -2 , mean Ca mass ranged from 1.75 to 4.19 g m -2 , mean Mg mass ranged from 0.12 to 0.36 g m -2 , and mean K mass ranged from 0.20 to 0.55 g m -2 . No significant pre-treatment differences were observed among the treatment plots for total mass or any of the elemental masses. Weighted mean decay class across all treatment plots was 3.5, with no significant differences among the treatments.
In July 2016, the first ice applications resulted in a general stepwise increase in CWD mass from the Control to the High treatment plots ( Fig 6 ). Significantly greater CWD mass was deposited on the Mid treatment plots compared to the Control plots, and significantly greater CWD mass was deposited on the High treatment plots compared to the other treatment plots ( Fig 6 ). Mean decay classes differed significantly among treatments. Decay classes were lowest (i.e., the wood was least decayed) for the High and Mid×2 treatment plots (weighted mean decay classes = 1.3 for both), mid-range for the Mid treatment plots (weighted mean decay class = 1.7), and highest for the Control and Low treatment plots (weighted mean decay classes = 2.3 and 2.6, respectively). Little additional CWD fell between July and November 2016 ( Fig 6 ), and the sample size (only 12 quadrats total with measurable CWD) was too low for meaningful statistical comparisons.

Following the second year of ice applications to the Mid×2 plots, a larger mass of CWD was deposited on the Mid×2 plots compared to the other treatment plots (July 2017, Fig 6 ). This amount was similar to the CWD mass deposited after the 2016 Mid and Mid×2 ice applications. Mean decay classes differed significantly among the treatments. Decay classes were lower in the High, Mid×2 and Mid treatment plots (weighted mean decay classes = 2.6, 2.1, and 2.4, respectively) compared to the Control and Low treatment plots (weighted mean decay classes = 3.7 and 3.6, respectively). For the Mid×2 treatments, decay classes were greater for CWD collected in July 2017 compared to CWD collected in July 2016 (weighted mean decay class = 2.08 vs 1.27, respectively; P < 0.001). Little additional CWD fell between July and November 2017 ( Fig 6 ). Decay classes differed significantly among the treatments, with mean decay class in Mid and Mid×2 treatment plots (weighted mean decay classes = 2.7 and 2.5, respectively) lower than the Control plots (weighted mean decay class = 3.6). The Mid treatment had a lower decay class than the High treatment plots as well (weighted mean decay classes = 2.7 and 3.6, respectively). Thus, nearly all the CWD resulting from the experimental ice applications fell during and immediately after the applications, the CWD was generally less decayed in the Mid, Mid×2 and High treatment plots compared to the Control and Low treatment plots, and the CWD that fell in 2017 had a greater component of older, more decayed wood than the CWD that fell in 2016.
Total mass of CWD nutrient elements generally followed those of total CWD mass ( Table 5 ).
Tree canopy damage assessments
For the damage assessments for all trees per plot combined, tree canopy damage indices were not significantly different among the treatments prior to icing (May 2015). In August 2016, the combined mean tree canopy damage indices were significantly greater in the Mid and High treatment plots compared to the Control and Low treatment plots, with the Mid×2 at an intermediate value. In August 2017, the combined mean tree canopy damage indices were significantly greater in the Mid×2 treatment plots compared to the Control plots ( Fig 7 ). In August 2018, the combined mean tree canopy damage indices were significantly greater in the Mid, Mid×2, and High treatment plots compared to the Controls, and by July 2019, the combined mean tree canopy damage indices were significantly higher in all four treatments compared to the Controls ( Fig 7 ).

Different letters denote significant differences among treatments ( P <0.05) within a sampling period. Vertical lines are 1 standard error.
The combined mean canopy tree damage indices increased in all treatments, including the Control, over the five years of the study, but the Mid, Mid×2 and High treatment plots showed a greater increase in damage over time than the Control and Low treatment plots, which resulted in the significantly higher damage indices for the assessments completed in the summers of 2018 and 2019 ( Fig 7 ).
For individual species, American beech had numerically the lowest initial values for mean canopy tree damage indices (1.2 in the Control treatment to 1.6 in the Mid treatment), followed by yellow birch (1.2 in the Mid×2 treatment to 2.3 in the Mid treatment), red maple (1.3 in the Mid×2 treatment to 2.6 in the Control treatment), and then sugar maple (1.6 in the Mid treatment to 2.6 in the Control treatment) ( Fig 7 ).
For American beech, no significant differences were observed in mean tree canopy damage indices among treatments prior to icing (May 2015) ( Fig 7 ). By August 2019, the mean tree canopy damage index for American beech trees in the control plots had not changed whereas values for the indices for the treated plots had increased by 46 to 74%. Initial mean tree damage indices were more variable for yellow birch, sugar maple and red maple than for American beech. By August 2019, mean tree canopy damage indices for the trees in the Control plots for these three species showed only minor changes (0 to +18%), whereas values in treated plots had changed by +38 to +133% for yellow birch, -3 to +67% for sugar maple and +50 to +140% for red maple. The largest numerical increases in mean tree damage indices for each species were in the most severe treatments, with mean indices increasing by 1 unit in the High treatment for American beech, 2 units in the High treatment for yellow birch, 1.5 units in the High treatment for sugar maple, and 1.7 units in the Mid×2 treatment for red maple. Overall, the percent change in mean tree canopy damage indices over the four years of the study was numerically greatest for red maple (in Mid×2 treatment), > yellow birch > American beech > sugar maple; the increases in absolute values of mean tree canopy damage index were numerically greater for yellow birch > red maple (in Mid×2 treatment) > sugar maple > American beech.
Due to small sample size, few of the species year × treatment comparisons were statistically significant ( Table 6 ). Of the 24 possible year × treatment pair-wise comparisons prior to icing (May 2015), 18 showed no significant pairwise differences, five showed tree canopy damage indices greater in sugar maple (2), red maple (2) and yellow birch (1) than American beech, and one showed tree canopy damage index in red maple greater than yellow birch. By August 2019, of the 24 possible year × treatment pair-wise comparisons prior to icing, 20 showed no significant pairwise differences, three showed tree canopy damage indices greater in sugar maple (2) and red maple (1) than American beech, and one showed tree canopy damage index in red maple greater than yellow birch.
Our methods and results demonstrate the feasibility of (i) creating a gradient of experimental ice storms and (ii) creating experimental ice storms in consecutive years. The treatment levels were chosen to reflect the amount of ice accretion that triggers National Weather Service ice storm warnings from the Mid-Atlantic to the New England and New York states (6.4 and 12.7 mm radial ice accretion), and then to extend this to a more extreme level (19.1 mm radial ice accretion), and to include an extreme frequency (12.7 mm radial ice accretion in two consecutive years). This initial work establishes the framework to evaluate the response to and recovery from this experimental suite of ice storms for decades to come.
Radial ice accretion was used as a measure of disturbance intensity. Ice accretion is difficult to characterize and quantify as its buildup varies on surfaces of different materials, diameters, shapes and inclinations [ 39 ]. Moreover, measurements in larger storms are dangerous. Pleas for better measurements of ice accretion have been made since the early 1960s [ 40 ]. Here, a passive ice collector was deployed to create a standardized, uniform and easily replicated collection surface for ice accretion measurements. Ice accretion measured with calipers produced similar values to measurements of water volume when estimated accretions were < 10 mm, with few icicles visibly present. However, caliper ice measurements underestimated ice accretion compared to the water volume measurements when estimated accretion was > 10 mm and abundant icicle formation was observed. This result highlights the difficulty of measuring ice loading with calipers when icicles are present. Combining both methods probably provided the best metric of icing intensity on these experimental plots. More work is needed to develop simple, low cost measurement protocols for ice accretion to standardize collection surfaces and accretion angles and determine how to account for icicle formation.
Fine and coarse woody debris inputs
The most immediate ecological impact of ice storms on forests is creation of canopy gaps as twigs, branches, tree tops and eventually entire trees break and snap under the load of the accumulated ice. In a related paper on the canopy impacts of this ice experiment, Fahey et al. [ 28 ] showed significant post-treatment declines in leaf area index of 27 to 37%, increases in gap light index of up to 200%, and shifts in the vertical structure of the canopy by the summer of 2017 compared to pre-treatment values. They found that changes in canopy properties were significantly related to both ice accretion as well as the annual total mass of FWD coupled with fall foliar litterfall.
Results reported here complement the work of Fahey et al. [ 28 ] by quantifying the magnitude and timing of inputs of the FWD and CWD creating the changes in canopy structure following icing. Although these inputs were generally commensurate with treatments (Figs (Figs5 5 and and6), 6 ), it is noteworthy that the mass of FWD was significantly greater in the 2017 Mid×2-yr2 treatment compared to both the 2016 Mid and Mid×2-yr1, despite the same target icing (13 mm). Similarly, the mass of CWD was significantly greater and more decayed in the 2017 Mid×2-yr2 treatment compared to both the 2016 Mid and Mid×2-yr1. We propose five non-exclusive explanations for this result: (i) the first year of mid-level icing did not break or damage all the susceptible twigs and branches in the canopy; (ii) the first year of icing broke branches that remained suspended in the canopy until they fell in response to the second year of icing, (iii) the first year of icing weakened or damaged branches that were then more susceptible to breaking during the second year of icing; (iv) the crown loss following the first year of ice treatment opened up access for greater water penetration/ice buildup on remaining twigs and branches, and reduced internal crown support that otherwise could have prevented the excessive bending and additional breakage and loss of canopy components; and (v) for FWD, the amount of time between icing and litter collection was greater in 2017 (70 days) than 2016 (13 to 37 days), although mean and maximum wind speeds between icing and collections were comparable. In any case, these results demonstrate an interactive effect of ice storm frequency and severity that would not be identified without our experimental approach.
Carbon inputs in FWD and CWD, in general, followed mass inputs, with step-wise increases in input commensurate with icing in 2016 and a pulse of C input in the Mid×2-yr2 treatment plots in 2017. The FWD C inputs following the single events in this ice storm experiment ranged from 18% of the average annual fine litter C inputs at HBEF in the Low treatment to 82% of the average annual inputs in the Mid×2-yr2 treatment ( Table 7 ). The CWD C flux inputs following the single events in this experiment ranged from 16% of the average annual CWD C inputs at HBEF in the Low treatment plots, to approximately equal to the average annual CWD C inputs in the Mid, Midx2-yr1 and Midx2-yr 2 treatment plots, to almost five times greater than the average annual CWD C inputs in the high treatment plots ( Table 7 ). These inputs, especially for the High treatment plots, represent a large transfer of C from the canopy to the soil, and accordingly a large amount of organic debris to be managed by foresters and local municipalities. As woody tissues typically have high C/N ratios and high lignin concentrations [ 41 ] these inputs may take years to decades to decompose.
Values are the mean ± SE.
1 FWD and CWD from Fahey et al. [ 42 ]. CWD is sum of coarse litterfall (20 g C m -2 yr -1 ) and coarse woody debris (120 g C m -2 yr -1 ).
2 1998 ice accretion from Rhoads et al. [ 8 ]; CWD from Fahey et al. [ 42 ]; FWD from Fahey (unpubl.) calculated from dry matter divided by 2.
3 FWD and CWD from Rustad and Campbell (17).
4 ice accretion from volume measurements.
Experimental inputs of FWD C and CWD C can also be compared to inputs incurred at HBEF during the natural ice storm of 1998 ( Table 7 ). This storm severely impacted parts of the south facing watersheds at the HBEF [ 5 , 23 ]. The FWD C inputs in the Low treatment plots of this experiment were roughly similar to those recorded at mid elevations at HBEF (31 vs 29 g C/m 2 , respectively) ( Table 7 ) after the 1998 ice storm, where the amounts of ice accretions were similar (5.7 mm ice for experiment; 5.9 mm ice for natural storm). The FWD C inputs in the Mid and High treatment plots were approximately two to four times the amount of FWD C inputs at high elevations at HBEF after the 1998 ice storm where ice amounts were roughly similar (8.5 to 16.4 mm ice for experiment and 9.4 mm ice for natural storm) ( Table 7 ). The CWD C inputs in the experimental plots with roughly equivalent icing to that of the natural ice storm (Midx2-yr1, Midx2-yr2 and High) ranged from 27% to 155% the CWD C recorded after the natural ice storm ( Table 7 ).
This current experiment was designed after a pilot ice storm experiment conducted at HBEF in 2012 at a similar elevation and forest type to this experiment [ 17 ]. Fine woody debris C inputs for the Mid and Mid×2-yr1 treatments (which had the same target ice accretion as the pilot study (12.7 mm)) were approximately half of the FWD C inputs of the pilot study; FWD C inputs for the Mid×2-yr2 treatments (which has the same target ice accretion as the pilot study but was applied in a second consecutive year) were approximately equal to the FWD C inputs of the pilot study ( Table 7 ). Coarse woody debris C inputs for the Mid and Mid×2-yr1 treatments (which had the same target ice accretion as the pilot study (12.7 mm)) were 64 and 53 percent of the CWD C inputs of the pilot study, respectively; CWD C inputs for the Mid×2-yr2 treatments (which had the same target ice accretion as the pilot study but was applied in a second consecutive year) were 84 percent of the pilot study ( Table 7 ).
Differences in FWD C and CWD C inputs among the several experimental and the natural icing events at HBEF reflect the many factors that interact to influence forest response to ice storms. These include natural variations in species composition, stand age, tree and stand health, soils, and antecedent or postcedent event weather conditions, as well as experimental artifacts such as the more rapid icing (~4 hours) during the experiments versus the natural event (~3 days).
In addition to C inputs, fine and coarse woody debris play an important role in recycling nutrients in northern forest ecosystems. Nitrogen, P and Ca can all be limiting in these forests [ 43 ]. Additions of these elements in woody materials replenish forest floor nutrients and, as they are slowly mineralized over time, stimulate future growth. Magnesium and K are also critical for tree health and to repair wounds following injury or insect infestation [ 44 ]. The simulated ice storms in this study resulted in significant inputs of N, P, Ca, Mg and K to these forest plots. The nutrient inputs reported here for the combined CWD and FWD measured in the Control (2016 and 2017) and Low treatment plots in 2016 were less than or equal to the amounts reported by Gosz et al. [ 45 ]for annual litter inputs in perennial tissues for a mixed hardwood site at a comparable elevation at Hubbard Brook ( Table 8 ). The nutrient inputs reported here for the Mid, Mid×2-yr1 and High treatment plots in 2016 were 137 to1048% higher than those reported by Gosz et al. (1972); and were 318 to 617% higher for the Mid×2_yr2 treatment plots in 2017. Thus the nutrient transfers in these single simulated storm events were equivalent to up to 10 years of average annual nutrient inputs at this site.
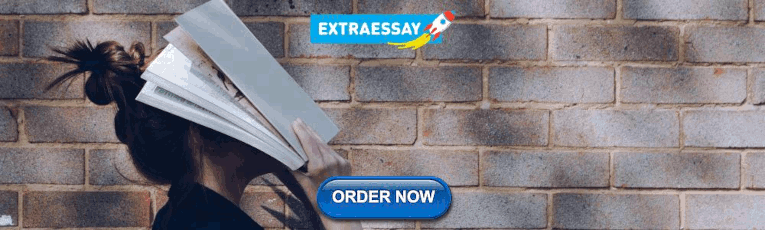
Tree canopy damage
The results from the tree canopy damage assessments demonstrated that tree response to ice-induced crown injury unfolds over a period of years. For all species combined, the indices of canopy damage increased over time, with significant difference between Mid and High treatment plots vs Control and Low treatment plots only emerging after two to three years following icing ( Fig 7 ). Similar trends were evident for the four individual deciduous species which had representation in all the plots. It is still early in the recovery period, and despite the high levels of icing and the increase in tree canopy damage indices, few trees were characterized as class 4 (> 75% damage). Continued monitoring of these trees will be needed to reveal the full extent of the damage over time. These results are consistent with the evolution of disease and mortality following ice storms as observed by Shortle et al. [ 21 ] and Deschenes et al. [ 46 ]. Delayed, lagged and/or persistent declines in tree health following ice storm-induced crown damage may be due to a combination of C starvation (due to loss of canopy foliage and increased C requirements for wound closure and defense) and secondary attacks by insects, pests and pathogens [ 21 , 47 ].
Four deciduous species were present in all the treatments and provided limited evidence for species-specific differences in response to icing. For example, red maple and yellow birch trees showed the greatest change in tree canopy damage indices over the four years of the study. In contrast, American beech had the lowest initial tree canopy damage indices for all the species, and showed the smallest change in this index in response to the repeated ice applications. It is notable that these responses are for the overstory American beech which were relatively healthy, with little sign of beech bark disease, a major disease that impacts the health of American beech across its range [ 48 ]. Observationally, understory American beech trees were considerably bent over by the weight of the ice, and stayed bowed over for the duration of the study, contributing to the canopy affects described by Fahey et al. [ 49 ]. The sugar maple in these stands had the highest initial tree canopy damage indices for all the species, and exhibited only moderate increases in response to the treatments. These observations can be contrasted to those reported by Shortle et al. [ 21 ]. In their study of 60–120 year old forest stands across New England impacted by the 1998 ice storm, American beech sustained the most damage, followed by yellow birch and then sugar maple. This discrepancy in the susceptibility of American beech to icing between the two studies may be due to the relative lack of a pre-existing stressor such as beech bark disease, the shorter amount of time between the icing and the sampling (as impacts evolve over time), and the small sample size in this study compared to the more comprehensive review including a greater range of sites impacted by pre-existing conditions, as presented by Shortle et al. [ 21 ].
Despite these patterns over time, few significant pair-wise species differences in tree canopy damage emerged among the four deciduous species. This may be due, in part, to the small sample size for most species, or to the fact that not all species were present in all treatments, which precluded additional species in the pairwise comparisons. Notably, sample sizes for the two coniferous species present in the plots—red spruce and balsam fir—were not robust enough for pair-wise comparisons. Coniferous species are known to be more resilient to ice storms than deciduous trees [ 50 ]. In order to answer the driving question of which species or age classes of species are more or less susceptible to ice storm damage, more synoptic studies of tree damage, likely following natural icing events, are needed.
Application of an experimental gradient approach to ice storm damage allowed for the identification of several thresholds for this forest stand. Light icing (i.e., target 6.4 mm ice) caused little twig or branch breakage. Although this amount of icing causes slippery conditions on roads and walkways, it had little impact on FWD and CWD in this forest, as reflected in the relatively low amounts of FWD and CWD in the Low treatment plots, which were not significantly different than the Control plots (Figs (Figs5 5 and and6). 6 ). The first thresholds in this forest was observed between 6.4- and 12.7-mm target ice accretions, when noticeable amounts of twigs and small branches were observed to break and fall to the ground. This was reflected in the significantly greater accumulations of FWD in the Mid and Mid×2-yr1 treatment plots compared to the Control plots in 2016, and significantly greater accumulation of FWD in the Mid×2-yr2 treatment plots compared to all other plots in 2017. It also contributed to the small increase in CWD in the Mid and Mid×2-yr1 treatment plots compared to the Control and Low treatment plots in 2016, and to the significant increase in CWD in the Mid×2-yr2 treatment plots compared to all other plots in 2017. A second threshold was observed between 12.7- and 19.1-mm target ice accretion, where whole tree tops bent under the weight of accumulated ice and were beginning to snap at the stem. This observation was reflected in the significant pulse of CWD in the High treatment plots compared to all other treatment plots in 2016. Similar tipping points were reported by Lemon [ 40 ] in a synthesis of observations from a suite of ice storms affecting forests in New York State, USA. Note that our experiment was conducted under calm conditions (maximum wind speed 1.8 m/s). Wind accentuates forest canopy ice damage, and the tipping points observed here could occur at lower ice accretions under higher wind conditions.
Hydrologic and chemical artifacts of treatments
In conducting field-scale experiments, potential methodological artifacts that may confound results must be considered. For icing experiments, an artifact can be collateral impacts on forest hydrologic and chemical budgets from the water used to generate ice treatments. Here, the water source for icing was the nearby Hubbard Brook. The chemistry of this stream, studied for over 50 years [ 51 ], is characterized as dilute [ 52 ] and thus was a good candidate as a water source. Results showed that the amount of water applied to experimental plots was within the range of a large precipitation event at Hubbard Brook (i.e., 5–16% of mean annual precipitation), and similar in magnitude to the water input in the natural ice storm of 1998, with water input to the Low treatment plots being less than water inputs in the natural storm (74%) and water inputs in the High treatment plots being more (254%) ( Table 3 ). Measured throughfall volume was lower than precipitation and ranged from 35 to 140% of the water input measured for the 1998 ice storm.
Chemical inputs in the treatment were measurable but well below commercial forest fertilization levels [ 53 , 54 ]. Here, total N inputs (NO 3 - + NH 4 + + DON) for the High treatment represented 7% of the average annual precipitation inputs for the site; total base cations input ranged from 63% (K+) to 300% (Ca 2+ ) and 317% (Mg + ) of the average annual precipitation inputs ( Table 3 ). All nutrient inputs were considerably higher than those in the naturally occurring 1998 ice storm ( Table 3 ), representing a possible experimental artifact that needs to be accounted for in future interpretations of biologic and chemical responses to ice storm treatments.
Conclusions
Currently, a knowledge gap exists on the short- to long-term ecological consequences of extreme weather disturbances. These types of events are problematic to study because they are heterogeneous in time and space, difficult to predict, and disrupt scientific and social infrastructure when they occur, making access to experimental instruments and research sites challenging. Extreme event experiments can help fill this gap, but they can be difficult and even risky to undertake.
Initial results from this study provide quantitative measurements of FWD and CWD mass and nutrient transfers from forest canopies to soil in response to different intensities and frequencies of canopy ice accretion. These metrics of response can help foresters, emergency managers, town planners, electric power utilities, arborists, winter recreationists and others be better prepared for the aftermath of ice storms. In particular, results suggest that mature northern hardwood forests such as the one studied here will sustain little damage below 6.4 mm radial ice accretion, moderate damage with up to 12.7 mm of accretion, and significant damage to crowns with upwards of 19.1 mm of ice or more. Further, current ice storm warnings that are issued by the National Weather Service in New York and New England for ≥12.7 mm radial ice accretion may be too high because significant FWD and some CWD will likely have already fallen at that level of icing and damage will have been done. The 6.4 mm ice storm warnings as issued for the Mid-Atlantic states may provide a safer level to alert the public to pending tree damage from ice storms. Results also suggest that first responders need to be prepared for an equal or greater amount of organic debris inputs if large storms (here ≥12.7 mm radial ice accretion) occur in back-to-back years. Our results suggest that damaged trees, branches or whole trees that do not fall in the first year are more susceptible to further damage in an ensuing year.
This experiment was the first of its kind in creating a suite of experimental ice storms of different intensities and frequencies in a forest ecosystem. Recommendations for future work include: (i) increase intensity of icing to simulate a tipping point that marks a state change characterized by long-lasting alterations of stand composition or structure; (ii) increase frequency of icing within a single year and over a greater number of consecutive years to better understand how resilient trees are to repeat ice storms and identify tipping points characterized by a state change; (iii) conduct a similar gradient of ice storms in different vegetation types and age classes to better understand what vegetation types and age classes are more or less susceptible to ice damage; (iv) conduct the experiment under different wind speeds, as greater damage is likely with higher wind velocities; and (v) increase plot size to encompass the complete canopies and root systems of a larger number of trees to minimize belowground interactions from adjacent non-iced trees. More work is also recommended to develop simple, low cost measurement protocols for ice accretion, including a refined design for the passive collector that optimizes collector surfaces, angles, and approaches to minimize icicle formation (Campbell et al. in review). Such collectors would allow researchers and citizen scientists to gather more standardized information on the intensity and frequency of icing events across the landscape.
The most interesting results from this experiment are likely those that will evolve over time as this forest ecosystem reorganizes and recovers from this suite of experimental ice storms. This study provides an experimental framework to study the short- and long-term impacts of these experimental ice storms on the structure and function of this northern hardwood forest now [ 28 , 55 , 56 ] and in the decades to come.
Supporting information
S1 photo license form, acknowledgments.
We thank the dozens of Hubbard Brook ‘ISE’ women and men who helped with the ice applications and associated field work. This experiment would not have been possible without these bold extreme weather enthusiasts. This manuscript is a contribution of the Hubbard Brook Ecosystem Study. Hubbard Brook is part of the Long-Term Ecological Research (LTER) network, which is supported by the National Science Foundation (DEB-1633026). The Hubbard Brook Experimental Forest is operated and maintained by the USDA Forest Service, Northern Research Station, Madison, WI.
Disclaimer of non-endorsement: Reference herein to any specific commercial products, process, or service by trade name, trademark, manufacturer, or otherwise, does not necessarily constitute or imply its endorsement, recommendation, or favoring by the United States Government. The views and opinions of authors expressed herein do not necessarily state or reflect those of the United States Government, and shall not be used for advertising or product endorsement purposes.
Funding Statement
This work was funded by an award from the National Science Foundation Directorate for Biological Sciences with the number 1457675 (NSF DEB 1457675) to CD, LR, JC, TF, PG, AND PS. This award provided support in the form of salaries for authors GS, GW, WL, and BL. The funder did not have any additional role in the study design, data collection and analysis, decision to publish, or preparation of the manuscript. The specific roles of these authors are articulated in the ‘author contributions’ section. The funder also provided support in the form of a contract to author FB. FB is an engineer and sole proprietor of Research Designs, and he provided specialized design services for this experiment. The funder had no role in the study design, data collection and analysis, decision to publish, or preparation of the manuscript relative to this contract. The specific role of FB is articulated in the ‘author contributions’ section.
Data Availability
Thank you for visiting nature.com. You are using a browser version with limited support for CSS. To obtain the best experience, we recommend you use a more up to date browser (or turn off compatibility mode in Internet Explorer). In the meantime, to ensure continued support, we are displaying the site without styles and JavaScript.
- View all journals
- My Account Login
- Explore content
- About the journal
- Publish with us
- Sign up for alerts
- Open access
- Published: 11 February 2021
Conservation machine learning: a case study of random forests
- Moshe Sipper 1 , 2 &
- Jason H. Moore 1
Scientific Reports volume 11 , Article number: 3629 ( 2021 ) Cite this article
5265 Accesses
19 Citations
17 Altmetric
Metrics details
- Computational science
- Computer science
- Scientific data
Conservation machine learning conserves models across runs, users, and experiments—and puts them to good use. We have previously shown the merit of this idea through a small-scale preliminary experiment, involving a single dataset source, 10 datasets, and a single so-called cultivation method—used to produce the final ensemble. In this paper, focusing on classification tasks, we perform extensive experimentation with conservation random forests, involving 5 cultivation methods (including a novel one introduced herein— lexigarden ), 6 dataset sources, and 31 datasets. We show that significant improvement can be attained by making use of models we are already in possession of anyway, and envisage the possibility of repositories of models (not merely datasets, solutions, or code), which could be made available to everyone, thus having conservation live up to its name, furthering the cause of data and computational science.
Similar content being viewed by others
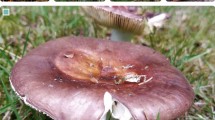
Mushroom data creation, curation, and simulation to support classification tasks
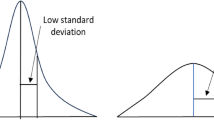
Dataset meta-level and statistical features affect machine learning performance
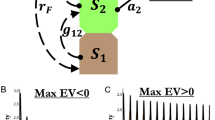
Interpreting random forest analysis of ecological models to move from prediction to explanation
Introduction.
We recently presented the idea of conservation machine learning , wherein machine learning (ML) models are saved across multiple runs, users, and experiments 1 . Conservation ML is essentially an “add-on” meta-algorithm, which can be applied to any collection of models (or even sub-models), however they were obtained: via ensemble or non-ensemble methods, collected over multiple runs, gathered from different modelers, a priori intended to be used in conjunction with others—or simply plucked a posteriori, and so forth.
A random forest (RF) is an oft-used ensemble technique that employs a forest of decision-tree classifiers on various sub-samples of the dataset, with random subsets of the features for node splits. It uses majority voting (for classification problems) or averaging (for regression problems) to improve predictive accuracy and control over-fitting 2 .
Reference 3 presented a method for constructing ensembles from libraries of thousands of models. They used a simple hill-climbing procedure to build the final ensemble, and successfully tested their method on 7 problems. They further examined three alternatives to their selection procedure to reduce overfitting. Pooling algorithms, such as stacked generalization 4 , and super learner 5 , have also proven successful. There also exists a body of knowledge regarding ensemble pruning 6 .
We believe the novelty of conservation machine learning, herein applied to random forests, is two-fold. First and foremost, we envisage the possibility of vast repositories of models (not merely datasets, solutions, or code). Consider the common case wherein several research groups have been tackling an extremely hard problem (e.g., 7 ), each group running variegated ML algorithms over several months (maybe years). It would not be hard to imagine that the number of models produced over time would run into the millions (quite easily more). Most of these models would be discarded unflinchingly, with only a minute handful retained, and possibly reported upon in the literature. We advocate making all models available to everyone, thus having conservation live up to its name, furthering the cause of data and computational science. Our second contribution in this paper is the introduction of a new ensemble cultivation method— lexigarden .
In 1 we offered a discussion and a preliminary proof-of-concept of conservation ML, involving a single dataset source, 10 datasets, and a single so-called cultivation method. Herein, focusing on classification tasks, we perform extensive experimentation involving 5 cultivation methods, including the newly introduced lexigarden (“ Ensemble cultivation ”), 6 dataset sources, and 31 datasets (“ Datasets ”). Upon describing the setup (“ Experimental setup ”), we show promising results (“ Results ”), followed by a discussion (“ Discussion ”) and concluding remarks (“ Concluding remarks ”).
Ensemble cultivation
Conservation ML begins with amassing a collection of models—through whatever means. Herein, we will collect models saved over multiple runs of RF training.
Once in possession of a collection of fitted models it is time to produce a final ensemble. We examine five ways of doing so:
Jungle. Use all collected fitted models to form class predictions through majority voting, where each model votes for a single class.
Super-ensemble. Use an ensemble of ensembles, namely an ensemble of RFs, with prediction done through majority voting, where each RF votes for a single class.
To clarify, assume we perform 100 runs of RFs of size 100. We are then in possession of a jungle of size 10,000 decision trees, and a super-ensemble of size 100 RFs. Both perform predictions through majority voting.
Order-based (or ranking-based) pruning. We implemented two methods of ensemble pruning 6 . The first, ranking-based, sorts the jungle from best model (over training set) to worst, and then selects the top n models for the ensemble, with n being a specified parameter
Clustering-based pruning. The second ensemble-pruning method performs k-means clustering over all model output vectors, with a given number of clusters, k , and then produces an ensemble by collecting the top-scoring (over training set) model of each cluster.
Lexigarden . A new method, introduce herein and described below, based on lexicase selection, a performant selection technique for evolutionary algorithms, with selection being one of the primary steps in such algorithms 8 , 9 . This step mimics the role of natural selection in nature, by probabilistically selecting fitter individuals from the evolving population, which will then undergo pseudo-genetic modification operators.
Lexicase selection selects individuals by filtering a pool of individuals which, before filtering, typically contains the entire population. The filtering is accomplished in steps, each of which filters according to performance on a single test case. Lexicase selection has been used productively within the field of evolutionary algorithms 10 , 11 . Herein, we co-opt it to cultivate a “garden” of select trees from the jungle, introducing the lexigarden algorithm. Lexigarden generates a garden of a specified size, whose models were selected through lexicase selection.
Algorithm 1 provides the pseudocode. Lexigarden receives a jungle of models, a dataset along with target values, and the number of models the generated garden is to contain. The lexicase function begins by randomly shuffling the dataset, after which it successively iterates through it, retaining only the models that provide a correct answer for each instance. In the end we are left with either a single model or a small number of models, which are precisely those that have correctly classified the subset of the dataset engendered through the looping process. The lexicase function is called as many times as needed to fill the garden with models. Lexigarden ends up generating a highly diverse subset of all the models by picking ones that each excels on a particular random subset of instances.
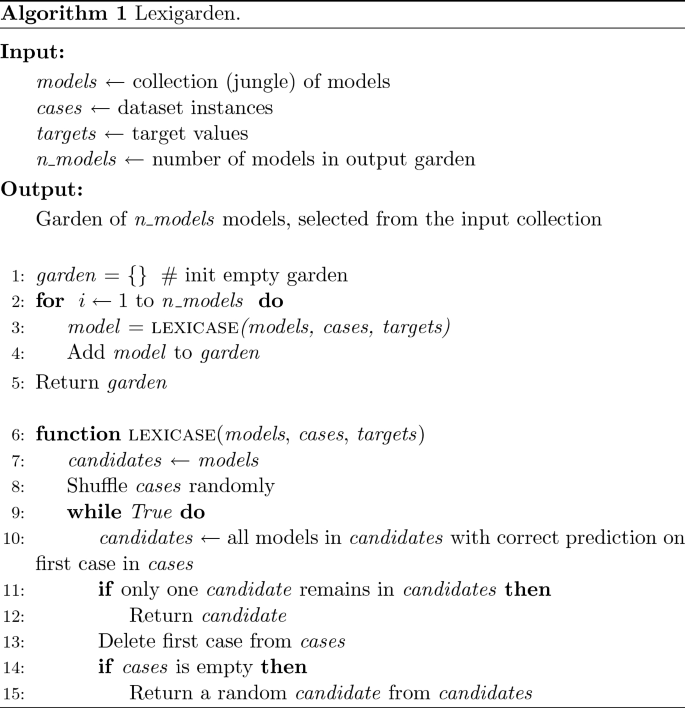
To compose a variegated collection of classification datasets for the experiments described in the next section we turned to 6 different sources:
Easy . Scikit-learn’s 12 “easy” classification datasets, where near-perfect performance is expected as par for the course: iris, wine, cancer, digits.
Clf . Datasets produced through make_classification , a Scikit-learn function that, “initially creates clusters of points normally distributed (std=1) about vertices of an n_informative -dimensional hypercube with sides of length 2*class_sep and assigns an equal number of clusters to each class.” 13
HIBACHI . A method and software for simulating complex biological and biomedical data 14 .
GAMETES . Software that generates complex biallelic single nucleotide polymorphism (SNP) disease models for simulation studies. We generated a 2-way and a 3-way biallelic pure, strict, epistatic model with a heritability of 1, referred to in related work as the Xor model 15 .
OpenML . A repository of over 21,000 datasets, of which we selected problems designated as “Highest Impact”, with a mix of number of samples, features, and classes 16 .
PMLB . The Penn Machine Learning Benchmark repository is an accessible, curated, and developing public benchmark resource to facilitate identification of the strengths and weaknesses of different machine learning methodologies 17 .
Note that in addition to there being 6 dataset sources, there is also a mix of dataset repositories (Easy, OpenML, PMLB) and dataset generators (Clf, HIBACHI, GAMETES). Figure 1 shows a “bird’s-eye view” of the total of 31 datasets.
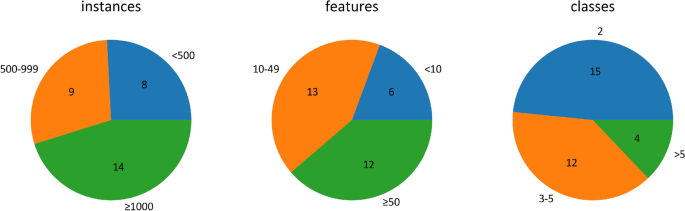
A “bird’s-eye view” of the 31 datasets used in this study: number of instances (left), number of features (center), and number of classes (right).
Experimental setup
The experiments were based on Scikit-learn’s RandomForestClassifier function, which we used with its default values (our aim here was not to improve RFs per se but to show that conservation can significantly improve a base ML algorithm) 12 , 13 .
The experimental setup is shown in Algorithm 2. For each replicate experiment we created 5 folds, for 5-fold cross validation. For each fold the dataset was split into a training set of 4 folds and the left-out test fold. 100 runs were conducted per fold. Each run consisted of fitting a 100-tree RF to the traning set and testing the fitted RF on the test set. In addition, all trees were saved into a jungle and all RFs were saved into a super-ensemble; these could then be tested on the test set.
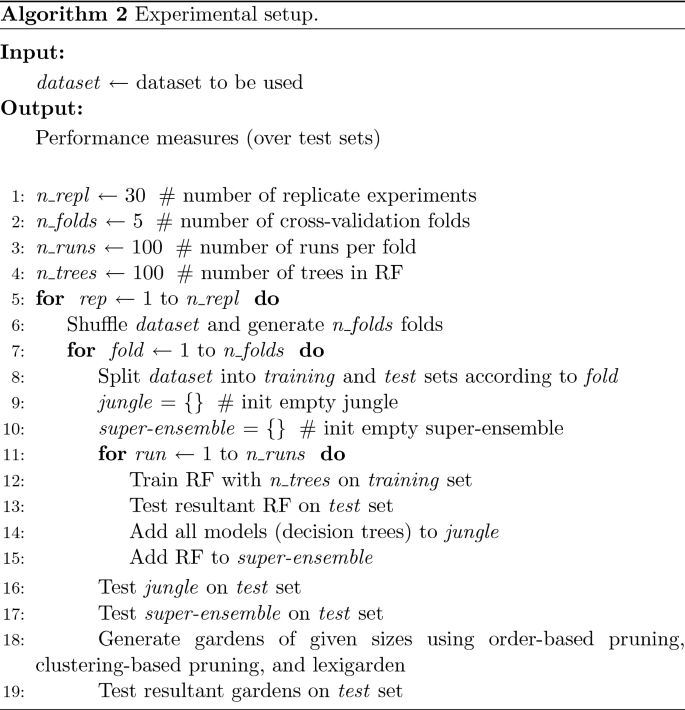
The jungle also served as fodder for the cultivation methods of “ Ensemble cultivation ”. For every training epoch, we generated gardens of given sizes (see Table 1 ) using the three cultivation methods: order-based pruning, clustering-based pruning, and lexigarden. These gardens could then also be tested on the test set.
Table 1 shows the results of our experiments. Each line in the table presents the results for a single dataset, involving 30 replicate experiments, as delineated in “ Experimental setup ”. We show the mean performance of RFs alongside the improvement of the 5 conservation methods discussed in “ Ensemble cultivation ”.
In addition to reporting improvement values we also report their statistical significance, as assessed by 10,000-round permutation tests. The permutation tests focused on the mean test scores across all replicates and folds, comparing each ensemble (mean) to the original RFs. We report on two p-value significance levels: \(<0.001\) and \(<0.05\) .
Focusing on random forests for classification we performed a study of the newly introduced idea of conservation machine learning. It is interesting to note that—case in point—our experiments herein alone produced almost fifty million models (31 datasets \(\times \) 30 replicates \(\times \) 5 folds \(\times \) 100 runs \(\times \) 100 decision trees = 46,500,000).
As can be seen in Table 1 , we attained statistically significant improvement for all datasets, except for the four “easy” problems, where a standard RF’s accuracy was close to 1 to begin with, and little improvment could be eked out. All but the super-ensemble method ranked highest on several datasets: the jungle method attained the best improvement for 8 datasets, order-based pruning attained the best improvement for 10 datasets, clustering-based pruning attained the best improvement for 2 datasets, and lexigarden attained the best improvement for 8 datasets (we excluded “easy” datasets from this count).
In summary, our results show that conservation random forests are able to improve performance through ensemble cultivation by making use of models we are already in possession of anyway.
There is a cost attached to conserving models, involving memory and computation time. The former is probably less significant, since saving millions and even billions of models requires storage space well within our reach. Computation time of a jungle or super-ensemble is obviously more costly than an ensemble of a lesser size (or a single model), but if the performance benefits are deemed worthwhile then time should not pose an issue. Computing a garden’s output involves only a minor increase in computation time, and a one-time computation of the garden’s members. As pointed out recently by 18 , improvements in software, algorithms, and hardware architecture can bring a much-needed boost. For example, they showed that a sample Python program, when coded in Java, produced a 10.8 \(\times \) speedup, and coding it in C produced a 47 \(\times \) speedup; moreover, tailoring the code to exploit specific features of the hardware gained an additional 1300 \(\times \) speedup.
Concluding remarks
There are many possible avenues for future exploration:
We focused on classification tasks, which leaves open the exploration of other types of tasks, such as regression and clustering.
We examined random forests, with other forms of ensemble techniques—such as sequential, boosting algorithms—yet to be explored.
Non-ensemble techniques deserve study as well.
It is also possible to amass models that were obtained through different ML methods (or even non-ML methods).
We used simple majority voting to compute the output of jungles, super-ensembles, and gardens. More sophisticated methods could be explored.
We offered lexigarden as a novel supplement to the cultivation-method toolkit. Other cultivation techniques could be devised.
As noted in 1 , current cloud repositories usually store code, datasets, and leaderboards. One might consider a new kind of model archive, storing a plethora of models, which could provide bountiful grist for the ML mill.
While we focused on random forests herein, we note again that conservation ML is essentially an “add-on” meta-algorithm that can be applied to any collection of models, however they were obtained.
We hope to see this idea receiving attention in the future.
Code availability
The code is available at https://github.com/EpistasisLab .
Sipper, M., & Moore, J.H. Conservation machine learning. BioData Min. 13 (9) (2020).
Ho, T.K. Random decision forests. In Proceedings of 3rd International Conference on Document Analysis and Recognition , vol. 1, pp. 278–282. IEEE (1995).
Caruana, R., Niculescu-Mizil, A., Crew, G., & Ksikes, A. Ensemble selection from libraries of models. In In Proceedings of the 21st International Conference on Machine Learning , pp. 137–144. ACM Press (2004).
David, H. Stacked generalization. Neural Netw. 5 (2), 241–259 (1992).
Article Google Scholar
Van der Laan, M. J., Polley, E. C. & Hubbard, A. E. Super learner. Stat. Appl. Genet. Mol. Biol. 6 (1) (2007).
Tsoumakas, G., Partalas, I., & Vlahavas, I. An ensemble pruning primer. In Applications of Supervised and Unsupervised Ensemble Methods (eds Okun, O. & Valentini, G.) 1–13 (Springer, Berlin, Heidelberg, 2009).
Bycroft, C. et al. The UK biobank resource with deep phenotyping and genomic data. Nature 562 (7726), 203–209 (2018).
Article ADS CAS Google Scholar
Metevier, B., Saini, A. K., & Spector, L. Lexicase selection beyond genetic programming. In Banzhaf, W., Spector, L., & Sheneman, L., editors, Genetic Programming Theory and Practice XVI , pp. 123–136. Springer (2019).
Spector L. Assessment of problem modality by differential performance of lexicase selection in genetic programming: A preliminary report. In Proceedings of the 14th Annual Conference Companion on Genetic and Evolutionary Computation , pp. 401–408. ACM (2012).
Helmuth, T., Spector, L. & Matheson, J. Solving uncompromising problems with lexicase selection. IEEE Trans. Evol. Comput. 19 (5), 630–643 (2014).
Helmuth, T., McPhee, N. F., & Spector, L. Lexicase selection for program synthesis: A diversity analysis. In Riolo, R., Worzel, W.P., Kotanchek, M., & Kordon, A., editors, Genetic Programming Theory and Practice XIII , pp. 151–167, Cham. Springer International Publishing (2016).
Pedregosa, F. et al. Scikit-learn: machine learning in python. J. Mach. Learn. Res. 12 , 2825–2830 (2011).
MathSciNet MATH Google Scholar
Scikit-learn: Machine learning in Python. https://scikit-learn.org/ . Accessed: 2020-06-09 (2020).
Moore, J. H., Shestov, M., Schmitt, P., & Olson, R. S. A heuristic method for simulating open-data of arbitrary complexity that can be used to compare and evaluate machine learning methods. In Pacific Symposium on Biocomputing , volume 23, pp. 259–267. World Scientific (2018).
Urbanowicz, R. J. et al. GAMETES: a fast, direct algorithm for generating pure, strict, epistatic models with random architectures. BioData Min. 5 (1), 16 (2012).
Vanschoren, J., van Rijn, J. N., Bischl, B. & Torgo, L. OpenML: Networked science in machine learning. SIGKDD Explor. 15 (2), 49–60 (2013).
Olson, R. S., La Cava, W., Orzechowski, P., Urbanowicz, R. J. & Moore, J. H. PMLB: a large benchmark suite for machine learning evaluation and comparison. BioData Min. 10 (1), 36 (2017).
Leiserson, C.E. et al. There’s plenty of room at the top: What will drive computer performance after moore’s law?. Science 368 (6495) (2020).
Download references
Acknowledgements
We thank Patryk Orzechowski for his help with the HIBACHI datasets, and Ryan Urbanowicz for helping out with the GAMETES datasets. This work was supported by National Institutes of Health (USA) grants LM010098, LM012601, AI116794.
Author information
Authors and affiliations.
Institute for Biomedical Informatics, University of Pennsylvania, Philadelphia, PA, 19104-6021, USA
Moshe Sipper & Jason H. Moore
Department of Computer Science, Ben-Gurion University, Beer Sheva, 84105, Israel
Moshe Sipper
You can also search for this author in PubMed Google Scholar
Contributions
M.S. wrote conceived the basic idea, ran the experiments, and wrote up the paper draft J.H.M. provided statistical analysis and paper improvements All authors reviewed the manuscript.
Corresponding author
Correspondence to Moshe Sipper .
Ethics declarations
Competing interests.
The authors declare no competing interests.
Additional information
Publisher's note.
Springer Nature remains neutral with regard to jurisdictional claims in published maps and institutional affiliations.
Rights and permissions
Open Access This article is licensed under a Creative Commons Attribution 4.0 International License, which permits use, sharing, adaptation, distribution and reproduction in any medium or format, as long as you give appropriate credit to the original author(s) and the source, provide a link to the Creative Commons licence, and indicate if changes were made. The images or other third party material in this article are included in the article's Creative Commons licence, unless indicated otherwise in a credit line to the material. If material is not included in the article's Creative Commons licence and your intended use is not permitted by statutory regulation or exceeds the permitted use, you will need to obtain permission directly from the copyright holder. To view a copy of this licence, visit http://creativecommons.org/licenses/by/4.0/ .
Reprints and permissions
About this article
Cite this article.
Sipper, M., Moore, J.H. Conservation machine learning: a case study of random forests. Sci Rep 11 , 3629 (2021). https://doi.org/10.1038/s41598-021-83247-4
Download citation
Received : 23 August 2020
Accepted : 01 February 2021
Published : 11 February 2021
DOI : https://doi.org/10.1038/s41598-021-83247-4
Share this article
Anyone you share the following link with will be able to read this content:
Sorry, a shareable link is not currently available for this article.
Provided by the Springer Nature SharedIt content-sharing initiative
This article is cited by
Identification of colorectal cancer progression-associated intestinal microbiome and predictive signature construction.
- Jungang Liu
- Xiaoliang Huang
- Weizhong Tang
Journal of Translational Medicine (2023)
Enhancing manufacturing process by predicting component failures using machine learning
- Raihanus Saadat
- Sharifah Mashita Syed-Mohamad
- Pantea Keikhosrokiani
Neural Computing and Applications (2022)
By submitting a comment you agree to abide by our Terms and Community Guidelines . If you find something abusive or that does not comply with our terms or guidelines please flag it as inappropriate.
Quick links
- Explore articles by subject
- Guide to authors
- Editorial policies
Sign up for the Nature Briefing: AI and Robotics newsletter — what matters in AI and robotics research, free to your inbox weekly.

- 0 Shopping Cart
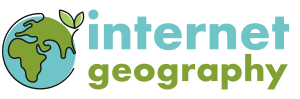
Epping Forest Case Study
A small scale ecosystem
Epping forest is an ancient, deciduous woodland to the northeast of London. It is all that remains of a more extensive forest that colonised England at the end of the last ice age . The forest is approximately 19 kilometres (12 miles) long from north to south but no more than 4 kilometres (2.5 mi) from east to west at its widest point.
The forest, managed for over 1000 years, has been used for hunting and timber resources. Recreation is the leading role of the forest now.
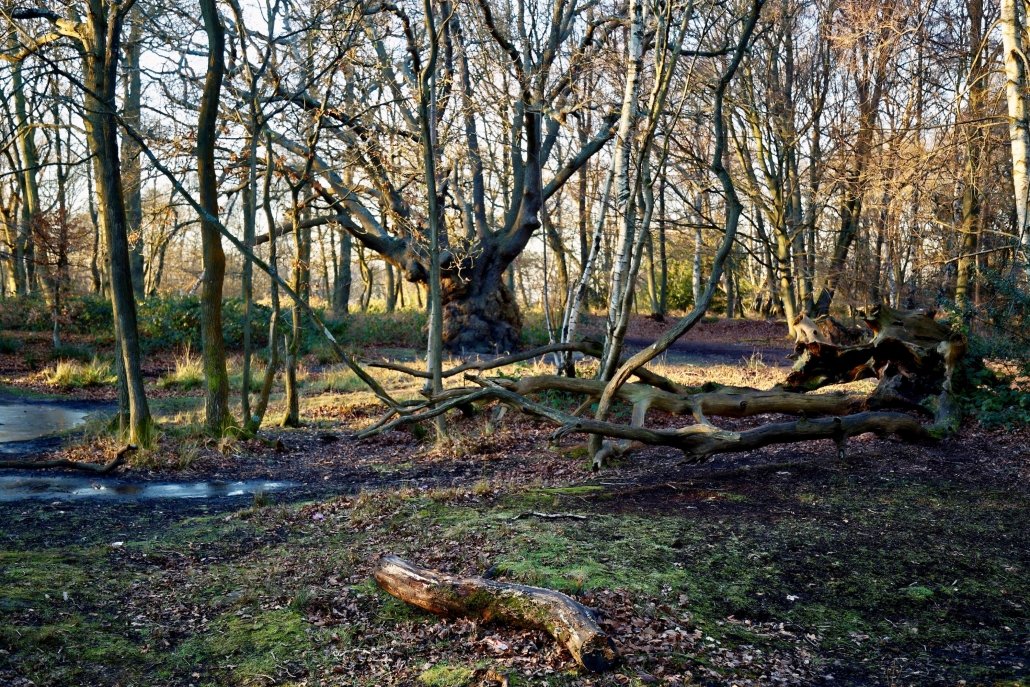
Epping Forest
The forest is designated as a Site of Special Scientific Interest (SSSI), meaning it is a protected environment.
Due to its careful management, Epping Forest has a complex food web composed of thousands of species. The forest has:
- a wide variety of native tree species that include beech, elm, oak and ash;
- a shrub layer consisting of hazel and holly, along with grasses, brambles, fern, bracken and flowering plants;
- 177 species of lichen and moss
- many primary consumers, including insects and small mammals, and deer, along with 38 species of birds
- secondary consumers such as owls, adders and foxes;
- 700 species of fungi, important decomposers, which are common due to a large amount of deadwood;
- over 100 lakes and ponds provide essential habitats for numerous fauna species (animals) and flora (plants).
Epping Forest food web
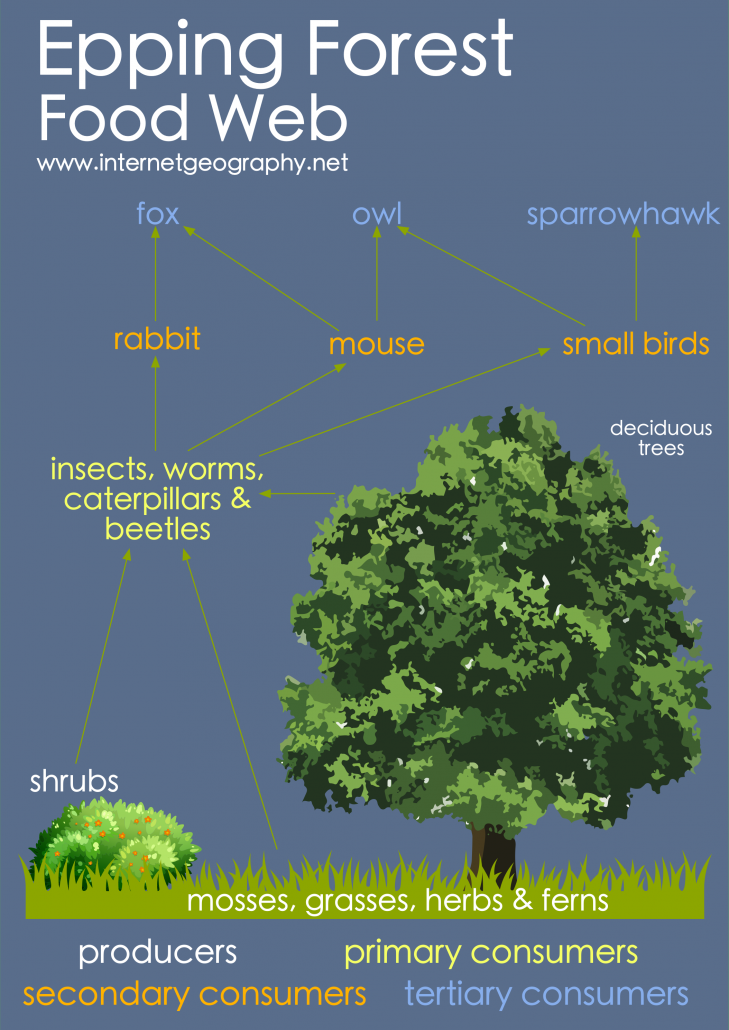
Epping Forest Food Web
How is the Epping Forest ecosystem interdependent?
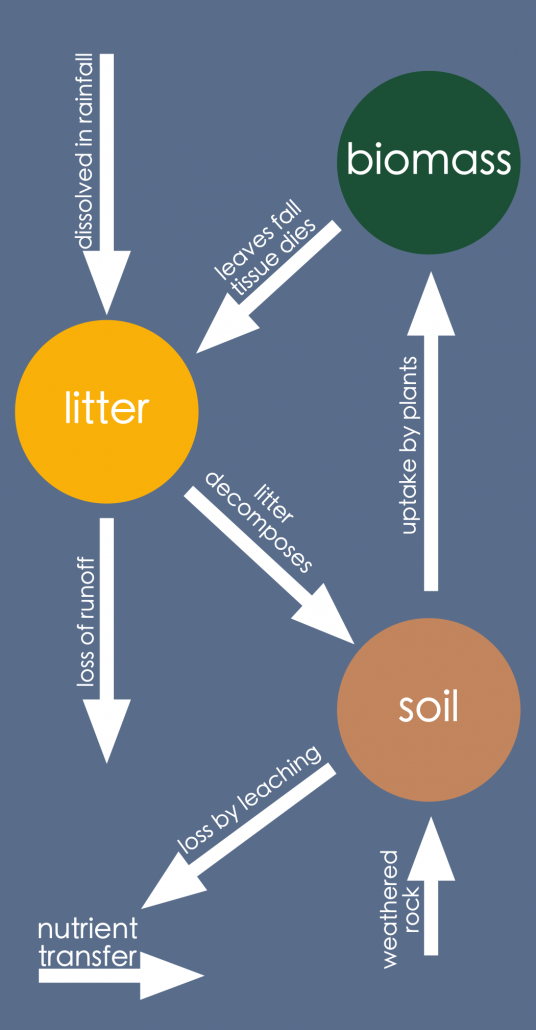
Epping Forest Nutrient Cycle
Sustainable Management of Epping Forest
Recreation is controlled within the forest, including having designated car parking areas, a visitor centre, provision of rubbish bins and leaflets on how to use the forest to protect the environment. Paths for walking and riding are open to public use. There are currently nine Forest Keepers, three Senior Forest Keepers, and one Head Keeper employed to protect the forest environment. These strategies are sustainable because they protect the forest for future generations.
Along the roads, vegetation is cut back so that deer are aware of traffic and reduce the risk of motor vehicle collisions. Maintaining the foliage protects the native deer population from road traffic accidents. This is a form of sustainable management as it helps protect current and future deer populations.
Cattle have been reintroduced in some areas as grazing supports the growth of some flora.
Epping forest conservation volunteers undertake tasks every Sunday to support the biodiversity of the forest. Duties include cutting back vegetation, rubbish and scrub clearance, removing silt and maintaining ponds and bogs.
Ecosystems on a larger, global scale are known as biomes. Find out more here .
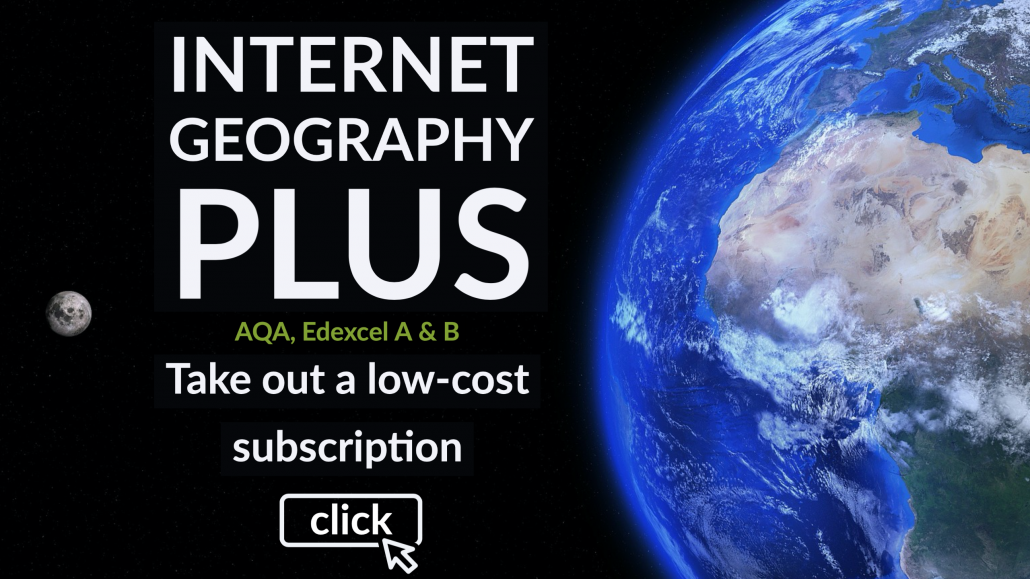
Premium Resources
Please support internet geography.
If you've found the resources on this page useful please consider making a secure donation via PayPal to support the development of the site. The site is self-funded and your support is really appreciated.
Related Topics
Use the images below to explore related GeoTopics.
UK Ecosystems
Topic home, what is a tropical rainforest, share this:.
- Click to share on Twitter (Opens in new window)
- Click to share on Facebook (Opens in new window)
- Click to share on Pinterest (Opens in new window)
- Click to email a link to a friend (Opens in new window)
- Click to share on WhatsApp (Opens in new window)
- Click to print (Opens in new window)
If you've found the resources on this site useful please consider making a secure donation via PayPal to support the development of the site. The site is self-funded and your support is really appreciated.
Search Internet Geography
Top posts and pages.

Latest Blog Entries

Pin It on Pinterest
- Click to share
- Print Friendly
- Ways to Give
- Contact an Expert
- Explore WRI Perspectives
Filter Your Site Experience by Topic
Applying the filters below will filter all articles, data, insights and projects by the topic area you select.
- All Topics Remove filter
- Climate filter site by Climate
- Cities filter site by Cities
- Energy filter site by Energy
- Food filter site by Food
- Forests filter site by Forests
- Freshwater filter site by Freshwater
- Ocean filter site by Ocean
- Business filter site by Business
- Economics filter site by Economics
- Finance filter site by Finance
- Equity & Governance filter site by Equity & Governance
Search WRI.org
Not sure where to find something? Search all of the site's content.
Forest Landscape Restoration Is More than Planting Trees: 3 Case Studies from the Western US
- agriculture
- restoration
Aaron Reuben, a communications officer at IUCN, is the lead author of this blog post. It originally appeared on IUCN’s blog.
A chainsaw breaks the peace of a quiet forest in central Oregon. Soon, the crash of a tree follows and the rustle of debris dragged and piled. When the weather cools the next sound will be a crackling one, as great heaps of wood are lit and fires rage.
What we are witness to is a classic scene of deforestation, right? The clearing of virgin woods to make way for cattle, crops or homes?
Wrong. In some cases, this scene is actually one of lands being restored and rejuvenated.
Restoration: It’s Not Just Tree-Planting
Landscape restoration, or bringing productivity back to degraded lands, takes many forms. In much of the western United States, it can mean cutting trees. And setting fires, removing hills, or even, sometimes, unleashing destructive rodents.
Perhaps we better explain. Restoration always comes with a purpose. In the U.S. Pacific Northwest, the purpose is often to make forest landscapes more resilient to climate change, drought or pest disturbance. Paradoxically, this is often accomplished by removing trees from dense forests to reconstruct more natural and open structures, burning undergrowth to encourage greater plant diversity, or cutting soft meandering brooks and meadows across sharp, human-altered streams.
Three short stories of landscape restoration in the western United States follow. They detail projects that are successfully bringing health and productivity back to degraded lands in unexpected ways. Each takes a landscape approach that, as you will see, goes far beyond merely planting trees.
1. Thinning and Burning
Most government agencies wish they could have a mascot as recognizable and effective as Smokey the Bear , the U.S. Forest Service’s cartoon grizzly bear who, since the 1940s, beseeched Americans to help prevent forest fires. Smokey was the face of a successful movement, begun well before the First World War, to stamp out wildfires in American forests. Today Smokey is recognized by 95 percent of American adults and nearly all forests in America bear a legacy of fire prevention .
The trouble is that fire is a natural element of the ecology of many U.S. forests, a fact any forester will happily tell you now . More than 100 years of fire suppression have left U.S. public lands—and many private lands—overstocked with dense trees that should have burned decades ago. If those lands were to catch fire now, the resulting fires would burn hotter, higher and much longer than normal, killing trees naturally designed to survive smaller fires and destroying other forest goods and services, like clean waterways.
To avoid catastrophic burns, which would kill old growth stands, threaten rural communities and clog waterways for years, the U.S. Forest Service and its partners in the Collaborative Forest Landscape Restoration Program routinely remove trees from dense stands (a process called “thinning”), and carefully burn the underbrush and litter below the trees to mimic natural fire. The resulting forest is more open – a structure that leads to greater plant diversity. Burned plant matter enriches the soil and the landscape has less wood and debris to fuel future fires.

In the last 5 years, the 23 projects of the US Forest Services’ Collaborative Forest Landscape Restoration Program have treated more than 1.45 million acres to reduce the risk of catastrophic wildfire, resulting in healthier forests with greater plant diversity and more resilience to pests and climate change. Walking through one restoration site last May, with smoke in our eyes and warm ash under our feet, it was hard to imagine that a burned forest is a restored one. But “this is what the ecosystem calls for,” said Maret Pajutee, forest ecologist for the US Forest Service in central Oregon. What is needed here, surprisingly, is not more trees, but fewer. And more fire.
2. Putting Beavers to Work
You know you are standing on the bank of a beaver managed stream when your feet start getting wet. What you thought was terra firma proves more porous, and water suddenly seems to run everywhere. Beavers hate the sound of flowing rivers and, as quickly as they arrive, subtly turn streams to wetlands and re-channel rivers by building dams. Today, with a changing climate , beavers may be what arid forest regions need.
“With the climate changing we want this system to store more water for us,” said Bob Hassmiller, a hydrologist with the U.S. Forest Service. Though many still view the aquatic rodents as pests, beavers make hydrologists like Hassmiller happy. Beaver activity alters and slows the flow of water through a watershed, he said, “providing natural reservoirs we can benefit from.”When water flows start to drop late in the summer season, beaver-dammed streams will continue to release water and, importantly, provide more flows for fish.

On a chilly day in May Hassmiller stood, pants tucked into tall rubber boots, beside a newly dammed stream running along the Malheur National Forest in Oregon. “Since the beavers have arrived,” he said, “we’ve seen more habitat for fish, and better stream temperatures.” Hassmiller and his colleagues in the Malheur have been using beavers to manage streamside restoration for a few years now, letting the non-human engineers transform landscapes altered by historic human uses. “We’re just glad they’re here.”
3. Aspen Restoration
Walking through eastern Oregon’s forest can be a monotonous process. The ponderosa pine ( Pinus ponderosa ) completely dominates hundreds of thousands of acres of forest in Oregon. With one exception. Very occasionally, you will come across a row of green and white trees, similar-looking and stacked in a pyramid, with a tall tree in the center giving way to short fellows on the sides – the quaking aspen ( Populus tremuloides ).
While aspen are so ubiquitous as to be a nuisance in some parts of the United States, in Oregon they are prized as biodiversity boons: Many species of woodpeckers and songbirds prefer to forage and nest in their boughs; certain mushrooms and insects favor their rich soils; and migrating deer and elk find nourishment in their sprouts and bark even in winter. That is why aspen is a key species for restoration in Oregon, said Trent Seager , PhD candidate in forest ecology at Oregon State University.

Because fire was suppressed in the region for more than 100 years, aspen, which typically return quickly from a fire and use fires to colonize new ground, have slowly disappeared from parts of Oregon’s forests. Because they prefer to grow in water-rich deep soil, and elk eat them when they are small, it can be deviously hard to plant aspen successfully.
So how do you restore a tree that is a challenge to plant?
“Slowly,” said Seager. Aspen grow in a clonal way, with a central tree sending underground root suckers outward to start new trees. This system works pretty well, and new stems in a clonal stand may appear up to 30–40 meters (98–131 feet) from a parent tree. An individual aspen can live for more than 100 years above ground, but the clones of the colony themselves can last for several hundred. The trick for aspen restoration is to find a good aspen stand and help it grow.
“We will remove the pines encroaching on an aspen stand to give the aspen more water, light and nutrients to expand,” said Seager. “And when we thin and burn across the larger landscape, that increases forage for elk and deer and frees up more water at the landscape level for aspen across the area .”
Forest landscape restoration is about more than just planting trees. It involves people coming together to identify the problems degradation causes in their area and finding the solutions that can bring a balanced mix of goods and services back to the land. In many of the forests in the Northwestern US water is a limiting factor. In a fire activated landscape, thinning makes the land healthier, taking pressure off remaining trees and allowing old growth forests to survive low-intensity fires. Allowing beavers to return to the land can bring more water year-round, improving fish stocks and forest health. And releasing old aspen stands from the encroachment of pines can increase biodiversity and wildlife forage.
We hope these stories can inspire those outside the US Northwest to think creatively about improving their landscapes. But some questions invariably arise:
Is the challenge facing the US, where fire was suppressed for decades, comparable to the challenges facing other countries? In many places the battle is to bring trees back to degraded lands, not remove them.
The US Forest Service has worked hard to change its image from a timber logging organization to an organization working towards resilience in human managed landscapes. However, are they depleting their carbon stocks by thinning and burning ( as some have suggested )? Can their 15 million hectare restoration commitment to the Bonn Challenge be considered equal to the 15 million hectares of agroforestry restoration expected from Ethiopia or the eight million hectares of forest landscape restoration committed from the Democratic Republic of the Congo ?
As we move towards more human mediated landscapes, with mounting pressures from climate change and the need for resilience, will landscape engineering become a more prevalent form of landscape restoration governance?
Maybe restoration as a concept is evolving. Before jumping into another tree planting campaign, we should remember to assess what is truly needed for a resilient landscape. It may not always be more trees.
These case studies originate from presentations made at the first International Seminar on Forest Landscape Restoration , hosted by the US Forest Service, World Resources Institute and IUCN. IUCN's contribution to the seminar made possible through support from UK aid, from the UK government.
Relevant Work
Clear waters: restoring fish, culture and land in central oregon, once degraded, west virginia's monongahela national forest is restored to glory, 3 myths and facts about forest and landscape restoration, pressing questions about ecosystem restoration, answered, how you can help.
WRI relies on the generosity of donors like you to turn research into action. You can support our work by making a gift today or exploring other ways to give.
Stay Informed
World Resources Institute 10 G Street NE Suite 800 Washington DC 20002 +1 (202) 729-7600
© 2024 World Resources Institute
Envision a world where everyone can enjoy clean air, walkable cities, vibrant landscapes, nutritious food and affordable energy.
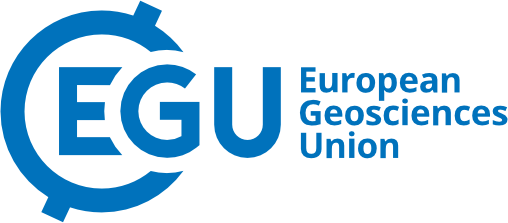
Article
- Volume 21, issue 9
- BG, 21, 2297–2311, 2024
- Peer review
- Related articles
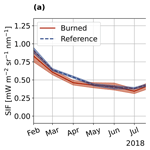
Monitoring the impact of forest changes on carbon uptake with solar-induced fluorescence measurements from GOME-2A and TROPOMI for an Australian and Chinese case study
Juliëtte c. s. anema, klaas folkert boersma, piet stammes, gerbrand koren, william woodgate, philipp köhler, christian frankenberg, jacqui stol.
Reliable and robust monitoring tools are crucial to assess the effectiveness of land mitigation techniques (LMTs) in enhancing carbon uptake, enabling informed decision making by policymakers. This study, addressing one of the scientific goals of the EU Horizon 2020 Land Use Based Mitigation for Resilient Climate Pathways (LANDMARC) project, examines the feasibility of using satellite solar-induced fluorescence (SIF) in combination with other satellite data as a monitoring proxy to evaluate the effects of LMTs on carbon uptake. Two distinct cases are explored: (1) instantaneous vegetation destruction caused by a 2019 eucalyptus wildfire in southeast Australia and (2) gradual forest gain resulting from reforestation efforts in northern China in 2007–2012. The cases are monitored using SIF from the TROPOspheric Monitoring Instrument (TROPOMI) and Global Ozone Monitoring Experiment-2A (GOME-2A), respectively. Comparing the temporal variability in SIF across the affected areas and nearby reference areas reveals that vegetation dynamics changed as a consequence of the land-use changes in both cases. Specifically, in the Australia case, TROPOMI demonstrated an immediate reduction in the SIF signal of 0.6 mW m −2 sr −1 nm −1 ( − 72 %) over the eucalypt forest right after the fire. Exploiting the strong correspondence between TROPOMI SIF and gross primary productivity (GPP) at the nearby representative eddy covariance Tumbarumba site and through the FluxSat product, we estimate that the Australian fire led to a loss in GPP of 130–200 GgC in the first 8 months after the fire. Over the northern Chinese provinces of Gansu, Shaanxi, Sichuan, Chongqing, and Shanxi, we report an increase in GOME-2A summertime SIF of 0.1–0.2 mW m −2 sr −1 nm −1 , coinciding with reforestation efforts between 2007 and 2012. This increase in the SIF signal is likely driven by a combination of increasingly favourable natural conditions and by the reforestation effort itself. A multivariate model that takes into account growth factors such as water availability and maximum temperature as well as satellite-derived forest-cover data explains the observed variability in GOME-2A SIF in the Chinese case reasonably well ( R 2 =0.72 ). The model suggests that increases in both forest cover and soil moisture have led, in step, to the observed increase in vegetation activity over northern China. In that region, for every 100 km 2 of additional forest cover, SIF increases by 0.1 mW m −2 sr −1 nm −1 between 2007 and 2012. Our study highlights that the use of satellite-based SIF, together with supporting in situ, modelled, and satellite data, allows us to monitor the impact of LMT implementation on regional carbon uptake as long as the scale of the LMT is of sufficient spatial extent.
- Article (PDF, 3775 KB)
- Supplement (979 KB)
- Article (3775 KB)
- Full-text XML

Anema, J. C. S., Boersma, K. F., Stammes, P., Koren, G., Woodgate, W., Köhler, P., Frankenberg, C., and Stol, J.: Monitoring the impact of forest changes on carbon uptake with solar-induced fluorescence measurements from GOME-2A and TROPOMI for an Australian and Chinese case study, Biogeosciences, 21, 2297–2311, https://doi.org/10.5194/bg-21-2297-2024, 2024.
Photosynthetic carbon uptake by the terrestrial biosphere plays a crucial part in the carbon cycle and is the largest flux that contributes to the carbon sink. Over the last 60 years, the terrestrial biosphere captured an amount of carbon equivalent to approximately 32 % of the total anthropogenic emissions in that period ( Friedlingstein et al. , 2020 ) . However, the ongoing efficacy of this photosynthetic carbon flux, or gross primary productivity (GPP), is not assured due to feedback mechanisms driven by climate change and human activity (e.g. Brook et al. , 2013 ; Huntzinger et al. , 2017 ) . Preserving the terrestrial biosphere's pivotal role in dampening the rise in atmospheric CO 2 concentrations is crucial to address the current climate crisis. In response to this urgency, land mitigation techniques (LMTs) play a key role as negative carbon technology in national climate strategies ( United Nations , 2016 ) and in the climate pathways of the Intergovernmental Panel on Climate Change ( Rogelj et al. , 2018 ) . These techniques include a variety of practices, such as reforestation and fire management, aimed at enhancing the land's carbon assimilation and preventing carbon stock loss.
The potential of large-scale LMT implementation is exemplified by observed greening trends over actively reforested areas across central to northern China ( Chu et al. , 2019 ; Gerlein-Safdi et al. , 2020 ) . However, persisting uncertainty regarding the LMTs' effectiveness in enhancing GPP and carbon sequestration in the long term limits their widespread implementation (e.g. Harper et al. , 2018 ) . The need for robust quantification of changing GPP following land-cover change is further emphasized by the uncertain impacts of rising atmospheric CO 2 concentrations and climate change itself on the terrestrial biosphere ( Schimel et al. , 2015 ; Smith et al. , 2020 ; Duffy et al. , 2021 ; Schurgers et al. , 2018 ) . Understanding the efficiency and applicability of LMTs is essential for effective climate action. Therefore, the Horizon 2020 LANDMARC (Land Use Based Mitigation for Resilient Climate Pathways) project ( https://www.landmarc2020.eu/ , last access: 8 June 2023) has developed monitoring tools, including satellite-based remote sensing, to study the feasibility of negative emission monitoring using multiple LMT cases worldwide as test cases.
The recent development of satellite-based measurements of solar-induced chlorophyll fluorescence (SIF) from atmospheric sensors has opened up promising opportunities to monitor the GPP dynamics ( Frankenberg et al. , 2011 ; Joiner et al. , 2011 ) . Chlorophyll fluorescence (660–850 nm) is the re-emission of the absorbed photosynthetically active radiation (APAR) by the leaf chlorophyll during photosynthesis ( Frankenberg and Berry , 2017 ) . The emission of fluorescence dissipates part of the excitation energy from APAR that is not transported to and used by the photochemical reactions in the plant – making both fluorescence and GPP dependent on APAR ( Porcar-Castell et al. , 2014 ) . SIF measurements depict the captured chlorophyll photons integrated over the canopy within the field of view and thus directly follow the dynamics of photosynthetic activity. Therefore, SIF follows GPP more consistently than traditional greenness parameter observations such as NDVI (e.g. Magney et al. , 2019 ; Gerlein-Safdi et al. , 2020 ) .
As part of one of the research objectives of the LANDMARC project, the main goal of this study is to assess the feasibility of satellite-based SIF in combination with other satellite data to monitor the effect of land mitigation techniques (LMTs) on carbon uptake. Monitoring tools could potentially benefit from the ability of SIF to track GPP dynamics consistently across various biomes and scales ( Zhang and Schwärzel , 2017 ; Verma et al. , 2017 ; Dechant et al. , 2020 ) and from its demonstrated sensitivity to climate stress ( Song et al. , 2018 ; Ač et al. , 2015 ) . Previous studies indicated the ability of SIF to evaluate changes in land cover ( Gerlein-Safdi et al. , 2020 ; Guo et al. , 2021 ) . However, to our knowledge, the use of SIF aligned with supporting satellite data to quantify the isolated effect of land-use change on GPP has not been assessed.
Here, we use TROPOMI and GOME-2A SIF to monitor two cases of forest-cover change. First, we use monthly TROPOMI SIF to track the aftermath of a 2019 wildfire in southeast Australia, impacting around 210 km 2 of eucalypt forest. Next, we use summertime GOME-2A SIF to monitor multi-year reforestation efforts in northern China from 2007–2012. The Australian case acts as a proof of concept due to its immediate and distinct single-cause land-cover change, while the Chinese case tests how SIF can be used to monitor the combined effect of gradual forest-cover change and variability in growth conditions (water and radiation availability) ( Gao et al. , 2019 ; Lin et al. , 2020 ; Wang et al. , 2022 ) . Satellite-based data, including MODIS burned-area data and Landsat-derived annual land-cover data, are used to evaluate the spatial and temporal extent of the changed forest cover in both cases. Subsequently, a comparative analysis between the impacted and reference areas was conducted.
Our study addresses the following research questions. (1) Is it possible to monitor changes in vegetation dynamics that are linked to instantaneous or gradual land-cover changes over scales that can be resolved by the TROPOMI and GOME-2A sensors? (2) How can we quantify the impact of instantaneous land-use change on carbon uptake? (3) How can we use remotely sensed metrics to improve our understanding of climate variability and forest-cover change on interannual SIF?
2.1 Space-based SIF observations
Satellite measurements of SIF are powerful proxies for vegetation growth, and they have been used in many studies to monitor the changes in GPP. Retrieval of SIF from satellite measurements, e.g. from GOME-2A and TROPOMI that offer near-daily global coverage, relies on the relative filling in of solar Fraunhofer lines in the Earth's radiance spectra. The apparent depth of the Fraunhofer lines is not affected by scattering or reflection from clouds or by the Earth's surface. However, if vegetation is present on the surface, the addition of solar-induced fluorescence (SIF) as a spectrally continuous surface light source dampens the relative depth of the lines, and this (relative) filling in of the Fraunhofer lines is a measure of the strength of fluorescence ( Joiner et al. , 2011 ; Frankenberg et al. , 2011 ) . The spectral-fitting window is made wide enough to cover multiple strong Fraunhofer lines and sufficiently narrow as to limit the presence of atmospheric absorption features. Spectral-basis functions derived from observations over vegetation-free non-fluorescent areas are used to describe the impact of atmospheric interaction and possible instrumental artefacts – the reference set. Accordingly, the SIF signal is computed by fitting the satellite-observed spectrum with a modelled reflectance spectrum assembled from the spectral-basis functions. Uncertainty in the SIF data emerges from measurement noise, the presence of optically thick clouds in the satellite field of view, and the fitting procedure, the latter depending on the quality and representativeness of the spectral-basis functions. To mitigate uncertainty, SIF observations with cloud cover fractions below 0.2 are used.
2.1.1 SIF retrieved from TROPOMI
In this study, we use level-2 SIF (740 nm) data retrieved from the TROPOspheric Monitoring Instrument (TROPOMI) by Caltech ( Köhler et al. , 2018 ) . TROPOMI is an atmospheric spectrometer on board the Sentinel-5 Precursor that performs in a wide spectral range from the ultraviolet to the shortwave-infrared. The instrument was launched on 13 October 2017 and flies in a low-Earth polar sun-synchronous orbit with equatorial crossing at 13:30 local solar time (LST) and with a repeat cycle of 17 d ( Veefkind et al. , 2012 ) . The 2600 km wide swath enables almost daily global coverage. TROPOMI SIF, with a spatial resolution of 7 × 3.5 km 2 at nadir, is retrieved from a subset of the NIR band with a selected window ranging from 743 to 758 nm. TROPOMI SIF has a single-pixel 1 σ uncertainty in the range of 0.3–0.5 mW m −2 sr −1 nm −1 ( Köhler et al. , 2018 ) .
TROPOMI SIF has been previously used to track photosynthetic activity following extreme precipitation events in the US ( Turner et al. , 2021 ) , during the dry season in the Amazon forest ( Doughty et al. , 2019 ) , and over evergreen forests ( Magney et al. , 2019 ) . Furthermore, it is extensively validated with GPP from multiple eddy covariance towers and with Orbiting Carbon Observatory-2 (OCO-2) SIF ( Li and Xiao , 2022 ; Köhler et al. , 2018 ) . Until ESA's FLEX mission with a spatial resolution of 300 m ( Drusch et al. , 2016 ) is launched (in 2025), TROPOMI SIF is arguably the most appropriate data product to track photosynthetic activity from vegetation on a global scale from space. The main limiters in detecting the impact of LMTs on vegetation activity are the spatial scale of a TROPOMI pixel ( ± 20 km 2 ) and the data not being available before April 2018.
2.1.2 SIF retrieved from GOME-2A
We also use clear-sky, gridded (level-3) SIF data from the Royal Netherlands Meteorological Institute (KNMI) Sun-Induced Fluorescence of Terrestrial Ecosystems Retrieval (SIFTER) v2 product ( Kooreman et al. , 2020 ; Van Schaik et al. , 2020 ) retrieved from the Global Ozone Monitoring Experiment-2A (GOME-2A) sensor. The data have a spatial resolution of 0.5° × 0.5°. GOME-2A was launched on 6 November 2006 aboard the Meteorological Operational (Metop-A) satellite and flies in a sun-synchronous orbit with equatorial crossing at 09:30 LST. The GOME-2A swath covers 1920 km, resulting in a spatial resolution of 80 × 40 km 2 at nadir. Due to a swath reduction on 15 July 2013, the spectral resolution thereafter was 40 × 40 km 2 . Despite the 160-times-coarser resolution of GOME-2A in comparison to TROPOMI, the GOME-2A SIF data are of relevance due to the long temporal record, starting from 2007. The GOME-2A SIF product is retrieved from a subset of band 4 with a selected window ranging from 734 to 758 nm, and the SIFTER retrieval principle is similar to that of TROPOMI SIF. GOME-2A SIF has a single-pixel uncertainty on the order of 0.6 mW m −2 sr −1 nm −1 ( Van Schaik et al. , 2020 ) . The retrieval is considered most reliable in the period before the sensor switch in July 2013 ( Van Schaik et al. , 2020 ) . After the July 2013 switch, degradation of the GOME-2A sensor and subsequent calibration updates in different level-1 data versions have posed difficulties in deriving a consistent, bias-free data record. Post hoc degradation corrections introduced in Van Schaik et al. ( 2020 ) have (at least partly) improved the consistency of the GOME-2A SIF data record in the post-2013 years.
We exclusively use the GOME-2A data from the years 2007–2012 for two main reasons. Firstly, this enables a constant satellite viewing geometry throughout the analysed time series. Secondly, we limit level-1 calibration issues associated with strong instrument degradation and unresolved differences in level-1 calibration post-processing within the dataset ( Van Schaik et al. , 2020 ; Gerlein-Safdi et al. , 2020 ) . Additionally, we applied a year-specific multiplicative correction to the SIF data, addressing the effect of instrument degradation on SIF. The corrections are determined over China's Gobi Desert, where vegetation is mostly absent and a constant near-zero SIF signal is expected. Supplement S1 describes the correction procedure in detail, and we note here that the (multiplicative) correction factors have values between 0.85 and 1.12 and that their application improves the correspondence between GOME-2A SIF and FluxSat GPP over northern China (Fig. S3).
Various applications have shown the data to be of good use for detecting the effects of heatwaves on GPP in Europe ( Wang et al. , 2020 ) , quantifying GPP over Africa ( Getachew Mengistu et al. , 2021 ) , and mapping the impact of El Niño on forest productivity in the Amazon ( Fancourt et al. , 2022 ) . GOME-2A SIF has been validated with GPP from various eddy covariance flux towers in Africa ( Getachew Mengistu et al. , 2021 ) .
2.2 Gross primary productivity (GPP) data
2.2.1 gpp from tumbarumba flux tower.
This study uses estimated GPP data from the Tumbarumba OzFlux eddy covariance flux tower site as a ground reference to the TROPOMI SIF observations (AU-TUM, 35.66° N, 148.15° E; Stol and Kitchen , 2022 ) . The Tumbarumba site is located in the Bago State Forest and is predominately covered by eucalyptus forest. GPP is not directly measured but is calculated using partitioning methods by the extraction of modelled respiration from the measured net ecosystem exchange (NEE) of CO 2 . NEE at the site is quantified using the eddy covariance method, which involves simultaneously measuring vertical wind speed and CO 2 concentration ( Moene and Van Dam , 2014 ) .
For our analysis, we use the quality-checked and gap-filled daily GPP (in gC m 2 d −1 ) from the level-6 data. The Tumbarumba data are processed following the standard tool developed by OzFlux, which is described in more detail in Isaac et al. ( 2017 ) . To match the recording time of TROPOMI SIF and account for the impact of the Dunn's Road fire that affected the OzFlux site in December 2020, GPP data between February 2018 and November 2020 are used.
2.2.2 FluxSat GPP product
The modelled global FluxSat GPP product is used as independent GPP data to compare against SIF and the OzFlux GPP data for the Australia case study. The dataset contains daily averaged GPP estimates (gC m −2 d −1 ) at a spatial resolution of 0.05° × 0.05° over the period from March 2000 to August 2020. The FluxSat GPP product was found to agree well with GPP from flux towers ( Joiner et al. , 2018 ) , and TROPOMI-inferred GPP ( Turner et al. , 2021 ) and has previously been used to quantify changes in carbon uptake (e.g. Byrne et al. , 2021 ; Bacour et al. , 2019 ) .
The global FluxSat GPP ( Joiner et al. , 2018 ) product is derived from the evaluation and training of satellite-data-driven models that are based on the light use efficiency concept ( Monteith , 1972 ) , which states that there is a linear relationship between GPP and the absorbed photosynthetically active radiation. The product's objective is to estimate GPP accurately at a high temporal (daily) and spatial resolution. MODIS reflectance (MCD43) data serve as vegetation index inputs for the model. Furthermore, the models are trained and calibrated using a subset of eddy covariance flux data that contains data across various plant types. In addition, GOME-2A SIF (v27; Joiner et al. , 2013 , 2016 ) , a different SIF product from NASA, is used in the FluxSat calibration procedure to delineate highly productive regions that are not well fitted and are underestimated using the global regression model. Guanter et al. ( 2014 ) found that high GPP values from cropland deviated from the linear relationship. Therefore, highly productive regions were trained and calibrated separately using an eddy covariance GPP subset that met the high SIF and GPP criteria ( Joiner et al. , 2018 ) . As GOME-2A SIF is merely used to identify productive areas, FluxSat GPP estimates remain largely independent from SIF.
We first investigate the case of a 2019 eucalyptus wildfire in southeastern Australia to evaluate the ability of SIF to capture the fire-induced change in vegetation dynamics. Lightning strikes on 16 January 2019 ignited multiple fires across remote forested areas in Victoria, southeast Australia. Strong northwesterly winds propagated and coalesced several individual fires into a clustered eucalyptus fire, which is locally known as the Nunnett–Timbarra River fire due to its extent between the Nunnett State Forest and the Timbarra River. Burning for nearly 4 weeks, the wildfire impacted around 210 km 2 of eucalyptus forest spanning from 37.35–37.2° S to 148.0–148.2° E. The impacted area had not previously been affected by wildfires within the 25 years prior to the event ( Lindenmayer and Taylor , 2020 ) . The fire has been documented to have caused a sharp reduction in leaf biomass in January 2019 but left eucalypt tree stems and branches mostly intact, according to witness reports by the Australian Forestry Service.
Figure 1 shows the location of the area impacted by the Nunnett–Timbarra River fire in the context of the wider biogeography of southeast Australia. Notably, Fig. 1 c and d show that prior to the fire, the area enclosed by the solid line (which would burn down in early 2019) as well as the surrounding area bound by the dashed line (which was spared from the fire) had high levels of SIF and GPP, indicating vigorous growth conditions in both areas under normal circumstances.
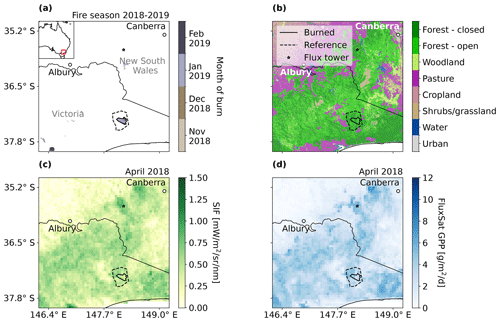
Figure 1 Location and extent of the Nunnett–Timbarra River fire and selected reference area. Maps of (a) the burned area per month of burn from the MODIS MCD64A1 Burned Area product ( Giglio et al. , 2015 ) , (b) dominant land cover over the area with classification based on the 2015 Land Cover Dataset ( Lymburner et al. , 2015 ) , (c) monthly mean TROPOMI SIF (0.05° × 0.05°), and (d) monthly mean FluxSat GPP (0.05° × 0.05°) in April 2018. The outlines of the burned area (approximately 210 km 2 ) and reference area (approximately 975 km 2 ) are indicated by the solid and dashed lines, respectively. Location of the Tumbarumba OzFlux site (AU-TUM) used in this study is shown as a black star.
3.1 Reduction in SIF following the eucalyptus forest fire
We evaluate the spatial and temporal trends in TROPOMI SIF over the burned area from February 2018 until November 2020. The monitoring period ends in November 2020 to avoid potential interference from subsequent wildfire events that occurred in December 2020 and affected the studied region ( Bowman et al. , 2021 ) . The extent of the eucalyptus fire is identified using the MODIS MCD64A1 Burned Area monthly product ( Giglio et al. , 2015 ) (Fig. 2 a), and the impacted burned area is defined as the region encompassing the MODIS burned pixels, indicated by the solid lines in Figs. 1 and 2 a and b. Next, for comparison, we carefully selected a reference area surrounding the burned area that remained unaffected by the fire. This reference area was chosen to closely match the biogeography of the impacted area, consisting of a mixture of open and closed eucalyptus forest (Fig. 1 b). SIF observations over the reference area are assumed to represent the forest dynamics over the burned area as if the fire had not taken place.
To evaluate temporal trends in SIF over both areas, we categorized TROPOMI level-2 pixels as either “burned” or “reference” based on their spatial overlap with each respective area. Criteria were implemented for each group to ensure pixel exclusivity and minimize interference. Pixels were labelled as burned if the burned-area fraction exceeded 50 %. Pixels were labelled as reference if they comprised less than 30 % of the burned area. Subsequently, within each group, the SIF values were averaged on a monthly basis. Note that throughout the reporting of the results, all uncertainty values are stated in standard error (SE).
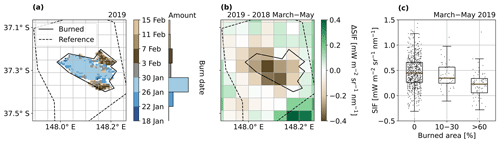
Figure 2 Impact of the 2019 eucalyptus fire on TROPOMI SIF over time and in comparison to the unburned reference area and pre-fire conditions. Map of (a) the burned area and specific burn data at a 1 km scale from the MODIS MCD64A1 Burned Area product and (b) the difference in SIF between post- (2019) and pre-fire (2018) conditions in March–May (autumn). Brown indicates higher SIF in 2018 (pre-fire); green indicates higher SIF in 2019 (post-fire). (c) Correlation between SIF in March–May 2019 (post-fire) and the coverage of the 500 m burned-area pixels by the SIF pixels, in percentage.
Figure 2 b shows the difference in SIF between autumn 2019 (post-fire) and 2018 (pre-fire). Over the area that burned down, autumnal SIF values in 2019 decreased significantly compared those in to 2018. In contrast, SIF at the reference area remained consistent across both years. Furthermore, the lowest SIF values (0.25 mW m −2 sr −1 nm −1 ) coincide with pixels with burned-area fractions higher than 60 %, whereas SIF is much higher (0.45 mW m −2 sr −1 nm −1 ) in pixels that had not burned, as illustrated in Fig. 2 c. We conclude that TROPOMI SIF is capable of capturing the strong reduction in vegetation activity over the burned area relative to the unburned reference area, noting that the affected area extends over some 200 km 2 , equivalent to eight individual TROPOMI pixels.
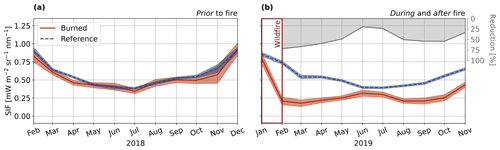
Figure 3 Time series of SIF over the burned and reference areas (a) prior to the fire in 2018 and (b) during and after the fire in 2019. The shaded red and blue areas represent the standard error (SE) in monthly SIF over the burned and reference areas, respectively. The right y axis of panel (b) shows the reduction in SIF (in %) over the burned area ( F B ) as compared to SIF over the reference area ( F R ), which is calculated as 1 - F R - F B F R . Prior to monthly averaging, SIF pixels beyond ± 2 σ from their respective seasonal group mean were removed.
Figure 3 a shows the seasonal cycle in 2018 (pre-fire) inferred from TROPOMI SIF over the to-be-burned and reference areas. Prior to the fire event, the observed SIF values indicate consistency and strong agreement between the two areas ( r =0.97 ; Fig. S4a). However, shortly after the fire, a substantial reduction in SIF over the burned area becomes evident (Fig. 3 b). This immediate fire-induced impact is highlighted by a striking 72 % decrease in February SIF values compared to those of the reference area. In the subsequent months, the vegetation activity over the burned area remained low but non-zero, with an averaged SIF of 0.26 ± 0.01 mW m −2 sr −1 nm −1 between February and August 2019. During springtime (September–November), vegetation recovery was noticeable, with SIF values reaching 0.43 ± 0.04 mW m −2 sr −1 nm −1 by November. In fact, SIF grew faster in springtime over the burned area than over the reference area, with respective increases of 208 % and 144 %, hinting at accelerated regrowth in a moderately burned area, a trend which has also been reported in Zambia and Alaska ( Chungu et al. , 2020 ; Madani et al. , 2021 ) .
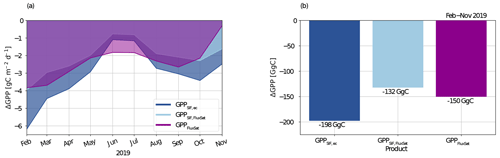
Figure 4 Reduction in GPP following the eucalyptus wildfire over the first 8 months post-fire (February–November 2019). (a) Time series of reduced GPP and (b) bar plot of total reduction obtained from SIF-based GPP using data from the Tumbarumba eddy covariance site (GPP SIF, ec ), SIF-based GPP using FluxSat GPP (GPP SIF, FluxSat ) over Tumbarumba, and FluxSat GPP (GPP FluxSat ), shown in dark blue, light blue, and purple, respectively.
3.2 Quantifying the fire-induced reduction in GPP
Next, based on the observed reduction in SIF, we quantify the fire-induced reduction in GPP. Previous studies found space-based SIF observations to be proportional to GPP at the canopy scale. This proportionality is defined by biome-specific linear relationships that are widely used to scale SIF to GPP (e.g. Turner et al. , 2020 ; Liu et al. , 2017 ; Sun et al. , 2018 ) . Building upon this recognized relationship between SIF and GPP, we assume that the relative reduction in SIF (Sect. 3.1 ) represents the loss in GPP ( Δ SIF ∝ Δ GPP). Consequently, we express the fire-induced change in GPP over the burned area Δ M B ( t ) (in gC m −2 d −1 ) over month t as the product of the relative change in SIF ( Δ F B ( t ) ) and the “baseline” GPP ( M R ( t )) over the reference area (as if no fire had occurred):
To compute the SIF-based GPP over the reference area ( M R ( t )), we first characterize the SIF–GPP relationship using GPP data from a representative eddy covariance site (see Supplement S2). Specifically, GPP data from the nearby Tumbarumba (AU-TUM) site ( Stol and Kitchen , 2022 ) are used to compare against TROPOMI SIF. We obtain the SIF-based GPP over the reference area using the following equation:
where F R ( t ) represents the SIF evolution over the reference area and M TUM F TUM is the local SIF–GPP relationship over the Tumbarumba flux site. We hold the empirical SIF–GPP relationship over Tumbarumba to be applicable to our study area. This is supported by (i) the similar biogeography of lowland eucalyptus forest in both areas (Fig. 1 b), (ii) the strong spatiotemporal correlation between TROPOMI SIF over Tumbarumba and over the reference area in the year prior to the fire ( r =0.92 ; Fig. S4b), and (iii) the good correlation between FluxSat GPP estimates over Tumbarumba and over the reference area in the year prior to the fire ( r =0.79 ; Fig. S4c). Moreover, the discrepancy between SIF–GPP FluxSat over Tumbarumba and the Nunnett–Timbarra River area is small: on the order of 4 % (Supplement S2.1)
As an alternative, we also compute SIF–GPP relationships over Tumbarumba using TROPOMI SIF and modelled FluxSat GPP. The temporal dynamics of TROPOMI SIF exhibit strong consistency with both the GPP estimated from eddy covariance flux tower data ( r =0.91 ) and the modelled (FluxSat) GPP ( r =0.94 ), providing some confidence in both estimates. However, the SIF–GPP relationship based on the flux tower GPP measurements is 1.6 times stronger than the relationship based on the FluxSat GPP data (Table S2.1, Fig. S5a). This discrepancy likely arises from higher GPP estimates from the eddy covariance flux tower – nearly twice that of the estimates from FluxSat (Fig. S5b) – and inherited uncertainties and biases in the estimates of the FluxSat GPP product. Our findings are consistent with Joiner et al. ( 2018 ) , who also observed magnitude differences up to a factor of 2 between FluxSat GPP and independent FLUXNET 2015 GPP data at some sites. Despite differing footprint sizes between the Tumbarumba tower and FluxSat model, this is not expected to significantly impact the uncertainty, given the extensive land cover around the tower (Supplement S2.1).
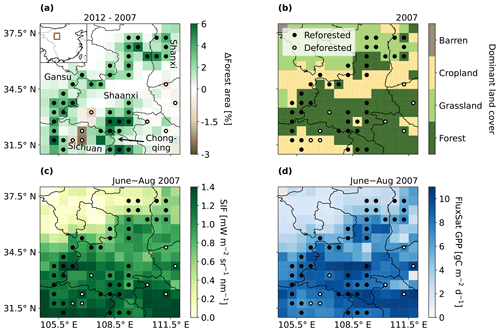
Figure 5 Location and extent of the reforestation efforts between 2007 and 2012 over the study region in northern China. Maps of (a) changed forest cover in 2012 with respect to 2007 (in % of cell area) obtained from the China land-cover dataset (CLCD) ( Yang and Huang , 2021 ) , with green indicating higher forest coverage in 2012 (reforestation) and brown indicating higher forest coverage in 2007 (deforestation) and (b) dominant land cover in 2007 with classification based on the CLCD, aggregated over the 0.5° × 0.5° GOME-2A SIF grid. Croplands are shown in yellow, grasslands in light green, and forests in dark green. Maps of (c) GOME-2A SIF ( Van Schaik et al. , 2020 ) and (d) FluxSat GPP ( Joiner et al. , 2018 ) , averaged over summertime (June–August) 2007. Selected reforested cells and deforested cells are indicated by black-filled and white-filled circles, respectively.
Finally, we estimate the reduction in GPP employing SIF–GPP relationships that use (1) the eddy covariance GPP data and (2) FluxSat GPP data over Tumbarumba – hereon referred to as GPP SIF, ec and GPP SIF, FluxSat , respectively. Additionally, we compute the reduction in GPP from the FluxSat GPP data directly over the burned and reference areas, referred to as GPP FluxSat . Figure S6 shows an overview of the three approaches to compute Δ GPP.
Figure 4 a shows the estimated fire-induced reductions in GPP over time. The SIF-based approaches, GPP SIF, ec and GPP SIF, FluxSat , show a sharp reduction in GPP immediately after the fire. This is followed by smaller GPP deficits in winter, when vegetation activity is low. The FluxSat data (GPP FluxSat ) also show a direct local impact of the wildfire on GPP. However, the FluxSat data suggest an almost complete recovery in November, in contrast to our SIF-based results.
When integrated over the period from February to November 2019 and across the burned area, we find SIF-based GPP losses Δ GPP SIF, ec and Δ GPP SIF, FluxSat of 198 and 132 GgC, respectively. The SIF-based GPP losses reflect a reduction of 48 % with respect to GPP over the same period in the reference area. Thus, the estimated SIF-based losses are in the range of 130–200 GgC over the initial 8 months post-fire. For comparison, Δ GPP FluxSat indicates a fire-induced loss of 150 GgC over the burned area between February–November compared to the reference area. The total GPP FluxSat over the burned area during this period is 58 % less than over the same period and area in 2018 (pre-fire conditions).
An uncertainty propagation analysis, described in Supplement S2.1, indicates a total uncertainty of approximately 60 % in the estimated SIF-based GPP losses. This total uncertainty is primarily driven by the use of the SIF–GPP relationship at Tumbarumba, where no fire occurred, to derive our GPP-loss estimate via Eq. ( 2 ) over the Nunnett–Timbarra River area that burned down – introducing an estimated uncertainty of 50 %. This uncertainty was determined by comparing SIF and FluxSat GPP relationships under pre-fire and post-fire conditions (see point 2 in Supplement S2.1). We conclude that estimating changes in GPP based on land-cover changes is possible with TROPOMI SIF in combination with empirical SIF–GPP relationships, at least when such land-cover changes occur over a spatial scale of a few hundred km 2 .
We now turn to examining how GOME-2A can be used to monitor the impact of reforestation on carbon uptake in parts of northern China. Our study area includes Shaanxi province, southwestern Shanxi, eastern Gansu, and part of northern Sichuan and Chongqing. This region spans approximately 31 to 38° N and 105.0 to 111.5° W (Fig. 5 a). Over the past 15 years, the area has undergone various greening campaigns like Grain for Green, the Natural Forest Conservation Program, and the Three-North Shelterbelt Program ( Delang and Yuan , 2015 ; Kong et al. , 2021 ) . These efforts have led to observable positive trends in vegetation activity ( Gerlein-Safdi et al. , 2020 ; Lin et al. , 2020 ; Li et al. , 2015 ; Sun et al. , 2015 ; Cao et al. , 2009 ) .
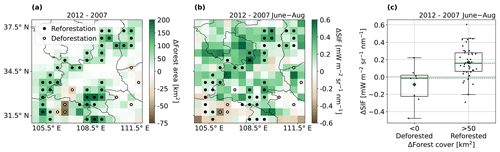
Figure 6 Impact of increased forest coverage in northern China between 2007 and 2012. Maps of changed (a) forest coverage (in km 2 ) calculated from the China land-cover dataset ( Yang and Huang , 2021 ) and (b) summertime GOME-2A SIF during the 6 years. Green indicates higher forest coverage/SIF in 2012 and brown indicates higher forest coverage/SIF in 2007. (c) Contrast in GOME-2A SIF trends between 2007 and 2012 over the deforested and reforested cells. The reforested and deforested cells are indicated by black-filled and white-filled circles in (a) and (b) . The SIF data are obtained from the GOME-2A SIFTER v2 dataset by Van Schaik et al. ( 2020 )
Figure 5 a and b show that most of the forest gain occurred within and at the borders of Shaanxi province, predominantly over areas dominated by forest or cropland cover. Concurrently, Fig. 5 c and d show that high SIF and FluxSat GPP values generally occur in the vegetated southern part of the domain, including the regions where reforestation has taken place, with lower average SIF and GPP values observed in the drier northern part of the region.
4.1 Coinciding increase in forest cover and SIF
We first examine the 2007–2012 variability in summertime (June–August) GOME-2A SIF, when vegetation productivity is at a maximum. To evaluate the changes in SIF, we used the first Landsat-derived annual China land-cover dataset (CLCD) ( Yang and Huang , 2021 ) . These satellite-based data categorize nine major land types such as cropland, forest, and grassland at a spatial resolution of 30 m. Specifically, we extracted forest-coverage data for each year between 2007 and 2012, aggregated over the 0.5° × 0.5° GOME-2A SIF grid. The conversion to forest, from any type of land cover, was determined by quantifying the change in forest cover relative to the 2007 baseline. We then compared changes in summertime SIF between 2007 and 2012 over two contrasting groups of cells: reforested ( n =42 ) and deforested ( n =8 ). We classify cells as “reforested” if they gained more than 2 % of their area in forest coverage (approximately 50 km 2 ) and as “deforested” ( n =8 ) if they lost forest coverage during this period, as shown in Fig. 6 a. Cells in both groups consistently gained or lost forest coverage with every passing year from 2007 to 2012.
The majority of reforested cells showed an increase in average summertime SIF, whereas most deforested cells experienced a decrease (Fig. 6 b). Figure 6 c shows the clear contrast in SIF trends across cells that gained forest coverage compared to those that lost forest coverage. Reforested cells exhibited a significant increase in SIF of 0.17 ± 0.03 mW m −2 sr −1 nm −1 , while SIF decreased by 0.09 ± 0.07 mW m −2 sr −1 nm −1 over the deforested cells between 2007 and 2012. The contrasting trends in vegetation activity indicated by GOME-2A SIF over the reforested and deforested cells are confirmed by co-sampled independent data from the near-infrared reflectance of vegetation (NIRv) and FluxSat GPP (Fig. 7 a and b).
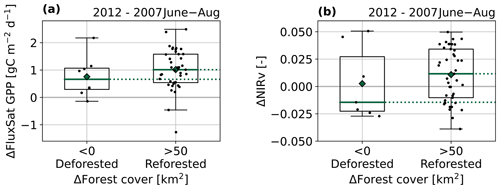
Figure 7 Contrast in changed vegetation activity between deforested and reforested cells between 2012 and 2007, shown using (a) FluxSat GPP and (b) the near-infrared reflectance of vegetation (NIRv) retrieved from MODIS.
Temporal SIF variability across northern China is driven not only by reforestation alone but also by co-occurring changes in local climate factors that influence plant growth, such as water availability, heat, and the presence of photosynthetically active radiation ( Zhang and Ye , 2021 ; Gao et al. , 2019 ; Lin et al. , 2020 ) . To examine the co-occurring impact of climate variability and changes in forest cover, we use satellite-based soil moisture and maximum temperature. Soil moisture data were obtained from the Surface Soil Moisture (Active) product ( Dorigo et al. , 2019 ) and the daily maximum temperature was derived from hourly 2 m temperature from ERA5-Land data ( Muñoz Sabater , 2019 ) . Both were aggregated to the 0.5° GOME-2A SIF grid. The summers from 2007 to 2012 in Shaanxi and surrounding provinces are characterized by soil moisture values (50 %–55 %; Fig. S7b) that are generally good for plant growth, showed a slight increase over time (1 % yr −1 ), and had relatively stable (average) maximum temperatures (Fig. S7a). The summer of 2010 was somewhat of an exception, with a maximum temperature more than 1 °C below the multi-annual mean, which we interpret as indicating lower radiation levels. Overall, we expect that interannual variability in climate factors favoured forest growth between 2007 and 2012 in both the reforested and deforested areas, in line with previous studies (e.g. Zhang et al. , 2022 ) . Overall conditions for vegetation growth appear more favourable in cells where reforestation occurred, as maximum temperatures were more moderate (23 °C) and soil moisture was higher (53 %) in the cells with reforestation than in cells with deforestation.
4.2 Interpretation of temporal variability in SIF
We constructed a simple model that expresses the local year-to-year variability in summertime SIF as a linear combination of soil moisture ( S ; in %), maximum temperature ( T ; in °C) averaged over spring to summertime (March–August), and the total forest coverage ( A ; in km 2 ): Eq. ( 3 ). This model serves to explore the impact of different climatic drivers on observed SIF, representing vegetation activity, alongside the effect of change in vegetation. The selection of this model is motivated by three factors: (i) soil moisture promotes the thriving of vegetation by enhancing the water availability, (ii) increasing temperature stimulates vegetation activity until an optimal temperature is reached ( Gao et al. , 2019 ) and is a proxy for photosynthetic active radiation, and (iii) total photosynthetic carbon uptake increases with increasing vegetation cover. Note that the climate parameters are averaged over the spring and summer period (March–Aug) to account for the concurrent and lagging effects on the vegetation activity over summer (e.g. Sun et al. , 2021 ).
We use a multivariate-least-squares regression to optimize the combined fit coefficients that best explain the observed SIF over the reforested and deforested cells. Modelled SIF as a function of the year (between 2007 and 2012), F mod ( t ), is expressed as
where α , β , γ , and δ represent the coefficients that minimize the discrepancies ( ϵ ) between F mod ( t ) and the observed GOME-2A time series F obs ( t ). The predictors of soil moisture, maximum temperature, and forest cover are indicated by S ( t ), T ( t ), and A ( t ), respectively, over the year t . To ensure sufficient statistics, we pooled the time series of SIF ( n =6 ) from all 50 selected cells, resulting in a single cell-ensemble fit. Overall, the modelled variability in SIF matches the measured variability reasonably well ( R 2 =0.57 ; n =300 ). Figure 8 a shows the correlation between the observed ( F obs ) and modelled ( F mod ) SIF over all cells.
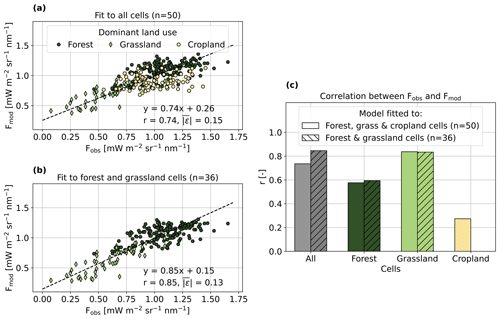
Figure 8 Results from the regression model in Eq. ( 3 ) fitted to (a) all cells ( n =50 ) and (b) exclusively forest and grassland cells ( n =36 ). Panel (c) shows the correlation between observed ( F obs ) and modelled ( F mod ) SIF for each dominant land type and for both fits.
However, the model's performance varied by land-use type across the region (Fig. 8 c). Notably, it demonstrated good agreement between observed and modelled values for cells dominated by grassland ( r =0.84 ) or forest cover ( r =0.58 ) but struggled to represent the dynamics in cropland cells ( r =0.27 ). This suggests distinct differences in the underlying dynamics and complexities in converting cropland areas into forest. Previous studies reported that croplands were often located in drier areas with less forest cover to begin with, reducing the vigour with which vegetation increases in response to reforestation ( Qu et al. , 2020 ) .
We therefore exclusively fitted our final model to data from the 36 cells dominated by grassland or forest cover ( n =216 ; Fig. 8 b and c). By excluding cropland cells, the fit significantly improved, evident from the higher R 2 of 0.72. The improved correlation between the observed and modelled SIF suggests that the model better captures the underlying dynamics. Note that the impact of different factors on the long-term vegetation dynamics is ecosystem-specific; therefore, our model is unique to the fitted area and period. Next, to evaluate the explanatory power of including forest coverage in our model, we constructed an alternative model that solely fits the first two terms on the right-hand side of Eq. ( 3 ), i.e. the climate parameters, to the observed summertime SIF. Doing so allows us to compute the adjusted R 2 of our model (Eq. 3 ) and the alternate model to enable fair comparison between the models containing three and two predictors. The alternate model had an adjusted R 2 of 0.66. With the inclusion of forest-cover data, the model's performance improved, achieving an adjusted R 2 of 0.71 – suggesting the added value of including changed forest coverage in conjunction with climate variability in the model.
Table 1 Regression results of the model fitted to GOME-2A summertime SIF temporal variability over reforested and deforested cells with a dominant land use of grassland or forest ( n =36 ). The model was fitted to data spanning 2007–2012. Uncertainty is given in standard error (SE).
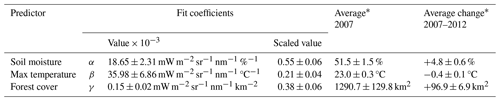
* Over reforested cells ( =31 ).
Download Print Version | Download XLSX
The model's fit coefficients presented in Table 1 demonstrate, as hypothesized, that increases in the predictors positively influence SIF. Soil moisture and forest-cover variability explain most of the variability in GOME-2A SIF, as indicated by the scaled values of the fit coefficients (which consider predictor standard deviations). This is also expected, given the relatively strong increases in soil moisture ( + 5 %) and in forest cover ( + 97 km 2 ) compared to the modest changes in maximum temperature over the provinces from 2007 to 2012. The effect of changes in soil moisture cannot be disentangled from the effect of changes in forest cover on vegetation activity. However, our results show a positive impact of the forest-cover increase on vegetation activity. This is likely occurring in tandem with the changes in soil moisture because soils in regions with increased tree cover tend to hold on to their soil moisture better. We also tested a model including an additional (fourth) interaction term between soil moisture and reforestation, but this did not lead to an improved R 2 , adjusted between modelled and observed SIF. We conclude that vegetation activity following reforestation efforts increases most over the temperate areas in northern China classified as grassland and forest, with already relatively high soil moisture. On average, these areas show high covariance between changes in soil moisture and changes in forest cover, indicating that both factors explain the increase in summertime SIF.
Satellite-based solar-induced fluorescence (SIF) observations, a measure of plant photosynthesis, emerge as a promising tool to track changes in carbon uptake in response to land-use change. In this study we demonstrated that TROPOMI SIF captures sharp local reductions in vegetation activity – and thus carbon uptake in the aftermath of a large forest fire in southeastern Australia, the Nunnett–Timbarra River fire, in 2019 – and also that TROPOMI SIF tracks rapid recovery of vegetation activity in the affected area. By careful cross-evaluation of TROPOMI SIF with coinciding tower- and model-based estimates of global primary productivity (GPP), we estimate that the Nunnett–Timbarra River fire caused a reduction in SIF by on average 0.2 mW m −2 sr −1 nm −1 , corresponding to 130–200 GgC that would otherwise have been stored by the 210 km 2 eucalypt forest if no fire had occurred in the first 8 post-fire months. This shows that TROPOMI SIF is capable of monitoring sudden changes in vegetation activity at a scale of some 20 × 10 km 2 , and this predicts excellent potential for the Fluorescence Explorer (FLEX, to be launched in 2025) to monitor changes in carbon uptake from land management techniques, which often occur at much smaller spatial scales than the Nunnett–Timbarra River fire.
We also found increases in GOME-2A SIF of 0.1–0.2 mW m −2 sr −1 nm −1 over the provinces of Shaanxi, Gansu, Sichuan, and Chonqing in northern China in the period between 2007–2012, when China effectuated a large-scale reforestation programme aimed at reversing desertification. In contrast, in areas where deforestation occurred, GOME-2A SIF showed a reduction in SIF of 0.1 m −2 sr −1 nm −1 over the same period. The possibility of tracking reforestation (and deforestation) with GOME-2A SIF is supported by independent and co-sampled measurements from MODIS NIRv and by the FluxSat model product over the same areas also indicating increased (and decreased) vegetation activity. The relatively coarse-scale GOME-2A SIF measurements (40 × 80 km 2 ) indicate that vegetation activity increased the most in regions with relatively high soil moisture content and substantial reforestation as diagnosed by the China land-cover dataset. This suggests that reforestation is effective in enhancing carbon storage in the relatively wet and temperate regions of northern China but less so in the subtropical parts of the provinces, which are drier and hotter. Interpreting the observed temporal variability in GOME-2A SIF from 2007–2012 with a simple model that accounts for simultaneous spatiotemporal changes in soil moisture, in maximum temperature, and in forest cover, we found that the observed SIF changes in northern China can be explained well ( R 2 =0.72 ) over areas with forests and grasslands that underwent reforestation. Reforestation efforts, in step with soil moisture increases, appear to be driving the observed increase in SIF. This implies that the impact of reforestation on increases in SIF should be interpreted in the context of simultaneous increases in soil moisture.
Satellite-based SIF observations emerge as a promising method to facilitate the tracking of carbon uptake in response to land-use change. Through the analysis of two distinct cases of forest-cover change, we demonstrated the applicability of SIF as a monitoring tool in combination with satellite data that map the land-use change. The supporting data facilitate the pinpointing of SIF cells aligned with land-cover changes. Our comparative approach revealed distinct changes in SIF dynamics related to shifts in forest coverage. This underscores the capability of SIF to capture the impact of land-cover change, whether driven by distinct abrupt events or gradual transitions, on regional vegetation activity.
Our work underscores the fact that satellite measurements of SIF are useful to monitor changes in carbon uptake following land-use change as long as the spatial and temporal scales at which these occur are sharply demarcated. In this study, the availability of high-resolution MODIS burned-area data (pixel size 1 × 1 km 2 ) and TROPOMI SIF (pixel size 7 × 3.5 km 2 ) made it possible to detect sharp changes in vegetation activity following a devastating fire over a relatively large area. It is also possible to attribute observed increases in GOME-2A SIF to changes that occur much more slowly and on an even larger spatial scale, such as the reforestation efforts in northern China, but this requires the careful selection and co-sampling of explanatory biogeographical data such as soil moisture, temperature, and forest cover.
The code needed to co-sample spatiotemporal changes in SIF and in supporting data is available upon request from the first author.
All data used in this study are publicly available. The GOME-2 SIF data are provided by KNMI within the framework of the EUMETSAT Satellite Application Facility on Atmospheric Composition Monitoring (AC SAF). KNMI GOME-2A SIF data can be downloaded from https://doi.org/10.21944/gome2a-sifter-v2-sun-induced-fluorescence ( Kooreman et al. , 2020 ) .
The supplement related to this article is available online at: https://doi.org/10.5194/bg-21-2297-2024-supplement .
JCSA and KFB designed the study and led the writing. JCSA performed the data analysis. PS contributed to the interpretation of the results and to the review of all versions of the manuscript and figures. GK provided and conducted the analysis of the MODIS NIRv data over northern China. PK and CF contributed to the interpretation of the results of TROPOMI SIF over Australia. WW and JS provided the OzFlux data and contributed to the determination of the validity of SIF–GPP over Australia. All co-authors reviewed the paper.
The contact author has declared that none of the authors has any competing interests.
Publisher’s note: Copernicus Publications remains neutral with regard to jurisdictional claims made in the text, published maps, institutional affiliations, or any other geographical representation in this paper. While Copernicus Publications makes every effort to include appropriate place names, the final responsibility lies with the authors.
This work received funding from the European Union's Horizon 2020 research and innovations programme under grant agreement no. 869367 (EU LANDMARC project) and from the European Organization for the Exploitation of Meteorological Satellites (EUMETSAT) Atmospheric Composition Satellite Application Facility (AC-SAF). We acknowledge the free use of SIFTER sun-induced fluorescence data from the (GOME-2A) sensor from https://www.temis.nl , last access: 5 October 2022. Furthermore, we would like to thank LANDMARC colleagues Markus Donat from the Barcelona Supercomputing Center (BSC) for the discussion on intercomparison of SIF with climate variables and Moritz Laub from ETH Zürich for the discussion of the wildfire impact on the carbon cycle perturbation.
This research has been supported by the Horizon 2020 research and innovation programme (grant no. 869367) and the European Organization for the Exploitation of Meteorological Satellites (EUMETSAT) Atmospheric Composition Satellite Application Facility (AC-SAF).
This paper was edited by Anja Rammig and reviewed by two anonymous referees.
Ač, A., Malenovský, Z., Olejníčková, J., Gallé, A., Rascher, U., and Mohammed, G.: Meta-analysis assessing potential of steady-state chlorophyll fluorescence for remote sensing detection of plant water, temperature and nitrogen stress, Remote Sens. Environ., 168, 420–436, https://doi.org/10.1016/j.rse.2015.07.022 , 2015. a
Bacour, C., Maignan, F., MacBean, N., Porcar-Castell, A., Flexas, J., Frankenberg, C., Peylin, P., Chevallier, F., Vuichard, N., and Bastrikov, V.: Improving Estimates of Gross Primary Productivity by Assimilating Solar-Induced Fluorescence Satellite Retrievals in a Terrestrial Biosphere Model Using a Process-Based SIF Model, J. Geophys. Res.-Biogeo., 124, 3281–3306, https://doi.org/10.1029/2019JG005040 , 2019. a
Bowman, D. M., Williamson, G. J., Price, O. F., Ndalila, M. N., and Bradstock, R. A.: Australian forests, megafires and the risk of dwindling carbon stocks, Plant Cell Environ., 44, 347–355, https://doi.org/10.1111/pce.13916 , 2021. a
Brook, B. W., Ellis, E. C., Perring, M. P., Mackay, A. W., and Blomqvist, L.: Does the terrestrial biosphere have planetary tipping points?, Trend. Ecol. Evol., 28, 396–401, https://doi.org/10.1016/j.tree.2013.01.016 , 2013. a
Byrne, B., Liu, J., Yin, Y., and Bowman, K.: The carbon cycle of southeast Australia during 2019–2020: Drought, fires and subsequent recovery This manuscript is a preprint and has been submitted for publication as a, AGU Adv., 2, 1–31, 2021. a
Cao, S., Chen, L., and Yu, X.: Impact of China's Grain for Green Project on the landscape of vulnerable arid and semi-arid agricultural regions: A case study in northern Shaanxi Province, J. Appl. Ecol., 46, 536–543, https://doi.org/10.1111/j.1365-2664.2008.01605.x , 2009. a
Chu, X. X., Zhan, J., Li, Z., Zhang, F., and Qi, W.: Assessment on forest carbon sequestration in the Three-North Shelterbelt Program region, China, J. Clean. Prod., 215, 382–389, https://doi.org/10.1016/j.jclepro.2018.12.296 , 2019. a
Chungu, D., Ng'andwe, P., Mubanga, H., and Chileshe, F.: Fire alters the availability of soil nutrients and accelerates growth of Eucalyptus grandis in Zambia, J. Forestry Res., 31, 1637–1645, https://doi.org/10.1007/s11676-019-00977-y , 2020. a
Dechant, B., Ryu, Y., Badgley, G., Zeng, Y., Berry, J. A., Zhang, Y., Goulas, Y., Li, Z., Zhang, Q., Kang, M., Li, J., and Moya, I.: Canopy structure explains the relationship between photosynthesis and sun-induced chlorophyll fluorescence in crops, Remote Sens. Environ., 241, 111733, https://doi.org/10.1016/j.rse.2020.111733 , 2020. a
Delang, C. O. and Yuan, Z.: China's Grain for Green Program, Springer, ISBN 9783319115047, https://doi.org/10.1007/978-3-319-11505-4 , 2015. a
Dorigo, W., Preimesberger, W., Reimer, C., Van der Schalie, R., Pasik, A., De Jeu, R., and Paulik, C.: Soil moisture gridded data from 1978 to present, v201912.0.0., https://cds.climate.copernicus.eu/cdsapp#!/dataset/satellite-soil-moisture?tab=overview (last access: 2 September 2022), 2019. a
Doughty, R., Köhler, P., Frankenberg, C., Magney, T. S., Xiao, X., Qin, Y., Wu, X., and Moore, B.: TROPOMI reveals dry-season increase of solar-induced chlorophyll fluorescence in the Amazon forest, P. Natl. Acad. Sci. USA, 116, 22393–22398, https://doi.org/10.1073/pnas.1908157116 , 2019. a
Drusch, M., Moreno, J., Del Bello, U., Franco, R., Goulas, Y., Huth, A., Kraft, S., Middleton, E. M., Miglietta, F., Mohammed, G., Nedbal, L., Rascher, U., Schüttemeyer, D., and Verhoef, W.: The FLuorescence EXplorer Mission Concept – ESA's Earth Explorer 8, IEEE Trans. Geosci. Remote Sens., 55, 1273–1284, https://doi.org/10.1109/TGRS.2016.2621820 , 2016. a
Duffy, K. A., Schwalm, C. R., Arcus, V. L., Koch, G. W., Liang, L. L., and Schipper, L. A.: How close are we to the temperature tipping point of the terrestrial biosphere?, Sci. Adva., 7, 1–9, https://doi.org/10.1126/sciadv.aay1052 , 2021. a
Fancourt, M., Ziv, G., Boersma, K. F., Tavares, J., Wang, Y., and Galbraith, D.: Background climate conditions regulated the photosynthetic response of Amazon forests to the 2015/2016 El Nino-Southern Oscillation event, Commun. Earth Environ., 3, 1–9, https://doi.org/10.1038/s43247-022-00533-3 , 2022. a
Frankenberg, C. and Berry, J.: Solar induced chlorophyll fluorescence: Origins, relation to photosynthesis and retrieval, 1986, Elsevier, ISBN 9780128032206, https://doi.org/10.1016/B978-0-12-409548-9.10632-3 , 2017. a
Frankenberg, C., Fisher, J. B., Worden, J., Badgley, G., Saatchi, S. S., Lee, J. E., Toon, G. C., Butz, A., Jung, M., Kuze, A., and Yokota, T.: New global observations of the terrestrial carbon cycle from GOSAT: Patterns of plant fluorescence with gross primary productivity, Geophys. Res. Lett., 38, 1–6, https://doi.org/10.1029/2011GL048738 , 2011. a , b
Friedlingstein, P., O'Sullivan, M., Jones, M. W., Andrew, R. M., Hauck, J., Olsen, A., Peters, G. P., Peters, W., Pongratz, J., Sitch, S., Le Quéré, C., Canadell, J. G., Ciais, P., Jackson, R. B., Alin, S., Aragão, L. E. O. C., Arneth, A., Arora, V., Bates, N. R., Becker, M., Benoit-Cattin, A., Bittig, H. C., Bopp, L., Bultan, S., Chandra, N., Chevallier, F., Chini, L. P., Evans, W., Florentie, L., Forster, P. M., Gasser, T., Gehlen, M., Gilfillan, D., Gkritzalis, T., Gregor, L., Gruber, N., Harris, I., Hartung, K., Haverd, V., Houghton, R. A., Ilyina, T., Jain, A. K., Joetzjer, E., Kadono, K., Kato, E., Kitidis, V., Korsbakken, J. I., Landschützer, P., Lefèvre, N., Lenton, A., Lienert, S., Liu, Z., Lombardozzi, D., Marland, G., Metzl, N., Munro, D. R., Nabel, J. E. M. S., Nakaoka, S.-I., Niwa, Y., O'Brien, K., Ono, T., Palmer, P. I., Pierrot, D., Poulter, B., Resplandy, L., Robertson, E., Rödenbeck, C., Schwinger, J., Séférian, R., Skjelvan, I., Smith, A. J. P., Sutton, A. J., Tanhua, T., Tans, P. P., Tian, H., Tilbrook, B., van der Werf, G., Vuichard, N., Walker, A. P., Wanninkhof, R., Watson, A. J., Willis, D., Wiltshire, A. J., Yuan, W., Yue, X., and Zaehle, S.: Global Carbon Budget 2020, Earth Syst. Sci. Data, 12, 3269–3340, https://doi.org/10.5194/essd-12-3269-2020 , 2020. a
Gao, J., Jiao, K., and Wu, S.: Investigating the spatially heterogeneous relationships between climate factors and NDVI in China during 1982 to 2013, J. Geogr. Sci., 29, 1597–1609, https://doi.org/10.1007/s11442-019-1682-2 , 2019. a , b , c
Gerlein-Safdi, C., Keppel-Aleks, G., Wang, F., Frolking, S., and Mauzerall, D. L.: Satellite Monitoring of Natural Reforestation Efforts in China's Drylands, One Earth, 2, 98–108, https://doi.org/10.1016/j.oneear.2019.12.015 , 2020. a , b , c , d , e
Getachew Mengistu, A., Mengistu Tsidu, G., Koren, G., Kooreman, M. L., Folkert Boersma, K., Tagesson, T., Ardö, J., Nouvellon, Y., and Peters, W.: Sun-induced fluorescence and near-infrared reflectance of vegetation track the seasonal dynamics of gross primary production over Africa, Biogeosciences, 18, 2843–2857, https://doi.org/10.5194/bg-18-2843-2021 , 2021. a , b
Giglio, L., Justice, C., Boschetti, L., and Roy, D.: MCD64A1 MODIS/Terra + Aqua Burned Area Monthly L3 Global 500m SIN Grid V006, NASA EOSDIS Land Processes DAAC [data set], https://doi.org/10.5067/MODIS/MCD64A1.006 , 2015. a , b
Guanter, L., Zhang, Y., Jung, M., Joiner, J., Voigt, M., Berry, J. A., Frankenberg, C., Huete, A. R., Zarco-Tejada, P., Lee, J. E., Moran, M. S., Ponce-Campos, G., Beer, C., Camps-Valls, G., Buchmann, N., Gianelle, D., Klumpp, K., Cescatti, A., Baker, J. M., and Griffis, T. J.: Global and time-resolved monitoring of crop photosynthesis with chlorophyll fluorescence, P. Natl. Acad. Sci. USA, 111, E1327–E1333, https://doi.org/10.1073/pnas.1320008111 , 2014. a
Guo, M., Li, J., Yu, F., Yin, S., Huang, S., and Wen, L.: Estimation of post-fire vegetation recovery in boreal forests using solar-induced chlorophyll fluorescence (SIF) data, Int. J. Wildland Fire, 30, 365–377, https://doi.org/10.1071/WF20162 , 2021. a
Harper, A. B., Powell, T., Cox, P. M., House, J., Huntingford, C., Lenton, T. M., Sitch, S., Burke, E., Chadburn, S. E., Collins, W. J., Comyn-Platt, E., Daioglou, V., Doelman, J. C., Hayman, G., Robertson, E., van Vuuren, D., Wiltshire, A., Webber, C. P., Bastos, A., Boysen, L., Ciais, P., Devaraju, N., Jain, A. K., Krause, A., Poulter, B., and Shu, S.: Land-use emissions play a critical role in land-based mitigation for Paris climate targets, Nat. Commun., 9, 2938, https://doi.org/10.1038/s41467-018-05340-z , 2018. a
Huntzinger, D. N., Michalak, A. M., Schwalm, C., Ciais, P., King, A. W., Fang, Y., Schaefer, K., Wei, Y., Cook, R. B., Fisher, J. B., Hayes, D., Huang, M., Ito, A., Jain, A. K., Lei, H., Lu, C., Maignan, F., Mao, J., Parazoo, N., Peng, S., Poulter, B., Ricciuto, D., Shi, X., Tian, H., Wang, W., Zeng, N., and Zhao, F.: Uncertainty in the response of terrestrial carbon sink to environmental drivers undermines carbon-climate feedback predictions, Sci. Rep., 7, 1–8, https://doi.org/10.1038/s41598-017-03818-2 , 2017. a
Isaac, P., Cleverly, J., McHugh, I., Van Gorsel, E., Ewenz, C., and Beringer, J.: OzFlux data: Network integration from collection to curation, Biogeosciences, 14, 2903–2928, https://doi.org/10.5194/bg-14-2903-2017 , 2017. a
Joiner, J., Yoshida, Y., Vasilkov, A. P., Yoshida, Y., Corp, L. A., and Middleton, E. M.: First observations of global and seasonal terrestrial chlorophyll fluorescence from space, Biogeosciences, 8, 637–651, https://doi.org/10.5194/bg-8-637-2011 , 2011. a , b
Joiner, J., Guanter, L., Lindstrot, R., Voigt, M., Vasilkov, A. P., Middleton, E. M., Huemmrich, K. F., Yoshida, Y., and Frankenberg, C.: Global monitoring of terrestrial chlorophyll fluorescence from moderate-spectral-resolution near-infrared satellite measurements: methodology, simulations, and application to GOME-2, Atmos. Meas. Tech., 6, 2803–2823, https://doi.org/10.5194/amt-6-2803-2013 , 2013. a
Joiner, J., Yoshida, Y., Guanter, L., and Middleton, E. M.: New methods for the retrieval of chlorophyll red fluorescence from hyperspectral satellite instruments: Simulations and application to GOME-2 and SCIAMACHY, Atmos. Meas. Tech., 9, 3939–3967, https://doi.org/10.5194/amt-9-3939-2016 , 2016. a
Joiner, J., Yoshida, Y., Zhang, Y., Duveiller, G., Jung, M., Lyapustin, A., Wang, Y., and Tucker, C. J.: Estimation of terrestrial global gross primary production (GPP) with satellite data-driven models and eddy covariance flux data, Remote Sens., 10, 1–38, https://doi.org/10.3390/rs10091346 , 2018. a , b , c , d , e
Köhler, P., Frankenberg, C., Magney, T. S., Guanter, L., Joiner, J., and Landgraf, J.: Global Retrievals of Solar-Induced Chlorophyll Fluorescence With TROPOMI: First Results and Intersensor Comparison to OCO-2, Geophys. Res. Lett., 45, 10456–10463, https://doi.org/10.1029/2018GL079031 , 2018. a , b , c
Kong, Z. H., Stringer, L. C., Paavola, J., and Lu, Q.: Situating China in the global effort to combat desertification, Land, 10, 1–22, https://doi.org/10.3390/land10070702 , 2021. a
Kooreman, M. L., Boersma, K. F., van Schaik, E., van Versendaal, R., Cacciari, A., and Tuinder, O. N. E.: SIFTER sun-induced vegetation fluorescence data from GOME-2A (Version 2.0), Royal Netherlands Meteorological Institute (KNMI) [data set], https://doi.org/10.21944/gome2a-sifter-v2-sun-induced-fluorescence , 2020. a , b
Li, S., Yang, S., Liu, X., Liu, Y., and Shi, M.: NDVI-based analysis on the influence of climate change and human activities on vegetation restoration in the shaanxi-gansu-ningxia region, central China, Remote Sens., 7, 11163–11182, https://doi.org/10.3390/rs70911163 , 2015. a
Li, X. and Xiao, J.: TROPOMI observations allow for robust exploration of the relationship between solar-induced chlorophyll fluorescence and terrestrial gross primary production, Remote Sens. Environ., 268, 112748, https://doi.org/10.1016/j.rse.2021.112748 , 2022. a
Lin, X., Niu, J., Berndtsson, R., Yu, X., Zhang, L., and Chen, X.: Ndvi dynamics and its response to climate change and reforestation in Northern China, Remote Sens., 12, 1–15, https://doi.org/10.3390/rs12244138 , 2020. a , b , c
Lindenmayer, D. B. and Taylor, C.: New spatial analyses of Australian wildfires highlight the need for new fire, resource, and conservation policies, P. Natl. Acad. Sci. USA, 117, 12481–12485, https://doi.org/10.1073/pnas.2002269117 , 2020. a
Liu, L., Guan, L., and Liu, X.: Directly estimating diurnal changes in GPP for C 3 and C 4 crops using far-red sun-induced chlorophyll fluorescence, Agr. Forest Meteorol., 232, 1–9, https://doi.org/10.1016/j.agrformet.2016.06.014 , 2017. a
Lymburner, L., Tan, P., McIntyre, A., Tankappan, M., and Sixsmith, J.: Dynamic Land Cover Dataset Version 2.1 [data set], https://pid.geoscience.gov.au/dataset/ga/83868 (last access: 30 September 2021), 2015. a
Madani, N., Parazoo, N. C., Kimball, J. S., Reichle, R. H., Chatterjee, A., Watts, J. D., Saatchi, S., Liu, Z., Endsley, A., Tagesson, T., Rogers, B. M., Xu, L., Wang, J. A., Magney, T., and Miller, C. E.: The Impacts of Climate and Wildfire on Ecosystem Gross Primary Productivity in Alaska, J. Geophys. Res.-Biogeo., 126, 1–14, https://doi.org/10.1029/2020JG006078 , 2021. a
Magney, T. S., Bowling, D. R., Logan, B. A., Grossmann, K., Stutz, J., Blanken, P. D., Burns, S. P., Cheng, R., Garcia, M. A., Köhler, P., Lopez, S., Parazoo, N. C., Raczka, B., Schimel, D., and Frankenberg, C.: Mechanistic evidence for tracking the seasonality of photosynthesis with solar-induced fluorescence, P. Natl. Acad. Sci. USA, 116, 11640–11645, https://doi.org/10.1073/pnas.1900278116 , 2019. a , b
Moene, A. F. and Van Dam, J. C.: Transport in the atmosphere-vegetation-soil continuum, Cambridge University Press, https://doi.org/10.1017/CBO9781139043137 , 2014. a
Monteith, J.: Solar Radiation And Productivity in Tropical Ecosystems, Society, 9, 747–766, 1972. a
Muñoz Sabater, J.: ERA5-Land hourly data from 1950 to present, Copernicus Climate Change Service (C3S) Climate Data Store (CDS) [date set], https://doi.org/10.24381/cds.e2161bac (last access: 1 September 2022), 2019. a
Porcar-Castell, A., Tyystjärvi, E., Atherton, J., Van Der Tol, C., Flexas, J., Pfündel, E. E., Moreno, J., Frankenberg, C., and Berry, J. A.: Linking chlorophyll a fluorescence to photosynthesis for remote sensing applications: Mechanisms and challenges, J. Exp. Bot., 65, 4065–4095, https://doi.org/10.1093/jxb/eru191 , 2014. a
Qu, S., Wang, L., Lin, A., Yu, D., Yuan, M., and Li, C.: Distinguishing the impacts of climate change and anthropogenic factors on vegetation dynamics in the Yangtze River Basin, China, Ecol. Indic., 108, 105724, https://doi.org/10.1016/j.ecolind.2019.105724 , 2020. a
Rogelj, J., Shindell, D., Jiang, K., Fifita, S., Forster, P., Ginzburg, V., Handa, C., Kheshgi, H., Kobayashi, S., Kriegler, E., Mundaca, L., Séférian, R., and Vilariño, M.: Mitigation Pathways Compatible with 1.5 °C in the Context of Sustainable Development. In: Global Warming of 1.5 °C, An IPCC Special Report on the impacts of global warming of 1.5 °C above pre-industrial levels and related global greenhouse gas emission pathw, IPCC special report Global Warming of 1.5 °C, 82 pp., https://www.ipcc.ch/site/assets/uploads/sites/2/2019/02/SR15_Chapter2_Low_Res.pdf (last access: 16 Juni 2023), 2018. a
Schimel, D., Stephens, B. B., and Fisher, J. B.: Effect of increasing CO 2 on the terrestrial carbon cycle, P. Natl. Acad. Sci. USA, 112, 436–441, https://doi.org/10.1073/pnas.1407302112 , 2015. a
Schurgers, G., Ahlström, A., Arneth, A., Pugh, T. A., and Smith, B.: Climate Sensitivity Controls Uncertainty in Future Terrestrial Carbon Sink, Geophys. Res. Lett., 45, 4329–4336, https://doi.org/10.1029/2018GL077528 , 2018. a
Smith, W. K., Fox, A. M., MacBean, N., Moore, D. J., and Parazoo, N. C.: Constraining estimates of terrestrial carbon uptake: new opportunities using long-term satellite observations and data assimilation, New Phytol., 225, 105–112, https://doi.org/10.1111/nph.16055 , 2020. a
Song, L., Guanter, L., Guan, K., You, L., Huete, A., Ju, W., and Zhang, Y.: Satellite sun-induced chlorophyll fluorescence detects early response of winter wheat to heat stress in the Indian Indo-Gangetic Plains, Glob. Change Biol., 24, 4023–4037, https://doi.org/10.1111/gcb.14302 , 2018. a
Stol, J. and Kitchen, M.: Tumbarumba Flux Data Release 2022_v1 (Version 1.0), Terrestrial Ecosystem Research Network [data set], https://doi.org/10.25901/esrb-5609 , 2022. a , b
Sun, H., Wang, J., Xiong, J., Bian, J., Jin, H., Cheng, W., and Li, A.: Vegetation Change and Its Response to Climate Change in Yunnan Province, China, Adv. Meteorol., 2021, 8857589, https://doi.org/10.1155/2021/8857589 , 2021. a
Sun, W., Song, X., Mu, X., Gao, P., Wang, F., and Zhao, G.: Spatiotemporal vegetation cover variations associated with climate change and ecological restoration in the Loess Plateau, Agr. Forest Meteorol., 209/210, 87–99, https://doi.org/10.1016/j.agrformet.2015.05.002 , 2015. a
Sun, Y., Frankenberg, C., Jung, M., Joiner, J., Guanter, L., Köhler, P., and Magney, T.: Overview of Solar-Induced chlorophyll Fluorescence (SIF) from the Orbiting Carbon Observatory-2: Retrieval, cross-mission comparison, and global monitoring for GPP, Remote Sens. Environ., 209, 808–823, https://doi.org/10.1016/j.rse.2018.02.016 , 2018. a
Turner, A. J., Köhler, P., Magney, T. S., Frankenberg, C., Fung, I., and Cohen, R. C.: Extreme events driving year-to-year differences in gross primary productivity across the US, Biogeosciences, 18, 6579–6588, https://doi.org/10.5194/bg-18-6579-2021 , 2021. a , b
Turner, A. J., Köhler, P., Magney, T. S., Frankenberg, C., Fung, I., and Cohen, R. C.: A double peak in the seasonality of California's photosynthesis as observed from space, Biogeosciences, 17, 405–422, https://doi.org/10.5194/bg-17-405-2020 , 2020. a
United Nations: Conference of the Parties Twenty – second session Marrakech, 7–18 November 2016 Item X of the provisional agenda, Aggregate effect of the intended nationally determined contributions: an update, 07126, 16–7126, http://unfccc.int/resource/docs/2016/cop22/eng/02.pdf (last access: 16 Juni 2023), 2016. a
Van Schaik, E., Kooreman, M. L., Stammes, P., Gijsbert Tilstra, L., Tuinder, O. N., Sanders, A. F., Verstraeten, W. W., Lang, R., Cacciari, A., Joiner, J., Peters, W., and Folkert Boersma, K.: Improved SIFTER v2 algorithm for long-term GOME-2A satellite retrievals of fluorescence with a correction for instrument degradation, Atmos. Meas. Techn., 13, 4295–4315, https://doi.org/10.5194/amt-13-4295-2020 , 2020. a , b , c , d , e , f , g
Veefkind, J. P., Aben, I., McMullan, K., Förster, H., de Vries, J., Otter, G., Claas, J., Eskes, H. J., de Haan, J. F., Kleipool, Q., van Weele, M., Hasekamp, O., Hoogeveen, R., Landgraf, J., Snel, R., Tol, P., Ingmann, P., Voors, R., Kruizinga, B., Vink, R., Visser, H., and Levelt, P. F.: TROPOMI on the ESA Sentinel-5 Precursor: A GMES mission for global observations of the atmospheric composition for climate, air quality and ozone layer applications, Remote Sens. Environ., 120, 70–83, https://doi.org/10.1016/j.rse.2011.09.027 , 2012. a
Verma, M., Schimel, D., Evans, B., Frankenberg, C., Beringer, J., Drewry, D. T., Magney, T., Marang, I., Hutley, L., Moore, C., and Eldering, A.: Effect of environmental conditions on the relationship between solar-induced fluorescence and gross primary productivity at an OzFlux grassland site, J. Geophys. Res.-Biogeo., 122, 716–733, https://doi.org/10.1002/2016JG003580 , 2017. a
Wang, S., Zhang, Y., Ju, W., Porcar-Castell, A., Ye, S., Zhang, Z., Brümmer, C., Urbaniak, M., Mammarella, I., Juszczak, R., and Folkert Boersma, K.: Warmer spring alleviated the impacts of 2018 European summer heatwave and drought on vegetation photosynthesis, Agr. Forest Meteorol., 295, 108195, https://doi.org/10.1016/j.agrformet.2020.108195 , 2020. a
Wang, S., Li, R., Wu, Y., and Zhao, S.: Effects of multi-temporal scale drought on vegetation dynamics in Inner Mongolia from 1982 to 2015, China, Ecol. Indic., 136, 108666, https://doi.org/10.1016/j.ecolind.2022.108666 , 2022. a
Yang, J. and Huang, X.: The 30 m annual land cover dataset and its dynamics in China from 1990 to 2019, Earth Syst. Sci. Data, 13, 3907–3925, https://doi.org/10.5194/essd-13-3907-2021 , 2021. a , b , c
Zhang, L. and Schwärzel, K.: China's land resources dilemma: Problems, outcomes, and options for sustainable land restoration, Sustainability, 9, 2362, https://doi.org/10.3390/su9122362 , 2017. a
Zhang, W., Wei, F., Horion, S., Fensholt, R., Forkel, M., and Brandt, M.: Global quantification of the bidirectional dependency between soil moisture and vegetation productivity, Agr. Forest Meteorol., 313, 108735, https://doi.org/10.1016/j.agrformet.2021.108735 , 2022. a
Zhang, Y. and Ye, A.: Quantitatively distinguishing the impact of climate change and human activities on vegetation in mainland China with the improved residual method, GISci. Remote Sens., 58, 235–260, https://doi.org/10.1080/15481603.2021.1872244 , 2021. a
- Introduction
- Forest loss following the eucalyptus fire in southeast Australia
- Reforestation in north-central China
- Conclusions and discussion
- Code availability
- Data availability
- Author contributions
- Competing interests
- Acknowledgements
- Financial support
- Review statement
Forest Conservation at the Crossroads? A Case Study of a Timber Producing Ejido in Quintana Roo, México
Journal Title
Journal issn, volume title, description, permanent link, collections.
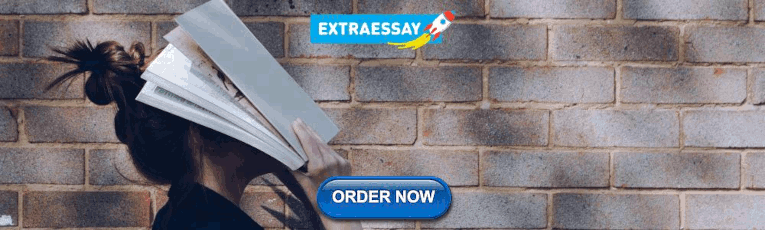
IMAGES
VIDEO
COMMENTS
A Hubbard Brook ice storm experiment. Joe Klementovich. Nestled in the White Mountains of New Hampshire is a forest. It sits in its own little valley, full of wispy birch trees, beeches, and grand ...
Chapter 2. What is the take home message from the Core Case Study "Experimenting with a Forest"? Click the card to flip 👆. Interrupting or altering natural processes can lead to negative effects. Runoff water increased by 30-40% and runoff nutrients increased by 6 to 8 times due to clear-cut logging. Click the card to flip 👆. 1 / 32.
Chapter 2 page 30 Case study's "who, what, where, why, when?" for APES winter final Learn with flashcards, games, and more — for free. ... Core Case Study: Experimenting With the Forest Who? Click the card to flip 👆 ...
Case studies: the value of research networks and long-term studies. As described above, long-term research in an ecosystem context brought considerable rigor to forest soils research. ... In 1908, the first experimental forest, the Fort Valley Experiment Station near Flagstaff, AZ, was established with a focus on ponderosa pine (Pinus ponderosa ...
Here using data from the first ecosystem-scale Free-Air CO2 Enrichment (FACE) experiment in a mature forest, we constructed a comprehensive ecosystem carbon budget to track the fate of carbon as ...
Controversial forestry experiment will be largest-ever in United States. At the Elliott State Forest in Oregon, researchers will explore how best to balance timber production with conservation. By ...
41 retrospective studies, natural experiments, large manipulations, and modeling efforts focused on ... 57 or high-intensity logging (see review in Ellison et al. 2005a for detailed case-studies). ... 80 Here, we describe the Harvard Forest Hemlock Removal Experiment (HF-HeRE), a
The Fire and Smoke Model Evaluation Experiment is a rare chance for researchers to study a large fire from start to finish 1. It is part of a broader push to gather data from wildfires in western ...
Although case studies have their place, experiments facilitate better understanding of the mechanisms influencing ecological processes, and thus should not be cast aside simply because they are difficult to do at landscape scales. ... (2011) A large-scale forest fragmentation experiment: the stability of altered forest ecosystems project. Phil ...
The present system of 80 experimental forests and ranges began in 1908. Many experimental forest are more than 50 years old. The system provides places for long-term science and management studies in major vegetation types of the 195,000,000 acres (790,000 km 2) of public land administered by the Forest Service. [4]
Study with Quizlet and memorize flashcards containing terms like Core Case Study: Experimenting with a forest, What should scientists do when making observation, experiments and models?, Peer review and more.
Where restoration sites are close to forest remnants, the framework species method works well. Framework tree species may be planted to complement ANR in small nuclei (case study 1), in larger plots (case study 2) or to form wildlife corridors (case study 3), depending on local ecological and economic conditions.
CASE STUDY Experimenting with a Forest 2.1 What Do Scientists Do? Engineering Focus: What Is Engineering? EXPLORERS AT WORK John Francis 2.2 What Is Matter? 2.3 What Is Energy? 2.4 What Are Systems? TYING IT ALL TOGETHER The Hubbard Brook Forest Experiment CHAPTER 3 ECOSYSTEM DYNAMICS EXPLORERS AT WORK Eco-Paradise at Serra Bonita, with Vitor ...
Students will know how to describe the forest community in their schoolyard and will be able to explain differences between two species in terms of one of the following: general health, location, density, carbon storage, or basal area. ... Questions arise out of scientific experiments that lead to other experiments. Data ... This case study ...
Extreme event experiments can help fill this gap, but they can be difficult and even risky to undertake. Initial results from this study provide quantitative measurements of FWD and CWD mass and nutrient transfers from forest canopies to soil in response to different intensities and frequencies of canopy ice accretion.
Ecology is a leading journal publishing original research and synthesis papers on all aspects of ecology, with particular emphasis on cutting-edge research and new concepts.
Focusing on random forests for classification we performed a study of the newly introduced idea of conservation machine learning. It is interesting to note that—case in point—our experiments ...
A middle ground between spontaneous natural regeneration and traditional tree-planting, assisted natural regeneration (ANR) is a set of ecosystem restoration techniques where local people - leaning on their knowledge of the land and on ancestral or local traditions - help trees and native vegetation naturally recover by eliminating threats to their growth and survival. This study analyzes ...
Epping forest is an ancient, deciduous woodland to the northeast of London. It is all that remains of a more extensive forest that colonised England at the end of the last ice age. The forest is approximately 19 kilometres (12 miles) long from north to south but no more than 4 kilometres (2.5 mi) from east to west at its widest point.
Study with Quizlet and memorize flashcards containing terms like What is the take home message from the Core Case Study "Experimenting with a Forest"? How much did runoff of water and nutrients increase due to clear-cut logging?, science, scientific hypothesis and more.
In the last 5 years, the 23 projects of the US Forest Services' Collaborative Forest Landscape Restoration Program have treated more than 1.45 million acres to reduce the risk of catastrophic wildfire, resulting in healthier forests with greater plant diversity and more resilience to pests and climate change. Walking through one restoration site last May, with smoke in our eyes and warm ash ...
Abstract. Reliable and robust monitoring tools are crucial to assess the effectiveness of land mitigation techniques (LMTs) in enhancing carbon uptake, enabling informed decision making by policymakers. This study, addressing one of the scientific goals of the EU Horizon 2020 Land Use Based Mitigation for Resilient Climate Pathways (LANDMARC) project, examines the feasibility of using ...
Study with Quizlet and memorize flashcards containing terms like What is the take home message from the Core Case Study "Experimenting with a Forest"? How much did runoff of water and nutrients increase due to clear-cut logging?, Science, scientific hypothesis and more.
"As part of the search for ways to combine conservation and development in the forested tropics, a number of projects to promote sustained yield forest management with a high degree of participation from local rural communities emerged during the 1980s (Richards 1991). One of the most internationally recognized initiatives has been that of the Plan Piloto Forestal (PPF) in Quintana Roo ...