The Law of Small Numbers in Financial Markets: Theory and Evidence
We build a model of the law of small numbers (LSN)—the incorrect belief that even small samples represent the properties of the underlying population—to study its implications for trading behavior and asset prices. In our model, a belief in the LSN induces investors to expect short-term price trends to revert and long-term price trends to persist. As a result, asset prices exhibit short-term momentum and long-term reversals. The model can reconcile the coexistence of the disposition effect and return extrapolation. In addition, it makes new predictions about investor behavior, including return patterns before purchases and sales, a weakened disposition effect for long-term holdings, doubling down in buying, a positive correlation between doubling down and the disposition effect, and heterogeneous selling propensities to past returns. By testing these predictions using account-level transaction data, we show that the LSN provides a parsimonious way of understanding a variety of puzzles about investor behavior.
We thank Nicholas Barberis, Francesca Bastianello, James Choi, Paul Fontanier, Cary Frydman, David Hirshleifer, Marco Sammon, Dimitri Vayanos, and seminar participants at the City University of Hong Kong, Cornell University, the London School of Economics, the University of Florida, and the University of Notre Dame for helpful discussions and comments. We are grateful to Terrance Odean for providing the data. This paper supersedes an earlier working paper “Investor Behavior Under the Law of Small Numbers.” Peng acknowledges financial support received during Ph.D. from a Whitebox Advisors research grant. Allen Hu and Andrew Wang provided excellent research assistance. The views expressed herein are those of the authors and do not necessarily reflect the views of the National Bureau of Economic Research.
MARC RIS BibTeΧ
Download Citation Data
- data appendix
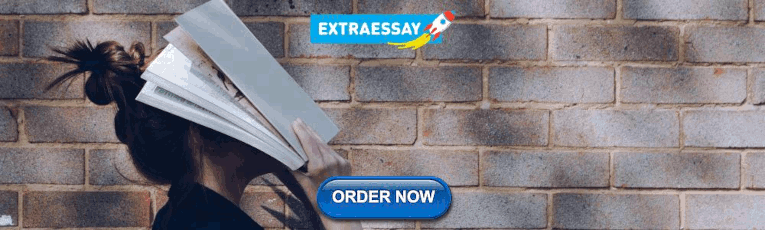
Working Groups
More from nber.
In addition to working papers , the NBER disseminates affiliates’ latest findings through a range of free periodicals — the NBER Reporter , the NBER Digest , the Bulletin on Retirement and Disability , the Bulletin on Health , and the Bulletin on Entrepreneurship — as well as online conference reports , video lectures , and interviews .
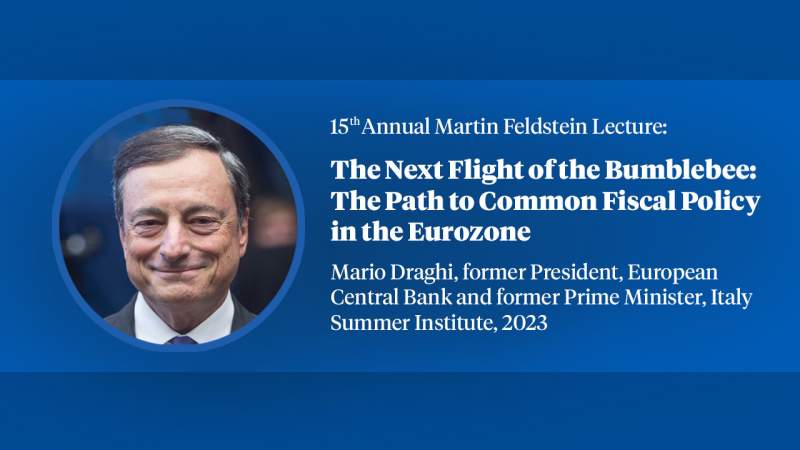
The effect of ETFs on financial markets: a literature review
- Published: 28 February 2020
- Volume 34 , pages 165–178, ( 2020 )
Cite this article
- Luca J. Liebi ORCID: orcid.org/0000-0003-4189-463X 1
5907 Accesses
18 Citations
Explore all metrics
Exchange-traded funds (ETFs) belong to the fastest growing investment products worldwide. Within 15 years, total assets invested in ETFs have twenty-folded, reaching over $3.7 trillion at the end of 2018. Increasing demand for passive investments, coupled with high liquidity and low transaction costs, are key advantages of ETFs compared to their closest substitutes such as traditional index funds. Besides the continuous growth of ETFs, the Flash Crash in 2010 triggered detailed investigations by regulators on how ETFs affect the financial market. This literature review provides a broad overview of recent academic studies analyzing the effect of ETFs on liquidity, price discovery, volatility, and comovement of the underlying securities.
This is a preview of subscription content, log in via an institution to check access.
Access this article
Price includes VAT (Russian Federation)
Instant access to the full article PDF.
Rent this article via DeepDyve
Institutional subscriptions
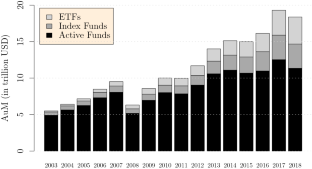
Similar content being viewed by others
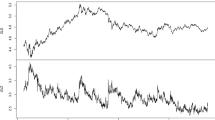
Re-examining the leverage effect and gold’s safe haven properties with the utilization of the implied volatility of gold: a non-parametric quantile regression approach
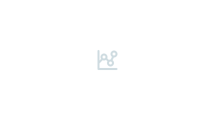
Return and volatility of emerging markets leveraged ETFs
The Downturn of Stock Market and the Irrational Exuberance of Leveraged Funds: A Case Study on Yinhuaxinli in SZSE
U.S. Securities and Exchange Commission ( 1988 ).
Investment Company Institute ( 2019 ).
CFTC ( 2010a ), CFTC ( 2010b ).
Adams, Z., Glück, T.: Financialization in commodity markets: a passing trend or the new normal? J. Bank. Finance 60 , 93–111 (2015)
Article Google Scholar
Agapova, A.: Conventional mutual index funds versus exchange-traded funds. J. Financial Markets 14 , 323–343 (2011)
Agarwal, V., Hanouna, P.E., Moussawi, R., Stahel, C.W.: (2018) Do ETFs increase the commonality in liquidity of underlying stocks? Working paper, Villanova University
Antoniewicz, R.S., Heinrichs, J.: (2014) Understanding exchange-traded funds: How ETFs work. In: ICI Research Perspective, vol. 20, pp. 11–13
Appel, I.R., Gormley, T.A., Keim, D.B.: Passive investors, not passive owners. J. Financ. Econ. 121 , 111–141 (2016)
Bae, K.H., Wang, J., Kang, J.K.: (2012) The costs of ETF membership: valuation effect of ETFs on underlying firms, Working paper, York University
Barberis, N., Shleifer, A., Wurgler, J.: Comovement. J. Financ. Econ. 75 , 283–317 (2005)
Ben-David, I., Franzoni, F., Moussawi, R.: Hedge fund stock trading in the financial crisis of 2007–2009. Rev. Financ. Stud. 25 , 1–54 (2012)
Bae, K.H., Wang, J., Kang, J.K.: (2016) Exchange traded funds (ETFs), Working paper, Ohio State University
Ben-David, I., Franzoni, F., Moussawi, R.: Do ETFs increase volatility? J. Finance 73 , 2471–2535 (2018)
Blocher, J., Whaley, R.E.: (2016) Two-sided markets in asset management: exchange-traded funds and securities lending, Working paper, Vanderbilt University
Boehmer, B., Boehmer, E.: Trading your neighbor’s ETFs: competition or fragmentation? J. Bank. Finance 27 , 1667–1703 (2003)
Borkovec, M., Domowitz, I., Serbin, V., Yegerman, H.: Liquidity and price discovery in exchange-traded funds: one of several possible lessons from the flash crash. J. Index Invest. 1 , 24–42 (2010)
Bradley, H.S., Litan, R.E.: (2010) Choking the recovery: why new growth companies aren’t going public and unrecognized risks of future market disruptions, Working paper, Ewing Marion Kauffman Foundation
Bradley, H.S., Litan, R.E.: (2011) ETFs and the present danger to capital formation, Working paper, Ewing Marion Kauffman Foundation
Carhart, M.M.: On persistence in mutual fund performance. J. Finance 52 , 57–82 (1997)
Carlson, M. (2007) A brief history of the 1987 stock market crash with a discussion of the federal reserve response, Working paper, Finance and Economics Discussion Series
CFTC (2010a) Findings regarding the market events of may 6, 2010, Report of the Staffs of the CFTC and SEC to the Joint Advisory Committee on Emerging Regulatory Issues
CFTC (2010b) Preliminary findings regarding the market events of may 6, 2010, Report of the Staffs of the CFTC and SEC to the Joint Advisory Committee on Emerging Regulatory Issues
Chan, W., Shelton, B., Wu, Y.: Volatility spillovers arising from the financialization of commodities. J. Risk Financ. Manag. 11 , 72 (2018)
Chang, Y.-C., Hong, H., Liskovich, I.: Regression discontinuity and the price effects of stock market indexing. Rev. Financ. Stud. 28 , 212–246 (2015)
Cheng, M., Madhavan, A.: The dynamics of leveraged and inverse exchange-traded funds. J. Invest. Manag. 7 , 43–62 (2009)
Google Scholar
Curcio, R.J., Anderson, R.I., Guirguis, H., Boney, V.: Have leveraged and traditional ETFs impacted the volatility of real estate stock prices? Appl. Financ. Econ. 22 , 709–722 (2012)
Da, Z., Shive, S.: (2013) When the bellwether dances to noise: evidence from exchange-traded funds, Working paper, University of Notre Dame
Dannhauser, C.D.: The impact of innovation: evidence from corporate bond ETFs. J. Financ. Econ. 125 , 537–560 (2017)
Deville, L., Gresse, C., de Séverac, B.: Direct and indirect effects of index ETFs on spot-futures pricing and liquidity: evidence from the CAC 40 index. Eur. Financ. Manag. 20 , 352–373 (2014)
Diebold, F.X., Yilmaz, K.: Better to give than to receive: predictive directional measurement of volatility spillovers. Int. J. Forecast. 28 , 57–66 (2012)
Engle, R.: Dynamic conditional correlation: a simple class of multivariate generalized autoregressive conditional heteroskedasticity models. J. Bus. Econ. Stat. 20 , 339–350 (2002)
Foucher, I., Gray, K.: Exchange-traded funds: evolution of benefits, vulnerabilities and risks. Bank Canada Financ. Syst. Rev., 37–46 (2014)
Gastineau, G.L.: Exchange-traded funds. J. Portf. Manag. 27 , 88–96 (2001)
Glosten, L., Nallareddy, S., Zou, Y.: (2015) ETF trading and informational efficiency of underlying securities. Management Science. forthcoming
Greenwood, R.M., Sosner, N.: Trading patterns and excess comovement of stock returns. Financ. Anal. J. 63 , 69–81 (2007)
Gruber, M.J.: Another puzzle: the growth in actively managed mutual funds. J. Finance 51 , 783–810 (1996)
Hamm, S.J.W.: (2014) The effect of ETFs on stock liquidity, Working paper, Tulane University
Hegde, S.P., McDermott, J.B.: The market liquidity of DIAMONDS, Q’s, and their underlying stocks. J. Bank. Finance 28 , 1043–1067 (2004)
Hill, J.M.: The evolution and success of index strategies in ETFs. Financ. Anal. J. 72 , 8–13 (2016)
Investment Company Institute (2019) Investment company fact book–a review of trends and activities in the U.S. investment company industry, vol. 59, pp. 194–202
Israeli, D., Lee, C., Sridharan, S.: Is there a dark side to exchange traded funds? An information perspective. Rev. Account. Stud. 22 , 1048–1083 (2017)
Jensen, M.C.: The performance of mutual funds in the period 1945–1964. J. Finance 23 , 389–416 (1968)
Krause, T., Ehsani, S., Lien, D.: Exchange-traded funds, liquidity and volatility. Appl. Financ. Econ. 24 , 1617–1630 (2014)
Lettau, M., Madhavan, A.: Exchange-traded funds 101 for economists. J. Econ. Perspect. 32 , 135–153 (2018)
Lin, C.-C., Chiang, M.-H.: Volatility effect of ETFs on the constituents of the underlying Taiwan 50 Index. Appl. Financ. Econ. 15 , 1315–1322 (2005)
Madhavan, A.: Exchange-traded funds, market structure, and the flash crash. Financ. Anal. J. 68 , 20–35 (2012)
Madhavan, A., Sobczyk, A.: Price dynamics and liquidity of exchange-traded funds. J. Invest. Manag. 14 , 1–17 (2016)
Malamud, S.: (2016) A dynamic equilibrium model of ETFs, Working paper, Swiss Finance Institute
Marshall, B.R., Nguyen, N.H., Visaltanachoti, N.: (2015) ETF liquidity, Working paper, Massey University
Morck, R., Yang, F.: (2001) The mysterious growing value of S&P 500 membership, Working paper, University of Alberta
Pan, K., Zeng, Y.: (2017) ETF arbitrage under liquidity mismatch, Working paper, Harvard University
Richie, N., Madura, J.: Impact of the QQQ on liquidity and risk of the underlying stocks. Q. Rev. Econ. Finance 47 , 411–421 (2007)
Staer, A., Sottile, P.: Equivalent volume and comovement. Q. Rev. Econ. Finance 68 , 143–157 (2018)
Trainor, W.J.: Do leveraged ETFs increase volatility. Technol. Invest. 1 , 215–220 (2010)
U.S Securities and Exchange Commission (1988) The October 1987 market break, A report by the Division of Market Regulation
Wang, H., Xu, L.: Do exchange-traded fund flows increase the volatility of the underlying index? Evidence from the emerging market in China. Account. Finance 58 , 1525–1548 (2019)
Wermers, R., Xue, J.: (2015) Intraday ETF trading and the volatility of the underlying, Working paper, University of Maryland
Wurgler, J.: (2010) On the economic consequences of index-linked investing, Working paper, New York University
Download references
Acknowledgements
The author thanks Prof. Dr. Manuel Ammann as well as Prof. Dr. Markus Schmid for their constructive and insightful comments.
Author information
Authors and affiliations.
Swiss Institute of Banking and Finance, University of St. Gallen, Unterer Graben 21, 9000, St. Gallen, Switzerland
Luca J. Liebi
You can also search for this author in PubMed Google Scholar
Corresponding author
Correspondence to Luca J. Liebi .
Additional information
Publisher's note.
Springer Nature remains neutral with regard to jurisdictional claims in published maps and institutional affiliations.
Rights and permissions
Reprints and permissions
About this article
Liebi, L.J. The effect of ETFs on financial markets: a literature review. Financ Mark Portf Manag 34 , 165–178 (2020). https://doi.org/10.1007/s11408-020-00349-1
Download citation
Published : 28 February 2020
Issue Date : June 2020
DOI : https://doi.org/10.1007/s11408-020-00349-1
Share this article
Anyone you share the following link with will be able to read this content:
Sorry, a shareable link is not currently available for this article.
Provided by the Springer Nature SharedIt content-sharing initiative
- Exchange-traded funds
- Price discovery
JEL Classification
- Find a journal
- Publish with us
- Track your research
Thank you for visiting nature.com. You are using a browser version with limited support for CSS. To obtain the best experience, we recommend you use a more up to date browser (or turn off compatibility mode in Internet Explorer). In the meantime, to ensure continued support, we are displaying the site without styles and JavaScript.
- View all journals
- My Account Login
- Explore content
- About the journal
- Publish with us
- Sign up for alerts
- Open access
- Published: 04 February 2021
Sentiment correlation in financial news networks and associated market movements
- Xingchen Wan 1 na1 ,
- Jie Yang 2 , 3 na1 ,
- Slavi Marinov 4 ,
- Jan-Peter Calliess 1 ,
- Stefan Zohren 1 &
- Xiaowen Dong 1
Scientific Reports volume 11 , Article number: 3062 ( 2021 ) Cite this article
22k Accesses
28 Citations
7 Altmetric
Metrics details
- Complex networks
- Computational science
- Information technology
In an increasingly connected global market, news sentiment towards one company may not only indicate its own market performance, but can also be associated with a broader movement on the sentiment and performance of other companies from the same or even different sectors. In this paper, we apply NLP techniques to understand news sentiment of 87 companies among the most reported on Reuters for a period of 7 years. We investigate the propagation of such sentiment in company networks and evaluate the associated market movements in terms of stock price and volatility. Our results suggest that, in certain sectors, strong media sentiment towards one company may indicate a significant change in media sentiment towards related companies measured as neighbours in a financial network constructed from news co-occurrence. Furthermore, there exists a weak but statistically significant association between strong media sentiment and abnormal market return as well as volatility. Such an association is more significant at the level of individual companies, but nevertheless remains visible at the level of sectors or groups of companies.
Similar content being viewed by others
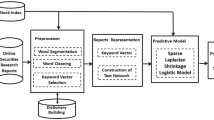
Incorporating textual network improves Chinese stock market analysis
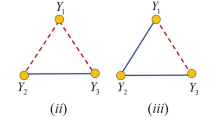
Loss of structural balance in stock markets
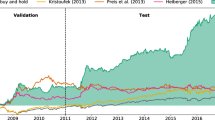
Revisiting the use of web search data for stock market movements
Introduction.
Complexity and inter-dependencies have been the defining features of most modern financial markets: a myriad of ever-changing interactions between market participants, financial assets and relations with broader macroeconomic factors have all contributed to intricate market dynamics. Yet, with recent examples of failures of financial systems triggering broader economic avalanches 1 , it has been both an empirical and an academic goal to untangle and shed new insights into the mechanisms of financial markets.
While classical economic models have often been found inadequate in explaining the lower-level market dynamics 2 , there has been increasing interest and success, to varying degrees, in applying data-driven and computational modelling approaches in this context. One prominent example is the recent attempt to model financial markets as computational networks, with the individual components (for example, the individual stocks or other financial assets) being the nodes and the correlation or some other form of relation between these components being edges 3 , 4 , 5 , 6 . This model and its variants have been used, for example, to model the propagation of systemic risk in wider economic systems at a global scale 7 and to assess default risk propagation in sectorial networks 8 .
Concurrently, with the advancement of natural language processing (NLP) techniques 9 , 10 , the algorithmic analysis of large-scale unstructured data that were previously not amenable to numerical processing (such as text data in financial news articles or research reports) has become possible. In this aspect, works have been dedicated to constructing networks of news and sentiment for various tasks, including sentiment analysis and risk/volatility monitoring 11 , 12 . Nonetheless, to the best of our knowledge, there still is a lack of studies combining the study of the correlation of sentiment and the corresponding market movements: graph-based market dynamics analysis and NLP-based analysis on financial markets have been largely taken as independent directions so far—while there have been studies on the market impact of sentiment 13 , 14 , the analysis has been mostly restricted to the investigation on individual companies. This is clearly inadequate and sub-optimal: the evolution and collective dynamics of news and the sentiment network can be equally important, and non-trivial relations may exist between them that go beyond the individual company level.
In this work, we aim to bridge this gap by combining network analysis and NLP techniques to study sentiment correlation and its associated movements of the financial market. We focus on a collection of companies (which also contain many with the world’s largest market capitalisation) that appear most frequently in news on Reuters. Specifically, we acquire news articles from Reuters on those companies of interest from 2007 to 2013: the period was deemed to span the late stages of pre-crisis boom, the 2008 Recession, the European debt crisis, and many important turns of events in the global markets. We apply NLP techniques to distill sentiment information and construct a network of 87 target companies based on the news information. We then perform community detection on this network and find that the groups of highly related companies obtained are often in close agreement with the conventional sectorial classification.
The news occurrence network allows us to study correlation dynamics of sentiment: we identify the events when the target companies experience extreme changes in sentiment, and find that these events are often correlated with movements of sentiment in the aforementioned groups of related companies. To analyse the market movements associated with sentiment, we further acquire daily Bloomberg market data on the target companies during the same period. We relate the sentiment events to market movements, and find that these events in expectation induce unusual movements in return and volatility in not only the companies experiencing the events themselves, but also at the sectorial or group level. In light of those findings, we would also like to advocate the pipeline of analysis used in this paper as a general-purpose, real-time framework for network analysis of financial news sentiment correlation and the corresponding market movements.
Evolution of sentiment
Through NLP techniques, we detect entities (i.e., companies) from financial news articles on Reuters, and merge entities that co-reference the same company. We compute a sentiment value for each of the companies that appears in a news article (see “ Methods ” section for details of the NLP processing). We end up selecting 87 companies in 9 sectors, listed in Table S1 . In Fig. 1 a, we visualize the evolution of news sentiment of the 87 companies for 27 quarters in our data. Each cell represents the average sentiment of the corresponding company in a specific quarter. Sentiment value is represented using cell color, with positive sentiment in red and negative sentiment in blue. We observe that banks in the U.S. ( LEHMQ, JPM, GS ) experienced strong negative sentiment around the financial crisis in September 2008, which indicates that those US banks were significantly affected in the crisis. However, the sentiment of Canadian banks ( BMO, RY ) did not suffer as much from the financial crisis, which is in line with the perception at the time 15 .
In Figure S2 , we furthermore present the average sentiment of each sector for each quarter, obtained by averaging the sentiment of all the companies in the sector. We observe that, companies in the “Technology” sector had a relatively stable sentiment distribution along all the quarters, but those in the “Financial Services” sector was more volatile. Overall, most sectors had negative sentiment in the financial crisis quarters, which clearly implied the impact of the crisis. Sentiment of the “Energy” and “Financial Services” sectors were negative in most quarters, which is consistent with sentiment experienced by individual companies in those sectors.
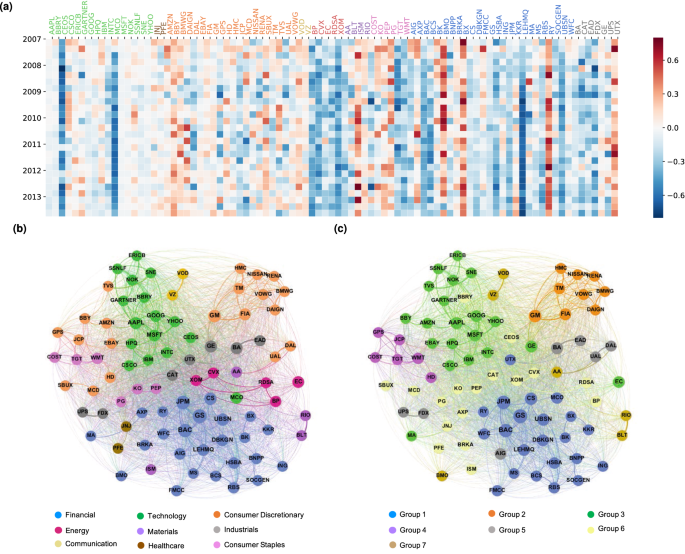
News co-occurrence network and sentiment distribution. ( a ) Sentiment distribution for 87 target companies in 27 quarters from 2007 to 2013. Colors in x-tick represent different sectors; ( b ) news co-occurrence network colored with sector; ( c ) news co-occurrence network colored with detected groups.
News co-occurrence network
The financial market is a system with complex inter-dependencies between companies. Co-appearance of companies in the news may reveal the underlying relations between them, thus provide a unique perspective to study such inter-dependencies. To this end, we first construct a news coverage matrix where each row represents a company and each column represents a news in the period of study. The ij -th entry of the matrix is the number of news articles in which company i appear on news j . From the news coverage matrix, we construct a weighted news co-occurrence network using the first year (2007) of the news data, where nodes represent companies and edges capture the relationships between pairs of companies. The edge weight \(e_{ij}\) between a pair of nodes i and j is defined as the cosine distance between the corresponding row vectors of the news coverage matrix. To analyse the structure of the news co-occurrence network, we apply community detection 16 on the network (we detail the technique in “ Methods ” section) to yield 7 major groups of companies, a number that is comparable to the 9 ground-truth sectors: it is worth noting that some sectors (such as Communications and Healthcare. See Table S1 ) are very small, and as such forcing the community detection method to produce the exact number of corresponding groups by tweaking the timescale parameter leads to unsatisfactory partitioning of groups. We present the groups obtained in Fig. 1 c, and we also present the visualisation of the companies’ “ground-truth” Bloomberg sector grouping in Fig. 1 b. We use the software package Gephi 17 for the visualisations, and companies in the same sector or group are labeled with the same color.
Through the comparison between Fig. 1 b, c, we observe a high-level consistency between the major company groups detected in the co-occurrence network and the “ground-truth” sectors (see Figure S5 in Supplementary information for a detailed distribution of sectors in each company group). Furthermore, we also find that while the median edge weight between two companies not belonging to the same sector ( out-sector ) is 0.00229, the median weight between two companies belonging to the same sector ( in-sector ) is 0.0157 (see Figure S1 in Supplementary Information for the probability distributions of weights). These suggest that we may largely recover the sectors simply by looking at how often the companies are mentioned together within a rather short timespan of a year.
Nonetheless, the network reveals more interesting information when the groups and sectors differ , which potentially contains insights beyond what sector classification offers. Specifically, taking explicitly into account of the different weight distributions for in-sector and out-sector companies (that the in-sector company pairs are expected to have larger weights), we use statistical criteria to isolate the outlier company pairs of the weight distributions. These outliers are either the company pairs not belonging to the same sector yet exhibiting extraordinarily strong co-occurrence (for the case of outliers in the out-sector distribution) or company pairs belonging to the same sector but exhibiting particularly strong links, which potentially reveals more fine-grained information about the relations of companies within sectors (for the case of outliers in the in-sector distributions) and we include the details of the filtering procedure in “ Methods ” section. We show the lists of interesting company pairs we find in Tables S3 and S4 of Supplementary Information. To give some specific examples, in the former category (Table S3 ), we find that while being classified as “Consumer Discretionary”, AMZN (Amazon) has strong links with both Technology companies (e.g. GOOG (Google) and AAPL (Apple)) and retail companies (e.g. WMT (Walmart)), which are nominally classified under “Consumer Staples”; this actually is reflective of the market position and operating model of AMZN. We also find interesting links such as BKRA (Berkshire Hathaway) - PG (Proctor & Gamble) and BKRA - KO (Coca-Cola), which could be explained by the large stake BKRA holds for both PG and KO. In the latter category (Table S4 ), we find that especially strong links are often between companies that are often direct competitors and/or with considerable overlap in business interests within the same sector, e.g., VOD (Vodafone)—VZ (Verizon), and GS (Goldman Sachs)—MS (Morgan Stanley).
We also directly examine the composition of the clusters and compare and contrast with the ground-truth classification in Table S2 and Figure S5 in the Supplementary information: Groups 1 and 2 are dominated by Financials and Consumer Discretionary companies (actually Group 2 is dominated by automobile manufacturers, which are a sub-division within the broader Consumer Discretionary sector. This suggests that finer-grained information can be obtained from the co-occurrence network). On the other hand, Groups 3–5 are dominated by a few different sectors, where such differences are usually interpretable: for example, while classified by Bloomberg in the “Consumer Discretionary” category, eBay (EBAY) and Amazon (AMZN) are grouped with other companies in “Technology”. Similarly, we also observe that Group 4 is dominated by more traditional offline retail companies, even though their sectors are split between “Consumer Discretionary” and “Consumer Staples”. Finally, we see a larger amount of disagreement in the final two groups (Groups 6 and 7), where many clusters of companies too small to be standalone groups are included. The discussions here highlight that the news co-occurrence network may provide additional insight into the relations between the companies that is not reflected in the sector segmentation; more importantly, the network can be constructed dynamically, and is thus capable of capturing the evolution of the relations between companies over time.
This latter point motivates us to build a co-occurrence network for each quarter of the year and investigate the evolution of positions of companies in the network using the eigenvector and betweenness centrality measures. In Figure S6 in Supplementary information, we show results for six top companies from Technology and Financial sectors (Google, Microsoft, Apple, Goldman Sachs, JP Morgan, and Lehman Brothers). It can be seen that Google and Apple had an increasing centrality in the network while Microsoft had a decreasing one in the seven years investigated. The three financial companies had decreasing centrality and Lehman Brothers faded quickly after its bankruptcy during the crisis. This therefore provides a unique angle to investigate the dynamic standings of companies in the financial markets.
We further study the structure of the news co-occurrence network over time, and the results are summarised in Figure S7 in Supplementary information. Figure S7 (a) shows the average degree of the co-occurrence network for each quarter. The network average degree reached the peak in the last quarter of 2008, which corresponds to the escalation of the financial crisis. This might be due to more companies being reported and discussed together during events of catastrophic failure in the market 4 . The increasing connectedness of the network can also be seen from its clustering coefficient and average path length shown in Figure S7 (b). In terms of clustering structure, we compare in Figure S7 (c) the auto-detected groups based on the co-occurrence network for different quarters against the ground-truth sectors, using normalised mutual information (NMI) and F1 as measures of group similarity. We observe that the similarity is generally increasing. This might be contributed by the general increasing number of financial news articles over the period of the seven years, which helps reflect more accurately the relations between the companies.
Sentiment dynamics in related companies
The inter-dependencies within the financial market may suggest that a strong media sentiment towards one company, often caused by a significant real-world event, could have a spillover effect on related companies. To this end, we first detect days on which there is a significant upward or downward change in the sentiment score of a target company, which we denote as event days . There are a few key rationales why we focus on sentiment events instead of the entire day-by-day sentiment time series: firstly, by focusing only on the days when there are significant changes in sentiment, we implicitly filter out the noises that are inevitably present in the sentiment time series. Secondly, sentiment time series especially between related companies can be themselves correlated to some extent, as financial news outlets such as Reuters often simultaneously report a number of related companies. In this paper we are primarily interested in investigating how strong sentiment in one company spills over to related companies, hence such effect would be a confounding factor. However, we find that by focusing on the sentiment events only, we largely remove the said correlation as we find the sentiment events of different companies are largely uncorrelated (See Figure S4 in Supplementary Information for the pairwise correlation matrix of all target companies, and the readers are referred to “ Methods ” section for details).
We then examine the average sentiment of related companies before and after the event days, where related companies are defined as nearest neighbours of the target company in the news co-occurrence network (see “ Methods ” section for more details about the event days and related companies). It is worth noting that the related companies do not need to experience sentiment events themselves. Finally, for ease of presentation, we aggregate results for different target companies by the groups shown in Fig. 1 c and Table S2 in Supplementary information.
The results are presented in Fig. 2 . We conduct a non-parametric Mann-Whitney U-test 18 on the null hypothesis that the change in group aggregate sentiment is zero, and marked statistically significant points in red ( \(p < 0.01\) ) and orange ( \(0.01 \le p < 0.05\) ). We find that there exists a clear positive relationship between the sentiment of the individual company and the group sentiment for several company groups, although the strength of this relationship varies across groups. For example, in Group 1 which mainly consists of financial services companies, negative sentiment change in one company often correlates with large movement across the group, while positive sentiment does not correlate with statistically significant group movement. The same patterns are observed for companies in Groups 3, 5 and 6. On the other hand, for Groups 2 and 4 which are both related to the consumer product-related companies (both Consumer Discretionary and Consumer Staples sectors—See Figure S5 in Supplementary information), the synchronised group sentiment movements from positive and negative events of individual companies are often more symmetrically felt.
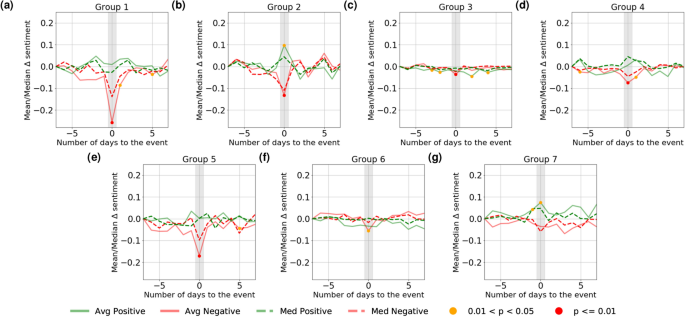
Change in mean and median group aggregate sentiment score of the major groups.
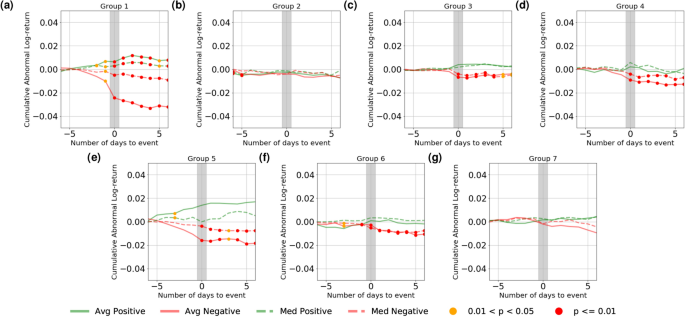
Change in mean and median CAR of the companies experiencing sentiment events, computed over all events over the time period and averaged over each company group.
Market movements associated with sentiment
Media sentiment is often associated with potential market movements. To begin with, it is important to investigate the sources of the media sentiment, for sentiment would be of limited usefulness to either explain or potentially predict market performance, if a significant amount of the sentiment is itself derived from commenting the market (especially so since Reuters does publish articles purely commenting on market performance). We argue that at least from a typical sample, most sentiment, at least on event days where there are massive changes in sentiment , is primarily derived from underlying fundamental events, instead of trivially mirroring the market performance (see “ Methods ” section for full methodology of the experiments conducted and conclusions drawn).
We then compute the cumulative abnormal return (CAR) and daily realised volatility for the individual companies around the days of sentiment events and aggregate the results based on the 7 company groups mentioned in the previous section (see “ Methods ” section for details). The results are shown in Fig. 3 for CAR and in Fig. 4 for daily volatility. Following the methodology of Ranco et al. 19 , we test statistical significance on CAR. However, differing from the z-test used in their studies which entails Gaussianity assumption, we again use the Mann-Whitney U-test 18 for statistical significance, both in conformity with the previous section and to avoid making any assumption on the underlying distributions. In Fig. 3 , we test against the null hypothesis that the CAR is zero; In Fig. 4 , we make the assumption that the volatility before the event days are drawn from a stationary distribution, and test against the hypothesis that the volatility after the events are drawn from the same distribution. Statistically significant points are marked in red ( \(p < 0.01\) ) and orange ( \(0.01 \le p < 0.05\) ).
The results demonstrate that for most groups, there exists a clear relation between media sentiment and market performance: while there are few statistically significant deviations from zero in CAR before sentiment events (with a few exceptions), the CAR patterns diverge remarkably after the sentiment events. Generally speaking, positive sentiment promotes upward price movements whereas negative one promotes price declines and as a result both types of events elicit elevation in volatility. For some company groups, this effect lingers on for a few days as the CAR continues to decline or improve gradually after the initial change on the event day. This effect again differs across different groups: it is, for example, most prominent in Finance-dominated Group 1, which seems to suggest that financial companies are more sensitive to sentiment change, both in terms of the correlated movement in group sentiment (as shown in Fig. 2 a) and the market reaction (as shown in Figs. 3 a, 4 a). Similar to Fig. 2 where negative events are shown to be associated with greater movement in group sentiment, we also observe that negative events also correlate with larger market movements. It is also interesting to observe that in a number of cases the perceived effect of sentiment on volatility is not only observed on the event day, but after event days as well, suggesting the lingering effect of extreme sentiment movements and potential predictive uses of large sentiment movements for market participants.
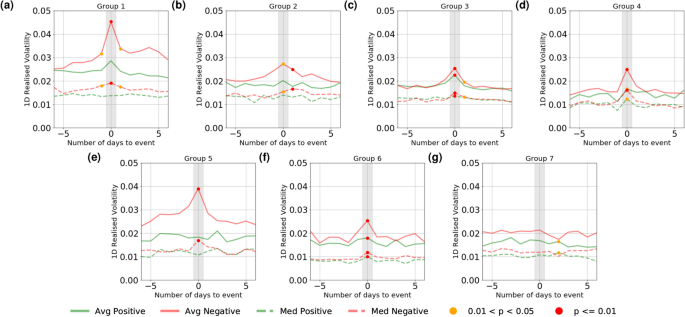
Change in mean and median daily volatility of the companies experiencing sentiment events, computed over all events over the time period and averaged over each company group.
The correlation of media sentiment across companies suggests that market movements may also be studied at a collective level for groups of companies. At the collective level, the complex interactions between companies preclude the simple analysis of average statistics as in the individual case. Instead, we computed the implied probability density function (PDF) of the histograms of abnormal return (AR) and volatility in each group on the days before, on, and after the days when there are extraordinary changes in group aggregate sentiment events (see “ Methods ” section for detail). We discuss in this section results for one company group, i.e., Group 2, and results for several other groups are presented in Figures S8–S11 in Supplementary information.
From Fig. 5 , we note that while the effect at the group level is unsurprisingly weaker, there are still noticeable deviations in the profiles of distributions on event days. This suggests that links exist not only between an individual company’s sentiment and price movement, but also at a collective level. Specifically, for the Industrial-dominated Group 2, we observe that when the group-aggregated sentiment experiences extraordinary changes in one direction, there is a noticeable shift in the probability mass of the distribution of AR towards the opposite direction. For volatility, there is a noticeable elevation in probability mass on both types of sentiment events, suggesting that on these days the market movements of the group as a whole are likely to be more volatile. Such observations, especially the elevation in volatility, are also observed in a number of other groups, as shown in Figures S8–S11. Interestingly, on Fig. 3 , Group 2 seems to exhibit the least correlation between sentiment and market movement at the individual company level; this suggests that analysis without considering network effects might prove inadequate in our context.
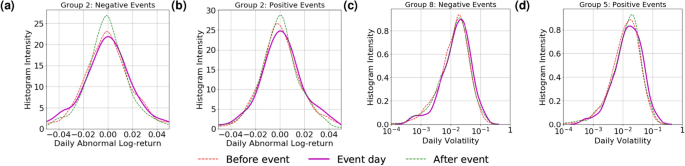
Probability density function of AR and daily realised volatility of Group 2 companies, computed over all group-aggregated sentiment events over the time period: ( a ) AR around negative events , ( b ) AR around positive events , ( c ) Volatility around negative events , ( d ) Volatility around positive events .
In this work, we have taken a first step in integrating NLP-based financial news sentiment analysis and network analysis of financial markets. In particular, we propose a novel pipeline that dynamically analyses the evolution of stocks of major companies using a network constructed on the basis of financial news and sentiments. We extend this framework to analyse the inter-connectedness of sentiment by considering the correlation of sentiment of individual companies to groups of related companies. We also demonstrate the practical utility of this network representation, as we find a non-negligible market movement associated with sentiment beyond the individual company level.
We believe that the present study points to an exciting direction of future research to improve the understanding of financial markets, the ultimate goal of this work. Up to this point, analysis of media sentiment and market movements in the existing literature has been largely focused on individual companies or financial assets. However, our work provides evidence suggesting that taking into account the dynamic interplay between these companies or assets and a shift from single company to at least sector/group level would be beneficial. We believe that this better understanding and quantification of sentiment correlation (which has been largely neglected) will be crucial, and could lead to better financial decision-making at various levels, from market participants to market regulators. Specifically, for market participants, a deeper understanding of news sentiment and market movement beyond the level of individual companies may help them make more informed investment decisions. For market regulators, insights about the collective dynamics of news co-occurrence, media sentiment, and associated market movement may provide them with a more holistic picture of the financial market, and allows for the monitoring of systemic risk in the market as well as designs of effective intervention mechanisms. Finally, apart from the empirical findings based on retrospective analysis, the pipeline proposed in the paper is highly valuable in its own right in that it provides a real-time network analysis tool on sentiment and market movements.
Nonetheless, it is important to also bear in mind the limitations of our present study. First, we only analyse news articles from one particular outlet (i.e. Reuters), which might subject us to hidden bias and scarcity of data. To partially ameliorate the second effect, we limit our focus to the largest companies that receive an adequate amount of coverage from Reuters news. Future work might consider extending the scope by including a broader range of companies and markets. Second, we are also limited by the present state-of-the-art of NLP analysis on effective entity recognition and sentiment prediction, which remain open research questions in the NLP community. In this aspect, the most obvious next step would be to employ more advanced techniques once they become available, and to use a more domain-specific corpus related to finance jargon, for example, for model training. Third, in the present work, we focus on the correlation of sentiments and associated market movements based on the historical data. In particular, no analysis of causality has been attempted; nevertheless, we note that, in many cases, the associated market movements took place after the sentiment movements (for example, see Fig. 3 —while the largest movement in log-return is often seen on event days, the same trend can often be extended to a longer period of time after the event day). Therefore, an immediate extension work would be to investigate any causal link between sentiment and market movement, a study we believe could be potentially extremely useful for financial decision-making. Fourth, the current analysis of sentiment effect ignores some of the possible interfering effects. For example, we ignore overlaps of multiple sentiment events from different companies which might be highly related. Future work could seek to rectify this by devising methods to control for such possible interfering effects. Finally, we acknowledge that, for the ease of analysis and data acquisition, we have only looked at historical data, and we hope to extend the study to more recent period of time, especially in view of the recent market turbulence and the possible changing market landscape in recent years due to increased automation 20 .
We focus on the investigation of network relations between companies through NLP-based analysis of financial news text. We first collect the raw text of financial news and then use a named entity recognition system to automatically identify the organisations in the financial news. The recognized organizations were cleaned and 87 target companies were kept in a post-processing step (as detailed below). A state-of-the-art sentiment prediction model is used to predict the sentiment score of those identified companies when they appear in the news. This allows us to build time series of company sentiment and study its dynamics and market movement using the news co-occurrence network of the companies.
We use the financial news on Reuters collected from October 2006 to November 2013, which are publicly available 21 . Table 1 shows some statistics of the data set. We select the data from January 1st, 2007 to September 30th, 2013 to include 27 full quarters. For market data, we collect daily closing price and volatility data of 87 target companies (methods to identify these companies are outlined in the next section) using the Bloomberg Terminal 22 .
Named entity recognition
We use a state-of-the-art deep learning based named entity recognition (NER) system NCRF++ 23 to automatically extract entities from financial news text. We use a character-level convolutional neural network (CNN) 24 with a word-level long short-term memory (LSTM) 25 network to extract the text features and use the conditional random field (CRF) 26 as the decoding layer. The NER system is trained on the CoNLL 2003 dataset 27 . Only the identified entities with type “organisation” are kept for the following steps.
As one organisation may have various name formats (e.g., “Apple Inc.”,“AAPL”,“Apple” all refer to the same organisation), we normalise the identified organisation names using the following rules: 1) we manually set a number of criteria to aggregate entities with short and full names. For example, “XXX LLC” \(\rightarrow\) “XXX” and “XXX Group” \(\rightarrow\) “XXX”; 2) we automatically disambiguate entities through brackets. In more detail, we utilise the bracket information within the news text and extract the mapping pairs. For example, “expanded partnership with International Business Machines Corp (IBM)” implies that “IBM” is the abbreviation of text “International Business Machines Corp”.
We keep the organisations that are consistently mentioned more than 4 times in the news in each of the 27 quarters, which resulted in 145 frequent organisations. Among those frequent organisations, we select 87 companies with a relevant price ticker in the Bloomberg terminal. We categorise the 87 companies into 9 sectors based on the Bloomberg sector list in 2018. Detailed company list and sector information are shown in Table S1 .
In this paper, we conduct a series of investigations on the News Co-occurrence Network. Firstly, we perform Community Detection on the network to obtain clusters of related companies: Given the news co-occurrence network constructed from the data of the first year, we apply the Louvain modularity method 16 to detect the communities of the related companies which we term the “groups” in this paper. We present a high-level overview of the Louvain modularity method as follows. The Louvain method uses modularity as the objective function Q it aims to maximise:
where the summation is over all edges in the network, \(e_{i, j}\) is the weight of the edge connecting nodes i and j (in our case, the cosine similarity measure between companies in terms of news appearance), \(k_i\) and \(k_j\) are the sum of all weights of the edges attached to nodes i and j , respectively, \(c_i\) and \(c_j\) are the communities i and j belong to (in our case, the company groups), respectively, and \(\delta\) is the Kronecker delta function with \(\delta (x, y) = 1\) if \(x = y\) and \(\delta (x,y)=0\) otherwise. The Louvain method initialises by assigning each node its own community and then computes the change in modularity, \(\Delta Q\) , by removing node i from its own community and moving it into each community i is connected to. Once computed for all communities, the node i is assigned to the community that leads to largest \(\Delta Q\) , if any increase \(\Delta Q\) is possible (otherwise the community of node i remains unchanged). This process is repeated sequentially for all nodes until no further \(\Delta Q > 0\) is possible.
After concluding the aforementioned procedures the algorithm starts the second phase, where nodes of the same community are now represented as nodes in a new network and the first phase can be re-applied again. This two-phased pass continues until there is no change in the computed communities and the algorithm terminates. We use the Python implementation of the Louvain modularity method ( https://github.com/taynaud/python-louvain ), which further complements the aforementioned Louvain modularity method with the multiscale feature 28 —in this work, we set the timescale parameter associated with this feature to the default value of 1.0.
Given the clusters of related companies obtained from the aforementioned procedures, we also compare and contrast these clusters with the ground-truth sectors provided by Bloomberg, and we are particularly interested in isolating the interesting pairs of companies where the clusters reveal relations different from the sector information, or otherwise provides more informative insights. Given that we find company pairs belonging to the same sector are also more likely to have stronger weights amongst them (and hence more likely to appear in the same cluster. See Figure S1 ), we design filtering criteria that explicitly take account of the different weight distributions of the companies that belong to the same sectors ( in-sector ) and those do not ( out-sector ). We isolate the outlier companies pairs defined as those with edge weights above 75th percentile + 1.5 Interquartile Range (IQR) in both categories.
Sentiment prediction
The identification of sentiment polarity for a given entity in a context is a classical “targeted sentiment analysis” NLP task 29 . Given the identified entities, we use a state-of-the-art attentive neural network model to predict the sentiment of given entities 30 . The model was trained using the text corpus made publicly available from a previous study 31 . For each sentence with given entities, our sentiment model assigns each entity a sentiment value ranging from − 1 to 1, where − 1 represents the most negative polarity and 1 the most positive one. Our sentiment model utilises the full context information of the sentence in assigning sentiment value to the target entity.
One entity may have been mentioned multiple times in the news during the period of analysis (e.g., one day or one quarter). In this case, we use the average sentiment among all mentions and we define a news article to be sentiment-bearing if it contains an overall non-neutral sentiment towards any target companies. This yields a sentiment time series of any desired temporal frequency for each of the target companies. When we considered the sentiment score at a group level, we simply aggregate the sentiment scores of all constituent entities of that group by averaging those scores.
Sources of sentiment
As mentioned, to support the rigour of the conclusions drawn we investigate the sources of the sentiment; however, since it is infeasible to manually classify the source for each sentence, we focus on a small yet sufficiently typical sample of sentences: we first examine the distribution of the non-neutral sentiment contained in articles, as only a fraction of all the sentences in each articles carry non-zero sentiment, and it is possible to trace total amount of sentiment contained in an article back to individual contribution of these sentences. We find that the distribution of the sentiment across articles is highly skewed, with top 9.2% articles accounting for 50% of the sentiment directed to any of our 87 target companies (see Figure S3 in Supplementary Information for details). With this showing the top few articles disproportionately account for a large amount of sentiment overall, we manually inspect all sentences with non-zero sentiment scores in the top-20 articles with most sentiments, and we find that only 1 article has a considerable amount of sentiment that is derived from market commentary. Upon visual inspection, this trend also at least extends to the top-50 articles with the most sentiment. Furthermore, on a daily basis, we also manually look into the top-5 days with the largest magnitudes of sentiment scores (often accompanied with large price movements as well) for 3 representative companies (AAPL (Apple), GS (Goldman Sachs) and GM (General Motors)). By inspection, it is evident that most of the sentiment comes from comments on the fundamentals rather than simply commenting on the market. As a singular example, on 20 Apr 2010 Goldman Sachs (GS) experienced negative shock in sentiment. We manually screened the first 50 sentences with non-zero sentiment scores on that day, and only 4 are some sort of commentary. Even for the 4 market comments, none is directly commenting on the market performance of GS on that day . The vast majority of the sentiment on that day directed to GS derives from the reporting a negative real-world event about GS. We find that the similar patterns hold for other days and for other companies.
Sentiment event days and group aggregate sentiment
To distill the most significant information from the sentiment time series, we extract the days where the entity or the group of entities experienced extraordinary changes in the computed sentiment scores. We denote these as event days , which are defined as days on which the sentiment score of the entity or the group of entities exceeded 2 standard deviations above or below the average sentiment in the preceding 180 trading days. Based on the direction of this movement, we term the event days as positive or negative event days, where appropriate.
As discussed, we are primarily interested in investigating how strong sentiment in one company correlates with behaviour of related companies and it is thus important to first examine the level of correlation between the sentiment of different companies itself, and this is another reason why we focus on the sentiment events. By modelling the daily sentiment as a multivariate time series for all the companies considered, a factor analysis model with 5 latent factors (similar to the model used in Vassallo et al, 2019 32 ) is capable of explaining 55% of the total variance; on the sentiment event series (i.e. the time series with only 3 possible values: − 1 (negative event), 0 (no event) and 1 (positive event)), the same model explains 2.3%. This suggests that the strong, transient sentiments are often driven by company-specific events, instead of market- or sector-coordinated movements.
To investigate the sentiment dynamics amongst companies, we construct a news co-occurrence network as described in the main text (i.e., representing individual companies as nodes of the graph with edges being the pairwise cosine distances of vectors corresponding to the companies in the news coverage matrix). To showcase the potential predictive value of our method, we construct the networks dynamically : on each event day described above, we only consider the news in the window of 60 trading days preceding the day itself \([T - 60, T)\) . With this dynamic network which evolves as a function of time, for a company c experiencing an event day on time \(t = T\) , we compute the aggregate sentiment of the companies that are top- k nearest neighbours on the network measured by the edge weight; in this work, unless otherwise specified we take \(k = 10\) , and it is worth noting that since the networks are dynamically constructed, the nearest neighbours are in general different at a different time t . Formally, the sentiment score \(\bar{s_c}\) for the nearest neighbours of company c at time \(t = T\) is given by:
where \(s(c_i)|_{t=T}\) is the sentiment score of company \(c_i\) at time \(t=T\) , and \(e_{c,c'}\) denotes the pairwise edge weight between companies c and \(c'\) where \(c'\) in this case is a member of the neighbours N ( c ) of company c in the network. To compare the group sentiment evolution around sentiment days, \(\bar{s_c}\) is then computed for each day in the range of \([T-7, T+7]\) around the event days to produce \(\{\bar{s_c}|_{t=T-7}, \ldots , \bar{s_c}|_{t=T}\) (event day) \(, \ldots \bar{s_c}|_{t=T+7}\}\) . Notice that there is one such series defined on each of the event days for each of the companies that experienced at least one event day. We first average over all event days to obtain one series per company. For the sake of better presentation, we then further aggregate by averaging over the groups of the companies (see Table S2 ) to condense 87 series to 7, as we expect the companies in the same group to behave more similarly. Formally, following the notation in Eq. ( 2 ), the final group aggregate sentiment score of a group of companies G , s ( G ), which is the quantity represented in Fig. 2 and discussed in the preceding section, can be mathematically represented by the two level aggregation:
where E ( c ) is the set of event days of company c over the period of time considered. It is worth emphasising that this quantity reflects the group sentiment as a whole and is independent of timestamp T and is only dependent on \(\tau\) , which is the the number of days relative to the sentiment day.
While it is infeasible to conduct the above screening procedure for all articles considering the vast number of sentences contained, we argue that at least from a typical sample, most sentiment, at least on event days where there are massive changes in sentiment , is primarily derived from underlying fundamental events, instead of trivially mirroring the market performance. We argue this is also reasonable: qualitatively, while Reuters does publish some articles purely for market commentary, we do expect the majority of the daily articles especially on days with important breaking news to be dedicated to reporting the underlying events, which should be the primary drivers of both the market and the sentiment. For the latter category of articles, the market performance is usually only commented on sparingly, and it is thus expected that the sentiment is primarily driven by the underlying events.
Market data processing
To accurately quantify the market movements associated with sentiment, we process the market data to exclude other possible confounding factors. Specifically, we compute the cumulative abnormal return (CAR) in the 7 trading days leading up to and immediately after the sentiment event days, defined in the previous section. Consider an event day on day T , on an arbitrary day around that day t , the CAR is given by:
where \(\epsilon _t\) denotes he abnormal return (AR) on day t , and the summation is over \(t-T_s+1\) trading days, where \(T_s\) is set to a fixed value of 7 trading days before the event day.
The AR is the excess return over the expected return from the Capital Asset Pricing Model (CAPM). Here we follow Ranco et al. 19 to select the CAPM model 33 over the more popular Fama-French model 34 , 35 : this is because we both favour the simplicity of CAPM model and expect that the additional factors included in Fama-French model (i.e. the factors explaining the outperformance of small-cap over large-cap companies, and that of value stocks over growth stocks) would be largely consistent over our selection of companies, which are predominantly large-cap and usually fall to the “growth” bracket. The market model decomposes the return of a single stock at time t , \(R_t = \log \Big (\frac{P(t)}{P(t-1)}\Big )\) ( P ( t ) is simply the closing price at day t ), with three components in a linear manner: \(\beta\) , which captures the return that can be explained by the movement of the entire market (usually represented by the index log-return \(R_m\) ); \(\alpha\) , which is the idiosyncratic return over the index; and \(\epsilon\) , a stochastic term to explain any residual influence that is the AR in our context:
Here, we compute parameters \(\alpha\) and \(\beta\) for each stock on each trading day based on its performance as compared to the index return in the previous 180 trading days. We select the MSCI World Index since the list of our selected companies include large-cap companies over a global range of developed markets. The readers are referred to Table S1 for a detailed list of companies and their corresponding sector listing. The null hypothesis is based on the market model that any return of a single stock can be explained by \(\alpha\) and \(\beta\) , and \(\epsilon\) is assumed to be a zero-mean stochastic quantity. Any \(\epsilon\) that significantly differs from 0 suggests there exist factors affecting the stock return that are not explained by the market model; one possible such factor is, in our case, movement in the sentiment of the company to which the stock belongs, which influences this particular stock only but not the index (at least to a lesser extent).
To model daily volatility, we use the absolute value of the daily log-return as a proxy \(\sigma (t) \approx |\log \frac{P(t)}{P(t-1)}|\) . While this deviates from the exact definition of volatility as the standard deviation of the log-return, this approximation has been shown empirically to correlate strongly with the actual volatility 36 and has been commonly used in the literature 37 , 38 . This metric therefore measures the magnitude of market reaction, regardless of the direction of the response.
As a final modelling step, given the market movement series \(\{CAR|_{t=T-7}, \ldots , CAR|_{t=T+7}\}\) and \(\{\sigma |_{t=T-7}, ..., \sigma |_{t=T+7}\}\) for each company around each event day similar to the group aggregate sentiment score described in the preceding section, we apply the similar final aggregation steps to obtain one series per company group. While descriptive statistics like median and mean are usually sufficient to model the market movements on an individual company level, in a group level, we also consider the distributions of AR and volatility approximated by histograms and kernel density estimation (KDE). Specifically, within each group defined in Table S2 , we first compute a group level sentiment time series by aggregating the sentiment time series of all the members of the group. Next, on market data, without aggregation, we categorize the elements of the CAR and volatility series of the related companies into before event day \(t \in [T-7, T)\) , on event day \(t = T\) , after event day \(t \in (T, T+7]\) . Within each category, over the entire period of time considered, we obtain the histograms of AR and volatility - by the market model described in the preceding section, \(AR \sim \mathscr {N}(0, \sigma ^2)\) . With the histograms, we then apply KDE to obtain a continuous approximation \(\hat{p}(x)\) to the probability density function (PDF) of AR p ( x ):
where n is the number of data points we use to estimate the distribution, \(\{x_i\}\) are the observed market data (AR or volatility) and h is the bandwidth, which we estimate automatically from the Seaborn visualisation package 39 . Finally, it is worth noting that to ensure a rather accurate estimation of the distribution functions, we only apply KDE when we have more than 20 sentiment events in a particular group.
Data availability
Data, materials and code that are necessary to reproduce the results described in this paper, including 1) the news coverage matrix, 2) the sentiment scores, and 3) the news co-occurrence network, are available from the corresponding author(s) upon reasonable request. Market data of equivalent quality used in this study are publicly available from platforms such as Yahoo! Finance.
Longstaff, F. A. The subprime credit crisis and contagion in financial markets. J. Financial Econ. 97 , 436–450 (2010).
Article Google Scholar
Battiston, S. et al. Complexity theory and financial regulation. Science 351 , 818–819 (2016).
Article ADS CAS Google Scholar
Mantegna, R. N. Hierarchical structure in financial markets. Eur. Phys. J. B Condens. Matter Complex Syst. 11 , 193–197 (1999).
Article CAS Google Scholar
Almog, A. & Shmueli, E. Structural entropy: monitoring correlation-based networks over time with application to financial markets. Sci. Rep. 9 , 1–13 (2019).
Onnela, J.-P., Chakraborti, A., Kaski, K., Kertesz, J. & Kanto, A. Asset trees and asset graphs in financial markets. Phys. Scr. 2003 , 48 (2003).
Heimo, T., Kaski, K. & Saramäki, J. Maximal spanning trees, asset graphs and random matrix denoising in the analysis of dynamics of financial networks. Phys. A Stat. Mech. Appl. 388 , 145–156 (2009).
Starnini, M., Boguñá, M. & Serrano, M. The interconnected wealth of nations: Shock propagation on global trade-investment multiplex networks. Sci. Rep. 9 (1), 1–10 (2019).
Barja, A. et al. Assessing the risk of default propagation in interconnected sectoral financial networks. EPJ Data Sci. 8 , 32 (2019).
Mitchell, M., Aguilar, J., Wilson, T. & Van Durme, B. Open domain targeted sentiment. In Proceedings of the 2013 conference on empirical methods in natural language processing (EMNLP), pp. 1643–1654 (2013).
Li, J. & Hovy, E. Sentiment analysis on the people’s daily. In Proceedings of the 2014 conference on empirical methods in natural language processing (EMNLP), pp. 467–476 (2014).
Forss, T. & Sarlin, P. News-sentiment networks as a company risk indicator. J. Netw. Theory Finance 4 , 65–86 (2018).
Mizuno, T., Ohnishi, T. & Watanabe, T. Novel and topical business news and their impact on stock market activity. EPJ Data Sci. 6 , 26 (2017).
Si, J. et al. Exploiting topic based twitter sentiment for stock prediction. In Proceedings of the 51st annual meeting of the association for computational linguistics (volume 2: short papers) , pp. 24–29 (2013).
Mittal, A. & Goel, A. Stock prediction using twitter sentiment analysis. Standford University, CS229 . http://cs229.stanford.edu/proj2011/GoelMittal-StockMarketPredictionUsingTwitterSentimentAnalysis.pdf (2012).
Carmichael, K. Canada dodged the worst of the financial crisis, and it wasn’t just dumb luck. https://business.financialpost.com/news/economy/canada-dodged-the-worst-of-the-financial-crisis-and-it-wasnt-just-dumb-luck (2018).
Blondel, V. D., Guillaume, J.-L., Lambiotte, R. & Lefebvre, E. Fast unfolding of communities in large networks. J. Stat. Mech. Theory Exp. 2008 , P10008 (2008).
Bastian, M., Heymann, S. & Jacomy, M. Gephi: An open source software for exploring and manipulating networks. In Proceedings of the international AAAI conference on web and social media, pp. 361–362 (2009).
Mann, H. B. & Whitney, D. R. On a test of whether one of two random variables is stochastically larger than the other. Ann. Math. Stat. 18 , 50–60 (1947).
Article MathSciNet Google Scholar
Ranco, G., Aleksovski, D., Caldarelli, G., Grčar, M. & Mozetič, I. The effects of twitter sentiment on stock price returns. PLoS ONE 10 , e0138441 (2015).
Amaro, S. sell-offs could be down to machines that control 80% of the US stock market, fund manager says (2018).
Ding, X., Zhang, Y., Liu, T. & Duan, J. Using structured events to predict stock price movement: An empirical investigation. In Proceedings of the 2014 conference on empirical methods in natural language processing (EMNLP) , pp. 1415–1425 (2014).
Bloomberg, L. P. Market information from selected companies from 1 Jan 2007 to 31 Dec 2013.
Yang, J. & Zhang, Y. NCRF++: An open-source neural sequence labeling toolkit. In Proceedings of the 56th annual meeting of the association for computational linguistics (2018).
LeCun, Y. et al. Backpropagation applied to handwritten zip code recognition. Neural Comput. 1 , 541–551 (1989).
Hochreiter, S. & Schmidhuber, J. Long short-term memory. Neural Comput. 9 , 1735–1780 (1997).
Lafferty, J., McCallum, A. & Pereira, F. C. Conditional random fields: Probabilistic models for segmenting and labeling sequence data. In Proceedings of the eighteenth international conference on machine learning (ICML) , pp. 282–289 (2001).
Tjong Kim Sang, E. F. & De Meulder, F. Introduction to the conll-2003 shared task: Language-independent named entity recognition. In Proceedings of the seventh conference on natural language learning at HLT-NAACL , pp. 142–147 (2003).
Lambiotte, R., Delvenne, J.-C. & Barahona, M. Random walks, Markov processes and the multiscale modular organization of complex networks. IEEE Trans. Netw. Sci. Eng. 2 (1), 76–90 (2014).
Jiang, L., Yu, M., Zhou, M., Liu, X. & Zhao, T. Target-dependent twitter sentiment classification. In Proceedings of the 49th annual meeting of the association for computational linguistics: Human language technologies , pp. 151–160 (2011).
Liu, J. & Zhang, Y. Attention modeling for targeted sentiment. In Proceedings of the 15th conference of the European chapter of the association for computational linguistics: volume 2, short papers , pp. 572–577 (2017).
Zhang, M., Zhang, Y. & Vo, D.-T. Gated neural networks for targeted sentiment analysis. In Proceedings of the AAAI conference on artificial intelligence , pp. 3087–3093 (2016).
Vassallo, D., Bormetti, G. & Lillo, F. A tale of two sentiment scales: Disentangling short-run and long-run components in multivariate sentiment dynamics. arXiv:1910.01407 (2019).
Fama, E. F. & French, K. R. The capital asset pricing model: Theory and evidence. J. Econ. Perspect. 18 , 25–46 (2004).
Fama, E. F. & French, K. R. The cross-section of expected stock returns. J. Finance 47 , 427–465 (1992).
Fama, E. F. & French, K. R. A five-factor asset pricing model. J. Financial Econ. 116 , 1–22 (2015).
Longin, F. & Solnik, B. Extreme correlation of international equity markets. J. Finance 56 , 649–676 (2001).
Saha, A., Malkiel, B. G. & Grecu, A. The clustering of extreme movements: Stock prices and the weather. J. Invest. Manag. 1 , 20 (2009).
Google Scholar
Masoliver, J. & Perelló, J. Multiple time scales and the exponential Ornstein–Uhlenbeck stochastic volatility model. Quant. Finance 6 , 423–433 (2006).
Waskom, M. et al. mwaskom/seaborn: v0.10.1 (april 2020), https://doi.org/10.5281/zenodo.3767070 (2020).
Download references
Acknowledgements
X.W., J.Y., J.-P.C., S.Z. and X.D. thank the Oxford-Man Institute of Quantitative Finance for supporting this research. S.M. thanks Man AHL for supporting this research.
Author information
These authors contributed equally: Xingchen Wan and Jie Yang.
Authors and Affiliations
Oxford-Man Institute of Quantitative Finance, University of Oxford, Oxford, UK
Xingchen Wan, Jan-Peter Calliess, Stefan Zohren & Xiaowen Dong
School of Public Health, Zhejiang University, Hangzhou, China
Harvard Medical School, Harvard University, Cambridge, MA, USA
Man AHL, London, UK
Slavi Marinov
You can also search for this author in PubMed Google Scholar
Contributions
X.W., J.Y., J.-P.C., S.Z. and X.D. conceived and designed the study. X.W. and J.Y. performed the analysis. S.M., J.-P.C., S.Z. and X.D. helped analyse and interpret the results. All authors wrote, reviewed and approved the manuscript.
Corresponding authors
Correspondence to Xingchen Wan or Jie Yang .
Ethics declarations
Competing interests.
The authors declare no competing interests.
Additional information
Publisher's note.
Springer Nature remains neutral with regard to jurisdictional claims in published maps and institutional affiliations.
Supplementary Information
Supplementary information., rights and permissions.
Open Access This article is licensed under a Creative Commons Attribution 4.0 International License, which permits use, sharing, adaptation, distribution and reproduction in any medium or format, as long as you give appropriate credit to the original author(s) and the source, provide a link to the Creative Commons licence, and indicate if changes were made. The images or other third party material in this article are included in the article's Creative Commons licence, unless indicated otherwise in a credit line to the material. If material is not included in the article's Creative Commons licence and your intended use is not permitted by statutory regulation or exceeds the permitted use, you will need to obtain permission directly from the copyright holder. To view a copy of this licence, visit http://creativecommons.org/licenses/by/4.0/ .
Reprints and permissions
About this article
Cite this article.
Wan, X., Yang, J., Marinov, S. et al. Sentiment correlation in financial news networks and associated market movements. Sci Rep 11 , 3062 (2021). https://doi.org/10.1038/s41598-021-82338-6
Download citation
Received : 03 July 2020
Accepted : 15 January 2021
Published : 04 February 2021
DOI : https://doi.org/10.1038/s41598-021-82338-6
Share this article
Anyone you share the following link with will be able to read this content:
Sorry, a shareable link is not currently available for this article.
Provided by the Springer Nature SharedIt content-sharing initiative
This article is cited by
Dataset on sentiment-based cryptocurrency-related news and tweets in english and malay language.
- Nur Azmina Mohamad Zamani
- Norhaslinda Kamaruddin
- Ahmad Muhyiddin B. Yusof
Language Resources and Evaluation (2024)
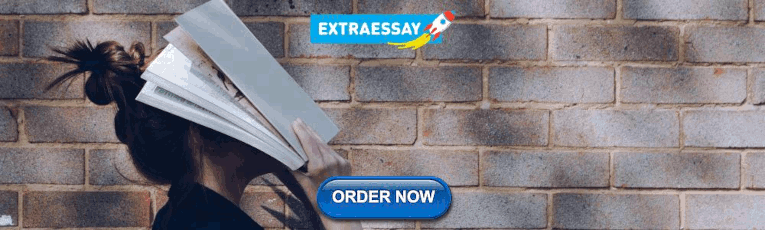
New drugs and stock market: a machine learning framework for predicting pharma market reaction to clinical trial announcements
- Semen Budennyy
- Alexey Kazakov
- Leonid Zhukov
Scientific Reports (2023)
Sentence Level Sentimental Analysis with Neural Network Using RSS News Feed on Stock Market Informations
- Seethiraju L. V. V. D. Sarma
- D. VenkataSekhar
- Gudipati Murali
SN Computer Science (2023)
Impact of public news sentiment on stock market index return and volatility
- Gianluca Anese
- Marco Corazza
- Loriana Pelizzon
Computational Management Science (2023)
Exploring spatiotemporal changes in the multi-granularity emotions of people in the city: a case study of Nanchang, China
- Chaoyang Fang
Computational Urban Science (2022)
By submitting a comment you agree to abide by our Terms and Community Guidelines . If you find something abusive or that does not comply with our terms or guidelines please flag it as inappropriate.
Quick links
- Explore articles by subject
- Guide to authors
- Editorial policies
Sign up for the Nature Briefing: AI and Robotics newsletter — what matters in AI and robotics research, free to your inbox weekly.

- Browse All Articles
- Newsletter Sign-Up
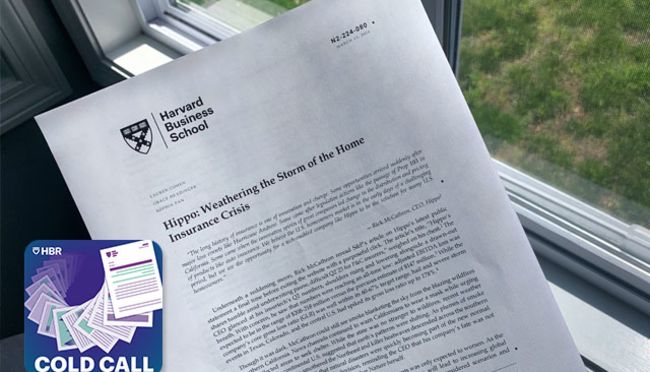
- 04 Jun 2024
- Cold Call Podcast
How One Insurtech Firm Formulated a Strategy for Climate Change
The Insurtech firm Hippo was facing two big challenges related to climate change: major loss ratios and rate hikes. The company used technologically empowered services to create its competitive edge, along with providing smart home packages, targeting risk-friendly customers, and using data-driven pricing. But now CEO and president Rick McCathron needed to determine how the firm’s underwriting model could account for the effects of high-intensity weather events. Harvard Business School professor Lauren Cohen discusses how Hippo could adjust its strategy to survive a new era of unprecedented weather catastrophes in his case, “Hippo: Weathering the Storm of the Home Insurance Crisis.”
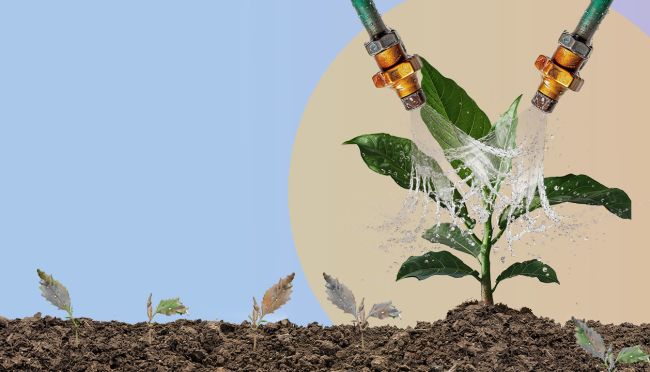
- 22 Apr 2024
- Research & Ideas
When Does Impact Investing Make the Biggest Impact?
More investors want to back businesses that contribute to social change, but are impact funds the only approach? Research by Shawn Cole, Leslie Jeng, Josh Lerner, Natalia Rigol, and Benjamin Roth challenges long-held assumptions about impact investing and reveals where such funds make the biggest difference.
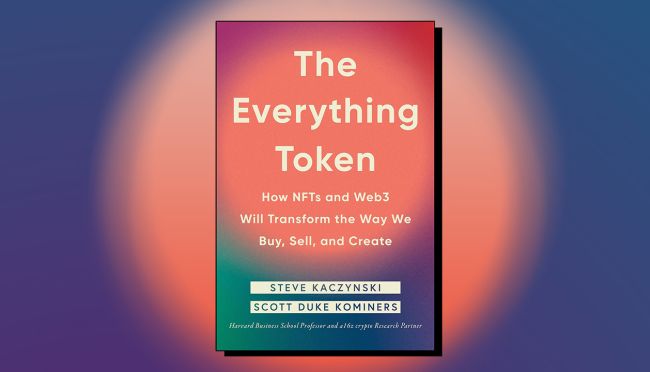
- 23 Jan 2024
More Than Memes: NFTs Could Be the Next Gen Deed for a Digital World
Non-fungible tokens might seem like a fad approach to selling memes, but the concept could help companies open new markets and build communities. Scott Duke Kominers and Steve Kaczynski go beyond the NFT hype in their book, The Everything Token.
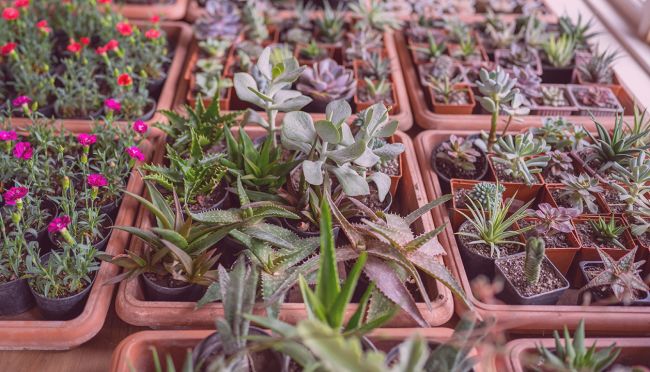
- 12 Sep 2023
How Can Financial Advisors Thrive in Shifting Markets? Diversify, Diversify, Diversify
Financial planners must find new ways to market to tech-savvy millennials and gen Z investors or risk irrelevancy. Research by Marco Di Maggio probes the generational challenges that advisory firms face as baby boomers retire. What will it take to compete in a fintech and crypto world?
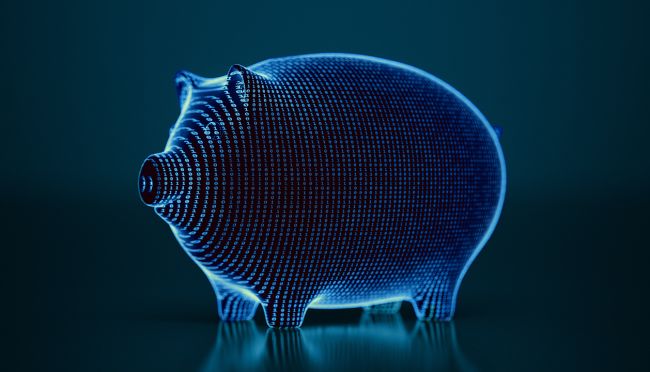
- 17 Aug 2023
‘Not a Bunch of Weirdos’: Why Mainstream Investors Buy Crypto
Bitcoin might seem like the preferred tender of conspiracy theorists and criminals, but everyday investors are increasingly embracing crypto. A study of 59 million consumers by Marco Di Maggio and colleagues paints a shockingly ordinary picture of today's cryptocurrency buyer. What do they stand to gain?
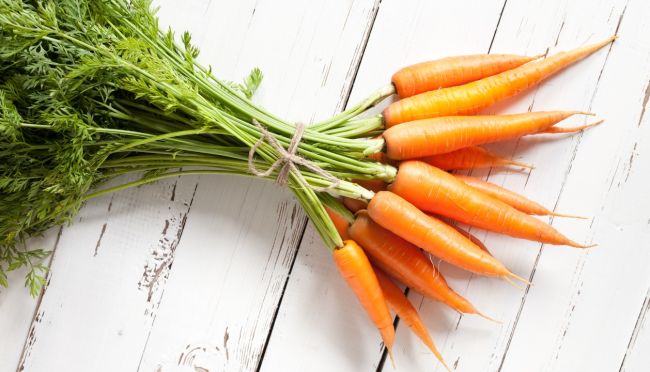
- 17 Jul 2023
Money Isn’t Everything: The Dos and Don’ts of Motivating Employees
Dangling bonuses to checked-out employees might only be a Band-Aid solution. Brian Hall shares four research-based incentive strategies—and three perils to avoid—for leaders trying to engage the post-pandemic workforce.
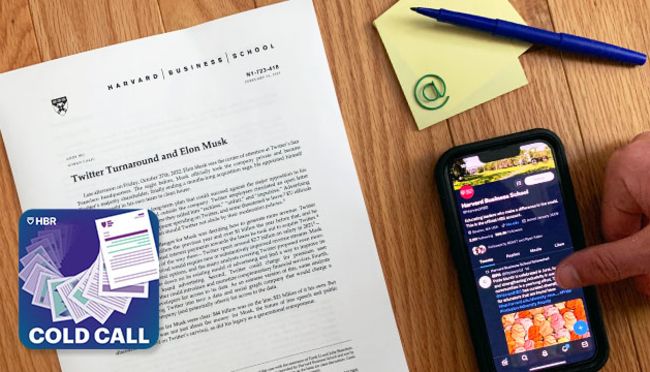
- 20 Jun 2023
Elon Musk’s Twitter Takeover: Lessons in Strategic Change
In late October 2022, Elon Musk officially took Twitter private and became the company’s majority shareholder, finally ending a months-long acquisition saga. He appointed himself CEO and brought in his own team to clean house. Musk needed to take decisive steps to succeed against the major opposition to his leadership from both inside and outside the company. Twitter employees circulated an open letter protesting expected layoffs, advertising agencies advised their clients to pause spending on Twitter, and EU officials considered a broader Twitter ban. What short-term actions should Musk take to stabilize the situation, and how should he approach long-term strategy to turn around Twitter? Harvard Business School assistant professor Andy Wu and co-author Goran Calic, associate professor at McMaster University’s DeGroote School of Business, discuss Twitter as a microcosm for the future of media and information in their case, “Twitter Turnaround and Elon Musk.”
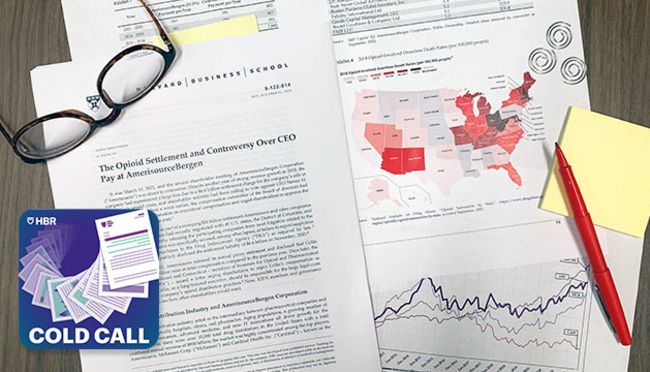
- 06 Jun 2023
The Opioid Crisis, CEO Pay, and Shareholder Activism
In 2020, AmerisourceBergen Corporation, a Fortune 50 company in the drug distribution industry, agreed to settle thousands of lawsuits filed nationwide against the company for its opioid distribution practices, which critics alleged had contributed to the opioid crisis in the US. The $6.6 billion global settlement caused a net loss larger than the cumulative net income earned during the tenure of the company’s CEO, which began in 2011. In addition, AmerisourceBergen’s legal and financial troubles were accompanied by shareholder demands aimed at driving corporate governance changes in companies in the opioid supply chain. Determined to hold the company’s leadership accountable, the shareholders launched a campaign in early 2021 to reject the pay packages of executives. Should the board reduce the executives’ pay, as of means of improving accountability? Or does punishing the AmerisourceBergen executives for paying the settlement ignore the larger issue of a business’s responsibility to society? Harvard Business School professor Suraj Srinivasan discusses executive compensation and shareholder activism in the context of the US opioid crisis in his case, “The Opioid Settlement and Controversy Over CEO Pay at AmerisourceBergen.”
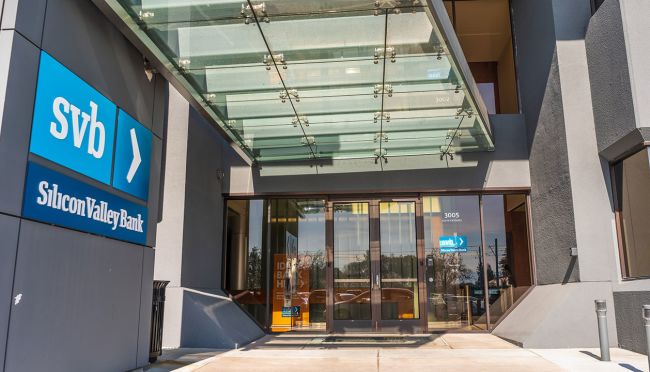
- 16 May 2023
- In Practice
After Silicon Valley Bank's Flameout, What's Next for Entrepreneurs?
Silicon Valley Bank's failure in the face of rising interest rates shook founders and funders across the country. Julia Austin, Jeffrey Bussgang, and Rembrand Koning share key insights for rattled entrepreneurs trying to make sense of the financing landscape.
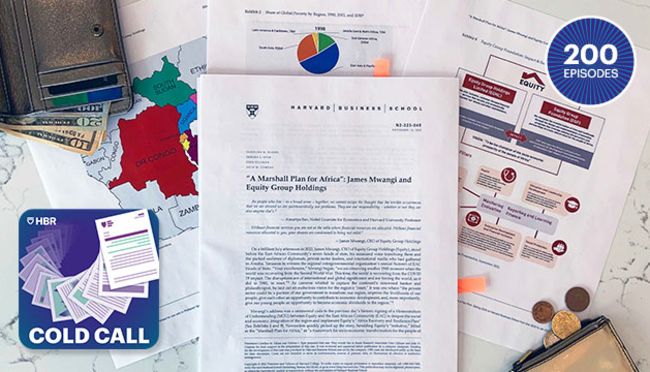
- 27 Apr 2023
Equity Bank CEO James Mwangi: Transforming Lives with Access to Credit
James Mwangi, CEO of Equity Bank, has transformed lives and livelihoods throughout East and Central Africa by giving impoverished people access to banking accounts and micro loans. He’s been so successful that in 2020 Forbes coined the term “the Mwangi Model.” But can we really have both purpose and profit in a firm? Harvard Business School professor Caroline Elkins, who has spent decades studying Africa, explores how this model has become one that business leaders are seeking to replicate throughout the world in her case, “A Marshall Plan for Africa': James Mwangi and Equity Group Holdings.” As part of a new first-year MBA course at Harvard Business School, this case examines the central question: what is the social purpose of the firm?
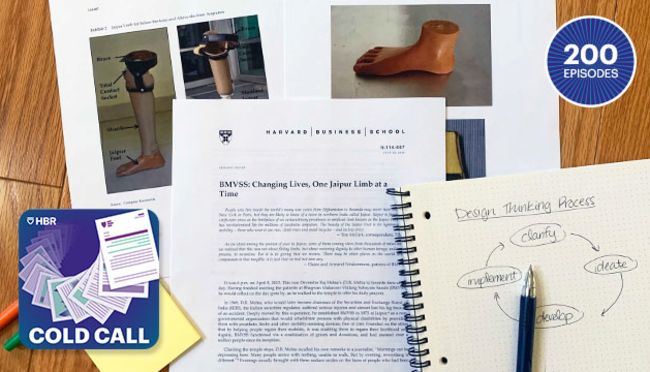
- 25 Apr 2023
Using Design Thinking to Invent a Low-Cost Prosthesis for Land Mine Victims
Bhagwan Mahaveer Viklang Sahayata Samiti (BMVSS) is an Indian nonprofit famous for creating low-cost prosthetics, like the Jaipur Foot and the Stanford-Jaipur Knee. Known for its patient-centric culture and its focus on innovation, BMVSS has assisted more than one million people, including many land mine survivors. How can founder D.R. Mehta devise a strategy that will ensure the financial sustainability of BMVSS while sustaining its human impact well into the future? Harvard Business School Dean Srikant Datar discusses the importance of design thinking in ensuring a culture of innovation in his case, “BMVSS: Changing Lives, One Jaipur Limb at a Time.”
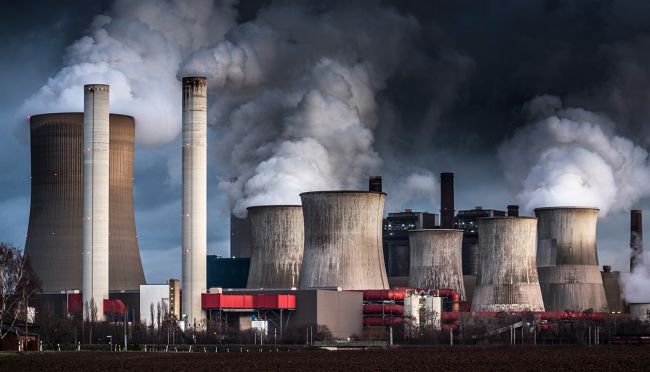
- 18 Apr 2023
What Happens When Banks Ditch Coal: The Impact Is 'More Than Anyone Thought'
Bank divestment policies that target coal reduced carbon dioxide emissions, says research by Boris Vallée and Daniel Green. Could the finance industry do even more to confront climate change?
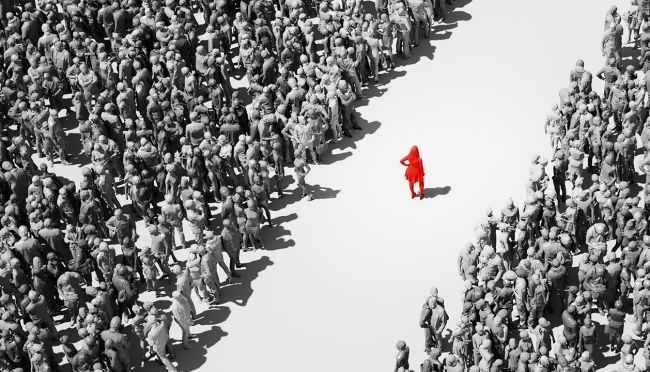
The Best Person to Lead Your Company Doesn't Work There—Yet
Recruiting new executive talent to revive portfolio companies has helped private equity funds outperform major stock indexes, says research by Paul Gompers. Why don't more public companies go beyond their senior executives when looking for top leaders?
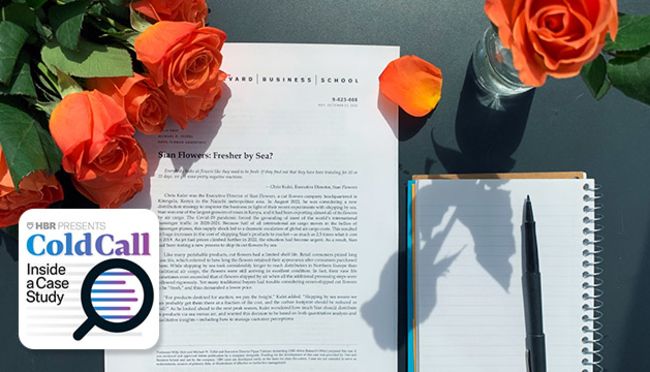
- 11 Apr 2023
A Rose by Any Other Name: Supply Chains and Carbon Emissions in the Flower Industry
Headquartered in Kitengela, Kenya, Sian Flowers exports roses to Europe. Because cut flowers have a limited shelf life and consumers want them to retain their appearance for as long as possible, Sian and its distributors used international air cargo to transport them to Amsterdam, where they were sold at auction and trucked to markets across Europe. But when the Covid-19 pandemic caused huge increases in shipping costs, Sian launched experiments to ship roses by ocean using refrigerated containers. The company reduced its costs and cut its carbon emissions, but is a flower that travels halfway around the world truly a “low-carbon rose”? Harvard Business School professors Willy Shih and Mike Toffel debate these questions and more in their case, “Sian Flowers: Fresher by Sea?”
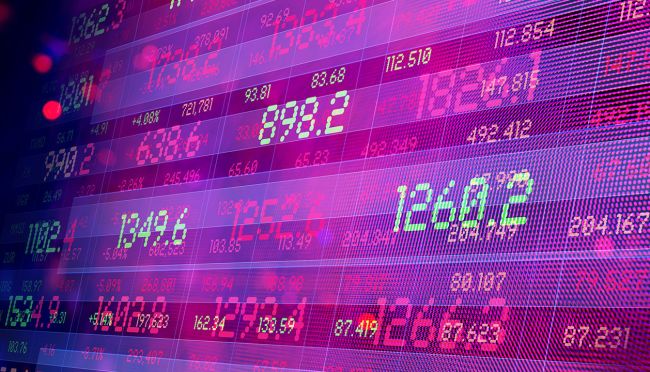
Is Amazon a Retailer, a Tech Firm, or a Media Company? How AI Can Help Investors Decide
More companies are bringing seemingly unrelated businesses together in new ways, challenging traditional stock categories. MarcAntonio Awada and Suraj Srinivasan discuss how applying machine learning to regulatory data could reveal new opportunities for investors.
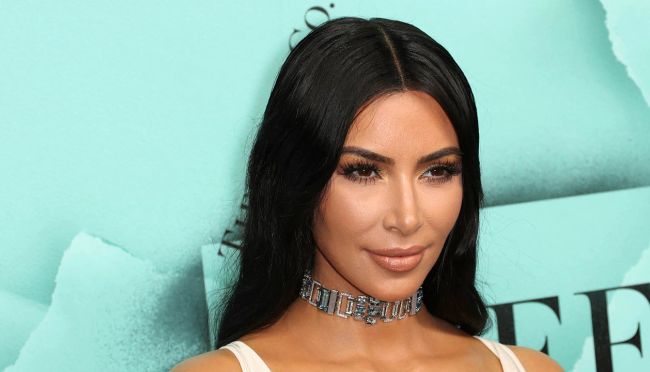
- 07 Apr 2023
When Celebrity ‘Crypto-Influencers’ Rake in Cash, Investors Lose Big
Kim Kardashian, Lindsay Lohan, and other entertainers have been accused of promoting crypto products on social media without disclosing conflicts. Research by Joseph Pacelli shows what can happen to eager investors who follow them.
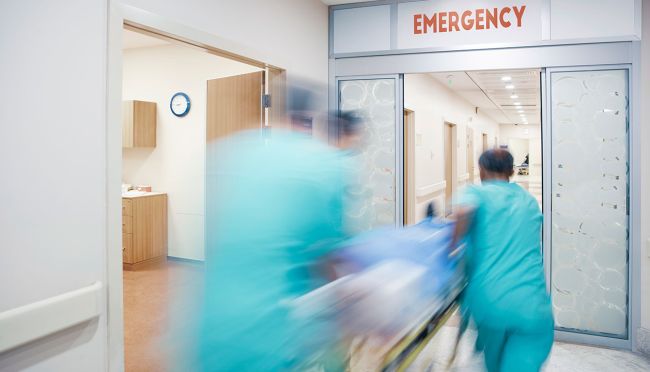
- 31 Mar 2023
Can a ‘Basic Bundle’ of Health Insurance Cure Coverage Gaps and Spur Innovation?
One in 10 people in America lack health insurance, resulting in $40 billion of care that goes unpaid each year. Amitabh Chandra and colleagues say ensuring basic coverage for all residents, as other wealthy nations do, could address the most acute needs and unlock efficiency.
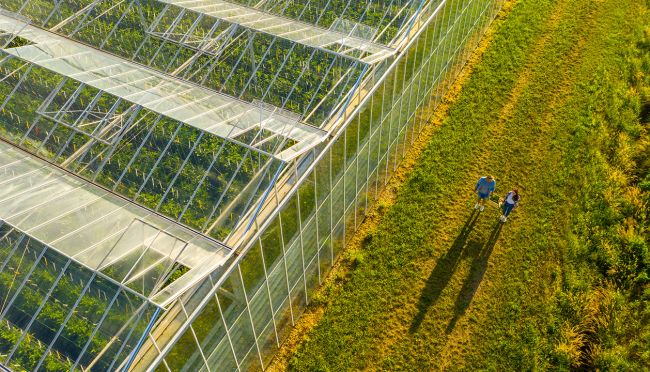
- 23 Mar 2023
As Climate Fears Mount, More Investors Turn to 'ESG' Funds Despite Few Rules
Regulations and ratings remain murky, but that's not deterring climate-conscious investors from paying more for funds with an ESG label. Research by Mark Egan and Malcolm Baker sizes up the premium these funds command. Is it time for more standards in impact investing?
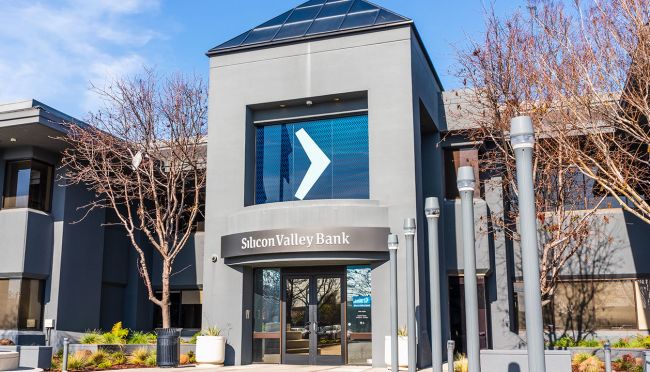
- 14 Mar 2023
What Does the Failure of Silicon Valley Bank Say About the State of Finance?
Silicon Valley Bank wasn't ready for the Fed's interest rate hikes, but that's only part of the story. Victoria Ivashina and Erik Stafford probe the complex factors that led to the second-biggest bank failure ever.
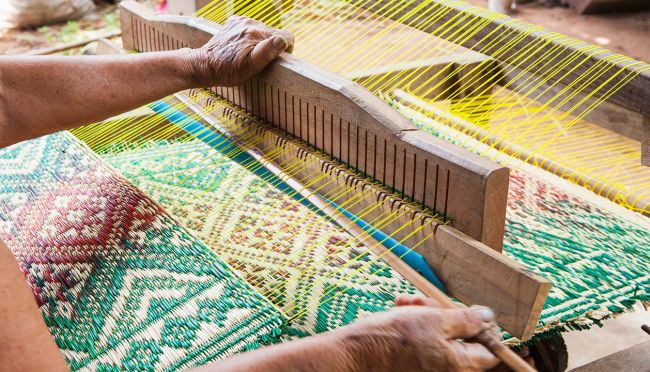
- 13 Mar 2023
What Would It Take to Unlock Microfinance's Full Potential?
Microfinance has been seen as a vehicle for economic mobility in developing countries, but the results have been mixed. Research by Natalia Rigol and Ben Roth probes how different lending approaches might serve entrepreneurs better.
Help | Advanced Search
Economics > General Economics
Title: financial performance and innovation: evidence from usa, 1998-2023.
Abstract: This study explores the relationship between R&D intensity, as a measure of innovation, and financial performance among S&P 500 companies over 100 quarters from 1998 to 2023, including multiple crisis periods. It challenges the conventional wisdom that larger companies are more prone to innovate, using a comprehensive dataset across various industries. The analysis reveals diverse associations between innovation and key financial indicators such as firm size, assets, EBITDA, and tangibility. Our findings underscore the importance of innovation in enhancing firm competitiveness and market positioning, highlighting the effectiveness of countercyclical innovation policies. This research contributes to the debate on the role of R&D investments in driving firm value, offering new insights for both academic and policy discussions.
Submission history
Access paper:.
- Other Formats

References & Citations
- Google Scholar
- Semantic Scholar
BibTeX formatted citation

Bibliographic and Citation Tools
Code, data and media associated with this article, recommenders and search tools.
- Institution
arXivLabs: experimental projects with community collaborators
arXivLabs is a framework that allows collaborators to develop and share new arXiv features directly on our website.
Both individuals and organizations that work with arXivLabs have embraced and accepted our values of openness, community, excellence, and user data privacy. arXiv is committed to these values and only works with partners that adhere to them.
Have an idea for a project that will add value for arXiv's community? Learn more about arXivLabs .
FinancialResearch.gov
Conferences, 2024 financial stability conference – call for papers.
Published: June 4, 2024
Share on Facebook Share on Linked In Logo for Twitter
The Federal Reserve Bank of Cleveland and the Office of Financial Research invite the submission of research and policy-oriented papers for the 2024 Financial Stability Conference on November 21–22, 2024. The conference will be held in person in Cleveland, Ohio, and virtually.
Markets and institutions, increasingly interconnected, are being challenged by the dizzying pace of changes in the financial system, accelerating the buildup of risk and threats to solvency. Regulatory adaptations add another layer of complexity to the issue. Increasingly sophisticated algorithms and the rise of generative artificial intelligence may create new vulnerabilities across the system as banks, nonbank financial institutions, and financial markets exploit nascent opportunities. The twelfth annual conference will explore how firms and markets can become resilient or even antifragile and how regulators can encourage and accommodate needed changes.
Conference Format
The conference will bring together policymakers, market participants, and researchers in two types of sessions:
- Policy Discussions These sessions include keynote addresses and panel discussions in which participants from industry, regulatory agencies, and academia share their insights.
- Research Forums These forums follow the format of an academic workshop and comprise sessions to discuss submitted papers.
We welcome submissions of research on topics related to potential financial stability risks faced by financial markets and institutions, sources of financial system resilience, and related public policy. Conference topics include but are not limited to the following:
Emerging Risks
As the financial system continues to evolve, new risks emerge along with new businesses, new strategies, and new technologies. Old problems take on new dimensions as fiscal and monetary policies adapt to new economic and political realities, thereby adding new stresses to regulatory frameworks that themselves struggle to adapt. As information technology moves risk out of closely regulated sectors, it also creates new vulnerabilities from cyber-attacks. A rapidly changing physical environment and the prospect of nonhuman intelligences add even more uncertainty.
- Financial stability concerns related to faster payments and equity transactions such as the implementation of t+1 settlement
- The financial stability implications of generative AI and deep learning
- Cryptocurrencies, smart contracts, and blockchain
- Cyber-attacks
- Climate risk
- Interaction of monetary policy with macroprudential supervision
- Sources of resilience in the financial sector
Financial Institutions
A riskier macroeconomic environment poses challenges for financial institutions and their supervisors. Risk management tools and strategies will be tested by fluctuations in inflation and output and by new regulations designed to mitigate vulnerabilities. Network effects, including interactions with a rapidly evolving fintech and crypto sector, may lead to further risks at a systemic level. How are institutions adapting to these risks and associated regulatory changes? How prepared are regulators and policymakers? Are existing microprudential and macroprudential toolkits sufficient?
- Bank lending to nonbank financial institutions (NBFI)
- Insurance markets
- Banking as a service (BaaS)
- Regional banks
- Interest rate risk
- Risks of rapid growth
- Unrealized losses on balance sheets and mark-to-market accounting
- Impact of reforms to lenders of last resort, deposit Insurance, capital rules, and the FHLB system
Financial Markets
Inflation and the associated responses of central banks around the world have contributed to stress to financial markets that has not been seen in the recent past. Financial stability threats may arise from resulting reallocations through volatility spikes, fire sales, and financial contagion. The continued development of algorithms, decentralized finance (DeFi), and complex artificial intelligence has the potential to add novel risks to financial markets. To what extent do investors recognize these risks, and how does recognition affect investors’ allocations? How does opacity resulting from deficiencies in reporting, risk management, and operation standards for these risks affect investor behavior?
- Risks associated with high levels and issuance of public debt (for example, recent volatility around Treasury funding announcements, concerns about primary dealers and principal trading firms, the SEC’s recent rule about what defines a dealer and what that might mean for Treasury markets)
- Short-term funding
- Implications of deficits, central bank balance sheet policies, and financial stability
- The impact of technological innovation on financial markets
Real Estate Markets
Real estate is often one of the sectors most affected by and can be a cause of financial instability. Construction and housing play a major role in the transmission of monetary policy, and real estate-based lending remains a major activity of banks, insurance companies, and mortgage companies. A complex and active securities market ties together financial institutions and markets in both residential and commercial real estate.
- Commercial real estate (CRE)
- Nonbank originators and servicers
- International contagion
- Implications of remote work and the impact of COVID-19
- Effects of monetary policy on real estate markets
Scientific Committee
- Vikas Agarwal, Georgia State University
- Marco Di Maggio, Harvard University
- Michael Fleming, Federal Reserve Bank of New York
- Rod Garratt, University of California, Santa Barbara
- Mariassunta Giannetti, Stockholm School of Economics
- Arpit Gupta, New York University, Stern School of Business
- Zhiguo He, Stanford University
- Zhaogang Song, Johns Hopkins University
- Russell R. Wermers, Robert H. Smith School of Business, The University of Maryland at College Park
Paper Submission Procedure
The deadline for submissions is Friday, July 5, 2024. Please submit completed papers through Conference Maker . Notification of acceptance will be provided by Friday, September 6, 2024. Final conference papers are due on Friday, November 1, 2024. In-person paper presentations are preferred. Questions should be directed to [email protected] .
Back to Conferences
You are now leaving the OFR’s website.
You will be redirected to:
You are now leaving the OFR Website. The website associated with the link you have selected is located on another server and is not subject to Federal information quality, privacy, security, and related guidelines. To remain on the OFR Website, click 'Cancel'. To continue to the other website you selected, click 'Proceed'. The OFR does not endorse this other website, its sponsor, or any of the views, activities, products, or services offered on the website or by any advertiser on the website.
Thank you for visiting www.financialresearch.gov.
Banking on Trust: Supervisory Transparency and Depositors’ Actions

We explore the role of institutional trust in influencing depositors’ reactions to bank super-visory actions in India. Utilizing a unique event that led to unexpected penalties on banks and the quasi-random nature of branch locations, we find news of penalties on some banks leading to deposit withdrawals from offending and neighboring nonoffending branches. Such withdrawals are more pronounced in regions with lower trust in public institutions, including trust in courts and banks. Trust is associated with information access and the quality of local services. We find limited evidence that credit and economic activity also decline in regions with deposit withdrawals.
More Research From These Scholars
Bank supervision and managerial control systems: the case of minority lending, community membership and reciprocity in lending: evidence from informal markets, access to credit in informal economies: does financial information matter.
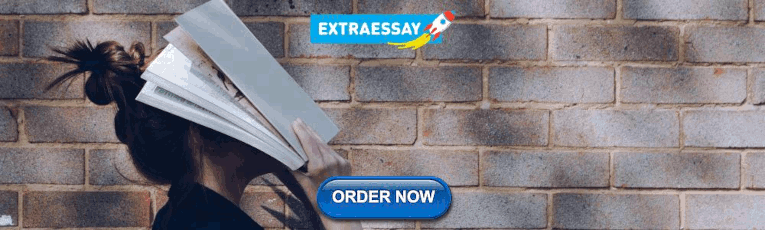
COMMENTS
by Carolin E. Pflueger, Emil Siriwardane, and Adi Sunderam. This paper sheds new light on connections between financial markets and the macroeconomy. It shows that investors' appetite for risk—revealed by common movements in the pricing of volatile securities—helps determine economic outcomes and real interest rates.
The Journal of Financial Markets publishes high quality original research on applied and theoretical issues related to securities trading and pricing. Area of coverage includes the analysis and design of trading mechanisms, optimal order placement strategies, the role of information in securities …. View full aims & scope.
The Journal of Finance publishes leading research across all the major fields of financial research. It is the most widely cited academic journal on finance. Each issue of the journal reaches over 8,000 academics, finance professionals, libraries, government and financial institutions around the world. Published six times a year, the journal is the official publication of The American Finance ...
The Journal of Finance publishes leading research across all the major fields of financial research. It is the most widely cited academic journal on finance and one of the most widely cited journals in economics as well. Each issue of the journal reaches over 8,000 academics, finance professionals, libraries, government and financial ...
Explore the latest full-text research PDFs, articles, conference papers, preprints and more on FINANCIAL MARKETS. Find methods information, sources, references or conduct a literature review on ...
This paper looks at how the social sciences study financial markets by making a very short survey of Financial Economics, Behavioural Finance, Sociology and International Political Economy in an ...
Many research papers (39 out of 110) employ AI as a predictive instrument for forecasting stock prices, performance and volatility. In 23 papers, AI is employed in classification problems and warning systems to detect credit risk and frauds, as well as to monitor firm or bank performance. ... Classification-based financial markets prediction ...
2.3 Classification of Financial Markets and Monetary Policy Research In framing financial market and moneta ry policy research, there is the need to establish and describe the classification of re ...
Financial Markets and Portfolio Management (FMPM), addresses all areas of finance, including financial markets, portfolio theory and wealth management, asset pricing, corporate finance, corporate governance, alternative investments, risk management, and regulation.. Official publication of the Swiss Financial Analysts Association. Serves as a bridge between innovative research and its ...
About the Journal. We specialize in the publication of original studies in the fields of finance and economics, specifically in the areas of financial markets, institutions and instruments. Financial Markets, Institutions and Instruments bridges the gap between the academic and professional finance communities. Submit your paper to this journal.
Qualitative Research in Financial Markets is dedicated to publishing research that employs qualitative methods and tools to explore contemporary issues in corporate finance and financial market behaviour across the world. The journal welcomes high quality submissions of empirical papers and analyses of methodological debates in the field.
DOI 10.3386/w32519. Issue Date May 2024. We build a model of the law of small numbers (LSN)—the incorrect belief that even small samples represent the properties of the underlying population—to study its implications for trading behavior and asset prices. In our model, a belief in the LSN induces investors to expect short-term price trends ...
In response to the stock market crash, the Security Exchange Commission (SEC) published a white paper analyzing the causes of the collapse. The key finding of the report was that index futures and program trading caused the crash. When markets started to decline, investors sold index futures, whereby S&P500 index futures fell by 29%.
Evolution of sentiment. Through NLP techniques, we detect entities (i.e., companies) from financial news articles on Reuters, and merge entities that co-reference the same company.
Conlisk (1996) presented a number of papers dealing with limited rationality; Duxbury (2015) made a research of perceptions provided by experimental studies examining financial decisions and market behavior; whereas Libby et al. (2002) compiled a series of experimental papers in finance to illustrate how experiments can be conducted successfully.
It has been 55 years since the publication of Eugene Fama's paper "Random Walks in Stock Market Prices" (Fama 1965b) in the pages of the Financial Analysts Journal (Journal). Footnote 1 The profound impact of his work is still being felt today. Up to that publication, making money on Wall Street had been considered easy.
2. Literature review. The role of investment behaviour holds critical importance in determining the performance of financial markets. Three factors were considered in investment behaviour: risk perception, satisfaction, and rate of profitability (Nguyen et al., Citation 2020).The moderating variable that was taken in this research is the uncertainty of Covid-19 and how it impacts the financial ...
Finance Research Letters invites submissions in all areas of finance, broadly defined. Finance Research Letters offers and ensures the rapid publication of important new results in these areas. We aim to provide a rapid response to papers, with all papers undergoing a desk review by one of the …. View full aims & scope.
In this paper, I provide an overview of the research on the real effects of financial reporting on investing and financing decisions made by firms. Accounting can improve investment efficiency and affect nearly every aspect of the financing decision by reducing information asymmetry and improving monitoring.
This paper reviews and explores the way that predictive analytic methods are used by scholars in finance, and in computational fields of study, to make forecasts. A systemic identification of methods and use cases is presented, specifically drawn from data sourced either internally or externally from financial Information Systems (IS).
New research on finance from Harvard Business School faculty on issues and topics including corporate investment, governance, and accounting management. ... Financial planners must find new ways to market to tech-savvy millennials and gen Z investors or risk irrelevancy. Research by Marco Di Maggio probes the generational challenges that ...
This paper examines the systemic implications of the supply of liquid safe assets, such as Treasury bills. The paper explores how liquid safe assets facilitate the trades of risky assets. The paper finds that financial markets may be remarkably resilient to changes in the stock of liquid assets. (Working Paper no. 15-23)
This study explores the relationship between R&D intensity, as a measure of innovation, and financial performance among S&P 500 companies over 100 quarters from 1998 to 2023, including multiple crisis periods. It challenges the conventional wisdom that larger companies are more prone to innovate, using a comprehensive dataset across various industries. The analysis reveals diverse associations ...
Notification of acceptance will be provided by Friday, September 6, 2024. Final conference papers are due on Friday, November 1, 2024. In-person paper presentations are preferred. Questions should be directed to [email protected]. The 2024 Financial Stability Conference hosted by the OFR and Federal Reserve Bank of ...
We will clarify many of the empirical studies, which focused on studying the relationship between the various fundamental analysis models, and show how its predictive ability of future stock value in different financial markets; developed and emerging as follows. 3.1. In the developed markets: In a study by Penman and Sougiannis (1998) aimed at ...
Research Rangahau. Discover impactful research at New Zealand's top-ranked research university. ... This paper introduces students to the principal capital markets in Australasia, their associated institutions, markets, regulators and their financial functions. Teaching Periods and Locations. 18B (HAM) Hamilton, On-campus. 18B (HAM) B Trimester ...
The structure of financial markets is a constantly developing organism displaying an ever-changing pattern of the weight in the overall financial market structure of its constituents such as ...
We explore the role of institutional trust in influencing depositors' reactions to bank super-visory actions in India. Utilizing a unique event that led to unexpected penalties on banks and the quasi-random nature of branch locations, we find news of penalties on some banks leading to deposit withdrawals from offending and neighboring nonoffending branches.
A Feature Paper should be a substantial original Article that involves several techniques or approaches, provides an outlook for future research directions and describes possible research applications. Feature papers are submitted upon individual invitation or recommendation by the scientific editors and must receive positive feedback from the ...
Acknowledging that high-quality, easily available investment research supports deep capital markets, listed companies, and economic growth, the UK Financial Conduct Authority (FCA) recently issued a consultation paper proposing to give UK buyside firms an additional option for how to pay for investment research. The so-called "new" option allows for the bundling of payments for third-party ...