Thank you for visiting nature.com. You are using a browser version with limited support for CSS. To obtain the best experience, we recommend you use a more up to date browser (or turn off compatibility mode in Internet Explorer). In the meantime, to ensure continued support, we are displaying the site without styles and JavaScript.
- View all journals
- My Account Login
- Explore content
- About the journal
- Publish with us
- Sign up for alerts
- Open access
- Published: 10 October 2022
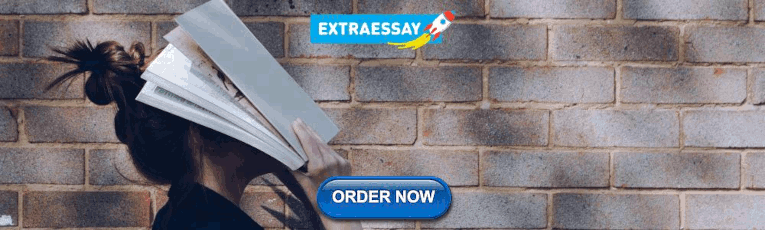
Health effects associated with smoking: a Burden of Proof study
- Xiaochen Dai ORCID: orcid.org/0000-0002-0289-7814 1 , 2 ,
- Gabriela F. Gil 1 ,
- Marissa B. Reitsma 1 ,
- Noah S. Ahmad 1 ,
- Jason A. Anderson 1 ,
- Catherine Bisignano 1 ,
- Sinclair Carr 1 ,
- Rachel Feldman 1 ,
- Simon I. Hay ORCID: orcid.org/0000-0002-0611-7272 1 , 2 ,
- Jiawei He 1 , 2 ,
- Vincent Iannucci 1 ,
- Hilary R. Lawlor 1 ,
- Matthew J. Malloy 1 ,
- Laurie B. Marczak 1 ,
- Susan A. McLaughlin 1 ,
- Larissa Morikawa ORCID: orcid.org/0000-0001-9749-8033 1 ,
- Erin C. Mullany 1 ,
- Sneha I. Nicholson 1 ,
- Erin M. O’Connell 1 ,
- Chukwuma Okereke 1 ,
- Reed J. D. Sorensen 1 ,
- Joanna Whisnant 1 ,
- Aleksandr Y. Aravkin 1 , 3 ,
- Peng Zheng 1 , 2 ,
- Christopher J. L. Murray ORCID: orcid.org/0000-0002-4930-9450 1 , 2 &
- Emmanuela Gakidou ORCID: orcid.org/0000-0002-8992-591X 1 , 2
Nature Medicine volume 28 , pages 2045–2055 ( 2022 ) Cite this article
24k Accesses
38 Citations
169 Altmetric
Metrics details
- Risk factors
Matters Arising to this article was published on 14 April 2023
As a leading behavioral risk factor for numerous health outcomes, smoking is a major ongoing public health challenge. Although evidence on the health effects of smoking has been widely reported, few attempts have evaluated the dose–response relationship between smoking and a diverse range of health outcomes systematically and comprehensively. In the present study, we re-estimated the dose–response relationships between current smoking and 36 health outcomes by conducting systematic reviews up to 31 May 2022, employing a meta-analytic method that incorporates between-study heterogeneity into estimates of uncertainty. Among the 36 selected outcomes, 8 had strong-to-very-strong evidence of an association with smoking, 21 had weak-to-moderate evidence of association and 7 had no evidence of association. By overcoming many of the limitations of traditional meta-analyses, our approach provides comprehensive, up-to-date and easy-to-use estimates of the evidence on the health effects of smoking. These estimates provide important information for tobacco control advocates, policy makers, researchers, physicians, smokers and the public.
Similar content being viewed by others
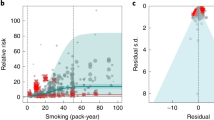
The Burden of Proof studies: assessing the evidence of risk
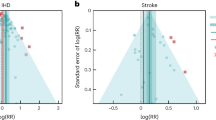
Health effects associated with exposure to secondhand smoke: a Burden of Proof study
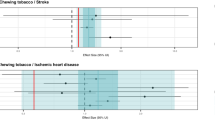
Health effects associated with chewing tobacco: a Burden of Proof study
Among both the public and the health experts, smoking is recognized as a major behavioral risk factor with a leading attributable health burden worldwide. The health risks of smoking were clearly outlined in a canonical study of disease rates (including lung cancer) and smoking habits in British doctors in 1950 and have been further elaborated in detail over the following seven decades 1 , 2 . In 2005, evidence of the health consequences of smoking galvanized the adoption of the first World Health Organization (WHO) treaty, the Framework Convention on Tobacco Control, in an attempt to drive reductions in global tobacco use and second-hand smoke exposure 3 . However, as of 2020, an estimated 1.18 billion individuals globally were current smokers and 7 million deaths and 177 million disability-adjusted life-years were attributed to smoking, reflecting a persistent public health challenge 4 . Quantifying the relationship between smoking and various important health outcomes—in particular, highlighting any significant dose–response relationships—is crucial to understanding the attributable health risk experienced by these individuals and informing responsive public policy.
Existing literature on the relationship between smoking and specific health outcomes is prolific, including meta-analyses, cohort studies and case–control studies analyzing the risk of outcomes such as lung cancer 5 , 6 , 7 , chronic obstructive pulmonary disease (COPD) 8 , 9 , 10 and ischemic heart disease 11 , 12 , 13 , 14 due to smoking. There are few if any attempts, however, to systematically and comprehensively evaluate the landscape of evidence on smoking risk across a diverse range of health outcomes, with most current research focusing on risk or attributable burden of smoking for a specific condition 7 , 15 , thereby missing the opportunity to provide a comprehensive picture of the health risk experienced by smokers. Furthermore, although evidence surrounding specific health outcomes, such as lung cancer, has generated widespread consensus, findings about the attributable risk of other outcomes are much more heterogeneous and inconclusive 16 , 17 , 18 . These studies also vary in their risk definitions, with many comparing dichotomous exposure measures of ever smokers versus nonsmokers 19 , 20 . Others examine the distinct risks of current smokers and former smokers compared with never smokers 21 , 22 , 23 . Among the studies that do analyze dose–response relationships, there is large variation in the units and dose categories used in reporting their findings (for example, the use of pack-years or cigarettes per day) 24 , 25 , which complicates the comparability and consolidation of evidence. This, in turn, can obscure data that could inform personal health choices, public health practices and policy measures. Guidance on the health risks of smoking, such as the Surgeon General’s Reports on smoking 26 , 27 , is often based on experts’ evaluation of heterogenous evidence, which, although extremely useful and well suited to carefully consider nuances in the evidence, is fundamentally subjective.
The present study, as part of the Global Burden of Diseases, Risk Factors, and Injuries Study (GBD) 2020, re-estimated the continuous dose–response relationships (the mean risk functions and associated uncertainty estimates) between current smoking and 36 health outcomes (Supplementary Table 1 ) by identifying input studies using a systematic review approach and employing a meta-analytic method 28 . The 36 health outcomes that were selected based on existing evidence of a relationship included 16 cancers (lung cancer, esophageal cancer, stomach cancer, leukemia, liver cancer, laryngeal cancer, breast cancer, cervical cancer, colorectal cancer, lip and oral cavity cancer, nasopharyngeal cancer, other pharynx cancer (excluding nasopharynx cancer), pancreatic cancer, bladder cancer, kidney cancer and prostate cancer), 5 cardiovascular diseases (CVDs: ischemic heart disease, stroke, atrial fibrillation and flutter, aortic aneurysm and peripheral artery disease) and 15 other diseases (COPD, lower respiratory tract infections, tuberculosis, asthma, type 2 diabetes, Alzheimer’s disease and related dementias, Parkinson’s disease, multiple sclerosis, cataracts, gallbladder diseases, low back pain, peptic ulcer disease, rheumatoid arthritis, macular degeneration and fractures). Definitions of the outcomes are described in Supplementary Table 1 . We conducted a separate systematic review for each risk–outcome pair with the exception of cancers, which were done together in a single systematic review. This approach allowed us to systematically identify all relevant studies indexed in PubMed up to 31 May 2022, and we extracted relevant data on risk of smoking, including study characteristics, following a pre-specified template (Supplementary Table 2 ). The meta-analytic tool overcomes many of the limitations of traditional meta-analyses by incorporating between-study heterogeneity into the uncertainty of risk estimates, accounting for small numbers of studies, relaxing the assumption of log(linearity) applied to the risk functions, handling differences in exposure ranges between comparison groups, and systematically testing and adjusting for bias due to study designs and characteristics. We then estimated the burden-of-proof risk function (BPRF) for each risk–outcome pair, as proposed by Zheng et al. 29 ; the BPRF is a conservative risk function defined as the 5th quantile curve (for harmful risks) that reflects the smallest harmful effect at each level of exposure consistent with the available evidence. Given all available data for each outcome, the risk of smoking is at least as harmful as the BPRF indicates.
We used the BPRF for each risk–outcome pair to calculate risk–outcome scores (ROSs) and categorize the strength of evidence for the association between smoking and each health outcome using a star rating from 1 to 5. The interpretation of the star ratings is as follows: 1 star (*) indicates no evidence of association; 2 stars (**) correspond to a 0–15% increase in risk across average range of exposures for harmful risks; 3 stars (***) represent a 15–50% increase in risk; 4 stars (****) refer to >50–85% increase in risk; and 5 stars (*****) equal >85% increase in risk. The thresholds for each star rating were developed in consultation with collaborators and other stakeholders.
The increasing disease burden attributable to current smoking, particularly in low- and middle-income countries 4 , demonstrates the relevance of the present study, which quantifies the strength of the evidence using an objective, quantitative, comprehensive and comparative framework. Findings from the present study can be used to support policy makers in making informed smoking recommendations and regulations focusing on the associations for which the evidence is strongest (that is, the 4- and 5-star associations). However, associations with a lower star rating cannot be ignored, especially when the outcome has high prevalence or severity. A summary of the main findings, limitations and policy implications of the study is presented in Table 1 .
We evaluated the mean risk functions and the BPRFs for 36 health outcomes that are associated with current smoking 30 (Table 2 ). Following the Preferred Reporting Items for Systematic Reviews and Meta-Analyses (PRISMA) guidelines 31 for each of our systematic reviews, we identified studies reporting relative risk (RR) of incidence or mortality from each of the 36 selected outcomes for smokers compared with nonsmokers. We reviewed 21,108 records, which were identified to have been published between 1 May 2018 and 31 May 2022; this represents the most recent time period since the last systematic review of the available evidence for the GBD at the time of publication. The meta-analyses reported in the present study for each of the 36 health outcomes are based on evidence from a total of 793 studies published between 1970 and 2022 (Extended Data Fig. 1 – 5 and Supplementary Information 1.5 show the PRISMA diagrams for each outcome). Only prospective cohort and case–control studies were included for estimating dose–response risk curves, but cross-sectional studies were also included for estimating the age pattern of smoking risk on cardiovascular and circulatory disease (CVD) outcomes. Details on each, including the study’s design, data sources, number of participants, length of follow-up, confounders adjusted for in the input data and bias covariates included in the dose–response risk model, can be found in Supplementary Information 2 and 3 . The theoretical minimum risk exposure level used for current smoking was never smoking or zero 30 .
Five-star associations
When the most conservative interpretation of the evidence, that is, the BPRF, suggests that the average exposure (15th–85th percentiles of exposure) of smoking increases the risk of a health outcome by >85% (that is, ROS > 0.62), smoking and that outcome are categorized as a 5-star pair. Among the 36 outcomes, there are 5 that have a 5-star association with current smoking: laryngeal cancer (375% increase in risk based on the BPRF, 1.56 ROS), aortic aneurysm (150%, 0.92), peripheral artery disease (137%, 0.86), lung cancer (107%, 0.73) and other pharynx cancer (excluding nasopharynx cancer) (92%, 0.65).
Results for all 5-star risk–outcome pairs are available in Table 2 and Supplementary Information 4.1 . In the present study, we provide detailed results for one example 5-star association: current smoking and lung cancer. We extracted 371 observations from 25 prospective cohort studies and 53 case–control studies across 25 locations (Supplementary Table 3 ) 5 , 6 , 32 , 33 , 34 , 35 , 36 , 37 , 38 , 39 , 40 , 41 , 42 , 43 , 44 , 45 , 46 , 47 , 48 , 49 , 50 , 51 , 52 , 53 , 54 , 55 , 56 , 57 , 58 , 59 , 60 , 61 , 62 , 63 , 64 , 65 , 66 , 67 , 68 , 69 , 70 , 71 , 72 , 73 , 74 , 75 , 76 , 77 , 78 , 79 , 80 , 81 , 82 , 83 , 84 , 85 , 86 , 87 , 88 , 89 , 90 , 91 , 92 , 93 , 94 , 95 , 96 , 97 , 98 , 99 , 100 , 101 , 102 , 103 , 104 , 105 , 106 , 107 . Exposure ranged from 1 pack-year to >112 pack-years, with the 85th percentile of exposure being 50.88 pack-years (Fig. 1a ).
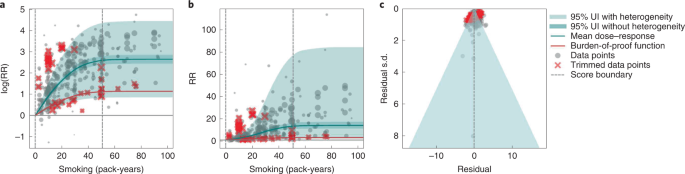
a , The log(RR) function. b , RR function. c , A modified funnel plot showing the residuals (relative to 0) on the x axis and the estimated s.d. that includes reported s.d. and between-study heterogeneity on the y axis.
We found a very strong and significant harmful relationship between pack-years of current smoking and the RR of lung cancer (Fig. 1b ). The mean RR of lung cancer at 20 pack-years of smoking was 5.11 (95% uncertainty interval (UI) inclusive of between-study heterogeneity = 1.84–14.99). At 50.88 pack-years (85th percentile of exposure), the mean RR of lung cancer was 13.42 (2.63–74.59). See Table 2 for mean RRs at other exposure levels. The BPRF, which represents the most conservative interpretation of the evidence (Fig. 1a ), suggests that smoking in the 15th–85th percentiles of exposure increases the risk of lung cancer by an average of 107%, yielding an ROS of 0.73.
The relationship between pack-years of current smoking and RR of lung cancer is nonlinear, with diminishing impact of further pack-years of smoking, particularly for middle-to-high exposure levels (Fig. 1b ). To reduce the effect of bias, we adjusted observations that did not account for more than five confounders, including age and sex, because they were the significant bias covariates identified by the bias covariate selection algorithm 29 (Supplementary Table 7 ). The reported RRs across studies were very heterogeneous. Our meta-analytic method, which accounts for the reported uncertainty in both the data and between-study heterogeneity, fit the data and covered the estimated residuals well (Fig. 1c ). After trimming 10% of outliers, we still detected publication bias in the results for lung cancer. See Supplementary Tables 4 and 7 for study bias characteristics and selected bias covariates, Supplementary Fig. 5 for results without 10% trimming and Supplementary Table 8 for observed RR data and alternative exposures across studies for the remaining 5-star pairs.
Four-star associations
When the BPRF suggests that the average exposure of smoking increases the risk of a health outcome by 50–85% (that is, ROS > 0.41–0.62), smoking is categorized as having a 4-star association with that outcome. We identified three outcomes with a 4-star association with smoking: COPD (72% increase in risk based on the BPRF, 0.54 ROS), lower respiratory tract infection (54%, 0.43) and pancreatic cancer (52%, 0.42).
In the present study, we provide detailed results for one example 4-star association: current smoking and COPD. We extracted 51 observations from 11 prospective cohort studies and 4 case–control studies across 36 locations (Supplementary Table 3 ) 6 , 8 , 9 , 10 , 78 , 108 , 109 , 110 , 111 , 112 , 113 , 114 , 115 , 116 . Exposure ranged from 1 pack-year to 100 pack-years, with the 85th percentile of exposure in the exposed group being 49.75 pack-years.
We found a strong and significant harmful relationship between pack-years of current smoking and RR of COPD (Fig. 2b ). The mean RR of COPD at 20 pack-years was 3.17 (1.60–6.55; Table 2 reports RRs at other exposure levels). At the 85th percentile of exposure, the mean RR of COPD was 6.01 (2.08–18.58). The BPRF suggests that average smoking exposure raises the risk of COPD by an average of 72%, yielding an ROS of 0.54. The results for the other health outcomes that have an association with smoking rated as 4 stars are shown in Table 2 and Supplementary Information 4.2 .
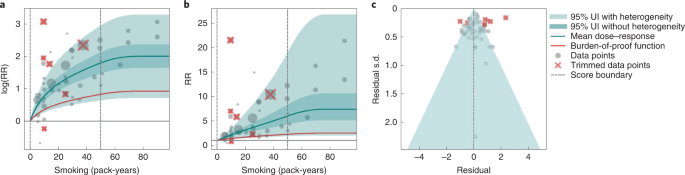
a , The log(RR) function. b , RR function. c , A modified funnel plot showing the residuals (relative to 0) on th e x axis and the estimated s.d. that includes the reported s.d. and between-study heterogeneity on the y axis.
The relationship between smoking and COPD is nonlinear, with diminishing impact of further pack-years of current smoking on risk of COPD, particularly for middle-to-high exposure levels (Fig. 2a ). To reduce the effect of bias, we adjusted observations that did not account for age and sex and/or were generated for individuals aged >65 years 116 , because they were the two significant bias covariates identified by the bias covariate selection algorithm (Supplementary Table 7 ). There was large heterogeneity in the reported RRs across studies, and our meta-analytic method fit the data and covered the estimated residuals well (Fig. 2b ). Although we trimmed 10% of outliers, publication bias was still detected in the results for COPD. See Supplementary Tables 4 and 7 for study bias characteristics and selected bias covariates, Supplementary Fig. 5 for results without 10% trimming and Supplementary Table 8 for reported RR data and alternative exposures across studies for the remaining health outcomes that have a 4-star association with smoking.
Three-star associations
When the BPRF suggests that the average exposure of smoking increases the risk of a health outcome by 15–50% (or, when protective, decreases the risk of an outcome by 13–34%; that is, ROS >0.14–0.41), the association between smoking and that outcome is categorized as having a 3-star rating. We identified 15 outcomes with a 3-star association: bladder cancer (40% increase in risk, 0.34 ROS); tuberculosis (31%, 0.27); esophageal cancer (29%, 0.26); cervical cancer, multiple sclerosis and rheumatoid arthritis (each 23–24%, 0.21); lower back pain (22%, 0.20); ischemic heart disease (20%, 0.19); peptic ulcer and macular degeneration (each 19–20%, 0.18); Parkinson's disease (protective risk, 15% decrease in risk, 0.16); and stomach cancer, stroke, type 2 diabetes and cataracts (each 15–17%, 0.14–0.16).
We present the findings on smoking and type 2 diabetes as an example of a 3-star risk association. We extracted 102 observations from 24 prospective cohort studies and 4 case–control studies across 15 locations (Supplementary Table 3 ) 117 , 118 , 119 , 120 , 121 , 122 , 123 , 124 , 125 , 126 , 127 , 128 , 129 , 130 , 131 , 132 , 133 , 134 , 135 , 136 , 137 , 138 , 139 , 140 , 141 , 142 , 143 , 144 . The exposure ranged from 1 cigarette to 60 cigarettes smoked per day, with the 85th percentile of exposure in the exposed group being 26.25 cigarettes smoked per day.
We found a moderate and significant harmful relationship between cigarettes smoked per day and the RR of type 2 diabetes (Fig. 3b ). The mean RR of type 2 diabetes at 20 cigarettes smoked per day was 1.49 (1.18–1.90; see Table 2 for other exposure levels). At the 85th percentile of exposure, the mean RR of type 2 diabetes was 1.54 (1.20–2.01). The BPRF suggests that average smoking exposure raises the risk of type 2 diabetes by an average of 16%, yielding an ROS of 0.15. See Table 2 and Supplementary Information 4.3 for results for the additional health outcomes with an association with smoking rated as 3 stars.
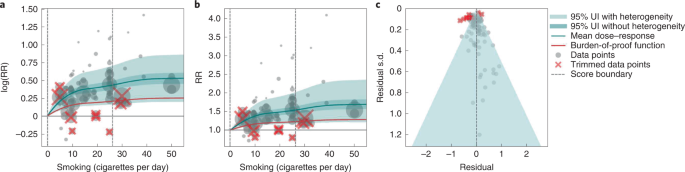
a , The log(RR) function. b , RR function. c , A modified funnel plot showing the residuals (relative to 0) on the x axis and the estimated s.d. that includes the reported s.d. and between-study heterogeneity on the y axis.
The relationship between smoking and type 2 diabetes is nonlinear, particularly for high exposure levels where the mean risk curve becomes flat (Fig. 3a ). We adjusted observations that were generated in subpopulations, because it was the only significant bias covariate identified by the bias covariate selection algorithm (Supplementary Table 7 ). There was moderate heterogeneity in the observed RR data across studies and our meta-analytic method fit the data and covered the estimated residuals extremely well (Fig. 3b,c ). After trimming 10% of outliers, we still detected publication bias in the results for type 2 diabetes. See Supplementary Tables 4 and 7 for study bias characteristics and selected bias covariates, Supplementary Fig. 5 for results without 10% trimming and Supplementary Table 8 for observed RR data and alternative exposures across studies for the remaining 3-star pairs.
Two-star associations
When the BPRF suggests that the average exposure of smoking increases the risk of an outcome by 0–15% (that is, ROS 0.0–0.14), the association between smoking and that outcome is categorized as a 2-star rating. We identified six 2-star outcomes: nasopharyngeal cancer (14% increase in risk, 0.13 ROS); Alzheimer’s and other dementia (10%, 0.09); gallbladder diseases and atrial fibrillation and flutter (each 6%, 0.06); lip and oral cavity cancer (5%, 0.05); and breast cancer (4%, 0.04).
We present the findings on smoking and breast cancer as an example of a 2-star association. We extracted 93 observations from 14 prospective cohort studies and 9 case–control studies across 14 locations (Supplementary Table 3 ) 84 , 87 , 145 , 146 , 147 , 148 , 149 , 150 , 151 , 152 , 153 , 154 , 155 , 156 , 157 , 158 , 159 , 160 , 161 , 162 , 163 , 164 , 165 . The exposure ranged from 1 cigarette to >76 cigarettes smoked per day, with the 85th percentile of exposure in the exposed group being 34.10 cigarettes smoked per day.
We found a weak but significant relationship between pack-years of current smoking and RR of breast cancer (Extended Data Fig. 6 ). The mean RR of breast cancer at 20 pack-years was 1.17 (1.04–1.31; Table 2 reports other exposure levels). The BPRF suggests that average smoking exposure raises the risk of breast cancer by an average of 4%, yielding an ROS of 0.04. See Table 2 and Supplementary Information 4.4 for results on the additional health outcomes for which the association with smoking has been categorized as 2 stars.
The relationship between smoking and breast cancer is nonlinear, particularly for high exposure levels where the mean risk curve becomes flat (Extended Data Fig. 6a ). To reduce the effect of bias, we adjusted observations that were generated in subpopulations, because it was the only significant bias covariate identified by the bias covariate selection algorithm (Supplementary Table 7 ). There was heterogeneity in the reported RRs across studies, but our meta-analytic method fit the data and covered the estimated residuals (Extended Data Fig. 6b ). After trimming 10% of outliers, we did not detect publication bias in the results for breast cancer. See Supplementary Tables 4 and 7 for study bias characteristics and selected bias covariates, Supplementary Fig. 5 for results without 10% trimming and Supplementary Table 8 for observed RR data and alternative exposures across studies for the remaining 2-star pairs.
One-star associations
When average exposure to smoking does not significantly increase (or decrease) the risk of an outcome, once between-study heterogeneity and other sources of uncertainty are accounted for (that is, ROS < 0), the association between smoking and that outcome is categorized as 1 star, indicating that there is not sufficient evidence for the effect of smoking on the outcome to reject the null (that is, there may be no association). There were seven outcomes with an association with smoking that rated as 1 star: colorectal and kidney cancer (each –0.01 ROS); leukemia (−0.04); fractures (−0.05); prostate cancer (−0.06); liver cancer (−0.32); and asthma (−0.64).
We use smoking and prostate cancer as examples of a 1-star association. We extracted 78 observations from 21 prospective cohort studies and 1 nested case–control study across 15 locations (Supplementary Table 3 ) 157 , 160 , 166 , 167 , 168 , 169 , 170 , 171 , 172 , 173 , 174 , 175 , 176 , 177 , 178 , 179 , 180 , 181 , 182 , 183 , 184 , 185 . The exposure among the exposed group ranged from 1 cigarette to 90 cigarettes smoked per day, with the 85th percentile of exposure in the exposed group being 29.73 cigarettes smoked per day.
Based on our conservative interpretation of the data, we did not find a significant relationship between cigarettes smoked per day and the RR of prostate cancer (Fig. 4B ). The exposure-averaged BPRF for prostate cancer was 0.94, which was opposite null from the full range of mean RRs, such as 1.16 (0.89–1.53) at 20 cigarettes smoked per day. The corresponding ROS was −0.06, which is consistent with no evidence of an association between smoking and increased risk of prostate cancer. See Table 2 and Supplementary Information 4.5 for results for the additional outcomes that have a 1-star association with smoking.
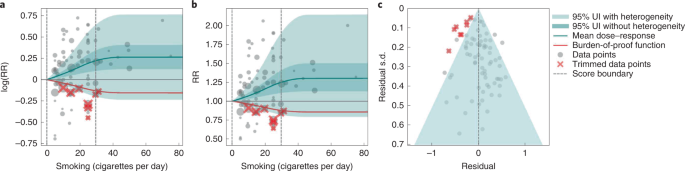
The relationship between smoking and prostate cancer is nonlinear, particularly for middle-to-high exposure levels where the mean risk curve becomes flat (Fig. 4a ). We did not adjust for any bias covariate because no significant bias covariates were selected by the algorithm (Supplementary Table 7 ). The RRs reported across studies were very heterogeneous, but our meta-analytic method fit the data and covered the estimated residuals well (Fig. 4b,c ). The ROS associated with the BPRF is −0.05, suggesting that the most conservative interpretation of all evidence, after accounting for between-study heterogeneity, indicates an inconclusive relationship between smoking exposure and the risk of prostate cancer. After trimming 10% of outliers, we still detected publication bias in the results for prostate cancer, which warrants further studies using sample populations. See Supplementary Tables 4 and 7 for study bias characteristics and selected bias covariates, Supplementary Fig. 5 for results without 10% trimming and Supplementary Table 8 for observed RR data and alternative exposures across studies for the remaining 1-star pairs.
Age-specific dose–response risk for CVD outcomes
We produced age-specific dose–response risk curves for the five selected CVD outcomes ( Methods ). The ROS associated with each smoking–CVD pair was calculated based on the reference risk curve estimated using all risk data regardless of age information. Estimation of the BPRF, calculation of the associated ROS and star rating of the smoking–CVD pairs follow the same rules as the other non-CVD smoking–outcome pairs (Table 1 and Supplementary Figs. 2 – 4 ). Once we had estimated the reference dose–response risk curve for each CVD outcome, we determined the age group of the reference risk curve. The reference age group is 55–59 years for all CVD outcomes, except for peripheral artery disease, the reference age group for which is 60–64 years. We then estimated the age pattern of smoking on all CVD outcomes (Supplementary Fig. 2 ) and calculated age attenuation factors of the risk for each age group by comparing the risk of each age group with that of the reference age group, using the estimated age pattern (Supplementary Fig. 3 ). Last, we applied the draws of age attenuation factors of each age group to the dose–response risk curve for the reference age group to produce the age group-specific dose–response risk curves for each CVD outcome (Supplementary Fig. 4 ).
Using our burden-of-proof meta-analytic methods, we re-estimated the dose–response risk of smoking on 36 health outcomes that had previously been demonstrated to be associated with smoking 30 , 186 . Using these methods, which account for both the reported uncertainty of the data and the between-study heterogeneity, we found that 29 of the 36 smoking–outcome pairs are supported by evidence that suggests a significant dose–response relationship between smoking and the given outcome (28 with a harmful association and 1 with a protective association). Conversely, after accounting for between-study heterogeneity, the available evidence of smoking risk on seven outcomes (that is, colon and rectum cancer, kidney cancer, leukemia, prostate cancer, fractures, liver cancer and asthma) was insufficient to reject the null or draw definitive conclusions on their relationship to smoking. Among the 29 outcomes that have evidence supporting a significant relationship to smoking, 8 had strong-to-very-strong evidence of a relationship, meaning that, given all the available data on smoking risk, we estimate that average exposure to smoking increases the risk of those outcomes by >50% (4- and 5-star outcomes). The currently available evidence for the remaining 21 outcomes with a significant association with current smoking was weak to moderate, indicating that smoking increases the risk of those outcomes by at least >0–50% (2- and 3-star associations).
Even under our conservative interpretation of the data, smoking is irrefutably harmful to human health, with the greatest increases in risk occurring for laryngeal cancer, aortic aneurysm, peripheral artery disease, lung cancer and other pharynx cancer (excluding nasopharynx cancer), which collectively represent large causes of death and ill-health. The magnitude of and evidence for the associations between smoking and its leading health outcomes are among the highest currently analyzed in the burden-of-proof framework 29 . The star ratings assigned to each smoking–outcome pair offer policy makers a way of categorizing and comparing the evidence for a relationship between smoking and its potential health outcomes ( https://vizhub.healthdata.org/burden-of-proof ). We found that, for seven outcomes in our analysis, there was insufficient or inconsistent evidence to demonstrate a significant association with smoking. This is a key finding because it demonstrates the need for more high-quality data for these particular outcomes; availability of more data should improve the strength of evidence for whether or not there is an association between smoking and these health outcomes.
Our systematic review approach and meta-analytic methods have numerous benefits over existing systematic reviews and meta-analyses on the same topic that use traditional random effects models. First, our approach relaxes the log(linear) assumption, using a spline ensemble to estimate the risk 29 . Second, our approach allows variable reference groups and exposure ranges, allowing for more accurate estimates regardless of whether or not the underlying relative risk is log(linear). Furthermore, it can detect outliers in the data automatically. Finally, it quantifies uncertainty due to between-study heterogeneity while accounting for small numbers of studies, minimizing the risk that conclusions will be drawn based on spurious findings.
We believe that the results for the association between smoking and each of the 36 health outcomes generated by the present study, including the mean risk function, BPRF, ROS, average excess risk and star rating, could be useful to a range of stakeholders. Policy makers can formulate their decisions on smoking control priorities and resource allocation based on the magnitude of the effect and the consistency of the evidence relating smoking to each of the 36 outcomes, as represented by the ROS and star rating for each smoking–outcome association 187 . Physicians and public health practitioners can use the estimates of average increased risk and the star rating to educate patients and the general public about the risk of smoking and to promote smoking cessation 188 . Researchers can use the estimated mean risk function or BPRF to obtain the risk of an outcome at a given smoking exposure level, as well as uncertainty surrounding that estimate of risk. The results can also be used in the estimation of risk-attributable burden, that is, the deaths and disability-adjusted life-years due to each outcome that are attributable to smoking 30 , 186 . For the general public, these results could help them to better understand the risk of smoking and manage their health 189 .
Although our meta-analysis was comprehensive and carefully conducted, there are limitations to acknowledge. First, the bias covariates used, although carefully extracted and evaluated, were based on observable study characteristics and thus may not fully capture unobserved characteristics such as study quality or context, which might be major sources of bias. Second, if multiple risk estimates with different adjustment levels were reported in a given study, we included only the fully adjusted risk estimate and modeled the adjustment level according to the number of covariates adjusted for (rather than which covariates were adjusted for) and whether a standard adjustment for age and sex had been applied. This approach limited our ability to make full use of all available risk estimates in the literature. Third, although we evaluated the potential for publication bias in the data, we did not test for other forms of bias such as when studies are more consistent with each other than expected by chance 29 . Fourth, our analysis assumes that the relationships between smoking and health outcomes are similar across geographical regions and over time. We do not have sufficient evidence to quantify how the relationships may have evolved over time because the composition of smoking products has also changed over time. Perhaps some of the heterogeneity of the effect sizes in published studies reflects this; however, this cannot be discerned with the currently available information.
In the future, we plan to include crude and partially adjusted risk estimates in our analyses to fully incorporate all available risk estimates, to model the adjusted covariates in a more comprehensive way by mapping the adjusted covariates across all studies comprehensively and systematically, and to develop methods to evaluate additional forms of potential bias. We plan to update our results on a regular basis to provide timely and up-to-date evidence to stakeholders.
To conclude, we have re-estimated the dose–response risk of smoking on 36 health outcomes while synthesizing all the available evidence up to 31 May 2022. We found that, even after factoring in the heterogeneity between studies and other sources of uncertainty, smoking has a strong-to-very-strong association with a range of health outcomes and confirmed that smoking is irrefutably highly harmful to human health. We found that, due to small numbers of studies, inconsistency in the data, small effect sizes or a combination of these reasons, seven outcomes for which some previous research had found an association with smoking did not—under our meta-analytic framework and conservative approach to interpreting the data—have evidence of an association. Our estimates of the evidence for risk of smoking on 36 selected health outcomes have the potential to inform the many stakeholders of smoking control, including policy makers, researchers, public health professionals, physicians, smokers and the general public.
For the present study, we used a meta-analytic tool, MR-BRT (metaregression—Bayesian, regularized, trimmed), to estimate the dose–response risk curves of the risk of a health outcome across the range of current smoking levels along with uncertainty estimates 28 . Compared with traditional meta-analysis using linear mixed effect models, MR-BRT relaxes the assumption of a log(linear) relationship between exposure and risk, incorporates between-study heterogeneity into the uncertainty of risk estimates, handles estimates reported across different exposure categories, automatically identifies and trims outliers, and systematically tests and adjusts for bias due to study designs and characteristics. The meta-analytic methods employed by the present study followed the six main steps proposed by Zheng et al. 28 , 29 , namely: (1) enacting a systematic review approach and data extraction following a pre-specified and standardized protocol; (2) estimating the shape of the relationship between exposure and RR; (3) evaluating and adjusting for systematic bias as a function of study characteristics and risk estimation; (4) quantifying between-study heterogeneity while adjusting for within-study correlation and the number of studies; (5) evaluating potential publication or reporting biases; and (6) estimating the mean risk function and the BPRF, calculating the ROS and categorizing smoking–outcome pairs using a star-rating scheme from 1 to 5.
The estimates for our primary indicators of this work—mean RRs across a range of exposures, BRPFs, ROSs and star ratings for each risk–outcome pair—are not specific to or disaggregated by specific populations. We did not estimate RRs separately for different locations, sexes (although the RR of prostate cancer was estimated only for males and of cervical and breast cancer only for females) or age groups (although this analysis was applied to disease endpoints in adults aged ≥30 years only and, as detailed below, age-specific estimates were produced for the five CVD outcomes).
The present study complies with the PRISMA guidelines 190 (Supplementary Tables 9 and 10 and Supplementary Information 1.5 ) and Guidelines for Accurate and Transparent Health Estimates Reporting (GATHER) recommendations 191 (Supplementary Table 11 ). The study was approved by the University of Washington Institutional Review Board (study no. 9060). The systematic review approach was not registered.
Selecting health outcomes
In the present study, current smoking is defined as the current use of any smoked tobacco product on a daily or occasional basis. Health outcomes were initially selected using the World Cancer Research Fund criteria for convincing or probable evidence as described in Murray et al. 186 . The 36 health outcomes that were selected based on existing evidence of a relationship included 16 cancers (lung cancer, esophageal cancer, stomach cancer, leukemia, liver cancer, laryngeal cancer, breast cancer, cervical cancer, colorectal cancer, lip and oral cavity cancer, nasopharyngeal cancer, other pharynx cancer (excluding nasopharynx cancer), pancreatic cancer, bladder cancer, kidney cancer and prostate cancer), 5 CVDs (ischemic heart disease, stroke, atrial fibrillation and flutter, aortic aneurysm and peripheral artery disease) and 15 other diseases (COPD, lower respiratory tract infections, tuberculosis, asthma, type 2 diabetes, Alzheimer’s disease and related dementias, Parkinson’s disease, multiple sclerosis, cataracts, gallbladder diseases, low back pain, peptic ulcer disease, rheumatoid arthritis, macular degeneration and fracture). Definitions of the outcomes are described in Supplementary Table 1 .
Step 1: systematic review approach to literature search and data extraction
Informed by the systematic review approach we took for the GBD 2019 (ref. 30 ), for the present study we identified input studies in the literature using a systematic review approach for all 36 smoking–outcome pairs using updated search strings to identify all relevant studies indexed in PubMed up to 31 May 2022 and extracted data on smoking risk estimates. Briefly, the studies that were extracted represented several types of study design (for example, cohort and case–control studies), measured exposure in several different ways and varied in their choice of reference categories (where some compared current smokers with never smokers, whereas others compared current smokers with nonsmokers or former smokers). All these study characteristics were catalogued systematically and taken into consideration during the modeling part of the analysis.
In addition, for CVD outcomes, we also estimated the age pattern of risk associated with smoking. We applied a systematic review of literature approach for smoking risk for the five CVD outcomes. We developed a search string to search for studies reporting any association between binary smoking status (that is, current, former and ever smokers) and the five CVD outcomes from 1 January 1970 to 31 May 2022, and included only studies reporting age-specific risk (RR, odds ratio (OR), hazard ratio (HR)) of smoking status. The inclusion criteria and results of the systematic review approach are reported in accordance with PRISMA guidelines 31 . Details for each outcome on the search string used in the systematic review approach, refined inclusion and exclusion criteria, data extraction template and PRISMA diagram are given in Supplementary Information 1 . Title and/or abstract screening, full text screening and data extraction were conducted by 14 members of the research team and extracted data underwent manual quality assurance by the research team to verify accuracy.
Selecting exposure categories
Cumulative exposure in pack-years was the measure of exposure used for COPD and all cancer outcomes except for prostate cancer, to reflect the risk of both duration and intensity of current smoking on these outcomes. For prostate cancer, CVDs and all the other outcomes except for fractures, we used cigarette-equivalents smoked per day as the exposure for current smoking, because smoking intensity is generally thought to be more important than duration for these outcomes. For fractures, we used binary exposure, because there were few studies examining intensity or duration of smoking on fractures. The smoking–outcome pairs and the corresponding exposures are summarized in Supplementary Table 4 and are congruent with the GBD 2019 (refs. 30 , 186 ).
Steps 2–5: modeling dose–response RR of smoking on the selected health outcomes
Of the six steps proposed by Zheng et al. 29 , steps 2–5 cover the process of modeling dose–response risk curves. In step 2, we estimated the shape (or the ‘signal’) of the dose–response risk curves, integrating over different exposure ranges. To relax the log(linear) assumption usually applied to continuous dose–response risk and make the estimates robust to the placement of spline knots, we used an ensemble spline approach to fit the functional form of the dose–response relationship. The final ensemble model was a weighted combination of 50 models with random knot placement, with the weight of each model proportional to measures of model fit and total variation. To avoid the influence of extreme data and reduce publication bias, we trimmed 10% of data for each outcome as outliers. We also applied a monotonicity constraint to ensure that the mean risk curves were nondecreasing (or nonincreasing in the case of Parkinson’s disease).
In step 3, following the GRADE approach 192 , 193 , we quantified risk of bias across six domains, namely, representativeness of the study population, exposure, outcome, reverse causation, control for confounding and selection bias. Details about the bias covariates are provided in Supplementary Table 4 . We systematically tested for the effect of bias covariates using metaregression, selected significant bias covariates using the Lasso approach 194 , 195 and adjusted for the selected bias covariates in the final risk curve.
In step 4, we quantified between-study heterogeneity accounting for within-study correlation, uncertainty of the heterogeneity, as well as small number of studies. Specifically, we used a random intercept in the mixed-effects model to account for the within-study correlation and used a study-specific random slope with respect to the ‘signal’ to capture between-study heterogeneity. As between-study heterogeneity can be underestimated or even zero when the number of studies is small 196 , 197 , we used Fisher’s information matrix to estimate the uncertainty of the heterogeneity 198 and incorporated that uncertainty into the final results.
In step 5, in addition to generating funnel plots and visually inspecting for asymmetry (Figs. 1c , 2c , 3c and 4c and Extended Data Fig. 6c ) to identify potential publication bias, we also statistically tested for potential publication or reporting bias using Egger’s regression 199 . We flagged potential publication bias in the data but did not correct for it, which is in line with the general literature 10 , 200 , 201 . Full details about the modeling process have been published elsewhere 29 and model specifications for each outcome are in Supplementary Table 6 .
Step 6: estimating the mean risk function and the BPRF
In the final step, step 6, the metaregression model inclusive of the selected bias covariates from step 3 (for example, the highest adjustment level) was used to predict the mean risk function and its 95% UI, which incorporated the uncertainty of the mean effect, between-study heterogeneity and the uncertainty in the heterogeneity estimate accounting for small numbers of studies. Specifically, 1,000 draws were created for each 0.1 level of doses from 0 pack-years to 100 pack-years or cigarette-equivalents smoked per day using the Bayesian metaregression model. The mean of the 1,000 draws was used to estimate the mean risk at each exposure level, and the 25th and 95th draws were used to estimate the 95% UIs for the mean risk at each exposure level.
The BPRF 29 is a conservative estimate of risk function consistent with the available evidence, correcting for both between-study heterogeneity and systemic biases related to study characteristics. The BPRF is defined as either the 5th (if harmful) or 95th (if protective) quantile curve closest to the line of log(RR) of 0, which defines the null (Figs. 1a , 2b , 3a and 4a ). The BPRF represents the smallest harmful (or protective) effect of smoking on the corresponding outcome at each level of exposure that is consistent with the available evidence. A BPRF opposite null from the mean risk function indicates that insufficient evidence is available to reject null, that is, that there may not be an association between risk and outcome. Likewise, the further the BPRF is from null on the same side of null as the mean risk function, the higher the magnitude and evidence for the relationship. The BPRF can be interpreted as indicating that, even accounting for between-study heterogeneity and its uncertainty, the log(RR) across the studied smoking range is at least as high as the BPRF (or at least as low as the BPRF for a protective risk).
To quantify the strength of the evidence, we calculated the ROS for each smoking–outcome association as the signed value of the log(BPRF) averaged between the 15th and 85th percentiles of observed exposure levels for each outcome. The ROS is a single summary of the effect of smoking on the outcome, with higher positive ROSs corresponding to stronger and more consistent evidence and a higher average effect size of smoking and a negative ROS, suggesting that, based on the available evidence, there is no significant effect of smoking on the outcome after accounting for between-study heterogeneity.
For ease of communication, we further classified each smoking–outcome association into a star rating from 1 to 5. Briefly, 1-star associations have an ROS <0, indicating that there is insufficient evidence to find a significant association between smoking and the selected outcome. We divided the positive ROSs into ranges 0.0–0.14 (2-star), >0.14–0.41 (3-star), >0.41–0.62 (4-star) and >0.62 (5-star). These categories correspond to excess risk ranges for harmful risks of 0–15%, >15–50%, >50–85% and >85%. For protective risks, the ranges of exposure-averaged decreases in risk by star rating are 0–13% (2 stars), >13–34% (3 stars), >34–46% (4 stars) and >46% (5 stars).
Among the 36 smoking–outcome pairs analyzed, smoking fracture was the only binary risk–outcome pair, which was due to limited data on the dose–response risk of smoking on fracture 202 . The estimation of binary risk was simplified because the RR was merely a comparison between current smokers and nonsmokers or never smokers. The concept of ROS for continuous risk can naturally extend to binary risk because the BPRF is still defined as the 5th percentile of the effect size accounting for data uncertainty and between-study heterogeneity. However, binary ROSs must be divided by 2 to make them comparable with continuous ROSs, which were calculated by averaging the risk over the range between the 15th and the 85th percentiles of observed exposure levels. Full details about estimating mean risk functions, BPRFs and ROSs for both continuous and binary risk–outcome pairs can be found elsewhere 29 .
Estimating the age-specific risk function for CVD outcomes
For non-CVD outcomes, we assumed that the risk function was the same for all ages and all sexes, except for breast, cervical and prostate cancer, which were assumed to apply only to females or males, respectively. As the risk of smoking on CVD outcomes is known to attenuate with increasing age 203 , 204 , 205 , 206 , we adopted a four-step approach for GBD 2020 to produce age-specific dose–response risk curves for CVD outcomes.
First, we estimated the reference dose–response risk of smoking for each CVD outcome using dose-specific RR data for each outcome regardless of the age group information. This step was identical to that implemented for the other non-CVD outcomes. Once we had generated the reference curve, we determined the age group associated with it by calculating the weighted mean age across all dose-specific RR data (weighted by the reciprocal of the s.e.m. of each datum). For example, if the weighted mean age of all dose-specific RR data was 56.5, we estimated the age group associated with the reference risk curve to be aged 55–59 years. For cohort studies, the age range associated with the RR estimate was calculated as a mean age at baseline plus the mean/median years of follow-up (if only the maximum years of follow-up were reported, we would halve this value and add it to the mean age at baseline). For case–control studies, the age range associated with the OR estimate was simply the reported mean age at baseline (if mean age was not reported, we used the midpoint of the age range instead).
In the third step, we extracted age group-specific RR data and relevant bias covariates from the studies identified in our systematic review approach of age-specific smoking risk on CVD outcomes, and used MR-BRT to model the age pattern of excess risk (that is, RR-1) of smoking on CVD outcomes with age group-specific excess RR data for all CVD outcomes. We modeled the age pattern of smoking risk on CVDs following the same steps we implemented for modeling dose–response risk curves. In the final model, we included a spline on age, random slope on age by study and the bias covariate encoding exposure definition (that is, current, former and ever smokers), which was picked by the variable selection algorithm 28 , 29 . When predicting the age pattern of the excess risk of smoking on CVD outcomes using the fitted model, we did not include between-study heterogeneity to reduce uncertainty in the prediction.
In the fourth step, we calculated the age attenuation factors of excess risk compared with the reference age group for each CVD outcome as the ratio of the estimated excess risk for each age group to the excess risk for the reference age group. We performed the calculation at the draw level to obtain 1,000 draws of the age attenuation factors for each age group. Once we had estimated the age attenuation factors, we carried out the last step, which consisted of adjusting the risk curve for the reference age group from step 1 using equation (1) to produce the age group-specific risk curves for each CVD outcome:
We implemented the age adjustment at the draw level so that the uncertainty of the age attenuation factors could be naturally incorporated into the final adjusted age-specific RR curves. A PRISMA diagram detailing the systematic review approach, a description of the studies included and the full details about the methods are in Supplementary Information 1.5 and 5.2 .
Estimating the theoretical minimum risk exposure level
The theoretical minimum risk exposure level for smoking was 0, that is, no individuals in the population are current or former smokers.
Model validation
The validity of the meta-analytic tool has been extensively evaluated by Zheng and colleagues using simulation experiments 28 , 29 . For the present study, we conducted two additional sensitivity analyses to examine how the shape of the risk curves was impacted by applying a monotonicity constraint and trimming 10% of data. We present the results of these sensitivity analyses in Supplementary Information 6 . In addition to the sensitivity analyses, the dose–response risk estimates were also validated by plotting the mean risk function along with its 95% UI against both the extracted dose-specific RR data from the studies included and our previous dose–response risk estimates from the GBD 2019 (ref. 30 ). The mean risk functions along with the 95% UIs were validated based on data fit and the level, shape and plausibility of the dose–response risk curves. All curves were validated by all authors and reviewed by an external expert panel, comprising professors with relevant experience from universities including Johns Hopkins University, Karolinska Institute and University of Barcelona; senior scientists working in relevant departments at the WHO and the Center for Disease Control and Prevention (CDC) and directors of nongovernmental organizations such as the Campaign for Tobacco-Free Kids.
Statistical analysis
Analyses were carried out using R v.3.6.3, Python v.3.8 and Stata v.16.
Statistics and reproducibility
The study was a secondary analysis of existing data involving systematic reviews and meta-analyses. No statistical method was used to predetermine sample size. As the study did not involve primary data collection, randomization and blinding, data exclusions were not relevant to the present study, and, as such, no data were excluded and we performed no randomization or blinding. We have made our data and code available to foster reproducibility.
Reporting summary
Further information on research design is available in the Nature Research Reporting Summary linked to this article.
Data availability
The findings from the present study are supported by data available in the published literature. Data sources and citations for each risk–outcome pair can be downloaded using the ‘download’ button on each risk curve page currently available at https://vizhub.healthdata.org/burden-of-proof . Study characteristics and citations for all input data used in the analyses are also provided in Supplementary Table 3 , and Supplementary Table 2 provides a template of the data collection form.
Code availability
All code used for these analyses is publicly available online ( https://github.com/ihmeuw-msca/burden-of-proof ).
Doll, R. & Hill, A. B. Smoking and carcinoma of the lung. Br. Med. J. 2 , 739–748 (1950).
Article CAS PubMed PubMed Central Google Scholar
Di Cicco, M. E., Ragazzo, V. & Jacinto, T. Mortality in relation to smoking: the British Doctors Study. Breathe 12 , 275–276 (2016).
Article PubMed PubMed Central Google Scholar
World Health Organization. WHO Framework Convention on Tobacco Control 36 (WHO, 2003).
Dai, X., Gakidou, E. & Lopez, A. D. Evolution of the global smoking epidemic over the past half century: strengthening the evidence base for policy action. Tob. Control 31 , 129–137 (2022).
Article PubMed Google Scholar
Dikshit, R. P. & Kanhere, S. Tobacco habits and risk of lung, oropharyngeal and oral cavity cancer: a population-based case-control study in Bhopal, India. Int. J. Epidemiol. 29 , 609–614 (2000).
Article CAS PubMed Google Scholar
Liaw, K. M. & Chen, C. J. Mortality attributable to cigarette smoking in Taiwan: a 12-year follow-up study. Tob. Control 7 , 141–148 (1998).
Gandini, S. et al. Tobacco smoking and cancer: a meta-analysis. Int. J. Cancer 122 , 155–164 (2008).
Deng, X., Yuan, C. & Chang, D. Interactions between single nucleotide polymorphism of SERPINA1 gene and smoking in association with COPD: a case–control study. Int. J. Chron. Obstruct. Pulmon. Dis. 12 , 259–265 (2017).
Leem, A. Y., Park, B., Kim, Y. S., Jung, J. Y. & Won, S. Incidence and risk of chronic obstructive pulmonary disease in a Korean community-based cohort. Int. J. Chron. Obstruct. Pulmon. Dis. 13 , 509–517 (2018).
Forey, B. A., Thornton, A. J. & Lee, P. N. Systematic review with meta-analysis of the epidemiological evidence relating smoking to COPD, chronic bronchitis and emphysema. BMC Pulmon. Med. 11 , 36 (2011).
Article Google Scholar
Tan, J. et al. Smoking, blood pressure, and cardiovascular disease mortality in a large cohort of Chinese men with 15 years follow-up. Int. J. Environ. Res. Public Health 15 , E1026 (2018).
Doll, R., Peto, R., Boreham, J. & Sutherland, I. Mortality in relation to smoking: 50 years’ observations on male British doctors. Br. Med. J. 328 , 1519 (2004).
Huxley, R. R. & Woodward, M. Cigarette smoking as a risk factor for coronary heart disease in women compared with men: a systematic review and meta-analysis of prospective cohort studies. Lancet 378 , 1297–1305 (2011).
Hbejan, K. Smoking effect on ischemic heart disease in young patients. Heart Views 12 , 1–6 (2011).
Chao, H. et al. A meta-analysis of active smoking and risk of meningioma. Tob. Induc. Dis. 19 , 34 (2021).
Shi, H., Shao, X. & Hong, Y. Association between cigarette smoking and the susceptibility of acute myeloid leukemia: a systematic review and meta-analysis. Eur. Rev. Med Pharm. Sci. 23 , 10049–10057 (2019).
CAS Google Scholar
Macacu, A., Autier, P., Boniol, M. & Boyle, P. Active and passive smoking and risk of breast cancer: a meta-analysis. Breast Cancer Res. Treat. 154 , 213–224 (2015).
Pujades-Rodriguez, M. et al. Heterogeneous associations between smoking and a wide range of initial presentations of cardiovascular disease in 1 937 360 people in England: lifetime risks and implications for risk prediction. Int. J. Epidemiol. 44 , 129–141 (2015).
Kanazir, M. et al. Risk factors for hepatocellular carcinoma: a case-control study in Belgrade (Serbia). Tumori 96 , 911–917 (2010).
Pytynia, K. B. et al. Matched-pair analysis of survival of never smokers and ever smokers with squamous cell carcinoma of the head and neck. J. Clin. Oncol. 22 , 3981–3988 (2004).
Barengo, N. C., Antikainen, R., Harald, K. & Jousilahti, P. Smoking and cancer, cardiovascular and total mortality among older adults: the Finrisk Study. Prev. Med. Rep. 14 , 100875 (2019).
Guo, Y. et al. Modifiable risk factors for cognitive impairment in Parkinson’s disease: a systematic review and meta-analysis of prospective cohort studies. Mov. Disord. 34 , 876–883 (2019).
Aune, D., Vatten, L. J. & Boffetta, P. Tobacco smoking and the risk of gallbladder disease. Eur. J. Epidemiol. 31 , 643–653 (2016).
Qin, L., Deng, H.-Y., Chen, S.-J. & Wei, W. Relationship between cigarette smoking and risk of chronic myeloid leukaemia: a meta-analysis of epidemiological studies. Hematology 22 , 193–200 (2017).
Petrick, J. L. et al. Tobacco, alcohol use and risk of hepatocellular carcinoma and intrahepatic cholangiocarcinoma: the Liver Cancer Pooling Project. Br. J. Cancer 118 , 1005–1012 (2018).
United States Department of Health, Education and Welfare. Smoking and Health. Report of the Advisory Committee on Smoking and Health to the Surgeon General of the United States Public Health Service https://www.cdc.gov/tobacco/data_statistics/sgr/index.htm (US DHEW, 1964).
United States Public Health Service Office of the Surgeon General & National Center for Chronic Disease Prevention and Health Promotion (US) Office on Smoking and Health. Smoking Cessation: A Report of the Surgeon General . (US Department of Health and Human Services, 2020).
Zheng, P., Barber, R., Sorensen, R. J. D., Murray, C. J. L. & Aravkin, A. Y. Trimmed constrained mixed effects models: formulations and algorithms. J. Comput. Graph Stat. 30 , 544–556 (2021).
Zheng, P. et al. The Burden of Proof studies: assessing the evidence of risk. Nat. Med. in press (2022).
Reitsma, M. B. et al. Spatial, temporal, and demographic patterns in prevalence of smoking tobacco use and attributable disease burden in 204 countries and territories, 1990–2019: a systematic analysis from the Global Burden of Disease Study 2019. Lancet 397 , 2337–2360 (2021).
Moher, D., Liberati, A., Tetzlaff, J. & Altman, D. G. Preferred reporting items for systematic reviews and meta-analyses: the PRISMA statement. Br. Med. J. 339 , b2535 (2009).
Liu, Z. Y., He, X. Z. & Chapman, R. S. Smoking and other risk factors for lung cancer in Xuanwei, China. Int. J. Epidemiol. 20 , 26–31 (1991).
Brownson, R. C., Reif, J. S., Keefe, T. J., Ferguson, S. W. & Pritzl, J. A. Risk factors for adenocarcinoma of the lung. Am. J. Epidemiol. 125 , 25–34 (1987).
Marugame, T. et al. Lung cancer death rates by smoking status: comparison of the Three-Prefecture Cohort study in Japan to the Cancer Prevention Study II in the USA. Cancer Sci. 96 , 120–126 (2005).
Dosemeci, M., Gokmen, I., Unsal, M., Hayes, R. B. & Blair, A. Tobacco, alcohol use, and risks of laryngeal and lung cancer by subsite and histologic type in Turkey. Cancer Causes Control 8 , 729–737 (1997).
Freedman, N. D. et al. Impact of changing US cigarette smoking patterns on incident cancer: risks of 20 smoking-related cancers among the women and men of the NIH-AARP cohort. Int. J. Epidemiol. 45 , 846–856 (2016).
Bae, J.-M. et al. Lung cancer incidence by smoking status in Korean men: 16 years of observations in the Seoul Male Cancer Cohort study. J. Korean Med. Sci. 28 , 636–637 (2013).
Everatt, R., Kuzmickienė, I., Virvičiūtė, D. & Tamošiūnas, A. Cigarette smoking, educational level and total and site-specific cancer: a cohort study in men in Lithuania. Eur. J. Cancer Prev. 23 , 579–586 (2014).
Nordlund, L. A., Carstensen, J. M. & Pershagen, G. Are male and female smokers at equal risk of smoking-related cancer: evidence from a Swedish prospective study. Scand. J. Public Health 27 , 56–62 (1999).
Siemiatycki, J., Krewski, D., Franco, E. & Kaiserman, M. Associations between cigarette smoking and each of 21 types of cancer: a multi-site case–control study. Int. J. Epidemiol. 24 , 504–514 (1995).
Chyou, P. H., Nomura, A. M. & Stemmermann, G. N. A prospective study of the attributable risk of cancer due to cigarette smoking. Am. J. Public Health 82 , 37–40 (1992).
Potter, J. D., Sellers, T. A., Folsom, A. R. & McGovern, P. G. Alcohol, beer, and lung cancer in postmenopausal women. The Iowa Women’s Health Study. Ann. Epidemiol. 2 , 587–595 (1992).
Chyou, P. H., Nomura, A. M., Stemmermann, G. N. & Kato, I. Lung cancer: a prospective study of smoking, occupation, and nutrient intake. Arch. Environ. Health 48 , 69–72 (1993).
Pesch, B. et al. Cigarette smoking and lung cancer–relative risk estimates for the major histological types from a pooled analysis of case–control studies. Int. J. Cancer 131 , 1210–1219 (2012).
Jöckel, K. H. et al. Occupational and environmental hazards associated with lung cancer. Int. J. Epidemiol. 21 , 202–213 (1992).
Jöckel, K. H., Ahrens, W., Jahn, I., Pohlabeln, H. & Bolm-Audorff, U. Occupational risk factors for lung cancer: a case-control study in West Germany. Int. J. Epidemiol. 27 , 549–560 (1998).
Lei, Y. X., Cai, W. C., Chen, Y. Z. & Du, Y. X. Some lifestyle factors in human lung cancer: a case-control study of 792 lung cancer cases. Lung Cancer 14 , S121–S136 (1996).
Pawlega, J., Rachtan, J. & Dyba, T. Evaluation of certain risk factors for lung cancer in Cracow (Poland)—a case–control study. Acta Oncol. 36 , 471–476 (1997).
Mao, Y. et al. Socioeconomic status and lung cancer risk in Canada. Int. J. Epidemiol. 30 , 809–817 (2001).
Barbone, F., Bovenzi, M., Cavallieri, F. & Stanta, G. Cigarette smoking and histologic type of lung cancer in men. Chest 112 , 1474–1479 (1997).
Matos, E., Vilensky, M., Boffetta, P. & Kogevinas, M. Lung cancer and smoking: a case–control study in Buenos Aires, Argentina. Lung Cancer 21 , 155–163 (1998).
Simonato, L. et al. Lung cancer and cigarette smoking in Europe: an update of risk estimates and an assessment of inter-country heterogeneity. Int. J. Cancer 91 , 876–887 (2001).
Risch, H. A. et al. Are female smokers at higher risk for lung cancer than male smokers? A case–control analysis by histologic type. Am. J. Epidemiol. 138 , 281–293 (1993).
Sankaranarayanan, R. et al. A case–control study of diet and lung cancer in Kerala, south India. Int. J. Cancer 58 , 644–649 (1994).
Band, P. R. et al. Identification of occupational cancer risks in British Columbia. Part I: methodology, descriptive results, and analysis of cancer risks, by cigarette smoking categories of 15,463 incident cancer cases. J. Occup. Environ. Med. 41 , 224–232 (1999).
Becher, H., Jöckel, K. H., Timm, J., Wichmann, H. E. & Drescher, K. Smoking cessation and nonsmoking intervals: effect of different smoking patterns on lung cancer risk. Cancer Causes Control 2 , 381–387 (1991).
Brockmöller, J., Kerb, R., Drakoulis, N., Nitz, M. & Roots, I. Genotype and phenotype of glutathione S-transferase class mu isoenzymes mu and psi in lung cancer patients and controls. Cancer Res. 53 , 1004–1011 (1993).
PubMed Google Scholar
Vena, J. E., Byers, T. E., Cookfair, D. & Swanson, M. Occupation and lung cancer risk. An analysis by histologic subtypes. Cancer 56 , 910–917 (1985).
Cascorbi, I. et al. Homozygous rapid arylamine N -acetyltransferase (NAT2) genotype as a susceptibility factor for lung cancer. Cancer Res. 56 , 3961–3966 (1996).
CAS PubMed Google Scholar
Chiazze, L., Watkins, D. K. & Fryar, C. A case–control study of malignant and non-malignant respiratory disease among employees of a fiberglass manufacturing facility. Br. J. Ind. Med 49 , 326–331 (1992).
CAS PubMed PubMed Central Google Scholar
Ando, M. et al. Attributable and absolute risk of lung cancer death by smoking status: findings from the Japan Collaborative Cohort Study. Int. J. Cancer 105 , 249–254 (2003).
De Matteis, S. et al. Are women who smoke at higher risk for lung cancer than men who smoke? Am. J. Epidemiol. 177 , 601–612 (2013).
He, Y. et al. Changes in smoking behavior and subsequent mortality risk during a 35-year follow-up of a cohort in Xi’an, China. Am. J. Epidemiol. 179 , 1060–1070 (2014).
Nishino, Y. et al. Cancer incidence profiles in the Miyagi Cohort Study. J. Epidemiol. 14 , S7–S11 (2004).
Papadopoulos, A. et al. Cigarette smoking and lung cancer in women: results of the French ICARE case–control study. Lung Cancer 74 , 369–377 (2011).
Shimazu, T. et al. Alcohol and risk of lung cancer among Japanese men: data from a large-scale population-based cohort study, the JPHC study. Cancer Causes Control 19 , 1095–1102 (2008).
Tindle, H. A. et al. Lifetime smoking history and risk of lung cancer: results from the Framingham Heart Study. J. Natl Cancer Inst. 110 , 1201–1207 (2018).
PubMed PubMed Central Google Scholar
Yong, L. C. et al. Intake of vitamins E, C, and A and risk of lung cancer. The NHANES I epidemiologic followup study. First National Health and Nutrition Examination Survey. Am. J. Epidemiol. 146 , 231–243 (1997).
Hansen, M. S. et al. Sex differences in risk of smoking-associated lung cancer: results from a cohort of 600,000 Norwegians. Am. J. Epidemiol. 187 , 971–981 (2018).
Boffetta, P. et al. Tobacco smoking as a risk factor of bronchioloalveolar carcinoma of the lung: pooled analysis of seven case-control studies in the International Lung Cancer Consortium (ILCCO). Cancer Causes Control 22 , 73–79 (2011).
Yun, Y. D. et al. Hazard ratio of smoking on lung cancer in Korea according to histological type and gender. Lung 194 , 281–289 (2016).
Suzuki, I. et al. Risk factors for lung cancer in Rio de Janeiro, Brazil: a case–control study. Lung Cancer 11 , 179–190 (1994).
De Stefani, E., Deneo-Pellegrini, H., Carzoglio, J. C., Ronco, A. & Mendilaharsu, M. Dietary nitrosodimethylamine and the risk of lung cancer: a case–control study from Uruguay. Cancer Epidemiol. Biomark. Prev. 5 , 679–682 (1996).
Google Scholar
Kreuzer, M. et al. Risk factors for lung cancer in young adults. Am. J. Epidemiol. 147 , 1028–1037 (1998).
Armadans-Gil, L., Vaqué-Rafart, J., Rosselló, J., Olona, M. & Alseda, M. Cigarette smoking and male lung cancer risk with special regard to type of tobacco. Int. J. Epidemiol. 28 , 614–619 (1999).
Kubík, A. K., Zatloukal, P., Tomásek, L. & Petruzelka, L. Lung cancer risk among Czech women: a case–control study. Prev. Med. 34 , 436–444 (2002).
Rachtan, J. Smoking, passive smoking and lung cancer cell types among women in Poland. Lung Cancer 35 , 129–136 (2002).
Thun, M. J. et al. 50-year trends in smoking-related mortality in the United States. N. Engl. J. Med. 368 , 351–364 (2013).
Zatloukal, P., Kubík, A., Pauk, N., Tomásek, L. & Petruzelka, L. Adenocarcinoma of the lung among women: risk associated with smoking, prior lung disease, diet and menstrual and pregnancy history. Lung Cancer 41 , 283–293 (2003).
Hansen, M. S., Licaj, I., Braaten, T., Lund, E. & Gram, I. T. The fraction of lung cancer attributable to smoking in the Norwegian Women and Cancer (NOWAC) Study. Br. J. Cancer 124 , 658–662 (2021).
Zhang, P. et al. Association of smoking and polygenic risk with the incidence of lung cancer: a prospective cohort study. Br. J. Cancer 126 , 1637–1646 (2022).
Weber, M. F. et al. Cancer incidence and cancer death in relation to tobacco smoking in a population-based Australian cohort study. Int. J. Cancer 149 , 1076–1088 (2021).
Guo, L.-W. et al. A risk prediction model for selecting high-risk population for computed tomography lung cancer screening in China. Lung Cancer 163 , 27–34 (2022).
Mezzoiuso, A. G., Odone, A., Signorelli, C. & Russo, A. G. Association between smoking and cancers among women: results from the FRiCaM multisite cohort study. J. Cancer 12 , 3136–3144 (2021).
Hawrysz, I., Wadolowska, L., Slowinska, M. A., Czerwinska, A. & Golota, J. J. Adherence to prudent and mediterranean dietary patterns is inversely associated with lung cancer in moderate but not heavy male Polish smokers: a case–control study. Nutrients 12 , E3788 (2020).
Huang, C.-C., Lai, C.-Y., Tsai, C.-H., Wang, J.-Y. & Wong, R.-H. Combined effects of cigarette smoking, DNA methyltransferase 3B genetic polymorphism, and DNA damage on lung cancer. BMC Cancer 21 , 1066 (2021).
Viner, B., Barberio, A. M., Haig, T. R., Friedenreich, C. M. & Brenner, D. R. The individual and combined effects of alcohol consumption and cigarette smoking on site-specific cancer risk in a prospective cohort of 26,607 adults: results from Alberta’s Tomorrow Project. Cancer Causes Control 30 , 1313–1326 (2019).
Park, E. Y., Lim, M. K., Park, E., Oh, J.-K. & Lee, D.-H. Relationship between urinary 4-(methylnitrosamino)-1-(3-pyridyl)-1-butanol and lung cancer risk in the general population: a community-based prospective cohort study. Front. Oncol. 11 , 611674 (2021).
De Stefani, E., Deneo-Pellegrini, H., Mendilaharsu, M., Carzoglio, J. C. & Ronco, A. Dietary fat and lung cancer: a case–control study in Uruguay. Cancer Causes Control 8 , 913–921 (1997).
Wünsch-Filho, V., Moncau, J. E., Mirabelli, D. & Boffetta, P. Occupational risk factors of lung cancer in São Paulo, Brazil. Scand. J. Work Environ. Health 24 , 118–124 (1998).
Hu, J. et al. A case-control study of diet and lung cancer in northeast China. Int. J. Cancer 71 , 924–931 (1997).
Jia, G., Wen, W., Massion, P. P., Shu, X.-O. & Zheng, W. Incorporating both genetic and tobacco smoking data to identify high-risk smokers for lung cancer screening. Carcinogenesis 42 , 874–879 (2021).
Rusmaully, J. et al. Risk of lung cancer among women in relation to lifetime history of tobacco smoking: a population-based case–control study in France (the WELCA study). BMC Cancer 21 , 711 (2021).
Jin, K. et al. Tobacco smoking modifies the association between hormonal factors and lung cancer occurrence among post-menopausal Chinese women. Transl. Oncol. 12 , 819–827 (2019).
Tse, L. A., Wang, F., Wong, M. C.-S., Au, J. S.-K. & Yu, I. T.-S. Risk assessment and prediction for lung cancer among Hong Kong Chinese men. BMC Cancer 22 , 585 (2022).
Huang, C.-C. et al. Joint effects of cigarette smoking and green tea consumption with miR-29b and DNMT3b mRNA expression in the development of lung cancer. Genes 13 , 836 (2022).
Hosseini, M. et al. Environmental risk factors for lung cancer in Iran: a case–control study. Int. J. Epidemiol. 38 , 989–996 (2009).
Naghibzadeh-Tahami, A. et al. Is opium use associated with an increased risk of lung cancer? A case–control study. BMC Cancer 20 , 807 (2020).
Shimatani, K., Ito, H., Matsuo, K., Tajima, K. & Takezaki, T. Cumulative cigarette tar exposure and lung cancer risk among Japanese smokers. Jpn J. Clin. Oncol. 50 , 1009–1017 (2020).
Lai, C.-Y. et al. Genetic polymorphism of catechol- O -methyltransferase modulates the association of green tea consumption and lung cancer. Eur. J. Cancer Prev. 28 , 316–322 (2019).
Schwartz, A. G. et al. Hormone use, reproductive history, and risk of lung cancer: the Women’s Health Initiative studies. J. Thorac. Oncol. 10 , 1004–1013 (2015).
Kreuzer, M., Gerken, M., Heinrich, J., Kreienbrock, L. & Wichmann, H.-E. Hormonal factors and risk of lung cancer among women? Int. J. Epidemiol. 32 , 263–271 (2003).
Sreeja, L. et al. Possible risk modification by CYP1A1, GSTM1 and GSTT1 gene polymorphisms in lung cancer susceptibility in a South Indian population. J. Hum. Genet. 50 , 618–627 (2005).
Siemiatycki, J. et al. Are the apparent effects of cigarette smoking on lung and bladder cancers due to uncontrolled confounding by occupational exposures? Epidemiology 5 , 57–65 (1994).
Chan-Yeung, M. et al. Risk factors associated with lung cancer in Hong Kong. Lung Cancer 40 , 131–140 (2003).
Lawania, S., Singh, N., Behera, D. & Sharma, S. Xeroderma pigmentosum complementation group D polymorphism toward lung cancer susceptibility survival and response in patients treated with platinum chemotherapy. Future Oncol. 13 , 2645–2665 (2017).
De Stefani, E. et al. Mate drinking and risk of lung cancer in males: a case-control study from Uruguay. Cancer Epidemiol. Biomark. Prev. 5 , 515–519 (1996).
Pérez-Padilla, R. et al. Exposure to biomass smoke and chronic airway disease in Mexican women. A case-control study. Am. J. Respir. Crit. Care Med. 154 , 701–706 (1996).
Zhang, X.-R. et al. Glucosamine use, smoking and risk of incident chronic obstructive pulmonary disease: a large prospective cohort study. Br. J. Nutr . https://doi.org/10.1017/S000711452100372X (2021).
Johannessen, A., Omenaas, E., Bakke, P. & Gulsvik, A. Incidence of GOLD-defined chronic obstructive pulmonary disease in a general adult population. Int. J. Tuberc. Lung Dis. 9 , 926–932 (2005).
Fox, J. Life-style and mortality: a large-scale census-based cohort study in Japan. J. Epidemiol. Community Health 45 , 173 (1991).
Article PubMed Central Google Scholar
Thomson, B. et al. Low-intensity daily smoking and cause-specific mortality in Mexico: prospective study of 150 000 adults. Int. J. Epidemiol. 50 , 955–964 (2021).
van Durme, Y. M. T. A. et al. Prevalence, incidence, and lifetime risk for the development of COPD in the elderly: the Rotterdam study. Chest 135 , 368–377 (2009).
Li, L. et al. SERPINE2 rs16865421 polymorphism is associated with a lower risk of chronic obstructive pulmonary disease in the Uygur population: a case–control study. J. Gene Med. 21 , e3106 (2019).
Ganbold, C. et al. The cumulative effect of gene-gene interactions between GSTM1 , CHRNA3 , CHRNA5 and SOD3 gene polymorphisms combined with smoking on COPD risk. Int. J. Chron. Obstruct. Pulmon. Dis. 16 , 2857–2868 (2021).
Omori, H. et al. Twelve-year cumulative incidence of airflow obstruction among Japanese males. Intern. Med. 50 , 1537–1544 (2011).
Manson, J. E., Ajani, U. A., Liu, S., Nathan, D. M. & Hennekens, C. H. A prospective study of cigarette smoking and the incidence of diabetes mellitus among US male physicians. Am. J. Med. 109 , 538–542 (2000).
Lv, J. et al. Adherence to a healthy lifestyle and the risk of type 2 diabetes in Chinese adults. Int. J. Epidemiol. 46 , 1410–1420 (2017).
Waki, K. et al. Alcohol consumption and other risk factors for self-reported diabetes among middle-aged Japanese: a population-based prospective study in the JPHC study cohort I. Diabet. Med. 22 , 323–331 (2005).
Meisinger, C., Döring, A., Thorand, B. & Löwel, H. Association of cigarette smoking and tar and nicotine intake with development of type 2 diabetes mellitus in men and women from the general population: the MONICA/KORA Augsburg Cohort Study. Diabetologia 49 , 1770–1776 (2006).
Huh, Y. et al. Association of smoking status with the risk of type 2 diabetes among young adults: a nationwide cohort study in South Korea. Nicotine Tob. Res. 24 , 1234–1240 (2022).
Sawada, S. S., Lee, I.-M., Muto, T., Matuszaki, K. & Blair, S. N. Cardiorespiratory fitness and the incidence of type 2 diabetes: prospective study of Japanese men. Diabetes Care 26 , 2918–2922 (2003).
Will, J. C., Galuska, D. A., Ford, E. S., Mokdad, A. & Calle, E. E. Cigarette smoking and diabetes mellitus: evidence of a positive association from a large prospective cohort study. Int. J. Epidemiol. 30 , 540–546 (2001).
Nakanishi, N., Nakamura, K., Matsuo, Y., Suzuki, K. & Tatara, K. Cigarette smoking and risk for impaired fasting glucose and type 2 diabetes in middle-aged Japanese men. Ann. Intern. Med. 133 , 183–191 (2000).
Sairenchi, T. et al. Cigarette smoking and risk of type 2 diabetes mellitus among middle-aged and elderly Japanese men and women. Am. J. Epidemiol. 160 , 158–162 (2004).
Hou, X. et al. Cigarette smoking is associated with a lower prevalence of newly diagnosed diabetes screened by OGTT than non-smoking in Chinese men with normal weight. PLoS ONE 11 , e0149234 (2016).
Hu, F. B. et al. Diet, lifestyle, and the risk of type 2 diabetes mellitus in women. N. Engl. J. Med. 345 , 790–797 (2001).
Teratani, T. et al. Dose-response relationship between tobacco or alcohol consumption and the development of diabetes mellitus in Japanese male workers. Drug Alcohol Depend. 125 , 276–282 (2012).
Kawakami, N., Takatsuka, N., Shimizu, H. & Ishibashi, H. Effects of smoking on the incidence of non-insulin-dependent diabetes mellitus. Replication and extension in a Japanese cohort of male employees. Am. J. Epidemiol. 145 , 103–109 (1997).
Patja, K. et al. Effects of smoking, obesity and physical activity on the risk of type 2 diabetes in middle-aged Finnish men and women. J. Intern. Med. 258 , 356–362 (2005).
White, W. B. et al. High-intensity cigarette smoking is associated with incident diabetes mellitus in Black adults: the Jackson Heart Study. J. Am. Heart Assoc. 7 , e007413 (2018).
Uchimoto, S. et al. Impact of cigarette smoking on the incidence of Type 2 diabetes mellitus in middle-aged Japanese men: the Osaka Health Survey. Diabet. Med . 16 , 951–955 (1999).
Rimm, E. B., Chan, J., Stampfer, M. J., Colditz, G. A. & Willett, W. C. Prospective study of cigarette smoking, alcohol use, and the risk of diabetes in men. Br. Med. J. 310 , 555–559 (1995).
Article CAS Google Scholar
Hilawe, E. H. et al. Smoking and diabetes: is the association mediated by adiponectin, leptin, or C-reactive protein? J. Epidemiol. 25 , 99–109 (2015).
InterAct, Consortium et al. Smoking and long-term risk of type 2 diabetes: the EPIC-InterAct study in European populations. Diabetes Care 37 , 3164–3171 (2014).
Jee, S. H., Foong, A. W., Hur, N. W. & Samet, J. M. Smoking and risk for diabetes incidence and mortality in Korean men and women. Diabetes Care 33 , 2567–2572 (2010).
Rasouli, B. et al. Smoking and the risk of LADA: results from a Swedish population-based case-control study. Diabetes Care 39 , 794–800 (2016).
Wannamethee, S. G., Shaper, A. G. & Perry, I. J., British Regional Heart Study. Smoking as a modifiable risk factor for type 2 diabetes in middle-aged men. Diabetes Care 24 , 1590–1595 (2001).
Radzeviciene, L. & Ostrauskas, R. Smoking habits and type 2 diabetes mellitus in women. Women Health 58 , 884–897 (2018).
Carlsson, S., Midthjell, K. & Grill, V., Nord-Trøndelag Study. Smoking is associated with an increased risk of type 2 diabetes but a decreased risk of autoimmune diabetes in adults: an 11-year follow-up of incidence of diabetes in the Nord-Trøndelag study. Diabetologia 47 , 1953–1956 (2004).
Akter, S. et al. Smoking, smoking cessation, and the risk of type 2 diabetes among Japanese adults: Japan Epidemiology Collaboration on Occupational Health Study. PLoS ONE 10 , e0132166 (2015).
Pirie, K. et al. The 21st century hazards of smoking and benefits of stopping: a prospective study of one million women in the UK. Lancet 381 , 133–141 (2013).
Park, C.-H. et al. [The effect of smoking status upon occurrence of impaired fasting glucose or type 2 diabetes in Korean men]. J. Prev. Med. Public Health 41 , 249–254 (2008).
Doi, Y. et al. Two risk score models for predicting incident Type 2 diabetes in Japan. Diabet. Med. 29 , 107–114 (2012).
van den Brandt, P. A. A possible dual effect of cigarette smoking on the risk of postmenopausal breast cancer. Eur. J. Epidemiol. 32 , 683–690 (2017).
Dossus, L. et al. Active and passive cigarette smoking and breast cancer risk: results from the EPIC cohort. Int. J. Cancer 134 , 1871–1888 (2014).
Kawai, M., Malone, K. E., Tang, M.-T. C. & Li, C. I. Active smoking and the risk of estrogen receptor-positive and triple-negative breast cancer among women ages 20 to 44 years. Cancer 120 , 1026–1034 (2014).
Reynolds, P. et al. Active smoking, household passive smoking, and breast cancer: evidence from the California Teachers Study. J. Natl Cancer Inst. 96 , 29–37 (2004).
Ellingjord-Dale, M. et al. Alcohol, physical activity, smoking, and breast cancer subtypes in a large, nested case-control study from the Norwegian Breast Cancer Screening Program. Cancer Epidemiol. Biomark. Prev. 26 , 1736–1744 (2017).
Arthur, R. et al. Association between lifestyle, menstrual/reproductive history, and histological factors and risk of breast cancer in women biopsied for benign breast disease. Breast Cancer Res. Treat. 165 , 623–631 (2017).
Luo, J. et al. Association of active and passive smoking with risk of breast cancer among postmenopausal women: a prospective cohort study. Br. Med. J. 342 , d1016 (2011).
White, A. J., D’Aloisio, A. A., Nichols, H. B., DeRoo, L. A. & Sandler, D. P. Breast cancer and exposure to tobacco smoke during potential windows of susceptibility. Cancer Causes Control 28 , 667–675 (2017).
Gram, I. T. et al. Breast cancer risk among women who start smoking as teenagers. Cancer Epidemiol. Biomark. Prev. 14 , 61–66 (2005).
Gammon, M. D. et al. Cigarette smoking and breast cancer risk among young women (United States). Cancer Causes Control 9 , 583–590 (1998).
Magnusson, C., Wedrén, S. & Rosenberg, L. U. Cigarette smoking and breast cancer risk: a population-based study in Sweden. Br. J. Cancer 97 , 1287–1290 (2007).
Chu, S. Y. et al. Cigarette smoking and the risk of breast cancer. Am. J. Epidemiol. 131 , 244–253 (1990).
Lemogne, C. et al. Depression and the risk of cancer: a 15-year follow-up study of the GAZEL cohort. Am. J. Epidemiol. 178 , 1712–1720 (2013).
Morabia, A., Bernstein, M., Héritier, S. & Khatchatrian, N. Relation of breast cancer with passive and active exposure to tobacco smoke. Am. J. Epidemiol. 143 , 918–928 (1996).
Conlon, M. S. C., Johnson, K. C., Bewick, M. A., Lafrenie, R. M. & Donner, A. Smoking (active and passive), N -acetyltransferase 2, and risk of breast cancer. Cancer Epidemiol. 34 , 142–149 (2010).
Ozasa, K., Japan Collaborative Cohort Study for Evaluation of Cancer. Smoking and mortality in the Japan Collaborative Cohort Study for Evaluation of Cancer (JACC). Asian Pac. J. Cancer Prev. 8 , 89–96 (2007).
Jones, M. E., Schoemaker, M. J., Wright, L. B., Ashworth, A. & Swerdlow, A. J. Smoking and risk of breast cancer in the Generations Study cohort. Breast Cancer Res. 19 , 118 (2017).
Bjerkaas, E. et al. Smoking duration before first childbirth: an emerging risk factor for breast cancer? Results from 302,865 Norwegian women. Cancer Causes Control 24 , 1347–1356 (2013).
Gram, I. T., Little, M. A., Lund, E. & Braaten, T. The fraction of breast cancer attributable to smoking: the Norwegian women and cancer study 1991–2012. Br. J. Cancer 115 , 616–623 (2016).
Li, C. I., Malone, K. E. & Daling, J. R. The relationship between various measures of cigarette smoking and risk of breast cancer among older women 65–79 years of age (United States). Cancer Causes Control 16 , 975–985 (2005).
Xue, F., Willett, W. C., Rosner, B. A., Hankinson, S. E. & Michels, K. B. Cigarette smoking and the incidence of breast cancer. Arch. Intern. Med. 171 , 125–133 (2011).
Parker, A. S., Cerhan, J. R., Putnam, S. D., Cantor, K. P. & Lynch, C. F. A cohort study of farming and risk of prostate cancer in Iowa. Epidemiology 10 , 452–455 (1999).
Sawada, N. et al. Alcohol and smoking and subsequent risk of prostate cancer in Japanese men: the Japan Public Health Center-based prospective study. Int. J. Cancer 134 , 971–978 (2014).
Hiatt, R. A., Armstrong, M. A., Klatsky, A. L. & Sidney, S. Alcohol consumption, smoking, and other risk factors and prostate cancer in a large health plan cohort in California (United States). Cancer Causes Control 5 , 66–72 (1994).
Cerhan, J. R. et al. Association of smoking, body mass, and physical activity with risk of prostate cancer in the Iowa 65+ Rural Health Study (United States). Cancer Causes Control 8 , 229–238 (1997).
Watters, J. L., Park, Y., Hollenbeck, A., Schatzkin, A. & Albanes, D. Cigarette smoking and prostate cancer in a prospective US cohort study. Cancer Epidemiol. Biomark. Prev. 18 , 2427–2435 (2009).
Butler, L. M., Wang, R., Wong, A. S., Koh, W.-P. & Yu, M. C. Cigarette smoking and risk of prostate cancer among Singapore Chinese. Cancer Causes Control 20 , 1967–1974 (2009).
Lotufo, P. A., Lee, I. M., Ajani, U. A., Hennekens, C. H. & Manson, J. E. Cigarette smoking and risk of prostate cancer in the physicians’ health study (United States). Int. J. Cancer 87 , 141–144 (2000).
Hsing, A. W. et al. Diet, tobacco use, and fatal prostate cancer: results from the Lutheran Brotherhood Cohort Study. Cancer Res. 50 , 6836–6840 (1990).
Veierød, M. B., Laake, P. & Thelle, D. S. Dietary fat intake and risk of prostate cancer: a prospective study of 25,708 Norwegian men. Int. J. Cancer 73 , 634–638 (1997).
Meyer, J., Rohrmann, S., Bopp, M. & Faeh, D. & Swiss National Cohort Study Group. Impact of smoking and excess body weight on overall and site-specific cancer mortality risk. Cancer Epidemiol. Biomark. Prev . 24 , 1516–1522 (2015).
Putnam, S. D. et al. Lifestyle and anthropometric risk factors for prostate cancer in a cohort of Iowa men. Ann. Epidemiol. 10 , 361–369 (2000).
Taghizadeh, N., Vonk, J. M. & Boezen, H. M. Lifetime smoking history and cause-specific mortality in a cohort study with 43 years of follow-up. PLoS ONE 11 , e0153310 (2016).
Park, S.-Y. et al. Racial/ethnic differences in lifestyle-related factors and prostate cancer risk: the Multiethnic Cohort Study. Cancer Causes Control 26 , 1507–1515 (2015).
Nomura, A. M., Lee, J., Stemmermann, G. N. & Combs, G. F. Serum selenium and subsequent risk of prostate cancer. Cancer Epidemiol. Biomark. Prev. 9 , 883–887 (2000).
Rodriguez, C., Tatham, L. M., Thun, M. J., Calle, E. E. & Heath, C. W. Smoking and fatal prostate cancer in a large cohort of adult men. Am. J. Epidemiol. 145 , 466–475 (1997).
Rohrmann, S. et al. Smoking and risk of fatal prostate cancer in a prospective U.S. study. Urology 69 , 721–725 (2007).
Giovannucci, E. et al. Smoking and risk of total and fatal prostate cancer in United States health professionals. Cancer Epidemiol. Biomark. Prev. 8 , 277–282 (1999).
Rohrmann, S. et al. Smoking and the risk of prostate cancer in the European Prospective Investigation into Cancer and Nutrition. Br. J. Cancer 108 , 708–714 (2013).
Lund Nilsen, T. I., Johnsen, R. & Vatten, L. J. Socio-economic and lifestyle factors associated with the risk of prostate cancer. Br. J. Cancer 82 , 1358–1363 (2000).
Hsing, A. W., McLaughlin, J. K., Hrubec, Z., Blot, W. J. & Fraumeni, J. F. Tobacco use and prostate cancer: 26-year follow-up of US veterans. Am. J. Epidemiol. 133 , 437–441 (1991).
Murray, C. J. L. et al. Global burden of 87 risk factors in 204 countries and territories, 1990–2019: a systematic analysis for the Global Burden of Disease Study 2019. Lancet 396 , 1223–1249 (2020).
Bero, L. A. & Jadad, A. R. How consumers and policymakers can use systematic reviews for decision making. Ann. Intern. Med. 127 , 37–42 (1997).
Centers for Disease Control and Prevention (CDC). Cigarette smoking among adults and trends in smoking cessation—United States, 2008. MMWR Morb. Mortal. Wkly Rep. 58 , 1227–1232 (2009).
Prochaska, J. O. & Goldstein, M. G. Process of smoking cessation: implications for clinicians. Clin. Chest Med. 12 , 727–735 (1991).
Page, M. J. et al. The PRISMA 2020 statement: an updated guideline for reporting systematic reviews. Br. Med. J. 372 , n71 (2021).
Stevens, G. A. et al. Guidelines for Accurate and Transparent Health Estimates Reporting: the GATHER statement. Lancet 388 , e19–e23 (2016).
BMJ Best Practice. What is GRADE? https://bestpractice.bmj.com/info/us/toolkit/learn-ebm/what-is-grade (BMJ, 2021).
The GRADE Working Group. GRADE handbook . https://gdt.gradepro.org/app/handbook/handbook.html (The GRADE Working Group, 2013).
Efron, B., Hastie, T., Johnstone, I. & Tibshirani, R. Least angle regression. Ann. Stat. 32 , 407–499 (2004).
Tibshirani, R. Regression shrinkage and selection via the lasso. J. R. Stat. Soc. Ser. B Stat. Methodol. 58 , 267–288 (1996).
von Hippel, P. T. The heterogeneity statistic I2 can be biased in small meta-analyses. BMC Med. Res. Methodol. 15 , 35 (2015).
Kontopantelis, E., Springate, D. A. & Reeves, D. A re-analysis of the Cochrane Library data: the dangers of unobserved heterogeneity in meta-analyses. PLoS ONE 8 , e69930 (2013).
Biggerstaff, B. J. & Tweedie, R. L. Incorporating variability in estimates of heterogeneity in the random effects model in meta-analysis. Stat. Med. 16 , 753–768 (1997).
Egger, M., Smith, G. D., Schneider, M. & Minder, C. Bias in meta-analysis detected by a simple, graphical test. Br. Med. J. 315 , 629–634 (1997).
Lee, P. N., Forey, B. A. & Coombs, K. J. Systematic review with meta-analysis of the epidemiological evidence in the 1900s relating smoking to lung cancer. BMC Cancer 12 , 385 (2012).
Rücker, G., Carpenter, J. R. & Schwarzer, G. Detecting and adjusting for small-study effects in meta-analysis. Biometr. J. 53 , 351–368 (2011).
Wu, Z.-J., Zhao, P., Liu, B. & Yuan, Z.-C. Effect of cigarette smoking on risk of hip fracture in men: a meta-analysis of 14 prospective cohort studies. PLoS ONE 11 , e0168990 (2016).
Thun, M. J. et al. in Cigarette Smoking Behaviour in the United States: changes in cigarette-related disease risks and their implication for prevention and control (eds Burns, D.M. et al.) Tobacco Control Monograph No. 8 Ch. 4 (National Cancer Institute, 1997).
Tolstrup, J. S. et al. Smoking and risk of coronary heart disease in younger, middle-aged, and older adults. Am. J. Public Health 104 , 96–102 (2014).
Jonas, M. A., Oates, J. A., Ockene, J. K. & Hennekens, C. H. Statement on smoking and cardiovascular disease for health care professionals. American Heart Association. Circulation 86 , 1664–1669 (1992).
Khan, S. S. et al. Cigarette smoking and competing risks for fatal and nonfatal cardiovascular disease subtypes across the life course. J. Am. Heart Assoc. 10 , e021751 (2021).
Download references
Acknowledgements
Research reported in this publication was supported by the Bill & Melinda Gates Foundation and Bloomberg Philanthropies. The content is solely the responsibility of the authors and does not necessarily represent the official views of the funders. The study funders had no role in study design, data collection, data analysis, data interpretation, writing of the final report or the decision to publish.
We thank the Tobacco Metrics Team Advisory Group for their valuable input and review of the work. The members of the Advisory Group are: P. Allebeck, R. Chandora, J. Drope, M. Eriksen, E. Fernández, H. Gouda, R. Kennedy, D. McGoldrick, L. Pan, K. Schotte, E. Sebrie, J. Soriano, M. Tynan and K. Welding.
Author information
Authors and affiliations.
Institute for Health Metrics and Evaluation, University of Washington, Seattle, WA, USA
Xiaochen Dai, Gabriela F. Gil, Marissa B. Reitsma, Noah S. Ahmad, Jason A. Anderson, Catherine Bisignano, Sinclair Carr, Rachel Feldman, Simon I. Hay, Jiawei He, Vincent Iannucci, Hilary R. Lawlor, Matthew J. Malloy, Laurie B. Marczak, Susan A. McLaughlin, Larissa Morikawa, Erin C. Mullany, Sneha I. Nicholson, Erin M. O’Connell, Chukwuma Okereke, Reed J. D. Sorensen, Joanna Whisnant, Aleksandr Y. Aravkin, Peng Zheng, Christopher J. L. Murray & Emmanuela Gakidou
Department of Health Metrics Sciences, School of Medicine, University of Washington, Seattle, WA, USA
Xiaochen Dai, Simon I. Hay, Jiawei He, Peng Zheng, Christopher J. L. Murray & Emmanuela Gakidou
Department of Applied Mathematics, University of Washington, Seattle, WA, USA
- Aleksandr Y. Aravkin
You can also search for this author in PubMed Google Scholar
Contributions
X.D., S.I.H., S.A.M., E.C.M., E.M.O., C.J.L.M. and E.G. managed the estimation or publications process. X.D. and G.F.G. wrote the first draft of the manuscript. X.D. and P.Z. had primary responsibility for applying analytical methods to produce estimates. X.D., G.F.G., N.S.A., J.A.A., S.C., R.F., V.I., M.J.M., L.M., S.I.N., C.O., M.B.R. and J.W. had primary responsibility for seeking, cataloguing, extracting or cleaning data, and for designing or coding figures and tables. X.D., G.F.G., M.B.R., N.S.A., H.R.L., C.O. and J.W. provided data or critical feedback on data sources. X.D., J.H., R.J.D.S., A.Y.A., P.Z., C.J.L.M. and E.G. developed methods or computational machinery. X.D., G.F.G., M.B.R., S.I.H., J.H., R.J.D.S., A.Y.A., P.Z., C.J.L.M. and E.G. provided critical feedback on methods or results. X.D., G.F.G., M.B.R., C.B., S.I.H., L.B.M., S.A.M., A.Y.A. and E.G. drafted the work or revised it critically for important intellectual content. X.D., S.I.H., L.B.M., E.C.M., E.M.O. and E.G. managed the overall research enterprise.
Corresponding author
Correspondence to Xiaochen Dai .
Ethics declarations
Competing interests.
The authors declare no competing interests.
Peer review
Peer review information.
Nature Medicine thanks Frederic Sitas and the other, anonymous, reviewer(s) for their contribution to the peer review of this work. Primary Handling Editors: Jennifer Sargent and Ming Yang, in collaboration with the Nature Medicine team.
Additional information
Publisher’s note Springer Nature remains neutral with regard to jurisdictional claims in published maps and institutional affiliations.
Extended data
Extended data fig. 1 prisma 2020 flow diagram for an updated systematic review of the smoking and tracheal, bronchus, and lung cancer risk-outcome pair..
The PRISMA flow diagram of an updated systematic review on the relationship between smoking and lung cancer conducted on PubMed to update historical review from previous cycles of the Global Burden of Disease Study. Template is from: Page MJ, McKenzie JE, Bossuyt PM, Boutron I, Hoffmann TC, Mulrow CD, et al. The PRISMA 2020 statement: an updated guideline for reporting systematic reviews. BMJ 2021;372:n71. doi: 10.1136/bmj.n71. For more information, visit: http://www.prisma-statement.org/ .
Extended Data Fig. 2 PRISMA 2020 flow diagram for an updated systematic review of the Smoking and Chronic obstructive pulmonary disease risk-outcome pair.
The PRISMA flow diagram of an updated systematic review on the relationship between smoking and chronic obstructive pulmonary disease conducted on PubMed to update historical review from previous cycles of the Global Burden of Disease Study. Template is from: Page MJ, McKenzie JE, Bossuyt PM, Boutron I, Hoffmann TC, Mulrow CD, et al. The PRISMA 2020 statement: an updated guideline for reporting systematic reviews. BMJ 2021;372:n71. doi: 10.1136/bmj.n71. For more information, visit: http://www.prisma-statement.org/ .
Extended Data Fig. 3 PRISMA 2020 flow diagram for an updated systematic review of the Smoking and Diabetes mellitus type 2 risk- outcome pair.
The PRISMA flow diagram of an updated systematic review on the relationship between smoking and type 2 diabetes conducted on PubMed to update historical review from previous cycles of the Global Burden of Disease Study. Template is from: Page MJ, McKenzie JE, Bossuyt PM, Boutron I, Hoffmann TC, Mulrow CD, et al. The PRISMA 2020 statement: an updated guideline for reporting systematic reviews. BMJ 2021;372:n71. doi: 10.1136/bmj.n71. For more information, visit: http://www.prisma-statement.org/ .
Extended Data Fig. 4 PRISMA 2020 flow diagram for an updated systematic review of the Smoking and Breast cancer risk-outcome pair.
The PRISMA flow diagram of an updated systematic review on the relationship between smoking and breast cancer conducted on PubMed to update historical review from previous cycles of the Global Burden of Disease Study. Template is from: Page MJ, McKenzie JE, Bossuyt PM, Boutron I, Hoffmann TC, Mulrow CD, et al. The PRISMA 2020 statement: an updated guideline for reporting systematic reviews. BMJ 2021;372:n71. doi: 10.1136/bmj.n71. For more information, visit: http://www.prisma-statement.org/ .
Extended Data Fig. 5 PRISMA 2020 flow diagram for an updated systematic review of the Smoking and Prostate cancer risk-outcome pair.
The PRISMA flow diagram of an updated systematic review on the relationship between smoking and prostate cancer conducted on PubMed to update historical review from previous cycles of the Global Burden of Disease Study. Template is from: Page MJ, McKenzie JE, Bossuyt PM, Boutron I, Hoffmann TC, Mulrow CD, et al. The PRISMA 2020 statement: an updated guideline for reporting systematic reviews. BMJ 2021;372:n71. doi: 10.1136/bmj.n71. For more information, visit: http://www.prisma-statement.org/ .
Extended Data Fig. 6 Smoking and Breast Cancer.
a , log-relative risk function. b , relative risk function. c , A modified funnel plot showing the residuals (relative to 0) on the x-axis and the estimated standard deviation (SD) that includes reported SD and between-study heterogeneity on the y-axis.
Supplementary information
Supplementary information.
Supplementary Information 1: Data source identification and assessment. Supplementary Information 2: Data inputs. Supplementary Information 3: Study quality and bias assessment. Supplementary Information 4: The dose–response RR curves and their 95% UIs for all smoking–outcome pairs. Supplementary Information 5: Supplementary methods. Supplementary Information 6: Sensitivity analysis. Supplementary Information 7: Binary smoking–outcome pair. Supplementary Information 8: Risk curve details. Supplementary Information 9: GATHER and PRISMA checklists.
Rights and permissions
Open Access This article is licensed under a Creative Commons Attribution 4.0 International License, which permits use, sharing, adaptation, distribution and reproduction in any medium or format, as long as you give appropriate credit to the original author(s) and the source, provide a link to the Creative Commons license, and indicate if changes were made. The images or other third party material in this article are included in the article’s Creative Commons license, unless indicated otherwise in a credit line to the material. If material is not included in the article’s Creative Commons license and your intended use is not permitted by statutory regulation or exceeds the permitted use, you will need to obtain permission directly from the copyright holder. To view a copy of this license, visit http://creativecommons.org/licenses/by/4.0/ .
Reprints and permissions
About this article
Cite this article.
Dai, X., Gil, G.F., Reitsma, M.B. et al. Health effects associated with smoking: a Burden of Proof study. Nat Med 28 , 2045–2055 (2022). https://doi.org/10.1038/s41591-022-01978-x
Download citation
Received : 11 April 2022
Accepted : 28 July 2022
Published : 10 October 2022
Issue Date : October 2022
DOI : https://doi.org/10.1038/s41591-022-01978-x
Share this article
Anyone you share the following link with will be able to read this content:
Sorry, a shareable link is not currently available for this article.
Provided by the Springer Nature SharedIt content-sharing initiative
This article is cited by
- Gabriela F. Gil
- Jason A. Anderson
- Emmanuela Gakidou
Nature Communications (2024)
- Luisa S. Flor
Nature Medicine (2024)
Metabolic profiling of smoking, associations with type 2 diabetes and interaction with genetic susceptibility
- Sofia Carlsson
European Journal of Epidemiology (2024)
Global burden of prostate cancer attributable to smoking among males in 204 countries and territories, 1990–2019
- Hanfei Zhang
- Dingping Huang
- Daqing Hong
BMC Cancer (2023)
Reply to: Concerns about the Burden of Proof studies
- Susan A. McLaughlin
- Christopher J. L. Murray
Nature Medicine (2023)
Quick links
- Explore articles by subject
- Guide to authors
- Editorial policies
Sign up for the Nature Briefing: Cancer newsletter — what matters in cancer research, free to your inbox weekly.

Loading metrics
Open Access
Peer-reviewed
Research Article
Assessing the impact of healthcare research: A systematic review of methodological frameworks
Roles Conceptualization, Data curation, Formal analysis, Funding acquisition, Investigation, Methodology, Validation, Writing – original draft, Writing – review & editing
Affiliation Centre for Patient Reported Outcomes Research, Institute of Applied Health Research, College of Medical and Dental Sciences, University of Birmingham, Birmingham, United Kingdom

Roles Conceptualization, Formal analysis, Funding acquisition, Methodology, Project administration, Supervision, Validation, Writing – review & editing
* E-mail: [email protected]
Roles Data curation, Formal analysis, Methodology, Validation, Writing – review & editing
Roles Formal analysis, Methodology, Supervision, Validation, Writing – review & editing
- Samantha Cruz Rivera,
- Derek G. Kyte,
- Olalekan Lee Aiyegbusi,
- Thomas J. Keeley,
- Melanie J. Calvert
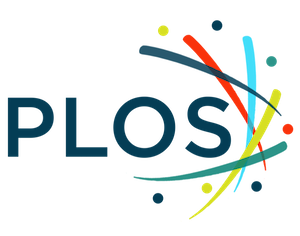
- Published: August 9, 2017
- https://doi.org/10.1371/journal.pmed.1002370
- Reader Comments
Increasingly, researchers need to demonstrate the impact of their research to their sponsors, funders, and fellow academics. However, the most appropriate way of measuring the impact of healthcare research is subject to debate. We aimed to identify the existing methodological frameworks used to measure healthcare research impact and to summarise the common themes and metrics in an impact matrix.
Methods and findings
Two independent investigators systematically searched the Medical Literature Analysis and Retrieval System Online (MEDLINE), the Excerpta Medica Database (EMBASE), the Cumulative Index to Nursing and Allied Health Literature (CINAHL+), the Health Management Information Consortium, and the Journal of Research Evaluation from inception until May 2017 for publications that presented a methodological framework for research impact. We then summarised the common concepts and themes across methodological frameworks and identified the metrics used to evaluate differing forms of impact. Twenty-four unique methodological frameworks were identified, addressing 5 broad categories of impact: (1) ‘primary research-related impact’, (2) ‘influence on policy making’, (3) ‘health and health systems impact’, (4) ‘health-related and societal impact’, and (5) ‘broader economic impact’. These categories were subdivided into 16 common impact subgroups. Authors of the included publications proposed 80 different metrics aimed at measuring impact in these areas. The main limitation of the study was the potential exclusion of relevant articles, as a consequence of the poor indexing of the databases searched.
Conclusions
The measurement of research impact is an essential exercise to help direct the allocation of limited research resources, to maximise research benefit, and to help minimise research waste. This review provides a collective summary of existing methodological frameworks for research impact, which funders may use to inform the measurement of research impact and researchers may use to inform study design decisions aimed at maximising the short-, medium-, and long-term impact of their research.
Author summary
Why was this study done.
- There is a growing interest in demonstrating the impact of research in order to minimise research waste, allocate resources efficiently, and maximise the benefit of research. However, there is no consensus on which is the most appropriate tool to measure the impact of research.
- To our knowledge, this review is the first to synthesise existing methodological frameworks for healthcare research impact, and the associated impact metrics by which various authors have proposed impact should be measured, into a unified matrix.
What did the researchers do and find?
- We conducted a systematic review identifying 24 existing methodological research impact frameworks.
- We scrutinised the sample, identifying and summarising 5 proposed impact categories, 16 impact subcategories, and over 80 metrics into an impact matrix and methodological framework.
What do these findings mean?
- This simplified consolidated methodological framework will help researchers to understand how a research study may give rise to differing forms of impact, as well as in what ways and at which time points these potential impacts might be measured.
- Incorporating these insights into the design of a study could enhance impact, optimizing the use of research resources.
Citation: Cruz Rivera S, Kyte DG, Aiyegbusi OL, Keeley TJ, Calvert MJ (2017) Assessing the impact of healthcare research: A systematic review of methodological frameworks. PLoS Med 14(8): e1002370. https://doi.org/10.1371/journal.pmed.1002370
Academic Editor: Mike Clarke, Queens University Belfast, UNITED KINGDOM
Received: February 28, 2017; Accepted: July 7, 2017; Published: August 9, 2017
Copyright: © 2017 Cruz Rivera et al. This is an open access article distributed under the terms of the Creative Commons Attribution License , which permits unrestricted use, distribution, and reproduction in any medium, provided the original author and source are credited.
Data Availability: All relevant data are within the paper and supporting files.
Funding: Funding was received from Consejo Nacional de Ciencia y Tecnología (CONACYT). The funders had no role in study design, data collection and analysis, decision to publish, or preparation of the manuscript ( http://www.conacyt.mx/ ).
Competing interests: I have read the journal's policy and the authors of this manuscript have the following competing interests: MJC has received consultancy fees from Astellas and Ferring pharma and travel fees from the European Society of Cardiology outside the submitted work. TJK is in full-time paid employment for PAREXEL International.
Abbreviations: AIHS, Alberta Innovates—Health Solutions; CAHS, Canadian Academy of Health Sciences; CIHR, Canadian Institutes of Health Research; CINAHL+, Cumulative Index to Nursing and Allied Health Literature; EMBASE, Excerpta Medica Database; ERA, Excellence in Research for Australia; HEFCE, Higher Education Funding Council for England; HMIC, Health Management Information Consortium; HTA, Health Technology Assessment; IOM, Impact Oriented Monitoring; MDG, Millennium Development Goal; NHS, National Health Service; MEDLINE, Medical Literature Analysis and Retrieval System Online; PHC RIS, Primary Health Care Research & Information Service; PRISMA, Preferred Reporting Items for Systematic Reviews and Meta-Analyses; PROM, patient-reported outcome measures; QALY, quality-adjusted life year; R&D, research and development; RAE, Research Assessment Exercise; REF, Research Excellence Framework; RIF, Research Impact Framework; RQF, Research Quality Framework; SDG, Sustainable Development Goal; SIAMPI, Social Impact Assessment Methods for research and funding instruments through the study of Productive Interactions between science and society
Introduction
In 2010, approximately US$240 billion was invested in healthcare research worldwide [ 1 ]. Such research is utilised by policy makers, healthcare providers, and clinicians to make important evidence-based decisions aimed at maximising patient benefit, whilst ensuring that limited healthcare resources are used as efficiently as possible to facilitate effective and sustainable service delivery. It is therefore essential that this research is of high quality and that it is impactful—i.e., it delivers demonstrable benefits to society and the wider economy whilst minimising research waste [ 1 , 2 ]. Research impact can be defined as ‘any identifiable ‘benefit to, or positive influence on the economy, society, public policy or services, health, the environment, quality of life or academia’ (p. 26) [ 3 ].
There are many purported benefits associated with the measurement of research impact, including the ability to (1) assess the quality of the research and its subsequent benefits to society; (2) inform and influence optimal policy and funding allocation; (3) demonstrate accountability, the value of research in terms of efficiency and effectiveness to the government, stakeholders, and society; and (4) maximise impact through better understanding the concept and pathways to impact [ 4 – 7 ].
Measuring and monitoring the impact of healthcare research has become increasingly common in the United Kingdom [ 5 ], Australia [ 5 ], and Canada [ 8 ], as governments, organisations, and higher education institutions seek a framework to allocate funds to projects that are more likely to bring the most benefit to society and the economy [ 5 ]. For example, in the UK, the 2014 Research Excellence Framework (REF) has recently been used to assess the quality and impact of research in higher education institutions, through the assessment of impact cases studies and selected qualitative impact metrics [ 9 ]. This is the first initiative to allocate research funding based on the economic, societal, and cultural impact of research, although it should be noted that research impact only drives a proportion of this allocation (approximately 20%) [ 9 ].
In the UK REF, the measurement of research impact is seen as increasingly important. However, the impact element of the REF has been criticised in some quarters [ 10 , 11 ]. Critics deride the fact that REF impact is determined in a relatively simplistic way, utilising researcher-generated case studies, which commonly attempt to link a particular research outcome to an associated policy or health improvement despite the fact that the wider literature highlights great diversity in the way research impact may be demonstrated [ 12 , 13 ]. This led to the current debate about the optimal method of measuring impact in the future REF [ 10 , 14 ]. The Stern review suggested that research impact should not only focus on socioeconomic impact but should also include impact on government policy, public engagement, academic impacts outside the field, and teaching to showcase interdisciplinary collaborative impact [ 10 , 11 ]. The Higher Education Funding Council for England (HEFCE) has recently set out the proposals for the REF 2021 exercise, confirming that the measurement of such impact will continue to form an important part of the process [ 15 ].
With increasing pressure for healthcare research to lead to demonstrable health, economic, and societal impact, there is a need for researchers to understand existing methodological impact frameworks and the means by which impact may be quantified (i.e., impact metrics; see Box 1 , 'Definitions’) to better inform research activities and funding decisions. From a researcher’s perspective, understanding the optimal pathways to impact can help inform study design aimed at maximising the impact of the project. At the same time, funders need to understand which aspects of impact they should focus on when allocating awards so they can make the most of their investment and bring the greatest benefit to patients and society [ 2 , 4 , 5 , 16 , 17 ].
Box 1. Definitions
- Research impact: ‘any identifiable benefit to, or positive influence on, the economy, society, public policy or services, health, the environment, quality of life, or academia’ (p. 26) [ 3 ].
- Methodological framework: ‘a body of methods, rules and postulates employed by a particular procedure or set of procedures (i.e., framework characteristics and development)’ [ 18 ].
- Pathway: ‘a way of achieving a specified result; a course of action’ [ 19 ].
- Quantitative metrics: ‘a system or standard of [quantitative] measurement’ [ 20 ].
- Narrative metrics: ‘a spoken or written account of connected events; a story’ [ 21 ].
Whilst previous researchers have summarised existing methodological frameworks and impact case studies [ 4 , 22 – 27 ], they have not summarised the metrics for use by researchers, funders, and policy makers. The aim of this review was therefore to (1) identify the methodological frameworks used to measure healthcare research impact using systematic methods, (2) summarise common impact themes and metrics in an impact matrix, and (3) provide a simplified consolidated resource for use by funders, researchers, and policy makers.
Search strategy and selection criteria
Initially, a search strategy was developed to identify the available literature regarding the different methods to measure research impact. The following keywords: ‘Impact’, ‘Framework’, and ‘Research’, and their synonyms, were used during the search of the Medical Literature Analysis and Retrieval System Online (MEDLINE; Ovid) database, the Excerpta Medica Database (EMBASE), the Health Management Information Consortium (HMIC) database, and the Cumulative Index to Nursing and Allied Health Literature (CINAHL+) database (inception to May 2017; see S1 Appendix for the full search strategy). Additionally, the nonindexed Journal of Research Evaluation was hand searched during the same timeframe using the keyword ‘Impact’. Other relevant articles were identified through 3 Internet search engines (Google, Google Scholar, and Google Images) using the keywords ‘Impact’, ‘Framework’, and ‘Research’, with the first 50 results screened. Google Images was searched because different methodological frameworks are summarised in a single image and can easily be identified through this search engine. Finally, additional publications were sought through communication with experts.
Following Preferred Reporting Items for Systematic Reviews and Meta-Analyses (PRISMA) guidelines (see S1 PRISMA Checklist ), 2 independent investigators systematically screened for publications describing, evaluating, or utilising a methodological research impact framework within the context of healthcare research [ 28 ]. Papers were eligible if they included full or partial methodological frameworks or pathways to research impact; both primary research and systematic reviews fitting these criteria were included. We included any methodological framework identified (original or modified versions) at the point of first occurrence. In addition, methodological frameworks were included if they were applicable to the healthcare discipline with no need of modification within their structure. We defined ‘methodological framework’ as ‘a body of methods, rules and postulates employed by a particular procedure or set of procedures (i.e., framework characteristics and development)’ [ 18 ], whereas we defined ‘pathway’ as ‘a way of achieving a specified result; a course of action’ [ 19 ]. Studies were excluded if they presented an existing (unmodified) methodological framework previously available elsewhere, did not explicitly describe a methodological framework but rather focused on a single metric (e.g., bibliometric analysis), focused on the impact or effectiveness of interventions rather than that of the research, or presented case study data only. There were no language restrictions.
Data screening
Records were downloaded into Endnote (version X7.3.1), and duplicates were removed. Two independent investigators (SCR and OLA) conducted all screening following a pilot aimed at refining the process. The records were screened by title and abstract before full-text articles of potentially eligible publications were retrieved for evaluation. A full-text screening identified the publications included for data extraction. Discrepancies were resolved through discussion, with the involvement of a third reviewer (MJC, DGK, and TJK) when necessary.
Data extraction and analysis
Data extraction occurred after the final selection of included articles. SCR and OLA independently extracted details of impact methodological frameworks, the country of origin, and the year of publication, as well as the source, the framework description, and the methodology used to develop the framework. Information regarding the methodology used to develop each methodological framework was also extracted from framework webpages where available. Investigators also extracted details regarding each framework’s impact categories and subgroups, along with their proposed time to impact (‘short-term’, ‘mid-term’, or ‘long-term’) and the details of any metrics that had been proposed to measure impact, which are depicted in an impact matrix. The structure of the matrix was informed by the work of M. Buxton and S. Hanney [ 2 ], P. Buykx et al. [ 5 ], S. Kuruvila et al. [ 29 ], and A. Weiss [ 30 ], with the intention of mapping metrics presented in previous methodological frameworks in a concise way. A consensus meeting with MJC, DGK, and TJK was held to solve disagreements and finalise the data extraction process.
Included studies
Our original search strategy identified 359 citations from MEDLINE (Ovid), EMBASE, CINAHL+, HMIC, and the Journal of Research Evaluation, and 101 citations were returned using other sources (Google, Google Images, Google Scholar, and expert communication) (see Fig 1 ) [ 28 ]. In total, we retrieved 54 full-text articles for review. At this stage, 39 articles were excluded, as they did not propose new or modified methodological frameworks. An additional 15 articles were included following the backward and forward citation method. A total of 31 relevant articles were included in the final analysis, of which 24 were articles presenting unique frameworks and the remaining 7 were systematic reviews [ 4 , 22 – 27 ]. The search strategy was rerun on 15 May 2017. A further 19 publications were screened, and 2 were taken forward to full-text screening but were ineligible for inclusion.
- PPT PowerPoint slide
- PNG larger image
- TIFF original image
https://doi.org/10.1371/journal.pmed.1002370.g001
Methodological framework characteristics
The characteristics of the 24 included methodological frameworks are summarised in Table 1 , 'Methodological framework characteristics’. Fourteen publications proposed academic-orientated frameworks, which focused on measuring academic, societal, economic, and cultural impact using narrative and quantitative metrics [ 2 , 3 , 5 , 8 , 29 , 31 – 39 ]. Five publications focused on assessing the impact of research by focusing on the interaction process between stakeholders and researchers (‘productive interactions’), which is a requirement to achieve research impact. This approach tries to address the issue of attributing research impact to metrics [ 7 , 40 – 43 ]. Two frameworks focused on the importance of partnerships between researchers and policy makers, as a core element to accomplish research impact [ 44 , 45 ]. An additional 2 frameworks focused on evaluating the pathways to impact, i.e., linking processes between research and impact [ 30 , 46 ]. One framework assessed the ability of health technology to influence efficiency of healthcare systems [ 47 ]. Eight frameworks were developed in the UK [ 2 , 3 , 29 , 37 , 39 , 42 , 43 , 45 ], 6 in Canada [ 8 , 33 , 34 , 44 , 46 , 47 ], 4 in Australia [ 5 , 31 , 35 , 38 ], 3 in the Netherlands [ 7 , 40 , 41 ], and 2 in the United States [ 30 , 36 ], with 1 model developed with input from various countries [ 32 ].
https://doi.org/10.1371/journal.pmed.1002370.t001
Methodological framework development
The included methodological frameworks varied in their development process, but there were some common approaches employed. Most included a literature review [ 2 , 5 , 7 , 8 , 31 , 33 , 36 , 37 , 40 – 46 ], although none of them used a recognised systematic method. Most also consulted with various stakeholders [ 3 , 8 , 29 , 31 , 33 , 35 – 38 , 43 , 44 , 46 , 47 ] but used differing methods to incorporate their views, including quantitative surveys [ 32 , 35 , 43 , 46 ], face-to-face interviews [ 7 , 29 , 33 , 35 , 37 , 42 , 43 ], telephone interviews [ 31 , 46 ], consultation [ 3 , 7 , 36 ], and focus groups [ 39 , 43 ]. A range of stakeholder groups were approached across the sample, including principal investigators [ 7 , 29 , 43 ], research end users [ 7 , 42 , 43 ], academics [ 3 , 8 , 39 , 40 , 43 , 46 ], award holders [ 43 ], experts [ 33 , 38 , 39 ], sponsors [ 33 , 39 ], project coordinators [ 32 , 42 ], and chief investigators [ 31 , 35 ]. However, some authors failed to identify the stakeholders involved in the development of their frameworks [ 2 , 5 , 34 , 41 , 45 ], making it difficult to assess their appropriateness. In addition, only 4 of the included papers reported using formal analytic methods to interpret stakeholder responses. These included the Canadian Academy of Health Sciences framework, which used conceptual cluster analysis [ 33 ]. The Research Contribution [ 42 ], Research Impact [ 29 ], and Primary Health Care & Information Service [ 31 ] used a thematic analysis approach. Finally, some authors went on to pilot their framework, which shaped refinements on the methodological frameworks until approval. Methods used to pilot the frameworks included a case study approach [ 2 , 3 , 30 , 32 , 33 , 36 , 40 , 42 , 44 , 45 ], contrasting results against available literature [ 29 ], the use of stakeholders’ feedback [ 7 ], and assessment tools [ 35 , 46 ].
Major impact categories
1. primary research-related impact..
A number of methodological frameworks advocated the evaluation of ‘research-related impact’. This encompassed content related to the generation of new knowledge, knowledge dissemination, capacity building, training, leadership, and the development of research networks. These outcomes were considered the direct or primary impacts of a research project, as these are often the first evidenced returns [ 30 , 62 ].
A number of subgroups were identified within this category, with frameworks supporting the collection of impact data across the following constructs: ‘research and innovation outcomes’; ‘dissemination and knowledge transfer’; ‘capacity building, training, and leadership’; and ‘academic collaborations, research networks, and data sharing’.
1 . 1 . Research and innovation outcomes . Twenty of the 24 frameworks advocated the evaluation of ‘research and innovation outcomes’ [ 2 , 3 , 5 , 7 , 8 , 29 – 39 , 41 , 43 , 44 , 46 ]. This subgroup included the following metrics: number of publications; number of peer-reviewed articles (including journal impact factor); citation rates; requests for reprints, number of reviews, and meta-analysis; and new or changes in existing products (interventions or technology), patents, and research. Additionally, some frameworks also sought to gather information regarding ‘methods/methodological contributions’. These advocated the collection of systematic reviews and appraisals in order to identify gaps in knowledge and determine whether the knowledge generated had been assessed before being put into practice [ 29 ].
1 . 2 . Dissemination and knowledge transfer . Nineteen of the 24 frameworks advocated the assessment of ‘dissemination and knowledge transfer’ [ 2 , 3 , 5 , 7 , 29 – 32 , 34 – 43 , 46 ]. This comprised collection of the following information: number of conferences, seminars, workshops, and presentations; teaching output (i.e., number of lectures given to disseminate the research findings); number of reads for published articles; article download rate and number of journal webpage visits; and citations rates in nonjournal media such as newspapers and mass and social media (i.e., Twitter and blogs). Furthermore, this impact subgroup considered the measurement of research uptake and translatability and the adoption of research findings in technological and clinical applications and by different fields. These can be measured through patents, clinical trials, and partnerships between industry and business, government and nongovernmental organisations, and university research units and researchers [ 29 ].
1 . 3 . Capacity building , training , and leadership . Fourteen of 24 frameworks suggested the evaluation of ‘capacity building, training, and leadership’ [ 2 , 3 , 5 , 8 , 29 , 31 – 35 , 39 – 41 , 43 ]. This involved collecting information regarding the number of doctoral and postdoctoral studentships (including those generated as a result of the research findings and those appointed to conduct the research), as well as the number of researchers and research-related staff involved in the research projects. In addition, authors advocated the collection of ‘leadership’ metrics, including the number of research projects managed and coordinated and the membership of boards and funding bodies, journal editorial boards, and advisory committees [ 29 ]. Additional metrics in this category included public recognition (number of fellowships and awards for significant research achievements), academic career advancement, and subsequent grants received. Lastly, the impact metric ‘research system management’ comprised the collection of information that can lead to preserving the health of the population, such as modifying research priorities, resource allocation strategies, and linking health research to other disciplines to maximise benefits [ 29 ].
1 . 4 . Academic collaborations , research networks , and data sharing . Lastly, 10 of the 24 frameworks advocated the collection of impact data regarding ‘academic collaborations (internal and external collaborations to complete a research project), research networks, and data sharing’ [ 2 , 3 , 5 , 7 , 29 , 34 , 37 , 39 , 41 , 43 ].
2. Influence on policy making.
Methodological frameworks addressing this major impact category focused on measurable improvements within a given knowledge base and on interactions between academics and policy makers, which may influence policy-making development and implementation. The returns generated in this impact category are generally considered as intermediate or midterm (1 to 3 years). These represent an important interim stage in the process towards the final expected impacts, such as quantifiable health improvements and economic benefits, without which policy change may not occur [ 30 , 62 ]. The following impact subgroups were identified within this category: ‘type and nature of policy impact’, ‘level of policy making’, and ‘policy networks’.
2 . 1 . Type and nature of policy impact . The most common impact subgroup, mentioned in 18 of the 24 frameworks, was ‘type and nature of policy impact’ [ 2 , 7 , 29 – 38 , 41 – 43 , 45 – 47 ]. Methodological frameworks addressing this subgroup stressed the importance of collecting information regarding the influence of research on policy (i.e., changes in practice or terminology). For instance, a project looking at trafficked adolescents and women (2003) influenced the WHO guidelines (2003) on ethics regarding this particular group [ 17 , 21 , 63 ].
2 . 2 . Level of policy impact . Thirteen of 24 frameworks addressed aspects surrounding the need to record the ‘level of policy impact’ (international, national, or local) and the organisations within a level that were influenced (local policy makers, clinical commissioning groups, and health and wellbeing trusts) [ 2 , 5 , 8 , 29 , 31 , 34 , 38 , 41 , 43 – 47 ]. Authors considered it important to measure the ‘level of policy impact’ to provide evidence of collaboration, coordination, and efficiency within health organisations and between researchers and health organisations [ 29 , 31 ].
2 . 3 . Policy networks . Five methodological frameworks highlighted the need to collect information regarding collaborative research with industry and staff movement between academia and industry [ 5 , 7 , 29 , 41 , 43 ]. A policy network emphasises the relationship between policy communities, researchers, and policy makers. This relationship can influence and lead to incremental changes in policy processes [ 62 ].
3. Health and health systems impact.
A number of methodological frameworks advocated the measurement of impacts on health and healthcare systems across the following impact subgroups: ‘quality of care and service delivering’, ‘evidence-based practice’, ‘improved information and health information management’, ‘cost containment and effectiveness’, ‘resource allocation’, and ‘health workforce’.
3 . 1 . Quality of care and service delivery . Twelve of the 24 frameworks highlighted the importance of evaluating ‘quality of care and service delivery’ [ 2 , 5 , 8 , 29 – 31 , 33 – 36 , 41 , 47 ]. There were a number of suggested metrics that could be potentially used for this purpose, including health outcomes such as quality-adjusted life years (QALYs), patient-reported outcome measures (PROMs), patient satisfaction and experience surveys, and qualitative data on waiting times and service accessibility.
3 . 2 . Evidence-based practice . ‘Evidence-based practice’, mentioned in 5 of the 24 frameworks, refers to making changes in clinical diagnosis, clinical practice, treatment decisions, or decision making based on research evidence [ 5 , 8 , 29 , 31 , 33 ]. The suggested metrics to demonstrate evidence-based practice were adoption of health technologies and research outcomes to improve the healthcare systems and inform policies and guidelines [ 29 ].
3 . 3 . Improved information and health information management . This impact subcategory, mentioned in 5 of the 24 frameworks, refers to the influence of research on the provision of health services and management of the health system to prevent additional costs [ 5 , 29 , 33 , 34 , 38 ]. Methodological frameworks advocated the collection of health system financial, nonfinancial (i.e., transport and sociopolitical implications), and insurance information in order to determine constraints within a health system.
3 . 4 . Cost containment and cost-effectiveness . Six of the 24 frameworks advocated the subcategory ‘cost containment and cost-effectiveness’ [ 2 , 5 , 8 , 17 , 33 , 36 ]. ‘Cost containment’ comprised the collection of information regarding how research has influenced the provision and management of health services and its implication in healthcare resource allocation and use [ 29 ]. ‘Cost-effectiveness’ refers to information concerning economic evaluations to assess improvements in effectiveness and health outcomes—for instance, the cost-effectiveness (cost and health outcome benefits) assessment of introducing a new health technology to replace an older one [ 29 , 31 , 64 ].
3 . 5 . Resource allocation . ‘Resource allocation’, mentioned in 6frameworks, can be measured through 2 impact metrics: new funding attributed to the intervention in question and equity while allocating resources, such as improved allocation of resources at an area level; better targeting, accessibility, and utilisation; and coverage of health services [ 2 , 5 , 29 , 31 , 45 , 47 ]. The allocation of resources and targeting can be measured through health services research reports, with the utilisation of health services measured by the probability of providing an intervention when needed, the probability of requiring it again in the future, and the probability of receiving an intervention based on previous experience [ 29 , 31 ].
3 . 6 . Health workforce . Lastly, ‘health workforce’, present in 3 methodological frameworks, refers to the reduction in the days of work lost because of a particular illness [ 2 , 5 , 31 ].
4. Health-related and societal impact.
Three subgroups were included in this category: ‘health literacy’; ‘health knowledge, attitudes, and behaviours’; and ‘improved social equity, inclusion, or cohesion’.
4 . 1 . Health knowledge , attitudes , and behaviours . Eight of the 24 frameworks suggested the assessment of ‘health knowledge, attitudes, behaviours, and outcomes’, which could be measured through the evaluation of levels of public engagement with science and research (e.g., National Health Service (NHS) Choices end-user visit rate) or by using focus groups to analyse changes in knowledge, attitudes, and behaviour among society [ 2 , 5 , 29 , 33 – 35 , 38 , 43 ].
4 . 2 . Improved equity , inclusion , or cohesion and human rights . Other methodological frameworks, 4 of the 24, suggested capturing improvements in equity, inclusion, or cohesion and human rights. Authors suggested these could be using a resource like the United Nations Millennium Development Goals (MDGs) (superseded by Sustainable Development Goals [SDGs] in 2015) and human rights [ 29 , 33 , 34 , 38 ]. For instance, a cluster-randomised controlled trial in Nepal, which had female participants, has demonstrated the reduction of neonatal mortality through the introduction of maternity health care, distribution of delivery kits, and home visits. This illustrates how research can target vulnerable and disadvantaged groups. Additionally, this research has been introduced by the World Health Organisation to achieve the MDG ‘improve maternal health’ [ 16 , 29 , 65 ].
4 . 3 . Health literacy . Some methodological frameworks, 3 of the 24, focused on tracking changes in the ability of patients to make informed healthcare decisions, reduce health risks, and improve quality of life, which were demonstrably linked to a particular programme of research [ 5 , 29 , 43 ]. For example, a systematic review showed that when HIV health literacy/knowledge is spread among people living with the condition, antiretroviral adherence and quality of life improve [ 66 ].
5. Broader economic impacts.
Some methodological frameworks, 9 of 24, included aspects related to the broader economic impacts of health research—for example, the economic benefits emerging from the commercialisation of research outputs [ 2 , 5 , 29 , 31 , 33 , 35 , 36 , 38 , 67 ]. Suggested metrics included the amount of funding for research and development (R&D) that was competitively awarded by the NHS, medical charities, and overseas companies. Additional metrics were income from intellectual property, spillover effects (any secondary benefit gained as a repercussion of investing directly in a primary activity, i.e., the social and economic returns of investing on R&D) [ 33 ], patents granted, licences awarded and brought to the market, the development and sales of spinout companies, research contracts, and income from industry.
The benefits contained within the categories ‘health and health systems impact’, ‘health-related and societal impact’, and ‘broader economic impacts’ are considered the expected and final returns of the resources allocated in healthcare research [ 30 , 62 ]. These benefits commonly arise in the long term, beyond 5 years according to some authors, but there was a recognition that this could differ depending on the project and its associated research area [ 4 ].
Data synthesis
Five major impact categories were identified across the 24 included methodological frameworks: (1) ‘primary research-related impact’, (2) ‘influence on policy making’, (3) ‘health and health systems impact’, (4) ‘health-related and societal impact’, and (5) ‘broader economic impact’. These major impact categories were further subdivided into 16 impact subgroups. The included publications proposed 80 different metrics to measure research impact. This impact typology synthesis is depicted in ‘the impact matrix’ ( Fig 2 and Fig 3 ).
CIHR, Canadian Institutes of Health Research; HTA, Health Technology Assessment; PHC RIS, Primary Health Care Research & Information Service; RAE, Research Assessment Exercise; RQF, Research Quality Framework.
https://doi.org/10.1371/journal.pmed.1002370.g002
AIHS, Alberta Innovates—Health Solutions; CAHS, Canadian Institutes of Health Research; IOM, Impact Oriented Monitoring; REF, Research Excellence Framework; SIAMPI, Social Impact Assessment Methods for research and funding instruments through the study of Productive Interactions between science and society.
https://doi.org/10.1371/journal.pmed.1002370.g003
Commonality and differences across frameworks
The ‘Research Impact Framework’ and the ‘Health Services Research Impact Framework’ were the models that encompassed the largest number of the metrics extracted. The most dominant methodological framework was the Payback Framework; 7 other methodological framework models used the Payback Framework as a starting point for development [ 8 , 29 , 31 – 35 ]. Additional methodological frameworks that were commonly incorporated into other tools included the CIHR framework, the CAHS model, the AIHS framework, and the Exchange model [ 8 , 33 , 34 , 44 ]. The capture of ‘research-related impact’ was the most widely advocated concept across methodological frameworks, illustrating the importance with which primary short-term impact outcomes were viewed by the included papers. Thus, measurement of impact via number of publications, citations, and peer-reviewed articles was the most common. ‘Influence on policy making’ was the predominant midterm impact category, specifically the subgroup ‘type and nature of policy impact’, in which frameworks advocated the measurement of (i) changes to legislation, regulations, and government policy; (ii) influence and involvement in decision-making processes; and (iii) changes to clinical or healthcare training, practice, or guidelines. Within more long-term impact measurement, the evaluations of changes in the ‘quality of care and service delivery’ were commonly advocated.
In light of the commonalities and differences among the methodological frameworks, the ‘pathways to research impact’ diagram ( Fig 4 ) was developed to provide researchers, funders, and policy makers a more comprehensive and exhaustive way to measure healthcare research impact. The diagram has the advantage of assorting all the impact metrics proposed by previous frameworks and grouping them into different impact subgroups and categories. Prospectively, this global picture will help researchers, funders, and policy makers plan strategies to achieve multiple pathways to impact before carrying the research out. The analysis of the data extraction and construction of the impact matrix led to the development of the ‘pathways to research impact’ diagram ( Fig 4 ). The diagram aims to provide an exhaustive and comprehensive way of tracing research impact by combining all the impact metrics presented by the different 24 frameworks, grouping those metrics into different impact subgroups, and grouping these into broader impact categories.
NHS, National Health Service; PROM, patient-reported outcome measure; QALY, quality-adjusted life year; R&D, research and development.
https://doi.org/10.1371/journal.pmed.1002370.g004
This review has summarised existing methodological impact frameworks together for the first time using systematic methods ( Fig 4 ). It allows researchers and funders to consider pathways to impact at the design stage of a study and to understand the elements and metrics that need to be considered to facilitate prospective assessment of impact. Users do not necessarily need to cover all the aspects of the methodological framework, as every research project can impact on different categories and subgroups. This review provides information that can assist researchers to better demonstrate impact, potentially increasing the likelihood of conducting impactful research and reducing research waste. Existing reviews have not presented a methodological framework that includes different pathways to impact, health impact categories, subgroups, and metrics in a single methodological framework.
Academic-orientated frameworks included in this review advocated the measurement of impact predominantly using so-called ‘quantitative’ metrics—for example, the number of peer-reviewed articles, journal impact factor, and citation rates. This may be because they are well-established measures, relatively easy to capture and objective, and are supported by research funding systems. However, these metrics primarily measure the dissemination of research finding rather than its impact [ 30 , 68 ]. Whilst it is true that wider dissemination, especially when delivered via world-leading international journals, may well lead eventually to changes in healthcare, this is by no means certain. For instance, case studies evaluated by Flinders University of Australia demonstrated that some research projects with non-peer-reviewed publications led to significant changes in health policy, whilst the studies with peer-reviewed publications did not result in any type of impact [ 68 ]. As a result, contemporary literature has tended to advocate the collection of information regarding a variety of different potential forms of impact alongside publication/citations metrics [ 2 , 3 , 5 , 7 , 8 , 29 – 47 ], as outlined in this review.
The 2014 REF exercise adjusted UK university research funding allocation based on evidence of the wider impact of research (through case narrative studies and quantitative metrics), rather than simply according to the quality of research [ 12 ]. The intention was to ensure funds were directed to high-quality research that could demonstrate actual realised benefit. The inclusion of a mixed-method approach to the measurement of impact in the REF (narrative and quantitative metrics) reflects a widespread belief—expressed by the majority of authors of the included methodological frameworks in the review—that individual quantitative impact metrics (e.g., number of citations and publications) do not necessary capture the complexity of the relationships involved in a research project and may exclude measurement of specific aspects of the research pathway [ 10 , 12 ].
Many of the frameworks included in this review advocated the collection of a range of academic, societal, economic, and cultural impact metrics; this is consistent with recent recommendations from the Stern review [ 10 ]. However, a number of these metrics encounter research ‘lag’: i.e., the time between the point at which the research is conducted and when the actual benefits arise [ 69 ]. For instance, some cardiovascular research has taken up to 25 years to generate impact [ 70 ]. Likewise, the impact may not arise exclusively from a single piece of research. Different processes (such as networking interactions and knowledge and research translation) and multiple individuals and organisations are often involved [ 4 , 71 ]. Therefore, attributing the contribution made by each of the different actors involved in the process can be a challenge [ 4 ]. An additional problem associated to attribution is the lack of evidence to link research and impact. The outcomes of research may emerge slowly and be absorbed gradually. Consequently, it is difficult to determine the influence of research in the development of a new policy, practice, or guidelines [ 4 , 23 ].
A further problem is that impact evaluation is conducted ‘ex post’, after the research has concluded. Collecting information retrospectively can be an issue, as the data required might not be available. ‘ex ante’ assessment is vital for funding allocation, as it is necessary to determine the potential forthcoming impact before research is carried out [ 69 ]. Additionally, ex ante evaluation of potential benefit can overcome the issues regarding identifying and capturing evidence, which can be used in the future [ 4 ]. In order to conduct ex ante evaluation of potential benefit, some authors suggest the early involvement of policy makers in a research project coupled with a well-designed strategy of dissemination [ 40 , 69 ].
Providing an alternate view, the authors of methodological frameworks such as the SIAMPI, Contribution Mapping, Research Contribution, and the Exchange model suggest that the problems of attribution are a consequence of assigning the impact of research to a particular impact metric [ 7 , 40 , 42 , 44 ]. To address these issues, these authors propose focusing on the contribution of research through assessing the processes and interactions between stakeholders and researchers, which arguably take into consideration all the processes and actors involved in a research project [ 7 , 40 , 42 , 43 ]. Additionally, contributions highlight the importance of the interactions between stakeholders and researchers from an early stage in the research process, leading to a successful ex ante and ex post evaluation by setting expected impacts and determining how the research outcomes have been utilised, respectively [ 7 , 40 , 42 , 43 ]. However, contribution metrics are generally harder to measure in comparison to academic-orientated indicators [ 72 ].
Currently, there is a debate surrounding the optimal methodological impact framework, and no tool has proven superior to another. The most appropriate methodological framework for a given study will likely depend on stakeholder needs, as each employs different methodologies to assess research impact [ 4 , 37 , 41 ]. This review allows researchers to select individual existing methodological framework components to create a bespoke tool with which to facilitate optimal study design and maximise the potential for impact depending on the characteristic of their study ( Fig 2 and Fig 3 ). For instance, if researchers are interested in assessing how influential their research is on policy making, perhaps considering a suite of the appropriate metrics drawn from multiple methodological frameworks may provide a more comprehensive method than adopting a single methodological framework. In addition, research teams may wish to use a multidimensional approach to methodological framework development, adopting existing narratives and quantitative metrics, as well as elements from contribution frameworks. This approach would arguably present a more comprehensive method of impact assessment; however, further research is warranted to determine its effectiveness [ 4 , 69 , 72 , 73 ].
Finally, it became clear during this review that the included methodological frameworks had been constructed using varied methodological processes. At present, there are no guidelines or consensus around the optimal pathway that should be followed to develop a robust methodological framework. The authors believe this is an area that should be addressed by the research community, to ensure future frameworks are developed using best-practice methodology.
For instance, the Payback Framework drew upon a literature review and was refined through a case study approach. Arguably, this approach could be considered inferior to other methods that involved extensive stakeholder involvement, such as the CIHR framework [ 8 ]. Nonetheless, 7 methodological frameworks were developed based upon the Payback Framework [ 8 , 29 , 31 – 35 ].
Limitations
The present review is the first to summarise systematically existing impact methodological frameworks and metrics. The main limitation is that 50% of the included publications were found through methods other than bibliographic databases searching, indicating poor indexing. Therefore, some relevant articles may not have been included in this review if they failed to indicate the inclusion of a methodological impact framework in their title/abstract. We did, however, make every effort to try to find these potentially hard-to-reach publications, e.g., through forwards/backwards citation searching, hand searching reference lists, and expert communication. Additionally, this review only extracted information regarding the methodology followed to develop each framework from the main publication source or framework webpage. Therefore, further evaluations may not have been included, as they are beyond the scope of the current paper. A further limitation was that although our search strategy did not include language restrictions, we did not specifically search non-English language databases. Thus, we may have failed to identify potentially relevant methodological frameworks that were developed in a non-English language setting.
In conclusion, the measurement of research impact is an essential exercise to help direct the allocation of limited research resources, to maximise benefit, and to help minimise research waste. This review provides a collective summary of existing methodological impact frameworks and metrics, which funders may use to inform the measurement of research impact and researchers may use to inform study design decisions aimed at maximising the short-, medium-, and long-term impact of their research.
Supporting information
S1 appendix. search strategy..
https://doi.org/10.1371/journal.pmed.1002370.s001
S1 PRISMA Checklist. Preferred Reporting Items for Systematic Reviews and Meta-Analyses (PRISMA) checklist.
https://doi.org/10.1371/journal.pmed.1002370.s002
Acknowledgments
We would also like to thank Mrs Susan Bayliss, Information Specialist, University of Birmingham, and Mrs Karen Biddle, Research Secretary, University of Birmingham.
- View Article
- PubMed/NCBI
- Google Scholar
- 3. HEFCE. REF 2014: Assessment framework and guidance on submissions 2011 [cited 2016 15 Feb]. Available from: http://www.ref.ac.uk/media/ref/content/pub/assessmentframeworkandguidanceonsubmissions/GOS%20including%20addendum.pdf .
- 8. Canadian Institutes of Health Research. Developing a CIHR framework to measure the impact of health research 2005 [cited 2016 26 Feb]. Available from: http://publications.gc.ca/collections/Collection/MR21-65-2005E.pdf .
- 9. HEFCE. HEFCE allocates £3.97 billion to universities and colleges in England for 2015–1 2015. Available from: http://www.hefce.ac.uk/news/newsarchive/2015/Name,103785,en.html .
- 10. Stern N. Building on Success and Learning from Experience—An Independent Review of the Research Excellence Framework 2016 [cited 2016 05 Aug]. Available from: https://www.gov.uk/government/uploads/system/uploads/attachment_data/file/541338/ind-16-9-ref-stern-review.pdf .
- 11. Matthews D. REF sceptic to lead review into research assessment: Times Higher Education; 2015 [cited 2016 21 Apr]. Available from: https://www.timeshighereducation.com/news/ref-sceptic-lead-review-research-assessment .
- 12. HEFCE. The Metric Tide—Report of the Independent Review of the Role of Metrics in Research Assessment and Management 2015 [cited 2016 11 Aug]. Available from: http://www.hefce.ac.uk/media/HEFCE,2014/Content/Pubs/Independentresearch/2015/The,Metric,Tide/2015_metric_tide.pdf .
- 14. LSE Public Policy Group. Maximizing the impacts of your research: A handbook for social scientists. http://www.lse.ac.uk/government/research/resgroups/LSEPublicPolicy/Docs/LSE_Impact_Handbook_April_2011.pdf . London: LSE; 2011.
- 15. HEFCE. Consultation on the second Research Excellence Framework. 2016.
- 18. Merriam-Webster Dictionary 2017. Available from: https://www.merriam-webster.com/dictionary/methodology .
- 19. Oxford Dictionaries—pathway 2016 [cited 2016 19 June]. Available from: http://www.oxforddictionaries.com/definition/english/pathway .
- 20. Oxford Dictionaries—metric 2016 [cited 2016 15 Sep]. Available from: https://en.oxforddictionaries.com/definition/metric .
- 21. WHO. WHO Ethical and Safety Guidelines for Interviewing Trafficked Women 2003 [cited 2016 29 July]. Available from: http://www.who.int/mip/2003/other_documents/en/Ethical_Safety-GWH.pdf .
- 31. Kalucy L, et al. Primary Health Care Research Impact Project: Final Report Stage 1 Adelaide: Primary Health Care Research & Information Service; 2007 [cited 2016 26 Feb]. Available from: http://www.phcris.org.au/phplib/filedownload.php?file=/elib/lib/downloaded_files/publications/pdfs/phcris_pub_3338.pdf .
- 33. Canadian Academy of Health Sciences. Making an impact—A preferred framework and indicators to measure returns on investment in health research 2009 [cited 2016 26 Feb]. Available from: http://www.cahs-acss.ca/wp-content/uploads/2011/09/ROI_FullReport.pdf .
- 39. HEFCE. RAE 2008—Guidance in submissions 2005 [cited 2016 15 Feb]. Available from: http://www.rae.ac.uk/pubs/2005/03/rae0305.pdf .
- 41. Royal Netherlands Academy of Arts and Sciences. The societal impact of applied health research—Towards a quality assessment system 2002 [cited 2016 29 Feb]. Available from: https://www.knaw.nl/en/news/publications/the-societal-impact-of-applied-health-research/@@download/pdf_file/20021098.pdf .
- 48. Weiss CH. Using social research in public policy making: Lexington Books; 1977.
- 50. Kogan M, Henkel M. Government and research: the Rothschild experiment in a government department: Heinemann Educational Books; 1983.
- 51. Thomas P. The Aims and Outcomes of Social Policy Research. Croom Helm; 1985.
- 52. Bulmer M. Social Science Research and Government: Comparative Essays on Britain and the United States: Cambridge University Press; 2010.
- 53. Booth T. Developing Policy Research. Aldershot, Gower1988.
- 55. Kalucy L, et al Exploring the impact of primary health care research Stage 2 Primary Health Care Research Impact Project Adelaide: Primary Health Care Research & Information Service (PHCRIS); 2009 [cited 2016 26 Feb]. Available from: http://www.phcris.org.au/phplib/filedownload.php?file=/elib/lib/downloaded_files/publications/pdfs/phcris_pub_8108.pdf .
- 56. CHSRF. Canadian Health Services Research Foundation 2000. Health Services Research and Evidence-based Decision Making [cited 2016 February]. Available from: http://www.cfhi-fcass.ca/migrated/pdf/mythbusters/EBDM_e.pdf .
- 58. W.K. Kellogg Foundation. Logic Model Development Guide 2004 [cited 2016 19 July]. Available from: http://www.smartgivers.org/uploads/logicmodelguidepdf.pdf .
- 59. United Way of America. Measuring Program Outcomes: A Practical Approach 1996 [cited 2016 19 July]. Available from: https://www.bttop.org/sites/default/files/public/W.K.%20Kellogg%20LogicModel.pdf .
- 60. Nutley S, Percy-Smith J and Solesbury W. Models of research impact: a cross sector review of literature and practice. London: Learning and Skills Research Centre 2003.
- 61. Spaapen J, van Drooge L. SIAMPI final report [cited 2017 Jan]. Available from: http://www.siampi.eu/Content/SIAMPI_Final%20report.pdf .
- 63. LSHTM. The Health Risks and Consequences of Trafficking in Women and Adolescents—Findings from a European Study 2003 [cited 2016 29 July]. Available from: http://www.oas.org/atip/global%20reports/zimmerman%20tip%20health.pdf .
- 70. Russell G. Response to second HEFCE consultation on the Research Excellence Framework 2009 [cited 2016 04 Apr]. Available from: http://russellgroup.ac.uk/media/5262/ref-consultation-response-final-dec09.pdf .
You are here
Ongoing research.
At any given time, HEI has a variety of ongoing studies at various stages of completion. Here, you will find a list of studies with more detailed information for each, including abstracts and posters presented at HEI Annual Conferences. We also indicate which studies have been completed and are currently undergoing HEI’s rigorous review process.
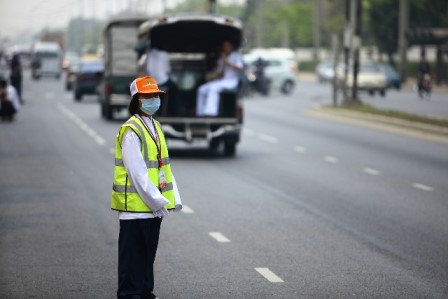
Accountability Studies
Covid-19, air pollution, and health studies, enhanced exposure studies.
- Global Health Studies
- Multiple Pollutant Epidemiology Studies
Quantifying Real-World Impacts of Non-Tailpipe Particulate Matter Emissions
Traffic exposure and health studies, wildland fires and agricultural burning studies, walter a. rosenblith award, ongoing studies, quantifying marginal societal health benefits of transportation emission reductions in the united states and canada.
Carleton University, Canada
The investigators will develop and apply a source‐ and location-specific database of mortality benefits per ton emissions reduction of NOx and other pollutants.
Impact of exposure to air pollution on asthma: A multi-exposure assessment
University of Copenhagen, Denmark
This New Investigator Award study will evaluate whether air pollution is associated with increased incidence of asthma in 3 cohorts in Denmark.
Traffic-related air pollution and birth weight: the roles of noise, placental function, green space, physical activity, and socioeconomic status (FRONTIER)
ISGlobal, Barcelona Institute for Global Health, Spain
In this study, the investigators will recruit pregnant women in Barcelona and assess the effects of in-utero exposure to traffic-related pollution on birth weight, fetal growth trajectories, and placental function for each pregnancy. The investigators will estimate exposure to several pollutants and transportation noise and evaluate the roles of socioeconomic status, green space, physical activity, diet, and stress.
Intersections as hot spots: assessing the contribution of localized non-tailpipe emissions and noise on the association between traffic and children’s health
University of Southern California
This study will assess the effects of metals from nontailpipe emissions on asthma and lung function in the most recent cohort of the Children’s Health Study in Southern California (recruited during 2002-2012), using available filters with particulate matter samples. The investigators will estimate exposure to several pollutants and transportation noise and evaluate the roles of socioeconomic status, green space, physical activity, diet, and stress.
Health effects of air pollution components, noise and socioeconomic status (“HERMES”)
Danish Cancer Society Research Center, Copenhagen, Denmark
This study will assess myocardial infarction, stroke, diabetes, and biomarkers related to cardiovascular disease and diabetes in three large Danish cohorts. The investigators will estimate exposure to several pollutants and transportation noise and evaluate the roles of socioeconomic status, green space, physical activity, diet, and stress.
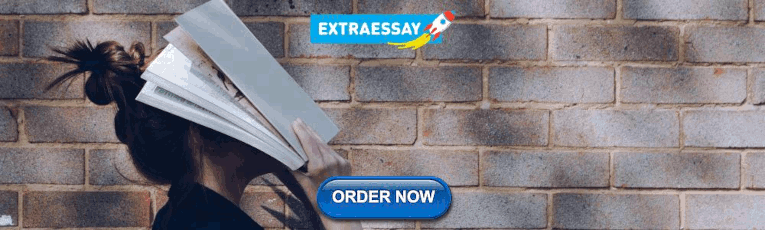
Assessing national health and educational benefits of the EPA’s school bus retrofit and replacement program: A randomized controlled trial design
University of Michigan
This study will evaluate the National Clean Diesel Rebate Program, a lottery program that allocates available funding to school districts across the United States to replace or retrofit old-technology diesel powered school buses. Adar and colleagues will compare student health and educational performance in districts with and without such funding.
Air pollutants and the gut microbiota and metabolome during early life: Implications for childhood obesity
University of Colorado, Boulder
This New Investigator Award study will investigate whether prenatal and/or early-life exposure to air pollutants affects the infant gut microbiota and fecal metabolome, thereby altering infant growth trajectories in the first two years of life. Dr. Alderete plans to study this in an ongoing longitudinal cohort of Hispanic mother-infant pairs in California with existing validated clinical assessments of infant growth trajectories. She will also use gut microbial profiling and high-resolution fecal metabolomics profiles to understand the mechanisms underlying the obesogenic effects of air pollutants in early life.
Comparing the estimated health impacts of long-term exposure to traffic-related air pollution using fixed-site, mobile, and deep learning models
McGill University, Canada
This study will evaluate health impacts of long-term exposures to traffic-related air pollution using exposure estimates from fixed-site and mobile measurement campaigns, as well as deep learning models, in Toronto and Montreal, Canada. The investigators will compare exposure estimates generated by these models to present-day and historical measurements, and to each other.
Accounting for the health benefits of air pollution regulations in China, 2008–2020
Boston University
This study will evaluate the major national regulatory policies that were implemented in China from 2008–2018. The investigators will focus on regulations in particular regions that target specific sources, such as coal combustion, and how they have reduced ambient concentrations of fine particles (and their components).
Australian fires and perinatal health risks
Yale University
This study is investigating risk of birth outcomes and perinatal mortality from fire-related PM 2.5 . The interdisciplinary team is developing fire modeling methods to estimate PM 2.5 specifically from fires for Australia with improved fire emissions inventories and Lagrangian modeling.
Contributions of prescribed fire to air quality and health
Georgia Institute of Technology
The objectives of this study are to: 1) Estimate the contributions of prescribed burning to both fine particulate matter (PM 2.5 ) and ozone (O 3 ) levels in the Southeastern US; 2) Quantify the effects of prescribed fire smoke on rates of emergency department (ED) visits; and 3) Assess how the observed health impacts vary across strata defined by age, sex, and socioeconomic markers.
Environmental and health benefits of mobile source and electricity generating unit policies to reduce particulate pollution
Emory University
University of Rochester Medical Center
In this HEI-funded project, the investigators will build on their previous accountability analyses in Atlanta, New York, and Los Angeles to characterize the health impacts of MV and EGU policy implementation.
Studies funded under RFA Health Outcomes Research – Assessing the Health Outcomes of Air Quality Actions
How do household energy interventions work?
This study will focus on a coal ban and heat pump subsidy program in the Beijing, China, region. They are building on an existing panel study that is following about 966 people who live in 50 villages around Beijing. Half the villages are subject to the policy, the other half are not.
The TRANSIT Accountability Study: Assessing impacts of vehicle emission regulations and local congestion policies on birth outcomes associated with traffic air pollution
Oregon State University
This study will assess the effects of emission-control measures on birth outcomes associated with traffic-related air pollution in Texas. Hystad and colleagues will evaluate air quality changes associated with national motor vehicle emissions regulations, as well as a diverse array of local congestion reduction programs implemented in Texas over the past 25 years.
HEI funded five different COVID-19 studies under RFA 20-1B . The first of these studies to be published, led by Zorana Jovanovic Andersen of the University of Copenhagen, Denmark, is presented in Research Report 214 . Studies in progress or under review are listed below. This research is taking place in the United States, Europe, and Asia and it focuses on two key areas:
- Accountability studies: Evaluating how interventions to control the pandemic might have affected emissions, air pollution, and human health.
- Susceptibility studies: Evaluating how air pollution exposure might affect the COVID-19 disease course, and how race, ethnicity, and socioeconomic status might also play a role.
Effect of air pollution reductions on mortality during the COVID-19 lockdown: A natural experiment study
This multicountry study will evaluate whether changes in mortality are associated with changes in ambient NO 2 and PM 2.5 levels before, during, and after the lockdown and disentangle the short-term effects of NO 2 versus PM 2.5 on mortality. The analysis will be conducted in four countries: China, Germany, Italy, and the United States.
Ambient air pollution and COVID-19 in California
University of California, Davis
This study will investigate the chronic and acute effects of air pollution exposure on COVID-19 incidence, mortality, and long-term complications, including onset of disease formation. Long- (2017−2018) and short- (2020) term exposures will be linked to a large medical records database in Southern California, with assessments at both the neighborhood and individual level.
Race, Ethnicity, and Air pollution in COVID-19 Hospitalization OUTcomes (REACH OUT)
Columbia University
In this study the investigators will conduct a retrospective evaluation of the interactions between long-term exposure to air pollution and neighborhood vulnerability to adverse COVID-19 outcomes. They will investigate both single and multipollutant air pollution exposures in relation to COVID-19 hospitalization, inpatient length of stay, ICU admission, ventilator use, and death among a racially diverse population in New York City.
Air pollution in relation to COVID-19 morbidity and mortality: A large population-based cohort study in Catalonia, Spain (COVAIR-CAT)
Barcelona Institute for Global Health (ISGlobal), Spain
This study will test whether long- or short-term exposure to air pollution increases the risk of COVID-19 hospital admissions or mortality and identify vulnerable subgroups among 6 million residents of Catalonia, Spain. The investigators will link air pollution exposures to residents’ addresses and inpatient and outpatient electronic medical records.
Accounting for mobility in air pollution exposure estimates in studies on long-term health effects
Swiss Tropical and Public Health Institute and Basel University, Switzerland
This study aims to improve our understanding of the contribution of individual mobility in air pollution exposure estimates. The investigators will use location tracking on a mobile phone application for 2,000 individuals in the Netherlands and Switzerland.
Comparison of Long-term Air Pollution Exposure assessment based on mobile monitoring, low-cost sensors, dispersion modelling and routine monitoring-based models (CLAIRE)
Utrecht University, Netherlands
Hoek and colleagues will prepare maps of modeled annual average air pollution across the Netherlands, validate the maps using new measurements from over 100 sites, and evaluate the performance of several exposure models. The investigators will conduct cross-comparisons to evaluate how different exposure assessment methods compare in their ability to predict long-term pollutant concentrations, with a particular focus on spatial variability of pollutants.
Investigating the consequences of Measurement Error of gradually more sophisticated long-term personal exposure models in assessing health effects: the LOndon Study (MELONS)
King's College London, United Kingdom
This study will investigate the consequences of measurement error on estimates of health effects of long-term exposure to outdoor air pollution in London by developing increasingly sophisticated exposure models.The investigators plan to compare exposure models that account for mobility, are based on exposure estimates at the residential address, and are based on concentrations measured at the nearest air pollution monitor.
Optimizing exposure assessment for inference about air pollution effects with application to the aging brain
University of Washington
This study will compare and contrast scientific and logistical benefits of different approaches to air pollution exposure assessment. The investigators will leverage large air pollution datasets obtained from low-cost sensors, mobile monitoring, and passive samplers. They will apply the exposure assessment approaches to determine associations with cognitive decline and dementia incidence in an ongoing cohort study, Adult Changes in Thought Air Pollution (ACT-AP).
Assessing the impact of non-exhaust emissions from traffic on the asthmatic airway
Queen Mary University of London, United Kingdom
In this study, participants with mild to moderate asthma will ride stationary bicycles on sidewalks in three London, UK environments (busy road characterized by stop-and-go traffic, high speed continuous traffic, and urban background) to measure how exposure to traffic with different mixtures of non-tailpipe and tailpipe emissions affects lung function of asthmatic adults.
Improved characterization of the real-world contributions and impacts of non-tailpipe particulate matter emissions
University of Toronto, Canada
This study will measure concentrations of non-tailpipe particulate matter across Toronto, Canada to determine how much non-tailpipe pollution people might breathe in everyday life and how to better measure these exposures in the future.
Studies funded under RFA 13-1, Improving Assessement of Near-Road Exposure to Traffic Related Pollution, and RFA 17-1, Assessing Adverse Health Effects of Exposure to Traffic-Related Air Pollution, Noise, and Their Interactions With Socio-Economic Status.
Designing optimal policies for reducing air pollution-related health inequities
Harvard University
Dr. Nethery’s study seeks to develop statistical methods for characterizing spatial and racial and ethnic variation in health effects associated with exposure to fine particulate matter (PM 2.5 ) across the United States, and to design potential policies for reducing PM 2.5 -attributable health inequities.
What's in the air? Engaging Native American youth in the Northern Plains to reduce air pollution
Dr. Ornelas Van Horne’s study seeks to advance a community-academic partnership with Missouri Breaks Industries Research (an American Indian-owned research center serving Indigenous communities and others in North and South Dakota) and Columbia University to characterize sources of air pollution and identify community-driven solutions through an emissions reduction plan.
COUPH: COpenhagen Ultrafine Particles and Health
This New Investigator Award study seeks to provide novel exposure–response functions for the effects of long-term exposure to ultrafine particles on several mortality and morbidity outcomes, while adjusting for exposures to other traffic-related air pollutants, road traffic noise, and socioeconomic status. The study makes use of a new Danish cohort of 650,000 adults.
Robust statistical approaches to understanding the causal effect of air pollution mixtures
University of Florida
This New Investigator Award study seeks to develop statistical methodology that allows for complex relationships between air pollution and health outcomes to be used to estimate causal effects of multivariate exposures. Additionally, the proposed methodology will allow for evaluation of separate subgroups in the population to identify the most vulnerable subgroups.
Urban air and noise pollution in sub-Saharan Africa: A study of prenatal exposures, birth outcomes, and sleep disturbances in infants
University of Massachusetts, Amherst
This New Investigator Award study seeks to examine associations of long-term maternal exposures to PM 2.5 , NO 2 , and environmental noise with adverse birth outcomes in Accra, Ghana. The investigators also seek to determine the effect of these exposures on the risk of lower respiratory infections in infants.
Air pollution source impacts at fine scales for long-term regulatory accountability and environmental justice
George Mason University
The primary objective of this Walter A. Rosenblith New Investigator Award study is to create fine-scale daily PM2.5 source impacts from major source and fuel categories from 2011-2020. This objective builds on recent work developing fine-scale daily air pollution exposure products in the United States, which have driven recent epidemiological studies on air pollution health effects.
Air pollution exposure and prefrontal connectivity in early adolescence
This New Investigator Award study is evaluating how fine-particle exposure affects the development of prefrontal connections and emotional behaviors during the transition from childhood to adolescence and whether this, in turn, contributes to greater risk for neuropsychiatric disorders.
Health Effects
Smoking leads to disease and disability and harms nearly every organ of the body.
More than 16 million Americans are living with a disease caused by smoking. For every person who dies because of smoking, at least 30 people live with a serious smoking-related illness. Smoking causes cancer, heart disease, stroke, lung diseases, diabetes, and chronic obstructive pulmonary disease (COPD), which includes emphysema and chronic bronchitis. Smoking also increases risk for tuberculosis, certain eye diseases, and problems of the immune system, including rheumatoid arthritis.
Secondhand smoke exposure contributes to approximately 41,000 deaths among nonsmoking adults and 400 deaths in infants each year. Secondhand smoke causes stroke, lung cancer, and coronary heart disease in adults. Children who are exposed to secondhand smoke are at increased risk for sudden infant death syndrome, acute respiratory infections, middle ear disease, more severe asthma, respiratory symptoms, and slowed lung growth.
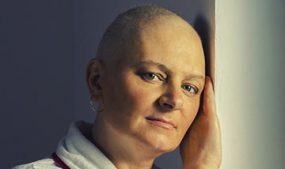
Tobacco use increases the risk for many types of cancer, such as Lung cancer.
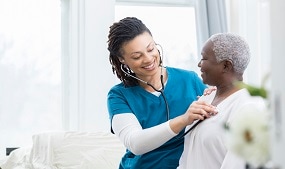
Heart Disease
Studies show a direct link between cigarette smoking and coronary heart disease.
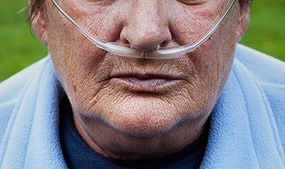
Smoking, including during the teenage years, increases the risk of dying from COPD.
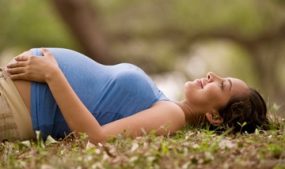
Smoking during pregnancy increases the risk for pregnancy complications.
Related Materials
- Health Effects of Cigarette Smoking
- Health Effects of Secondhand Smoke
- Tobacco-Related Mortality
- 50 Years of Progress: A Report of the Surgeon General [PDF–35.9 MB]
- U.S. Department of Health and Human Services. The Health Consequences of Smoking—50 Years of Progress: A Report of the Surgeon General . Atlanta: U.S. Department of Health and Human Services, Centers for Disease Control and Prevention, National Center for Chronic Disease Prevention and Health Promotion, Office on Smoking and Health, 2014 [accessed 2016 Dec 20].
To receive email updates about Smoking & Tobacco Use, enter your email address:
- Tips From Former Smokers ®
- Division of Cancer Prevention and Control
- Lung Cancer
- National Comprehensive Cancer Control Program
- Division of Reproductive Health

Exit Notification / Disclaimer Policy
- The Centers for Disease Control and Prevention (CDC) cannot attest to the accuracy of a non-federal website.
- Linking to a non-federal website does not constitute an endorsement by CDC or any of its employees of the sponsors or the information and products presented on the website.
- You will be subject to the destination website's privacy policy when you follow the link.
- CDC is not responsible for Section 508 compliance (accessibility) on other federal or private website.

An official website of the United States government
Here’s how you know
Official websites use .gov A .gov website belongs to an official government organization in the United States.
Secure .gov websites use HTTPS A lock ( Lock A locked padlock ) or https:// means you’ve safely connected to the .gov website. Share sensitive information only on official, secure websites.
JavaScript appears to be disabled on this computer. Please click here to see any active alerts .
Wildland Fire Research: Health Effects Research
Larger and more intense wildfires are creating the potential for greater smoke production and chronic exposures in the U.S., particularly in the West. Wildfires increase air pollution in surrounding areas and can affect regional air quality.
The effects of smoke from wildfires can range from eye and respiratory tract irritation to more serious disorders, including reduced lung function, bronchitis, exacerbation of asthma and heart failure, and premature death. Children, pregnant women, and the elderly are especially vulnerable to smoke exposure. Emissions from wildfires are known to cause increased visits to hospitals and clinics by those exposed to smoke.
It is important to more fully understand the human health effects associated with short- and long-term exposures to smoke from wildfires as well as prescribed fires, referred together as wildland fires. Research is being conducted to advance understanding of the health effects from different types of fires as well as combustion phases. Researchers want to know:
- What is the full extent of health effects from smoke exposure?
- Who is most at risk?
- Are there differences in health effects from different wildfire fuel types or combustion phases (burning versus flaming)?
- What strategies and approaches are most effective in protecting public health?
- What are the environmental, social and economic impacts of wildfire emissions?
Ongoing Research Highlights
- A novel laboratory combustion system provides the capability to control components of fire conditions and smoke that may affect health. Animal and invitro toxicology studies are ongoing to determine how wildland fire smoke impacts health.
- Researchers are partnering in a study with the Missoula City-County Health Department in Montana, University of Montana, and the Hoopa Valley Tribe in California, to measure air pollutants when smoke episodes are anticipated. The objectives of the research-- called the Wildfire Advancing Science Partnerships for Indoor Reductions of Smoke Exposures (ASPIRE) Study--are to compare indoor and outdoor fine particulate matter (PM 2.5 ) concentrations and develop strategies for reducing indoor pollutant in public buildings during wildland fire smoke events. PM 2.5 is the pollutant that is a primary health threat from smoke exposures. ASPIRE Study
- Animal toxicology studies are under way to explore the physiological mechanisms of action resulting from smoke exposure to better understand what happens in the body that results in severe lung and heart health problems.
- Learn more about the Comparative Assessment of the Impacts of Prescribed Fire Versus Wildfire (CAIF): A Case Study in the Western U.S. (Released Sep 2021)
- EPA researchers are studying the characteristics, elements, networks, relationships, and processes that contribute to an effective smoke-ready communities plan to protect public health. The study team is using a collaborative approach with partners to study the process of collaboration as well as influencing factors and early outcomes that may be related to this approach. Smoke-Ready Communities Research to Prepare for Wildfires
- A crowdsourcing study, in conjunction with the development of a smartphone app – Smoke Sense – is evaluating public health communication during smoke episodes to assess the magnitude of health outcomes. The results can be used to both estimate the economic value associated with avoiding these health outcomes and examine how health risk communication strategies affect behavior and reduce public health burdens during smoke episodes. Smoke Sense App
- Studies are being conducted to ascertain whether weatherized households have a protective effect in keeping out outdoor air pollution from wildfire smoke. Researchers are comparing health outcomes for weatherized and non-weatherized homes during wildfire season in Colorado. The research is supported by a STAR grant on Indoor Air and Climate Change .
- Air Research Home
- Air Monitoring & Emissions
- Air Quality Modeling
- Wildland Fires
- Health Effects from Air Pollution
- Air & Energy
- Outreach, Tools & Resources
Advertisement
Supported by
Covid Vaccine Side Effects: 4 Takeaways From Our Investigation
Thousands of Americans believe they experienced rare but serious side effects. But confirming a link is a difficult task.
- Share full article

By Apoorva Mandavilli
Apoorva Mandavilli spent more than a year talking to dozens of experts in vaccine science, policymakers and people who said they had experienced serious side effects after receiving a Covid-19 vaccine.
Soon after their arrival in late December 2020, the Covid-19 vaccines turned the pandemic around and opened a path back to normalcy. They prevented about 14.4 million deaths worldwide, according to one estimate .
In a small percentage of people, they also produced side effects.
Over the course of more than a year, The New York Times talked to 30 people who said they had been harmed by Covid vaccines. Their symptoms may turn out to be unrelated to the shots. But they — along with more than a dozen experts — felt federal officials are not doing enough to investigate their complaints.
All vaccines carry some risk of side effects. More than 270 million Americans received about 677 million doses of the Covid vaccines, and even rare side effects — occurring, say, in just 0.001 percent of patients — might mean thousands of recipients were affected.
Indeed, more than 13,000 have submitted claims to a government fund that compensates people for Covid vaccine injuries. So far, however, only a dozen people have been compensated, nearly all of them for a heart problem caused by the vaccines.
Here are four takeaways from our investigation.
For most people, the benefits of Covid vaccines outweigh any risks.
Even the best vaccines and drugs have some side effects. That does not negate their benefits, nor does it suggest that people should stop taking them.
The rotavirus vaccine, for example, is an unmitigated success, but it can lead to intussusception — a life-threatening condition in which the intestine folds in on itself — in about 0.02 percent of children who are vaccinated.
Some side effects caused by the Covid vaccines may be equally rare. Researchers in Hong Kong analyzed that country’s health records and found that about seven of every million doses of Pfizer-BioNTech vaccine triggered a bout of shingles serious enough to require hospitalization.
Other side effects are slightly more common. The Covid vaccines may lead to myocarditis, or inflammation of the heart, in one of every 10,000 adolescent males. (Myocarditis is one of the four serious side effects acknowledged by federal health officials.)
Deaths from the vaccines are vanishingly rare , despite claims from some conspiracy theorists that vaccines have led to a spike in mortality rates.
More intensive analysis may indicate that in some groups, like young men, the benefit of Covid shots may no longer outweigh the risks. But for the majority of Americans, the vaccines continue to be far safer than contracting Covid itself.
Federal surveillance has found some side effects but may miss others.
To detect problems with vaccines, federal agencies rely on multiple databases. The largest, the Vaccine Adverse Event Reporting System, is useful for generating hypotheses, but contains unverified accounts of harms. Other databases combine electronic health records and insurance claims.
These systems spotted blood-clotting problems associated with the Johnson & Johnson vaccine and a potential risk of stroke after mRNA immunizations, which is still under investigation. But federal researchers trailed Israeli scientists in picking up myocarditis as a problem among young men.
The American health care system is fragmented, with medical records stored by multiple companies that do not collaborate. Electronic health records do not all describe symptoms the same way, making comparisons difficult. Insurance claims databases may have no record of shots administered at mass vaccination sites.
Federal systems may also miss symptoms that defy easy description or diagnosis.
Proving vaccination led to an illness is complicated.
Among the hundreds of millions of Americans who were immunized against Covid, there were deaths, heart attacks, strokes, miscarriages and autoimmune illnesses. How to distinguish illnesses caused by the vaccine from those that would have happened anyway?
The rarer the condition, the harder it is to answer this question.
Merely judging by the timing — the appearance of a particular problem after vaccination — can be misleading. Most famously, childhood vaccines were mistakenly linked to autism because the first noticeable features often coincided with the immunization schedule.
Serious side effects may first turn up in animal studies of vaccines. But few such studies were possible given the nation’s desperate timeline in 2020. Clinical trials of the vaccines were intended to test their effectiveness, but they were far from big enough to detect side effects that may occur only in a few people per million doses.
Most independent studies of side effects have not been large enough to detect rare events, nor to exclude their possibility; others have looked only for a preset list of symptoms and might have missed the rare outliers.
An expert panel convened by the National Academies concluded in April that for most side effects, there was not enough data to accept or reject a link to Covid vaccination.
Understanding the full range of side effects may take years.
Federal health officials acknowledge four major side effects of Covid vaccines — not including the temporary injection site pain, fever and malaise that may accompany the shots.
But in federal databases, thousands of Americans have reported that Covid vaccines caused ringing in the ears, dizziness, brain fog, sharp fluctuations in blood pressure and heart rate, new or relapsed autoimmune conditions , hives , vision problems , kidney disorders, tingling , numbness and a loss of motor skills.
Some studies have examined reports of side effects and largely concluded that there was no link . Closer scrutiny may reveal that many, perhaps most, of the other reported side effects are unrelated to immunization. Most of them are also associated with Covid , and may be the result of undiagnosed infections. But without in-depth studies, it is impossible to be sure, experts said.
Apoorva Mandavilli is a reporter focused on science and global health. She was a part of the team that won the 2021 Pulitzer Prize for Public Service for coverage of the pandemic. More about Apoorva Mandavilli
- International edition
- Australia edition
- Europe edition
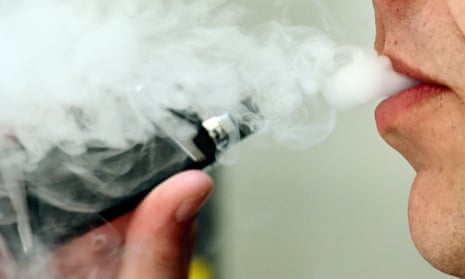
Chemicals in vapes could be highly toxic when heated, research finds
AI analysis of 180 vape flavours finds that products contain 127 ‘acutely toxic’ chemicals, 153 ‘health hazards’ and 225 ‘irritants’
Chemicals used to produce vapes could be acutely toxic when heated and inhaled, according to research .
Vaping devices heat the liquid flavouring to high temperatures to form an aerosol that is then inhaled. They contain chemicals including vegetable glycerin, propylene glycol, nicotine and flavourings, blended in various amounts.
Previous experiments have shown that some fruit-flavoured vapes – such as strawberry, melon and blueberry – produce dangerous compounds called volatile carbonyls due to this heating process.
These compounds are known to have health implications for chronic obstructive pulmonary disease (COPD), cardiovascular disease and cancers.
With so many chemicals used in tens of thousands of different vape products, conducting experiments to test every brand and flavour for toxicity could take decades of research.
Instead, the study used AI to analyse the chemical composition of 180 vape flavours and simulate how they decompose when heated. The research, published in Scientific Reports , predicted that vapes produce 127 “acutely toxic” chemicals, 153 “health hazards” and 225 “irritants”.
Nearly every flavour put through the AI predictor showed at least one product that was classified as a health hazard, with many predicting several. The toxins were associated with vapes containing no nicotine, as well as those with.
The research team at RCSI University of Medicine and Health Sciences, Dublin , conclude there is a “potential public health threat facing the 4.5 million vapers in the UK” and an urgent need for “enhanced restrictions” on flavours and regulations that are reflective of the health risks of vaping, especially for young people.
In January, the government announced that it would ban disposable vapes and restrict sweet and fruity flavours . Lead author Donal O’Shea, professor of chemistry at RCSI, said that the UK government should go further and remove all flavours from vapes.
It is crucial to understand the impact of flavoured vapes on health “before it’s too late”, he added.
“It is plausible that we are on the cusp of a new wave of chronic diseases that will emerge 15 to 20 years from now due to these exposures.”
Given the popularity of flavoured vapes among non-smoking teenagers and young adults, understanding the long-term effects of these products on public health, morbidity and mortality is crucial, the study concludes.
“Without comprehensive regulation, as we try to treat the nicotine addictions of older tobacco smokers, there is a substantial risk of transferring new health issues to younger generations.”
Responding to the findings, a Department of Health and Social Care spokesperson said: “The health advice is clear – if you don’t smoke, don’t vape and children should never vape.
“That’s why we are banning disposable vapes and our tobacco and vapes bill includes powers to limit flavours, packaging and displays of vapes to reduce the appeal to children.
“It is clear that flavours like cotton candy and cherry cola are deliberately being targeted at children, not adult smokers trying to quit, which is completely unacceptable. That is why we are taking decisive action and will be restricting vape flavours.”
Prof Sanjay Agrawal, the Royal College of Physicians’ special adviser on tobacco, said that while vaping can be a very effective way to break the addiction to tobacco, it should only be used for this purpose.
“Vaping is not risk-free, so those who don’t smoke, including children and young people, should not vape either,” he said.
John Dunne, director general at the trade body the UK Vaping Industry Association, said: “The science on vaping is very clear, it is the most effective way for smokers to quit and is at least 95% less harmful than smoking. Every chemical used in vaping e-liquid in the UK is stringently tested, including analysing chemicals when heated, and is only approved for use by the UK government if it is deemed safe.”
Most viewed
REVIEW article
Bifidobacterium exopolysaccharides: new insights into engineering strategies, physicochemical functions, and immunomodulatory effects on host health.
- 1 Department of Food Biotechnology, Branch for Northwest and West Region, Agricultural Biotechnology Research Institute of Iran, Agricultural Research, Education and Extension Organization (AREEO), Tabriz, Iran
- 2 Regenerative Medicine Research Center (RMRC), Health Technology Institute, Kermanshah University of Medical Sciences, Kermanshah, Iran
Bifidobacteria are a prominent type of bacteria that have garnered significant research attention for their exceptional probiotic properties and capacity to produce exopolysaccharides (EPSs). These compounds exhibit diverse physical, chemical, and biological characteristics, prompting numerous investigations into their potential applications. Researchers have noted their beneficial effects as immune modulators within the host’s body across various industries. Extensive research has been conducted on the immunomodulatory effects of bifidobacteria-derived EPSs, with emerging engineering strategies aimed at enhancing their immune-modulating capabilities. Understanding the structure, physicochemical properties, and biological activities of these compounds is crucial for their effective utilization across different industries. Our review encompassed numerous studies exploring Bifidobacterium and its metabolites, including EPSs, across various sectors, drawing from diverse databases. The distinctive properties of EPSs have spurred investigations into their applications, revealing their potential to bolster the immune system, combat inflammation, and treat various ailments. Additionally, these compounds possess antioxidant and antimicrobial properties, making them suitable for incorporation into a range of products spanning food, health, and medicine.
1 Introduction
Bifidobacteria, a genus within the Actinobacteria branch, are Gram-positive, anaerobic bacteria shaped like bacilli, acknowledged as a pivotal bacterial group in the intestinal microbial community, particularly during natural childbirth and infancy. First isolated in 1899 by Tissier from the feces of breastfed babies, this genus encompasses over 50 different species, including notable examples such as Bifidobacterium bifidum , Bifidobacterium longum , Bifidobacterium breve , Bifidobacterium animal , Bifidobacterium adolescentis , Bifidobacterium pseudostreptococcus , and Bifidobacterium pseudolongum ( Schell et al., 2002 ; Fanning et al., 2012b ; Eshaghi et al., 2017 ; Ruiz et al., 2017 ; Hidalgo-Cantabrana et al., 2018 ; Turroni et al., 2019 ; Yao et al., 2021 ; Li et al., 2023 ; Nicolescu et al., 2023 ; Pacyga-Prus et al., 2023 ).
In infants, bifidobacteria typically account for about 90% of intestinal bacteria, while in adults, this proportion decreases to 3%–5%. The mode of infant feeding significantly impacts the establishment of bifidobacteria in the gut, with breastfed infants showing higher levels compared to formula-fed counterparts ( Liu et al., 2014 ; Ruiz et al., 2017 ; Choi et al., 2022 ; Li et al., 2023 ). These bacteria have the capability to produce metabolites known as exopolysaccharides (EPSs) during fermentation. Traditionally, EPSs play a vital role in fermented dairy products owing to its gelling and thickening properties, which also offer potential health benefits ( Salazar et al., 2009 ; Sørensen et al., 2022 ). Due to their unique physiological and biological properties, these compounds can help strengthen the body’s immune system and effectively combat inflammation and various diseases, as well as serving as biological additives in various products such as food, pharmaceuticals, and health products ( Xu et al., 2019 ; Angelin and Kavitha, 2020 ; Korcz and Varga, 2021 ; Netrusov et al., 2023 ).
In particular, EPSs, with their diverse chemical and structural composition, perform various functions across industries including agriculture, dairy, biofilm formation, cosmetics, etc., demonstrating their biotechnological significance ( Netrusov et al., 2023 ). EPSs are a type of polysaccharide ( Lim et al., 2020 ) and carbohydrate polymer widely distributed in various organisms, including plants, animals, microorganisms, and others ( Yue et al., 2023a ). EPS produced by lactic acid bacteria typically consists of glucose, galactose, and rhamnose sugar units in varying ratios, commonly found in genera such as bifidobacteria and lactobacilli ( Kaur and Dey, 2023 ). These polysaccharides can either form a capsule when covalently attached to the cell surface, known as capsular polysaccharides (CPSs; Chen et al., 2017 ; Angelin and Kavitha, 2020 ; Alessandri et al., 2021 ), or be secreted into the surrounding environment of the cell, easily released in the growth environment, creating a slimy coating, and are then referred to as EPSs, which are crucial for the formation of bacterial biofilms ( Alessandri et al., 2021 ; Xie et al., 2023 ).
The primary function of Bifidobacterium EPS is to shield these bacteria from acidity and bile salts during transit through the digestive system, thereby enhancing their adhesion to the intestinal mucosa ( Llamas-Arriba et al., 2019 ). Consequently, Bifidobacteria are commonly utilized for the direct production of EPS in fermented products ( Xu et al., 2019 ). Additionally, EPSs play various roles in protecting bacteria against osmotic stress ( Bhagat et al., 2021 ), desiccation ( Carezzano et al., 2023 ), extreme temperatures, salinity, UV rays, chemical agents such as antibiotics and heavy metals ( Shukla et al., 2017 ), phagocytosis, and bacteriophage attacks ( Laiño et al., 2016 ; Schmid, 2018 ; Angelin and Kavitha, 2020 ; Korcz and Varga, 2021 ; Netrusov et al., 2023 ). Hence, they serve as vital biological components in the interaction between microorganisms and the host ( Laiño et al., 2016 ).
Certain species of Bifidobacterium , such as B. longum , B. breve , B. bifidum , B. adolescentis , B. catenulatum , and B. infantis , possess the capability to produce EPSs ( Salazar et al., 2009 ; Hidalgo-Cantabrana et al., 2014b ). These EPSs varieties exhibit a wide array of properties, serving as preservatives in food products, enhancing the immune system, acting as antimicrobial agents, and functioning as antioxidants ( Fanning et al., 2012b ; Laiño et al., 2016 ; Yue et al., 2023a ). Moreover, they demonstrate antitumor potential ( Wang et al., 2019 ), along with properties such as anti-diabetic, anti-inflammatory bowel, anti-aging, immune modulation, wound healing, and blood cholesterol reduction ( Laiño et al., 2016 ; Llamas-Arriba et al., 2019 ; Yue et al., 2023a ).
For instance, EPS derived from B. longum w11 has exhibited antioxidant activity in vitro and has been shown to regulate cellular oxidative stress ( Inturri et al., 2017a ). Furthermore, research indicates that bifidobacteria can mitigate the progression or symptoms of various diseases, including colorectal cancer, diarrhea, necrotizing enterocolitis, and inflammatory bowel disease ( Liu et al., 2019 ). In a particular study, EPS isolated from lactobacilli and bifidobacteria demonstrated efficacy in attenuating the inflammatory response of enterotoxigenic E. coli on pig intestinal enterocytes ( Wachi et al., 2014 ). Additionally, bifidobacteria have been observed to interact with human immune cells and modulate specific pathways involved in both innate and adaptive immune responses ( Ruiz et al., 2017 ). By employing strategies such as optimizing cultivation conditions, as well as genetic and metabolic engineering, it becomes feasible to tailor the performance, structural, and functional characteristics of bacterial EPSs ( Hidalgo-Cantabrana et al., 2014b ).
2 Composition of Bifidobacterium EPS
EPS produced by bifidobacteria are recognized as potentially biologically active compounds. They exhibit a diverse range of structures and are primarily synthesized in response to various environmental stimuli ( Nicolescu et al., 2023 ). Research indicates that the production rate of EPS by these bacteria is influenced by factors such as strain variation, environmental composition, and culture conditions, including temperature, pH, and carbon-nitrogen ratio ( Angelin and Kavitha, 2020 ; Mohd Nadzir et al., 2021 ).
EPS can be categorized into two groups based on their properties, namely homopolysaccharides (HoPSs), composed of a single monosaccharide, and heteropolysaccharides (HePSs), consisting of one or more types of monosaccharides ( Salazar et al., 2016 ; Angelin and Kavitha, 2020 ; Netrusov et al., 2023 ; Salimi and Farrokh, 2023 ). While homopolysaccharides are produced by certain lactic acid bacteria, their production in bifidobacteria remains unidentified ( Hidalgo-Cantabrana et al., 2012 ; Castro-Bravo et al., 2018b ; Lynch et al., 2018 ). In bifidobacteria, HePs consist of various repeating monosaccharide units, predominantly D-glucose, D-galactose, L-rhamnose, and occasionally, N-acetylated monosaccharides such as N-acetyl-glucosamine (GluNAc) and N-acetyl-galactosamine (GalNAc), as well as fucose, glucuronic acid, glycerol, or mannose, which may be branched or unbranched ( Castro-Bravo et al., 2018b ; Angelin and Kavitha, 2020 ; Jurášková et al., 2022 ; Netrusov et al., 2023 ).
Examples of heteropolysaccharides produced by bacteria include xanthan, alginate, valan, kefir, golan, and hyaluronic acid ( Mohd Nadzir et al., 2021 ). The structure of the repeating units is elucidated using nuclear magnetic resonance and other chromatographic methods ( Hidalgo-Cantabrana et al., 2012 ; Castro-Bravo et al., 2018b ).
In a study investigating approximately 30 EPS from Bifidobacterium strains using various chromatographic methods, the main monosaccharides identified were D-galactose, found in all Bifidobacterium EPS, followed by D-glucose, present in over half of them, and finally L-rhamnose, found in half of the Bifidobacterium EPS. However, exceptions exist where the proportion of rhamnose is higher in certain B. animalis subsp. EPS ( Hidalgo-Cantabrana et al., 2012 ). Additionally, studies indicate that Bifidobacterium strains containing D-mannose exhibit a higher rate of EPS production compared to L-mannose strains ( Chen et al., 2017 ). Table 1 illustrating the structure of EPS units produced by several bifidobacteria strains.
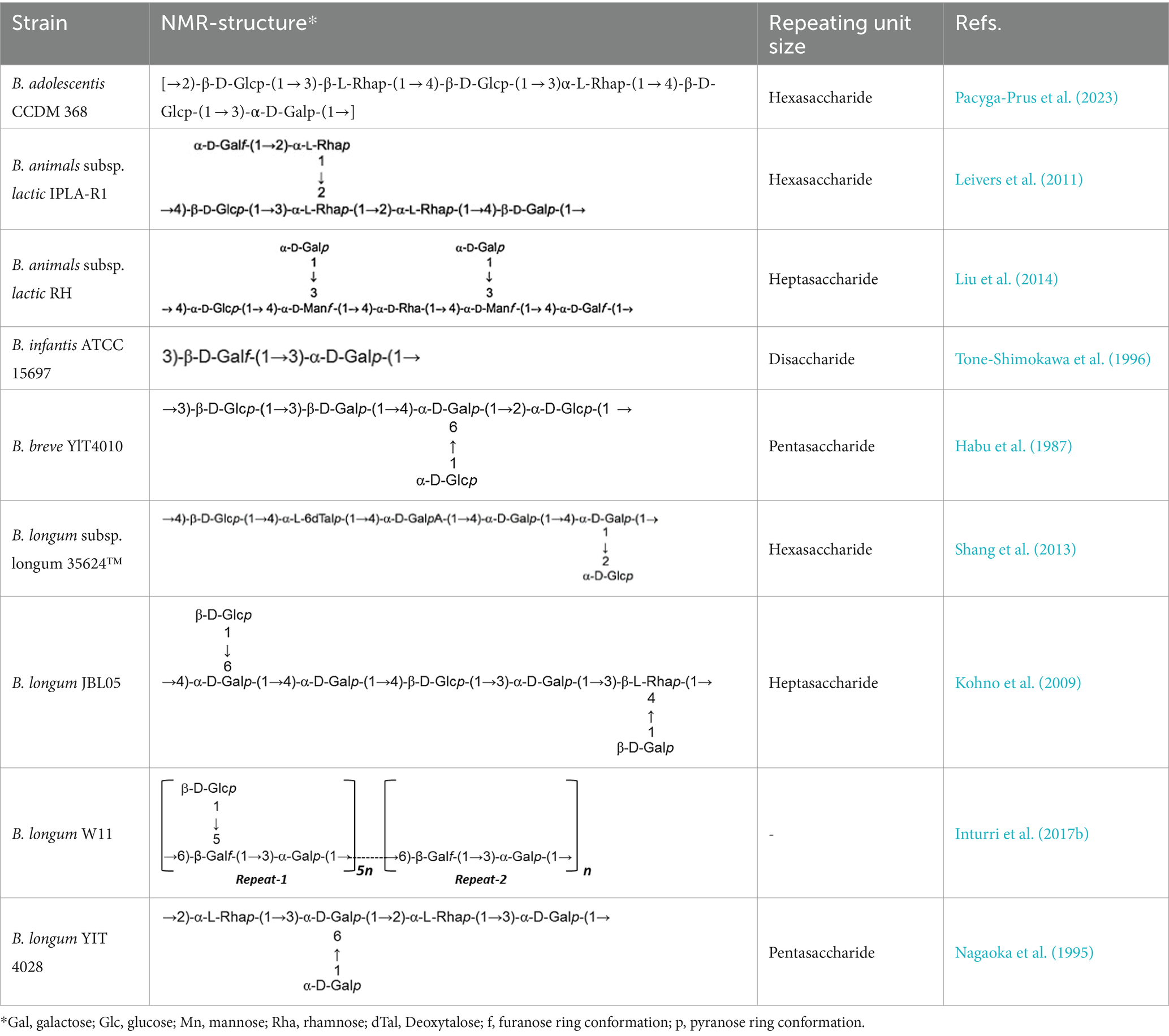
Table 1 . Unit structures of EPS synthesized by Bifidobacterium determined by NMR techniques.
Both quantitative and qualitative methods are utilized to detect and identify EPS produced by bifidobacteria. Qualitative techniques encompass electron microscopy (EM) and confocal laser scanning microscopy (CLSM), providing visual insights into EPS structure and morphology. On the quantitative front, various methods are employed to analyze EPS composition including liquid chromatography (HPLC), gas chromatography ( Han et al., 2016 ), colorimetric methods, size exclusion chromatography (SEC), ion exclusion chromatography ( Konieczna et al., 2012 ), nuclear magnetic resonance spectroscopy (NMR), and Fourier transforms infrared spectroscopy (FTIR). These methods, either individually or in combination, enable comprehensive analyses of EPS composition, thereby contributing to a deeper understanding of their properties and potential applications ( Korcz and Varga, 2021 ).
3 Pathway of EPS biosynthesis in bifidobacteria
The mechanism underlying EPS synthesis in the Bifidobacterium genus remains incompletely understood due to the elusive structure and composition of Bifidobacterium EPS. Previous studies have revealed that most bifidobacteria lack genes associated with HoPSs synthesis, which encode enzymes like glycan sucrase and fructan sucrase. However, a pathway for HePSs synthesis in bifidobacteria has been postulated based on the predicted functions of eps genes ( Hidalgo-Cantabrana et al., 2014b ; Castro-Bravo et al., 2018b ).
HePSs polymers possess a complex composition, and their synthesis involves multiple enzymes and proteins, rendering the process intricate ( Castro-Bravo et al., 2018b ; Whitfield et al., 2020 ). Enzymes involved in HePSs biosynthesis can be categorized into four groups:
1. Hexokinase: These enzymes activate glucose to glucose-6-phosphate.
2. Uridine-5′diphosphate (UDP)-glucose pyrophosphorylase: They catalyze the conversion of glucose-1-phosphate to UDP-glucose, a critical molecule in EPS synthesis.
3. Glycosyltransferases: These enzymes transfer sugar nucleotides to a glycosyl carrier lipid.
4. Wzx protein (flipase) and ABC transporters: These groups of enzymes are involved in the polymerization and transport of EPS units across the cytoplasmic membrane. Wzx protein acts as a flipase, ejecting EPS repeat units bound to a lipid carrier across the membrane, while ABC transporters transport single repeating units attached to the lipid carrier UDP-C55 ( Willis and Whitfield, 2013 ).
The synthesis of HePSs in bifidobacteria involves several steps:
1. Synthesis of repeating sugar units within the cytoplasm.
2. Cytoplasmic assembly of the EPS unit.
3. Export of the repeating EPS units to the extracellular side.
4. Polymerization and determination of the length of the final skeleton chain, with all steps except polymerization occurring in the cytoplasm ( Cuthbertson et al., 2009 ; Zannini et al., 2016 ; Yang et al., 2019 ; Angelin and Kavitha, 2020 ).
Initially, glucose is converted into glucose-6-phosphate by intracellular hexokinase enzymes. Subsequently, glucose-6-phosphate is converted into glucose-1-phosphate by the enzyme phosphoglucomutase. UDP-glucose, essential for EPS synthesis, is then formed from glucose-1-phosphate by uridine diphosphate glucose pyrophosphorylase.
In the subsequent step, glycosyltransferase priming enzymes link the first monosaccharide from Pischas or the activated sugar nucleotide to a membrane-bound isoprenoid lipid carrier [Undecaprenyl phosphate (C55)]. Successive glycosyltransferases catalyze the glycosidic bond between new nucleotide sugars and the initial monosaccharide, leading to the addition of more sugar fragments. The structure of each oligopolysaccharide varies depending on the number and characteristics of Glycosyltransferases ( Castro-Bravo et al., 2018a , b ; Wang et al., 2019 ; Angelin and Kavitha, 2020 ).
Carrier lipids, identified as isoprenoid alcohols, have their terminal alcohol groups connected to remaining monosaccharides via a pyrophosphate bridge. These carrier lipids may undergo modifications such as acetylation, acylation, sulfosylation, and methylation, if necessary.
Finally, the synthesized polymers are secreted to the extracellular side using two secretory systems: ABC transporters and the flippase-polymerase complex (WZX-WZY). Most eps clusters in Bifidobacterium strains indicate the existence of both systems in this genus. In the Wzx-Wzy-dependent pathway, the protein flippase (Wzx) ejects the EPS repeat units bound to the lipid carrier across the membrane, followed by a polymerase (Wzy) that transfers the repeating units outside the cell. The final chain length is determined by protein tyrosine kinase (Wzz; Hidalgo-Cantabrana et al., 2014b ; Castro-Bravo et al., 2018a ). A schematic representation of the hypothetical EPS biosynthesis pathway in Bifidobacterium, dependent on the Wzx-Wzy pathway, is depicted in Figure 1 .
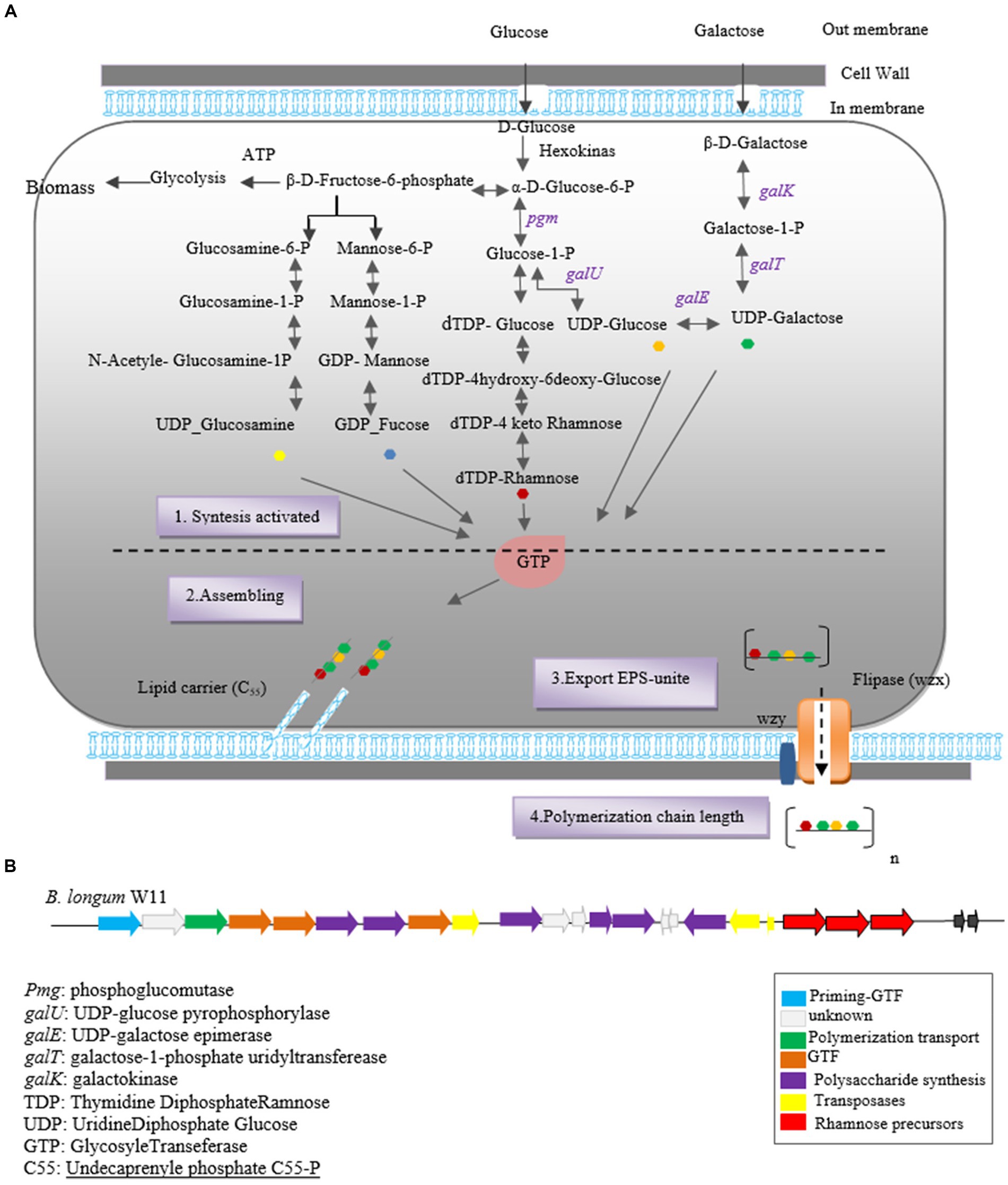
Figure 1 . Heteropolysaccharide biosynthesis pathway in Bifidobacterium genus (A) and gene cluster of Bifidobacterium longum W11 (B).
The biosynthesis of EPSs in Bifidobacterium species involves two key stages: the synthesis of precursor sugar nucleotides and the EPS cluster, which includes genes responsible for sugar nucleotide production and EPS biosynthesis enzymes. Notable genes involved in precursor sugar nucleotide synthesis include galK, galE, galT, galU, rmlA, rmlB1 , and rmlCD , along with early glycosyltransferases. These genes have been identified in B. longum subspecies and strains NCC2705, DJO10A, and B. longum subsp. longum CRC 002 ( Audy et al., 2010 ).
The eps gene cluster comprises genes encoding EPS biosynthesis enzymes and proteins. In silico analysis of the eps cluster has revealed a conserved gene set, including the predicted glycosyltransferase Primary ( p_gtf ), which catalyzes the synthesis of the EPS unit in the initial step. This gene is typically present in all eps clusters and is crucial for EPS production in the Bifidobacterium genus ( Ferrario et al., 2016 ). For instance, the eps cluster of B. longum W11 has been described ( Inturri et al., 2017b ) as predicted in Figure 1B .
The first identification of EPS genes within the Bifidobacterium genome was reported by Lee and O’Mallivan (2010) , uncovering predicted genes involved in EPS synthesis within these clusters ( Castro-Bravo et al., 2018a ). Subsequently, the sequence of the eps cluster responsible for EPS synthesis in B. animalis subsp. lactis was elucidated, along with the structure of the high molecular weight (HMW) rhamnose-rich repeating unit EPS produced by strain IPLA-R1 ( Leivers et al., 2011 ).
Subsequent research and genetic analysis by Hidalgo et al. in 2014, focusing on 28 completed Bifidobacterium genomes, shed light on the diversity of the eps cluster among Bifidobacterium strains. For instance, B. animalis subsp. IPLA R1 was found to harbor a 54.3 kb eps cluster containing 42 genes, exhibiting variation across Bifidobacterium subspecies ( Hidalgo-Cantabrana et al., 2014b ; Ferrario et al., 2016 ). On the other hand, B. bifidum E3 strain’s eps cluster comprised 20 genes, indicating variation even within species ( Yue et al., 2023a ).
Moreover, higher diversity was observed in B. adolescentis and B. breve strains, highlighting strain-dependent variation in eps clusters. Notably, B. breve UCC2003 strain was found to possess three eps clusters, namely eps1, eps2a , and eps2b ( Fanning et al., 2012a ; Ferrario et al., 2016 ). However, despite the presence of a conserved eps2 cluster in the genome of B. pullorum LMG21816, this strain exhibited a negative EPS phenotype under tested conditions. It was suggested that the absence of p-gtf indicates the incompleteness of the eps cluster in this strain, resulting in the lack of EPS production ( Ferrario et al., 2016 ).
In a study conducted by Ferrario et al. (2016) , the genomes of 48 bifidobacteria strains available in the gene bank were analyzed to identify potential eps clusters. The researchers utilized the p-gtf sequence as a molecular marker to retrieve eps genome sequences, except for B. bifidum LMG11041. This study corroborated findings from a previous study by Hidalgo et al. in 2014, which indicated a lack of common structural organization in the eps biosynthesis clusters of bifidobacteria. However, the study revealed consistent interspecies diversity among strains possessing eps clusters, particularly in terms of cluster length, number, and predicted gene functions. The size of eps gene clusters varied significantly among Bifidobacterium strains, ranging from 9 genes identified in the eps region of B. mongoliense to 55 genes in B. dentium ( Ferrario et al., 2016 ).
Notably, the glycosyltransferase enzyme, catalyzing the initial step of EPS synthesis, was found encoded within all eps clusters of the studied Bifidobacterium strains ( Hidalgo-Cantabrana et al., 2014b ; Castro-Bravo et al., 2018b ; Zhou et al., 2019 ). However, interspecies variation in the genetic content responsible for EPS synthesis in Bifidobacterium indicates the flexibility of the genome within this genus ( Zhou et al., 2019 ).
It’s noteworthy that there’s no common structural organization observed among species and strains within the Bifidobacterium genus. Furthermore, the G + C content of most EPS clusters in this genus are lower than that of the entire genome, suggesting horizontal acquisition of these genes. The likely donors of these genes are inhabitants with which bifidobacteria share a common environment, such as members of Lactobacillaceae and Lachnospiraceae. This suggests a dynamic evolutionary process shaping EPS synthesis within the Bifidobacterium genus ( Chaib De Mares et al., 2015 ).
4 Bifidobacterium EPS engineering strategy
EPS engineering strategies aim to optimize the performance and unique properties of EPS for diverse applications in food, medicine, and industry, driven by insights into structure–function relationships. These strategies encompass interventions at the polymer synthesis stage through various treatments or at the biosynthesis level, thereby influencing structural composition. A straightforward approach to engineer EPS involves enzymatic modification, utilizing polysaccharide hydrolases and lyases, which serve as endoglycosidases or exoglycosidases. Typically sourced from microorganisms or their bacteriophages, these enzymes facilitate the alteration of EPS structure, enabling tailored functionalities ( Boels et al., 2001 ).
Moreover, targeted manipulation of regulatory proteins offers another avenue to enhance EPS productivity. By augmenting the transcription of operons responsible for encoding EPS biosynthesis proteins, productivity can be significantly increased ( Schmid et al., 2015 ). Additionally, EPS structures can be customized through modifications such as acetylation, phosphorylation, and sulfonation, enabling the attainment of desired functionalities. While these modifications have been extensively studied in lactobacillus strains, their exploration within the Bifidobacterium genus remains limited.
However, alternative strategies for modifying EPS in Bifidobacterium include altering molecular weight, adding or removing substituents and monomer sugars from side chains, and overexpressing genes encoding enzymes in EPS biosynthesis pathways. Additionally, housekeeping genes involved in sugar nucleotide formation play a crucial role. Given the abundance of the CRISPR-Cas system in bifidobacteria, gene editing using the CRISPR system offers a promising avenue for modifying EPS with new biological activities ( Xu et al., 2019 ; Zuo et al., 2020 ; Sun and Zhang, 2021 ; Salimi and Farrokh, 2023 ).
In a study by Castro-Bravo et al. (2017) , a novel double crossover recombination strategy was utilized in bifidobacteria. Specifically, they targeted the Balate-1410 gene, which encodes a protein responsible for polymer chain elongation in Bifidobacterium animalis subsp. lactis DSM10140. Through this approach, they replaced the wild-type gene with a mutant variant, resulting in a mucoid phenotype. In essence, this research demonstrated that the ability to produce EPS in B. animalis DSM10140T could be reinstated by introducing a point mutation into the Balat_1410 gene, which plays a crucial role in EPS chain elongation. Analysis using NMR and SEC-MALS revealed that the mutant strain produced EPS with a higher molecular weight compared to the wild type. Furthermore, chemical and physical analyses confirmed the successful introduction of the mutation related to high molecular weight EPS in the recombinant strain ( Castro-Bravo et al., 2017 ). This study showcases the potential of genetic manipulation techniques in enhancing EPS properties in Bifidobacterium , paving the way for future advancements in EPS engineering within this genus.
The impact of genes involved in nucleotide sugar production and priming glycosyltransferase (PGTF), which initiates the assembly of EPS repeat units by adding the first sugar-1-phosphate to a lipophilic carrier, is crucial in EPS engineering strategies. In a study conducted by Audy et al. (2010) , the focus was on the expression of genes associated with sugar nucleotide production and EPS biosynthesis in Bifidobacterium longum subspCRC002. Their findings revealed that genes responsible for EPS biosynthesis were clustered within one or two transcription units, notably including PGTF, along with sugar nucleotide precursors for glucose, galactose, and rhamnose. Targeting these specific genes significantly influenced EPS production in this strain. Also B. longum subsp. CRC002 showed strong metabolic activity leading to increased production of EPS composed of glucose and galactose when PGTF-related genes were targeted. In addition, the expression of nucleotide sugar genes peaked at the exponential growth stage, indicating their importance in EPS biosynthesis. This study underscores the significance of targeting PGTF as a key enzyme in the biosynthetic pathway of EPS. By manipulating the expression of genes associated with nucleotide sugar production and PGTF, researchers can effectively enhance EPS production and tailor EPS composition to meet specific requirements for various applications ( Audy et al., 2010 ).
In a study conducted by Hickey et al. (2021) , they reported that strains of Bifidobacterium breve UCC2003 and B. breve JCM7017, which naturally produce EPS (WT), were compared with isogenic strains lacking EPS (EPS-mutants). The researchers observed that both WT strains lost their ability to produce EPS upon mutation, serving as positive controls for EPS deposition assays. Furthermore, the study investigated the impact of different carbohydrate sources on EPS production. Screening both B. breve strains in media containing glucose, lactose, and maltose revealed that while the WT strains did not precipitate EPS over a 6-h period, their EPS-mutants did. Additionally, the study explored the modulation of EPS on the cytokine response of Bone Marrow-Derived Macrophages (BMDM) and Dendritic Cells (BMDCs). When both EPS-isogenic strains were cultured with primary BMDM for 24 h, the absence of EPS from B. breve UCC2003 led to increased cytokine responses, with BMDM secreting TNF-α and IL10. Conversely, the absence of EPS from B. breve JCM7017 resulted in reduced cytokine responses. BMDCs did not exhibit significant TNF-α or IL10 production in response to any bacterial strain ( Hickey et al., 2021 ).
5 Interaction between Bifidobacterial EPS and immune system
The immune system comprises two main components: innate and acquired immunity. The innate immune system, which is inherited and non-specific, serves to protect the host against microbial invasion and tissue damage ( Ashraf and Shah, 2014 ; Hato and Dagher, 2015 ; Yatim and Lakkis, 2015 ). It operates with a short-term memory and includes various components such as the skin, phagocytic cells like macrophages, dendritic cells (DCs), neutrophils, and protein molecules like the complement and coagulation systems ( Yatim and Lakkis, 2015 ; Kellie and Al-Mansour, 2017 ). In contrast, the acquired immune response is specific to target antigens and involves receptors expressed on B and T lymphocytes. This response becomes prominent a few days after encountering the antigen. Communication between the innate and adaptive immune systems is primarily facilitated by antigen-presenting dendritic cells ( Yatim and Lakkis, 2015 ). Although the mechanisms of action of innate and acquired immunity differ, their cooperation is essential for mounting a fully effective immune response. This collaboration ensures a comprehensive defense against pathogens and other harmful agents ( Hato and Dagher, 2015 ; Kellie and Al-Mansour, 2017 ).
The gastrointestinal tract (GIT) is home to a diverse array of microorganisms, including bacteria belonging to the genus Bifidobacterium , which play a crucial role in promoting host health.
In recent decades, the immunomodulatory capabilities of Bifidobacterium bacteria in interaction with human immune cells have garnered significant scientific interest. The modulating ability of strains within this bacterial genus has been linked to their production of EPSs ( Hidalgo-Cantabrana et al., 2012 ). Bifidobacterium EPS exhibit anti-inflammatory and antimicrobial properties, contributing to their ability to regulate the immune system. Inflammation, which is a normal tissue repair process in response to infections and tissue damage, can lead to various inflammatory reactions such as pain, swelling, and fever due to the production of nitric oxide (NO) and prostaglandin E2 (PGE2; Angelin and Kavitha, 2020 ; Choi et al., 2022 ). Prolonged inflammation can result in excessive or insufficient production of pro-inflammatory cytokines, including IL-6 and TNF-α, and suppression of anti-inflammatory cytokines like IL-10, leading to inflammatory diseases and cancer ( Angelin and Kavitha, 2020 ). Bifidobacterium EPSs have been demonstrated to modulate the inflammatory response of immune cells. For instance, EPS extracted and purified from B. longum BCRC 14634 exhibit mild immunomodulatory activity on J77A.1 macrophages by increasing IL-10 secretion and decreasing TNF-α levels ( Wu et al., 2010 ). Other activities of EPS in modulating the host’s immune system include enhancing the proliferation of T and B lymphocytes, increasing natural killer (NK) cell activity, boosting the phagocytic capacity of mononuclear cells, inducing cytokine production, and enhancing overall host immune defense against pathogens ( Angelin and Kavitha, 2020 ).
Moreover, Bifidobacterium plays a crucial role in reducing the risk of infection and preventing gastrointestinal cancers and inflammatory diseases such as inflammatory bowel disease (IBD; Rajoka et al., 2018 ). For instance, B. longum subsp. longum 35,624 has demonstrated clinical efficacy in irritable bowel syndrome, and comparison with a mutant derivative lacking EPS production illustrated the preventive role of EPS ( Schiavi et al., 2016 ). Bifidobacterium EPS also exert their modulatory effects through antigen-presenting cells (APCs) or dendritic cells. EPS induce dendritic cells to secrete cytokines, leading to the differentiation of naïve T cells into regulatory T cells, which suppress inhibitory T cells, thereby promoting immune balance ( Ashraf and Shah, 2014 ).
Various in vitro and in vivo models have been utilized to study the immunomodulatory activity of EPSs produced by Bifidobacterium strains. These models include peripheral blood mononuclear cells (PBMCs), mouse spleen cells, macrophage-like cell lines, and Gut Associated Lymphatic Tissues (GALT). Additionally, enterocytes such as the CaCo-2 or HT29 cell models have been employed in certain studies to investigate the immunomodulatory potential of Bifidobacterium bacteria due to their direct exposure to the intestinal environment, which could play a pivotal role in the initiation of bifidobacteria -host interactions ( Ruiz et al., 2017 ).
In vivo models using human, mouse, and rat PBMCs have also been employed, and several instances of in vitro and in vivo models demonstrating immune responses are detailed in Table 2 . The immunomodulatory capacity of Bifidobacterium EPS suggests potential health benefits for humans. However, differences in EPS structure and immune regulation between strains of the same Bifidobacterium species can lead to variations in their immunomodulatory effects. There is great structural variation in EPS polymers produced by bifidobacteria, even between strains of the same species ( Hidalgo-Cantabrana et al., 2014b ; Ferrario et al., 2016 ). In addition to differences in glycosidic bonds and degree of branching, changes in monosaccharide components and their amounts have been observed for different bifidobacteria strains ( Hidalgo-Cantabrana et al., 2014b ; Inturri et al., 2017b ). This diversity could, in principle, lead to a large number of distinct EPS structures and theoretically to different immunomodulatory effects on the host ( Hickey et al., 2021 ). For instance, B. breve UCC2003, with a thicker EPS layer, exhibits a more anti-inflammatory phenotype compared to B. breve JCM7017, as evidenced by modulation of macrophage IL-10 and TNFα and dendritic cell expression of Tnfa , Il6 , Il12a , and Il23a . Murine B. pseudolongum UMB287 MBP-01 EPS increases intestinal Tregs, whereas porcine-derived B. pseudolongum ATCC25526 EPS does not. However, EPS from both strains lead to increased dendritic cells (DCs), mesenteric lymph node (MLN) DCs, and intestinal MLN macrophages ( Hickey et al., 2021 ; Gavzy et al., 2023 ).
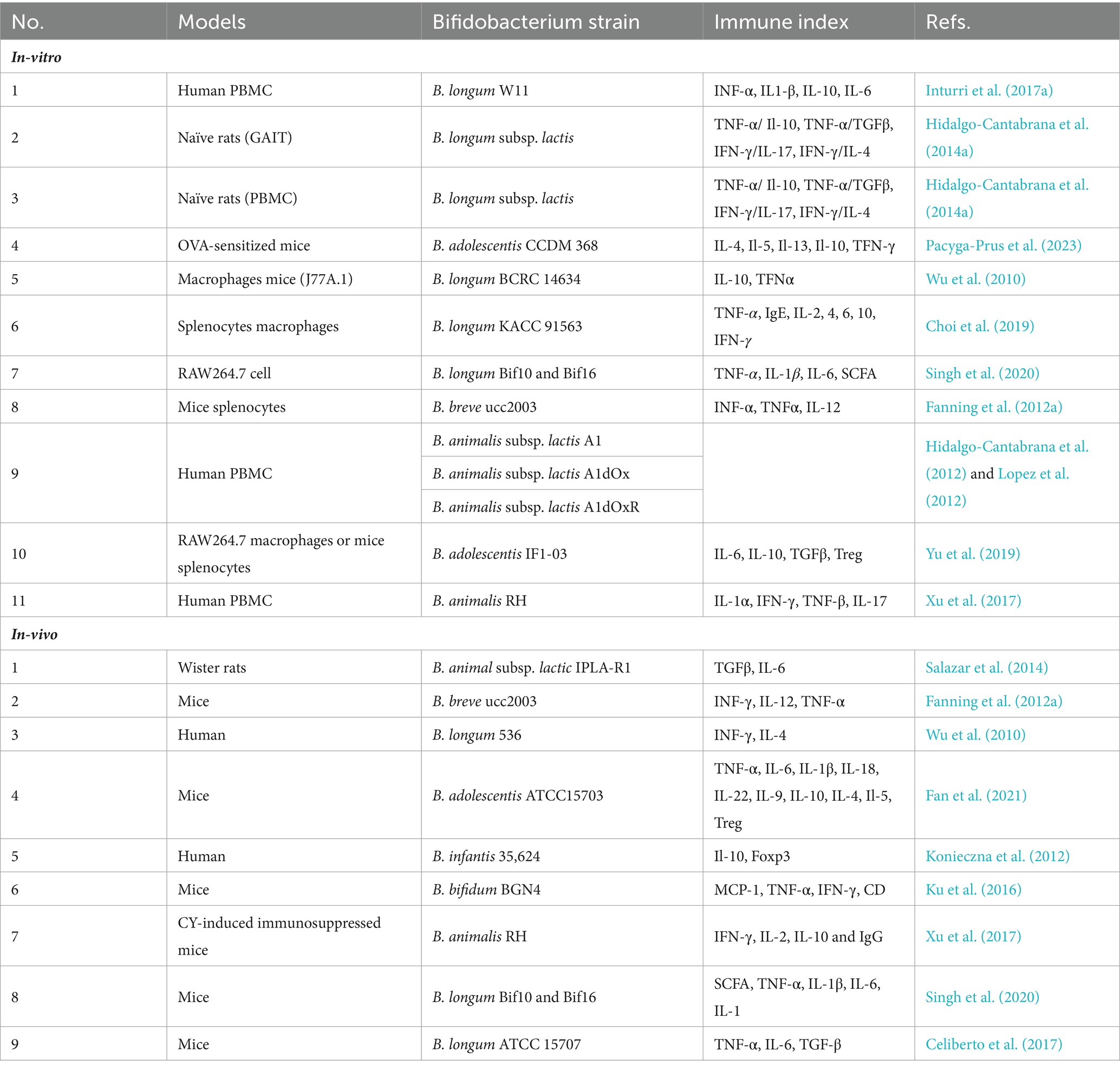
Table 2 . In-vitro and in-vivo models to study the Bifidobacterium EPSs immunomodulatory potential.
The modulatory effects of Bifidobacterium EPS depend on factors such as molecular weight and chemical composition. Generally, EPS with low molecular weight induce higher levels of cytokines, while those with high molecular weight induce a lower cytokine secretion or decrease the TNFα/IL-10 ratio, indicating an anti-inflammatory effect ( Lopez et al., 2012 ; Salazar et al., 2014 ). For example, EPS from B. animalis subsp. lactis IPLA-R1, with a high rhamnose percentage and molecular weight, increases IL-10 production in PBMC models and decreases TNFα production in human colon biopsies ( Hidalgo-Cantabrana et al., 2015 ). Moreover, studies have shown correlations between EPS polymer composition, structure, and size, and the corresponding immune response, suggesting that the physical and chemical characteristics of EPS influence their immunomodulatory properties ( Lopez et al., 2012 ).
Similar findings were reported in a study involving co-incubation of purified EPS from three strains of B. animalis (A1, A1dOx, and A1dOxR) with PBMCs. The analysis revealed that the A1dOxR extracellular polymer caused less release of pro- and anti-inflammatory cytokines compared to other strains, attributed to its higher molecular weight. Additionally, EPS from Bifidobacterium adolescentis strains IF1-11 and IF1-03, with high molecular weight, induced different cytokine profiles when co-cultured with macrophage RAW264.7 and mouse spleen cells, with strain IF1-03 exhibiting anti-inflammatory effects, while strain IF1-11 showed pro-inflammatory effects ( Alessandri et al., 2019 ).
Dendritic cells (DCs) represent a specialized subset of myeloid cells that respond to infection by capturing antigens, processing them into smaller peptides, and subsequently presenting them to T lymphocytes. As primary antigen-presenting cells (APCs), DCs serve as a crucial link between innate and adaptive immunity. Both mouse dendritic cells and human blood mononuclear cells hold promise for future investigations into the modulatory potential of EPSs derived from Bifidobacterium strains.
In vitro studies involving the treatment of DCs with Bifidobacterium bifidum PRI1, followed by co-culture with naïve CD4 T cells, have demonstrated enhanced induction of regulatory T cells (Tregs) and production of interleukin-10 (IL-10; Gavzy et al., 2023 ). These findings underscore the immunomodulatory capabilities of Bifidobacterium EPS, particularly in promoting immune tolerance and anti-inflammatory responses mediated by Tregs and IL-10. Such studies pave the way for further exploration of the interaction between Bifidobacterium -derived EPS and DCs, shedding light on their potential therapeutic applications in immune-related disorders.
One of the extensively researched aspects of immune system modulation by probiotic bacteria is the regulation of cytokine production. Cytokines, which are protein molecules synthesized by immune cells, play diverse roles in defense mechanisms, including inflammation, B and T lymphocyte differentiation, immune system activation, and eradication of foreign antigens. Additionally, cytokines can significantly contribute to the pathogenesis of autoimmune and immune-mediated kidney diseases ( Yatim and Lakkis, 2015 ). Probiotic bacteria can induce the secretion of cytokines from intestinal epithelial cells in a strain-specific manner. For instance, in a study involving mice treated with Bifidobacterium adolescentis ATCC15703, lower levels of pro-inflammatory cytokines such as TNFα, IL-6, IL-1β, IL-18, IL-22, and IL-9 were observed compared to the control group. Conversely, higher levels of the anti-inflammatory cytokine IL-10 and the cytokines IL-4 and IL-5, along with increased regulatory T cells (Tregs), were detected in the colons of colitis mice receiving B. adolescentis ATCC15703 ( Gavzy et al., 2023 ). These findings underscore the strain-specific immunomodulatory effects of probiotic bacteria and highlight their potential therapeutic implications in inflammatory conditions.
The impact of EPS produced by Bifidobacterium longum W11 on the immune response of peripheral blood mononuclear cells (PBMCs), both with and without ConA stimulation, was investigated. Specifically, in unstimulated PBMCs, EPS induced the production of IL-6 at higher concentrations and IL-10 only at lower concentrations. Moreover, when PBMCs were stimulated with ConA, EPS increased the production of various cytokines, except for IL-10 ( Inturri et al., 2017a ). Cytokines serve as soluble mediators of host defense responses, playing crucial roles in both specific and non-specific mechanisms for eliminating foreign antigens ( Ashraf and Shah, 2014 ). The cell surface components of B. longum strains NCC 2705, ATCC 15707, and BIF53 have been shown to stimulate the production of IL-10 and TNFα in isolated peripheral blood mononuclear cells ( Gavzy et al., 2023 ).
Macrophages play a crucial role in recognizing bacteria and, upon activation by microbial metabolites like polysaccharides, they engage in bacterial killing through phagocytosis, secrete cytokines for immune modulation, and present antigens to helper T cells. Notably, intestinal macrophages exhibit restrained proinflammatory cytokine production in response to various inflammatory stimuli, including microbial components ( Wu et al., 2010 ).
In a study investigating the immunomodulatory and anti-inflammatory properties of EPSs from B. longum subsp. infantis E4, conducted on RAW264.7 cells, spleen lymphocytes, and mouse NK cells in vitro , it was found that EPS enhanced the growth and phagocytic activity of RAW264.7 macrophages, increased spleen lymphocyte proliferation, and boosted NK cell activity. These findings suggest that EPS derived from B. infantis E4 possesses immune-modulating and anti-inflammatory properties, potentially serving as a prebiotic for promoting future health maintenance. Hence, EPS from B. longum subsp. infantis E4 could be considered a functional food ingredient with modulatory and anti-inflammatory effects on immune cells, thus broadening the scope of immune modulators ( Yue et al., 2023b ).
In another study, EPS produced by B. longum strain BCRC 14634 was observed to induce increased production of the anti-inflammatory cytokine IL-10 by murine macrophages, compared to baseline conditions. Additionally, the presence of EPS was associated with lower levels of the pro-inflammatory cytokine TNFα, compared to lipopolysaccharides ( Wu et al., 2010 ).
6 Application of Bifidobacterium EPS in the food industry
Beneficial microorganisms like lactic acid bacteria and bifidobacteria possess the capability to produce postbiotic bioactive substances, including EPSs. Leveraging their technological advantages, they are extensively employed as starter cultures in the production of fermented food products ( Xu et al., 2019 ), as well as functional foods ( Sørensen et al., 2022 ), which can directly or indirectly impact human health ( Castro-Bravo et al., 2018b ; Xu et al., 2019 ).
In the food industry, these microorganisms serve as functional additives, contributing to the production of products with desirable attributes. Particularly in the dairy sector, EPSs function as thickeners, emulsifiers, and stabilizers without imparting unpleasant tastes. They prevent water separation in cheese, resulting in a softer and creamier product, thereby increasing cheese yield. Moreover, they enhance yogurt viscosity and water holding capacity ( Xu et al., 2019 ).
Another notable application of EPS is in bakery products, where it increases bread volume and moisture content, resulting in a softer texture for both gluten-containing and gluten-free bread. Additionally, EPSs mitigate staling by impeding starch retrogradation, thereby improving shelf life owing to their water-binding properties ( Xu et al., 2019 ). However, despite their numerous advantages, EPSs can also have detrimental effects. For instance, EPS-producing bacteria can spoil alcoholic beverages like beer and wine. Furthermore, EPS synthesis and the formation of intestinal plaque and biofilm can lead to health issues in the food industry.
As bifidobacteria are commonly utilized as probiotics in dairy products, there is a growing interest in exploring the potential of EPS-producing Bifidobacterium strains as functional starters for low-fat yogurt production ( Prasanna et al., 2013 ). Both lactic acid bacteria and bifidobacteria are known to produce EPS, contributing to the texture and mouthfeel of yogurt, a popular fermented milk product.
In the context of health-conscious consumers preferring low-fat dairy options, the production of low-fat yogurt presents certain challenges, such as compromised texture and taste, characterized by high synthesis and low viscosity. While thickeners can address these issues, regulations prohibiting the addition of stabilizers in yogurt have led to the exploration of EPSs as viable alternatives in the European Union ( Xu et al., 2019 ).
The texture of yogurt is influenced by various factors including milk heating, pH, fermentation duration, milk composition (particularly protein or fat content), as well as the concentration and structure of EPS produced by the starter culture, which plays a pivotal role in yogurt gelation. Thus, the selection of strains with higher EPS production is crucial. However, studies indicate a weak correlation between EPS quantity and resulting effects on rheological properties, underscoring the significance of EPS structures (e.g., monosaccharide composition, charge, molecular weight, degree of branching, and backbone stiffness) and interactions between EPSs and milk components, especially proteins, in determining yogurt texture ( Gentès et al., 2011 ; Han et al., 2016 ). Key textural characteristics of yogurt, including viscosity, syneresis (whey separation), gelation pH, and gel firmness, are considered essential factors in product quality and consumer acceptance ( Xu et al., 2019 ).
The EPS produced by B. infantis CCUG 52486 and B. infantis NCIMB 702205 exhibits notable emulsification activity and favorable rheological properties, leading to enhanced viscosity in fermented low-fat milk. Among these strains, B. longum subsp. infantis CCUG 52486 stands out as particularly promising. It can be effectively incorporated into yogurt starter cultures to produce low-fat yogurt with probiotic benefits while simultaneously improving the physicochemical and rheological characteristics of the product ( Prasanna et al., 2013 ).
Presently, there are 39 species of lactic acid bacteria and 5 species of bifidobacteria that have been granted Generally Recognized as Safe (GRAS) status by the European Food Safety Authority ( Hazards et al., 2022 ). These strains are also included in the Qualified Presumption of Safety (QPS) list, making their application in food matrices more accessible. However, it’s worth noting that neither the EFSA nor the FDA have established any health claims for the use of EPS from lactic acid bacteria in food products ( Hazards et al., 2022 ).
7 Conclusion and future perspective
In recent years, there has been growing interest in the EPS produced by Bifidobacterium bacteria. EPS serve as a crucial external protection and covering for Bifidobacterium , offering resilience against the surrounding environment. The synthesis of EPS involves a complex interplay of molecules, proteins, and enzymes, including glycosyl transferase and polymerases. The structural and chemical characteristics of EPS determine their diverse functions, rendering them beneficial in various industries such as agriculture, dairy, cosmetics, and pharmaceuticals.
In silico analysis conducted on available Bifidobacterium genomes has revealed a lack of consensus structural organization in EPS clusters, unlike those identified in LAB- eps clusters. However, some common features of bifido- eps clusters, such as high inter- and intraspecific organizational diversity, are observed, with the exception of B. animalis subsp. lactis . Additionally, the EPS cluster generally exhibits a lower G + C content compared to the entire bifidobacterial genome. The increasing availability of genomes in the future will offer researchers opportunities for genetic and metabolic engineering to tailor EPS production for use in the food or pharmaceutical industries.
Despite the growing interest, limited research has been conducted on in vitro and in vivo models to assess the immunomodulatory activity of EPS-producing bifidobacteria. Further scientific investigation is needed to enhance EPS efficiency and conduct in vivo studies to explore their therapeutic properties fully. This will enable researchers to harness the full potential of EPS in various applications.
Author contributions
MS: Writing – original draft. BH: Conceptualization, Project administration, Writing – original draft. YN: Writing – review & editing.
The author(s) declare that no financial support was received for the research, authorship, and/or publication of this article.
Conflict of interest
The authors declare that the research was conducted in the absence of any commercial or financial relationships that could be construed as a potential conflict of interest.
Publisher’s note
All claims expressed in this article are solely those of the authors and do not necessarily represent those of their affiliated organizations, or those of the publisher, the editors and the reviewers. Any product that may be evaluated in this article, or claim that may be made by its manufacturer, is not guaranteed or endorsed by the publisher.
Alessandri, G., Ossiprandi, M. C., MacSharry, J., van Sinderen, D., and Ventura, M. (2019). Bifidobacterial dialogue with its human host and consequent modulation of the immune system. Front. Immunol. 10:2348. doi: 10.3389/fimmu.2019.02348
PubMed Abstract | Crossref Full Text | Google Scholar
Alessandri, G., van Sinderen, D., and Ventura, M. (2021). The genus Bifidobacterium: from genomics to functionality of an important component of the mammalian gut microbiota. Comput. Struct. Biotechnol. J. 19, 1472–1487. doi: 10.1016/j.csbj.2021.03.006
Altmann, F., Kosma, P., O’Callaghan, A., Leahy, S., Bottacini, F., Molloy, E., et al. (2016). Genome analysis and characterisation of the exopolysaccharide produced by Bifidobacterium longum subsp. longum 35624™. PLoS One 11:e0162983. doi: 10.1371/journal.pone.0162983
Angelin, J., and Kavitha, M. (2020). Exopolysaccharides from probiotic bacteria and their health potential. Int. J. Biol. Macromol. 162, 853–865. doi: 10.1016/j.ijbiomac.2020.06.190
Ashraf, R., and Shah, N. P. (2014). Immune system stimulation by probiotic microorganisms. Crit. Rev. Food Sci. Nutr. 54, 938–956. doi: 10.1080/10408398.2011.619671
Crossref Full Text | Google Scholar
Audy, J., Labrie, S., Roy, D., and LaPointe, G. (2010). Sugar source modulates exopolysaccharide biosynthesis in Bifidobacterium longum subsp. longum CRC 002. Microbiology 156, 653–664. doi: 10.1099/mic.0.033720-0
Bhagat, N., Raghav, M., Dubey, S., and Bedi, N. (2021). Bacterial exopolysaccharides: insight into their role in plant abiotic stress tolerance. J. Microbiol. Biotechnol. 31, 1045–1059. doi: 10.4014/jmb.2105.05009
Boels, I. C., van Kranenburg, R., Hugenholtz, J., Kleerebezem, M., and De Vos, W. M. (2001). Sugar catabolism and its impact on the biosynthesis and engineering of exopolysaccharide production in lactic acid bacteria. Int. Dairy J. 11, 723–732. doi: 10.1016/S0958-6946(01)00116-9
Carezzano, M. E., Alvarez Strazzi, F. B., Pérez, V., Bogino, P., and Giordano, W. (2023). Exopolysaccharides synthesized by Rhizospheric Bacteria: a review focused on their roles in protecting plants against stress. Appl. Microbiol. 3, 1249–1261. doi: 10.3390/applmicrobiol3040086
Castro-Bravo, N., Hidalgo-Cantabrana, C., Rodriguez-Carvajal, M. A., Ruas-Madiedo, P., and Margolles, A. (2017). Gene replacement and fluorescent labeling to study the functional role of exopolysaccharides in Bifidobacterium animalis subsp. lactis. Front. Microbiol. 8:1405. doi: 10.3389/fmicb.2017.01405
Castro-Bravo, N., Sánchez, B., Margolles, A., and Ruas-Madiedo, P. (2018a). “Biological activities and applications of bifidobacterial exopolysaccharides: from the bacteria and host perspective” in The Bifidobacteria and related organisms . eds. P. Mattarelli, B. Biavati, and B. J. B. Wood (Elsevier), 177–193.
Google Scholar
Castro-Bravo, N., Wells, J. M., Margolles, A., and Ruas-Madiedo, P. (2018b). Interactions of surface exopolysaccharides from Bifidobacterium and Lactobacillus within the intestinal environment. Front. Microbiol. 9:2426. doi: 10.3389/fmicb.2018.02426
Celiberto, L. S., Bedani, R., Dejani, N. N., Ivo de Medeiros, A., Sampaio Zuanon, J. A., Spolidorio, L. C., et al. (2017). Effect of a probiotic beverage consumption ( Enterococcus faecium CRL 183 and Bifidobacterium longum ATCC 15707) in rats with chemically induced colitis. PLoS One 12:e0175935. doi: 10.1371/journal.pone.0175935
Chaib De Mares, M., Hess, J., Floudas, D., Lipzen, A., Choi, C., Kennedy, M., et al. (2015). Horizontal transfer of carbohydrate metabolism genes into ectomycorrhizal a manita. New Phytol. 205, 1552–1564. doi: 10.1111/nph.13140
Chen, Q., Lu, X., Guo, X., Guo, Q., and Li, D. (2017). Metabolomics characterization of two Apocynaceae plants, Catharanthus roseus and Vinca minor , using GC-MS and LC-MS methods in combination. Molecules 22:997. doi: 10.3390/molecules22060997
Choi, M., Lee, Y., Lee, N.-K., Bae, C. H., Park, D. C., Paik, H.-D., et al. (2019). Immunomodulatory effects by Bifidobacterium longum KACC 91563 in mouse splenocytes and macrophages. J. Microbiol. Biotechnol. 29, 1739–1744. doi: 10.4014/jmb.1812.12002
Choi, Y. J., Shin, S.-H., and Shin, H. S. (2022). Immunomodulatory effects of Bifidobacterium spp. and use of Bifidobacterium breve and Bifidobacterium longum on acute diarrhea in children. J. Microbiol. Biotechnol. 32, 1186–1194. doi: 10.4014/jmb.2206.06023
Cuthbertson, L., Mainprize, I. L., Naismith, J. H., and Whitfield, C. (2009). Pivotal roles of the outer membrane polysaccharide export and polysaccharide copolymerase protein families in export of extracellular polysaccharides in gram-negative bacteria. Microbiol. Mol. Biol. Rev. 73, 155–177. doi: 10.1128/MMBR.00024-08
Eshaghi, M., Bibalan, M. H., Rohani, M., Esghaei, M., Douraghi, M., Talebi, M., et al. (2017). Bifidobacterium obtained from mother’s milk and their infant stool; a comparative genotyping and antibacterial analysis. Microb. Pathog. 111, 94–98. doi: 10.1016/j.micpath.2017.08.014
Fan, L., Qi, Y., Qu, S., Chen, X., Li, A., Hendi, M., et al. (2021). B. adolescentis ameliorates chronic colitis by regulating Treg/Th2 response and gut microbiota remodeling. Gut Microbes 13, 1–17. doi: 10.1080/19490976.2020.1826746
Fanning, S., Hall, L. J., Cronin, M., Zomer, A., MacSharry, J., Goulding, D., et al. (2012a). Bifidobacterial surface-exopolysaccharide facilitates commensal-host interaction through immune modulation and pathogen protection. Proc. Natl. Acad. Sci. 109, 2108–2113. doi: 10.1073/pnas.1115621109
Fanning, S., Hall, L. J., and van Sinderen, D. (2012b). Bifidobacterium breve UCC2003 surface exopolysaccharide production is a beneficial trait mediating commensal-host interaction through immune modulation and pathogen protection. Gut Microbes 3, 420–425. doi: 10.4161/gmic.20630
Ferrario, C., Milani, C., Mancabelli, L., Lugli, G. A., Duranti, S., Mangifesta, M., et al. (2016). Modulation of the eps-ome transcription of bifidobacteria through simulation of human intestinal environment. FEMS Microbiol. Ecol. 92:fiw056. doi: 10.1093/femsec/fiw056
Gavzy, S. J., Kensiski, A., Lee, Z. L., Mongodin, E. F., Ma, B., and Bromberg, J. S. (2023). Bifidobacterium mechanisms of immune modulation and tolerance. Gut Microbes 15:2291164. doi: 10.1080/19490976.2023.2291164
Gentès, M.-C., St-Gelais, D., and Turgeon, S. L. (2011). Gel formation and rheological properties of fermented milk with in situ exopolysaccharide production by lactic acid bacteria. Dairy Sci. Technol. 91, 645–661. doi: 10.1007/s13594-011-0039-0
Habu, Y., Nagaoka, M., Yokokura, T., and Azuma, I. (1987). Structural studies of cell wall polysaccharides from Bifidobacterium breve YIT 4010 and related Bifidobacterium species. J. Biochem. 102, 1423–1432. doi: 10.1093/oxfordjournals.jbchem.a122188
Han, X., Yang, Z., Jing, X., Yu, P., Zhang, Y., Yi, H., et al. (2016). Improvement of the texture of yogurt by use of exopolysaccharide producing lactic acid bacteria. Biomed. Res. Int. 2016, 1–6. doi: 10.1155/2016/7945675
Hato, T., and Dagher, P. C. (2015). How the innate immune system senses trouble and causes trouble. Clin. J. Am. Soc. Nephrol. 10, 1459–1469. doi: 10.2215/cjn.04680514
Hazards, E. P. O. B., Koutsoumanis, K., Allende, A., Alvarez-Ordóñez, A., Bolton, D., Bover-Cid, S., et al. (2022). Update of the list of QPS-recommended biological agents intentionally added to food or feed as notified to EFSA 15: suitability of taxonomic units notified to EFSA until September 2021. EFSA J. 20:e07045. doi: 10.2903/j.efsa.2022.7045
Hickey, A., Stamou, P., Udayan, S., Ramón-Vázquez, A., Esteban-Torres, M., Bottacini, F., et al. (2021). Bifidobacterium breve exopolysaccharide blocks dendritic cell maturation and activation of CD4+ T cells. Front. Microbiol. 12:653587. doi: 10.3389/fmicb.2021.653587
Hidalgo-Cantabrana, C., Delgado, S., Ruiz, L., Ruas-Madiedo, P., Sánchez, B., and Margolles, A. (2018). “Bifidobacteria and their health-promoting effects” in Bugs as Drugs: Therapeutic Microbes for the Prevention and Treatment of Disease . eds. R. A. Britton and P. D. Cani (Wiley), 73–98.
Hidalgo-Cantabrana, C., López, P., Gueimonde, M., de Los Reyes-Gavilán, C. G., Suárez, A., Margolles, A., et al. (2012). Immune modulation capability of exopolysaccharides synthesised by lactic acid bacteria and bifidobacteria. Probiotics Antimicrob Proteins 4, 227–237. doi: 10.1007/s12602-012-9110-2
Hidalgo-Cantabrana, C., Nikolic, M., López, P., Suárez, A., Miljkovic, M., Kojic, M., et al. (2014a). Exopolysaccharide-producing Bifidobacterium animalis subsp. lactis strains and their polymers elicit different responses on immune cells from blood and gut associated lymphoid tissue. Anaerobe 26, 24–30. doi: 10.1016/j.anaerobe.2014.01.003
Hidalgo-Cantabrana, C., Sánchez, B., Álvarez-Martín, P., López, P., Martínez-Álvarez, N., Delley, M., et al. (2015). A single mutation in the gene responsible for the mucoid phenotype of Bifidobacterium animalis subsp. lactis confers surface and functional characteristics. Appl. Environ. Microbiol. 81, 7960–7968. doi: 10.1128/AEM.02095-15
Hidalgo-Cantabrana, C., Sánchez, B., Milani, C., Ventura, M., Margolles, A., and Ruas-Madiedo, P. (2014b). Genomic overview and biological functions of exopolysaccharide biosynthesis in Bifidobacterium spp. Appl. Environ. Microbiol. 80, 9–18. doi: 10.1128/AEM.02977-13
Inturri, R., Mangano, K., Santagati, M., Intrieri, M., Di Marco, R., and Blandino, G. (2017a). Immunomodulatory effects of Bifidobacterium longum W11 produced exopolysaccharide on cytokine production. Curr. Pharm. Biotechnol. 18, 883–889. doi: 10.2174/1389201019666171226151551
Inturri, R., Molinaro, A., Di Lorenzo, F., Blandino, G., Tomasello, B., Hidalgo-Cantabrana, C., et al. (2017b). Chemical and biological properties of the novel exopolysaccharide produced by a probiotic strain of Bifidobacterium longum . Carbohydr. Polym. 174, 1172–1180. doi: 10.1016/j.carbpol.2017.07.039
Jurášková, D., Ribeiro, S. C., and Silva, C. C. (2022). Exopolysaccharides produced by lactic acid bacteria: from biosynthesis to health-promoting properties. Food Secur. 11:156. doi: 10.3390/foods11020156
Kaur, N., and Dey, P. (2023). Bacterial exopolysaccharides as emerging bioactive macromolecules: from fundamentals to applications. Res. Microbiol. 174:104024. doi: 10.1016/j.resmic.2022.104024
Kellie, S., and Al-Mansour, Z. (2017). “Overview of the immune system” in Micro and nanotechnology in vaccine development . eds. M. S. Czynski and I. Toth (Elsevier), 63–81.
Kohno, M., Suzuki, S., Kanaya, T., Yoshino, T., Matsuura, Y., Asada, M., et al. (2009). Structural characterization of the extracellular polysaccharide produced by Bifidobacterium longum JBL05. Carbohydr. Polym. 77, 351–357. doi: 10.1016/j.carbpol.2009.01.013
Konieczna, P., Akdis, C. A., Quigley, E. M., Shanahan, F., and O’Mahony, L. (2012). Portrait of an immunoregulatory Bifidobacterium. Gut Microbes 3, 261–266. doi: 10.4161/gmic.20358
Korcz, E., and Varga, L. (2021). Exopolysaccharides from lactic acid bacteria: techno-functional application in the food industry. Trends Food Sci. Technol. 110, 375–384. doi: 10.1016/j.tifs.2021.02.014
Ku, S., Park, M. S., Ji, G. E., and You, H. J. (2016). Review on Bifidobacterium bifidum BGN4: functionality and nutraceutical applications as a probiotic microorganism. Int. J. Mol. Sci. 17:1544. doi: 10.3390/ijms17091544
Laiño, J., Villena, J., Kanmani, P., and Kitazawa, H. (2016). Immunoregulatory effects triggered by lactic acid bacteria exopolysaccharides: new insights into molecular interactions with host cells. Microorganisms 4:27. doi: 10.3390/microorganisms4030027
Lee, J. H., and O’Sullivan, D. J. (2010). Genomic insights into bifidobacteria. Microbiol. Mol. Biol. R. 74, 378–416.
Leivers, S., Hidalgo-Cantabrana, C., Robinson, G., Margolles, A., Ruas-Madiedo, P., and Laws, A. P. (2011). Structure of the high molecular weight exopolysaccharide produced by Bifidobacterium animalis subsp. lactis IPLA-R1 and sequence analysis of its putative eps cluster. Carbohydr. Res. 346, 2710–2717. doi: 10.1016/j.carres.2011.09.010
Li, J., Wang, J., Wang, M., Zheng, L., Cen, Q., Wang, F., et al. (2023). Bifidobacterium: a probiotic for the prevention and treatment of depression. Front. Microbiol. 14:1174800. doi: 10.3389/fmicb.2023.1174800
Lim, P., Loke, C., Ho, Y., and Tan, H. (2020). Cholesterol homeostasis associated with probiotic supplementation in vivo. J. Appl. Microbiol. 129, 1374–1388. doi: 10.1111/jam.14678
Liu, G., Chen, H., Chen, J., Wang, X., Gu, Q., and Yin, Y. (2019). Effects of bifidobacteria-produced exopolysaccharides on human gut microbiota in vitro. Appl. Microbiol. Biotechnol. 103, 1693–1702. doi: 10.1007/s00253-018-9572-6
Liu, L., Qin, Y., Wang, Y., Li, H., Shang, N., and Li, P. (2014). Complete genome sequence of Bifidobacterium animalis RH, a probiotic bacterium producing exopolysaccharides. J. Biotechnol. 189, 86–87. doi: 10.1016/j.jbiotec.2014.08.041
Llamas-Arriba, M. G., Peiroten, A., Puertas, A. I., Prieto, A., Lopez, P., Pardo, M. A., et al. (2019). Heteropolysaccharide-producing bifidobacteria for the development of functional dairy products. LWT 102, 295–303. doi: 10.1016/j.lwt.2018.12.044
Lopez, P., Monteserin, D. C., Gueimonde, M., de los Reyes-Gavilan, C. G., Margolles, A., Suarez, A., et al. (2012). Exopolysaccharide-producing Bifidobacterium strains elicit different in vitro responses upon interaction with human cells. Food Res. Int. 46, 99–107. doi: 10.1016/j.foodres.2011.11.020
Lynch, K. M., Zannini, E., Coffey, A., and Arendt, E. K. (2018). Lactic acid bacteria exopolysaccharides in foods and beverages: isolation, properties, characterization, and health benefits. Annu. Rev. Food Sci. Technol. 9, 155–176. doi: 10.1146/annurev-food-030117-012537
Mohd Nadzir, M., Nurhayati, R. W., Idris, F. N., and Nguyen, M. H. (2021). Biomedical applications of bacterial exopolysaccharides: a review. Polymers 13:530. doi: 10.3390/polym13040530
Nagaoka, M., Shibata, H., Kimura, I., Hashimoto, S., Kimura, K., Sawada, H., et al. (1995). Structural studies on a cell wall polysaccharide from Bifidobacterium longum YIT4028. Carbohydr. Res. 274, 245–249. doi: 10.1016/0008-6215(95)00076-6
Netrusov, A. I., Liyaskina, E. V., Kurgaeva, I. V., Liyaskina, A. U., Yang, G., and Revin, V. V. (2023). Exopolysaccharides producing Bacteria: a review. Microorganisms 11:1541. doi: 10.3390/microorganisms11061541
Nicolescu, C. M., Bumbac, M., Buruleanu, C. L., Popescu, E. C., Stanescu, S. G., Georgescu, A. A., et al. (2023). Biopolymers produced by lactic acid Bacteria: characterization and food application. Polymers 15:1539. doi: 10.3390/polym15061539
Pacyga-Prus, K., Jakubczyk, D., Sandström, C., Šrůtková, D., Pyclik, M. J., Leszczyńska, K., et al. (2023). Polysaccharide BAP1 of Bifidobacterium adolescentis CCDM 368 is a biologically active molecule with immunomodulatory properties. Carbohydr. Polym. 315:120980. doi: 10.1016/j.carbpol.2023.120980
Prasanna, P., Grandison, A., and Charalampopoulos, D. (2013). Microbiological, chemical and rheological properties of low fat set yoghurt produced with exopolysaccharide (EPS) producing Bifidobacterium strains. Food Res. Int. 51, 15–22. doi: 10.1016/j.foodres.2012.11.016
Rajoka, M. S. R., Jin, M., Haobin, Z., Li, Q., Shao, D., Jiang, C., et al. (2018). Functional characterization and biotechnological potential of exopolysaccharide produced by Lactobacillus rhamnosus strains isolated from human breast milk. LWT 89, 638–647. doi: 10.1016/j.lwt.2017.11.034
Ruiz, L., Delgado, S., Ruas-Madiedo, P., Sánchez, B., and Margolles, A. (2017). Bifidobacteria and their molecular communication with the immune system. Front. Microbiol. 8:2345. doi: 10.3389/fmicb.2017.02345
Salazar, N., Gueimonde, M., De Los Reyes-Gavilán, C. G., and Ruas-Madiedo, P. (2016). Exopolysaccharides produced by lactic acid bacteria and bifidobacteria as fermentable substrates by the intestinal microbiota. Crit. Rev. Food Sci. Nutr. 56, 1440–1453. doi: 10.1080/10408398.2013.770728
Salazar, N., López, P., Garrido, P., Moran, J., Cabello, E., Gueimonde, M., et al. (2014). Immune modulating capability of two exopolysaccharide-producing Bifidobacterium strains in a Wistar rat model. Biomed. Res. Int. 2014, 1–9. doi: 10.1155/2014/106290
Salazar, N., Prieto, A., Leal, J., Mayo, B., Bada-Gancedo, J. C., de Los Reyes-Gavilán, C., et al. (2009). Production of exopolysaccharides by Lactobacillus and Bifidobacterium strains of human origin, and metabolic activity of the producing bacteria in milk. J. Dairy Sci. 92, 4158–4168. doi: 10.3168/jds.2009-2126
Salimi, F., and Farrokh, P. (2023). Recent advances in the biological activities of microbial exopolysaccharides. World J. Microbiol. Biotechnol. 39:213. doi: 10.1007/s11274-023-03660-x
Schell, M. A., Karmirantzou, M., Snel, B., Vilanova, D., Berger, B., Pessi, G., et al. (2002). The genome sequence of Bifidobacterium longum reflects its adaptation to the human gastrointestinal tract. Proc. Natl. Acad. Sci. 99, 14422–14427. doi: 10.1073/pnas.212527599
Schiavi, E., Gleinser, M., Molloy, E., Groeger, D., Frei, R., Ferstl, R., et al. (2016). The surface-associated exopolysaccharide of Bifidobacterium longum 35624 plays an essential role in dampening host proinflammatory responses and repressing local TH17 responses. Appl. Environ. Microbiol. 82, 7185–7196. doi: 10.1128/AEM.02238-16
Schmid, J. (2018). Recent insights in microbial exopolysaccharide biosynthesis and engineering strategies. Curr. Opin. Biotechnol. 53, 130–136. doi: 10.1016/j.copbio.2018.01.005
Schmid, J., Sieber, V., and Rehm, B. (2015). Bacterial exopolysaccharides: biosynthesis pathways and engineering strategies. Front. Microbiol. 6:147519. doi: 10.3389/fmicb.2015.00496
Shang, N., Xu, R., and Li, P. (2013). Structure characterization of an exopolysaccharide produced by Bifidobacterium animalis RH. Carbohydr. Polym. 91, 128–134. doi: 10.1016/j.carbpol.2012.08.012
Shukla, P. J., Nathani, N. M., and Dave, B. P. (2017). Marine bacterial exopolysaccharides [EPSs] from extreme environments and their biotechnological applications. Int J Res Biosci 6, 20–32
Singh, S., Bhatia, R., Khare, P., Sharma, S., Rajarammohan, S., Bishnoi, M., et al. (2020). Anti-inflammatory Bifidobacterium strains prevent dextran sodium sulfate induced colitis and associated gut microbial dysbiosis in mice. Sci. Rep. 10:18597. doi: 10.1038/s41598-020-75702-5
Sørensen, H. M., Rochfort, K. D., Maye, S., MacLeod, G., Brabazon, D., Loscher, C., et al. (2022). Exopolysaccharides of lactic acid bacteria: production, purification and health benefits towards functional food. Nutrients 14:2938. doi: 10.3390/nu14142938
Sun, X., and Zhang, J. (2021). Bacterial exopolysaccharides: chemical structures, gene clusters and genetic engineering. Int. J. Biol. Macromol. 173, 481–490. doi: 10.1016/j.ijbiomac.2021.01.139
Tone-Shimokawa, Y., Toida, T., and Kawashima, T. (1996). Isolation and structural analysis of polysaccharide containing galactofuranose from the cell walls of Bifidobacterium infantis . J. Bacteriol. 178, 317–320. doi: 10.1128/jb.178.1.317-320.1996
Turroni, F., Duranti, S., Milani, C., Lugli, G. A., van Sinderen, D., and Ventura, M. (2019). Bifidobacterium bifidum : a key member of the early human gut microbiota. Microorganisms 7:544. doi: 10.3390/microorganisms7110544
Wachi, S., Kanmani, P., Tomosada, Y., Kobayashi, H., Yuri, T., Egusa, S., et al. (2014). Lactobacillus delbrueckii TUA 4408 L and its extracellular polysaccharides attenuate enterotoxigenic E scherichia coli-induced inflammatory response in porcine intestinal epitheliocytes via T oll-like receptor-2 and 4. Mol. Nutr. Food Res. 58, 2080–2093. doi: 10.1002/mnfr.201400218
Wang, L., Wang, Y., Li, Q., Tian, K., Xu, L., Liu, G., et al. (2019). Exopolysaccharide, isolated from a novel strain Bifidobacterium breve lw01 possess an anticancer effect on head and neck cancer–genetic and biochemical evidences. Front. Microbiol. 10:1044. doi: 10.3389/fmicb.2019.01044
Whitfield, C., Wear, S. S., and Sande, C. (2020). Assembly of bacterial capsular polysaccharides and exopolysaccharides. Annu. Rev. Microbiol. 74, 521–543. doi: 10.1146/annurev-micro-011420-075607
Willis, L. M., and Whitfield, C. (2013). Structure, biosynthesis, and function of bacterial capsular polysaccharides synthesized by ABC transporter-dependent pathways. Carbohydr. Res. 378, 35–44. doi: 10.1016/j.carres.2013.05.007
Wu, M.-H., Pan, T.-M., Wu, Y.-J., Chang, S.-J., Chang, M.-S., and Hu, C.-Y. (2010). Exopolysaccharide activities from probiotic bifidobacterium: immunomodulatory effects (on J774A. 1 macrophages) and antimicrobial properties. Int. J. Food Microbiol. 144, 104–110. doi: 10.1016/j.ijfoodmicro.2010.09.003
Xie, Y., Ye, Z., Deng, H., Sun, W., Wan, X., He, X., et al. (2023). Screening of exopolysaccharide-producing Enterobacter aerogenes NJ1023 and its cadaverine biosynthesis promotion. Front. Microbiol. 14:1200123. doi: 10.3389/fmicb.2023.1200123
Xu, Y., Cui, Y., Yue, F., Liu, L., Shan, Y., Liu, B., et al. (2019). Exopolysaccharides produced by lactic acid bacteria and Bifidobacteria: structures, physiochemical functions and applications in the food industry. Food Hydrocoll. 94, 475–499. doi: 10.1016/j.foodhyd.2019.03.032
Xu, R., Shen, Q., Wu, R., and Li, P. (2017). Structural analysis and mucosal immune regulation of exopolysaccharide fraction from Bifidobacterium animalis RH. Food Agric. Immunol. 28, 1226–1241. doi: 10.1080/09540105.2017.1333578
Yang, X., Liang, Q., Chen, Y., and Wang, B. (2019). Alteration of methanogenic archaeon by ethanol contribute to the enhancement of biogenic methane production of lignite. Front. Microbiol. 10:2323. doi: 10.3389/fmicb.2019.02323
Yao, S., Zhao, Z., Wang, W., and Liu, X. (2021). Bifidobacterium longum : protection against inflammatory bowel disease. J. Immunol. Res. 2021, 1–11. doi: 10.1155/2021/8030297
Yatim, K. M., and Lakkis, F. G. (2015). A brief journey through the immune system. Clin. J. Am. Soc. Nephrol. 10, 1274–1281. doi: 10.2215/CJN.10031014
Yu, R., Zuo, F., Ma, H., and Chen, S. (2019). Exopolysaccharide-producing Bifidobacterium adolescentis strains with similar adhesion property induce differential regulation of inflammatory immune response in Treg/Th17 axis of DSS-colitis mice. Nutrients 11:782. doi: 10.3390/nu11040782
Yue, Y., Wang, Y., Han, Y., Zhang, Y., Cao, T., Huo, G., et al. (2023a). Genome analysis of Bifidobacterium Bifidum E3, structural characteristics, and antioxidant properties of exopolysaccharides. Food Secur. 12:2988. doi: 10.3390/foods12162988
Yue, Y., Wang, Y., Yang, R., Liu, D., Cheng, Y., Li, S., et al. (2023b). Exopolysaccharides of Bifidobacterium longum subsp. infantis E4 on the immune and anti-inflammatory effects in vitro. J. Funct. Foods 107:105699. doi: 10.1016/j.jff.2023.105699
Zannini, E., Waters, D. M., Coffey, A., and Arendt, E. K. (2016). Production, properties, and industrial food application of lactic acid bacteria-derived exopolysaccharides. Appl. Microbiol. Biotechnol. 100, 1121–1135. doi: 10.1007/s00253-015-7172-2
Zhou, Y., Cui, Y., and Qu, X. (2019). Exopolysaccharides of lactic acid bacteria: structure, bioactivity and associations: a review. Carbohydr. Polym. 207, 317–332. doi: 10.1016/j.carbpol.2018.11.093
Zuo, F., Chen, S., and Marcotte, H. (2020). Engineer probiotic bifidobacteria for food and biomedical applications-current status and future prospective. Biotechnol. Adv. 45:107654. doi: 10.1016/j.biotechadv.2020.107654
Keywords: Bifidobacterium , exopolysaccharide, lactic acid bacteria, probiotics, immune system modulation
Citation: Sadeghi M, Haghshenas B and Nami Y (2024) Bifidobacterium exopolysaccharides: new insights into engineering strategies, physicochemical functions, and immunomodulatory effects on host health. Front. Microbiol . 15:1396308. doi: 10.3389/fmicb.2024.1396308
Received: 05 March 2024; Accepted: 26 April 2024; Published: 06 May 2024.
Reviewed by:
Copyright © 2024 Sadeghi, Haghshenas and Nami. This is an open-access article distributed under the terms of the Creative Commons Attribution License (CC BY) . The use, distribution or reproduction in other forums is permitted, provided the original author(s) and the copyright owner(s) are credited and that the original publication in this journal is cited, in accordance with accepted academic practice. No use, distribution or reproduction is permitted which does not comply with these terms.
*Correspondence: Babak Haghshenas, [email protected] ; Yousef Nami, [email protected]
Disclaimer: All claims expressed in this article are solely those of the authors and do not necessarily represent those of their affiliated organizations, or those of the publisher, the editors and the reviewers. Any product that may be evaluated in this article or claim that may be made by its manufacturer is not guaranteed or endorsed by the publisher.
You are using an outdated browser. Please upgrade your browser to improve your experience.
It appears JavaScript is disabled. To get the most out of the website we recommend enabling JavaScript in your browser.
- News and events
RCSI research warns of hazardous health risks from flavoured vapes
- General news
New RCSI research has uncovered the potentially harmful substances that are produced when e-liquids in vaping devices are heated for inhalation.
The study, published in Scientific Reports , highlights the urgent need for public health policies concerning flavoured vapes.
The research team in RCSI’s Department of Chemistry used artificial intelligence (AI) to simulate the effects of heating e-liquid flavour chemicals found in nicotine vapes. They included all 180 known e-liquid flavour chemicals, predicting the new compounds formed when these substances are heated within a vaping device immediately prior to inhalation.
The analysis revealed the formation of many hazardous chemicals including 127 that are classified as ‘acute toxic’, 153 as ‘health hazards’ and 225 as ‘irritants’. Notably, these included a group of chemicals called volatile carbonyls (VCs), which are known to pose health risks. Sources for VCs were predicted to be the most popular fruit, candy and dessert-flavoured products.
Lead author Professor Donal O’Shea , Professor of Chemistry and Head of Department, said the findings are very concerning: “We wanted to understand, before it’s too late, the likely impact flavoured vapes are having on the health of the growing number of vapers. Our findings indicate a significantly different profile of chemical hazards compared to what we are familiar with from traditional tobacco smoking.
“It is plausible that we are on the cusp of a new wave of chronic diseases that will emerge 15 to 20 years from now due to these exposures. We hope this research will help people make more informed choices and contribute to the conversation on the potential long-term health risks and the regulation of vaping, which this research suggests should be comprehensive.”
The study also highlighted the complexities introduced by the huge array of flavours available in vaping products, which include 180 different chemicals blended in various amounts. This cocktail of chemicals, primarily derived from the food industry where they have a good safety record for specific uses, were never intended to be heated to high temperatures for inhalation.
As vaping devices vary widely and are often user-customised, the temperature control and resulting chemical reactions can differ, increasing the unpredictability of potential health risks. This variability requires further research using the AI framework established in this study, which could also lead to the development of risk reports for individual flavours, providing an informative public health policy resource.
Considering the popularity of flavoured vapes among non-smoking teenagers and young adults, understanding the long-term effects of these products on public health, morbidity and mortality is crucial. This study demonstrates that without comprehensive regulation, as we try to treat the nicotine addictions of older tobacco smokers, there is a substantial risk of transferring new health issues to younger generations.
The research was carried out in conjunction with IBM Research – Tokyo and was supported by Science Foundation Ireland (SFI) and the Irish Research Council through the SFI-IRC Pathway Programme for Dr Dan Wu. Details are published in the paper ‘Forecasting vaping health risks through neural network model prediction of flavour pyrolysis reactions’ in Scientific Reports .
Latest news
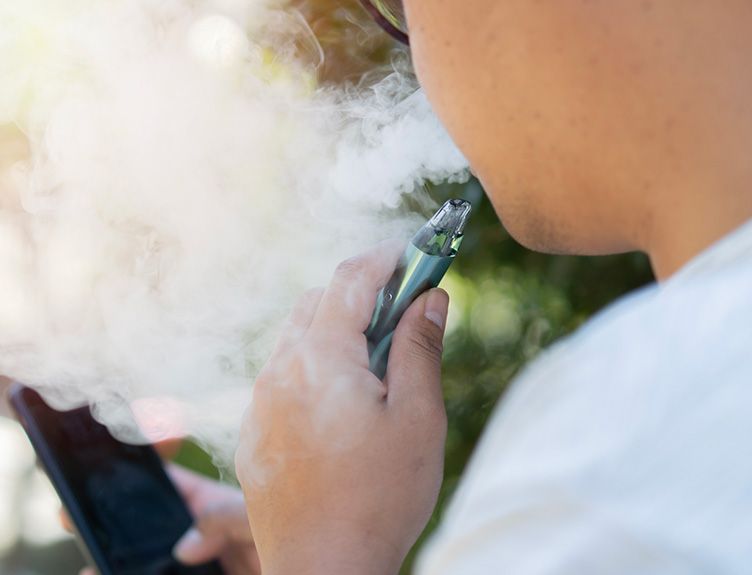
08 May 2024
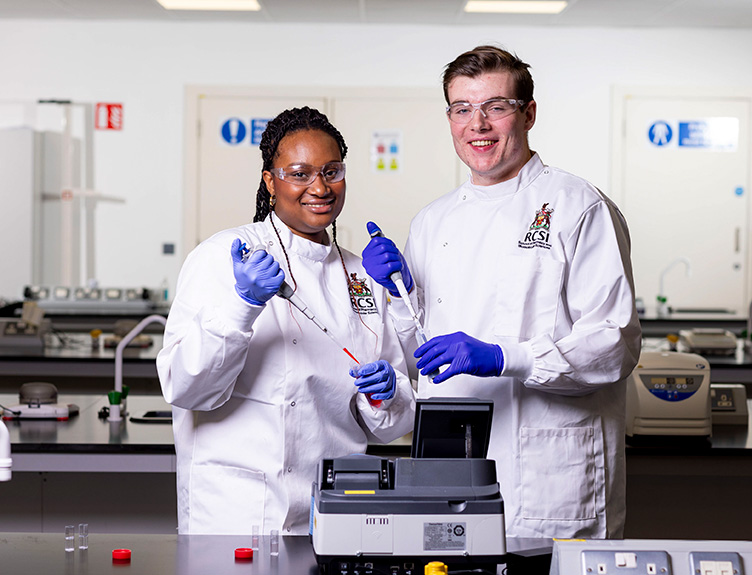
07 May 2024
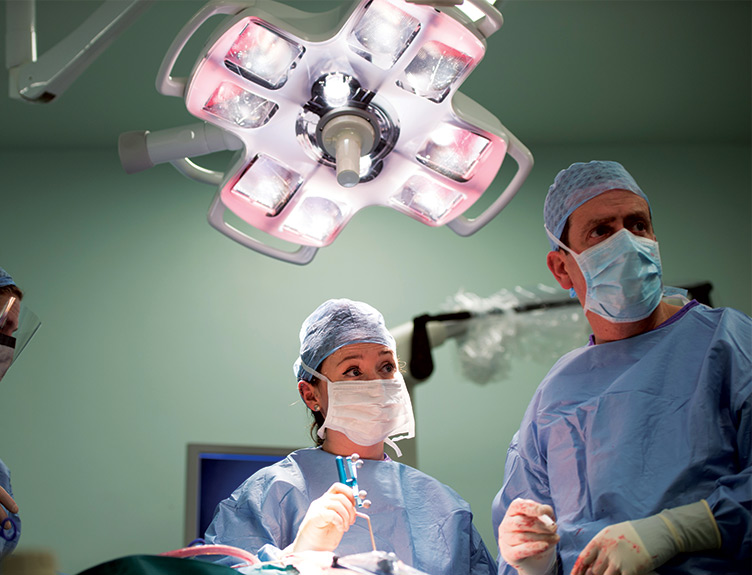
- Search the site GO Please fill out this field.
- Newsletters
Health Benefits of Turmeric (Curcumin)
:max_bytes(150000):strip_icc():format(webp)/20220908_135328-0c8907e94f384cbfbfe09a9c660bdda3.jpg)
Turmeric is a vibrantly colored spice traditionally used in Indian cuisine. The spice, sometimes called Indian saffron, grows naturally in southeastern Asia and has been used medicinally for centuries. Turmeric's "claim to fame" is that it contains a powerful antioxidant called curcumin.
Thanks to curcumin, turmeric has anti-inflammatory and antioxidant properties. The compound might reduce the risk of several diseases, like type 2 diabetes, heart disease, and cancer. The majority of studies have been done in animals or in vitro (human cells in Petri dishes). Few studies have been conducted in humans, so the health benefits of turmeric are still uncertain.
Turmeric spices and supplements are made from the rhizome (root) of the plant, which are ground up. Read on to learn about turmeric, including its possible health benefits, side effects, and more.
krisanapong detraphiphat / Getty Images
Shown To Improve Metabolic Syndrome
Metabolic syndrome , also called insulin resistance syndrome, is a condition that increases your risk for type 2 diabetes, stroke, and heart disease. It's characterized by having three or more risk factors, such as high blood sugar, triglycerides, and blood pressure. Other risk factors include having abdominal obesity and low HDL ("good") cholesterol. One in three adults has metabolic syndrome.
A small study published in 2016 examined the effects of supplementing with curcumin in people with metabolic syndrome. The researchers tested pro-inflammatory cytokines, or proteins associated with metabolic syndrome.
The study authors found that curcumin significantly reduced cytokines in the participants' blood samples over eight weeks. This study was small, so larger ones are needed to confirm this benefit.
Helps Alleviate Depression and Anxiety Symptoms
Depression is a common and serious mental health condition that causes feelings of sadness, low energy, low appetite, and loss of pleasure. Nearly 17% of people will experience depression in their life, usually during their late teens or early 20s.
A review published in 2020 of nine studies looked at the effects turmeric may have on depression and anxiety . The researchers found that people supplementing with turmeric saw significant improvements in symptoms. The study authors concluded that the studies were too small to base any medical decisions, and further studies are needed.
Lessens Inflammation
Inflammation is part of the immune system's defenses. The immune system sends out inflammatory cells and chemicals, like cytokines, to protect and heal your body when you encounter germs or injure yourself. Chronic inflammation means your immune system may be sending out this response all the time, even when it's not needed. This can lead to pain, fatigue, depression, weight gain, infections, acid reflux, and other issues.
A review published in 2015 of seven clinical trials looked at turmeric and oxidative stress (an imbalance between harmful chemicals and antioxidants). The researchers found that taking turmeric supplements for more than six weeks resulted in fewer oxidative stress markers and increased antioxidants.
It's worth noting that these clinical trials were small. The study authors said larger studies are needed before any benefits can be fully understood.
May Improve Kidney Disease in People with Diabetes
Renal disease, or kidney disease , affects one-third of all people with diabetes. Excess sugar in the blood can damage the kidney's blood vessels over time if diabetes goes untreated. Kidney disease usually develops slowly over the course of many years.
Research has found that supplementing with turmeric can lead to improvements in tests that measure markers of kidney damage. Turmeric might lower levels of albumin in the urine. Albumin is a muscle-building protein that's normally in your blood but shows up in urine when there's kidney damage.
May Inhibit Cancer Cells
Cancer refers to a number of diseases that all have one thing in common: cells begin to grow abnormally and spread and harm nearby body tissues. Cancer is the second leading cause of death in the United States, exceeded only by deaths due to heart disease.
Many studies have been conducted on turmeric and cancer. Most have only looked at animals or in vitro. Few studies have been conducted on humans, but some evidence suggests that curcumin nanoparticles may slow the growth of cancer cells. Curcumin may even shrink tumor size and weight. Larger studies done on humans are needed before anything can be definitively proven.
Might Improve Pain With Arthritis
Arthritis is inflammation of the joints, or where two bones meet, like your knee or elbow. There are many types of arthritis, each with its own cause and treatment. Symptoms of arthritis include joint pain, swelling, and stiffness. One in four adults in the United States has arthritis, and it's a leading cause of disability.
Turmeric is known to have anti-inflammatory properties, which can be helpful with inflammatory diseases like arthritis. Some evidence suggests that turmeric can modify pro-inflammatory cells called cytokines. This can reduce inflammation in people with osteoarthritis (OA), the most common type of arthritis. The studies that have looked at turmeric's effects on arthritis are too small to allow for a definite recommendation for its use.
How To Use
Turmeric is a spice used in cooking in many parts of the world. The spice is used in India in curries, in Japan in tea, and in the United States as a supplement and a spice. The curcumin in turmeric is also available in creams, energy drinks, cosmetics, and soap.
The body poorly absorbs curcumin unless black pepper is added to it. If you use turmeric in cooking, try adding black pepper to get the most health benefits. Turmeric is also a fat-soluble substance, so you need to eat it with a source of fat for your body to absorb it well. Pair turmeric with fat sources, such as avocado or cheese.
The Food and Drug Administration (FDA) has approved curcuminoids (anything isolated from turmeric ) and calls them "generally recognized as safe." Supplements from turmeric with 4,000–8,000 milligrams (mg) per day are recognized as safe.
Is It Safe?
Turmeric is considered safe if eaten in foods or beverages or applied to the skin. It's unknown if turmeric is safe for people who are pregnant or breastfeeding , as it has not been thoroughly studied in these groups.
Some companies are developing turmeric or curcumin products that have increased bioavailability so the body absorbs them better. It's important to remember that reformulated herbs may increase the harmful effects of the supplement.
Potential Drug Interactions
Herbs and supplements , like regular medications, are capable of causing negative interactions if taken with other drugs or supplements. Turmeric is known to decrease the body's ability to absorb certain drugs, like medications for cancer or heart disease. The spice may also increase your risk for adverse effects when taken with antidepressants , antibiotics, diabetes medications, or allergy medications.
The available evidence does not provide enough conclusions to make any hard-and-fast rule. It's important to speak with a healthcare provider before starting turmeric supplements.
Where To Find
Turmeric is a supplement available in capsule form in most grocery stores or pharmacies. The FDA does not regulate supplements to the same extent as medications.
Supplement manufacturers are responsible for testing the safety of their products. The FDA, in contrast, controls what types of claims the product labels can have. The FDA has the authority to act against any adulterated or mislabeled product after it reaches the market. Make sure you find a brand that's been third-party tested to ensure you get a pure formulation of the correct supplement.
Turmeric grows naturally in India and other parts of Southeast Asia. Many grocery stores sell fresh turmeric in their produce section and as a dried spice in the aisle with other seasonings.
Can You Take Too Much?
It's possible to take too much turmeric, but it's not likely. A study published in 2017 found that heavy doses may be toxic to cells. Other research has shown that supplements of less than 8,000 mg daily are safe. More research is needed to find the best dose of turmeric.
Turmeric is complex and difficult to study because it easily changes in your body and reaches your bloodstream. Some manufacturers have produced turmeric or curcumin products that have better absorption rates. It's important to read the label clearly and speak with a healthcare provider about the amount of turmeric you take if you choose to take it.
Turmeric Supplement Recommendations
Health 's team of experts, including registered dietitians, research and review supplements rigorously , evaluating products on several criteria, including safety, efficacy, dosage, quality of ingredients, and testing certifications. Our complete guide to turmeric supplements can be found here , but these are our two top picks.
Thorne Research Curcumin Phytosome (Meriva)
Courtesy of Amazon
This NSF Certified for Sport product contains a clinically researched form of curcumin that's well absorbed and free from artificial flavors and colors. Each two-capsule serving contains 1,000 mg of curcumin per serving.
NOW Turmeric Curcumin
These affordable capsules have a short ingredient list and are third-party tested. Each one-capsule serving contains 630 mg of curcumin per serving.
Turmeric Side Effects
Although rare, turmeric supplementation may cause side effects in some people. These side effects may include:
- Diarrhea or other gastrointestinal (GI) discomfort
A Quick Review
Turmeric shows promise as an anti-inflammatory and antioxidant. The spice has possible beneficial effects for people with cancer, metabolic syndrome, depression, arthritis, or other inflammatory conditions. More research is needed to understand turmeric's full effects on human health.
Try using turmeric as a spice in your cooking if you like the flavor. Speak with a healthcare provider, especially if you are taking other medications, if you choose to supplement with turmeric or curcumin.
Frequently Asked Questions
Turmeric is a spice in the ginger family that has a peppery taste. Some people liken the taste of turmeric to that of mustard.
Turmeric is likely safe to take daily. Just remember to not take more than 4,000–8,000 mg per day, and speak with a healthcare provider before starting turmeric supplements.
It's important to talk to a healthcare provider before starting turmeric supplements. Those with acid reflux, arrhythmia (irregular heartbeat), bleeding disorders, and gallbladder problems may need to steer clear of turmeric . The effects of the spice in pregnant and breastfeeding people is also unclear.
Hewlings SJ, Kalman DS. Curcumin: A review of its effects on human health . Foods . 2017;6(10):92. doi:10.3390/foods6100092
National Center for Complementary and Integrative Health. Turmeric .
National Heart, Lung, and Blood Institute. What is metabolic syndrome?
Panahi Y, Hosseini M, Khalili N, et al. Effects of curcumin on serum cytokine concentrations in subjects with metabolic syndrome: A post-hoc analysis of a randomized controlled trial . Biomed Pharmacother . 2016;(82)578-582. doi:10.1016/j.biopha.2016.05.037
American Psychiatric Association. What is depression?
Fusar-Poli L, Vozza L, Gabbiadini A, et al. Curcumin for depression: A meta-analysis . Crit Rev Food Sci Nutr . 2020;60(15):2643-2653. doi:10.1080/10408398.2019.1653260
Pahwa R, Goyal A, Jialal I, et al. Chronic Inflammation . In: StatPearls . StatPearls Publishing; 2024.
Sahebkar A, Serban M, Sorin U et al. Effect of curcuminoids on oxidative stress: A systematic review and meta-analysis of randomized controlled trials . J. Funct. Foods . 2015;18:898-909. doi:10.1016/j.jff.2015.01.005
National Institute of Diabetes and Digestive and Kidney Diseases. Diabetic kidney disease .
Weir MA, Walsh M, Cuerden MS, et al. The effect of micro-particle curcumin on chronic kidney disease progression: The MPAC-CKD randomized clinical trial . Nephrol Dial Transplant . 2023;38(10):2192-2200. doi:10.1093/ndt/gfad037
Centers for Disease Control and Prevention. Cancer statistics at a glance .
Ombredane A, Silva V, Andrade L. In vivo efficacy and toxicity of curcumin nanoparticles in breast cancer treatment: A systematic review . Front Oncol . 2021;11(61). doi:10.3389/fonc.2021.612903
Centers for Disease Control and Prevention. Arthritis .
Daily JW, Yang M, Park S. Efficacy of turmeric extracts and curcumin for alleviating the symptoms of joint arthritis: A systematic review and meta-analysis of randomized clinical trials . J Med Food . 2016;19(8):717-729. doi:10.1089/jmf.2016.3705
Stohs SJ, Chen O, Ray SD, et al. Highly bioavailable forms of curcumin and promising avenues for curcumin-based research and application: A review . Molecules . 2020;25(6):1397. doi:10.3390/molecules25061397
Bahramsoltani R, Rahimi R, Farzaei MH. Pharmacokinetic interactions of curcuminoids with conventional drugs: A review . J Ethnopharmacol . 2017;209:1-12. doi:10.1016/j.jep.2017.07.022
Food and Drug Administration. Dietary supplements .
Hatfield J, Saad S, Housewright C. Dietary supplements and bleeding . Proc (Bayl Univ Med Cent) . 2022;35(6):802-807. doi:10.1080/08998280.2022.2121575
Modi M, Modi K. Ginger root . In: StatPearls . StatPearls Publishing; 2024.
Related Articles
Appointments at Mayo Clinic
Kratom: unsafe and ineffective.
Users swear by kratom for mood enhancement and fatigue reduction, but safety issues and questions about its effectiveness abound.
If you read health news or visit vitamin stores, you may have heard about kratom, a supplement that is sold as an energy booster, mood enhancer, pain reliever and antidote for opioid withdrawal. However, the truth about kratom is more complicated, and the safety problems related to its use are concerning.
Kratom is an herbal extract that comes from the leaves of an evergreen tree (Mitragyna speciosa) grown in Southeast Asia. Kratom leaves can be chewed, and dry kratom can be swallowed or brewed. Kratom extract can be used to make a liquid product. The liquid form is often marketed as a treatment for muscle pain, or to suppress appetite and stop cramps and diarrhea. Kratom is also sold as a treatment for panic attacks.
Kratom is believed to act on opioid receptors. At low doses, kratom acts as a stimulant, making users feel more energetic. At higher doses, it reduces pain and may bring on euphoria. At very high doses, it acts as a sedative, making users quiet and perhaps sleepy. Some people who practice Asian traditional medicine consider kratom to be a substitute for opium.
Some people take kratom to avoid the symptoms of opioid withdrawal and because kratom may be bought more easily than prescription drugs.
Kratom is also used at music festivals and in other recreational settings. People who use kratom for relaxation report that because it is plant-based, it is natural and safe. However, the amount of active ingredient in kratom plants can vary greatly, making it difficult to gauge the effect of a given dose. Depending on what is in the plant and the health of the user, taking kratom may be very dangerous. Claims about the benefits of kratom can't be rated because reliable evidence is lacking.
Side effects and safety concerns
Although people who take kratom believe in its value, researchers who have studied kratom think its side effects and safety problems more than offset any potential benefits. Poison control centers in the United States received about 1,800 reports involving use of kratom from 2011 through 2017, including reports of death. About half of these exposures resulted in serious negative outcomes such as seizures and high blood pressure. Five of the seven infants who were reported to have been exposed to kratom went through withdrawal. Kratom has been classified as possibly unsafe when taken orally.
Kratom has a number of known side effects, including:
- Weight loss
- Chills, nausea and vomiting
- Changes in urine and constipation
- Liver damage
- Muscle pain
Kratom also affects the mind and nervous system:
- Hallucinations and delusion
- Depression and delusion
- Breathing suppression
- Seizure, coma and death
Kratom takes effect after five to 10 minutes, and its effects last two to five hours. The effects of kratom become stronger as the quantity taken increases. In animals, kratom appears to be more potent than morphine. Exposure to kratom has been reported in an infant who was breastfed by a mother taking kratom.
Many of the problems that occur with pain medications happen when these drugs are used at high doses or over a long period of time. It's not known exactly what level of kratom is toxic in people, but as with pain medications and recreational drugs, it is possible to overdose on kratom.
Research shows little promise
At one time, some researchers believed that kratom might be a safe alternative to opioids and other prescription pain medications. However, studies on the effects of kratom have identified many safety concerns and no clear benefits.
Kratom has been reported to cause abnormal brain function when taken with prescription medicines. When this happens, you may experience a severe headache, lose your ability to communicate or become confused.
In a study testing kratom as a treatment for symptoms of opioid withdrawal, people who took kratom for more than six months reported withdrawal symptoms similar to those that occur after opioid use. Too, people who use kratom may begin craving it and require treatments given for opioid addiction, such as naloxone (Narcan) and buprenorphine (Buprenex).
Kratom also adversely affects infant development. When kratom is used during pregnancy, the baby may be born with symptoms of withdrawal that require treatment.
In addition, substances that are made from kratom may be contaminated with salmonella bacteria. As of April 2018, more than 130 people in 38 states became ill with Salmonella after taking kratom. Salmonella poisoning may be fatal, and the U.S. Food and Drug Administration has linked more than 35 deaths to Salmonella-tainted kratom. Salmonella contamination has no obvious signs, so the best way to avoid becoming ill is to avoid products that may contain it.
Kratom is not currently regulated in the United States, and federal agencies are taking action to combat false claims about kratom. In the meantime, your safest option is to work with your doctor to find other treatment options.
There is a problem with information submitted for this request. Review/update the information highlighted below and resubmit the form.
From Mayo Clinic to your inbox
Sign up for free and stay up to date on research advancements, health tips, current health topics, and expertise on managing health. Click here for an email preview.
Error Email field is required
Error Include a valid email address
To provide you with the most relevant and helpful information, and understand which information is beneficial, we may combine your email and website usage information with other information we have about you. If you are a Mayo Clinic patient, this could include protected health information. If we combine this information with your protected health information, we will treat all of that information as protected health information and will only use or disclose that information as set forth in our notice of privacy practices. You may opt-out of email communications at any time by clicking on the unsubscribe link in the e-mail.
Thank you for subscribing!
You'll soon start receiving the latest Mayo Clinic health information you requested in your inbox.
Sorry something went wrong with your subscription
Please, try again in a couple of minutes
- Chien GCC, et al. Is kratom the new "legal high" on the block?: The case of an emerging opioid receptor agonist with substance abuse potential. Pain Physician. 2017;20:E195.
- Feng L, et al. New psychoactive substances of natural origin: A brief review. Journal of Food and Drug Analysis. 2017;25:461.
- Griffin III OH, et al. Do you get what you paid for? An examination of products advertised as kratom. Journal of Psychoactive Drugs. 2016;48:330.
- Drug Enforcement Administration. Kratom (Mitragyna speciosa korth). https://www.deadiversion.usdoj.gov/drug_chem_info/kratom.pdf. Accessed April 17, 2018.
- Yusoff NHM, et al. Opioid receptors mediate the acquisition, but not the expression of mitragynine-induced conditioned place preference in rats. Behavioural Brain Research. 2017;332:1.
- Diep J, et al. Kratom, an emerging drug of abuse: A case report of overdose and management of withdrawal. Anesthesia & Analgesia Case Reports. In press. Accessed May 2, 2018.
- Swogger MT, et al. Experiences of kratom users: A qualitative analysis. Journal of Psychoactive Drugs. 2015;47:360.
- Fox J, et al. Drugs of abuse and novel psychoactive substances at outdoor music festivals in Colorado. Substance Use & Misuse. In press. Accessed May 2, 2018.
- Kowalczuk AP, et al. Comprehensive methodology for identification of kratom in police laboratories. Forensic Science International. 2013;233:238.
- Fluyua D, et al. Biochemical benefits, diagnosis, and clinical risks of kratom. Frontiers in Psychiatry. 2017;8:62.
- Castillo A, et al. Posterior reversible leukoencephalopathy syndrome after kratom ingestion. Baylor University Medical Center Proceedings. 2017;30:355.
- Grundmann O. Patterns of kratom use and health impact in the US — Results from an online survey. Drug and Alcohol Dependence. 2017;176:63.
- Drago JD, et al. The harm in kratom. The Oncologist. 2017;22:1010.
- Pizarro-Osilla C. Introducing…kratom. In press. Accessed May 2, 2018.
- Kruegel AC, et al. The medicinal chemistry and neuropharmacology of kratom: A preliminary discussion of a promising medicinal plant and analysis of its potential for abuse. Neuropharmacology. In press. Accessed May 2, 2018.
- Ismail I, et al. Kratom and future treatment for the opioid addiction and chronic pain: Periculo beneficium? Current Drug Targets. In press. Accessed May 2, 2018.
- Singh D, et al. Kratom (Mitragyna speciosa) dependence, withdrawal symptoms and cravings in regular users. Drug and Alcohol Dependence. 2014;139:132.
- Swogger MT, et al. Kratom use and mental health: A systematic review. Drug and Alcohol Dependence. 2018;183:134.
- Food and Drug Administration. FDA investigates multistate outbreak of salmonella infections linked to products reported to contain kratom. https://www.fda.gov/Food/RecallsOutbreaksEmergencies/Outbreaks/ucm597265.htm. Accessed April 17, 2018.
- Food and Drug Administration. Statement from FDA Commissioner Scott Gottlieb, M.D., on FDA advisory about deadly risks associated with kratom. https://www.fda.gov/NewsEvents/Newsroom/PressAnnouncements/ucm584970.htm. Accessed April 17, 2018.
- Voelker R. Crackdown on false claims to ease opioid withdrawal symptoms. JAMA. 2018;319:857.
- Post S. Kratom exposures reported to United States poison control centers: 2011-2017. Clinical Toxicology. Published online February 20, 2019.
- Drug Enforcement Administration. Kratom—drug fact sheet. https://www.dea.gov/sites/default/files/2020-06/Kratom-2020.pdf. Accessed January 26, 2022.
- Therapeutic Research Center. Kratom. https://naturalmedicines.therapeuticresearch.com/databases/food,-herbs-supplements/professional.aspx?productid=1513. Accessed January 26, 2022.
- Umbehr G, et al. Acute liver injury following short-term use of the herbal supplement kratom. JAAPA. 2022;35:39.
- Medication-free hypertension control
- Alcohol: Does it affect blood pressure?
- Alpha blockers
- Ambien: Is dependence a concern?
- Angiotensin-converting enzyme (ACE) inhibitors
- Angiotensin II receptor blockers
- Antidepressant withdrawal: Is there such a thing?
- Antidepressants and alcohol: What's the concern?
- Antidepressants and weight gain: What causes it?
- Antidepressants: Can they stop working?
- Antidepressants for children and teens
- Antidepressants: Side effects
- Antidepressants: Selecting one that's right for you
- Antidepressants: Which cause the fewest sexual side effects?
- Anxiety: A cause of high blood pressure?
- Atypical antidepressants
- Automated external defibrillators: Do you need an AED?
- Beta blockers
- Beta blockers: Do they cause weight gain?
- Beta blockers: How do they affect exercise?
- Bipolar disorder
- Bipolar disorder and alcoholism: Are they related?
- Bipolar disorder in children: Is it possible?
- Bipolar medications and weight gain
- Bipolar treatment: I vs. II
- Blood pressure: Can it be higher in one arm?
- Blood pressure chart
- Blood pressure cuff: Does size matter?
- Blood pressure: Does it have a daily pattern?
- Blood pressure: Is it affected by cold weather?
- Blood pressure medication: Still necessary if I lose weight?
- Blood pressure medications: Can they raise my triglycerides?
- Blood pressure readings: Why higher at home?
- Blood pressure tip: Get more potassium
- Caffeine and hypertension
- Calcium channel blockers
- Calcium supplements: Do they interfere with blood pressure drugs?
- Can whole-grain foods lower blood pressure?
- Central-acting agents
- Choosing blood pressure medicines
- Clinical depression: What does that mean?
- Depression and anxiety: Can I have both?
- Depression, anxiety and exercise
- What is depression? A Mayo Clinic expert explains.
- Depression in women: Understanding the gender gap
- Depression (major depressive disorder)
- Depression: Supporting a family member or friend
- Diuretics: A cause of low potassium?
- High blood pressure and exercise
- Free blood pressure machines: Are they accurate?
- Home blood pressure monitoring
- Heart arrhythmia
- Heart Rhythm Conditions
- High blood pressure (hypertension)
- High blood pressure and cold remedies: Which are safe?
- High blood pressure and sex
- High blood pressure dangers
- How opioid use disorder occurs
- How to tell if a loved one is abusing opioids
- What is hypertension? A Mayo Clinic expert explains.
- Hypertension FAQs
- Hypertensive crisis: What are the symptoms?
- Insomnia: How do I stay asleep?
- Insomnia treatment: Cognitive behavioral therapy instead of sleeping pills
- Intervention: Help a loved one overcome addiction
- Isolated systolic hypertension: A health concern?
- Kratom for opioid withdrawal
- Lack of sleep: Can it make you sick?
- L-arginine: Does it lower blood pressure?
- Low blood pressure (hypotension)
- Male depression: Understanding the issues
- MAOIs and diet: Is it necessary to restrict tyramine?
- Marijuana and depression
- Medications and supplements that can raise your blood pressure
- Menopause and high blood pressure: What's the connection?
- Mental health: Overcoming the stigma of mental illness
- Mental health providers: Tips on finding one
- Mental illness
- Monoamine oxidase inhibitors (MAOIs)
- Natural remedies for depression: Are they effective?
- Nervous breakdown: What does it mean?
- Opioid stewardship: What is it?
- Pain and depression: Is there a link?
- Prescription drug abuse
- Prescription sleeping pills: What's right for you?
- Pulse pressure: An indicator of heart health?
- Reactive attachment disorder
- Resperate: Can it help reduce blood pressure?
- Selective serotonin reuptake inhibitors (SSRIs)
- Serotonin and norepinephrine reuptake inhibitors (SNRIs)
- Sleep deprivation: A cause of high blood pressure?
- Stress and high blood pressure
- Tapering off opioids: When and how
- Teen depression
- Teen drug abuse
- Nutrition and pain
- Pain rehabilitation
- Self-care approaches to treating pain
- Treatment-resistant depression
- Tricyclic antidepressants and tetracyclic antidepressants
- Unexplained weight loss
- Valerian: A safe and effective herbal sleep aid?
- Vasodilators
- How to measure blood pressure using a manual monitor
- How to measure blood pressure using an automatic monitor
- What is blood pressure?
- Vitamin B-12 and depression
- Can a lack of vitamin D cause high blood pressure?
- What are opioids and why are they dangerous?
- White coat hypertension
- Wrist blood pressure monitors: Are they accurate?
- Mayo Clinic Minute: Do not share pain medication
- Mayo Clinic Minute: Avoid opioids for chronic pain
- Mayo Clinic Minute: Be careful not to pop pain pills
Mayo Clinic does not endorse companies or products. Advertising revenue supports our not-for-profit mission.
- Opportunities
Mayo Clinic Press
Check out these best-sellers and special offers on books and newsletters from Mayo Clinic Press .
- Mayo Clinic on Incontinence - Mayo Clinic Press Mayo Clinic on Incontinence
- The Essential Diabetes Book - Mayo Clinic Press The Essential Diabetes Book
- Mayo Clinic on Hearing and Balance - Mayo Clinic Press Mayo Clinic on Hearing and Balance
- FREE Mayo Clinic Diet Assessment - Mayo Clinic Press FREE Mayo Clinic Diet Assessment
- Mayo Clinic Health Letter - FREE book - Mayo Clinic Press Mayo Clinic Health Letter - FREE book
- Kratom - unsafe and ineffective
Your gift holds great power – donate today!
Make your tax-deductible gift and be a part of the cutting-edge research and care that's changing medicine.
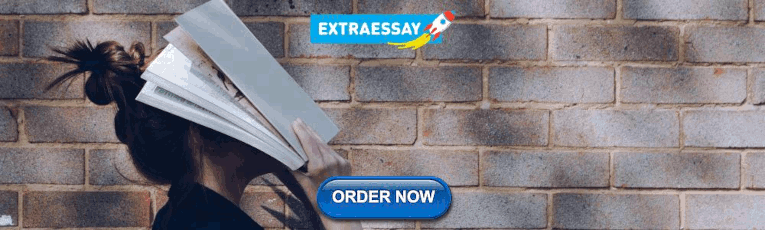
IMAGES
COMMENTS
Because greenhouse gases and air pollutants stem from the same sources of fuel combustion (), reducing fossil fuel and biofuel burning will achieve multiple improvements in public health.Table 1 shows some of the main sources of emissions for key air pollutants, which also emit carbon dioxide. These include anthropogenic fuel combustion for transportation, electricity generation, and ...
Health effects are also closely dependent on country, area, season, and time. An extended exposure duration to the pollutant should incline to long-term health effects in relation also to the above factors. ... At this point, international cooperation in terms of research, development, administration policy, monitoring, and politics is vital ...
Research on Health Effects from Air Pollution. Decades of research have shown that air pollutants such as ozone and particulate matter (PM) increase the amount and seriousness of lung and heart disease and other health problems. More investigation is needed to further understand the role poor air quality plays in causing detrimental effects to ...
Clinical noise research should further extend the evidence base of exposure-mediated mental health effects and also investigate non-pharmacological mitigation strategies (e.g. coping mechanisms ...
The Lancet Commission on pollution and health reported that pollution was responsible for 9 million premature deaths in 2015, making it the world's largest environmental risk factor for disease and premature death. We have now updated this estimate using data from the Global Burden of Diseases, Injuriaes, and Risk Factors Study 2019. We find that pollution remains responsible for approximately ...
The climate-health link has been the target of more research in recent years and it is also receiving increasing attention from the public and in both public health and climate communication literature. 2 39-41 However, the health framing of climate change information is still underused in climate communications, and researchers suggest we ...
A subset of PM, fine particulate matter (PM 2.5) is 30 times thinner than a human hair. It can be inhaled deeply into lung tissue and contribute to serious health problems. PM 2.5 accounts for most health effects due to air pollution in the U.S. Volatile organic compounds (VOC) vaporize at or near room temperature—hence, the designation ...
Climate change is directly contributing to humanitarian emergencies from heatwaves, wildfires, floods, tropical storms and hurricanes and they are increasing in scale, frequency and intensity. Research shows that 3.6 billion people already live in areas highly susceptible to climate change. Between 2030 and 2050, climate change is expected to ...
The cumulative effect of loss of livelihood, displacement, disrupted social cohesion and uncertainty from climate change can also result in longer-term mental health disorders 17. Research is ...
We identified three outcomes with a 4-star association with smoking: COPD (72% increase in risk based on the BPRF, 0.54 ROS), lower respiratory tract infection (54%, 0.43) and pancreatic cancer ...
Human Health Impacts of Climate Change. Climate change impacts human health in both direct and indirect ways 1 , 2 . Extreme heat waves, rising sea level, changes in precipitation resulting in flooding and droughts, and intense hurricanes can directly cause injury, illness, and even death 3 . The effects of climate change can also indirectly ...
As the world gets hotter and more crowded, our engines continue to pump out dirty emissions, and half the world has no access to clean fuels or technologies (e.g. stoves, lamps), the very air we breathe is growing dangerously polluted: nine out of ten people now breathe polluted air, which kills 7 million people every year. The health effects of air pollution are serious - one third of ...
Climate Effects on Health. Climate change, together with other natural and human-made health stressors, influences human health and disease in numerous ways. Some existing health threats will intensify and new health threats will emerge. Not everyone is equally at risk. Important considerations include age, economic resources, and location.
The results of each HEI-funded project undergo peer-review by outside scientists and the Health Review Committee. The HEI Research Reports contain the Investigator's Report and the Review Committee's evaluation of the study, summarized in a Commentary or short Critique. ISSN 1041-5505 (print) ISSN 2688-6855 (online)
Methods and findings. Two independent investigators systematically searched the Medical Literature Analysis and Retrieval System Online (MEDLINE), the Excerpta Medica Database (EMBASE), the Cumulative Index to Nursing and Allied Health Literature (CINAHL+), the Health Management Information Consortium, and the Journal of Research Evaluation from inception until May 2017 for publications that ...
Further research into individual effects of carbohydrate forms in diverse populations is needed to understand the complex relationship between sugar and health. ... (project number 50182) (S.E.B.), the National Health and Medical Research Council (NHMRC; project number GNT1146417) (S.E.B.), and the Australian Research Council's Discovery ...
Research is also underway to better understand the health effects associated with low levels of exposure to PFAS over long periods of time, especially in children. What We Know about Health Effects. Current peer-reviewed scientific studies have shown that exposure to certain levels of PFAS may lead to: Reproductive effects such as decreased ...
HEI's research program has addressed many important questions about the health effects of a variety of ambient air pollutants over the past two decades. These include carbon monoxide, ozone, particulate matter, and nitrogen oxides, which are regulated in the United States by the National Ambient Air Quality Standards. In addition, many air toxics and fuel additives have been studied, including ...
Health Effects. Smoking leads to disease and disability and harms nearly every organ of the body. More than 16 million Americans are living with a disease caused by smoking. For every person who dies because of smoking, at least 30 people live with a serious smoking-related illness. Smoking causes cancer, heart disease, stroke, lung diseases ...
It is important to more fully understand the human health effects associated with short- and long-term exposures to smoke from wildfires as well as prescribed fires, referred together as wildland fires. Research is being conducted to advance understanding of the health effects from different types of fires as well as combustion phases.
National Institutes of Health (NIH)
Based on the type, timing and severity of the applied stimulus, stress can exert various actions on the body ranging from alterations in homeostasis to life-threatening effects and death. In many cases, the pathophysiological complications of disease arise from stress and the subjects exposed to stress, e.g. those that work or live in stressful ...
But in federal databases, thousands of Americans have reported that Covid vaccines caused ringing in the ears, dizziness, brain fog, sharp fluctuations in blood pressure and heart rate, new or ...
The research team at RCSI University of Medicine and Health Sciences, Dublin, conclude there is a "potential public health threat facing the 4.5 million vapers in the UK" and an urgent need ...
Extensive research has been conducted on the immunomodulatory effects of bifidobacteria-derived EPSs, with emerging engineering strategies aimed at enhancing their immune-modulating capabilities. Understanding the structure, physicochemical properties, and biological activities of these compounds is crucial for their effective utilization ...
Future research on the health effects of replacement PFAS and mechanistic studies on legacy PFAS must apply "lessons learned" such as those highlighted in the present review. There are only a handful of PFAS with enough health effects data for use in decision-making, as evidenced by state-led standard setting. ...
The study, published in Scientific Reports, highlights the urgent need for public health policies concerning flavoured vapes. The research team in RCSI's Department of Chemistry used artificial intelligence (AI) to simulate the effects of heating e-liquid flavour chemicals found in nicotine vapes. They included all 180 known e-liquid flavour ...
Other research has shown that supplements of less than 8,000 mg daily are safe. ... More research is needed to understand turmeric's full effects on human health.
The health effects of radiation can be divided into two, and show subtly different relationships between dose and effect. Early, deterministic or tissue effects are seen at high doses (>1 Sv), associated with cell killing in the tissues exposed, and show a direct correlation with dose. We are used to seeing these effects in cancer patients ...
Five of the seven infants who were reported to have been exposed to kratom went through withdrawal. Kratom has been classified as possibly unsafe when taken orally. Kratom has a number of known side effects, including: Weight loss. Dry mouth. Chills, nausea and vomiting. Changes in urine and constipation. Liver damage.