- Advanced search
- Peer review

Discover relevant research today

Advance your research field in the open

Reach new audiences and maximize your readership
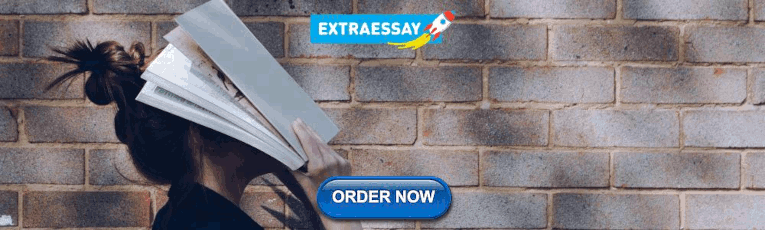
ScienceOpen puts your research in the context of
Publications
For Publishers
ScienceOpen offers content hosting, context building and marketing services for publishers. See our tailored offerings
- For academic publishers to promote journals and interdisciplinary collections
- For open access journals to host journal content in an interactive environment
- For university library publishing to develop new open access paradigms for their scholars
- For scholarly societies to promote content with interactive features
For Institutions
ScienceOpen offers state-of-the-art technology and a range of solutions and services
- For faculties and research groups to promote and share your work
- For research institutes to build up your own branding for OA publications
- For funders to develop new open access publishing paradigms
- For university libraries to create an independent OA publishing environment
For Researchers
Make an impact and build your research profile in the open with ScienceOpen
- Search and discover relevant research in over 93 million Open Access articles and article records
- Share your expertise and get credit by publicly reviewing any article
- Publish your poster or preprint and track usage and impact with article- and author-level metrics
- Create a topical Collection to advance your research field
Create a Journal powered by ScienceOpen
Launching a new open access journal or an open access press? ScienceOpen now provides full end-to-end open access publishing solutions â embedded within our smart interactive discovery environment. A modular approach allows open access publishers to pick and choose among a range of services and design the platform that fits their goals and budget.
Continue reading âCreate a Journal powered by ScienceOpenâ
What can a Researcher do on ScienceOpen?
ScienceOpen provides researchers with a wide range of tools to support their research â all for free. Here is a short checklist to make sure you are getting the most of the technological infrastructure and content that we have to offer. What can a researcher do on ScienceOpen? Continue reading âWhat can a Researcher do on ScienceOpen?â
ScienceOpen on the Road
Upcoming events.
- 20 â 22 February â ResearcherToReader Conferece
Past Events
- 09 November â Webinar for the Discoverability of African Research
- 26 â 27 October â Attending the Workshop on Open Citations and Open Scholarly Metadata
- 18 â 22 October â ScienceOpen at Frankfurt Book Fair.
- 27 â 29 September â Attending OA Tage, Berlin .
- 25 â 27 September â ScienceOpen at Open Science Fair
- 19 â 21 September â OASPA 2023 Annual Conference .
- 22 â 24 May â ScienceOpen sponsoring Pint of Science, Berlin.
- 16-17 May â ScienceOpen at 3rd AEUP Conference.
- 20 â 21 April â ScienceOpen attending Scaling Small: Community-Owned Futures for Open Access Books .
- 18 â 20 April â ScienceOpen at the London Book Fair .
What is ScienceOpen?
- Smart search and discovery within an interactive interface
- Researcher promotion and ORCID integration
- Open evaluation with article reviews and Collections
- Business model based on providing services to publishers
Live Twitter stream
Some of our partners:.

When you choose to publish with PLOS, your research makes an impact. Make your work accessible to all, without restrictions, and accelerate scientific discovery with options like preprints and published peer review that make your work more Open.
- PLOS Biology
- PLOS Climate
- PLOS Complex Systems
- PLOS Computational Biology
- PLOS Digital Health
- PLOS Genetics
- PLOS Global Public Health
- PLOS Medicine
- PLOS Mental Health
- PLOS Neglected Tropical Diseases
- PLOS Pathogens
- PLOS Sustainability and Transformation
- PLOS Collections
Breaking boundaries. Empowering researchers. Opening Science.
PLOS is a nonprofit, Open Access publisher empowering researchers to accelerate progress in science and medicine by leading a transformation in research communication.
Every country. Every career stage. Every area of science. Hundreds of thousands of researchers choose PLOS to share and discuss their work. Together, we collaborate to make science, and the process of publishing science, fair, equitable, and accessible for the whole community.
FEATURED COMMUNITIES
- Molecular Biology
- Microbiology
- Neuroscience
- Cancer Treatment and Research
RECENT ANNOUNCEMENTS
Written by Lindsay Morton Over 4 years: 74k+ eligible articles. Nearly 85k signed reviews. More than 30k published peer review history…
The latest quarterly update to the Open Science Indicators (OSIs) dataset was released in December, marking the one year anniversary of OSIs…
PLOS JOURNALS
PLOS publishes a suite of influential Open Access journals across all areas of science and medicine. Rigorously reported, peer reviewed and immediately available without restrictions, promoting the widest readership and impact possible. We encourage you to consider the scope of each journal before submission, as journals are editorially independent and specialized in their publication criteria and breadth of content.
PLOS Biology PLOS Climate PLOS Computational Biology PLOS Digital Health PLOS Genetics PLOS Global Public Health PLOS Medicine PLOS Neglected Tropical Diseases PLOS ONE PLOS Pathogens PLOS Sustainability and Transformation PLOS Water
Now open for submissions:
PLOS Complex Systems PLOS Mental Health
ADVANCING OPEN SCIENCE
Open opportunities for your community to see, cite, share, and build on your research. PLOS gives you more control over how and when your work becomes available.
Ready, set, share your preprint. Authors of most PLOS journals can now opt-in at submission to have PLOS post their manuscript as a preprint to bioRxiv or medRxiv.
All PLOS journals offer authors the opportunity to increase the transparency of the evaluation process by publishing their peer review history.
We have everything you need to amplify your reviews, increase the visibility of your work through PLOS, and join the movement to advance Open Science.
FEATURED RESOURCES
Ready to submit your manuscript to PLOS? Find everything you need to choose the journal that’s right for you as well as information about publication fees, metrics, and other FAQs here.
We have everything you need to write your first review, increase the visibility of your work through PLOS, and join the movement to advance Open Science.
Transform your research with PLOS. Submit your manuscript

The Trusted Solution for Open Access Publishing

Fully Open Access Topical Journals
IEEE offers over 30 technically focused gold fully open access journals spanning a wide range of fields.

Hybrid Open Access Journals
IEEE offers 180+ hybrid journals that support open access, including many of the top-cited titles in the field. These titles have Transformative Status under Plan S.

IEEE Access
The multidisciplinary, gold fully open access journal of the IEEE, publishing high quality research across all of IEEEâs fields of interest.
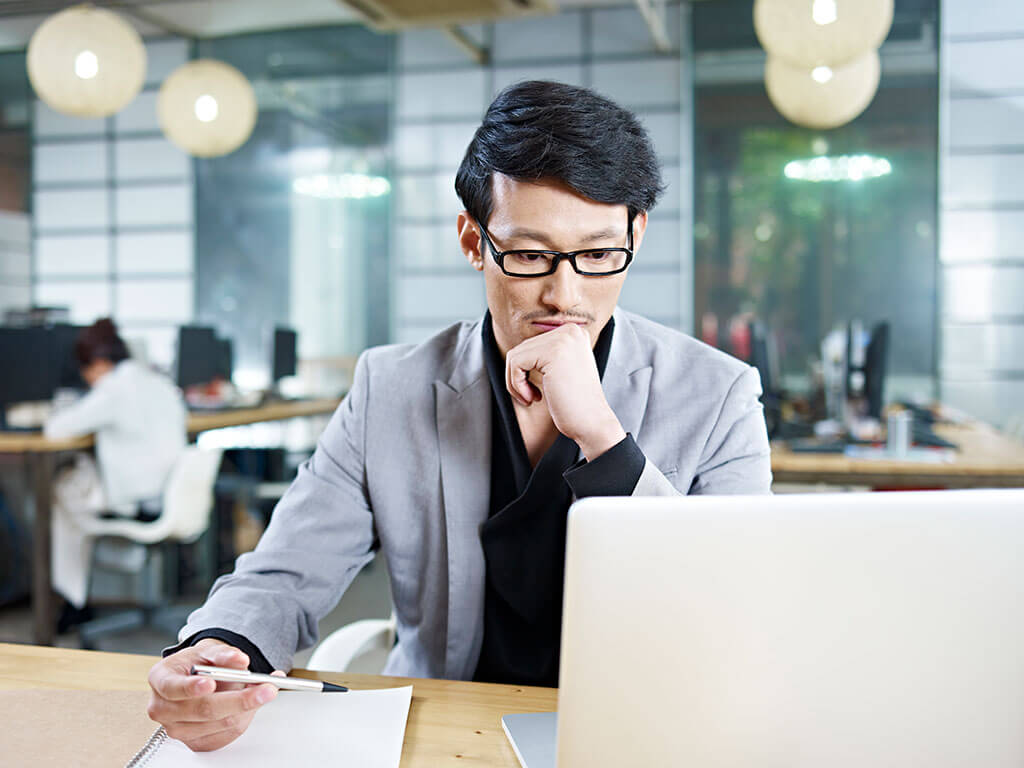
About IEEE Open
Many authors in todayâs publishing environment want to make access to research freely available to all reader communities. To help authors gain maximum exposure for their groundbreaking research, IEEE provides a variety of open access options to meet the needs of authors and institutions.
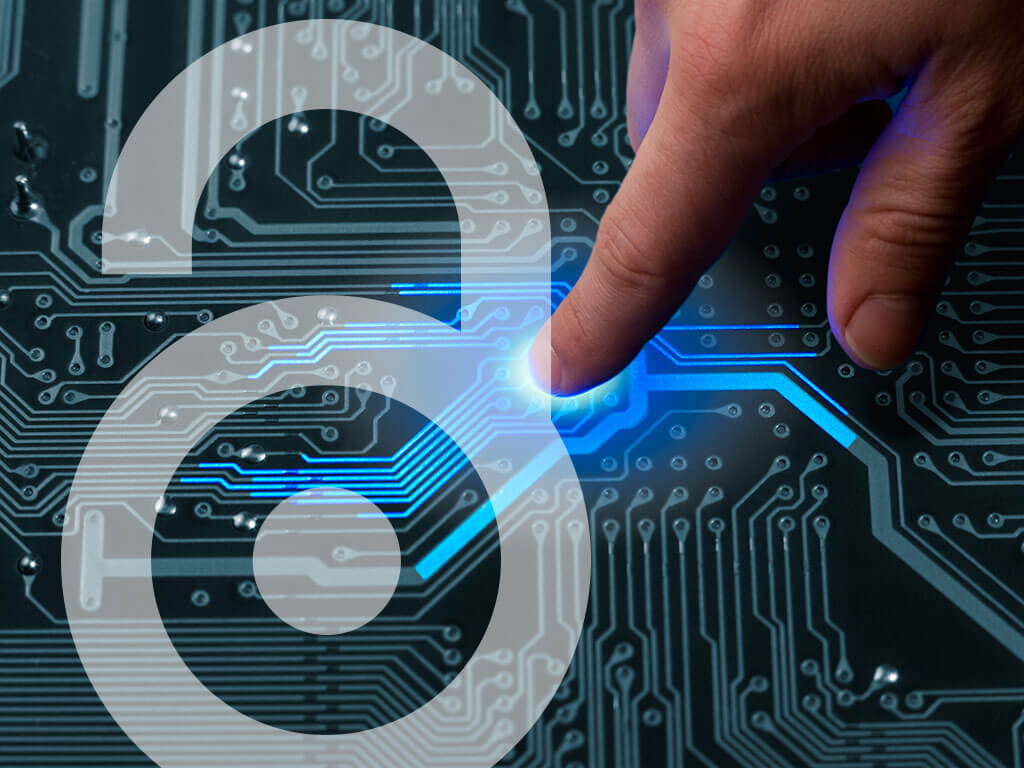
Call for Papers
Browse our fully open access topical journals and submit a paper.
News & Events
IEEE Announces 6 New Fully Open Access Journals and 3 Hybrid Journals Coming in 2024
IEEE Commits its Entire Hybrid Journal Portfolio to Transformative Journal Status Aligned with Plan S
IEEE and CRUI Sign Three-Year Transformative Agreement to Accelerate Open Access Publishing in Italy
New IEEE Open Access Journals Receive First Impact Factors
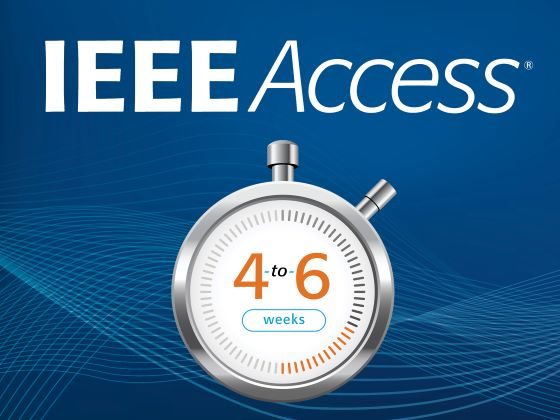
IEEE Access, a Multidisciplinary, Open Access Journal
IEEE Access is a multidisciplinary, online-only, gold fully open access journal, continuously presenting the results of original research or development across all IEEE fields of interest. Supported by article processing charges (APCs), its hallmarks are rapid peer review, a submission-to-publication time of 4 to 6 weeks, and articles that are freely available to all readers.
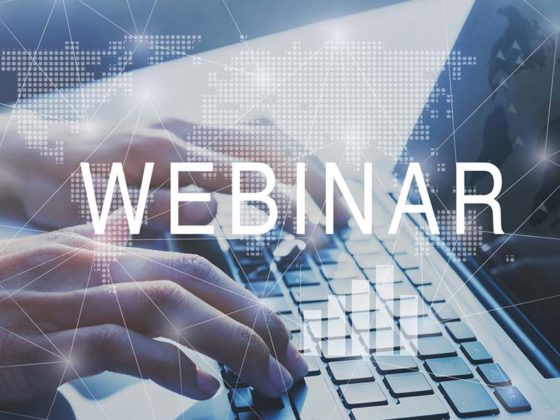
Now On-Demand
How to publish open access with ieee.
This newly published on-demand webinar will provide authors with best practices in preparing a manuscript, navigating the journal submission process, and important tips to help an author get published. It will also review the opportunities authors and academic institutions have to enhance the visibility and impact of their research by publishing in the many open access options available from IEEE.
Register Now
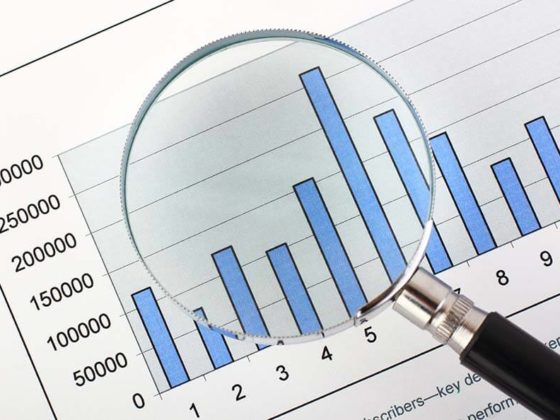
IEEE Publications Dominate Latest Citation Rankings
Each year, the Journal Citation ReportsÂź (JCR) from Web of Science Group examines the influence and impact of scholarly research journals. JCR reveals the relationship between citing and cited journals, offering a systematic, objective means to evaluate the worldâs leading journals. The 2022 JCR study, released in June 2023, reveals that IEEE journals continue to maintain rankings at the top of their fields.
A researcherâs complete guide to open access papers
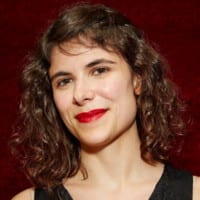
Mathilde Darbier
Marketing Communications Manager
Open access is one of the most effective ways of ensuring your findings can be read and built upon by a broad audience. Sharing your papers and data without restrictions can help to build a better research culture, and lead to faster, more advanced outcomes for the global challenges we face today.
Open access isnât an easy concept to grasp, however. In this blog, we provide you with a full overview of the various aspects of open access. We also cover the tools designed to help you discover freely-accessible papers and journals, including the Web of Science âą and Journal Citation Reports âą.
- what open access is and how it developed
- the advantages of open access resources
- what to look out for when publishing open access papers
- the different types of open access available
- the costs involved in open access
- where you can find open access journals and papers
Looking for open access articles? Watch our video to quickly find and focus your search in the Web of Science.
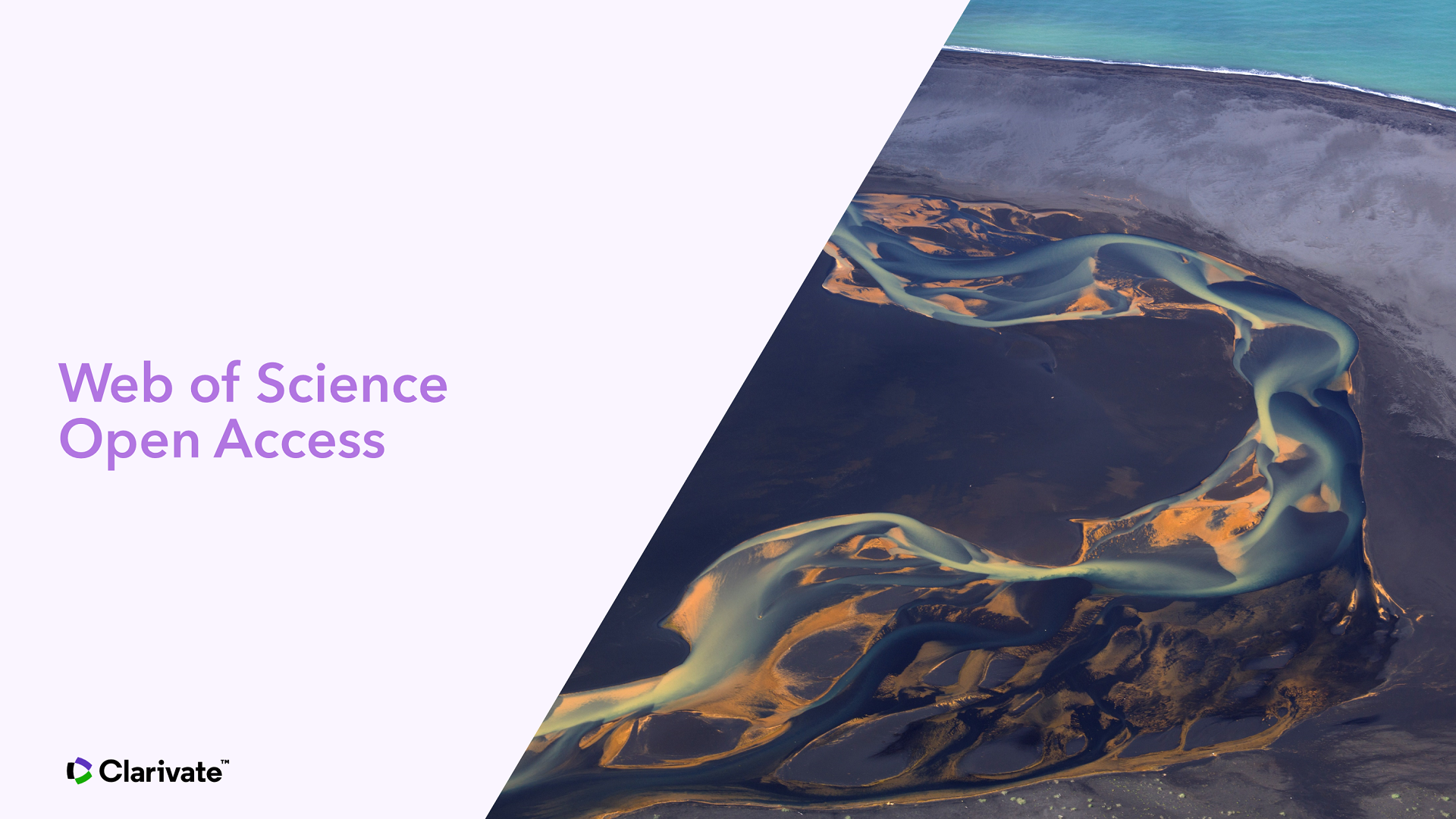
What is open access and how did it develop?
Open access (OA) is the name for free, digital, full-text scientific and academic material made available online. As defined by Creative Commons, open access papers are âdigital, online, free of charge, and free of most copyright and licensing restrictions.â 1 The 1990s saw the beginning of the open access movement brought on by the widespread availability of the World Wide Web, although researchers in physics and computer science had been self-archiving work on the internet long before this method of publication was officially named open access. Self-archiving articles into an online depository helped researchers share their papers more widely, optimizing access and maximizing its subsequent impact.
What are the advantages of making papers open access?
One of the greatest benefits of making your material open access is that you can disseminate your research more rapidly and to a broader audience. Your work will be available to a wider set of researchers, including to researchers and students from a diverse setting, helping them advance their work more quickly and enrich their learning without restriction 2 . This widespread distribution helps share new ideas, stimulate new studies, and greatly improves research and discovery in a vast number of academic disciplines. It may also increase your chances of more citations and impact. 2
Benefit from open access data in the Web of Science and Journal Citation Reports
The Web of Science is one of the most trusted solutions for researchers to discover open access publications. Our publisher-neutral approach means that you can quickly find papers that are not only free to read, but also from reputable sources worth your time and attention.
Using the Web of Science, you can access more than 14 million peer-reviewed open access papers. 32% of 2015 to 2019 Web of Science Core Collectionâą records point to open access content.
Watch this video or read our blog to learn more about how to discover open access content on the Web of Science. This also extends to the Journal Citation Reports, where we included new open access publication data in early 2020 ( find out more ). This helps the research community better understand the contribution of gold open access content to the literature and its influence on scholarly discourse.
The different types of open access
There are no single, agreed-upon definitions of open access types. However, there are five relatively common types of open access worth knowing about, regardless of whether theyâre âofficiallyâ accepted:
These different types of open access describe various ways to make academic work freely available online. We discuss these in more detail below (click any of the above links to skip to this section). First, hereâs a short summary about Creative Commons Licences.
Creative Commons Licences
Open access papers sometimes have lenient copyright and licensing restrictions depending on the open access route they have been published through, allowing anyone on the internet to read, download, copy and distribute material within reasonable use.
Derivative work can also be produced using some open access papers, providing the original author is credited. Creative Commons licences help you share scholarly material legally online with standardized copyright licences. Below is a brief explanation of the different Creative Commons licences available.
With Creative Commons licences covered, what are the differences between open access types?
Green open access
Green open access makes the author responsible for making an article freely available and archiving it, whether it is archived by sharing it through an institutionâs repository, a personal website or another public archive.
Some versions of Green OA papers may not have been copyedited, but may have been peer reviewed:
- Pre-publication Green refers to the version of your work before it has been submitted to a journal, and is sometimes called the pre-print version.
- Post-publication Green refers to the final draft of your work that has been accepted for publication by a journal, before it has been copyedited, typeset and proofread. It is also referred to as the post-print version.
The publisher will keep a copy of the full, peer reviewed version of your work, which is called the Version of Record (VOR) and readers can access these reviewed, full-text versions of the paper for a fee. This version is not Green open access, but alternative versions such as the pre-publication and post-publication version can be accessed under Green open access. The rights for reuse may be limited with Green open access, and access to Green OA papers may be limited by a publisher embargo period. An embargo period is when access to scholarly articles is not open to readers who have not paid for access. Different journals may have different embargo periods, so it is important to find out if the journal you have chosen to publish with will apply one to your work.
Bronze open access No open access fee is paid for Bronze open access, with the publisher choosing to make material freely available online. 5 Publishers are entitled to revoke open access rights to Bronze materials at any time, leading some to debate whether this is in line with true open access criteria.
Gold open access
Gold open access means the publisher is responsible for making the published academic material freely available online. Gold open access papers mean that the Version of Record is published and made freely available online. A Creative Commons licence will be applied to Gold open access papers in most cases. The Version of Record will be the final, peer reviewed paper.
Gold OA will not charge readers to access a paper, instead often charging an article processing charge (APC) to cover the publishing and distribution costs, for which the author isnât always responsible. An institution or funder may pay the APC. A key benefit of Gold open access publishing is that as the author, you will retain copyright over your work under a Creative Commons licence. The full, unrestricted reuse of published work, providing the original author is cited, is allowed with Gold OA.
Platinum and Diamond open access In the Platinum and Diamond open access models, authors, institutions, and funders do not pay open access fees, and material is made free to read online. The publisher will pay any fees applied during the publication process. Platinum and Diamond open access models are popular with university presses that account for publishing costs in their budgets.
Hybrid open access
Hybrid open access is a mixed model where journals publish both Hybrid and subscription content. It allows authors to pay an article publication charge and publish specific work as Gold open access papers.
As an author, you can benefit from Hybrid open access because it allows you to publish with trusted journals. Authors often are more concerned about which journal is best to publish with than which business model (i.e. subscription or open access) journals use.
This can help a journal transition to operating on an open access business model as it will increase the amount of open access content its community is publishing.
Despite these advantages, Hybrid open access is not without its critics. Some take issue with the practice of so-called âdouble dippingâ, where publishers charge institutions twice for the same content: authors who make their papers available as OA, and libraries who subscribe to the journal. With a number of charges applied to the publication process, itâs important to know what fees apply to making your work open access, and who is responsible for paying them.
What are the costs involved with open access?
There are a huge number of journals to submit academic work to, and it can be hard to know which journal is right for your work.
If you are the author of a paper, you may have to pay a fee to publish your work, or your research funder or institution may pay the fees in part or in full for you. A 2011 report showed that open access publication fees were only paid with personal funding in 12% Â of cases, with funders paying in 59% of cases, and universities in 24% of cases. 6
APCs, also known as publication fees, are applied by many open access journals to account for peer reviewing and editorial costs, and to make material available in both open access journals or hybrid journals. There are still journals that do not apply article processing charges, but these charges are the most common way journals generate their income.
Luckily, as an author you may not always have to pay the full fees when publishing your work. For instance, some libraries offer deals to publishers, charging reduced rate fees if they publish your work in specific open access journals. This means you may be able to save money on article processing charges when submitting your papers to these peer reviewed journals.
In some cases, charges may be lifted due to financial hardship or due to the economic status of an authorâs geographic location. If you do not have funding for APCs, ask the journalâs editorial team for their waiver policy. We also recommend checking whether the Directory of Open Access Journals (DOAJ) lists the journal you would like to be published in. Make sure you also read our blog to learn how products like Journal Citation Reports and the Master Journal List âąÂ help you find the right open access journal for your research in the fastest possible time. You can also watch our on demand webinar on the same topic.
Where can I find open access journals, papers and data?
There are a number of online tools that can help you source OA journals and papers, and below are just a few.
- The Web of Science allows you to discover world-class research literature from specially selected, high-quality journals, and users can easily access millions of peer-reviewed open access articles. You can also use Kopernio , a free browser plugin featured in the Web of Science to get one click access to your PDF faster using open access alternatives when the PDF you are looking for is not available via your existing institutional subscription. Watch our video to learn more .
- Master Journal List Manuscript Matcher is the ultimate place to begin your search for journals. It is a free tool that helps you narrow down your journal options based on your research topic and goals, with special filters for open access journals
- Journal Citation Reports is the most powerful product for journal intelligence. It uses transparent, publisher-neutral data and statistics to provide unique insights into a journalâs role, influence and the open access options available to you.
- Directory of Open Access Journals is a community-built directory that provides access to peer reviewed journals.
- PubMed Central is run by the National Institute of Health and is a full-text archive of biomedical and life sciences journals, which increases visibility of scholarly material.
- Check.Submit. is an international, cross-sector initiative offering tools and resources to help you identify trustworthy journals for your research.
- ROAD allows you to search for OA papers by name, subject or ISSN number.
If youâre looking for open access data , make sure you also check out the Web of Science Data Citation Index âą. It boasts 9.7 million datasets sourced from 380 repositories.
Open access is central to advancing discovery and improving education worldwide. It helps authors distribute their work more widely, and enables researchers like you to access quality, often peer reviewed work for free.
To ensure you can get the most out of open access publishing, donât forget to check out our video about discovering open access content on the Web of Science. If you want to better understand the open access journals available when publishing your work, this blog (and on-demand webinar) will point you to the right tools to use.
Subscribe to receive regular updates on how to research smarter
Related posts
Reimagining research impact: introducing web of science research intelligence.
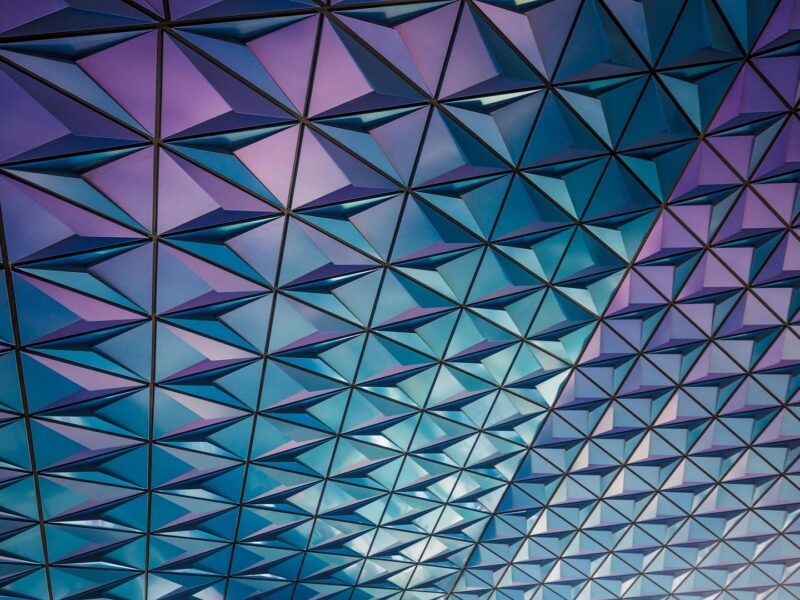
Beyond discovery: AI and the future of the Web of Science
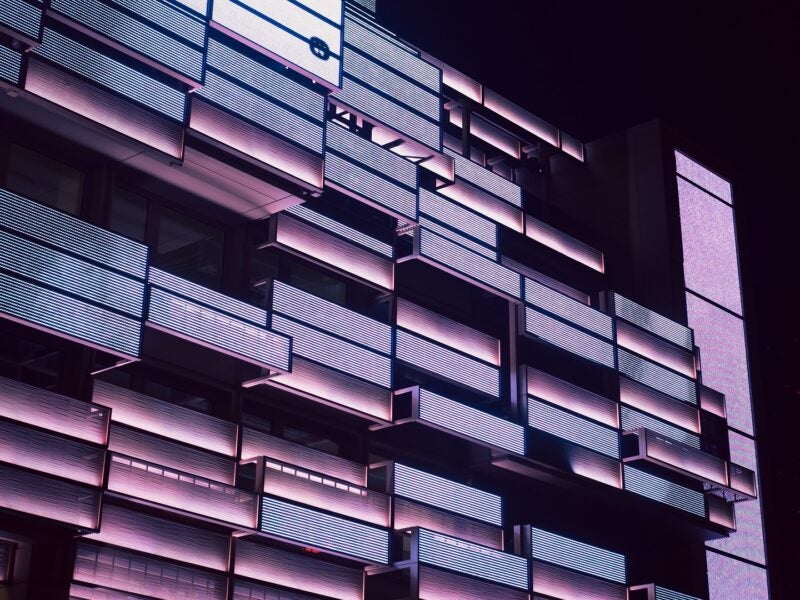
Clarivate welcomes the Barcelona Declaration on Open Research Information
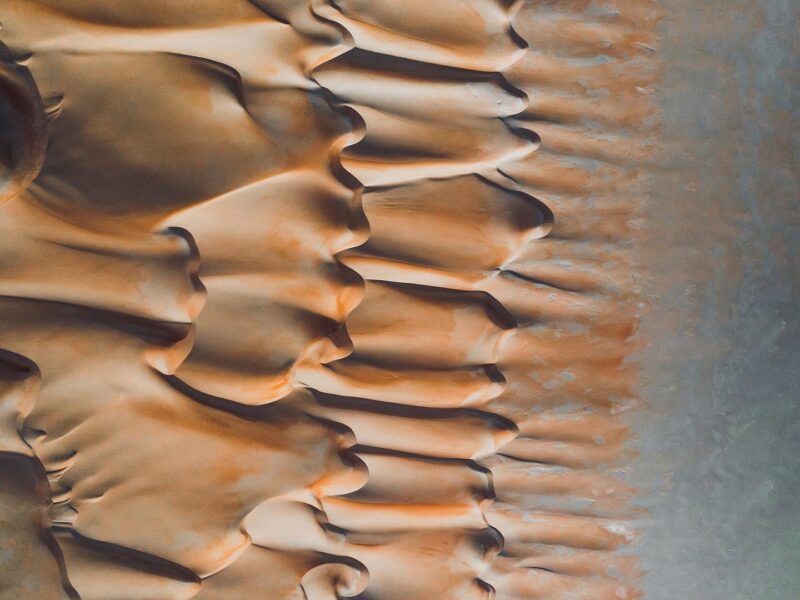
Thank you for visiting nature.com. You are using a browser version with limited support for CSS. To obtain the best experience, we recommend you use a more up to date browser (or turn off compatibility mode in Internet Explorer). In the meantime, to ensure continued support, we are displaying the site without styles and JavaScript.
- View all journals
- My Account Login
- Explore content
- About the journal
- Publish with us
- Sign up for alerts
- Open access
- Published: 07 June 2023
CORE: A Global Aggregation Service for Open Access Papers
- Petr Knoth  ORCID: orcid.org/0000-0003-1161-7359 1 ,
- Drahomira Herrmannova  ORCID: orcid.org/0000-0002-2730-1546 1  nAff2 ,
- Matteo Cancellieri 1 ,
- Lucas Anastasiou 1 ,
- Nancy Pontika 1 ,
- Samuel Pearce 1 ,
- Bikash Gyawali 1 &
- David Pride 1 Â
Scientific Data volume  10 , Article number: 366 ( 2023 ) Cite this article
7036 Accesses
76 Altmetric
Metrics details
- Research data
This paper introduces CORE, a widely used scholarly service, which provides access to the worldâs largest collection of open access research publications, acquired from a global network of repositories and journals. CORE was created with the goal of enabling text and data mining of scientific literature and thus supporting scientific discovery, but it is now used in a wide range of use cases within higher education, industry, not-for-profit organisations, as well as by the general public. Through the provided services, CORE powers innovative use cases, such as plagiarism detection, in market-leading third-party organisations. CORE has played a pivotal role in the global move towards universal open access by making scientific knowledge more easily and freely discoverable. In this paper, we describe COREâs continuously growing dataset and the motivation behind its creation, present the challenges associated with systematically gathering research papers from thousands of data providers worldwide at scale, and introduce the novel solutions that were developed to overcome these challenges. The paper then provides an in-depth discussion of the services and tools built on top of the aggregated data and finally examines several use cases that have leveraged the CORE dataset and services.
Similar content being viewed by others
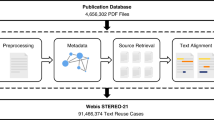
A large dataset of scientific text reuse in Open-Access publications
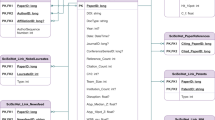
SciSciNet: A large-scale open data lake for the science of science research
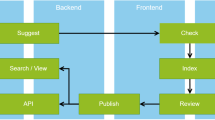
re3data â Indexing the Global Research Data Repository Landscape Since 2012
Introduction.
Scientific literature contains some of the most important information we have assembled as a species, such as how to treat diseases, solve difficult engineering problems, and answer many of the worldâs challenges we are facing today. The entire body of scientific literature is growing at an enormous rate with an annual increase of more than 5 million articles (almost 7.2 million papers were published in 2022 according to Crossref, the largest Digital Object Identifier (DOI) registration agency). Furthermore, it was estimated that the amount of research published each year increases by about 10% annually 1 . At the same time, an ever growing amount of research literature, which has been estimated to be well over 1 million publications per year in 2015 2 , is being published as open access (OA), and can therefore be read and processed with limited or no copyright restrictions. As reading this knowledge is now beyond the capacities of any human being, text mining offers the potential to not only improve the way we access and analyse this knowledge 3 , but can also lead to new scientific insights 4 .
However, systematically gathering scientific literature to enable automated methods to process it at scale is a significant problem. Scientific literature is spread across thousands of publishers, repositories, journals, and databases, which often lack common data exchange protocols and other support for inter-operability. Even when protocols are in place, the lack of infrastructure for collecting and processing this data, as well as restrictive copyrights and the fact that OA is not yet the default publishing route in most parts of the world further complicate the machine processing of scientific knowledge.
To alleviate these issues and support text and data mining of scientific literature we have developed CORE ( https://core.ac.uk/ ). CORE aggregates open access research papers from thousands of data providers from all over the world including institutional and subject repositories, open access and hybrid journals. CORE is the largest collection of OA literatureâat the time of writing this article, it provides a single point of access to scientific literature collected from over ten thousand data providers worldwide and it is constantly growing. It provides a number of ways for accessing its data for both users and machines, including a free API and a complete dump of its data.
As of January 2023, there are 4,700 registered API users and 2,880 registered dataset and more than 70 institutions have registered to use CORE Recommender in their repository systems.
The main contributions of this work are the development of COREâs continuously growing dataset and the tools and services built on top of this corpus. In this paper, we describe the motivation behind the datasetâs creation and the challenges and methods of assembling it and keeping it continuously up-to-date. Overcoming the challenges posed by creating a collection of research papers of this scale required devising innovative solutions to harvesting and resource management. Our key innovations in this area which have contributed to the improvement of the process of aggregating research literature include:
Devising methods to extend the functionality of existing widely-adopted metadata exchange protocols which were not designed for content harvesting, to enable efficient harvesting of research papersâ full texts.
Developing a novel harvesting approach (referred to here as CHARS) which allows us to continuously utilise the available compute resources while providing improved horizontal scalability, recoverability, and reliability.
Designing an efficient algorithm for scheduling updates of harvested resources which optimises the recency of our data while effectively utilising the compute resources available to us.
This paper is organised as follows. First, in the remainder of this section, we present several use cases requiring large scale text and data mining of scientific literature, and explain the challenges in obtaining data for these tasks. Next, we present the data offered by CORE and our approach for systematically gathering full text open access articles from thousands of repositories and key scientific publishers.
Terminology
In digital libraries the term record is typically used to denote a digital object such as text, image, or video. In this paper and when referring to data in CORE, we use the term metadata record to refer to the metadata of a research publication, i.e. the title, authors, abstract, project funding details, etc., and the term full text record to describe a metadata record which has an associated full text.
We use the term data provider to refer to any database or a dataset from which we harvest records. Data providers harvested by CORE include disciplinary and institutional repositories, publishers and other databases.
When talking about open access (OA) to scientific literature, we refer to the Budapest Open Access Initiative (BOAI) definition which defines OA as âfree availability on the public internet, permitting any users to read, download, copy, distribute, print, search, or link to the full texts of these articles, crawl them for indexing, pass them as data to software, or use them for any other lawful purposeâ ( https://www.budapestopenaccessinitiative.org/read ). There are two routes to open access, 1) OA repositories and 2) OA journals. The first can be achieved by self-archiving (depositing) publications in repositories (green OA), and the latter by directly publishing articles in OA journals (gold OA).
Text and Data Mining of Scientific Literature
Text and data mining (TDM) is the discovery by a computer of new, previously unknown information, by automatically extracting information from different written resources ( http://bit.ly/jisc-textm ). The broad goal of TDM of scientific literature is to build tools that can retrieve useful information from digital documents, improve access to these documents, or use these documents to support scientific discovery. OA and TDM of scientific literature have one thing in commonâthey both aim to improve access to scientific knowledge for people. While OA aims to widen the availability of openly available research, TDM aims to improve our ability to discover, understand and interpret scientific knowledge.
TDM of scientific literature is being used in a growing number of applications, many of which were until recently not viable due to the difficulties associated with accessing the data from across many publishers and other data providers. Because many use cases involving text and data mining can only realise their full potential when they are executed on an as large corpus of research papers as possible, these data access difficulties have rendered many of the uses cases described below very difficult to achieve. For example, to reliably detect plagiarism in newly submitted publications it is necessary to have access to an always up-to-date dataset of published literature spanning all disciplines. Based on data needs, scientific literature TDM use cases can be broadly categorised into the following two categories, which are shown in Fig. 1 :
A priori defined sample use cases: Use cases which require access to a subset of scientific publications that can be specified prior to the execution of the use case. For example, gathering the list of all trialled treatments for a particular disease in the period 2000â2010 is a typical example of such a use case.
Undefined sample use cases: Use cases which cannot be completed using data samples that are defined a priori. The execution of such use cases might require access to data not known prior to the execution or may require access to all data available. Plagiarism detection is a typical example of such use case.
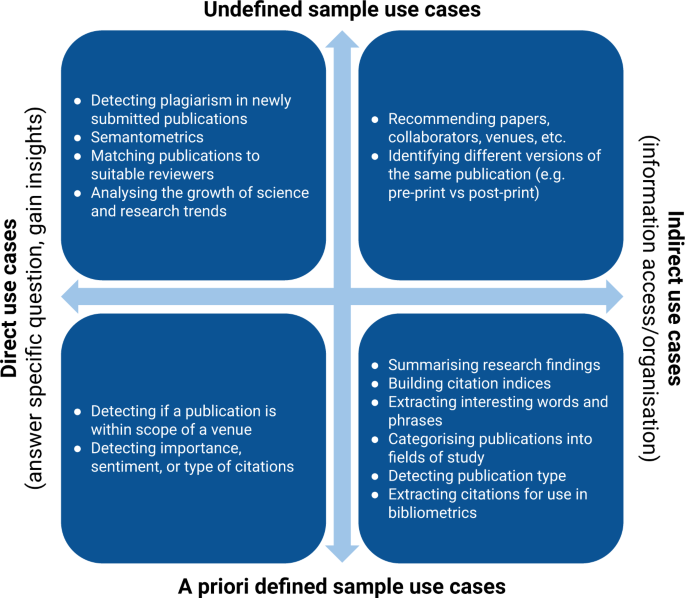
Example uses cases of text and data mining of scientific literature. Depending on data needs, TDM uses can be categorised into a) a priori defined sample use cases, and b) undefined sample use cases. Furthermore, TDM use cases can broadly be categorised into 1) indirect applications which aim to improve access to and organisation of literature and 2) direct applications which focus on answering specific questions or gaining insights.
However, there are a number of factors that significantly complicate access to data for these applications. The needed data is often spread across many publishers, repositories, and other databases, often lacking interoperability (these factors will be further discussed in the next section). Consequently, researchers and developers working in these areas typically invest a considerable amount of time in corpus collection, which could be up to 90% of the total investigation time 5 . For many, this task can even prove impossible due to technical restrictions and limitations of publisher platforms, some of which will be discussed in the next section. Consequently, there is a need for a global, continuously updated, and downloadable dataset of full text publications to enable such analysis.
Challenges in machine access to scientific literature
Probably the largest obstacle to the effective and timely retrieval of relevant research literature is that it may be stored in a wide variety of locations with little to no interoperability: repositories of individual institutions, publisher databases, conference and journal websites, pre-print databases, and other locations, each of which typically offers different means for accessing their data. While repositories often implement a standard protocol for metadata harvesting, the Open Archives Initiative Protocol for Metadata Harvesting (OAI-PMH), publishers typically allow access to their data through custom made APIs, which are not standardised and are subject to changes 6 . Other data sources may provide static data dumps in a variety of formats or not offer programmatic access to their data at all.
However, even when publication metadata can be obtained, other steps involved in the data collection process complicate the creation of a final dataset suitable for TDM applications. For example, the identification of scientific publications within all downloaded documents, matching these publications correctly to the original publication metadata, and their conversion from formats used in publishing, such as the PDF format, into a textual representation suitable for text and data mining, are just some of the additional difficulties involved in this process. The typical minimum steps involved in this process are illustrated in Fig. 2 . As there are no widely adopted solutions providing interoperability across different platforms, custom harvesting solutions need to be created for each.
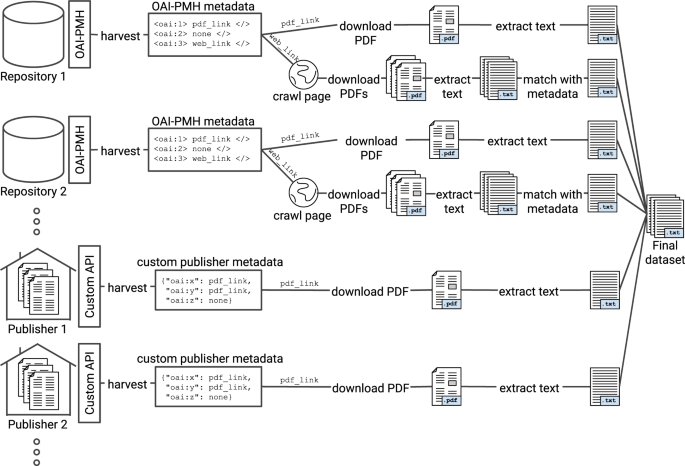
Example illustration of the data collection process. The figure depicts the typical minimum steps which are necessary to produce a dataset for TDM of scientific literature. Depending on the use case, tens or hundreds of different data sources may need to be accessed, each potentially requiring a different processâfor example accessing a different set of API methods or a different process for downloading publication full text. Furthermore, depending on the use case, additional steps may be needed, such as extraction of references, identification of duplicate items or detection of the publicationâs language. In the context of CORE, we provide the details of this process in Section Methods.
Challenges in systematically gathering open access research literature
Open access journals and repositories are increasingly becoming the central providers of open access content, in part thanks to the introduction of funder and institutional open access policies 7 . Open access repositories include institutional repositories such as the University of Cambridge Repository https://www.repository.cam.ac.uk/ , and subject repositories such arXiv https://arxiv.org/ . As of February 2023, there are 6,015 open access repositories indexed in the Directory of Open Access Repositories http://v2.sherpa.ac.uk/opendoar/ (OpenDOAR), as well as 18,935 open access journals indexed in the Directory of Open Access Journals https://doaj.org/ (DOAJ). However, open access research literature can be stored in a wide variety of other locations, including publisher and conference websites, individual researcher websites, and elsewhere. Consequently, a system for harvesting open access content needs to be able to harvest effectively from thousands of data providers. Furthermore, a large number of open access repositories (69.4% of repositories indexed in OpenDOAR as of January 2018) expose their data through the OAI-PMH protocol while often not providing any alternatives. An open access harvesting system therefore also needs to be able to effectively utilise OAI-PMH for open access content harvesting. However, these two requirementsâharvesting from thousands of data providers and utilising OAI-PMH for content harvestingâpose a number of significant scalability challenges.
Challenges related to harvesting from thousands of data providers
Open access data providers vary greatly in size, with some hosting millions of documents while others host a significantly lower number. New documents are added and old documents are often updated by data providers daily.
Different geographic locations and internet connection speeds may result in vastly differing times needed to harvest information from different providers, even when their size in terms of publication numbers is the same. As illustrated in Table 1 , there are also a variety of OAI-PMH implementations across commonly used repository platforms providing significantly different harvesting performance. To construct this table, we analysed OAI-PMH metadata harvesting performances of 1,439 repositories in CORE, covering eight different repository platforms. It should be noted that the OAI-PMH protocol only necessitates metadata to be expressed in the Dublin Core (DC) format. However, it also can be extended to express the metadata in other formats. Because the Dublin-Core standard is constrained to just 15 elements, it is not uncommon for OAI-PMH repositories to also use and extended metadata format such as Rioxx ( https://rioxx.net ) or the OpenAIRE Guidelines ( https://www.openaire.eu/openaire-guidelines-for-literature-institutional-and-thematic-repositories ).
Additionally, harvesting is limited not only by factors related to the data providers, but also by the compute resources (hardware) available to the aggregator. As many use cases listed in the Introduction, such as in plagiarism detection or systematic review automation, require access to very recent data, ensuring that the harvested data stays recent and that the compute resources are utilised efficiently both pose significant challenges.
To overcome these challenges, we designed the CORE Harvesting System (CHARS) which relies on two key principles. The first is the application of the microservices software principles to open access content harvesting 8 . The second is our strategy we denote pro-active harvesting , which means that providers are scheduled automatically according to current need. This strategy is implemented in the harvesting Scheduler (Section CHARS_architecture). The Scheduler uses a formula we designed for prioritising data providers.
The combination of the Scheduler with CHARS microservices architecture enables us to schedule harvesting according to current compute resource utilisation, thus greatly increasing our harvesting efficiency. Since switching from a fixed-schedule approach described above to pro-active harvesting, we have been able to greatly improve the data recency of our collection as well as to increase the size of the collection threefold within the span of three years.
Challenges related to the use of OAI-PMH protocol for content harvesting
As explained above, OAI-PMH is currently the standard method for exchanging data across repositories. While the OAI-PMH protocol was originally been designed for metadata harvesting only, it has been, due to its wide adoption and lack of alternatives, used as an entry point for full text harvesting. Full text harvesting is achieved by extracting URLs from the metadata records collected through OAI-PMH, the extracted URLs are then used to discover the location of the actual resource 9 . However, there are a number of limitations of the OAI-PMH protocol which make it unsuitable for large-scale content harvesting:
It directly supports only metadata harvesting, meaning additional functionality has to be implemented in order to use it for content harvesting.
The location of full text links in the OAI-PMH metadata is not standardised and the OAI-PMH metadata records typically contain multiple links. From the metadata it is not clear which of these links points to the described representation of the resource and in many cases none of them does so directly. Therefore, all possible links to the resource itself have to be extracted from the metadata and tested to identify the correct resource. Furthermore, OAI-PMH does not facilitate any validation for ensuring the discovered resource is truly the described resource. In order to overcome this issues, the adoption of the RIOXX https://rioxx.net/ metadata format or the OpenAIRE guidelines https://guidelines.openaire.eu/ has been promoted. However, the issue of unambiguously connecting metadata records and the described resource is still present.
The architecture of the OAI-PMH protocol is inherently sequential, which makes it ill-suited for harvesting from very large repositories. This is because the processing of large repositories cannot be parallelised and it is not possible to recover the harvesting in case of failures.
Scalability across different implementations of OAI-PMH differs dramatically. Our analysis (Table 1 ) shows that performance can differ significantly also when only a single repository software is considered 10 .
Other limitations include difficulties in incremental harvesting, reliability issues, metadata interoperability issues, and scalability issues 11 .
We have designed solutions to overcome a number of these issues, which have enabled us to efficiently and effectively utilise OAI-PMH to harvest open access content from repositories. We present these solutions in Section Using OAI-PMH for content harvesting. While we currently rely on a variety of solutions and workarounds to enable content harvesting through OAI-PMH, most of the limitations listed in this section could also be addressed by adopting more sophisticated data exchange protocols, such as the ResourceSync ( http://www.openarchives.org/rs/1.1/resourcesync ) protocol which was designed with content harvesting in mind 10 and the adoption in the systems of data providers we support.
Our solution
In the above sections we have highlighted a critical need for many researchers and organisations globally for large-scale always up-to-date seamless machine access to scientific literature originating from thousands of data providers at full text level. Providing this seamless access has become both a defining goal and a feature of CORE and has enabled other researchers to design and test innovative methods on CORE data, often powered by artificial intelligence processes. In order to put together this vast continuously updated dataset, we had to overcome a number of research challenges, such as those related to the lack of interoperability, scalability, regular content synchronisation, content redundancy and inconsistency. Our key innovation in this area is the improvement of the process of aggregating research literature , as specified in the Introduction section.
This underpinning research has allowed CORE to become a leading provider of open access papers. The amount of data made available by CORE has been growing since 2011 12 and is continuously kept up to date. As of February 2023, CORE provides access to over 291 million metadata records and 32.8 million full text open access articles, making it the worldâs largest archive of open access research papers, significantly larger than PubMed, arXiv and JSTOR datasets.
Whilst there are other publication databases that could be initially viewed as similar to CORE, such as BASE or Unpaywall, we will demonstrate the significant differences that set CORE apart and show how it provides access to a unique, harmonised corpus of Open Access literature. A major difference between these existing services is that CORE is completely free to use for the end user, it hosts full text content, and offers several methods for accessing its data for machine processing. Consequently, it removes the need to harvest and pre-process full text for text mining, since CORE provides plain text access to the full texts via its raw data services, eliminating the need for text and data miners to work on PDF formats. A detailed comparison of other publication databases is provided in the Discussion. In addition, CORE enables building powerful services on top of the collected full texts, supporting all the categories of use cases outlined in the Use cases section.
As of today, CORE provides three services for accessing its raw data: API, dataset, and a FastSync service. The CORE API provides real-time machine access to both metadata and full texts of research papers. It is intended for building applications that need reliable access to a fraction of CORE data at any time. CORE provides a RESTful API. Users can register for an API key to access the service. Full documentation and Python notebooks containing code examples can be found on the CORE documentation pages online ( https://api.core.ac.uk/docs/v3 ). The CORE Dataset can be used to download CORE data in bulk. Finally, CORE FastSync enables third party systems to keep an always up to date copy of all CORE data within their infrastructure. Content can be transferred as soon as it becomes available in CORE using a data synchronisation service on top of the ResourceSync protocol 13 optimised by us for improved synchronisation scalability with an on-demand resource dumps capability. CORE FastSync provides fast, incremental and enterprise data synchronisation.
CORE is the largest up-to-date full text open access dataset as well as one of the most widely used services worldwide supporting access to freely available research literature. CORE regularly releases data dumps licensed as ODC-By, making the data freely available for both commercial and non-commercial purposes. Access to CORE data via the API is provided freely to individuals conducting work in their own personal capacity and to public research organisations for unfunded research purposes. CORE offers licenses to commercial organisations wanting to use CORE services to obtain a convenient way of accessing CORE data with a guaranteed level of service support. CORE is operated as a not-for-profit entity by The Open University and this business model makes it possible for CORE to remain free for the >99.99% of its users.
A large number of commercial organisations have benefited from these licenses in areas as diverse as plagiarism detection in research, building specialised scholarly publication search engines, developing scientific assistants and machine translation systems and supporting education etc. https://core.ac.uk/about/endorsements/partner-projects . The CORE data servicesâCORE API and Dataset, have been used by over 7,000 experts to analyse data, develop text-mining applications and to embed CORE into existing production systems.
Additionally, more than 70 repository systems have registered to use the CORE Recommender and the service is notably used by prestigious institutions, including the University of Cambridge and by popular pre-prints services such as arXiv.org. Other CORE services are the CORE Discovery and the CORE Repository Dashboard. The first was released on July 2019 and at the time of writing it has more than 5000 users. The latter is a tool designed specifically for repository managers which provides access to a range of tools for managing the content within their repositories. The CORE Repository Dashboard is currently used by 499 users from 36 countries.
In the rest of this paper we describe the CORE dataset and the methods of assembling it and keeping it continuously up-to-date. We also present the services and tools built on top of the aggregated corpus and provide several examples of how the CORE dataset has been used to create real-world applications addressing specific use-cases.
As highlighted in the Introduction, CORE is a continuously growing dataset of scientific publications for both human and machine processing. As we will show in this section, it is a global dataset spanning all disciplines and containing publications aggregated from more than ten thousand data providers including disciplinary and institutional repositories, publishers, and other databases. To improve access to the collected publications, CORE performs a number of data enrichment steps. These include metadata and full text extraction, language and DOI detection, and linking with other databases. Furthermore, CORE provides a number of services which are built on top of the data: a publications recommender ( https://core.ac.uk/services/recommender/ ), CORE Discovery service ( https://core.ac.uk/services/discovery/ ) (a tool for discovering OA versions of scientific publications), and a dashboard for repository managers ( https://core.ac.uk/services/repository-dashboard/ ).
Dataset size
As of February 2023, CORE is the worldâs largest dataset of open access papers (comparison with other systems is provided in the Discussion). CORE hosts over 291 million metadata records including over 34 million articles with full text written in 82 languages and aggregated from over ten thousand data providers located in 150 countries. Full details of CORE Dataset size are presented in Table 2 . In the table, âMetadata recordsâ represent all valid (not retracted, deleted, or for some other reason withdrawn) records in CORE. It can be seen that about 13% of records in CORE contain full text. This number represents records for which a manuscript was successfully downloaded and converted to plain text. However, a much higher proportion of records contains links to additional freely available full text articles hosted by third-party providers. Based on analysing a subset of our data, we estimate that about 48% of metadata records in CORE fall into this category, indicating that CORE is likely to contain links to open access full texts for 139 million articles. Due to the nature of academic publishing there will be instances where multiple versions of the same paper are deposited in different repositories. For example, an early version of an article can be deposited by an author to a pre-print server such as arXiv or BiorXiv and then a later version uploaded to an institutional repository. Identifying and matching these different versions is a significant undertaking. CORE has carried out research to develop techniques based on locality sensitive hashing for duplicates identification 8 and integrated these into its ingestion pipeline to link versions of papers from across the network of OA repositories and group these under a single works entity. The large number of records in CORE translates directly into the size of the dataset in bytes as the uncompressed version of the dataset including PDFs is about 100 TB. The compressed version of the CORE dataset with plain texts only amounts to 393 GB and uncompressed to 3.5 TBs.
Recent studies have estimated that around 24%â28% of all articles are available free to read 2 , 14 . There are a number of reasons why the proportion of full text content in CORE is lower than these estimates. The main reason is likely that a significant proportion of the free to read articles represents content hosted on platform with many restrictions for machine accessibility, i.e. some repositories severely restrict or fully prohibit content harvesting 9 .
The growth of CORE has been made possible thanks to the introduction of a novel harvesting system and the creation of an efficient harvesting scheduler, both of which are described in the Methods section. The growth of metadata and full text records in CORE is shown in Fig. 3 . Finally, Fig. 4 shows age of publications in CORE.
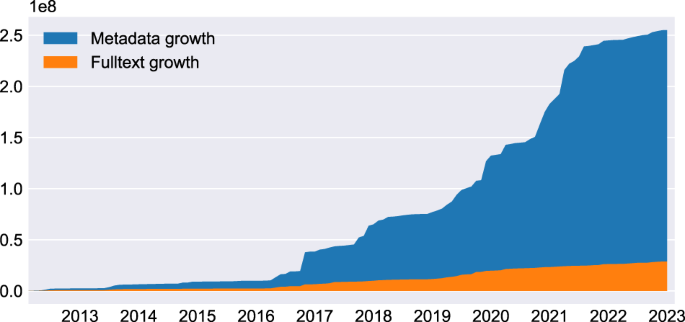
Growth of records in CORE per month since February 2012. âFull text growthâ represents growth of records containing full text, while âMetadata growthâ represents growth of records without full text, i.e. the two numbers do not overlap. The two area plots are stacked on top of each other, their sum therefore represents the total number of records in CORE.
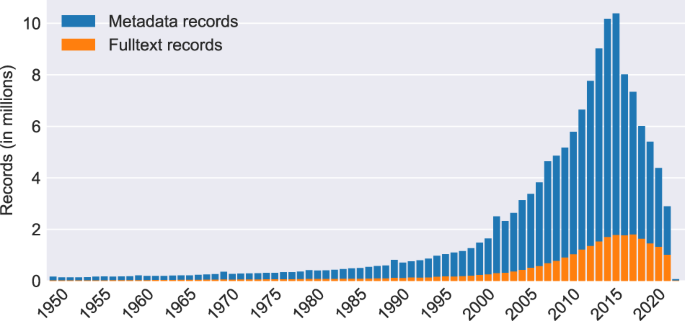
Age of publications in CORE. Similarly as in Fig. 3 , the âMetadataâ and âFull textâ records bars are stacked on top of each other.
Data sources and languages
As of February 2023, CORE was aggregating content from 10,744 data sources. These data sources include institutional repositories (for example the USC Digital Library or the University of Michigan Library Repository), academic publishers (Elsevier, Springer), open access journals (PLOS), subject repositories, including those hosting eprints (arXiv, bioRxiv, ZENODO, PubMed Central) and aggregators (e.g. DOAJ). The ten largest data sources in CORE are shown in Table 3 . To calculate the total number of data providers in CORE, we consider aggregators and publishers as one data source despite each aggregating data from multiple sources. A full list of all data providers can be found on the CORE website. ( https://core.ac.uk/data-providers ).
The data providers aggregated by CORE are located in 150 different countries. Figure 5 shows the top ten countries in terms of number of data providers aggregated by CORE from each country alongside the top ten languages. The geographic spread of repositories is largely reflective of the size of the research economy in those countries. We see the US, Japan, Germany, Brazil and the UK all in the top six. One result that at first may appear surprising is the significant number of repositories in Indonesia, enough to place them at the top of the list. An article in Nature in 2019 showed that Indonesia may be the worldâs OA leader, finding that 81% of 20,000 journal articles published in 2017 with an Indonesia-affiliated author are available to read for free somewhere online. ( https://www.nature.com/articles/d41586-019-01536-5 ). Additionally, there are a large number of Indonesian open-access journals registered with Crossref. This subsequently leads to a much higher number of individual repositories in this country.
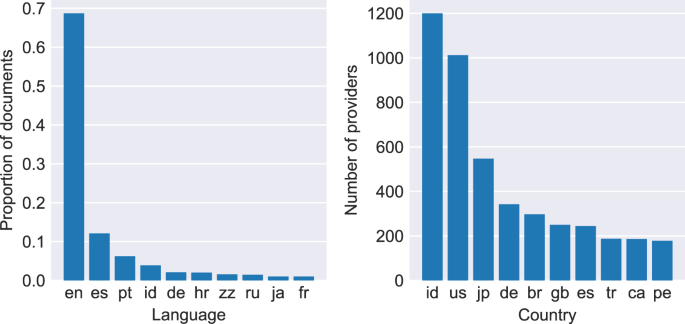
Top ten languages and top ten provider locations in CORE.
As part of the enrichment process, CORE performs language detection. Language is either extracted from the attached metadata where available or identified automatically from full text in case it is not available in metadata. More than 80% of all documents with language information are in English. Overall, CORE contains publications in a variety of languages, the top 10 of which are shown in Fig. 5 .
Document types
The CORE dataset comprises a collection of documents gathered from various sources, many of which contain articles of different types. Consequently, aside of research articles from journals and conferences, it includes other types of research outputs such as research theses, presentations, and technical reports. To distinguish different types of articles, CORE has implemented a method of automatically classifying documents into one of the following four categories 15 : (1) research article, (2) thesis, (3) presentation, (4) unknown (for articles not belonging into any of the previous three categories). This method is based on a supervised machine learning model trained on article full texts. Figure 6 shows the distribution of articles in CORE into these four categories. It can be seen that the collection aggregated by CORE consists predominantly of research articles. We have observed in the data collected from repositories that the vast majority of research theses deposited in repositories has full text associated with the metadata. As this is not always the case for research articles, and as Fig. 6 is produced on articles with full text only, we expect that the proportion of research articles compared to research theses in CORE is actually higher across the entire collection.
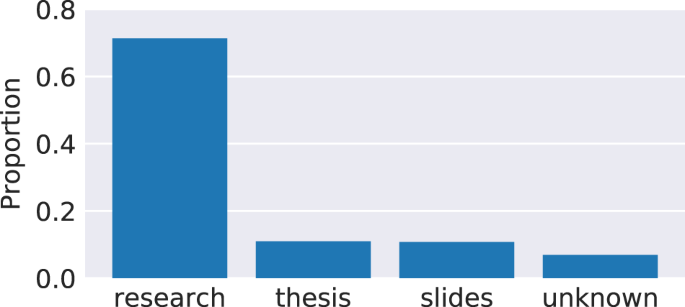
Distribution of document types.
Research disciplines
To analyse the distribution of disciplines in CORE we have leveraged a third-party service. Figure 7 shows a subject distribution of a sample of 20,758,666 publications in CORE. For publications with multiple subjects we count the publication towards each discipline.
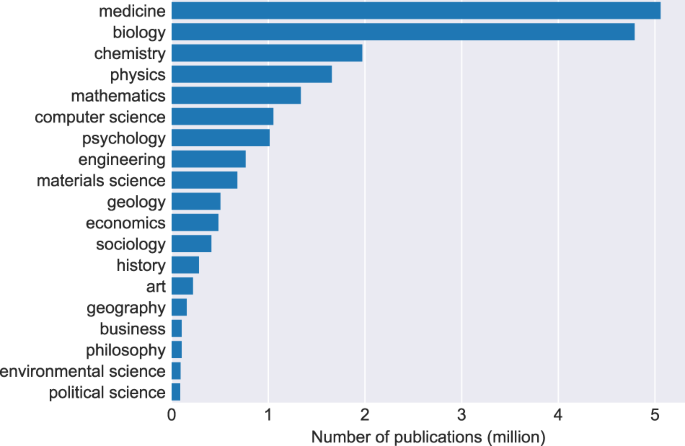
Subject distribution of a sample of 20,758,666 CORE publications.
The subject for each article was obtained using Microsoft Academic ( https://academic.microsoft.com/home ) prior to its retirement in November 2021. Our results are consistent with other studies, which have reported Biology, Medicine, and Physics to be the largest disciplines in terms of number of publications 16 , 17 , suggesting that the distribution of articles in CORE is representative of research publications in general.
Additional CORE Tools and Services
CORE has built several additional tools for a range of stakeholders including institutions, repository managers and researchers from across all scientific domains. Details of usage of these services is covered in the Uptake of CORE section.
The Dashboard provides a suite of tools for repository management, content enrichment, metadata quality assessment and open access compliance checking. Further, it can provide statistics regarding content downloads and suggestions for improving the efficiency of harvesting and the quality of metadata.
CORE Discovery helps users to discover freely accessible copies of research papers. There are several methods for interacting with the Discovery tool. First, as a plugin for repositories, enriching metadata only pages in repositories with links to open access copies of full text documents. Second, via a browser extension for researchers and anyone interested in reading scientific documents. And finally as an API service for developers.
Recommender
The recommender is a plugin for repositories, journal systems and web interfaces that provides suggestions on relevant articles to the one currently displayed. Its purpose is to support users in discovering articles of interest from across the network of open access repositories. It is notably used by prestigious institutions, including the University of Cambridge and by popular pre-prints services such as arXiv.org.
OAI Resolver
An OAI (Open Archives Initiative) identifier is a unique identifier of a metadata record. OAI identifiers are used in the context of repositories using the Open Archives Initiative Protocol for Metadata Harvesting (OAI-PMH). OAI Identifiers are viable persistent identifiers for repositories that can be, as opposed to DOIs, minted in a distributed fashion and cost-free, and which can be resolvable directly to the repository rather than to the publisher. The CORE OAI Resolver can resolve any OAI identifier to either a metadata page of the record in CORE or route it directly to the relevant repository page. This approach has the potential to increase the importance of repositories in the process of disseminating knowledge.
Uptake of CORE
As of February 2023, CORE averages over 40 million monthly active users and is the top 10th website in the category Science and Education according to SimilarWeb ( https://www.similarweb.com/ ). There are currently 4,700 registered API users and 2,880 registered dataset users. The CORE Dashboard is currently used by 499 institutional repositories to manage their open access content, monitor content download statistics, manage issues with metadata within the repository and ensure compliance with OA funder policies, notably REF in the U.K. The CORE Discovery plugin has been integrated into 434 repositories and the browser extension has been downloaded by more than 5,000 users via the Google Chrome Web Store ( https://chrome.google.com/webstore/category/extensions ). The CORE Recommender has been embedded in 70 repository systems including the University of Cambridge and arXiv.
In this section we discuss differences between CORE and other open access aggregation services and present several real-word use cases where CORE was used to develop services to support science. In this section we also present our future plans.
Existing open access aggregation services
Currently there are a number of open access aggregation services available (Table 4 ), with some examples being BASE ( https://base-search.net/ ), OpenAIRE ( https://www.openaire.eu/ ), Unpaywall ( http://unpaywall.org/ ), Paperity ( https://paperity.org/ ). BASE (Bielfield Academic Search Engine) is a global metadata harvesting service. It harvests repositories and journals via OAI-PMH and exposes the harvested content through an API and a dataset. OpenAIRE is a network of open access data providers who support open access policies. Even though in the past the project focused on European repositories, it has recently expanded by including institutional and subject repositories from outside Europe. A key focus of OpenAIRE is to assist the European Council to monitor compliance of its open access policies. OpenAIRE data is exposed via an API. Paperity is a service which harvests publications from open access journals. Paperity harvests both metadata and full text but does not host full texts. SHARE (Shared Access Research Ecosystem) is a harvester of open access content from US repositories. Its aim is to assist with the White House Office of Science and Technology Policy (OSTP) open access policies compliance. Even though SHARE harvests both metadata and full text it does not host the latter. Unpaywall is not primarily a harvester, but rather collects content from Crossref, whenever a free to read available version can be retrieved. It processes both metadata and full text but does not host them. It exposes the discovered links to documents through an API.
CORE differs from these services in a number of ways. CORE is currently the largest database of full text OA documents. In addition, CORE offers via its API a rich metadata record for each item in its collection which includes additional enrichments, contrary, for example, to Unpaywallâs API, which focuses only on delivering to the user information as to whether a free to read version is available. CORE also provides the largest number of links to OA content. To simplify access to data for end users it provides a number of ways for accessing its collection. All of the above services are free to use for research purposes however both CORE and Unpaywall also offer services to commercial partners on a paid-for basis.
Existing publication databases
Apart from OA aggregation services, a number of other services exists for searching and downloading scientific literature (Table 5 ). One of the main publication databases is Crossref ( https://www.crossref.org/ ), an authoritative index of DOI identifiers. Its primary function is to maintain metadata information associated with each DOI. The metadata stored by Crossref includes both OA and non-OA records. Crossref does not store publication full text, but for many publications provides full text links. As of February 2023, 5.9âm records in Crossref were associated with an explicit Creative Commons license (we have used the Crossref API to determine this number). Although Crossref provides an API, it does not offer its data for download in bulk, or provide a data sync service.
The remaining services from Table 5 can be roughly grouped into the following two categories: 1) citation indices, 2) academic search engines and scholarly graphs. The two major citation indices are Elsevierâs Scopus ( https://www.elsevier.com/solutions/scopus ) and Clarivateâs Web of Science ( https://clarivate.com/webofsciencegroup/solutions/web-of-science/ ), both of which are premium subscription services. Google Scholar, the best known academic search engine does not provide an API for accessing its data and does not permit crawling its website. Semantic Scholar ( https://www.semanticscholar.org/ ) is a relatively new academic search service which aims to create an âintelligent academic search engineâ 18 . Dimensions ( https://www.dimensions.ai/ ) is a service focused on data analysis. It integrates publications, grants, policy documents, and metrics. 1findr ( https://1findr.1science.com/home ) is a curated abstract indexing service. It provides links to full text, but no API or a dataset for download.
The added value of CORE
There are other services that claim to provide access to a large dataset of open access papers. In particular, Unpaywall 2 , claim to provide access to 46.4 million free to read articles, and BASE, who state they provide access to full texts of about 60% of their 300 million metadata records. However, these statistics are not directly comparable to the numbers we report and are a product of a different focus of these two projects. This is because both the analysis of BASE and now Unpaywall define âproviding access toâ in terms of having a list of URLs from which a human user can navigate to the full text of the resource. This means that both Unpaywall and BASE do not collect these full text resources, which is also why they do not face many of the challenges we described in the Introduction. Using this approach, we could say that the CORE Dataset provides access to approximately 139 million full texts, i.e. about 48% of our 291 million metadata records point to a URL from which a human can navigate to the full text. However, to people concerned with text and data mining of scientific literature, it makes little sense to count URLs pointing to many different domains on the Web as the number of full texts made available.
As a result, our 32.8 million statistic refers to the number of OA documents we identified, downloaded, extracted text from, validated their relationship to the metadata record and the full texts of which we host on the CORE servers and make available to others. In contrast, BASE and Unpaywall do not aggregate the full texts of the resources they provide access to and consequently do not offer the means to interact with the full texts of these resources or offer bulk download capability of these resources for text analytics over scholarly literature.
We have also integrated CORE data with the OpenMinTeD infrastructure, a European Commission funded project which aimed to provide a platform for text mining of scholarly literature in the cloud 6 .
A number of academia and industry partners have utilised CORE in their services. In this section we present three existing uses of CORE demonstrating how CORE can be utilised to support text and data mining use cases.
Since 2017, CORE has been collaborating with a range of scholarly search and discovery systems. These include Naver ( https://naver.com/ ), Lean Library ( https://www.leanlibrary.com/ ) and Ontochem ( https://ontochem.com/ ). As part of this work, CORE serves as a provider of full text copies of reserch papers to existing records in these systems (Lean Library) or even supplies both metadata and full texts for indexing (Ontochem, NAVER). This collaboration also benefits COREâs data providers as it expands and increases the visibility of their content.
In 2019, CORE entered into a collaboration with Turnitin, a global leader in plagiarism detection software. By using the CORE FastSync service, Turnitinâs proprietary web crawler searches through COREâs global database of open access content and metadata to check for text similarity. This partnership enables Turnitin to significantly enlarge its content database in a fast and efficient manner. In turn, it also helps protect open access content from misuse, thus protecting authors and institutions.
As of February 2023, CORE Recommender 19 is actively running in over 70 repositories including the University of Cambridge institutional repository and arXiv.org among others. The purpose of the recommender is to improve the discoverability of research outputs by providing suggestions for similar research papers both within the collection of the hosting repository and the CORE collection. Repository managers can install the recommender to advance the accessibility of other scientific papers and outreach to other scientific communities, since the CORE Recommender acts as a gate to millions of open access research papers. The recommender is integrated with the CORE search functionality and is also offered as a plugin for all repository software, for example EPrints, DSpace, etc. as well as open access journals and any other webpage. Based on the fact that CORE harvests open repositories, the recommender only displays research articles where the full text is available as open access, i.e. for immediate use, without access barriers or limited rightsâ restrictions. Through the recommender, CORE promotes the widest discoverability and distribution of the open access scientific papers.
Future work
An ongoing goal of CORE is to keep growing the collection to become a single point of access to all of worldâs open access research. However, there are a number of other ways we are planning to improve both the size and ease of access to the collection. The CORE Harvesting System was designed to enable adding new harvesting steps and enrichment tasks. There remains scope for adding more of such enrichments. Some of these are machine learning powered, such as classification of scientific citations 20 . Further, CORE is currently developing new methodologies to identify and link different versions of the same article. The proposed system, titled CORE Works, will leverage COREâs central position in the OA infrastructure landscape and will link different versions of the same paper using a unique identifier. We will continue to keep linking the CORE collection to scholarly entities from other services, thereby making CORE data participate in a global scholarly knowledge graph.
In the Introduction section we focused on a a number of challenges researchers face when collecting research literature for text and data mining. In this section, we instead focus on the perspective of a research literature aggregator, i.e. a system whose goal is to continuously provide seamless access to research literature aggregated from thousands of data providers worldwide in a way that enables the resulting research publication collection to be used by others in production applications. We describe the challenges we had to overcome to build this collection and to keep it continuously up-to-date, and present the key technical innovations which allowed us to greatly increase the size of the CORE collection and become a leading provider of open access literature which we illustrate using our content growth statistics.
CORE Harvesting system (CHARS)
CORE Harvesting System (CHARS) is the backbone of our harvesting process. CHARS uses the Harvesting Scheduler (Section CHARS_architecture) to select data providers to be processed next. It manages all the running processes (tasks) and ensures the available compute resources are well utilised.
Prior to implementing CHARS, CORE was centralised around data providers rather than around individual tasks needed to harvest and process these data providers (e.g. metadata download and parsing, full text download, etc.). Consequently, even though the scaling up and the continuation of this system was possible, the infrastructure was not horizontally scalable and the architecture suffered from tight coupling of services. This was not consistent with COREâs high availability requirements and was regularly causing problems in the complexity of maintenance. In response to these challenges, we designed CHARS using a microservices architecture, i.e. using small manageable autonomous components that work together as part of a larger infrastructure 21 . One of the key benefits of microservices-oriented architecture is that the implementation focus can be put on the individual components which can be improved and redeployed as frequently as needed and independently of the rest of the infrastructure. As the process of open access content harvesting can be inherently split into individual consecutive tasks, a microservices-oriented architecture presents a natural fit for aggregation systems like CHARS.
Tasks involved in open access content harvesting
The harvesting process can be described as a pipeline where each task performs a certain action and where the output of each task feeds into the next task. The input to this pipeline is a set of data providers and the final output is a system populated with records of research papers available from them. The main types of key tasks currently performed as part of COREâs harvesting system are (Fig. 8 ):
Metadata download: The metadata exposed by a data provider via OAI-PMH are downloaded and stored in the file system (typically as an XML). The downloading process is sequential, i.e. a repository provides typically between 100â1,000 metadata records per request and a resumption token. This token is then used to provide the next batch. As a result, full harvesting can a significant amount of time (hours-days) for large data providers. Therefore, this process has been implemented to provide resilience to a range of communication failures.
Metadata extraction : Metadata extraction parses, cleans, and harmonises the downloaded metadata and stores them into the CORE internal data structure (database). The harmonisation and cleaning process addresses the fact that different data providers/repository platforms describe the same information in different ways (syntactic heterogeneity) as well as having different interpretations for the same information (semantic heterogeneity).
Full text download : Using links extracted from the metadata CORE attempts to download and store publication manuscripts. This process is non-trivial and is further described in the Using OAI-PMH for content harvesting section.
Information extraction : Plain text from the downloaded manuscripts is extracted and processed to create a semi-structured representation. This process includes a range of information extraction tasks, such as references extraction.
Enrichment : The enrichment task works by increasing both metadata and full text harvested from the data providers with additional data from multiple sources. Some of the enrichments are performed directly by specific tasks in the pipeline such as language detection and document type detection. The remaining enrichments that involve external datasets are performed externally and independently to the CHARS pipeline and ingested into the dataset as described in the Enrichments section.
Indexing : The final step in the harvesting pipeline is indexing the harvested data. The resulting index powers COREâs services, including search, API and FastSync.
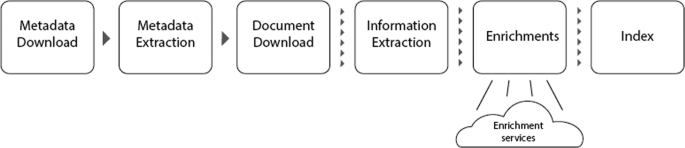
CORE Harvesting Pipeline. Each tasksâ output produces the input for the following task. In some cases the input is considered as a whole, for example all the content harvested from a data provider, while in other cases, the output is split in multiple small tasks performed on a record level.
Scalable infrastructure requirements
Based on the experience obtained while developing and maintaining our harvesting system as well as taking into consideration the features of the CiteSeerX 22 architecture, we have defined a set of requirements for a scalable harvesting infrastructure 8 . These requirements are generic and apply to any aggregation or digital library scenario. These requirements informed and are reflected in the architecture design of CHARS (Section CHARS architecture):
Easy to maintain: The system should be easy to manage, maintain, fix, and improve.
High levels of automation: The system should be completely autonomous while allowing manual interaction.
Fail fast: Items in the harvesting pipeline should be validated immediately after a task is performed, instead of having only one and final validation at the end of the pipeline. This has the benefit of recognising issues and enabling fixes earlier in the process.
Easy to troubleshoot: Possible code bugs should be easily discerned.
Distributed and scalable: The addition of more compute resources should allow scalability, be transparent and replicable.
No single point of failure: A single crash should not affect the whole harvesting pipeline, individual tasks should work independently.
Decoupled from user-facing systems: Any failure in the ingestion processing services should not have an immediate impact on user-facing services.
Recoverable: When a harvesting task stops, either manually or due to a failure, the system should be able to recover and resume the task without manual intervention.
Performance observable: The systemâs progress must be properly logged at all times and overlay monitoring services should be set up to provide a transparent overview of the servicesâ progress at all times, to allow early detection of scalability problems and identification of potential bottlenecks.
CHARS architecture
An overview of CHARS is shown in Fig. 9 . The system consists of the following main software components:
Scheduler: it becomes active when a task finishes. It monitors resource utilisation and selects and submits data providers to be harvested.
Queue (Qn): a messaging system that assists with communication between parts of the harvesting pipeline. Every individual task, such as metadata download, metadata parsing, full text download, and language detection, has its own message queue.
Worker (W i ): an independent and standalone application capable of executing a specific task. Every individual task has its own set of workers.

CORE Harvesting System.
A complete harvest of a data provider can be described as follows. When an existing task finishes, the scheduler is activated and informed of the result. It then uses the formula described in Appendix A to assign a score to each data provider. Depending on current resource utilisation, i.e. if there are any idle workers, and the number of data providers already scheduled for harvesting, the data provider with the highest score is then placed in the first queue Q 1 which contains data providers scheduled for metadata download. Once one of the metadata download workers W i -W j becomes available, a data provider is taken out of the queue and a new download of its metadata starts. Upon completion, the worker notifies the scheduler and, if the task is completed successfully, places the data provider in the next queue. This process continues until the data provider passes through the entire pipeline.
While some of the tasks in the pipeline need to be performed at the granularity of data providers, specifically metadata download and parsing, other tasks, such as full text extraction and language detection, can be performed at the granularity of individual records. While these tasks are originally scheduled at the granularity of data providers, only the individual records of a selected data provider which require processing are subsequently independently placed in the appropriate queue. Workers assigned to these tasks then process the individual records in the queue and they move through the pipeline once completed.
A more detailed description of CHARS, which includes technologies used to implement it, as well as other details can be found in 8 .
The harvesting scheduler is a component responsible for identifying data providers which need to be harvested next and placing these data providers in the harvesting queue. In the original design of CORE, our harvesting schedule was created manually, assigning the same harvesting frequency to every data provider. However, we found this approach inefficient as it does not scale due to the varying data providers size, differences in the update frequency of their databases and the maximum data delivery speeds of their repository platforms. To address these limitations, we designed the CHARS scheduler according to our new concept of âpro-active harvesting.â This means that the scheduler is event driven. It is triggered whenever the underlying hardware infrastructure has resources available to determine which data provider should be harvested next. The underlying idea is to maximise the number of ingested documents over a unit of time. The pseudocode and the formula we use to determine which repository to harvest next is described in Algorithm 1.
The size of the metadata download queue, i.e. the queue which represents an entry into the harvesting pipeline, is kept limited in order to keep the system responsive to the prioritisation of data providers. A long queue makes prioritising data providers harder, as it is not known beforehand how long the processing of a particular data provider will take. An appropriate size of the queue ensures a good balance between the reactivity and utilisation of the available resources.
Using OAI-PMH for content harvesting
We now describe the third key technical innovation which enables us to harvest full text content (as opposed to just metadata) from data providers using the OAI-PMH protocol. This process represents one step in the harvesting pipeline (Fig. 9 ), specifically, the third step which is activated after data provider metadata have been downloaded and parsed.
The OAI-PMH protocol was originally designed for metadata harvesting only, but due to its wide adoption and lack of alternatives it has been used as an entry point for full text harvesting from repositories. Full text harvesting is achieved by using URLs found in the metadata records to discover the location of the actual resource and subsequently downloading it 9 . We summarised the key challenges of this approach in the Challenges related to the use of OAI-PMH protocol for content harvesting section. The algorithm follows a depth first search strategy with prioritisation and finishes as soon as the first matching document is found.
The procedure works in the following way. First, all metadata records from a selected data provider with no full text are collected. Those records for which full text download was attempted within the retry period ( RP ) (usually six months) are filtered out. This is to avoid repeatedly downloading URLs that do not lead to the sought after documents. The downside of this approach is that if a data provider updates a link in the metadata, it might take up to the duration of the retry period to acquire the full text.
Algorithm 1
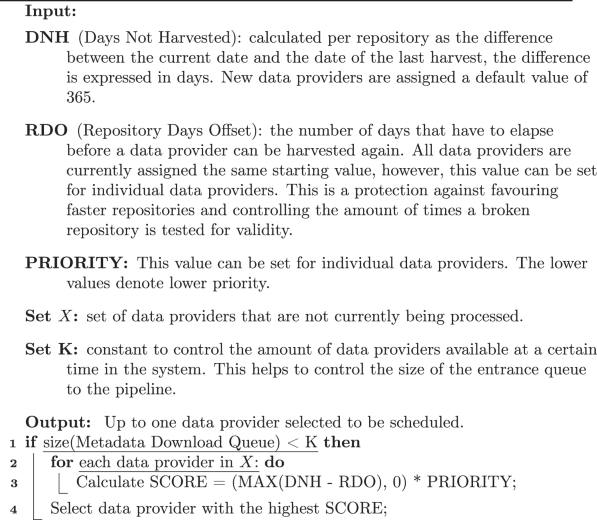
Next, the records are further filtered using a set of rules and heuristics we developed to a) increase the chances of identifying the URL leading to the described document quickly and b) to ensure that we identify the correct document. These filtering rules include:
Accepted file extensions: URLs are filtered according to a list of accepted file extensions. URLs ending with extensions such as .pptx that clearly indicate that the URL does not link to the required resource are removed from the list.
Same domain policy: URLs in the OAI-PMH metadata can link to any resources and domains. For example, a common practice is to provide a link to the associated presentation, dataset, or another related resource. As these are often stored in external databases, filtering out all URLs that lead to an external domain, i.e. domain different than the domain of the data provider, presents a simple method of avoiding the download of resources which with very high likelihood do not represent the target document. Exceptions include dx.doi.org and hdl.handle.net domains whose purpose is to provide a persistent identifier pointing to the document. The same domain policy is disabled for data providers which are aggregators and link to many different domains by design.
Provider-specific crawling heuristics: Many data providers follow a specific pattern when composing URLs. For example, a link to a full text document may be composed of the following parts: data provider URL â+â record handle â+â .pdf . For data providers utilising such patterns, URLs may be composed automatically where the relevant information (record handle) is known to us from the metadata. These generated URLs are then added to the list of URLs obtained from the metadata.
Prioritising certain URLs: As it is more likely for PDF URL to contain the target record than for an HTML URL, the final step is to sort URLs according to file and URL type. Highest priority is assigned to URLs that uses repository software specific patterns to identify full text, document, and PDF filetypes, while the lowest priority is assigned to hdl.handle.net URLs.
The system then attempts to request the document at each URL and download it. After each download, checks are performed to determine whether the downloaded document represents the target record. Currently, the downloaded document has to be a valid PDF with a title matching the original metadata record. If the target record is identified, the downloaded document is stored and the download process for that record ends. If the downloaded document contains an HTML page, URLs are extracted from this page and filtered using the same method mentioned above. This is because it is common in some of the most widely used repository systems such as DSpace for the documents not to be directly referenced from within the metadata records. Instead, the metadata records typically link to an HTML overview page of the document. To deal with this problem, we use the concept of harvesting levels. A maximum harvesting level corresponds to the maximum search depth for the referenced document. The algorithm finishes either as soon as the first matching document is found or after all the available URLs up to the maximum harvesting level have been exhausted. Algorithm 2 describes our approach for collecting the full texts using the OAI-PMH protocol. The algorithm follows a depth first search strategy with prioritisation and finishes as soon as the first matching document is found.
Algorithm 2
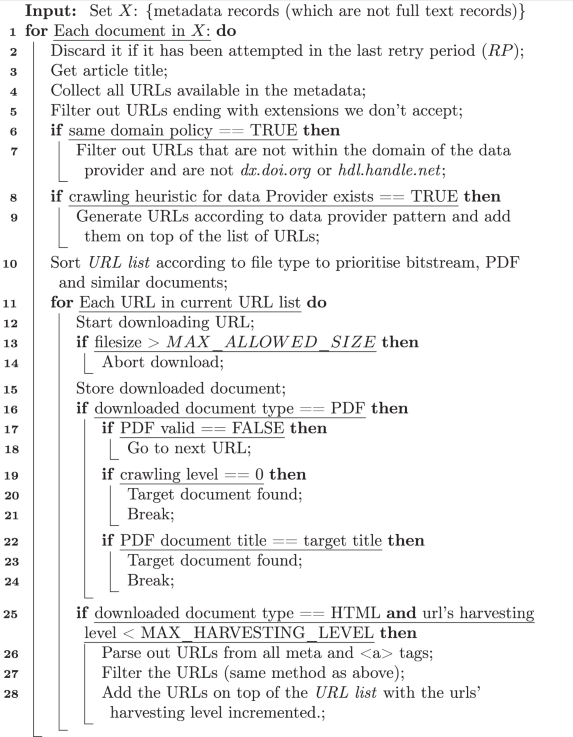
CHARS limitations
Despite overcoming the key issues to scalable harvesting of content from repositories, there still remains a number of important challenges. The first relates to the difficulty of estimating the optimal number of workers in our system to run efficiently. While the worker allocation is still largely established empirically, we are investigating more sophisticated approaches based on formal models of distributed computation, such as Petri Nets. This will allow us to investigate new approaches to dynamically allocating and launching workers to optimise the usage of our resources.
Enrichments
Conceptually, two types of enrichment processes are used within CORE: 1) an online enrichment process enriching a single record at the time of it being processed by the CHARS pipeline and 2) a periodic offline enrichment process which enriches a record based on information in external datasets (Fig. 10 ).
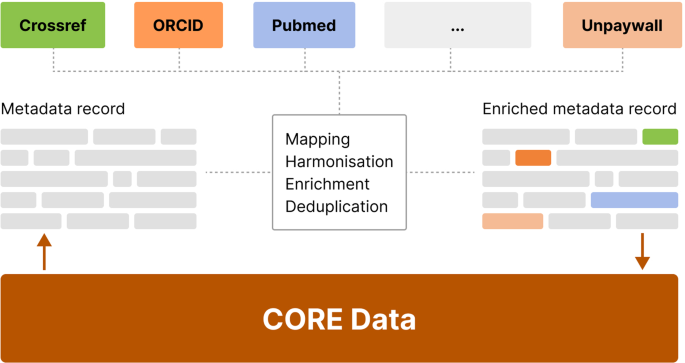
CORE Offline Enrichments.
Online enrichments
Online enrichments are fully integrated into the CHARS pipeline described earlier in this section. These enrichments generally involve the application of machine learning models and rule-based tools to gather additional insights about the record, such as language detection, document type detection. As opposed to offline enrichments, online enrichments are always performed just once for a given record. The following is a list of the current enrichments performed online:
Article type detection: A machine learning algorithm assigns each publication one of the following four types: presentation, thesis, research paper, other. In the future we may include other types.
Language identification: This task uses third-party libraries to identify the language based on the full text of a document. The resulting language is then compared to the one provided by the metadata record. Some heuristics are applied to disambiguate and harmonise languages.
Offline enrichments
Offline enrichments are carried out by means of gathering a range of information from large third-party scholarly datasets (research graphs). Such information includes metadata that do not necessarily change, such as a DOI identifier, as well as metadata that evolve, such as the number of citations. Especially due to the latter, CORE performs offline enrichments periodically, i.e. all records in CORE go through this process repeatedly at specified time intervals (currently once per month).
The process is depicted in Fig. 10 . The initial mapping of a record is carried out using a DOI, if available. However, as the majority of records from repositories do not come with a DOI, we carry out a matching process against the Crossref database using a subset of metadata fields including title, authors and year. Once the mapping is performed, we can harmonise fields as well as gather a wide range of additional useful data from relevant external databases, thereby enriching the CORE record. Such data include, ORCID identifiers, citation information, additional links to freely available full texts, field of study information and PubMed identifiers. Our solution is based on a set of map-reduce tasks to enrich the dataset and implemented on a Cloudera Enterprise Data Hub ( https://www.cloudera.com/products/enterprise-data-hub.html ) 23 , 24 , 25 , 26 .
Data availability
CORE provides several large data dumps of the processed and aggregated data under the ODC-BY licence ( https://core.ac.uk/documentation/dataset ). The only condition for both commercial and non-commercial reuse of these datasets is to acknowledge the use of CORE in their outputs. Additionally, CORE makes its API and most recent data dump freely available to registered individual users and researchers. Please note that CORE claims no rights in the aggregated content itself which is open access and therefore freely available to everyone. All CORE data rights correspond to the sui generis database rights of the aggregated and processed collection.
Licences for CORE services, such as the API and FastSync, are available for commercial users wishing to benefit from convenient access to CORE data with guaranteed level of customer support. The organisation running CORE, i.e. The Open University, is a charitable organisation fully committed to the Open Research mission. CORE is a signatory of the Principles of Open Scholarly Infrastructure (POSI) ( https://openscholarlyinfrastructure.org/posse ). No profit generation is practised. Instead, COREâs income from licences to commercial parties is used solely to provide sustainability by means of enabling CORE to become less reliant on unstable project grants, thus offsetting and reducing the cost of CORE to the taxpayer. This is done in full compliance with the principles and best practices of sustainable open science infrastructure.
Code availability
CORE consists of multiple services. Most of our source code is open source and available in our public repository on GitHub ( https://github.com/oacore/ ). As of today, we are unfortunately not yet able to provide the source code to our data ingestion module. However, as we want to be as transparent as possible with our community, we have documented in this paper the key algorithms and processes which we apply using pseudocode.
Bornmann, L. & Mutz, R. Growth rates of modern science: A bibliometric analysis based on the number of publications and cited references. JASIST 66 (11), 2215â2222 (2015).
CAS Â Google Scholar Â
Piwowar, H. et al . The State of OA: A large-scale analysis of the prevalence and impact of Open Access articles. PeerJ 6 , e4375 (2018).
Article  PubMed  PubMed Central  Google Scholar Â
Saggion, H. & Ronzano, F. Scholarly data mining: making sense of scientific literature. 2017 ACM/IEEE Joint Conference on Digital Libraries (JCDL) : 1â2 (2017).
Kim, E. et al . Materials synthesis insights from scientific literature via text extraction and machine learning. Chemistry of Materials 29 (21), 9436â9444 (2017).
Article  CAS  Google Scholar Â
Jacobs, N. & Ferguson, N. Bringing the UKâs open access research outputs together: Barriers on the Berlin road to open access. Jisc Repository (2014).
Knoth, P., Pontika, N. Aggregating Research Papers from Publishersâ Systems to Support Text and Data Mining: Deliberate Lack of Interoperability or Not? In: INTEROP2016 (2016).
Herrmannova, D., Pontika, N. & Knoth, P. Do Authors Deposit on Time? Tracking Open Access Policy Compliance. Proceedings of the 2019 ACM/IEEE Joint Conference on Digital Libraries , Urbana-Champaign, IL (2019).
Cancellieri, M., Pontika, N., Pearce, S., Anastasiou, L. & Knoth, P. Building Scalable Digital Library Ingestion Pipelines Using Microservices. Proceedings of the 11th International Conference on Metadata and Semantics Research (MTSR 2017) : 275â285. Springer (2017).
Knoth, P. From open access metadata to open access content: two principles for increased visibility of open access content. Proceedings of the 2013 Open Repositories Conference , Charlottetown, Prince Edward Island, Canada (2013).
Knoth, P.; Cancellieri, M. & Klein, M. Comparing the Performance of OAI-PMH with ResourceSync. Proceedings of the 2019 Open Repositories Conference , Hamburg, Germany (2019).
Kapidakis, S. Metadata Synthesis and Updates on Collections Harvested Using the Open Archive Initiative Protocol for Metadata Harvesting. Digital Libraries for Open Knowledge. TPDL 2018. Lecture Notes in Computer Science 11057 , 16â31 (2018).
Google Scholar Â
Knoth, P. and Zdrahal, Z. CORE: three access levels to underpin open access. D-Lib Magazine 18 (11/12) (2012).
Haslhofer, B. et al . ResourceSync: leveraging sitemaps for resource synchronization. Proceedings of the 22nd International Conference on World Wide Web : 11â14 (2013).
Khabsa, M. & Giles, C. L. The number of scholarly documents on the public web. PLOS One 9 (5), e93949 (2014).
Article  ADS  PubMed  PubMed Central  Google Scholar Â
Charalampous, A. & Knoth, P. Classifying document types to enhance search and recommendations in digital libraries. Research and Advanced Technology for Digital Libraries. TPDL 2017. Lecture Notes in Computer Science 10450 , 181â192 (2017).
Rosvall, M. & Bergstrom, C. T. Maps of random walks on complex networks reveal community structure. Proceedings of the National Academy of Sciences 105 (4), 1118â1123 (2008).
Article  ADS  CAS  Google Scholar Â
DâAngelo, C. A. & Abramo, G. Publication rates in 192 research fields of the hard sciences. Proceedings of the 15th ISSI Conference : 915â925 (2015).
Ammar, W. et al . Construction of the Literature Graph in Semantic Scholar. Proceedings of the 2018 Conference of the North American Chapter of the Association for Computational Linguistics: Human Language Technologies , Volume 3 (Industry Papers): 84â91 (2018).
Knoth, P. et al . Towards effective research recommender systems for repositories. Open Repositories , Bozeman, USA (2017).
Pride, D. & Knoth, P. An Authoritative Approach to Citation Classification. Proceedings of the 2020 ACM/IEEE Joint Conference on Digital Libraries (JCDL 2020), VirtualâChina (2020).
Newman, S. Building microservices: designing fine-grained systems. OâReilly Media, Inc. (2015).
Li, H. et al . CiteSeer Ï : a scalable autonomous scientific digital library. Proceedings of the 1st International Conference on Scalable Information Systems , ACM (2006).
Bastian, H., Glasziou, P. & Chalmers, I. Seventy-five trials and eleven systematic reviews a day: how will we ever keep up? PLoS medicine 7 (9), e1000326 (2010).
Shojania, K. G. et al . How quickly do systematic reviews go out of date? A survival analysis. Annals of internal medicine 147 (4), 224â233 (2007).
Article  PubMed  Google Scholar Â
Tsafnat, G. et al . Systematic review automation technologies. Systematic reviews 3 (1), 74 (2014).
Harzing, A.-W. & Alakangas, S. Microsoft Academic is one year old: The Phoenix is ready to leave the nest. Scientometrics 112 (3), 1887â1894 (2017).
Article  Google Scholar Â
Download references
Acknowledgements
We would like to acknowledge the generous support of Jisc, under a number of grants and service contracts with The Open University. These included projects CORE, ServiceCORE, UK Aggregation (1 and 2) and DiggiCORE, which was co-funded by Jisc with NWO. Since 2015, CORE has been supported in three iterations under the Jisc Digital ServicesâCORE (JDSCORE) service contract with The Open University. Within Jisc, we would like to thank primarily the CORE project managers, Andy McGregor, Alastair Dunning, Neil Jacobs and Balviar Notay. We would also like to thank the European Commission for funding that contributed to CORE, namely OpenMinTeD (739563) and EOSC Pilot (654021). We would like to show our gratitude to all current CORE Team members who contributed to CORE but are not authors of the manuscript, namely Valeriy Budko, Ekaterine Chkhaidze, Viktoriia Pavlenko, Halyna Torchylo, Andrew Vasilyev and Anton Zhuk. We would like to show our gratitude to all past CORE Team members who have contributed to CORE over the years, namely Lucas Anastasiou, Giorgio Basile, Aristotelis Charalampous, Josef Harag, Drahomira Herrmannova, Alexander Huba, Bikash Gyawali, Tomas Korec, Dominika Koroncziova, Magdalena Krygielova, Catherine Kuliavets, Sergei Misak, Jakub Novotny, Gabriela Pavel, Vojtech Robotka, Svetlana Rumyanceva, Maria Tarasiuk, Ian Tindle, Bethany Walker and Viktor Yakubiv, Zdenek Zdrahal and Anna Zelinska.
Author information
Drahomira Herrmannova
Present address: Oak Ridge National Laboratory Oak Ridge, Oak Ridge, TN, USA
Authors and Affiliations
Knowledge Media Institute, The Open University Walton Hall, Milton Keynes, UK
Petr Knoth, Drahomira Herrmannova, Matteo Cancellieri, Lucas Anastasiou, Nancy Pontika, Samuel Pearce, Bikash Gyawali & David Pride
You can also search for this author in PubMed  Google Scholar
Contributions
P.K. is the Founder and Head of CORE. He conceived the idea and has been the project lead since the start in 2011. He researched and created the first version of CORE, acquired funding, built the team, and has been managing and leading all research and development. M.C., L.A., S.P. and P.K. designed, worked out all technical details, and implemented significant parts of the system including CHARS, the harvesting scheduler, and the OAI-PMH content harvesting method. All authors contributed to the maintenance, operation and improvements of the system. D.H. drafted the initial version of the manuscript based on consultations with P.K. D.P. and P.K. wrote the final manuscript with additional input from L.A. and N.P. D.H., M.C. and L.A. performed the data analysis for the paper and D.H. produced the figures. D.H., D.P., B.G. and L.A. participated in research activities and tasks related to CORE following the instructions and directly supervised by P.K.
Corresponding author
Correspondence to Petr Knoth .
Ethics declarations
Competing interests.
The authors declare no competing interests.
Additional information
Publisherâs note Springer Nature remains neutral with regard to jurisdictional claims in published maps and institutional affiliations.
Rights and permissions
Open Access This article is licensed under a Creative Commons Attribution 4.0 International License, which permits use, sharing, adaptation, distribution and reproduction in any medium or format, as long as you give appropriate credit to the original author(s) and the source, provide a link to the Creative Commons license, and indicate if changes were made. The images or other third party material in this article are included in the articleâs Creative Commons license, unless indicated otherwise in a credit line to the material. If material is not included in the articleâs Creative Commons license and your intended use is not permitted by statutory regulation or exceeds the permitted use, you will need to obtain permission directly from the copyright holder. To view a copy of this license, visit http://creativecommons.org/licenses/by/4.0/ .
Reprints and permissions
About this article
Cite this article.
Knoth, P., Herrmannova, D., Cancellieri, M. et al. CORE: A Global Aggregation Service for Open Access Papers. Sci Data 10 , 366 (2023). https://doi.org/10.1038/s41597-023-02208-w
Download citation
Received : 18 May 2021
Accepted : 03 May 2023
Published : 07 June 2023
DOI : https://doi.org/10.1038/s41597-023-02208-w
Share this article
Anyone you share the following link with will be able to read this content:
Sorry, a shareable link is not currently available for this article.
Provided by the Springer Nature SharedIt content-sharing initiative
Quick links
- Explore articles by subject
- Guide to authors
- Editorial policies
Sign up for the Nature Briefing newsletter â what matters in science, free to your inbox daily.

đșđŠÂ  make metadata, not war
A comprehensive bibliographic database of the worldâs scholarly literature
The worldâs largest collection of open access research papers, machine access to our vast unique full text corpus, core features, indexing the worldâs repositories.
We serve the global network of repositories and journals
Comprehensive data coverage
We provide both metadata and full text access to our comprehensive collection through our APIs and Datasets
Powerful services
We create powerful services for researchers, universities, and industry
Cutting-edge solutions
We research and develop innovative data-driven and AI solutions
Committed to the POSI
Cost-free PIDs for your repository
OAI identifiers are unique identifiers minted cost-free by repositories. Ensure that your repository is correctly configured, enabling the CORE OAI Resolver to redirect your identifiers to your repository landing pages.
OAI IDs provide a cost-free option for assigning Persistent Identifiers (PIDs) to your repository records. Learn more.
Who we serve?
Enabling others to create new tools and innovate using a global comprehensive collection of research papers.
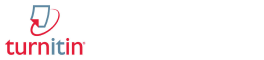
â Our partnership with CORE will provide Turnitin with vast amounts of metadata and full texts that we can ... â Show more
Gareth Malcolm, Content Partner Manager at Turnitin
Academic institutions.
Making research more discoverable, improving metadata quality, helping to meet and monitor open access compliance.
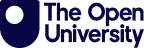
â COREâs role in providing a unified search of repository content is a great tool for the researcher and ex... â Show more
Nicola Dowson, Library Services Manager at Open University
Researchers & general public.
Tools to find, discover and explore the wealth of open access research. Free for everyone, forever.

â With millions of research papers available across thousands of different systems, CORE provides an invalu... â Show more
Jon Tennant, Rogue Paleontologist and Founder of the Open Science MOOC
Helping funders to analyse, audit and monitor open research and accelerate towards open science.
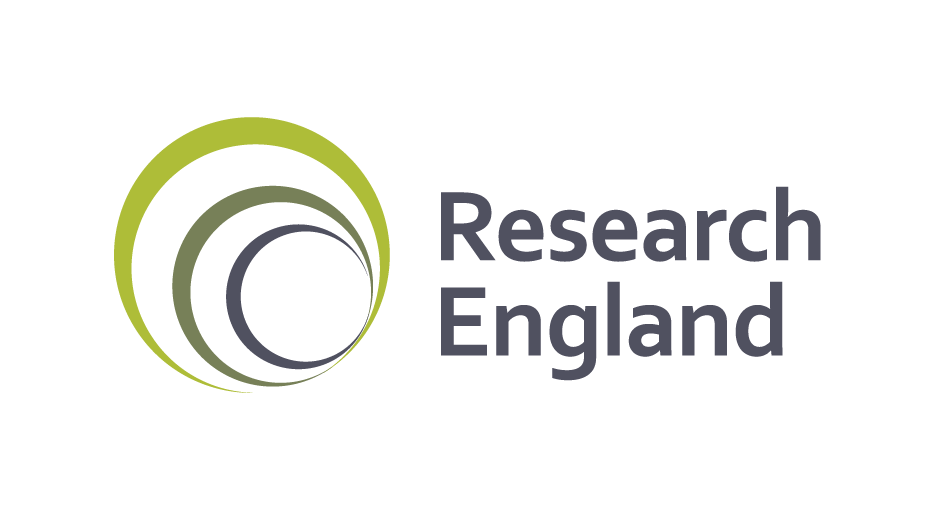
â Aggregation plays an increasingly essential role in maximising the long-term benefits of open access, hel... â Show more
Ben Johnson, Research Policy Adviser at Research England
Our services, access to raw data.
Create new and innovative solutions.
Content discovery
Find relevant research and make your research more visible.
Managing content
Manage how your research content is exposed to the world.
Companies using CORE
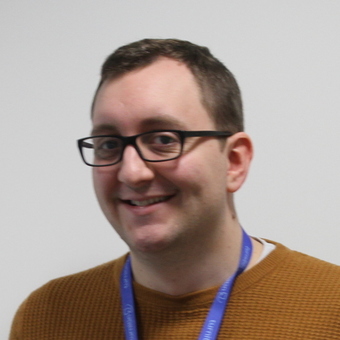
Gareth Malcolm
Content Partner Manager at Turnitin
Our partnership with CORE will provide Turnitin with vast amounts of metadata and full texts that we can utilise in our plagiarism detection software.
Academic institution using CORE
Kathleen Shearer
Executive Director of the Confederation of Open Access Repositories (COAR)
CORE has significantly assisted the academic institutions participating in our global network with their key mission, which is their scientific content exposure. In addition, CORE has helped our content administrators to showcase the real benefits of repositories via its added value services.
Partner projects
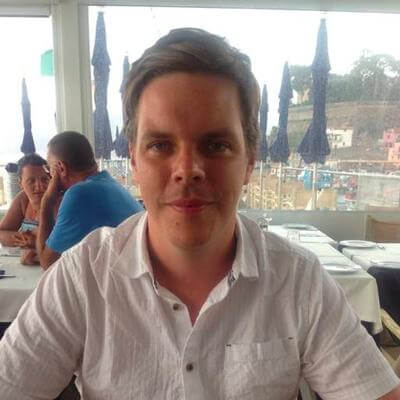
Ben Johnson
Research Policy Adviser
Aggregation plays an increasingly essential role in maximising the long-term benefits of open access, helping to turn the promise of a 'research commons' into a reality. The aggregation services that CORE provides therefore make a very valuable contribution to the evolving open access environment in the UK.
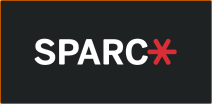
The Journal of Open Source Software is a developer friendly , open access journal for research software packages.
Committed to publishing quality research software with zero article processing charges or subscription fees.
Recently Published Papers 2447
Scas dashboard: a tool to intuitively and interactively analyze slurm cluster usage.
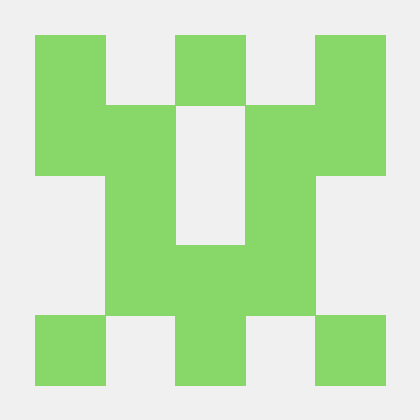
STITCHES: a Python package to amalgamate existing Earth system model output into new scenario realizations
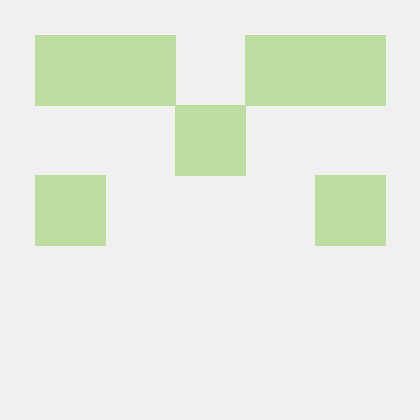
planetMagFields: A Python package for analyzing and plotting planetary magnetic field data
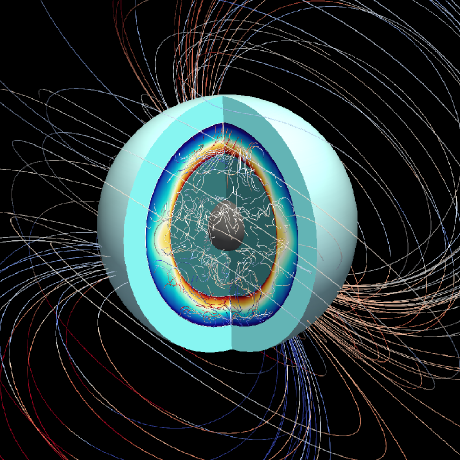
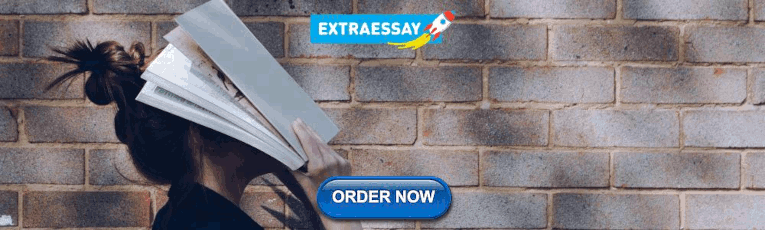
21cmSense v2: A modular, open-source 21 cm sensitivity calculator
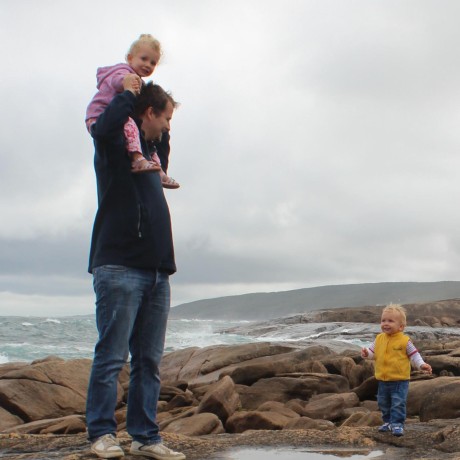
Spelunker: A quick-look Python pipeline for JWST NIRISS FGS Guide Star Data
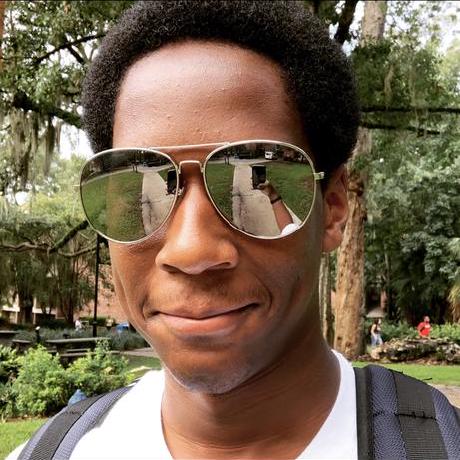
SIMIO-continuum: Connecting simulations to ALMA observations
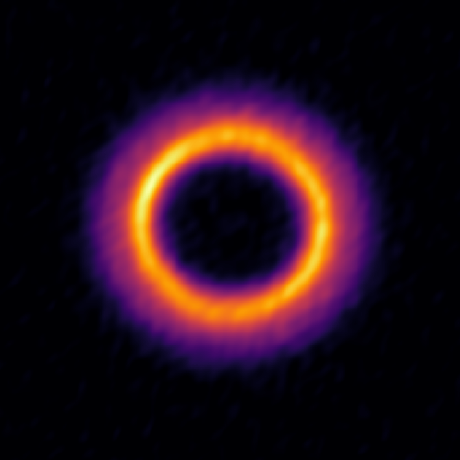
QSOnic: fast quasar continuum fitting
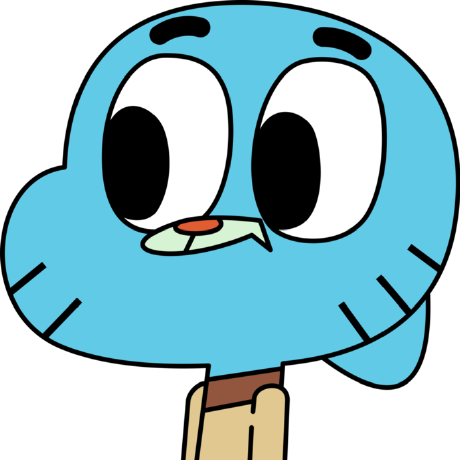
Contextualized: Heterogeneous Modeling Toolbox
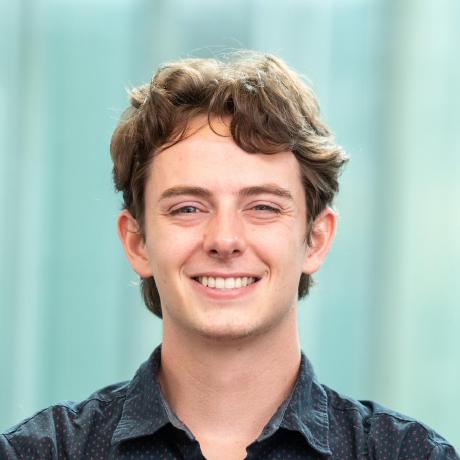
spINAR: An R Package for Semiparametric and Parametric Estimation and Bootstrapping of Integer-Valued Autoregressive (INAR) Models
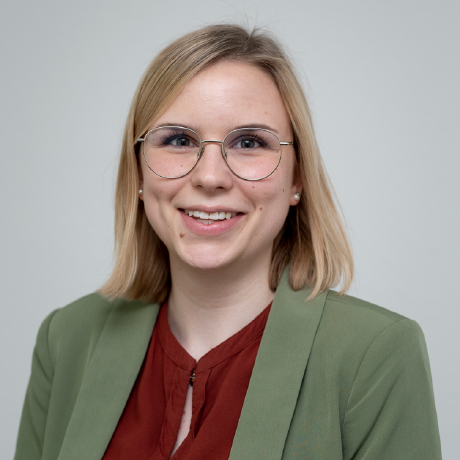
CalibrateEmulateSample.jl: Accelerated Parametric Uncertainty Quantification
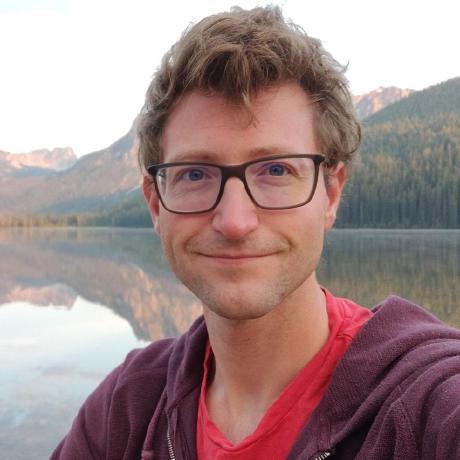
Journal of Open Source Software is an affiliate of the Open Source Inititative .
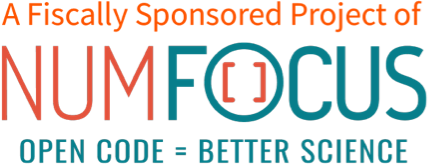
Journal of Open Source Software is part of Open Journals , which is a NumFOCUS-sponsored project .
Table of Contents Public user content licensed CC BY 4.0 unless otherwise specified. ISSN 2475-9066
- Mission and history
- Platform features
- Library Advisory Group
- Whatâs in JSTOR
- For Librarians
- For Publishers
Open research reports
JSTOR hosts a growing curated collection of more than 50,000 open research reports from 187 think tanks and research institutes from around the world. These publications are freely accessible to everyone on JSTOR and discoverable as their own content type alongside journals, books, and primary sources. We update research reports on our platform each month as they become available through contributing institutes.
Download the list (xlsx) of contributing policy institutes.
Research reports provide current analysis on many of todayâs most discussed and debated issues from a diversity of ideological and international perspectives representing 40 countries and 29 languages. A sample of topics would include: climate change, border security, fake news, cybersecurity, electric vehicles, artificial intelligence, energy policy, gender issues, terrorism, remote learning, recent trends in business and economics, and various public health issues, including COVID-19.
Although the briefs, papers, and reports published by these institutes are not peer-reviewed, they are written by policy experts and members of the academic community who are fellows in residence. This is content that impacts policy, both foreign and domestic. It is also increasingly used by faculty in their classrooms for its currency, breadth, and accessibility.
JSTORâs research reports cover seven Areas of Focus: Business & Economics, Critical Race & Ethnic Studies, Education, Gender & Sexuality, Public Health, Security Studies, and Sustainability.
Browse research reports
Why research reports on JSTOR?
Input from faculty and librarians revealed that although research reports were for the most part freely available outside of JSTOR, they were hard to find and not easily discoverable alongside relevant material. It was also difficult for students to differentiate between the most credible research reports and a growing corpus of questionable sources on the Web.
JSTOR has attempted to redress these issues by centralizing a curated collection of think tank research reports on a single platform, making this content freely available to all JSTOR users, and enhancing its discoverability through comprehensive searching and the application of rich metadata.
MIT Libraries home DSpace@MIT
- DSpace@MIT Home
MIT Open Access Articles
The MIT Open Access Articles collection consists of scholarly articles written by MIT-affiliated authors that are made available through DSpace@MIT under the MIT Faculty Open Access Policy, or under related publisher agreements. Articles in this collection generally reflect changes made during peer-review.
Version details are supplied for each paper in the collection:
- Original manuscript: author's manuscript prior to formal peer review
- Author's final manuscript: final author's manuscript post peer review, without publisher's formatting or copy editing
- Final published version: final published article, as it appeared in a journal, conference proceedings, or other formally published context (this version appears here only if allowable under publisher's policy)
Some peer-reviewed scholarly articles are available through other DSpace@MIT collections, such as those for departments, labs, and centers.
If you are an MIT community member who wants to deposit an article into the this collection, you will need to log in to do so. If you don't have an account, please contact us.
More information:
- Working with MIT's open access policy
- Submitting a paper under the policy
- FAQ about the policy
Recent Submissions

Biofilm formation of Pseudomonas aeruginosa in spaceflight is minimized on lubricant impregnated surfaces ï»ż
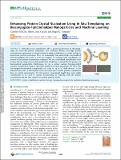
Enhancing Protein Crystal Nucleation Using In Situ Templating on Bioconjugate-Functionalized Nanoparticles and Machine Learning ï»ż
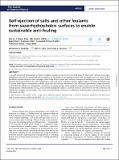
Self-ejection of salts and other foulants from superhydrophobic surfaces to enable sustainable anti-fouling ï»ż

- Search Search
- CN (Chinese)
- DE (German)
- ES (Spanish)
- FR (Français)
- JP (Japanese)
- Open Research
- Booksellers
- Peer Reviewers
- Springer Nature Group â
- Fundamentals of open research
- Gold or Green routes to open research
- Benefits of open research
- Open research timeline
- Whitepapers
- About overview
- Journal pricing FAQs
- Publishing an OA book
- Journals & books overview
- OA article funding
- Article OA funding and policy guidance
- OA book funding
- Book OA funding and policy guidance
- Funding & support overview
- Open access agreements
- Springer Nature journal policies
- APC waivers and discounts
- Springer Nature book policies
- Publication policies overview
Open access journals
The worldâs most significant open access portfolio, we have published over 124,000 open access articles via gold open access across disciplines âfrom the life sciences to the humanities, representing 33% of all springer nature articles in 2020. authors can also publish their article under an open access licence in more than 2,200 of our hybrid journals..
Our portfolio focuses on robust and insightful research, supporting the development of new areas of knowledge and making ideas and information accessible around the globe.
Across our publishing imprints there are leading multidisciplinary and community-focused journals that offer rigorous, high-impact open access. Many of our titles are also published in partnership with academic societies, enabling them to achieve their own open research ambitions.
OA articles published via Gold OA
Hybrid OA journals
Open access books
Fully open access journals
Download a list of our fully open access journals, including APC and licence information.
This list indicates the standard article processing charge (APC) and default licence for each journal. Where CC BY is listed, the default version is CC BY 4.0. APCs are payable for articles upon acceptance. While we make every effort to keep this list updated, please note that APCs are subject to change and may vary from the price listed. For further information on the licences and other currencies available, self-archiving embargoes, manuscript deposition, and abstracting & indexing, visit the individual journalâs website. VAT or local taxes will be added where applicable.
Questions about paying for open access?
View our frequently asked questions about article processing charges (APCs).
Visit our imprint sites
Hybrid journals
Download a list of our hybrid journals, including Springer Open Choice titles. We publish more than 2,200 journals that offer open access at the article level, allowing optional open access in the majority of Springer Nature's subscription-based journals.
This list indicates the standard article processing charge (APC) for each journal. APCs are payable for articles upon acceptance. While we make every effort to keep this list updated, please note that APCs are subject to change and may vary from the price listed. For further information on the licences and other currencies available, self-archiving embargoes, manuscript deposition, and abstracting & indexing, visit the individual journalâs website. VAT or local taxes will be added where applicable.
Find out more by imprint
Springer open choice, springer nature hybrid journals on nature.com, palgrave macmillan hybrid journals, stay up to date.
Here to foster information exchange with the library community
Connect with us on LinkedIn and stay up to date with news and development.
- Tools & Services
- Account Development
- Sales and account contacts
- Professional
- Press office
- Locations & Contact
We are a world leading research, educational and professional publisher. Visit our main website for more information.
- © 2024 Springer Nature
- General terms and conditions
- Your US State Privacy Rights
- Your Privacy Choices / Manage Cookies
- Accessibility
- Legal notice
- Help us to improve this site, send feedback.
Open research in computer science
Spanning networks and communications to security and cryptology to big data, complexity, and analytics, SpringerOpen and BMC publish one of the leading open access portfolios in computer science. Learn about our journals and the research we publish here on this page.
Highly-cited recent articles
Spotlight on.
EPJ Data Science
See how EPJ Data Science brings attention to data science
Reasons to publish in Human-centric Computing and Information Sciences
Download this handy infographic to see all the reasons why Human-centric Computing and Information Sciences is a great place to publish.
We've asked a few of our authors about their experience of publishing with us.
What authors say about publishing in our journals:
Fast, transparent, and fair. - EPJ Data Science Easy submission process through online portal. - Journal of Cloud Computing Patient support and constant reminder at every phase. - Journal of Cloud Computing Quick and relevant. - Journal of Big Data âââââââ
How to Submit Your Manuscript
Your browser needs to have JavaScript enabled to view this video
Computer science blog posts
Read the latest from the SpringerOpen blog
The SpringerOpen blog highlights recent noteworthy research of general interest published in our open access journals.
Failed to load RSS feed.
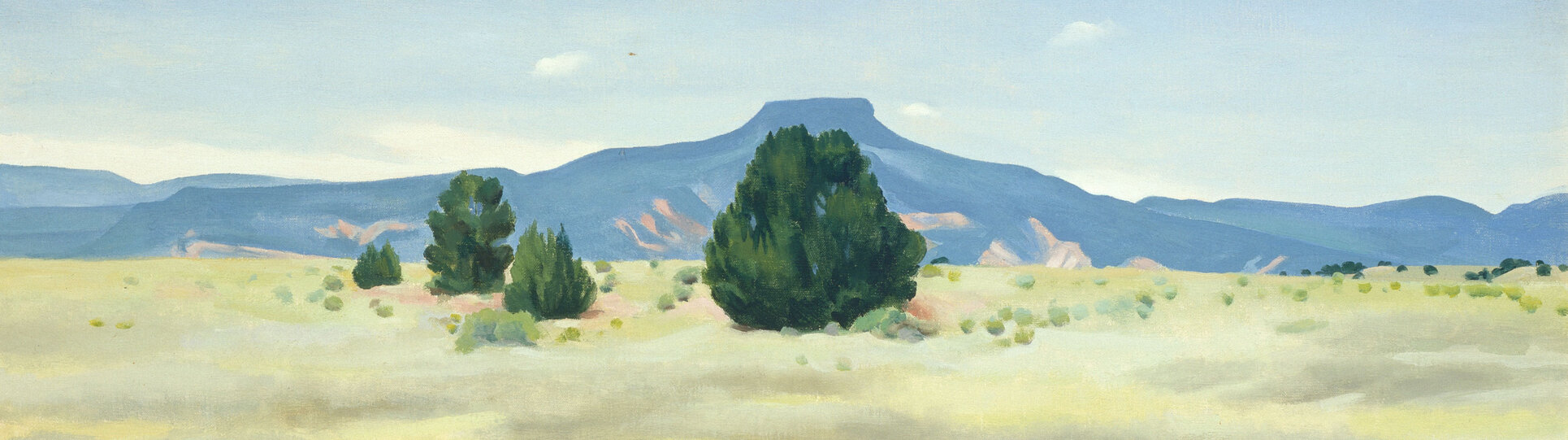
Explore millions of high-quality primary sources and images from around the world, including artworks, maps, photographs, and more.
Explore migration issues through a variety of media types
- Part of The Streets are Talking: Public Forms of Creative Expression from Around the World
- Part of The Journal of Economic Perspectives, Vol. 34, No. 1 (Winter 2020)
- Part of Cato Institute (Aug. 3, 2021)
- Part of University of California Press
- Part of Open: Smithsonian National Museum of African American History & Culture
- Part of Indiana Journal of Global Legal Studies, Vol. 19, No. 1 (Winter 2012)
- Part of R Street Institute (Nov. 1, 2020)
- Part of Leuven University Press
- Part of UN Secretary-General Papers: Ban Ki-moon (2007-2016)
- Part of Perspectives on Terrorism, Vol. 12, No. 4 (August 2018)
- Part of Leveraging Lives: Serbia and Illegal Tunisian Migration to Europe, Carnegie Endowment for International Peace (Mar. 1, 2023)
- Part of UCL Press
Harness the power of visual materials—explore more than 3 million images now on JSTOR.
Enhance your scholarly research with underground newspapers, magazines, and journals.
Explore collections in the arts, sciences, and literature from the worldâs leading museums, archives, and scholars.
open source intelligence Recently Published Documents
Total documents.
- Latest Documents
- Most Cited Documents
- Contributed Authors
- Related Sources
- Related Keywords
The effect of ISO/IEC 27001 standard over open-source intelligence
The Internetâs emergence as a global communication medium has dramatically expanded the volume of content that is freely accessible. Through using this information, open-source intelligence (OSINT) seeks to meet basic intelligence requirements. Although open-source information has historically been synonymous with strategic intelligence, todayâs consumers range from governments to corporations to everyday people. This paper aimed to describe open-source intelligence and to show how to use a few OSINT resources. In this article, OSINT (a combination of public information, social engineering, open-source information, and internet information) was examined to define the present situation further, and suggestions were made as to what could happen in the future. OSINT is gaining prominence, and its application is spreading into different areas. The primary difficulty with OSINT is separating relevant bits from large volumes of details. Thus, this paper proposed and illustrated three OSINT alternatives, demonstrating their existence and distinguishing characteristics. The solution analysis took the form of a presentation evaluation, during which the usage and effects of selected OSINT solutions were reported and observed. The paperâs results demonstrate the breadth and dispersion of OSINT solutions. The mechanism by which OSINT data searches are returned varies greatly between solutions. Combining data from numerous OSINT solutions to produce a detailed summary and interpretation involves work and the use of multiple disjointed solutions, both of which are manual. Visualization of results is anticipated to be a potential theme in the production of OSINT solutions. Individualsâ data search and analysis abilities are another trend worth following, whether to optimize the productivity of currently accessible OSINT solutions or to create more advanced OSINT solutions in the future.
Applications of Open Source Intelligence in Crisis AnalysisâA COVID-19 Case Study
I know where you are going: predicting flight destinations of corporate and state aircraft.
As data of aircraft movements have become freely accessible on a large scale through means of crowdsourcing, their open source intelligence (OSINT) value has been illustrated in many different domains. Potentially sensitive movements of all stakeholders outside commercial aviation are potentially affected, from corporate jets to military and government aircraft. Until now, this OSINT value was shown only on historical data, where automated analysis on flight destinations has been effective to find information on potential mergers & acquisition deals or diplomatic relationships between governments. In practice, obtaining such information as early as possible is crucial. Hence, in this work, we predict the destinations of state and corporate aircraft on live data, while the targets are still in the air. We use machine learning algorithms to predict the area of landing up to 2 h in advance. We evaluate our approach on more than 500,000 flights during 2018 obtained from the OpenSky Network.
Analisis Forensik Konten dan Timestamp pada Aplikasi Tiktok
<p><em>The Tiktok application is one of the social media platform applications that often finds many loopholes to get the identity of the application's users. TikTok has experienced tremendous growth by reaching 1.5 billion users in 2019. This research uses </em><em>an</em><em> Open-Source Intelligence (OSINT) method as a standard in the research phase to reveal the timestamps obtained from the TikTok application. The method used in this research is the National Institute of Standard Technology (NIST). Th</em><em>e</em><em> research uses forensic tools, namely Browser History Capture/Viewer, Video Cache Viewer, Unfurl and Urlebird. The result of this research show</em><em>s </em><em>a complete description of all digital artifacts and timestamps obtained from TikTok content. Furthermore, </em><em>by </em><em>using the results of the analysis in th</em><em>e research</em><em>, it is </em><em>expected</em><em> that </em><em>the research</em><em> can </em><em>help to </em><em>reconstruct the content and </em><em>to </em><em>search for keywords from the timestamp in th</em><em>e</em><em> TikTok application.</em></p>
Meta-analysis of transcriptional regulatory networks for lipid metabolism in neural cells from schizophrenia patients based on an open-source intelligence approach
Open source intelligence.
AbstractOpen Source Intelligence (OSINT) has gained importance in more fields of application than just in intelligence agencies. This paper provides an overview of the fundamental methods used to conduct OSINT investigations and presents different use cases where OSINT techniques are applied. Different models of the information cycle applied to OSINT are addressed. Additionally, the terms data, information, and intelligence are explained and correlated with the intelligence cycle. AÂ classification system for entities during OSINT investigations is introduced. By presenting the capabilities of modern search engines, techniques for research within social networks and for penetration tests, the fundamental methods used for information gathering are explained. Furthermore, possible countermeasures to protect oneâs privacy against the misuse of openly available information as well as the legal environment in Germany, and the ethical perspective are discussed.
Az internetes biztonsĂĄg Ă©s az OSINT összefĂŒggĂ©sei
Az OSINT (Open Source Intelligence) mozaikszĂł mint a nyĂlt forrĂĄsĂș hĂrszerzĂ©s jelentĆsĂ©ge az elmĂșlt Ă©vekben egyre nagyobb szerepet kap az egyes szolgĂĄlatok Ă©letĂ©ben, hiszen a digitĂĄlis informĂĄciĂłk megszerzĂ©se Ă©s birtoklĂĄsa Ășj kihĂvĂĄsok elĂ© ĂĄllĂtja a szakembereket. A legtöbben biztosan hallottuk mĂĄr azt a közhelyet, miszerint âami egyszer felkerĂŒl az internetre, az ott is maradâ. Ezt szem elĆtt tartva kell mindennapi munkĂĄnkat vĂ©geznĂŒnk, hiszen soha nem tudhatjuk, hogy ki Ă©s mikor hasznĂĄlhat fel ellenĂŒnk olyan szemĂ©lyes informĂĄciĂłkat, amelyek megszerzĂ©sĂ©hez mi magunk szolgĂĄltattuk az adatokat valamikor a közel- vagy rĂ©gmĂșltban. Jelen ĂrĂĄsban az OSINT jelenetĆsĂ©gĂ©nek bemutatĂĄsĂĄt követĆen a digitĂĄlis tudatossĂĄg fontossĂĄgĂĄt hangsĂșlyozva rĂĄmutatok nĂ©hĂĄny tipikus gyenge pontra, Ășgymint a gyermekek Ă©s az idĆsebb korosztĂĄly e tĂ©ren hiĂĄnyos ismeretei, az operĂĄciĂłs rendszerek, mĂĄs programok adatgyƱjtĂ©sei, a kiszivĂĄrgott vagy gyƱjtött adatbĂĄzisok jelentette veszĂ©lyek, az adatvesztĂ©s körĂŒlmĂ©nyei Ă©s következmĂ©nyei. KitĂ©rek tovĂĄbbĂĄ az egyre bĆvĂŒlĆ speciĂĄlis adatgyƱjtĆ cĂ©lszoftverekre is. Ezek kapcsĂĄn megoldĂĄsi javaslatokat vĂĄzolok fel, amelyek hasznos segĂtsĂ©get jelenthetnek akĂĄr a prevenciĂłt, akĂĄr a vesztesĂ©gek minimalizĂĄlĂĄsĂĄt illetĆen.
Medien der Forensik
In der kriminaltechnischen Praxis beginnt Forensik materialiter und lokal: mit Spuren am Ereignisort einer Tat. Medien der Forensik operieren als Tatortmedien und formieren Medientatorte. Sie prozessieren Datenspuren und SpurmedialitĂ€ten. In digitalen Medienkulturen ist - im Off offizieller Forensik - eine Konjunktur medienforensischer Semantiken, Verfahren, Praktiken zu beobachten, denen Simon Rothöhler nachgeht: in Alltags- und Popkultur (True Crime), in der Praxis kĂŒnstlerischer Forschung (Forensic Architecture), in zivilgesellschaftlich-investigativen Kontexten (Open Source Intelligence).
Cyber Crime Investigation: Landscape, Challenges, and Future Research Directions
As technology has become pivotal a part of life, it has also become a part of criminal life. Criminals use new technology developments to commit crimes, and investigators must adapt to these changes. Many people have, and will become, victims of cybercrime, making it even more important for investigators to understand current methods used in cyber investigations. The two general categories of cyber investigations are digital forensics and open-source intelligence. Cyber investigations are affecting more than just the investigators. They must determine what tools they need to use based on the information that the tools provide and how effectively the tools and methods work. Tools are any application or device used by investigators, while methods are the process or technique of using a tool. This survey compares the most common methods available to investigators to determine what kind of evidence the methods provide, and which of them are the most effective. To accomplish this, the survey establishes criteria for comparison and conducts an analysis of the tools in both mobile digital forensic and open-source intelligence investigations. We found that there is no single tool or method that can gather all the evidence that investigators require. Many of the tools must be combined to be most effective. However, there are some tools that are more useful than others. Out of all the methods used in mobile digital forensics, logical extraction and hex dumps are the most effective and least likely to cause damage to the data. Among those tools used in open-source intelligence, natural language processing has more applications and uses than any of the other options.
Open-Source Intelligence as the New Introduction in the Graduate Cybersecurity Curriculum
Export citation format, share document.
Help | Advanced Search
Computer Science > Robotics
Title: robocar: a rapidly deployable open-source platform for autonomous driving research.
Abstract: This paper introduces RoboCar, an open-source research platform for autonomous driving developed at the University of Luxembourg. RoboCar provides a modular, cost-effective framework for the development of experimental Autonomous Driving Systems (ADS), utilizing the 2018 KIA Soul EV. The platform integrates a robust hardware and software architecture that aligns with the vehicle's existing systems, minimizing the need for extensive modifications. It supports various autonomous driving functions and has undergone real-world testing on public roads in Luxembourg City. This paper outlines the platform's architecture, integration challenges, and initial test results, offering insights into its application in advancing autonomous driving research. RoboCar is available to anyone at this https URL and is released under an open-source MIT license.
Submission history
Access paper:.
- Other Formats
References & Citations
- Google Scholar
- Semantic Scholar
BibTeX formatted citation

Bibliographic and Citation Tools
Code, data and media associated with this article, recommenders and search tools.
- Institution
arXivLabs: experimental projects with community collaborators
arXivLabs is a framework that allows collaborators to develop and share new arXiv features directly on our website.
Both individuals and organizations that work with arXivLabs have embraced and accepted our values of openness, community, excellence, and user data privacy. arXiv is committed to these values and only works with partners that adhere to them.
Have an idea for a project that will add value for arXiv's community? Learn more about arXivLabs .
Open Innovation in Schools: A New Imperative for Organising Innovation in Education?
- Original research
- Open access
- Published: 27 November 2023
Cite this article
You have full access to this open access article
- Marcus Pietsch  ORCID: orcid.org/0000-0002-9836-6793 1 ,
- Colin Cramer  ORCID: orcid.org/0000-0003-3720-9708 2 ,
- Chris Brown  ORCID: orcid.org/0000-0002-9759-9624 3 ,
- Burak Aydin  ORCID: orcid.org/0000-0003-4462-1784 1 , 4 &
- Jasmin Witthöft  ORCID: orcid.org/0000-0003-3578-0230 1 Â
1672 Accesses
1 Altmetric
Explore all metrics
Schools are considered knowledge-creating organisations that find it difficult to develop and implement innovations on their own. Knowledge mobilisation is seen as the key to overcoming this problem. In particular, the use of external sources of knowledge is regarded as an important lever for change. However, there is a lack of concepts and empirical studies in educational research on the extent to which external knowledge is used for innovation in schools. Based on a sample of Nâ=â411 schools, this article explores whether the concept of open innovation can be used in the context of education. Originating from the field of innovation research, open innovation regimes are seen as imperative if organisations are to create and benefit from technology. Multinomial logistic regression analyses show that mobilising external knowledge significantly increases the likelihood of implementing innovations in schools. A machine-learning approach reveals that it is necessary to tailor open innovation regimes to the specific conditions of any given school. In particular, with regard to the use of new technologies and innovations in the field of digitalisation, open innovation can be a lever for change.
Similar content being viewed by others
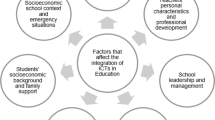
Impacts of digital technologies on education and factors influencing schools' digital capacity and transformation: A literature review
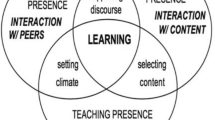
Investigating blended learning interactions in Philippine schools through the community of inquiry framework
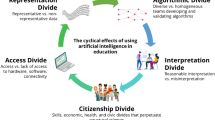
The cyclical ethical effects of using artificial intelligence in education
Avoid common mistakes on your manuscript.
1 Introduction
In the face of current crises and to keep up with social and technological developments, schools are now, more than ever, required to implement innovations, some of which are long overdue (Brown & Luzmore, 2021 ; Schwabsky et al., 2020 ; Serdyukov, 2017 ; Tan et al., 2021 ). Innovations can lead to changes in school structure and functioning (Damanpour, 1988 ), are closely linked with experimentation and finding new approaches and ideas for educating children (Lubienski & Perry, 2019 ) and are ultimately enacted at the classroom level (Vincent-Lancrin et al., 2019 ). However, although innovation is seen as important for school effectiveness and countries around the world invest a lot of money in it, there are only a few empirical studies on innovation in education. Furthermore, the findings from this scant evidence base are inconclusive (Schwabsky et al., 2020 ; Serdyukov, 2017 ; Zimmer et al., 2017 ).
Due to the lack of adequate measurement models, little is known about what concrete innovations schools implement (Vincent-Lancrin et al., 2014 , 2019 ). Accordingly, Serdyukov ( 2017 ), looking at one of the largest studies on this topic to date (Vincent-Lancrin et al., 2014 ), concludes that the list of innovations selected and reported in it is disappointingly unimpressive. In addition, little is known about which conditions in schools are conducive to successful innovation (Schwabsky et al., 2020 ), and there are too few empirical studies that examine (strategic) innovation and related knowledge management in schools (Cheng, 2021 ): that is, practices by which schools incorporate and coordinate the generation, dissemination and application of knowledge for innovating teaching and learning (Cordeiro et al., 2022 ).
Outside of educational research, in organisational and innovation research, however, the concept of open innovation has been considered imperative for organising innovation (Bogers et al., 2019 ) and especially for creating and profiting from technology (Chesbrough, 2003a ). Open innovation theory suggests that organisations can and should use both external and internal knowledge to drive their innovation efforts and is seen as a lever to improve innovation performance (Bigliardi et al., 2020 ; Bogers et al., 2019 ; Chesbrough, 2003a ). In particular, when the organisation of innovation processes is tailored to the specific needs of an organisation (Chesbrough, 2003a , 2012 ). Although, the concept of open innovation is used in the context of public administration (De Connick et al., 2021 ; Kankanhalli et al., 2017 ), it has hardly, to date, been applied in educational research and practice (Pietsch et al., 2023a ).
A principal reason for this lack of application is that research on innovation in education itself is, on the one hand, still in its infancy (Vincent-Lacarin et al., 2014 ). Allied to this nascent state of the art, is that such research is also rarely linked to other, more mainstream research, on innovation (HalĂĄsz, 2018 ). At the same time, however, the definition of innovation in education is more or less similar to that of other organisations (Schwabsky et al., 2020 ); and it has long been assumed in educational research that schools improve and change when they succeed in using newly created and acquired knowledge as a basis for changes to the beliefs, understanding and actions of all those involved in a school (Frost, 2012 ; Hanson, 2001 ). Nevertheless, introducing innovation in schools is considered a complex undertaking that requires a broad mobilisation of knowledge (Greany, 2018 ), as well as the active involvement and support of all stakeholders, from policymakers to learners (Serdyukov, 2017 ). Consequently, sourcing and sharing knowledge within schools as well as with outside people, communities and/or organisations is therefore seen as particularly important, especially for the implementation of sustainable innovation (Prenger et al., 2022 ).
Against this background, our article examines whether the concept of open innovation is transferable to educational research and whether and to what extent, open innovation regimes can lead to innovation outcomes in schools. More specifically, we examine whether and the extent to which the use of internal and external education-related knowledge is associated with different innovations in schools. For this purpose, we use a random sample of schools ( N â=â411) from Germany and estimate latent multinomial logistic regression models as well as apply a machine-learning approach. Herein, our study aims to fill the mentioned research gap by addressing the following research questions:
Do schools incorporate external knowledge for internal innovation?
Does externally mobilised knowledge (open innovation) increase the likelihood of innovations being introduced in schools compared to knowledge mobilisation within schools (closed innovation)?
Do closed and open innovation mechanisms interact with school-specific innovation conditions and contexts?
2 Innovation in Schools
Innovation in education is crucial for promoting improvements and sustainable development in schools (Nguyen et al., 2021 ). However, innovation is a multifaceted term that might attract a wide range of meaning and implications (Nicholls, 2018 ). In general, however, innovation can be described as the intentional emergence and implementation of new ideas, processes and solutions that imply both purposefulness and novelty (Damanpour, 1988 , 1991 ; Rogers, 1995 ). In the context of education, innovation (as an outcome) is considered a subset of public sector innovation (OECD, 2009 ) and defined as âa new or improved product or process (or combination thereof) that differs significantly from the unitâs previous products or processes and that has been made available to potential users (product) or brought into use by the unit (process)â (OECD & Eurostat, 2018 , p. 60; see also Vincent-Lancrin et al., 2014 , 2019 ).
According to Goldenbaum ( 2012 , p. 81), innovations in the school context can be characterized more precisely as the following: âInnovations (âŠ) tend to be relatively new, targeted, intentional, and planned measures that bring about changes or improvements in the school education system (macro-level), in the individual school (meso-level) and/ or in the classroom or social interactions (micro-level)â. In short, this mean: Innovations in the context of schooling can be implemented at different levels of an education system and always involve at least the following three aspects: 1. they are fundamental in nature; 2. they are intentional and planned; and 3. there is an intention to improve or change (Nicholls, 2018 ).
In contrary to the business sector, innovation in the public service sector and thus in schools, is rarely driven by the pursuit of financial growth. The motivation to innovate arises from different sources that are, in most parts, connected with cultural, societal, or political changes and transitions (Goldenbaum, 2012 ): with main drivers of innovation in schools often being local competition between institutions and the regressive effects of large-scale, standardized reform strategies (Sahlberg, 2016 ). In addition, there are other external driving forces requiring schools or whole education systems to innovate, i.e., disruptive changes of educational environments like the COVID-19 pandemic (Pietsch et al., ), natural catastrophes and disasters (Brown & Luzmore, 2021 ), or man-made wars (and their consequences) (Kruszewska & Lavrenova, 2022 ).
However, as Tyack and Tobinâs ( 1994 ) findings demonstrate, implementing innovation in schools is difficult because such institutions have a grammar of schooling in relation to long-standing structures (e.g. subject-based teaching, age-based classes, fixed timetables) that influence many aspects of teaching and absorbs innovative efforts. As research shows, the reasons for this are many. For instance, the structural characteristics of schools are limiting, with schools serving multiple constituents, making changes hard to plan and predict. Further, schools are responsible for passing down civic and cultural knowledge and thus have a certain obligation to preserve the past (Brown & Luzmore, 2021 ; (Pietsch et al., 2023a , 2023b ); Tye, 2000 ). Accordingly, even after innovations are introduced, changes in schools often regress after a while, with stakeholders and organisations returning to former behaviors and structures (Hopkins, 2013 ). As a result, schools tend towards the maintenance of stability by adopting incremental changes (Cuban, 2020 ), making it difficult for them to either innovate bottom-up, on their own, or integrate more radical top-down innovations (Elmore, 1996 ; OECD, 2015 ). Thus, successful innovation in schools requires a continuous and complex mobilisation of knowledge (Greany, 2018 ; Prenger et al., 2022 ) and the integration of this knowledge into existing organisational structures and routines (Daâas et al., 2020 ; Tappel et al., 2023 ).
3 Closed and Open Innovation
As school autonomy has increased worldwide (Hanushek et al., 2013 ; Hargreaves & Shirley, 2012 ), schools are increasingly expected to become innovative and effective as, according to the underlying assumptions of this approach, they were freed from bureaucracy whilst also being incentivised to compete with other schools for the best ideas and outcomes (Greany & Waterhouse, 2016 ; Preston et al., 2012 ). As a result, decision-making power has been increasingly shifted to schools, with an emphasis placed on teaching and learning, and a new focus established on school capacity building (Honig & Rainey, 2012 ). A key assumption here is that schools are knowledge creating organisations (Hargreaves, 1999 ) and so should (be expected to) develop innovative professional practices and processes internally (McCharen et al., 2011 ). As a consequence, as Hargreaves ( 1999 , p. 125) argues, âschools which create professional knowledge are likely to display characteristics similar to those of high-technology firms that are demonstrably successful in knowledge creation in response to the dual demand for higher R&D [Research and Development] productivity and shorter development lead times.â
Outside of educational research, Chesbrough ( 2003b ) refers to such an innovation paradigm as closed innovation and argues that innovation processes in organisations that follow such an approach tend to be inward-oriented and strictly focused on progress and success. In this regard, the closed innovation model assumes that all knowledge providing the basis for innovation and change both can be and is produced internally by an organisation (Marques, 2014 ). Consequently, in this view, successful innovation requires control (Chesbrough, 2003b ) and can only develop within organisations in a linear way, with scant regard for external expertise; subsequently leaving a majority of ideas unexploited (Chesbrough, 2012 ). Simultaneously, however, research indicates that such an approach to innovation is unable to cope with fast-changing and globally connected environments, meaning that closed and linear innovation models alone cannot be successful in the long run (Bigliardi et al., 2020 ). This argument also applies to schools, which often lack the capability and/or resources to change and innovate on their own (Bryck, 2010 ; Slavin, 2005 ).
Conversely, open innovation is seen as a key driver that helps organisations to overcome such constraints and to innovate in a dynamically evolving environment despite their own limited resources (Chesbrough, 2003a ). The paradigm of open innovation is defined as âa distributed innovation process based on purposively managed knowledge flows across organizational boundaries, using pecuniary and non-pecuniary mechanisms in line with the organization's business modelâ (Chesbrough & Bogers, 2014 , p. 17). This paradigm highlights the importance of open and cooperative research strategies which target both in and outflows of knowledge to accelerate internal innovation. According to the open innovation paradigm, different ways of inventing new ideas and technologies exist. Either they result from internal knowledge and need external paths to market, or they develop through external knowledge using internal paths to become successful (Chesbrough, 2006 ). Organisations must be open to external inputs, ideas, and contributors and allow internal knowledge to go beyond organisational boundaries to realise their full potential (Chesbrough, 2012 ). Inbound open innovation (integrating external knowledge) is the first level of open innovation, which enriches internal knowledge by integrating knowledge from external sources due to collaboration. This phase requires opening up processes and allowing innovation to develop beyond organisational boundaries. The second step is outbound open innovation (disseminating internal knowledge) involving the transfer of internal knowledge outside the organization and offering excellent chances for exchange and cooperation with other organizations. Further, it supports the main idea of open innovation that new ideas and expert knowledge do not emerge within closed organisational structures. The third and last phase is the coupled process (combining inbound and outbound mechanisms) which links inbound and outbound open innovation to develop shared values, strategic alliances, and networks between organizations (Gassmann et al., 2010 ; Pietsch et al., 2023a ).
At the same time, however, both theory and empirical evidence indicate that for open innovation to be effective and achieve innovation outcomes, open innovation mechanisms must be fully aligned with an organisation's business model (Chesbrough, 2003a , 2012 ; West & Bogers, 2014 ). In other words, aligned with the description of an organisation and how it functions to achieve its goals (Massa et al, 2017 ): particularly in relation to: (i) configuration of knowledge co-creation within an organsisation; (ii) the permeability of knowledge flows across and within organisational boundaries; and (iii) the degree of collaboration with external knowledge providers (RamĂrez-Montoya et al., 2022 ; Saebi & Foss, 2015 ). Furthermore, any such alignment will also vary with an organisation's internal and external contextual characteristics (Huizingh, 2011 ).
4.1 Study Context
Against this background, our study focusses on the differential effects of closed and open innovation in schools on various innovation outcomes. The context of this study is Germany, a nation comprising 16 federal states that are fully responsible for their individual school system. Despite these differences, students across all 16 states attend primary schools from the age of 6 to at least 10Â years. Subsequently, however, students, once reaching fifth grade (at age 11) progress into a highly-structured and differentiated secondary school system. Nonetehless the traditional division into three school types ( Hauptschule, Realschule and Gymnasium ) has been successively reformed in most federal states ( LĂ€nder ) and in many places various forms of comprehensive schools have also been introduced. Yet, at the same time, innovation across all schools implemented very slowly in Germany, however, especially in the area of digitalisation. For example, in 2018, just before the start of the COVID-19 pandemic, only about 3.2% of schools in Germany had equipped all teachers with mobile devices (for comparison, the EU average was 25.9%; Eickelmann et al., 2019 ).
The database of our study is drawn from the third wave of the Leadership in German Schools (LineS) study. Data was collected between August and November 2021 across Germany. The longitudinal study surveyed a random sample of school leaders that is representative of Germany in each of the measurement waves (Cramer et al., 2021 ; Dedering & Pietsch, 2023 ; Röhl et al., 2022 ). Besides recurrent topics that are surveyed in every wave, specific topics are also used to highlight a subject focus in every single wave. The emphasis of the 2021 survey was on (open) innovation in schools. The forsa Institute for Social Research and Statistical Analysis , a leading survey and polling company in Germany, collected the data as a field service provider. Participants were recruited via its omnibus and omninet panels: here, a random sample of around 1,000 people aged 14 and above is interviewed on a mixed-topic daily basis, with questions also asking on the current occupation. Thus, school leaders ( N â=â411) can be identified on a random basis, leading to a nationally representative sample for general schools in Germany. Participants received personalised access to an online questionnaire, hosted also by forsa. Of these, N â=â103 school leaders already participated in the previous two waves, and an additional refreshment sample of N â=â308 (Watson & Lynn, 2021 ) was surveyed. In our analysis we use cross-sectional data from all these sub-samples since data on open innovation has only been collected in this wave so far.
Of our sample, N â=â247 (63.3%) of the school leaders surveyed were female and Nâ=â163 (36.7%) male. The average age was 52.9Â years, with a standard deviation of 6.9Â years. Respondents had been working as school leaders for an average of 9.5Â years at the time of the survey. N â=â35 (8.5%) of respondents work in private schools, N â=â374 (91.0%) work in public schools, while N â=â2 (0.5%) did not respond to this question. Of the schools they lead, 63 (15.3%) are located in a village, hamlet or rural area (population less than 3,000); 106 (25.8%) are located in a small town (population 3,000 to approximately 15,000); 141 (34.3%) are located in a small city (population 15,000 to approximately 100,000); 75 (18.2%) are located in a medium size city (population 100,000 to approximately 1 million); and 25 (6.1%) are located in a large city (population over 1 million). It should be noted that, for one school, contextual data were missing.
In order to be able to present the school form in an internationally comprehensible way, we report education levels according to the International Standard Classification of Education (ISCED; UNESCO Institute for Statistics, 2012). ISCED classifies education systems according to uniform criteria: ISCED 1 refers to âprimary educationâ and covers the first to fourth school years in Germany; ISCED 2 refers to âlower secondary educationâ and covers the fifth to the tenth school years; while ISCED 3 refers to âhigher secondary educationâ and covers the eleventh to thirteenth school years. Within our sample, therefore: 53.2% are primary, 38.8% are secondary, 8.0% are other schools, including 2.0% special needs schools. On average, 381 students were enrolled in the participantsâ schools, with a standard deviation of 316 and a 5 to 95th percentile range of 60 to 1,027 students.
4.3 Measures
The questionnaire we employed for the survey comprised 35 item blocks. In addition to the items and scales relevant to the study, these include, for example, standardized constructs on self-efficacy (Röhl et al., 2022 ), stress experience, ambidexterity (Dedering & Pietsch, 2023 ; Pietsch et al., 2023a , 2023b ), and career choice and turnover intentions of school leaders (Cramer et al., 2021 ). For this reason, we use only a selection of items and scales in our study. That is, those that are relevant to answering our research questions. To minimise common method bias (Podsakoff et al., 2012 ), measures were taken, both in the instrumentâs design and in data collection. For example, item wording and scale properties varied across scales, and both item blocks and individual items within blocks were rotated and scrambled randomly across individual surveys. We used the following variables as part of our study (also see â Appendix â):
Innovations , our dependent variable, were measured by adapting items from the European Community Innovation Survey (CIS; Behrens et al., 2017 ), which is based on the Organisation for Economic Co-operation and Developmentâs (OECD/Eurostat, 2018 ) Oslo guidelines for collecting, reporting and using data on innovation. Accordingly, we treat innovation as something that is new for a school, but not necessarily new for the entire education sector. In a first step, school leaders were asked whether innovations in pedagogical work, i.e., teaching and instruction, had been introduced at their school in the past 12Â months, using a binary-coded item (0â=âno, were not introduced, 1â=âyes, were introduced). Specifically the wording of this question was:
Have any process innovations, i.e., innovations or noticeable changes that affect the pedagogical work of the school, been introduced at your school in the last 12Â months?
If school leaders answered yes to this question, as a second step they were asked to indicate up to three of the most important innovations in the past 12Â months, using free-form fields that were ranked by importance. Related to these statements, they then, in a third step, were required to justify, again in free-form fields, why they considered these innovations important and, as a fourth step, rate how radical these are for their school on a scale ranging from 1 (incremental innovationsâimproving and/or supplementing and/or adapting what already exists) to 10 (radical innovationsâintroducing something completely new).
According to the data provided by school leaders, 78.8 percent of schools introduced innovations affecting teaching and instruction in the past 12Â months; 19.2 percent of schools, however, did not introduce innovations; with no data on innovations were provided by two percent of school leaders. These innovations were seen by school leaders as comparatively radical for their schools ( M â=â6.31, SD â=â2.73). For our analyses, the open-ended responses of what school leaders perceived as the most important innovation for their school during the last 12Â months were coded and grouped into the following five categories: (a) innovating digital teaching and learning (64%), i.e., increases in the (appropriate) use of digital media in classroom; (b) innovating traditional teaching and learning (10%), i.e., the development of new, creative task formats; (c) innovating digitalisation (4%), i.e. the introduction of digital devices and software; (d) innovating social interaction (7%), i.e., the introduction of school-based parental involvement, and (e) other innovations (15%), e.g. the introduction of vocational orientation. Exemplary answers are provided in Table 1 .
Open innovation was measured following Laursen and Salter ( 2006 ) and thus refers to inbound open innovation. Hence, it considers the diversity of external knowledge sources for innovation, i.e. open innovation breadth, and the intensity of use of those sources, i.e. open innovation depth. To capture a schoolsâ open innovation orientation, school leaders were asked where the external knowledge for innovations for teaching, that were introduced in the last 12 months in their schools, came from (base question: âNow we would like to know where the knowledge came from for pedagogical innovations, i.e. teaching and instruction, introduced at your school in the last 12 months.â). In total, there were eight different response options (item stem: âThe knowledge we used for the innovations came fromâŠâ), (a) parents, guardians, (b) other schools, (c) authorities, state institutes, (d) universities and other scientific institutions, (e) independent school-improvement consultants, (f) commercial companies, (g) professional trainings and/or conventions, and (h) professional literature. All items were measured on a six-point scale ranging from ânot at allâ to âto an exceptionally high degreeâ. Open innovation depth represents the mean of those items. The open innovation depth scaleâs internal consistency, reported as McDonaldâs omega (1999), was Ï â=â0.76. These items were also used to determine open innovation breadth by first coding the ânot at allâ category as zero and the other five categories as one, and then summing the number of all external knowledge sources and dividing by eight. This index of open innovation breadth thus has a minimum of zero (no sources of external innovation used) and a maximum of one (all possible sources of external innovation used). The open innovation breadth scaleâs internal consistency was Ï â=â0.80.
Closed innovation refers to the amount of internal knowledge a school used for generating, developing and implementing pedagogical innovations in teaching and instruction during the 12 recent months and was captured with one item. Accordingly, school leader respondents were asked to what degree the knowledge for pedagogical innovations at the school came from within the school itself or from the schoolâs teachers (base question: âNow we would like to know where the knowledge came from for pedagogical innovations, i.e. teaching and instruction, introduced at your school in the last 12Â months.â, item: âThe knowledge we used for the innovations came from the school itself/ the teachers of our school.â). The item was measured on a six-point scale ranging from ânot at allâ to âto an exceptionally high degreeâ.
As open innovation theory suggests that open innovation systems need to be aligned with an organisation's business model and tailored to the specific conditions and structural characteristics of an organisation (Chesbrough, 2003a , 2012 ), following Becker ( 2005 ), Bernerth et al. ( 2018 ) and Spector and Brannick ( 2011 ), we also included control variables in our model. These were: school size or type, for which there is evidence of a relationship with innovation and change in education (Haelermans & Blank, 2012 ; Luyten et al, 2014 ); as well as variables related to the innovation orientation within schools (that is, innovation conditions and school leadership). Specifically, our innovation conditions include scales on innovation climate, teacher innovativeness and school leaders' innovation networking activities. These constructs were surveyed by adapting scales from Popa et al. ( 2017 ), OECD ( 2019 ) as well as from Slavec Gomezel and Rangus ( 2019 ). Further detail on each is provided below.
The first of these innovation conditions, innovation climate ( Ï â=â0.80) comprised three items measured on a five-point scale ranging from âneverâ to âvery oftenâ. An example item is: âOur school provides time and resources for teachers to generate, share/exchange, and experiment with innovative ideas/solutionsâ. Teacher innovativeness ( Ï â=â0.88) comprised four items that could be rated on a four-point scale, ranging from âtotally disagreeâ to âtotally agreeâ. (example item: âMost teachers in this school strive to develop new ideas for teaching and learningâ). School leadersâ innovation networking activities , were measured by asking school leaders how many hours per week on average they did spent in the last 12Â months maintaining existing external contacts (e.g., face-to-face, e-mail, telephone, video conference) with people with whom they discuss strategic school matters (e.g., finance, school improvement, innovations). This was an open-ended question, so school leaders could enter numbers ranging from zero to 99Â h per week.
School leadership was measured following Pietsch et al. ( 2019 ) and thus intending to capture leadership for learning, a blend of âinstructional leadership, transformational leadership, and shared leadershipâ (Hallinger, 2011 , p. 126). All leadership items were measured on a four-point scale ranging from âvery rarely or neverâ to âvery oftenâ. Instructional leadership ( Ï â=â0.75) was measured using two items from the Programme for International Student Assessment (PISA, OECD, 2014 ) (example item: âI ensure that teachers work according to the schoolâs educational goalsâ). Transformational leadership (Ïâ=â0.77) was captured with four items from the Multifactor Leadership Questionnaire (MLQ, Bass et al., 1995 ), indicating idealized influence, inspirational motivation, intellectual stimulation, and individualized consideration (example item: âI seek different perspectives when solving problemsâ). Shared leadership was measured with one item (for a discussion on single item measures, see: Allen et al., 2022 ) from the Teaching and Learning International Survey (TALIS; OECD, 2019 ) (example item:âI provide staff with opportunities to participate in school decision-makingâ).
4.4 Analytical Strategy
As a first step in investigating the effects of open and closed innovation practices on different types of educational innovation in schools, we constructed latent multinomial logistic regression models in M plus 8.4 (Muthén & Muthén, 2017 ). The reference group comprised of schools where, according to the surveyed school leaders, no innovations in teaching and instruction had been introduced in the last 12 months. Prior to analysis we standardised all continuous predictor variables with a mean of 0 and a standard deviation of 1, so that a one-unit change in the standardised predictor is actually a standard deviation change in the original predictor variable. Accordingly, the relevance of these variables, even if originally measured on different metrics, can be directly compared.
We report unstandardised beta coefficients and odd-ratios (OR), that compare each innovation type group: innovating digital teaching and learning; innovating traditional teaching and learning; innovating digitalisation; innovating social interaction; and other innovationsâto the reference group of schools without innovations with respect to the reported predictors. Here, an OR of 1 indicates no effect, whereas an OR above 1 represents a positive effect, and a value below 1 indicates a negative effect (Hosmer et al., 2013 ). For example, an OR of 2 for a predictor means that it doubles the odds that the innovation under study will be introduced compared to no innovation being introduced. With an OR of 0.5, the opposite is true: the predictor reduces the odds of introducing the innovation under study by half compared to introducing no innovation.
Starting from a base model (Model 1), which only includes traditional closed innovation practices as a predictor, we successively include further sets of predictors. Model 2 adds open innovation breadth and depth. Model 3 additionally introduces control variables relevant to innovation within the school, i.e. leadership, innovation climate, etc., and contextual characteristics of the schools. Due to the small number of cases of other schools, we modelled school type a dichotomous variableânot ISCED 1 versus ISCED 1 (coded 0/1)âin our analyses.
To investigate interactions between innovation mechanisms, school conditions and contexts for innovation, and thus answering our third research question, we applied a classification and regression tree (CART) procedure (Breiman et al., 1984 ) using R . This machine learning approach was utilized to predict the innovation type with the same predictors included in Model 3 of the logistic regression model. The rpart function with its default settings (Therneau & Atkinson, 2014 ) was chosen to implement the algorithm that divides data into subsets based on the predictive power of independent variables. Thus, in terms of prediction accuracy, CART is expected to outperform multinomial logistic regression due to its robustness for outliers and non-linear relations, for example interactions among independent variables. CART is also expected to perform similarly when the associations between study variables are not complex. Another advantage of CART is that it conveniently handles missing data given that any observation with a non-missing value for the dependent variable and at least one predictor is not discarded. Hence, a successful CART implementation can serve as a sensitivity analysis and can reveal additional predictive information.
As the amount of missing data was low (2.1%), we used a full information maximum likelihood (FIML) procedure for handling missing data. Since the data for our study were collected from a single instrument, we also tested for the possibility of a common method bias using Harmanâs single-factor test (Harman, 1960 ). This test investigates whether a single factor or a general factor emerges to explain the majority of the covariance in the independent and dependent variables of an empirical study. Accordingly, we loaded all items in our study in an un-rotated exploratory factor analysis to see whether a single factor emerges or whether a general factor accounts for much of the covariance between the measured variables. This analysis evidenced that 18.6 percent of the covariance between the items under study could be explained by a single factor, far below the cut-off value of 50 percent (Lance et al., 2010 ), indicating a low likelihood of common method bias.
5.1 Descriptive Statistics, Correlations and Univariate Analyses
Table 2 presents the means ( M ), standard deviations ( SD ) and correlations of our within-school study variables. Regarding RQ1, it appears that the schools in our sample for instructional innovation derive much more knowledge from closed innovation ( M â=â4.45) than from open innovation ( M â=â2.39) processes ( W (1)â=â992.587, p â<â0.001). Results further show that school leaders' innovation network closeness is not statistically significantly correlated with any of the other model variables and that significant correlations with all other model variables can be demonstrated for teachers' innovativeness, i.e. teachersâ receptivity, openness and willingness to adopt change (Buske, 2018 ; Fullan, 2015 ).
According to the school leaders surveyed, the external knowledge for internal innovations in schools came primarily from professional trainings and conferences ( M â=â3.50), followed by knowledge flowing in from other schools ( M â=â2.97) and knowledge stemming from relevant professional literature ( M â=â2.64). On the other hand, little external knowledge for internal innovation came from government agencies ( M â=â2.29), universities ( M â=â2.09), and parents ( M â=â2.09). The knowledge of commercial companies ( M â=â1.79) and independent school consultants ( M â=â1.66) was almost not used at all to introduce pedagogical innovations in schools.
5.2 Multinomial Logistic Regressions
To answer RQ2, we investigated the impact of closed innovation practices in schools on innovation outcomes by applying multinomial logistic regressions, with âno innovationâ in teaching and instruction during the last 12Â months being the reference (see Tables 3 , 4 , 5 ). Results of model 1 indicate that closed innovation practices in schools, hence, using internal available knowledge for pedagogical changes, is positively associated with both digital (ORâ=â2.042, p â<â0.001) and traditional teaching and learning (ORâ=â1.719, p â<â0.05) as well as with innovating digitalisation (ORâ=â2.340, p â<â0.05), innovating social interactions (ORâ=â2.558, p â<â0.05), and other relevant innovations, such as the introduction of vocational orientation or strengthening social work in schools (ORâ=â1.874, p â<â0.001). Thus, if schools take advantage of teachersâ internal knowledge, this increases the odds that such innovations will be introduced by about 100 percent, i.e., doubling the probability that such an event will occur.
Subsequently, we added open innovation measures to our analysis. Model 2 demonstrates the mixed effects of open innovation in schools. First, positive effects of closed innovation processes for innovations in schools can still be observed, even if open innovation processes are considered in the model. Second, we see that the effects of incorporating external knowledge for innovation in schools, i.e., innovation depth, are disproportionately larger with regards to innovations in digital teaching and learning, since we can observe significant correlations of open innovation depth with innovations in digital (ORâ=â4.568, p â<â0.001) as well as in traditional teaching and learning (ORâ=â8.603 p â<â0.001) in schools. Thus, when schools use a lot of external knowledge to innovate internally, the likelihood of introducing such innovations increases by five to eight times. We find an even stronger effect of open innovation depth for the digitalisation of schools (ORâ=â11.597, p â<â0.001). However, the diversity of knowledge sources, i.e. open innovation breadth, has a negative effect on all reported innovations (all p â<â0.05), with the exception of the social interaction innovation ( p â>â0.05). This is particularly noticeable with regard to innovations in traditional teaching and learning, such as the introduction of individualised learning or peer teaching within the classroom. Here, open innovation breadth is associated with an OR of 0.141 ( p â<â0.001), meaning that a school is more than 85 percent less likely to innovate in traditional teaching and learning if it draws on as many external sources of knowledge as possible.
In the final Model 3, in addition to closed and open innovation mechanisms, we also consider within-school innovation characteristics as well as school contextual conditions as control variables. This does not fundamentally change the relationship between innovation in schools and closed and open innovation mechanisms. However, two things stand out: first, controlling for covariates in the model, we no longer find both a significant effect for the influence of closed innovation mechanisms on digital innovations and an effect of open innovation breadth on the innovation of social interactions in schools (both effects p â>â0.05). In general, therefore, model 3 also shows that the intensity of knowledge inflow in schools, i.e. open innovation depth, has a far greater effect on teaching and learning related innovations in schools than closed innovation processes. With regard to digitalisation in schools and innovations in the area of social interaction, it is even exclusively open innovation that plays a demonstrable role in whether or not corresponding innovations are subsequently introduced at schools. But here, too, it is evident that it is not the diversity, i.e. open innovation breadth, but the quantity of knowledge, i.e. innovation depth, that has a positive influence, even when we control for school innovations and contextual features.
5.3 Classification and Regression Tree Analysis (CART)
To answer RQ3, we further examined interactions between our predictors and their joint effects on innovation in schools. As even âvalid controls are possibly endogenous and therefore represent a combination of several different causal mechanismsâ (HĂŒnermund & Louw, 2020 , p. 1) we investigated potential interactions of predictor variables by applying a machine learning approach in a final step, called CART. Unlike logistic regression, this approach does not develop a prediction equation, but explores the data set by partitioning the data along the predictor axes into subsets with homogeneous values of the dependent variable, allowing for multiple interactions between the predictor variables (Krzywinski & Altman, 2017 ).
Multinomial CART results indicate that eight out of 12 predictors have importance to predict the type of innovation with the following weights: open innovation depth 26%, closed innovation 22%, school size 19%, open innovation breadth 18%, ISCED 6%, teacher innovativeness 4%, transformational leadership 2% and innovation climate 2%. CART results are depicted in Fig. 1 using the R package rattle (Williams, 2011 ), so illustrating the interactions among variables to predict innovation type as frequencies reported in each node. For example, the first node, before partitioning, reports 208 as the frequency for innovating digital teaching and learning (type-1); 31 for innovating traditional teaching and learning (type-2); 12 for innovating digitalisation (type-3); 23 for innovating social interaction (type-4); 50 for other innovations (type-5), and 87 for no innovation (type-6). The header in each node shows the highest frequency, for example 1 for the first node. Results show that open innovation is the strongest predictor for teaching-related innovations in schools. It is striking, however, that closed innovation is the decisive predictor when it comes to the implementation of innovations in schools in general since all other predictors depend on closed innovation or interact with the closed innovation mechanisms of schools. It is also striking that an above-average use of closed innovation is used in schools that are not particularly small (less than one standard deviation below the mean) and that open innovation depth comes into play especially when closed innovation is below average.
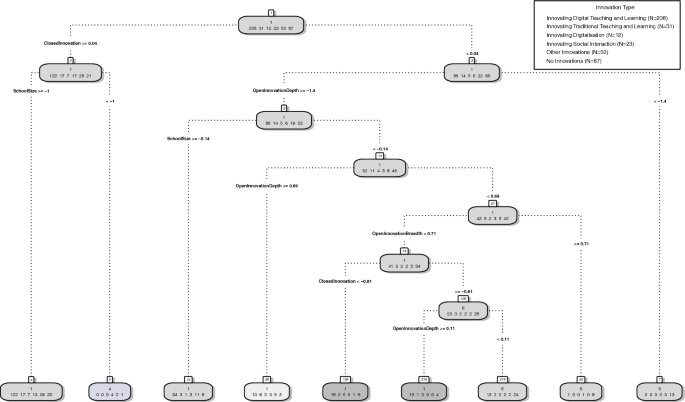
Classification and regression tree (CART) model for innovation in schools
To compare CARTâs predictive accuracy with the multinomial logistic regression model for our final analysis we employed 5000 resamples using the caret package (Kuhn, 2008 ). Here the median value of predictive accuracy was 0.50 for the multinomial regression and 0.57 for CART indicating a slightly better performance for the latter. Overall based on the CART results it can be argued that relations between the study variables are rather complex than linear and that the effectiveness of open innovation a) is key to innovation in schools, b) depends on closed innovation mechanisms and c) interacts with conditions of the respective school.
6 Discussion
The findings show that both closed and open innovation depth affect innovations in schools. Specifically, open innovation depth has a far greater effect on innovation in teaching and learning (traditional and digital) than effects of closed innovation. These findings are consistent with findings from general organisational and innovation research (Bogers et al., 2019 ; De Coninck et al., 2021 ). They also show that schools need stimuli to open up to externally inspired innovations and so overcome their traditionally strong self-recursiveness if they are to keep pace with social and technical developments. For instance, teacher professional development should be sensitive to questions, such as how teachers understand proposed innovation, how those can be enacted, and what fosters the adaption of external knowledge to the local conditions (Silver et al., 2019 ).
What is striking, however, is that open innovation depth is the only innovation mechanism having a significant effect on digitalisation in schools, when contextual factors are taken into account. This finding supports the notion that open innovation is particularly useful in enabling schools to benefit from new technologies (Chesbrough, 2003a ): especially when existing staff are unlikely to have an understanding of, or experience relating to, such technologies. The finding that open innovation breadth has negative effects on all innovations also seems significant. This result is also consistent with the findings of other studies that find a curvilinear relationship between open innovation breadth and innovation performance (Laursen & Salter, 2006 ; Shi et al., 2019 ; Terjersen & Patel, 2017 ).
Accordingly, also in schools such a potential over-search (Shi et al., 2019 ) seems to make it difficult to identify and allocate resources to valuable sources of knowledge, which in turn might have a negative impact on innovation performance. In other words, too many parallel activities undertaken all at once may lead to a diffusion of forces instead of a concentration of activities, which is not conducive but even a hindrance to the innovations aimed at. There is thus a danger of a failure trap (Pietsch et al., 2023b ), i.e., a parallelism or succession of ever new measures, which leads to no sustainable innovation. This parallelism also applies to teaching, when teachers constantly explore new teaching concepts and methods inspired by continuing professional development activities or other external sources, without following a specific strategy or didactic concept. In this respect, open innovation in pedagogical practice must not be misunderstood as an aimless experimentation as a result of multiple external inspirations. Rather, the professional action of teachers in the classroom is tied to reasoned trade-offs between options.
Indeed, there is seemingly a particular risk of the emergence of failure traps in education systems because, when it comes to education, reforms regularly form part of the political discourse, and innovations (intended to solve perceived educational issues) are often introduced to schools from the outside. Depending on the regularity of this introduction, such policy-making could even prevent schools from using external knowledge in the sense of (self-motivated) proactive open innovation. In this respect, the role of education policy, such as with medicine, should perhaps not be to prescribe specific innovations per se, but to demand and promote open innovation (Jiao et al., 2022 ).
It is further striking that the open and closed innovation mechanisms are not dichotomous categories, but rather interwoven mechanisms. As depicted in Fig. 1 , open innovationâs effectiveness is present when the score for closed innovation is low. In line with Marques ( 2014 ), we therefore argue that both mechanisms are closely intertwined and that an interplay of closed and open innovation regimes is important when it comes to educational innovation and change. This finding also suggests that it is important for externally mobilised knowledge, i.e. open innovation depth, to be linked to the knowledge already available in the school in order for it to become effective. As a result, our study also contributes to the theoretical discussion in innovation research and in particular to the debate on how to organise innovation through distributed approaches. It is argued here that when organisations combine internal and external search strategies to access different resources for innovation, new challenges arise from the complementary management of these knowledge sources (Lakhani et al., 2013 ; Tushman et al., 2012 ). Our empirical analyses clearly demonstrate this, and suggest that the simultaneous pursuit of multiple types of organisational boundaries means that schools have to deal with complex, often internally conflicting innovation logics and their structural and procedural requirements. Our study is the first to show therefore that, even in schools, the ability to identify and acquire relevant external knowledge, and to link it to internal knowledge through transformation and exploitation, is significantly determined by the ability of a school and its actors to benefit from open innovation mechanisms. Thus, a schoolâs absorptive capacity (Daâas & Qadach, 2020 ) is an important determinant of the effective use of external knowledge (Lichtenthaler & Lichtenthaler, 2009 ; Lowik et al, 2017 ). In the end, it is likely to be of particular relevance whether innovations at the level of the school as an organization also reach pedagogical practice through the actions of teachers in the classroom, about which little is yet known.
Finally, the effect of open innovation depth on innovations generally varies with other inner-school conditions as well as school contexts. This also corresponds to the assumptions and findings on open innovation research from other fields (Chesbrough, 2003a , 2006 , 2012 ): The more closely open and closed innovation regimes are tailored to the conditions and needs of a school, the more likely they are to be effective. In this regard, it is striking that most of the contextual variables we examined have only a minor influence on the innovation outcome in schools, but that school size is a crucial characteristic in determining whether or not closed innovation mechanisms are sufficient for innovation in schools. Particularly in schools of above-average and below-average size, open innovation seems to act as an additional resource to closed innovation mechanisms and to mitigate possible resource constraints. This finding is also connectable to empirical findings outside of education research, which indicates a relationship between organisational size and organisational innovation (Aldieri & Vinci, 2019 ; Mote et al., 2016 ).
As in other studies, it can, finally, be seen that the relationships are not linear but complex, especially when it comes to technology and digitalisation (Lee & Xia, 2006 ). This also underlines the need to prepare teachers for a future-proof profession in such a way that they deal appropriately with this complexity and uncertainty in the field of pedagogical action, so that they do not implement external knowledge into the school in a decontextualized way (Cramer et al., 2023 ). Methodologically, our study here demonstrates the potential of machine learning approaches for the study of non-linear and complex relationships in educational research (Hilbert et al., 2021 . In particular, it highlights the value of such methods as a tool for testing and pruning theories, and as a catalyst for broadening the range of explanations that a theory can contain in organisational research in general (Leavitt et al., 2021 ), and in educational innovation and effectiveness research in particular (Hu et al., 2022 ).
7 Limitations
As far as we know, our study is one of the first to empirically examine Chesbroughsâ (2003a) concept of open innovation in the school context. Although our investigation accordingly reveals many possibilities for further research, it also has several limitations. The first relates to it cross-sectional rather than time-series nature, and thus our ability (or lack thereof) to generalise results gathered from one point in time in Germany. Consequently, on the one hand, causality can only be inferred, but cannot be demonstrated. Related is that we cannot assess whether the reported effects are similar in other contexts. We were also unable to investigate how open and closed innovation processes emerge and develop and to what extent these two innovation paradigms interact with each other dynamically over time (Chiaroni et al., 2011 ). Further, our analyses are based on self-reports of school leaders, so neither misreporting nor perceptual distance (Tafvelin et al., 2017 ) between leaders and other organisational members, i.e. teachers, can be completely ruled out. Accordingly, there is also the possibility that micro-level innovations regarding individual teachers and classrooms were not perceived and reported. In measuring open innovation, we followed Laursen and Salter ( 2006 ). Accordingly, our list of potential knowledge sources is not exhaustive and could theoretically be expanded (or contracted) to include (or exclude) other options. Furthermore, following the model, we did not investigate whether there are individual knowledge sources that are particularly relevant, as we were interested in comparing our findings with those of studies that also followed Laursen and Salter ( 2006 ). Hence, future research should try to apply longitudinal designs, gather data at different levels of schools and across various contexts, and should investigate whether there are specific sources of knowledge that are particularly relevant to stimulating innovation in schools.
8 Conclusion, Implications and Future Research
Our findings provide first and preliminary evidence that the concept of open innovation can be applied in the context of education. It is obvious that schools are highly dependent on external knowledge if they want to keep up with social and technological developments. Using the knowledge available in the school, i.e. closed innovation, seems to be no longer enough. The extent to which external knowledge effectively influences internal innovation depends on the respective conditions of the individual school. In particular, the mobilisation of internal school knowledge is an important prerequisite for externally mobilised knowledge to become effective. Especially when it comes to the use of new technologies and innovation in the field of digitalisation, open innovation can be a lever for change in schools.
Regarding policy and practice in education, this means that it is important to prepare schools and school staff (both in teacher education and while qualifying school leaders) to be open to appropriate knowledge flows, e.g. by strengthening open innovation mindsets (Bogers et al., 2019 ; Chesbrough, 2017 ; Engelsberger et al., 2022 ) and (individual) absorptive capacity (Aliasghar et al., 2019 ; Lichtenthaler & Lichtenthaler, 2009 ; Spithoven et al., 2010 ). As schools âare historically weak at knowledge sharing within and across schoolsâ (Fullan, 2002 , p. 409), it might also be necessary to address possible negative attitudes of teachers towards externally acquired knowledge in order to overcome possible ânot-invented-hereâ barriers (Antons & Piller, 2015 ; West & Bogers, 2014 ). Given that managing broad and heterogeneous sources of knowledge requires a substantial share of management time and attention (Aliasghar et al., 2020 ), and that an accompanying over-search can ultimately lead to negative effects in pedagogical innovation in schools (Pietsch et al., 2023a ; Shi et al., 2019 ), it also seems necessary to enable school leaders to direct both individual and school capacities towards influential innovation opportunities. It is a desideratum to better understand how exactly innovations at the level of the school as an organization ultimately show up in pedagogical practice in the classroom or in teacher action.
This is also followed by perspectives for further research: Given the generally very limited evidence on the influence of inbound open innovation on the development of process innovations (Aliasghar et al., 2020 ) and the fact that especially complicated process innovations, which were the subject of our study, can cause high transaction and opportunity costs for external knowledge mobilisation (Shi et al., 2019 ), it makes sense in principle to identify configurations for optimal knowledge flows within, into and between schools. Further, we currently know very little about teachersâ and school leadersâ mindsets and attitudes towards knowledge creation and sharing for innovation within and across schools (Berson et al., 2015 ) and nothing at all about this with regard to the concept of open innovation. Accordingly, on the one hand, it seems purposeful to investigate the applicability of these concepts to school and teaching. On the other hand, it might be promising to investigate their connection with the innovative capacity and innovation performance of schools in future research.
Data Availability
The datasets used and/or analysed during the current study are available from the corresponding author on reasonable request.
Aldieri, L., & Vinci, C. P. (2019). Firm size and sustainable innovation: A theoretical and empirical analysis. Sustainability, 11 (10), 2775. https://doi.org/10.3390/su11102775
Article  Google Scholar Â
Aliasghar, O., Rose, E. L., & Chetty, S. (2019). Where to search for process innovations? The mediating role of absorptive capacity and its impact on process innovation. Industrial Marketing Management, 82 , 199â212. https://doi.org/10.1016/j.indmarman.2019.01.014
Aliasghar, O., Sadeghi, A., & Rose, E. L. (2020). Process innovation in small- and medium-sized enterprises: The critical roles of external knowledge sourcing and absorptive capacity. Journal of Small Business Management . https://doi.org/10.1080/00472778.2020.1844491
Allen, M. S., Iliescu, D., & Greiff, S. (2022). Single item measures in psychological science. European Journal of Psychological Assessment, 38 (1), 1â5. https://doi.org/10.1027/1015-5759/a000699
Antons, D., & Piller, F. T. (2015). Opening the black box of ânot invented hereâ: Attitudes, decision biases, and behavioral consequences. Academy of Management Perspectives, 29 (2), 193â217. https://doi.org/10.5465/amp.2013.0091
Bass, B. M., & Avolio, B. J. (1995). MLQ multifactor leadership questionnaire. Technical report . Mind Garden.
Google Scholar Â
Becker, T. E. (2005). Potential problems in the statistical control of variables in organizational research: A qualitative analysis with recommendations. Organizational Research Methods, 8 (3), 274â289. https://doi.org/10.1177/1094428105278021
Behrens, V., Berger, M., Hud, M., et al. (2017). Innovation activities of firms in Germany: Results of the German CIS 2012 and 2014 background report on the surveys of the Mannheim Innovation Panel conducted in the years 2013 to 2016 . ZEW - Leibniz Centre for European Economic Research.
Bernerth, J. B., Cole, M. S., Taylor, E. C., & Walker, H. J. (2018). Control variables in leadership research: A qualitative and quantitative review. Journal of Management, 44 (1), 131â160. https://doi.org/10.1177/0149206317690586
Berson, Y., Daâas, R., & Waldman, D. A. (2015). How do leaders and their teams bring about organizational learning and outcomes? Personnel Psychology, 68 (1), 79â108. https://doi.org/10.1111/peps.12071
Bigliardi, B., Ferraro, G., Filippelli, S., & Galati, F. (2020). The past, present and future of open innovation. European Journal of Innovation Management, 24 (4), 1130â1161. https://doi.org/10.1108/ejim-10-2019-0296
Bogers, M., Chesbrough, H., Heaton, S., & Teece, D. J. (2019). Strategic management of open innovation: A dynamic capabilities perspective. California Management Review, 62 (1), 77â94. https://doi.org/10.1177/0008125619885150
Breiman, L., Friedman, J. H., Olshen, R. A., & Stone, C. J. (1984). Classification and regression trees . Wadsworth.
Brown, C., & Luzmore, R. (2021). Educating tomorrow: Learning for the post-pandemic world. Emerald Publishing Limited . https://doi.org/10.1108/9781800436602
Brown, C., MacGregor, S., Flood, J., & Malin, J. (2022). Facilitating research-informed educational practice for inclusion: Survey findings from 147 teachers and school leaders in England. Frontiers in Education . https://doi.org/10.3389/feduc.2022.890832
Brown, C., White, R., & Kelly, A. (2021). Teachers as educational change agents: What do we currently know? Findings from a systematic review. Emerald Open Research, 3 , 26. https://doi.org/10.35241/emeraldopenres.14385.1
Bryk, A. S. (2010). Organizing schools for improvement. Phi Delta Kappan, 91 (7), 23â30. https://doi.org/10.1177/003172171009100705
Buske, R. (2018). The principal as a key actor in promoting teachersâ innovativeness: Analyzing the innovativeness of teaching staff with variance-based partial least square modeling. School Effectiveness and School Improvement, 29 (2), 262â284. https://doi.org/10.1080/09243453.2018.1427606
Cheng, E. C. (2021). Knowledge management for improving school strategic planning. Educational Management Administration and Leadership, 49 (5), 824â840. https://doi.org/10.1177/1741143220918255
Chesbrough, H. W. (2003a). Open innovation: The new imperative for creating and profiting from technology . Harvard Business Press.
Chesbrough, H. (2003b). The logic of open innovation. California Management Review, 45 (3), 33â58. https://doi.org/10.1177/000812560304500301
Chesbrough, H. (2006). Open innovation: A new paradigm for understanding industrial innovation. In H. Chesbrough, W. Vanhaverbeke, & J. West (Eds.), Open innovation: Researching a new paradigm (pp. 1â12). Oxford University Press.
Chapter  Google Scholar Â
Chesbrough, H. (2012). open innovation: Where weâve been and where weâre going. Research-Technology Management, 55 (4), 20â27. https://doi.org/10.5437/08956308x5504085
Chesbrough, H. (2017). The future of open innovation. Research-Technology Management, 60 (1), 35â38. https://doi.org/10.1080/08956308.2017.1255054
Chesbrough, H. W., & Bogers, M. (2014). Explicating open innovation: Clarifying an emerging paradigm for understanding innovation. In H. W. Chesbrough, W. Vanhaverbeke, & J. West (Eds.), New frontiers in open innovation (pp. 3â28). Oxford University Press. https://doi.org/10.1093/acprof:oso/9780199682461.003.0001
Chiaroni, D., Chiesa, V., & Frattini, F. (2011). The open innovation journey: How firms dynamically implement the emerging innovation management paradigm. Technovation, 31 (1), 34â43. https://doi.org/10.1016/j.technovation.2009.08.007
Chu, K. W. (2016a). Beginning a journey of knowledge management in a secondary school. Journal of Knowledge Management, 20 (2), 364â385. https://doi.org/10.1108/jkm-04-2015-0155
Chu, K. W. (2016b). Leading knowledge management in a secondary school. Journal of Knowledge Management, 20 (5), 1104â1147. https://doi.org/10.1108/jkm-10-2015-0390
Cordeiro, M. D. M., Oliveira, M., & Sanchez-Segura, M. I. (2022). The influence of the knowledge management processes on results in basic education schools. Journal of Knowledge Management, 26 (10), 2699â2717. https://doi.org/10.1108/JKM-07-2021-0579
Cramer, C., Brown, C., & Aldridge, D. (2023). Meta-reflexivity and teacher professionalism: Facilitating multiparadigmatic teacher education to achieve a future-proof profession. Journal of Teacher Education . https://doi.org/10.1177/00224871231162295
Cramer, C., GroĂ Ophoff, J., Pietsch, M., & Tulowitzki, P. (2021). Schulleitung in Deutschland: reprĂ€sentative Befunde zur AttraktivitĂ€t, zu Karrieremotiven und zu Arbeitsplatzwechselabsichten. Ddsâdie Deutsche Schule, 113 (2), 132â148. https://doi.org/10.31244/dds.2021.02.02
Cuban, L. (2020). Reforming the grammar of schooling again and again. American Journal of Education, 126 (4), 665â671. https://doi.org/10.1086/709959
Daas, R. A., & Qadach, M. (2020). Examining organizational absorptive capacity construct: A validation study in the school context. Leadership and Policy in Schools, 19 (3), 327â345. https://doi.org/10.1080/15700763.2018.1554155
Damanpour, F. (1988). Innovation type, radicalness, and the adoption process. Communication Research, 15 (5), 545â567. https://doi.org/10.1177/009365088015005003
Damanpour, F. (1991). Organizational innovation: A meta-analysis of effects of determinants and moderators. Academy of Management Journal, 34 (3), 555â590. https://doi.org/10.5465/256406
De Coninck, B., GascĂł-HernĂĄndez, M., Viaene, S., & Leysen, J. (2021). Determinants of open innovation adoption in public organizations: a systematic review. Public Management Review . https://doi.org/10.1080/14719037.2021.2003106
Dedering, K., & Pietsch, M. (2023). School leader trust and collective teacher innovativeness: on individual and organisational ambidexterityâs mediating role. Educational Review . https://doi.org/10.1080/00131911.2023.2195593
Eickelmann, B., Bos, W., Gerick, J., Goldhammer, F., Schaumburg, H., Schwippert, K., Senkbeil, M., & Vahrenhold, J. (Eds.). (2019). ICILS 2018 #Deutschland: Computer- und informationsbezogene Kompetenzen von SchĂŒlerinnen und SchĂŒlern im zweiten internationalen Vergleich und Kompetenzen im Bereich Computational Thinking (1st ed.). Waxmann.
Elmore, R. (1996). Getting to scale with good educational practice. Harvard Educational Review, 66 (1), 1â27. https://doi.org/10.17763/haer.66.1.g73266758j348t33
Engelsberger, A., Halvorsen, B., Cavanagh, J., & Bartram, T. (2022). Human resources management and open innovation: The role of open innovation mindset. Asia Pacific Journal of Human Resources, 60 (1), 194â215. https://doi.org/10.1111/1744-7941.12281
Frost, D. (2012). From professional development to system change: Teacher leadership and innovation. Professional Development in Education, 38 (2), 205â227. https://doi.org/10.1080/19415257.2012.657861
Fullan, M. (2002). The role of leadership in the promotion of knowledge management in schools. Teachers and Teaching, 8 (3), 409â419. https://doi.org/10.1080/135406002100000530
Fullan, M. (2015). The new meaning of educational change (5th ed.). Amsterdam University Press.
Gassmann, O., Enkel, E., & Chesbrough, H. (2010). The future of open innovation. R&D Management, 40 (3), 213â221. https://doi.org/10.1111/j.1467-9310.2010.00605.x
Goldenbaum, A. (2012). Innovationsmanagement in Schulen: Eine empirische Untersuchung zur Implementation eines Sozialen Lernprogramms . VS.
Greany, T. (2018). Innovation is possible, itâs just not easy: Improvement, innovation and legitimacy in Englandâs autonomous and accountable school system. Educational Management Administration and Leadership, 46 (1), 65â85. https://doi.org/10.1177/1741143216659297
Greany, T., & Waterhouse, J. (2016). Rebels against the system. International Journal of Educational Management, 30 (7), 1188â1206. https://doi.org/10.1108/ijem-11-2015-0148
Hadfield, M., Jopling, M., Noden, C., OâLeary, D., & Stott, A. (2006). What does the existing knowledge base tell us about the impact of networking and collaboration? A review of network-based innovations in education in the UK . National College for School Leadership.
Haelermans, C., & Blank, J. L. (2012). Is a schoolsâ performance related to technical change? A study on the relationship between innovations and secondary school productivity. Computers and Education, 59 (3), 884â892. https://doi.org/10.1016/j.compedu.2012.03.027
HalĂĄsz, G. (2018). Measuring innovation in education: The outcomes of a national education sector innovation survey. European Journal of Education, 53 (4), 557â573. https://doi.org/10.1111/ejed.12299
Hallinger, P. (2011). Leadership for learning: Lessons from 40 years of empirical research. Journal of Educational Administration, 49 (2), 125â142. https://doi.org/10.1108/09578231111116699
Hallinger, P., Wang, W., Chen, C., & Liare, D. (2015). Assessing instructional leadership with the principal instructional management rating scale . Springer.
Book  Google Scholar Â
Hanson, M. (2001). Institutional theory and educational change. Educational Administration Quarterly, 37 (5), 637â661. https://doi.org/10.1177/00131610121969451
Hanushek, E. A., Link, S., & Woessmann, L. (2013). Does school autonomy make sense everywhere? Panel estimates from PISA. Journal of Development Economics, 104 , 212â232. https://doi.org/10.1016/j.jdeveco.2012.08.002
Hargreaves, A., & Shirley, D. (2012). The fourth way: The inspiring future for educational change. Corwin Press . https://doi.org/10.4135/9781452219523
Hargreaves, D. H. (1999). The knowledge-creating school. British Journal of Educational Studies, 47 (2), 122â144. https://doi.org/10.1111/1467-8527.00107
Harman, H. H. (1960). Modern factor analysis . University of Chicago Press.
Hilbert, S., Coors, S., Kraus, E., Bischl, B., Lindl, A., Frei, M., & Stachl, C. (2021). Machine learning for the educational sciences. Review of Education . https://doi.org/10.1002/rev3.3310
Honig, M. I., & Rainey, L. R. (2012). Autonomy and school improvement: What do we know and where do we go from here? Educational Policy, 26 (3), 465â495. https://doi.org/10.1177/0895904811417590
Hopkins, D. (2013). Exploding the myths of school reform. School Leadership and Management, 33 (4), 304â321. https://doi.org/10.1080/13632434.2013.793493
Hosmer, D. W., Lemeshow, S., & Sturdivant, R. X. (2013). Applied logistic regression . Wiley. https://doi.org/10.1002/9781118548387
Hu, J., Peng, Y., & Ma, H. (2022). Examining the contextual factors of science effectiveness: A machine learning-based approach. School Effectiveness and School Improvement, 33 (1), 21â50. https://doi.org/10.1080/09243453.2021.1929346
Huizingh, E. K. (2011). Open innovation: State of the art and future perspectives. Technovation, 31 (1), 2â9. https://doi.org/10.1016/j.technovation.2010.10.002
HĂŒnermund, P., & Louw, B. (2020). On the nuisance of control variables in regression analysis (working paper no. 4). Cornell University. https://doi.org/10.48550/arXiv.2005.10314
Jiao, H., Yang, J., & Cui, Y. (2022). Institutional pressure and open innovation: The moderating effect of digital knowledge and experience-based knowledge. Journal of Knowledge Management, 26 (10), 2499â2527. https://doi.org/10.1108/JKM-01-2021-0046
Kankanhalli, A., Zuiderwijk, A., & Tayi, G. K. (2017). Open innovation in the public sector: A research agenda. Government Information Quarterly, 34 (1), 84â89. https://doi.org/10.1016/j.giq.2016.12.002
Kruszewska, A., & Lavrenova, M. (2022). The educational opportunities of Ukrainian children at the time of the Russian invasion: Perspectives from teachers. Education, 3â13 , 1â14. https://doi.org/10.1080/03004279.2022.2083211
Krzywinski, M., & Altman, N. (2017). Classification and regression trees. Nature Methods, 14 (8), 757â758. https://doi.org/10.1038/nmeth.4370
Kuhn, M. (2008). Building predictive models in R using the caret package. Journal of Statistical Software, 28 , 1â26. https://doi.org/10.18637/jss.v028.i05
Lakhani, K., Lifshitz-Assaf, H., & Tushman, M. (2013). Open innovation and organizational boundaries: Task decomposition, knowledge distribution, and the locus of innovation. In A. Grandori (Ed.), Handbook of economic organization: Integrating economic and organizational theory (pp. 355â382). Elgar.
Lance, C. E., Dawson, B., Birkelbach, D., & Hoffman, B. J. (2010). Method effects, measurement error, and substantive conclusions. Organizational Research Methods, 13 (3), 435â455. https://doi.org/10.1177/1094428109352528
Laursen, K., & Salter, A. (2006). Open for innovation: The role of openness in explaining innovation performance among UK manufacturing firms. Strategic Management Journal, 27 (2), 131â150. https://doi.org/10.1002/smj.507
Leavitt, K., Schabram, K., Hariharan, P., & Barnes, C. M. (2021). Ghost in the machine: On organizational theory in the age of machine learning. Academy of Management Review, 46 (4), 750â777. https://doi.org/10.5465/amr.2019.0247
Lee, G., & Xia, W. (2006). Organizational size and IT innovation adoption: A meta-analysis. Information and Management, 43 (8), 975â985. https://doi.org/10.1016/j.im.2006.09.003
Lichtenthaler, U., & Lichtenthaler, E. (2009). A capability-based framework for open innovation: Complementing absorptive capacity. Journal of Management Studies, 46 (8), 1315â1338. https://doi.org/10.1111/j.1467-6486.2009.00854.x
Lowik, S., Kraaijenbrink, J., & Groen, A. J. (2017). Antecedents and effects of individual absorptive capacity: A micro-foundational perspective on open innovation. Journal of Knowledge Management, 21 (6), 1319â1341. https://doi.org/10.1108/JKM-09-2016-0410
Lubienski, C., & Perry, L. (2019). The third sector and innovation: Competitive strategies, incentives, and impediments to change. Journal of Educational Administration, 57 (4), 329â344. https://doi.org/10.1108/jea-10-2018-0193
Luyten, H., Hendriks, M., & Scheerens, J. (2014). School size effects revisited: A qualitative and quantitative review of the research evidence in primary and secondary education . Springer.
Marques, J. P. C. (2014). Closed versus open innovation: Evolution or Combination? International Journal of Business and Management . https://doi.org/10.5539/ijbm.v9n3p196
Massa, L., Tucci, C. L., & Afuah, A. (2017). A critical assessment of business model research. Academy of Management Annals, 11 (1), 73â104. https://doi.org/10.5465/annals.2014.0072
McCharen, B., Song, J., & Martens, J. (2011). School Innovation. Educational Management Administration and Leadership, 39 (6), 676â694. https://doi.org/10.1177/1741143211416387
Mote, J., Jordan, G., Hage, J., Hadden, W., & Clark, A. (2016). Too big to innovate? Exploring organizational size and innovation processes in scientific research. Science and Public Policy, 43 (3), 332â337. https://doi.org/10.1093/scipol/scv045
MuthĂ©n, L. K. & MuthĂ©n, B. O. (2017). Mplus userâs guide . MuthĂ©n & MuthĂ©n.
Nguyen, D., Pietsch, M., & GĂŒmĂŒĆ, S. (2021). Collective teacher innovativeness in 48 countries: Effects of teacher autonomy, collaborative culture, and professional learning. Teaching and Teacher Education, 106 , 103463. https://doi.org/10.1016/j.tate.2021.103463
Nicholls, A. (2018). Managing educational innovations . Routledge.
OECD. (2009). Working out change: Systemic innovation in vocational education and training . OECD.
OECD. (2014). PISA 2012 technical report . OECD. Retrieved December 30, 2022, from https://www.oecd.org/pisa/pisaproducts/PISA-2012-technical-report-final.pdf
OECD. (2015). Schooling redesigned . OECD. https://doi.org/10.1787/9789264245914-en
OECD/Eurostat. (2018). Oslo manual 2018: Guidelines for collecting, reporting and using data on innovation . OECD. https://doi.org/10.1787/9789264304604-en
OECD. (2019). TALIS 2018 technical report . OECD. Retrieved December 29, 2022, from https://www.oecd.org/education/talis/TALIS_2018_Technical_Report.pdf
Pietsch, M., Brown, C., Aydin, B., & Cramer, C. (2023a). Open innovation networks: A driver for knowledge mobilisation in schools? Journal of Professional Capital and Community, 8 (3), 202â218. https://doi.org/10.1108/JPCC-02-2023-0012
Pietsch, M., Tulowitzki, P., & Cramer, C. (2023b). Innovating teaching and instruction in turbulent times: The dynamics of principalsâ exploration and exploitation activities. Journal of Educational Change, 24 (3), 549â581. https://doi.org/10.1007/s10833-022-09458-2
Pietsch, M., Tulowitzki, P., & Koch, T. (2019). On the differential and shared effects of leadership for learning on teachersâ organizational commitment and job satisfaction: A multilevel perspective. Educational Administration Quarterly, 55 (5), 705â741. https://doi.org/10.1177/0013161X18806346
Podsakoff, P. M., MacKenzie, S. B., & Podsakoff, N. P. (2012). Sources of method bias in social science research and recommendations on how to control It. Annual Review of Psychology, 63 (1), 539â569. https://doi.org/10.1146/annurev-psych-120710-100452
Popa, S., Soto-Acosta, P., & Martinez-Conesa, I. (2017). Antecedents, moderators, and outcomes of innovation climate and open innovation: An empirical study in SMEs. Technological Forecasting and Social Change, 118 , 134â142. https://doi.org/10.1016/j.techfore.2017.02.014
Prenger, R., Tappel, A. P. M., Poortman, C. L., & Schildkamp, K.(2022). How can educational innovations become sustainable? A review of the empirical literature. Frontiers in Education , 7, Article 970715. https://doi.org/10.3389/feduc.2022.970715
Preston, C., Goldring, E., Berends, M., & Cannata, M. (2012). School innovation in district context: Comparing traditional public schools and charter schools. Economics of Education Review, 31 (2), 318â330. https://doi.org/10.1016/j.econedurev.2011.07.016
RamĂrez-Montoya, M. S., Castillo-MartĂnez, I. M., Sanabria-Z, J., & Miranda, J. (2022). Complex thinking in the framework of Education 4.0 and Open Innovation: A systematic literature review. Journal of Open Innovation: Technology, Market, and Complexity, 8 (1), 4. https://doi.org/10.3390/joitmc8010004
Rogers, E. (1995). Diffusion of innovations (4th ed.). The Free Press.
Röhl, S., Pietsch, M., & Cramer, C. (2022). School leadersâ self-efficacy and its impact on innovation: Findings of a repeated measurement study. Educational Management Administration and Leadership . https://doi.org/10.1177/17411432221132482
Saebi, T., & Foss, N. J. (2015). Business models for open innovation: Matching heterogeneous open innovation strategies with business model dimensions. European Management Journal, 33 (3), 201â213. https://doi.org/10.1016/j.emj.2014.11.002
Sahlberg P (2016). The global educational reform movement and its impact on schooling. In K. Mundy, A. Green, B. Lingard, & A. Verger (Eds.), The handbook of global education policy (pp. 128â144). Wiley. https://doi.org/10.1002/9781118468005.ch7
Schwabsky, N., Erdogan, U., & Tschannen-Moran, M. (2020). Predicting school innovation: The role of collective efficacy and academic press mediated by faculty trust. Journal of Educational Administration, 58 (2), 246â262. https://doi.org/10.1108/JEA-02-2019-0029
Serdyukov, P. (2017). Innovation in education: What works, what doesnât, and what to do about it? Journal of Research in Innovative Teaching & Learning, 10 (1), 4â33. https://doi.org/10.1108/JRIT-10-2016-0007
Shi, X., Zhang, Q., & Zheng, Z. (2019). The double-edged sword of external search in collaboration networks: Embeddedness in knowledge networks as moderators. Journal of Knowledge Management, 23 (10), 2135â2160. https://doi.org/10.1108/jkm-04-2018-0226
Silver, R. E., Kogut, G., & Huynh, T. C. D. (2019). Learning ânewâ instructional strategies: Pedagogical innovation, teacher professional development, understanding and concerns. Journal of Teacher Education, 70 (5), 552â566. https://doi.org/10.1177/0022487119844712
Slavec Gomezel, A., & Rangus, K. (2019). Open innovation: It starts with the leaderâs openness. Innovation, 21 (4), 533â551. https://doi.org/10.1080/14479338.2019.1615376
Slavin, R.E. (2005). Sand, bricks, and seeds: school change strategies and readiness for reform. In D. Hopkins (Ed.), The practice and theory of school improvement (pp. 265â279). Springer. https://doi.org/10.1007/1-4020-4452-615
Spector, P. E., & Brannick, M. T. (2011). Methodological urban legends: The misuse of statistical control variables. Organizational Research Methods, 14 (2), 287â305. https://doi.org/10.1177/1094428110369842
Spithoven, A., Clarysse, B., & Knockaert, M. (2010). Building absorptive capacity to organise inbound open innovation in traditional industries. Technovation, 30 (2), 130â141. https://doi.org/10.1016/j.technovation.2009.08.004
Tafvelin, S., von Thiele Schwarz, U., & Hasson, H. (2017). In agreement? Leader-team perceptual distance in organizational learning affects work performance. Journal of Business Research, 75 , 1â7. https://doi.org/10.1016/j.jbusres.2017.01.016
Tan, S. C., Chan, C., Bielaczyc, K., Ma, L., Scardamalia, M., & Bereiter, C. (2021). Knowledge building: Aligning education with needs for knowledge creation in the digital age. Educational Technology Research and Development, 69 (4), 2243â2266. https://doi.org/10.1007/s11423-020-09914-x
Tappel, A. P., Poortman, C. L., Schildkamp, K., & Visscher, A. J. (2023). Promoting sustainable educational innovation using the Sustainability Meter. Journal of Professional Capital and Community, 8 (3), 234â255. https://doi.org/10.1108/JPCC-02-2023-0008
Terjesen, S., & Patel, P. C. (2017). In Search of process innovations: The role of search depth, search breadth, and the industry environment. Journal of Management, 43 (5), 1421â1446. https://doi.org/10.1177/0149206315575710
Therneau, T. M., & Atkinson, B. (2014). An introduction to recursive partitioning using the rpart routines. R package version 4.1â19. http://cran.r-project.org/web/packages/rpart/rpart.pdf
Tushman, M. L., Lakhani, K. R., & Lifshitz-Assaf, H. (2012). Open innovation and organization design. Journal of Organization Design, 1 (1), 24â27.
Tyack, D., & Tobin, W. (1994). The âgrammarâ of schooling: Why has it been so hard to change? American Educational Research Journal, 31 (3), 453â479. https://doi.org/10.3102/00028312031003453
Tye, B. B. (2000). Hard Truths: Uncovering the Deep Structure of Schooling . Teachersâ College Press.
Vincent-Lancrin, S., KÀrkkÀinen, K., Pfotenhauer, S., Atkinson, A., Jocotin, G., & Rimini, M. (2014). Measuring innovation in education . OECD.
Vincent-Lancrin, S., Urgel, J., Kar, S., & Jacotin, G. (2019). Measuring innovation in education 2019: What has changed in the classroom? OECD . https://doi.org/10.1787/9789264311671-en
Watson, N., & Lynn, P. (2021). Refreshment sampling for longitudinal surveys. Advances in Longitudinal Survey Methodology . https://doi.org/10.1002/9781119376965.ch1
West, J., & Bogers, M. (2014). Leveraging external sources of innovation: A review of research on open innovation. Journal of Product Innovation Management, 31 (4), 814â831. https://doi.org/10.1111/jpim.12125
Williams, G. (2011). Data mining with Rattle and R: The art of excavating data for knowledge discovery . Springer.
Zimmer, R., Henry, G. T., & Kho, A. (2017). The effects of school turnaround in Tennesseeâs achievement school district and innovation zones. Educational Evaluation and Policy Analysis, 39 (4), 670â696. https://doi.org/10.3102/0162373717705729
Download references
Open Access funding enabled and organized by Projekt DEAL. This work was supported by the German Research Foundation (DFG) by a DFG Heisenberg grant under Grant No. 451458391 (PI 618/4-1).
Author information
Authors and affiliations.
Leuphana University of LĂŒneburg, UniversitĂ€tsallee 1, 21335, LĂŒneburg, Germany
Marcus Pietsch, Burak Aydin & Jasmin Witthöft
Eberhard Karls University of TĂŒbingen, Wilhelmstrasse 31, 72070, TĂŒbingen, Germany
Colin Cramer
University of Warwick, Coventry, CV4 7AL, UK
Chris Brown
Ege Universitesi, Erzene Mah Bornova, Izmir, TR, 35040, Turkey
Burak Aydin
You can also search for this author in PubMed  Google Scholar
Corresponding author
Correspondence to Marcus Pietsch .
Ethics declarations
Conflict of interest.
The authors have not disclosed any competing interests.
Additional information
Publisher's note.
Springer Nature remains neutral with regard to jurisdictional claims in published maps and institutional affiliations.
Appendix: Items and Scales
1.1 innovation outcome.
Introduction: We would now like to know from you whether, and if so, to what extent process innovations, i.e. innovations or changes in the pedagogical work of the school that did not previously exist at your school, have been introduced at your school in the last 12Â months.
1.1.1 Measurement of Innovativeness
Have process innovations, i.e. innovations or noticeable changes that affect the pedagogical work of the school, been introduced at your school in the last 12Â months?
Item measured on a binary scale (0â=âno; 1â=âyes).
1.1.2 Measurement of Concrete Innovations
(If yes) What were the most important innovations in this area in the last 12Â months? Please name a maximum of three examples, ordered by importance!
Free-form fields
1.1.3 Justification of Relevance of Innovations
(always related to the each mentioned innovation) Please explain in one sentence why this innovation was important for your school.
1.1.4 Measurement of Innovation Radicalness
(always related to each mentioned innovation) Are these changes incremental (improving and/or supplementing and/or adapting what already exists) or radical (introducing something completely new) for your school?
Item measured on a ten-point scale (1â=âincremental to 10â=âradical).
For all questions on innovation, this explanation was shown to the study participants throughout the questionnaire block: "Process innovations include new or noticeably changed processes with regard to the pedagogical work of the school (e.g. teaching and instruction)".
1.2 Closed and Open Innovation Depth and Breadth
Base Question: Now we would like to know where the knowledge came from for pedagogical innovations introduced at your school in the last 12 months. The knowledge that we used for the innovations cameâŠ
âŠfrom the school itself/ the teachers of our school.â+â
âŠfrom parents and guardians.*
âŠfrom other schools.*
âŠfrom school authorities, other authorities or official institutions, e.g. state institutes.*
âŠfrom academic institutions, e.g. universities.*
âŠfrom freelance or independent school improvement consultants.*
âŠfrom commercial enterprises.*
âŠfrom professional trainings and/or conventions.*
âŠfrom professional literature.*
â+â closed innovation , * open innovation.
All items were measured on a six-point scale (1â=ânot at all to 6â=âto an exceptionally high degree).
1.3 Innovation Climate
Base Question: How often are teachers offered the following opportunities at your school?
Our school provides time and resources for teachers to generate, share/exchange, and experiment with innovative ideas/solutions.
Our teachers frequently encounter nonroutine and challenging work that stimulates creativity.
Our teachers are recognized and rewarded for their creativity and innovative ideas.
All items were measured on a five-point scale (1â=ânever 5â=âvery often).
1.4 Collective Teacher Innovativeness
Base Question: Thinking about the teachers in your school, how strongly do you agree or disagree with the following statements?
Most teachers in my school strive to develop new ideas for teaching and learning.
Most teachers in my school are open to change.
Most teachers in my school search for new ways to solve problems.
Most teachers in my school provide practical support to each other for the application of new ideas.
All items were measured on a four-point scale (1â=âstrongly disagree to 4â=âstrongly agree).
1.5 Innovation Network Closeness
In the last 12Â months, how many hours per week on average did you spend maintaining existing contacts (e.g. in person on site, via email, by telephone, as a video conference) with people outside the school with whom you discussed school strategy matters (e.g. finances, school development, innovations)?
Free-form field (option: 0 to 99)
1.6 School Leadership for Learning
Base Question: Now we would like to know something about your leadership behaviour. For this purpose, statements that describe you as a leader are listed below. Please answer all questions quickly and trust your spontaneous judgement. How do you assess yourself in your current leadership role?
I talk optimistically about the future.+
I seek different perspectives when solving problems.+
I talk with teachers about their most important values and beliefs.+â
I help teachers in my school to develop their strengths.+â
I provide opportunities for teachers to actively participate in school decisions.*
I ensure that teachers work according to the schoolâs educational goals.#
When a teacher brings up a classroom problem, we solve the problem together.#
â+â transformational leadership , * shared leadership, #instructional leadership.
All items were measured on a four-point scale (1â=âvery rarely or never to 4â=âvery often).
Rights and permissions
Open Access This article is licensed under a Creative Commons Attribution 4.0 International License, which permits use, sharing, adaptation, distribution and reproduction in any medium or format, as long as you give appropriate credit to the original author(s) and the source, provide a link to the Creative Commons licence, and indicate if changes were made. The images or other third party material in this article are included in the article's Creative Commons licence, unless indicated otherwise in a credit line to the material. If material is not included in the article's Creative Commons licence and your intended use is not permitted by statutory regulation or exceeds the permitted use, you will need to obtain permission directly from the copyright holder. To view a copy of this licence, visit http://creativecommons.org/licenses/by/4.0/ .
Reprints and permissions
About this article
Pietsch, M., Cramer, C., Brown, C. et al. Open Innovation in Schools: A New Imperative for Organising Innovation in Education?. Tech Know Learn (2023). https://doi.org/10.1007/s10758-023-09705-2
Download citation
Accepted : 07 November 2023
Published : 27 November 2023
DOI : https://doi.org/10.1007/s10758-023-09705-2
Share this article
Anyone you share the following link with will be able to read this content:
Sorry, a shareable link is not currently available for this article.
Provided by the Springer Nature SharedIt content-sharing initiative
- Closed innovation
- Innovation performance
- Knowledge creation
- Knowledge mobilisation
- Open innovation
- Find a journal
- Publish with us
- Track your research
Purdue Online Writing Lab Purdue OWLÂź College of Liberal Arts
Welcome to the Purdue Online Writing Lab
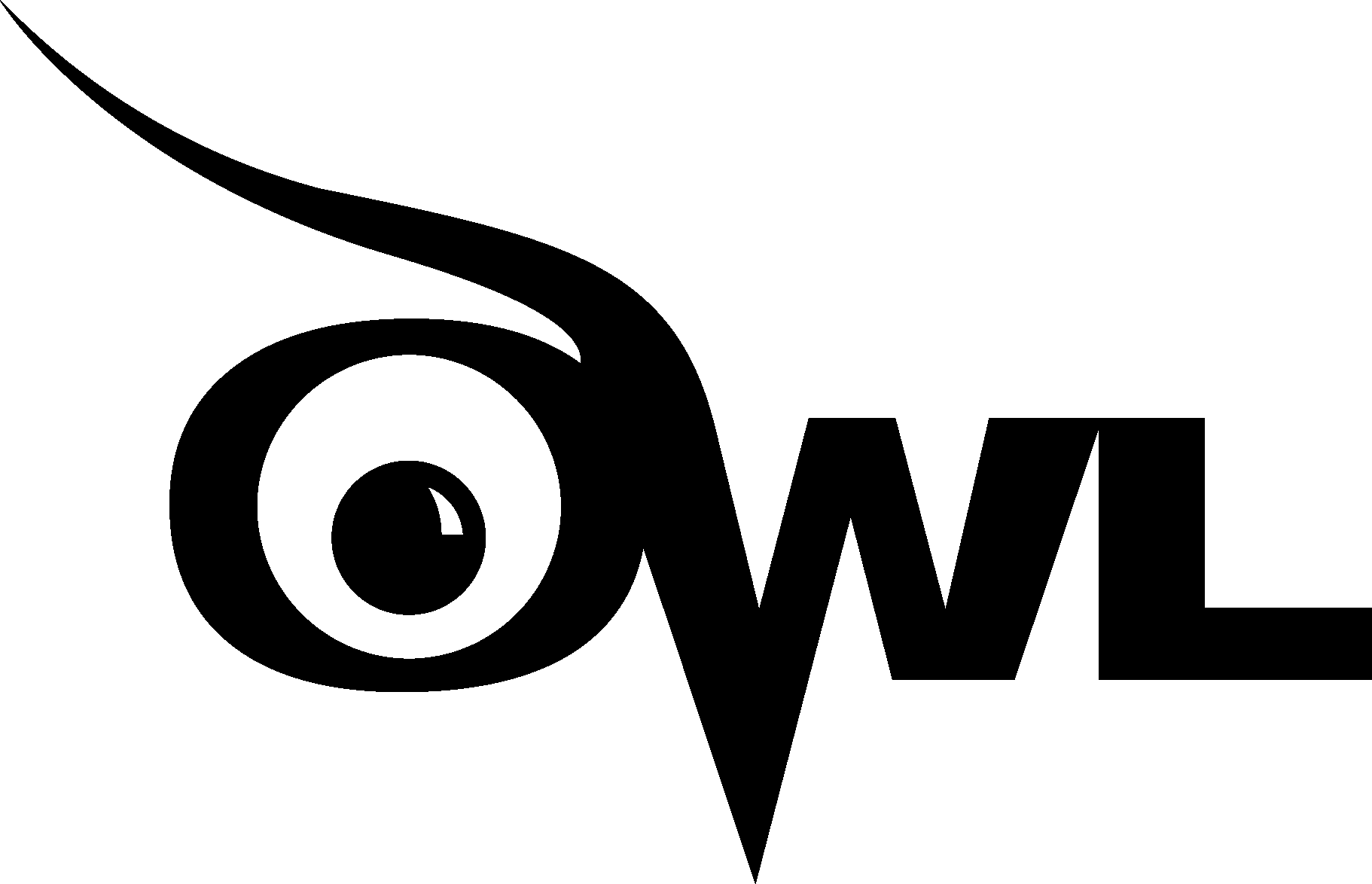
Welcome to the Purdue OWL
This page is brought to you by the OWL at Purdue University. When printing this page, you must include the entire legal notice.
Copyright ©1995-2018 by The Writing Lab & The OWL at Purdue and Purdue University. All rights reserved. This material may not be published, reproduced, broadcast, rewritten, or redistributed without permission. Use of this site constitutes acceptance of our terms and conditions of fair use.
The Online Writing Lab at Purdue University houses writing resources and instructional material, and we provide these as a free service of the Writing Lab at Purdue. Students, members of the community, and users worldwide will find information to assist with many writing projects. Teachers and trainers may use this material for in-class and out-of-class instruction.
The Purdue On-Campus Writing Lab and Purdue Online Writing Lab assist clients in their development as writers—no matter what their skill level—with on-campus consultations, online participation, and community engagement. The Purdue Writing Lab serves the Purdue, West Lafayette, campus and coordinates with local literacy initiatives. The Purdue OWL offers global support through online reference materials and services.
A Message From the Assistant Director of Content Development
The Purdue OWL® is committed to supporting students, instructors, and writers by offering a wide range of resources that are developed and revised with them in mind. To do this, the OWL team is always exploring possibilties for a better design, allowing accessibility and user experience to guide our process. As the OWL undergoes some changes, we welcome your feedback and suggestions by email at any time.
Please don't hesitate to contact us via our contact page if you have any questions or comments.
All the best,
Social Media
Facebook twitter.
- Introduction
- Conclusions
- Article Information
Models were stratified by age, cohort (sex), and calendar time, and adjusted for Southern European/Mediterranean ancestry (yes/no), married (yes/no), living alone (yes/no), smoking status (never, former, current smoker 1-14 cigarettes/d, 15-24 cigarettes/d, or â„25 cigarettes/d), physical activity (<3.0, 3.0-8.9, 9.0-17.9, 18.0-26.9, â„27.0 metabolic equivalent of taskâh/wk), multivitamin use (yes/no), history of hypertension (yes/no), history of hypercholesterolemia (yes/no), history of diabetes (yes/no), in women postmenopausal status and menopausal hormone use (premenopausal, postmenopausal [no, past, or current hormone use]), total energy intake (kcal/d), family history of dementia (yes/no), history of depression (yes/no), census socioeconomic status (9-variable score, in quintiles), and body mass index calculated as weight in kilograms divided by height in meters squared (<23, 23-25, 25-30, 30-35, â„35). Pooled results were obtained by pooling the datasets of the cohorts. AMED score is without monounsaturated:saturated fats intake ratio component. AHEI score is without polyunsaturated fats intake component. HR indicates hazard ratio.
a Reference value.
b P â<â.05.
Substitution analysis of 5 g/d intake of olive oil for the equivalent amount of butter, other vegetable oils, mayonnaise, and margarine. All Cox proportional hazards models were stratified by age and calendar time. Models were adjusted for Southern European/Mediterranean ancestry (yes/no), married (yes/no), living alone (yes/no), smoking status (never, former, current smoker 1-14 cigarettes/d, 15-24 cigarettes/d, or â„25 cigarettes/d), alcohol intake (0, 0.1-4.9, 5.0-9.9, 10.0-14.9, and â„15.0 g/d), physical activity (<3.0, 3.0-8.9, 9.0-17.9, 18.0-26.9, â„27.0 metabolic equivalent of taskâh/wk), multivitamin use (yes/no), history of hypertension (yes/no), history of hypercholesterolemia (yes/no), in women postmenopausal status and menopausal hormone use (premenopausal, postmenopausal [no, past, or current hormone use]), total energy intake (kcal/d), family history of dementia (yes/no), history of depression (yes/no), census socioeconomic status (9-variable score, in quintiles), body mass index calculated as weight in kilograms divided by height in meters squared (<23, 23-25, 25-30, 30-35, â„35), red meat, fruits and vegetables, nuts, soda, whole grains intake (in quintiles), and trans-fat. Pooled results were obtained by pooling the data sets of the cohorts and Cox proportional hazards model 3 was further stratified by cohort (sex). HR indicates hazard ratio.
eTable 1. Odds Ratios for Dementia-Related Mortality by APOE4 Allelic Dosage
eTable 2. Risk of Death With Dementia (Composite Outcome) According to Categories of Total Olive Oil
eTable 3. Joint Associations of Olive Oil Intake and AMED (A), and AHEI (B) With Dementia-Related Mortality Risk
eTable 4. Risk of Dementia-Related Mortality According to Categories of Total Olive Oil in the Genomic DNA Subsample
eFigure. Subgroup Analyses for 5g/d Increase in Olive Oil Intake With Dementia-Related Mortality Risk
eTable 5. Risk of Dementia-Related Mortality According to Categories of Total Olive Oil Without Stopping Diet Update Upon Report of Intermediate Non-Fatal Events
eTable 6. Risk of Dementia Mortality According to Categories of Total Olive Oil Applying a 4-Year Lag Period Between Dietary Intake and Dementia Mortality
eTable 7. Risk of Dementia-Related Mortality According to Categories of Total Olive Oil Adjusting for Other Covariates
eTable 8. Risk of Mortality From Dementia and Other Causes of Death According to Categories of Total Olive Oil Applying a Competing Risk Model
eReferences
Data Sharing Statement
See More About
Sign up for emails based on your interests, select your interests.
Customize your JAMA Network experience by selecting one or more topics from the list below.
- Academic Medicine
- Acid Base, Electrolytes, Fluids
- Allergy and Clinical Immunology
- American Indian or Alaska Natives
- Anesthesiology
- Anticoagulation
- Art and Images in Psychiatry
- Artificial Intelligence
- Assisted Reproduction
- Bleeding and Transfusion
- Caring for the Critically Ill Patient
- Challenges in Clinical Electrocardiography
- Climate and Health
- Climate Change
- Clinical Challenge
- Clinical Decision Support
- Clinical Implications of Basic Neuroscience
- Clinical Pharmacy and Pharmacology
- Complementary and Alternative Medicine
- Consensus Statements
- Coronavirus (COVID-19)
- Critical Care Medicine
- Cultural Competency
- Dental Medicine
- Dermatology
- Diabetes and Endocrinology
- Diagnostic Test Interpretation
- Drug Development
- Electronic Health Records
- Emergency Medicine
- End of Life, Hospice, Palliative Care
- Environmental Health
- Equity, Diversity, and Inclusion
- Facial Plastic Surgery
- Gastroenterology and Hepatology
- Genetics and Genomics
- Genomics and Precision Health
- Global Health
- Guide to Statistics and Methods
- Hair Disorders
- Health Care Delivery Models
- Health Care Economics, Insurance, Payment
- Health Care Quality
- Health Care Reform
- Health Care Safety
- Health Care Workforce
- Health Disparities
- Health Inequities
- Health Policy
- Health Systems Science
- History of Medicine
- Hypertension
- Images in Neurology
- Implementation Science
- Infectious Diseases
- Innovations in Health Care Delivery
- JAMA Infographic
- Law and Medicine
- Leading Change
- Less is More
- LGBTQIA Medicine
- Lifestyle Behaviors
- Medical Coding
- Medical Devices and Equipment
- Medical Education
- Medical Education and Training
- Medical Journals and Publishing
- Mobile Health and Telemedicine
- Narrative Medicine
- Neuroscience and Psychiatry
- Notable Notes
- Nutrition, Obesity, Exercise
- Obstetrics and Gynecology
- Occupational Health
- Ophthalmology
- Orthopedics
- Otolaryngology
- Pain Medicine
- Palliative Care
- Pathology and Laboratory Medicine
- Patient Care
- Patient Information
- Performance Improvement
- Performance Measures
- Perioperative Care and Consultation
- Pharmacoeconomics
- Pharmacoepidemiology
- Pharmacogenetics
- Pharmacy and Clinical Pharmacology
- Physical Medicine and Rehabilitation
- Physical Therapy
- Physician Leadership
- Population Health
- Primary Care
- Professional Well-being
- Professionalism
- Psychiatry and Behavioral Health
- Public Health
- Pulmonary Medicine
- Regulatory Agencies
- Reproductive Health
- Research, Methods, Statistics
- Resuscitation
- Rheumatology
- Risk Management
- Scientific Discovery and the Future of Medicine
- Shared Decision Making and Communication
- Sleep Medicine
- Sports Medicine
- Stem Cell Transplantation
- Substance Use and Addiction Medicine
- Surgical Innovation
- Surgical Pearls
- Teachable Moment
- Technology and Finance
- The Art of JAMA
- The Arts and Medicine
- The Rational Clinical Examination
- Tobacco and e-Cigarettes
- Translational Medicine
- Trauma and Injury
- Treatment Adherence
- Ultrasonography
- Users' Guide to the Medical Literature
- Vaccination
- Venous Thromboembolism
- Veterans Health
- Women's Health
- Workflow and Process
- Wound Care, Infection, Healing
Get the latest research based on your areas of interest.
Others also liked.
- Download PDF
- X Facebook More LinkedIn
Tessier A , Cortese M , Yuan C, et al. Consumption of Olive Oil and Diet Quality and Risk of Dementia-Related Death. JAMA Netw Open. 2024;7(5):e2410021. doi:10.1001/jamanetworkopen.2024.10021
Manage citations:
© 2024
- Permissions
Consumption of Olive Oil and Diet Quality and Risk of Dementia-Related Death
- 1 Department of Nutrition, Harvard T.H. Chan School of Public Health, Boston, Massachusetts
- 2 School of Public Health, the Second Affiliated Hospital, Zhejiang University School of Medicine, Hangzhou, China
- 3 Department of Epidemiology, Harvard T.H. Chan School of Public Health, Boston, Massachusetts
- 4 Channing Division of Network Medicine, Department of Medicine, Brigham and Womenâs Hospital and Harvard Medical School, Boston, Massachusetts
- 5 Department of Public Health and Novo Nordisk Foundation Center for Basic Metabolic Research, Faculty of Health and Medical Sciences, University of Copenhagen, Copenhagen, Denmark
Question  Is the long-term consumption of olive oil associated with dementia-related death risk?
Findings  In a prospective cohort study of 92âŻ383 adults observed over 28 years, the consumption of more than 7 g/d of olive oil was associated with a 28% lower risk of dementia-related death compared with never or rarely consuming olive oil, irrespective of diet quality.
Meaning  These results suggest that olive oil intake represents a potential strategy to reduce dementia mortality risk.
Importance  Age-standardized dementia mortality rates are on the rise. Whether long-term consumption of olive oil and diet quality are associated with dementia-related death is unknown.
Objective  To examine the association of olive oil intake with the subsequent risk of dementia-related death and assess the joint association with diet quality and substitution for other fats.
Design, Setting, and Participants  This prospective cohort study examined data from the Nursesâ Health Study (NHS; 1990-2018) and Health Professionals Follow-Up Study (HPFS; 1990-2018). The population included women from the NHS and men from the HPFS who were free of cardiovascular disease and cancer at baseline. Data were analyzed from May 2022 to July 2023.
Exposures  Olive oil intake was assessed every 4 years using a food frequency questionnaire and categorized as (1) never or less than once per month, (2) greater than 0 to less than or equal to 4.5 g/d, (3) greater than 4.5 g/d to less than or equal to 7 g/d, and (4) greater than 7 g/d. Diet quality was based on the Alternative Healthy Eating Index and Mediterranean Diet score.
Main Outcome and Measure  Dementia death was ascertained from death records. Multivariable Cox proportional hazards regressions were used to estimate hazard ratios (HRs) and 95% CIs adjusted for confounders including genetic, sociodemographic, and lifestyle factors.
Results  Of 92âŻ383 participants, 60âŻ582 (65.6%) were women and the mean (SD) age was 56.4 (8.0) years. During 28 years of follow-up (2âŻ183âŻ095 person-years), 4751 dementia-related deaths occurred. Individuals who were homozygous for the apolipoprotein Δ4 ( APOE Δ4 ) allele were 5 to 9 times more likely to die with dementia. Consuming at least 7 g/d of olive oil was associated with a 28% lower risk of dementia-related death (adjusted pooled HR, 0.72 [95% CI, 0.64-0.81]) compared with never or rarely consuming olive oil ( P for trendâ<â.001); results were consistent after further adjustment for APOE Δ4 . No interaction by diet quality scores was found. In modeled substitution analyses, replacing 5 g/d of margarine and mayonnaise with the equivalent amount of olive oil was associated with an 8% (95% CI, 4%-12%) to 14% (95% CI, 7%-20%) lower risk of dementia mortality. Substitutions for other vegetable oils or butter were not significant.
Conclusions and Relevance  In US adults, higher olive oil intake was associated with a lower risk of dementia-related mortality, irrespective of diet quality. Beyond heart health, the findings extend the current dietary recommendations of choosing olive oil and other vegetable oils for cognitive-related health.
One-third of older adults die with Alzheimer disease or another dementia. 1 While deaths from diseases such as stroke and heart disease have been decreasing over the past 20 years, age-standardized dementia mortality rates have been on the rise. 2 The Mediterranean diet has gained in popularity owing to its recognized, multifaceted health benefits, particularly on cardiovascular outcomes. 3 Accruing evidence suggests this dietary pattern also has a beneficial effect on cognitive health. 4 As part of the Mediterranean diet, olive oil may exert anti-inflammatory and neuroprotective effects due to its high content of monounsaturated fatty acids and other compounds with antioxidant properties such as vitamin E and polyphenols. 5 A substudy conducted as part of the Prevencion con Dieta Mediterranea (PREDIMED) randomized trial provided evidence that higher intake of olive oil for 6.5 years combined with adherence to a Mediterranean diet was protective of cognitive decline when compared with a low-fat control diet. 6 - 8
Given that most previous studies on olive oil consumption and cognition were conducted in Mediterranean countries, 7 - 10 studying the US population, where olive oil consumption is generally lower, could offer unique insights. Recently, we showed that olive oil consumption was associated with a lower risk of total and cause-specific mortality in large US prospective cohort studies, including a 29% (95% CI, 22%-36%) lower risk for neurodegenerative disease mortality in participants who consumed more than 7 g/d of olive oil compared with little or none. 11 However, this previous analysis was not designed to examine the association of olive oil and diet quality with dementia-related mortality, and therefore the latter remains unclear.
In this study, we examined the association between total olive oil consumption and the subsequent risk of dementia-related mortality in 2 large prospective studies of US women and men. Additionally, we evaluated the joint associations of diet quality (adherence to the Mediterranean diet and Alternative Healthy Eating Index [AHEI] score) and olive oil consumption with the risk of dementia-related mortality. We also estimated the difference in the risk of dementia-related mortality when other dietary fats were substituted with an equivalent amount of olive oil.
Analyses were performed in 2 large US prospective cohorts: the Nursesâ Health Study I (NHS) and the Health Professionals Follow-Up Study (HPFS). The NHS was initiated in 1976 and recruited 121âŻ700 US female registered nurses aged 30 to 55 years. 12 The HPFS was established in 1986 and included 51âŻ525 male health professionals aged 40 to 75 years. 13 The cohorts have been described elsewhere. 12 , 13 Lifestyle factors and medical history were assessed biennially through mailed questionnaires, with a follow-up rate greater than 90%. Baseline for this analysis was 1990, which is when the food frequency questionnaires (FFQs) first included information on olive oil consumption.
Participants with a history of cardiovascular disease (CVD) or cancer at baseline, with missing data on olive oil consumption, or who reported implausible total energy intakes (<500 or >3500 kcal/d for women and <800 or >4200 kcal/d for men) were excluded. The completion of the questionnaire self-selected cognitively highly functioning women and men. In total, 60âŻ582 women and 31âŻ801 men were included. The study protocol was approved by the institutional review boards of the Brigham and Womenâs Hospital and Harvard T.H. Chan School of Public Health, which deemed the participantsâ completion of the questionnaire to be considered as implied consent. This report followed the Strengthening the Reporting of Observational Studies in Epidemiology ( STROBE ) reporting guideline.
Dietary intake was measured using a validated greater than 130-item FFQ administered in 1990 and every 4 years thereafter. The validity and reliability of the FFQ have been described previously. 14 Participants were asked how frequently they consumed specific foods, including types of fats and oils used for cooking or added to meals in the past 12 months. Total olive oil intake was determined by summing up answers to 3 questions related to olive oil consumption (ie, olive oil used for salad dressings, olive oil added to food or bread, and olive oil used for baking and frying at home). The equivalent of 1 tablespoon of olive oil was considered to be 13.5 g. Intakes of other fats and nutrients were calculated using the United States Department of Agriculture and Harvard University Food Composition Database, 15 and biochemical analyses. The nutritional composition of olive oil and other types of fat, as well as trends of types of fat intake in the NHS and HPFS, have been reported previously. 11
Adherence to the Mediterranean diet was assessed using a modified version of the 9-point Alternative Mediterranean index (AMED) score. 16 Adherence to the AHEI (0-110), previously associated with lower risk of chronic disease, was also computed. 17 Higher scores indicated better overall diet quality.
The apolipoprotein E Δ4 ( APOE Δ4 ) allele is known to interfere with lipid and glucose metabolism such that it increases the risk of dementia. 18 ï»ż APOE genotyping was conducted in a subset of 27âŻ296 participants. Blood samples were collected between 1989 and 1990 in the NHS and between 1993 and 1995 in the HPFS. NHS participants who had not provided blood samples were invited to contribute buccal samples from 2002 to 2004. DNA was extracted with the ReturPureGene DNA Isolation Kit (Gentra Systems). The APOE genotype was determined using a Taqman Assay (Applied Biosystems) 19 in 5069 participants, and through imputation from multiple genome-wide association studies, 20 which has shown high accuracy, 20 in the remaining subset.
Deaths were ascertained from state vital statistics records and the National Death Index or by reports from next of kin or the postal authorities. The follow-up for mortality exceeded 98% in these cohorts. Dementia deaths were determined by physician review of medical records, autopsy reports, or death certificates. Dementia deaths were those in which dementia was listed as the underlying cause of death, or as a contributing cause of death, or as reported by the family, in the absence of a more likely cause. The International Classification of Diseases, Eighth Revision (ICD-8) was used in the NHS and ICD-9 in the HPFS, which were the revisions used at the inception of those cohorts. Dementia deaths included codes 290.0 (senile dementia, simple type), 290.1 (presenile dementia), and 331.0 (Alzheimer disease). To test the validity of the dementia mortality outcome, we examined the likelihood of dementia mortality by APOE Δ4 allelic dosage (eTable 1 in Supplement 1 ). 18 A composite outcome was also created including both participants who reported having dementia during follow-up and later died, with those who had dementia reported on their death certificate.
Participants completed biennial questionnaires reporting updates on body weight, smoking, physical activity, multivitamin use, menopausal status, and postmenopausal hormone use in women, family history of dementia, self-report of chronic diseases, and ancestry. History of depression was identified based on antidepressive medication use and self-report of depression. Socioeconomic status (SES) was established through a composite score derived from home address details and various factors such as income, education, and housing; the composite score methods are described in a previous report. 21 Body mass index (BMI) was obtained by dividing the weight in kilograms by the height in meters squared.
In each cohort, age-stratified Cox proportional hazard models were used to evaluate the association of olive oil intake with dementia-related mortality. Participant person-time was calculated from baseline until end of follow-up (June 30, 2018, in NHS; January 31, 2018, in HPFS), loss to follow-up, or death, whichever came first. The cumulative average (mean) of olive oil intake from all available FFQs, from baseline until 2014 (or loss to follow-up or death), was used as the exposure. Because potential diet modifications following cancer or CVD diagnosis may not represent long-term diet, we ceased updating dietary variables upon report of these conditions. For missing covariates, we carried forward nonmissing values from previous questionnaires and assigned median values for continuous variables.
Participants were categorized by olive oil intake frequency: never or less than once per month (reference group), greater than 0 to less than or equal to 4.5 g/d, greater than 4.5 g/d to less than or equal to 7 g/d, and greater than 7 g/d. P values for linear trends were obtained using the Wald test on a continuous variable represented by the median intake of each category. Multivariable Cox proportional hazard models were used to estimate the hazard ratios (HRs) and 95% CIs for dementia mortality according to categories of olive oil intake, separately in each cohort. Participants were censored at death from causes other than dementia. Model 1 was stratified for age and calendar time. Multivariable model 2 was adjusted for Southern European/Mediterranean ancestry, married, living alone, smoking, alcohol intake, physical activity, multivitamin use, history of hypertension and hypercholesterolemia, in women postmenopausal status and menopausal hormone use, total energy intake, family history of dementia, history of depression, census SES, and BMI. Multivariable model 3 was further adjusted for intake of red meat, fruits and vegetables, nuts, soda, whole grains, and trans-fat, all indicative of diet quality.
In a secondary analysis we used the composite outcome for dementia-related deaths. We also repeated the main analysis in the genotyping subsample. We carried out mediation analyses to calculate the percentage of the association between olive oil intake and dementia-related mortality that is attributable to CVD, hypercholesterolemia, hypertension, and diabetes. We also performed stratified analyses by prespecified subgroups (eMethods in Supplement 1 ).
A joint analysis was performed to test whether olive oil intake (never or <1/mo, >0 to â€7g/d, and >7g/d) and the AMED or the AHEI score (tertiles) combined as the exposure was associated with dementia mortality. In substitution analyses, we assessed the risk of dementia-related mortality by replacing 5 g/d of different fat sources, including margarine, mayonnaise, butter, and a combination of other vegetable oils (corn, safflower, soybean, and canola), with olive oil. Both continuous variables as 5-g/d increments were included in a multivariable model 3, mutually adjusted for other types of fat. The difference in the coefficients obtained for olive oil and the substituted fat provided the estimated HR and 95% CI for substituting 5 g/d of olive oil for an equivalent amount of the other fats.
Several exploratory sensitivity analyses were performed including a 4-year lagged analysis, analyses adjusting for other covariates, a cause-specific competing risk model and analyses excluding participants who self-reported having dementia at baseline (nâ=â12) (eMethods in Supplement 1 ). Analyses were performed from May 2022 to July 2023 using SAS version 9.4 (SAS Institute). All statistical tests were 2-sided with an αâ=â.05.
Over 2âŻ183âŻ095 person-years of follow-up, this study documented a total of 4751 dementia deaths (3473 in NHS and 1278 in HPFS; 37âŻ649 total deaths). Among 92âŻ383 participants included at baseline in 1990, 60âŻ582 (65.6%) were women, and the mean (SD) age was 56.4 (8.0) years. Mean (SD) olive oil intake was 1.3 (2.5) g/d in both NHS and HPFS; the mean (SD) adherence score for the Mediterranean diet was 4.5 (1.9) points in the NHS and 4.2 (1.9) points in the HPFS; and the mean (SD) AHEI diet quality score was 52.5 (11.1) points in the NHS and 53.4 (11.6) points in the HPFS.
Table 1 shows baseline characteristics of participants categorized by total olive oil intake. Participants with a higher olive oil intake (>7 g/d) at baseline had an overall higher caloric intake, but not a higher BMI, had better diet quality, had higher alcohol intake, were more physically active, and were less likely to smoke compared with those never consuming olive oil or less than once per month ( Table1 ). Individuals who were homozygous for the APOE Δ4 allele were 5.5 to 9.4 times more likely to die with dementia compared with noncarriers for the APOE e4 allele (Ï 2 ï»ż P â<â.001) (eTable 1 in Supplement 1 ).
Olive oil intake was inversely associated with dementia-related mortality in age-stratified and multivariable-adjusted models ( Table 2 ). Compared with participants with the lowest olive oil intake, the pooled HR for dementia-related death among participants with the highest olive oil intake (>7 g/d) was 0.72 (95% CI, 0.64-0.81), after adjusting for sociodemographic and lifestyle factors. The association between each 5-g increment in olive oil consumption with dementia-related death was also inverse and significant in the pooled analysis. The multivariable-adjusted HR for dementia-related death for the highest compared with the lowest olive oil intake (>7 g/d) was 0.67 (95% CI, 0.59-0.77) for women and 0.87 (95% CI, 0.69-1.09) for men ( Table 2 ). Olive oil intake in 5-g increments was inversely associated with dementia-related mortality in women (HR, 0.88 [95% CI, 0.84-0.93]), but not in men (HR, 0.96 [95% CI, 0.88-1.04]). Analyses remained consistent when using the composite outcome for death with dementia (eTable 2 in Supplement 2 ). In the genotyping subsample, the results remained unchanged after further adjusting for the APOE Δ4 allelic genotype (multivariable-adjusted pooled HR comparing high vs low olive oil intake, 0.66 [95% CI, 0.54-0.81]; P for trendâ<â.001) (eTable 4 in Supplement 1 ). Pooled mediation analyses found that CVD, hypercholesterolemia, hypertension, and diabetes did not significantly attenuate the association (unchanged HRs with and without adjusting for the intermediate; data not shown).
In joint analyses, participants with the highest olive oil intake had a lower risk for dementia-related mortality, irrespective of their AMED score (28% to 34% lower risk compared with participants in the combined low olive oil and high AMED) ( Figure 1 A; eTable 3 in Supplement 1 ) and of their AHEI (27% to 38% lower risk compared with participants with low olive oil and high AHEI) ( Figure 1 B; eTable 3 in Supplement 1 ).
Replacing 5 g/d of mayonnaise with 5 g/d of olive oil was associated with a 14% (95% CI, 7%-20%) lower risk of dementia-related mortality in pooled multivariable-adjusted models ( Figure 2 ). As for the substitution of 5 g/d of margarine with the equivalent amount of olive oil, we estimated an 8% (95% CI, 4%-12%) lower risk. Substitutions of other vegetable oils or butter with olive oil were not statistically significant.
Exploratory subgroup analyses (eFigure in Supplement 1 ) showed associations between higher olive oil intake and lower risk of dementia-related mortality across most subgroups. No statistically significant associations were found in participants with a family history of dementia, living alone, using a multivitamin, and in nonâ APOE Δ4 carriers. Results from exploratory sensitivity analyses (eTables 5-8 in Supplement 1 ) were comparable with the findings from the main analysis (eResults in Supplement 1 ).
In 2 large US prospective cohorts of men and women, we found that participants who consumed more than 7 g/d of olive oil had 28% lower risk of dying from dementia compared with participants who never or rarely consumed olive oil. This association remained significant after adjustment for diet quality scores including adherence to the Mediterranean diet. We estimated that substituting 5 g/d of margarine and mayonnaise with olive oil was associated with significantly lower dementia-related death risk, but not when substituting butter and other vegetable oils. These findings provide evidence to support dietary recommendations advocating for the use of olive oil and other vegetable oils as a potential strategy to maintain overall health and prevent dementia.
In the NHS and HPFS, a lower risk of neurodegenerative disease mortality, including dementia mortality, was observed with higher olive oil consumption (HR, 0.81 [95% CI, 0.78-0.84]). 11 Evidence that pertains to cognitive decline or incident dementia is more widely available than it is for dementia mortality. 6 , 22 In the French Three-City Study (nâ=â6947), participants with the highest olive oil intake were 17% (95% CI, 1%-29%) less likely to experience a 4-year cognitive decline related to visual memory, but no association was found for verbal fluency (odds ratio [OR], 0.85 [95% CI, 0.70-1.03]). 22 Furthermore, participants with a higher intake of olive oil (moderate or intensive vs never) had a lower risk of verbal fluency and visual memory cognitive impairment. Potential sex differences were not investigated. In the PREDIMED trial, which supplemented a Mediterranean-style diet with extra-virgin olive oil (1 L/wk/household) or nuts (30 g/d), 23 the authors investigated cognitive effects and status in 285 and 522 cognitively healthy participants using global and in-depth neuropsychological battery testing. Although the study was not originally designed for cognitive outcomes and the effect of olive oil cannot be isolated, after 6.5 years, the olive oil group exhibited improved cognitive performance in verbal fluency and memory tests compared with a low-fat diet (control), and they were less prone to develop mild cognitive impairment (OR, 0.34 [95% CI, 0.12-0.97]; nâ=â285). 6 Global cognitive performance was higher in both the olive oil and the nut groups compared with the control post trial (nâ=â522). 8 These studies were conducted in Europe, in populations with typically higher olive oil intake compared with US populations.
Observational studies and some trials have consistently found associations between following diets such as the Mediterranean, DASH, MIND, and AHEI, and prudent patterns to healthier brain structure, 24 reduced cognitive impairment and Alzheimer risk, and improved cognitive function. 4 In our study, those with the highest olive oil intake (>7 g/d) had the lowest dementia-related death risk compared with those with minimal intake (never or less than once per month), regardless of diet quality. This highlights a potentially specific role for olive oil. Still, the group with both high AHEI scores and high olive oil intake exhibited the lowest dementia mortality risk (HR, 0.68 [95% CI, 0.58-0.79]; reference: low AHEI score and low olive oil intake), suggesting that combining higher diet quality with higher olive oil intake may confer enhanced benefit.
Olive oil consumption may lower dementia mortality by improving vascular health. 18 Several clinical trials support the effect of olive oil in reducing CVD via improved endothelial function, coagulation, lipid metabolism, oxidative stress, platelet aggregation and decreased inflammation. 25 Nonetheless, the results of our study remained independent of hypertension and hypercholesterolemia. Mild cognitive impairment, Alzheimer disease, and related dementias were associated with abnormal blood brain barrier permeability, possibly allowing the crossing of neurotoxic molecules into the brain. 26 Mechanistical evidence from animal 27 - 29 and human studies 9 , 30 have shown that phenolic compounds in olive oil, particularly extra-virgin olive oil, may attenuate inflammation, oxidative stress and restore blood brain barrier function, thereby reducing brain amyloid-ÎČ and tau-related pathologies and improving cognitive function. However, incident CVD, hypercholesterolemia, hypertension, and diabetes were not significant mediators of the association between olive oil intake and dementia-related death in our study.
The association was significant in both sexes but did not remain in men after full adjustment of the model. Some previous research has reported cognitive-related sex differences. Evidence from trials also showed sex- and/or gender-specific responses to lifestyle interventions for preventing cognitive decline, possibly due to differences in brain structure, hormones (sex) and social factors (gender). 31 Olive oil intake may be protective of dementia and related mortality, particularly in women. Nonetheless, we did not observe significant heterogeneity or interaction of cohort by olive oil intake on the risk of fatal dementia. Sex and gender differences should be carefully considered in future studies examining the association or effect of olive oil on cognitive-related outcomes to improve our understanding.
We found that using olive oil instead of margarine and mayonnaise, but not butter and other vegetable oils, was associated with a lower risk of dementia-related death. At the time of the study, margarine and mayonnaise contained considerable levels of hydrogenated trans-fats. The latter were strongly associated with all-cause mortality, CVD, type 2 diabetes, and dementia, 32 , 33 which may explain the lower dementia-related death risk observed when replacing it with olive oil. The US Food and Drug Administration banned manufacturers from adding partially hydrogenated oils to foods in 2020. 34 Future studies examining intake of trans-fatâfree margarine will be informative. Although the substitution of butter with olive oil was found to be associated with a lower risk of type 2 diabetes, CVD, and total mortality, 11 we did not find an association with the risk of dementia mortality. Although these previous studies did not examine the associations for butter per se, intake of regular fat dairy products, including cheese, yogurt, and milk, was reported to be either not associated or inversely associated with lower cognitive function, cognitive decline, and dementia. 35 - 37
Our cohort analyses include several strengths, namely the long follow-up period and large sample size with a high number of dementia death cases. Also, we included genotyping of the APOE Δ4 allele in a large subsample of participants to reduce potential confounding attributed to this well-known risk factor for Alzheimer disease. Additionally, our repeated diet measurements, weight, and lifestyle variables permitted us to account for long-term olive oil intake and confounding factors. Furthermore, the use of dietary cumulative average updates reduced random measurement error by considering within-person variations in intake.
This study has limitations. The possibility of reverse causation cannot be excluded due to the observational nature of our study. However, the 4-year lagged analysis results, consistent with the primary analysis, suggest that olive oil intake is predictive of dementia mortality rather than a consequence of premorbid dementia. While it is plausible that higher olive oil intake could be indicative of a healthier diet and higher SES, our results remained consistent after accounting for the latter. Despite adjusting for key covariates, residual confounding may remain due to unmeasured factors. Also, our study was conducted among health professionals. While this minimizes the potential confounding effects of socioeconomic factors and likely increases reporting due to a high level of education, this may also limit generalizability. Our population was predominantly of non-Hispanic White participants, limiting generalizability to more diverse populations. Additionally, we could not differentiate among various types of olive oil that differ in their polyphenols and other nonlipid bioactive compounds content.
This study found that in US adults, particularly women, consuming more olive oil was associated with lower risk of dementia-related mortality, regardless of diet quality. Substituting olive oil intake for margarine and mayonnaise was associated with lower risk of dementia mortality and may be a potential strategy to improve longevity free of dementia. These findings extend the current dietary recommendations of choosing olive oil and other vegetable oils to the context of cognitive health and related mortality.
Accepted for Publication: March 6, 2024.
Published: May 6, 2024. doi:10.1001/jamanetworkopen.2024.10021
Open Access: This is an open access article distributed under the terms of the CC-BY License . © 2024 Tessier AJ et al. JAMA Network Open .
Corresponding Authors: Anne-Julie Tessier, RD, PhD ( [email protected] ), and Marta Guasch-Ferré, PhD ( [email protected] ), Department of Nutrition, Harvard T.H. Chan School of Public Health, 655 Huntington Ave, Bldg 2, Boston, MA 02115.
Author Contributions: Drs Tessier and Guasch-Ferré had full access to all of the data in the study and take responsibility for the integrity of the data and the accuracy of the data analysis.
Concept and design: Tessier, Chavarro, Hu, Willett, Guasch-Ferré.
Acquisition, analysis, or interpretation of data: Tessier, Cortese, Yuan, Bjornevik, Ascherio, Wang, Chavarro, Stampfer, Willett, Guasch-Ferré.
Drafting of the manuscript: Tessier.
Critical review of the manuscript for important intellectual content: Tessier, Cortese, Yuan, Bjornevik, Ascherio, Wang, Chavarro, Stampfer, Hu, Willett, Guasch-Ferré.
Statistical analysis: Tessier, Cortese, Wang, Willett, Guasch-Ferré.
Obtained funding: Chavarro, Stampfer, Hu, Guasch-Ferré.
Administrative, technical, or material support: Cortese, Yuan, Stampfer, Hu.
Supervision: Chavarro, Hu, Guasch-Ferré.
Conflict of Interest Disclosures: Dr Cortese reported a speaker honorarium from Roche outside the submitted work. Dr Ascherio reported receiving speaker honoraria from WebMD, Prada Foundation, Biogen, Moderna, Merck, Roche, and Glaxo-Smith-Kline. No other disclosures were reported.
Funding/Support: This study is supported by the research grant R21 AG070375 from the National Institutes of Health to Dr Guasch-Ferré. The NHS, NHSII and HPFS are supported by grants from the National Institutes of Health (UM1 CA186107, P01 CA87969, U01 CA167552, P30 DK046200, HL034594, HL088521, HL35464, HL60712). Dr Tessier is supported by the Canadian Institutes of Health Research (CIHR) Postdoctoral Fellowship Award. Dr Guasch-Ferré is supported the Novo Nordisk Foundation grant NNF23SA0084103.
Role of the Funder/Sponsor: The funders had no role in the design and conduct of the study; collection, management, analysis, and interpretation of the data; preparation, review, or approval of the manuscript; and decision to submit the manuscript for publication.
Data Sharing Statement: See Supplement 2 .
- Register for email alerts with links to free full-text articles
- Access PDFs of free articles
- Manage your interests
- Save searches and receive search alerts
The BIGGEST CVEs of Q1 2024: Get the quarterly report
Attack surface management: A practitioner’s guide
Vulcan Cyber helped an enterprise payment platform reduce critical risk by 50% | Learn how >>
Vulcan Cyber named a RBVM Leader by The OMDIA Universe >>
New Whitepaper: The Top 5 Mistakes That Everyone in Vulnerability Remediation is Making
The Platform
Vulcan Connectors
Why Vulcan Cyber?
Vulcan Free FREE TOOL
CAPABILITIES
Vulnerability aggregation
Vulnerability correlation
Risk prioritization
Remediation orchestration
Risk reporting
Exposure management
Vulnerability Risk Management (RBVM)
Application Security Posture Management (ASPM)
Cloud Vulnerability Management
Financial services
Federal organizations
Retail industry
The Forrester Waveâą
Voyager18 Research
MITRE Mapper FREE TOOL
2023 Vulnerability Watch
2024 Vulnerability Watch NEW REPORT
The CyberRisk Summit - June 2024
GET TO KNOW US
Awards & accolades
GET IN TOUCH
Partner program
Deal registration
How-to guides
The complete guide to open-source security.
Everything you need to know about open-source security, including 7 best practices to shield your organization from a breach.
In this article
What is open-source security , which is better for security: open-source or proprietary software, 7 best practices and strategies to maintain security posture across open-source projects, top 10 open-source security tools and integrations, white paper.
The best free and open source tools for cyber risk assessment and mitigation
This article explores the threats, opportunities and best practices for open-source security. Following high-profile vulnerabilities such as the XZ backdoor threat , the unique challenges presented by open-source technology have come to the fore.
Here’s what you need to know.
TL;DR: Open-source security at a glance
Open-source security is comprised of best practices and measures designed to protect open-source software (OSS) from major threats and vulnerabilities.
Open-source is less secure than proprietary software since it is publically available and anyone can contribute to code in repositories, including malicious actors.
7 best practices include:
- Performing regular code reviews and audits
- Securing your Git repositories
- Implementing automated security testing
Understanding license compliance policies
- Searching vulnerability databases for vulnerable OSS packages
- Conducting a thorough OSS assessment
- Implementing threat modeling
Open-source security encompasses best practices and security measures designed to protect open-source software (OSS) projects from threats and vulnerabilities.
Open-source software (OSS) remains a staple for developers thanks in part to many connected communities, ease of use, and contributors to help review code.
However open-source software (OSS) presents major security challenges for organizations. A prime illustration of this is the case of GitHub, where a critical vulnerability was discovered in an open-source repository , leading to the exposure of over 4,000 repositories in a repojacking attack.
The npm registry alone contained 691 malicious packages, potentially installed inadvertently by developers.
malicious open-source packages found in npm and PyPI registries.
Yet, despite these challenges, developers made 301 million total contributions to open-source projects across GitHub in 2023.
There are numerous open-source security tools available to scan third-party libraries and dependencies for critical vulnerabilities.
When we talk security, both open-source and proprietary software have their advantages and disadvantages.
Open-source has many dedicated communities with incredibly talented and helpful developers who contribute to projects together.
If a bug arises, it will be most likely discussed, giving you a head start in patching or upgrading any software to the latest version.
The bad news is that open-source is public, which means anyone can access it at any given time, including a malicious actor.
Applications built with open-source code contain an average of seven vulnerabilities and 44% of those programs contain critical vulnerabilities.
Public access to open-source code increases the risk of backdoors or introducing insecure code into the CI/CD pipeline , potentially compromising the security of the entire software supply chain .
Third-party open-source libraries can also contain hidden vulnerabilities that can impact other projects if left unpatched.
Letâs dive deeper into the overall advantages and disadvantages of open-source vs proprietary software. Which is the right fit for your organization and existing infrastructure?
Open-source vs proprietary software
Perform regular code reviews and audits.
Research found that 84% of codebases have at least one open-source vulnerability. Without proper code reviews and audits, these vulnerabilities can remain undetected and make it into production.
Not only is the organization at risk, but their customers face potential malicious attacks as a result. Regular code reviews are also essential for ensuring that the projectâs codebases meet compliance best practices.
Secure your Git repositories:
Storing credentials in repositories is risky business. In 2023, 12.8 million secrets were accidentally leaked on public GitHub repositories by developers – a 28% increase from the previous year.
The exposed secrets contained API keys, OAuth tokens, TLS/SSL certificates, and credentials to log into cloud services.
Organizations must routinely review their repositories and remove any stored credentials that are no longer in use to prevent secrets exposure. Limit access to code contributors and ensure that 2FA is always enabled.
Implement automated security testing
Testing for vulnerabilities early in the SDLC is imperative. Automated security scanning tools such as Sast, Dast, and SCA can easily identify potential security risks in the codebase before they escalate into more serious issues during later stages of deployment.
These tools provide DevOps teams with plenty of time to remediate critical vulnerabilities and patch software before it gets pushed into production.
Open-source projects often utilize third-party libraries that are governed by specific licenses. Understanding the various license policies can help prevent potential copyright lawsuits or violations of any licensing agreements.
This is especially important as most open-source projects are heavily dependent on collaborations and contributions from a large connected community of developers such as GitHub or Stack Overflow.
Search vulnerability databases for vulnerable OSS packages
Routinely check vulnerability databases such as CVE details and the National Vulnerability Database (NVD) from NIST for OSS packages that might have been impacted in a potential breach.
The XZ Utils backdoor (CVE-2024-3094) recently made headlines when malicious actors exploited a massive software supply chain vulnerability, causing ripples in the Linux community.
Vulcan Cyber suggests following CISA recommendations of downgrading to an unaffected XZ Utils version (v5.6.0 and previous versions) and conducting thorough checks for any signs of suspicious activity on systems running the affected versions.
Conduct a thorough OSS assessment
A cyber risk assessment performed early can spare you the expenses of a potential breach later on. A security assessment of your OSS packages should include a thorough code review, license compliance check, and dependency analysis to remove any outdated components if needed.
Comprehensive vulnerability scanning should also be performed to identify any potentially malicious OSS packages.
Implement threat modeling:
Threat modeling helps identify all assets that are at high risk for a potential attack. These assets include user credentials, source code, and sensitive data.
Once identified, security teams are then able to determine appropriate mitigation strategies, such as implementing tighter access controls to Git repositories and cloud services.
Learn >> The best free and open-source tools for cyber risk assessment and mitigation
Category: Code scanning
About: The Sonatype code scanner automatically enforces open-source security policies and blocks bad component downloads.
GitHub Code Scanning
About: Analyze code in a GitHub repository to find security vulnerabilities and coding errors.
GitHub Dependabot
Category: Dependency review
About: Dependabot allows you to review code-project vulnerabilities and fix vulnerable dependencies in your repository.
Category: Threat intelligence
About: MISP is a threat intelligence and visibility tool. You can import and integrate MISP, threatintel, or OSINT feed from third parties.
Category: Network scanning
About: Network Mapper, commonly known as Nmap, is an open-source network scanning tool that allows users to discover hosts and services by sending packets and analyzing their responses.
Category: Code collaboration
About: GitLab is an open-source code repository and collaborative software development platform for DevSecOps.
JFrog Artifactory OSS
Category: Artifact/binary scanning
About JFrog Artifactory OSS enables you to manage Java binary artifacts centrally.
Vulcan Cyber integrates with JFrog Xray to keep your software supply chain secured.
JFrog Xray is a SCA solution that natively integrates with Artifactory. It identifies vulnerabilities in open-source and license compliance violations.
Category: K8s cluster monitoring
About: Prometheus is an open-source monitoring and alerting toolkit originally built at SoundCloud.
It offers insights into the health and performance of Kubernetes clusters through a collection of metrics which it stores as time series data.
About: Wazuh is an open-source security platform that blends XDR and SIEM capabilities for endpoints and cloud workloads.
It provides threat intelligence features such as vulnerability detection and log data analysis.
Category: CI/CD security
About: Jenkins is an open-source CI/CD server that helps developers automate the process of building, testing, and deploying software applications.
Strengthen your open-source security with Vulcan Cyber
A malicious open-source package can create a ripple effect in your software supply chain and put your entire organization at risk for a breach.
The Vulcan Cyber platform provides total visibility over your entire software supply chain in a single operational view.
Get a better understanding of your assets at risk and prioritize mitigating vulnerable application code. Vulcan Cyber provides security teams with contextualized insights from 20+ threat intelligence feeds.
Improve application vulnerability management and open-source security with Vulcan Cyber.
Get a demo to learn more.
Free for risk owners
Set up in minutes to aggregate and prioritize cyber risk across all your assets and attack vectors.
âThe only free RBVM tool out there The only free RBVM tool lorem ipsum out there. The onlyâ.
Name Namerson Head of Cyber Security Strategy
Free for risk owners.
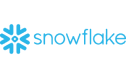
Platform Overview
Vulcan Free
Vulnerability Aggregation
Vulnerability Correlation
Vulnerability Intelligence
Vulnerability Prioritization
Management Orchestration
Collaborative Security
Risk Reporting
Exposure Management
Risk-Based Vulnerability Management
Application Vulnerability Management
Financial Services
Federal Organizations
Retail Industry
CYBER RISK HUB
Vulnerability Management Basics
MITRE Mapper
CyberRisk Summit
Become a Partner
Deal Registration
Awards and Recognition
Copyright © 2024 Vulcan Cyber. All rights reserved. Privacy Policy | Terms of Use
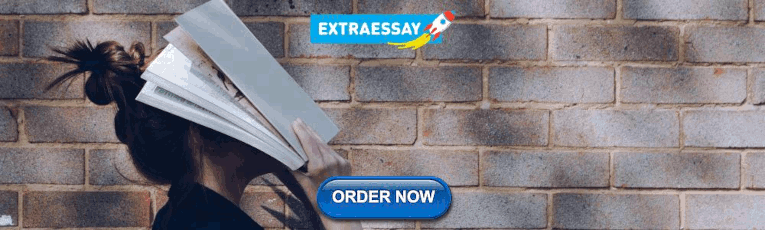
IMAGES
VIDEO
COMMENTS
About the directory. DOAJ is a unique and extensive index of diverse open access journals from around the world, driven by a growing community, and is committed to ensuring quality content is freely available online for everyone. DOAJ is committed to keeping its services free of charge, including being indexed, and its data freely available.
Make an impact and build your research profile in the open with ScienceOpen. Search and discover relevant research in over 93 million Open Access articles and article records; Share your expertise and get credit by publicly reviewing any article; Publish your poster or preprint and track usage and impact with article- and author-level metrics; Create a topical Collection to advance your ...
When you choose to publish with PLOS, your research makes an impact. Make your work accessible to all, without restrictions, and accelerate scientific discovery with options like preprints and published peer review that make your work more Open. ... PLOS publishes a suite of influential Open Access journals across all areas of science and ...
The SpringerOpen portfolio has grown tremendously since its launch in 2010, so that we now offer researchers from all areas of science, technology, medicine, the humanities and social sciences a place to publish open access in journals. Publishing with SpringerOpen makes your work freely available online for everyone, immediately upon ...
arXiv is a free distribution service and an open-access archive for nearly 2.4 million scholarly articles in the fields of physics, mathematics, computer science, quantitative biology, quantitative finance, statistics, electrical engineering and systems science, and economics. Materials on this site are not peer-reviewed by arXiv.
JSTOR hosts a growing number of public collections, including Artstor's Open Access collections, from museums, archives, libraries, and scholars worldwide. Research reports. A curated set of more than 34,000 research reports from more than 140 policy institutes selected with faculty, librarian, and expert input.
IEEE Access, a Multidisciplinary, Open Access Journal. IEEE Access is a multidisciplinary, online-only, gold fully open access journal, continuously presenting the results of original research or development across all IEEE fields of interest. Supported by article processing charges (APCs), its hallmarks are rapid peer review, a submission-to ...
Hybrid open access is a mixed model where journals publish both Hybrid and subscription content. It allows authors to pay an article publication charge and publish specific work as Gold open access papers. As an author, you can benefit from Hybrid open access because it allows you to publish with trusted journals.
Open access (OA) refers to the free, immediate, online availability of research outputs such as journal articles or books, combined with the rights to use these outputs fully in the digital environment. OA content is open to all, with no access fees. Open research goes beyond the boundaries of publications to consider all research outputs ...
Abstract. This paper introduces CORE, a widely used scholarly service, which provides access to the world's largest collection of open access research publications, acquired from a global ...
Aggregation plays an increasingly essential role in maximising the long-term benefits of open access, helping to turn the promise of a 'research commons' into a reality. The aggregation services that CORE provides therefore make a very valuable contribution to the evolving open access environment in the UK. Show all.
The Journal of Open Source Software is a. developer friendly. , open access journal for research software packages. Committed to publishing quality research software with zero article processing charges or subscription fees. Submit a paper to JOSS đ Volunteer to review. Explore Papers Documentation Learn More.
Open research reports. JSTOR hosts a growing curated collection of more than 50,000 open research reports from 187 think tanks and research institutes from around the world. These publications are freely accessible to everyone on JSTOR and discoverable as their own content type alongside journals, books, and primary sources. We update research ...
MIT Open Access Articles. The MIT Open Access Articles collection consists of scholarly articles written by MIT-affiliated authors that are made available through DSpace@MIT under the MIT Faculty Open Access Policy, or under related publisher agreements. Articles in this collection generally reflect changes made during peer-review.
The world's most significant open access portfolio. We have published over 124,000 open access articles via Gold open access across disciplines -from the life sciences to the humanities, representing 33% of all Springer Nature articles in 2020. Authors can also publish their article under an open access licence in more than 2,200 of our ...
Open research in computer science. Spanning networks and communications to security and cryptology to big data, complexity, and analytics, SpringerOpen and BMC publish one of the leading open access portfolios in computer science. Learn about our journals and the research we publish here on this page.
1. Introduction. Free Open-Source Software (FOSS) is essentially seen as a key pillar for many public and private sector organizations due to potential benefits that generally include cost reduction, improved security and interoperability, as well as a substantial increase in system quality and ability (Sanchez et al., 2020).Thus, as with many governments (AlMheiri et al., 2018, Silic and Back ...
OATD.org aims to be the best possible resource for finding open access graduate theses and dissertations published around the world. Metadata (information about the theses) comes from over 1100 colleges, universities, and research institutions. OATD currently indexes 7,241,108 theses and dissertations. About OATD (our FAQ). Visual OATD.org
This paper accomplishes these two goals and demonstrates that: 1) managers of free and open source software projects do change the distribution rights of their source code through a change in the ...
The Open Research Library (ORL) is planned to include all Open Access book content worldwide on one platform for user-friendly discovery, offering a seamless experience navigating more than 20,000 Open Access books.
Enrich your research with primary sources Enrich your research with primary sources. ... Part of Open: Smithsonian ... (Nov. 1, 2020) Part of Leuven University Press. Part of UN Secretary-General Papers: Ban Ki-moon (2007-2016) Part of Perspectives on Terrorism, Vol. 12, No. 4 (August 2018)
The recent surge in open-source Large Language Models (LLMs), such as LLaMA, Falcon, and Mistral, provides diverse options for AI practitioners and researchers. However, most LLMs have only released partial artifacts, such as the final model weights or inference code, and technical reports increasingly limit their scope to high-level design choices and surface statistics. These choices hinder ...
Through using this information, open-source intelligence (OSINT) seeks to meet basic intelligence requirements. Although open-source information has historically been synonymous with strategic intelligence, today's consumers range from governments to corporations to everyday people. This paper aimed to describe open-source intelligence and to ...
This paper introduces RoboCar, an open-source research platform for autonomous driving developed at the University of Luxembourg. RoboCar provides a modular, cost-effective framework for the development of experimental Autonomous Driving Systems (ADS), utilizing the 2018 KIA Soul EV. The platform integrates a robust hardware and software architecture that aligns with the vehicle's existing ...
The Medical Physics publishes papers helping health professionals perform their responsibilities more effectively and efficiently. Skip to Article Content ... Software from publicly funded research should be free and open source for research. Brendan M. Whelan, Brendan M. Whelan. University of Sydney, Image X Institute, Sydney, New South Wales ...
Schools are considered knowledge-creating organisations that find it difficult to develop and implement innovations on their own. Knowledge mobilisation is seen as the key to overcoming this problem. In particular, the use of external sources of knowledge is regarded as an important lever for change. However, there is a lack of concepts and empirical studies in educational research on the ...
The Online Writing Lab at Purdue University houses writing resources and instructional material, and we provide these as a free service of the Writing Lab at Purdue.
Meta AI's first Llama model release in February 2023 was a big breakthrough for openly available LLM and was a pivotal moment for open(-source) LLMs. So, naturally, everyone was excited about the Llama 2 release last year. Now, the Llama 3 models, which Meta AI has started to roll out, are similarly exciting.. While Meta is still training some of their largest models (e.g., the 400B variant ...
Funding/Support: This study is supported by the research grant R21 AG070375 from the National Institutes of Health to Dr Guasch-Ferré. The NHS, NHSII and HPFS are supported by grants from the National Institutes of Health (UM1 CA186107, P01 CA87969, U01 CA167552, P30 DK046200, HL034594, HL088521, HL35464, HL60712).
White paper. The best free and open source tools for cyber risk assessment and mitigation. Download. This article explores the threats, opportunities and best practices for open-source security. ... Research found that 84% of codebases have at least one open-source vulnerability. Without proper code reviews and audits, these vulnerabilities can ...