Templates for college and university assignments
Include customizable templates in your college toolbox. stay focused on your studies and leave the assignment structuring to tried and true layout templates for all kinds of papers, reports, and more..
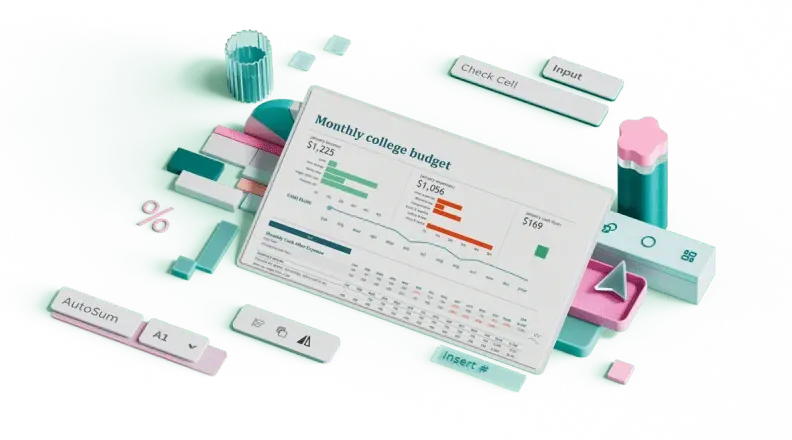
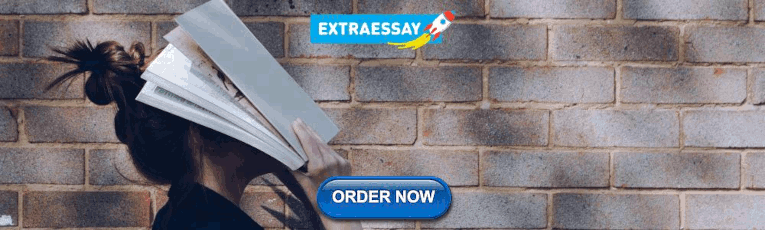
Keep your college toolbox stocked with easy-to-use templates
Work smarter with higher-ed helpers from our college tools collection. Presentations are on point from start to finish when you start your project using a designer-created template; you'll be sure to catch and keep your professor's attention. Staying on track semester after semester takes work, but that work gets a little easier when you take control of your scheduling, list making, and planning by using trackers and planners that bring you joy. Learning good habits in college will serve you well into your professional life after graduation, so don't reinvent the wheel—use what is known to work!

Understanding Assignments
What this handout is about.
The first step in any successful college writing venture is reading the assignment. While this sounds like a simple task, it can be a tough one. This handout will help you unravel your assignment and begin to craft an effective response. Much of the following advice will involve translating typical assignment terms and practices into meaningful clues to the type of writing your instructor expects. See our short video for more tips.
Basic beginnings
Regardless of the assignment, department, or instructor, adopting these two habits will serve you well :
- Read the assignment carefully as soon as you receive it. Do not put this task off—reading the assignment at the beginning will save you time, stress, and problems later. An assignment can look pretty straightforward at first, particularly if the instructor has provided lots of information. That does not mean it will not take time and effort to complete; you may even have to learn a new skill to complete the assignment.
- Ask the instructor about anything you do not understand. Do not hesitate to approach your instructor. Instructors would prefer to set you straight before you hand the paper in. That’s also when you will find their feedback most useful.
Assignment formats
Many assignments follow a basic format. Assignments often begin with an overview of the topic, include a central verb or verbs that describe the task, and offer some additional suggestions, questions, or prompts to get you started.
An Overview of Some Kind
The instructor might set the stage with some general discussion of the subject of the assignment, introduce the topic, or remind you of something pertinent that you have discussed in class. For example:
“Throughout history, gerbils have played a key role in politics,” or “In the last few weeks of class, we have focused on the evening wear of the housefly …”
The Task of the Assignment
Pay attention; this part tells you what to do when you write the paper. Look for the key verb or verbs in the sentence. Words like analyze, summarize, or compare direct you to think about your topic in a certain way. Also pay attention to words such as how, what, when, where, and why; these words guide your attention toward specific information. (See the section in this handout titled “Key Terms” for more information.)
“Analyze the effect that gerbils had on the Russian Revolution”, or “Suggest an interpretation of housefly undergarments that differs from Darwin’s.”
Additional Material to Think about
Here you will find some questions to use as springboards as you begin to think about the topic. Instructors usually include these questions as suggestions rather than requirements. Do not feel compelled to answer every question unless the instructor asks you to do so. Pay attention to the order of the questions. Sometimes they suggest the thinking process your instructor imagines you will need to follow to begin thinking about the topic.
“You may wish to consider the differing views held by Communist gerbils vs. Monarchist gerbils, or Can there be such a thing as ‘the housefly garment industry’ or is it just a home-based craft?”
These are the instructor’s comments about writing expectations:
“Be concise”, “Write effectively”, or “Argue furiously.”
Technical Details
These instructions usually indicate format rules or guidelines.
“Your paper must be typed in Palatino font on gray paper and must not exceed 600 pages. It is due on the anniversary of Mao Tse-tung’s death.”
The assignment’s parts may not appear in exactly this order, and each part may be very long or really short. Nonetheless, being aware of this standard pattern can help you understand what your instructor wants you to do.
Interpreting the assignment
Ask yourself a few basic questions as you read and jot down the answers on the assignment sheet:
Why did your instructor ask you to do this particular task?
Who is your audience.
- What kind of evidence do you need to support your ideas?
What kind of writing style is acceptable?
- What are the absolute rules of the paper?
Try to look at the question from the point of view of the instructor. Recognize that your instructor has a reason for giving you this assignment and for giving it to you at a particular point in the semester. In every assignment, the instructor has a challenge for you. This challenge could be anything from demonstrating an ability to think clearly to demonstrating an ability to use the library. See the assignment not as a vague suggestion of what to do but as an opportunity to show that you can handle the course material as directed. Paper assignments give you more than a topic to discuss—they ask you to do something with the topic. Keep reminding yourself of that. Be careful to avoid the other extreme as well: do not read more into the assignment than what is there.
Of course, your instructor has given you an assignment so that they will be able to assess your understanding of the course material and give you an appropriate grade. But there is more to it than that. Your instructor has tried to design a learning experience of some kind. Your instructor wants you to think about something in a particular way for a particular reason. If you read the course description at the beginning of your syllabus, review the assigned readings, and consider the assignment itself, you may begin to see the plan, purpose, or approach to the subject matter that your instructor has created for you. If you still aren’t sure of the assignment’s goals, try asking the instructor. For help with this, see our handout on getting feedback .
Given your instructor’s efforts, it helps to answer the question: What is my purpose in completing this assignment? Is it to gather research from a variety of outside sources and present a coherent picture? Is it to take material I have been learning in class and apply it to a new situation? Is it to prove a point one way or another? Key words from the assignment can help you figure this out. Look for key terms in the form of active verbs that tell you what to do.
Key Terms: Finding Those Active Verbs
Here are some common key words and definitions to help you think about assignment terms:
Information words Ask you to demonstrate what you know about the subject, such as who, what, when, where, how, and why.
- define —give the subject’s meaning (according to someone or something). Sometimes you have to give more than one view on the subject’s meaning
- describe —provide details about the subject by answering question words (such as who, what, when, where, how, and why); you might also give details related to the five senses (what you see, hear, feel, taste, and smell)
- explain —give reasons why or examples of how something happened
- illustrate —give descriptive examples of the subject and show how each is connected with the subject
- summarize —briefly list the important ideas you learned about the subject
- trace —outline how something has changed or developed from an earlier time to its current form
- research —gather material from outside sources about the subject, often with the implication or requirement that you will analyze what you have found
Relation words Ask you to demonstrate how things are connected.
- compare —show how two or more things are similar (and, sometimes, different)
- contrast —show how two or more things are dissimilar
- apply—use details that you’ve been given to demonstrate how an idea, theory, or concept works in a particular situation
- cause —show how one event or series of events made something else happen
- relate —show or describe the connections between things
Interpretation words Ask you to defend ideas of your own about the subject. Do not see these words as requesting opinion alone (unless the assignment specifically says so), but as requiring opinion that is supported by concrete evidence. Remember examples, principles, definitions, or concepts from class or research and use them in your interpretation.
- assess —summarize your opinion of the subject and measure it against something
- prove, justify —give reasons or examples to demonstrate how or why something is the truth
- evaluate, respond —state your opinion of the subject as good, bad, or some combination of the two, with examples and reasons
- support —give reasons or evidence for something you believe (be sure to state clearly what it is that you believe)
- synthesize —put two or more things together that have not been put together in class or in your readings before; do not just summarize one and then the other and say that they are similar or different—you must provide a reason for putting them together that runs all the way through the paper
- analyze —determine how individual parts create or relate to the whole, figure out how something works, what it might mean, or why it is important
- argue —take a side and defend it with evidence against the other side
More Clues to Your Purpose As you read the assignment, think about what the teacher does in class:
- What kinds of textbooks or coursepack did your instructor choose for the course—ones that provide background information, explain theories or perspectives, or argue a point of view?
- In lecture, does your instructor ask your opinion, try to prove their point of view, or use keywords that show up again in the assignment?
- What kinds of assignments are typical in this discipline? Social science classes often expect more research. Humanities classes thrive on interpretation and analysis.
- How do the assignments, readings, and lectures work together in the course? Instructors spend time designing courses, sometimes even arguing with their peers about the most effective course materials. Figuring out the overall design to the course will help you understand what each assignment is meant to achieve.
Now, what about your reader? Most undergraduates think of their audience as the instructor. True, your instructor is a good person to keep in mind as you write. But for the purposes of a good paper, think of your audience as someone like your roommate: smart enough to understand a clear, logical argument, but not someone who already knows exactly what is going on in your particular paper. Remember, even if the instructor knows everything there is to know about your paper topic, they still have to read your paper and assess your understanding. In other words, teach the material to your reader.
Aiming a paper at your audience happens in two ways: you make decisions about the tone and the level of information you want to convey.
- Tone means the “voice” of your paper. Should you be chatty, formal, or objective? Usually you will find some happy medium—you do not want to alienate your reader by sounding condescending or superior, but you do not want to, um, like, totally wig on the man, you know? Eschew ostentatious erudition: some students think the way to sound academic is to use big words. Be careful—you can sound ridiculous, especially if you use the wrong big words.
- The level of information you use depends on who you think your audience is. If you imagine your audience as your instructor and they already know everything you have to say, you may find yourself leaving out key information that can cause your argument to be unconvincing and illogical. But you do not have to explain every single word or issue. If you are telling your roommate what happened on your favorite science fiction TV show last night, you do not say, “First a dark-haired white man of average height, wearing a suit and carrying a flashlight, walked into the room. Then a purple alien with fifteen arms and at least three eyes turned around. Then the man smiled slightly. In the background, you could hear a clock ticking. The room was fairly dark and had at least two windows that I saw.” You also do not say, “This guy found some aliens. The end.” Find some balance of useful details that support your main point.
You’ll find a much more detailed discussion of these concepts in our handout on audience .
The Grim Truth
With a few exceptions (including some lab and ethnography reports), you are probably being asked to make an argument. You must convince your audience. It is easy to forget this aim when you are researching and writing; as you become involved in your subject matter, you may become enmeshed in the details and focus on learning or simply telling the information you have found. You need to do more than just repeat what you have read. Your writing should have a point, and you should be able to say it in a sentence. Sometimes instructors call this sentence a “thesis” or a “claim.”
So, if your instructor tells you to write about some aspect of oral hygiene, you do not want to just list: “First, you brush your teeth with a soft brush and some peanut butter. Then, you floss with unwaxed, bologna-flavored string. Finally, gargle with bourbon.” Instead, you could say, “Of all the oral cleaning methods, sandblasting removes the most plaque. Therefore it should be recommended by the American Dental Association.” Or, “From an aesthetic perspective, moldy teeth can be quite charming. However, their joys are short-lived.”
Convincing the reader of your argument is the goal of academic writing. It doesn’t have to say “argument” anywhere in the assignment for you to need one. Look at the assignment and think about what kind of argument you could make about it instead of just seeing it as a checklist of information you have to present. For help with understanding the role of argument in academic writing, see our handout on argument .
What kind of evidence do you need?
There are many kinds of evidence, and what type of evidence will work for your assignment can depend on several factors–the discipline, the parameters of the assignment, and your instructor’s preference. Should you use statistics? Historical examples? Do you need to conduct your own experiment? Can you rely on personal experience? See our handout on evidence for suggestions on how to use evidence appropriately.
Make sure you are clear about this part of the assignment, because your use of evidence will be crucial in writing a successful paper. You are not just learning how to argue; you are learning how to argue with specific types of materials and ideas. Ask your instructor what counts as acceptable evidence. You can also ask a librarian for help. No matter what kind of evidence you use, be sure to cite it correctly—see the UNC Libraries citation tutorial .
You cannot always tell from the assignment just what sort of writing style your instructor expects. The instructor may be really laid back in class but still expect you to sound formal in writing. Or the instructor may be fairly formal in class and ask you to write a reflection paper where you need to use “I” and speak from your own experience.
Try to avoid false associations of a particular field with a style (“art historians like wacky creativity,” or “political scientists are boring and just give facts”) and look instead to the types of readings you have been given in class. No one expects you to write like Plato—just use the readings as a guide for what is standard or preferable to your instructor. When in doubt, ask your instructor about the level of formality they expect.
No matter what field you are writing for or what facts you are including, if you do not write so that your reader can understand your main idea, you have wasted your time. So make clarity your main goal. For specific help with style, see our handout on style .
Technical details about the assignment
The technical information you are given in an assignment always seems like the easy part. This section can actually give you lots of little hints about approaching the task. Find out if elements such as page length and citation format (see the UNC Libraries citation tutorial ) are negotiable. Some professors do not have strong preferences as long as you are consistent and fully answer the assignment. Some professors are very specific and will deduct big points for deviations.
Usually, the page length tells you something important: The instructor thinks the size of the paper is appropriate to the assignment’s parameters. In plain English, your instructor is telling you how many pages it should take for you to answer the question as fully as you are expected to. So if an assignment is two pages long, you cannot pad your paper with examples or reword your main idea several times. Hit your one point early, defend it with the clearest example, and finish quickly. If an assignment is ten pages long, you can be more complex in your main points and examples—and if you can only produce five pages for that assignment, you need to see someone for help—as soon as possible.
Tricks that don’t work
Your instructors are not fooled when you:
- spend more time on the cover page than the essay —graphics, cool binders, and cute titles are no replacement for a well-written paper.
- use huge fonts, wide margins, or extra spacing to pad the page length —these tricks are immediately obvious to the eye. Most instructors use the same word processor you do. They know what’s possible. Such tactics are especially damning when the instructor has a stack of 60 papers to grade and yours is the only one that low-flying airplane pilots could read.
- use a paper from another class that covered “sort of similar” material . Again, the instructor has a particular task for you to fulfill in the assignment that usually relates to course material and lectures. Your other paper may not cover this material, and turning in the same paper for more than one course may constitute an Honor Code violation . Ask the instructor—it can’t hurt.
- get all wacky and “creative” before you answer the question . Showing that you are able to think beyond the boundaries of a simple assignment can be good, but you must do what the assignment calls for first. Again, check with your instructor. A humorous tone can be refreshing for someone grading a stack of papers, but it will not get you a good grade if you have not fulfilled the task.
Critical reading of assignments leads to skills in other types of reading and writing. If you get good at figuring out what the real goals of assignments are, you are going to be better at understanding the goals of all of your classes and fields of study.
You may reproduce it for non-commercial use if you use the entire handout and attribute the source: The Writing Center, University of North Carolina at Chapel Hill
Make a Gift
EL Education Curriculum
You are here.
- ELA 2019 G6:M4:U2:L12
Analyze a Hidden Figure: Katherine Johnson
In this lesson, daily learning targets, ongoing assessment.
- Technology and Multimedia
Supporting English Language Learners
Materials from previous lessons, new materials, closing & assessments, you are here:.
- ELA 2019 Grade 6
- ELA 2019 G6:M4
- ELA 2019 G6:M4:U2
Like what you see?
Order printed materials, teacher guides and more.
How to order
Help us improve!
Tell us how the curriculum is working in your classroom and send us corrections or suggestions for improving it.
Leave feedback
Focus Standards: These are the standards the instruction addresses.
- RI.6.1, RI.6.3, W.6.1, W.6.9b
Supporting Standards: These are the standards that are incidental—no direct instruction in this lesson, but practice of these standards occurs as a result of addressing the focus standards.
- RL.6.10, RI.6.8, W.6.10
- I can analyze how the author introduces and elaborates on Katherine Johnson's character in the text. (RI.6.3)
- I can gather evidence and develop reasoning for an argument essay about Katherine Johnson's remarkable accomplishments. (W.6.1)
- I can share my independent research reading with my peers. (RL.6.10, RI.6.10)
- Opening A: Entrance Ticket: Unit 2, Lesson 12 (RI.6.1, RI.6.8, W.6.1, W.6.10)
- Work Time A: Gist on sticky notes
- Work Time B: Collaborative Argument Evidence note-catcher (RI.6.1, RI.6.3, W.6.1, W.6.9b, W.6.10)
- Prepare students for the Jigsaw protocol:
- Review the Jigsaw protocol as needed to ensure clear directions and smooth transitions.
- Strategically group students into A or B groups.
- Display the jigsaw groups and each group's reading assignments on chart paper or an external computer monitor for students for easy reference.
- Read chapters 13 and 16 in Hidden Figures in advance to identify plot points and vocabulary that may require clarification or sensitivity.
- Review the Independent Reading Sample Plans to determine the best way for students to reflect on and share what they have learned about the module topic from their independent work.
- Prepare copies of handouts for students (see Materials list).
- Post the learning targets and applicable anchor charts (see Materials list).
Tech and Multimedia
- Work Time B: Display jigsaw groups and reading assignments on chart paper or an external monitor for students to reference easily.
- Work Time B: Display a brief video or infographic about air traffic control to enhance comprehension of Katherine Johnson's contributions to air traffic safety during the reading of chapter 13 in Hidden Figures .
Supports guided in part by CA ELD Standards 6.I.B.6, 6.I.B.7, 6.I.B.8, and 6.II.C.6.
Important Points in the Lesson Itself
- To support ELLs, this lesson features another jigsaw. In two groups, students read and gather evidence from a chapter from Hidden Figures about Katherine Johnson. Being responsible for reading and thoroughly comprehending only one chapter, instead of two, helps boost ELLs' confidence. Also, as in Lessons 9-10, students are not expected to share their jigsaw findings with their home groups until the following lesson, giving students adequate time to work in expert groups to discuss the text and complete their Collaborative Argument Evidence note-catchers .
- ELLs may find it challenging to interpret some of the technical vocabulary used in chapter 13 to describe Katherine's work with the Flight Research Division. If helpful, support student comprehension of this chapter with small sketches or graphs that illustrate the chapter's concepts.
- Collaborative Argument Evidence note-catcher (for teacher reference) (from Module 4, Unit 2, Lesson 9, Opening A)
- Gists: Hidden Figures anchor chart (example for teacher reference) (from Module 4, Unit 2, Lesson 1, Work Time A)
- Gists: Hidden Figures anchor chart (one for display; from Module 4, Unit 2, Lesson 1, Work Time A)
- Text Guide: Hidden Figures (for teacher reference) (from Module 4, Unit 2, Lesson 1, Work Time A)
- Independent Reading Sample Plans (for teacher reference) (from the Tools page )
- Work to Become Ethical People anchor chart (one for display; from Module 1, Unit 1, Lesson 1, Work Time A)
- Hidden Figures (Young Readers' Edition) (text; one per student; from Module 4, Unit 1, Lesson 1, Opening A)
- Collaborative Argument Evidence note-catcher (one per student; from Module 4, Unit 2, Lesson 9, Opening A)
- Entrance Ticket: Unit 2, Lesson 12 (example for teacher reference)
- Entrance Ticket: Unit 2, Lesson 12 (one per student)
- Sticky notes (one per student)
- Synopsis: Hidden Figures , Chapters 13 and 16 (one per student)
Each unit in the 6-8 Language Arts Curriculum has two standards-based assessments built in, one mid-unit assessment and one end of unit assessment. The module concludes with a performance task at the end of Unit 3 to synthesize students' understanding of what they accomplished through supported, standards-based writing.
Copyright © 2013-2024 by EL Education, New York, NY.
Get updates about our new K-5 curriculum as new materials and tools debut.
Help us improve our curriculum..
Tell us what’s going well, share your concerns and feedback.
Terms of use . To learn more about EL Education, visit eleducation.org
- Affiliate Program

- UNITED STATES
- 台灣 (TAIWAN)
- TÜRKIYE (TURKEY)
- Academic Editing Services
- - Research Paper
- - Journal Manuscript
- - Dissertation
- - College & University Assignments
- Admissions Editing Services
- - Application Essay
- - Personal Statement
- - Recommendation Letter
- - Cover Letter
- - CV/Resume
- Business Editing Services
- - Business Documents
- - Report & Brochure
- - Website & Blog
- Writer Editing Services
- - Script & Screenplay
- Our Editors
- Client Reviews
- Editing & Proofreading Prices
- Wordvice Points
- Partner Discount
- Plagiarism Checker
- APA Citation Generator
- MLA Citation Generator
- Chicago Citation Generator
- Vancouver Citation Generator
- - APA Style
- - MLA Style
- - Chicago Style
- - Vancouver Style
- Writing & Editing Guide
- Academic Resources
- Admissions Resources
How to Use Tables & Graphs in a Research Paper
It might not seem very relevant to the story and outcome of your study, but how you visually present your experimental or statistical results can play an important role during the review and publication process of your article. A presentation that is in line with the overall logical flow of your story helps you guide the reader effectively from your introduction to your conclusion.
If your results (and the way you organize and present them) don’t follow the story you outlined in the beginning, then you might confuse the reader and they might end up doubting the validity of your research, which can increase the chance of your manuscript being rejected at an early stage. This article illustrates the options you have when organizing and writing your results and will help you make the best choice for presenting your study data in a research paper.
Why does data visualization matter?
Your data and the results of your analysis are the core of your study. Of course, you need to put your findings and what you think your findings mean into words in the text of your article. But you also need to present the same information visually, in the results section of your manuscript, so that the reader can follow and verify that they agree with your observations and conclusions.
The way you visualize your data can either help the reader to comprehend quickly and identify the patterns you describe and the predictions you make, or it can leave them wondering what you are trying to say or whether your claims are supported by evidence. Different types of data therefore need to be presented in different ways, and whatever way you choose needs to be in line with your story.
Another thing to keep in mind is that many journals have specific rules or limitations (e.g., how many tables and graphs you are allowed to include, what kind of data needs to go on what kind of graph) and specific instructions on how to generate and format data tables and graphs (e.g., maximum number of subpanels, length and detail level of tables). In the following, we will go into the main points that you need to consider when organizing your data and writing your result section .
Table of Contents:
Types of data , when to use data tables .
- When to Use Data Graphs
Common Types of Graphs in Research Papers
Journal guidelines: what to consider before submission.
Depending on the aim of your research and the methods and procedures you use, your data can be quantitative or qualitative. Quantitative data, whether objective (e.g., size measurements) or subjective (e.g., rating one’s own happiness on a scale), is what is usually collected in experimental research. Quantitative data are expressed in numbers and analyzed with the most common statistical methods. Qualitative data, on the other hand, can consist of case studies or historical documents, or it can be collected through surveys and interviews. Qualitative data are expressed in words and needs to be categorized and interpreted to yield meaningful outcomes.
Quantitative data example: Height differences between two groups of participants Qualitative data example: Subjective feedback on the food quality in the work cafeteria
Depending on what kind of data you have collected and what story you want to tell with it, you have to find the best way of organizing and visualizing your results.
When you want to show the reader in detail how your independent and dependent variables interact, then a table (with data arranged in columns and rows) is your best choice. In a table, readers can look up exact values, compare those values between pairs or groups of related measurements (e.g., growth rates or outcomes of a medical procedure over several years), look at ranges and intervals, and select specific factors to search for patterns.
Tables are not restrained to a specific type of data or measurement. Since tables really need to be read, they activate the verbal system. This requires focus and some time (depending on how much data you are presenting), but it gives the reader the freedom to explore the data according to their own interest. Depending on your audience, this might be exactly what your readers want. If you explain and discuss all the variables that your table lists in detail in your manuscript text, then you definitely need to give the reader the chance to look at the details for themselves and follow your arguments. If your analysis only consists of simple t-tests to assess differences between two groups, you can report these results in the text (in this case: mean, standard deviation, t-statistic, and p-value), and do not necessarily need to include a table that simply states the same numbers again. If you did extensive analyses but focus on only part of that data (and clearly explain why, so that the reader does not think you forgot to talk about the rest), then a graph that illustrates and emphasizes the specific result or relationship that you consider the main point of your story might be a better choice.
When to Use Data Graphs
Graphs are a visual display of information and show the overall shape of your results rather than the details. If used correctly, a visual representation helps your (or your reader’s) brain to quickly understand large amounts of data and spot patterns, trends, and exceptions or outliers. Graphs also make it easier to illustrate relationships between entire data sets. This is why, when you analyze your results, you usually don’t just look at the numbers and the statistical values of your tests, but also at histograms, box plots, and distribution plots, to quickly get an overview of what is going on in your data.
Line graphs
When you want to illustrate a change over a continuous range or time, a line graph is your best choice. Changes in different groups or samples over the same range or time can be shown by lines of different colors or with different symbols.
Example: Let’s collapse across the different food types and look at the growth of our four fish species over time.
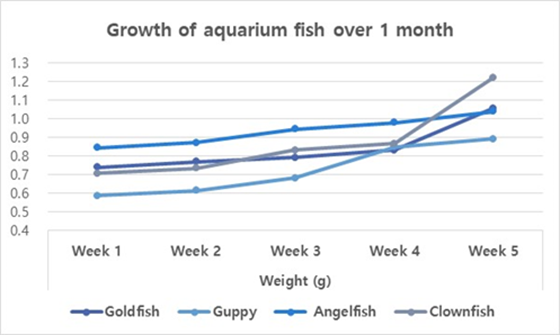
You should use a bar graph when your data is not continuous but divided into categories that are not necessarily connected, such as different samples, methods, or setups. In our example, the different fish types or the different types of food are such non-continuous categories.
Example: Let’s collapse across the food types again and also across time, and only compare the overall weight increase of our four fish types at the end of the feeding period.
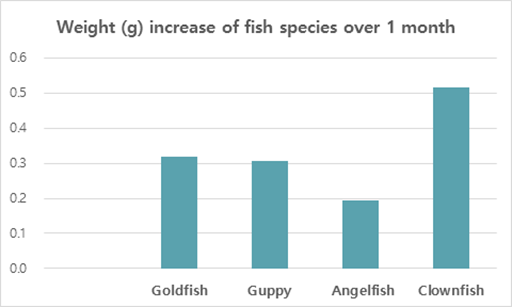
Scatter plots
Scatter plots can be used to illustrate the relationship between two variables — but note that both have to be continuous. The following example displays “fish length” as an additional variable–none of the variables in our table above (fish type, fish food, time) are continuous, and they can therefore not be used for this kind of graph.
As you see, these example graphs all contain less data than the table above, but they lead the reader to exactly the key point of your results or the finding you want to emphasize. If you let your readers search for these observations in a big table full of details that are not necessarily relevant to the claims you want to make, you can create unnecessary confusion. Most journals allow you to provide bigger datasets as supplementary information, and some even require you to upload all your raw data at submission. When you write up your manuscript, however, matching the data presentation to the storyline is more important than throwing everything you have at the reader.
Don’t forget that every graph needs to have clear x and y axis labels , a title that summarizes what is shown above the figure, and a descriptive legend/caption below. Since your caption needs to stand alone and the reader needs to be able to understand it without looking at the text, you need to explain what you measured/tested and spell out all labels and abbreviations you use in any of your graphs once more in the caption (even if you think the reader “should” remember everything by now, make it easy for them and guide them through your results once more). Have a look at this article if you need help on how to write strong and effective figure legends .
Even if you have thought about the data you have, the story you want to tell, and how to guide the reader most effectively through your results, you need to check whether the journal you plan to submit to has specific guidelines and limitations when it comes to tables and graphs. Some journals allow you to submit any tables and graphs initially (as long as tables are editable (for example in Word format, not an image) and graphs of high enough resolution.
Some others, however, have very specific instructions even at the submission stage, and almost all journals will ask you to follow their formatting guidelines once your manuscript is accepted. The closer your figures are already to those guidelines, the faster your article can be published. This PLOS One Figure Preparation Checklist is a good example of how extensive these instructions can be – don’t wait until the last minute to realize that you have to completely reorganize your results because your target journal does not accept tables above a certain length or graphs with more than 4 panels per figure.
Some things you should always pay attention to (and look at already published articles in the same journal if you are unsure or if the author instructions seem confusing) are the following:
- How many tables and graphs are you allowed to include?
- What file formats are you allowed to submit?
- Are there specific rules on resolution/dimension/file size?
- Should your figure files be uploaded separately or placed into the text?
- If figures are uploaded separately, do the files have to be named in a specific way?
- Are there rules on what fonts to use or to avoid and how to label subpanels?
- Are you allowed to use color? If not, make sure your data sets are distinguishable.
If you are dealing with digital image data, then it might also be a good idea to familiarize yourself with the difference between “adjusting” for clarity and visibility and image manipulation, which constitutes scientific misconduct . And to fully prepare your research paper for publication before submitting it, be sure to receive proofreading services , including journal manuscript editing and research paper editing , from Wordvice’s professional academic editors .
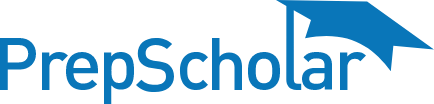
Choose Your Test
Sat / act prep online guides and tips, 10 popular types: free printable graph paper.
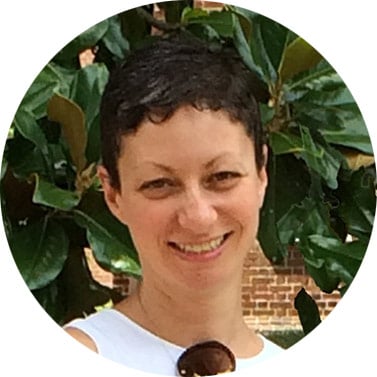
Miscellaneous
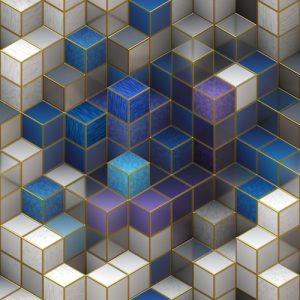
Do you need to print out some graph paper for an assignment, a project, or just for fun? Are you curious about the different types and what they're used for? Then you've come to the right place to browse and print out whatever graph paper you need!
What Is Graph Paper?
In the most basic sense, graph paper is paper that comes pre-printed with faint lines that make up a grid . There are all different ways to accomplish this, but the most common is a grid made of squares composed of parallel horizontal and vertical lines.
You’re probably already familiar with ruled paper—paper that comes pre-printed with horizontal lines that you can use to write on neatly. Graph paper provides assistance in a similar way, but with a grid that anchors your work to two dimensions rather than just one.
You’ll most likely need graph paper for math and math-based work. For example, as a beginning student, you can use it for visual representations of numbers when learning multiplication or addition. Later in school, you’ll find it’s much easier to use grid paper to graph geometric figures on a XY-plane than blank or ruled paper. Finally, of course, any schematic, drawing, or design that needs to be to-scale and precise is easier to do on grid paper.
However, you can also use graph paper for fun. It's perfect for creating complex designs for interior design, quilting, beading, embroidery, and knitting. It's also an excellent tool for games that feature maps or strategic divisions of space: for instance, most role-playing games use graph paper to show where characters are in relation to terrain, each other, and enemies.
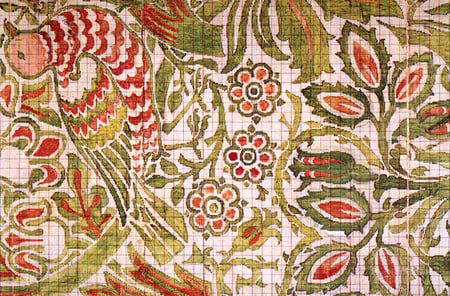
This complex textile design uses graph paper to figure out how to create a repeating pattern.
Download Free Graph Paper
Depending on your project, you’ll need a different kind of grid on your graph paper. I've created 10 printable graph paper PDFs (with alternate JPG versions) to meet your needs.
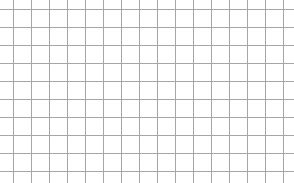
Quad-Ruled Graph Paper
Here are several versions of the most standard kind of graph paper —the kind that is made up of squares.
Use this paper for math projects like graphing lines or functions, for science projects like creating charts of experiment results, or for developing creative symmetrical or repeating designs.
- ⅛ inch squares: JPG Version | PDF Version
- ¼ inch squares (most common size): JPG version | PDF version
- ½ inch squares: JPG version | PDF version
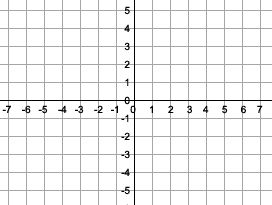
Quad-Ruled Graph Paper with XY-Coordinates
If you’re doing a lot of coordinate geometry, save yourself some time with graph paper that already has an XY-axis on it !
- Full-page coordinate grid: JPG version | PDF version
- Half-page coordinate grids: JPG version | PDF version
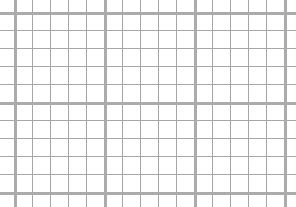
Multi-Line Graph Paper
This graph paper features a standard ¼ inch quad grid overlaid with slightly heavier lines in intervals of 4 or 5 squares .
Use it to learn skip-counting when first discovering numbers, or use it to easily create bar graphs and other kinds of visual data representation
- ¼ inch minor lines, with major lines every 4 squares: JPG version | PDF version
- ¼ inch minor lines, with major lines every 5 squares: JPG version | PDF version
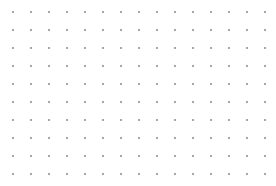
Dot paper is graph paper that only marks the corners of the grid squares and leaves out their sides .
Use it for charts and designs, particularly if you’d like a less visually cluttered result. Because the paper only has dots, any lines you draw won't have to compete with the pre-printed lines that standard quad paper has.
- ¼ inch spaced dots: JPG version | PDF version
- ½ inch spaced dots: JPG version | PDF version
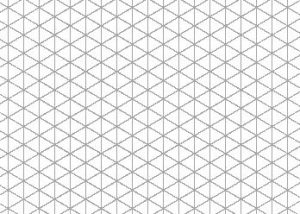
Isometric (Triangle Grid) Paper
In this kind of graph paper, instead of squares, the grid is made up out of equilateral triangles .
Use it to create 3-dimensional drawings for designs like woodworking plans, interior space planning, or graphing on an XYZ-axis. Draw your vertical lines normally, and then draw any horizontal lines using the sides of the triangles. Because the grid creates angled cubes, your drawings are instantly in 3D.
- ¼ inch isometric graph paper: JPG version | PDF version
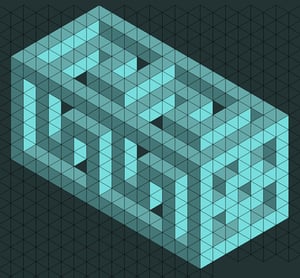
Here's a cool example of what you can do with isometric paper. (Image: tygerbaer2013 via Deviant Art )
Expert Tips for Using Graph Paper in Math
If you're using graph paper to learn math, let me share a few ideas for how to get the most productive use out of this tool.
For Beginner Students
For younger students, you can use graph paper to help with concepts like:
The number line. Drawing a number line on graph paper automatically correctly spaces each segment. You can use the cross-lines to represent units, fives, tens, or hundreds.
Addition and subtraction. Use different color pencils to add or remove squares from a whole. Alternatively, you can use a number line to show that addition and subtraction are ways or moving up or down on it.
Multiplication and division. Use rectangular shapes to demonstrate how 3x8 yields the same result as 4x6 by graphing 3 rows of 8, then graphing 4 rows of six, and then counting the resulting squares.
Fractions. Divide a rectangle into a variety of equal parts with colored pencils to show how many equal parts can make up a whole.
Mapping an environment. Connect our 3D world to 2D space by making a map of the room you’re in, or of your street.
For More Advanced Learning
As you continue in school, graph paper should become one of the many tools in your problem-solving arsenal.
Automatically use graph paper. Remember that you may find it easier to solve problems with graph paper even if they don’t explicitly say to use it. For example, many geometry problems are easier to figure out when you see them represented visually.
Graph paper makes the best scratch paper. Use grid paper as your math scratch paper—it will force you to be neater and more precise when organizing proofs, reducing formulas, and so on.
What's Next?
Learning coordinate geometry and struggling with some of the concepts? Use our guides to the four graph quadrants and completing the square to help fill in some of the gaps.
What graph-related math do you need to know for the SAT/ACT? Find out with our articles about coordinate geometry in the SAT and ACT .
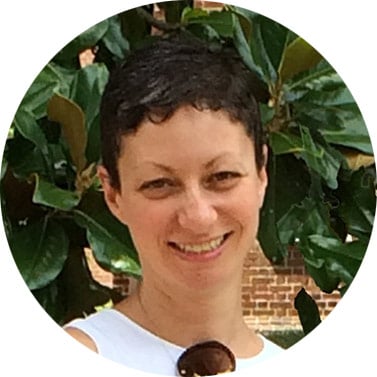
Anna scored in the 99th percentile on her SATs in high school, and went on to major in English at Princeton and to get her doctorate in English Literature at Columbia. She is passionate about improving student access to higher education.
Ask a Question Below
Have any questions about this article or other topics? Ask below and we'll reply!
Improve With Our Famous Guides
- For All Students
The 5 Strategies You Must Be Using to Improve 160+ SAT Points
How to Get a Perfect 1600, by a Perfect Scorer
Series: How to Get 800 on Each SAT Section:
Score 800 on SAT Math
Score 800 on SAT Reading
Score 800 on SAT Writing
Series: How to Get to 600 on Each SAT Section:
Score 600 on SAT Math
Score 600 on SAT Reading
Score 600 on SAT Writing
Free Complete Official SAT Practice Tests
What SAT Target Score Should You Be Aiming For?
15 Strategies to Improve Your SAT Essay
The 5 Strategies You Must Be Using to Improve 4+ ACT Points
How to Get a Perfect 36 ACT, by a Perfect Scorer
Series: How to Get 36 on Each ACT Section:
36 on ACT English
36 on ACT Math
36 on ACT Reading
36 on ACT Science
Series: How to Get to 24 on Each ACT Section:
24 on ACT English
24 on ACT Math
24 on ACT Reading
24 on ACT Science
What ACT target score should you be aiming for?
ACT Vocabulary You Must Know
ACT Writing: 15 Tips to Raise Your Essay Score
How to Get Into Harvard and the Ivy League
How to Get a Perfect 4.0 GPA
How to Write an Amazing College Essay
What Exactly Are Colleges Looking For?
Is the ACT easier than the SAT? A Comprehensive Guide
Should you retake your SAT or ACT?
When should you take the SAT or ACT?
Stay Informed

Get the latest articles and test prep tips!
Looking for Graduate School Test Prep?
Check out our top-rated graduate blogs here:
GRE Online Prep Blog
GMAT Online Prep Blog
TOEFL Online Prep Blog
Holly R. "I am absolutely overjoyed and cannot thank you enough for helping me!”
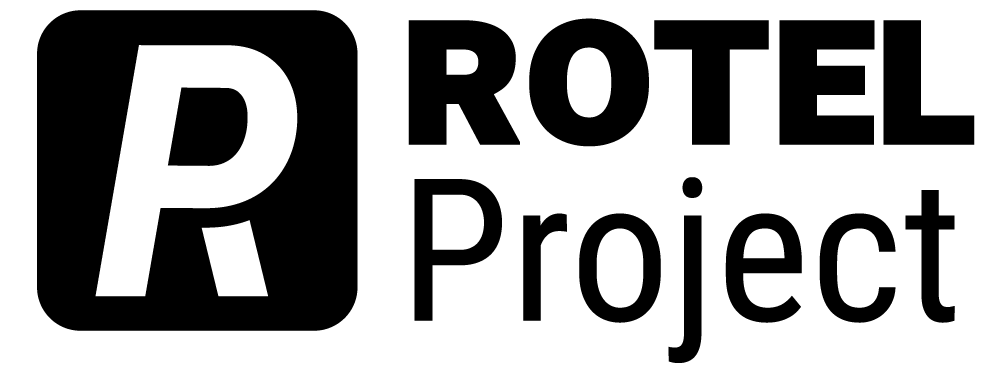
Want to create or adapt books like this? Learn more about how Pressbooks supports open publishing practices.
Examining Sample Assignment 1: Summary and Analysis
In this chapter and in the next three, I walk through example assignments and how you might analyze them to better understand your task.
This assignment comes from one of my first-year writing classes. It’s a fairly typical early assignment in my first-year writing classes, one that asks students to read a text and engage with it in some way. In this case, the readings include the same one I use as a model in the first section of this book , though the actual assignment differs a bit.
The ability to read critically and summarize accurately is a crucial academic skill. The ability to use ideas from one text to guide understanding in another text is similarly crucial. This assignment helps you practice both of these skills.
Your summary will need to explain the key concepts in Mitchell’s article and to explain the main points in the article that you choose to work with. In class, we will work specifically on critical reading strategies to understand how authors make claims and connect those claims to one another. We will also work on techniques for writing strong summaries that accurately represent an author’s work.
Your summaries of these texts should be between 300 and 400 words of your final paper.
In this part of your paper, you will make connections between Mitchell’s concepts and the specific situation described in the article you have chosen. Specifically, you must try to explain the situation in your article using the terms “segregated coexistence” and “living in community” as Mitchell explains them. Think about questions like the following:
- Does the article you have chosen describe a situation that could be considered “segregated coexistence”? If so, what is that situation and how well does it align with “segregated coexistence” as Mitchell describes it?
- Similarly, does the article you have chosen describe a situation that could be considered “living in community”? If so, what is that situation and how well does it align with “living in community” as Mitchell describes it?
- Are there ways in which Mitchell’s terms don’t apply or don’t cover the issue well enough? How so?
Note that this part of your paper should be between 400 and 500 words long, longer than your summaries. While accurately summarizing is important, readers at the college level are more interested in seeing your thinking, so this part should be longer than your summary.
When I comment on your summary and analysis, I will be looking to see how well you have met the goals of the assignment. That is, I will be looking for how accurately and thoroughly you have summarized the articles and how well you have explained and provided support for your analysis. If you only provide summaries of the articles without analysis, your project will not be successful. Instead, your project should demonstrate your critical reading and thinking skills.
Your summary and analysis will also need to meet the standard expectations of good college-level academic writing, which we will be working on during the term. Your purpose and focus will need to be clear and well explained. You will need to provide your reader with sufficient detail in your summary and your response so that your explanations are clear and thorough. You will also need to provide structural cues that enable your reader to follow the logic of your thinking. And your prose will need to be well written both stylistically and grammatically.
Examining the Verbs in Key Sentences
When I read this assignment, I find three key sentences that tell us what we’re supposed to do in this assignment.
Before going on, try to find the key sentences in the Summary and Analysis assignment. Then, read on to see if you agree with my choices.
Let’s look at them.
First Sentence for Examination
To start, there is a sentence summarizing the assignment at the top. Sentences pulled out like this are often important:
Summarize the ideas of “segregated coexistence” and “living in community” from Mitchell’s essay and analyze how those ideas apply to the situation described in an additional article (see sources below).
The verbs here are pretty direct: summarize and analyze.
- What are you summarizing? Mitchell’s ideas
- What are you analyzing? How those ideas apply to the situation in the second article you have chosen
Second Sentence for Examination
There’s another key sentence at the beginning of the “Summaries” section:
Your summary will need to explain the key concepts in Mitchell’s article and to explain the main points in the article that you choose to work with.
The verbs here are less helpful, at least until we look at the words around them.
When someone tells you that you “will need” to do something, you know that they mean that you “must” do it. If we substitute “must” for “will need,” we get a bit more help:
Your summary must explain the key concepts in Mitchell’s article and must explain the main points in the article that you choose to work with.
“Choose” is not terribly important for our purposes because it’s just identifying the second source that we are working with. “Explain,” however, seems to be very important.
Here we get a focus for our summary work:
- Explain the key concepts in Mitchell’s article (which have been identified in the first sentence we analyzed)
- Explain the main points in the article we’ve chosen
In this sentence, we have more detail about what “summarizing” looks like for this assignment.
Third Sentence for Examination
To understand the “analyzing” part of the assignment, we have a couple of sentences at the beginning of the “Analysis” section. I’m including two sentences since the second sentence begins with “specifically,” which indicates that it’s providing more detail about the first:
In this part of your paper, you will make connections between Mitchell’s concepts and the specific situation described in the article you have chosen . Specifically, you must try to explain the situation in your article using the terms “segregated coexistence” and “living in community” as Mitchell explains them.
These verbs require a bit of adjustment before our task will be clear. “Will make” doesn’t tell us much without the following word “connections,” without which we don’t know what we are making. However, “will make connections” can also be understood as simply “connect.” Here’s the sentence with this adjustment (eliminating a few more words to make the sentence grammatically correct:
In this part of your paper, you will connect Mitchell’s concepts and the specific situation described in the article you have chosen . Specifically, you must try to explain the situation in your article using the terms “segregated coexistence” and “living in community” as Mitchell explains them.
Similarly, “must try” doesn’t help us until we look at the words that tell us what we are trying to do. In this case, “must try to explain” is the idea we need to focus on. “Must try” in this sentence is an indication that our professor wants us to make effort, but explaining is really the work here:
In this part of your paper, you will connect Mitchell’s concepts and the specific situation described in the article you have chosen . Specifically, you must explain the situation in your article using the terms “segregated coexistence” and “living in community” as Mitchell explains them.
As with the sentence earlier, “have chosen” just indicates our second article, which is why I skipped that one.
The last “explains” is worth looking at in a bit more detail. In this case, the verb is not about your doing the explaining, but rather the fact that Mitchell has done some. From this sentence, we know that we must use the two identified terms in the same way that Mitchell does.
So, in the analysis part of our paper, we need to do the following:
- Connect Mitchell’s concepts, which we summarized in the summary section of the paper, to the situation in our second article.
- To do this effectively, we need to use Mitchell’s terms.
Applying Bloom
Having done this analysis, we now have a better sense of the intellectual work of this assignment:
- Summary Part 1: Explain Mitchell’s key ideas
- Summary Part 2: Explain the main points in our second article
- Analysis: Use Mitchell’s ideas to explain the situation in our second article.
Before jumping into the next section, take what you know about the task in the sample assignment and see which types of knowledge and which cognitive processes you believe the assignment is looking for.
After you read the rest of this chapter, decide whether or not you agree with my analysis.
Kinds of Cognitive Processes
First, the verbs.
The summary section of the assignment focused on explaining the key ideas in both articles. It can be helpful to move “up” the pyramid or the side of the grid with the cognitive processes to help us figure this out.
We aren’t being asked to remember, since we can look up the information, but we are being asked to understand both Mitchell’s concepts and the main points from the second article. Notice that on the grid version, summarizing appears at the intersection of factual knowledge and the cognitive process of understanding.
When we look at connections, though, “understanding” doesn’t seem to be enough. Yes, we have to understand, but we’re trying to make those connections (remember the original wording?), and “understanding” seems to be more about making sense of ideas that others have already put together.
The next step is “ applying .” If we look only at the grid, applying doesn’t seem to work, but the pyramids explain this one a bit differently. If applying means to “use information in new situations” or “use information in a new (but similar) form,” the term seems to work, right? The assignment asks us to use Mitchell’s terms to explain the situation in the second article. That sounds like an application to me!
But what about “analysis” in the title of the assignment? Look at the explanation of analyzing on the grid: “Break material into constituent parts and determine how parts relate to one another and to an overall structure of purpose.” Similarly, the pyramids describe analyzing as making connections and exploring relationships.
We aren’t doing this kind of work if we look only at Mitchell’s article; there, we are simply explaining what Mitchell means (i.e., summarizing). But when we get to the second article, we have to do more than just apply Mitchell’s terms. We have to divide up the ideas in that article into ideas that are connected to “segregated coexistence” and ideas that are connected to “living in community.”
To do this successfully, we need to explain how these connections work. This means that it’s not enough to identify specific ideas as either one or the other. We also need to make those connections clear to our reader. Those explanations are kinds of analysis .
The verbs in the assignment do not ask us to make arguments or critique ideas, so Bloom’s “evaluate” doesn’t apply in this assignment. Similarly, we aren’t really “creating” something new, beyond the vague idea that what we write should be in our own words for the most part. These two cognitive processes don’t apply much, if at all, here.
To summarize, looking at the verbs and assignment, we seem to be working in the cognitive realms of understanding, applying, and analyzing.
Kinds of Knowledge
While the verbs tell us about the cognitive processes that we are being asked to use, the examination of those key sentences can also help us focus on the information that we will need to complete the task. While much of this was obvious as we explored the verbs, I’ll break it down a bit here to complete the example.
In this case, we will need to know/understand the following:
- Mitchell’s key terms (“segregated coexistence” and “living in community”)
- The main ideas in our second article
- The connections between Mitchell’s concepts and the ideas in our second article
The first two would be factual knowledge, according to Bloom’s Taxonomy. We should be able to go to the article and find those ideas. We aren’t developing those terms or ideas; we are simply recording them. To do that, we have to understand them, but that’s a cognitive process, and we’ll come back to that in a minute.
The connections, however, aren’t factual. Our chosen article doesn’t use Mitchell’s terms directly, so we have to create those connections ourselves. If you look at the descriptions, you’ll see that this type of knowledge is called “ conceptual ,” which specifically is about organizing factual knowledge.
I don’t see anything here that is asking us to work with procedural (how to) knowledge or metacognition (thinking about thinking), so we are just working with the first two types of information.
Putting It Together
In this assignment, we are being asked to use factual and conceptual knowledge to understand, apply, and analyze.
The assignment comes in two parts. The first part is focused on summarizing Mitchell’s two key concepts and the main points from the second article. This part, then, stays firmly in the factual realm. We’re not supposed to talk about our opinions of any of these ideas or start making connections between them in this section. If we fail to present the factual information (e.g., we are missing one summary or the other; or we misread the article so our summary isn’t accurate), we will not succeed at this part. Also, because this is the more basic part of the assignment (lower on the pyramids and grid), if we don’t do this part accurately, odds are good that our analysis part won’t be as successful as we would like.
The second part, what the assignment calls “analysis,” is really a combination of applying and analyzing. We have to understand the main points, too, but mostly, we would do that in the first part of the assignment. In the “analysis,” we need to explain how the ideas in the second article can be categorized using Mitchell’s terms. We’re applying Mitchell, but we also have to explain if our assignment is going to be successful.
At this point, I have beaten this assignment into submission, but I’m hoping you can see the value in taking an assignment apart like this.
Reading and Writing Successfully in College: A Guide for Students Copyright © 2023 by Patricia Lynne is licensed under a Creative Commons Attribution-ShareAlike 4.0 International License , except where otherwise noted.
Share This Book
- U.S. Locations
- UMGC Europe
- Learn Online
- Find Answers
- 855-655-8682
- Current Students
Online Guide to Writing and Research
The research process, explore more of umgc.
- Online Guide to Writing
The Research Assignment Introduction
When tasked with writing a research paper, you are able to “dig in” to a topic, idea, theme, or question in greater detail. In your academic career, you will be assigned several assignments that require you to “research” something and then write about it. Sometimes you can choose a topic and sometimes a topic is assigned to you.
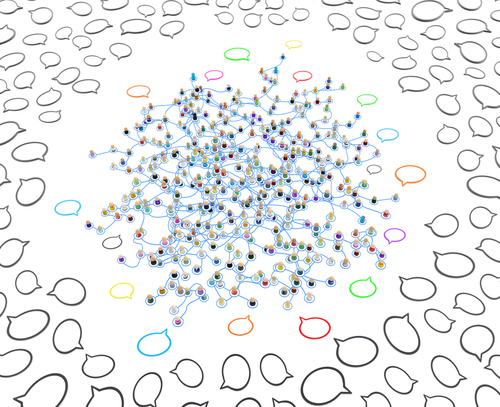
Either way, look at this assignment as an opportunity to learn more about something and to add your voice to the discourse community about said topic. Your professor is assigning you the task to give you a chance to learn more about something and then share that newfound knowledge with the professor and your academic peers. In this way, you contribute meaningfully to the existing scholarship in that subject area. You are then creating a research space for yourself and for other researchers who may follow you.
Mailing Address: 3501 University Blvd. East, Adelphi, MD 20783 This work is licensed under a Creative Commons Attribution-NonCommercial-ShareAlike 4.0 International License . © 2022 UMGC. All links to external sites were verified at the time of publication. UMGC is not responsible for the validity or integrity of information located at external sites.
Table of Contents: Online Guide to Writing
Chapter 1: College Writing
How Does College Writing Differ from Workplace Writing?
What Is College Writing?
Why So Much Emphasis on Writing?
Chapter 2: The Writing Process
Doing Exploratory Research
Getting from Notes to Your Draft
Introduction
Prewriting - Techniques to Get Started - Mining Your Intuition
Prewriting: Targeting Your Audience
Prewriting: Techniques to Get Started
Prewriting: Understanding Your Assignment
Rewriting: Being Your Own Critic
Rewriting: Creating a Revision Strategy
Rewriting: Getting Feedback
Rewriting: The Final Draft
Techniques to Get Started - Outlining
Techniques to Get Started - Using Systematic Techniques
Thesis Statement and Controlling Idea
Writing: Getting from Notes to Your Draft - Freewriting
Writing: Getting from Notes to Your Draft - Summarizing Your Ideas
Writing: Outlining What You Will Write
Chapter 3: Thinking Strategies
A Word About Style, Voice, and Tone
A Word About Style, Voice, and Tone: Style Through Vocabulary and Diction
Critical Strategies and Writing
Critical Strategies and Writing: Analysis
Critical Strategies and Writing: Evaluation
Critical Strategies and Writing: Persuasion
Critical Strategies and Writing: Synthesis
Developing a Paper Using Strategies
Kinds of Assignments You Will Write
Patterns for Presenting Information
Patterns for Presenting Information: Critiques
Patterns for Presenting Information: Discussing Raw Data
Patterns for Presenting Information: General-to-Specific Pattern
Patterns for Presenting Information: Problem-Cause-Solution Pattern
Patterns for Presenting Information: Specific-to-General Pattern
Patterns for Presenting Information: Summaries and Abstracts
Supporting with Research and Examples
Writing Essay Examinations
Writing Essay Examinations: Make Your Answer Relevant and Complete
Writing Essay Examinations: Organize Thinking Before Writing
Writing Essay Examinations: Read and Understand the Question
Chapter 4: The Research Process
Planning and Writing a Research Paper
Planning and Writing a Research Paper: Ask a Research Question
Planning and Writing a Research Paper: Cite Sources
Planning and Writing a Research Paper: Collect Evidence
Planning and Writing a Research Paper: Decide Your Point of View, or Role, for Your Research
Planning and Writing a Research Paper: Draw Conclusions
Planning and Writing a Research Paper: Find a Topic and Get an Overview
Planning and Writing a Research Paper: Manage Your Resources
Planning and Writing a Research Paper: Outline
Planning and Writing a Research Paper: Survey the Literature
Planning and Writing a Research Paper: Work Your Sources into Your Research Writing
Research Resources: Where Are Research Resources Found? - Human Resources
Research Resources: What Are Research Resources?
Research Resources: Where Are Research Resources Found?
Research Resources: Where Are Research Resources Found? - Electronic Resources
Research Resources: Where Are Research Resources Found? - Print Resources
Structuring the Research Paper: Formal Research Structure
Structuring the Research Paper: Informal Research Structure
The Nature of Research
The Research Assignment: How Should Research Sources Be Evaluated?
The Research Assignment: When Is Research Needed?
The Research Assignment: Why Perform Research?
Chapter 5: Academic Integrity
Academic Integrity
Giving Credit to Sources
Giving Credit to Sources: Copyright Laws
Giving Credit to Sources: Documentation
Giving Credit to Sources: Style Guides
Integrating Sources
Practicing Academic Integrity
Practicing Academic Integrity: Keeping Accurate Records
Practicing Academic Integrity: Managing Source Material
Practicing Academic Integrity: Managing Source Material - Paraphrasing Your Source
Practicing Academic Integrity: Managing Source Material - Quoting Your Source
Practicing Academic Integrity: Managing Source Material - Summarizing Your Sources
Types of Documentation
Types of Documentation: Bibliographies and Source Lists
Types of Documentation: Citing World Wide Web Sources
Types of Documentation: In-Text or Parenthetical Citations
Types of Documentation: In-Text or Parenthetical Citations - APA Style
Types of Documentation: In-Text or Parenthetical Citations - CSE/CBE Style
Types of Documentation: In-Text or Parenthetical Citations - Chicago Style
Types of Documentation: In-Text or Parenthetical Citations - MLA Style
Types of Documentation: Note Citations
Chapter 6: Using Library Resources
Finding Library Resources
Chapter 7: Assessing Your Writing
How Is Writing Graded?
How Is Writing Graded?: A General Assessment Tool
The Draft Stage
The Draft Stage: The First Draft
The Draft Stage: The Revision Process and the Final Draft
The Draft Stage: Using Feedback
The Research Stage
Using Assessment to Improve Your Writing
Chapter 8: Other Frequently Assigned Papers
Reviews and Reaction Papers: Article and Book Reviews
Reviews and Reaction Papers: Reaction Papers
Writing Arguments
Writing Arguments: Adapting the Argument Structure
Writing Arguments: Purposes of Argument
Writing Arguments: References to Consult for Writing Arguments
Writing Arguments: Steps to Writing an Argument - Anticipate Active Opposition
Writing Arguments: Steps to Writing an Argument - Determine Your Organization
Writing Arguments: Steps to Writing an Argument - Develop Your Argument
Writing Arguments: Steps to Writing an Argument - Introduce Your Argument
Writing Arguments: Steps to Writing an Argument - State Your Thesis or Proposition
Writing Arguments: Steps to Writing an Argument - Write Your Conclusion
Writing Arguments: Types of Argument
Appendix A: Books to Help Improve Your Writing
Dictionaries
General Style Manuals
Researching on the Internet
Special Style Manuals
Writing Handbooks
Appendix B: Collaborative Writing and Peer Reviewing
Collaborative Writing: Assignments to Accompany the Group Project
Collaborative Writing: Informal Progress Report
Collaborative Writing: Issues to Resolve
Collaborative Writing: Methodology
Collaborative Writing: Peer Evaluation
Collaborative Writing: Tasks of Collaborative Writing Group Members
Collaborative Writing: Writing Plan
General Introduction
Peer Reviewing
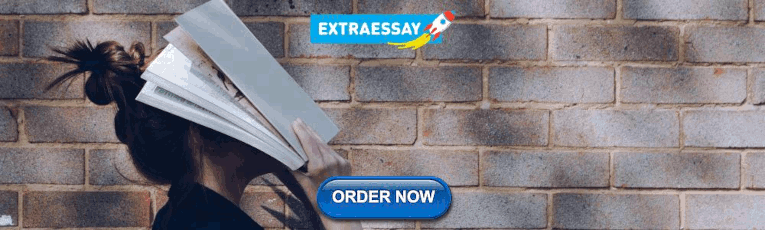
Appendix C: Developing an Improvement Plan
Working with Your Instructor’s Comments and Grades
Appendix D: Writing Plan and Project Schedule
Devising a Writing Project Plan and Schedule
Reviewing Your Plan with Others
By using our website you agree to our use of cookies. Learn more about how we use cookies by reading our Privacy Policy .
- Skip to main content
- Skip to secondary menu
- Skip to primary sidebar
- Skip to footer
Erin Wright Writing
Writing-Related Software Tutorials
How to Create and Customize Charts in Microsoft Word
By Erin Wright

Charts offer a concise and visually appealing way to present numeric information. This tutorial explains the basics of creating and customizing charts in Microsoft Word. We’ll cover five topics:
- How to insert a chart
- How to update existing data in a chart
- How to resize a chart
- How to reposition a chart
- How to change chart colors
These steps apply to all seventeen of Word’s prebuilt chart types:
Important Note: Word provides many ways to customize charts—many more than can reasonably be covered in one tutorial. So, this tutorial presents the basic methods I believe will be most useful for the majority of users.
Before we begin…
What about Figures and Graphs?
In the writing world, charts and graphs fall under the umbrella term figures , which also includes photos, drawings, maps, and musical scores.
Graphs are generally considered a type of chart. Therefore, the term chart is used throughout this tutorial. However, all the steps shown here also apply to visuals typically considered to be graphs, such as line graphs.
This tutorial is also available as a YouTube video showing all the steps in real time.
Watch more than 150 other writing-related software tutorials on my YouTube channel .
The images below are from Word for Microsoft 365. The steps are the same in Word 2021, Word 2019, Word 2016, and Word 2013. However, your interface may look slightly different in those older versions of the software.
How to Insert a Chart
- Place your cursor where you want to insert the chart.
- Select the Insert tab in the ribbon.

- Select the Chart button in the Illustrations group.

- Select a chart type from the left side of the Insert Chart dialog box.

Pro Tip: Hover your pointer over the example image in the center of the Insert Chart dialog box to see a larger example of the chosen chart type.
- Select a subtype of the selected chart.
The available subtypes will depend on the selected chart. Common charts such as pie charts and bar charts offer attractive 3-D options.

- Select the OK button to close the Insert Chart dialog box and insert the chart.

- Enter labels and numbers into the spreadsheet by typing over the example data. Add additional labels and numbers or delete the example data as necessary.

- Select the X to close the spreadsheet.

- (Optional Step) Select the Chart Elements button to the right of the chart if you want to add or remove the title, data labels, or the legend. (Click inside the border to select the chart if the right-side buttons are not visible.)

How to Update Existing Data in a Chart
- Right-click the chart.
- Select Edit Data from the shortcut menu.

Pro Tip: Select the arrow next to Edit Data and select Edit Data in Excel if you want to update your chart in Excel rather than Word’s spreadsheet.
- Edit your data in the spreadsheet (see figure 6).
- Select the X to close the spreadsheet and apply your changes (see figure 7).
How to Resize a Chart
Charts can be resized by dragging the border or by using exact dimensions (e.g., 3” x 4”).
Basic Method: Resize a Chart by Dragging the Border
- Click inside the border to reveal the resizing handles.

- Click and hold one of the handles as you drag the chart to the appropriate size.
- The corner handles provide movement in all directions.
- The side handles provide horizontal movement.
- The top and bottom handles provide vertical movement.
Advanced Method: Resize a Chart to Exact Dimensions
- Click inside the border to select the chart.
- Select the Layout Options button to the right of the chart.

- Select See more from the Layout Options menu.

- Select the Size tab in the Layout dialog box.

- (Optional Step) Select Lock aspect ratio if you want to maintain the current shape.

- Enter the dimensions in the Height and Width boxes. If you selected Lock aspect ratio , you only have to enter one of these numbers.

- Select the OK button to close the Layout dialog box and apply your new dimensions.

How to Reposition a Chart
You can customize your chart’s placement on the page by changing its alignment and text wrapping. Text wrapping determines how charts and other figures are positioned in relation to the surrounding text.
- Select the Home tab in the ribbon.

- Select the Align Left , Center , or Align Right button in the Paragraph group.

- (Optional Step) Select the Layout Options button to the right of the chart for text wrapping options.

Your position changes will be applied immediately.
How to Change Chart Colors
You can choose a prebuilt color palette for your whole chart or select custom colors for individual elements.
See the bonus section below for information about using RGB, HSL, and Hex color codes.
Basic Method: Choose a Prebuilt Color Palette
- Select the Chart Styles button to the right of the chart.

- Select the Color tab in the shortcut menu.

- Select a color palette.

Your new color palette will be applied immediately.
Advanced Method: Choose Custom Colors
- Select and then right-click the individual chart element you want to change.
- Select the Fill button in the shortcut menu.

- Select a color from the drop-down menu or choose More Fill Colors for additional options.

Your new color will be applied immediately.
Bonus Section: How to Use RGB, HSL, and Hex Color Codes in a Chart
Word lets you use RGB (Red, Green, Blue) and HSL (Hue, Saturation, Lightness) color codes in your charts. In addition, you can use Hex color codes if you are using an updated version of Word for Microsoft 365 (formerly Office 365).
- Select the Fill button in the shortcut menu (see figure 23).
- Select More Fill Colors from the drop-down menu.

- Select the Custom tab in the Colors dialog box.

- Select RGB or HSL from the Color model menu or enter a code in the Hex box.

- Enter your RGB or HSL code into the appropriate boxes. (Skip this step if you are using a Hex code.)

- Select the OK button to close the Colors dialog box and apply your color change.

Related Resources
Three Ways to Insert Tables in Microsoft Word
How to Save Tables and Figures as Images in Microsoft Word (PC & Mac)
How to Update Table and Figure Numbers in Microsoft Word
How to Change the Style of Table Titles and Figure Captions in Microsoft Word
How to Create and Update a List of Tables or Figures in Microsoft Word
How to Write Figure Captions for Graphs, Charts, Photos, Drawings, and Maps
How to Write Table Titles
How to Reference Tables and Figures in Text
Updated November 27, 2022
- Microsoft Word Tutorials
- Adobe Acrobat Tutorials
- PowerPoint Tutorials
- Writing Tips
- Editing Tips
- Writing-Related Resources
Free Online Graph Paper
Welcome! Here you will find an assortment of free printable online graph paper. All graph papers a available as free downloadable PDF. They come in all sizes and orientations, from letter to 11x17 - to poster size. Both landscape or portrait.
5mm Graph Paper
This is a standard Cartesian system graphing paper. There are horizontal and vertical lines 5mm apart. Graph paper is often used in engineering, it's common to see engineering graph paper printed on light green paper. View details
1/4" Inch Graph Paper
Also know as Quad paper four boxes make up an inch. Sometimes it's also been referred to quadrille paper. The only difference between this an the other graph paper listed here is the size of the boxes. View details
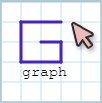
Virtual Online Graph Paper
This virtual graph paper lets you draw lines and write text on it right from your computer. If you make a mistake you can easily undo it. It will remember what you draw unless you erase it so you don't have to worry about losing it. And to top it off it's printable. View details
10 Squares Per Inch Graph Paper
Working with inches? Having 10 squares per inch gives you a nice even number to work with that is both manageable and precise. View details
Dot paper, or dotted paper is like graph paper. Only instead of lines there are dots. It's a good alternative to the more typical graph appear. Having dots instead of lines can be useful for designers. This kind of paper is also used in some games. View details
Centimeter Graph Paper
This is standard graph paper similiar to the graph paper above except of course the lines are 1 centimeter apart instead. View details
1/2" Half Inch Graph Paper
The half inch graph paper can handily function as a two dimensional ruler. View details
1" One-Inch Graph Paper
The larger size graph paper be useful when using the graph paper for measuring. Also when using it with underdeveloped motor skills, for example when working with children. It's also handy when giving presentations where the audience has to be able to see it from far away. View details
Isometric Graph Paper
This graph paper is used to draw three-dimensional figures. It has lines representing all three dimensions: length, width, and height. It can be used for isometric art, architectural designs, and plotting three-dimensional functions. Perfect for drawing sketches and drafting plans. View details
Log Graph Paper
Log is short for logarithmic, this graph paper is used to plot data where the values change exponentially. That is the values go up and down drastically very quickly. This graph paper has sections that compress large ranges of values allowing you to plot large and small numbers while still being able to see everything. View details
Polar Graph Paper
A traditional grid with horizontal and vertical lines is designed to plot Cartesian coordinates where a location is represented with a horizontal and vertical location. Polar coordinates represent another coordinate system where a location is specified by the angle and distance from a fixed point. This graph paper allows you to plot those points. View details
Looking for a particular paper size?
- A4 Graph Paper
- 11x17 Graph Paper
- Legal Graph Paper
- A3 Graph Paper
- A2 Graph Paper
- Poster Graph Paper
- Movie Poster Graph Paper
What is Graph Paper?
Graph paper is paper meant to be written and drawn on. It is made of up fine lines arranged vertically and horizontally as to create many small boxes.
What is Graph Paper used for?
Graph paper is useful when you want to draw things to some kind of scale, instead of measuring each line with a ruler as you draw it you let the graph paper serve as a guide. Graph paper is available in many different measurements, for example each box can be centimeter or an inch in length.
For example anything using the cartesian system can make use of graph paper since the cartesian system is essentially a grid. This makes graph paper ideal for taking notes on math related subjects. For example to plot and study lines, functions, and data.
Other uses of graph paper:
You can use graph paper as a two dimensional ruler. Instead of placing the ruler on the object you can place the object on the paper.
You can also use it to do multi digit math. Having multiple numbers in a small space can make it confusing to determine which numbers should be added, subtracted, multiplied, etc. Graph papers keep those number neat and aligned.
It can also be used as a writing aid, specially for children learning to write. For example you can use a three block row. Capital letters can take up the top two rows. Lowercase letters should take up only the second row. Letters that dip under the line like the lowercase "p", "g", and "y" can take up the bottom two rows. Also a block can help children separate words which is helpful since children often have problems with spacing.
You can fill in blocks in black or color to create graph art.
You can use it to create crossword puzzles.
You can use it to create a maze.
You can use it to play the dot game with dots, this works when the grid lines are very light, that allows you to use a pen or pencil to darken them, also it helps if the grids are large.
Like it? Share it.
If you found this site useful can do me a favor and help others by sharing it? Thanks you.
Clustering source code from automated assessment of programming assignments
- Regular Paper
- Open access
- Published: 15 May 2024
Cite this article
You have full access to this open access article
- José Carlos Paiva 1 , 2 ,
- José Paulo Leal 1 , 2 &
- Álvaro Figueira 1 , 2
Clustering of source code is a technique that can help improve feedback in automated program assessment. Grouping code submissions that contain similar mistakes can, for instance, facilitate the identification of students’ difficulties to provide targeted feedback. Moreover, solutions with similar functionality but possibly different coding styles or progress levels can allow personalized feedback to students stuck at some point based on a more developed source code or even detect potential cases of plagiarism. However, existing clustering approaches for source code are mostly inadequate for automated feedback generation or assessment systems in programming education. They either give too much emphasis to syntactical program features, rely on expensive computations over pairs of programs, or require previously collected data. This paper introduces an online approach and implemented tool—AsanasCluster—to cluster source code submissions to programming assignments. The proposed approach relies on program attributes extracted from semantic graph representations of source code, including control and data flow features. The obtained feature vector values are fed into an incremental k -means model. Such a model aims to determine the closest cluster of solutions, as they enter the system, timely, considering clustering is an intermediate step for feedback generation in automated assessment. We have conducted a twofold evaluation of the tool to assess (1) its runtime performance and (2) its precision in separating different algorithmic strategies. To this end, we have applied our clustering approach on a public dataset of real submissions from undergraduate students to programming assignments, measuring the runtimes for the distinct tasks involved: building a model, identifying the closest cluster to a new observation, and recalculating partitions. As for the precision, we partition two groups of programs collected from GitHub. One group contains implementations of two searching algorithms, while the other has implementations of several sorting algorithms. AsanasCluster matches and, in some cases, improves the state-of-the-art clustering tools in terms of runtime performance and precision in identifying different algorithmic strategies. It does so without requiring the execution of the code. Moreover, it is able to start the clustering process from a dataset with only two submissions and continuously partition the observations as they enter the system.
Similar content being viewed by others
Adaptive Clustering of Codes for Assessment in Introductory Programming Courses
Enhancing Computer Science Education by Automated Analysis of Students’ Code Submissions
Use of Machine Learning Methods in the Assessment of Programming Assignments
Avoid common mistakes on your manuscript.
1 Introduction
Learning to program requires extensive and varied practice, obtained through solving a wide range of programming assignments supported with accurate, timely, and formative feedback [ 2 , 10 , 30 ]. Such feedback cannot be guaranteed manually on learners’ demand, as instructors can neither verify the code attempts for all learners in a class nor are always available outside classes. Thus, scalable and automatic techniques to assess programming assignments have long been investigated to address this need and are still a target of increasing research interest [ 35 ].
The clustering of source code has been initially introduced into the automated assessment of programming assignments for plagiarism detection purposes [ 31 ]. By grouping submissions that exhibit high similarity, the space of possible cases of plagiarism reduces considerably, enabling a more thorough pairwise inspection on them [ 34 , 50 ]. Multiple strategies to measure similarity have been proposed, including structural [ 9 , 20 , 33 , 39 ], semantical [ 4 , 5 , 7 ], and behavioral [ 21 , 28 , 49 ] approaches. Eventually, clustering has emerged as a promising technique to support the generation of feedback on the correctness of solutions and how to progress after mistakes. Having solutions with similar functionality, code complexity, structure, or behavior together can, for instance, facilitate the delivery of targeted feedback on common mistakes from learners by grouping submissions that contain similar errors or misconceptions [ 11 , 18 ] and enable the generation of personalized feedback to improve a program based on a solution adopting a similar strategy but correct [ 6 , 22 ].
Nevertheless, clustering source code is a complex task. On the one hand, approaches often require computing an edit distance between each pair of solutions (e.g., abstract syntax tree edit distance), which is expensive. On the other hand, the quality of the clusters is highly-dependent not only on the selected representation of source code but also on the model used and the available data. Therefore, most of the proposed approaches perform poorly in providing feedback in programming courses. These either: (1) overly focus on syntax and/or require exact matching of program features, generating a large number of clusters as a consequence [ 14 , 17 ]; (2) rely on expensive pairwise computations [ 19 , 32 ]; (3) require a large amount of previously generated data [ 37 , 44 ]; or (4) are specialized in a specific type of assignment (e.g., dynamic programming) and not generalizable [ 22 ].
This work proposes an approach and tool—AsanasCluster—to cluster correct source code submissions to programming assignments based on their algorithmic strategies. To this end, we extract the control flow graph (CFG), which encodes the execution order of individual statements of a program, and data flow graph (DFG), which describes how data variables get updated between instructions, from the submitted source code. The combined information of these representations captures the key aspects of the algorithmic strategy adopted in the original program [ 12 ], ignoring its syntax. As even computing the pairwise graph edit distance of these simplified representations would be expensive, we rather compile a vector of features from them, which is used as the input to the clustering model. This model is an incremental mini-batch k -means variant [ 43 ] of the popular Lloyd’s classic k -means algorithm [ 26 ]. Such a model moves clusters’ centers as new correct submissions enter the system, reducing training time considerably when compared to re-training the model on the complete dataset.
The ultimate goal of this clustering process is to, given an incorrect solution, determine the closest cluster considering all correct submissions up to date. From this cluster, we select a correct solution, which theoretically follows the same algorithmic strategy, to compare against the wrong program and generate personalized feedback for the student. Consequently, the runtime of the clustering process must allow for near real-time feedback, and considering feedback generation is a subsequent task. Moreover, it is important that the model solution adopts the same strategy as the incorrect one if it is a valid approach, to support students’ development in their own line of thought. Hence, we evaluate AsanasCluster on a public dataset—PROGpedia [ 36 ]—both regarding the runtimes and the effectiveness in identifying different algorithmic solution strategies.
The remainder of this paper is organized as follows. Section 2 presents some of the most important works involving the clustering of source code for the automated assessment of programming assignments. Section 3 reviews the necessary concepts for the correct understanding of this work. Section 4 describes the proposed approach. Section 5 demonstrates the effectiveness of this approach using a public dataset of real submissions to programming assignments. Finally, Sect. 6 discusses and summarizes the contributions of this work.
2 Related work
Earlier approaches for clustering source code in programming education are based on textual similarity. These approaches often involve the extraction of tokens or selection of keywords from the source code, followed by pairwise comparison using some well-known distance metric or common text mining techniques [ 24 , 31 , 34 ]. While such approaches can inherit much from text clustering, they are generally very sensitive to changes in code structure or formatting.
A popular program representation used in clustering approaches is abstract syntax trees (ASTs), as they capture just enough information to understand the structure of the code. Such clustering approaches compute similarity using distances in feature space [ 15 , 16 ], string edit distance [ 42 ], tree edit distance [ 19 ], or normalization [ 41 , 47 ]. For instance, Codewebs [ 32 ] customizes and employs a set of semantics-preserving AST transformations to normalize and cluster student submissions.
Luxton-Reilly et al. [ 29 ] claim that different solutions have distinct structural variations, which can be encoded using control flow graphs (CFGs). This means that clustering source codes by their control flow structures divide them into categories. OverCode [ 14 ] and CLARA [ 17 ] combine these structures with dynamic information on variable values to cluster solutions. However, these techniques generate a large number of clusters as they focus excessively on the syntactic details of the source code.
SemCluster [ 37 ] uses a vector representation of programs based on semantic program features, which can be used with standard clustering algorithms such as k -means. The features include control flow features and data flow features. The former describes how the problem space is partitioned into sub-spaces (i.e., the control flow paths), while the latter captures the frequency of occurrence of distinct pairs of successive values of individual variables in test executions.
Using deep learning to learn program embeddings from token sequences, ASTs, CFGs, program states, or other program representations is the recent trend in program clustering [ 27 , 38 , 40 , 44 , 45 ]. Nevertheless, training such models still requires considerable effort and a meticulous selection of inputs. Finally, other clustering approaches specialize in specific programming problems such as dynamic programming [ 22 ] and interactive programs [ 8 ].
3 Definitions
In this section, we present the concepts of control flow graph (CFG), evaluation order graph (EOG), data flow graph (DFG), and k -means clustering that form the basis of the proposed approach.
3.1 Control flow graph
A control flow graph (CFG) is a directed graph \(G = (N, E, n_0, n_f)\) , where N represents the set of nodes, E is the set of directed edges (i.e., pairs of elements of N ), and \(n_0, n_f\) correspond to the entry and exit nodes, respectively. The set of nodes \(N = \{n_1, n_2,...\} \cup \{n_0, n_f\}\) corresponds to basic blocks, i.e., maximal-length sequences of branch-free instructions of a program. The set of edges E represents control dependencies between the nodes. The two extra nodes \(n_0, n_f\) , which represent the node through which the control enters the graph (entry node \(n_0\) ) and the node through which the control exits the graph (exit node \(n_f\) ), are added such that each node of the graph has at most two successors.
The CFG captures the control flow behavior of a program, considering the possible paths and decisions taken during program execution. It provides a structured representation of the control flow of the program, supporting program analysis, optimization, and the understanding of its behavior.
3.2 Evaluation order graph
The evaluation order graph (EOG) [ 46 ] is a directed graph \(G = (N, E)\) , where N represents the set of nodes and E is the set of directed edges, designed to capture the order in which code is executed, similarly to a CFG, but on a finer level of granularity, i.e., including the order in which expressions and sub-expressions are evaluated. The nodes of the EOG are the same nodes as those of the abstract syntax tree of the program, whereas an edge \((n_i, n_j)\) means that \(n_j\) is evaluated after \(n_i\) .
The differences between the EOG and the CFG, which connects basic blocks of statements, are only a few, particularly: methods without explicit return statements have an edge in the EOG to a virtual return node; the EOG considers opening blocks (e.g., { ) as separate nodes; the EOG uses separate nodes for the if keyword and the condition; and the EOG considers a method header as a node.
3.3 Data flow graph
A data flow graph (DFG) is a directed graph \(G = (N, E)\) , where N is the set of nodes and E is the set of directed edges. Each node within the set \(N = \{n_1, n_2,...\}\) denotes a distinct computational unit or instruction, whereas the directed edges \((n_i, n_j)\) for \(n_i, n_j \in N\) within the set E represent the data dependencies, i.e., the output data of \(n_i\) is consumed by \(n_j\) . Such visualization enables a clear view of the data processing pipeline (i.e., the flow of data along the edges establishes the sequence in which operations should be executed), supporting the analysis and optimization of the program through the identification of parallel execution possibilities.
3.4 K -means clustering
K -means clustering method is a popular unsupervised machine learning technique for partitioning a set of observations (or data points) into k different clusters. Firstly, the k initial centroids are randomly selected, where k is a user-defined parameter. Each data point d is then assigned to the closest mean (or centroid), and the collection of points assigned to a centroid forms a cluster. Afterward, the centroid of each cluster is updated based on all points in the cluster. This iterative procedure is repeated until no changes occur in the clusters.
The method can be formally defined as follows. Consider \(D = \{ d_1,..., d_n \}\) is the set of observations to be clustered, where each \(d_i \in \mathbb {R}^m\) is represented by a m -dimensional feature vector. Then, k -means partitions the data points in D into K clusters with centroids \(C^{*} = \{C_1,...,C_k\}\) such that \(\sum _{i=1}^K\sum _{d \in C_i} dist(d, \mu _i)\) is minimal, where \(\mu _i = \frac{1}{C_i}\sum _{d \in C_i} d\) is the centroid of cluster \(C_i\) and “dist” is the distance function used. There are many distance metrics that can be used, such as the squared Euclidean distance, i.e., \(dist(d, \mu )=||d-\mu ||^2\) , and the cosine distance, i.e., \(dist(d, \mu )=(d \cdot \mu ) / (||d||. ||\mu ||)\) . The best one depends on the dataset composition.
Even though this problem is known to be NP-hard, such gradient descent methods generally converge to a local optimum if seeded with an initial set of k observations drawn uniformly and randomly from D [ 3 ]. Bottou et al. [ 3 ] used this property to propose an online stochastic gradient descent variant that computes a gradient descent step on one observation at a time, which makes it converge faster on large datasets but degrading the quality of clusters (due to stochastic noise). Sculley [ 43 ] proposes an optimization for k -means clustering by processing mini-batches rather than individual data points, which tend to have lower stochastic noise and are not affected in terms of cost when datasets grow large with redundant observations.
4 Clustering source code with AsanasCluster
This section introduces the design and the implementation of a tool, named AsanasCluster, to cluster correct source code solutions submitted to programming assignments in real time. This approach addresses a few gaps in existing techniques. First, it groups programs by their algorithmic strategy from a high-level perspective, which generates fewer clusters than most existing clustering approaches. Second, it extracts and relies on a vector of features from the semantic graph representations of the program, avoiding expensive pairwise computations such as the graph edit distance across the complete dataset. Lastly, it follows an incremental clustering model, meaning that solutions are assigned to clusters as they enter the system rather than all at once. Such a model not only reduces the time to discover the closest cluster to a new observation considerably but also enables this task to run with up-to-date information on submitted solutions.
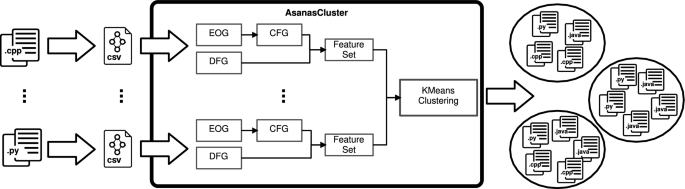
Scheme of how AsanasCluster works on a high level
The workflow of AsanasCluster is illustrated in Fig. 1 . Given a set of existing solutions P to a programming assignment, for each new program p , received as input, it generates both an EOG and a DFG using an adaptation of an existing Kotlin library [ 13 ], designed to extract the code property graph (which includes the representations needed) out of source code. This step guarantees support for programs written in either Python, Java, C, or C++. The obtained EOG is transformed into a CFG through a process involving edge contraction, i.e., for every edge whose source has an out-degree of one and destination has an in-degree of one is contracted. These two final representations, DFG and CFG, are analyzed to compute the control flow and data flow features that compose the feature vector of a program, (described in Subsection 4.1 ). Finally, the resulting feature vector is fed into the k -means clustering algorithm implemented (refer to Subsection 4.1 for details).
4.1 Feature engineering
One key characteristic of the proposed approach lies in the representation of the program used. The clustering process aims to separate source code solutions by their algorithmic strategy, i.e., a sequence of instructions executed in a well-defined order to solve a problem or calculate a function. The flow of execution of a program, i.e., the order in which the instructions execute, is, thus, an essential aspect of the algorithmic strategy. Combining this with knowledge of the data dependencies among these instructions, the algorithmic strategy is largely covered [ 12 ]. The former information is captured by the CFG (or the EOG), whereas the latter is encoded in the DFG, as explained in Subsections 3.1 and 3.3 .
To obtain these representations, we firstly adapted a Kotlin library [ 13 ], initially developed to extract the code property graph (CPG) [ 48 ] out of source code written in either Python, Java, C, or C++. The CPG is a data structure combining the AST, DFG, and EOG, designed to mine large codebases for programming patterns that represent security vulnerabilities. As this representation includes the required information, our adaptation consists of adding a feature to the library for exporting the CPG in comma-separated value (CSV) format. The exported artifact is composed of two CSV files: one containing the description of the nodes, including ID, type of construct, token, and location, and the other describing the edges, including source, location, origin (AST, EOG, or DFG), among other information of its specific origin (e.g., variable identifier for edges of the DFG). While both the EOG and the CFG encode the control flow of a program, the latter is a significantly smaller graph. Hence, before further computations, the obtained EOG is transformed into a CFG through a process involving edge contraction, i.e., every edge whose source has an out-degree of one and destination has an in-degree of one is contracted.
Clustering by the CFG and the DFG would require measuring two pairwise graph edit distances over the full dataset. These are complex operations whose computational cost grows exponentially on the graphs and dataset size. Therefore, our approach is rather feature-based. We derive a feature vector composed of numeric values calculated from the characteristics of both graphs, CFG and DFG. This vector contains 11 features, namely: connected_components , the number of connected components in the control flow graph (i.e., being an intra-procedural representation, the multiple procedures have no connection in the graph); loop_statements , the number of loop statements (e.g., for , foreach , while , and do... while ) in the program; conditional_statements , the number of conditional statements (e.g., if ) in the program; cycles , the number of different cycles in the control flow graph; paths , the number of different paths in the control flow graph; cyclomatic_complexity , a software metric that measures the complexity of a program by analyzing its control flow (i.e., it provides a quantitative measure of the number of possible execution paths in the program); variable_count , the number of variables used in the program, excluding variables which are never read; total_reads , the total number of read operations on variables; total_writes , the total number of write operations on variables; max_reads , the maximum number of read operations on single variable; and max_writes , the maximum number of write operations on single variable. Table 1 summarizes the features of the model.
As the order of execution of instructions has the most relevance in the algorithmic strategy of the solution, we decided to split the weight of the data flow features. Among these, the variable_count weighs more, as the others are dependent on it by definition. The summed weight of all these features is the same as that of a single control flow feature. Moreover, we have scaled the data so that it has zero mean and unit variance. For that, running means and variances are maintained for each feature. Even though, for being incremental, the exact means and variances are not known in advance, this does not have a detrimental impact in the long term.
Having a high number of features in the model makes it more difficult to manage and may even add noise, as some of these features can be redundant. To prevent this, the correlation of the 11 features of our model has been measured using Pearson’s correlation coefficient [ 23 ] on the 16 programming exercises of PROGpedia dataset [ 36 ]. The correlation coefficient has values from \(-1\) to 1: A value closer to 0 implies weaker correlation (i.e., 0 is no correlation); a value closer to 1 means stronger positive correlation; and a value closer to \(-1\) implies stronger negative correlation. Each programming exercise is analyzed separately and casts a vote on pairs with a correlation above 0.9. For pairs with half or more of the total votes, a member is eliminated. Nevertheless, in this case, no correlated pair has been identified with these conditions.
4.2 Clustering model
The values of the final feature vector are given as input to the k -means clustering algorithm implemented (see Subsection 3.4 ). This specific implementation starts by randomly instantiating k centroids, according to a Gaussian distribution. The value of k is the main hyper-parameter of the model and sets the limit on the number of formed clusters. As the goal is to have as many clusters as the number of algorithmic solution strategies, an adequate value would be greater or equal to the expected count of different strategies. We have limited the maximum amount of clusters to 16 as the possibility of an academic-level programming assignment having more than 16 algorithmic solution strategies can be neglected. Nevertheless, this value can be defined explicitly, per assignment.
Given a new submission, more precisely the feature vector extracted from it, we first identify the closest centroid. This is done by measuring the distance from the new observation to each centroid, using a certain distance metric, and selecting the minimum of these distances. In this case, we tried the Manhattan distance, Euclidean distance, and cosine distance in two sets of submissions to programming assignments with well-defined algorithmic solution strategies. The Euclidean distance revealed a lower average error index (0) than the Manhattan (0.3) and cosine (0.25) distances and, thus, was applied. After identifying the centroid (and cluster) to which the new observation belongs, the centroid’s position “moves” in the direction of the new element. The amount by which to move the centroid is a product of their scalar distance and the learning rate. The learning rate is the inverse of the number of solutions assigned to a cluster during the process, i.e., as the number of elements increases, the effect of new elements is reduced.
The pseudocode of this clustering process is presented in Algorithm 1. It assumes that the feature vector is provided as the solution object, ignoring the extraction of the graph representations and subsequent computation of the feature vector values. Moreover, when centroids “move,” the closest centroid is re-identified for previous solutions.
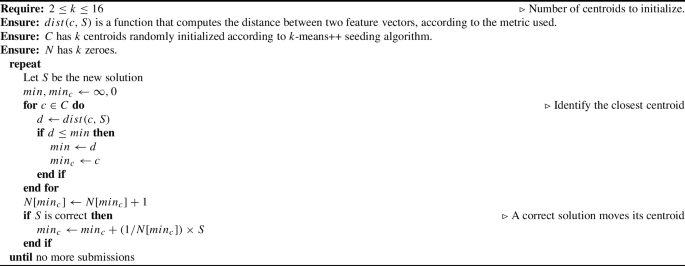
Pseudocode of the k -means clustering process
4.3 Mooshak integration
AsanasCluster aims to integrate into automated assessment engines, consuming their submissions’ data both offline (i.e., previously submitted solutions) and in real time (i.e., new submissions entering the system). To this end, AsanasCluster has two modes. One builds a clustering model from all existing submissions to a specified programming assignment. The other loads a clustering model saved into the disk and identifies the closest cluster to the given submission, including it in the model if it is an accepted solution.
Mooshak [ 25 ] is one of the existing systems providing automated assessment capabilities and the one selected for the development and testing of AsanasCluster. Mooshak uses the file system as the object database, storing and retrieving data in Tcl-code files organized in directories. Therefore, the submissions’ metadata is stored alongside the source code and extracted CSV files of the CPG in the submission folder.
For building a clustering model, AsanasCluster simply iterates the submissions’ directory and, for each submission folder, loads the CPG and processes it into the model (if accepted). When a new submission enters the system, AsanasCluster acts as the last evaluator of Mooshak, adding the submission into the model and echoing the classification of the previous evaluator. If the submission has a rejection classification, the identification of the closest cluster is also printed, and model updates get discarded.
5 Evaluation
This section presents the results of the evaluation of the accuracy and time adequacy of AsanasCluster for automated assessment of programming assignments. To this end, we have evaluated the performance of clustering on a public collection—PROGpedia [ 36 ]—of source code submitted to 16 programming assignments on Mooshak [ 25 ] in undergraduate Computer Science courses within multiple years of the 2003–2020 time span. The dataset comprises a total of 9117 submissions. As we intend to use the clustering output as input to a program repair tool, we separate the submissions not only by programming exercise but also by programming language. Only solutions written in C/C++ (C17), Java (Java 8), and Python (version 3) were considered (Note: version within parentheses means “compatible with” not an exact match). All tests run on a Dell XPS 15 9570.
5.1 Runtime
Our goal is to use AsanasCluster as an intermediate step in the automated assessment of programming assignments. While no time limit for a single evaluation is formally defined in the literature of automated assessment of programming assignments, one minute is a reasonable limit for a task that is meant to be nearly real time [ 1 ]. To evaluate the scalability of AsanasCluster, we measure the amount of time required to (1) build a clustering model with past submissions from scratch, (2) discover a new correct solution, and (3) determine the cluster of a new submitted solution. As for (1), we have built models for the set of correct solutions from PROGpedia [ 36 ], separating data by programming exercise and language. Table 2 summarizes the composition of the dataset regarding submissions, including the number of submissions and the average lines of code for each pair assignment/programming language. For (2), a new correct solution has been developed. Finally, in (3) we select randomly a wrong attempt.
Building a clustering model on a set of submissions (1) requires four steps. First, search and select the adequate solutions (i.e., accepted solutions written in the programming language of the model) from the directory containing all submissions to an assignment. Second, generate the needed semantic graph representations, i.e., the DFG and the CFG. Third, compute the feature vector from the representations. Finally, build the k -means model, processing existing observations. Table 3 presents the size of the solutions’ sets, building times, and the number of generated clusters for each pair (programming assignment, programming language).
The maximum model’s building time is 9 min and 30 s for the 152 Java submissions to programming assignment 53 , which demands the implementation of a graph searching algorithm. As expected, the amount of submissions has the greatest impact on training performance when compared to the programming language or complexity of the programming assignment. However, the complexity of the solutions also affects the runtime negatively. For instance, processing the 205 submissions to programming assignment 34 , which requires sorting a vector of numbers, takes less 9 s than the 152 to assignment 53 .
The number of generated clusters has no noticeable correlation with either the number of submissions or the programming language. The median number of clusters for the models built is 4. The set of solutions written in C for exercise 42 has 9 clusters, which is the highest amount of clusters identified for the evaluated sets.
Table 4 depicts the time needed to (2) process a new solution into the model and (3) identify the best cluster for a new submitted solution, using the built models. On average, it takes 5,397 s to learn (2) and 4,910 s to predict the cluster of a new observation (3). There is no task running for 7 or more seconds. (6,981 is the worst case.)
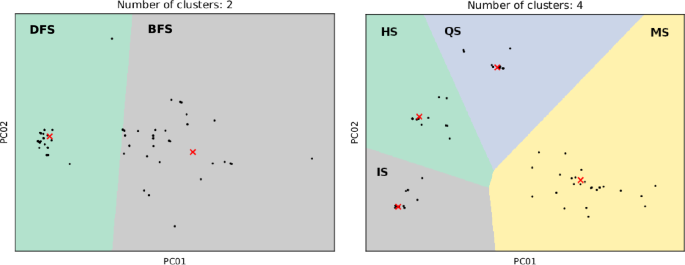
2-Component PCA visualization of the clustering of implementations of graph searching (left) and sorting algorithms (right)
5.2 Error index
This analysis aims to validate the effectiveness of AsanasCluster in separating the different algorithmic strategies implemented in solutions. To this end, we consider a simple metric ( 1 ), which we named Error Index. The Error Index takes values between 0 and 1, where 0 indicates that all solutions were correctly grouped. A solution is considered wrongly grouped if it is in the cluster of a different strategy (i.e., a cluster belongs to the algorithmic strategy with most solutions in it). This metric purposely ignores the case where solutions adopting the same algorithmic strategy spread across different clusters. The reason is that we understand those solutions can still be quite distinct.
To evaluate this, we conduct two separate tasks. The first task consists of clustering a set of 100 different implementations of two graph searching algorithms, 50 depth-first search, and 50 breadth-first search. These programs were collected during an Algorithm Design and Analysis class. In the second task, we cluster a collection of 100 programs from GitHub implementing sorting algorithms, namely heap, merge, insertion, and quick sort. There are 25 samples of each sorting algorithm.
Figure 2 illustrates the 2-component principal component analysis (PCA) visualization of the resulting clusters for both tasks. (PC1 and PC2 explain 83% and 6% of the variability, respectively, in the left chart, and 60% and 24% in the right chart.) The red crosses indicate clusters’ centroids. On the left chart, there are four clusters. The green cluster contains the 50 points corresponding to the depth-first search implementations. The breadth-first search implementations are assigned to the gray cluster. On the right, four clusters match the different sorting algorithms: insertion sort (gray), heap sort (green), quick sort (blue), and merge sort (yellow). Note that some points are not visible as they share the same (or very close) values of the feature vector. In both experiments, there is one cluster corresponding to each of the different included algorithmic strategies, and solutions are split evenly by these clusters. Furthermore, the Error Index of both tasks evaluates to 0, as there is no cluster with solutions of different algorithmic strategies.
Extending the collection of the second task with one implementation of the radix sort algorithm also does not affect the Error Index. As depicted in the 2-component PCA visualization of Fig. 3 (PC1 and PC2 explain 61% and 23% of the variability, respectively), a new cluster (blue) is created with this new solution, whereas the existing clusters are not changed. However, adding a few implementations of the selection sort algorithm increases the Error Index. As its implementation is semantically similar to insertion sort, they are both assigned to the same cluster until there are enough samples to form a new cluster. For instance, including 3 implementations results in an Error Index of 0.03 (3 incorrectly grouped solutions out of 103).
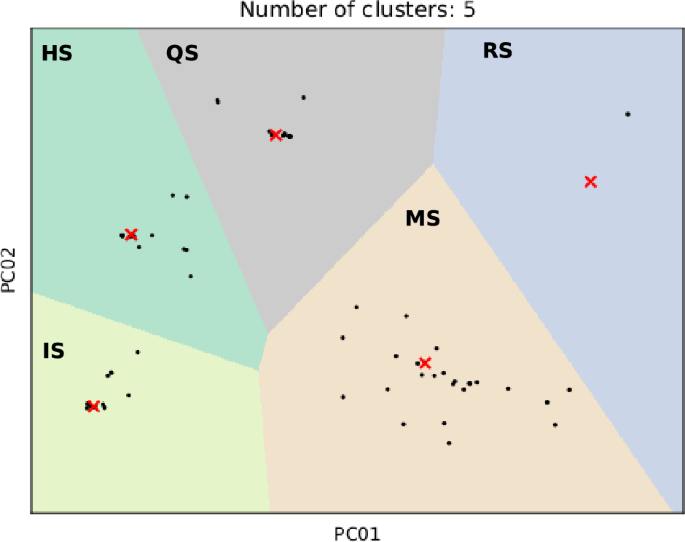
2-Component PCA visualization of the clustering of implementations of sorting algorithms, adding a single radix sort implementation
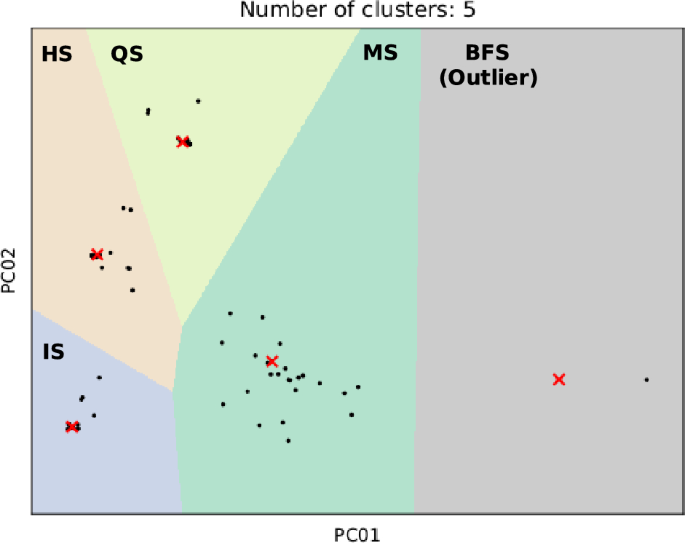
2-Component PCA visualization of the clustering of implementations of sorting algorithms, adding a breadth-first search implementation
To demonstrate how AsanasCluster handles unrelated solutions submitted intentionally or accidentally, we have added a breadth-first search implementation to the clustering model of the sorting algorithms. The result is depicted in the 2-component PCA visualization of Fig. 4 . (PC1 and PC2 explain 63% and 21% of the variability, respectively.) The unrelated solution is isolated in a new cluster (gray area), while other clusters remain unaffected. Therefore, the Error Index is 0.
5.3 Discussion
There are a few tools presented in the literature that introduce clustering approaches comparable to the one described in this paper, namely SemCluster [ 37 ], OverCode [ 14 ], and CLARA [ 17 ]. The evaluation of SemCluster includes a comparison with the latter tools (OverCode and CLARA). Even though calculating the similarity between two implementations with small sizes, such as those referred in Table 2 , can be performed in a short period of time, clustering a new solution requires pairwise comparison between the new solution and each of the existing. The evaluation of SemCluster demonstrates this has a tremendous impact in terms of runtime of the approaches (e.g., CLARA tool can take more than 100 min for programs with less than 100 lines of code).
SemCluster reveals a better runtime performance and precision in identifying different algorithmic solution strategies than the existing tools [ 37 ]. Nevertheless, none of the proposed approaches is incremental, i.e., they require rebuilding the clustering model on every new submission. This takes much more than a minute in any of the analyzed tools, even for small size programs with less than 50 lines of code. Assuming that representations of source code are stored between model training sessions, SemCluster still has a median runtime of 18 s for the average time needed to recalculate models (i.e., when adding an element), possibly reaching 30 s.
Due to the unavailability of dataset used in [ 37 ], the evaluation of the runtime performance described in this paper applies our tool on identical tasks but using a different, publicly available, dataset [ 36 ]. This dataset contains 16 assignments of various complexities, delivered at multiple stages of undergraduate CS courses, using several distinct algorithms with implementations written in C, C++, Java, and Python. The composition of the dataset is fairly similar to the dataset used in [ 37 ], as shown in Table 2 . In these conditions, our tool has median runtimes of 4 (Python) and 5 (C, C++, and Java) seconds to identify the cluster of a new solution and integrate it into the model. In the worst case, it can take up to 7 s.
Regarding the precision in identifying the different algorithmic solution strategies, SemCluster has proven its effectiveness in two tasks. Firstly, it successfully separates 100 solutions to an assignment involving sorting algorithms by 4 clusters, according to the adopted algorithm: bubble sort, quicksort, and none specifically (two clusters). Lastly, it can perfectly partition 100 programs into 2 clusters depending on the searching algorithm applied, i.e., depth-first and breadth-first search. Similarly, the experiment conducted to evaluate AsanasCluster achieved optimal results in the two tasks, as described in Subsection 5.2 .
Therefore, considering its inclusion in the process of automated assessment of programming assignments, AsanasCluster can achieve better performance than the most similar tools presented in the literature. Nevertheless, the main benefits are (1) not requiring the execution of the code to extract the feature vector and (2) being able to start the clustering process from a dataset with only two submissions and recalculate clusters.
5.4 Threats to validity
Only a direct comparison with SemCluster [ 37 ] (i.e., using the same dataset) would allow us to demonstrate an improvement of the state of the art in terms of runtime and precision in separating algorithmic strategies. Unfortunately, neither the dataset nor the tool is publicly available and was not also made available upon request to the authors. We have, however, tried to select a similarly complex dataset with a few even larger solutions on average, considering the lines of code.
Furthermore, we have evaluated our approach to small-to-medium size programs typically found in introductory programming problems. While this is in line with related work, we aim to validate the extension of our approach to larger programs, as found in more advanced courses, in future work.
6 Conclusion
This paper presents a novel online approach to clustering source code for supporting the automatic assessment of programming assignments based on quantitative program features extracted from the programs’ semantic graph representations, namely the CFG and the DFG. This approach aims to (1) generate a number of clusters close to the number of different algorithmic solution strategies, (2) avoid expensive pairwise computations, and (3) learn incrementally, i.e., every solution processed becomes part of the model’s “knowledge” for subsequent observations.
Even though the evaluation presents some building times close to 10 min (see Table 3 ), building a model from scratch is a step performed only once (when loading the programming assignment) in online clustering approaches, with no effect on a submission’s assessment time. In fact, the assessment of a submission involves recalculating centroids including the new observation and/or determining the closest cluster, which takes under 7 s in all trials performed (see Table 4 ). Such a delay (below one minute) is acceptable for automated assessment of programming assignments. Furthermore, the experiments conducted to measure the precision of clustering reveal great accuracy in separating different algorithmic strategies.
Our goal is to integrate AsanasCluster as the first step of our workflow to repair incorrect student attempts. For a given programming assignment, we rely on AsanasCluster to cluster the correct student solutions. Given an incorrect student program, we identify the cluster of solutions most similar to the submitted program and compare it against one of the solutions in the selected cluster, generating the most pertaining modifications that get the student to the correct solution. Furthermore, the tool has the potential to be applied in many other automated reasoning tasks in programming education and beyond (e.g., learning analytics, similarity detection, and fault localization).
Availability of data and materials
The datasets generated and/or analyzed during the current study are available in the PROGpedia repository, https://doi.org/10.5281/zenodo.7449056 .
Ala-Mutka, K.M.: A survey of automated assessment approaches for programming assignments. Comput. Sci. Educ. 15 (2), 83–102 (2005). https://doi.org/10.1080/08993400500150747
Article Google Scholar
Bennedsen, J., Caspersen, M.E.: Failure rates in introductory programming. SIGCSE Bull. 39 (2), 32–36 (2007). https://doi.org/10.1145/1272848.1272879
Bottou, L., Bengio, Y.: Convergence properties of the k-means algorithms. In: Proceedings of the 7th International Conference on Neural Information Processing Systems, pp. 585–592. MIT Press, Cambridge, MA, USA, NIPS’94 (1994)
Chae, D.K., Ha, J., Kim, S.W., et al.: Software plagiarism detection: a graph-based approach. In: Proceedings of the 22nd ACM International Conference on Information & Knowledge Management, pp. 1577–1580. Association for Computing Machinery, New York, NY, USA, CIKM ’13 (2013). https://doi.org/10.1145/2505515.2507848
Chen, R., Hong, L., Lu, C., et al.: Author identification of software source code with program dependence graphs. In: Proceedings of the 2010 IEEE 34th Annual Computer Software and Applications Conference Workshops, pp. 281–286. IEEE Computer Society, USA, COMPSACW ’10 (2010). https://doi.org/10.1109/COMPSACW.2010.56
Chow, S., Yacef, K., Koprinska, I., et al.: Automated data-driven hints for computer programming students. In: Adjunct Publication of the 25th Conference on User Modeling, Adaptation and Personalization, pp. 5–10. Association for Computing Machinery, New York, NY, USA, UMAP ’17 (2017). https://doi.org/10.1145/3099023.3099065
Cosma, G., Joy, M.: An approach to source-code plagiarism detection and investigation using latent semantic analysis. IEEE Trans. Comput. 61 (3), 379–394 (2012). https://doi.org/10.1109/TC.2011.223
Article MathSciNet Google Scholar
Drummond, A., Lu, Y., Chaudhuri, S., et al.: Learning to grade student programs in a massive open online course. In: Proceedings of the 2014 IEEE International Conference on Data Mining, pp. 785–790. IEEE Computer Society, USA, ICDM ’14 (2014). https://doi.org/10.1109/ICDM.2014.142
Durić, Z., Gašević, D.: A source code similarity system for plagiarism detection. Comput. J. 56 (1), 70–86 (2012). https://doi.org/10.1093/comjnl/bxs018
Elmaleh, J., Shankararaman, V.: Improving student learning in an introductory programming course using flipped classroom and competency framework. In: 2017 IEEE Global Engineering Education Conference (EDUCON), pp. 49–55. IEEE, Athens, Greece (2017). https://doi.org/10.1109/EDUCON.2017.7942823
Emerson, A., Smith, A., Rodriguez, F.J., et al.: Cluster-based analysis of novice coding misconceptions in block-based programming. In: Proceedings of the 51st ACM Technical Symposium on Computer Science Education. Association for Computing Machinery, New York, NY, USA, SIGCSE ’20, pp. 825–831 (2020). https://doi.org/10.1145/3328778.3366924
Feautrier, P.: Dataflow analysis of array and scalar references. Int. J. Parallel Prog. 20 (1), 23–53 (1991). https://doi.org/10.1007/BF01407931
Fraunhofer AISEC: Code Property Graph (2023). https://github.com/Fraunhofer-AISEC/cpg . Accessed 20 May 2023
Glassman, E.L., Scott, J., Singh, R., et al.: Overcode: visualizing variation in student solutions to programming problems at scale. ACM Trans. Comput. Hum. Interact. 22 (2), 25 (2015). https://doi.org/10.1145/2699751
Gross, S., Zhu, X., Hammer, B., et al.: Cluster based feedback provision strategies in intelligent tutoring systems. In: Cerri, S.A., Clancey, W.J., Papadourakis, G., et al. (eds.) Intelligent Tutoring Systems, pp. 699–700. Springer, Berlin (2012). https://doi.org/10.1007/978-3-642-30950-2_127
Chapter Google Scholar
Gross, S., Mokbel, B., Hammer, B., et al.: Towards providing feedback to students in absence of formalized domain models. In: Lane, H.C., Yacef, K., Mostow, J., et al. (eds.) Artificial Intelligence in Education, pp. 644–648. Springer, Berlin (2013). https://doi.org/10.1007/978-3-642-39112-5_79
Gulwani, S., Radiček, I., Zuleger, F.: Automated clustering and program repair for introductory programming assignments. In: Proceedings of the 39th ACM SIGPLAN Conference on Programming Language Design and Implementation, pp. 465–480. Association for Computing Machinery, New York, NY, USA, PLDI 2018 (2018). https://doi.org/10.1145/3192366.3192387
Head, A., Glassman, E., Soares, G., et al.: Writing reusable code feedback at scale with mixed-initiative program synthesis. In: Proceedings of the Fourth (2017) ACM Conference on Learning @ Scale, pp. 89–98. Association for Computing Machinery, New York, NY, USA, L@S ’17 (2017). https://doi.org/10.1145/3051457.3051467
Huang, J., Piech, C., Nguyen, A., et al.: Syntactic and functional variability of a million code submissions in a machine learning MOOC. In: Walker, E., Looi, C. (eds.) Proceedings of the Workshops at the 16th International Conference on Artificial Intelligence in Education AIED 2013, CEUR Workshop Proceedings, vol 1009. CEUR-WS.org, Memphis, TN, USA, pp. 25–32 (2013). https://ceur-ws.org/Vol-1009/0105.pdf
Inoue, U., Wada, S.: Detecting plagiarisms in elementary programming courses. In: 2012 9th International Conference on Fuzzy Systems and Knowledge Discovery. IEEE, Chongqing, China, pp. 2308–2312 (2012). https://doi.org/10.1109/FSKD.2012.6234186
Jhi, Y.C., Wang, X., Jia, X., et al.: Value-based program characterization and its application to software plagiarism detection. In: Proceedings of the 33rd International Conference on Software Engineering. Association for Computing Machinery, New York, NY, USA, ICSE ’11, pp. 756–765 (2011). https://doi.org/10.1145/1985793.1985899
Kaleeswaran, S., Santhiar, A., Kanade, A., et al.: Semi-supervised verified feedback generation. In: Proceedings of the 2016 24th ACM SIGSOFT International Symposium on Foundations of Software Engineering. Association for Computing Machinery, New York, NY, USA, FSE 2016, pp. 739–750 (2016). https://doi.org/10.1145/2950290.2950363
Kirch, W. (ed.): Pearson’s Correlation Coefficient, pp. 1090–1091. Springer, Dordrecht (2008)
Google Scholar
Koivisto, T., Hellas, A.: Evaluating CodeClusters for effectively providing feedback on code submissions. In: 2022 IEEE Frontiers in Education Conference (FIE). IEEE, pp. 1–9 (2022). https://doi.org/10.1109/FIE56618.2022.9962751
Leal, J.P., Silva, F.: Mooshak: a web-based multi-site programming contest system. Softw. Pract. Exp. 33 (6), 567–581 (2003). https://doi.org/10.1002/spe.522
Lloyd, S.: Least squares quantization in PCM. IEEE Trans. Inf. Theor. 28 (2), 129–137 (1982). https://doi.org/10.1109/TIT.1982.1056489
Luo, L., Zeng, Q.: Solminer: mining distinct solutions in programs. In: Proceedings of the 38th International Conference on Software Engineering Companion. Association for Computing Machinery, New York, NY, USA, ICSE ’16, pp. 481–490 (2016). https://doi.org/10.1145/2889160.2889202
Luo, L., Ming, J., Wu, D., et al.: Semantics-based obfuscation-resilient binary code similarity comparison with applications to software plagiarism detection. In: Proceedings of the 22nd ACM SIGSOFT International Symposium on Foundations of Software Engineering. Association for Computing Machinery, New York, NY, USA, FSE 2014, pp. 389–400 (2014). https://doi.org/10.1145/2635868.2635900
Luxton-Reilly, A., Denny, P., Kirk, D., et al.: On the differences between correct student solutions. In: Proceedings of the 18th ACM Conference on Innovation and Technology in Computer Science Education. Association for Computing Machinery, New York, NY, USA, ITiCSE ’13, pp. 177–182 (2013). https://doi.org/10.1145/2462476.2462505
Luxton-Reilly, A., Simon Albluwi, I., et al.: Introductory programming: a systematic literature review. In: Proceedings Companion of the 23rd Annual ACM Conference on Innovation and Technology in Computer Science Education. Association for Computing Machinery, New York, NY, USA, ITiCSE 2018 Companion, pp. 55–106 (2018). https://doi.org/10.1145/3293881.3295779
Moussiades, L., Vakali, A.: PDetect: a clustering approach for detecting plagiarism in source code datasets. Comput. J. 48 (6), 651–661 (2005). https://doi.org/10.1093/comjnl/bxh119
Nguyen, A., Piech, C., Huang, J., et al.: Codewebs: scalable homework search for massive open online programming courses. In: Proceedings of the 23rd International Conference on World Wide Web. Association for Computing Machinery, New York, NY, USA, WWW ’14, pp. 491–502 (2014). https://doi.org/10.1145/2566486.2568023
Ohmann, T., Rahal, I.: Efficient clustering-based source code plagiarism detection using PIY. Knowl. Inf. Syst. 43 (2), 445–472 (2014). https://doi.org/10.1007/s10115-014-0742-2
Ohmann, T., Rahal, I.: Efficient clustering-based source code plagiarism detection using PIY. Knowl. Inf. Syst. 43 (2), 445–472 (2015). https://doi.org/10.1007/s10115-014-0742-2
Paiva, J.C., Leal, J.P., Figueira, A.: Automated assessment in computer science education: a state-of-the-art review. ACM Trans. Comput. Educ. (2022). https://doi.org/10.1145/3513140
Paiva, J.C., Leal, J.P., Figueira, Á.: Progpedia: collection of source-code submitted to introductory programming assignments. Data Brief 46 , 108887 (2023). https://doi.org/10.1016/j.dib.2023.108887
Perry, DM., Kim, D., Samanta, R., et al.: Semcluster: clustering of imperative programming assignments based on quantitative semantic features. In: Proceedings of the 40th ACM SIGPLAN Conference on Programming Language Design and Implementation. Association for Computing Machinery, New York, NY, USA, PLDI 2019, pp. 860–873 (2019). https://doi.org/10.1145/3314221.3314629
Piech, C., Huang, J., Nguyen, A., et al.: Learning program embeddings to propagate feedback on student code. In: Proceedings of the 32nd International Conference on International Conference on Machine Learning, vol. 37, pp. 1093–1102. JMLR.org, ICML’15 (2015)
Poon, J.Y., Sugiyama, K., Tan, Y.F., et al.: Instructor-centric source code plagiarism detection and plagiarism corpus. In: Proceedings of the 17th ACM Annual Conference on Innovation and Technology in Computer Science Education. Association for Computing Machinery, New York, NY, USA, ITiCSE ’12, pp. 122–127 (2012). https://doi.org/10.1145/2325296.2325328
Pu, Y., Narasimhan, K., Solar-Lezama, A., et al.: Sk_p: a neural program corrector for MOOCs. In: Companion Proceedings of the 2016 ACM SIGPLAN International Conference on Systems, Programming, Languages and Applications: Software for Humanity. Association for Computing Machinery, New York, NY, USA, SPLASH Companion 2016, pp. 39–40 (2016). https://doi.org/10.1145/2984043.2989222
Rivers, K., Koedinger, K.R.: A canonicalizing model for building programming tutors. In: Cerri, S.A., Clancey, W.J., Papadourakis, G., et al. (eds.) Intelligent Tutoring Systems. Springer, Berlin, pp. 591–593 (2012). https://doi.org/10.1007/978-3-642-30950-2_80
Rivers, K., Koedinger, K.R.: Automatic generation of programming feedback: a data-driven approach. In: The First Workshop on AI-supported Education for Computer Science (AIEDCS 2013), pp. 50–59. Memphis, USA (2013)
Sculley, D.: Web-scale k-means clustering. In: Proceedings of the 19th International Conference on World Wide Web. Association for Computing Machinery, New York, NY, USA, WWW ’10, pp. 1177–1178 (2010). https://doi.org/10.1145/1772690.1772862
Wang, K., Singh, R., Su, Z.: Dynamic neural program embedding for program repair (2018). https://doi.org/10.48550/arXiv.1711.07163
Wang, K., Singh, R., Su, Z.: Search, align, and repair: data-driven feedback generation for introductory programming exercises. In: Proceedings of the 39th ACM SIGPLAN Conference on Programming Language Design and Implementation. Association for Computing Machinery, New York, NY, USA, PLDI 2018, pp. 481–495 (2018). https://doi.org/10.1145/3192366.3192384
Weiss, K., Banse, C.: A language-independent analysis platform for source code (2022). https://doi.org/10.48550/arXiv.2203.08424
Xu, S., Chee, Y.S.: Transformation-based diagnosis of student programs for programming tutoring systems. IEEE Trans. Softw. Eng. 29 (4), 360–384 (2003). https://doi.org/10.1109/TSE.2003.1191799
Yamaguchi, F., Golde, N., Arp, D., et al.: Modeling and discovering vulnerabilities with code property graphs. In: 2014 IEEE Symposium on Security and Privacy, pp. 590–604. IEEE, Berkeley, CA, USA (2014). https://doi.org/10.1109/SP.2014.44
Zhang, F., Wu, D., Liu, P., et al.: Program logic based software plagiarism detection. In: 2014 IEEE 25th International Symposium on Software Reliability Engineering, pp. 66–77. IEEE, Naples, Italy (2014). https://doi.org/10.1109/ISSRE.2014.18
Ďuračík, M., Kršák, E., Hrkút, P.: Scalable source code plagiarism detection using source code vectors clustering. In: 2018 IEEE 9th International Conference on Software Engineering and Service Science (ICSESS), pp. 499–502. IEEE, Beijing, China (2018). https://doi.org/10.1109/ICSESS.2018.8663708
Download references
Acknowledgements
Not applicable.
Open access funding provided by FCT|FCCN (b-on). J.C.P.’s work is funded by the FCT—Fundação para a Ciência e a Tecnologia (Portuguese Foundation for Science and Technology), Portugal—for the PhD Grant 2020.04430.BD.
Author information
Authors and affiliations.
CRACS, INESC TEC, Rua do Campo Alegre, 4169-007, Porto, Portugal
José Carlos Paiva, José Paulo Leal & Álvaro Figueira
DCC, FCUP, Rua do Campo Alegre, 4169-007, Porto, Portugal
You can also search for this author in PubMed Google Scholar
Contributions
JCP, JPL, and ÁF contributed to conceptualization; methodology; project administration; validation; and visualization; JCP was involved in data curation, funding acquisition; investigation; resources; and writing—original draft, and provided software; and JPL and ÁF contributed to supervision. All authors have read and agreed to the published version of the manuscript.
Corresponding author
Correspondence to José Carlos Paiva .
Ethics declarations
Conflict of interest.
The authors declare that they have no conflict of interest.
Additional information
Publisher's note.
Springer Nature remains neutral with regard to jurisdictional claims in published maps and institutional affiliations.
Rights and permissions
Open Access This article is licensed under a Creative Commons Attribution 4.0 International License, which permits use, sharing, adaptation, distribution and reproduction in any medium or format, as long as you give appropriate credit to the original author(s) and the source, provide a link to the Creative Commons licence, and indicate if changes were made. The images or other third party material in this article are included in the article’s Creative Commons licence, unless indicated otherwise in a credit line to the material. If material is not included in the article’s Creative Commons licence and your intended use is not permitted by statutory regulation or exceeds the permitted use, you will need to obtain permission directly from the copyright holder. To view a copy of this licence, visit http://creativecommons.org/licenses/by/4.0/ .
Reprints and permissions
About this article
Paiva, J.C., Leal, J.P. & Figueira, Á. Clustering source code from automated assessment of programming assignments. Int J Data Sci Anal (2024). https://doi.org/10.1007/s41060-024-00554-5
Download citation
Received : 03 January 2024
Accepted : 15 April 2024
Published : 15 May 2024
DOI : https://doi.org/10.1007/s41060-024-00554-5
Share this article
Anyone you share the following link with will be able to read this content:
Sorry, a shareable link is not currently available for this article.
Provided by the Springer Nature SharedIt content-sharing initiative
- Programming learning
- Automated assessment
- Programming assignments
- Semantic graph
- Find a journal
- Publish with us
- Track your research
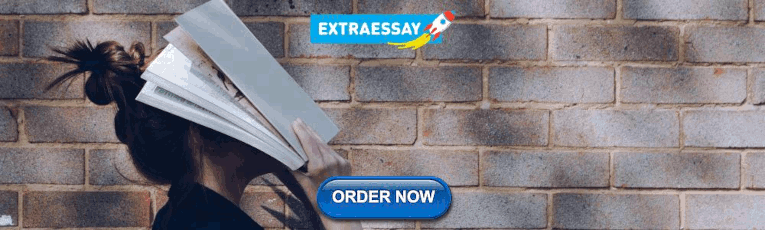
IMAGES
VIDEO
COMMENTS
Describe each of these using simple examples: role, audience, format, and topic. (It may be helpful to write the elements on chart paper or a bulletin board for future reference). Model how to write responses to the prompts, and discuss the key elements as a class. Teachers should keep this as simple and concise as possible for younger students.
Hey guys here is a video on Chart paper decoration ideas for school / how to make chart papersPLEASE SUBSCRIBE HERE: https://www.youtube.com/channel/UCAXpDlE...
Indent the first line of every paragraph of text 0.5 in. using the tab key or the paragraph-formatting function of your word-processing program. Page numbers: Put a page number in the top right corner of every page, including the title page or cover page, which is page 1. Student papers do not require a running head on any page.
Templates for college and university assignments. Include customizable templates in your college toolbox. Stay focused on your studies and leave the assignment structuring to tried and true layout templates for all kinds of papers, reports, and more. Category. Color. Create from scratch. Show all.
They are also used to make a paper or article more readable by removing numeric or listed data from the text. Tables are typically used to present raw data, not when you want to show a relationship between variables. Figures are visual presentations of results. They come in the form of graphs, charts, drawings, photos, or maps.
What this handout is about. The first step in any successful college writing venture is reading the assignment. While this sounds like a simple task, it can be a tough one. This handout will help you unravel your assignment and begin to craft an effective response. Much of the following advice will involve translating typical assignment terms ...
Work Time B: Display jigsaw groups and reading assignments on chart paper or an external monitor for students to reference easily. Work Time B: Display a brief video or infographic about air traffic control to enhance comprehension of Katherine Johnson's contributions to air traffic safety during the reading of chapter 13 in Hidden Figures.
Graph paper is required to perform math subjects and science disciplines accurately. Many courses require graph paper and instructors may penalize students who do not use graph paper. As a result, it is important to have plenty of graph paper ready for classes, homework assignments, and tests.
In a table, readers can look up exact values, compare those values between pairs or groups of related measurements (e.g., growth rates or outcomes of a medical procedure over several years), look at ranges and intervals, and select specific factors to search for patterns. Tables are not restrained to a specific type of data or measurement.
Isometric (Triangle Grid) Paper. In this kind of graph paper, instead of squares, the grid is made up out of equilateral triangles. Use it to create 3-dimensional drawings for designs like woodworking plans, interior space planning, or graphing on an XYZ-axis. Draw your vertical lines normally, and then draw any horizontal lines using the sides ...
Tables, Images, & Appendices. For some papers and reports, you may choose to add a table, graph, chart, or image within the body of the draft. Or you may choose to include an appendix at the end of your paper. These can help to provide a visual representation of data or other information that you wish to relay to your reader.
When you read the assignment prompt, you should do the following: • Look for action verbs. Verbs like analyze, compare, discuss, explain, make an argument, propose a solution, trace, or research can help you understand what you're being asked to do with an assignment. Unless the instructor has specified otherwise, most of your paper ...
Tables and figures are each numbered separately, in the order they are referred to in your text. For example, the first table you refer to is Table 1; the fourth figure you refer to is Figure 4. The title should clearly and straightforwardly describe the content of the table or figure. Omit articles to keep it concise.
Plotting graphs is an essential component of analysing experimental results in GCSE science and A-Level sciences. This video demonstrates a methodical appro...
Your summaries of these texts should be between 300 and 400 words of your final paper. Analysis. In this part of your paper, you will make connections between Mitchell's concepts and the specific situation described in the article you have chosen. ... In this assignment, we are being asked to use factual and conceptual knowledge to understand ...
The Research Assignment Introduction. When tasked with writing a research paper, you are able to "dig in" to a topic, idea, theme, or question in greater detail. In your academic career, you will be assigned several assignments that require you to "research" something and then write about it.
Tables and figures taken from other sources are numbered and presented in the same format as your other tables and figures. Refer to them as Table 1, Figure 3, etc., but include an in-text citation after you mention them to acknowledge the source. In-text citation example. The results in Table 1 (Ajzen, 1991, p. 179) show that ….
Figure 9. Edit Data option. Pro Tip: Select the arrow next to Edit Data and select Edit Data in Excel if you want to update your chart in Excel rather than Word's spreadsheet. Edit your data in the spreadsheet (see figure 6). Select the X to close the spreadsheet and apply your changes (see figure 7).; How to Resize a Chart. Charts can be resized by dragging the border or by using exact ...
Using ChatGPT for Assignments | Tips & Examples. Published on February 13, 2023 by Jack Caulfield and Tobias Solis. Revised on November 16, 2023. People are still figuring out the best use cases for ChatGPT, the popular chatbot based on a powerful AI language model.This article provides some ideas for how to use ChatGPT and other AI tools to assist with your academic writing.
These graphing worksheets will produce a polar coordinate grid for the students to use in polar coordinate graphing problems. These Graphing Worksheets are perfect for teachers, homeschoolers, moms, dads, and children looking for some practice in Graphing problems and graph paper for various types of problems.
Week Of. Most assignment charts will include a 'Week of:' section and are generally formatted to cover the span of a week. Place this section somewhere toward the top of the chart. You can type ...
Here you will find an assortment of free printable online graph paper. All graph papers a available as free downloadable PDF. They come in all sizes and orientations, from letter to 11x17 - to poster size. Both landscape or portrait.
Border design for projects | Border design for school assignments #viral #project #assignment #projectzomboid #projects #design #border #borderdesigns #frame #template #suggestion #paper #design #blank #illustration #flip #symbol #note #certificate #book #connection #office #logo #shadow #network #web #floral #document #silhoutte #bookpages #business #pagedecorations #people #webpage #banner # ...
This paper introduces an online approach and implemented tool—AsanasCluster—to cluster source code submissions to programming assignments. The proposed approach relies on program attributes extracted from semantic graph representations of source code, including control and data flow features.