Click through the PLOS taxonomy to find articles in your field.
For more information about PLOS Subject Areas, click here .
Loading metrics
Open Access
Peer-reviewed
Research Article
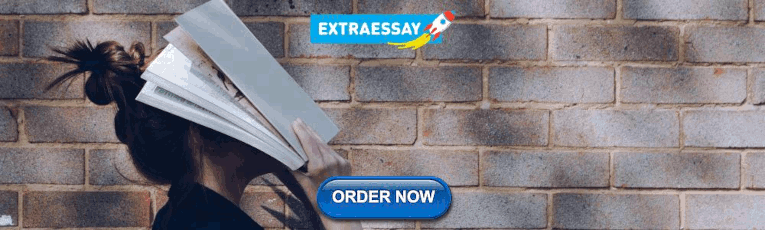
Recent quantitative research on determinants of health in high income countries: A scoping review
Roles Conceptualization, Data curation, Formal analysis, Investigation, Methodology, Project administration, Software, Visualization, Writing – original draft, Writing – review & editing
* E-mail: [email protected]
Affiliation Centre for Health Economics Research and Modelling Infectious Diseases, Vaccine and Infectious Disease Institute, University of Antwerp, Antwerp, Belgium

Roles Conceptualization, Data curation, Funding acquisition, Project administration, Resources, Supervision, Validation, Visualization, Writing – review & editing
- Vladimira Varbanova,
- Philippe Beutels
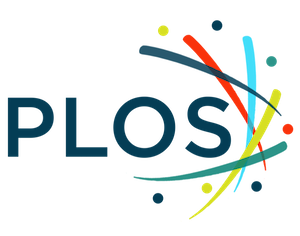
- Published: September 17, 2020
- https://doi.org/10.1371/journal.pone.0239031
- Peer Review
- Reader Comments
Identifying determinants of health and understanding their role in health production constitutes an important research theme. We aimed to document the state of recent multi-country research on this theme in the literature.
We followed the PRISMA-ScR guidelines to systematically identify, triage and review literature (January 2013—July 2019). We searched for studies that performed cross-national statistical analyses aiming to evaluate the impact of one or more aggregate level determinants on one or more general population health outcomes in high-income countries. To assess in which combinations and to what extent individual (or thematically linked) determinants had been studied together, we performed multidimensional scaling and cluster analysis.
Sixty studies were selected, out of an original yield of 3686. Life-expectancy and overall mortality were the most widely used population health indicators, while determinants came from the areas of healthcare, culture, politics, socio-economics, environment, labor, fertility, demographics, life-style, and psychology. The family of regression models was the predominant statistical approach. Results from our multidimensional scaling showed that a relatively tight core of determinants have received much attention, as main covariates of interest or controls, whereas the majority of other determinants were studied in very limited contexts. We consider findings from these studies regarding the importance of any given health determinant inconclusive at present. Across a multitude of model specifications, different country samples, and varying time periods, effects fluctuated between statistically significant and not significant, and between beneficial and detrimental to health.
Conclusions
We conclude that efforts to understand the underlying mechanisms of population health are far from settled, and the present state of research on the topic leaves much to be desired. It is essential that future research considers multiple factors simultaneously and takes advantage of more sophisticated methodology with regards to quantifying health as well as analyzing determinants’ influence.
Citation: Varbanova V, Beutels P (2020) Recent quantitative research on determinants of health in high income countries: A scoping review. PLoS ONE 15(9): e0239031. https://doi.org/10.1371/journal.pone.0239031
Editor: Amir Radfar, University of Central Florida, UNITED STATES
Received: November 14, 2019; Accepted: August 28, 2020; Published: September 17, 2020
Copyright: © 2020 Varbanova, Beutels. This is an open access article distributed under the terms of the Creative Commons Attribution License , which permits unrestricted use, distribution, and reproduction in any medium, provided the original author and source are credited.
Data Availability: All relevant data are within the manuscript and its Supporting Information files.
Funding: This study (and VV) is funded by the Research Foundation Flanders ( https://www.fwo.be/en/ ), FWO project number G0D5917N, award obtained by PB. The funder had no role in study design, data collection and analysis, decision to publish, or preparation of the manuscript.
Competing interests: The authors have declared that no competing interests exist.
Introduction
Identifying the key drivers of population health is a core subject in public health and health economics research. Between-country comparative research on the topic is challenging. In order to be relevant for policy, it requires disentangling different interrelated drivers of “good health”, each having different degrees of importance in different contexts.
“Good health”–physical and psychological, subjective and objective–can be defined and measured using a variety of approaches, depending on which aspect of health is the focus. A major distinction can be made between health measurements at the individual level or some aggregate level, such as a neighborhood, a region or a country. In view of this, a great diversity of specific research topics exists on the drivers of what constitutes individual or aggregate “good health”, including those focusing on health inequalities, the gender gap in longevity, and regional mortality and longevity differences.
The current scoping review focuses on determinants of population health. Stated as such, this topic is quite broad. Indeed, we are interested in the very general question of what methods have been used to make the most of increasingly available region or country-specific databases to understand the drivers of population health through inter-country comparisons. Existing reviews indicate that researchers thus far tend to adopt a narrower focus. Usually, attention is given to only one health outcome at a time, with further geographical and/or population [ 1 , 2 ] restrictions. In some cases, the impact of one or more interventions is at the core of the review [ 3 – 7 ], while in others it is the relationship between health and just one particular predictor, e.g., income inequality, access to healthcare, government mechanisms [ 8 – 13 ]. Some relatively recent reviews on the subject of social determinants of health [ 4 – 6 , 14 – 17 ] have considered a number of indicators potentially influencing health as opposed to a single one. One review defines “social determinants” as “the social, economic, and political conditions that influence the health of individuals and populations” [ 17 ] while another refers even more broadly to “the factors apart from medical care” [ 15 ].
In the present work, we aimed to be more inclusive, setting no limitations on the nature of possible health correlates, as well as making use of a multitude of commonly accepted measures of general population health. The goal of this scoping review was to document the state of the art in the recent published literature on determinants of population health, with a particular focus on the types of determinants selected and the methodology used. In doing so, we also report the main characteristics of the results these studies found. The materials collected in this review are intended to inform our (and potentially other researchers’) future analyses on this topic. Since the production of health is subject to the law of diminishing marginal returns, we focused our review on those studies that included countries where a high standard of wealth has been achieved for some time, i.e., high-income countries belonging to the Organisation for Economic Co-operation and Development (OECD) or Europe. Adding similar reviews for other country income groups is of limited interest to the research we plan to do in this area.
In view of its focus on data and methods, rather than results, a formal protocol was not registered prior to undertaking this review, but the procedure followed the guidelines of the PRISMA statement for scoping reviews [ 18 ].
We focused on multi-country studies investigating the potential associations between any aggregate level (region/city/country) determinant and general measures of population health (e.g., life expectancy, mortality rate).
Within the query itself, we listed well-established population health indicators as well as the six world regions, as defined by the World Health Organization (WHO). We searched only in the publications’ titles in order to keep the number of hits manageable, and the ratio of broadly relevant abstracts over all abstracts in the order of magnitude of 10% (based on a series of time-focused trial runs). The search strategy was developed iteratively between the two authors and is presented in S1 Appendix . The search was performed by VV in PubMed and Web of Science on the 16 th of July, 2019, without any language restrictions, and with a start date set to the 1 st of January, 2013, as we were interested in the latest developments in this area of research.
Eligibility criteria
Records obtained via the search methods described above were screened independently by the two authors. Consistency between inclusion/exclusion decisions was approximately 90% and the 43 instances where uncertainty existed were judged through discussion. Articles were included subject to meeting the following requirements: (a) the paper was a full published report of an original empirical study investigating the impact of at least one aggregate level (city/region/country) factor on at least one health indicator (or self-reported health) of the general population (the only admissible “sub-populations” were those based on gender and/or age); (b) the study employed statistical techniques (calculating correlations, at the very least) and was not purely descriptive or theoretical in nature; (c) the analysis involved at least two countries or at least two regions or cities (or another aggregate level) in at least two different countries; (d) the health outcome was not differentiated according to some socio-economic factor and thus studied in terms of inequality (with the exception of gender and age differentiations); (e) mortality, in case it was one of the health indicators under investigation, was strictly “total” or “all-cause” (no cause-specific or determinant-attributable mortality).
Data extraction
The following pieces of information were extracted in an Excel table from the full text of each eligible study (primarily by VV, consulting with PB in case of doubt): health outcome(s), determinants, statistical methodology, level of analysis, results, type of data, data sources, time period, countries. The evidence is synthesized according to these extracted data (often directly reflected in the section headings), using a narrative form accompanied by a “summary-of-findings” table and a graph.
Search and selection
The initial yield contained 4583 records, reduced to 3686 after removal of duplicates ( Fig 1 ). Based on title and abstract screening, 3271 records were excluded because they focused on specific medical condition(s) or specific populations (based on morbidity or some other factor), dealt with intervention effectiveness, with theoretical or non-health related issues, or with animals or plants. Of the remaining 415 papers, roughly half were disqualified upon full-text consideration, mostly due to using an outcome not of interest to us (e.g., health inequality), measuring and analyzing determinants and outcomes exclusively at the individual level, performing analyses one country at a time, employing indices that are a mixture of both health indicators and health determinants, or not utilizing potential health determinants at all. After this second stage of the screening process, 202 papers were deemed eligible for inclusion. This group was further dichotomized according to level of economic development of the countries or regions under study, using membership of the OECD or Europe as a reference “cut-off” point. Sixty papers were judged to include high-income countries, and the remaining 142 included either low- or middle-income countries or a mix of both these levels of development. The rest of this report outlines findings in relation to high-income countries only, reflecting our own primary research interests. Nonetheless, we chose to report our search yield for the other income groups for two reasons. First, to gauge the relative interest in applied published research for these different income levels; and second, to enable other researchers with a focus on determinants of health in other countries to use the extraction we made here.
- PPT PowerPoint slide
- PNG larger image
- TIFF original image
https://doi.org/10.1371/journal.pone.0239031.g001
Health outcomes
The most frequent population health indicator, life expectancy (LE), was present in 24 of the 60 studies. Apart from “life expectancy at birth” (representing the average life-span a newborn is expected to have if current mortality rates remain constant), also called “period LE” by some [ 19 , 20 ], we encountered as well LE at 40 years of age [ 21 ], at 60 [ 22 ], and at 65 [ 21 , 23 , 24 ]. In two papers, the age-specificity of life expectancy (be it at birth or another age) was not stated [ 25 , 26 ].
Some studies considered male and female LE separately [ 21 , 24 , 25 , 27 – 33 ]. This consideration was also often observed with the second most commonly used health index [ 28 – 30 , 34 – 38 ]–termed “total”, or “overall”, or “all-cause”, mortality rate (MR)–included in 22 of the 60 studies. In addition to gender, this index was also sometimes broken down according to age group [ 30 , 39 , 40 ], as well as gender-age group [ 38 ].
While the majority of studies under review here focused on a single health indicator, 23 out of the 60 studies made use of multiple outcomes, although these outcomes were always considered one at a time, and sometimes not all of them fell within the scope of our review. An easily discernable group of indices that typically went together [ 25 , 37 , 41 ] was that of neonatal (deaths occurring within 28 days postpartum), perinatal (fetal or early neonatal / first-7-days deaths), and post-neonatal (deaths between the 29 th day and completion of one year of life) mortality. More often than not, these indices were also accompanied by “stand-alone” indicators, such as infant mortality (deaths within the first year of life; our third most common index found in 16 of the 60 studies), maternal mortality (deaths during pregnancy or within 42 days of termination of pregnancy), and child mortality rates. Child mortality has conventionally been defined as mortality within the first 5 years of life, thus often also called “under-5 mortality”. Nonetheless, Pritchard & Wallace used the term “child mortality” to denote deaths of children younger than 14 years [ 42 ].
As previously stated, inclusion criteria did allow for self-reported health status to be used as a general measure of population health. Within our final selection of studies, seven utilized some form of subjective health as an outcome variable [ 25 , 43 – 48 ]. Additionally, the Health Human Development Index [ 49 ], healthy life expectancy [ 50 ], old-age survival [ 51 ], potential years of life lost [ 52 ], and disability-adjusted life expectancy [ 25 ] were also used.
We note that while in most cases the indicators mentioned above (and/or the covariates considered, see below) were taken in their absolute or logarithmic form, as a—typically annual—number, sometimes they were used in the form of differences, change rates, averages over a given time period, or even z-scores of rankings [ 19 , 22 , 40 , 42 , 44 , 53 – 57 ].
Regions, countries, and populations
Despite our decision to confine this review to high-income countries, some variation in the countries and regions studied was still present. Selection seemed to be most often conditioned on the European Union, or the European continent more generally, and the Organisation of Economic Co-operation and Development (OECD), though, typically, not all member nations–based on the instances where these were also explicitly listed—were included in a given study. Some of the stated reasons for omitting certain nations included data unavailability [ 30 , 45 , 54 ] or inconsistency [ 20 , 58 ], Gross Domestic Product (GDP) too low [ 40 ], differences in economic development and political stability with the rest of the sampled countries [ 59 ], and national population too small [ 24 , 40 ]. On the other hand, the rationales for selecting a group of countries included having similar above-average infant mortality [ 60 ], similar healthcare systems [ 23 ], and being randomly drawn from a social spending category [ 61 ]. Some researchers were interested explicitly in a specific geographical region, such as Eastern Europe [ 50 ], Central and Eastern Europe [ 48 , 60 ], the Visegrad (V4) group [ 62 ], or the Asia/Pacific area [ 32 ]. In certain instances, national regions or cities, rather than countries, constituted the units of investigation instead [ 31 , 51 , 56 , 62 – 66 ]. In two particular cases, a mix of countries and cities was used [ 35 , 57 ]. In another two [ 28 , 29 ], due to the long time periods under study, some of the included countries no longer exist. Finally, besides “European” and “OECD”, the terms “developed”, “Western”, and “industrialized” were also used to describe the group of selected nations [ 30 , 42 , 52 , 53 , 67 ].
As stated above, it was the health status of the general population that we were interested in, and during screening we made a concerted effort to exclude research using data based on a more narrowly defined group of individuals. All studies included in this review adhere to this general rule, albeit with two caveats. First, as cities (even neighborhoods) were the unit of analysis in three of the studies that made the selection [ 56 , 64 , 65 ], the populations under investigation there can be more accurately described as general urban , instead of just general. Second, oftentimes health indicators were stratified based on gender and/or age, therefore we also admitted one study that, due to its specific research question, focused on men and women of early retirement age [ 35 ] and another that considered adult males only [ 68 ].
Data types and sources
A great diversity of sources was utilized for data collection purposes. The accessible reference databases of the OECD ( https://www.oecd.org/ ), WHO ( https://www.who.int/ ), World Bank ( https://www.worldbank.org/ ), United Nations ( https://www.un.org/en/ ), and Eurostat ( https://ec.europa.eu/eurostat ) were among the top choices. The other international databases included Human Mortality [ 30 , 39 , 50 ], Transparency International [ 40 , 48 , 50 ], Quality of Government [ 28 , 69 ], World Income Inequality [ 30 ], International Labor Organization [ 41 ], International Monetary Fund [ 70 ]. A number of national databases were referred to as well, for example the US Bureau of Statistics [ 42 , 53 ], Korean Statistical Information Services [ 67 ], Statistics Canada [ 67 ], Australian Bureau of Statistics [ 67 ], and Health New Zealand Tobacco control and Health New Zealand Food and Nutrition [ 19 ]. Well-known surveys, such as the World Values Survey [ 25 , 55 ], the European Social Survey [ 25 , 39 , 44 ], the Eurobarometer [ 46 , 56 ], the European Value Survey [ 25 ], and the European Statistics of Income and Living Condition Survey [ 43 , 47 , 70 ] were used as data sources, too. Finally, in some cases [ 25 , 28 , 29 , 35 , 36 , 41 , 69 ], built-for-purpose datasets from previous studies were re-used.
In most of the studies, the level of the data (and analysis) was national. The exceptions were six papers that dealt with Nomenclature of Territorial Units of Statistics (NUTS2) regions [ 31 , 62 , 63 , 66 ], otherwise defined areas [ 51 ] or cities [ 56 ], and seven others that were multilevel designs and utilized both country- and region-level data [ 57 ], individual- and city- or country-level [ 35 ], individual- and country-level [ 44 , 45 , 48 ], individual- and neighborhood-level [ 64 ], and city-region- (NUTS3) and country-level data [ 65 ]. Parallel to that, the data type was predominantly longitudinal, with only a few studies using purely cross-sectional data [ 25 , 33 , 43 , 45 – 48 , 50 , 62 , 67 , 68 , 71 , 72 ], albeit in four of those [ 43 , 48 , 68 , 72 ] two separate points in time were taken (thus resulting in a kind of “double cross-section”), while in another the averages across survey waves were used [ 56 ].
In studies using longitudinal data, the length of the covered time periods varied greatly. Although this was almost always less than 40 years, in one study it covered the entire 20 th century [ 29 ]. Longitudinal data, typically in the form of annual records, was sometimes transformed before usage. For example, some researchers considered data points at 5- [ 34 , 36 , 49 ] or 10-year [ 27 , 29 , 35 ] intervals instead of the traditional 1, or took averages over 3-year periods [ 42 , 53 , 73 ]. In one study concerned with the effect of the Great Recession all data were in a “recession minus expansion change in trends”-form [ 57 ]. Furthermore, there were a few instances where two different time periods were compared to each other [ 42 , 53 ] or when data was divided into 2 to 4 (possibly overlapping) periods which were then analyzed separately [ 24 , 26 , 28 , 29 , 31 , 65 ]. Lastly, owing to data availability issues, discrepancies between the time points or periods of data on the different variables were occasionally observed [ 22 , 35 , 42 , 53 – 55 , 63 ].
Health determinants
Together with other essential details, Table 1 lists the health correlates considered in the selected studies. Several general categories for these correlates can be discerned, including health care, political stability, socio-economics, demographics, psychology, environment, fertility, life-style, culture, labor. All of these, directly or implicitly, have been recognized as holding importance for population health by existing theoretical models of (social) determinants of health [ 74 – 77 ].
https://doi.org/10.1371/journal.pone.0239031.t001
It is worth noting that in a few studies there was just a single aggregate-level covariate investigated in relation to a health outcome of interest to us. In one instance, this was life satisfaction [ 44 ], in another–welfare system typology [ 45 ], but also gender inequality [ 33 ], austerity level [ 70 , 78 ], and deprivation [ 51 ]. Most often though, attention went exclusively to GDP [ 27 , 29 , 46 , 57 , 65 , 71 ]. It was often the case that research had a more particular focus. Among others, minimum wages [ 79 ], hospital payment schemes [ 23 ], cigarette prices [ 63 ], social expenditure [ 20 ], residents’ dissatisfaction [ 56 ], income inequality [ 30 , 69 ], and work leave [ 41 , 58 ] took center stage. Whenever variables outside of these specific areas were also included, they were usually identified as confounders or controls, moderators or mediators.
We visualized the combinations in which the different determinants have been studied in Fig 2 , which was obtained via multidimensional scaling and a subsequent cluster analysis (details outlined in S2 Appendix ). It depicts the spatial positioning of each determinant relative to all others, based on the number of times the effects of each pair of determinants have been studied simultaneously. When interpreting Fig 2 , one should keep in mind that determinants marked with an asterisk represent, in fact, collectives of variables.
Groups of determinants are marked by asterisks (see S1 Table in S1 Appendix ). Diminishing color intensity reflects a decrease in the total number of “connections” for a given determinant. Noteworthy pairwise “connections” are emphasized via lines (solid-dashed-dotted indicates decreasing frequency). Grey contour lines encircle groups of variables that were identified via cluster analysis. Abbreviations: age = population age distribution, associations = membership in associations, AT-index = atherogenic-thrombogenic index, BR = birth rate, CAPB = Cyclically Adjusted Primary Balance, civilian-labor = civilian labor force, C-section = Cesarean delivery rate, credit-info = depth of credit information, dissatisf = residents’ dissatisfaction, distrib.orient = distributional orientation, EDU = education, eHealth = eHealth index at GP-level, exch.rate = exchange rate, fat = fat consumption, GDP = gross domestic product, GFCF = Gross Fixed Capital Formation/Creation, GH-gas = greenhouse gas, GII = gender inequality index, gov = governance index, gov.revenue = government revenues, HC-coverage = healthcare coverage, HE = health(care) expenditure, HHconsump = household consumption, hosp.beds = hospital beds, hosp.payment = hospital payment scheme, hosp.stay = length of hospital stay, IDI = ICT development index, inc.ineq = income inequality, industry-labor = industrial labor force, infant-sex = infant sex ratio, labor-product = labor production, LBW = low birth weight, leave = work leave, life-satisf = life satisfaction, M-age = maternal age, marginal-tax = marginal tax rate, MDs = physicians, mult.preg = multiple pregnancy, NHS = Nation Health System, NO = nitrous oxide emissions, PM10 = particulate matter (PM10) emissions, pop = population size, pop.density = population density, pre-term = pre-term birth rate, prison = prison population, researchE = research&development expenditure, school.ref = compulsory schooling reform, smoke-free = smoke-free places, SO = sulfur oxide emissions, soc.E = social expenditure, soc.workers = social workers, sugar = sugar consumption, terror = terrorism, union = union density, UR = unemployment rate, urban = urbanization, veg-fr = vegetable-and-fruit consumption, welfare = welfare regime, Wwater = wastewater treatment.
https://doi.org/10.1371/journal.pone.0239031.g002
Distances between determinants in Fig 2 are indicative of determinants’ “connectedness” with each other. While the statistical procedure called for higher dimensionality of the model, for demonstration purposes we show here a two-dimensional solution. This simplification unfortunately comes with a caveat. To use the factor smoking as an example, it would appear it stands at a much greater distance from GDP than it does from alcohol. In reality however, smoking was considered together with alcohol consumption [ 21 , 25 , 26 , 52 , 68 ] in just as many studies as it was with GDP [ 21 , 25 , 26 , 52 , 59 ], five. To aid with respect to this apparent shortcoming, we have emphasized the strongest pairwise links. Solid lines connect GDP with health expenditure (HE), unemployment rate (UR), and education (EDU), indicating that the effect of GDP on health, taking into account the effects of the other three determinants as well, was evaluated in between 12 to 16 studies of the 60 included in this review. Tracing the dashed lines, we can also tell that GDP appeared jointly with income inequality, and HE together with either EDU or UR, in anywhere between 8 to 10 of our selected studies. Finally, some weaker but still worth-mentioning “connections” between variables are displayed as well via the dotted lines.
The fact that all notable pairwise “connections” are concentrated within a relatively small region of the plot may be interpreted as low overall “connectedness” among the health indicators studied. GDP is the most widely investigated determinant in relation to general population health. Its total number of “connections” is disproportionately high (159) compared to its runner-up–HE (with 113 “connections”), and then subsequently EDU (with 90) and UR (with 86). In fact, all of these determinants could be thought of as outliers, given that none of the remaining factors have a total count of pairings above 52. This decrease in individual determinants’ overall “connectedness” can be tracked on the graph via the change of color intensity as we move outwards from the symbolic center of GDP and its closest “co-determinants”, to finally reach the other extreme of the ten indicators (welfare regime, household consumption, compulsory school reform, life satisfaction, government revenues, literacy, research expenditure, multiple pregnancy, Cyclically Adjusted Primary Balance, and residents’ dissatisfaction; in white) the effects on health of which were only studied in isolation.
Lastly, we point to the few small but stable clusters of covariates encircled by the grey bubbles on Fig 2 . These groups of determinants were identified as “close” by both statistical procedures used for the production of the graph (see details in S2 Appendix ).
Statistical methodology
There was great variation in the level of statistical detail reported. Some authors provided too vague a description of their analytical approach, necessitating some inference in this section.
The issue of missing data is a challenging reality in this field of research, but few of the studies under review (12/60) explain how they dealt with it. Among the ones that do, three general approaches to handling missingness can be identified, listed in increasing level of sophistication: case-wise deletion, i.e., removal of countries from the sample [ 20 , 45 , 48 , 58 , 59 ], (linear) interpolation [ 28 , 30 , 34 , 58 , 59 , 63 ], and multiple imputation [ 26 , 41 , 52 ].
Correlations, Pearson, Spearman, or unspecified, were the only technique applied with respect to the health outcomes of interest in eight analyses [ 33 , 42 – 44 , 46 , 53 , 57 , 61 ]. Among the more advanced statistical methods, the family of regression models proved to be, by and large, predominant. Before examining this closer, we note the techniques that were, in a way, “unique” within this selection of studies: meta-analyses were performed (random and fixed effects, respectively) on the reduced form and 2-sample two stage least squares (2SLS) estimations done within countries [ 39 ]; difference-in-difference (DiD) analysis was applied in one case [ 23 ]; dynamic time-series methods, among which co-integration, impulse-response function (IRF), and panel vector autoregressive (VAR) modeling, were utilized in one study [ 80 ]; longitudinal generalized estimating equation (GEE) models were developed on two occasions [ 70 , 78 ]; hierarchical Bayesian spatial models [ 51 ] and special autoregressive regression [ 62 ] were also implemented.
Purely cross-sectional data analyses were performed in eight studies [ 25 , 45 , 47 , 50 , 55 , 56 , 67 , 71 ]. These consisted of linear regression (assumed ordinary least squares (OLS)), generalized least squares (GLS) regression, and multilevel analyses. However, six other studies that used longitudinal data in fact had a cross-sectional design, through which they applied regression at multiple time-points separately [ 27 , 29 , 36 , 48 , 68 , 72 ].
Apart from these “multi-point cross-sectional studies”, some other simplistic approaches to longitudinal data analysis were found, involving calculating and regressing 3-year averages of both the response and the predictor variables [ 54 ], taking the average of a few data-points (i.e., survey waves) [ 56 ] or using difference scores over 10-year [ 19 , 29 ] or unspecified time intervals [ 40 , 55 ].
Moving further in the direction of more sensible longitudinal data usage, we turn to the methods widely known among (health) economists as “panel data analysis” or “panel regression”. Most often seen were models with fixed effects for country/region and sometimes also time-point (occasionally including a country-specific trend as well), with robust standard errors for the parameter estimates to take into account correlations among clustered observations [ 20 , 21 , 24 , 28 , 30 , 32 , 34 , 37 , 38 , 41 , 52 , 59 , 60 , 63 , 66 , 69 , 73 , 79 , 81 , 82 ]. The Hausman test [ 83 ] was sometimes mentioned as the tool used to decide between fixed and random effects [ 26 , 49 , 63 , 66 , 73 , 82 ]. A few studies considered the latter more appropriate for their particular analyses, with some further specifying that (feasible) GLS estimation was employed [ 26 , 34 , 49 , 58 , 60 , 73 ]. Apart from these two types of models, the first differences method was encountered once as well [ 31 ]. Across all, the error terms were sometimes assumed to come from a first-order autoregressive process (AR(1)), i.e., they were allowed to be serially correlated [ 20 , 30 , 38 , 58 – 60 , 73 ], and lags of (typically) predictor variables were included in the model specification, too [ 20 , 21 , 37 , 38 , 48 , 69 , 81 ]. Lastly, a somewhat different approach to longitudinal data analysis was undertaken in four studies [ 22 , 35 , 48 , 65 ] in which multilevel–linear or Poisson–models were developed.
Regardless of the exact techniques used, most studies included in this review presented multiple model applications within their main analysis. None attempted to formally compare models in order to identify the “best”, even if goodness-of-fit statistics were occasionally reported. As indicated above, many studies investigated women’s and men’s health separately [ 19 , 21 , 22 , 27 – 29 , 31 , 33 , 35 , 36 , 38 , 39 , 45 , 50 , 51 , 64 , 65 , 69 , 82 ], and covariates were often tested one at a time, including other covariates only incrementally [ 20 , 25 , 28 , 36 , 40 , 50 , 55 , 67 , 73 ]. Furthermore, there were a few instances where analyses within countries were performed as well [ 32 , 39 , 51 ] or where the full time period of interest was divided into a few sub-periods [ 24 , 26 , 28 , 31 ]. There were also cases where different statistical techniques were applied in parallel [ 29 , 55 , 60 , 66 , 69 , 73 , 82 ], sometimes as a form of sensitivity analysis [ 24 , 26 , 30 , 58 , 73 ]. However, the most common approach to sensitivity analysis was to re-run models with somewhat different samples [ 39 , 50 , 59 , 67 , 69 , 80 , 82 ]. Other strategies included different categorization of variables or adding (more/other) controls [ 21 , 23 , 25 , 28 , 37 , 50 , 63 , 69 ], using an alternative main covariate measure [ 59 , 82 ], including lags for predictors or outcomes [ 28 , 30 , 58 , 63 , 65 , 79 ], using weights [ 24 , 67 ] or alternative data sources [ 37 , 69 ], or using non-imputed data [ 41 ].
As the methods and not the findings are the main focus of the current review, and because generic checklists cannot discern the underlying quality in this application field (see also below), we opted to pool all reported findings together, regardless of individual study characteristics or particular outcome(s) used, and speak generally of positive and negative effects on health. For this summary we have adopted the 0.05-significance level and only considered results from multivariate analyses. Strictly birth-related factors are omitted since these potentially only relate to the group of infant mortality indicators and not to any of the other general population health measures.
Starting with the determinants most often studied, higher GDP levels [ 21 , 26 , 27 , 29 , 30 , 32 , 43 , 48 , 52 , 58 , 60 , 66 , 67 , 73 , 79 , 81 , 82 ], higher health [ 21 , 37 , 47 , 49 , 52 , 58 , 59 , 68 , 72 , 82 ] and social [ 20 , 21 , 26 , 38 , 79 ] expenditures, higher education [ 26 , 39 , 52 , 62 , 72 , 73 ], lower unemployment [ 60 , 61 , 66 ], and lower income inequality [ 30 , 42 , 53 , 55 , 73 ] were found to be significantly associated with better population health on a number of occasions. In addition to that, there was also some evidence that democracy [ 36 ] and freedom [ 50 ], higher work compensation [ 43 , 79 ], distributional orientation [ 54 ], cigarette prices [ 63 ], gross national income [ 22 , 72 ], labor productivity [ 26 ], exchange rates [ 32 ], marginal tax rates [ 79 ], vaccination rates [ 52 ], total fertility [ 59 , 66 ], fruit and vegetable [ 68 ], fat [ 52 ] and sugar consumption [ 52 ], as well as bigger depth of credit information [ 22 ] and percentage of civilian labor force [ 79 ], longer work leaves [ 41 , 58 ], more physicians [ 37 , 52 , 72 ], nurses [ 72 ], and hospital beds [ 79 , 82 ], and also membership in associations, perceived corruption and societal trust [ 48 ] were beneficial to health. Higher nitrous oxide (NO) levels [ 52 ], longer average hospital stay [ 48 ], deprivation [ 51 ], dissatisfaction with healthcare and the social environment [ 56 ], corruption [ 40 , 50 ], smoking [ 19 , 26 , 52 , 68 ], alcohol consumption [ 26 , 52 , 68 ] and illegal drug use [ 68 ], poverty [ 64 ], higher percentage of industrial workers [ 26 ], Gross Fixed Capital creation [ 66 ] and older population [ 38 , 66 , 79 ], gender inequality [ 22 ], and fertility [ 26 , 66 ] were detrimental.
It is important to point out that the above-mentioned effects could not be considered stable either across or within studies. Very often, statistical significance of a given covariate fluctuated between the different model specifications tried out within the same study [ 20 , 49 , 59 , 66 , 68 , 69 , 73 , 80 , 82 ], testifying to the importance of control variables and multivariate research (i.e., analyzing multiple independent variables simultaneously) in general. Furthermore, conflicting results were observed even with regards to the “core” determinants given special attention, so to speak, throughout this text. Thus, some studies reported negative effects of health expenditure [ 32 , 82 ], social expenditure [ 58 ], GDP [ 49 , 66 ], and education [ 82 ], and positive effects of income inequality [ 82 ] and unemployment [ 24 , 31 , 32 , 52 , 66 , 68 ]. Interestingly, one study [ 34 ] differentiated between temporary and long-term effects of GDP and unemployment, alluding to possibly much greater complexity of the association with health. It is also worth noting that some gender differences were found, with determinants being more influential for males than for females, or only having statistically significant effects for male health [ 19 , 21 , 28 , 34 , 36 , 37 , 39 , 64 , 65 , 69 ].
The purpose of this scoping review was to examine recent quantitative work on the topic of multi-country analyses of determinants of population health in high-income countries.
Measuring population health via relatively simple mortality-based indicators still seems to be the state of the art. What is more, these indicators are routinely considered one at a time, instead of, for example, employing existing statistical procedures to devise a more general, composite, index of population health, or using some of the established indices, such as disability-adjusted life expectancy (DALE) or quality-adjusted life expectancy (QALE). Although strong arguments for their wider use were already voiced decades ago [ 84 ], such summary measures surface only rarely in this research field.
On a related note, the greater data availability and accessibility that we enjoy today does not automatically equate to data quality. Nonetheless, this is routinely assumed in aggregate level studies. We almost never encountered a discussion on the topic. The non-mundane issue of data missingness, too, goes largely underappreciated. With all recent methodological advancements in this area [ 85 – 88 ], there is no excuse for ignorance; and still, too few of the reviewed studies tackled the matter in any adequate fashion.
Much optimism can be gained considering the abundance of different determinants that have attracted researchers’ attention in relation to population health. We took on a visual approach with regards to these determinants and presented a graph that links spatial distances between determinants with frequencies of being studies together. To facilitate interpretation, we grouped some variables, which resulted in some loss of finer detail. Nevertheless, the graph is helpful in exemplifying how many effects continue to be studied in a very limited context, if any. Since in reality no factor acts in isolation, this oversimplification practice threatens to render the whole exercise meaningless from the outset. The importance of multivariate analysis cannot be stressed enough. While there is no “best method” to be recommended and appropriate techniques vary according to the specifics of the research question and the characteristics of the data at hand [ 89 – 93 ], in the future, in addition to abandoning simplistic univariate approaches, we hope to see a shift from the currently dominating fixed effects to the more flexible random/mixed effects models [ 94 ], as well as wider application of more sophisticated methods, such as principle component regression, partial least squares, covariance structure models (e.g., structural equations), canonical correlations, time-series, and generalized estimating equations.
Finally, there are some limitations of the current scoping review. We searched the two main databases for published research in medical and non-medical sciences (PubMed and Web of Science) since 2013, thus potentially excluding publications and reports that are not indexed in these databases, as well as older indexed publications. These choices were guided by our interest in the most recent (i.e., the current state-of-the-art) and arguably the highest-quality research (i.e., peer-reviewed articles, primarily in indexed non-predatory journals). Furthermore, despite holding a critical stance with regards to some aspects of how determinants-of-health research is currently conducted, we opted out of formally assessing the quality of the individual studies included. The reason for that is two-fold. On the one hand, we are unaware of the existence of a formal and standard tool for quality assessment of ecological designs. And on the other, we consider trying to score the quality of these diverse studies (in terms of regional setting, specific topic, outcome indices, and methodology) undesirable and misleading, particularly since we would sometimes have been rating the quality of only a (small) part of the original studies—the part that was relevant to our review’s goal.
Our aim was to investigate the current state of research on the very broad and general topic of population health, specifically, the way it has been examined in a multi-country context. We learned that data treatment and analytical approach were, in the majority of these recent studies, ill-equipped or insufficiently transparent to provide clarity regarding the underlying mechanisms of population health in high-income countries. Whether due to methodological shortcomings or the inherent complexity of the topic, research so far fails to provide any definitive answers. It is our sincere belief that with the application of more advanced analytical techniques this continuous quest could come to fruition sooner.
Supporting information
S1 checklist. preferred reporting items for systematic reviews and meta-analyses extension for scoping reviews (prisma-scr) checklist..
https://doi.org/10.1371/journal.pone.0239031.s001
S1 Appendix.
https://doi.org/10.1371/journal.pone.0239031.s002
S2 Appendix.
https://doi.org/10.1371/journal.pone.0239031.s003
- View Article
- Google Scholar
- PubMed/NCBI
- 75. Dahlgren G, Whitehead M. Policies and Strategies to Promote Equity in Health. Stockholm, Sweden: Institute for Future Studies; 1991.
- 76. Brunner E, Marmot M. Social Organization, Stress, and Health. In: Marmot M, Wilkinson RG, editors. Social Determinants of Health. Oxford, England: Oxford University Press; 1999.
- 77. Najman JM. A General Model of the Social Origins of Health and Well-being. In: Eckersley R, Dixon J, Douglas B, editors. The Social Origins of Health and Well-being. Cambridge, England: Cambridge University Press; 2001.
- 85. Carpenter JR, Kenward MG. Multiple Imputation and its Application. New York: John Wiley & Sons; 2013.
- 86. Molenberghs G, Fitzmaurice G, Kenward MG, Verbeke G, Tsiatis AA. Handbook of Missing Data Methodology. Boca Raton: Chapman & Hall/CRC; 2014.
- 87. van Buuren S. Flexible Imputation of Missing Data. 2nd ed. Boca Raton: Chapman & Hall/CRC; 2018.
- 88. Enders CK. Applied Missing Data Analysis. New York: Guilford; 2010.
- 89. Shayle R. Searle GC, Charles E. McCulloch. Variance Components: John Wiley & Sons, Inc.; 1992.
- 90. Agresti A. Foundations of Linear and Generalized Linear Models. Hoboken, New Jersey: John Wiley & Sons Inc.; 2015.
- 91. Leyland A. H. (Editor) HGE. Multilevel Modelling of Health Statistics: John Wiley & Sons Inc; 2001.
- 92. Garrett Fitzmaurice MD, Geert Verbeke, Geert Molenberghs. Longitudinal Data Analysis. New York: Chapman and Hall/CRC; 2008.
- 93. Wolfgang Karl Härdle LS. Applied Multivariate Statistical Analysis. Berlin, Heidelberg: Springer; 2015.
- Research article
- Open access
- Published: 03 February 2021
A review of the quantitative effectiveness evidence synthesis methods used in public health intervention guidelines
- Ellesha A. Smith ORCID: orcid.org/0000-0002-4241-7205 1 ,
- Nicola J. Cooper 1 ,
- Alex J. Sutton 1 ,
- Keith R. Abrams 1 &
- Stephanie J. Hubbard 1
BMC Public Health volume 21 , Article number: 278 ( 2021 ) Cite this article
3614 Accesses
5 Citations
3 Altmetric
Metrics details
The complexity of public health interventions create challenges in evaluating their effectiveness. There have been huge advancements in quantitative evidence synthesis methods development (including meta-analysis) for dealing with heterogeneity of intervention effects, inappropriate ‘lumping’ of interventions, adjusting for different populations and outcomes and the inclusion of various study types. Growing awareness of the importance of using all available evidence has led to the publication of guidance documents for implementing methods to improve decision making by answering policy relevant questions.
The first part of this paper reviews the methods used to synthesise quantitative effectiveness evidence in public health guidelines by the National Institute for Health and Care Excellence (NICE) that had been published or updated since the previous review in 2012 until the 19th August 2019.The second part of this paper provides an update of the statistical methods and explains how they address issues related to evaluating effectiveness evidence of public health interventions.
The proportion of NICE public health guidelines that used a meta-analysis as part of the synthesis of effectiveness evidence has increased since the previous review in 2012 from 23% (9 out of 39) to 31% (14 out of 45). The proportion of NICE guidelines that synthesised the evidence using only a narrative review decreased from 74% (29 out of 39) to 60% (27 out of 45).An application in the prevention of accidents in children at home illustrated how the choice of synthesis methods can enable more informed decision making by defining and estimating the effectiveness of more distinct interventions, including combinations of intervention components, and identifying subgroups in which interventions are most effective.
Conclusions
Despite methodology development and the publication of guidance documents to address issues in public health intervention evaluation since the original review, NICE public health guidelines are not making full use of meta-analysis and other tools that would provide decision makers with fuller information with which to develop policy. There is an evident need to facilitate the translation of the synthesis methods into a public health context and encourage the use of methods to improve decision making.
Peer Review reports
To make well-informed decisions and provide the best guidance in health care policy, it is essential to have a clear framework for synthesising good quality evidence on the effectiveness and cost-effectiveness of health interventions. There is a broad range of methods available for evidence synthesis. Narrative reviews provide a qualitative summary of the effectiveness of the interventions. Meta-analysis is a statistical method that pools evidence from multiple independent sources [ 1 ]. Meta-analysis and more complex variations of meta-analysis have been extensively applied in the appraisals of clinical interventions and treatments, such as drugs, as the interventions and populations are clearly defined and tested in randomised, controlled conditions. In comparison, public health studies are often more complex in design, making synthesis more challenging [ 2 ].
Many challenges are faced in the synthesis of public health interventions. There is often increased methodological heterogeneity due to the inclusion of different study designs. Interventions are often poorly described in the literature which may result in variation within the intervention groups. There can be a wide range of outcomes, whose definitions are not consistent across studies. Intermediate, or surrogate, outcomes are often used in studies evaluating public health interventions [ 3 ]. In addition to these challenges, public health interventions are often also complex meaning that they are made up of multiple, interacting components [ 4 ]. Recent guidance documents have focused on the synthesis of complex interventions [ 2 , 5 , 6 ]. The National Institute for Health and Care Excellence (NICE) guidance manual provides recommendations across all topics that are covered by NICE and there is currently no guidance that focuses specifically on the public health context.
Research questions
A methodological review of NICE public health intervention guidelines by Achana et al. (2014) found that meta-analysis methods were not being used [ 3 ]. The first part of this paper aims to update and compare, to the original review, the meta-analysis methods being used in evidence synthesis of public health intervention appraisals.
The second part of this paper aims to illustrate what methods are available to address the challenges of public health intervention evidence synthesis. Synthesis methods that go beyond a pairwise meta-analysis are illustrated through the application to a case study in public health and are discussed to understand how evidence synthesis methods can enable more informed decision making.
The third part of this paper presents software, guidance documents and web tools for methods that aim to make appropriate evidence synthesis of public health interventions more accessible. Recommendations for future research and guidance production that can improve the uptake of these methods in a public health context are discussed.
Update of NICE public health intervention guidelines review
Nice guidelines.
The National Institute for Health and Care Excellence (NICE) was established in 1999 as a health authority to provide guidance on new medical technologies to the NHS in England and Wales [ 7 ]. Using an evidence-based approach, it provides recommendations based on effectiveness and cost-effectiveness to ensure an open and transparent process of allocating NHS resources [ 8 ]. The remit for NICE guideline production was extended to public health in April 2005 and the first recommendations were published in March 2006. NICE published ‘Developing NICE guidelines: the manual’ in 2006, which has been updated since, with the most recent in 2018 [ 9 ]. It was intended to be a guidance document to aid in the production of NICE guidelines across all NICE topics. In terms of synthesising quantitative evidence, the NICE recommendations state: ‘meta-analysis may be appropriate if treatment estimates of the same outcome from more than 1 study are available’ and ‘when multiple competing options are being appraised, a network meta-analysis should be considered’. The implementation of network meta-analysis (NMA), which is described later, as a recommendation from NICE was introduced into the guidance document in 2014, with a further update in 2018.
Background to the previous review
The paper by Achana et al. (2014) explored the use of evidence synthesis methodology in NICE public health intervention guidelines published between 2006 and 2012 [ 3 ]. The authors conducted a systematic review of the methods used to synthesise quantitative effectiveness evidence within NICE public health guidelines. They found that only 23% of NICE public health guidelines used pairwise meta-analysis as part of the effectiveness review and the remainder used a narrative summary or no synthesis of evidence at all. The authors argued that despite significant advances in the methodology of evidence synthesis, the uptake of methods in public health intervention evaluation is lower than other fields, including clinical treatment evaluation. The paper concluded that more sophisticated methods in evidence synthesis should be considered to aid in decision making in the public health context [ 3 ].
The search strategy used in this paper was equivalent to that in the previous paper by Achana et al. (2014)[ 3 ]. The search was conducted through the NICE website ( https://www.nice.org.uk/guidance ) by searching the ‘Guidance and Advice List’ and filtering by ‘Public Health Guidelines’ [ 10 ]. The search criteria included all guidance documents that had been published from inception (March 2006) until the 19th August 2019. Since the original review, many of the guidelines had been updated with new documents or merged. Guidelines that remained unchanged since the previous review in 2012 were excluded and used for comparison.
The guidelines contained multiple documents that were assessed for relevance. A systematic review is a separate synthesis within a guideline that systematically collates all evidence on a specific research question of interest in the literature. Systematic reviews of quantitative effectiveness, cost-effectiveness evidence and decision modelling reports were all included as relevant. Qualitative reviews, field reports, expert opinions, surveillance reports, review decisions and other supporting documents were excluded at the search stage.
Within the reports, data was extracted on the types of review (narrative summary, pairwise meta-analysis, network meta-analysis (NMA), cost-effectiveness review or decision model), design of included primary studies (randomised controlled trials or non-randomised studies, intermediate or final outcomes, description of outcomes, outcome measure statistic), details of the synthesis methods used in the effectiveness evaluation (type of synthesis, fixed or random effects model, study quality assessment, publication bias assessment, presentation of results, software). Further details of the interventions were also recorded, including whether multiple interventions were lumped together for a pairwise comparison, whether interventions were complex (made up of multiple components) and details of the components. The reports were also assessed for potential use of complex intervention evidence synthesis methodology, meaning that the interventions that were evaluated in the review were made up of components that could potentially be synthesised using an NMA or a component NMA [ 11 ]. Where meta-analysis was not used to synthesis effectiveness evidence, the reasons for this was also recorded.
Search results and types of reviews
There were 67 NICE public health guidelines available on the NICE website. A summary flow diagram describing the literature identification process and the list of guidelines and their reference codes are provided in Additional files 1 and 2 . Since the previous review, 22 guidelines had not been updated. The results from the previous review were used for comparison to the 45 guidelines that were either newly published or updated.
The guidelines consisted of 508 documents that were assessed for relevance. Table 1 shows which types of relevant documents were available in each of the 45 guidelines. The median number of relevant articles per guideline was 3 (minimum = 0, maximum = 10). Two (4%) of the NICE public health guidelines did not report any type of systematic review, cost-effectiveness review or decision model (NG68, NG64) that met the inclusion criteria. 167 documents from 43 NICE public health guidelines were systematic reviews of quantitative effectiveness, cost-effectiveness or decision model reports and met the inclusion criteria.
Narrative reviews of effectiveness were implemented in 41 (91%) of the NICE PH guidelines. 14 (31%) contained a review that used meta-analysis to synthesise the evidence. Only one (1%) NICE guideline contained a review that implemented NMA to synthesise the effectiveness of multiple interventions; this was the same guideline that used NMA in the original review and had been updated. 33 (73%) guidelines contained cost-effectiveness reviews and 34 (76%) developed a decision model.
Comparison of review types to original review
Table 2 compares the results of the update to the original review and shows that the types of reviews and evidence synthesis methodologies remain largely unchanged since 2012. The proportion of guidelines that only contain narrative reviews to synthesise effectiveness or cost-effectiveness evidence has reduced from 74% to 60% and the proportion that included a meta-analysis has increased from 23% to 31%. The proportion of guidelines with reviews that only included evidence from randomised controlled trials and assessed the quality of individual studies remained similar to the original review.
Characteristics of guidelines using meta-analytic methods
Table 3 details the characteristics of the meta-analytic methods implemented in 24 reviews of the 14 guidelines that included one. All of the reviews reported an assessment of study quality, 12 (50%) reviews included only data from randomised controlled trials, 4 (17%) reviews used intermediate outcomes (e.g. uptake of chlamydia screening rather than prevention of chlamydia (PH3)), compared to the 20 (83%) reviews that used final outcomes (e.g. smoking cessation rather than uptake of a smoking cessation programme (NG92)). 2 (8%) reviews only used a fixed effect meta-analysis, 19 (79%) reviews used a random effects meta-analysis and 3 (13%) did not report which they had used.
An evaluation of the intervention information reported in the reviews concluded that 12 (50%) reviews had lumped multiple (more than two) different interventions into a control versus intervention pairwise meta-analysis. Eleven (46%) of the reviews evaluated interventions that are made up of multiple components (e.g. interventions for preventing obesity in PH47 were made up of diet, physical activity and behavioural change components).
21 (88%) of the reviews presented the results of the meta-analysis in the form of a forest plot and 22 (92%) presented the results in the text of the report. 20 (83%) of the reviews used two or more forms of presentation for the results. Only three (13%) reviews assessed publication bias. The most common software to perform meta-analysis was RevMan in 14 (58%) of the reviews.
Reasons for not using meta-analytic methods
The 143 reviews of effectiveness and cost effectiveness that did not use meta-analysis methods to synthesise the quantitative effectiveness evidence were searched for reasons behind this decision. 70 reports (49%) did not give a reason for not synthesising the data using a meta-analysis and 164 reasons were reported which are displayed in Fig. 1 . Out of the remaining reviews, multiple reasons for not using a meta-analysis were given. 53 (37%) of the reviews reported at least one reason due to heterogeneity. 30 (21%) decision model reports did not give a reason and these are categorised separately. 5 (3%) reviews reported that meta-analysis was not applicable or feasible, 1 (1%) reported that they were following NICE guidelines and 5 (3%) reported that there were a lack of studies.
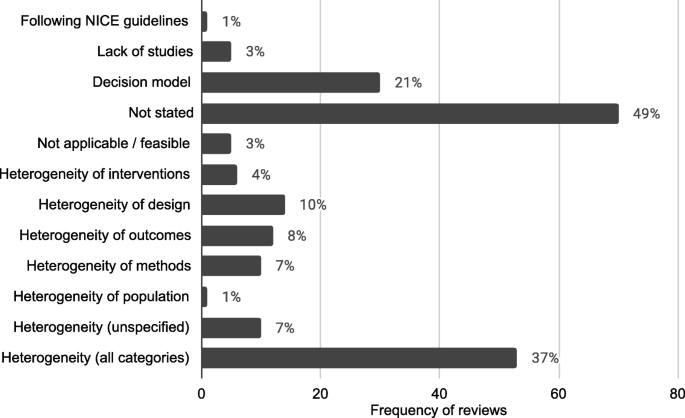
Frequency and proportions of reasons reported for not using statistical methods in quantitative evidence synthesis in NICE PH intervention reviews
The frequency of reviews and guidelines that used meta-analytic methods were plotted against year of publication, which is reported in Fig. 2 . This showed that the number of reviews that used meta-analysis were approximately constant but there is some suggestion that the number of meta-analyses used per guideline increased, particularly in 2018.
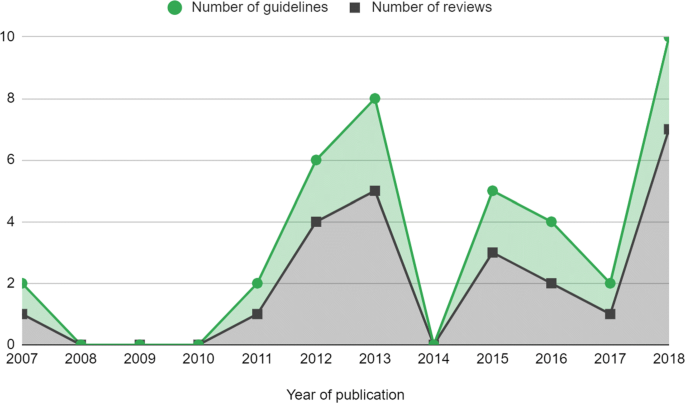
Number of meta-analyses in NICE PH guidelines by year. Guidelines that were published before 2012 had been updated since the previous review by Achana et al. (2014) [ 3 ]
Comparison of meta-analysis characteristics to original review
Table 4 compares the characteristics of the meta-analyses used in the evidence synthesis of NICE public health intervention guidelines to the original review by Achana et al. (2014) [ 3 ]. Overall, the characteristics in the updated review have not much changed from those in the original. These changes demonstrate that the use of meta-analysis in NICE guidelines has increased but remains low. Lumping of interventions still appears to be common in 50% of reviews. The implications of this are discussed in the next section.
Application of evidence synthesis methodology in a public health intervention: motivating example
Since the original review, evidence synthesis methods have been developed and can address some of the challenges of synthesising quantitative effectiveness evidence of public health interventions. Despite this, the previous section shows that the uptake of these methods is still low in NICE public health guidelines - usually limited to a pairwise meta-analysis.
It has been shown in the results above and elsewhere [ 12 ] that heterogeneity is a common reason for not synthesising the quantitative effectiveness evidence available from systematic reviews in public health. Statistical heterogeneity is the variation in the intervention effects between the individual studies. Heterogeneity is problematic in evidence synthesis as it leads to uncertainty in the pooled effect estimates in a meta-analysis which can make it difficult to interpret the pooled results and draw conclusions. Rather than exploring the source of the heterogeneity, often in public health intervention appraisals a random effects model is fitted which assumes that the study intervention effects are not equivalent but come from a common distribution [ 13 , 14 ]. Alternatively, as demonstrated in the review update, heterogeneity is used as a reason to not undertake any quantitative evidence synthesis at all.
Since the size of the intervention effects and the methodological variation in the studies will affect the impact of the heterogeneity on a meta-analysis, it is inappropriate to base the methodological approach of a review on the degree of heterogeneity, especially within public health intervention appraisal where heterogeneity seems inevitable. Ioannidis et al. (2008) argued that there are ‘almost always’ quantitative synthesis options that may offer some useful insights in the presence of heterogeneity, as long as the reviewers interpret the findings with respect to their limitations [ 12 ].
In this section current evidence synthesis methods are applied to a motivating example in public health. This aims to demonstrate that methods beyond pairwise meta-analysis can provide appropriate and pragmatic information to public health decision makers to enable more informed decision making.
Figure 3 summarises the narrative of this part of the paper and illustrates the methods that are discussed. The red boxes represent the challenges in synthesising quantitative effectiveness evidence and refers to the section within the paper for more detail. The blue boxes represent the methods that can be applied to investigate each challenge.
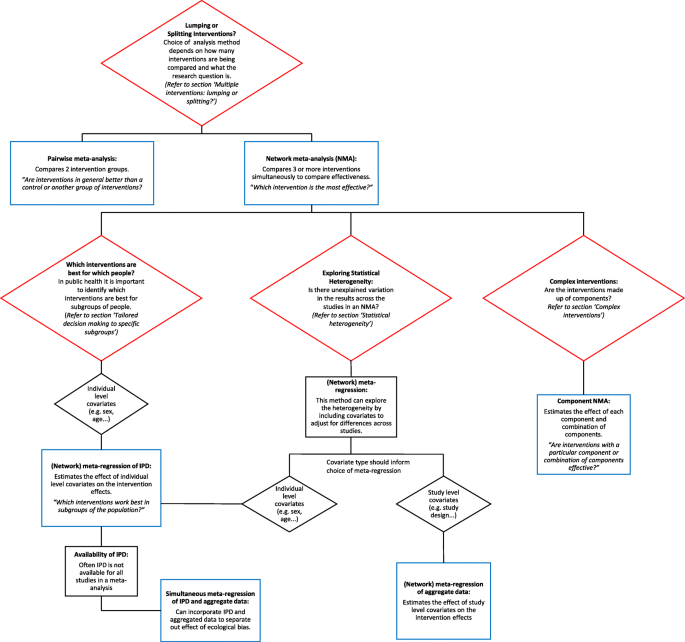
Summary of challenges that are faces in the evidence synthesis of public health interventions and methods that are discussed to overcome these challenges
Evaluating the effect of interventions for promoting the safe storage of cleaning products to prevent childhood poisoning accidents
To illustrate the methodological developments, a motivating example is used from the five year, NIHR funded, Keeping Children Safe Programme [ 15 ]. The project included a Cochrane systematic review that aimed to increase the use of safety equipment to prevent accidents at home in children under five years old. This application is intended to be illustrative of the benefits of new evidence synthesis methods since the previous review. It is not a complete, comprehensive analysis as it only uses a subset of the original dataset and therefore the results are not intended to be used for policy decision making. This example has been chosen as it demonstrates many of the issues in synthesising effectiveness evidence of public health interventions, including different study designs (randomised controlled trials, observational studies and cluster randomised trials), heterogeneity of populations or settings, incomplete individual participant data and complex interventions that contain multiple components.
This analysis will investigate the most effective promotional interventions for the outcome of ‘safe storage of cleaning products’ to prevent childhood poisoning accidents. There are 12 studies included in the dataset, with IPD available from nine of the studies. The covariate, single parent family, is included in the analysis to demonstrate the effect of being a single parent family on the outcome. In this example, all of the interventions are made up of one or more of the following components: education (Ed), free or low cost equipment (Eq), home safety inspection (HSI), and installation of safety equipment (In). A Bayesian approach using WinBUGS was used and therefore credible intervals (CrI) are presented with estimates of the effect sizes [ 16 ].
The original review paper by Achana et al. (2014) demonstrated pairwise meta-analysis and meta-regression using individual and cluster allocated trials, subgroup analyses, meta-regression using individual participant data (IPD) and summary aggregate data and NMA. This paper firstly applies NMA to the motivating example for context, followed by extensions to NMA.
Multiple interventions: lumping or splitting?
Often in public health there are multiple intervention options. However, interventions are often lumped together in a pairwise meta-analysis. Pairwise meta-analysis is a useful tool for two interventions or, alternatively in the presence of lumping interventions, for answering the research question: ‘are interventions in general better than a control or another group of interventions?’. However, when there are multiple interventions, this type of analysis is not appropriate for informing health care providers which intervention should be recommended to the public. ‘Lumping’ is becoming less frequent in other areas of evidence synthesis, such as for clinical interventions, as the use of sophisticated synthesis techniques, such as NMA, increases (Achana et al. 2014) but lumping is still common in public health.
NMA is an extension of the pairwise meta-analysis framework to more than two interventions. Multiple interventions that are lumped into a pairwise meta-analysis are likely to demonstrate high statistical heterogeneity. This does not mean that quantitative synthesis could not be undertaken but that a more appropriate method, NMA, should be implemented. Instead the statistical approach should be based on the research questions of the systematic review. For example, if the research question is ‘are any interventions effective for preventing obesity?’, it would be appropriate to perform a pairwise meta-analysis comparing every intervention in the literature to a control. However, if the research question is ‘which intervention is the most effective for preventing obesity?’, it would be more appropriate and informative to perform a network meta-analysis, which can compare multiple interventions simultaneously and identify the best one.
NMA is a useful statistical method in the context of public health intervention appraisal, where there are often multiple intervention options, as it estimates the relative effectiveness of three or more interventions simultaneously, even if direct study evidence is not available for all intervention comparisons. Using NMA can help to answer the research question ‘what is the effectiveness of each intervention compared to all other interventions in the network?’.
In the motivating example there are six intervention options. The effect of lumping interventions is shown in Fig. 4 , where different interventions in both the intervention and control arms are compared. There is overlap of intervention and control arms across studies and interpretation of the results of a pairwise meta-analysis comparing the effectiveness of the two groups of interventions would not be useful in deciding which intervention to recommend. In comparison, the network plot in Fig. 5 illustrates the evidence base of the prevention of childhood poisonings review comparing six interventions that promote the use of safety equipment in the home. Most of the studies use ‘usual care’ as a baseline and compare this to another intervention. There are also studies in the evidence base that compare pairs of the interventions, such as ‘Education and equipment’ to ‘Equipment’. The plot also demonstrates the absence of direct study evidence between many pairs of interventions, for which the associated treatment effects can be indirectly estimated using NMA.
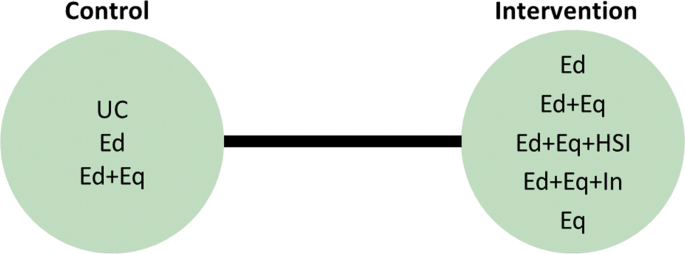
Network plot to illustrate how pairwise meta-analysis groups the interventions in the motivating dataset. Notation UC: Usual care, Ed: Education, Ed+Eq: Education and equipment, Ed+Eq+HSI: Education, equipment, and home safety inspection, Ed+Eq+In: Education, equipment and installation, Eq: Equipment
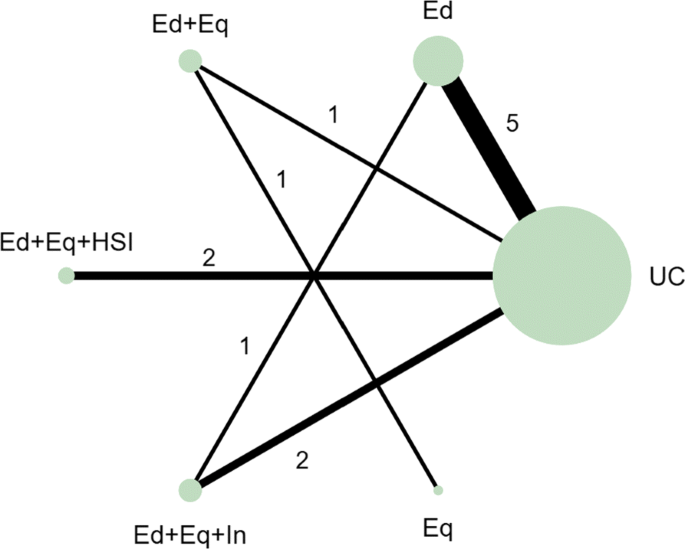
Network plot for the safe storage of cleaning products outcome. Notation UC: Usual care, Ed: Education, Ed+Eq: Education and equipment, Ed+Eq+HSI: Education, equipment, and home safety inspection, Ed+Eq+In: Education, equipment and installation, Eq: Equipment
An NMA was fitted to the motivating example to compare the six interventions in the studies from the review. The results are reported in the ‘triangle table’ in Table 5 [ 17 ]. The top right half of the table shows the direct evidence between pairs of the interventions in the corresponding rows and columns by either pooling the studies as a pairwise meta-analysis or presenting the single study results if evidence is only available from a single study. The bottom left half of the table reports the results of the NMA. The gaps in the top right half of the table arise where no direct study evidence exists to compare the two interventions. For example, there is no direct study evidence comparing ‘Education’ (Ed) to ‘Education, equipment and home safety inspection’ (Ed+Eq+HSI). The NMA, however, can estimate this comparison through the direct study evidence as an odds ratio of 3.80 with a 95% credible interval of (1.16, 12.44). The results suggest that the odds of safely storing cleaning products in the Ed+Eq+HSI intervention group is 3.80 times the odds in the Ed group. The results demonstrate a key benefit of NMA that all intervention effects in a network can be estimated using indirect evidence, even if there is no direct study evidence for some pairwise comparisons. This is based on the consistency assumption (that estimates of intervention effects from direct and indirect evidence are consistent) which should be checked when performing an NMA. This is beyond the scope of this paper and details on this can be found elsewhere [ 18 ].
NMA can also be used to rank the interventions in terms of their effectiveness and estimate the probability that each intervention is likely to be the most effective. This can help to answer the research question ‘which intervention is the best?’ out of all of the interventions that have provided evidence in the network. The rankings and associated probabilities for the motivating example are presented in Table 6 . It can be seen that in this case the ‘education, equipment and home safety inspection’ (Ed+Eq+HSI) intervention is ranked first, with a 0.87 probability of being the best intervention. However, there is overlap of the 95% credible intervals of the median rankings. This overlap reflects the uncertainty in the intervention effect estimates and therefore it is important that the interpretation of these statistics clearly communicates this uncertainty to decision makers.
NMA has the potential to be extremely useful but is underutilised in the evidence synthesis of public health interventions. The ability to compare and rank multiple interventions in an area where there are often multiple intervention options is invaluable in decision making for identifying which intervention to recommend. NMA can also include further literature in the analysis, compared to a pairwise meta-analysis, by expanding the network to improve the uncertainty in the effectiveness estimates.
Statistical heterogeneity
When heterogeneity remains in the results of an NMA, it is useful to explore the reasons for this. Strategies for dealing with heterogeneity involve the inclusion of covariates in a meta-analysis or NMA to adjust for the differences in the covariates across studies [ 19 ]. Meta-regression is a statistical method developed from meta-analysis that includes covariates to potentially explain the between-study heterogeneity ‘with the aim of estimating treatment-covariate interactions’ (Saramago et al. 2012). NMA has been extended to network meta-regression which investigates the effect of trial characteristics on multiple intervention effects. Three ways have been suggested to include covariates in an NMA: single covariate effect, exchangeable covariate effects and independent covariate effects which are discussed in more detail in the NICE Technical Support Document 3 [ 14 ]. This method has the potential to assess the effect of study level covariates on the intervention effects, which is particularly relevant in public health due to the variation across studies.
The most widespread method of meta-regression uses study level data for the inclusion of covariates into meta-regression models. Study level covariate data is when the data from the studies are aggregated, e.g. the proportion of participants in a study that are from single parent families compared to dual parent families. The alternative to study level data is individual participant data (IPD), where the data are available and used as a covariate at the individual level e.g. the parental status of every individual in a study can be used as a covariate. Although IPD is considered to be the gold standard for meta-analysis, aggregated level data is much more commonly used as it is usually available and easily accessible from published research whereas IPD can be hard to obtain from study authors.
There are some limitations to network meta-regression. In our motivating example, using the single parent covariate in a meta-regression would estimate the relative difference in the intervention effects of a population that is made up of 100% single parent families compared to a population that is made up of 100% dual parent families. This interpretation is not as useful as the analysis that uses IPD, which would give the relative difference of the intervention effects in a single parent family compared to a dual parent family. The meta-regression using aggregated data would also be susceptible to ecological bias. Ecological bias is where the effect of the covariate is different at the study level compared to the individual level [ 14 ]. For example, if each study demonstrates a relationship between a covariate and the intervention but the covariate is similar across the studies, a meta-regression of the aggregate data would not demonstrate the effect that is observed within the studies [ 20 ].
Although meta-regression is a useful tool for investigating sources of heterogeneity in the data, caution should be taken when using the results of meta-regression to explain how covariates affect the intervention effects. Meta-regression should only be used to investigate study characteristics, such as the duration of intervention, which will not be susceptible to ecological bias and the interpretation of the results (the effect of intervention duration on intervention effectiveness) would be more meaningful for the development of public health interventions.
Since the covariate of interest in this motivating example is not a study characteristic, meta-regression of aggregated covariate data was not performed. Network meta-regression including IPD and aggregate level data was developed by Samarago et al. (2012) [ 21 ] to overcome the issues with aggregated data network meta-regression, which is discussed in the next section.
Tailored decision making to specific sub-groups
In public health it is important to identify which interventions are best for which people. There has been a recent move towards precision medicine. In the field of public health the ‘concept of precision prevention may [...] be valuable for efficiently targeting preventive strategies to the specific subsets of a population that will derive maximal benefit’ (Khoury and Evans, 2015). Tailoring interventions has the potential to reduce the effect of inequalities in social factors that are influencing the health of the population. Identifying which interventions should be targeted to which subgroups can also lead to better public health outcomes and help to allocate scarce NHS resources. Research interest, therefore, lies in identifying participant level covariate-intervention interactions.
IPD meta-analysis uses data at the individual level to overcome ecological bias. The interpretation of IPD meta-analysis is more relevant in the case of using participant characteristics as covariates since the interpretation of the covariate-intervention interaction is at the individual level rather than the study level. This means that it can answer the research question: ‘which interventions work best in subgroups of the population?’. IPD meta-analyses are considered to be the gold standard for evidence synthesis since it increases the power of the analysis to identify covariate-intervention interactions and it has the ability to reduce the effect of ecological bias compared to aggregated data alone. IPD meta-analysis can also help to overcome scarcity of data issues and has been shown to have higher power and reduce the uncertainty in the estimates compared to analysis including only summary aggregate data [ 22 ].
Despite the advantages of including IPD in a meta-analysis, in reality it is often very time consuming and difficult to collect IPD for all of the studies [ 21 ]. Although data sharing is becoming more common, it remains time consuming and difficult to collect IPD for all studies in a review. This results in IPD being underutilised in meta-analyses. As an intermediate solution, statistical methods have been developed, such as the NMA in Samarago et al. (2012), that incorporates both IPD and aggregate data. Methods that simultaneously include IPD and aggregate level data have been shown to reduce uncertainty in the effect estimates and minimise ecological bias [ 20 , 21 ]. A simulation study by Leahy et al. (2018) found that an increased proportion of IPD resulted in more accurate and precise NMA estimates [ 23 ].
An NMA including IPD, where it is available, was performed, based on the model presented in Samarago et al. (2012) [ 21 ]. The results in Table 7 demonstrates the detail that this type of analysis can provide to base decisions on. More relevant covariate-intervention interaction interpretations can be obtained, for example the regression coefficients for covariate-intervention interactions are the individual level covariate intervention interactions or the ‘within study interactions’ that are interpreted as the effect of being in a single parent family on the effectiveness of each of the interventions. For example, the effect of Ed+Eq compared to UC in a single parent family is 1.66 times the effect of Ed+Eq compared to UC in a dual parent family but this is not an important difference as the credible interval crosses 1. The regression coefficients for the study level covariate-intervention interactions or the ‘between study interactions’ can be interpreted as the relative difference in the intervention effects of a population that is made up of 100% single parent families compared to a population that is made up of 100% dual parent families.
- Complex interventions
In many public health research settings the complex interventions are comprised of a number of components. An NMA can compare all of the interventions in a network as they are implemented in the original trials. However, NMA does not tell us which components of the complex intervention are attributable to this effect. It could be that particular components, or the interacting effect of multiple components, are driving the effectiveness and other components are not as effective. Often, trials have not directly compared every combination of components as there are so many component combination options, it would be inefficient and impractical. Component NMA was developed by Welton et al. (2009) to estimate the effect of each component of the complex interventions and combination of components in a network, in the absence of direct trial evidence and answers the question: ‘are interventions with a particular component or combination of components effective?’ [ 11 ]. For example, for the motivating example, in comparison to Fig. 5 , which demonstrates the interventions that an NMA can estimate effectiveness, Fig. 6 demonstrates all of the possible interventions of which the effectiveness can be estimated in a component NMA, given the components present in the network.
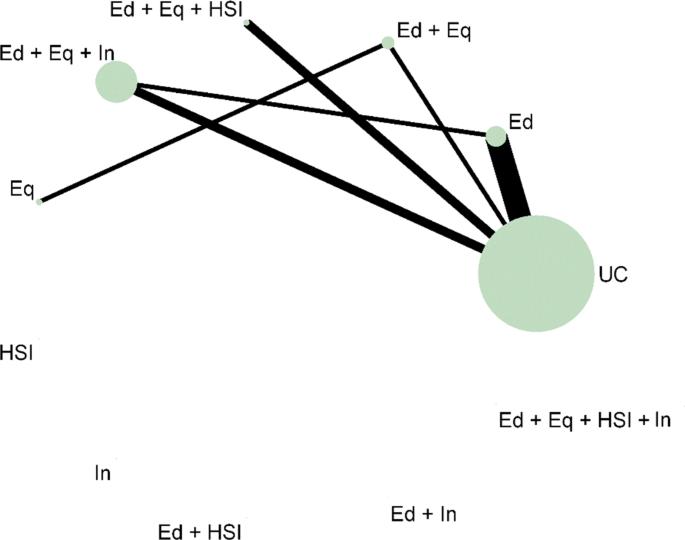
Network plot that illustrates how component network meta-analysis can estimate the effectiveness of intervention components and combinations of components, even when they are not included in the direct evidence. Notation UC: Usual care, Ed: Education, Eq: Equipment, Installation, Ed+Eq: Education and equipment, Ed+HSI: Education and home safety inspection, Ed+In: Education and installation, Eq+HSI: Equipment and home safety inspection, Eq+In: equipment and installation, HSI+In: Home safety inspection and installation, Ed+Eq+HSI: Education, equipment, and home safety inspection, Ed+Eq+In: Education, equipment and installation, Eq+HSI+In: Equipment, home safety inspection and installation, Ed+Eq+HSI+In: Education, equipment, home safety inspection and installation
The results of the analyses of the main effects, two way effects and full effects models are shown in Table 8 . The models, proposed in the original paper by Welton et al. (2009), increase in complexity as the assumptions regarding the component effects relax [ 24 ]. The main effects component NMA assumes that the components in the interventions each have separate, independent effects and intervention effects are the sum of the component effects. The two-way effects models assumes that there are interactions between pairs of the components, so the effects of the interventions are more than the sum of the effects. The full effects model assumes that all of the components and combinations of the components interact. Component NMA did not provide further insight into which components are likely to be the most effective since all of the 95% credible intervals were very wide and overlapped 1. There is a lot of uncertainty in the results, particularly in the 2-way and full effects models. A limitation of component NMA is that there are issues with uncertainty when data is scarce. However, the results demonstrate the potential of component NMA as a useful tool to gain better insights from the available dataset.
In practice, this method has rarely been used since its development [ 24 – 26 ]. It may be challenging to define the components in some areas of public health where many interventions have been studied. However, the use of meta-analysis for planning future studies is rarely discussed and component NMA would provide a useful tool for identifying new component combinations that may be more effective [ 27 ]. This type of analysis has the potential to prioritise future public health research, which is especially useful where there are multiple intervention options, and identify more effective interventions to recommend to the public.
Further methods / other outcomes
The analysis and methods described in this paper only cover a small subset of the methods that have been developed in meta-analysis in recent years. Methods that aim to assess the quality of evidence supporting a NMA and how to quantify how much the evidence could change due to potential biases or sampling variation before the recommendation changes have been developed [ 28 , 29 ]. Models adjusting for baseline risk have been developed to allow for different study populations to have different levels of underlying risk, by using the observed event rate in the control arm [ 30 , 31 ]. Multivariate methods can be used to compare the effect of multiple interventions on two or more outcomes simultaneously [ 32 ]. This area of methodological development is especially appealing within public health where studies assess a broad range of health effects and typically have multiple outcome measures. Multivariate methods offer benefits over univariate models by allowing the borrowing of information across outcomes and modelling the relationships between outcomes which can potentially reduce the uncertainty in the effect estimates [ 33 ]. Methods have also been developed to evaluate interventions with classes or different intervention intensities, known as hierarchical interventions [ 34 ]. These methods were not demonstrated in this paper but can also be useful tools for addressing challenges of appraising public health interventions, such as multiple and surrogate outcomes.
This paper only considered an example with a binary outcome. All of the methods described have also been adapted for other outcome measures. For example, the Technical Support Document 2 proposed a Bayesian generalised linear modelling framework to synthesise other outcome measures. More information and models for continuous and time-to-event data is available elsewhere [ 21 , 35 – 38 ].
Software and guidelines
In the previous section, meta-analytic methods that answer more policy relevant questions were demonstrated. However, as shown by the update to the review, methods such as these are still under-utilised. It is suspected from the NICE public health review that one of the reasons for the lack of uptake of methods in public health could be due to common software choices, such as RevMan, being limited in their flexibility for statistical methods.
Table 9 provides a list of software options and guidance documents that are more flexible than RevMan for implementing the statistical methods illustrated in the previous section to make these methods more accessible to researchers.
In this paper, the network plot in Figs. 5 and 6 were produced using the networkplot command from the mvmeta package [ 39 ] in Stata [ 61 ]. WinBUGS was used to fit the NMA in this paper by adapting the code in the book ‘Evidence Synthesis for Decision Making in Healthcare’ which also provides more detail on Bayesian methods and assessing convergence of Bayesian models [ 45 ]. The model for including IPD and summary aggregate data in an NMA was based on the code in the paper by Saramago et al. (2012). The component NMA in this paper was performed in WinBUGS through R2WinBUGS, [ 47 ] using the code in Welton et al. (2009) [ 11 ].
WinBUGS is a flexible tool for fitting complex models in a Bayesian framework. The NICE Decision Support Unit produced a series of Evidence Synthesis Technical Support Documents [ 46 ] that provide a comprehensive technical guide to methods for evidence synthesis and WinBUGS code is also provided for many of the models. Complex models can also be performed in a frequentist framework. Code and commands for many models are available in R and STATA (see Table 9 ).
The software, R2WinBUGS, was used in the analysis of the motivating example. Increasing numbers of researchers are using R and so packages that can be used to link the two softwares by calling BUGS models in R, packages such as R2WinBUGS, can improve the accessibility of Bayesian methods [ 47 ]. The new R package, BUGSnet, may also help to facilitate the accessibility and improve the reporting of Bayesian NMA [ 48 ]. Webtools have also been developed as a means of enabling researchers to undertake increasingly complex analyses [ 52 , 53 ]. Webtools provide a user-friendly interface to perform statistical analyses and often help in the reporting of the analyses by producing plots, including network plots and forest plots. These tools are very useful for researchers that have a good understanding of the statistical methods they want to implement as part of their review but are inexperienced in statistical software.
This paper has reviewed NICE public health intervention guidelines to identify the methods that are currently being used to synthesise effectiveness evidence to inform public health decision making. A previous review from 2012 was updated to see how method utilisation has changed. Methods have been developed since the previous review and these were applied to an example dataset to show how methods can answer more policy relevant questions. Resources and guidelines for implementing these methods were signposted to encourage uptake.
The review found that the proportion of NICE guidelines containing effectiveness evidence summarised using meta-analysis methods has increased since the original review, but remains low. The majority of the reviews presented only narrative summaries of the evidence - a similar result to the original review. In recent years, there has been an increased awareness of the need to improve decision making by using all of the available evidence. As a result, this has led to the development of new methods, easier application in standard statistical software packages, and guidance documents. Based on this, it would have been expected that their implementation would rise in recent years to reflect this, but the results of the review update showed no such increasing pattern.
A high proportion of NICE guideline reports did not provide a reason for not applying quantitative evidence synthesis methods. Possible explanations for this could be time or resource constraints, lack of statistical expertise, being unaware of the available methods or poor reporting. Reporting guidelines, such as the Preferred Reporting Items for Systematic Reviews and Meta-Analyses (PRISMA), should be updated to emphasise the importance of documenting reasons for not applying methods, as this can direct future research to improve uptake.
Where it was specified, the most common reported reason for not conducting a meta-analysis was heterogeneity. Often in public health, the data is heterogeneous due to the differences between studies in population, design, interventions or outcomes. A common misconception is that the presence of heterogeneity implies that it is not possible to pool the data. Meta-analytic methods can be used to investigate the sources of heterogeneity, as demonstrated in the NMA of the motivating example, and the use of IPD is recommended where possible to improve the precision of the results and reduce the effect of ecological bias. Although caution should be exercised in the interpretation of the results, quantitative synthesis methods provide a stronger basis for making decisions than narrative accounts because they explicitly quantify the heterogeneity and seek to explain it where possible.
The review also found that the most common software to perform the synthesis was RevMan. RevMan is very limited in its ability to perform advanced statistical analyses, beyond that of pairwise meta-analysis, which might explain the above findings. Standard software code is being developed to help make statistical methodology and application more accessible and guidance documents are becoming increasingly available.
The evaluation of public health interventions can be problematic due to the number and complexity of the interventions. NMA methods were applied to a real Cochrane public health review dataset. The methods that were demonstrated showed ways to address some of these issues, including the use of NMA for multiple interventions, the inclusion of covariates as both aggregated data and IPD to explain heterogeneity, and the extension to component network meta-analysis for guiding future research. These analyses illustrated how the choice of synthesis methods can enable more informed decision making by allowing more distinct interventions, and combinations of intervention components, to be defined and their effectiveness estimated. It also demonstrated the potential to target interventions to population subgroups where they are likely to be most effective. However, the application of component NMA to the motivating example has also demonstrated the issues around uncertainty if there are a limited number of studies observing the interventions and intervention components.
The application of methods to the motivating example demonstrated a key benefit of using statistical methods in a public health context compared to only presenting a narrative review – the methods provide a quantitative estimate of the effectiveness of the interventions. The uncertainty from the credible intervals can be used to demonstrate the lack of available evidence. In the context of decision making, having pooled estimates makes it much easier for decision makers to assess the effectiveness of the interventions or identify when more research is required. The posterior distribution of the pooled results from the evidence synthesis can also be incorporated into a comprehensive decision analytic model to determine cost-effectiveness [ 62 ]. Although narrative reviews are useful for describing the evidence base, the results are very difficult to summarise in a decision context.
Although heterogeneity seems to be inevitable within public health interventions due to their complex nature, this review has shown that it is still the main reported reason for not using statistical methods in evidence synthesis. This may be due to guidelines that were originally developed for clinical treatments that are tested in randomised conditions still being applied in public health settings. Guidelines for the choice of methods used in public health intervention appraisals could be updated to take into account the complexities and wide ranging areas in public health. Sophisticated methods may be more appropriate in some cases than simpler models for modelling multiple, complex interventions and their uncertainty, given the limitations are also fully reported [ 19 ]. Synthesis may not be appropriate if statistical heterogeneity remains after adjustment for possible explanatory covariates but details of exploratory analysis and reasons for not synthesising the data should be reported. Future research should focus on the application and dissemination of the advantages of using more advanced methods in public health, identifying circumstances where these methods are likely to be the most beneficial, and ways to make the methods more accessible, for example, the development of packages and web tools.
There is an evident need to facilitate the translation of the synthesis methods into a public health context and encourage the use of methods to improve decision making. This review has shown that the uptake of statistical methods for evaluating the effectiveness of public health interventions is slow, despite advances in methods that address specific issues in public health intervention appraisal and the publication of guidance documents to complement their application.
Availability of data and materials
The dataset supporting the conclusions of this article is included within the article.
Abbreviations
National institute for health and care excellence
- Network meta-analysis
Individual participant data
Home safety inspection
Installation
Credible interval
Preferred reporting items for systematic reviews and meta-analyses
Dias S, Welton NJ, Sutton AJ, Ades A. NICE DSU Technical Support Document 2: A Generalised Linear Modelling Framework for Pairwise and Network Meta-Analysis of Randomised Controlled Trials: National Institute for Health and Clinical Excellence; 2011, p. 98. (Technical Support Document in Evidence Synthesis; TSD2).
Higgins JPT, López-López JA, Becker BJ, et al.Synthesising quantitative evidence in systematic reviews of complex health interventions. BMJ Global Health. 2019; 4(Suppl 1):e000858. https://doi.org/10.1136/bmjgh-2018-000858 .
Article PubMed PubMed Central Google Scholar
Achana F, Hubbard S, Sutton A, Kendrick D, Cooper N. An exploration of synthesis methods in public health evaluations of interventions concludes that the use of modern statistical methods would be beneficial. J Clin Epidemiol. 2014; 67(4):376–90.
Article PubMed Google Scholar
Craig P, Dieppe P, Macintyre S, Michie S, Nazareth I, Petticrew M. Developing and evaluating complex interventions: the new medical research council guidance. Int J Nurs Stud. 2013; 50(5):587–92.
Caldwell DM, Welton NJ. Approaches for synthesising complex mental health interventions in meta-analysis. Evidence-Based Mental Health. 2016; 19(1):16–21.
Melendez-Torres G, Bonell C, Thomas J. Emergent approaches to the meta-analysis of multiple heterogeneous complex interventions. BMC Med Res Methodol. 2015; 15(1):47.
Article CAS PubMed PubMed Central Google Scholar
NICE. NICE: Who We Are. https://www.nice.org.uk/about/who-we-are . Accessed 19 Sept 2019.
Kelly M, Morgan A, Ellis S, Younger T, Huntley J, Swann C. Evidence based public health: a review of the experience of the national institute of health and clinical excellence (NICE) of developing public health guidance in England. Soc Sci Med. 2010; 71(6):1056–62.
NICE. Developing NICE Guidelines: The Manual. https://www.nice.org.uk/process/pmg20/chapter/introduction-and-overview . Accessed 19 Sept 2019.
NICE. Public Health Guidance. https://www.nice.org.uk/guidance/published?type=ph . Accessed 19 Sept 2019.
Welton NJ, Caldwell D, Adamopoulos E, Vedhara K. Mixed treatment comparison meta-analysis of complex interventions: psychological interventions in coronary heart disease. Am J Epidemiol. 2009; 169(9):1158–65.
Ioannidis JP, Patsopoulos NA, Rothstein HR. Reasons or excuses for avoiding meta-analysis in forest plots. BMJ. 2008; 336(7658):1413–5.
Higgins JP, Thompson SG. Quantifying heterogeneity in a meta-analysis. Stat Med. 2002; 21(11):1539–58.
Article Google Scholar
Dias S, Sutton A, Welton N, Ades A. NICE DSU Technical Support Document 3: Heterogeneity: Subgroups, Meta-Regression, Bias and Bias-Adjustment: National Institute for Health and Clinical Excellence; 2011, p. 76.
Kendrick D, Ablewhite J, Achana F, et al.Keeping Children Safe: a multicentre programme of research to increase the evidence base for preventing unintentional injuries in the home in the under-fives. Southampton: NIHR Journals Library; 2017.
Google Scholar
Lunn DJ, Thomas A, Best N, et al.WinBUGS - A Bayesian modelling framework: Concepts, structure, and extensibility. Stat Comput. 2000; 10:325–37. https://doi.org/10.1023/A:1008929526011 .
Dias S, Caldwell DM. Network meta-analysis explained. Arch Dis Child Fetal Neonatal Ed. 2019; 104(1):8–12. https://doi.org/10.1136/archdischild-2018-315224. http://arxiv.org/abs/https://fn.bmj.com/content/104/1/F8.full.pdf.
Dias S, Welton NJ, Sutton AJ, Caldwell DM, Lu G, Ades A. NICE DSU Technical Support Document 4: Inconsistency in Networks of Evidence Based on Randomised Controlled Trials: National Institute for Health and Clinical Excellence; 2011. (NICE DSU Technical Support Document in Evidence Synthesis; TSD4).
Cipriani A, Higgins JP, Geddes JR, Salanti G. Conceptual and technical challenges in network meta-analysis. Ann Intern Med. 2013; 159(2):130–7.
Riley RD, Steyerberg EW. Meta-analysis of a binary outcome using individual participant data and aggregate data. Res Synth Methods. 2010; 1(1):2–19.
Saramago P, Sutton AJ, Cooper NJ, Manca A. Mixed treatment comparisons using aggregate and individual participant level data. Stat Med. 2012; 31(28):3516–36.
Lambert PC, Sutton AJ, Abrams KR, Jones DR. A comparison of summary patient-level covariates in meta-regression with individual patient data meta-analysis. J Clin Epidemiol. 2002; 55(1):86–94.
Article CAS PubMed Google Scholar
Leahy J, O’Leary A, Afdhal N, Gray E, Milligan S, Wehmeyer MH, Walsh C. The impact of individual patient data in a network meta-analysis: an investigation into parameter estimation and model selection. Res Synth Methods. 2018; 9(3):441–69.
Freeman SC, Scott NW, Powell R, Johnston M, Sutton AJ, Cooper NJ. Component network meta-analysis identifies the most effective components of psychological preparation for adults undergoing surgery under general anesthesia. J Clin Epidemiol. 2018; 98:105–16.
Pompoli A, Furukawa TA, Efthimiou O, Imai H, Tajika A, Salanti G. Dismantling cognitive-behaviour therapy for panic disorder: a systematic review and component network meta-analysis. Psychol Med. 2018; 48(12):1945–53.
Rücker G, Schmitz S, Schwarzer G. Component network meta-analysis compared to a matching method in a disconnected network: A case study. Biom J. 2020. https://doi.org/10.1002/bimj.201900339 .
Efthimiou O, Debray TP, van Valkenhoef G, Trelle S, Panayidou K, Moons KG, Reitsma JB, Shang A, Salanti G, Group GMR. GetReal in network meta-analysis: a review of the methodology. Res Synth Methods. 2016; 7(3):236–63.
Salanti G, Del Giovane C, Chaimani A, Caldwell DM, Higgins JP. Evaluating the quality of evidence from a network meta-analysis. PLoS ONE. 2014; 9(7):99682.
Article CAS Google Scholar
Phillippo DM, Dias S, Welton NJ, Caldwell DM, Taske N, Ades A. Threshold analysis as an alternative to grade for assessing confidence in guideline recommendations based on network meta-analyses. Ann Intern Med. 2019; 170(8):538–46.
Dias S, Welton NJ, Sutton AJ, Ades AE. NICE DSU Technical Support Document 5: Evidence Synthesis in the Baseline Natural History Model: National Institute for Health and Clinical Excellence; 2011, p. 29. (NICE DSU Technical Support Document in Evidence Synthesis; TSD5).
Achana FA, Cooper NJ, Dias S, Lu G, Rice SJ, Kendrick D, Sutton AJ. Extending methods for investigating the relationship between treatment effect and baseline risk from pairwise meta-analysis to network meta-analysis. Stat Med. 2013; 32(5):752–71.
Riley RD, Jackson D, Salanti G, Burke DL, Price M, Kirkham J, White IR. Multivariate and network meta-analysis of multiple outcomes and multiple treatments: rationale, concepts, and examples. BMJ (Clinical research ed.) 2017; 358:j3932. https://doi.org/10.1136/bmj.j3932 .
Achana FA, Cooper NJ, Bujkiewicz S, Hubbard SJ, Kendrick D, Jones DR, Sutton AJ. Network meta-analysis of multiple outcome measures accounting for borrowing of information across outcomes. BMC Med Res Methodol. 2014; 14(1):92.
Owen RK, Tincello DG, Keith RA. Network meta-analysis: development of a three-level hierarchical modeling approach incorporating dose-related constraints. Value Health. 2015; 18(1):116–26.
Jansen JP. Network meta-analysis of individual and aggregate level data. Res Synth Methods. 2012; 3(2):177–90.
Donegan S, Williamson P, D’Alessandro U, Garner P, Smith CT. Combining individual patient data and aggregate data in mixed treatment comparison meta-analysis: individual patient data may be beneficial if only for a subset of trials. Stat Med. 2013; 32(6):914–30.
Saramago P, Chuang L-H, Soares MO. Network meta-analysis of (individual patient) time to event data alongside (aggregate) count data. BMC Med Res Methodol. 2014; 14(1):105.
Thom HH, Capkun G, Cerulli A, Nixon RM, Howard LS. Network meta-analysis combining individual patient and aggregate data from a mixture of study designs with an application to pulmonary arterial hypertension. BMC Med Res Methodol. 2015; 15(1):34.
Gasparrini A, Armstrong B, Kenward MG. Multivariate meta-analysis for non-linear and other multi-parameter associations. Stat Med. 2012; 31(29):3821–39.
Chaimani A, Higgins JP, Mavridis D, Spyridonos P, Salanti G. Graphical tools for network meta-analysis in stata. PLoS ONE. 2013; 8(10):76654.
Rücker G, Schwarzer G, Krahn U, König J. netmeta: Network meta-analysis with R. R package version 0.5-0. 2014. R package version 0.5-0. Availiable: http://CRAN.R-project.org/package=netmeta .
van Valkenhoef G, Kuiper J. gemtc: Network Meta-Analysis Using Bayesian Methods. R package version 0.8-2. 2016. Available online at: https://CRAN.R-project.org/package=gemtc .
Lin L, Zhang J, Hodges JS, Chu H. Performing arm-based network meta-analysis in R with the pcnetmeta package. J Stat Softw. 2017; 80(5):1–25. https://doi.org/10.18637/jss.v080.i05 .
Rücker G, Schwarzer G. Automated drawing of network plots in network meta-analysis. Res Synth Methods. 2016; 7(1):94–107.
Welton NJ, Sutton AJ, Cooper N, Abrams KR, Ades A. Evidence Synthesis for Decision Making in Healthcare, vol. 132. UK: Wiley; 2012.
Book Google Scholar
Dias S, Welton NJ, Sutton AJ, Ades AE. Evidence synthesis for decision making 1: introduction. Med Decis Making Int J Soc Med Decis Making. 2013; 33(5):597–606. https://doi.org/10.1177/0272989X13487604 .
Sturtz S, Ligges U, Gelman A. R2WinBUGS: a package for running WinBUGS from R. J Stat Softw. 2005; 12(3):1–16.
Béliveau A, Boyne DJ, Slater J, Brenner D, Arora P. Bugsnet: an r package to facilitate the conduct and reporting of bayesian network meta-analyses. BMC Med Res Methodol. 2019; 19(1):196.
Neupane B, Richer D, Bonner AJ, Kibret T, Beyene J. Network meta-analysis using R: a review of currently available automated packages. PLoS ONE. 2014; 9(12):115065.
White IR. Multivariate random-effects meta-analysis. Stata J. 2009; 9(1):40–56.
Chaimani A, Salanti G. Visualizing assumptions and results in network meta-analysis: the network graphs package. Stata J. 2015; 15(4):905–50.
Owen RK, Bradbury N, Xin Y, Cooper N, Sutton A. MetaInsight: An interactive web-based tool for analyzing, interrogating, and visualizing network meta-analyses using R-shiny and netmeta. Res Synth Methods. 2019; 10(4):569–81. https://doi.org/10.1002/jrsm.1373 .
Freeman SC, Kerby CR, Patel A, Cooper NJ, Quinn T, Sutton AJ. Development of an interactive web-based tool to conduct and interrogate meta-analysis of diagnostic test accuracy studies: MetaDTA. BMC Med Res Methodol. 2019; 19(1):81.
Nikolakopoulou A, Higgins JPT, Papakonstantinou T, Chaimani A, Del Giovane C, Egger M, Salanti G. CINeMA: An approach for assessing confidence in the results of a network meta-analysis. PLoS Med. 2020; 17(4):e1003082. https://doi.org/10.1371/journal.pmed.1003082 .
Viechtbauer W. Conducting meta-analyses in R with the metafor package. J Stat Softw. 2010; 36(3):1–48.
Freeman SC, Carpenter JR. Bayesian one-step ipd network meta-analysis of time-to-event data using royston-parmar models. Res Synth Methods. 2017; 8(4):451–64.
Riley RD, Lambert PC, Staessen JA, Wang J, Gueyffier F, Thijs L, Boutitie F. Meta-analysis of continuous outcomes combining individual patient data and aggregate data. Stat Med. 2008; 27(11):1870–93.
Debray TP, Moons KG, van Valkenhoef G, Efthimiou O, Hummel N, Groenwold RH, Reitsma JB, Group GMR. Get real in individual participant data (ipd) meta-analysis: a review of the methodology. Res Synth Methods. 2015; 6(4):293–309.
Tierney JF, Vale C, Riley R, Smith CT, Stewart L, Clarke M, Rovers M. Individual Participant Data (IPD) Meta-analyses of Randomised Controlled Trials: Guidance on Their Use. PLoS Med. 2015; 12(7):e1001855. https://doi.org/10.1371/journal.pmed.1001855 .
Stewart LA, Clarke M, Rovers M, Riley RD, Simmonds M, Stewart G, Tierney JF. Preferred reporting items for a systematic review and meta-analysis of individual participant data: the prisma-ipd statement. JAMA. 2015; 313(16):1657–65.
StataCorp. Stata Statistical Software: Release 16. College Station: StataCorp LLC; 2019.
Cooper NJ, Sutton AJ, Abrams KR, Turner D, Wailoo A. Comprehensive decision analytical modelling in economic evaluation: a bayesian approach. Health Econ. 2004; 13(3):203–26.
Download references
Acknowledgements
We would like to acknowledge Professor Denise Kendrick as the lead on the NIHR Keeping Children Safe at Home Programme that originally funded the collection of the evidence for the motivating example and some of the analyses illustrated in the paper.
ES is funded by a National Institute for Health Research (NIHR), Doctoral Research Fellow for this research project. This paper presents independent research funded by the National Institute for Health Research (NIHR). The views expressed are those of the authors and not necessarily those of the NHS, the NIHR or the Department of Health and Social Care. The funding bodies played no role in the design of the study and collection, analysis, and interpretation of data and in writing the manuscript.
Author information
Authors and affiliations.
Department of Health Sciences, University of Leicester, Lancaster Road, Leicester, UK
Ellesha A. Smith, Nicola J. Cooper, Alex J. Sutton, Keith R. Abrams & Stephanie J. Hubbard
You can also search for this author in PubMed Google Scholar
Contributions
ES performed the review, analysed the data and wrote the paper. SH supervised the project. SH, KA, NC and AS provided substantial feedback on the manuscript. All authors have read and approved the manuscript.
Corresponding author
Correspondence to Ellesha A. Smith .
Ethics declarations
Ethics approval and consent to participate.
Not applicable.
Consent for publication
Competing interests.
KA is supported by Health Data Research (HDR) UK, the UK National Institute for Health Research (NIHR) Applied Research Collaboration East Midlands (ARC EM), and as a NIHR Senior Investigator Emeritus (NF-SI-0512-10159). The views expressed are those of the author(s) and not necessarily those of the NIHR or the Department of Health and Social Care. KA has served as a paid consultant, providing unrelated methodological advice, to; Abbvie, Amaris, Allergan, Astellas, AstraZeneca, Boehringer Ingelheim, Bristol-Meyers Squibb, Creativ-Ceutical, GSK, ICON/Oxford Outcomes, Ipsen, Janssen, Eli Lilly, Merck, NICE, Novartis, NovoNordisk, Pfizer, PRMA, Roche and Takeda, and has received research funding from Association of the British Pharmaceutical Industry (ABPI), European Federation of Pharmaceutical Industries & Associations (EFPIA), Pfizer, Sanofi and Swiss Precision Diagnostics. He is a Partner and Director of Visible Analytics Limited, a healthcare consultancy company.
Additional information
Publisher’s note.
Springer Nature remains neutral with regard to jurisdictional claims in published maps and institutional affiliations.
Supplementary Information
Additional file 1.
Key for the Nice public health guideline codes. Available in NICEGuidelinesKey.xlsx .
Additional file 2
NICE public health intervention guideline review flowchart for the inclusion and exclusion of documents. Available in Flowchart.JPG .
Rights and permissions
Open Access This article is licensed under a Creative Commons Attribution 4.0 International License, which permits use, sharing, adaptation, distribution and reproduction in any medium or format, as long as you give appropriate credit to the original author(s) and the source, provide a link to the Creative Commons licence, and indicate if changes were made. The images or other third party material in this article are included in the article’s Creative Commons licence, unless indicated otherwise in a credit line to the material. If material is not included in the article’s Creative Commons licence and your intended use is not permitted by statutory regulation or exceeds the permitted use, you will need to obtain permission directly from the copyright holder. To view a copy of this licence, visit http://creativecommons.org/licenses/by/4.0/ . The Creative Commons Public Domain Dedication waiver ( http://creativecommons.org/publicdomain/zero/1.0/ ) applies to the data made available in this article, unless otherwise stated in a credit line to the data.
Reprints and permissions
About this article
Cite this article.
Smith, E.A., Cooper, N.J., Sutton, A.J. et al. A review of the quantitative effectiveness evidence synthesis methods used in public health intervention guidelines. BMC Public Health 21 , 278 (2021). https://doi.org/10.1186/s12889-021-10162-8
Download citation
Received : 22 September 2020
Accepted : 04 January 2021
Published : 03 February 2021
DOI : https://doi.org/10.1186/s12889-021-10162-8
Share this article
Anyone you share the following link with will be able to read this content:
Sorry, a shareable link is not currently available for this article.
Provided by the Springer Nature SharedIt content-sharing initiative
- Meta-analysis
- Systematic review
- Public health
- Decision making
- Evidence synthesis
BMC Public Health
ISSN: 1471-2458
- Submission enquiries: [email protected]
- General enquiries: [email protected]
Quantitative research
Affiliation.
- 1 Faculty of Health and Social Care, University of Hull, Hull, England.
- PMID: 25828021
- DOI: 10.7748/ns.29.31.44.e8681
This article describes the basic tenets of quantitative research. The concepts of dependent and independent variables are addressed and the concept of measurement and its associated issues, such as error, reliability and validity, are explored. Experiments and surveys – the principal research designs in quantitative research – are described and key features explained. The importance of the double-blind randomised controlled trial is emphasised, alongside the importance of longitudinal surveys, as opposed to cross-sectional surveys. Essential features of data storage are covered, with an emphasis on safe, anonymous storage. Finally, the article explores the analysis of quantitative data, considering what may be analysed and the main uses of statistics in analysis.
Keywords: Experiments; measurement; nursing research; quantitative research; reliability; surveys; validity.
- Biomedical Research / methods*
- Double-Blind Method
- Evaluation Studies as Topic
- Longitudinal Studies
- Randomized Controlled Trials as Topic
- United Kingdom
- - Google Chrome
Intended for healthcare professionals
- Access provided by Google Indexer
- My email alerts
- BMA member login
- Username * Password * Forgot your log in details? Need to activate BMA Member Log In Log in via OpenAthens Log in via your institution

Search form
- Advanced search
- Search responses
- Search blogs
- Using data for...
Using data for improvement
Read the full collection.
- Related content
- Peer review
- Amar Shah , chief quality officer and consultant forensic psychiatrist, national improvement lead for the Mental Health Safety Improvement Programme
- East London NHS Foundation Trust, London, E1 8DE, UK
- amarshah{at}nhs.net @DrAmarShah
What you need to know
Both qualitative and quantitative data are critical for evaluating and guiding improvement
A family of measures, incorporating outcome, process, and balancing measures, should be used to track improvement work
Time series analysis, using small amounts of data collected and displayed frequently, is the gold standard for using data for improvement
We all need a way to understand the quality of care we are providing, or receiving, and how our service is performing. We use a range of data in order to fulfil this need, both quantitative and qualitative. Data are defined as “information, especially facts and numbers, collected to be examined and considered and used to help decision-making.” 1 Data are used to make judgements, to answer questions, and to monitor and support improvement in healthcare ( box 1 ). The same data can be used in different ways, depending on what we want to know or learn.
Defining quality improvement 2
Quality improvement aims to make a difference to patients by improving safety, effectiveness, and experience of care by:
Using understanding of our complex healthcare environment
Applying a systematic approach
Designing, testing, and implementing changes using real-time measurement for improvement
Within healthcare, we use a range of data at different levels of the system:
Patient level—such as blood sugar, temperature, blood test results, or expressed wishes for care)
Service level—such as waiting times, outcomes, complaint themes, or collated feedback of patient experience
Organisation level—such as staff experience or financial performance
Population level—such as mortality, quality of life, employment, and air quality.
This article outlines the data we need to understand the quality of care we are providing, what we need to capture to see if care is improving, how to interpret the data, and some tips for doing this more effectively.
Sources and selection criteria
This article is based on my experience of using data for improvement at East London NHS Foundation Trust, which is seen as one of the world leaders in healthcare quality improvement. Our use of data, from trust board to clinical team, has transformed over the past six years in line with the learning shared in this article. This article is also based on my experience of teaching with the Institute for Healthcare Improvement, which guides and supports quality improvement efforts across the globe.
What data do we need?
Healthcare is a complex system, with multiple interdependencies and an array of factors influencing outcomes. Complex systems are open, unpredictable, and continually adapting to their environment. 3 No single source of data can help us understand how a complex system behaves, so we need several data sources to see how a complex system in healthcare is performing.
Avedis Donabedian, a doctor born in Lebanon in 1919, studied quality in healthcare and contributed to our understanding of using outcomes. 4 He described the importance of focusing on structures and processes in order to improve outcomes. 5 When trying to understand quality within a complex system, we need to look at a mix of outcomes (what matters to patients), processes (the way we do our work), and structures (resources, equipment, governance, etc).
Therefore, when we are trying to improve something, we need a small number of measures (ideally 5-8) to help us monitor whether we are moving towards our goal. Any improvement effort should include one or two outcome measures linked explicitly to the aim of the work, a small number of process measures that show how we are doing with the things we are actually working on to help us achieve our aim, and one or two balancing measures ( box 2 ). Balancing measures help us spot unintended consequences of the changes we are making. As complex systems are unpredictable, our new changes may result in an unexpected adverse effect. Balancing measures help us stay alert to these, and ought to be things that are already collected, so that we do not waste extra resource on collecting these.
Different types of measures of quality of care
Outcome measures (linked explicitly to the aim of the project).
Aim— To reduce waiting times from referral to appointment in a clinic
Outcome measure— Length of time from referral being made to being seen in clinic
Data collection— Date when each referral was made, and date when each referral was seen in clinic, in order to calculate the time in days from referral to being seen
Process measures (linked to the things you are going to work on to achieve the aim)
Change idea— Use of a new referral form (to reduce numbers of inappropriate referrals and re-work in obtaining necessary information)
Process measure— Percentage of referrals received that are inappropriate or require further information
Data collection— Number of referrals received that are inappropriate or require further information each week divided by total number of referrals received each week
Change idea— Text messaging patients two days before the appointment (to reduce non-attendance and wasted appointment slots)
Process measure— Percentage of patients receiving a text message two days before appointment
Data collection— Number of patients each week receiving a text message two days before their appointment divided by the total number of patients seen each week
Process measure— Percentage of patients attending their appointment
Data collection— Number of patients attending their appointment each week divided by the total number of patients booked in each week
Balancing measures (to spot unintended consequences)
Measure— Percentage of referrers who are satisfied or very satisfied with the referral process (to spot whether all these changes are having a detrimental effect on the experience of those referring to us)
Data collection— A monthly survey to referrers to assess their satisfaction with the referral process
Measure— Percentage of staff who are satisfied or very satisfied at work (to spot whether the changes are increasing burden on staff and reducing their satisfaction at work)
Data collection— A monthly survey for staff to assess their satisfaction at work
How should we look at the data?
This depends on the question we are trying to answer. If we ask whether an intervention was efficacious, as we might in a research study, we would need to be able to compare data before and after the intervention and remove all potential confounders and bias. For example, to understand whether a new treatment is better than the status quo, we might design a research study to compare the effect of the two interventions and ensure that all other characteristics are kept constant across both groups. This study might take several months, or possibly years, to complete, and would compare the average of both groups to identify whether there is a statistically significant difference.
This approach is unlikely to be possible in most contexts where we are trying to improve quality. Most of the time when we are improving a service, we are making multiple changes and assessing impact in real-time, without being able to remove all confounding factors and potential bias. When we ask whether an outcome has improved, as we do when trying to improve something, we need to be able to look at data over time to see how the system changes as we intervene, with multiple tests of change over a period. For example, if we were trying to improve the time from a patient presenting in the emergency department to being admitted to a ward, we would likely be testing several different changes at different places in the pathway. We would want to be able to look at the outcome measure of total time from presentation to admission on the ward, over time, on a daily basis, to be able to see whether the changes made lead to a reduction in the overall outcome. So, when looking at a quality issue from an improvement perspective, we view smaller amounts of data but more frequently to see if we are improving over time. 2
What is best practice in using data to support improvement?
Best practice would be for each team to have a small number of measures that are collectively agreed with patients and service users as being the most important ways of understanding the quality of the service being provided. These measures would be displayed transparently so that all staff, service users, and patients and families or carers can access them and understand how the service is performing. The data would be shown as time series analysis, to provide a visual display of whether the service is improving over time. The data should be available as close to real-time as possible, ideally on a daily or weekly basis. The data should prompt discussion and action, with the team reviewing the data regularly, identifying any signals that suggest something unusual in the data, and taking action as necessary.
The main tools used for this purpose are the run chart and the Shewhart (or control) chart. The run chart ( fig 1 ) is a graphical display of data in time order, with a median value, and uses probability-based rules to help identify whether the variation seen is random or non-random. 2 The Shewhart (control) chart ( fig 2 ) also displays data in time order, but with a mean as the centre line instead of a median, and upper and lower control limits (UCL and LCL) defining the boundaries within which you would predict the data to be. 6 Shewhart charts use the terms “common cause variation” and “special cause variation,” with a different set of rules to identify special causes.

A typical run chart
- Download figure
- Open in new tab
- Download powerpoint

A typical Shewhart (or control) chart
Is it just about numbers?
We need to incorporate both qualitative and quantitative data to help us learn about how the system is performing and to see if we improve over time. Quantitative data express quantity, amount, or range and can be measured numerically—such as waiting times, mortality, haemoglobin level, cash flow. Quantitative data are often visualised over time as time series analyses (run charts or control charts) to see whether we are improving.
However, we should also be capturing, analysing, and learning from qualitative data throughout our improvement work. Qualitative data are virtually any type of information that can be observed and recorded that is not numerical in nature. Qualitative data are particularly useful in helping us to gain deeper insight into an issue, and to understand meaning, opinion, and feelings. This is vital in supporting us to develop theories about what to focus on and what might make a difference. 7 Examples of qualitative data include waiting room observation, feedback about experience of care, free-text responses to a survey.
Using qualitative data for improvement
One key point in an improvement journey when qualitative data are critical is at the start, when trying to identify “What matters most?” and what the team’s biggest opportunity for improvement is. The other key time to use qualitative data is during “Plan, Do, Study, Act” (PDSA) cycles. Most PDSA cycles, when done well, rely on qualitative data as well as quantitative data to help learn about how the test fared compared with our original theory and prediction.
Table 1 shows four different ways to collect qualitative data, with advantages and disadvantages of each, and how we might use them within our improvement work.
Different ways to collect qualitative data for improvement
- View inline
Tips to overcome common challenges in using data for improvement?
One of the key challenges faced by healthcare teams across the globe is being able to access data that is routinely collected, in order to use it for improvement. Large volumes of data are collected in healthcare, but often little is available to staff or service users in a timescale or in a form that allows it to be useful for improvement. One way to work around this is to have a simple form of measurement on the unit, clinic, or ward that the team own and update. This could be in the form of a safety cross 8 or tally chart. A safety cross ( fig 3 ) is a simple visual monthly calendar on the wall which allows teams to identify when a safety event (such as a fall) occurred on the ward. The team simply colours in each day green when no fall occurred, or colours in red the days when a fall occurred. It allows the team to own the data related to a safety event that they care about and easily see how many events are occurring over a month. Being able to see such data transparently on a ward allows teams to update data in real time and be able to respond to it effectively.

Example of a safety cross in use
A common challenge in using qualitative data is being able to analyse large quantities of written word. There are formal approaches to qualitative data analyses, but most healthcare staff are not trained in these methods. Key tips in avoiding this difficulty are ( a ) to be intentional with your search and sampling strategy so that you collect only the minimum amount of data that is likely to be useful for learning and ( b ) to use simple ways to read and theme the data in order to extract useful information to guide your improvement work. 9 If you want to try this, see if you can find someone in your organisation with qualitative data analysis skills, such as clinical psychologists or the patient experience or informatics teams.
Education into practice
What are the key measures for the service that you work in?
Are these measures available, transparently displayed, and viewed over time?
What qualitative data do you use in helping guide your improvement efforts?
How patients were involved in the creation of this article
Service users are deeply involved in all quality improvement work at East London NHS Foundation Trust, including within the training programmes we deliver. Shared learning over many years has contributed to our understanding of how best to use all types of data to support improvement. No patients have had input specifically into this article.
This article is part of a series commissioned by The BMJ based on ideas generated by a joint editorial group with members from the Health Foundation and The BMJ , including a patient/carer. The BMJ retained full editorial control over external peer review, editing, and publication. Open access fees and The BMJ ’s quality improvement editor post are funded by the Health Foundation.
Competing interests: I have read and understood the BMJ Group policy on declaration of interests and have no relevant interests to declare.
Provenance and peer review: Commissioned; externally peer reviewed.
This is an Open Access article distributed in accordance with the Creative Commons Attribution Non Commercial (CC BY-NC 4.0) license, which permits others to distribute, remix, adapt, build upon this work non-commercially, and license their derivative works on different terms, provided the original work is properly cited and the use is non-commercial. See: http://creativecommons.org/licenses/by-nc/4.0/ .
- ↵ Cambridge University Press. Cambridge online dictionary , 2008. https://dictionary.cambridge.org/ .
- Provost LP ,
- Braithwaite J
- Neuhauser D
- Donabedian A
- Mohammed MA
- Davidoff F ,
- Dixon-Woods M ,
- Leviton L ,
- ↵ Flynn M. Quality & Safety—The safety cross system: simple and effective. https://www.inmo.ie/MagazineArticle/PrintArticle/11155 .
Quantitative Methods
The Quantitative Methods (QM) field of study provides students with the necessary quantitative and analytical skills to approach and solve problems in public health and clinical research and practice. This field is designed for mid-career health professionals, research scientists, and MD/MPH specific dual/joint-degree students.
Through a competency-based curriculum, health professionals in the MPH-45 receive the analytical and statistical knowledge and skills required for successful public health practice and research. In addition to providing broad perspectives on general aspects of public health, the QM field of study provides an excellent foundation for those interested in pursuing academic careers in the health sciences.
Degree programs
The Master of Public Health 45-credit degree provides established professionals with the specialized skills and powerful global network needed to progress their careers in public health.
- Abbreviation: MPH-45 QM
- Degree format: On campus
- Time commitment: Full-time or part-time
- Average program length: One year full-time; two years part-time
Student interests
The Quantitative Methods (QM) field of study is uniquely designed for mid-career health professionals, research scientists, and MD/MPH students. Students who choose QM are passionate about clinical and population-based health research, and dedicated to learning the tools necessary for implementation.
Career outcomes
Graduates of the Master of Public Health (MPH) 45-credit program with the Quantitative Methods (QM) field of study are prepared to fulfill professional positions in clinical and population-based health research in government, health care institutions, and private industry.
Quantitative Research in Healthcare Simulation: An Introduction and Discussion of Common Pitfalls
- First Online: 14 November 2019
Cite this chapter
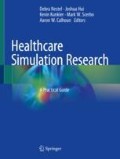
- Aaron W. Calhoun 6 ,
- Joshua Hui 7 &
- Mark W. Scerbo 8
2391 Accesses
In contrast to qualitative research, quantitative research focuses primarily on the testing of hypotheses using variables that are measured numerically and analyzed using statistical procedures. If appropriately designed, quantitative approaches provide the ability to establish causal relationships between variables. Hypothesis testing is a critical component of quantitative methods, and requires appropriately framed research questions, knowledge of the appropriate literature, and guidance from relevant theoretical frameworks. Within the field of simulation, two broad categories of quantitative research exist: studies that investigate the use of simulation as a variable and studies using simulation to investigate other questions and issues. In this chapter we review common study designs and introduce some key concepts pertaining to measurement and statistical analysis. We conclude the chapter with a survey of common errors in quantitative study design and implementation.
This is a preview of subscription content, log in via an institution to check access.
Access this chapter
- Available as PDF
- Read on any device
- Instant download
- Own it forever
- Available as EPUB and PDF
- Compact, lightweight edition
- Dispatched in 3 to 5 business days
- Free shipping worldwide - see info
Tax calculation will be finalised at checkout
Purchases are for personal use only
Institutional subscriptions
Babbie ER. The practice of social research. 12th ed. Belmont: Wadsworth Cengage; 2010.
Google Scholar
Mujis D. Doing quantitative research in education with SPSS. 2nd ed. London: SAGE Publications; 2010.
Vogt WP. Dictionary of statistics and methodology. 2nd ed. London: SAGE Publications; 1999.
Kessler D, Pusic M, Chang TP, Fein DM, Grossman D, Mehta R, et al. Impact of just-in-time and just-in-place simulation on intern success with infant lumbar puncture. Pediatrics. 2015;135(5):e1237–46.
Article Google Scholar
Calhoun AW, Sutton ERH, Barbee AP, McClure B, Bohnert C, Forest R, et al. Compassionate options for pediatric EMS (COPE): addressing communication skills. Prehosp Emerg Care. 2017;21(3):334–43.
Sullivan GM, Sargeant J. Qualities of qualitative research: part I. J Grad Med Educ. 2011;3(4):449–52.
Crandall SJ, Caelleigh AS, Steinecke A. Reference to the literature and documentation. Acad Med. 2001;76(9):925–7.
Picho K, Artino AR Jr. 7 deadly sins in educational research. J Grad Med Educ. 2016;8(4):483–7.
Cook DA, Beckman TJ, Bordage G. Quality of reporting of experimental studies in medical education: a systematic review. Med Educ. 2007;41(8):737–45.
Bernhard HR. Social research methods, qualitative and quantitative approaches. 2nd ed. London: SAGE Publications; 2013.
Evans BC, Coon DW, Ume E. Use of theoretical frameworks as a pragmatic guide for mixed methods studies: a methodological necessity? J Mix Methods Res. 2011;5(4):276–92.
Morgan DL. Paradigms lost and pragmatism regained: methodological implications of combining qualitative and quantitative methods. J Mixed Methods Res. 2007;1(1):48–76.
Tavakol M, Sandars J. Quantitative and qualitative methods in medical education research: AMEE Guide No 90: part II. Med Teach. 2014;36(10):838–48.
Ingham-Broomfield RA. Nurse’s guide to quantitative research. Aust J Adv Nurs. 2014;32(2):32–8.
Neuman WL. Social research methods: qualitative and quantitative approaches. 7th ed. Edinburgh Gate: Pearson Education Limited; 2014.
Lopreiato JO, editor. Healthcare simulation dictionary. Rockville: Agency for Healthcare Research and Quality; 2016.
Issenberg SB, McGaghie WC, Petrusa ER, Lee Gordon D, Scalese RJ. Features and uses of high-fidelity medical simulations that lead to effective learning: a BEME systematic review. Med Teach. 2005;27(1):10–28.
Calhoun AW, Bhanji F, Sherbino J, Hatala R. Simulation for high-stakes assessment in pediatric emergency medicine. Clin Pediatr Emerg Med. 2016;17(3):212–23.
Calhoun AW, Donoghue A, Adler M. Assessment in pediatric simulation. In: Grant V, Cheng A, editors. Comprehensive healthcare simulation: pediatrics. Cham: Springer International; 2016. p. 77–94.
Chapter Google Scholar
Cook DA, Brydges R, Ginsburg S, Hatala R. A contemporary approach to validity arguments: a practical guide to Kane’s framework. Med Educ. 2015;49(6):560–75.
Downing SM. Validity: on meaningful interpretation of assessment data. Med Educ. 2003;37(9):830–7.
Messick S. Meaning and values in test validation: the science and ethics of assessment. Educ Res. 1989;18(2):5–11.
Vetter TR. Fundamentals of research data and variables: the devil is in the details. Anesth Analg. 2017;125:1375–80.
Sullivan GM, Feinn R. Using effect size-or why the P value is not enough. J Grad Med Educ. 2012;4(3):279–82.
Sullivan GM. Is there a role for spin doctors in Med Ed research? J Grad Med Educ. 2014;6(3):405–7.
Feise RJ. Do multiple outcomes measures require P-value adjustment? BMC Med Res Methodol. 2002;2(8):1–4.
Noble WS. How does multiple testing correction work? Nat Biotechnol. 2009;27(12):1135–7.
Article CAS Google Scholar
Quertemont E. How to statistically show the absence of an effect. Psychol Belg. 2011;51(2):109–27.
Download references
Author information
Authors and affiliations.
Department of Pediatrics, University of Louisville School of Medicine, Louisville, KY, USA
Aaron W. Calhoun
Emergency Medicine, Kaiser Permanente, Los Angeles Medical Center, Los Angeles, CA, USA
Department of Psychology, Old Dominion University, Norfolk, VA, USA
Mark W. Scerbo
You can also search for this author in PubMed Google Scholar
Corresponding author
Correspondence to Aaron W. Calhoun .
Editor information
Editors and affiliations.
Monash Institute for Health and Clinical Education, Monash University, Clayton, VIC, Australia
Debra Nestel
Department of Surgery, University of Maryland, Baltimore, Baltimore, MD, USA
Kevin Kunkler
Rights and permissions
Reprints and permissions
Copyright information
© 2019 Springer Nature Switzerland AG
About this chapter
Calhoun, A.W., Hui, J., Scerbo, M.W. (2019). Quantitative Research in Healthcare Simulation: An Introduction and Discussion of Common Pitfalls. In: Nestel, D., Hui, J., Kunkler, K., Scerbo, M., Calhoun, A. (eds) Healthcare Simulation Research. Springer, Cham. https://doi.org/10.1007/978-3-030-26837-4_21
Download citation
DOI : https://doi.org/10.1007/978-3-030-26837-4_21
Published : 14 November 2019
Publisher Name : Springer, Cham
Print ISBN : 978-3-030-26836-7
Online ISBN : 978-3-030-26837-4
eBook Packages : Biomedical and Life Sciences Biomedical and Life Sciences (R0)
Share this chapter
Anyone you share the following link with will be able to read this content:
Sorry, a shareable link is not currently available for this article.
Provided by the Springer Nature SharedIt content-sharing initiative
- Publish with us
Policies and ethics
- Find a journal
- Track your research

An official website of the United States government
Here's how you know
The .gov means it’s official. Federal government websites often end in .gov or .mil. Before sharing sensitive information, make sure you’re on a federal government site.
The site is secure. The https:// ensures that you are connecting to the official website and that any information you provide is encrypted and transmitted securely.
Common Data Types in Public Health Research
Quantitative data.
- Quantitative data is measurable, often used for comparisons, and involves counting of people, behaviors, conditions, or other discrete events (Wang, 2013).
- Quantitative data uses numbers to determine the what, who, when, and where of health-related events (Wang, 2013).
- Examples of quantitative data include: age, weight, temperature, or the number of people suffering from diabetes.
Qualitative Data
- Qualitative data is a broad category of data that can include almost any non-numerical data.
- Qualitative data uses words to describe a particular health-related event (Romano).
- This data can be observed, but not measured.
- Involves observing people in selected places and listening to discover how they feel and why they might feel that way (Wang, 2013).
- Examples of qualitative data include: male/female, smoker/non-smoker, or questionnaire response (agree, disagree, neutral).
- Measuring organizational change.
- Measures of clinical leadership in implementing evidence-based guidelines.
- Patient perceptions of quality of care.
Data Sources
Primary data sources.
- Primary data analysis in which the same individual or team of researchers designs, collects, and analyzes the data, for the purpose of answering a research question (Koziol & Arthur, nd).
Advantages to Using Primary Data
- You collect exactly the data elements that you need to answer your research question (Romano).
- You can test an intervention, such as an experimental drug or an educational program, in the purest way (a double-blind randomized controlled trial (Romano).
- You control the data collection process, so you can ensure data quality, minimize the number of missing values, and assess the reliability of your instruments (Romano).
Secondary Data Sources
- Existing data collected for another purposes, that you use to answer your research question (Romano).
Advantages of Working with Secondary Data
- Large samples
- Can provide population estimates : for example state data can be combined across states to get national estimates (Shaheen, Pan, & Mukherjee).
- Less expensive to collect than primary data (Romano)
- It takes less time to collect secondary data (Romano).
- You may not need to worry about informed consent, human subjects restriction (Romano).
Issues in Using Secondary Data
- Study design and data collection already completed (Koziol & Arthur, nd).
- Data may not facilitate particular research question o Information regarding study design and data collection procedures may be scarce.
- Data may potentially lack depth (the greater the breadth the harder it is to measure any one construct in depth) (Koziol & Arthur, nd).
- Certain fields or departments (e.g., experimental programs) may place less value on secondary data analysis (Koziol & Arthur, nd).
- Often requires special techniques to analyze statistically the data.
Log in using your username and password
- Search More Search for this keyword Advanced search
- Latest content
- Current issue
- Write for Us
- BMJ Journals More You are viewing from: Google Indexer
You are here
- Volume 21, Issue 4
- How to appraise quantitative research
- Article Text
- Article info
- Citation Tools
- Rapid Responses
- Article metrics
This article has a correction. Please see:
- Correction: How to appraise quantitative research - April 01, 2019

- Xabi Cathala 1 ,
- Calvin Moorley 2
- 1 Institute of Vocational Learning , School of Health and Social Care, London South Bank University , London , UK
- 2 Nursing Research and Diversity in Care , School of Health and Social Care, London South Bank University , London , UK
- Correspondence to Mr Xabi Cathala, Institute of Vocational Learning, School of Health and Social Care, London South Bank University London UK ; cathalax{at}lsbu.ac.uk and Dr Calvin Moorley, Nursing Research and Diversity in Care, School of Health and Social Care, London South Bank University, London SE1 0AA, UK; Moorleyc{at}lsbu.ac.uk
https://doi.org/10.1136/eb-2018-102996
Statistics from Altmetric.com
Request permissions.
If you wish to reuse any or all of this article please use the link below which will take you to the Copyright Clearance Center’s RightsLink service. You will be able to get a quick price and instant permission to reuse the content in many different ways.
Introduction
Some nurses feel that they lack the necessary skills to read a research paper and to then decide if they should implement the findings into their practice. This is particularly the case when considering the results of quantitative research, which often contains the results of statistical testing. However, nurses have a professional responsibility to critique research to improve their practice, care and patient safety. 1 This article provides a step by step guide on how to critically appraise a quantitative paper.
Title, keywords and the authors
The authors’ names may not mean much, but knowing the following will be helpful:
Their position, for example, academic, researcher or healthcare practitioner.
Their qualification, both professional, for example, a nurse or physiotherapist and academic (eg, degree, masters, doctorate).
This can indicate how the research has been conducted and the authors’ competence on the subject. Basically, do you want to read a paper on quantum physics written by a plumber?
The abstract is a resume of the article and should contain:
Introduction.
Research question/hypothesis.
Methods including sample design, tests used and the statistical analysis (of course! Remember we love numbers).
Main findings.
Conclusion.
The subheadings in the abstract will vary depending on the journal. An abstract should not usually be more than 300 words but this varies depending on specific journal requirements. If the above information is contained in the abstract, it can give you an idea about whether the study is relevant to your area of practice. However, before deciding if the results of a research paper are relevant to your practice, it is important to review the overall quality of the article. This can only be done by reading and critically appraising the entire article.
The introduction
Example: the effect of paracetamol on levels of pain.
My hypothesis is that A has an effect on B, for example, paracetamol has an effect on levels of pain.
My null hypothesis is that A has no effect on B, for example, paracetamol has no effect on pain.
My study will test the null hypothesis and if the null hypothesis is validated then the hypothesis is false (A has no effect on B). This means paracetamol has no effect on the level of pain. If the null hypothesis is rejected then the hypothesis is true (A has an effect on B). This means that paracetamol has an effect on the level of pain.
Background/literature review
The literature review should include reference to recent and relevant research in the area. It should summarise what is already known about the topic and why the research study is needed and state what the study will contribute to new knowledge. 5 The literature review should be up to date, usually 5–8 years, but it will depend on the topic and sometimes it is acceptable to include older (seminal) studies.
Methodology
In quantitative studies, the data analysis varies between studies depending on the type of design used. For example, descriptive, correlative or experimental studies all vary. A descriptive study will describe the pattern of a topic related to one or more variable. 6 A correlational study examines the link (correlation) between two variables 7 and focuses on how a variable will react to a change of another variable. In experimental studies, the researchers manipulate variables looking at outcomes 8 and the sample is commonly assigned into different groups (known as randomisation) to determine the effect (causal) of a condition (independent variable) on a certain outcome. This is a common method used in clinical trials.
There should be sufficient detail provided in the methods section for you to replicate the study (should you want to). To enable you to do this, the following sections are normally included:
Overview and rationale for the methodology.
Participants or sample.
Data collection tools.
Methods of data analysis.
Ethical issues.
Data collection should be clearly explained and the article should discuss how this process was undertaken. Data collection should be systematic, objective, precise, repeatable, valid and reliable. Any tool (eg, a questionnaire) used for data collection should have been piloted (or pretested and/or adjusted) to ensure the quality, validity and reliability of the tool. 9 The participants (the sample) and any randomisation technique used should be identified. The sample size is central in quantitative research, as the findings should be able to be generalised for the wider population. 10 The data analysis can be done manually or more complex analyses performed using computer software sometimes with advice of a statistician. From this analysis, results like mode, mean, median, p value, CI and so on are always presented in a numerical format.
The author(s) should present the results clearly. These may be presented in graphs, charts or tables alongside some text. You should perform your own critique of the data analysis process; just because a paper has been published, it does not mean it is perfect. Your findings may be different from the author’s. Through critical analysis the reader may find an error in the study process that authors have not seen or highlighted. These errors can change the study result or change a study you thought was strong to weak. To help you critique a quantitative research paper, some guidance on understanding statistical terminology is provided in table 1 .
- View inline
Some basic guidance for understanding statistics
Quantitative studies examine the relationship between variables, and the p value illustrates this objectively. 11 If the p value is less than 0.05, the null hypothesis is rejected and the hypothesis is accepted and the study will say there is a significant difference. If the p value is more than 0.05, the null hypothesis is accepted then the hypothesis is rejected. The study will say there is no significant difference. As a general rule, a p value of less than 0.05 means, the hypothesis is accepted and if it is more than 0.05 the hypothesis is rejected.
The CI is a number between 0 and 1 or is written as a per cent, demonstrating the level of confidence the reader can have in the result. 12 The CI is calculated by subtracting the p value to 1 (1–p). If there is a p value of 0.05, the CI will be 1–0.05=0.95=95%. A CI over 95% means, we can be confident the result is statistically significant. A CI below 95% means, the result is not statistically significant. The p values and CI highlight the confidence and robustness of a result.
Discussion, recommendations and conclusion
The final section of the paper is where the authors discuss their results and link them to other literature in the area (some of which may have been included in the literature review at the start of the paper). This reminds the reader of what is already known, what the study has found and what new information it adds. The discussion should demonstrate how the authors interpreted their results and how they contribute to new knowledge in the area. Implications for practice and future research should also be highlighted in this section of the paper.
A few other areas you may find helpful are:
Limitations of the study.
Conflicts of interest.
Table 2 provides a useful tool to help you apply the learning in this paper to the critiquing of quantitative research papers.
Quantitative paper appraisal checklist
- 1. ↵ Nursing and Midwifery Council , 2015 . The code: standard of conduct, performance and ethics for nurses and midwives https://www.nmc.org.uk/globalassets/sitedocuments/nmc-publications/nmc-code.pdf ( accessed 21.8.18 ).
- Gerrish K ,
- Moorley C ,
- Tunariu A , et al
- Shorten A ,
Competing interests None declared.
Patient consent Not required.
Provenance and peer review Commissioned; internally peer reviewed.
Correction notice This article has been updated since its original publication to update p values from 0.5 to 0.05 throughout.
Linked Articles
- Miscellaneous Correction: How to appraise quantitative research BMJ Publishing Group Ltd and RCN Publishing Company Ltd Evidence-Based Nursing 2019; 22 62-62 Published Online First: 31 Jan 2019. doi: 10.1136/eb-2018-102996corr1
Read the full text or download the PDF:
How Has Quantitative Analysis Changed Health Care?
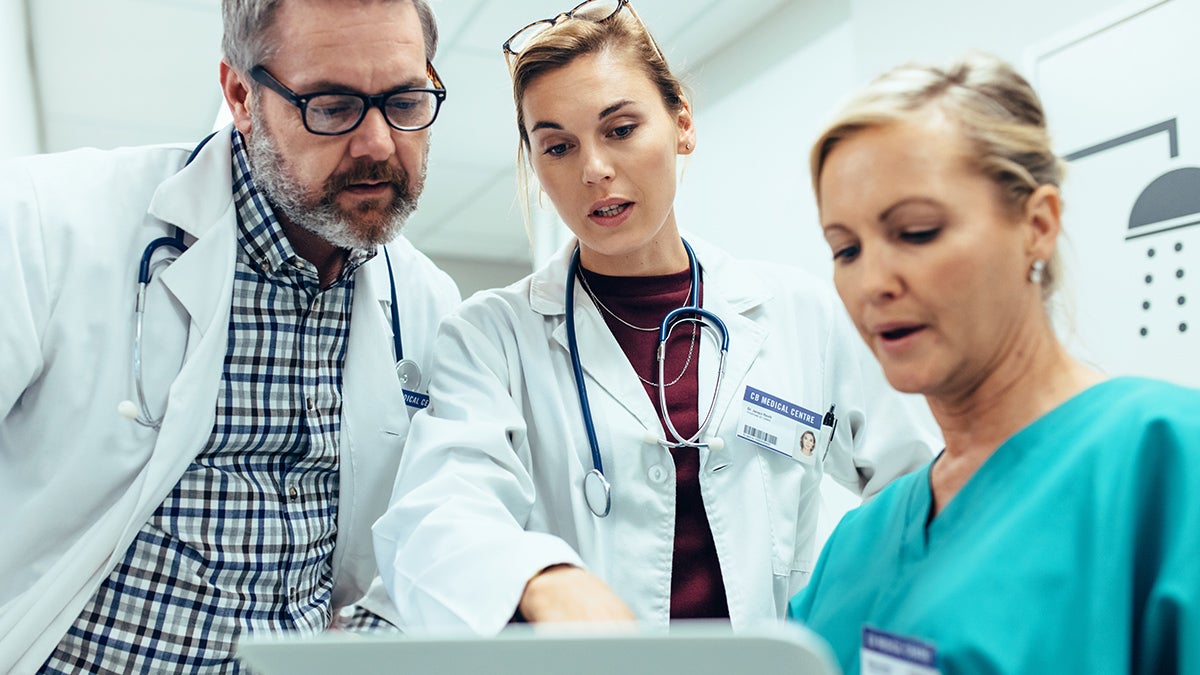
Fill out the form below and we'll email you more information about UCF's online healthcare programs.
- Name * First Last
- Degree * Autism Spectrum Disorders Executive Master of Health Administration, EMHA Forensic Science, MS Fundraising Gender Studies Health Informatics and Information Management, BS Health Services Administration, BS Healthcare Simulation Healthcare Systems Engineering Certificate Healthcare Systems Engineering, MS Integrative General Studies, BGS Interdisciplinary Studies – Diversity Studies Leadership Track, BA Interdisciplinary Studies, BA/BS Master of Public Administration, MPA Master of Science in Healthcare Informatics Master of Social Work Online Nonprofit Management Nonprofit Management, MNM Nursing Education Nursing Practice, DNP, Advanced Track Nursing Practice, DNP, Executive Track Nursing, BS Nursing, BSN to PhD Nursing, MSN Nursing, PhD Project Engineering Psychology, BS Public Administration Research Administration Certificate Research Administration, MRA Systems Engineering
Privacy Notice
In health care, groundbreaking solutions often follow a new capacity for measurement and pattern finding. For example, developing the ability to measure blood glucose levels led to better treatments of diabetes. Florence Nightingale changed nursing forever with her careful measurements of hospital care outcomes. Today, we’re in the midst of an even more significant change in the health care industry: Troves of data are mixing with technologies newly powerful enough to adequately analyze them. As a result, the unprecedented pattern-finding power of quantitative analysis is remaking the health care industry.
Quantitative analysis refers to the process of using complex mathematical or statistical modeling to make sense of data and potentially to predict behavior. Though quantitative analysis is well-established in the fields of economics and finance, cutting-edge quantitative analysis has only recently become possible in health care. Some experts insist that the unfurling of QA in health care will radically change the industry—and how all of us maintain our health and are treated when we’re sick.
It will be up to professionals in the transforming field of health care information technology to make the most of the opportunities borne from these expanding data sets. But what are a few of the specific ways in which quantitative analysis could improve health care?
Stronger Research
Dr. Richard Biehl, former education coordinator of the online Master of Science in Health Care Systems Engineering program at the University of Central Florida, explains that QA stands to change the face of research in the health care field, because, suddenly, it may become very easy to test the strength of correlations between thousands of variables with the touch of a button. In other words, no researcher will need to make the concerted decision to build a study around a question such as “Is this particular allele driving lipid metabolism?” Powerful analytical tools driven by QA will be able to point researchers in the direction of promising correlations between variables they might not have realized were linked.
“We used to get the data to support our research; now we’re getting the data to suggest our research,” Dr. Biehl says. “That’s very, very different.”
The upshot? The field of health care research will become a much more targeted and efficient space—and more likely to regularly uncover lifesaving treatments.
Saving Time, Money, and Lives Through Efficiency and Safety
New QA tools will decrease wait times and call patients into doctors’ offices only when a visit is necessary. As more and more data is crunched to determine, for example, what bodily indicators tend to precede a heart attack, the provider (who will be monitoring the patient’s vital signs via wearable devices) will be able to alert the patient when his or her indicators are trending in a worrisome direction. That means paying for fewer checkup appointments when one is healthy.
Even more importantly, QA tools will allow health care professionals to decrease the impact of human error in prescribing medication and invasive health care procedures. More data can save lives by uncovering complicated patterns (in physiology, DNA, diet, or lifestyle) that help explain why certain medications can prove dangerous for some.
Making Sure Supply Meets Demand
Certain geographic locations and clinical specialties are already facing doctor shortages as mergers and acquisitions reform the health care landscape and financial difficulties force providers to close their doors. But by filling in the picture of oversupply and undersupply around the country, QA can help providers plug holes where they need to.
“Making sure there’s an adequate supply of health care in the right places, in the right specialties, and at the right times, is a health care systems engineering challenge,” Dr. Biehl says.
Amid all the exciting possibilities, QA’s application to health care is still newer than other industries and faces challenges. This type of analysis requires that variables be recorded as numerical data so that they can be analyzed with statistical tools—a format that health care has struggled to conform with, as much of its outcome data is recorded as “positive” or “negative.”
Additionally, QA statistical tools work best when fed with huge amounts of data, as more data makes for clearer patterns and stronger conclusions. Big data analysts at corporations such as Amazon and Google—where every click is tracked and measured—have been collecting unprecedented amounts of data to feed into complex statistical tools for years. Health care has yet to catch up, but it likely will once more wearable technology options, such as expanded versions of Fitbit devices and trackers embedded in up-and-coming “internet of things” appliances, track more and more users’ every move, bite, and night of sleep.
“Once we start collecting all this personal, wearable data from people, health care will start to look more like the Googles and Amazons of the world,” Dr. Biehl says. “We’ll have hundreds of millions of people collecting tens of thousands of data points a day. We’ll finally have big data; we’re heading in that direction.”
Additional Resources
https://www.worldcat.org/wcpa/servlet/DCARead?standardNo=0787971642&standardNoType=1&excerpt=true https://bizfluent.com/info-8168865-benefits-quantitative-research-health-care.html https://www.ruralhealthinfo.org/community-health/rural-toolkit/4/quantitative-qualitative https://www.dotmed.com/news/story/37262
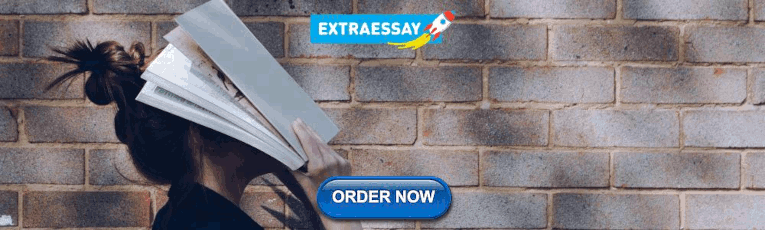
UCF’s Online Healthcare Degrees
- Autism Spectrum Disorders
- Executive Master of Health Administration, EMHA
- Forensic Science, MS
- Fundraising
- Gender Studies
- Health Informatics and Information Management, BS
- Health Services Administration, BS
- Healthcare Simulation
- Healthcare Systems Engineering Certificate
- Healthcare Systems Engineering, MS
- Integrative General Studies, BGS
- Interdisciplinary Studies – Diversity Studies Leadership Track, BA
- Interdisciplinary Studies, BA/BS
- Master of Public Administration, MPA
- Master of Science in Healthcare Informatics
- Master of Social Work Online
- Nonprofit Management
- Nonprofit Management, MNM
- Nursing Education
- Nursing Practice, DNP, Advanced Track
- Nursing Practice, DNP, Executive Track
- Nursing, BS
- Nursing, BSN to PhD
- Nursing, MSN
- Nursing, PhD
- Project Engineering
- Psychology, BS
- Public Administration
- Research Administration Certificate
- Research Administration, MRA
- Systems Engineering
You May Also Enjoy
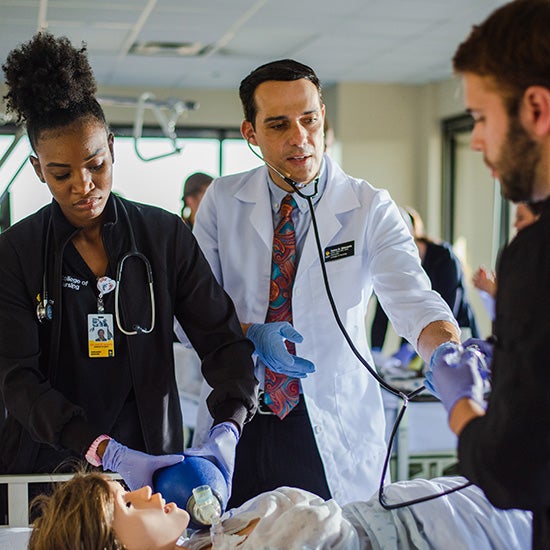
- Get the Job
- Resumes and CVs
- Applications
- Cover Letters
- Professional References
Professional Licenses and Exams
- Get a Promotion
- Negotiation
- Professional Ethics
- Professionalism
- Dealing with Coworkers
- Dealing with Bosses
Communication Skills
Managing the office, disabilities, harassment and discrimination, unemployment.
- Career Paths
- Compare Careers
- Switching Careers
- Training and Certifications
- Start a Company
- Internships and Apprenticeships
- Entry Level Jobs
- College Degrees
Growth Trends for Related Jobs
What are the benefits of quantitative research in health care.

Most scientific research will follow one of two approaches - it can be either qualitative or quantitative. Health care research is often based on quantitative methods in which, by definition, information is quantifiable. That is, the variables used in research are measured and recorded as numerical data that can be analyzed by means of statistical tools. The use of quantitative research in health care has several benefits.
The main strength of quantitative methods is in their usefulness in producing factual and reliable outcome data. After the effects of a given drug or treatment have been tested on a sample population, the statistic record of the observed outcomes will provide objective results generalizable to larger populations. The statistical methods associated with quantitative research are well suited for figuring out ways to maximize dependent variables on the basis of independents, which translates into a capability for identifying and applying the interventions that can maximize the quality and quantity of life for a patient.
Reductionism
Quantitative researchers are often accused of reductionism; they take a complex phenomena and reduce them to a few essential numbers, loosing every nuance in the process. However, this reductionism is a two-edged sword with a very significant benefit. By reducing health cases to their essentials, a very large number of them can be taken into consideration for any given study. Large, statistically representative samples that would be unfeasible in qualitative studies can be easily analyzed using quantitative methods.
Evidence-Based Health Research
Given the benefits of quantitative methods in health care, evidence-based medicine seeks to use scientific methods to determine which drugs and procedures are best for treating diseases. At the core of evidence-based practice is the systematic and predominantly quantitative review of randomized controlled trials. Because quantitative researchers tend to use similar statistical methods, experiments and trials performed in different institutions and at different times and places can be aggregated together in large meta-analysis. Thus, quantitative research on health care can build on previous studies, accumulating a body of evidence regarding the effectiveness of different treatments.
Mixed Methods
Evidence-based medicine, and quantitative methods overall, are sometimes accused of leading to "cookbook" medicine. Some of the phenomena of interest to health researchers are of a qualitative nature and, almost by definition, inaccessible to quantitative tools -- for example, the lived experiences of the patient, his social interactions or his perspective of the doctor-patient interaction. However, judicious researchers can find a combination of qualitative and quantitative approaches so the strengths of each method reinforce those of the other. For instance, qualitative methods can be used for the creative generation of hypotheses or research questions, adding a human touch to the rigorous quantitative approach.
Related Articles
Pros & cons of descriptive research →.

Types of Medical Scientists →
What is a hypothesis →.

The Duties of a Biochemist →

Pros & Cons of Evidence-Based Practice →
Career Objectives of Biochemists →

- "Research Design: Qualitative, Quantitative, and Mixed Methods Approaches" ; John W. Creswell; 2009
- Health Promotion Practice; "Appraising Quantitative Research in Health Education: Guidelines for Public Health Educators"; Leonard Jack Jr et al; 2010
- British Medical Journal; "Evidence Based Medicine: What it is and What it isn't"; David L Sackett et al; 1996
Alan Valdez started his career reviewing video games for an obscure California retailer in 2003 and has been writing weekly articles on science and technology for Grupo Reforma since 2006. He got his Bachelor of Science in engineering from Monterrey Tech in 2003 and moved to the U.K., where he is currently doing research on competitive intelligence applied to the diffusion of innovations.
bymuratdeniz/E+/GettyImages
- Job Descriptions
- Law Enforcement Job Descriptions
- Administrative Job Descriptions
- Healthcare Job Descriptions
- Sales Job Descriptions
- Fashion Job Descriptions
- Education Job Descriptions
- Salary Insights
- Journalism Salaries
- Healthcare Salaries
- Military Salaries
- Engineering Salaries
- Teaching Salaries
- Accessibility
- Privacy Notice
- Cookie Notice
- Copyright Policy
- Contact Us
- Find a Job
- Manage Preferences
- California Notice of Collection
- Terms of Use
- Open access
- Published: 11 April 2024
The role of champions in the implementation of technology in healthcare services: a systematic mixed studies review
- Sissel Pettersen 1 ,
- Hilde Eide 2 &
- Anita Berg 1
BMC Health Services Research volume 24 , Article number: 456 ( 2024 ) Cite this article
239 Accesses
Metrics details
Champions play a critical role in implementing technology within healthcare services. While prior studies have explored the presence and characteristics of champions, this review delves into the experiences of healthcare personnel holding champion roles, as well as the experiences of healthcare personnel interacting with them. By synthesizing existing knowledge, this review aims to inform decisions regarding the inclusion of champions as a strategy in technology implementation and guide healthcare personnel in these roles.
A systematic mixed studies review, covering qualitative, quantitative, or mixed designs, was conducted from September 2022 to March 2023. The search spanned Medline, Embase, CINAHL, and Scopus, focusing on studies published from 2012 onwards. The review centered on health personnel serving as champions in technology implementation within healthcare services. Quality assessments utilized the Mixed Methods Appraisal Tool (MMAT).
From 1629 screened studies, 23 were included. The champion role was often examined within the broader context of technology implementation. Limited studies explicitly explored experiences related to the champion role from both champions’ and health personnel’s perspectives. Champions emerged as promoters of technology, supporting its adoption. Success factors included anchoring and selection processes, champions’ expertise, and effective role performance.
The specific tasks and responsibilities assigned to champions differed across reviewed studies, highlighting that the role of champion is a broad one, dependent on the technology being implemented and the site implementing it. Findings indicated a correlation between champion experiences and organizational characteristics. The role’s firm anchoring within the organization is crucial. Limited evidence suggests that volunteering, hiring newly graduated health personnel, and having multiple champions can facilitate technology implementation. Existing studies predominantly focused on client health records and hospitals, emphasizing the need for broader research across healthcare services.
Conclusions
With a clear mandate, dedicated time, and proper training, health personnel in champion roles can significantly contribute professional, technological, and personal competencies to facilitate technology adoption within healthcare services. The review finds that the concept of champions is a broad one and finds varied definitions of the champion role concept. This underscores the importance of describing organizational characteristics, and highlights areas for future research to enhance technology implementation strategies in different healthcare settings with support of a champion.
Peer Review reports
Digital health technologies play a transformative role in healthcare service systems [ 1 , 2 ]. The utilization of technology and digitalization is essential for ensuring patient safety, delivering high quality, cost-effective, and sustainable healthcare services [ 3 , 4 ]. The implementation of technology in healthcare services is a complex process that demands systematic changes in roles, workflows, and service provision [ 5 , 6 ].
The successful implementation of new technologies in healthcare services relies on the adaptability of health professionals [ 7 , 8 , 9 ]. Champions have been identified as a key factor in the successful implementation of technology among health personnel [ 10 , 11 , 12 ]. However, they have rarely been studied as an independent strategy; instead, they are often part of a broader array of strategies in implementation studies (e.g., Hudson [ 13 ], Gullslett and Bergmo [ 14 ]). Prior research has frequently focused on determining the presence or absence of champions [ 10 , 12 , 15 ], as well as investigating the characteristics of individuals assuming the champion role (e.g., George et al. [ 16 ], Shea and Belden [ 17 ]).
Recent reviews on champions [ 18 , 19 , 20 ] have studied their effects on adherence to guidelines, implementation of innovations and facilitation of evidence-based practice. While these reviews suggest that having champions yields positive effects, they underscore the importance for studies that offer detailed insights into the champion’s role concerning specific types of interventions.
There is limited understanding of the practical role requirements and the actual experiences of health personnel in performing the champion role in the context of technology implementation within healthcare services. Further, this knowledge is needed to guide future research on the practical, professional, and relational prerequisites for health personnel in this role and for organizations to successfully employ champions as a strategy in technology implementation processes.
This review seeks to synthesize the existing empirical knowledge concerning the experiences of those in the champion role and the perspectives of health personnel involved in technology implementation processes. The aim is to contribute valuable insights that enhance our understanding of practical role requirements, the execution of the champion role, and best practices in this domain.
The term of champions varies [ 10 , 19 ] and there is a lack of explicit conceptualization of the term ‘champion’ in the implementation literature [ 12 , 18 ]. Various terms for individuals with similar roles also exist in the literature, such as implementation leader, opinion leader, facilitator, change agent, superuser and facilitator. For the purpose of this study, we have adopted the terminology utilized in the recent review by Rigby, Redley and Hutchinson [ 21 ] collectively referring to these roles as ‘champions’. This review aims to explore the experiences of health personnel in their role as champions and the experiences of health personnel interacting with them in the implementation of technology in the healthcare services.
Prior review studies on champions in healthcare services have employed various designs [ 10 , 18 , 19 , 20 ]. In this review, we utilized a comprehensive mixed studies search to identify relevant empirical studies [ 22 ]. The search was conducted utilizing the Preferred Reporting Items for Systematic and Meta-Analysis (PRISMA) guidelines, ensuring a transparent and comprehensive overview that can be replicated or updated by others [ 23 ]. The study protocol is registered in PROSPERO (ID CRD42022335750), providing a more comprehensive description of the methods [ 24 ]. A systematic mixed studies review, examining research using diverse study designs, is well-suited for synthesizing existing knowledge and identifying gaps by harnessing the strengths of both qualitative and quantitative methods [ 22 ]. Our search encompassed qualitative, quantitative, and mixed methods design to capture experiences with the role of champions in technology implementation.
Search strategy and study selection
Search strategy.
The first author, in collaboration with a librarian, developed the search strategy based on initial searches to identify appropriate terms and truncations that align with the eligibility criteria. The search was constructed utilizing a combination of MeSH terms and keywords related to technology, implementation, champion, and attitudes/experiences. Conducted in August/September 2022, the search encompassed four databases: Medline, Embase, CINAHL, and Scopus, with an updated search conducted in March 2023. The full search strategy for Medline is provided in Appendix 1 . The searches in Embase, CINAHL and Scopus employed the same strategy, with adopted terms and phrases to meet the requirements of each respective database.
Eligibility criteria
We included all empirical studies employing qualitative, quantitative, and mixed methods designs that detailed the experiences and/or attitudes of health personnel regarding the champions role in the implementation of technology in healthcare services. Articles in the English language published between 2012 and 2023 were considered. The selected studies involved technology implemented or adapted within healthcare services.
Conference abstract and review articles were excluded from consideration. Articles published prior 2012 were excluded as a result of the rapid development of technology, which could impact the experiences reported. Furthermore, articles involving surgical technology and pre-implementation studies were also excluded, as the focus was on capturing experiences and attitudes from the adoption and daily use of technology. The study also excluded articles that involved champions without clinical health care positions.
Study selection
A total of 1629 studies were identified and downloaded from the selected databases, with Covidence [ 25 ] utilized as a software platform for screening. After removing 624 duplicate records, all team members collaborated to calibrate the screening process utilizing the eligibility criteria on the initial 50 studies. Subsequently, the remaining abstracts were independently screened by two researchers, blinded to each other, to ensure adherence to the eligibility criteria. Studies were included if the title and abstract included the term champion or its synonyms, along with technology in healthcare services, implementation, and health personnel’s experiences or attitudes. Any discrepancies were resolved through consensus among all team members. A total of 949 abstracts were excluded for not meeting this inclusion condition. During the initial search, 56 remaining studies underwent full-text screening, resulting in identification of 22 studies qualified for review.
In the updated search covering the period September 2022 to March 2023, 64 new studies were identified. Of these, 18 studies underwent full-text screening, and one study was included in our review. The total number of included studies is 23. The PRISMA flowchart (Fig. 1 ) illustrates the process.
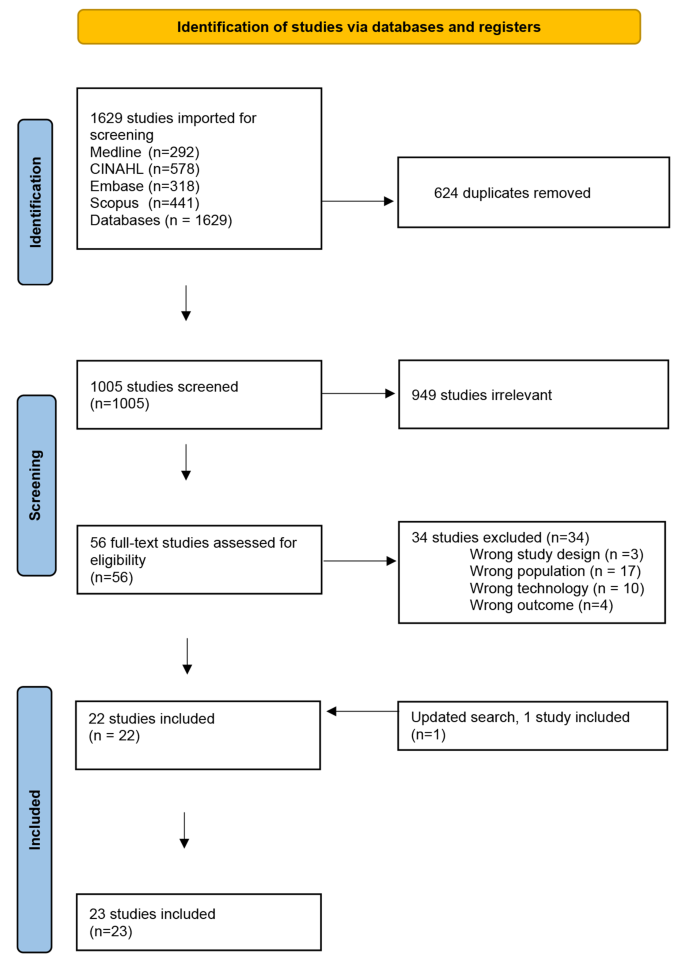
Flow Chart illustrating the study selection and screening process
Data extraction
The research team developed an extraction form for the included studies utilizing an Excel spreadsheet. Following data extraction, the information included the Name of Author(s) Year of publication, Country/countries, Title of the article, Setting, Aim, Design, Participants, and Sample size of the studies, Technology utilized in healthcare services, name/title utilized to describe the Champion Role, how the studies were analyzed and details of Attitude/Experience with the role of champion. Data extraction was conducted by SP, and the results were deliberated in a workshop with the other researchers AB, and HE until a consensus was reached. Any discrepancies were resolved through discussions. The data extraction was categorized into three categories: qualitative, quantitative, and mixed methods, in preparation for quality appraisal.
Quality appraisal
The MMAT [ 26 ] was employed to assess the quality of the 23 included studies. Specifically designed for mixed studies reviews, the MMAT allows for the appraisal of the methodological quality of studies falling into five categories. The studies in our review encompassed qualitative, quantitative descriptive, and mixed methods studies. The MMAT begins with two screening questions to confirm the empirical nature of this study. Subsequently, all studies were categorized by type and evaluated utilizing specific criteria based on their research methods, with ratings of ‘Yes,’ ‘No’ or ‘Can’t tell.’ The MMAT discourages overall scores in favor of providing a detailed explanation for each criterion. Consequently, we did not rely on the MMAT’s overall methodical quality scores and continued to include all 23 studies for our review. Two researchers independently scored the studies, and any discrepancies were discussed among all team members until a consensus was reached. The results of the MMAT assessments are provided in Appendix 2 .
Data synthesis
Based on discussions of this material, additional tables were formulated to present a comprehensive overview of the study characteristics categorized by study design, study settings, technology included, and descriptions/characteristics of the champion role. To capture attitudes and experiences associated with the champion role, the findings from the included studies were translated into narrative texts [ 22 ]. Subsequently, the reviewers worked collaboratively to conduct a thematic analysis, drawing inspiration from Braun and Clarke [ 27 ]. Throughout the synthesis process, multiple meetings were conducted to discern and define the emerging themes and subthemes.
The adopting of new technology in healthcare services can be perceived as both an event and a process. According to Iqbal [ 28 ], experience is defined as the knowledge and understanding gained after an event or the process of living through or undergoing an event. This review synthesizes existing empirical knowledge regarding the experiences of occupying the champion role, and the perspectives of health personnel interacting with champions in technology implementation processes.
Study characteristics
The review encompassed a total of 23 studies, and an overview of these studies is presented in Table 1 . Of these, fourteen studies employed a qualitative design, four had quantitative design, and five utilized a mixed method design. The geographical distribution revealed that the majority of studies were conducted in the USA (8), followed by Australia (5), England (4), Canada (2), Norway (2), Ireland (1), and Malaysia (1). In terms of settings, 11 studies were conducted in hospitals, five in primary health care, three in home-based care settings, and four in a mixed settings where two or more settings collaborated. Various technologies were employed across these studies, with client health records (7) and telemedicine (5) being the most frequently utilized. All studies included experiences from champions or health personnel collaborating with champions in their respective healthcare services. Only three studies had the champion role as a main objective [ 29 , 30 , 31 ]. The remaining studies described champions as one of the strategies in technology implementation processes, including 10 evaluation studies (including feasibility studies [ 32 , 33 , 34 ] and one cost-benefit study [ 30 ]).
Several studies underscored the importance of champions for successful implementation [ 29 , 30 , 31 , 34 , 35 , 36 , 37 , 38 , 40 , 41 , 42 , 43 , 49 ]. Four studies specifically highlighted champions as a key factor for success [ 34 , 36 , 37 , 43 ], and one study went further to describe champions as the most important factor for successful implementation [ 39 ]. Additionally, one study associated champions with reduced labor cost [ 30 ].
Thin descriptions, yet clear expectations for technology champions’ role and -attributes
The analyses revealed that the concept of champions in studies pertaining to technology implementation in healthcare services varies, primarily as a result of the diversity of terms utilized to describe the role combined with short role descriptions. Nevertheless, the studies indicated clear expectations for the champion’s role and associated attributes.
The term champion
The term champion was expressed in 20 different forms across the 23 studies included in our review. Three studies utilized multiple terms within the same study [ 32 , 47 , 48 ] and 15 different authors [ 29 , 32 , 33 , 35 , 36 , 37 , 39 , 40 , 41 , 42 , 43 , 44 , 46 , 47 , 50 ] employed the term with different compositions (Table 1 ). Furthermore, four authors utilized the term Super user [ 30 , 31 , 49 , 51 ], while four authors employed the terms Facilitator [ 38 ], IT clinician [ 48 ], Leader [ 45 ], and Manager [ 34 ], each in combination with more specific terms (such as local opinion leaders, IT nurse, or practice manager).
Most studies associated champion roles with specific professions. In seven studies, the professional title was explicitly linked to the concept of champions, such as physician champions or clinical nurse champions, or through the strategic selection of specific professions [ 29 , 33 , 36 , 40 , 43 , 47 , 50 ]. Additionally, some studies did not specify professions, but utilized terms like clinicians [ 45 ] or health professionals [ 41 ].
All included articles portray the champion’s role as facilitating implementation and daily use of technology among staff. In four studies, the champion’s role was not elaborated beyond indicating that the individual holding the role is confident with an interest in technology [ 35 , 41 , 42 , 44 ]. The champion’s role was explicitly examined in six studies [ 29 , 30 , 31 , 33 , 46 , 50 ]. Furthermore, seven studies described the champion in both the methods and results [ 32 , 36 , 38 , 47 , 48 , 49 , 51 ]. In ten of the studies, champions were solely mentioned in the results [ 34 , 35 , 37 , 39 , 38 , 39 , 40 , 41 , 42 , 43 , 44 , 45 ].
Eight studies provided a specific description or definition of the champion [ 29 , 30 , 31 , 32 , 38 , 48 , 49 , 50 ]. The champion’s role was described as involving training in the specific technology, being an expert on the technology, providing support and assisting peers when needed. In some instance, the champion had a role in leading the implementation [ 50 ], while in other situations, the champion operated as a mediator [ 48 ].
The champions tasks
In the included studies, the champion role encompassed two interrelated facilitators tasks: promoting the technology and supporting others in adopting the technology in their daily practice. Promoting the technology involved encouraging staff adaptation [ 32 , 34 , 35 , 37 , 40 , 41 , 49 ], generally described as being enthusiastic about the technology [ 32 , 35 , 37 , 41 , 48 ], influencing the attitudes and beliefs of colleagues [ 42 , 45 ] and legitimizing the introduction of the technology [ 42 , 46 , 48 ]. Supporting others in technology adaption involved training and teaching [ 31 , 35 , 38 , 40 , 51 ], as well as providing technical support [ 30 , 31 , 39 , 43 , 49 ] and social support [ 49 ]. Only four studies reported that the champions received their own training to enable them able to support their colleagues [ 30 , 31 , 39 , 48 ]. Furthermore, eight studies [ 32 , 34 , 38 , 40 , 48 , 49 , 50 , 51 ], specified that the champion role included leadership and management responsibilities, mentioning tasks such as planning, organizing, coordinating, and mediating technology adaption without providing further details.
Desirable champion attributes
To effectively fulfill their role, champions should ideally possess clinical expertise and experience [ 29 , 35 , 38 , 40 , 48 ], stay professionally updated [ 37 , 48 ], and possess knowledge of the organization and workflows [ 29 , 34 , 46 ]. They should have the ability to understand and communicate effectively with healthcare personnel [ 31 , 32 , 46 , 49 ] and be proficient in IT language [ 51 ]. Moreover, champions should demonstrate a general technological interest and competence, and competence, along with specific knowledge of the technology to be implemented [ 32 , 37 , 49 ]. It is also emphasized that they should command formal and/or informal respect and authority in the organization [ 36 , 45 ], be accessible to others [ 39 , 43 ], possess leadership qualities [ 34 , 37 , 38 , 46 ], and understand and balance the needs of stakeholders [ 43 ]. Lastly, the champions should be enthusiastic promoters of the technology, engaging and supporting others [ 31 , 32 , 33 , 34 , 37 , 39 , 40 , 41 , 43 , 49 ], while also effectively coping with cultural resistance to change [ 31 , 46 ].
Anchoring and recruiting for the champion role
The champions were organized differently within services, holding various positions in the organizations, and being recruited for the role in different ways.
Anchoring the champion role
The champion’s role is primarily anchored at two levels: the management level and/or the clinical level, with two studies having champions at both levels [ 34 , 49 ]. Those working with the management actively participated in the planning of the technology implementation [ 29 , 36 , 40 , 41 , 45 ]. Serving as advisors to management, they leveraged their clinical knowledge to guide the implementation in alignment with the necessities and possibilities of daily work routines in the clinics. Champions in this capacity experienced having a clear formal position that enabled them to fulfil their role effectively [ 29 , 40 ]. Moreover, these champions served as bridge builders between the management and department levels [ 36 , 45 ], ensuring the necessary flow of information in both directions.
Champions anchored at the clinic level played a pivotal role in the practical implementation and facilitation of the daily use of technology [ 31 , 33 , 35 , 37 , 38 , 43 , 48 , 51 ]. Additionally, these champions actively participated in meetings with senior management to discuss the technology and its implementation in the clinic. This position conferred potential influence over health personnel [ 33 , 35 ]. Champions at the clinic level facilitated collaboration between employees, management, and suppliers [ 48 ]. Fontaine et al. [ 36 ] identified respected champions at the clinical level, possessing authority and formal support from all leadership levels, as the most important factor for success.
Only one study reported that the champions received additional compensation for their role [ 36 ], while another study mentioned champions having dedicated time to fulfil their role [ 46 ]. The remaining studies did not provide this information.
Recruiting for the role as champion
Several studies have reported different experiences regarding the management’s selection of champions. A study highlighted the distinctions between a volunteered role and an appointed champion’s role [ 31 ]. Some studies underscored that appointed champions were chosen based on technological expertise and skills [ 41 , 48 , 51 ]. Moreover, the selection criteria included champions’ interest in the specific technology [ 42 ] or experiential skills [ 40 ]. The remaining studies did not provide this information.
While the champion role was most frequently held by health personnel with clinical experience, one study deviated by hiring 150 newly qualified nurses as champions [ 30 ] for a large-scale implementation of an Electronic Health Record (EHR). Opting for clinical novices assisted in reducing implementation costs, as it avoided disrupting daily tasks and interfering with daily operations. According to Bullard [ 30 ], these super-user nurses became highly sought after post-implementation as a result of their technological confidence and competence.
Reported experiences of champions and health personnel
Drawing from the experiences of both champions and health personnel, it is essential for a champion to possess a combination of general knowledge and specific champion characteristics. Furthermore, champions are required to collaborate with individuals both within and outside the organization. The subsequent paragraphs delineate these experiences, categorizing them into four subsets: champions’ contextual knowledge and expertise, preferred performance of the champion role, recognizing that a champion alone is insufficient, and distinguishing between reactive and proactive champions.
Champions’ contextual knowledge and know-how
Health personnel with experience interacting with champions emphasized that a champion must be familiar with the department and its daily work routines [ 35 , 40 ]. Knowledge of the department’s daily routines made it easier for champions to facilitate the adaptation of technology. However, there was a divergence of opinions on whether champions were required to possess extensive clinical experience to fulfil their role. In most studies, having an experienced and competent clinician as a champion instilled a sense of confidence among health personnel. Conversely, Bullard’s study [ 30 ] exhibited that health personnel were satisfied with newly qualified nurses in the role of champion, despite their initial skepticism.
It is a generally expected that champions should possess technological knowledge beyond that of other health professionals [ 37 , 41 ]. Some health personnel perceived the champions as uncritical promoters of technology, with the impression that health personnel were being compelled to utilize technology [ 46 ]. Champions could also overestimate the readiness of health personnel to implement a technology, especially during the early phases of the implementation process [ 32 ]. Regardless of whether the champion is at the management level or the clinic level, champions themselves have acknowledged the importance of providing time and space for innovation. Moreover, the recruitment of champions should span all levels of the organization [ 34 , 46 ]. Furthermore, champions must be familiar with daily work routines, work tools, and work surfaces [ 38 , 40 , 43 ].
Preferable performance of the champion role
The studies identified several preferable characteristics of successful champions. Health personnel favored champions utilizing positive words when discussing technology and exhibiting positive attitudes while facilitating and adapting it [ 33 , 34 , 37 , 38 , 41 , 46 ]. Additionally, champions who were enthusiastic and engaging were considered good role models for the adoption of technology. Successful champions were perceived as knowledgeable and adept problem solvers who motivated and supported health personnel [ 41 , 43 , 44 , 48 ]. They were also valued for being available and responding promptly when contacted [ 42 ]. Health professionals noted that champions perceived as competent garnered respect in the organization [ 40 ]. Moreover, some health personnel felt that some certain champions wielded a greater influence based on how they encouraged the use of the system [ 48 ]. It was also emphasized that health personnel needed to feel it was safe to provide feedback to champions, especially when encountering difficulties or uncertainties [ 49 ].
A champion is not enough
The role of champions proved to be more demanding than expected [ 29 , 31 , 38 ], involving tasks such as handling an overwhelming number of questions or actively participating in the installation process to ensure the technology functions effectively in the department [ 29 ]. Regardless of the organizational characteristics or the champion’s profile, appointing the champion as a “solo implementation agent” is deemed unsuitable. If the organization begins with one champion, it is recommended that this individual promptly recruits others into the role [ 42 ].
Health personnel, reliant on champions’ expertise, found it beneficial to have champions in all departments, and these champions had to be actively engaged in day-to-day operations [ 31 , 33 , 34 , 37 ]. Champions themselves also noted that health personnel increased their technological expertise through their role as champions in the department [ 39 ].
Furthermore, the successful implementation of technology requires the collaboration of various professions and support functions, a task that cannot be solely addressed by a champion [ 29 , 43 , 48 ]. In Orchard et. al.‘s study [ 34 ], champions explicitly emphasized the necessity of support from other personnel in the organization, such as those responsible for the technical aspects and archiving routines, to provide essential assistance.
According to health personnel, the role of champions is vulnerable in case they become sick or leave their position [ 42 , 51 ]. In some of the included studies, only one or a few hold the position of champion [ 37 , 38 , 42 , 48 ]. Two studies observed that their implementations were not completed because champions left or reassigned for various reasons [ 32 , 51 ]. The health professionals in the study by Owens and Charles [ 32 ] expressed that champions must be replaced in such cases. Further, the study of Olsen et al., 2021 [ 42 ] highlights the need for quicky building a champion network within the organization.
Reactive and proactive champions
Health personnel and champions alike noted that champions played both a reactive and proactive role. The proactive role entailed facilitating measures such as training and coordination [ 31 , 32 , 33 , 34 , 37 , 39 , 40 , 41 , 43 , 48 , 49 ] as initiatives to generate enthusiasm for the technology [ 31 , 32 , 33 , 34 , 35 , 37 , 39 , 40 , 41 , 43 , 49 ]. On the other hand, the reactive role entailed hands-on support and troubleshooting [ 30 , 31 , 39 , 43 , 49 ].
In a study presenting experiences from both health personnel and champions, Yuan et al. [ 31 ] found that personnel observed differences in the assistance provided by appointed and self-chosen champions. Appointed champions demonstrated the technology, answered questions from health personnel, but quickly lost patience and track of employees who had received training [ 31 ]. Health personnel perceived that self-chosen champions were proactive and well-prepared to facilitate the utilization of technology, communicating with the staff as a group and being more competent in utilizing the technology in daily practice [ 31 ]. Health personnel also noted that volunteer champions were supportive, positive, and proactive in promoting the technology, whereas appointed champions acted on request and had a more reactive approach [ 31 ].
This review underscores the breadth of the concept of champion and the significant variation in the champion’s role in implementation of technology in healthcare services. This finding supports the results from previous reviews [ 10 , 18 , 19 , 20 ]. The majority of studies meeting our inclusion criteria did not specifically focus on the experiences of champions and health personnel regarding the champion role, with the exception of studies by Bullard [ 30 ], Gui et al. [ 29 ], Helmer-Smith et al. [ 33 ], Hogan-Murphy et al. [ 46 ], Rea et al. [ 50 ], and Yuan et al. [ 31 ].
The 23 studies encompassed in this review utilized 20 different terms for the champion role. In most studies, the champion’s role was briefly described in terms of the duties it entailed or should entail. This may be linked to the fact that the role of champions was not the primary focus of the study, but rather one of the strategies in the implementation process being investigated. This result reinforces the conclusions drawn by Miech et al. [ 10 ] and Shea et al. [ 12 ] regarding the lack of united understandings of the concept. Furthermore, in Santos et al.‘s [ 19 ] review, champions were only operationalized through presence or absence in 71.4% of the included studies. However, our review finds that there is a consistent and shared understanding that champions should promote and support technology implementation.
Several studies advocate for champions as an effective and recommended strategy for implementing technology [ 30 , 31 , 33 , 34 , 35 , 36 , 37 , 38 , 39 , 40 , 42 , 43 , 45 , 46 ]. However, we identified that few studies exclusively explore health personnel`s experiences within the champion role when implementing technology in healthcare services.
This suggests a general lack of information essential for understanding the pros, cons, and prerequisites for champions as a strategy within this field of knowledge. However, this review identifies, on a general basis, the types of support and structures required for champions to perform their role successfully from the perspectives of health personnel, contributing to Shea’s conceptual model [ 12 ].
Regarding the organization of the role, this review identified champions holding both formal appointed and informal roles, working in management or clinical settings, being recruited for their clinical and/or technological expertise, and either volunteering or being hired with specific benefits for the role. Regardless of these variations, anchoring the role is crucial for both the individuals holding the champion role and the health personnel interacting with them. Anchoring, in this context, is associated with the clarity of the role’s content and a match between role expectations and opportunities for fulfilment. Furthermore, the role should be valued by the management, preferably through dedicated time and/or salary support [ 34 , 36 , 46 ]. Additionally, our findings indicate that relying on a “solo champion” is vulnerable to issues such as illness, turnover, excessive workload, and individual champion performance [ 32 , 37 ]. Based on these insights, it appears preferable to appoint multiple champions, with roles at both management and clinical levels [ 33 ].
Some studies have explored the selection of champions and its impact on role performance, revealing diverse experiences [ 30 , 31 ]. Notably, Bullard [ 30 ], stands out for emphasizing long clinical experience, and hiring newly trained nurses as superusers to facilitate the use of electronic health records. Despite facing initial reluctance, these newly trained nurses gradually succeeded in their roles. This underscores the importance of considering contextual factors in the champion selection [ 30 , 52 ]. In Bullard’s study [ 30 ], the collaboration between newly trained nurses as digital natives and clinical experienced health personnel proved beneficial, highlighting the need to align champion selection with the organization’s needs based on personal characteristics. This finding aligns with Melkas et al.‘s [ 9 ] argument that implementing technology requires a deeper understanding of users, access to contextual know-how, and health personnel’s tacit knowledge.
To meet role expectations and effectively leverage their professional and technological expertise, champions should embody personal qualities such as the ability to engage others, take a leadership role, be accessible, supportive, and communicate clearly. These qualities align with the key attributes for change in healthcare champions described by Bonawitz et al. [ 15 ]. These attributes include influence, ownership, physical presence, persuasiveness, grit, and a participative leadership style (p.5). These findings suggest that the active performance of the role, beyond mere presence, is crucial for champions to be a successful strategy in technology implementation. Moreover, the recruitment process is not inconsequential. Identifying the right person for the role and providing them with adequate training, organizational support, and dedicated time to fulfill their responsibilities emerge as an important factor based on the insights from champions and health personnel.
Strengths and limitations
While this study benefits from identifying various terms associated with the role of champions, it acknowledges the possibility of missing some studies as a result of diverse descriptions of the role. Nonetheless, a notable strength of the study lies in its specific focus on the health personnel’s experiences in holding the champion role and the broader experiences of health personnel concerning champions in technology implementation within healthcare services. This approach contributes valuable insights into the characteristics of experiences and attitudes toward the role of champions in implementing technology. Lastly, the study emphasizes the relationship between the experiences with the champion role and the organizational setting’s characteristics.
The champion role was frequently inadequately defined [ 30 , 33 , 34 , 35 , 36 , 37 , 39 , 41 , 42 , 43 , 44 , 45 , 46 , 47 , 51 ], aligning with previous reviews [ 17 , 19 , 21 ]. As indicated by van Laere and Aggestam [ 52 ], this lack of clarity complicates the identification and comparison of champions across studies. Studies that lacking a distinct definition of the champion’s role were consequently excluded. Only studies written in English were included, introducing the possibility of overlooking relevant studies based on our chosen terms for identifying the champion’s role. Most of the included studies focused on technology implementation in a general context, with champions being just one of several measures. This approach resulted in scant descriptions, as champions were often discussed in the results, discussion, or implications sections rather than being the central focus of the research.
As highlighted by Hall et al. [ 18 ]., methodological issues and inadequate reporting in studies of the champion role create challenges for conducting high-quality reviews, introducing uncertainty around the findings. We have adopted a similar approach to Santos et al. [ 19 ], including all studies even when some issues were identified during the quality assessment. Our review shares the same limitations as previous review by Santos et al. [ 19 ] on the champion role.
Practical implications, policy, and future research
The findings emphasize the significance of the relationship between experiences with the champion role and characteristics of organizational settings as crucial factors for success in the champion role. Clear anchoring of the role within the organization is vital and may impact routines, workflows, staffing, and budgets. Despite limited evidence on the experience of the champion’s role, volunteering, hiring newly graduated health personnel, and appointing more than one champion are identified as facilitators of technology implementation. This study underscores the need for future empirical research including clear descriptions of the champion roles, details on study settings and the technologies to be adopted. This will enable the determination of outcomes and success factors in holding champions in technology implementation processes, transferability of knowledge between contexts and technologies as well as enhance the comparability of studies. Furthermore, there is a need for studies to explore experiences with the champion role, preferably from the perspective of multiple stakeholders, as well as focus on the champion role within various healthcare settings.
This study emphasizes that champions can hold significant positions when provided with a clear mandate, dedicated time, and training, contributing their professional, technological, and personal competencies to expedite technology adoption within services. It appears to be an advantage if the health personnel volunteer or apply for the role to facilitate engaged and proactive champions. The implementation of technology in healthcare services demands efforts from the entire service, and the experiences highlighted in this review exhibits that champions can play an important role. Consequently, empirical studies dedicated to the champion role, employing robust designs based current knowledge, are still needed to provide solid understanding of how champions can be a successful initiative when implementing technology in healthcare services.
Data availability
This review relies exclusively on previously published studies. The datasets supporting the conclusions of this article are included within the article and its supplementary files: Description and characteristics of included studies in Table 1 , Study characteristics. The search strategy is provided in Appendix 1 , and the Critical Appraisal Summary of included studies utilizing MMAT is presented in Appendix 2 .
Abbreviations
Electronic Health Record
Implementation Outcomes Framework
Preferred Reporting Items for Systematics and Meta-Analysis
Meskó B, Drobni Z, Bényei É, Gergely B, Győrffy Z. Digital health is a cultural transformation of traditional healthcare. mHealth. 2017;3:38. https://doi.org/10.21037/mhealth.2017.08.07 .
Article PubMed PubMed Central Google Scholar
Pérez Sust P, Solans O, Fajardo JC, Medina Peralta M, Rodenas P, Gabaldà J, et al. Turning the crisis into an opportunity: Digital health strategies deployed during the COVID-19 outbreak. JMIR Public Health Surveill. 2020;6:e19106. https://doi.org/10.2196/19106 .
Alotaibi YK, Federico F. The impact of health information technology on patient safety. Saudi MedJ. 2017;38:117380. https://doi.org/10.15537/smj.2017.12.20631 .
Article Google Scholar
Kuoppamäki S. The application and deployment of welfare technology in Swedish municipal care: a qualitative study of procurement practices among municipal actors. BMC Health Serv Res. 2021;21:918. https://doi.org/10.1186/s12913-021-06944-w .
Kraus S, Schiavone F, Pluzhnikova A, Invernizzi AC. Digital transformation in healthcare: analyzing the current state-of-research. J Bus Res. 2021;123:557–67. https://doi.org/10.1016/j.jbusres.2020.10.030 .
Frennert S. Approaches to welfare technology in municipal eldercare. JTechnolHum. 2020;38:22646. https://doi.org/10.1080/15228835.2020.1747043 .
Konttila J, Siira H, Kyngäs H, Lahtinen M, Elo S, Kääriäinen M, et al. Healthcare professionals’ competence in digitalisation: a systematic review. Clin Nurs. 2019;28:74561. https://doi.org/10.1111/jocn.14710 .
Jacob C, Sanchez-Vazquez A, Ivory C. Social, organizational, and technological factors impacting clinicians’ adoption of mobile health tools: systematic literature review. JMIR mHealth uHealth. 2020;8:e15935. https://doi.org/10.2196/15935 .
Melkas H, Hennala L, Pekkarinen S, Kyrki V. Impacts of robot implementation on care personnel and clients in elderly-care institutions. Int J Med Inf. 2020;134:104041. https://doi.org/10.1016/j.ijmedinf.2019.104041 .
Miech EJ, Rattray NA, Flanagan ME, Damschroder L, Schmid AA, Damush TM. Inside help: an integrative review of champions in healthcare-related implementation. SAGE Open Med. 2018;6. https://doi.org/10.1177/2050312118773261 .
Foong HF, Kyaw BM, Upton Z, Tudor Car L. Facilitators and barriers of using digital technology for the management of diabetic foot ulcers: a qualitative systematic review. Int Wound J. 2020;17:126681. https://doi.org/10.1111/iwj.13396 .
Shea CM. A conceptual model to guide research on the activities and effects of innovation champions. Implement Res Pract. 2021;2. https://doi.org/10.1177/2633489521990443 .
Hudson D. Physician engagement strategies in health information system implementations. Healthc Manage Forum. 2023;36:86–9. https://doi.org/10.1177/08404704221131921 .
Article PubMed Google Scholar
Gullslett MK, Strand Bergmo T. Implementation of E-prescription for multidose dispensed drugs: qualitative study of general practitioners’’ experiences. JMIR HumFactors. 2022;9:e27431. https://doi.org/10.2196/27431 .
Bonawitz K, Wetmore M, Heisler M, Dalton VK, Damschroder LJ, Forman J, et al. Champions in context: which attributes matter for change efforts in healthcare? Implement Sci. 2020;15:62. https://doi.org/10.1186/s13012-020-01024-9 .
George ER, Sabin LL, Elliott PA, Wolff JA, Osani MC, McSwiggan Hong J, et al. Examining health care champions: a mixed-methods study exploring self and peer perspectives of champions. Implement Res Pract. 2022;3. https://doi.org/10.1177/26334895221077880 .
Shea CM, Belden CM. What is the extent of research on the characteristics, behaviors, and impacts of health information technology champions? A scoping review. BMC Med Inf Decis Mak. 2016;16:2. https://doi.org/10.1186/s12911-016-0240-4 .
Hall AM, Flodgren GM, Richmond HL, Welsh S, Thompson JY, Furlong BM, Sherriff A. Champions for improved adherence to guidelines in long-term care homes: a systematic review. Implement Sci Commun. 2021;2(1):85–85. https://doi.org/10.1186/s43058-021-00185-y .
Santos WJ, Graham ID, Lalonde M, Demery Varin M, Squires JE. The effectiveness of champions in implementing innovations in health care: a systematic review. Implement Sci Commun. 2022;3(1):1–80. https://doi.org/10.1186/s43058-022-00315-0 .
Wood K, Giannopoulos V, Louie E, Baillie A, Uribe G, Lee KS, Haber PS, Morley KC. The role of clinical champions in facilitating the use of evidence-based practice in drug and alcohol and mental health settings: a systematic review. Implement Res Pract. 2020;1:2633489520959072–2633489520959072. https://doi.org/10.1177/2633489520959072 .
Rigby K, Redley B, Hutchinson AM. Change agent’s role in facilitating use of technology in residential aged care: a systematic review. Int J Med Informatics. 2023;105216. https://doi.org/10.1016/j.ijmedinf.2023.105216 .
Pluye P, Hong QN. Combining the power of stories and the power of numbers: mixed methods research and mixed studies reviews. Annu Rev Public Health. 2014;35:29–45. https://doi.org/10.1146/annurev-publhealth-032013-182440 .
Page MJ, McKenzie JE, Bossuyt PM, Boutron I, Hoffmann TC, Mulrow CD, et al. The PRISMA 2020 statement: an updated guideline for reporting systematic reviews. BMJ. 2021;372:n71. https://doi.org/10.1136/bmj.n71 .
Pettersen S, Berg A, Eide H. Experiences and attitudes to the role of champions in implementation of technology in health services. A systematic review. PROSPERO. 2022. https://www.crd.york.ac.uk/prospero/display_record.php?ID=CRD42022335750 . Accessed [15 Feb 2023].
Covidence. Better Syst Rev Manag. https://www.covidence.org/ . 2023;26.
Hong QN, Fàbregues S, Bartlett G, Boardman F, Cargo M, Dagenais P, et al. The mixed methods Appraisal Tool (MMAT) version 2018 for information professionals and researchers. Educ Inf. 2018;34:285–91. https://doi.org/10.3233/EFI-180221 .
Braun V, Clarke V. Thematic analysis: a practical guide. 1st ed. SAGE; 2022.
Iqbal MP, Manias E, Mimmo L, Mears S, Jack B, Hay L, Harrison R. Clinicians’ experience of providing care: a rapid review. BMC Health Serv Res. 2020;20:1–10. https://doi.org/10.1186/s12913-020-05812-3 .
Gui X, Chen Y, Zhou X, Reynolds TL, Zheng K, Hanauer DA. Physician champions’ perspectives and practices on electronic health records implementation: challenges and strategies. JAMIA open. 2020;3:5361. https://doi.org/10.1093/jamiaopen/ooz051 .
Bullard KL. Cost effective staffing for an EHR implementation. Nurs Econ. 2016;34:726.
Google Scholar
Yuan CT, Bradley EH, Nembhard IM. A mixed methods study of how clinician ‘super users’ influence others during the implementation of electronic health records. BMC Med Inf Decis Mak. 2015;15:26. https://doi.org/10.1186/s12911-015-0154-6 .
Owens C, Charles N. Implementation of a text-messaging intervention for adolescents who self-harm (TeenTEXT): a feasibility study using normalisation process theory. Child Adolesc Psychiatry Ment Health. 2016;10:14. https://doi.org/10.1186/s13034-016-0101-z .
Helmer-Smith M, Fung C, Afkham A, Crowe L, Gazarin M, Keely E, et al. The feasibility of using electronic consultation in long-term care homes. JAm Med Dir Assoc. 2020;21:11661170e2. https://doi.org/10.1016/j.jamda.2020.03.003 .
Orchard J, Lowres N, Freedman SB, Ladak L, Lee W, Zwar N, et al. Screening for atrial fibrillation during influenza vaccinations by primary care nurses using a smartphone electrocardiograph (iECG): a feasibility study. Eur J Prev Cardiol. 2016;23:13–20. https://doi.org/10.1177/2047487316670255 .
Bee P, Lovell K, Airnes Z, Pruszynska A. Embedding telephone therapy in statutory mental health services: a qualitative, theory-driven analysis. BMC Psychiatry. 2016;16:56. https://doi.org/10.1186/s12888-016-0761-5 .
Fontaine P, Whitebird R, Solberg LI, Tillema J, Smithson A, Crabtree BF. Minnesota’s early experience with medical home implementation: viewpoints from the front lines. J Gen Intern Med. 2015;30(7):899–906. https://doi.org/10.1007/s11606-014-3136-y .
Kolltveit B-CH, Gjengedal E, Graue M, Iversen MM, Thorne S, Kirkevold M. Conditions for success in introducing telemedicine in diabetes foot care: a qualitative inquiry. BMC Nurs. 2017;16:2. https://doi.org/10.1186/s12912-017-0201-y .
Salbach NM, McDonald A, MacKay-Lyons M, Bulmer B, Howe JA, Bayley MT, et al. Experiences of physical therapists and professional leaders with implementing a toolkit to advance walking assessment poststroke: a realist evaluation. Phys Ther. 2021;101:1–11. https://doi.org/10.1093/ptj/pzab232 .
Schwarz M, Coccetti A, Draheim M, Gordon G. Perceptions of allied health staff of the implementation of an integrated electronic medical record across regional and metropolitan settings. Aust Health Rev. 2020;44:965–72. https://doi.org/10.1071/AH19024 .
Stewart J, McCorry N, Reid H, Hart N, Kee F. Implementation of remote asthma consulting in general practice in response to the COVID-19 pandemic: an evaluation using extended normalisation process theory. BJGP Open. 2022;6:1–10. https://doi.org/10.3399/BJGPO.2021.0189 .
Bennett-Levy J, Singer J, DuBois S, Hyde K. Translating mental health into practice: what are the barriers and enablers to e-mental health implementation by aboriginal and Torres Strait Islander health professionals? JMed. Internet Res. 2017;19:e1. https://doi.org/10.2196/jmir.6269 .
Olsen J, Peterson S, Stevens A. Implementing electronic health record-based National Diabetes Prevention Program referrals in a rural county. Public Health Nurs (Boston Mass). 2021;38(3):464–9. https://doi.org/10.1111/phn.12860 .
Yang L, Brown-Johnson CG, Miller-Kuhlmann R, Kling SMR, Saliba-Gustafsson EA, Shaw JG, et al. Accelerated launch of video visits in ambulatory neurology during COVID-19: key lessons from the Stanford experience. Neurology. 2020;95:305–11. https://doi.org/10.1212/WNL.0000000000010015 .
Article CAS PubMed Google Scholar
Buckingham SA, Sein K, Anil K, Demain S, Gunn H, Jones RB, et al. Telerehabilitation for physical disabilities and movement impairment: a service evaluation in South West England. JEval Clin Pract. 2022;28:108495. https://doi.org/10.1111/jep.13689 .
Chung OS, Robinson T, Johnson AM, Dowling NL, Ng CH, Yücel M, et al. Implementation of therapeutic virtual reality into psychiatric care: clinicians’ and service managers’’ perspectives. Front Psychiatry. 2022;12:791123. https://doi.org/10.3389/fpsyt.2021.791123 .
Hogan-Murphy D, Stewart D, Tonna A, Strath A, Cunningham S. Use of normalization process theory to explore key stakeholders’ perceptions of the facilitators and barriers to implementing electronic systems for medicines management in hospital settings. Res SocialAdm Pharm. 2021;17:398405. https://doi.org/10.1016/j.sapharm.2020.03.005 .
Moss SR, Martinez KA, Nathan C, Pfoh ER, Rothberg MB. Physicians’ views on utilization of an electronic health record-embedded calculator to assess risk for venous thromboembolism among medical inpatients: a qualitative study. TH Open. 2022;6:e33–9. https://doi.org/10.1055/s-0041-1742227 .
Yusof MM. A case study evaluation of a critical Care Information System adoption using the socio-technical and fit approach. Int J Med Inf. 2015;84:486–99. https://doi.org/10.1016/j.ijmedinf.2015.03.001 .
Dugstad J, Sundling V, Nilsen ER, Eide H. Nursing staff’s evaluation of facilitators and barriers during implementation of wireless nurse call systems in residential care facilities. A cross-sectional study. BMC Health Serv Res. 2020;20:163. https://doi.org/10.1186/s12913-020-4998-9 .
Rea K, Le-Jenkins U, Rutledge C. A technology intervention for nurses engaged in preventing catheter-associated urinary tract infections. Comput Inf Nurs. 2018;36:305–13. https://doi.org/10.1097/CIN.0000000000000429 .
Bail K, Davey R, Currie M, Gibson J, Merrick E, Redley B. Implementation pilot of a novel electronic bedside nursing chart: a mixed-methods case study. Aust Health Rev. 2020;44:672–6. https://doi.org/10.1071/AH18231 .
van Laere J, Aggestam L. Understanding champion behaviour in a health-care information system development project – how multiple champions and champion behaviours build a coherent whole. Eur J Inf Syst. 2016;25:47–63. https://doi.org/10.1057/ejis.2015.5 .
Download references
Acknowledgements
We would like to thank the librarian Malin E. Norman, at Nord university, for her assistance in the development of the search, as well as guidance regarding the scientific databases.
This study is a part of a PhD project undertaken by the first author, SP, and funded by Nord University, Norway. This research did not receive any specific grant from funding agencies in the public, commercial, as well as not-for-profit sectors.
Open access funding provided by Nord University
Author information
Authors and affiliations.
Faculty of Nursing and Health Sciences, Nord university, P.O. Box 474, N-7801, Namsos, Norway
Sissel Pettersen & Anita Berg
Centre for Health and Technology, Faculty of Health Sciences, University of South-Eastern Norway, PO Box 7053, N-3007, Drammen, Norway
You can also search for this author in PubMed Google Scholar
Contributions
The first author/SP has been the project manager and was mainly responsible for all phases of the study. The second and third authors HE and AB have contributed to screening, quality assessment, analysis and discussion of findings. Drafting of the final manuscript has been a collaboration between the first/SP and third athor/AB. The final manuscript has been approved by all authors.
Corresponding author
Correspondence to Sissel Pettersen .
Ethics declarations
Ethics approval and consent to participate.
This review does not involve the processing of personal data, and given the nature of this study, formal consent is not applicable.
Consent for publication
Not applicable.
Competing interests
The authors declare no competing interests.
Additional information
Publisher’s note.
Springer Nature remains neutral with regard to jurisdictional claims in published maps and institutional affiliations.
Electronic supplementary material
Below is the link to the electronic supplementary material.
Supplementary Material 1
Supplementary material 2, rights and permissions.
Open Access This article is licensed under a Creative Commons Attribution 4.0 International License, which permits use, sharing, adaptation, distribution and reproduction in any medium or format, as long as you give appropriate credit to the original author(s) and the source, provide a link to the Creative Commons licence, and indicate if changes were made. The images or other third party material in this article are included in the article’s Creative Commons licence, unless indicated otherwise in a credit line to the material. If material is not included in the article’s Creative Commons licence and your intended use is not permitted by statutory regulation or exceeds the permitted use, you will need to obtain permission directly from the copyright holder. To view a copy of this licence, visit http://creativecommons.org/licenses/by/4.0/ . The Creative Commons Public Domain Dedication waiver ( http://creativecommons.org/publicdomain/zero/1.0/ ) applies to the data made available in this article, unless otherwise stated in a credit line to the data.
Reprints and permissions
About this article
Cite this article.
Pettersen, S., Eide, H. & Berg, A. The role of champions in the implementation of technology in healthcare services: a systematic mixed studies review. BMC Health Serv Res 24 , 456 (2024). https://doi.org/10.1186/s12913-024-10867-7
Download citation
Received : 19 June 2023
Accepted : 14 March 2024
Published : 11 April 2024
DOI : https://doi.org/10.1186/s12913-024-10867-7
Share this article
Anyone you share the following link with will be able to read this content:
Sorry, a shareable link is not currently available for this article.
Provided by the Springer Nature SharedIt content-sharing initiative
- Technology implementation
- Healthcare personnel
- Healthcare services
- Mixed methods
- Organizational characteristics
- Technology adoption
- Role definitions
- Healthcare settings
- Systematic review
BMC Health Services Research
ISSN: 1472-6963
- General enquiries: [email protected]
22 Big Data in Healthcare Examples and Applications
Companies are turning to big data to reimagine every aspect of healthcare.

Big data is being utilized more and more in every industry , but the role it’s playing in healthcare may end up having the greatest impact on our lives.
Researchers, hospitals and physicians are turning to a vast network of healthcare data to understand clinical context, prevent future health issues and even find new treatment options. While there are many ways data is being used to impact healthcare, we’ve rounded up five areas — along with a few examples of companies and organizations working within each area — where big data is taking on some of the major challenges in healthcare.
Big Data in Healthcare Applications and Examples
Big data in healthcare applications.
- Cancer Research
- Disease Detection
- Population Health
- Pharmaceutical Research and Development
- Health Insurance Risk Assessment
Big Data and Cancer Research
Nearly all of us have been impacted by cancer in some way, and the search for new ways to combat the disease is in high gear. Researchers in both the private and public sector are dedicated to everything from researching a cure to finding more effective treatment options. Big data has changed the way these researchers understand the disease, providing access to patient information, trends and patterns never accessible before. The following are just a few of the companies using big data to make headway in the fight against cancer.
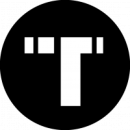
Location: Chicago, Illinois
Tempus is building the largest library of molecular and clinical data in the world with the goal of providing medical professionals with more clinical context for each patient’s cancer case. The Tempus platform collects and organizes data from lab reports, clinical notes, radiology scans and pathology images, accelerating oncology research and helping physicians make more personalized and informed treatment plans.
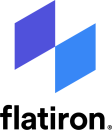
Flatiron Health
Location: New York, New York
Flatiron Health utilizes billions of data points from cancer patients to enhance research and gain new insights for patient care. Their solutions connect all players in the treatment of cancer, from oncologists and hospitals to academics and life science researchers, enabling them to learn from each patient.
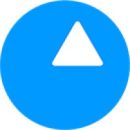
Oncora Medical
Location: Philadelphia, Pennsylvania
Oncora Medical is simplifying workflows for oncologists by blending machine learning, automation and big data into a single platform. With the company’s data analysis tools, oncologists can compile data and quickly add information to a patient’s health records. As a result, oncologists can review a patient’s radiology and pathology history faster, delivering more timely and personalized care to cancer patients.
Related Reading Big Data in Education: 9 Companies Delivering Insights to the Classroom
Big Data and Early Disease Detection
Early detection for diseases and complications is crucial for successful treatment. Whether it’s cancer, multiple sclerosis or a number of other conditions, screenings and other exams are often vital in staying ahead of disease. Here are a few examples of companies leveraging big data to improve early detection of disease and complications in patients.
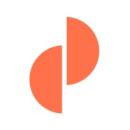
Location: Irving, Texas
Pieces is a cloud-based software company that collects data throughout the entire patient journey to improve both the quality and cost of care. The company’s flagship product, Pieces Decision Sciences, is a clinical engine that makes decisions and recommendations based on a variety of data such as lab results, vitals, and structured and unstructured data. The platform consistently works to identify possible interventions while also learning from clinical outcomes.
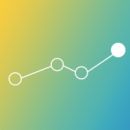
PeraHealth: The Rothman Index
Location: Charlotte, North Carolina
PeraHealth is the creator of the Rothman Index, a peer-reviewed, universal scoring system for the overall health of a patient. The score takes the data within electronic health records, vitals, lab results and nursing assessments to assign a score. The scores are provided in a visual graph and updated in real time to identify changes and keep track of the details, helping patients avoid complications.
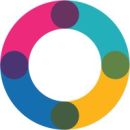
Prognos applies artificial intelligence to clinical data and manages Prognos Factor — a hub for multi-sourced diagnostic data. Their AI platform helps physicians apply treatments earlier, displays clinical trial opportunities, suggests therapy options and exposes care gaps for more than 30 conditions.
Big Data and Population Health
Different from public health, which focuses on how society can ensure healthier people, population health studies the patterns and conditions that affect the overall health of groups . Big data is an essential part of understanding population health because without data, patterns are difficult to pinpoint. The following are just a few examples of companies that are aggregating and organizing data to help healthcare organizations and researchers identify the patterns that can improve health conditions.
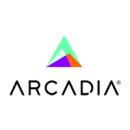
Location: Fully Remote
Arcadia ’s big data platform provides organizations throughout the healthcare landscape with actionable insights that enable them to “make more strategic decisions in support of their financial, clinical, and operational objectives.” In the area of population health management, for example, Arcadia’s analytics capabilities make it possible to identify and overcome care gaps.
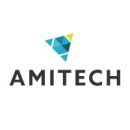
Amitech Solutions
Location: Creve Coeur, Missouri
Amitech Solutions applies data to the health field in multiple ways, from modern data management to healthcare analytics. Specifically, Amitech utilizes data for population health management solutions, combining physical and behavioral health data to identify risks and engage patients in their own healthcare.
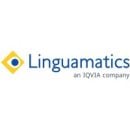
Linguamatics
Location: Marlborough, Massachusetts
Linguamatics mines the untapped, unstructured data in electronic health records for research and solutions in population health. By using natural language processing , Linguamatics can use unstructured patient data to identify lifestyle factors, build predictive models and detect high-risk patients.
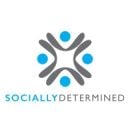
Socially Determined
Location: Washington, D.C.
Socially Determined takes a more holistic approach to population health by supplying healthcare organizations with social risk intelligence. The company’s platform SocialScape measures factors such as patients’ access to housing, transportation and food. Healthcare groups can then craft their strategies around these variables to deliver tailored care to specific populations.
Big Data and Pharmaceutical Research
Whether it be vaccines, synthetic insulin or simple antihistamines, medicines produced by the pharmaceutical industry play an important role in the treatment of disease. New drug discovery and creation depends on data to assess the viability and effectiveness of treatments. The following companies are using big data to help enhance pharmaceutical companies with research and development .
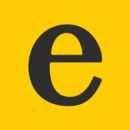
Location: San Mateo, California
Evidation has a mobile app that rewards users for healthy behaviors, provides access to health insights and offers opportunities to contribute to health research. Through Evidation, researchers can access everyday health data that informs their work and enables them to discover new ways of diagnosing, treating and managing various medical conditions. The company prioritizes giving app users control over their data, asking them for consent before their data can be accessed.
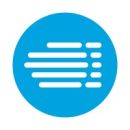
Location: Durham, North Carolina
IQVIA builds links between analytics, data and technology, so pharmacy leaders can complete faster and more effective clinical research. Besides boasting a dense healthcare database , the company leverages AI and machine learning to pinpoint the ideal patients for specific trials. Pharmacists can then run decentralized trials, compile data with IQVIA’s devices and jumpstart the research and development process.
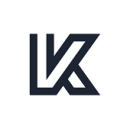
Kalderos is challenging the cost of pharmaceuticals through its drug discount management platform, which collects data from multiple sources and stakeholders to improve transparency among patients. Drug manufacturers, covered entities and payers can use the platform to collaborate too. The company hopes to promote trust and equity within the healthcare industry.
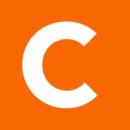
Location: Santa Clara, California
Although now part of the Cloudera family due to a merger , the Hortonworks data platform continues to help pharmaceutical companies and researchers gain a better view of pharmaceutical data. Because billions of records are integrated and made accessible, companies can answer questions that weren’t possible before. This sparks more effective research for clinical trials, improved safety, faster time to market and better health outcomes .
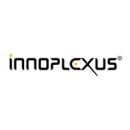
Location: Iselin, New Jersey (U.S. office)
Innoplexus is the creator of the iPlexus discovery tool that organizes millions of publications, articles, dissertations, thousands of clinical trials, drug profiles and congress articles into a concept-based research platform. The tool helps pharmaceutical companies find the relevant information needed for research and new drug discovery.
Related Reading 9 Examples of Big Data in Media and Entertainment
Big Data and Health Records
When it comes to healthcare and specifically health insurance , risk is often a large contributing factor in how patients access care. The following are a few examples of companies using big data to gain more insight into risk and ensure accuracy in adjustments.
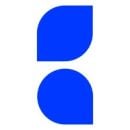
Blubyrd is designed to help surgical facilities and clinical practices compile and exchange data efficiently and securely. This data includes appointment schedules, procedure codes and equipment inventory.
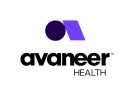
Avaneer Health
Avaneer Health works to improve the efficiency of data flow in the healthcare industry by giving network participants access to administrative help and secured transactions. Founded in 2020 by a collective of top healthcare industry leaders — including CVS, Anthem, Cleveland Clinic and more — the company’s platform relies on blockchain.
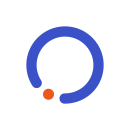
Particle Health
Particle Health makes an API platform that brings together patient records into a single secure place. With a simple query, developers can access clean and actionable data sets to use. The goal is for healthcare providers to use the data to make more meaningful recommendations to their patients.
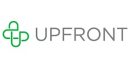
Upfront Healthcare
Upfront Healthcare ’s software platform uses data-driven personalization to improve communications between healthcare professionals and patients. For example, Upfront collects data — such as patient-reported outcomes, behavioral patterns, psychographic segments — and uses it to deliver relevant and timely messages to patients, whether it’s a reminder or a call to action.
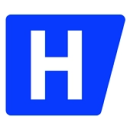
Human API streamlines the underwriting process by allowing teams to sift through detailed electronic health records. A health intelligence platform reviews patients’ health backgrounds with automated features and pinpoints any underlying conditions. This workflow reduces the time it takes to complete each application, leading to higher placement rates, larger volumes of applicants and improved customer experiences.
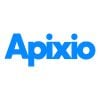
Apixio ’s data acquisition technology wrangles medical data from millions of files, claims, PDFs and other health records. With this information, Apixio’s coding application provides more accurate risk adjustment for healthcare providers.
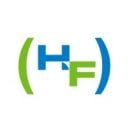
Health Fidelity
Health Fidelity helps healthcare providers and institutions find risks normally concealed in clinical charts. Their technology uses natural language processing to extract 100 percent of data within clinical charts and identify problems in care, assessment and documentation, which provides improved visibility for risk adjustment.
Rose Velazquez contributed reporting to this story.
Recent Big Data Articles
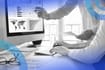

An official website of the United States government
The .gov means it’s official. Federal government websites often end in .gov or .mil. Before sharing sensitive information, make sure you’re on a federal government site.
The site is secure. The https:// ensures that you are connecting to the official website and that any information you provide is encrypted and transmitted securely.
- Publications
- Account settings
Preview improvements coming to the PMC website in October 2024. Learn More or Try it out now .
- Advanced Search
- Journal List
- Int J Prev Med
Qualitative Methods in Health Care Research
Vishnu renjith.
School of Nursing and Midwifery, Royal College of Surgeons Ireland - Bahrain (RCSI Bahrain), Al Sayh Muharraq Governorate, Bahrain
Renjulal Yesodharan
1 Department of Mental Health Nursing, Manipal College of Nursing Manipal, Manipal Academy of Higher Education, Manipal, Karnataka, India
Judith A. Noronha
2 Department of OBG Nursing, Manipal College of Nursing Manipal, Manipal Academy of Higher Education, Manipal, Karnataka, India
Elissa Ladd
3 School of Nursing, MGH Institute of Health Professions, Boston, USA
Anice George
4 Department of Child Health Nursing, Manipal College of Nursing Manipal, Manipal Academy of Higher Education, Manipal, Karnataka, India
Healthcare research is a systematic inquiry intended to generate robust evidence about important issues in the fields of medicine and healthcare. Qualitative research has ample possibilities within the arena of healthcare research. This article aims to inform healthcare professionals regarding qualitative research, its significance, and applicability in the field of healthcare. A wide variety of phenomena that cannot be explained using the quantitative approach can be explored and conveyed using a qualitative method. The major types of qualitative research designs are narrative research, phenomenological research, grounded theory research, ethnographic research, historical research, and case study research. The greatest strength of the qualitative research approach lies in the richness and depth of the healthcare exploration and description it makes. In health research, these methods are considered as the most humanistic and person-centered way of discovering and uncovering thoughts and actions of human beings.
Introduction
Healthcare research is a systematic inquiry intended to generate trustworthy evidence about issues in the field of medicine and healthcare. The three principal approaches to health research are the quantitative, the qualitative, and the mixed methods approach. The quantitative research method uses data, which are measures of values and counts and are often described using statistical methods which in turn aids the researcher to draw inferences. Qualitative research incorporates the recording, interpreting, and analyzing of non-numeric data with an attempt to uncover the deeper meanings of human experiences and behaviors. Mixed methods research, the third methodological approach, involves collection and analysis of both qualitative and quantitative information with an objective to solve different but related questions, or at times the same questions.[ 1 , 2 ]
In healthcare, qualitative research is widely used to understand patterns of health behaviors, describe lived experiences, develop behavioral theories, explore healthcare needs, and design interventions.[ 1 , 2 , 3 ] Because of its ample applications in healthcare, there has been a tremendous increase in the number of health research studies undertaken using qualitative methodology.[ 4 , 5 ] This article discusses qualitative research methods, their significance, and applicability in the arena of healthcare.
Qualitative Research
Diverse academic and non-academic disciplines utilize qualitative research as a method of inquiry to understand human behavior and experiences.[ 6 , 7 ] According to Munhall, “Qualitative research involves broadly stated questions about human experiences and realities, studied through sustained contact with the individual in their natural environments and producing rich, descriptive data that will help us to understand those individual's experiences.”[ 8 ]
Significance of Qualitative Research
The qualitative method of inquiry examines the 'how' and 'why' of decision making, rather than the 'when,' 'what,' and 'where.'[ 7 ] Unlike quantitative methods, the objective of qualitative inquiry is to explore, narrate, and explain the phenomena and make sense of the complex reality. Health interventions, explanatory health models, and medical-social theories could be developed as an outcome of qualitative research.[ 9 ] Understanding the richness and complexity of human behavior is the crux of qualitative research.
Differences between Quantitative and Qualitative Research
The quantitative and qualitative forms of inquiry vary based on their underlying objectives. They are in no way opposed to each other; instead, these two methods are like two sides of a coin. The critical differences between quantitative and qualitative research are summarized in Table 1 .[ 1 , 10 , 11 ]
Differences between quantitative and qualitative research
Qualitative Research Questions and Purpose Statements
Qualitative questions are exploratory and are open-ended. A well-formulated study question forms the basis for developing a protocol, guides the selection of design, and data collection methods. Qualitative research questions generally involve two parts, a central question and related subquestions. The central question is directed towards the primary phenomenon under study, whereas the subquestions explore the subareas of focus. It is advised not to have more than five to seven subquestions. A commonly used framework for designing a qualitative research question is the 'PCO framework' wherein, P stands for the population under study, C stands for the context of exploration, and O stands for the outcome/s of interest.[ 12 ] The PCO framework guides researchers in crafting a focused study question.
Example: In the question, “What are the experiences of mothers on parenting children with Thalassemia?”, the population is “mothers of children with Thalassemia,” the context is “parenting children with Thalassemia,” and the outcome of interest is “experiences.”
The purpose statement specifies the broad focus of the study, identifies the approach, and provides direction for the overall goal of the study. The major components of a purpose statement include the central phenomenon under investigation, the study design and the population of interest. Qualitative research does not require a-priori hypothesis.[ 13 , 14 , 15 ]
Example: Borimnejad et al . undertook a qualitative research on the lived experiences of women suffering from vitiligo. The purpose of this study was, “to explore lived experiences of women suffering from vitiligo using a hermeneutic phenomenological approach.” [ 16 ]
Review of the Literature
In quantitative research, the researchers do an extensive review of scientific literature prior to the commencement of the study. However, in qualitative research, only a minimal literature search is conducted at the beginning of the study. This is to ensure that the researcher is not influenced by the existing understanding of the phenomenon under the study. The minimal literature review will help the researchers to avoid the conceptual pollution of the phenomenon being studied. Nonetheless, an extensive review of the literature is conducted after data collection and analysis.[ 15 ]
Reflexivity
Reflexivity refers to critical self-appraisal about one's own biases, values, preferences, and preconceptions about the phenomenon under investigation. Maintaining a reflexive diary/journal is a widely recognized way to foster reflexivity. According to Creswell, “Reflexivity increases the credibility of the study by enhancing more neutral interpretations.”[ 7 ]
Types of Qualitative Research Designs
The qualitative research approach encompasses a wide array of research designs. The words such as types, traditions, designs, strategies of inquiry, varieties, and methods are used interchangeably. The major types of qualitative research designs are narrative research, phenomenological research, grounded theory research, ethnographic research, historical research, and case study research.[ 1 , 7 , 10 ]
Narrative research
Narrative research focuses on exploring the life of an individual and is ideally suited to tell the stories of individual experiences.[ 17 ] The purpose of narrative research is to utilize 'story telling' as a method in communicating an individual's experience to a larger audience.[ 18 ] The roots of narrative inquiry extend to humanities including anthropology, literature, psychology, education, history, and sociology. Narrative research encompasses the study of individual experiences and learning the significance of those experiences. The data collection procedures include mainly interviews, field notes, letters, photographs, diaries, and documents collected from one or more individuals. Data analysis involves the analysis of the stories or experiences through “re-storying of stories” and developing themes usually in chronological order of events. Rolls and Payne argued that narrative research is a valuable approach in health care research, to gain deeper insight into patient's experiences.[ 19 ]
Example: Karlsson et al . undertook a narrative inquiry to “explore how people with Alzheimer's disease present their life story.” Data were collected from nine participants. They were asked to describe about their life experiences from childhood to adulthood, then to current life and their views about the future life. [ 20 ]
Phenomenological research
Phenomenology is a philosophical tradition developed by German philosopher Edmond Husserl. His student Martin Heidegger did further developments in this methodology. It defines the 'essence' of individual's experiences regarding a certain phenomenon.[ 1 ] The methodology has its origin from philosophy, psychology, and education. The purpose of qualitative research is to understand the people's everyday life experiences and reduce it into the central meaning or the 'essence of the experience'.[ 21 , 22 ] The unit of analysis of phenomenology is the individuals who have had similar experiences of the phenomenon. Interviews with individuals are mainly considered for the data collection, though, documents and observations are also useful. Data analysis includes identification of significant meaning elements, textural description (what was experienced), structural description (how was it experienced), and description of 'essence' of experience.[ 1 , 7 , 21 ] The phenomenological approach is further divided into descriptive and interpretive phenomenology. Descriptive phenomenology focuses on the understanding of the essence of experiences and is best suited in situations that need to describe the lived phenomenon. Hermeneutic phenomenology or Interpretive phenomenology moves beyond the description to uncover the meanings that are not explicitly evident. The researcher tries to interpret the phenomenon, based on their judgment rather than just describing it.[ 7 , 21 , 22 , 23 , 24 ]
Example: A phenomenological study conducted by Cornelio et al . aimed at describing the lived experiences of mothers in parenting children with leukemia. Data from ten mothers were collected using in-depth semi-structured interviews and were analyzed using Husserl's method of phenomenology. Themes such as “pivotal moment in life”, “the experience of being with a seriously ill child”, “having to keep distance with the relatives”, “overcoming the financial and social commitments”, “responding to challenges”, “experience of faith as being key to survival”, “health concerns of the present and future”, and “optimism” were derived. The researchers reported the essence of the study as “chronic illness such as leukemia in children results in a negative impact on the child and on the mother.” [ 25 ]
Grounded Theory Research
Grounded theory has its base in sociology and propagated by two sociologists, Barney Glaser, and Anselm Strauss.[ 26 ] The primary purpose of grounded theory is to discover or generate theory in the context of the social process being studied. The major difference between grounded theory and other approaches lies in its emphasis on theory generation and development. The name grounded theory comes from its ability to induce a theory grounded in the reality of study participants.[ 7 , 27 ] Data collection in grounded theory research involves recording interviews from many individuals until data saturation. Constant comparative analysis, theoretical sampling, theoretical coding, and theoretical saturation are unique features of grounded theory research.[ 26 , 27 , 28 ] Data analysis includes analyzing data through 'open coding,' 'axial coding,' and 'selective coding.'[ 1 , 7 ] Open coding is the first level of abstraction, and it refers to the creation of a broad initial range of categories, axial coding is the procedure of understanding connections between the open codes, whereas selective coding relates to the process of connecting the axial codes to formulate a theory.[ 1 , 7 ] Results of the grounded theory analysis are supplemented with a visual representation of major constructs usually in the form of flow charts or framework diagrams. Quotations from the participants are used in a supportive capacity to substantiate the findings. Strauss and Corbin highlights that “the value of the grounded theory lies not only in its ability to generate a theory but also to ground that theory in the data.”[ 27 ]
Example: Williams et al . conducted a grounded theory research to explore the nature of relationship between the sense of self and the eating disorders. Data were collected form 11 women with a lifetime history of Anorexia Nervosa and were analyzed using the grounded theory methodology. Analysis led to the development of a theoretical framework on the nature of the relationship between the self and Anorexia Nervosa. [ 29 ]
Ethnographic research
Ethnography has its base in anthropology, where the anthropologists used it for understanding the culture-specific knowledge and behaviors. In health sciences research, ethnography focuses on narrating and interpreting the health behaviors of a culture-sharing group. 'Culture-sharing group' in an ethnography represents any 'group of people who share common meanings, customs or experiences.' In health research, it could be a group of physicians working in rural care, a group of medical students, or it could be a group of patients who receive home-based rehabilitation. To understand the cultural patterns, researchers primarily observe the individuals or group of individuals for a prolonged period of time.[ 1 , 7 , 30 ] The scope of ethnography can be broad or narrow depending on the aim. The study of more general cultural groups is termed as macro-ethnography, whereas micro-ethnography focuses on more narrowly defined cultures. Ethnography is usually conducted in a single setting. Ethnographers collect data using a variety of methods such as observation, interviews, audio-video records, and document reviews. A written report includes a detailed description of the culture sharing group with emic and etic perspectives. When the researcher reports the views of the participants it is called emic perspectives and when the researcher reports his or her views about the culture, the term is called etic.[ 7 ]
Example: The aim of the ethnographic study by LeBaron et al . was to explore the barriers to opioid availability and cancer pain management in India. The researchers collected data from fifty-nine participants using in-depth semi-structured interviews, participant observation, and document review. The researchers identified significant barriers by open coding and thematic analysis of the formal interview. [ 31 ]
Historical research
Historical research is the “systematic collection, critical evaluation, and interpretation of historical evidence”.[ 1 ] The purpose of historical research is to gain insights from the past and involves interpreting past events in the light of the present. The data for historical research are usually collected from primary and secondary sources. The primary source mainly includes diaries, first hand information, and writings. The secondary sources are textbooks, newspapers, second or third-hand accounts of historical events and medical/legal documents. The data gathered from these various sources are synthesized and reported as biographical narratives or developmental perspectives in chronological order. The ideas are interpreted in terms of the historical context and significance. The written report describes 'what happened', 'how it happened', 'why it happened', and its significance and implications to current clinical practice.[ 1 , 10 ]
Example: Lubold (2019) analyzed the breastfeeding trends in three countries (Sweden, Ireland, and the United States) using a historical qualitative method. Through analysis of historical data, the researcher found that strong family policies, adherence to international recommendations and adoption of baby-friendly hospital initiative could greatly enhance the breastfeeding rates. [ 32 ]
Case study research
Case study research focuses on the description and in-depth analysis of the case(s) or issues illustrated by the case(s). The design has its origin from psychology, law, and medicine. Case studies are best suited for the understanding of case(s), thus reducing the unit of analysis into studying an event, a program, an activity or an illness. Observations, one to one interviews, artifacts, and documents are used for collecting the data, and the analysis is done through the description of the case. From this, themes and cross-case themes are derived. A written case study report includes a detailed description of one or more cases.[ 7 , 10 ]
Example: Perceptions of poststroke sexuality in a woman of childbearing age was explored using a qualitative case study approach by Beal and Millenbrunch. Semi structured interview was conducted with a 36- year mother of two children with a history of Acute ischemic stroke. The data were analyzed using an inductive approach. The authors concluded that “stroke during childbearing years may affect a woman's perception of herself as a sexual being and her ability to carry out gender roles”. [ 33 ]
Sampling in Qualitative Research
Qualitative researchers widely use non-probability sampling techniques such as purposive sampling, convenience sampling, quota sampling, snowball sampling, homogeneous sampling, maximum variation sampling, extreme (deviant) case sampling, typical case sampling, and intensity sampling. The selection of a sampling technique depends on the nature and needs of the study.[ 34 , 35 , 36 , 37 , 38 , 39 , 40 ] The four widely used sampling techniques are convenience sampling, purposive sampling, snowball sampling, and intensity sampling.
Convenience sampling
It is otherwise called accidental sampling, where the researchers collect data from the subjects who are selected based on accessibility, geographical proximity, ease, speed, and or low cost.[ 34 ] Convenience sampling offers a significant benefit of convenience but often accompanies the issues of sample representation.
Purposive sampling
Purposive or purposeful sampling is a widely used sampling technique.[ 35 ] It involves identifying a population based on already established sampling criteria and then selecting subjects who fulfill that criteria to increase the credibility. However, choosing information-rich cases is the key to determine the power and logic of purposive sampling in a qualitative study.[ 1 ]
Snowball sampling
The method is also known as 'chain referral sampling' or 'network sampling.' The sampling starts by having a few initial participants, and the researcher relies on these early participants to identify additional study participants. It is best adopted when the researcher wishes to study the stigmatized group, or in cases, where findings of participants are likely to be difficult by ordinary means. Respondent ridden sampling is an improvised version of snowball sampling used to find out the participant from a hard-to-find or hard-to-study population.[ 37 , 38 ]
Intensity sampling
The process of identifying information-rich cases that manifest the phenomenon of interest is referred to as intensity sampling. It requires prior information, and considerable judgment about the phenomenon of interest and the researcher should do some preliminary investigations to determine the nature of the variation. Intensity sampling will be done once the researcher identifies the variation across the cases (extreme, average and intense) and picks the intense cases from them.[ 40 ]
Deciding the Sample Size
A-priori sample size calculation is not undertaken in the case of qualitative research. Researchers collect the data from as many participants as possible until they reach the point of data saturation. Data saturation or the point of redundancy is the stage where the researcher no longer sees or hears any new information. Data saturation gives the idea that the researcher has captured all possible information about the phenomenon of interest. Since no further information is being uncovered as redundancy is achieved, at this point the data collection can be stopped. The objective here is to get an overall picture of the chronicle of the phenomenon under the study rather than generalization.[ 1 , 7 , 41 ]
Data Collection in Qualitative Research
The various strategies used for data collection in qualitative research includes in-depth interviews (individual or group), focus group discussions (FGDs), participant observation, narrative life history, document analysis, audio materials, videos or video footage, text analysis, and simple observation. Among all these, the three popular methods are the FGDs, one to one in-depth interviews and the participant observation.
FGDs are useful in eliciting data from a group of individuals. They are normally built around a specific topic and are considered as the best approach to gather data on an entire range of responses to a topic.[ 42 Group size in an FGD ranges from 6 to 12. Depending upon the nature of participants, FGDs could be homogeneous or heterogeneous.[ 1 , 14 ] One to one in-depth interviews are best suited to obtain individuals' life histories, lived experiences, perceptions, and views, particularly while exporting topics of sensitive nature. In-depth interviews can be structured, unstructured, or semi-structured. However, semi-structured interviews are widely used in qualitative research. Participant observations are suitable for gathering data regarding naturally occurring behaviors.[ 1 ]
Data Analysis in Qualitative Research
Various strategies are employed by researchers to analyze data in qualitative research. Data analytic strategies differ according to the type of inquiry. A general content analysis approach is described herewith. Data analysis begins by transcription of the interview data. The researcher carefully reads data and gets a sense of the whole. Once the researcher is familiarized with the data, the researcher strives to identify small meaning units called the 'codes.' The codes are then grouped based on their shared concepts to form the primary categories. Based on the relationship between the primary categories, they are then clustered into secondary categories. The next step involves the identification of themes and interpretation to make meaning out of data. In the results section of the manuscript, the researcher describes the key findings/themes that emerged. The themes can be supported by participants' quotes. The analytical framework used should be explained in sufficient detail, and the analytic framework must be well referenced. The study findings are usually represented in a schematic form for better conceptualization.[ 1 , 7 ] Even though the overall analytical process remains the same across different qualitative designs, each design such as phenomenology, ethnography, and grounded theory has design specific analytical procedures, the details of which are out of the scope of this article.
Computer-Assisted Qualitative Data Analysis Software (CAQDAS)
Until recently, qualitative analysis was done either manually or with the help of a spreadsheet application. Currently, there are various software programs available which aid researchers to manage qualitative data. CAQDAS is basically data management tools and cannot analyze the qualitative data as it lacks the ability to think, reflect, and conceptualize. Nonetheless, CAQDAS helps researchers to manage, shape, and make sense of unstructured information. Open Code, MAXQDA, NVivo, Atlas.ti, and Hyper Research are some of the widely used qualitative data analysis software.[ 14 , 43 ]
Reporting Guidelines
Consolidated Criteria for Reporting Qualitative Research (COREQ) is the widely used reporting guideline for qualitative research. This 32-item checklist assists researchers in reporting all the major aspects related to the study. The three major domains of COREQ are the 'research team and reflexivity', 'study design', and 'analysis and findings'.[ 44 , 45 ]
Critical Appraisal of Qualitative Research
Various scales are available to critical appraisal of qualitative research. The widely used one is the Critical Appraisal Skills Program (CASP) Qualitative Checklist developed by CASP network, UK. This 10-item checklist evaluates the quality of the study under areas such as aims, methodology, research design, ethical considerations, data collection, data analysis, and findings.[ 46 ]
Ethical Issues in Qualitative Research
A qualitative study must be undertaken by grounding it in the principles of bioethics such as beneficence, non-maleficence, autonomy, and justice. Protecting the participants is of utmost importance, and the greatest care has to be taken while collecting data from a vulnerable research population. The researcher must respect individuals, families, and communities and must make sure that the participants are not identifiable by their quotations that the researchers include when publishing the data. Consent for audio/video recordings must be obtained. Approval to be in FGDs must be obtained from the participants. Researchers must ensure the confidentiality and anonymity of the transcripts/audio-video records/photographs/other data collected as a part of the study. The researchers must confirm their role as advocates and proceed in the best interest of all participants.[ 42 , 47 , 48 ]
Rigor in Qualitative Research
The demonstration of rigor or quality in the conduct of the study is essential for every research method. However, the criteria used to evaluate the rigor of quantitative studies are not be appropriate for qualitative methods. Lincoln and Guba (1985) first outlined the criteria for evaluating the qualitative research often referred to as “standards of trustworthiness of qualitative research”.[ 49 ] The four components of the criteria are credibility, transferability, dependability, and confirmability.
Credibility refers to confidence in the 'truth value' of the data and its interpretation. It is used to establish that the findings are true, credible and believable. Credibility is similar to the internal validity in quantitative research.[ 1 , 50 , 51 ] The second criterion to establish the trustworthiness of the qualitative research is transferability, Transferability refers to the degree to which the qualitative results are applicability to other settings, population or contexts. This is analogous to the external validity in quantitative research.[ 1 , 50 , 51 ] Lincoln and Guba recommend authors provide enough details so that the users will be able to evaluate the applicability of data in other contexts.[ 49 ] The criterion of dependability refers to the assumption of repeatability or replicability of the study findings and is similar to that of reliability in quantitative research. The dependability question is 'Whether the study findings be repeated of the study is replicated with the same (similar) cohort of participants, data coders, and context?'[ 1 , 50 , 51 ] Confirmability, the fourth criteria is analogous to the objectivity of the study and refers the degree to which the study findings could be confirmed or corroborated by others. To ensure confirmability the data should directly reflect the participants' experiences and not the bias, motivations, or imaginations of the inquirer.[ 1 , 50 , 51 ] Qualitative researchers should ensure that the study is conducted with enough rigor and should report the measures undertaken to enhance the trustworthiness of the study.
Conclusions
Qualitative research studies are being widely acknowledged and recognized in health care practice. This overview illustrates various qualitative methods and shows how these methods can be used to generate evidence that informs clinical practice. Qualitative research helps to understand the patterns of health behaviors, describe illness experiences, design health interventions, and develop healthcare theories. The ultimate strength of the qualitative research approach lies in the richness of the data and the descriptions and depth of exploration it makes. Hence, qualitative methods are considered as the most humanistic and person-centered way of discovering and uncovering thoughts and actions of human beings.
Financial support and sponsorship
Conflicts of interest.
There are no conflicts of interest.
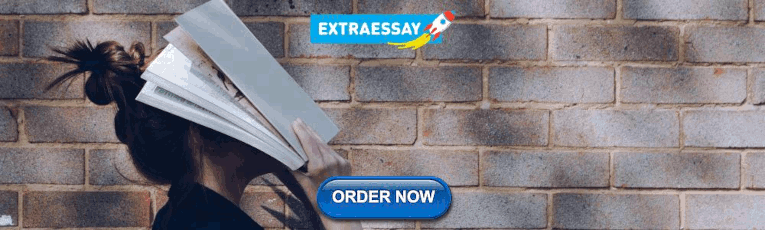
IMAGES
VIDEO
COMMENTS
1. BACKGROUND. Public and patient involvement (PPI) in health research has been defined as research being carried out "with" or "by" members of the public rather than "to," "about" or "for" them. 1 PPI covers a diverse range of approaches from "one off" information gathering to sustained partnerships. Tritter's conceptual framework for PPI distinguished between indirect ...
Background Identifying determinants of health and understanding their role in health production constitutes an important research theme. We aimed to document the state of recent multi-country research on this theme in the literature. Methods We followed the PRISMA-ScR guidelines to systematically identify, triage and review literature (January 2013—July 2019).
INTRODUCTION. Scientific research is usually initiated by posing evidenced-based research questions which are then explicitly restated as hypotheses.1,2 The hypotheses provide directions to guide the study, solutions, explanations, and expected results.3,4 Both research questions and hypotheses are essentially formulated based on conventional theories and real-world processes, which allow the ...
Many studies referred to the integration of qualitative and quantitative techniques as one of Q-methodology's key strengths, ... Studies using Q-methodology in healthcare research have increased over the past 5 years, suggesting a growing interest and acceptance of what was once considered a niche psychological tool . However, the use of Q ...
The complexity of public health interventions create challenges in evaluating their effectiveness. There have been huge advancements in quantitative evidence synthesis methods development (including meta-analysis) for dealing with heterogeneity of intervention effects, inappropriate 'lumping' of interventions, adjusting for different populations and outcomes and the inclusion of various ...
This article describes the basic tenets of quantitative research. The concepts of dependent and independent variables are addressed and the concept of measurement and its associated issues, such as error, reliability and validity, are explored. Experiments and surveys - the principal research designs in quantitative research - are described ...
This paper gives an overview of the main quantitative research designs relevant to nursing and healthcare. It outlines some strengths and weaknesses of the designs, provides examples to illustrate the different designs and examines some of the relevant statistical concepts.
Abstract. Quantitative research is the foundation for evidence-based global health practice and interventions. Preparing health research starts with a clear research question to initiate the study, careful planning using sound methodology as well as the development and management of the capacity and resources to complete the whole research cycle.
Quantitative Methods for Health Research, Second Edition is a practical learning resource for students, practitioners and researchers in public health, health care and related disciplines, providing both a course book and a useful introductory reference.
We use a range of data in order to fulfil this need, both quantitative and qualitative. Data are defined as "information, especially facts and numbers, collected to be examined and considered and used to help decision-making." 1 Data are used to make judgements, to answer questions, and to monitor and support improvement in healthcare ( box ...
The ability to critique research in a systematic way is an essential component of a health professional's role in order to deliver high quality, evidence-based healthcare. This chapter is intended to provide a simple overview of the way new researchers and health practitioners can understand and employ quantitative methods.
The Quantitative Methods (QM) field of study provides students with the necessary quantitative and analytical skills to approach and solve problems in public health and clinical research and practice. This field is designed for mid-career health professionals, research scientists, and MD/MPH specific dual/joint-degree students.
Quantitative research methods focus on variables that can be measured numerically and analyzed using statistical techniques [1,2,3].The primary advantage of this approach is its ability to investigate relationships between variables within a sample that can then be inferred to a larger population of interest while more consistently controlling for threats to validity.
Quantitative data uses numbers to determine the what, who, when, and where of health-related events (Wang, 2013). Examples of quantitative data include: age, weight, temperature, or the number of people suffering from diabetes. Qualitative Data. Qualitative data is a broad category of data that can include almost any non-numerical data.
Some nurses feel that they lack the necessary skills to read a research paper and to then decide if they should implement the findings into their practice. This is particularly the case when considering the results of quantitative research, which often contains the results of statistical testing. However, nurses have a professional responsibility to critique research to improve their practice ...
This publication is designed to help provide practicing health educators with basic tools helpful to facilitate a better understanding of quantitative research. This article describes the major components—title, introduction, methods, analyses, results and discussion sections—of quantitative research. Readers will be introduced to ...
Quantitative research methods are often used in public health research and evaluation to determine the needs of a community or population, examine associations among multiple factors, and compare outcomes across subpopulations based on demographic characteristics (e.g., age, gender, race, education, income). Quantitative data and analysis can ...
Combining quantitative and qualitative methods in a single study is not uncommon in social research, although, 'traditionally a gulf is seen to exist between qualitative and quantitative research with each belonging to distinctively different paradigms'. [] Within health research there has, more recently, been an upsurge of interest in the combined use of qualitative and quantitative methods ...
Quantitative analysis refers to the process of using complex mathematical or statistical modeling to make sense of data and potentially to predict behavior. Though quantitative analysis is well-established in the fields of economics and finance, cutting-edge quantitative analysis has only recently become possible in health care.
Health Research: Quantitative research is used in health research to study the effectiveness of medical treatments, identify risk factors for diseases, and track health outcomes over time. Researchers use statistical methods to analyze data from clinical trials, surveys, and other sources to inform medical practice and policy. ...
Quantitative research uses numerical data and statistical tools to test the effects of drugs or treatments on health outcomes. It can produce reliable and generalizable results, but also faces the criticism of reductionism and lack of qualitative perspective.
Quantitative research objectives can be to establish the incidence or prevalence of a health problem; the health personnel degree of adherence to a new intervention; or, the users' level of satisfaction with a service. Qualitative research aims at understanding what exists from social actors' perspectives.
Data extraction. The research team developed an extraction form for the included studies utilizing an Excel spreadsheet. Following data extraction, the information included the Name of Author(s) Year of publication, Country/countries, Title of the article, Setting, Aim, Design, Participants, and Sample size of the studies, Technology utilized in healthcare services, name/title utilized to ...
When it comes to healthcare and specifically health insurance, risk is often a large contributing factor in how patients access care. The following are a few examples of companies using big data to gain more insight into risk and ensure accuracy in adjustments. View Profile. Location: Fully Remote.
Volatile organic compound (VOC) emissions have attracted wide attention due to their impacts on atmospheric quality and public health. However, most studies reviewed certain aspects of natural VOCs (NVOCs) or anthropogenic VOCs (AVOCs) rather than comprehensively quantifying the hotspots and evolution trends of AVOCs and NVOCs. We combined the bibliometric method with the evolution tree and ...
Significance of Qualitative Research. The qualitative method of inquiry examines the 'how' and 'why' of decision making, rather than the 'when,' 'what,' and 'where.'[] Unlike quantitative methods, the objective of qualitative inquiry is to explore, narrate, and explain the phenomena and make sense of the complex reality.Health interventions, explanatory health models, and medical-social ...