
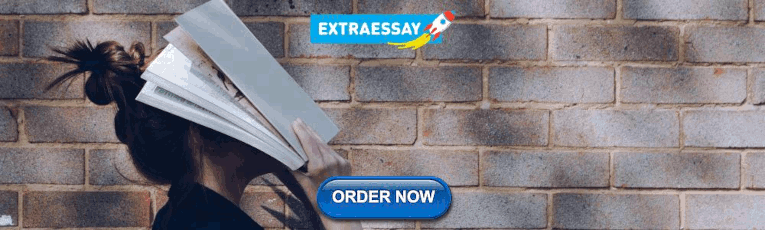
Biology Hypothesis
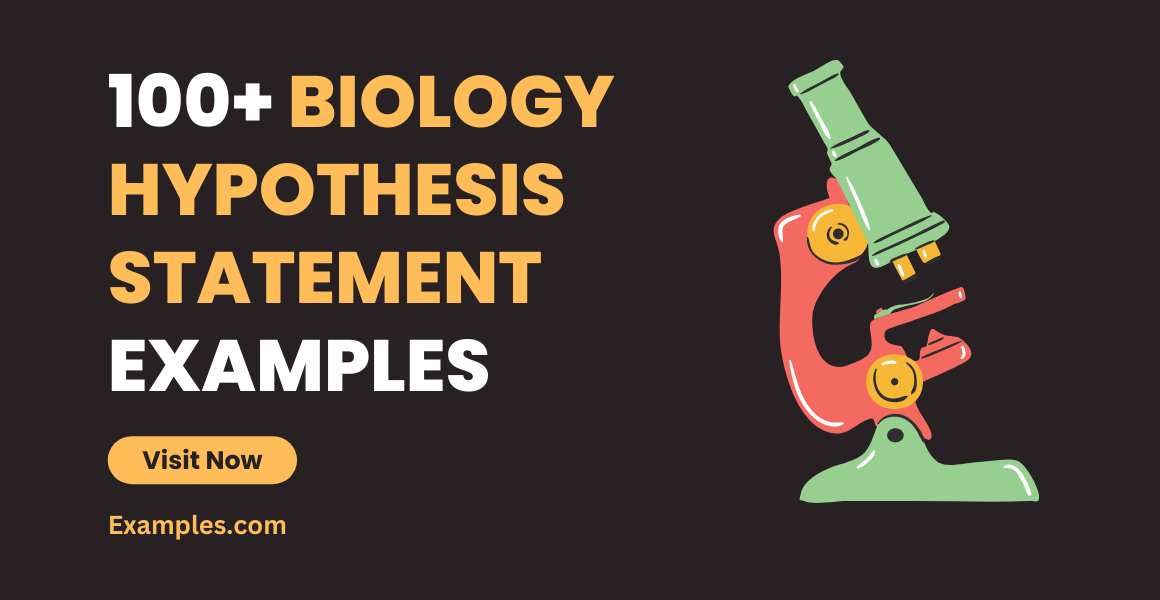
Delve into the fascinating world of biology with our definitive guide on crafting impeccable hypothesis thesis statements . As the foundation of any impactful biological research, a well-formed hypothesis paves the way for groundbreaking discoveries and insights. Whether you’re examining cellular behavior or large-scale ecosystems, mastering the art of the thesis statement is crucial. Embark on this enlightening journey with us, as we provide stellar examples and invaluable writing advice tailored for budding biologists.
What is a good hypothesis in biology?
A good hypothesis in biology is a statement that offers a tentative explanation for a biological phenomenon, based on prior knowledge or observation. It should be:
- Testable: The hypothesis should be measurable and can be proven false through experiments or observations.
- Clear: It should be stated clearly and without ambiguity.
- Based on Knowledge: A solid hypothesis often stems from existing knowledge or literature in the field.
- Specific: It should clearly define the variables being tested and the expected outcomes.
- Falsifiable: It’s essential that a hypothesis can be disproven. This means there should be a possible result that could indicate the hypothesis is incorrect.
What is an example of a hypothesis statement in biology?
Example: “If a plant is given a higher concentration of carbon dioxide, then it will undergo photosynthesis at an increased rate compared to a plant given a standard concentration of carbon dioxide.”
In this example:
- The independent variable (what’s being changed) is the concentration of carbon dioxide.
- The dependent variable (what’s being measured) is the rate of photosynthesis. The statement proposes a cause-and-effect relationship that can be tested through experimentation.
100 Biology Thesis Statement Examples
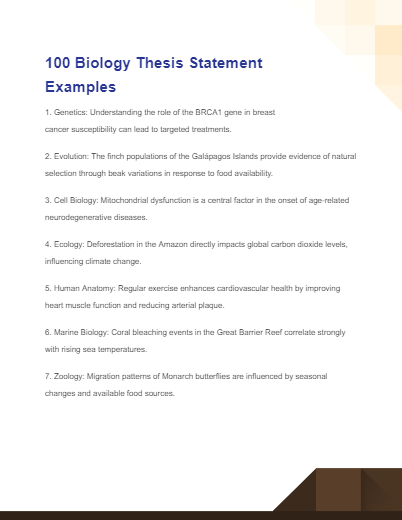
Size: 272 KB
Biology, as the study of life and living organisms, is vast and diverse. Crafting a good thesis statement in this field requires a clear understanding of the topic at hand, capturing the essence of the research aim. From genetics to ecology, from cell biology to animal behavior, the following examples will give you a comprehensive idea about forming succinct biology thesis statements.
Genetics: Understanding the role of the BRCA1 gene in breast cancer susceptibility can lead to targeted treatments.
2. Evolution: The finch populations of the Galápagos Islands provide evidence of natural selection through beak variations in response to food availability.
3. Cell Biology: Mitochondrial dysfunction is a central factor in the onset of age-related neurodegenerative diseases.
4. Ecology: Deforestation in the Amazon directly impacts global carbon dioxide levels, influencing climate change.
5. Human Anatomy: Regular exercise enhances cardiovascular health by improving heart muscle function and reducing arterial plaque.
6. Marine Biology: Coral bleaching events in the Great Barrier Reef correlate strongly with rising sea temperatures.
7. Zoology: Migration patterns of Monarch butterflies are influenced by seasonal changes and available food sources.
8. Botany: The symbiotic relationship between mycorrhizal fungi and plant roots enhances nutrient absorption in poor soil conditions.
9. Microbiology: The overuse of antibiotics in healthcare has accelerated the evolution of antibiotic-resistant bacterial strains.
10. Physiology: High altitude adaptation in certain human populations has led to increased hemoglobin production.
11. Immunology: The role of T-cells in the human immune response is critical in developing effective vaccines against viral diseases.
12. Behavioral Biology: Birdsong variations in sparrows can be attributed to both genetic factors and environmental influences.
13. Developmental Biology: The presence of certain hormones during fetal development dictates the differentiation of sex organs in mammals.
14. Conservation Biology: The rapid decline of bee populations worldwide is directly linked to the use of certain pesticides in agriculture.
15. Molecular Biology: The CRISPR-Cas9 system has revolutionized gene editing techniques, offering potential cures for genetic diseases.
16. Virology: The mutation rate of the influenza virus necessitates annual updates in vaccine formulations.
17. Neurobiology: Neural plasticity in the adult brain can be enhanced through consistent learning and cognitive challenges.
18. Ethology: Elephant herds exhibit complex social structures and matriarchal leadership.
19. Biotechnology: Genetically modified crops can improve yield and resistance but also pose ecological challenges.
20. Environmental Biology: Industrial pollution in freshwater systems disrupts aquatic life and can lead to loss of biodiversity.
21. Neurodegenerative Diseases: Amyloid-beta protein accumulation in the brain is a key marker for Alzheimer’s disease progression.
22. Endocrinology: The disruption of thyroid hormone balance leads to metabolic disorders and weight fluctuations.
23. Bioinformatics: Machine learning algorithms can predict protein structures with high accuracy, advancing drug design.
24. Plant Physiology: The stomatal closure mechanism in plants helps prevent water loss and maintain turgor pressure.
25. Parasitology: The lifecycle of the malaria parasite involves complex interactions between humans and mosquitoes.
26. Molecular Genetics: Epigenetic modifications play a crucial role in gene expression regulation and cell differentiation.
27. Evolutionary Psychology: Human preference for symmetrical faces is a result of evolutionarily advantageous traits.
28. Ecosystem Dynamics: The reintroduction of apex predators in ecosystems restores ecological balance and biodiversity.
29. Epigenetics: Maternal dietary choices during pregnancy can influence the epigenetic profiles of offspring.
30. Biochemistry: Enzyme kinetics in metabolic pathways reveal insights into cellular energy production.
31. Bioluminescence: The role of bioluminescence in deep-sea organisms serves as camouflage and communication.
32. Genetics of Disease: Mutations in the CFTR gene cause cystic fibrosis, leading to severe respiratory and digestive issues.
33. Reproductive Biology: The influence of pheromones on mate selection is a critical aspect of reproductive success in many species.
34. Plant-Microbe Interactions: Rhizobium bacteria facilitate nitrogen fixation in leguminous plants, benefiting both organisms.
35. Comparative Anatomy: Homologous structures in different species provide evidence of shared evolutionary ancestry.
36. Stem Cell Research: Induced pluripotent stem cells hold immense potential for regenerative medicine and disease modeling.
37. Bioethics: Balancing the use of genetic modification in humans with ethical considerations is a complex challenge.
38. Molecular Evolution: The study of orthologous and paralogous genes offers insights into evolutionary relationships.
39. Bioenergetics: ATP synthesis through oxidative phosphorylation is a fundamental process driving cellular energy production.
40. Population Genetics: The Hardy-Weinberg equilibrium model helps predict allele frequencies in populations over time.
41. Animal Communication: The complex vocalizations of whales serve both social bonding and long-distance communication purposes.
42. Biogeography: The distribution of marsupials in Australia and their absence elsewhere highlights the impact of geographical isolation on evolution.
43. Aquatic Ecology: The phenomenon of eutrophication in lakes is driven by excessive nutrient runoff and results in harmful algal blooms.
44. Insect Behavior: The waggle dance of honeybees conveys precise information about the location of food sources to other members of the hive.
45. Microbial Ecology: The gut microbiome’s composition influences host health, metabolism, and immune system development.
46. Evolution of Sex: The Red Queen hypothesis explains the evolution of sexual reproduction as a defense against rapidly evolving parasites.
47. Immunotherapy: Manipulating the immune response to target cancer cells shows promise as an effective cancer treatment strategy.
48. Epigenetic Inheritance: Epigenetic modifications can be passed down through generations, impacting traits and disease susceptibility.
49. Comparative Genomics: Comparing the genomes of different species sheds light on genetic adaptations and evolutionary divergence.
50. Neurotransmission: The dopamine reward pathway in the brain is implicated in addiction and motivation-related behaviors.
51. Microbial Biotechnology: Genetically engineered bacteria can produce valuable compounds like insulin, revolutionizing pharmaceutical production.
52. Bioinformatics: DNA sequence analysis reveals evolutionary relationships between species and uncovers hidden genetic information.
53. Animal Migration: The navigational abilities of migratory birds are influenced by magnetic fields and celestial cues.
54. Human Evolution: The discovery of ancient hominin fossils provides insights into the evolutionary timeline of our species.
55. Cancer Genetics: Mutations in tumor suppressor genes contribute to the uncontrolled growth and division of cancer cells.
56. Aquatic Biomes: Coral reefs, rainforests of the sea, host incredible biodiversity and face threats from climate change and pollution.
57. Genomic Medicine: Personalized treatments based on an individual’s genetic makeup hold promise for more effective healthcare.
58. Molecular Pharmacology: Understanding receptor-ligand interactions aids in the development of targeted drugs for specific diseases.
59. Biodiversity Conservation: Preserving habitat diversity is crucial to maintaining ecosystems and preventing species extinction.
60. Evolutionary Developmental Biology: Comparing embryonic development across species reveals shared genetic pathways and evolutionary constraints.
61. Plant Reproductive Strategies: Understanding the trade-offs between asexual and sexual reproduction in plants sheds light on their evolutionary success.
62. Parasite-Host Interactions: The coevolution of parasites and their hosts drives adaptations and counter-adaptations over time.
63. Genomic Diversity: Exploring genetic variations within populations helps uncover disease susceptibilities and evolutionary history.
64. Ecological Succession: Studying the process of ecosystem recovery after disturbances provides insights into resilience and stability.
65. Conservation Genetics: Genetic diversity assessment aids in formulating effective conservation strategies for endangered species.
66. Neuroplasticity and Learning: Investigating how the brain adapts through synaptic changes improves our understanding of memory and learning.
67. Synthetic Biology: Designing and engineering biological systems offers innovative solutions for medical, environmental, and industrial challenges.
68. Ethnobotany: Documenting the traditional uses of plants by indigenous communities informs both conservation and pharmaceutical research.
69. Ecological Niche Theory: Exploring how species adapt to specific ecological niches enhances our grasp of biodiversity patterns.
70. Ecosystem Services: Quantifying the benefits provided by ecosystems, like pollination and carbon sequestration, supports conservation efforts.
71. Fungal Biology: Investigating mycorrhizal relationships between fungi and plants illuminates nutrient exchange mechanisms.
72. Molecular Clock Hypothesis: Genetic mutations accumulate over time, providing a method to estimate evolutionary divergence dates.
73. Developmental Disorders: Unraveling the genetic and environmental factors contributing to developmental disorders informs therapeutic approaches.
74. Epigenetics and Disease: Epigenetic modifications contribute to the development of diseases like cancer, diabetes, and neurodegenerative disorders.
75. Animal Cognition: Studying cognitive abilities in animals unveils their problem-solving skills, social dynamics, and sensory perceptions.
76. Microbiota-Brain Axis: The gut-brain connection suggests a bidirectional communication pathway influencing mental health and behavior.
77. Neurological Disorders: Neurodegenerative diseases like Parkinson’s and Alzheimer’s have genetic and environmental components that drive their progression.
78. Plant Defense Mechanisms: Investigating how plants ward off pests and pathogens informs sustainable agricultural practices.
79. Conservation Genomics: Genetic data aids in identifying distinct populations and prioritizing conservation efforts for at-risk species.
80. Reproductive Strategies: Comparing reproductive methods in different species provides insights into evolutionary trade-offs and reproductive success.
81. Epigenetics in Aging: Exploring epigenetic changes in the aging process offers insights into longevity and age-related diseases.
82. Antimicrobial Resistance: Understanding the genetic mechanisms behind bacterial resistance to antibiotics informs strategies to combat the global health threat.
83. Plant-Animal Interactions: Investigating mutualistic relationships between plants and pollinators showcases the delicate balance of ecosystems.
84. Adaptations to Extreme Environments: Studying extremophiles reveals the remarkable ways organisms thrive in extreme conditions like deep-sea hydrothermal vents.
85. Genetic Disorders: Genetic mutations underlie numerous disorders like cystic fibrosis, sickle cell anemia, and muscular dystrophy.
86. Conservation Behavior: Analyzing the behavioral ecology of endangered species informs habitat preservation and restoration efforts.
87. Neuroplasticity in Rehabilitation: Harnessing the brain’s ability to rewire itself offers promising avenues for post-injury or post-stroke rehabilitation.
88. Disease Vectors: Understanding how mosquitoes transmit diseases like malaria and Zika virus is critical for disease prevention strategies.
89. Biochemical Pathways: Mapping metabolic pathways in cells provides insights into disease development and potential therapeutic targets.
90. Invasive Species Impact: Examining the effects of invasive species on native ecosystems guides management strategies to mitigate their impact.
91. Molecular Immunology: Studying the intricate immune response mechanisms aids in the development of vaccines and immunotherapies.
92. Plant-Microbe Symbiosis: Investigating how plants form partnerships with beneficial microbes enhances crop productivity and sustainability.
93. Cancer Immunotherapy: Harnessing the immune system to target and eliminate cancer cells offers new avenues for cancer treatment.
94. Evolution of Flight: Analyzing the adaptations leading to the development of flight in birds and insects sheds light on evolutionary innovation.
95. Genomic Diversity in Human Populations: Exploring genetic variations among different human populations informs ancestry, migration, and susceptibility to diseases.
96. Hormonal Regulation: Understanding the role of hormones in growth, reproduction, and homeostasis provides insights into physiological processes.
97. Conservation Genetics in Plant Conservation: Genetic diversity assessment helps guide efforts to conserve rare and endangered plant species.
98. Neuronal Communication: Investigating neurotransmitter systems and synaptic transmission enhances our comprehension of brain function.
99. Microbial Biogeography: Mapping the distribution of microorganisms across ecosystems aids in understanding their ecological roles and interactions.
100. Gene Therapy: Developing methods to replace or repair defective genes offers potential treatments for genetic disorders.
Scientific Hypothesis Statement Examples
This section offers diverse examples of scientific hypothesis statements that cover a range of biological topics. Each example briefly describes the subject matter and the potential implications of the hypothesis.
- Genetic Mutations and Disease: Certain genetic mutations lead to increased susceptibility to autoimmune disorders, providing insights into potential treatment strategies.
- Microplastics in Aquatic Ecosystems: Elevated microplastic levels disrupt aquatic food chains, affecting biodiversity and human health through bioaccumulation.
- Bacterial Quorum Sensing: Inhibition of quorum sensing in pathogenic bacteria demonstrates a potential avenue for novel antimicrobial therapies.
- Climate Change and Phenology: Rising temperatures alter flowering times in plants, impacting pollinator interactions and ecosystem dynamics.
- Neuroplasticity and Learning: The brain’s adaptability facilitates learning through synaptic modifications, elucidating educational strategies for improved cognition.
- CRISPR-Cas9 in Agriculture: CRISPR-engineered crops with enhanced pest resistance showcase a sustainable approach to improving agricultural productivity.
- Invasive Species Impact on Predators: The introduction of invasive prey disrupts predator-prey relationships, triggering cascading effects in terrestrial ecosystems.
- Microbial Contributions to Soil Health: Beneficial soil microbes enhance nutrient availability and plant growth, promoting sustainable agriculture practices.
- Marine Protected Areas: Examining the effectiveness of marine protected areas reveals their role in preserving biodiversity and restoring marine ecosystems.
- Epigenetic Regulation of Cancer: Epigenetic modifications play a pivotal role in cancer development, highlighting potential therapeutic targets for precision medicine.
Testable Hypothesis Statement Examples in Biology
Testability hypothesis is a critical aspect of a hypothesis. These examples are formulated in a way that allows them to be tested through experiments or observations. They focus on cause-and-effect relationships that can be verified or refuted.
- Impact of Light Intensity on Plant Growth: Increasing light intensity accelerates photosynthesis rates and enhances overall plant growth.
- Effect of Temperature on Enzyme Activity: Higher temperatures accelerate enzyme activity up to an optimal point, beyond which denaturation occurs.
- Microbial Diversity in Soil pH Gradients: Soil pH influences microbial composition, with acidic soils favoring certain bacterial taxa over others.
- Predation Impact on Prey Behavior: The presence of predators induces changes in prey behavior, resulting in altered foraging strategies and vigilance levels.
- Chemical Communication in Marine Organisms: Investigating chemical cues reveals the role of allelopathy in competition among marine organisms.
- Social Hierarchy in Animal Groups: Observing animal groups establishes a correlation between social rank and access to resources within the group.
- Effect of Habitat Fragmentation on Pollinator Diversity: Fragmented habitats reduce pollinator species richness, affecting plant reproductive success.
- Dietary Effects on Gut Microbiota Composition: Dietary shifts influence gut microbiota diversity and metabolic functions, impacting host health.
- Hybridization Impact on Plant Fitness: Hybrid plants exhibit varied fitness levels depending on the combination of parent species.
- Human Impact on Coral Bleaching: Analyzing coral reefs under different anthropogenic stresses identifies the main factors driving coral bleaching events.
Scientific Investigation Hypothesis Statement Examples in Biology
This section emphasizes hypotheses that are part of broader scientific investigations. They involve studying complex interactions or phenomena and often contribute to our understanding of larger biological systems.
- Genomic Variation in Human Disease Susceptibility: Genetic analysis identifies variations associated with increased risk of common diseases, aiding personalized medicine.
- Behavioral Responses to Temperature Shifts in Insects: Investigating insect responses to temperature fluctuations reveals adaptation strategies to climate change.
- Endocrine Disruptors and Amphibian Development: Experimental exposure to endocrine disruptors elucidates their role in amphibian developmental abnormalities.
- Microbial Succession in Decomposition: Tracking microbial communities during decomposition uncovers the succession patterns of different decomposer species.
- Gene Expression Patterns in Stress Response: Studying gene expression profiles unveils the molecular mechanisms underlying stress responses in plants.
- Effect of Urbanization on Bird Song Patterns: Urban noise pollution influences bird song frequency and complexity, impacting communication and mate attraction.
- Nutrient Availability and Algal Blooms: Investigating nutrient loading in aquatic systems sheds light on factors triggering harmful algal blooms.
- Host-Parasite Coevolution: Analyzing genetic changes in hosts and parasites over time uncovers coevolutionary arms races and adaptation.
- Ecosystem Productivity and Biodiversity: Linking ecosystem productivity to biodiversity patterns reveals the role of species interactions in ecosystem stability.
- Habitat Preference of Invasive Species: Studying the habitat selection of invasive species identifies factors promoting their establishment and spread.
Hypothesis Statement Examples in Biology Research
These examples are tailored for research hypothesis studies. They highlight hypotheses that drive focused research questions, often leading to specific experimental designs and data collection methods.
- Microbial Community Structure in Human Gut: Investigating microbial diversity and composition unveils the role of gut microbiota in human health.
- Plant-Pollinator Mutualisms: Hypothesizing reciprocal benefits in plant-pollinator interactions highlights the role of coevolution in shaping ecosystems.
- Chemical Defense Mechanisms in Insects: Predicting the correlation between insect feeding behavior and chemical defenses explores natural selection pressures.
- Evolutionary Significance of Mimicry: Examining mimicry in organisms demonstrates its adaptive value in predator-prey relationships and survival.
- Neurological Basis of Mate Choice: Proposing neural mechanisms underlying mate choice behaviors uncovers the role of sensory cues in reproductive success.
- Mycorrhizal Symbiosis Impact on Plant Growth: Investigating mycorrhizal colonization effects on plant biomass addresses nutrient exchange dynamics.
- Social Learning in Primates: Formulating a hypothesis on primate social learning explores the transmission of knowledge and cultural behaviors.
- Effect of Pollution on Fish Behavior: Anticipating altered behaviors due to pollution exposure highlights ecological consequences on aquatic ecosystems.
- Coevolution of Flowers and Pollinators: Hypothesizing mutual adaptations between flowers and pollinators reveals intricate ecological relationships.
- Genetic Basis of Disease Resistance in Plants: Identifying genetic markers associated with disease resistance enhances crop breeding programs.
Prediction Hypothesis Statement Examples in Biology
Predictive simple hypothesis involve making educated guesses about how variables might interact or behave under specific conditions. These examples showcase hypotheses that anticipate outcomes based on existing knowledge.
- Pesticide Impact on Insect Abundance: Predicting decreased insect populations due to pesticide application underscores ecological ramifications.
- Climate Change and Migratory Bird Patterns: Anticipating shifts in migratory routes of birds due to climate change informs conservation strategies.
- Ocean Acidification Effect on Coral Calcification: Predicting reduced coral calcification rates due to ocean acidification unveils threats to coral reefs.
- Disease Spread in Crowded Bird Roosts: Predicting accelerated disease transmission in densely populated bird roosts highlights disease ecology dynamics.
- Eutrophication Impact on Freshwater Biodiversity: Anticipating decreased freshwater biodiversity due to eutrophication emphasizes conservation efforts.
- Herbivore Impact on Plant Species Diversity: Predicting reduced plant diversity in areas with high herbivore pressure elucidates ecosystem dynamics.
- Predator-Prey Population Cycles: Predicting cyclical fluctuations in predator and prey populations showcases the role of trophic interactions.
- Climate Change and Plant Phenology: Anticipating earlier flowering times due to climate change demonstrates the influence of temperature on plant life cycles.
- Antibiotic Resistance in Bacterial Communities: Predicting increased antibiotic resistance due to overuse forewarns the need for responsible antibiotic use.
- Human Impact on Avian Nesting Success: Predicting decreased avian nesting success due to habitat fragmentation highlights conservation priorities.
How to Write a Biology Hypothesis – Step by Step Guide
A hypothesis in biology is a critical component of scientific research that proposes an explanation for a specific biological phenomenon. Writing a well-formulated hypothesis sets the foundation for conducting experiments, making observations, and drawing meaningful conclusions. Follow this step-by-step guide to create a strong biology hypothesis:
1. Identify the Phenomenon: Clearly define the biological phenomenon you intend to study. This could be a question, a pattern, an observation, or a problem in the field of biology.
2. Conduct Background Research: Before formulating a hypothesis, gather relevant information from scientific literature. Understand the existing knowledge about the topic to ensure your hypothesis builds upon previous research.
3. State the Independent and Dependent Variables: Identify the variables involved in the phenomenon. The independent variable is what you manipulate or change, while the dependent variable is what you measure as a result of the changes.
4. Formulate a Testable Question: Based on your background research, create a specific and testable question that addresses the relationship between the variables. This question will guide the formulation of your hypothesis.
5. Craft the Hypothesis: A hypothesis should be a clear and concise statement that predicts the outcome of your experiment or observation. It should propose a cause-and-effect relationship between the independent and dependent variables.
6. Use the “If-Then” Structure: Formulate your hypothesis using the “if-then” structure. The “if” part states the independent variable and the condition you’re manipulating, while the “then” part predicts the outcome for the dependent variable.
7. Make it Falsifiable: A good hypothesis should be testable and capable of being proven false. There should be a way to gather data that either supports or contradicts the hypothesis.
8. Be Specific and Precise: Avoid vague language and ensure that your hypothesis is specific and precise. Clearly define the variables and the expected relationship between them.
9. Revise and Refine: Once you’ve formulated your hypothesis, review it to ensure it accurately reflects your research question and variables. Revise as needed to make it more concise and focused.
10. Seek Feedback: Share your hypothesis with peers, mentors, or colleagues to get feedback. Constructive input can help you refine your hypothesis further.
Tips for Writing a Biology Hypothesis Statement
Writing a biology alternative hypothesis statement requires precision and clarity to ensure that your research is well-structured and testable. Here are some valuable tips to help you create effective and scientifically sound hypothesis statements:
1. Be Clear and Concise: Your hypothesis statement should convey your idea succinctly. Avoid unnecessary jargon or complex language that might confuse your audience.
2. Address Cause and Effect: A hypothesis suggests a cause-and-effect relationship between variables. Clearly state how changes in the independent variable are expected to affect the dependent variable.
3. Use Specific Language: Define your variables precisely. Use specific terms to describe the independent and dependent variables, as well as any conditions or measurements.
4. Follow the “If-Then” Structure: Use the classic “if-then” structure to frame your hypothesis. State the independent variable (if) and the expected outcome (then). This format clarifies the relationship you’re investigating.
5. Make it Testable: Your hypothesis must be capable of being tested through experimentation or observation. Ensure that there is a measurable and observable way to determine if it’s true or false.
6. Avoid Ambiguity: Eliminate vague terms that can be interpreted in multiple ways. Be precise in your language to avoid confusion.
7. Base it on Existing Knowledge: Ground your hypothesis in prior research or existing scientific theories. It should build upon established knowledge and contribute new insights.
8. Predict a Direction: Your hypothesis should predict a specific outcome. Whether you anticipate an increase, decrease, or a difference, your hypothesis should make a clear prediction.
9. Be Focused: Keep your hypothesis statement focused on one specific idea or relationship. Avoid trying to address too many variables or concepts in a single statement.
10. Consider Alternative Explanations: Acknowledge alternative explanations for your observations or outcomes. This demonstrates critical thinking and a thorough understanding of your field.
11. Avoid Value Judgments: Refrain from including value judgments or opinions in your hypothesis. Stick to objective and measurable factors.
12. Be Realistic: Ensure that your hypothesis is plausible and feasible. It should align with what is known about the topic and be achievable within the scope of your research.
13. Refine and Revise: Draft multiple versions of your hypothesis statement and refine them. Discuss and seek feedback from mentors, peers, or advisors to enhance its clarity and precision.
14. Align with Research Goals: Your hypothesis should align with the overall goals of your research project. Make sure it addresses the specific question or problem you’re investigating.
15. Be Open to Revision: As you conduct research and gather data, be open to revising your hypothesis if the evidence suggests a different outcome than initially predicted.
Remember, a well-crafted biology science hypothesis statement serves as the foundation of your research and guides your experimental design and data analysis. It’s essential to invest time and effort in formulating a clear, focused, and testable hypothesis that contributes to the advancement of scientific knowledge.
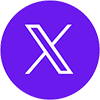
AI Generator
Text prompt
- Instructive
- Professional
10 Examples of Public speaking
20 Examples of Gas lighting
Have a language expert improve your writing
Run a free plagiarism check in 10 minutes, automatically generate references for free.
- Knowledge Base
- Methodology
- How to Write a Strong Hypothesis | Guide & Examples
How to Write a Strong Hypothesis | Guide & Examples
Published on 6 May 2022 by Shona McCombes .
A hypothesis is a statement that can be tested by scientific research. If you want to test a relationship between two or more variables, you need to write hypotheses before you start your experiment or data collection.
Table of contents
What is a hypothesis, developing a hypothesis (with example), hypothesis examples, frequently asked questions about writing hypotheses.
A hypothesis states your predictions about what your research will find. It is a tentative answer to your research question that has not yet been tested. For some research projects, you might have to write several hypotheses that address different aspects of your research question.
A hypothesis is not just a guess – it should be based on existing theories and knowledge. It also has to be testable, which means you can support or refute it through scientific research methods (such as experiments, observations, and statistical analysis of data).
Variables in hypotheses
Hypotheses propose a relationship between two or more variables . An independent variable is something the researcher changes or controls. A dependent variable is something the researcher observes and measures.
In this example, the independent variable is exposure to the sun – the assumed cause . The dependent variable is the level of happiness – the assumed effect .
Prevent plagiarism, run a free check.
Step 1: ask a question.
Writing a hypothesis begins with a research question that you want to answer. The question should be focused, specific, and researchable within the constraints of your project.
Step 2: Do some preliminary research
Your initial answer to the question should be based on what is already known about the topic. Look for theories and previous studies to help you form educated assumptions about what your research will find.
At this stage, you might construct a conceptual framework to identify which variables you will study and what you think the relationships are between them. Sometimes, you’ll have to operationalise more complex constructs.
Step 3: Formulate your hypothesis
Now you should have some idea of what you expect to find. Write your initial answer to the question in a clear, concise sentence.
Step 4: Refine your hypothesis
You need to make sure your hypothesis is specific and testable. There are various ways of phrasing a hypothesis, but all the terms you use should have clear definitions, and the hypothesis should contain:
- The relevant variables
- The specific group being studied
- The predicted outcome of the experiment or analysis
Step 5: Phrase your hypothesis in three ways
To identify the variables, you can write a simple prediction in if … then form. The first part of the sentence states the independent variable and the second part states the dependent variable.
In academic research, hypotheses are more commonly phrased in terms of correlations or effects, where you directly state the predicted relationship between variables.
If you are comparing two groups, the hypothesis can state what difference you expect to find between them.
Step 6. Write a null hypothesis
If your research involves statistical hypothesis testing , you will also have to write a null hypothesis. The null hypothesis is the default position that there is no association between the variables. The null hypothesis is written as H 0 , while the alternative hypothesis is H 1 or H a .
Hypothesis testing is a formal procedure for investigating our ideas about the world using statistics. It is used by scientists to test specific predictions, called hypotheses , by calculating how likely it is that a pattern or relationship between variables could have arisen by chance.
A hypothesis is not just a guess. It should be based on existing theories and knowledge. It also has to be testable, which means you can support or refute it through scientific research methods (such as experiments, observations, and statistical analysis of data).
A research hypothesis is your proposed answer to your research question. The research hypothesis usually includes an explanation (‘ x affects y because …’).
A statistical hypothesis, on the other hand, is a mathematical statement about a population parameter. Statistical hypotheses always come in pairs: the null and alternative hypotheses. In a well-designed study , the statistical hypotheses correspond logically to the research hypothesis.
Cite this Scribbr article
If you want to cite this source, you can copy and paste the citation or click the ‘Cite this Scribbr article’ button to automatically add the citation to our free Reference Generator.
McCombes, S. (2022, May 06). How to Write a Strong Hypothesis | Guide & Examples. Scribbr. Retrieved 22 April 2024, from https://www.scribbr.co.uk/research-methods/hypothesis-writing/
Is this article helpful?
Shona McCombes
Other students also liked, operationalisation | a guide with examples, pros & cons, what is a conceptual framework | tips & examples, a quick guide to experimental design | 5 steps & examples.
Module 1: Introduction to Biology
Experiments and hypotheses, learning outcomes.
- Form a hypothesis and use it to design a scientific experiment
Now we’ll focus on the methods of scientific inquiry. Science often involves making observations and developing hypotheses. Experiments and further observations are often used to test the hypotheses.
A scientific experiment is a carefully organized procedure in which the scientist intervenes in a system to change something, then observes the result of the change. Scientific inquiry often involves doing experiments, though not always. For example, a scientist studying the mating behaviors of ladybugs might begin with detailed observations of ladybugs mating in their natural habitats. While this research may not be experimental, it is scientific: it involves careful and verifiable observation of the natural world. The same scientist might then treat some of the ladybugs with a hormone hypothesized to trigger mating and observe whether these ladybugs mated sooner or more often than untreated ones. This would qualify as an experiment because the scientist is now making a change in the system and observing the effects.
Forming a Hypothesis
When conducting scientific experiments, researchers develop hypotheses to guide experimental design. A hypothesis is a suggested explanation that is both testable and falsifiable. You must be able to test your hypothesis through observations and research, and it must be possible to prove your hypothesis false.
For example, Michael observes that maple trees lose their leaves in the fall. He might then propose a possible explanation for this observation: “cold weather causes maple trees to lose their leaves in the fall.” This statement is testable. He could grow maple trees in a warm enclosed environment such as a greenhouse and see if their leaves still dropped in the fall. The hypothesis is also falsifiable. If the leaves still dropped in the warm environment, then clearly temperature was not the main factor in causing maple leaves to drop in autumn.
In the Try It below, you can practice recognizing scientific hypotheses. As you consider each statement, try to think as a scientist would: can I test this hypothesis with observations or experiments? Is the statement falsifiable? If the answer to either of these questions is “no,” the statement is not a valid scientific hypothesis.
Practice Questions
Determine whether each following statement is a scientific hypothesis.
Air pollution from automobile exhaust can trigger symptoms in people with asthma.
- No. This statement is not testable or falsifiable.
- No. This statement is not testable.
- No. This statement is not falsifiable.
- Yes. This statement is testable and falsifiable.
Natural disasters, such as tornadoes, are punishments for bad thoughts and behaviors.
a: No. This statement is not testable or falsifiable. “Bad thoughts and behaviors” are excessively vague and subjective variables that would be impossible to measure or agree upon in a reliable way. The statement might be “falsifiable” if you came up with a counterexample: a “wicked” place that was not punished by a natural disaster. But some would question whether the people in that place were really wicked, and others would continue to predict that a natural disaster was bound to strike that place at some point. There is no reason to suspect that people’s immoral behavior affects the weather unless you bring up the intervention of a supernatural being, making this idea even harder to test.
Testing a Vaccine
Let’s examine the scientific process by discussing an actual scientific experiment conducted by researchers at the University of Washington. These researchers investigated whether a vaccine may reduce the incidence of the human papillomavirus (HPV). The experimental process and results were published in an article titled, “ A controlled trial of a human papillomavirus type 16 vaccine .”
Preliminary observations made by the researchers who conducted the HPV experiment are listed below:
- Human papillomavirus (HPV) is the most common sexually transmitted virus in the United States.
- There are about 40 different types of HPV. A significant number of people that have HPV are unaware of it because many of these viruses cause no symptoms.
- Some types of HPV can cause cervical cancer.
- About 4,000 women a year die of cervical cancer in the United States.
Practice Question
Researchers have developed a potential vaccine against HPV and want to test it. What is the first testable hypothesis that the researchers should study?
- HPV causes cervical cancer.
- People should not have unprotected sex with many partners.
- People who get the vaccine will not get HPV.
- The HPV vaccine will protect people against cancer.
Experimental Design
You’ve successfully identified a hypothesis for the University of Washington’s study on HPV: People who get the HPV vaccine will not get HPV.
The next step is to design an experiment that will test this hypothesis. There are several important factors to consider when designing a scientific experiment. First, scientific experiments must have an experimental group. This is the group that receives the experimental treatment necessary to address the hypothesis.
The experimental group receives the vaccine, but how can we know if the vaccine made a difference? Many things may change HPV infection rates in a group of people over time. To clearly show that the vaccine was effective in helping the experimental group, we need to include in our study an otherwise similar control group that does not get the treatment. We can then compare the two groups and determine if the vaccine made a difference. The control group shows us what happens in the absence of the factor under study.
However, the control group cannot get “nothing.” Instead, the control group often receives a placebo. A placebo is a procedure that has no expected therapeutic effect—such as giving a person a sugar pill or a shot containing only plain saline solution with no drug. Scientific studies have shown that the “placebo effect” can alter experimental results because when individuals are told that they are or are not being treated, this knowledge can alter their actions or their emotions, which can then alter the results of the experiment.
Moreover, if the doctor knows which group a patient is in, this can also influence the results of the experiment. Without saying so directly, the doctor may show—through body language or other subtle cues—their views about whether the patient is likely to get well. These errors can then alter the patient’s experience and change the results of the experiment. Therefore, many clinical studies are “double blind.” In these studies, neither the doctor nor the patient knows which group the patient is in until all experimental results have been collected.
Both placebo treatments and double-blind procedures are designed to prevent bias. Bias is any systematic error that makes a particular experimental outcome more or less likely. Errors can happen in any experiment: people make mistakes in measurement, instruments fail, computer glitches can alter data. But most such errors are random and don’t favor one outcome over another. Patients’ belief in a treatment can make it more likely to appear to “work.” Placebos and double-blind procedures are used to level the playing field so that both groups of study subjects are treated equally and share similar beliefs about their treatment.
The scientists who are researching the effectiveness of the HPV vaccine will test their hypothesis by separating 2,392 young women into two groups: the control group and the experimental group. Answer the following questions about these two groups.
- This group is given a placebo.
- This group is deliberately infected with HPV.
- This group is given nothing.
- This group is given the HPV vaccine.
- a: This group is given a placebo. A placebo will be a shot, just like the HPV vaccine, but it will have no active ingredient. It may change peoples’ thinking or behavior to have such a shot given to them, but it will not stimulate the immune systems of the subjects in the same way as predicted for the vaccine itself.
- d: This group is given the HPV vaccine. The experimental group will receive the HPV vaccine and researchers will then be able to see if it works, when compared to the control group.
Experimental Variables
A variable is a characteristic of a subject (in this case, of a person in the study) that can vary over time or among individuals. Sometimes a variable takes the form of a category, such as male or female; often a variable can be measured precisely, such as body height. Ideally, only one variable is different between the control group and the experimental group in a scientific experiment. Otherwise, the researchers will not be able to determine which variable caused any differences seen in the results. For example, imagine that the people in the control group were, on average, much more sexually active than the people in the experimental group. If, at the end of the experiment, the control group had a higher rate of HPV infection, could you confidently determine why? Maybe the experimental subjects were protected by the vaccine, but maybe they were protected by their low level of sexual contact.
To avoid this situation, experimenters make sure that their subject groups are as similar as possible in all variables except for the variable that is being tested in the experiment. This variable, or factor, will be deliberately changed in the experimental group. The one variable that is different between the two groups is called the independent variable. An independent variable is known or hypothesized to cause some outcome. Imagine an educational researcher investigating the effectiveness of a new teaching strategy in a classroom. The experimental group receives the new teaching strategy, while the control group receives the traditional strategy. It is the teaching strategy that is the independent variable in this scenario. In an experiment, the independent variable is the variable that the scientist deliberately changes or imposes on the subjects.
Dependent variables are known or hypothesized consequences; they are the effects that result from changes or differences in an independent variable. In an experiment, the dependent variables are those that the scientist measures before, during, and particularly at the end of the experiment to see if they have changed as expected. The dependent variable must be stated so that it is clear how it will be observed or measured. Rather than comparing “learning” among students (which is a vague and difficult to measure concept), an educational researcher might choose to compare test scores, which are very specific and easy to measure.
In any real-world example, many, many variables MIGHT affect the outcome of an experiment, yet only one or a few independent variables can be tested. Other variables must be kept as similar as possible between the study groups and are called control variables . For our educational research example, if the control group consisted only of people between the ages of 18 and 20 and the experimental group contained people between the ages of 30 and 35, we would not know if it was the teaching strategy or the students’ ages that played a larger role in the results. To avoid this problem, a good study will be set up so that each group contains students with a similar age profile. In a well-designed educational research study, student age will be a controlled variable, along with other possibly important factors like gender, past educational achievement, and pre-existing knowledge of the subject area.
What is the independent variable in this experiment?
- Sex (all of the subjects will be female)
- Presence or absence of the HPV vaccine
- Presence or absence of HPV (the virus)
List three control variables other than age.
What is the dependent variable in this experiment?
- Sex (male or female)
- Rates of HPV infection
- Age (years)
Contribute!
Improve this page Learn More
- Revision and adaptation. Authored by : Shelli Carter and Lumen Learning. Provided by : Lumen Learning. License : CC BY-NC-SA: Attribution-NonCommercial-ShareAlike
- Scientific Inquiry. Provided by : Open Learning Initiative. Located at : https://oli.cmu.edu/jcourse/workbook/activity/page?context=434a5c2680020ca6017c03488572e0f8 . Project : Introduction to Biology (Open + Free). License : CC BY-NC-SA: Attribution-NonCommercial-ShareAlike
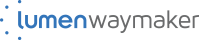
Writing an Introduction for a Scientific Paper
Dr. michelle harris, dr. janet batzli, biocore.
This section provides guidelines on how to construct a solid introduction to a scientific paper including background information, study question , biological rationale, hypothesis , and general approach . If the Introduction is done well, there should be no question in the reader’s mind why and on what basis you have posed a specific hypothesis.
Broad Question : based on an initial observation (e.g., “I see a lot of guppies close to the shore. Do guppies like living in shallow water?”). This observation of the natural world may inspire you to investigate background literature or your observation could be based on previous research by others or your own pilot study. Broad questions are not always included in your written text, but are essential for establishing the direction of your research.
Background Information : key issues, concepts, terminology, and definitions needed to understand the biological rationale for the experiment. It often includes a summary of findings from previous, relevant studies. Remember to cite references, be concise, and only include relevant information given your audience and your experimental design. Concisely summarized background information leads to the identification of specific scientific knowledge gaps that still exist. (e.g., “No studies to date have examined whether guppies do indeed spend more time in shallow water.”)
Testable Question : these questions are much more focused than the initial broad question, are specific to the knowledge gap identified, and can be addressed with data. (e.g., “Do guppies spend different amounts of time in water <1 meter deep as compared to their time in water that is >1 meter deep?”)
Biological Rationale : describes the purpose of your experiment distilling what is known and what is not known that defines the knowledge gap that you are addressing. The “BR” provides the logic for your hypothesis and experimental approach, describing the biological mechanism and assumptions that explain why your hypothesis should be true.
The biological rationale is based on your interpretation of the scientific literature, your personal observations, and the underlying assumptions you are making about how you think the system works. If you have written your biological rationale, your reader should see your hypothesis in your introduction section and say to themselves, “Of course, this hypothesis seems very logical based on the rationale presented.”
- A thorough rationale defines your assumptions about the system that have not been revealed in scientific literature or from previous systematic observation. These assumptions drive the direction of your specific hypothesis or general predictions.
- Defining the rationale is probably the most critical task for a writer, as it tells your reader why your research is biologically meaningful. It may help to think about the rationale as an answer to the questions— how is this investigation related to what we know, what assumptions am I making about what we don’t yet know, AND how will this experiment add to our knowledge? *There may or may not be broader implications for your study; be careful not to overstate these (see note on social justifications below).
- Expect to spend time and mental effort on this. You may have to do considerable digging into the scientific literature to define how your experiment fits into what is already known and why it is relevant to pursue.
- Be open to the possibility that as you work with and think about your data, you may develop a deeper, more accurate understanding of the experimental system. You may find the original rationale needs to be revised to reflect your new, more sophisticated understanding.
- As you progress through Biocore and upper level biology courses, your rationale should become more focused and matched with the level of study e ., cellular, biochemical, or physiological mechanisms that underlie the rationale. Achieving this type of understanding takes effort, but it will lead to better communication of your science.
***Special note on avoiding social justifications: You should not overemphasize the relevance of your experiment and the possible connections to large-scale processes. Be realistic and logical —do not overgeneralize or state grand implications that are not sensible given the structure of your experimental system. Not all science is easily applied to improving the human condition. Performing an investigation just for the sake of adding to our scientific knowledge (“pure or basic science”) is just as important as applied science. In fact, basic science often provides the foundation for applied studies.
Hypothesis / Predictions : specific prediction(s) that you will test during your experiment. For manipulative experiments, the hypothesis should include the independent variable (what you manipulate), the dependent variable(s) (what you measure), the organism or system , the direction of your results, and comparison to be made.
If you are doing a systematic observation , your hypothesis presents a variable or set of variables that you predict are important for helping you characterize the system as a whole, or predict differences between components/areas of the system that help you explain how the system functions or changes over time.
Experimental Approach : Briefly gives the reader a general sense of the experiment, the type of data it will yield, and the kind of conclusions you expect to obtain from the data. Do not confuse the experimental approach with the experimental protocol . The experimental protocol consists of the detailed step-by-step procedures and techniques used during the experiment that are to be reported in the Methods and Materials section.
Some Final Tips on Writing an Introduction
- As you progress through the Biocore sequence, for instance, from organismal level of Biocore 301/302 to the cellular level in Biocore 303/304, we expect the contents of your “Introduction” paragraphs to reflect the level of your coursework and previous writing experience. For example, in Biocore 304 (Cell Biology Lab) biological rationale should draw upon assumptions we are making about cellular and biochemical processes.
- Be Concise yet Specific: Remember to be concise and only include relevant information given your audience and your experimental design. As you write, keep asking, “Is this necessary information or is this irrelevant detail?” For example, if you are writing a paper claiming that a certain compound is a competitive inhibitor to the enzyme alkaline phosphatase and acts by binding to the active site, you need to explain (briefly) Michaelis-Menton kinetics and the meaning and significance of Km and Vmax. This explanation is not necessary if you are reporting the dependence of enzyme activity on pH because you do not need to measure Km and Vmax to get an estimate of enzyme activity.
- Another example: if you are writing a paper reporting an increase in Daphnia magna heart rate upon exposure to caffeine you need not describe the reproductive cycle of magna unless it is germane to your results and discussion. Be specific and concrete, especially when making introductory or summary statements.
Where Do You Discuss Pilot Studies? Many times it is important to do pilot studies to help you get familiar with your experimental system or to improve your experimental design. If your pilot study influences your biological rationale or hypothesis, you need to describe it in your Introduction. If your pilot study simply informs the logistics or techniques, but does not influence your rationale, then the description of your pilot study belongs in the Materials and Methods section.
How will introductions be evaluated? The following is part of the rubric we will be using to evaluate your papers.
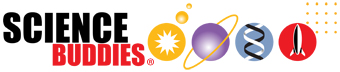
- Science Projects
- Project Guides
- STEM Activities
- Lesson Plans
- Video Lessons
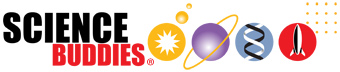
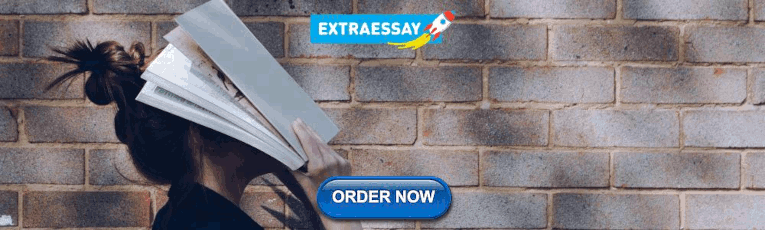
Writing a Hypothesis for Your Science Fair Project
What is a hypothesis.
When a scientist has a question they want to answer, they research what is already known about the topic. Then, they come up with their best answer to the question based on what they know. This is called a hypothesis. The hypothesis has to be testable. A scientist must be able to do an experiment to test if the hypothesis is a good answer to the question.
The hypothesis also leads to predictions, which are guesses about what will happen in the experiments. These predictions usually have the pattern "If ____ then ____". The predictions include what the scientist will change in the experiment (called the independent variable) and what they will observe or measure (called the dependent variable). A hypothesis can have more than one prediction, but usually one or two predictions are enough for a science project.
Examples of Hypotheses and Predictions
What if my hypothesis is wrong.
What if your science project data does not match your predictions? Is it bad that your hypothesis is wrong? Don't worry! The goal of a science project is not to prove your hypothesis right or wrong. The goal is to learn more about how the natural world works. Even in a science fair, judges can be impressed by a project that started with a bad hypothesis. What matters is that you understood your project, did a good experiment, and have ideas for how to make it better.
In fact, scientists never say their hypothesis is, right or wrong . Instead, they say that their experiment supports or does not support their hypothesis. This is because nature is complex, so it takes more than one experiment to figure everything out. For example, let's say you make a hypothesis that earthworms can't survive in places with cold winters. You predict that you'll find earthworms in Florida, but not in Alaska. When you dig in both places, you find earthworms in Florida, but not in Alaska. Your data supports your hypothesis, but you can't be sure there are no earthworms in Alaska. After all, you only searched a very small amount of all the dirt in Alaska. That's why scientists say the data supports their hypothesis instead of saying it proves their hypothesis. And just so you know, there are earthworms in Alaska!
What Makes a Good Hypothesis?
Assign these with Google Classroom:
- Hypothesis Quiz
- My Science Project Hypothesis (Student Worksheet)
Explore Our Science Videos
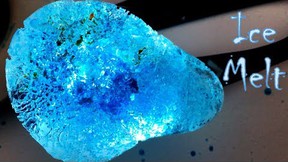
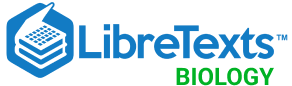
- school Campus Bookshelves
- menu_book Bookshelves
- perm_media Learning Objects
- login Login
- how_to_reg Request Instructor Account
- hub Instructor Commons
- Download Page (PDF)
- Download Full Book (PDF)
- Periodic Table
- Physics Constants
- Scientific Calculator
- Reference & Cite
- Tools expand_more
- Readability
selected template will load here
This action is not available.
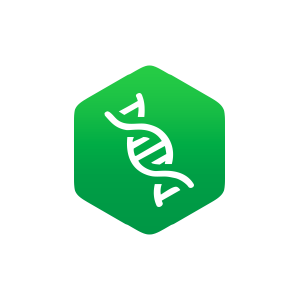
3.3: Putting it all together- Inferential statistics and hypothesis testing
- Last updated
- Save as PDF
- Page ID 32141
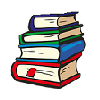
- Melissa Ha and Rachel Schleiger
- Yuba College & Butte College via ASCCC Open Educational Resources Initiative
What is a hypothesis and are there different kinds?
Biological (Scientific) hypothesis: An idea that proposes a tentative explanation about a phenomenon or a narrow set of phenomena observed in the natural world. This is the backbone of all scientific inquiry! As such it is important to have a solid biological hypothesis before moving forward in the scientific method (i.e. procedures, results, discussion). After the creation of a solid biological hypothesis, it can then be simplified into a statistical hypothesis (as defined below) that will become the basis for how the data will be analyzed and interpreted.
Statistical hypotheses: After defining a strong biological hypothesis, a statistical hypothesis can be created based on what you will predict will be the measured outcome(s) (dependent variable(s)). If a study has multiple measured outcomes there can be multiple statistical hypotheses. Each statistical hypothesis will have two components (Null and Alternative).
- Null hypothesis (Ho) –This hypothesis states that there is no relationship (or no pattern) between the independent and dependent variables.
- Alternative hypothesis (H1) – This hypothesis states that there is a relationship (or is a pattern) between the independent and dependent variables.
Independent versus dependent variables: For both biological and statistical hypotheses there should be two basic variables defined:
- Independent (explanatory) variable – It is usually what phenomena you think will affect the measure you are interested in (dependent variable).
- Dependent (response) variable – A dependent variable is what you measure in the experiment and what is affected during the experiment. The dependent variable responds to (depends on) the independent variable. In a scientific experiment, you cannot have a dependent variable without an independent variable.
Yellow-billed Cuckoo nests were counted during breeding season in degraded, restored, and intact riparian habitats to see overall habitat preference for nesting sites increased with habitat health.
- Scientific hypothesis: Yellow-billed Cuckoo will have habitat preferences because of habitat health/status.
- Statistical hypotheses: (Ho) There will be no differences in number of nests between habitats with different health/status. (H1) There will be more nests in restored and intact habitats compared to degraded.
- Independent variable = Habitat health/status
- Dependent variable = Number of nests counted
How do you make conclusions?
Finally, after defining the biological hypothesis, statistical hypothesis, and collecting all your data, a researcher can begin statistical analysis. A statistical test will mathematically “test” your data against the statistical hypothesis. The type of statistical test that is used depends on the type and quantity of variables in the study, as well as the question the researcher wants to ask. After computing the statistical test, the outcome will indicate which statistical hypothesis is more likely. This, in turn indicates to scientists what level of inference can be gained from the data compared to the biological hypothesis (the focus point of the study). Then a conclusion can be made based on the sample about the entire population. It is important to note that the process does not stop here. Scientists will want to continue to test this conclusion until a clear pattern emerges (or not) or to investigate similar but different questions.
Attribution
Rachel Schleiger ( CC-BY-NC )
If you're seeing this message, it means we're having trouble loading external resources on our website.
If you're behind a web filter, please make sure that the domains *.kastatic.org and *.kasandbox.org are unblocked.
To log in and use all the features of Khan Academy, please enable JavaScript in your browser.
AP®︎/College Biology
Course: ap®︎/college biology > unit 7.
- Taxonomy and the tree of life
- Discovering the tree of life
- Understanding and building phylogenetic trees
- Phylogenetic trees
Building a phylogenetic tree
Key points:.
- Phylogenetic trees represent hypotheses about the evolutionary relationships among a group of organisms.
- A phylogenetic tree may be built using morphological (body shape), biochemical, behavioral, or molecular features of species or other groups.
- In building a tree, we organize species into nested groups based on shared derived traits (traits different from those of the group's ancestor).
- The sequences of genes or proteins can be compared among species and used to build phylogenetic trees. Closely related species typically have few sequence differences, while less related species tend to have more.
Introduction
Overview of phylogenetic trees, the idea behind tree construction, example: building a phylogenetic tree.
- In the context of homework or a test, the question you are solving may tell you which traits are derived vs. ancestral.
- If you are doing your own research, you may have knowledge that allows you identify ancestral and derived traits (e.g., based on fossils).
- You may be given information about an outgroup , a species that's more distantly related to the species of interest than they are to one another.
Parsimony and pitfalls in tree construction
- We may not always be able to distinguish features that reflect shared ancestry ( homologous features) from features that are similar but arose independently ( analogous features arising by convergent evolution ). See an example Imagine that the tree below shows the actual evolutionary history of a group of rodents. In this tree, whiskers arise two independent times. If we didn't know the true history of the group and were trying to reconstruct it, we might interpret the whiskers as arising from a single event. The whisker data would then conflict with data for the other traits. A diagonal phylogenetic tree, labeled Convergent evolution of whiskers. The root splits at a point labeled Most recent common ancestor of A through E, to form two branches. A drawing of a rat-like creature is shown at this point. The left branch forms species A with the same drawing of a rat-like creature (no whiskers, no fuzzy tail, no big ears). The right branch passes through a point labeled Fuzzy tail (in green) and splits to form a pair of branches. From there, the left branch passes through a point labeled Whiskers (1) (in purple) and forms species B with a drawing of a rat-like creature with a fuzzy tail and whiskers. The right branch passes through a point labeled Big ears (in blue) and splits to form a pair of branches. From there, the left branch forms species C with a drawing of a rat-like creature with a fuzzy tail and big ears, no whiskers. The right branch passes through a point labeled Whiskers (2) (in purple) and splits to form species D and E. Species D and E have identical drawings of a rat-like creature with a fuzzy tail, big ears, and whiskers. The letters and drawings are labeled Present-day species.
- Traits can be gained and lost multiple times over the evolutionary history of a species. A species may have a derived trait, but then lose that trait (revert back to the ancestral form) over the course of evolution. See an example Imagine that the tree below shows the actual evolutionary history of a group of rodents. In this tree, species E undergoes a genetic change that causes it to lose its bushy tail and gain the skinny tail present in the group's ancestor. If we didn't know the true history of the group and were trying to reconstruct it, we might assume that the species E was descended from an ancestor without a bushy tail. Under this assumption, the tail data would conflict with data for other traits. A diagonal phylogenetic tree, labeled Gain and loss of fuzzy tail. The root splits at a point labeled Most recent common ancestor of A through E, to form two branches. A drawing of a rat-like creature is shown at this point. The left branch forms species A with the same drawing of a rat-like creature (no whiskers, no fuzzy tail, no big ears). The right branch passes through a point labeled Fuzzy tail (in green) and splits to form a pair of branches. From there, the left branch forms species B with a drawing of a rat-like creature with a fuzzy tail. The right branch passes through a point labeled Big ears (in blue) and splits to form a pair of branches. From there, the left branch forms species C with a drawing of a rat-like creature with a fuzzy tail and big ears. The right branch passes through a point labeled Whiskers (in purple) and splits to form two branches. From there, the left branch forms species D with a drawing of a rat-like creature with a fuzzy tail, big ears, and whiskers. The right branch passes through a point labeled Skinny tail (reversion) and forms species E with a drawing of a rat-like creature with a skinny tail, big ears, and whiskers. The letters and drawings are labeled Present-day species.
Using molecular data to build trees
- A larger number of differences corresponds to less related species
- A smaller number of differences corresponds to more related species
Attribution
Works cited.
- David Baum, "Reading a Phylogenetic Tree: The Meaning of Monophyletic Groups," Nature Education 1, no. 1 (2008): 190, http://www.nature.com/scitable/topicpage/reading-a-phylogenetic-tree-the-meaning-of-41956 .
Want to join the conversation?
- Upvote Button navigates to signup page
- Downvote Button navigates to signup page
- Flag Button navigates to signup page

Want to create or adapt books like this? Learn more about how Pressbooks supports open publishing practices.
Developing a Hypothesis
Rajiv S. Jhangiani; I-Chant A. Chiang; Carrie Cuttler; and Dana C. Leighton
Learning Objectives
- Distinguish between a theory and a hypothesis.
- Discover how theories are used to generate hypotheses and how the results of studies can be used to further inform theories.
- Understand the characteristics of a good hypothesis.
Theories and Hypotheses
Before describing how to develop a hypothesis, it is important to distinguish between a theory and a hypothesis. A theory is a coherent explanation or interpretation of one or more phenomena. Although theories can take a variety of forms, one thing they have in common is that they go beyond the phenomena they explain by including variables, structures, processes, functions, or organizing principles that have not been observed directly. Consider, for example, Zajonc’s theory of social facilitation and social inhibition (1965) [1] . He proposed that being watched by others while performing a task creates a general state of physiological arousal, which increases the likelihood of the dominant (most likely) response. So for highly practiced tasks, being watched increases the tendency to make correct responses, but for relatively unpracticed tasks, being watched increases the tendency to make incorrect responses. Notice that this theory—which has come to be called drive theory—provides an explanation of both social facilitation and social inhibition that goes beyond the phenomena themselves by including concepts such as “arousal” and “dominant response,” along with processes such as the effect of arousal on the dominant response.
Outside of science, referring to an idea as a theory often implies that it is untested—perhaps no more than a wild guess. In science, however, the term theory has no such implication. A theory is simply an explanation or interpretation of a set of phenomena. It can be untested, but it can also be extensively tested, well supported, and accepted as an accurate description of the world by the scientific community. The theory of evolution by natural selection, for example, is a theory because it is an explanation of the diversity of life on earth—not because it is untested or unsupported by scientific research. On the contrary, the evidence for this theory is overwhelmingly positive and nearly all scientists accept its basic assumptions as accurate. Similarly, the “germ theory” of disease is a theory because it is an explanation of the origin of various diseases, not because there is any doubt that many diseases are caused by microorganisms that infect the body.
A hypothesis , on the other hand, is a specific prediction about a new phenomenon that should be observed if a particular theory is accurate. It is an explanation that relies on just a few key concepts. Hypotheses are often specific predictions about what will happen in a particular study. They are developed by considering existing evidence and using reasoning to infer what will happen in the specific context of interest. Hypotheses are often but not always derived from theories. So a hypothesis is often a prediction based on a theory but some hypotheses are a-theoretical and only after a set of observations have been made, is a theory developed. This is because theories are broad in nature and they explain larger bodies of data. So if our research question is really original then we may need to collect some data and make some observations before we can develop a broader theory.
Theories and hypotheses always have this if-then relationship. “ If drive theory is correct, then cockroaches should run through a straight runway faster, and a branching runway more slowly, when other cockroaches are present.” Although hypotheses are usually expressed as statements, they can always be rephrased as questions. “Do cockroaches run through a straight runway faster when other cockroaches are present?” Thus deriving hypotheses from theories is an excellent way of generating interesting research questions.
But how do researchers derive hypotheses from theories? One way is to generate a research question using the techniques discussed in this chapter and then ask whether any theory implies an answer to that question. For example, you might wonder whether expressive writing about positive experiences improves health as much as expressive writing about traumatic experiences. Although this question is an interesting one on its own, you might then ask whether the habituation theory—the idea that expressive writing causes people to habituate to negative thoughts and feelings—implies an answer. In this case, it seems clear that if the habituation theory is correct, then expressive writing about positive experiences should not be effective because it would not cause people to habituate to negative thoughts and feelings. A second way to derive hypotheses from theories is to focus on some component of the theory that has not yet been directly observed. For example, a researcher could focus on the process of habituation—perhaps hypothesizing that people should show fewer signs of emotional distress with each new writing session.
Among the very best hypotheses are those that distinguish between competing theories. For example, Norbert Schwarz and his colleagues considered two theories of how people make judgments about themselves, such as how assertive they are (Schwarz et al., 1991) [2] . Both theories held that such judgments are based on relevant examples that people bring to mind. However, one theory was that people base their judgments on the number of examples they bring to mind and the other was that people base their judgments on how easily they bring those examples to mind. To test these theories, the researchers asked people to recall either six times when they were assertive (which is easy for most people) or 12 times (which is difficult for most people). Then they asked them to judge their own assertiveness. Note that the number-of-examples theory implies that people who recalled 12 examples should judge themselves to be more assertive because they recalled more examples, but the ease-of-examples theory implies that participants who recalled six examples should judge themselves as more assertive because recalling the examples was easier. Thus the two theories made opposite predictions so that only one of the predictions could be confirmed. The surprising result was that participants who recalled fewer examples judged themselves to be more assertive—providing particularly convincing evidence in favor of the ease-of-retrieval theory over the number-of-examples theory.
Theory Testing
The primary way that scientific researchers use theories is sometimes called the hypothetico-deductive method (although this term is much more likely to be used by philosophers of science than by scientists themselves). Researchers begin with a set of phenomena and either construct a theory to explain or interpret them or choose an existing theory to work with. They then make a prediction about some new phenomenon that should be observed if the theory is correct. Again, this prediction is called a hypothesis. The researchers then conduct an empirical study to test the hypothesis. Finally, they reevaluate the theory in light of the new results and revise it if necessary. This process is usually conceptualized as a cycle because the researchers can then derive a new hypothesis from the revised theory, conduct a new empirical study to test the hypothesis, and so on. As Figure 2.3 shows, this approach meshes nicely with the model of scientific research in psychology presented earlier in the textbook—creating a more detailed model of “theoretically motivated” or “theory-driven” research.
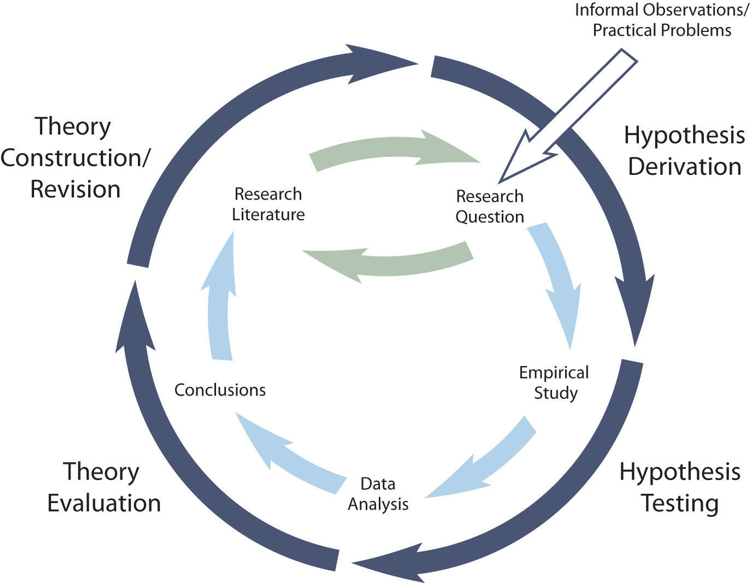
As an example, let us consider Zajonc’s research on social facilitation and inhibition. He started with a somewhat contradictory pattern of results from the research literature. He then constructed his drive theory, according to which being watched by others while performing a task causes physiological arousal, which increases an organism’s tendency to make the dominant response. This theory predicts social facilitation for well-learned tasks and social inhibition for poorly learned tasks. He now had a theory that organized previous results in a meaningful way—but he still needed to test it. He hypothesized that if his theory was correct, he should observe that the presence of others improves performance in a simple laboratory task but inhibits performance in a difficult version of the very same laboratory task. To test this hypothesis, one of the studies he conducted used cockroaches as subjects (Zajonc, Heingartner, & Herman, 1969) [3] . The cockroaches ran either down a straight runway (an easy task for a cockroach) or through a cross-shaped maze (a difficult task for a cockroach) to escape into a dark chamber when a light was shined on them. They did this either while alone or in the presence of other cockroaches in clear plastic “audience boxes.” Zajonc found that cockroaches in the straight runway reached their goal more quickly in the presence of other cockroaches, but cockroaches in the cross-shaped maze reached their goal more slowly when they were in the presence of other cockroaches. Thus he confirmed his hypothesis and provided support for his drive theory. (Zajonc also showed that drive theory existed in humans [Zajonc & Sales, 1966] [4] in many other studies afterward).
Incorporating Theory into Your Research
When you write your research report or plan your presentation, be aware that there are two basic ways that researchers usually include theory. The first is to raise a research question, answer that question by conducting a new study, and then offer one or more theories (usually more) to explain or interpret the results. This format works well for applied research questions and for research questions that existing theories do not address. The second way is to describe one or more existing theories, derive a hypothesis from one of those theories, test the hypothesis in a new study, and finally reevaluate the theory. This format works well when there is an existing theory that addresses the research question—especially if the resulting hypothesis is surprising or conflicts with a hypothesis derived from a different theory.
To use theories in your research will not only give you guidance in coming up with experiment ideas and possible projects, but it lends legitimacy to your work. Psychologists have been interested in a variety of human behaviors and have developed many theories along the way. Using established theories will help you break new ground as a researcher, not limit you from developing your own ideas.
Characteristics of a Good Hypothesis
There are three general characteristics of a good hypothesis. First, a good hypothesis must be testable and falsifiable . We must be able to test the hypothesis using the methods of science and if you’ll recall Popper’s falsifiability criterion, it must be possible to gather evidence that will disconfirm the hypothesis if it is indeed false. Second, a good hypothesis must be logical. As described above, hypotheses are more than just a random guess. Hypotheses should be informed by previous theories or observations and logical reasoning. Typically, we begin with a broad and general theory and use deductive reasoning to generate a more specific hypothesis to test based on that theory. Occasionally, however, when there is no theory to inform our hypothesis, we use inductive reasoning which involves using specific observations or research findings to form a more general hypothesis. Finally, the hypothesis should be positive. That is, the hypothesis should make a positive statement about the existence of a relationship or effect, rather than a statement that a relationship or effect does not exist. As scientists, we don’t set out to show that relationships do not exist or that effects do not occur so our hypotheses should not be worded in a way to suggest that an effect or relationship does not exist. The nature of science is to assume that something does not exist and then seek to find evidence to prove this wrong, to show that it really does exist. That may seem backward to you but that is the nature of the scientific method. The underlying reason for this is beyond the scope of this chapter but it has to do with statistical theory.
- Zajonc, R. B. (1965). Social facilitation. Science, 149 , 269–274 ↵
- Schwarz, N., Bless, H., Strack, F., Klumpp, G., Rittenauer-Schatka, H., & Simons, A. (1991). Ease of retrieval as information: Another look at the availability heuristic. Journal of Personality and Social Psychology, 61 , 195–202. ↵
- Zajonc, R. B., Heingartner, A., & Herman, E. M. (1969). Social enhancement and impairment of performance in the cockroach. Journal of Personality and Social Psychology, 13 , 83–92. ↵
- Zajonc, R.B. & Sales, S.M. (1966). Social facilitation of dominant and subordinate responses. Journal of Experimental Social Psychology, 2 , 160-168. ↵
A coherent explanation or interpretation of one or more phenomena.
A specific prediction about a new phenomenon that should be observed if a particular theory is accurate.
A cyclical process of theory development, starting with an observed phenomenon, then developing or using a theory to make a specific prediction of what should happen if that theory is correct, testing that prediction, refining the theory in light of the findings, and using that refined theory to develop new hypotheses, and so on.
The ability to test the hypothesis using the methods of science and the possibility to gather evidence that will disconfirm the hypothesis if it is indeed false.
Developing a Hypothesis Copyright © 2022 by Rajiv S. Jhangiani; I-Chant A. Chiang; Carrie Cuttler; and Dana C. Leighton is licensed under a Creative Commons Attribution-NonCommercial-ShareAlike 4.0 International License , except where otherwise noted.
Share This Book

An official website of the United States government
The .gov means it’s official. Federal government websites often end in .gov or .mil. Before sharing sensitive information, make sure you’re on a federal government site.
The site is secure. The https:// ensures that you are connecting to the official website and that any information you provide is encrypted and transmitted securely.
- Publications
- Account settings
Preview improvements coming to the PMC website in October 2024. Learn More or Try it out now .
- Advanced Search
- Journal List
- PMC10739548

Quantitative Modelling in Stem Cell Biology and Beyond: How to Make Best Use of It
Philip greulich.
1 School of Mathematical Sciences, University of Southampton, Southampton, UK
2 Institute for Life Sciences, University of Southampton, Southampton, UK
Purpose of Review
This article gives a broad overview of quantitative modelling approaches in biology and provides guidance on how to employ them to boost stem cell research, by helping to answer biological questions and to predict the outcome of biological processes.
Recent Findings
The twenty-first century has seen a steady increase in the proportion of cell biology publications employing mathematical modelling to aid experimental research. However, quantitative modelling is often used as a rather decorative element to confirm experimental findings, an approach which often yields only marginal added value, and is in many cases scientifically questionable.
Quantitative modelling can boost biological research in manifold ways, but one has to take some careful considerations before embarking on a modelling campaign, in order to maximise its added value, to avoid pitfalls that may lead to wrong results, and to be aware of its fundamental limitations, imposed by the risks of over-fitting and “universality”.
Introduction
Quantitative modelling is the use of mathematical or computational means to imitate real-world processes and to predict their outcomes. Historically, it has been used for centuries in the form of fundamental physical laws, such as Newton’s laws, while biological problems have been subject to mathematical modelling since the first half of the twentieth century. An example is the work of Luria and Delbrück in 1943 who employed mathematical models, tested on bacterial population data, to find that mutations occur without selection pressure [ 1 ]. Yet, for a long time, quantitative modelling has not been seen as a core ingredient of biological research. Only in the twenty-first century, the use of quantitative modelling has become ubiquitous, and the way how it is being viewed by the experimental biologists community has turned from widespread scepticism to being a staple ingredient in modern biological research papers, in particular, in developmental and stem cell biology [ 2 – 5 ].
While quantitative modelling is employed in a wide range of biological work, researchers may not always be fully aware how to utilise the opportunities it offers in a best way. Modelling is often seen as a way to confirm experimental findings by reproducing them in silico , and having a “model” for the sake of it is perceived as a way to boost a paper’s impact. However, when modelling is used as a mostly decorative element for an already accomplished experimental campaign, the actual benefit of it is often marginal and sometimes questionable at all.
When considering whether and how to use quantitative modelling in a biological context, one should ask three questions: (1) is there anything quantitative modelling can do which cannot be done by experimental means and standard statistical inference; i.e. can it provide sufficient added value? (2) Can modelling replace experiments and can thereby cut costs and save staff time? (3) If modelling could provide added value, how do I employ it effectively, to maximise the scientific value of the work? In the following, I wish to give some ideas and guidance on how to approach these questions and how to make best use of computational and mathematical modelling.
When assessing whether quantitative modelling can provide added value to a biological research project, one should first define what its purpose is. The most common purposes are the following:
- To confirm a hypothesis previously suggested experimentally, by fitting the corresponding model to the experimental data and reproducing it faithfully.
- To predict the outcome of a biological process, e.g. gene expression, protein folding, or morphogenesis.
- To answer research questions and test hypotheses, if this cannot be done by plain experimental means and standard statistical inference methods.
- To study intrinsic properties of models and relationships between different models theoretically.
In addition to those purposes come others which are nowadays implemented in established software and are often not explicitly perceived as “modelling”, like data clustering, inference of gene regulatory networks [ 6 – 8 ], and cell fate trajectory inference [ 9 – 12 ]. While those often seem to be straightforward statistical methods, they actually involve sophisticated models and model fitting, which require certain assumptions to be met and hyperparameters to be tuned.
In the following, I wish to assess under which circumstances quantitative modelling is fit for those purposes and how to maximise its added value.
Reconfirm an Experimental Finding by Modelling
When an experiment has delivered some interesting results, and a hypothesis has been suggested and tested through standard test statistics, one could be tempted to have a quantitative model representing that hypothesis to reproduce the experimental data. However, the added value of such an approach is questionable. On the one hand, if a hypothesis has been tested experimentally, having in addition a mathematical model of that hypothesis matching the data provides only a marginally improved certainty of this hypothesis. While modelling can be used to increase the certainty of a hypothesis, computing such a certainty requires not only to test the model of the suggested hypothesis itself, but also all other, competing but reasonable hypotheses need to be tested. 1 Otherwise, the added certainty from testing a mathematical model is undefined. On the other hand, having a fitted model matching the experimental data is by no means a guarantee that this model is correct. In particular, if the model is complex, with many free parameters, the model can easily be over-fitted , meaning that the parameters provide too much freedom for the model to be matched too closely to the data, fitting the noise rather than real trends [ 13 ]. The result is that a completely wrong model may fit the data. Even if the number of parameters is low, a model which may share some, but not necessarily the biologically crucial features with the correct model, may also fit the data (see discussion of “universality” in the section “ Universality: Curse and Opportunity ”. In both cases, such an approach not only has marginal benefit but may even yield wrong results, which when published stay in the public domain.
Predicting the Outcomes of Biological Processes
The second purpose is the prediction of the outcome of a biological process. Undeniably, quantitative models can be very powerful in achieving this, yet certain conditions need to be fulfilled to be successful. Most commonly, supervised machine learning is employed for this. Although often not seen as that, machine learning is also a form of mathematical modelling: for example, in deep learning, mathematical functions that resemble the connections of neurons ( artificial neural networks (ANN) ) are used, which are trained —that is, fitted—to data, in order to extrapolate trends from this data and thus to predict outcomes beyond the data regime [ 14 ]. Ironically, this usually leads to over-fitting of a “wrong model”, yet in a desired way: most of the time, the system to be predicted is not actually a neural network (for example, when predicting protein folding, such as by AlphaFold [ 15 ]), yet due to the vast number of parameters, those neural networks can fit almost any (labelled) data. However, despite over-fitting, machine learning yields excellent predictions—a feat that has not been entirely understood yet. 2 An additional method to improve the predictive power of ANNs is regularisation [ 16 ]: when fitting the ANN, large parameter variations are penalised in the evaluation of the fitting accuracy, so that the parameter space is effectively substantially reduced, leading to an excellent predictive power. Hence, the power of an ANN lies in its ability to be fitted to a large range of data, despite not representing the biological process on a model level. However, for machine learning to be predictive, it requires vast amounts of data, which usually only high-throughput assays, like next-generation sequencing, can deliver.
While powerful for predictions, machine learning approaches cannot reveal information about the underlying biology, since the models themselves do not reflect the biology (and are therefore also called a “blackbox” models). Furthermore, the predictions work only under the circumstances under which the training data has been collected; changes of circumstances, such as a change of phenotype from a mutation, or a change of experimental settings, cannot be predicted without new experimental data.
An alternative are mechanistic models that reflect directly the underlying real-world processes [ 17 ]. Such models describe underlying biological or biochemical processes in a mathematical form, usually either (1) in the form of differential equations, which express the time evolution of relevant biological quantities as their time derivatives on the one side, and explicit terms expressing the change rates of these quantities on the other side, or (2) as stochastic processes, which include some degree of randomness and whose predictions are probabilistic. Instead of providing a unique outcome, they predict a probability distribution of outcomes. The advantage of a mechanistic model, if trained appropriately and once tested to be predictive, is that it allows to test the effect of particular changes of circumstances, that is, changes in parameters, which can be expressed in terms of the model rules more easily than in a blackbox machine learning model. However, it is highly challenging to develop such a model. It must be complex enough to contain all relevant features, both for prediction and for comparability with the biological scenario, but at the same time, its free parameters must be few, to avoid over-fitting, since in such a case—in contrast to machine learning—fitting the wrong model would undermine the model’s purpose (even if it could be rendered predictive by regularisation). Hence, one can usually only use such a model if the majority of parameters are accurately known through measurements. An example is protein folding, where all relevant physico-chemical parameters of amino acid crosslinking and their thermal motion are accurately known. If not all parameters are known a priori, mechanistic models can rarely make quantitatively accurate de novo predictions, but are often able to predict certain qualitative behaviours of a system, for example, whether a certain quantity varies smoothly or abruptly (in a step-change manner) under a change of external parameters [ 18 ], or predict characteristic properties of differentiation, based on bifurcation theory [ 19 ].
Overall, quantitative modelling—which includes machine learning—has great potential to predict the outcome of biological processes, but there are limitations: machine learning requires vast amounts of data (which, however, can be obtained by high-throughput technologies) and can only make predictions for circumstances under which the data has been collected, while mechanistic modelling—which reflects the underlying biology and allows to predict changes in circumstances—requires less data but much pre-existing knowledge about the relevant parameters.
Answer Biological Questions Through Quantitative Modelling
When attempting to answer a biological question, experimental means and direct hypothesis testing may not be sufficient to yield a clear answer. The experimental data may contain the information relevant to answer the question, but it may not be readily extractable through direct statistical tools. An example is the search for cell fate choice patterns using genetic cell lineage tracing [ 20 •, 21 – 23 ] via the Cre-Lox recombinase system [ 24 – 26 ]. The Cre-Lox system allows labelling of cells with fluorescent proteins in an inheritable way, i.e. all of a labelled cell’s progeny caries that fluorescent marker, which allows to trace individual clones (see Fig. 1 A). When tissue is harvested and clones analysed, one can obtain the full statistical distribution of clone sizes and their composition of cell (sub-)types if appropriate markers are available. The issue is that, while this statistical distribution is the result of the cell fate choices and thus contains some information about them, it cannot directly reveal the cell fate choice patterns. The problem is twofold: (1) the data is a snapshot of clonal distributions at particular time points, while the biological question is about a dynamical process, i.e. the changes of cell type over time and upon cell division, and (2) the data is multicellular, about cell populations (a clone is a sub-population of cells), while the biological question is about the fate of single cells and their daughters upon division. Hence, experimental data and the biological question, that is, any associated hypothesis, do not match in terms of scale of time (static vs. dynamic) and cell number (single- vs. multicellular).

Hypothesis testing via quantitative modelling, exemplified on clonal statistics. A Depiction of lineage tracing via Cre-Lox recombination and clonal statistics. Transgenic animals carry a GFP gene preceded by a Stop sequence that is flanked by Lox constructs. The Lox-flanked Stop sequence is removed by a Cre recombinase which is expressed upon administration of tamoxifen, and thus, GFP is expressed. The GFP label is inherited to the progeny of the initial cell, which constitutes a clone and which grows over time upon Cre recombination (centre top, ©2014 SpringerNature. Reprinted, with permission, from [ 28 ]). The statistical distributions of clone sizes is recorded (centre bottom, data from P. H. Jones as published in [ 29 ]), yet it cannot directly distinguish between hypotheses of cell fate outcome (bottom). B Quantitative modelling can bridge the gap between hypotheses and data: each hypothesis represents the rules for a stochastic model of cell fate choice dynamics, which predicts the hypothesis’ expected clonal statistics. The latter can then be directly compared with the experimental data and tested (bottom, data from P. H. Jones, as published in [ 29 ]). C , D Illustration of universality. C Two models of stem cell fate choice in homeostasis, which differ in some features, yet predict the same clone size distribution and are thus indistinguishable through static cell lineage tracing data [ 30 ] (plot on bottom: ©2019 SpringerNature. Reprinted, with permission, from [ 31 ]). D ~ 800 randomly generated cell fate models can be categorised in only two universality classes, one predicting an exponential distribution in the long term limit and the other one a normal distribution if mean clone sizes are large (plots reprinted on CC-BY license from [ 32 ••]). The two classes are distinguished by only one predictive relevant property, namely, whether the number of stem cells is strictly conserved or not
At this point, quantitative modelling can help. It can bridge the gap between biological question/hypotheses and the experimental data, as depicted in Fig. 1 B. The key is that every candidate hypothesis can be interpreted as the rules for a mechanistic model, which can be formulated and evaluated mathematically or computationally, as a set of differential equations (deterministic dynamics) or as a stochastic process (which includes random noise), to generate “virtual data”, as would be predicted by that hypothesis. That prediction can then be directly compared with the data and be tested on it.
As an example, let us again consider the search for cell fate choice patterns. Let us assume that we have measured clonal distributions experimentally; yet, we cannot directly see the cell fate choice rules from this data. We can, however, take the possible candidate hypotheses and translate each of them into the update rules of a stochastic process. Then, these models can be evaluated to produce predicted clonal (probability) distributions as output, and the parameters of the models can be fitted by some optimisation method. Those predicted clonal distributions can now be directly compared with the data. Hence, the mathematical modelling turns hypotheses that cannot be compared with the data, into predicted clonal distributions that can be directly overlaid with the data (see Fig. 1 B).
Now, if we find that a model’s output cannot be fitted to the data, we can reasonably reject the corresponding hypothesis. However, we have to be careful how to interpret a fitting model output. Can we confirm a hypothesis and settle the question about cell fate choice rules if a predicted clonal distribution fits the data? The answer is a clear “no”; a prediction fitting the data does not confirm a hypothesis, since other candidate hypotheses might as well predict the data equally well or better. And other than being the exception, this rather is the rule. On the one hand, over-fitting, as described before, can allow a wrong model to be fitted to the data if it is formulated with too many free parameters. 3 Hence, if one does not have the knowledge of all parameters in a possibly very complex biological process, the only reasonable option is to simplify the model so far that the number of parameters is low enough to avoid over-fitting. Thus, many aspects of an initially complex model may need to be neglected (for stochastic models, this simplification means essentially that neglected features are assumed to be random and unbiased, which is covered by a stochastic model’s random noise). On the other hand, often more than one model can fit the data, even if the number of parameters is sufficiently low, since some features that distinguish models may not affect the predictions at all. This phenomenon is often called “universality”, which we discuss in the following section.
Universality: Curse and Opportunity
Universality is the phenomenon that different models can sometimes generate the same predictions with respect to a certain type of data, if some quantities, like mean values or passed time, are sufficiently large [ 27 ]. Models which yield the same predictions have some common features, called “predictive relevant”, but may differ substantially in others, called “predictive irrelevant” features (notably, predictive irrelevant features may yet be biologically relevant). Models that differ only in predictive irrelevant features, i.e. yielding the same predictions, can be categorised in one “universality class”, while those that differ in predictive relevant features belong to different universality classes. This has the unfortunate consequence that hypotheses that correspond to models of the same universality class will fit the data equally well and thus cannot be distinguished when the corresponding models are tested against that data. From this also follows that a fitting model does not mean that it is the “correct” model if any of the predictive irrelevant features are biologically relevant for the posed biological question, since any other model of the same universality class, but which may differ in biologically relevant, yet predictive irrelevant features, could fit the data as well.
Universality can have several origins:
- Weak convergence [ 33 ]: for stochastic processes—which model some degree of randomness—the phenomenon of “weak convergence” means that they generate statistics that converge over time, or if mean numbers are large, to the same limiting distributions, if the predictive relevant features are the same. The most common of these universal limiting distributions is the normal distribution. There is a vast number of random numbers and stochastic processes which all produce a normal distribution and thus are of the same universality class; only few predictive relevant features must be fulfilled for this: (1) the final outcome of the process is a sum of individual steps/random quantities, and (2) the mean value and variance of each step are bounded [ 34 ]. Furthermore, the number of steps (interpreted as time steps in a stochastic process) must be large. Notably, any statistical features of individual step sizes, beyond the boundedness of mean and variance, are predictive irrelevant and do not affect outcomes, if the number of steps is large.
- Non-dimensionalisation : in both stochastic and deterministic models, quantities and parameters contain physical units, and these units can be arbitrarily chosen. For example, instead of using “seconds” as time unit, one may want to choose the inverse of the cell division rate as time unit, whereby the cell division rate becomes trivially “one division per time unit”. This can be done with other parameters as well, which thus become predictive irrelevant. By non-dimensionalisation , 4 several different models may actually map to the same non-dimensionalised model, with a common prediction, and those thus form the same universality class.
- Universality of critical phenomena 5 : complex systems with many interacting components may display critical phenomena, like phase transitions, (e.g. liquid to gas or liquid blood that becomes a solid blood clot). Sufficiently close to the critical points, many models that differ in some features—i.e. the predictive irrelevant features—predict the same functional behaviour of the quantities describing the collective properties of those systems [ 35 , 36 ]. The predictive relevant features are usually very few and often categorical, for example, which quantities are conserved, what symmetries prevail, and whether the configurations of the system are continuous or discrete (countable in integer numbers).
As an example, consider two cell fate models in homeostasis, as depicted in Fig. 1 C. In model 1, a stem cell (S) divides, and upon this division, the daughter cells irreversibly choose their fate, to either remain a stem cell until the next division or to commit to differentiation (C). In model 2, cell divisions are constrained to be always asymmetric, with one cell remaining a stem cell (S) and the other one being primed for differentiation (D), while the cell types may also change independently of cell division, in a reversible way, that is, an S-cell can become a D-cell and a D-cell can reverse to become an S-cell again [ 30 ]. Despite these fundamental differences, both models predict the same clone size distribution (Fig. 1 C, bottom). Why is this, and what are the predictive relevant features those models share? To answer these questions requires some mathematical analysis, on which we will elaborate later (see also a detailed analysis in Ref. [ 32 ••]).
Besides structural features of models, the parameters of a model can be predictive relevant or irrelevant. (Predictive) Irrelevant parameters are those which do not change the model predictions at all when changed under conditions where universality prevails; relevant parameters are those which affect the model predictions. However, a minimal set of relevant parameters does not necessarily include the plain model parameters; often, predictive relevant parameters are the product or ratio of plain model parameters rather than the parameters themselves. For example, in model 1 of Fig. 1 B, the predicted distribution of C-cells depends only on the ratio of division rate and terminal differentiation rate, not explicitly on the individual parameters themselves[ 20 •]. 6 If one has found a best set of parameter values, then doubling both the division rate and the terminal differentiation rate leads to the same best fit, and thus, the “true” best set of parameters is not identifiable [ 37 ].
But universality also provides opportunities and thus may be a desired property: if we only wish to distinguish predictive relevant features, and accept for now that we cannot distinguish the predictive irrelevant ones, we do not need to test all models of a universality class but can choose the simplest model—having the lowest number of parameters and being the easiest one to evaluate and analyse—as a representative of that class and thus simplify the whole modelling campaign substantially. Since the predictive features are often categorical, the number of universality classes is usually very small, and thus, only a small number of models, one representative of each universality class, need to be tested. Furthermore, universality is to some extent essential for model testing: models always require some degree of simplification. Universality allows simplifications, that is, negligence of predictive irrelevant features, without compromising the predictive accuracy of a hypothesis/model. Without universality, that is, if all features were predictive relevant, every simplification would lead a model to deviate in its predictions from the data, and even a reasonably “true” model—when subject to some technically necessary simplifications—would not fit the data. This is usually not desired, since simplifications, and be it just for technical reasons, are often essential to evaluate models properly.
Could we overcome the limitations posed by universality? Universality emerges in view of the type of data and the circumstances under which it is collected; other types of data or a change of experimental settings may render certain predictive irrelevant features relevant and thus distinguishable. One could therefore try to obtain richer data with more features. For example, when assessing cell fate choices, one could try to directly observe them through intra-vital live imaging, to gain the time dimension as feature of the data, and with this, further details of the cell fate choices could be distinguished. While such experiments are possible in some circumstances (for example, to observe live cell fate choices in mouse epidermis [ 38 ]), they are more expensive in terms of money and effort, more invasive, or not possible in many tissues and situations. On the other hand, universality does not always emerge: usually only in limiting cases, e.g. when experiments are run over longer time scales or when numbers (such as clone sizes) are large, properties are genuinely universal [ 33 ]. When data is collected from experiments after shorter time scales or when numbers are smaller, for example, short-term cell lineage tracing after few cell divisions [ 28 , 39 ], the data, and related model outputs, are not universal, and more features could, in principle, be distinguished. However, this may lead to a trade-off one wishes to avoid: while model details are easier to distinguish for short-term data, reasonable and necessary simplifications to the model may lead to undesired deviations.
To summarise, there is no one-size-fits-all solution, and a lot of intuition is needed to balance the trade-offs between the opportunities and limitations of universality: on the one hand, one wishes to distinguish a sufficient number of features, i.e. having them predictive relevant; on the other hand, one wishes to simplify the models as much as possible, by neglecting predictive irrelevant features. Ideally, the predictive relevant features are the same as the ones relevant to the biological question; this cannot be assured, but appropriate choices of experimental settings can adapt universal features for our purposes, at least to some extent. Unfortunately, the predictive (ir-)relevant features which define the universality classes are often not known beforehand. Then, we may need to travel down the rocky route and follow the classical scientific method, according to K. Popper: come up with a set of all plausible hypotheses and test the corresponding models for all of them; reject those hypotheses which cannot be brought in accordance with the data through fitting, while those that fit (possibly more than one model) may then constitute the universality class of the “true” model. Without prior knowledge about universality classes, however, the number of candidate models to test could be extremely large and arbitrarily complex. Hence, in order to optimise a modelling approach, it is essential to gather some a priori knowledge about the universality classes and their predictive relevant features. This can only be obtained by a mathematical analysis of candidate models’ properties beforehand, as described in the following section.
Data-Free Model Analysis
Commonly, quantitative models are used in view of experimental data. The study of intrinsic properties of models from a plainly theoretical standpoint, without explicitly taking into account data, is often seen as a mere academic exercise. Nonetheless, studying the intrinsic properties of models, through mathematical and computational tools, can yield impactful insights irrespective of the particular data and can guide and boost experimental campaigns substantially. The crucial benefit of theoretical, data-free model analysis is that it can assess the intrinsic consistency of models and connections between them (that is, between hypotheses), yielding shortcuts for the modelling campaign and guiding experiments. For example, by studying models theoretically, one can:
- discard some hypotheses a priori, by finding that they are intrinsically not consistent, or are not consistent with the circumstances of experiments.
- find that some hypotheses imply each other or contradict each other.
- identify Universality classes and their predictive (ir-)relevant features.
This means that many candidate hypotheses may be redundant, be it because they are intrinsically inconsistent, or follow from/ contradict other hypothesis, or are indistinguishable from others. Needless to say that this may guide towards those experiments that are really needed, and one can identify experiments that are redundant before one starts designing them, thus saving a large amount of effort and costs. An example how hypotheses can be excluded is our study of generic models for tissue cell population dynamics that includes all types of cell fate choice dynamics, in homeostasis [ 40 ]. There, it is shown, by introducing rigorous definitions of the concepts of a stem cell type, self-renewal, and homeostasis, that in a homeostatic state, only models where self-renewing cells are at the apex of a lineage hierarchy can prevail; others can be discarded without an expensive model testing campaign. Hence, by using this knowledge, coming from purely theoretical, data-free model analysis, both a lot of modelling and experimental work can be saved. Consequently, data-free model analysis can yield highly valuable information about a biological system that can save a lot of experimental and computational work and thus a lot of money, since the theoretical work is often much cheaper to be done.
Finally, theoretical model analysis can be used to reduce the number of potential candidate hypotheses that need to be tested dramatically, by finding the possible universality classes and identifying the predictive relevant features that associate a model with a universality class. Numerically, this can be done by varying parameters and structural features randomly and test under which circumstances model predictions change and when not. If an appropriate mathematical formulation of the model is available, this can also be done by mathematically taking the limits under which the emergence of universality is suspected (for large times, system size, or close to bifurcations or phase transition points), or taking a coarse-graining process such as “renormalisation” to identify the model behaviour under large scales of time and space [ 41 , 42 ]. For example, in Ref. [ 32 ••], it was shown that in homeostasis, all cell fate models can be categorised in only two universality classes (with further sub-classes when different limits of parameters are considered 7 ). The only predictive relevant feature is a binary characteristic: whether the number of cells which retain self-renewal potential is strictly conserved over time, or not (see Fig. 1 D). This also explains why model 2 in Fig. 1 C, involving cell fate priming and reversibility, predicts the same clone size distribution as model 1: in both cases, the number of stem cells is not conserved, in model 1 via symmetric divisions; in model 2, if upon a division S → S + D, the D-cell turns into an S-cell. Hence, model 2 shares the (only) predictive relevant characteristic with model 1. Another example is the classification of models for cell differentiation via catastrophe theory [ 19 , 43 ]: many different dynamical systems behave in the same way close to points where the stability of the system changes (“bifurcations”), such as cells when they differentiate. Catastrophe theory was used to show that there are only few possibilities how cells can progress through differentiation [ 19 ], which again reduces the number of candidate models to be tested.
Conclusions
Quantitative modelling can aid biological research in many aspects, but one needs to take into consideration a few things before embarking onto a modelling campaign, in order to make optimal use of it and avoid wrong results. One risk of quantitative modelling, when the exact model is not known a priori and is to be tested on the data, is over-fitting: it is tempting to include a large number of biological details in a model, but this often comes at the cost of additional free parameters, which leads to the phenomenon that an over-fitted, wrong model can seemingly match the data with its output. This can lead to wrong conclusions about the underlying biological hypotheses. Hence, if one wishes to test a model, and thus any underlying hypotheses, the number of free parameters needs to remain low, which requires to keep a model lean, stripping it to the core features that are needed to test the underlying hypothesis.
However, even when a model contains sufficiently few parameters to avoid over-fitting, a matching model might not be the correct one. The reason behind this is “universality” the phenomenon that different models can yield the same predictions, if they possess the same “predictive relevant” features, even if they might differ in other, “predictive irrelevant” features—some of them which may yet be biologically relevant. This means that others than the “true” model may match the data as well. Thus, without prior knowledge about the predictive relevant features of universality classes, a match of model and data does not mean that the model is correct, and the only way to test hypotheses through modelling is by testing all reasonable candidate models and exclude those which do not fit.
While the prospect of testing all reasonable models, in conjunction with the rather discouraging fact that many may not be distinguishable at all, seems daunting, the existence of universality classes also provides opportunities, when the universality classes (which are usually very few) and their predictive relevant features are determined beforehand. In that case, only the simplest model, as representatives of each universality class, needs to be tested. If in addition, the features we wish to distinguish biologically are the same as the predictive relevant features, universality is not a problem, yet can simplify the modelling campaign a lot. However, this requires a thorough theoretical, data-free mathematical or computational analysis of the models in question, in order to identify the predictive features and universality classes of relevant models beforehand. In general, data-free theoretical analysis can simplify modelling and experimental research in multiple ways: apart from identifying universal features, one can find criteria to exclude certain models a priori and find connections between models/hypotheses that allow to imply some hypotheses from others, whereby experimental and modelling work can be reduced.
When the aim of modelling is to predict the outcome of a biological process, over-fitting and universality are not problems per se: if one has rich data, e.g. as provided by high-throughput assays, machine learning, together with appropriate tuning of hyperparameters and regularisation, can be used to achieve great predictive power, without necessarily correctly reflecting the underlying biology. However, due to this reason, machine learning does not provide understanding of underlying biological processes and can only make predictions under the conditions where the data was collected. Alternatively, mechanistic models—which directly reflect the underlying biology—can also be used to yield predictions, if almost all parameters are well known beforehand. Such models can in principle be adjusted to make predictions beyond the narrow setup of performed experiments, to perform “in silico” experiments. However, making use of mechanistic models for prediction is very challenging, not only due to the risk of over-fitting but also since, in particular when the situation is not universal (i.e. away from limiting situations), it is difficult or even impossible to find all relevant features. Thus, mechanistic models are rarely used in cell and developmental biology to make de novo predictions but are used in other realms of biology, for example, in epidemiology to predict the spread of infectious diseases [ 44 ].
To summarise, mathematical modelling can, when correctly done, provide plenty of added value to biological research. But care needs to be taken when drawing conclusions, since over-fitting or the existence of universality can mislead the model finding process.
Medical Research Council, MR/R026610/1, Philip Greulich.
Compliance with Ethical Standards
The author declares that he has no conflicts of interest.
This article does not contain any studies with human or animal subjects performed by any of the authors.
1 The certainty of a hypothesis can be computed as the "Bayesian posterior", but this requires the computation of likelihoods of all reasonable candidate hypotheses.
2 It has been suggested that the training procedure of ANNs exhibits an intrinsic bias towards simple functions that avoid fitting noise and extrapolate predictions well beyond the training data [ 45 , 46 ].
3 The number of parameters that can be fitted without over-fitting should normally scale with the number of data points, but as described in the “ Universality: Curse and Opportunity ” section, the phenomenon of “universality” can render parameters irrelevant which means that many instances of those parameters—and not only the “true” parameter values of the underlying real system—can yield a good fit, thus mimicking over-fitting.
4 Since quantities which bear physical units are also called “dimensions”.
5 This is the context in which the term “universality” has been historically introduced and canonically defined. The adaptation of this term to the other situations where predictive irrelevant features exist is non-canonical and not generally used as such in the literature.
6 The reason is that the probability distribution of cell numbers is a Poisson distribution, which is entirely determined by a single parameter, the mean value, which in this case is the cell division rate divided by the terminal differentiation rate.
7 The universality class corresponding to strictly conserved stem cell numbers can yield different predictions in different limits: a normal distribution in the limit of fast stem cell divisions and an exponential distribution for slow stem cell divisions. Thus, this class can be divided into two sub-universality classes.
Publisher's Note
Springer Nature remains neutral with regard to jurisdictional claims in published maps and institutional affiliations.
Papers of particular interest, published recently, have been highlighted as: • Of importance •• Of major importance
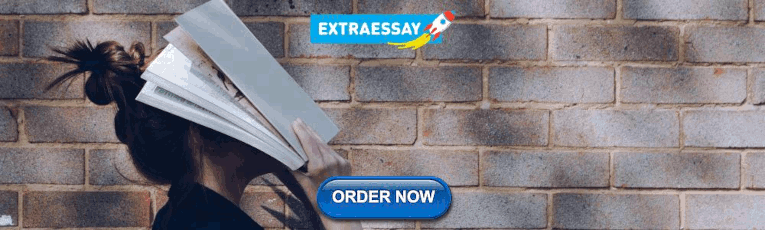
IMAGES
VIDEO
COMMENTS
A hypothesis in biology is a critical component of scientific research that proposes an explanation for a specific biological phenomenon. Writing a well-formulated hypothesis sets the foundation for conducting experiments, making observations, and drawing meaningful conclusions. Follow this step-by-step guide to create a strong biology ...
The scientific method. At the core of biology and other sciences lies a problem-solving approach called the scientific method. The scientific method has five basic steps, plus one feedback step: Make an observation. Ask a question. Form a hypothesis, or testable explanation. Make a prediction based on the hypothesis.
Developing a hypothesis (with example) Step 1. Ask a question. Writing a hypothesis begins with a research question that you want to answer. The question should be focused, specific, and researchable within the constraints of your project. Example: Research question.
Meaning. Biology. The study of living things. Observation. Noticing and describing events in an orderly way. Hypothesis. A scientific explanation that can be tested through experimentation or observation. Controlled experiment. An experiment in which only one variable is changed.
Step 5: Phrase your hypothesis in three ways. To identify the variables, you can write a simple prediction in if … then form. The first part of the sentence states the independent variable and the second part states the dependent variable. If a first-year student starts attending more lectures, then their exam scores will improve.
This statement will make an excellent hypothesis for the next study. The researchers should first test hypothesis C—whether or not the new vaccine can prevent HPV.[/hidden-answer] Experimental Design. You've successfully identified a hypothesis for the University of Washington's study on HPV: People who get the HPV vaccine will not get ...
Testing a Hypothesis. Credits. Biologists, and other scientists, study the world using a formal process referred to as the scientific method. The scientific method was first documented by Sir Francis Bacon (1561-1626) of England, and can be applied to almost all fields of study. The scientific method is founded upon observation, which then ...
When conducting scientific experiments, researchers develop hypotheses to guide experimental design. A hypothesis is a suggested explanation that is both testable and falsifiable. You must be able to test your hypothesis through observations and research, and it must be possible to prove your hypothesis false. For example, Michael observes that ...
The scientific process was used even in ancient times, but it was first documented by England's Sir Francis Bacon (1561-1626) ( Figure 1.2.1 1.2. 1 ), who set up inductive methods for scientific inquiry. The scientific method is not exclusively used by biologists but can be applied to almost anything as a logical problem solving method.
Start typing, then use the up and down arrows to select an option from the list.?
Although the terms "hypothesis" and "prediction" are often incorrectly used interchangeably, they refer to different but complementary concepts. A hypothesis attempts to explain the mechanism underlying a pattern, while a prediction states an expectation regarding the results. While challenging to construct, hypotheses provide powerful ...
hypothesis. science. scientific hypothesis, an idea that proposes a tentative explanation about a phenomenon or a narrow set of phenomena observed in the natural world. The two primary features of a scientific hypothesis are falsifiability and testability, which are reflected in an "If…then" statement summarizing the idea and in the ...
Dr. Michelle Harris, Dr. Janet Batzli,Biocore. This section provides guidelines on how to construct a solid introduction to a scientific paper including background information, study question, biological rationale, hypothesis, and general approach. If the Introduction is done well, there should be no question in the reader's mind why and on ...
A hypothesis is the best answer to a question based on what is known. Scientists take that best answer and do experiments to see if it still makes sense or if a better answer can be made. When a scientist has a question they want to answer, they research what is already known about the topic. Then, they come up with their best answer to the ...
After computing the statistical test, the outcome will indicate which statistical hypothesis is more likely. This, in turn indicates to scientists what level of inference can be gained from the data compared to the biological hypothesis (the focus point of the study). Then a conclusion can be made based on the sample about the entire population.
Formulating Hypotheses for Different Study Designs. Generating a testable working hypothesis is the first step towards conducting original research. Such research may prove or disprove the proposed hypothesis. Case reports, case series, online surveys and other observational studies, clinical trials, and narrative reviews help to generate ...
Phylogenetic trees represent hypotheses about the evolutionary relationships among a group of organisms. A phylogenetic tree may be built using morphological (body shape), biochemical, behavioral, or molecular features of species or other groups. In building a tree, we organize species into nested groups based on shared derived traits (traits ...
The technique used by the vast majority of biologists, and the technique that most of this handbook describes, is sometimes called "frequentist" or "classical" statistics. It involves testing a null hypothesis by comparing the data you observe in your experiment with the predictions of a null hypothesis. You estimate what the probability would ...
Vermeij's original formulation of the hypothesis of escalation is actually composed of two separate testable propositions: "Biological hazards due to competitors and predators have become more severe over the course of time in physically comparable habitats" (p. 49 in Vermeij 1987) and "traits that enhance the competitive and ...
The first is to raise a research question, answer that question by conducting a new study, and then offer one or more theories (usually more) to explain or interpret the results. This format works well for applied research questions and for research questions that existing theories do not address. The second way is to describe one or more ...
Quantitative modelling is the use of mathematical or computational means to imitate real-world processes and to predict their outcomes. Historically, it has been used for centuries in the form of fundamental physical laws, such as Newton's laws, while biological problems have been subject to mathematical modelling since the first half of the ...
The hypothesis is often written using the words "IF" and "THEN.". For example, "If I do not study, then I will fail the test.". The "if' and "then" statements reflect your independent and dependent variables. The hypothesis should relate back to your original question and must be testable.