Introduction to Quantitative Epidemiology
- First Online: 22 February 2022
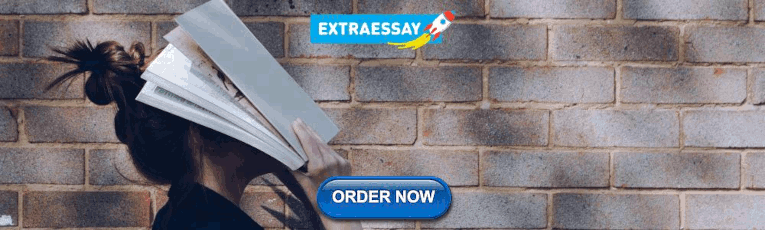
Cite this chapter
- Xinguang Chen 7
Part of the book series: Emerging Topics in Statistics and Biostatistics ((ETSB))
1063 Accesses
Epidemiology is essential for education, research, and practice in public health and medicine. As a scientific discipline, epidemiology covers four major tasks, including descriptive, etiological, translational, and methodological epidemiology. Descriptive epidemiology aims at quantifying the distribution of medical, health, or behavioral issues among people residing in a geographic area overtime; etiological epidemiology devotes to the understanding of causes and influential factors of any medical, health, or behavioral issue from onset, to progress and prognosis; translational epidemiology focuses on the transition of study findings from the descriptive and etiological epidemiology into interventions for disease prevention, treatment, and health promotion; and methodological epidemiology strives to develop new methods and innovatively use existing methods to deal with challenges in epidemiological research and practice.
Numbers speak louder than words.
This is a preview of subscription content, log in via an institution to check access.
Access this chapter
- Available as EPUB and PDF
- Read on any device
- Instant download
- Own it forever
- Compact, lightweight edition
- Dispatched in 3 to 5 business days
- Free shipping worldwide - see info
- Durable hardcover edition
Tax calculation will be finalised at checkout
Purchases are for personal use only
Institutional subscriptions
Brownson, R.C., Samet, J.M., Bensyl, D.M.: Applied epidemiology and public health: are we training the future generations appropriately? Ann. Epidemiol. 27 (2), 77–82 (2017)
Article Google Scholar
Chen, X., Chen, D.: Cusp catastrophe modeling in medical and health research. In: Chen, Wilson (eds.) Innovative Statistical Methods for Public Health Data, pp. 265–290. Springer (2015)
Chapter Google Scholar
Chen, X., Wang, K.: Geographic area-based rate as a novel indicator to enhance research and precision intervention for more effective HIV/AIDS control. Prev. Med. Rep. 5 , 301–307 (2017)
Chen, X., Yu, B.: Age and birth cohort-adjusted rates of suicide mortality among US male and female youth aged 10-19 years from 1999 to 2017. JAMA Netw. Open. 2 (9), e1911383 (2019)
Chen, X., Hu, H., Xu, X., Gong, J., Yan, Y., Li, F.: Probability sampling by connecting space with households using GIS/GPS technologies. J. Surv. Stud. Methodol. 6 , 149–168 (2018)
Cochran, W.G.: Sampling Techniques, 3rd edn. John Willey & Sons, New York (1977)
MATH Google Scholar
Doll, R., Hill, A.B.: Smoking and carcinoma of the lung. Br. Med. J. 2 (4682), 739–748 (1950)
Heckathorn, D.: Extensions of respondent-driven sampling: analyzing continuous variables and controlling for differential recruitment. Sociol. Methodol. 37 (1), 152–208 (2007)
Article MathSciNet Google Scholar
Henry, G.T.: Practical Sampling. Sage Publications, Newbury Park (1990)
Book Google Scholar
Higgins, C., Hodges, C.: Studies on prostatic cancer. 1. The effect of castration, of estrogen and of androgen injection on serum phosphatases in metastatic carcinoma of the prostate. Cancer Res. 1 , 293–297 (1941)
Google Scholar
Nelson, K.E., William, C.M.: Infectious Disease Epidemiology, 3rd edn. Jones & Bartlett Learning (2014)
Omran, A.R.: The epidemiological transition: a theory of the epidemiology of population change. Milkbank Q. 83 (4), 731–751 (2005)
Palinkas, et al.: Purposeful sampling for qualitative data collection and analysis in mixed method implantation research. Admin. Pol. Ment. Health. 42 (5), 533–544 (2015)
Pasteur, L.: The Physiological Theory of Fermentation and the Germ Theory and its Application to Medicine and Surgery Kessinger Legacy Reprint in 2010. Kessinger Publishing, LLC (1910)
Rothman, J., Greenland, S., Lash, T.L.: Modern Epidemiology, 3rd edn. Wolters Kluwer Health/Lippincott/Williams & Wilkins (2008)
Wang, K., Chen, X., Bird, V.Y., Gerke, T.A., Manini, T.M., Prosperi, M.: Association between age-related reductions in testosterone and risk of prostate cancer – an analysis of patient data with prostate diseases. Int. J. Cancer. 141 (9), 1783–1793 (2017)
Woodward, M.: Epidemiology: Study Design and Data Analysis, 3rd edn. CRC Press (2014)
Yu, B., Chen, X.: Age and birth cohort-adjusted rates of suicide mortality among US male and female youths aged 10 to 19 years from 1999 to 2017. JAMA Netw. Open. 2 (9), e1911383 (2019)
Download references
Author information
Authors and affiliations.
Department of Epidemiology, University of Florida, Gainesville, FL, USA
Xinguang Chen
You can also search for this author in PubMed Google Scholar
Rights and permissions
Reprints and permissions
Copyright information
© 2021 The Author(s), under exclusive license to Springer Nature Switzerland AG
About this chapter
Chen, X. (2021). Introduction to Quantitative Epidemiology. In: Quantitative Epidemiology. Emerging Topics in Statistics and Biostatistics . Springer, Cham. https://doi.org/10.1007/978-3-030-83852-2_1
Download citation
DOI : https://doi.org/10.1007/978-3-030-83852-2_1
Published : 22 February 2022
Publisher Name : Springer, Cham
Print ISBN : 978-3-030-83851-5
Online ISBN : 978-3-030-83852-2
eBook Packages : Mathematics and Statistics Mathematics and Statistics (R0)
Share this chapter
Anyone you share the following link with will be able to read this content:
Sorry, a shareable link is not currently available for this article.
Provided by the Springer Nature SharedIt content-sharing initiative
- Publish with us
Policies and ethics
- Find a journal
- Track your research
- Search Menu
- Sign in through your institution
- Advance articles
- Editor's Choice
- 100 years of the AJE
- Collections
- Author Guidelines
- Submission Site
- Open Access Options
- About American Journal of Epidemiology
- About the Johns Hopkins Bloomberg School of Public Health
- Journals Career Network
- Editorial Board
- Advertising and Corporate Services
- Self-Archiving Policy
- Dispatch Dates
- Journals on Oxford Academic
- Books on Oxford Academic
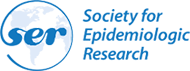
Article Contents
Abbreviations, a well-defined question, specifying the target population (and its relationship to the study sample), intermission: missing data, defining the outcome, specifying a measure of occurrence, the role of covariates, conclusions, acknowledgments.
- < Previous
A Framework for Descriptive Epidemiology
- Article contents
- Figures & tables
- Supplementary Data
Catherine R Lesko, Matthew P Fox, Jessie K Edwards, A Framework for Descriptive Epidemiology, American Journal of Epidemiology , Volume 191, Issue 12, December 2022, Pages 2063–2070, https://doi.org/10.1093/aje/kwac115
- Permissions Icon Permissions
In this paper, we propose a framework for thinking through the design and conduct of descriptive epidemiologic studies. A well-defined descriptive question aims to quantify and characterize some feature of the health of a population and must clearly state: 1) the target population, characterized by person and place, and anchored in time; 2) the outcome, event, or health state or characteristic; and 3) the measure of occurrence that will be used to summarize the outcome (e.g., incidence, prevalence, average time to event, etc.). Additionally, 4) any auxiliary variables will be prespecified and their roles as stratification factors (to characterize the outcome distribution) or nuisance variables (to be standardized over) will be stated. We illustrate application of this framework to describe the prevalence of viral suppression on December 31, 2019, among people living with human immunodeficiency virus (HIV) who had been linked to HIV care in the United States. Application of this framework highlights biases that may arise from missing data, especially 1) differences between the target population and the analytical sample; 2) measurement error; 3) competing events, late entries, loss to follow-up, and inappropriate interpretation of the chosen measure of outcome occurrence; and 4) inappropriate adjustment.
human immunodeficiency virus
North American AIDS Cohort Collaboration on Research and Design
Editor’s note: An invited commentary on this article appears on page 2071, and the authors’ response appears on page 2073.
Epidemiologic questions arguably exist on a continuum from purely descriptive to purely causal. To be concise, we ignore prediction questions here. There are several frameworks intended to help guide causal analyses ( 1 , 2 ), but the literature on theoretical and practical guidance for conducting descriptive analyses is limited. Here we present a framework for conducting descriptive epidemiologic studies. Many, if not all, of the considerations discussed in this framework apply to estimation of valid causal effects in a population, although they may be frequently overlooked. Where there may be differences in analytical decisions depending on the type of study question, we highlight them. We summarize guidance provided herein in Table 1 in the form of a checklist modeled after the Strengthening the Reporting of Observational Studies in Epidemiology (STROBE) guidelines ( 3 ).
Items That Should Be Included in Reports of Descriptive Studies
We define a descriptive epidemiologic question as one that aims to quantify some feature of the health of a population and, often, to characterize the distribution of that feature across the population. The estimand for causal analyses is a contrast of potential outcomes in a single population, where the potential outcomes are those we would expect to observe under some hypothetical intervention ( 1 , 4 – 7 ). The fundamental problem of causal inference is that we cannot observe all of these potential outcomes ( 8 ). The estimand for descriptive analyses is a function of the outcomes that occurred for everyone in the target population. The estimation challenge for descriptive analyses is that we may not completely observe all of the actual outcomes. A descriptive analysis might be cross-sectional or longitudinal; it might concern a dichotomous, categorical, or continuous outcome; and it might attempt to summarize the outcome in any number of ways (e.g., median time to some event, mean value, etc.). While much discussion focuses on the most common scenarios (e.g., dichotomous outcomes), this framework is intended to be applied to descriptive analyses for any combination of study designs, outcomes, and estimands.
We start with the premise that good epidemiologic questions are impactful and well-defined. An impactful question, if answered, would lead to knowledge that could inform action in the population it concerns ( 7 ). A well-defined question should be stated with enough specificity and clarity that answering it is at least theoretically possible.
A well-defined research question (causal or descriptive) states: 1) the target population, characterized by person and place, and anchored in time; 2) the outcome, event, or health state or characteristic; and 3) the measure of occurrence that will be used to summarize the outcome (e.g., incidence, prevalence, average time to event, etc.). A causal question requires specifying additional components, such as exposures and covariates that are thought to be confounders, effect modifiers, or mediators. For descriptive questions, consideration of additional variables is optional, but if auxiliary variables will be considered, a well-defined descriptive question will 4) prespecify any other variables of interest and how they will be considered (e.g., to characterize the population, as a stratification factor to characterize the outcome distribution, or as a “nuisance” variable that we would like to adjust for or standardize over). For a descriptive question, indiscriminate adjustment for these other variables can lead to uninterpretable results that may mislead ( 9 ); as such, researchers should be clear as to the purpose of adjustment in descriptive studies, understand the implications of such adjustments, and be cautious in interpreting adjusted statistics ( 10 ).
Example : We illustrate application of this framework to description of one portion of the human immunodeficiency virus (HIV) care continuum ( 11 ): What was the prevalence of viral suppression on December 31, 2019, among adults living with HIV who had been linked to HIV care (i.e., saw a clinician who was aware of their HIV status and had the ability to prescribe antiretroviral therapy) in the United States? We will explore specific components of this question to make it more well-defined (and tie those components to analytical decisions) below.
For a descriptive question, we define the target population as the group in which we would like to characterize the distribution of the outcome. The choice of target population is directly linked to the purpose of asking the question. The target population might be, for example, the population for which we will be providing public health services. The target population is not necessarily enumerated (in contrast to a cohort or a sample), but we do need to be able to define membership in terms of person, place, and time (here, time is used to define membership in the target population and does not relate directly to measurement of the outcome). For our example question, the target population is everyone living in the United States ( place ) who was aged ≥18 years, was infected and diagnosed with HIV, and attended ≥1 clinical visit for HIV care with a clinician who was aware of their infection and could prescribe antiretroviral medication ( person ) before December 31, 2019, and was alive through December 31, 2019 ( time ).
A well-defined question specifies the target population a priori. When data are available on a full census of the target population (e.g., through administrative records or public health surveillance), no sampling is needed. However, when data on the entire population cannot be obtained, we rely on data from a sample of the target population or a population that we hope is sufficiently representative of the target population with respect to both measured and unmeasured characteristics. The study sample is the enumerated set of individuals whose information is captured in a data set, among whom we attempt to measure occurrence of the outcome (after inclusion and exclusion criteria have been applied, if data were not collected using these criteria (e.g., administrative data)). Many descriptive and causal questions are answered using convenience samples without a clear sampling frame (e.g., people recruited using Web-based surveys, frequent clinic attendees, or people who sought medical care in a particular hospital system) and implicitly assume that the study sample is a random sample (perhaps conditional on covariates with known sampling probabilities) of the target population. Achieving a representative sample may involve considerable work and may be very resource-intensive ( 12 ). However, use of convenience samples often results in study samples that are different from the target population in unmeasurable ways, particularly when subjects must actively seek out or opt into participation ( 13 ).
On the topic of sampling and selection, it is also useful to define the analytical sample as a proper subset of the study sample in which disease occurrence is measured given practical limitations (e.g., excluding individuals in the study sample who are missing information on the outcome). We might use information from the analytical sample to attempt to quantify disease occurrence in the study sample, but we must rely on assumptions to do so (e.g., assuming data are missing at random and imputing missing data or reweighting study participants with complete data). For valid inferences, the incidence of the outcome in the sample must be able to stand in for the incidence in the target population. Here, the “sample” is either the analytical sample or the study sample represented by the analytical sample after any attempts to handle missing data. Given the many practical challenges enumerated above, the samples we rely on in our studies are rarely representative of the target population. If the distribution of risk factors for the health state differs between the study sample and the target population, we have a lack of generalizability ( 14 – 16 ); the absolute value (risk, prevalence, rate) of the outcome in the sample will differ from what we would have observed in the target population. Without applying quantitative approaches to generalize data from the sample to the target population, descriptive results will be biased. Except in special cases (e.g., when the selected estimand is the one scale on which effect measure modification is absent), if absolute measures differ between the sample and the target, most contrasts of the outcome across exposure groups in the sample will also be biased for the same contrasts in the target population (causal results will be biased) ( 14 – 16 ). If the underlying joint distribution of all causes of the outcome differs between the analytical sample and the study sample, we have selection bias ( 17 , 18 ). To recover an estimand relevant to the target population from an analytical sample with a different distribution of causes of the outcome, stratification and standardization methods may be appropriate.
Example : Recall that the target population is everyone living in the United States who had been linked to clinical care for HIV before December 31, 2019. There is mandated reporting in the United States of new HIV diagnoses and HIV viral load test results to public health surveillance agencies under national notifiable disease regulations, and the Centers for Disease Control and Prevention aggregates these data from all states and dependent areas. This might seem like a census of the target population. However, despite these mandates, not all diagnoses are reported, and people who move across state lines may be double-counted because of challenges with deduplication. Thus, the number of people with HIV infection may be inaccurate. Additionally, data rely on HIV viral load and CD4 cell-count laboratory tests as a proxy for clinical visits, and the proxy is imperfect ( 19 , 20 ); thus, we cannot accurately apply the second inclusion criterion for target population membership: linkage to clinical care. Alternatively, we might use data from the North American AIDS Cohort Collaboration on Research and Design (NA-ACCORD) ( 21 ) or another clinical cohort of people with HIV who have been linked to care. However, clinical cohort studies are often nested within academic medical centers, where the quality of care and wraparound services may differ (and thus the probability of the outcome, viral suppression, may differ), and may have stricter enrollment criteria (to preserve study resources) than we have used to define linkage to care for our target population.
There are other options for study samples we might try to leverage. We might even choose to estimate the parameter of interest in multiple samples and triangulate the results. The point is that there is rarely a single, perfect, existing study sample that can stand in for the target population. Therefore, if we wish to use existing data, identifying ways in which the study sample and the target population differ provides a framework for thinking about sources of bias and how we might adjust the estimate for better inferences.
A theme of many threats to descriptive and causal epidemiologic inference is that they can often be cast as missing-data problems ( 22 ). The ideal data set for answering our descriptive epidemiologic question includes a row for everyone in the target population and columns with values for the outcome and any covariates of interest. When the study sample is not a census of the target population, anyone in the target population who is not in the study sample will have missing data in some, if not all, columns. Indeed, without a clear sampling frame, we do not even know how many rows are missing from our ideal data set (and we cannot quantify the amount of missing data from this ideal study). Analyzing the study sample as if it were a random sample of the target population is akin to assuming that data are missing completely at random. If, instead, it is plausible to assume that data are missing at random conditional on covariates that are available for target population members who were not selected for the study sample, we could reweight or standardize the study sample to represent the full target population.
Example : The surveillance data include everyone in the target population (age ≥18 years, alive, diagnosed with HIV, and ≥1 HIV care visit before December 31, 2019), but they also include some people who are not in the target population (they include people who did not make ≥1 HIV care visit with a clinician who might prescribe antiretroviral medications), and we are unable to definitively identify people in the surveillance data who do not meet the inclusion criteria for the target population (we have to rely on laboratory tests as a proxy for clinical visits) ( 19 ). However, the surveillance data likely are closer to representing the target population than the NA-ACCORD data (which do not include everyone in the target population, although they do not include anyone who should be excluded from the target population). Therefore, we might use surveillance data for our primary analyses, but we might conduct secondary analyses that leverage the relative strengths of the different study samples and, for example, reweight NA-ACCORD data that include visits to resemble the target population implied by the surveillance data.
To describe the occurrence, frequency, or relative frequency of an outcome, we need an unambiguous definition of that outcome, and we must be able to apply that definition in our data. In the absence of a gold standard or the ability to apply that gold standard due to data or resource constraints, we must understand how imperfect sensitivity and specificity might affect our results. Measurement error has previously been described as a missing-data problem ( 22 ) in which the true outcome is missing and we overwrite that missing value with a mismeasured outcome. To the extent to which the mismeasured outcome is a poor substitute for the true outcome, our inferences will be biased.
Example : Our outcome is “viral suppression” on December 31, 2019, but there is no single, standard threshold for suppression. Prior studies have used plasma HIV RNA levels of <20, <50, <200, or <400 copies/mL ( 23 ). Lower thresholds will result in a lower estimate of the prevalence of viral suppression; for example, in an HIV clinical cohort in Baltimore, Maryland, the proportion of patients estimated to have a suppressed viral load in a given year from 2010 to 2018 was 75% if the threshold for suppression was set at <20 copies/mL but 89% if the threshold was set at <400 copies/mL ( 24 ). Failure to suppress viral load below a lower threshold may also be a more sensitive indicator of subsequent morbidity and mortality ( 24 – 28 ), but suppression below a higher threshold is more relevant as an indicator of an individual’s transmission potential ( 29 , 30 ), so our choice of threshold may depend on how our results will be used. Additionally, not everyone in either of our candidate study samples will have had a viral load measurement on December 31, 2019, exactly. Typically, researchers accept viral loads measured within a time window around some key date as indicative of the viral load on that key date. We must decide how wide a window we are willing to use to answer our question. The width we are willing to tolerate might depend on how frequently we anticipate viral load changes in the population. A wider window risks assigning a viral load value to December 31 that is inaccurate because viral load has changed since measurement, while a narrower window will result in a larger proportion of the cohort with a missing viral-load value.
We have multiple options for measures of occurrence, and like the proverbial blind men feeling the elephant, our choice of measure of occurrence might give us only part of the complete picture about the distribution of the outcome in the target population. Incidence tells us something about how frequently an event occurs over time. There are multiple measures of incidence; in the interest of space, we will restrict our discussion to risks and rates. If individuals are not followed over time and the event can recur, it may be difficult to distinguish the number of affected individuals from the number of events. Prevalent outcomes are often not of interest in causal investigations, as temporality is more challenging to determine and reverse causation is a potential problem. In addition, survival bias might affect results when considering prevalent exposures ( 31 , 32 ). Finally, prevalence is a function of the incidence of the condition and its duration, such that, if incidence is what is relevant to the question at hand, prevalence might be a misleading proxy. However, for descriptive questions designed to inform public-health planning for secondary or tertiary prevention measures, prevalence might be the most relevant measure of occurrence, as it reflects the population of people who might access those services.
Risk (the proportion of people free from disease at baseline who develop the outcome during the study period) is the foundation of many causal epidemiologic studies ( 33 ), particularly as the target trial framework ( 1 ) has gained in popularity. Risk is arguably the most easily interpretable measure of disease occurrence for the general public ( 33 ). We discuss rates (the number of events divided by a sum of person-time) as an alternative measure of incidence in a few paragraphs. Two complications for obtaining valid estimates of either measure of incidence, however, are competing events and incompletely observed person-time (left-truncation and right-censoring).
Competing events are events that preclude the event of interest from occurring and are theoretical if not practical problems for all outcomes other than all-cause mortality ( 34 ). In the presence of competing events, we have the option to report the conditional or unconditional risk (i.e., cumulative incidence function) ( 35 ). The conditional risk is the proportion of people free from disease at baseline that we would expect to develop the outcome during the study period if all competing events were prevented without changing the hazard of the event of interest; it is the risk “conditional” on removal of the competing event. It is estimated by censoring persons who experience a competing event and is the first and sometimes only estimand of risk that students of epidemiology are taught ( 36 ). It is also implied by the exponential formula for converting rates to risks. However, complete removal of the competing event is a hypothetical intervention, and the conditional risk is the risk under that often-infeasible intervention. If our goal is to describe the world as it exists, absent hypothetical interventions, the cumulative incidence function is recommended when the number of competing events is nontrivial ( 37 ). The cumulative incidence function (or, as is implied but is a less commonly used term, the unconditional risk) is the proportion of people free from disease at baseline who would develop the outcome of interest during the study period in the real world in which a competing event might remove them from follow-up and preclude them from ever developing the outcome of interest.
Risks can be calculated in the presence of late entries (left-truncation) and loss to follow-up (right-censoring) under strong assumptions about independence between entering/leaving the study and risk of the outcome ( 38 , 39 ). Left-truncation and right-censoring impute outcomes for people who did not survive to enroll in the study sample and for people who are censored ( 38 ). We can adjust for possible associations between censoring and the outcome (and resultant selection bias) using inverse probability of censoring weights ( 40 ). However, the resultant risks are interpretable as the risk that would have been observed if no one were lost to follow-up (a hypothetical intervention), and will be different from the natural course if loss to follow-up was associated with the outcome in ways not captured by covariates in the weight model or if loss to follow-up itself directly altered the risk of the outcome ( 18 , 40 ).
Finally, rates may occasionally be a useful measure of incidence as an alternative to risks, especially for descriptive studies. Risks are only defined relevant to a population free of, and biologically at risk for, the outcome at a particular time origin. When we would like to describe incidence across a time metric along which not all people were biologically at risk at the time origin, rates can appropriately exclude person-time not at risk and allow for reporting of smoothed incidence estimates. For example, when describing temporal trends for the incidence of HIV diagnoses since the beginning of the epidemic in the 1980s, there will be people who were not born (not at risk for the outcome) in the 1980s who should be counted in the target population in the 2010s. Perhaps in an idealized descriptive study, we would report the daily risk of HIV diagnosis restricted to people who were alive and at risk for HIV diagnosis at the start of each day. However, across 3 decades this may be computationally intensive and impractical given the granularity of data collection and reporting. We might instead report weekly, monthly, or yearly HIV diagnosis risk, but the wider the time interval across which we measure risk becomes, the greater the number of people in our target population who are not at risk at the start of the interval. How should we treat people born in December 1990 when calculating the risk of HIV diagnosis in 1990? In contrast, if we are willing to assume that the rate of HIV diagnosis across a calendar year is approximately constant, or if we assume that the average rate is a reasonable representation of the incidence in that year, rates could appropriately exclude person-time in which people are not biologically at risk. The assumption of a constant rate or the acceptability of an average rate for answering the study question should be plausible across the time intervals chosen, or time should be further discretized. Another benefit of rates is that they are straightforward to estimate when we do not have individual-level data, which is more common in descriptive analyses than in causal or predictive epidemiologic analyses. For example, rates are the standard measure of incidence used for notifiable diseases, where health departments count case reports to get the numerator and use midyear census estimates for the denominator.
Example : We have clearly specified in our research question that we are interested in the prevalence of viral suppression on December 31, 2019. People in our study sample with no viral load measurement in 2019 are lost to follow-up. Viral suppression is influenced by access to health care and is only possible if people are receiving antiretroviral therapy (except, in rare cases, for elite controllers) ( 41 ). In this setting, people who are lost to follow-up may have transferred to another clinic and may still be receiving treatment (if we are using NA-ACCORD data) or may have moved out of the jurisdiction (if we are using surveillance data), and we might assume that they have the same probability of viral suppression as people with a viral load measurement (censoring is appropriate; equivalently, we can restrict analyses to people with a measured viral load) ( 24 ). Alternatively, people who do not have a viral load measurement may have dropped out of clinical care and may not have access to antiretroviral therapy. The probability of viral suppression among these individuals is near 0 (we might think of loss to follow-up as a competing event and assign a value of “not suppressed” to persons who are lost to follow-up) ( 42 ). Understanding the assumptions and implications of different analytical decisions for these people is critical for making the right inference about the prevalence of the outcome.
When describing the prevalence or incidence of an outcome, we sometimes want to characterize the people who got the outcome according to covariates. Alternatively, we may want to account for nuisance variables, such as factors that differ between the study sample and the target population or between groups we plan to stratify by. When characterizing groups with the highest incidence of the outcome, bivariate results can make it challenging to understand how covariates interact to determine the distribution of disease. For example, if the prevalence of viral suppression is lower for cisgender women than for cisgender men and lower for Black patients than for White patients ( 43 ), what would we expect to see regarding the prevalence of viral suppression for cisgender White women relative to cisgender Black men? Stratifying on multiple variables simultaneously might be helpful in this setting, or we may want to employ theoretical models (e.g., conceptual frameworks for how variables influence risk of the outcome) or statistical strategies (e.g., supervised machine learning) to identify the most important variables if there are not enough data to stratify on all variables of interest. Conversely, when trying to understand whether one covariate is associated with the distribution of disease independently or merely because of its correlation with another covariate, a common approach is to put all covariates into a single model. However, this approach can lead to incorrect interpretations of the results and inappropriate recommendations for actions ( 44 ). Adjustment implies an intervention on the data and a distortion of reality—for example, “Would Black people still have lower prevalence of viral suppression if they had the same distribution of HIV acquisition risk factors as White people?”. Inappropriate adjustment may understate the magnitude of disparities ( 45 ) and adjusted statistics are prone to be interpreted causally, which could lead to inappropriate recommendations ( 9 ). We endorse reporting and primary interpretation of unadjusted results for descriptive studies and clear justification and proper interpretation in cases where adjustments are made.
Descriptive epidemiologic studies seek to characterize what is happening in the world to inform public health priorities, target interventions, and occasionally contrast with counterfactual scenarios to estimate intervention effects ( 46 , 47 ). Descriptive studies have value in their own right and not merely as stepping stools toward causal inference. Characterizing what is happening in the world requires that we be very clear about the particular slice of the world and the specific outcome we hope to study. Generalizability and selection biases can bias descriptive studies when study participation is associated with the outcome. Measurement error can bias descriptive studies when we do not use, or there is no gold-standard measure of, the outcome. Different measures of occurrence will provide different pictures of what is happening in the world. Censoring people who have a competing event or adjusting for covariates implies interventions on the data such that the results are a distorted version of reality. These are all basic epidemiologic principles that also affect the success of our attempts at causal effect estimation. Performing rigorous descriptive studies that accurately estimate a parameter of interest and are interpretable to clinicians and policy-makers will improve public health.
Author affiliations: Department of Epidemiology, Bloomberg School of Public Health, Johns Hopkins University, Baltimore, Maryland, United States (Catherine R. Lesko); Departments of Epidemiology and Global Health, School of Public Health, Boston University, Boston, Massachusetts, United States (Matthew P. Fox); and Department of Epidemiology, Gillings School of Global Public Health, University of North Carolina at Chapel Hill, Chapel Hill, North Carolina, United States (Jessie K. Edwards).
This work was supported by grants K01 AA028193, K01 AI125087, and R01 AI157758 from the National Institutes of Health.
Conflict of interest: none declared.
Hernán MA , Robins JM . Using big data to emulate a target trial when a randomized trial is not available . Am J Epidemiol . 2016 ; 183 ( 8 ): 758 – 764 .
Google Scholar
Petersen ML , van der Laan MJ . Causal models and learning from data: integrating causal modeling and statistical estimation . Epidemiology . 2014 ; 25 ( 3 ): 418 – 426 .
von Elm E , Altman DG , Egger M , et al. The Strengthening the Reporting of Observational Studies in Epidemiology (STROBE) Statement: guidelines for reporting observational studies . Int J Surg . 2014 ; 12 ( 12 ): 1495 – 1499 .
Robins JM . Data, design, and background knowledge in etiologic inference . Epidemiology . 2001 ; 12 ( 3 ): 313 – 320 .
Rubin DB . The design versus the analysis of observational studies for causal effects: parallels with the design of randomized trials . Stat Med . 2007 ; 26 ( 1 ): 20 – 36 .
Petersen ML . Commentary: applying a causal road map in settings with time-dependent confounding . Epidemiology . 2014 ; 25 ( 6 ): 898 – 901 .
Fox MP , Edwards JK , Platt R , et al. The critical importance of asking good questions: the role of epidemiology doctoral training programs . Am J Epidemiol . 2020 ; 189 ( 4 ): 261 – 264 .
Holland PW . Statistics and causal inference . J Am Stat Assoc . 1986 ; 81 ( 396 ): 945 – 960 .
Tennant PWG , Murray EJ . The quest for timely insights into COVID-19 should not come at the cost of scientific rigor . Epidemiology . 2021 ; 32 ( 1 ):e2.
Kaufman JS . Statistics, adjusted statistics, and maladjusted statistics . Am J Law Med . 2017 ; 43 ( 2-3 ): 193 – 208 .
Gardner EM , McLees MP , Steiner JF , et al. The spectrum of engagement in HIV care and its relevance to test-and-treat strategies for prevention of HIV infection . Clin Infect Dis . 2011 ; 52 ( 6 ): 793 – 800 .
Lee KK , Fitts MS , Conigrave JH , et al. Recruiting a representative sample of urban South Australian Aboriginal adults for a survey on alcohol consumption . BMC Med Res Methodol . 2020 ; 20 ( 1 ): 183 .
Offord C . How (not) to do an antibody survey for SARS-CoV-2. Scientist . https://www.the-scientist.com/news-opinion/how-not-to-do-an-antibody-survey-for-sars-cov-2-67488 . Published April 28, 2020 . Accessed April 8, 2022 .
Lesko CR , Buchanan AL , Westreich D , et al. Generalizing study results: a potential outcomes perspective . Epidemiology . 2017 ; 28 ( 4 ): 553 – 561 .
Dahabreh IJ , Robertson SE , Tchetgen EJ , et al. Generalizing causal inferences from individuals in randomized trials to all trial-eligible individuals . Biometrics . 2019 ; 75 ( 2 ): 685 – 694 .
Cole SR , Stuart EA . Generalizing evidence from randomized clinical trials to target populations: the ACTG 320 Trial . Am J Epidemiol . 2010 ; 172 ( 1 ): 107 – 115 .
Westreich D . Berkson’s bias, selection bias, and missing data . Epidemiology . 2012 ; 23 ( 1 ): 159 – 164 .
Hernán MA . Invited commentary: selection bias without colliders . Am J Epidemiol . 2017 ; 185 ( 11 ): 1048 – 1050 .
Rebeiro PF , Althoff KN , Lau B , et al. Laboratory measures as proxies for primary care encounters: implications for quantifying clinical retention among HIV-infected adults in North America . Am J Epidemiol . 2015 ; 182 ( 11 ): 952 – 960 .
Lesko CR , Sampson LA , Miller WC , et al. Measuring the HIV care continuum using public health surveillance data in the United States . J Acquir Immune Defic Syndr . 2015 ; 70 ( 5 ): 489 – 494 .
Gange SJ , Kitahata MM , Saag MS , et al. Cohort profile: the North American AIDS Cohort Collaboration on Research and Design (NA-ACCORD) . Int J Epidemiol . 2007 ; 36 ( 2 ): 294 – 301 .
Edwards JK , Cole SR , Westreich D . All your data are always missing: incorporating bias due to measurement error into the potential outcomes framework . Int J Epidemiol . 2015 ; 44 ( 4 ): 1452 – 1459 .
McMahon JH , Elliott JH , Bertagnolio S , et al. Viral suppression after 12 months of antiretroviral therapy in low- and middle-income countries: a systematic review . Bull World Health Organ . 2013 ; 91 ( 5 ): 377 – 385E .
Lesko CR , Chander G , Moore RD , et al. Variation in estimated viral suppression associated with the definition of viral suppression used . AIDS . 2020 ; 34 ( 10 ): 1519 – 1526 .
Hermans LE , Moorhouse M , Carmona S , et al. Effect of HIV-1 low-level viraemia during antiretroviral therapy on treatment outcomes in WHO-guided South African treatment programmes: a multicentre cohort study . Lancet Infect Dis . 2018 ; 18 ( 2 ): 188 – 197 .
Elvstam O , Medstrand P , Yilmaz A , et al. Virological failure and all-cause mortality in HIV-positive adults with low-level viremia during antiretroviral treatment . PLoS One . 2017 ; 12 ( 7 ):e0180761.
Antiretroviral Therapy Cohort Collaboration , Vandenhende MA , Ingle S , et al. Impact of low-level viremia on clinical and virological outcomes in treated HIV-1-infected patients . AIDS . 2015 ; 29 ( 3 ): 373 – 383 .
Laprise C , de Pokomandy A , Baril J-G , et al. Virologic failure following persistent low-level viremia in a cohort of HIV-positive patients: results from 12 years of observation . Clin Infect Dis . 2013 ; 57 ( 10 ): 1489 – 1496 .
Lesko CR , Lau B , Chander G , et al. Time spent with HIV viral load >1500 copies/mL among persons engaged in continuity HIV care in an urban clinic in the United States, 2010–2015 . AIDS Behav . 2018 ; 22 ( 11 ): 3443 – 3450 .
Quinn TC , Wawer MJ , Sewankambo N , et al. Viral load and heterosexual transmission of human immunodeficiency virus type 1. Rakai Project Study Group . N Engl J Med . 2000 ; 342 ( 13 ): 921 – 929 .
Prentice RL , Chlebowski RT , Stefanick ML , et al. Estrogen plus progestin therapy and breast cancer in recently postmenopausal women . Am J Epidemiol . 2008 ; 167 ( 10 ): 1207 – 1216 .
Lund JL , Richardson DB , Stürmer T . The active comparator, new user study design in pharmacoepidemiology: historical foundations and contemporary application . Curr Epidemiol Rep . 2015 ; 2 ( 4 ): 221 – 228 .
Cole SR , Hudgens MG , Brookhart MA , et al. Risk . Am J Epidemiol . 2015 ; 181 ( 4 ): 246 – 250 .
Lau B , Cole SR , Gange SJ . Competing risk regression models for epidemiologic data . Am J Epidemiol . 2009 ; 170 ( 2 ): 244 – 256 .
Edwards JK , Hester LL , Gokhale M , et al. Methodologic issues when estimating risks in pharmacoepidemiology . Curr Epidemiol Rep . 2016 ; 3 ( 4 ): 285 – 296 .
Rothman KJ , Lash TL , VanderWeele TJ , et al. Measures of occurrence. In: Modern Epidemiology . 4th ed. Philadelphia, PA : Wolters Kluwer N.V. ; 2021 : 53 – 77 .
Google Preview
Cole SR , Lau B , Eron JJ , et al. Estimation of the standardized risk difference and ratio in a competing risks framework: application to injection drug use and progression to AIDS after initiation of antiretroviral therapy . Am J Epidemiol . 2015 ; 181 ( 4 ): 238 – 245 .
Cole SR , Edwards JK , Naimi AI , et al. Hidden imputations and the Kaplan-Meier estimator . Am J Epidemiol . 2020 ; 189 ( 11 ): 1408 – 1411 .
Lesko CR , Edwards JK , Cole SR , et al. When to censor? Am J Epidemiol . 2018 ; 187 ( 3 ): 623 – 632 .
Howe CJ , Cole SR , Lau B , et al. Selection bias due to loss to follow up in cohort studies . Epidemiology . 2016 ; 27 ( 1 ): 91 – 97 .
Okulicz JF , Marconi VC , Landrum ML , et al. Clinical outcomes of elite controllers, viremic controllers, and long-term nonprogressors in the US Department of Defense HIV Natural History Study . J Infect Dis . 2009 ; 200 ( 11 ): 1714 – 1723 .
Edwards JK , Lesko CR , Herce ME , et al. Gone but not lost: implications for estimating HIV care outcomes when loss to clinic is not loss to care . Epidemiology . 2020 ; 31 ( 4 ): 570 – 577 .
Centers for Disease Control and Prevention . Monitoring Selected National HIV Prevention and Care Objectives by Using HIV Surveillance Data—United States and 6 Dependent Areas, 2019 . ( HIV Surveillance Supplemental Report , vol. 26, no. 2) . Atlanta, GA : Centers for Disease Control and Prevention ; 2021 . https://www.cdc.gov/hiv/pdf/library/reports/surveillance/cdc-hiv-surveillance-report-vol-26-no-2.pdf . Accessed November 29, 2021 .
Westreich D , Greenland S . The table 2 fallacy: presenting and interpreting confounder and modifier coefficients . Am J Epidemiol . 2013 ; 177 ( 4 ): 292 – 298 .
Zalla LC , Martin CL , Edwards JK , et al. A geography of risk: structural racism and COVID-19 mortality in the United States . Am J Epidemiol . 2021 ; 190 ( 8 ): 1439 – 1446 .
Westreich D . From exposures to population interventions: pregnancy and response to HIV therapy . Am J Epidemiol . 2014 ; 179 ( 7 ): 797 – 806 .
Edwards JK , Cole SR , Lesko CR , et al. An illustration of inverse probability weighting to estimate policy-relevant causal effects . Am J Epidemiol . 2016 ; 184 ( 4 ): 336 – 344 .
- epidemiology
- epidemiologic studies
- stratification
- outcome measures
- measurement error
- missing data
- viral suppression
- data analysis
Email alerts
Citing articles via, looking for your next opportunity.
- Recommend to your Library
Affiliations
- Online ISSN 1476-6256
- Print ISSN 0002-9262
- Copyright © 2024 Johns Hopkins Bloomberg School of Public Health
- About Oxford Academic
- Publish journals with us
- University press partners
- What we publish
- New features
- Open access
- Institutional account management
- Rights and permissions
- Get help with access
- Accessibility
- Advertising
- Media enquiries
- Oxford University Press
- Oxford Languages
- University of Oxford
Oxford University Press is a department of the University of Oxford. It furthers the University's objective of excellence in research, scholarship, and education by publishing worldwide
- Copyright © 2024 Oxford University Press
- Cookie settings
- Cookie policy
- Privacy policy
- Legal notice
This Feature Is Available To Subscribers Only
Sign In or Create an Account
This PDF is available to Subscribers Only
For full access to this pdf, sign in to an existing account, or purchase an annual subscription.
Thank you for visiting nature.com. You are using a browser version with limited support for CSS. To obtain the best experience, we recommend you use a more up to date browser (or turn off compatibility mode in Internet Explorer). In the meantime, to ensure continued support, we are displaying the site without styles and JavaScript.
- View all journals
- Explore content
- About the journal
- Publish with us
- Sign up for alerts
Epidemiology articles within Scientific Reports
Article 26 May 2024 | Open Access
Nonlinear associations between the ratio of family income to poverty and all-cause mortality among adults in NHANES study
- , Minghui Li
- & Zhenyu Zhai
Proteinuria and risk of ocular motor cranial nerve palsy: a nationwide population-based study
- , Kyungdo Han
- & Sei Yeul Oh
Article 25 May 2024 | Open Access
Association between obesity and the prevalence of dyslipidemia in middle-aged and older people: an observational study
- Chuanlei Zheng
- , Yanhong Liu
- & Qingfeng Wu
Determinants of echocardiographic epicardial adipose tissue in a general middle-aged population - The Cardiovascular Risk in Young Finns Study
- Behnoush Gustafsson
- , Suvi P. Rovio
- & Olli T. Raitakari
Article 24 May 2024 | Open Access
Body mass index is associated with clinical outcomes in idiopathic pulmonary fibrosis
- Hee-Young Yoon
- , Hoseob Kim
- & Jin Woo Song
Compliance with the 24-h Movement Guidelines for Portuguese children: differences between boys and girls
- João Martins
- , Miguel Ángel Tapia-Serrano
- & Pedro Antonio Sanchéz-Miguel
Article 23 May 2024 | Open Access
Exploring the association between cardiovascular health and bowel health
- , Mingyue Guo
- & Hong Yang
Cross-sectional study on exercise-related skin complaints among sports students at two German universities
- Karl Philipp Drewitz
- , Claudia Hasenpusch
- & Christian J. Apfelbacher
Article 22 May 2024 | Open Access
Static graph approximations of dynamic contact networks for epidemic forecasting
- Razieh Shirzadkhani
- , Shenyang Huang
- & Reihaneh Rabbany
Monitoring and evaluation of childhood stunting reduction program based on fish supplement product in North Sumatera, Indonesia
- Bens Pardamean
- , Rudi Nirwantono
- & Sarma Nursani Lumbanraja
Article 21 May 2024 | Open Access
Lifestyle and metabolic risk factors, and diabetes mellitus prevalence in European countries from three waves of the European Health Interview Survey
- Nóra Kovács
- , Balqees Shahin
- & Orsolya Varga
Article 20 May 2024 | Open Access
Validity and reliability of the Persian version of food preferences questionnaire (Persian-FPQ) in Iranian adolescents
- Zahra Heidari
- , Awat Feizi
- & Fahimeh Haghighatdoost
A person-centered approach to characterizing longitudinal ambulatory impairment in Parkinson's disease
- Farren B. S. Briggs
- , Douglas D. Gunzler
- & Steven A. Gunzler
Article 19 May 2024 | Open Access
The challenge of adopting a collaborative information system for independent healthcare workers in France: a comprehensive study
- Laurent Gaucher
- , Céline Puill
- & Frédéric Mougeot
Article 17 May 2024 | Open Access
Prevalence of hepatitis B and C viruses among migrant workers in Qatar
- Gheyath K. Nasrallah
- , Hiam Chemaitelly
- & Laith J. Abu-Raddad
Article 16 May 2024 | Open Access
Reanalysis of cluster randomised trial data to account for exposure misclassification using a per-protocol and complier-restricted approach
- Suzanne M. Dufault
- , Stephanie K. Tanamas
- & Katherine L. Anders
Self-reported depression and its risk factors among hypertensive patients, Morocco: a cross-sectional study
- Fatima Zahra Boukhari
- , Safae Belayachi
- & Touria Essayagh
Article 15 May 2024 | Open Access
Prevalence of free flap failure in mandibular osteoradionecrosis reconstruction: a systematic review and meta-analysis
- Evangelos Kostares
- , Michael Kostares
- & Maria Kantzanou
Article 14 May 2024 | Open Access
Seroprevalence of Toxoplasma gondii and Borrelia burgdorferi infections in patients with multiple sclerosis in Poland
- Agnieszka Pawełczyk
- , Katarzyna Donskow-Łysoniewska
- & Renata Welc-Falęciak
Distinct cytokine profiles in late pregnancy in Ugandan people with HIV
- Lisa M. Bebell
- , Joseph Ngonzi
- & Galit Alter
Article 11 May 2024 | Open Access
Arthritis is associated with high nutritional risk among older Canadian adults from the Canadian Longitudinal Study on Aging
- Roxanne Bennett
- , Thea A. Demmers
- & Lisa Kakinami
Country-report pattern corrections of new cases allow accurate 2-week predictions of COVID-19 evolution with the Gompertz model
- I. Villanueva
- , D. Conesa
- & E. Alvarez-Lacalle
Examining variations in body composition among patients with colorectal cancer according to site and disease stage
- Mayra Laryssa da Silva Nascimento
- , Nithaela Alves Bennemann
- & Ana Paula Trussardi Fayh
Article 10 May 2024 | Open Access
Effects of cooking with solid fuel on hearing loss in Chinese adults—Based on two cohort studies
- Xue-yun Mao
- , Miao Zheng
- & Wei-jun Zheng
The relationship between oxidative balance score and erectile dysfunction in the U.S. male adult population
- Mutong Chen
- , Zhongfu Zhang
- & Bentao Shi
Article 08 May 2024 | Open Access
Longitudinal patterns of natural hazard exposures and anxiety and depression symptoms among young adults in four low- and middle-income countries
- Ilan Cerna-Turoff
- , Joan A. Casey
- & Daniel Malinsky
Article 06 May 2024 | Open Access
A Bayesian spatio-temporal model of COVID-19 spread in England
- Xueqing Yin
- , John M. Aiken
- & Jonathan L. Bamber
The association of hypertension among married Indian couples: a nationally representative cross-sectional study
- Jithin Sam Varghese
- , Arpita Ghosh
- & Shivani A. Patel
Article 03 May 2024 | Open Access
Statistical analysis of three data sources for Covid-19 monitoring in Rhineland-Palatinate, Germany
- Maximilian Pilz
- , Karl-Heinz Küfer
- & Neele Leithäuser
Ideal cardiovascular health index and high-normal blood pressure in elderly people: evidence based on real-world data
- Yongcheng Ren
- , Lulu Cheng
- & Pengfei Wang
Personality traits explain the relationship between psychedelic use and less depression in a comparative study
- David K. Sjöström
- , Emma Claesdotter-Knutsson
- & Petri J. Kajonius
Effect of pooled tracheal sample testing on the probability of Mycoplasma hyopneumoniae detection
- Ana Paula Serafini Poeta Silva
- , Robert Mugabi
- & Maria Jose Clavijo
Socioeconomic and geographic inequalities in antenatal and postnatal care components in India, 2016–2021
- , Sohee Jung
- & Rockli Kim
Study of defensive behavior of a venomous snake as a new approach to understand snakebite
- João Miguel Alves-Nunes
- , Adriano Fellone
- & Otavio Augusto Vuolo Marques
Article 01 May 2024 | Open Access
Zika emergence, persistence, and transmission rate in Colombia: a nationwide application of a space-time Markov switching model
- Laís Picinini Freitas
- , Dirk Douwes-Schultz
- & Kate Zinszer
Article 30 April 2024 | Open Access
Characteristics and outcomes of patients admitted to intensive care units in Uganda: a descriptive nationwide multicentre prospective study
- Patience Atumanya
- , Peter. K. Agaba
- & Cornelius Sendagire
Development and validation of a health practitioner survey on ocular allergy
- Ereeny Mikhail
- , Mohammadreza Mohebbi
- & Cenk Suphioglu
Prediction and causal inference of hyperuricemia using gut microbiota
- Yuna Miyajima
- , Shigehiro Karashima
- & Shigefumi Okamoto
Forecasting the spread of COVID-19 based on policy, vaccination, and Omicron data
- Kyulhee Han
- , Bogyeom Lee
- & Taesung Park
Association between maternal heavy metal exposure and Kawasaki Disease, the Japan Environment and Children’s Study (JECS)
- Takanori Yanai
- , Satomi Yoshida
- & Takahiko Katoh
Article 29 April 2024 | Open Access
The mediating effect of internet addiction and the moderating effect of physical activity on the relationship between alexithymia and depression
- , Liangfan Duan
- & Tiancheng Zhang
Article 27 April 2024 | Open Access
Development and preliminary validation of a prediction formula of sodium and sodium-to-potassium ratio based on multiple regression using 24-h urines
- Marina Yamagishi
- , Ribeka Takachi
- & Norie Sawada
The burden of schizophrenia in the Middle East and North Africa region, 1990–2019
- Saeid Safiri
- , Maryam Noori
- & Ali-Asghar Kolahi
Oral microbial signatures associated with age and frailty in Canadian adults
- Vanessa DeClercq
- , Robyn J. Wright
- & Morgan G. I. Langille
Article 26 April 2024 | Open Access
Changing epidemiology of parvovirus B19 in the Netherlands since 1990, including its re-emergence after the COVID-19 pandemic
- Anne Russcher
- , Michiel van Boven
- & Aloys C. M. Kroes
Vegetation index and livestock practices as predictors of malaria transmission in Nigeria
- Oluyemi Okunlola
- , Segun Oloja
- & Oyetunde Oyeyemi
No bidirectional relationship between sleep phenotypes and risk of proliferative diabetic retinopathy: a two-sample Mendelian randomization study
- & Jing Wei
Article 25 April 2024 | Open Access
Development and validation of a smartwatch algorithm for differentiating physical activity intensity in health monitoring
- , Yuchen Du
- & Xun Xu
Estimating SARS-CoV-2 infection probabilities with serological data and a Bayesian mixture model
- Benjamin Glemain
- , Xavier de Lamballerie
- & Fabrice Carrat
Article 24 April 2024 | Open Access
Assessment of using Google Trends for real-time monitoring of infectious disease outbreaks: a measles case study
- , John Cameron Lang
- & Yao-Hsuan Chen
Browse broader subjects
- Medical research
- Public health
Quick links
- Explore articles by subject
- Guide to authors
- Editorial policies


Virome Sequencing Identifies H5N1 Avian Influenza in Wastewater from Nine Cities
- Find this author on Google Scholar
- Find this author on PubMed
- Search for this author on this site
- ORCID record for Michael J. Tisza
- ORCID record for Fuqing Wu
- For correspondence: [email protected]
- Info/History
- Preview PDF
Avian influenza (serotype H5N1) is a highly pathogenic virus that emerged in domestic waterfowl in 1996. Over the past decade, zoonotic transmission to mammals, including humans, has been reported. Although human to human transmission is rare, infection has been fatal in nearly half of patients who have contracted the virus in past outbreaks. The increasing presence of the virus in domesticated animals raises substantial concerns that viral adaptation to immunologically naïve humans may result in the next flu pandemic. Wastewater-based epidemiology (WBE) to track viruses was historically used to track polio and has recently been implemented for SARS-CoV2 monitoring during the COVID-19 pandemic. Here, using an agnostic, hybrid-capture sequencing approach, we report the detection of H5N1 in wastewater in nine Texas cities, with a total catchment area population in the millions, over a two-month period from March 4 th to April 25 th , 2024. Sequencing reads uniquely aligning to H5N1 covered all eight genome segments, with best alignments to clade 2.3.4.4b. Notably, 19 of 23 monitored sites had at least one detection event, and the H5N1 serotype became dominant over seasonal influenza over time. A variant analysis suggests avian or bovine origin but other potential sources, especially humans, could not be excluded. We report the value of wastewater sequencing to track avian influenza.
Competing Interest Statement
The authors have declared no competing interest.
Funding Statement
This work was supported by S.B. 1780, 87th Legislature, 2021 Reg. Sess. (Texas 2021) (E.B., A.W.M., and J.F.P.), NIH/NIAID (Grant number U19 AI44297) (A.W.M.), Baylor College of Medicine Melnick Seed (A.W.M) and Alkek Foundation Seed (J.F.P.), and Pandemic Threat Technology Center (P.A.P.).
Author Declarations
I confirm all relevant ethical guidelines have been followed, and any necessary IRB and/or ethics committee approvals have been obtained.
I confirm that all necessary patient/participant consent has been obtained and the appropriate institutional forms have been archived, and that any patient/participant/sample identifiers included were not known to anyone (e.g., hospital staff, patients or participants themselves) outside the research group so cannot be used to identify individuals.
I understand that all clinical trials and any other prospective interventional studies must be registered with an ICMJE-approved registry, such as ClinicalTrials.gov. I confirm that any such study reported in the manuscript has been registered and the trial registration ID is provided (note: if posting a prospective study registered retrospectively, please provide a statement in the trial ID field explaining why the study was not registered in advance).
I have followed all appropriate research reporting guidelines, such as any relevant EQUATOR Network research reporting checklist(s) and other pertinent material, if applicable.
Data Availability
All data produced are available online at https://zenodo.org/doi/10.5281/zenodo.11175923 and NCBI SRA BioProject: PRJNA966185
View the discussion thread.
Thank you for your interest in spreading the word about medRxiv.
NOTE: Your email address is requested solely to identify you as the sender of this article.

Citation Manager Formats
- EndNote (tagged)
- EndNote 8 (xml)
- RefWorks Tagged
- Ref Manager
- Tweet Widget
- Facebook Like
- Google Plus One
Subject Area
- Infectious Diseases (except HIV/AIDS)
- Addiction Medicine (324)
- Allergy and Immunology (633)
- Anesthesia (168)
- Cardiovascular Medicine (2402)
- Dentistry and Oral Medicine (289)
- Dermatology (207)
- Emergency Medicine (381)
- Endocrinology (including Diabetes Mellitus and Metabolic Disease) (852)
- Epidemiology (11799)
- Forensic Medicine (10)
- Gastroenterology (705)
- Genetic and Genomic Medicine (3770)
- Geriatric Medicine (350)
- Health Economics (637)
- Health Informatics (2409)
- Health Policy (940)
- Health Systems and Quality Improvement (905)
- Hematology (342)
- HIV/AIDS (787)
- Infectious Diseases (except HIV/AIDS) (13348)
- Intensive Care and Critical Care Medicine (769)
- Medical Education (369)
- Medical Ethics (105)
- Nephrology (401)
- Neurology (3524)
- Nursing (199)
- Nutrition (529)
- Obstetrics and Gynecology (681)
- Occupational and Environmental Health (669)
- Oncology (1835)
- Ophthalmology (539)
- Orthopedics (221)
- Otolaryngology (287)
- Pain Medicine (234)
- Palliative Medicine (66)
- Pathology (447)
- Pediatrics (1038)
- Pharmacology and Therapeutics (426)
- Primary Care Research (424)
- Psychiatry and Clinical Psychology (3192)
- Public and Global Health (6185)
- Radiology and Imaging (1293)
- Rehabilitation Medicine and Physical Therapy (751)
- Respiratory Medicine (832)
- Rheumatology (380)
- Sexual and Reproductive Health (374)
- Sports Medicine (324)
- Surgery (406)
- Toxicology (50)
- Transplantation (172)
- Urology (147)

A .gov website belongs to an official government organization in the United States.
A lock ( ) or https:// means you've safely connected to the .gov website. Share sensitive information only on official, secure websites.
- Guidelines and Guidance Library
- Core Practices
- Isolation Precautions Guideline
- Disinfection and Sterilization Guideline
- Environmental Infection Control Guidelines
- Hand Hygiene Guidelines
- Multidrug-resistant Organisms (MDRO) Management Guidelines
- Catheter-Associated Urinary Tract Infections (CAUTI) Prevention Guideline
- Tools and resources
- Evaluating Environmental Cleaning
What to know
This guideline provides recommendations for isolation precautions in healthcare settings.
Guideline for Isolation Precautions: Preventing Transmission of Infectious Agents in Healthcare Settings (2007)
Print Version of Guidelines
Updates, infection control.
CDC provides information on infection control and clinical safety to help reduce the risk of infections among healthcare workers, patients, and visitors.
For Everyone
Health care providers, public health.

An official website of the United States government
The .gov means it’s official. Federal government websites often end in .gov or .mil. Before sharing sensitive information, make sure you’re on a federal government site.
The site is secure. The https:// ensures that you are connecting to the official website and that any information you provide is encrypted and transmitted securely.
- Publications
- Account settings
Preview improvements coming to the PMC website in October 2024. Learn More or Try it out now .
- Advanced Search
- Journal List
- Cancers (Basel)

Breast Cancer—Epidemiology, Risk Factors, Classification, Prognostic Markers, and Current Treatment Strategies—An Updated Review
Sergiusz Łukasiewicz.
1 Department of Surgical Oncology, Center of Oncology of the Lublin Region St. Jana z Dukli, 20-091 Lublin, Poland; lp.lzoc@zciweisakulS (S.Ł.); [email protected] (A.S.)
Marcin Czeczelewski
2 Department of Forensic Medicine, Medical University of Lublin, 20-090 Lublin, Poland; [email protected] (M.C.); lp.teno@amrofa (A.F.)
Alicja Forma
3 Department of Human Anatomy, Medical University of Lublin, 20-090 Lublin, Poland; [email protected]
Robert Sitarz
Andrzej stanisławek.
4 Department of Oncology, Chair of Oncology and Environmental Health, Medical University of Lublin, 20-081 Lublin, Poland
Simple Summary
Breast cancer is the most common cancer among women. It is estimated that 2.3 million new cases of BC are diagnosed globally each year. Based on mRNA gene expression levels, BC can be divided into molecular subtypes that provide insights into new treatment strategies and patient stratifications that impact the management of BC patients. This review addresses the overview on the BC epidemiology, risk factors, classification with an emphasis on molecular types, prognostic biomarkers, as well as possible treatment modalities.
Breast cancer (BC) is the most frequently diagnosed cancer in women worldwide with more than 2 million new cases in 2020. Its incidence and death rates have increased over the last three decades due to the change in risk factor profiles, better cancer registration, and cancer detection. The number of risk factors of BC is significant and includes both the modifiable factors and non-modifiable factors. Currently, about 80% of patients with BC are individuals aged >50. Survival depends on both stage and molecular subtype. Invasive BCs comprise wide spectrum tumors that show a variation concerning their clinical presentation, behavior, and morphology. Based on mRNA gene expression levels, BC can be divided into molecular subtypes (Luminal A, Luminal B, HER2-enriched, and basal-like). The molecular subtypes provide insights into new treatment strategies and patient stratifications that impact the management of BC patients. The eighth edition of TNM classification outlines a new staging system for BC that, in addition to anatomical features, acknowledges biological factors. Treatment of breast cancer is complex and involves a combination of different modalities including surgery, radiotherapy, chemotherapy, hormonal therapy, or biological therapies delivered in diverse sequences.
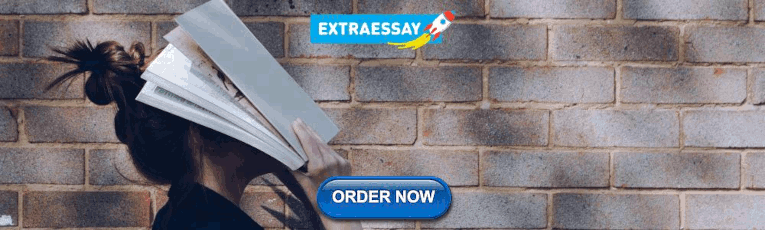
1. Introduction
Being characterized by six major hallmarks, carcinogenesis might occur in every cell, tissue, and organ, leading to the pathological alternations that result in a vast number of cancers. The major mechanisms that enable its progression include evasion of apoptosis, limitless capacity to divide, enhanced angiogenesis, resistance to anti-growth signals and induction of own growth signals, as well as the capacity to metastasize [ 1 ]. Carcinogenesis is a multifactorial process that is primarily stimulated by both—genetic predispositions and environmental causes. The number of cancer-related deaths is disturbingly increasing every year ranking them as one of the major causes of death worldwide. Even though a significant number of cancers do not always need to result in death, they significantly lower the quality of life and require larger costs in general.
Breast cancer is currently one of the most prevalently diagnosed cancers and the 5th cause of cancer-related deaths with an estimated number of 2.3 million new cases worldwide according to the GLOBOCAN 2020 data [ 2 ]. Deaths due to breast cancer are more prevalently reported (an incidence rate approximately 88% higher) in transitioning countries (Melanesia, Western Africa, Micronesia/Polynesia, and the Caribbean) compared to the transitioned ones (Australia/New Zealand, Western Europe, Northern America, and Northern Europe). Several procedures such as preventive behaviors in general as well as screening programs are crucial regarding a possible minimization of breast cancer incidence rate and the implementation of early treatment. Currently, it is the Breast Health Global Initiative (BHGI) that is responsible for the preparation of proper guidelines and the approaches to provide the most sufficient breast cancer control worldwide [ 3 ]. In this review article, we have focused on the female breast cancer specifically since as abovementioned, it currently constitutes the most prevalent cancer amongst females.
2. Breast Cancer Epidemiology
According to the WHO, malignant neoplasms are the greatest worldwide burden for women, estimated at 107.8 million Disability-Adjusted Life Years (DALYs), of which 19.6 million DALYs are due to breast cancer. [ 4 ]. Breast cancer is the most frequently diagnosed cancer in women worldwide with 2.26 million [95% UI, 2.24–2.79 million] new cases in 2020 [ 5 ]. In the United States, breast cancer alone is expected to account for 29% of all new cancers in women [ 6 ]. The 2018 GLOBOCAN data shows that age-standardized incidence rates (ASIR) of breast cancer are strongly and positively associated with the Human Development Index (HDI) [ 7 ]. According to 2020 data, the ASIR was the highest in very high HDI countries (75.6 per 100,000) while it was more than 200% lower in medium and low HDI countries (27.8 per 100,000 and 36.1 per 100,000 respectively) [ 5 ].
Besides being the most common, breast cancer is also the leading cause of cancer death in women worldwide. Globally, breast cancer was responsible for 684,996 deaths [95% UI, 675,493–694,633] at an age-adjusted rate of 13.6/100,000 [ 5 ]. Although incidence rates were the highest in developed regions, the countries in Asia and Africa shared 63% of total deaths in 2020 [ 5 ]. Most women who develop breast cancer in a high-income country will survive; the opposite is true for women in most low-income and many middle-income countries [ 8 ].
In 2020 breast cancer mortality-to-incidence ratio (MIR) as a representative indicator of 5-year survival rates [ 9 ] was 0.30 globally [ 5 ]. Taking into consideration the clinical extent of breast cancer, in locations with developed health care (Hong-Kong, Singapore, Turkey) the 5-year survival was 89.6% for localized and 75.4% for regional cancer. In less developed countries (Costa Rica, India, Philippines, Saudi Arabia, Thailand) the survival rates were 76.3% and 47.4% for localized and regional breast cancer respectively [ 10 ].
Breast cancer incidence and death rates have increased over the last three decades. Between 1990 and 2016 breast cancer incidence has more than doubled in 60/102 countries (e.g., Afghanistan, Philippines, Brazil, Argentina), whereas deaths have doubled in 43/102 countries (e.g., Yemen, Paraguay, Libya, Saudi Arabia) [ 11 ]. Current projections indicate that by 2030 the worldwide number of new cases diagnosed reach 2.7 million annually, while the number of deaths 0.87 million [ 12 ]. In low- and medium-income countries, the breast cancer incidence is expected to increase further due to the westernization of lifestyles (e.g., delayed pregnancies, reduced breastfeeding, low age at menarche, lack of physical activity, and poor diet), better cancer registration, and cancer detection [ 13 ].
3. Risk Factors of Breast Cancer
The number of risk factors of breast cancer is significant and includes both modifiable factors and non-modifiable factors ( Table 1 ).
Modifiable and non-modifiable risk factors of breast cancer.
3.1. Non-Modifiable Factors
3.1.1. female sex.
Female sex constitutes one of the major factors associated with an increased risk of breast cancer primarily because of the enhanced hormonal stimulation. Unlike men who present insignificant estrogen levels, women have breast cells which are very vulnerable to hormones (estrogen and progesterone in particular) as well as any disruptions in their balance. Circulating estrogens and androgens are positively associated with an increased risk of breast cancer [ 14 ]. The alternations within the physiological levels of the endogenous levels of sex hormones result in a higher risk of breast cancer in the case of premenopausal and postmenopausal women; these observations were also supported by the Endogenous Hormones and Breast Cancer Collaborative Group [ 15 , 16 , 17 ].
Less than 1% of all breast cancers occur in men. However, breast cancer in men is a rare disease that’s at the time of diagnosis tends to be more advanced than in women. The average age of men at the diagnosis is about 67. The important factors increase a man’s risk of breast cancer are: older age, BRCA2/BRCA1 mutations, increased estrogen levels, Klinefelter syndrome, family history of breast cancer, and radiation exposure [ 18 ].
3.1.2. Older Age
Currently, about 80% of patients with breast cancer are individuals aged >50 while at the same time more than 40% are those more than 65 years old [ 19 , 20 , 21 ]. The risk of developing breast cancer increases as follows—the 1.5% risk at age 40, 3% at age 50, and more than 4% at age 70 [ 22 ]. Interestingly, a relationship between a particular molecular subtype of cancer and a patient’s age was observed –aggressive resistant triple-negative breast cancer subtype is most commonly diagnosed in groups under 40 age, while in patients >70, it is luminal A subtype [ 21 ]. Generally, the occurrence of cancer in older age is not only limited to breast cancer; the accumulation of a vast number of cellular alternations and exposition to potential carcinogens results in an increase of carcinogenesis with time.
3.1.3. Family History
A family history of breast cancer constitutes a major factor significantly associated with an increased risk of breast cancer. Approximately 13–19% of patients diagnosed with breast cancer report a first-degree relative affected by the same condition [ 23 ]. Besides, the risk of breast cancer significantly increases with an increasing number of first-degree relatives affected; the risk might be even higher when the affected relatives are under 50 years old [ 24 , 25 , 26 ]. The incidence rate of breast cancer is significantly higher in all of the patients with a family history despite the age. This association is driven by epigenetic changes as well as environmental factors acting as potential triggers [ 27 ]. A family history of ovarian cancer—especially those characterized by BRCA1 and BRCA2 mutations—might also induce a greater risk of breast cancer [ 28 ].
3.1.4. Genetic Mutations
Several genetic mutations were reported to be highly associated with an increased risk of breast cancer. Two major genes characterized by a high penetrance are BRCA1 (located on chromosome 17) and BRCA2 (located on chromosome 13). They are primarily linked to the increased risk of breast carcinogenesis [ 29 ]. The mutations within the above-mentioned genes are mainly inherited in an autosomal dominant manner, however, sporadic mutations are also commonly reported. Other highly penetrant breast cancer genes include TP53 , CDH1 , PTEN , and STK11 [ 30 , 31 , 32 , 33 , 34 ]. Except for the increased risk of breast cancer, carriers of such mutations are more susceptible to ovarian cancer as well. A significant number of DNA repair genes that can interact with BRCA genes including ATM , PALB2 , BRIP1 , or CHEK2 , were reported to be involved in the induction of breast carcinogenesis; those are however characterized by a lower penetrance (moderate degree) compared to BRCA1 or BRCA2 ( Table 2 ) [ 29 , 35 , 36 , 37 , 38 ]. According to quite recent Polish research, mutations within the XRCC2 gene could also be potentially associated with an increased risk of breast cancer [ 39 ].
Major genes associated with an increased risk of breast cancer occurrence.
3.1.5. Race/Ethnicity
Disparities regarding race and ethnicity remain widely observed among individuals affected by breast cancer; the mechanisms associated with this phenomenon are not yet understood. Generally, the breast cancer incidence rate remains the highest among white non-Hispanic women [ 51 , 52 ]. Contrarily, the mortality rate due to this malignancy is significantly higher among black women; this group is also characterized by the lowest survival rates [ 53 ].
3.1.6. Reproductive History
Numerous studies confirmed a strict relationship between exposure to endogenous hormones—estrogen and progesterone in particular—and excessive risk of breast cancer in females. Therefore, the occurrence of specific events such as pregnancy, breastfeeding, first menstruation, and menopause along with their duration and the concomitant hormonal imbalance, are crucial in terms of a potential induction of the carcinogenic events in the breast microenvironment. The first full-term pregnancy at an early age (especially in the early twenties) along with a subsequently increasing number of births are associated with a reduced risk of breast cancer [ 54 , 55 ]. Besides, the pregnancy itself provides protective effects against potential cancer. However, protection was observed at approximately the 34th pregnancy week and was not confirmed for the pregnancies lasting for 33 weeks or less [ 56 ]. Women with a history of preeclampsia during pregnancy or children born to a preeclamptic pregnancy are at lower risk of developing breast cancer [ 57 ]. No association between the increased breast cancer risk and abortion was stated so far [ 58 ].
The dysregulated hormone levels during preeclampsia including increased progesterone and reduced estrogen levels along with insulin, cortisol, insulin-like growth factor-1, androgens, human chorionic gonadotropin, corticotropin-releasing factor, and IGF-1 binding protein deviating from the physiological ranges, show a protective effect preventing from breast carcinogenesis. The longer duration of the breastfeeding period also reduces the risk of both the ER/PR-positive and -negative cancers [ 59 ]. Early age at menarche is another risk factor of breast cancer; it is possibly also associated with a tumor grade and lymph node involvement [ 60 ]. Besides, the earlier age of the first menstruation could result in an overall poorer prognosis. Contrarily, early menopause despite whether natural or surgical, lowers the breast cancer risk [ 61 ].
3.1.7. Density of Breast Tissue
The density of breast tissue remains inconsistent throughout the lifetime; however, several categories including low-density, high-density, and fatty breasts have been established in clinical practice. Greater density of breasts is observed in females of younger age and lower BMI, who are pregnant or during the breastfeeding period, as well as during the intake of hormonal replacement therapy [ 62 ]. Generally, the greater breast tissue density correlates with the greater breast cancer risk; this trend is observed both in premenopausal and postmenopausal females [ 63 ]. It was proposed that screening of breast tissue density could be a promising, non-invasive, and quick method enabling rational surveillance of females at increased risk of cancer [ 64 ].
3.1.8. History of Breast Cancer and Benign Breast Diseases
Personal history of breast cancer is associated with a greater risk of a renewed cancerous lesions within the breasts [ 65 ]. Besides, a history of any other non-cancerous alternations in breasts such as atypical hyperplasia, carcinoma in situ, or many other proliferative or non-proliferative lesions, also increases the risk significantly [ 66 , 67 , 68 ]. The histologic classification of benign lesions and a family history of breast cancer are two factors that are strongly associated with breast cancer risk [ 66 ].
3.1.9. Previous Radiation Therapy
The risk of secondary malignancies after radiotherapy treatment remains an individual matter that depends on the patient’s characteristics, even though it is a quite frequent phenomenon that arises much clinical concern. Cancer induced by radiation therapy is strictly associated with an individual’s age; patients who receive radiation therapy before the age of 30, are at a greater risk of breast cancer [ 69 ]. The selection of proper radiotherapy technique is crucial in terms of secondary cancer risk—for instance, tangential field IMRT (2F-IMRT) is associated with a significantly lower risk compared to multiple-field IMRT (6F-IMRT) or double partial arcs (VMAT) [ 70 ]. Besides, the family history of breast cancer in patients who receive radiotherapy additionally enhances the risk of cancer occurrence [ 71 ]. However, Bartelink et al. showed that additional radiation (16 Gy) to the tumor bed combined with standard radiotherapy might decrease the risk of local recurrence [ 72 ].
3.2. Modifiable Factors
3.2.1. chosen drugs.
Data from some research indicates that the intake of diethylstilbestrol during pregnancy might be associated with a greater risk of breast cancer in children; this, however, remains inconsistent between studies and requires further evaluation [ 73 , 74 ]. The intake of diethylstilbestrol during pregnancy is associated with an increased risk of breast cancer not only in mothers but also in the offspring [ 75 ]. This relationship is observed despite the expression of neither estrogen nor progesterone receptors and might be associated with every breast cancer histological type. The risk increases with age; women at age of ≥40 years are nearly 1.9 times more susceptible compared to women under 40. Moreover, breast cancer risk increases with greater diethylstilbestrol doses [ 76 ]. Numerous researches indicate that females who use hormonal replacement therapy (HRT) especially longer than 5 or 7 years are also at increased risk of breast cancer [ 77 , 78 ]. Several studies indicated that the intake of chosen antidepressants, mainly paroxetine, tricyclic antidepressants, and selective serotonin reuptake inhibitors might be associated with a greater risk of breast cancer [ 79 , 80 ]. Lawlor et al. showed that similar risk might be achieved due to the prolonged intake of antibiotics; Friedman et al. observed that breast risk is mostly elevated while using tetracyclines [ 81 , 82 ]. Attempts were made to investigate a potential relationship between hypertensive medications, non-steroidal anti-inflammatory drugs, as well as statins, and an elevated risk of breast cancer, however, this data remains highly inconsistent [ 83 , 84 , 85 ].
3.2.2. Physical Activity
Even though the mechanism remains yet undeciphered, regular physical activity is considered to be a protective factor of breast cancer incidence [ 86 , 87 ]. Chen et al. observed that amongst females with a family history of breast cancer, physical activity was associated with a reduced risk of cancer but limited only to the postmenopausal period [ 88 ]. However, physical activity is beneficial not only in females with a family history of breast cancer but also in those without such a history. Contrarily to the above-mentioned study, Thune et al. pointed out more pronounced effects in premenopausal females [ 89 ]. There are several hypotheses aiming to explain the protective role of physical activity in terms of breast cancer incidence; physical activity might prevent cancer by reducing the exposure to the endogenous sex hormones, altering immune system responses or insulin-like growth factor-1 levels [ 88 , 90 , 91 ].
3.2.3. Body Mass Index
According to epidemiological evidence, obesity is associated with a greater probability of breast cancer. This association is mostly intensified in obese post-menopausal females who tend to develop estrogen-receptor-positive breast cancer. Yet, independently to menopausal status, obese women achieve poorer clinical outcomes [ 92 ]. Wang et al. showed that females above 50 years old with greater Body Mass Index (BMI) are at a greater risk of cancer compared to those with low BMI [ 93 ]. Besides, the researchers observed that greater BMI is associated with more aggressive biological features of tumor including a higher percentage of lymph node metastasis and greater size. Obesity might be a reason for greater mortality rates and a higher probability of cancer relapse, especially in premenopausal women [ 94 ]. Increased body fat might enhance the inflammatory state and affects the levels of circulating hormones facilitating pro-carcinogenic events [ 95 ]. Thus, poorer clinical outcomes are primarily observed in females with BMI ≥ 25 kg/m 2 [ 96 ]. Interestingly, postmenopausal women tend to present poorer clinical outcomes despite proper BMI values but namely due to excessive fat volume [ 97 ]. Greater breast cancer risk with regards to BMI also correlates with the concomitant family history of breast cancer [ 98 ].
3.2.4. Alcohol Intake
Numerous evidences confirm that excessive alcohol consumption is a factor that might enhance the risk of malignancies within the gastrointestinal tract; however, it was proved that it is also linked to the risk of breast cancer. Namely, it is not alcohol type but rather the content of alcoholic beverages that mostly affect the risk of cancer. The explanation for this association is the increased levels of estrogens induced by the alcohol intake and thus hormonal imbalance affecting the risk of carcinogenesis within the female organs [ 99 , 100 ]. Besides, alcohol intake often results in excessive fat gain with higher BMI levels, which additionally increases the risk. Other hypotheses include direct and indirect carcinogenic effects of alcohol metabolites and alcohol-related impaired nutrient intake [ 101 ]. Alcohol consumption was observed to increase the risk of estrogen-positive breast cancers in particular [ 102 ]. Consumed before the first pregnancy, it significantly contributes to the induction of morphological alterations of breast tissue, predisposing it to further carcinogenic events [ 103 ].
3.2.5. Smoking
Carcinogens found in tobacco are transported to the breast tissue increasing the plausibility of mutations within oncogenes and suppressor genes ( p53 in particular). Thus, not only active but also passive smoking significantly contributes to the induction of pro-carcinogenic events [ 104 ]. Besides, longer smoking history, as well as smoking before the first full-term pregnancy, are additional risk factors that are additionally pronounced in females with a family history of breast cancer [ 105 , 106 , 107 , 108 ].
3.2.6. Insufficient Vitamin Supplementation
Vitamins exert anticancer properties, which might potentially benefit in the prevention of several malignancies including breast cancer, however, the mechanism is not yet fully understood. Attempts are continually made to analyze the effects of vitamin intake (vitamin C, vitamin E, B-group vitamins, folic acid, multivitamin) on the risk of breast cancer, nevertheless, the data remains inconsistent and not sufficient to compare the results and draw credible data [ 108 ]. In terms of breast cancer, most studies are currently focused on vitamin D supplementation confirming its potentially protective effects [ 109 , 110 , 111 ]. High serum 25-hydroxyvitamin D levels are associated with a lower incidence rate of breast cancer in premenopausal and postmenopausal women [ 110 , 112 ]. Intensified expression of vitamin D receptors was shown to be associated with lower mortality rates due to breast cancer [ 113 ]. Even so, further evaluation is required since data remains inconsistent in this matter [ 108 , 114 ].
3.2.7. Exposure to Artificial Light
Artificial light at night (ALAN) has been recently linked to increased breast cancer risk. The probable causation might be a disrupted melatonin rhythm and subsequent epigenetic alterations [ 115 ]. According to the studies conducted so far, increased exposure to ALAN is associated with a significantly greater risk of breast cancer compared to individuals with lowered ALAN exposure [ 116 ]. Nonetheless, data regarding the excessive usage of LED electronic devices and increased risk of breast cancer is insufficient and requires further evaluation as some results are contradictory [ 116 ].
3.2.8. Intake of Processed Food/Diet
According to the World Health Organization (WHO), highly processed meat was classified as a Group 1 carcinogen that might increase the risk of not only gastrointestinal malignancies but also breast cancer. Similar observations were made in terms of an excessive intake of saturated fats [ 117 ]. Ultra-processed food is rich in sodium, fat, and sugar which subsequently predisposes to obesity recognized as another factor of breast cancer risk [ 118 ]. It was observed that a 10% increase of ultra-processed food in the diet is associated with an 11% greater risk of breast cancer [ 118 ]. Contrarily, a diet high in vegetables, fruits, legumes, whole grains, and lean protein is associated with a lowered risk of breast cancer [ 119 ]. Generally, a diet that includes food containing high amounts of n-3 PUFA, vitamin D, fiber, folate, and phytoestrogen might be beneficial as a prevention of breast cancer [ 120 ]. Besides, lower intake of n-6 PUFA and saturated fat is recommended. Several in vitro and in vivo studies also suggest that specific compounds found in green tea might present anti-cancer effects which has also been studied regarding breast cancer [ 121 ]. Similar properties were observed in case of turmeric-derived curcuminoids as well as sulforaphane (SFN) [ 122 , 123 ].
3.2.9. Exposure to Chemical
Chronic exposure to chemicals can promote breast carcinogenesis by affecting the tumor microenvironment subsequently inducing epigenetic alterations along with the induction of pro-carcinogenic events [ 124 ]. Females chronically exposed to chemicals present significantly greater plausibility of breast cancer which is further positively associated with the duration of the exposure [ 125 ]. The number of chemicals proposed to induce breast carcinogenesis is significant; so far, dichlorodiphenyltrichloroethane (DDT) and polychlorinated biphenyl (PCB) are mostly investigated in terms of breast cancer since early exposure to those chemicals disrupts the development of mammary glands [ 126 , 127 ]. A potential relationship was also observed in the case of increased exposure to polycyclic aromatic hydrocarbons (PAH), synthetic fibers, organic solvents, oil mist, and insecticides [ 128 ].
3.2.10. Other Drugs
Other drugs that might constitute potential risk factors for breast cancer include antibiotics, antidepressants, statins, antihypertensive medications (e.g., calcium channel blockers, angiotensin II-converting enzyme inhibitors), as well as NSAIDs (including aspirin, ibuprofen) [ 129 , 130 , 131 , 132 , 133 ].
4. Breast Cancer Classification
4.1. histological classification.
Invasive breast cancers (IBC) comprise wide spectrum tumors that show a variation concerning their clinical presentation, behavior, and morphology. The World Health Organization (WHO) distinguish at least 18 different histological breast cancer types [ 134 ].
Invasive breast cancer of no special type (NST), formerly known as invasive ductal carcinoma is the most frequent subgroup (40–80%) [ 135 ]. This type is diagnosed by default as a tumor that fails to be classified into one of the histological special types [ 134 ]. About 25% of invasive breast cancers present distinctive growth patterns and cytological features, hence, they are recognized as specific subtypes (e.g., invasive lobular carcinoma, tubular, mucinous A, mucinous B, neuroendocrine) [ 136 ].
Molecular classification independently from histological subtypes, invasive breast cancer can be divided into molecular subtypes based on mRNA gene expression levels. In 2000, Perou et al. on a sample of 38 breast cancers identified 4 molecular subtypes from microarray gene expression data: Luminal, HER2-enriched, Basal-like, and Normal Breast-like [ 137 ]. Further studies allowed to divide the Luminal group into two subgroups (Luminal A and B) [ 138 , 139 ]. The normal breast-like subtype has subsequently been omitted, as it is thought to represent sample contamination by normal mammary glands. In the Cancer Genome Atlas Project (TCGA) over 300 primary tumors were thoroughly profiled (at DNA, RNA, and protein levels) and combined in biological homogenous groups of tumors. The consensus clustering confirmed the distinction of four main breast cancer intrinsic subtypes based on mRNA gene expression levels only (Luminal A, Luminal B, HER2-enriched, and basal-like) [ 140 ]. Additionally, the 5th intrinsic subtype—claudin-low breast cancer was discovered in 2007 in an integrated analysis of human and murine mammary tumors [ 141 ].
In 2009, Parker et al. developed a 50-gene signature for subtype assignment, known as PAM50, that could reliably classify particular breast cancer into the main intrinsic subtypes with 93% accuracy [ 142 ]. PAM50 is now clinically implemented worldwide using the NanoString nCounter ® , which is the basis for the Prosigna ® test. The Prosigna ® combines the PAM50 assay as well as clinical information to assess the risk of distant relapse estimation in postmenopausal women with hormone receptor-positive, node-negative, or node-positive early-stage breast cancer patients, and is a daily-used tool assessing the indication of adjuvant chemotherapy [ 143 , 144 , 145 ].
4.2. Luminal Breast Cancer
Luminal breast cancers are ER-positive tumors that comprise almost 70% of all cases of breast cancers in Western populations [ 146 ]. Most commonly Luminal-like cancers present as IBC of no special subtype, but they may infrequently differentiate into invasive lobular, tubular, invasive cribriform, mucinous, and invasive micropapillary carcinomas [ 147 , 148 ]. Two main biological processes: proliferation-related pathways and luminal-regulated pathways distinguish Luminal-like tumors into Luminal A and B subtypes with different clinical outcomes.
Luminal A tumors are characterized by presence of estrogen-receptor (ER) and/or progesterone-receptor (PR) and absence of HER2. In this subtype the ER transcription factors activate genes, the expression of which is characteristic for luminal epithelium lining the mammary ducts [ 149 , 150 ]. It also presents a low expression of genes related to cell proliferation [ 151 ]. Clinically they are low-grade, slow-growing, and tend to have the best prognosis.
In contrast to subtype A, Luminal B tumors are higher grade and has worse prognosis. They are ER positive and may be PR negative and/or HER2 positive. Additionally, it has high expression of proliferation-related genes (e.g., MKI67 and AURKA) [ 152 , 153 , 154 ]. This subtype has lower expression of genes or proteins typical for luminal epithelium such as the PR [ 150 , 155 ] and FOXA1 [ 146 , 156 ], but not the ER [ 157 ]. ER is similarly expressed in both A and B subtypes and is used to distinguish luminal from non-luminal disease.
4.3. HER2-Enriched Breast Cancer
The HER2-enriched group makes up 10–15% of breast cancers. It is characterized by the high expression of the HER2 with the absence of ER and PR. This subtype mainly expresses proliferation—related genes and proteins (e.g., ERBB2/HER2 and GRB7), rather than luminal and basal gene and protein clusters [ 154 , 156 , 157 ]. Additionally, in the HER2-enriched subtype there is evidence of mutagenesis mediated by APOBEC3B. APOBEC3B is a subclass of APOBEC cytidine deaminases, which induce cytosine mutation biases and is a source of mutation clusters [ 158 , 159 , 160 ].
HER2-enriched cancers grow faster than luminal cancers and used to have the worst prognosis of subtypes before the introduction of HER2-targeted therapies. Importantly, the HER2-enriched subtype is not synonymous with clinically HER2-positive breast cancer because many ER-positive/HER2-positive tumors qualify for the luminal B group. Moreover, about 30% of HER2-enriched tumors are classified as clinically HER2-negative based on immunohistochemistry (IHC) and/or fluorescence in situ hybridization (FISH) methods [ 161 ].
4.4. Basal-Like/Triple-Negative Breast Cancer
The Triple-Negative Breast Cancer (TNBC) is a heterogeneous collection of breast cancers characterized as ER-negative, PR-negative, and HER2-negative. They constitute about 20% of all breast cancers. TNBC is more common among women younger than 40 years of age and African-American women [ 161 ]. The majority (approximately 80%) of breast cancers arising in BRCA1 germline mutation are TNBC, while 11–16% of all TNBC harbor BRCA1 or BRCA2 germline mutations. TNBC tends to be biologically aggressive and is often associated with a worse prognosis [ 162 ]. The most common histology seen in TNBC is infiltrating ductal carcinoma, but it may also present as medullary-like cancers with a prominent lymphocytic infiltrate; metaplastic cancers, which may show squamous or spindle cell differentiation; and rare special type cancers like adenoid cystic carcinoma (AdCC) [ 163 , 164 , 165 ].
The terms basal-like and TNBC have been used interchangeably; however, not all TNBC are of the basal type. On gene expression profiling, TNBCs can be subdivided into six subtypes: basal-like (BL1 and BL2), mesenchymal (M), mesenchymal stem-like (MSL), immunomodulatory (IM), and luminal androgen receptor (LAR), as well as an unspecified group (UNS) [ 166 , 167 ]. However, the clinical relevance of the subtyping still unclear, and more research is needed to clarify its impact on TNBC treatment decisions [ 168 ].
4.5. Claudin-Low Breast Cancer
Claudin-low (CL) breast cancers are poor prognosis tumors being mostly ER-negative, PR-negative, and HER2-negative. CL tumors account for 7–14% of all invasive breast cancers [ 147 ]. No differences in survival rates were observed between claudin-low tumors and other poor-prognosis subtypes (Luminal B, HER2-enriched, and Basal-like). CL subtype is characterized by the low expression of genes involved in cell-cell adhesion, including claudins 3, 4, and 7, occludin, and E-cadherin. Besides, these tumors show high expression of epithelial-mesenchymal transition (EMT) genes and stem cell-like gene expression patterns [ 169 , 170 ]. Moreover, CL tumors have marked immune and stromal cell infiltration [ 171 ]. Due to their less differentiated state and a preventive effect of the EMT-related transcription factor, ZEB1 CL tumors are often genomically stable [ 172 , 173 ].
4.6. Surrogate Markers Classification
In clinical practice, the key question is the discrimination between patients who will or will not benefit from particular therapies. By using molecular assays, more patients can be spared adjuvant chemotherapy, but these tests are associated with significant costs. Therefore, surrogate subgroups based on pathological morphology and widely available immunohistochemical (IHC) markers are used as a tool for risk stratification and guidance of adjuvant therapy [ 174 ]. A combination of the routine pathological markers ER, PR, and HER2 is used to classify tumors into intrinsic subtypes [ 175 ]. Semiquantitative evaluation of Ki-67 and PR is helpful for further typing of the Luminal subtype [ 176 , 177 ]. Moreover, evaluation of cytokeratin 5/6 and epidermal growth factor receptor is utilized to identify the Basal-like breast cancer among the TNBC [ 178 ].
In St. Gallen’s 2013 guidelines the IHC-based surrogate subtype classification was recommended for clinical decision making [ 179 ]. However, these IHC-based markers are only a surrogate and cannot establish the intrinsic subtype of any given cancer, with discordance rates between IHC-based markers and gene-based assays as high as 30% [ 180 ].
4.7. American Joint Committee on Cancer Classification
The baseline tool to estimate the likely prognosis of patients with breast cancer is the AJCC staging system that includes grading, immunohistochemistry biomarkers, and anatomical advancement of the disease. Since its inception in 1977, the American Joint Committee on Cancer (AJCC) has published an internationally accepted staging system based on anatomic findings: tumor size (T), nodal status (N), and metastases (M). However, gene expression profiling has identified several molecular subtypes of breast cancer [ 181 ]. The eighth edition of the AJCC staging manual (2018), outlines a new prognostic staging system for breast cancer that, in addition to anatomical features, acknowledges biological factors [ 182 ]. These factors—ER, PR, HER2, grade, and multigene assays—are recommended in practice to define prognosis [ 183 , 184 ].
The most widely used histologic grading system of breast cancer is the Elston-Ellis modification [ 185 ] of Scarff-Bloom-Richardson grading system [ 186 ], also known as the Nottingham grading system. The grade of a tumor is determined by assessing morphologic features: (a) formation of tubules, (b) mitotic count, (c) variability, and the size and shape of cellular nuclei. A score between 1 (most favorable) and 3 (least favorable) is assigned for each feature. Grade 1 corresponds to combined scores between 3 and 5, grade 2 corresponds to a combined score of 6 or 7, and grade 3 corresponds to a combined score of 8 or 9.
In addition to grading and biomarkers, the commercially available multigene assays provide additional prognostic information suitable for incorporation in the AJCC 8th edition. The 21-gene assay Oncotype DX ® assessed by reverse transcription-polymerase chain reaction (RT-PCR) was the only assay sufficiently evaluated and included in the staging system. This assay is valuable in the staging of patients with hormone receptor-positive, HER2-negative, node-negative tumors that are <5 cm. Patients with results of the assay (Recurrence Score) less than 11 had excellent disease-free survival at 6.9 years of 98.6% with endocrine therapy alone [ 187 ]. Hence, adjuvant systemic chemotherapy can be safely omitted in patients with a low-risk multigene assay [ 188 ].
The AJCC staging manual includes a pathological and a clinical-stage group. The clinical prognostic stage group should be utilized in all patients on initial evaluation before any systemic therapy. Clinical staging uses the TNM anatomical information, grading, and expression of these three biomarkers. When patients undergo surgical resection of their primary tumor, the post-resection anatomic information coupled with the pretreatment biomarker findings results in the final Pathologic Prognostic Stage Group.
The recent update of breast cancer staging by the biologic markers improved the outcome prediction in comparison to prior staging based only on anatomical features of the disease. The validation studies involving the reassessment of the Surveillance, Epidemiology, and End Results (SEER) database ( n = 209,304, 2010–2014) and the University of Texas MD Anderson Cancer Center database ( n = 3327, years of treatment 2007–2013) according to 8th edition AJCC manual proved the more accurate prognostic information [ 189 , 190 ].
5. Prognostic Biomarkers
5.1. estrogen receptor.
Estrogen receptor (ER) is an important diagnostic determinant since approximately 70–75% of invasive breast carcinomas are characterized by significantly enhanced ER expression [ 191 , 192 ]. Current practice requires the measurement of ER expression on both—primary invasive tumors and recurrent lesions. This procedure is mandatory to provide the selection of those patients who will most benefit from the implementation of the endocrine therapy mainly selective estrogen receptor modulators, pure estrogen receptor downregulators, or third-generation aromatase inhibitors [ 193 ]. Even though the diagnosis of altered expression of ER is particularly relevant in terms of the proper therapy selection, ER expression might also constitute a predictive factor—patients with high ER expression usually present significantly better clinical outcomes [ 194 ]. A relationship was observed between ER expression and the family history of breast cancer which further facilitates the utility of ER expression as a diagnostic biomarker of breast cancer especially in cases of familial risk [ 195 ]. Besides, Konan et al. reported that ERα-36 expression could constitute one of the potential targets of PR-positive cancers and a prognostic marker at the same time [ 196 ].
5.2. Progesterone Receptor
PR is highly expressed (>50%) in patients with ER-positive while quite rarely in those with ER-negative breast cancer [ 197 ]. PR expression is regulated by ER therefore, physiological values of PR inform about the functional ER pathway [ 197 ]. However, both ER and PR are abundantly expressed in breast cancer cells and both are considered as diagnostic and prognostic biomarkers of breast cancer (especially ER-positive ones) [ 198 ]. Greater PR expression is positively associated with the overall survival, time to recurrence, and time to either treatment failure or progression while lowered PR levels are usually related to a more aggressive course of the disease as well as poorer recurrence and prognosis [ 199 ]. Thus, favorable management of breast cancer patients highly depends on the assessment of PR expression. Nevertheless, the predictive value of PR expression still remains controversial [ 200 ].
5.3. Human Epidermal Growth Factor Receptor 2
The expression of human epidermal growth factor receptor 2 (HER2) accounts for approximately 15–25% of breast cancers and its status is primarily relevant in the choice of proper management with breast cancer patients; HER2 overexpression is one of the earliest events during breast carcinogenesis [ 201 ]. Besides, HER2 increases the detection rate of metastatic or recurrent breast cancers from 50% to even more than 80% [ 202 ]. Serum HER2 levels are considered to be a promising real-time marker of tumor presence or recurrence [ 203 ]. HER2 amplification leads to further overactivation of the pro-oncogenic signaling pathways leading to uncontrolled growth of cancer cells which corresponds with poorer clinical outcomes in the case of HER2-positive cancers [ 204 ]. Overexpression of HER2 also correlates with a significantly shorter disease-free period [ 205 ] as well as histologic type, pathologic state of cancer, and a number of axillary nodes with metastatic cancerous cells [ 205 ].
5.4. Antigen Ki-67
The Ki-67 protein is a cellular marker of proliferation and the Ki-67 proliferation index is an excellent marker to provide information about the proliferation of cancerous cells particularly in the case of breast cancer. The proliferative activities determined by Ki-67 reflect the aggressiveness of cancer along with the response to treatment and recurrence time [ 206 ]. Thus, Ki-67 is crucial in terms of the choice of the proper treatment therapy and the potential follow-ups due to recurrence. Though, due to several limitations of the analytical validity of Ki-67 immunohistochemistry, Ki-67 expression levels should be considered benevolently in terms of definite treatment decisions. Ki-67 might be considered as a potential prognostic factor as well; according to a meta-analysis of 68 studies involving 12,155 patients, the overexpression of Ki-67 is associated with poorer clinical outcomes of patients [ 207 ]. High expression of Ki-67 also reflects poorer survival rates of breast cancer patients [ 208 ]. There are speculations whether Ki-67 could be considered as a potential predictive marker, however, such data is still limited and contradictory.
Mib1 (antibody against Ki-67) proliferation index remains a reliable diagnostic biomarker of breast cancer, similarly to Ki-67. A decrease in both Mib1 and Ki-67 expression levels is associated with a good response of breast cancer patients to preoperative treatment [ 209 ]. Mib1 levels are significantly greater in patients with concomitant p53 mutations [ 210 ]. Mib1 assessment might be especially useful in cases of biopsy specimens small in size, inappropriate for neither mitotic index nor S-phase fraction evaluation [ 211 ].
5.6. E-Cadherin
E-cadherin is a critical protein in the epithelial-mesenchymal transition (EMT); loss of its expression leads to the gradual transformation into mesenchymal phenotype which is further associated with increased risk of metastasis. The utility of E-cadherin as a breast biomarker is yet questionable, however, some research indicated that its expression is potentially associated with several breast cancer characteristics such as tumor size, TNM stage, or lymph node status [ 212 ]. Low or even total loss of E-cadherin expression might be potentially useful in the determination of histologic subtype of breast cancer [ 213 , 214 ]. E-cadherin levels do not seem to be promising in terms of patients’ survival rates assessment, however, there are some reports indicating that higher levels of E-cadherin were associated with shorter survival rates in patients with invasive breast carcinoma [ 213 , 215 ]. Lowered E-cadherin expression is positively associated with lymph node metastasis [ 216 ].
5.7. Circulating Circular RNA
Circulating circular RNAs (circRNAs) belong to the group of non-coding RNA and were quite recently shown to be crucial in terms of several hallmarks of breast carcinogenesis including apoptosis, enhanced proliferation, or increased metastatic potential [ 217 ]. One of the most comprehensively described circRNAs, mostly specific to breast cancer include circFBXW7—which was proposed as a potential diagnostic biomarker as well as therapeutic tool for patients with triple-negative breast cancer (TNBC), as well as hsa_circ_0072309 which is abundantly expressed in breast cancer patients and usually associated with poorer survival rates [ 218 ]. Has_circ_0001785 is considered to be promising as a diagnostic biomarker of breast cancer [ 219 ]. The number of circRNAs dysregulated during breast carcinogenesis is significant; their expression might be either upregulated (e.g., has_circ_103110, circDENND4C) or downregulated (e.g., has_circ_006054, circ-Foxo3) [ 220 ]. Besides, specific circRNAs have been reported in different types of breast cancer such as TNBC, HER2-positive, and ER-positive [ 221 ]. Recently it was showed that an interaction between circRNAs and micro-RNA—namely in the form of Cx43/has_circ_0077755/miR-182 post-transcriptional axis, might predict breast cancer initiation as well as further prognosis. Cx43 is transmembrane protein responsible for epithelial homeostasis that mediates junction intercellular communication and its loss dysregulates post-transcriptional axes in breast cancer initiation [ 222 ].
Loss-of-function mutations in the TP53 (P53) gene have been found in numerous cancer types including osteosarcomas, leukemia, brain tumors, adrenocortical carcinomas, and breast cancers [ 223 , 224 ]. P53 protein is essential for normal cellular homeostasis and genome maintenance by mediating cellular stress responses including cell cycle arrest, apoptosis, DNA repair, and cellular senescence [ 225 ]. The silencing mutation of the P53 gene is evident at an early stage of cancer progression. In breast cancer, the prevalence of TP53 mutations is present in approximately 80% of patients with the TNBC and 10% of patients with Luminal A disease [ 226 ].
There have been many studies showing the prognostic role of p53 loss-of-function mutation in breast cancer [ 227 , 228 ]. However, the missense mutations may alters p53 properties causing not only a loss of wild-type function, but also acquisition novel activities-gain of function [ 229 ]. The IHC status of p53 has been proposed as a specific prognostic factor in TNBC, and a feature that divides TNBC into 2 distinct subgroups: a p53-negative normal breast-like TN subgroup, and a p53-positive basal-like subgroup with worse overall survival [ 230 , 231 , 232 ]. However, there is not enough evidence to utilize p53 gene mutational status or immunohistochemically measured protein for determining standardized prognosis in patients with breast cancer [ 233 ].
5.9. MicroRNA
MicroRNAs (miRNA) are a major class of endogenous non-coding RNA molecules (19–25 nucleotides) that have regulatory roles in multiple pathways [ 234 ]. Some miRNAs are related to the development, progression, and response of the tumor to therapy [ 235 ]. Several studies have investigated abnormally expressed miRNAs as biomarkers in breast cancer tissue samples. According to meta-analysis by Adhami et al. two miRNAs (miRNA-21 and miRNA-210) were upregulated consistently and six miRNAs (miRNA-145, miRNA-139-5p, miRNA-195, miRNA-99a, miRNA-497, and miRNA-205) were downregulated consistently in at least three studies [ 236 ].
The miRNA-21 overexpression was observed in TNBC tissues and was associated with enhanced invasion and proliferation of TNBC cells as well as downregulation of the PTEN expression [ 237 ]. Similarly, the high expression of miRNA-210 is related to tumor proliferation, invasion, and poor survival rates in breast cancer patients [ 238 , 239 ].
The miRNA-145 is an anti-cancer agent having the property of inhibiting migration and proliferation of breast cancer cells via regulating the TGF-β1 expression [ 240 ]. However, the miRNA-145 is downregulated in both plasma and tumors of breast cancer patients [ 241 ]. Similarly, miRNA-139-5p and miRNA-195 have tumor suppressor activity in various cancers [ 242 , 243 ].
Nevertheless, further clinical researches focusing on these miRNAs are needed to utilize them as reproducible, disease-specific markers that have a high level of specificity and sensitivity.
5.10. Tumor-Associated Macrophages
Macrophages are known for their immunomodulatory effects and they can be divided according to their phenotypes into M1- or M2-like states [ 244 , 245 ]. M1 macrophages secrete IL-12 and tumor necrosis factor with antimicrobial and antitumor effects. M2 macrophages produce cytokines, including IL-10, IL-1 receptor antagonist type II, and IL-1 decoy receptor. Therefore, macrophages with M1-like phenotype have been linked to good disease course while M2-like phenotype has been associated with adverse outcome, potentially through immunosuppression and the promotion of angiogenesis and tumor cell proliferation and invasion [ 246 , 247 ]. In literature, tumor-associated macrophages (TAMs) are associated with M2 macrophages which promote tumor growth and metastasis.
For breast cancer, studies have shown that the density of TAMs is related to hormone receptor status, stage, histologic grade, lymph node metastasis, and vascular invasion [ 248 , 249 , 250 , 251 ]. According to meta-analysis conducted by Zhao et al. high density of TAMs was related to overall survival disease-free survival [ 252 ].
Conversely, M1 polarized macrophages are linked to favorable prognoses in various cancers [ 253 , 254 , 255 ]. In breast cancer, the high density of M1-like macrophages predicted improved survival in patients with HER2+ phenotype and may be a potential prognostic marker [ 256 ].
However, further studies are needed to clarify the influence of macrophages on breast cancer biology as well as investigate the role of their intratumoral distribution and surface marker selection.
5.11. Inflammation-Based Models
The host inflammatory and immune responses in the tumor and its microenvironment are critical components in cancer development and progression [ 257 ]. The tumor-induced systemic inflammatory response leads to alterations of peripheral blood white blood cells [ 258 ]. Therefore, the relationship between peripheral blood inflammatory cells may serve as an accessible and early method of predicting patient prognosis. Recent studies have reported the predictive role of the inflammatory cell ratios: neutrophil-to-lymphocyte ratio, the lymphocyte-to-monocyte ratio, and the platelet-to-lymphocyte ratio for prognosis in different cancers [ 258 , 259 , 260 , 261 ].
5.11.1. The Neutrophil-to-Lymphocyte Ratio (NLR)
In an extensive study on 27,031 cancer patients, Proctor et al. analyzed the prognostic value of NLR and found a significant relationship between NLR and survival in various cancers including breast cancer [ 262 ]. There are pieces of evidence of the role of lymphocytes in breast cancer immunosurveillance [ 263 , 264 ]. Opposingly neutrophils suppress the cytolytic activity of lymphocytes, leading to enhanced angiogenesis and tumor growth and progression [ 265 ].
Azab et al. first reported that NLR before chemotherapy was an independent factor for long-term mortality and related it to age and tumor size in breast cancer [ 266 ]. In a recent meta-analysis by Guo et al., performed on 17,079 individuals, the high NLR level was associated with both poor overall survival as well as disease-free survival for breast cancer patients. Moreover, it was reported that association between NLR and overall survival was stronger in TNBC patients than in HER2-positive ones [ 267 ].
5.11.2. Lymphocyte-to-Monocyte Ratio
The association of the lymphocyte-to-monocyte ratio (LMR) with patients’ prognosis has been reported for several cancers [ 268 , 269 ]. As lymphocytes have an antitumor activity by inducing cytotoxic cell death and inhibiting tumor proliferation [ 270 ], the monocytes are involved in tumorigenesis, including differentiation into TAMs [ 246 , 247 , 271 ]. In the tumor microenvironment, cytokines, and free radicals that are secreted by monocytes and macrophages are associated with angiogenesis, tumor cell invasion, and metastasis [ 271 ].
A meta-analysis investigating the prognostic effect of LMR showed that low LMR levels are associated with shorter overall survival outcomes in Asian populations, TNBC patients, and patients with non-metastatic and mixed stages [ 272 ]. Moreover, high LMR levels are associated with favorable disease-free survival of breast cancer patients under neoadjuvant chemotherapy [ 273 ].
5.11.3. Platelet-to-Lymphocyte Ratio (PLR)
A high platelet count has been associated with poor prognosis in several types of cancers [ 274 , 275 , 276 ]. Platelets contain both pro-inflammatory molecules and cytokines (P-selectin, CD40L, and interleukin (IL)-1, IL-3, and IL-6) and many anti-inflammatory cytokines. Tumor angiogenesis and growth may be stimulated by the secretion of platelet-derived growth factor, vascular endothelial growth factor, transforming growth factor-beta, and platelet factor 4 [ 277 , 278 , 279 ].
A meta-analysis study investigated the prognostic importance of PLR by analyzing 5542 breast cancer patients. High PLR level was associated with poor prognosis (overall survival and disease-free survival), yet, its prognostic value was not determined for molecular subtypes of breast cancer. Nevertheless, an association was found between PLR and clinicopathological features of the tumor, including stage, lymph node metastasis, and distant metastasis [ 280 ]. In the aforementioned meta-analysis, there was a difference in the incidence of high levels of PLR between HER2 statuses [ 280 ], while other studies found a difference between hormone ER or PR statuses [ 281 , 282 ].
6. Treatment Strategies
6.1. surgery.
There are two major types of surgical procedures enabling the removal of breast cancerous tissues and those include (1) breast-conserving surgery (BCS) and (2) mastectomy. BCS—also called partial/segmental mastectomy, lumpectomy, wide local excision, or quadrantectomy—enables the removal of the cancerous tissue with simultaneous preservation of intact breast tissue often combined with plastic surgery technics called oncoplasty. Mastectomy is a complete removal of the breast and is often associated with immediately breast reconstruction. The removal of affected lymph nodes involves sentinel lymph node biopsy (SLNB) and axillary lymph node dissection (ALND). Even though BCS seems to be highly more beneficial for patients, those who were treated with this technique often show a tendency for a further need for a complete mastectomy [ 283 ]. However, usage of BCS is mostly related to significantly better cosmetic outcomes, lowered psychological burden of a patient, as well as reduced number of postoperative complications [ 284 ]. Guidelines of the European Society for Medical Oncology (ESMO) for patients with early breast cancer make the choice of therapy dependent to tumor size, feasibility of surgery, clinical phenotype, and patient’s willingness to preserve the breast [ 285 ].
6.2. Chemotherapy
Chemotherapy is a systemic treatment of BC and might be either neoadjuvant or adjuvant. Choosing the most appropriate one is individualized according to the characteristics of the breast tumor; chemotherapy might also be used in the secondary breast cancer. Neoadjuvant chemotherapy is used for locally advanced BC, inflammatory breast cancers, for downstaging large tumors to allow BCS or in small tumors with worse prognostics molecular subtypes (HER2 or TNBC) which can help to identify prognostics and predictive factors of response and can be provided intravenously or orally. Currently, treatment includes a simultaneous application of schemes 2–3 of the following drugs—carboplatin, cyclophosphamide, 5-fluorouracil/capecitabine, taxanes (paclitaxel, docetaxel), and anthracyclines (doxorubicin, epirubicin). The choice of the proper drug is of major importance since different molecular breast cancer subtypes respond differently to preoperative chemotherapy [ 286 ]. Preoperative chemotherapy is comparably effective to postoperative chemotherapy [ 287 ].
Even though chemotherapy is considered to be effective, its usage very often leads to several side effects including hair loss, nausea/vomiting, diarrhea, mouth sores, fatigue, increased susceptibility to infections, bone marrow supression, combined with leucopenia, anaemia, easier bruising or bleeding; other less frequent side effects include cardiomyopathy, neuropathy, hand-foot syndrome, impaired mental functions. In younger women, disruptions of the menstrual cycle and fertility issues might also appear. Special form of chemotherapy is electrochemotherapy which can be used in patients with breast cancer that has spread to the skin, however, it is still quite uncommon and not available in most clinics.
6.3. Radiation Therapy
Radiotherapy is local treatment of BC, typically provided after surgery and/or chemotherapy. It is performed to ensure that all of the cancerous cells remain destroyed, minimizing the possibility of breast cancer recurrence. Further, radiation therapy is favorable in the case of metastatic or unresectable breast cancer [ 288 ]. Choice of the type of radiation therapy depends on previous type of surgery or specific clinical situation; most common techniques include breast radiotherapy (always applied after BC), chest-wall radiotherapy (usually after mastectomy), and ‘breast boost’ (a boost of high-dose radiotherapy to the place of tumor bed as a complement of breast radiotherapy after BCS). Regarding breast radiotherapy specifically, several types are distinguished including
- (1) intraoperative radiation therapy (IORT)
- (2) 3D-conformal radiotherapy (3D-CRT)
- (3) intensity-modulated radiotherapy (IMRT)
- (4) brachytherapy—which refers to internal radiation in contrast to other above-mentioned techniques.
Irritation and darkening of the skin exposed to radiation, fatigue, and lymphoedema are one of the most common side effects of radiation therapy applied in breast cancer patients. Nonetheless, radiation therapy is significantly associated with the improvement of the overall survival rates of patients and lowered risk of recurrence [ 289 ].
6.4. Endocrinal (Hormonal) Therapy
Endocrinal therapy might be used either as a neoadjuvant or adjuvant therapy in patients with Luminal–molecular subtype of BC; it is effective in cases of breast cancer recurrence or metastasis. Since the expression of ERs, a very frequent phenomenon in breast cancer patients, its blockage via hormonal therapy is commonly used as one of the potential treatment modalities. Endocrinal therapy aims to lower the estrogen levels or prevents breast cancer cells to be stimulated by estrogen. Drugs that block ERs include selective estrogen receptor modulators (SERMs) (tamoxifen, toremifene) and selective estrogen receptor degraders (SERDs) (fulvestrant) while treatments that aim to lower the estrogen levels include aromatase inhibitors (AIs) (letrozole, anastrazole, exemestane) [ 290 , 291 ]. In the case of pre-menopausal women, ovarian suppression induced by oophorectomy, luteinizing hormone-releasing hormone analogs, or several chemotherapy drugs, are also effective in lowering estrogen levels [ 292 ]. However, approximately 50% of hormonoreceptor-positive breast cancer become progressively resistant to hormonal therapy during such treatment [ 293 ]. Endocrinal therapy combined with chemotherapy is associated with the reduction of mortality rates amongst breast cancer patients [ 294 ].
6.5. Biological Therapy
Biological therapy (targeted therapy) can be provided at every stage of breast therapy– before surgery as neoadjuvant therapy or after surgery as adjuvant therapy. Biological therapy is quite common in HER2-positive breast cancer patients; major drugs include trastuzumab, pertuzumab, trastuzumab deruxtecan, lapatinib, and neratinib [ 295 , 296 , 297 , 298 , 299 ]. Further, the efficacy of angiogenesis inhibitors such as a recombinant humanized monoclonal anti-VEGF antibody (rhuMAb VEGF) or bevacizumab are continuously investigated [ 300 ].
In the case of Luminal, HER2-negative breast cancer, pre-menopausal women more often receive everolimus -TOR inhibitor with exemestane while postmenopausal women often receive CDK 4–6 inhibitor palbociclib or ribociclib simultaneously, combined with hormonal therapy [ 301 , 302 , 303 ]. Two penultimate drugs along with abemaciclib and everolimus can also be used in HER2-negative and estrogen-positive breast cancer [ 304 , 305 ]. Atezolizumab is approved in triple-negative breast cancer, while denosumab is approved in case of metastasis to the bones [ 306 , 307 , 308 ].
7. Conclusions
In this review, we aimed to summarize and update the current knowledge about breast cancer with an emphasis on its current epidemiology, risk factors, classification, prognostic biomarkers, and available treatment strategies. Since both the morbidity and mortality rates of breast cancer have significantly increased over the past decades, it is an urgent need to provide the most effective prevention taking into account that modifiable risk factors might be crucial in providing the reduction of breast cancer incidents. So far, mammography and sonography is the most common screening test enabling quite an early detection of breast cancer. The continuous search for prognostic biomarkers and targets for the potential biological therapies has significantly contributed to the improvement of management and clinical outcomes of breast cancer patients.
Author Contributions
Conceptualization, A.F., R.S. and A.S.; critical review of literature, S.Ł., M.C., A.F., J.B., R.S., A.S.; writing—original draft preparation, M.C., A.F.; writing—review and editing, S.Ł., M.C., A.F., J.B., R.S., A.S.; supervision, R.S. All authors have read and agreed to the published version of the manuscript.
This research received no external funding.
Conflicts of Interest
The authors declare no conflict of interest.
Publisher’s Note: MDPI stays neutral with regard to jurisdictional claims in published maps and institutional affiliations.
Log in using your username and password
- Search More Search for this keyword Advanced search
- Latest content
- Current issue
- BMJ Journals More You are viewing from: Google Indexer
You are here
- Online First
- Pedestrian safety on the road to net zero: cross-sectional study of collisions with electric and hybrid-electric cars in Great Britain
- Article Text
- Article info
- Citation Tools
- Rapid Responses
- Article metrics

- http://orcid.org/0000-0003-4431-8822 Phil J Edwards ,
- Siobhan Moore ,
- Craig Higgins
- London School of Hygiene & Tropical Medicine , London , UK
- Correspondence to Dr Phil J Edwards, London School of Hygiene & Tropical Medicine, London WC1E 7HT, UK; phil.edwards{at}LSHTM.ac.uk
Background Plans to phase out fossil fuel-powered internal combustion engine (ICE) vehicles and to replace these with electric and hybrid-electric (E-HE) vehicles represent a historic step to reduce air pollution and address the climate emergency. However, there are concerns that E-HE cars are more hazardous to pedestrians, due to being quieter. We investigated and compared injury risks to pedestrians from E-HE and ICE cars in urban and rural environments.
Methods We conducted a cross-sectional study of pedestrians injured by cars or taxis in Great Britain. We estimated casualty rates per 100 million miles of travel by E-HE and ICE vehicles. Numerators (pedestrians) were extracted from STATS19 datasets. Denominators (car travel) were estimated by multiplying average annual mileage (using National Travel Survey datasets) by numbers of vehicles. We used Poisson regression to investigate modifying effects of environments where collisions occurred.
Results During 2013–2017, casualty rates per 100 million miles were 5.16 (95% CI 4.92 to 5.42) for E-HE vehicles and 2.40 (95%CI 2.38 to 2.41) for ICE vehicles, indicating that collisions were twice as likely (RR 2.15; 95% CI 2.05 to 2.26) with E-HE vehicles. Poisson regression found no evidence that E-HE vehicles were more dangerous in rural environments (RR 0.91; 95% CI 0.74 to 1.11); but strong evidence that E-HE vehicles were three times more dangerous than ICE vehicles in urban environments (RR 2.97; 95% CI 2.41 to 3.7). Sensitivity analyses of missing data support main findings.
Conclusion E-HE cars pose greater risk to pedestrians than ICE cars in urban environments. This risk must be mitigated as governments phase out petrol and diesel cars.
- WOUNDS AND INJURIES
- CLIMATE CHANGE
Data availability statement
Data are available in a public, open-access repository. Numerator data (numbers of pedestrians injured in collisions) are publicly available from the Road Safety Data (STATS19) datasets ( https://www.data.gov.uk/dataset/cb7ae6f0-4be6-4935-9277-47e5ce24a11f/road-safety-data ). Denominator data (100 million miles of car travel per year) may be estimated by multiplying average annual mileage by numbers of vehicle registrations (publicly available from Department for Transport, https://www.gov.uk/government/statistical-data-sets/veh02-licensed-cars ). Average annual mileage for E-HE and ICE vehicles may be estimated separately for urban and rural environments using data that may obtained under special licence from the National Travel Survey datasets ( http://doi.org/10.5255/UKDA-Series-2000037 ).
https://doi.org/10.1136/jech-2024-221902
Statistics from Altmetric.com
Request permissions.
If you wish to reuse any or all of this article please use the link below which will take you to the Copyright Clearance Center’s RightsLink service. You will be able to get a quick price and instant permission to reuse the content in many different ways.
WHAT IS ALREADY KNOWN ON THIS TOPIC
Electric cars are quieter than cars with petrol or diesel engines and may pose a greater risk to pedestrians.
The US National Highway Transportation Safety Agency found that during 2000–2007 the odds of an electric or hybrid-electric car causing a pedestrian injury were 35% greater than a car with a petrol or diesel engine.
The UK Transport Research Laboratory found the pedestrian casualty rate per 10 000 registered electric or hybrid-electric vehicles during 2005–2007 in Great Britain was lower than the rate for petrol or diesel vehicles.
WHAT THIS STUDY ADDS
In Great Britain during 2013–2017, pedestrians were twice as likely to be hit by an electric or hybrid-electric car than by a petrol or diesel car; the risks were higher in urban areas.
HOW THIS STUDY MIGHT AFFECT RESEARCH, PRACTICE OR POLICY
The greater risk to pedestrian safety posed by electric or hybrid-electric cars needs to be mitigated as governments proceed to phase out petrol and diesel cars.
Drivers of electric or hybrid-electric cars must be cautious of pedestrians who may not hear them approaching and may step into the road thinking it is safe to do so, particularly in towns and cities.
Introduction
Many governments have set targets to reach net-zero emissions to help mitigate the harms of climate change. Short-term health benefits of reduced emissions are expected from better air quality with longer-term benefits from reduced global temperatures. 1
Transition to electric and hybrid-electric (E-HE) cars
One such target is to phase out sales of new fossil fuel-powered internal combustion engine (ICE) vehicles and replace these with E-HE vehicles. 2 3
Pedestrian safety
Road traffic injuries are the leading cause of death for children and young adults. 4 A quarter of all road traffic deaths are of pedestrians. 5 Concerns have been raised that E-HE cars may be more hazardous to pedestrians than ICE cars, due to being quieter. 6 7 It has been hypothesised that E-HE cars pose a greater risk of injury to pedestrians in urban areas where background ambient noise levels are higher. 8 However, there has been relatively little empirical research on possible impacts of E-HE cars on pedestrian road safety. A study commissioned for the US National Highway Transportation Safety Agency based on data from 16 States found that the odds of an E-HE vehicle causing a pedestrian injury were 35% greater than an ICE vehicle. 9 In contrast, a study commissioned by the UK Department for Transport found pedestrian casualty rates from collisions with E-HE vehicles during 2005–2007 were lower than for ICE vehicles. 10 Possible reasons for these conflicting results are that the two studies used different designs and estimated different measures of relative risk—the first used a case–control design and estimated an OR, whereas the second used a cross-sectional study and estimated a rate ratio. ORs will often differ from rate ratios. 11 Other reasons include differences between the USA and the UK in the amount and quality of walking infrastructure. 12
Aim and objectives
We aimed to add to the evidence base on whether E-HE cars pose a greater injury risk to pedestrians than ICE cars by analysing road traffic injury data and travel survey data in Great Britain.
We sought to improve on the previous UK study by using distance travelled instead of number of registered vehicles as the measure of exposure in estimation of collision rates.
The objectives of this study were:
To estimate pedestrian casualty rates for E-HE and ICE vehicles and to compare these by calculating a rate ratio;
To assess whether or not the evidence supports the hypothesis that casualty rate ratios vary according to urban or rural environments. 8
Study design
This study was an analysis of differences in casualty rates of pedestrians per 100 million miles of E-HE car travel and rates per 100 million miles of ICE car travel.
This study was set in Great Britain between 2013 and 2017.
Participants
The study participants were all pedestrians reported to have been injured in a collision with a car or a taxi.
The exposure was the type of propulsion of the colliding vehicle, E-HE or ICE. E-HE vehicles were treated as a single powertrain type, regardless of the mode of operation that a hybrid vehicle was in at the time of collision (hybrid vehicles typically start in electric mode and change from battery to combustion engine at higher speeds). 13
The outcome of interest was a pedestrian casualty.
Effect modification by road environment
We used the urban–rural classification 14 of the roads on which the collisions occurred to investigate whether casualty rate ratios comparing E-HE with ICE vehicles differed between rural and urban environments.
Data sources/measurement
Numerator data (numbers of pedestrians injured in collisions) were extracted from the Road Safety Data (STATS19) datasets. 15
Denominator data (100 million miles of car travel per year) were estimated by multiplying average annual mileage by numbers of vehicle registrations. 16 Average annual mileage for E-HE and ICE vehicles was estimated separately for urban and rural environments using data obtained under special licence from the National Travel Survey (NTS) datasets. 17 We estimated average annual mileage for the years 2013–2017 because the NTS variable for the vehicle fuel type did not include ‘hybrid’ prior to 2013 and data from 2018 had not been uploaded to the UK data service due to problems with the archiving process (Andrew Kelly, Database Manager, NTS, Department for Transport, 23 March 2020, personal communication). Denominators were thus available for the years 2013–2017.
Data preparation
The datasets for collisions, casualties and vehicles from the STATS19 database were merged using a unique identification number for each collision.
Statistical methods
We calculated annual casualty rates for E-HE and ICE vehicles separately and we compared these by calculating a rate ratio. We used Poisson regression models to estimate rate ratios with 95% CIs and to investigate any modifying effects of the road environment in which the collisions occurred. For this analysis, our regression model included explanatory terms for the main effects of the road environment, plus terms for the interaction between type of propulsion and the road environment. The assumptions for Poisson regression were met in our study: we modelled count data (counts of pedestrians injured), traffic collisions were independent of each other, occurring in different places over time, and never occurring simultaneously. Data preparation, management and analyses were carried out using Microsoft Access 2019 and Stata V.16. 18
Sensitivity analysis
We conducted an extreme case analysis where all missing propulsion codes were assumed to be ICE vehicles (there were over a 100 times more ICE vehicles than E-HE vehicles on the roads in Great Britain during our study period, 16 so missing propulsion is more likely to have been ICE).
The sample size for this study included all available recorded road traffic collisions in Great Britain during the study period. We estimated that for our study to have 80% power at the 5% significance level to show a difference in casualty rates of 2 per 100 miles versus 5.5 per 100 miles, we would require 481 million miles of vehicle travel in each group (E-HE and ICE); whereas to have 90% power at the 1% significance level to show this difference, 911 million miles of vehicle travel would be required in each group. Our study includes 32 000 million miles of E-HE vehicle travel and 3 000 000 million miles of ICE vehicle travel and therefore our study was sufficiently powered to detect differences in casualty rates of these magnitudes.
Between 2013 and 2017, there were 916 713 casualties from reported road traffic collisions in Great Britain. 120 197 casualties were pedestrians. Of these pedestrians, 96 285 had been hit by a car or taxi. Most pedestrians—71 666 (74%) were hit by an ICE car or taxi. 1652 (2%) casualties were hit by an E-HE car or taxi. For 22 829 (24%) casualties, the vehicle propulsion code was missing. Most collisions occurred in urban environments and a greater proportion of the collisions with E-HE vehicles occurred in an urban environment (94%) than did collisions with ICE vehicles (88%) ( figure 1 ).
- Download figure
- Open in new tab
- Download powerpoint
Flow chart of pedestrian casualties in collisions with E-HE or ICE cars or taxis from reported road traffic collisions in Great Britain 2013–2017. E-HE, electric and hybrid-electric; ICE, internal combustion engine.
Main results
During the period 2013 to 2017, the average annual casualty rates of pedestrians per 100 million miles were 5.16 (95% CI 4.92 to 5.42) for E-HE vehicles and 2.40 (95% CI 2.38 to 2.41) for ICE vehicles, which indicates that collisions with pedestrians were on average twice as likely (RR 2.15 (95% CI 2.05 to 2.26), p<0.001) with E-HE vehicles as with ICE vehicles ( table 1 ).
- View inline
Pedestrian casualties due to collisions with cars or taxis from reported road traffic collisions in Great Britain 2013–2017—by vehicle propulsion type
In our extreme case analysis, the 22 829 pedestrian casualties where vehicle propulsion was missing were all assumed to have been struck by ICE vehicles. In this case, average casualty rates of pedestrians per 100 million miles were 3.16 (95% CI 3.14 to 3.18) for ICE vehicles, which would indicate that collisions with pedestrians were on average 63% more likely (RR 1.63 (95% CI 1.56 to 1.71), p<0.001) with E-HE vehicles than with ICE vehicles ( table 2 ).
Extreme case sensitivity analysis—pedestrian casualties due to collisions with cars or taxis from reported road traffic collisions in Great Britain 2013–2017 by vehicle propulsion type where 22 829 missing vehicle propulsion codes are assumed to be ICE vehicles
Relative risks according to road environment
Casualty rates were higher in urban than rural environments ( tables 3 and 4 ).
Pedestrian casualties due to collisions with cars or taxis from reported road traffic collisions in Great Britain 2013–2017—by vehicle propulsion type in urban road environments
Pedestrian casualties due to collisions with cars or taxis from reported road traffic collisions in Great Britain 2013–2017—by vehicle propulsion type in rural road environments
Urban environments
Collisions with pedestrians in urban environments were on average over two and a half times as likely (RR 2.69 (95% CI 2.56 to 2.83, p<0.001) with E-HE vehicles as with ICE vehicles ( table 3 ).
The extreme case sensitivity analysis showed collisions with pedestrians in urban environments were more likely with E-HE vehicles (RR 2.05; 95% CI 1.95 to 2.15).
Rural environments
Collisions with pedestrians in rural environments were equally likely (RR 0.91; 95% CI 0.74 to 1.11) with E-HE vehicles as with ICE vehicles ( table 4 ).
The extreme case sensitivity analysis found evidence that collisions with pedestrians in rural environments were less likely with E-HE vehicles (RR 0.68; 95% CI 0.55 to 0.83).
Results of Poisson regression analysis
Our Poisson regression model results ( table 5 ) showed that pedestrian injury rates were on average 9.28 (95% CI 9.07 to 9.49) times greater in urban than in rural environments. There was no evidence that E-HE vehicles were more dangerous than ICE vehicles in rural environments (RR 0.91; 95% CI 0.74 to 1.11), consistent with our finding in table 4 . There was strong evidence that E-HE vehicles were on average three times more dangerous than ICE vehicles in urban environments (RR 2.97; 95% CI 2.41 to 3.67).
Results of Poisson regression analysis of annual casualty rates of pedestrians per 100 million miles by road environment and the interaction between vehicle propulsion type and environment
Statement of principal findings
This study found that in Great Britain between 2013 and 2017, casualty rates of pedestrians due to collisions with E-HE cars and taxis were higher than those due to collisions with ICE cars and taxis. Our best estimate is that such collisions are on average twice as likely, and in urban areas E-HE vehicles are on average three times more dangerous than ICE vehicles, consistent with the theory that E-HE vehicles are less audible to pedestrians in urban areas where background ambient noise levels are higher.
Strengths and weaknesses of the study
There are several limitations to this study which are discussed below.
The data used were not very recent. However, ours is the most current analysis of E-HE vehicle collisions using the STATS19 dataset.
Before we can infer that E-HE vehicles pose a greater risk to pedestrians than ICE vehicles, we must consider whether our study is free from confounding and selection bias. Confounding occurs when the exposure and outcome share a common cause. 19 Confounders in this study would be factors that may both cause a traffic collision and also cause the exposure (use of an E-HE car). Younger, less experienced drivers (ie, ages 16–24) are more likely to be involved in a road traffic collision 20 and are also more likely to own an electric car. 21 Some of the observed increased risk of electric cars may therefore be due to younger drivers preferring electric cars. This would cause positive confounding, meaning that the true relative risk of electric cars is less than we have estimated in our study. Regarding selection bias, it is known that the STATS19 dataset does not include every road traffic casualty in Great Britain, as some non-fatal casualties are not reported to the police. 22 If casualties from collisions are reported to the police differentially according to the type of vehicle propulsion, this may have biased our results; however, there is no reason to suspect that a pedestrian struck by a petrol or diesel car is any more or less likely to report the collision to the police than one struck by an electric car.
We must also address two additional concerns as ours is a cross-sectional study: The accuracy of exposure assignment (including the potential for recall bias) and the adequacy of prevalence as a proxy for incidence. 23 First, the accuracy of exposure assignment and the potential for recall bias are not issues for this study, as the exposure (type of propulsion of the colliding vehicle, E-HE or ICE), is assigned independently of the casualties by the UK Department for Transport who link the vehicle registration number (VRN) of each colliding vehicle to vehicle data held by the UK Driver Vehicle and Licensing Agency (DVLA). 10 Second, we have not used prevalence as a proxy for incidence but have estimated incidence using total distance travelled by cars as the measure of exposure.
We may therefore reasonably infer from our study results that E-HE vehicles pose a greater risk to pedestrians than ICE vehicles in urban environments, and that part of the risk may be due to younger people’s preference for E-HE cars.
A major limitation of the STATS19 road safety dataset used in this study was that it did not contain a vehicle propulsion code for all vehicles in collisions with pedestrians. We excluded these vehicles from our primary analysis (a complete case analysis) and we also conducted an extreme case sensitivity analysis. We will now argue why imputation of missing vehicle propulsion codes would not have added value to this study. Vehicle propulsion data are obtained for the STATS19 dataset by the UK Department for Transport who link the VRN of each colliding vehicle recorded in STATS19 to vehicles data held by the UK DVLA. The STATS19 data on reported collisions and casualties are collected by a Police Officer when an injury road accident is reported to them; Most police officers write details of the casualties and the vehicles involved in their notebooks for transcription onto the STATS19 form later at the Police station. 24 The VRN is one of 18 items recorded on each vehicle involved in a collision. Items may occasionally be missed due to human error during this process. Where a VRN is missing, vehicle propulsion will be missing in the STATS19 dataset. The chance that any vehicle-related item is missing will be independent of any characteristics of the casualties involved and so the vehicle propulsion codes are missing completely at random (MCAR). As the missing propulsion data are very likely MCAR, the set of pedestrians with no missing data is a random sample from the source population and hence our complete case analysis for handling the missing data gives unbiased results. The extreme case sensitivity analysis we performed shows a possible result that could occur, and it demonstrates our conclusions in urban environments are robust to the missing data. Lastly, to impute the missing data would require additional variables which are related to the likelihood of a VRN being missing. Such variables were not available and therefore we do not believe a useful multiple imputation analysis could have been performed.
Strengths and weaknesses in relation to other studies
Our study uses hundreds of millions of miles of car travel as the denominators in our estimates of annual pedestrian casualty rates which is a more accurate measure of exposure to road hazards than the number of registered vehicles, which was used as the denominator in a previous study in the UK. 10 Our results differ to this previous study which found that pedestrian casualty rates from collisions with E-HE vehicles during 2005–2007 were lower than those from ICE vehicles. Our study has updated this previous analysis and shows that casualty rates due to E-HE vehicle collisions exceed those due to ICE vehicle collisions. Similarly, our study uses a more robust measure of risk (casualty rates per miles of car travel) than that used in a US study. 9 Our study results are consistent with this US study that found that the odds of an E-HE vehicle causing a pedestrian injury were 35% greater than an ICE vehicle. Brand et al 8 hypothesised, without any supporting data, that “hybrid and electric low-noise cars cause an increase in traffic collisions involving vulnerable road users in urban areas” and recommended that “further investigations have to be done with the increase of low-noise cars to prove our hypothesis right.” 8 We believe that our study is the first to provide empirical evidence in support of this hypothesis.
Meaning of the study: possible explanations and implications for clinicians and policymakers
More pedestrians are injured in Great Britain by petrol and diesel cars than by electric cars, but compared with petrol and diesel cars, electric cars pose a greater risk to pedestrians and the risk is greater in urban environments. One plausible explanation for our results is that background ambient noise levels differ between urban and rural areas, causing electric vehicles to be less audible to pedestrians in urban areas. Such differences may impact on safety because pedestrians usually hear traffic approaching and take care to avoid any collision, which is more difficult if they do not hear electric vehicles. This is consistent with audio-testing evidence in a small study of vision-impaired participants. 10 From a Public Health perspective, our results should not discourage active forms of transport beneficial to health, such as walking and cycling, rather they can be used to ensure that any potential increased traffic injury risks are understood and safeguarded against. A better transport policy response to the climate emergency might be the provision of safe, affordable, accessible and integrated public transport systems for all. 25
Unanswered questions and future research
It will be of interest to investigate the extent to which younger drivers are involved in collisions of E-HE cars with pedestrians.
If the braking distance of electric cars is longer, 26 and electric cars are heavier than their petrol and diesel counterparts, 27 these factors may increase the risks and the severity of injuries sustained by pedestrians and require investigation.
As car manufacturers continue to develop and equip new electric cars with Collision Avoidance Systems and Autonomous Emergency Braking to ensure automatic braking in cases where pedestrians or cyclists move into the path of an oncoming car, future research can repeat the analyses presented in this study to evaluate whether the risks of E-HE cars to pedestrians in urban areas have been sufficiently mitigated.
Conclusions
E-HE vehicles pose a greater risk to pedestrians than petrol and diesel powered vehicles in urban environments. This risk needs to be mitigated as governments proceed to phase out petrol and diesel cars.
Ethics statements
Patient consent for publication.
Not applicable.
Ethics approval
This study involves human participants and was approved by the LSHTM MSc Research Ethics Committee (reference #16400). The study uses the anonymised records of people injured in road traffic collisions, data which are routinely collected by UK police forces. The participants are unknown to the investigators and could not be contacted.
Acknowledgments
We thank Rebecca Steinbach for her advice on analysis of National Travel Survey data, Jonathan Bartlett for his advice on missing data, and Ben Armstrong for his advice on Poisson regression. We are grateful to the reviewers and to Dr C Mary Schooling, Associate Editor, whose comments helped us improve the manuscript. We are grateful to Jim Edwards and Graham Try for their comments on earlier versions of this manuscript.
- H Baqui A ,
- Benfield T , et al
- Gilchrist J
- ↵ WHO factsheet on road traffic injuries . Available : https://www.who.int/news-room/fact-sheets/detail/road-traffic-injuries#:~:text=Approximately%201.19%20million%20people%20die,adults%20aged%205%E2%80%9329%20years [Accessed 14 Apr 2024 ].
- ↵ Reported road casualties great Britain, annual report . 2022 . Available : https://www.gov.uk/government/statistics/reported-road-casualties-great-britain-annual-report-2022 [Accessed 14 Apr 2024 ].
- Maryland General Assembly
- Haas P , et al
- Morgan PA ,
- Muirhead M , et al
- Greenland S
- Buehler R ,
- Alternative Fuels Data Center
- Government-Statistics
- Department for Transport
- Department for Transport. (2023
- Hernán MA ,
- Hernández-Díaz S ,
- Barriers Direct
- Savitz DA ,
- Wellenius GA
- Transport Scotland
Contributors CH and PJE developed the idea for this study and supervised SM in performing the literature search, downloading, managing and analysing the data. SM wrote the first draft of the manuscript, which was the dissertation for her MSc in Public Health. PJE prepared the first draft of the manuscript for the journal. All authors assisted in editing and refining the manuscript. The corresponding author attests that all listed authors meet authorship criteria and that no others meeting the criteria have been omitted. PJE (guarantor) accepts full responsibility for the work and the conduct of the study, had access to the data and controlled the decision to publish.
Funding This study was conducted in part fulfilment of the Masters degree in Public Health at the London School of Hygiene & Tropical Medicine. The second author was self-funded for her studies for this degree.
Competing interests None declared.
Provenance and peer review Not commissioned; externally peer reviewed.
Read the full text or download the PDF:
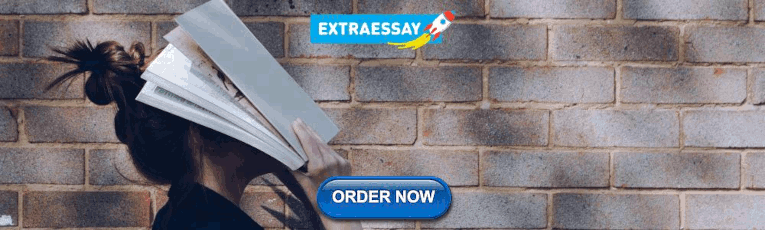
COMMENTS
8 Epidemiology: an introduction. This book. This book is intended to provide a practical yet critically informed review. of epidemiology. We consider epidemiology both as a toolkit of research ...
The American Journal of Epidemiology is publishing timely, high-quality articles to further the scientific discourse about COVID-19 and the understanding of the pandemic. Explore the papers. An official journal of John Hopkins Bloomberg School of Public Health. Publishes empirical research findings, opinion pieces, and methodological.
1 PDF. -- (Global burden of disease and injury series ; volume 4) 1.Communicable diseases - epidemiology I. Murray, Christopher J. L. ... viii Global Epidemiology of Infectious Diseases women immunized with at least 2 doses of tetanus toxoid, by WHO Region, 1993.....186 Chapter 7 7.1 Detection and prevalence of leprosy in five African ...
The classical definition of Greek origin. Epi -upon. Domos - the people. Ology - the study of. "the study of epidemics". Seven Uses of Epidemiology. To study the history of the health of the population. To diagnose the health of the community. To study the working of health services.
Basic epidemiology starts with a definition of epidemiology, introduces the his-tory of modern epidemiology, and provides examples of the uses and applications of epidemiology. Measurement of exposure and disease are covered in Chapter 2 and a summary of the different types of study designs and their strengths and limitations is provided in ...
the contributions of epidemiology to public health with the example of HIV/AIDS. 5.2.1 Example: Epidemiology and the HIV/AIDS Epidemic A good example of the important role of epidemiology in collaboration with other relevant public health and medical disciplines is the research upon the AIDS epi-demic.
1. INTRODUCTION. On 11 March, the World Health Organization (WHO) declared the coronavirus disease 2019 (COVID‐19) outbreak caused by the severe acute respiratory syndrome coronavirus 2 (SARS‐CoV‐2) a pandemic. 1 Currently, the deadly COVID‐19 has no effective therapy or vaccine. In addition, the signs of having COVID‐19 are nonspecific or can be absent, adding challenges to disease ...
Abstract. The severe acute respiratory syndrome coronavirus 2 (SARS-CoV-2), a zoonotic infection, is responsible for COVID-19 pandemic and also is known as a public health concern. However, so far, the origin of the causative virus and its intermediate hosts is yet to be fully determined. SARS-CoV-2 contains nearly 30,000 letters of RNA that ...
As an introduction to quantitative epidemiology, this chapter consists of 9 sections, covering key concepts and major tasks of epidemiology, paradigm of quantitative epidemiology, population , study population, sample, and sampling methods; methods to identify a problem, frame a problem into a research question, defend a selected topic by considering significance, innovation, feasibility, and ...
Chris Bain, MB BS (UQ), MPH, MSc (Harvard) is Reader in Epidemiology in the School of Population Health, University of Queensland. He has been teaching epidemiology to public health and medical students for over 3 decades and has co-authored a book on how to conduct a systematic review as well as more than 100 original epidemiology research papers.
Publishes papers on epidemiological advances and new developments throughout the. ... The IEA aim to facilitate communication amongst those engaged in research and teaching of epidemiology throughout the world, and to encourage its use in all fields of health including social, community, and preventative medicine. ... This PDF is available to ...
Epidemiology publishes original research from all fields of epidemiology. The journal also welcomes review articles and meta-analyses, novel hypotheses, descriptions and applications of new methods, and discussions of research theory or public health policy. We give special consideration to papers from developing countries. Official Journal of the The International Society for Environmental ...
According to the International Agency for Research on Cancer (IARC), in 2020 there were approximately 19.3 million new cases of cancer, and 10 million deaths by this disease, 6 while 23.8 million cases and 13.0 million deaths are projected to occur by 2030. 73 In this regard, it is clear the increasing role that environmental factors ...
A well-defined research question (causal or descriptive) states: 1) the target population, characterized by person and place, and anchored in time; 2) the outcome, event, or health state or characteristic; and 3) the measure of occurrence that will be used to summarize the outcome (e.g., incidence, prevalence, average time to event, etc.).
Principles of Epidemiology in Public Health Practice Third Edition An Introduction to Applied Epidemiology and Biostatistics ... Research has shown that these factors greatly influence learning ability. Each lesson in the course consists of reading, exercises, and a self-assessment quiz.
The thesis demonstrates the student's comprehensive knowledge of the substantive area of the study and the research methods used. It also represents the culminating product of the master's program in which students are expected to integrate and apply the concepts and methods learned in coursework.". 4.
Read the latest Research articles in Epidemiology from Scientific Reports. ... Changing epidemiology of parvovirus B19 in the Netherlands since 1990, including its re-emergence after the COVID-19 ...
Drafting a research paper 2017 3 | P a g e Background Publishing papers is a key part of an effective strategy to disseminate research results and communicate with your peers. The number of papers published in journals is increasing, as is the competition in getting a paper accepted in journals, with increasingly high rejection rates.
Gary Maartens, Connie Celum, Sharon R Lewin. HIV prevalence is increasing worldwide because people on antiretroviral therapy are living longer, although new infections decreased from 3·3 million in 2002, to 2·3 million in 2012. Global AIDS-related deaths peaked at 2·3 million in 2005, and decreased to 1·6 million by 2012.
2. ]. In this issue, after an introductory paper by Kotz et al, Kotz and Cals publish the first of a series of monthly compact one-page papers, each highlighting an essential step in preparing and writing a research paper. This series, containing a total of 12 one-pagers, originates from a PhD student course organized at Maastricht University ...
Here, using an agnostic, hybrid-capture sequencing approach, we report the detection of H5N1 in wastewater in nine Texas cities, with a total catchment area population in the millions, over a two-month period from March 4 th to April 25 th, 2024. Sequencing reads uniquely aligning to H5N1 covered all eight genome segments, with best alignments ...
Appendix A: Type and Duration of Precautions Recommended for Selected Infections and Conditions. Appendix A: Table 1. History of Guidelines for Isolation Precautions in Hospitals. Appendix A: Table 2. Clinical Syndromes or Conditions Warranting Empiric Transmission-Based Precautions in Addition to Standard Precautions. Appendix A. Table 3.
The validation studies involving the reassessment of the Surveillance, Epidemiology, and End Results (SEER) database (n = 209,304, 2010-2014) and the University of Texas MD Anderson Cancer Center database (n = 3327, years of treatment 2007-2013) according to 8th edition AJCC manual proved the more accurate prognostic information [189,190].
Background Plans to phase out fossil fuel-powered internal combustion engine (ICE) vehicles and to replace these with electric and hybrid-electric (E-HE) vehicles represent a historic step to reduce air pollution and address the climate emergency. However, there are concerns that E-HE cars are more hazardous to pedestrians, due to being quieter. We investigated and compared injury risks to ...