Have a language expert improve your writing
Run a free plagiarism check in 10 minutes, automatically generate references for free.
- Knowledge Base
- Methodology
- Doing Survey Research | A Step-by-Step Guide & Examples
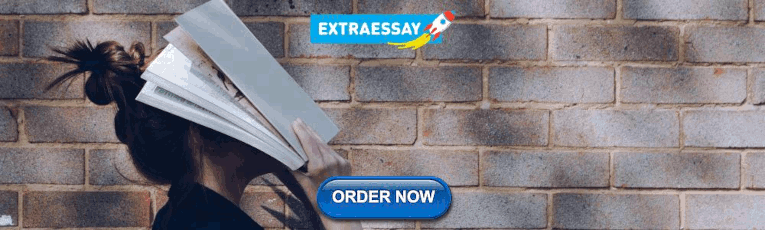
Doing Survey Research | A Step-by-Step Guide & Examples
Published on 6 May 2022 by Shona McCombes . Revised on 10 October 2022.
Survey research means collecting information about a group of people by asking them questions and analysing the results. To conduct an effective survey, follow these six steps:
- Determine who will participate in the survey
- Decide the type of survey (mail, online, or in-person)
- Design the survey questions and layout
- Distribute the survey
- Analyse the responses
- Write up the results
Surveys are a flexible method of data collection that can be used in many different types of research .
Table of contents
What are surveys used for, step 1: define the population and sample, step 2: decide on the type of survey, step 3: design the survey questions, step 4: distribute the survey and collect responses, step 5: analyse the survey results, step 6: write up the survey results, frequently asked questions about surveys.
Surveys are used as a method of gathering data in many different fields. They are a good choice when you want to find out about the characteristics, preferences, opinions, or beliefs of a group of people.
Common uses of survey research include:
- Social research: Investigating the experiences and characteristics of different social groups
- Market research: Finding out what customers think about products, services, and companies
- Health research: Collecting data from patients about symptoms and treatments
- Politics: Measuring public opinion about parties and policies
- Psychology: Researching personality traits, preferences, and behaviours
Surveys can be used in both cross-sectional studies , where you collect data just once, and longitudinal studies , where you survey the same sample several times over an extended period.
Prevent plagiarism, run a free check.
Before you start conducting survey research, you should already have a clear research question that defines what you want to find out. Based on this question, you need to determine exactly who you will target to participate in the survey.
Populations
The target population is the specific group of people that you want to find out about. This group can be very broad or relatively narrow. For example:
- The population of Brazil
- University students in the UK
- Second-generation immigrants in the Netherlands
- Customers of a specific company aged 18 to 24
- British transgender women over the age of 50
Your survey should aim to produce results that can be generalised to the whole population. That means you need to carefully define exactly who you want to draw conclusions about.
It’s rarely possible to survey the entire population of your research – it would be very difficult to get a response from every person in Brazil or every university student in the UK. Instead, you will usually survey a sample from the population.
The sample size depends on how big the population is. You can use an online sample calculator to work out how many responses you need.
There are many sampling methods that allow you to generalise to broad populations. In general, though, the sample should aim to be representative of the population as a whole. The larger and more representative your sample, the more valid your conclusions.
There are two main types of survey:
- A questionnaire , where a list of questions is distributed by post, online, or in person, and respondents fill it out themselves
- An interview , where the researcher asks a set of questions by phone or in person and records the responses
Which type you choose depends on the sample size and location, as well as the focus of the research.
Questionnaires
Sending out a paper survey by post is a common method of gathering demographic information (for example, in a government census of the population).
- You can easily access a large sample.
- You have some control over who is included in the sample (e.g., residents of a specific region).
- The response rate is often low.
Online surveys are a popular choice for students doing dissertation research , due to the low cost and flexibility of this method. There are many online tools available for constructing surveys, such as SurveyMonkey and Google Forms .
- You can quickly access a large sample without constraints on time or location.
- The data is easy to process and analyse.
- The anonymity and accessibility of online surveys mean you have less control over who responds.
If your research focuses on a specific location, you can distribute a written questionnaire to be completed by respondents on the spot. For example, you could approach the customers of a shopping centre or ask all students to complete a questionnaire at the end of a class.
- You can screen respondents to make sure only people in the target population are included in the sample.
- You can collect time- and location-specific data (e.g., the opinions of a shop’s weekday customers).
- The sample size will be smaller, so this method is less suitable for collecting data on broad populations.
Oral interviews are a useful method for smaller sample sizes. They allow you to gather more in-depth information on people’s opinions and preferences. You can conduct interviews by phone or in person.
- You have personal contact with respondents, so you know exactly who will be included in the sample in advance.
- You can clarify questions and ask for follow-up information when necessary.
- The lack of anonymity may cause respondents to answer less honestly, and there is more risk of researcher bias.
Like questionnaires, interviews can be used to collect quantitative data : the researcher records each response as a category or rating and statistically analyses the results. But they are more commonly used to collect qualitative data : the interviewees’ full responses are transcribed and analysed individually to gain a richer understanding of their opinions and feelings.
Next, you need to decide which questions you will ask and how you will ask them. It’s important to consider:
- The type of questions
- The content of the questions
- The phrasing of the questions
- The ordering and layout of the survey
Open-ended vs closed-ended questions
There are two main forms of survey questions: open-ended and closed-ended. Many surveys use a combination of both.
Closed-ended questions give the respondent a predetermined set of answers to choose from. A closed-ended question can include:
- A binary answer (e.g., yes/no or agree/disagree )
- A scale (e.g., a Likert scale with five points ranging from strongly agree to strongly disagree )
- A list of options with a single answer possible (e.g., age categories)
- A list of options with multiple answers possible (e.g., leisure interests)
Closed-ended questions are best for quantitative research . They provide you with numerical data that can be statistically analysed to find patterns, trends, and correlations .
Open-ended questions are best for qualitative research. This type of question has no predetermined answers to choose from. Instead, the respondent answers in their own words.
Open questions are most common in interviews, but you can also use them in questionnaires. They are often useful as follow-up questions to ask for more detailed explanations of responses to the closed questions.
The content of the survey questions
To ensure the validity and reliability of your results, you need to carefully consider each question in the survey. All questions should be narrowly focused with enough context for the respondent to answer accurately. Avoid questions that are not directly relevant to the survey’s purpose.
When constructing closed-ended questions, ensure that the options cover all possibilities. If you include a list of options that isn’t exhaustive, you can add an ‘other’ field.
Phrasing the survey questions
In terms of language, the survey questions should be as clear and precise as possible. Tailor the questions to your target population, keeping in mind their level of knowledge of the topic.
Use language that respondents will easily understand, and avoid words with vague or ambiguous meanings. Make sure your questions are phrased neutrally, with no bias towards one answer or another.
Ordering the survey questions
The questions should be arranged in a logical order. Start with easy, non-sensitive, closed-ended questions that will encourage the respondent to continue.
If the survey covers several different topics or themes, group together related questions. You can divide a questionnaire into sections to help respondents understand what is being asked in each part.
If a question refers back to or depends on the answer to a previous question, they should be placed directly next to one another.
Before you start, create a clear plan for where, when, how, and with whom you will conduct the survey. Determine in advance how many responses you require and how you will gain access to the sample.
When you are satisfied that you have created a strong research design suitable for answering your research questions, you can conduct the survey through your method of choice – by post, online, or in person.
There are many methods of analysing the results of your survey. First you have to process the data, usually with the help of a computer program to sort all the responses. You should also cleanse the data by removing incomplete or incorrectly completed responses.
If you asked open-ended questions, you will have to code the responses by assigning labels to each response and organising them into categories or themes. You can also use more qualitative methods, such as thematic analysis , which is especially suitable for analysing interviews.
Statistical analysis is usually conducted using programs like SPSS or Stata. The same set of survey data can be subject to many analyses.
Finally, when you have collected and analysed all the necessary data, you will write it up as part of your thesis, dissertation , or research paper .
In the methodology section, you describe exactly how you conducted the survey. You should explain the types of questions you used, the sampling method, when and where the survey took place, and the response rate. You can include the full questionnaire as an appendix and refer to it in the text if relevant.
Then introduce the analysis by describing how you prepared the data and the statistical methods you used to analyse it. In the results section, you summarise the key results from your analysis.
A Likert scale is a rating scale that quantitatively assesses opinions, attitudes, or behaviours. It is made up of four or more questions that measure a single attitude or trait when response scores are combined.
To use a Likert scale in a survey , you present participants with Likert-type questions or statements, and a continuum of items, usually with five or seven possible responses, to capture their degree of agreement.
Individual Likert-type questions are generally considered ordinal data , because the items have clear rank order, but don’t have an even distribution.
Overall Likert scale scores are sometimes treated as interval data. These scores are considered to have directionality and even spacing between them.
The type of data determines what statistical tests you should use to analyse your data.
A questionnaire is a data collection tool or instrument, while a survey is an overarching research method that involves collecting and analysing data from people using questionnaires.
Cite this Scribbr article
If you want to cite this source, you can copy and paste the citation or click the ‘Cite this Scribbr article’ button to automatically add the citation to our free Reference Generator.
McCombes, S. (2022, October 10). Doing Survey Research | A Step-by-Step Guide & Examples. Scribbr. Retrieved 14 May 2024, from https://www.scribbr.co.uk/research-methods/surveys/
Is this article helpful?
Shona McCombes
Other students also liked, qualitative vs quantitative research | examples & methods, construct validity | definition, types, & examples, what is a likert scale | guide & examples.

An official website of the United States government
The .gov means it’s official. Federal government websites often end in .gov or .mil. Before sharing sensitive information, make sure you’re on a federal government site.
The site is secure. The https:// ensures that you are connecting to the official website and that any information you provide is encrypted and transmitted securely.
- Publications
- Account settings
Preview improvements coming to the PMC website in October 2024. Learn More or Try it out now .
- Advanced Search
- Journal List
- J Adv Pract Oncol
- v.6(2); Mar-Apr 2015

Understanding and Evaluating Survey Research
A variety of methodologic approaches exist for individuals interested in conducting research. Selection of a research approach depends on a number of factors, including the purpose of the research, the type of research questions to be answered, and the availability of resources. The purpose of this article is to describe survey research as one approach to the conduct of research so that the reader can critically evaluate the appropriateness of the conclusions from studies employing survey research.
SURVEY RESEARCH
Survey research is defined as "the collection of information from a sample of individuals through their responses to questions" ( Check & Schutt, 2012, p. 160 ). This type of research allows for a variety of methods to recruit participants, collect data, and utilize various methods of instrumentation. Survey research can use quantitative research strategies (e.g., using questionnaires with numerically rated items), qualitative research strategies (e.g., using open-ended questions), or both strategies (i.e., mixed methods). As it is often used to describe and explore human behavior, surveys are therefore frequently used in social and psychological research ( Singleton & Straits, 2009 ).
Information has been obtained from individuals and groups through the use of survey research for decades. It can range from asking a few targeted questions of individuals on a street corner to obtain information related to behaviors and preferences, to a more rigorous study using multiple valid and reliable instruments. Common examples of less rigorous surveys include marketing or political surveys of consumer patterns and public opinion polls.
Survey research has historically included large population-based data collection. The primary purpose of this type of survey research was to obtain information describing characteristics of a large sample of individuals of interest relatively quickly. Large census surveys obtaining information reflecting demographic and personal characteristics and consumer feedback surveys are prime examples. These surveys were often provided through the mail and were intended to describe demographic characteristics of individuals or obtain opinions on which to base programs or products for a population or group.
More recently, survey research has developed into a rigorous approach to research, with scientifically tested strategies detailing who to include (representative sample), what and how to distribute (survey method), and when to initiate the survey and follow up with nonresponders (reducing nonresponse error), in order to ensure a high-quality research process and outcome. Currently, the term "survey" can reflect a range of research aims, sampling and recruitment strategies, data collection instruments, and methods of survey administration.
Given this range of options in the conduct of survey research, it is imperative for the consumer/reader of survey research to understand the potential for bias in survey research as well as the tested techniques for reducing bias, in order to draw appropriate conclusions about the information reported in this manner. Common types of error in research, along with the sources of error and strategies for reducing error as described throughout this article, are summarized in the Table .

Sources of Error in Survey Research and Strategies to Reduce Error
The goal of sampling strategies in survey research is to obtain a sufficient sample that is representative of the population of interest. It is often not feasible to collect data from an entire population of interest (e.g., all individuals with lung cancer); therefore, a subset of the population or sample is used to estimate the population responses (e.g., individuals with lung cancer currently receiving treatment). A large random sample increases the likelihood that the responses from the sample will accurately reflect the entire population. In order to accurately draw conclusions about the population, the sample must include individuals with characteristics similar to the population.
It is therefore necessary to correctly identify the population of interest (e.g., individuals with lung cancer currently receiving treatment vs. all individuals with lung cancer). The sample will ideally include individuals who reflect the intended population in terms of all characteristics of the population (e.g., sex, socioeconomic characteristics, symptom experience) and contain a similar distribution of individuals with those characteristics. As discussed by Mady Stovall beginning on page 162, Fujimori et al. ( 2014 ), for example, were interested in the population of oncologists. The authors obtained a sample of oncologists from two hospitals in Japan. These participants may or may not have similar characteristics to all oncologists in Japan.
Participant recruitment strategies can affect the adequacy and representativeness of the sample obtained. Using diverse recruitment strategies can help improve the size of the sample and help ensure adequate coverage of the intended population. For example, if a survey researcher intends to obtain a sample of individuals with breast cancer representative of all individuals with breast cancer in the United States, the researcher would want to use recruitment strategies that would recruit both women and men, individuals from rural and urban settings, individuals receiving and not receiving active treatment, and so on. Because of the difficulty in obtaining samples representative of a large population, researchers may focus the population of interest to a subset of individuals (e.g., women with stage III or IV breast cancer). Large census surveys require extremely large samples to adequately represent the characteristics of the population because they are intended to represent the entire population.
DATA COLLECTION METHODS
Survey research may use a variety of data collection methods with the most common being questionnaires and interviews. Questionnaires may be self-administered or administered by a professional, may be administered individually or in a group, and typically include a series of items reflecting the research aims. Questionnaires may include demographic questions in addition to valid and reliable research instruments ( Costanzo, Stawski, Ryff, Coe, & Almeida, 2012 ; DuBenske et al., 2014 ; Ponto, Ellington, Mellon, & Beck, 2010 ). It is helpful to the reader when authors describe the contents of the survey questionnaire so that the reader can interpret and evaluate the potential for errors of validity (e.g., items or instruments that do not measure what they are intended to measure) and reliability (e.g., items or instruments that do not measure a construct consistently). Helpful examples of articles that describe the survey instruments exist in the literature ( Buerhaus et al., 2012 ).
Questionnaires may be in paper form and mailed to participants, delivered in an electronic format via email or an Internet-based program such as SurveyMonkey, or a combination of both, giving the participant the option to choose which method is preferred ( Ponto et al., 2010 ). Using a combination of methods of survey administration can help to ensure better sample coverage (i.e., all individuals in the population having a chance of inclusion in the sample) therefore reducing coverage error ( Dillman, Smyth, & Christian, 2014 ; Singleton & Straits, 2009 ). For example, if a researcher were to only use an Internet-delivered questionnaire, individuals without access to a computer would be excluded from participation. Self-administered mailed, group, or Internet-based questionnaires are relatively low cost and practical for a large sample ( Check & Schutt, 2012 ).
Dillman et al. ( 2014 ) have described and tested a tailored design method for survey research. Improving the visual appeal and graphics of surveys by using a font size appropriate for the respondents, ordering items logically without creating unintended response bias, and arranging items clearly on each page can increase the response rate to electronic questionnaires. Attending to these and other issues in electronic questionnaires can help reduce measurement error (i.e., lack of validity or reliability) and help ensure a better response rate.
Conducting interviews is another approach to data collection used in survey research. Interviews may be conducted by phone, computer, or in person and have the benefit of visually identifying the nonverbal response(s) of the interviewee and subsequently being able to clarify the intended question. An interviewer can use probing comments to obtain more information about a question or topic and can request clarification of an unclear response ( Singleton & Straits, 2009 ). Interviews can be costly and time intensive, and therefore are relatively impractical for large samples.
Some authors advocate for using mixed methods for survey research when no one method is adequate to address the planned research aims, to reduce the potential for measurement and non-response error, and to better tailor the study methods to the intended sample ( Dillman et al., 2014 ; Singleton & Straits, 2009 ). For example, a mixed methods survey research approach may begin with distributing a questionnaire and following up with telephone interviews to clarify unclear survey responses ( Singleton & Straits, 2009 ). Mixed methods might also be used when visual or auditory deficits preclude an individual from completing a questionnaire or participating in an interview.
FUJIMORI ET AL.: SURVEY RESEARCH
Fujimori et al. ( 2014 ) described the use of survey research in a study of the effect of communication skills training for oncologists on oncologist and patient outcomes (e.g., oncologist’s performance and confidence and patient’s distress, satisfaction, and trust). A sample of 30 oncologists from two hospitals was obtained and though the authors provided a power analysis concluding an adequate number of oncologist participants to detect differences between baseline and follow-up scores, the conclusions of the study may not be generalizable to a broader population of oncologists. Oncologists were randomized to either an intervention group (i.e., communication skills training) or a control group (i.e., no training).
Fujimori et al. ( 2014 ) chose a quantitative approach to collect data from oncologist and patient participants regarding the study outcome variables. Self-report numeric ratings were used to measure oncologist confidence and patient distress, satisfaction, and trust. Oncologist confidence was measured using two instruments each using 10-point Likert rating scales. The Hospital Anxiety and Depression Scale (HADS) was used to measure patient distress and has demonstrated validity and reliability in a number of populations including individuals with cancer ( Bjelland, Dahl, Haug, & Neckelmann, 2002 ). Patient satisfaction and trust were measured using 0 to 10 numeric rating scales. Numeric observer ratings were used to measure oncologist performance of communication skills based on a videotaped interaction with a standardized patient. Participants completed the same questionnaires at baseline and follow-up.
The authors clearly describe what data were collected from all participants. Providing additional information about the manner in which questionnaires were distributed (i.e., electronic, mail), the setting in which data were collected (e.g., home, clinic), and the design of the survey instruments (e.g., visual appeal, format, content, arrangement of items) would assist the reader in drawing conclusions about the potential for measurement and nonresponse error. The authors describe conducting a follow-up phone call or mail inquiry for nonresponders, using the Dillman et al. ( 2014 ) tailored design for survey research follow-up may have reduced nonresponse error.
CONCLUSIONS
Survey research is a useful and legitimate approach to research that has clear benefits in helping to describe and explore variables and constructs of interest. Survey research, like all research, has the potential for a variety of sources of error, but several strategies exist to reduce the potential for error. Advanced practitioners aware of the potential sources of error and strategies to improve survey research can better determine how and whether the conclusions from a survey research study apply to practice.
The author has no potential conflicts of interest to disclose.
Faculty and researchers : We want to hear from you! We are launching a survey to learn more about your library collection needs for teaching, learning, and research. If you would like to participate, please complete the survey by May 17, 2024. Thank you for your participation!

- University of Massachusetts Lowell
- University Libraries
Survey Research: Design and Presentation
- Planning a Thesis Proposal
- Introduction to Survey Research Design
- Literature Review: Definition and Context
- Slides, Articles
- Evaluating Survey Results
- Related Library Databases
The goal of a proposal is to demonstrate that you are ready to start your research project by presenting a distinct idea, question or issue which has great interest for you, along with the method you have chosen to explore it.
The process of developing your research question is related to the literature review. As you discover more from your research, your question will be shaped by what you find.
The clarity of your idea dictates the plan for your dissertation or thesis work.
From the University of North Texas faculty member Dr. Abraham Benavides:

Elements of a Thesis Proposal
(Adapted from the Department of Communication, University of Washington)
Dissertation proposals vary but most share the following elements, though not necessarily in this order.
1. The Introduction
In three paragraphs to three or four pages very simply introduce your question. Use a narrative to style to engage readers. A well-known issue in your field, controversy surrounding some texts, or the policy implications of your topic are some ways to add context to the proposal.
2. Research Questions
State your question early in your proposal. Even if you are going to restate your research questionas part of the literature review, you may wish to mention it briefly at the end of the introduction.
Make sure if you have questions which follow from your main question that this is clearly indicated. The research questions should include any boundaries you have placed on your inquiry, for instance time, place, and topics. Terms with unusual meanings should be defined.
3. Literature Synthesis or Review
The proposal must be described within the broader body of scholarship around the topic. This is part of establishing the significance of your research. The discussion of the literature typically shows how your project will extend what’s already known.
In writing your literature review, think about the important theories and concepts related to your project and organize your discussion accordingly; you usually want to avoid a strictly chronological discussion (i.e., earliest study, next study, etc.).
What research is directly related to your topic? Discuss it thoroughly.
What literature provides context for your research? Discuss it briefly.
In your proposal you should avoid writing a genealogy of your field’s research. For instance, you don’t need to tell your committee about the development of research in the entire field in order to justify the particular project you propose. Instead, isolate the major areas of research within your field that are relevant to your project.
4. Significance of your Research Question
Good proposals leave readers with a clear understanding of the dissertation project’s overall significance. Consider the following:
- advancing theoretical understandings
- introducing new interpretations
- analyzing the relationship between variables
- testing a theory
- replicating earlier studies
- exploring the whether earlier findings can be demonstrated to hold true in new times, places, or circumstances
- refining a method of inquiry.
5. Research Method
The research method that will be used involves three levels of concern:
- overall research design
- delineation of the method
- procedures for executing it.
At the outset you have to show that your overall design is appropriate for the questions you’re posing.
Next, you need to outline your specific research method. What data will you analyze?
How will you collect the data? Supervisors sometimes expect proposals to sketch instruments (e.g., coding sheets, questionnaires, protocols) central to the project.
Third, what procedures will you follow as you conduct your research? What will you do with your data? A key here is your plan for analyzing data. You want to gather data in a form in which you can analyze it. [In this case the method is a survey administered to a group of people]. If appropriate, you should indicate what rules for interpretation or what kinds of statistical tests that you’ll use.
6. Tentative Dissertation Outline
Give your committee a sense of how your thesis will be organized. You can write a short (two- or three-sentence) paragraph summarizing what you expect to include in each section of the thesis.
7. Tentative Schedule for Completion
Be realistic in projecting your timeline. Don’t forget to include time for human subjects review, if appropriate .
8. References
If you didn’t use footnotes or endnotes throughout, you should include a list of references to the literature cited in the proposal.
9. Selected Bibliography of Other Sources
You might want to append a more extensive bibliography (check with your supervisor). If you include one, you might want to divide it into several subsections, for instance by concept, topic or field.
- << Previous: Introduction to Survey Research Design
- Next: Literature Review: Definition and Context >>
- Last Updated: Jan 22, 2024 2:05 PM
- URL: https://libguides.uml.edu/rohland_surveys
Dissertation surveys: Questions, examples, and best practices
Collect data for your dissertation with little effort and great results.
Dissertation surveys are one of the most powerful tools to get valuable insights and data for the culmination of your research. However, it’s one of the most stressful and time-consuming tasks you need to do. You want useful data from a representative sample that you can analyze and present as part of your dissertation. At SurveyPlanet, we’re committed to making it as easy and stress-free as possible to get the most out of your study.
With an intuitive and user-friendly design, our templates and premade questions can be your allies while creating a survey for your dissertation. Explore all the options we offer by simply signing up for an account—and leave the stress behind.
How to write dissertation survey questions
The first thing to do is to figure out which group of people is relevant for your study. When you know that, you’ll also be able to adjust the survey and write questions that will get the best results.
The next step is to write down the goal of your research and define it properly. Online surveys are one of the best and most inexpensive ways to reach respondents and achieve your goal.
Before writing any questions, think about how you’ll analyze the results. You don’t want to write and distribute a survey without keeping how to report your findings in mind. When your thesis questionnaire is out in the real world, it’s too late to conclude that the data you’re collecting might not be any good for assessment. Because of that, you need to create questions with analysis in mind.
You may find our five survey analysis tips for better insights helpful. We recommend reading it before analyzing your results.
Once you understand the parameters of your representative sample, goals, and analysis methodology, then it’s time to think about distribution. Survey distribution may feel like a headache, but you’ll find that many people will gladly participate.
Find communities where your targeted group hangs out and share the link to your survey with them. If you’re not sure how large your research sample should be, gauge it easily with the survey sample size calculator.
Need help with writing survey questions? Read our guide on well-written examples of good survey questions .
Dissertation survey examples
Whatever field you’re studying, we’re sure the following questions will prove useful when crafting your own.
At the beginning of every questionnaire, inform respondents of your topic and provide a consent form. After that, start with questions like:
- Please select your gender:
- What is the highest educational level you’ve completed?
- High school
- Bachelor degree
- Master’s degree
- On a scale of 1-7, how satisfied are you with your current job?
- Please rate the following statements:
- I always wait for people to text me first.
- Strongly Disagree
- Neither agree nor disagree
- Strongly agree
- My friends always complain that I never invite them anywhere.
- I prefer spending time alone.
- Rank which personality traits are most important when choosing a partner. Rank 1 - 7, where 1 is the most and 7 is the least important.
- Flexibility
- Independence
- How openly do you share feelings with your partner?
- Almost never
- Almost always
- In the last two weeks, how often did you experience headaches?
Dissertation survey best practices
There are a lot of DOs and DON’Ts you should keep in mind when conducting any survey, especially for your dissertation. To get valuable data from your targeted sample, follow these best practices:
Use the consent form.
The consent form is a must when distributing a research questionnaire. A respondent has to know how you’ll use their answers and that the survey is anonymous.
Avoid leading and double-barreled questions
Leading and double-barreled questions will produce inconclusive results—and you don’t want that. A question such as: “Do you like to watch TV and play video games?” is double-barreled because it has two variables.
On the other hand, leading questions such as “On a scale from 1-10 how would you rate the amazing experience with our customer support?” influence respondents to answer in a certain way, which produces biased results.
Use easy and straightforward language and questions
Don’t use terms and professional jargon that respondents won’t understand. Take into consideration their educational level and demographic traits and use easy-to-understand language when writing questions.
Mix close-ended and open-ended questions
Too many open-ended questions will annoy respondents. Also, analyzing the responses is harder. Use more close-ended questions for the best results and only a few open-ended ones.
Strategically use different types of responses
Likert scale, multiple-choice, and ranking are all types of responses you can use to collect data. But some response types suit some questions better. Make sure to strategically fit questions with response types.
Ensure that data privacy is a priority
Make sure to use an online survey tool that has SSL encryption and secure data processing. You don’t want to risk all your hard work going to waste because of poorly managed data security. Ensure that you only collect data that’s relevant to your dissertation survey and leave out any questions (such as name) that can identify the respondents.
Create dissertation questionnaires with SurveyPlanet
Overall, survey methodology is a great way to find research participants for your research study. You have all the tools required for creating a survey for a dissertation with SurveyPlanet—you only need to sign up . With powerful features like question branching, custom formatting, multiple languages, image choice questions, and easy export you will find everything needed to create, distribute, and analyze a dissertation survey.
Happy data gathering!
Sign up now
Free unlimited surveys, questions and responses.
Reference management. Clean and simple.
How to collect data for your thesis
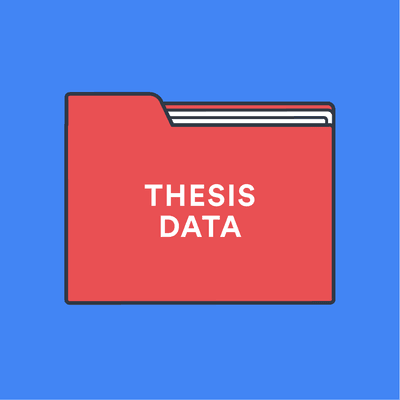
Collecting theoretical data
Search for theses on your topic, use content-sharing platforms, collecting empirical data, qualitative vs. quantitative data, frequently asked questions about gathering data for your thesis, related articles.
After choosing a topic for your thesis , you’ll need to start gathering data. In this article, we focus on how to effectively collect theoretical and empirical data.
Empirical data : unique research that may be quantitative, qualitative, or mixed.
Theoretical data : secondary, scholarly sources like books and journal articles that provide theoretical context for your research.
Thesis : the culminating, multi-chapter project for a bachelor’s, master’s, or doctoral degree.
Qualitative data : info that cannot be measured, like observations and interviews .
Quantitative data : info that can be measured and written with numbers.
At this point in your academic life, you are already acquainted with the ways of finding potential references. Some obvious sources of theoretical material are:
- edited volumes
- conference proceedings
- online databases like Google Scholar , ERIC , or Scopus
You can also take a look at the top list of academic search engines .
Looking at other theses on your topic can help you see what approaches have been taken and what aspects other writers have focused on. Pay close attention to the list of references and follow the bread-crumbs back to the original theories and specialized authors.
Another method for gathering theoretical data is to read through content-sharing platforms. Many people share their papers and writings on these sites. You can either hunt sources, get some inspiration for your own work or even learn new angles of your topic.
Some popular content sharing sites are:
With these sites, you have to check the credibility of the sources. You can usually rely on the content, but we recommend double-checking just to be sure. Take a look at our guide on what are credible sources?
The more you know, the better. The guide, " How to undertake a literature search and review for dissertations and final year projects ," will give you all the tools needed for finding literature .
In order to successfully collect empirical data, you have to choose first what type of data you want as an outcome. There are essentially two options, qualitative or quantitative data. Many people mistake one term with the other, so it’s important to understand the differences between qualitative and quantitative research .
Boiled down, qualitative data means words and quantitative means numbers. Both types are considered primary sources . Whichever one adapts best to your research will define the type of methodology to carry out, so choose wisely.
In the end, having in mind what type of outcome you intend and how much time you count on will lead you to choose the best type of empirical data for your research. For a detailed description of each methodology type mentioned above, read more about collecting data .
Once you gather enough theoretical and empirical data, you will need to start writing. But before the actual writing part, you have to structure your thesis to avoid getting lost in the sea of information. Take a look at our guide on how to structure your thesis for some tips and tricks.
The key to knowing what type of data you should collect for your thesis is knowing in advance the type of outcome you intend to have, and the amount of time you count with.
Some obvious sources of theoretical material are journals, libraries and online databases like Google Scholar , ERIC or Scopus , or take a look at the top list of academic search engines . You can also search for theses on your topic or read content sharing platforms, like Medium , Issuu , or Slideshare .
To gather empirical data, you have to choose first what type of data you want. There are two options, qualitative or quantitative data. You can gather data through observations, interviews, focus groups, or with surveys, tests, and existing databases.
Qualitative data means words, information that cannot be measured. It may involve multimedia material or non-textual data. This type of data claims to be detailed, nuanced and contextual.
Quantitative data means numbers, information that can be measured and written with numbers. This type of data claims to be credible, scientific and exact.
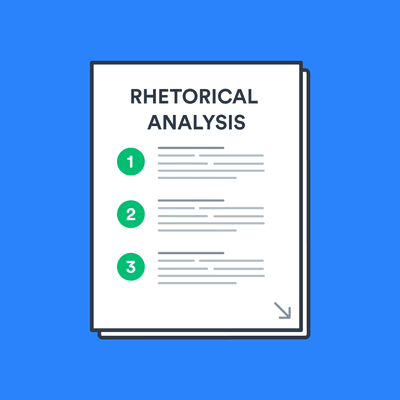

How To Write The Results/Findings Chapter
For qualitative studies (dissertations & theses).
By: Jenna Crossley (PhD). Expert Reviewed By: Dr. Eunice Rautenbach | August 2021
So, you’ve collected and analysed your qualitative data, and it’s time to write up your results chapter. But where do you start? In this post, we’ll guide you through the qualitative results chapter (also called the findings chapter), step by step.
Overview: Qualitative Results Chapter
- What (exactly) the qualitative results chapter is
- What to include in your results chapter
- How to write up your results chapter
- A few tips and tricks to help you along the way
- Free results chapter template
What exactly is the results chapter?
The results chapter in a dissertation or thesis (or any formal academic research piece) is where you objectively and neutrally present the findings of your qualitative analysis (or analyses if you used multiple qualitative analysis methods ). This chapter can sometimes be combined with the discussion chapter (where you interpret the data and discuss its meaning), depending on your university’s preference. We’ll treat the two chapters as separate, as that’s the most common approach.
In contrast to a quantitative results chapter that presents numbers and statistics, a qualitative results chapter presents data primarily in the form of words . But this doesn’t mean that a qualitative study can’t have quantitative elements – you could, for example, present the number of times a theme or topic pops up in your data, depending on the analysis method(s) you adopt.
Adding a quantitative element to your study can add some rigour, which strengthens your results by providing more evidence for your claims. This is particularly common when using qualitative content analysis. Keep in mind though that qualitative research aims to achieve depth, richness and identify nuances , so don’t get tunnel vision by focusing on the numbers. They’re just cream on top in a qualitative analysis.
So, to recap, the results chapter is where you objectively present the findings of your analysis, without interpreting them (you’ll save that for the discussion chapter). With that out the way, let’s take a look at what you should include in your results chapter.
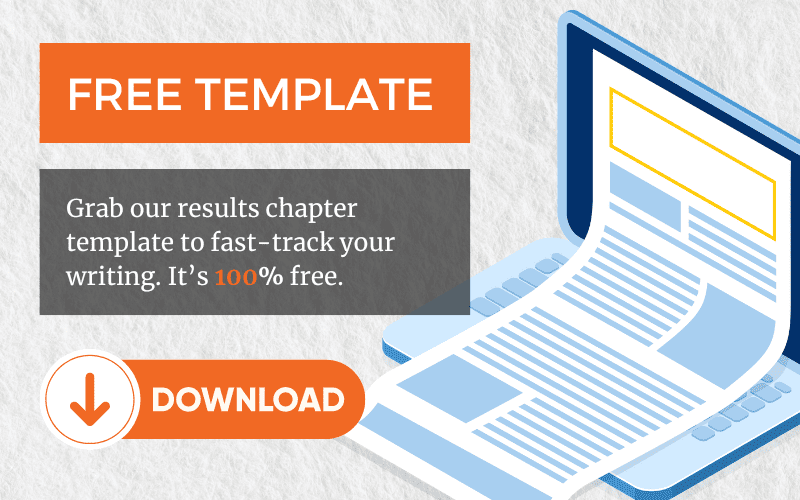
What should you include in the results chapter?
As we’ve mentioned, your qualitative results chapter should purely present and describe your results , not interpret them in relation to the existing literature or your research questions . Any speculations or discussion about the implications of your findings should be reserved for your discussion chapter.
In your results chapter, you’ll want to talk about your analysis findings and whether or not they support your hypotheses (if you have any). Naturally, the exact contents of your results chapter will depend on which qualitative analysis method (or methods) you use. For example, if you were to use thematic analysis, you’d detail the themes identified in your analysis, using extracts from the transcripts or text to support your claims.
While you do need to present your analysis findings in some detail, you should avoid dumping large amounts of raw data in this chapter. Instead, focus on presenting the key findings and using a handful of select quotes or text extracts to support each finding . The reams of data and analysis can be relegated to your appendices.
While it’s tempting to include every last detail you found in your qualitative analysis, it is important to make sure that you report only that which is relevant to your research aims, objectives and research questions . Always keep these three components, as well as your hypotheses (if you have any) front of mind when writing the chapter and use them as a filter to decide what’s relevant and what’s not.
Need a helping hand?
How do I write the results chapter?
Now that we’ve covered the basics, it’s time to look at how to structure your chapter. Broadly speaking, the results chapter needs to contain three core components – the introduction, the body and the concluding summary. Let’s take a look at each of these.
Section 1: Introduction
The first step is to craft a brief introduction to the chapter. This intro is vital as it provides some context for your findings. In your introduction, you should begin by reiterating your problem statement and research questions and highlight the purpose of your research . Make sure that you spell this out for the reader so that the rest of your chapter is well contextualised.
The next step is to briefly outline the structure of your results chapter. In other words, explain what’s included in the chapter and what the reader can expect. In the results chapter, you want to tell a story that is coherent, flows logically, and is easy to follow , so make sure that you plan your structure out well and convey that structure (at a high level), so that your reader is well oriented.
The introduction section shouldn’t be lengthy. Two or three short paragraphs should be more than adequate. It is merely an introduction and overview, not a summary of the chapter.
Pro Tip – To help you structure your chapter, it can be useful to set up an initial draft with (sub)section headings so that you’re able to easily (re)arrange parts of your chapter. This will also help your reader to follow your results and give your chapter some coherence. Be sure to use level-based heading styles (e.g. Heading 1, 2, 3 styles) to help the reader differentiate between levels visually. You can find these options in Word (example below).

Section 2: Body
Before we get started on what to include in the body of your chapter, it’s vital to remember that a results section should be completely objective and descriptive, not interpretive . So, be careful not to use words such as, “suggests” or “implies”, as these usually accompany some form of interpretation – that’s reserved for your discussion chapter.
The structure of your body section is very important , so make sure that you plan it out well. When planning out your qualitative results chapter, create sections and subsections so that you can maintain the flow of the story you’re trying to tell. Be sure to systematically and consistently describe each portion of results. Try to adopt a standardised structure for each portion so that you achieve a high level of consistency throughout the chapter.
For qualitative studies, results chapters tend to be structured according to themes , which makes it easier for readers to follow. However, keep in mind that not all results chapters have to be structured in this manner. For example, if you’re conducting a longitudinal study, you may want to structure your chapter chronologically. Similarly, you might structure this chapter based on your theoretical framework . The exact structure of your chapter will depend on the nature of your study , especially your research questions.
As you work through the body of your chapter, make sure that you use quotes to substantiate every one of your claims . You can present these quotes in italics to differentiate them from your own words. A general rule of thumb is to use at least two pieces of evidence per claim, and these should be linked directly to your data. Also, remember that you need to include all relevant results , not just the ones that support your assumptions or initial leanings.
In addition to including quotes, you can also link your claims to the data by using appendices , which you should reference throughout your text. When you reference, make sure that you include both the name/number of the appendix , as well as the line(s) from which you drew your data.
As referencing styles can vary greatly, be sure to look up the appendix referencing conventions of your university’s prescribed style (e.g. APA , Harvard, etc) and keep this consistent throughout your chapter.
Section 3: Concluding summary
The concluding summary is very important because it summarises your key findings and lays the foundation for the discussion chapter . Keep in mind that some readers may skip directly to this section (from the introduction section), so make sure that it can be read and understood well in isolation.
In this section, you need to remind the reader of the key findings. That is, the results that directly relate to your research questions and that you will build upon in your discussion chapter. Remember, your reader has digested a lot of information in this chapter, so you need to use this section to remind them of the most important takeaways.
Importantly, the concluding summary should not present any new information and should only describe what you’ve already presented in your chapter. Keep it concise – you’re not summarising the whole chapter, just the essentials.
Tips for writing an A-grade results chapter
Now that you’ve got a clear picture of what the qualitative results chapter is all about, here are some quick tips and reminders to help you craft a high-quality chapter:
- Your results chapter should be written in the past tense . You’ve done the work already, so you want to tell the reader what you found , not what you are currently finding .
- Make sure that you review your work multiple times and check that every claim is adequately backed up by evidence . Aim for at least two examples per claim, and make use of an appendix to reference these.
- When writing up your results, make sure that you stick to only what is relevant . Don’t waste time on data that are not relevant to your research objectives and research questions.
- Use headings and subheadings to create an intuitive, easy to follow piece of writing. Make use of Microsoft Word’s “heading styles” and be sure to use them consistently.
- When referring to numerical data, tables and figures can provide a useful visual aid. When using these, make sure that they can be read and understood independent of your body text (i.e. that they can stand-alone). To this end, use clear, concise labels for each of your tables or figures and make use of colours to code indicate differences or hierarchy.
- Similarly, when you’re writing up your chapter, it can be useful to highlight topics and themes in different colours . This can help you to differentiate between your data if you get a bit overwhelmed and will also help you to ensure that your results flow logically and coherently.
If you have any questions, leave a comment below and we’ll do our best to help. If you’d like 1-on-1 help with your results chapter (or any chapter of your dissertation or thesis), check out our private dissertation coaching service here or book a free initial consultation to discuss how we can help you.
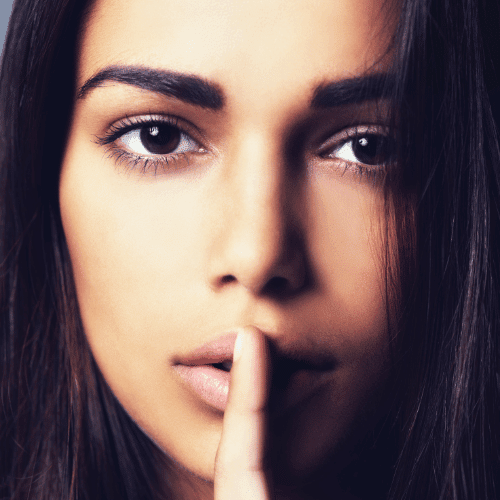
Psst... there’s more!
This post was based on one of our popular Research Bootcamps . If you're working on a research project, you'll definitely want to check this out ...
You Might Also Like:
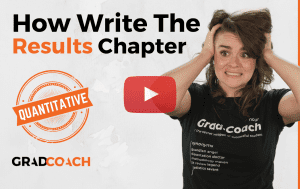
20 Comments
This was extremely helpful. Thanks a lot guys
Hi, thanks for the great research support platform created by the gradcoach team!
I wanted to ask- While “suggests” or “implies” are interpretive terms, what terms could we use for the results chapter? Could you share some examples of descriptive terms?
I think that instead of saying, ‘The data suggested, or The data implied,’ you can say, ‘The Data showed or revealed, or illustrated or outlined’…If interview data, you may say Jane Doe illuminated or elaborated, or Jane Doe described… or Jane Doe expressed or stated.
I found this article very useful. Thank you very much for the outstanding work you are doing.
What if i have 3 different interviewees answering the same interview questions? Should i then present the results in form of the table with the division on the 3 perspectives or rather give a results in form of the text and highlight who said what?
I think this tabular representation of results is a great idea. I am doing it too along with the text. Thanks
That was helpful was struggling to separate the discussion from the findings
this was very useful, Thank you.
Very helpful, I am confident to write my results chapter now.
It is so helpful! It is a good job. Thank you very much!
Very useful, well explained. Many thanks.
Hello, I appreciate the way you provided a supportive comments about qualitative results presenting tips
I loved this! It explains everything needed, and it has helped me better organize my thoughts. What words should I not use while writing my results section, other than subjective ones.
Thanks a lot, it is really helpful
Thank you so much dear, i really appropriate your nice explanations about this.
Thank you so much for this! I was wondering if anyone could help with how to prproperly integrate quotations (Excerpts) from interviews in the finding chapter in a qualitative research. Please GradCoach, address this issue and provide examples.
what if I’m not doing any interviews myself and all the information is coming from case studies that have already done the research.
Very helpful thank you.
This was very helpful as I was wondering how to structure this part of my dissertation, to include the quotes… Thanks for this explanation
This is very helpful, thanks! I am required to write up my results chapters with the discussion in each of them – any tips and tricks for this strategy?
Submit a Comment Cancel reply
Your email address will not be published. Required fields are marked *
Save my name, email, and website in this browser for the next time I comment.
- Print Friendly
- Skip to main content
- Skip to primary sidebar
- Skip to footer
- QuestionPro

- Solutions Industries Gaming Automotive Sports and events Education Government Travel & Hospitality Financial Services Healthcare Cannabis Technology Use Case NPS+ Communities Audience Contactless surveys Mobile LivePolls Member Experience GDPR Positive People Science 360 Feedback Surveys
- Resources Blog eBooks Survey Templates Case Studies Training Help center

Home Surveys
Survey Data Collection: Definition, Methods with Examples and Analysis
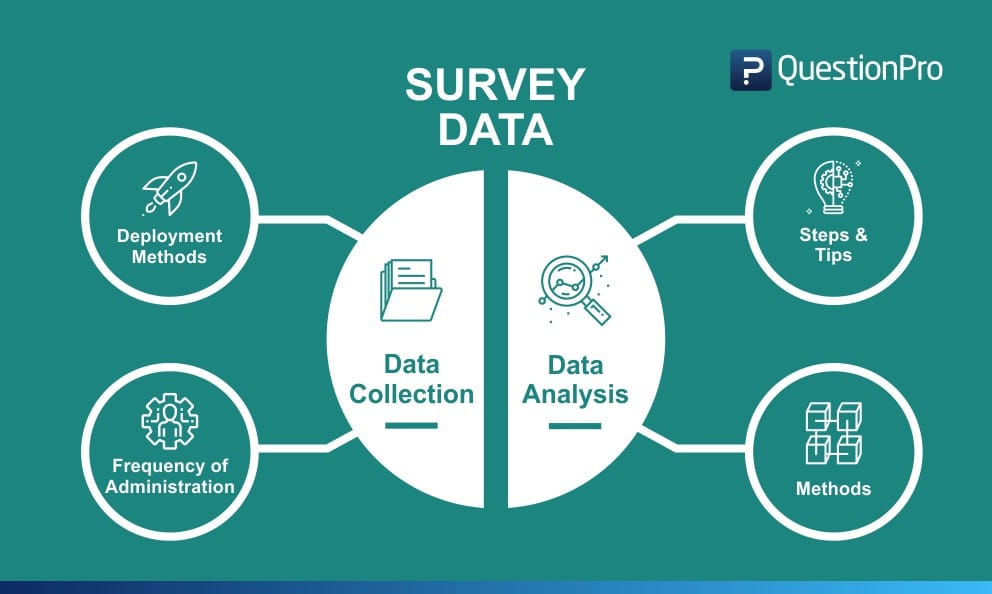
Survey Data: Definition
Survey data is defined as the resultant data that is collected from a sample of respondents that took a survey . This data is comprehensive information gathered from a target audience about a specific topic to conduct research. There are many methods used for survey data collection and statistical analysis .
Various mediums are used to collect feedback and opinions from the desired sample of individuals. While conducting survey research, researchers prefer multiple sources to gather data such as online surveys , telephonic surveys, face-to-face surveys, etc. The medium of collecting survey data decides the sample of people that are to be reached out to, to reach the requisite number of survey responses.
LEARN ABOUT: Survey Sample Sizes
Factors of collecting survey data such as how the interviewer will contact the respondent (online or offline), how the information is communicated to the respondents etc. decide the effectiveness of gathered data.
LEARN ABOUT: telephone survey
Survey Data Collection Methods with Examples
The methods used to collect survey data have evolved with the change in technology. From face-to-face surveys, telephonic surveys to now online and email surveys , the world of survey data collection has changed with time. Each survey data collection method has its pros and cons, and every researcher has a preference for gathering accurate information from the target sample.
The survey response rates for each of these data collection methods will differ as their reach and impact are always different. Different ways are chosen according to specific target population characteristics and the intent to examine human nature under various situations.
Types of survey data based on deployment methods:
There are four main survey data collection methods – Telephonic Surveys, Face-to-face Surveys, and Online Surveys.
- Online Surveys
Online surveys are the most cost-effective and can reach the maximum number of people in comparison to the other mediums. The performance of these surveys is much more widespread than the other data collection methods. In situations where there is more than one question to be asked to the target sample, certain researchers prefer conducting online surveys over the traditional face-to-face or telephone surveys.
Online surveys are effective and therefore require computational logic and branching technologies for exponentially more accurate survey data collection vs any other traditional means of surveying. They are straightforward in their implementation and take a minimum time of the respondents. The investment required for survey data collection using online surveys is also negligible in comparison to the other methods. The results are collected in real-time for researchers to analyze and decide corrective measures.
A very good example of an online survey is a hotel chain using an online survey to collect guest satisfaction metrics after a stay or an event at the property.
Learn more: Quality Of Life Survey Questions + Sample Questionnaire Template
Online surveys are safe and secure to conduct. As there is no in-person interaction or any direct form of communication, they are quite useful in times of global crisis. For instance, many organizations moved to contactless surveys during the pandemic. It helped them ensure that the employees are not experiencing any COVID-19 symptoms before they come to the office.
Learn more: Contactless Health-Screen Survey Questions + Sample Questionnaire Template
- Face-to-face Surveys
Gaining information from respondents via face-to-face mediums is much more effective than the other mediums because respondents usually tend to trust the surveyors and provide honest and clear feedback about the subject in-hand.
Researchers can easily identify whether their respondents are uncomfortable with the asked questions and can be extremely productive in case there are sensitive topics involved in the discussion. This online data collection method demands more cost-investment than in comparison to the other methods. According to the geographic segmentation or psychographic segmentation , researchers must be trained to gain accurate information.
For example, a job evaluation survey is conducted in person between an HR or a manager with the employee. This method works best face-to-face as the data collection can collect as accurate information as possible.
LEARN ABOUT: Workforce Planning Model
- Telephone Surveys
Telephone surveys require much lesser investment than face-to-face surveys. Depending on the required reach, telephone surveys cost as much or a little more than online surveys. Contacting respondents via the telephonic medium requires less effort and manpower than the face-to-face survey medium.
If interviewers are located at the same place, they can cross-check their questions to ensure error-free questions are asked to the target audience. The main drawback of conducting telephone surveys is that establishing a friendly equation with the respondent becomes challenging due to the bridge of the medium. Respondents are also highly likely to choose to remain anonymous in their feedback over the phone as the reliability associated with the researcher can be questioned.
For example, if a retail giant would like to understand purchasing decisions, they can conduct a telephonic, motivation, and buying experience survey to collect data about the entire purchasing experience.
LEARN ABOUT: Anonymous Surveys
- Paper Surveys
The other commonly used survey method is paper surveys. These surveys can be used where laptops, computers, and tablets cannot go, and hence they use the age-old method of data collection ; pen and paper. This method helps collect survey data in field research and helps strengthen the number of responses collected and the validity of these responses.
A popular example or use case of a paper survey is a fast-food restaurant survey, where the fast-food chain would like to collect feedback on its patrons’ dining experience.
Types of survey data based on the frequency at which they are administered:
Surveys can be divided into 3 distinctive types on the basis of the frequency of their distribution. They are:
- Cross-Sectional Surveys
Cross-sectional surveys is an observational research method that analyzes data of variables collected at one given point of time across a sample population or a pre-defined subset. The survey data from this method helps the researcher understand what the respondent feels at a certain point. It helps measure opinions in a particular situation.
For example, if the researcher would like to understand movie rental habits, a survey can be conducted across demographics and geographical locations. The cross-sectional study , for example, can help understand that males between 21-28 rent action movies and females between 35-45 rent romantic comedies. This survey data helps for the basis of a longitudinal study .
LEARN ABOUT: Real Estate Surveys
- Longitudinal Surveys
Longitudinal surveys are those surveys that help researchers to make an observation and collect data over an extended period of time. This survey data can be qualitative or quantitative in nature, and the survey creator does not interfere with the survey respondents.
For example, a longitudinal study can be carried out for years to help understand if mine workers are more prone to lung diseases. This study takes a year and discounts any pre-existing conditions.
- Retrospective Surveys
In retrospective surveys, researchers ask respondents to report events from the past. This survey method offers in-depth survey data but doesn’t take as long to complete. By deploying this kind of survey, researchers can gather data based on past experiences and beliefs of people.
For example, if hikers are asked about a certain hike – the conditions of the hiking trail, ease of hike, weather conditions, trekking conditions, etc. after they have completed the trek, it is a retrospective study.
LEARN ABOUT: Powerful Survey Generator
Survey Data Analysis
After the survey data has been collected, this data has to be analyzed to ensure it aids towards the end research objective. There are different ways of conducting this research and some steps to follow. They are as below:
Survey Data Analysis: Steps and Tips
There are four main steps of survey data analysis :
- Understand the most popular survey research questions: The survey format questions should align with the overall purpose of the survey. That is when the collected data will be effective in helping researchers. For example, if a seminar has been conducted, the researchers will send out a post-seminar feedback survey. The primary goal of this survey will be to understand whether the attendees are interested in attending future seminars. The question will be: “How likely are you to attend future seminars?” – Data collected for this question will decide the likelihood of success of future seminars.
- Filter obtained results using the cross-tabulation technique: Understand the various categories in the target audience and their thoughts using cross-tabulation format. For example, if there are business owners, administrators, students, etc. who attend the seminar, the data about whether they would prefer attending future seminars or not can be represented using cross-tabulation.
- Evaluate the derived numbers: Analyzing the gathered information is critical. How many of the attendees are of the opinion that they will be attending future seminars and how many will not – these facts need to be evaluated according to the results obtained from the sample.
- Draw conclusions: Weave a story with the collected and analyzed data. What was the intention of the survey research, and how does the survey data suffice that objective? – Understand that and develop accurate, conclusive results.
Survey Data Analysis Methods
Conducting a survey without having access to the resultant data and the inability to drawing conclusions from the survey data is pointless. When you conduct a survey, it is imperative to have access to its analytics. It is tough to analyze using traditional survey methods like pen and paper and also requires additional manpower. Survey data analysis becomes much easier when using advanced online data collection methods with an online survey platform such as market research survey software or customer survey software.
LEARN ABOUT: Top 12 Tips to Create A Good Survey
Statistical analysis can be conducted on the survey data to make sense of the data that has been collected. There are multiple data analysis methods of quantitative data . Some of the commonly used types are:
- Cross-tabulation: Cross-tabulation is the most widely used data analysis methods. It uses a basic tabulation framework to make sense of data. This statistical analysis method helps tabulate data into easily understandable rows and columns, and this helps draw parallels between different research parameters. It contains data that is mutually exclusive or have some connection with each other.
- Trend analysis: Trend analysis is a statistical analysis method that provides the ability to look at survey-data over a long period of time. This method helps plot aggregated response data over time allows drawing a trend line of the change, if any, of perceptions over time about a common variable.
- MaxDiff analysis: The MaxDiff analysis method is used to gauge what a customer prefers in a product or a service across multiple parameters. For example, a product’s feature list, the difference with the competition, ease of use and likert scale , pricing, etc. form the basis for maxdiff analysis. In a simplistic form, this method is also called the “best-worst” method. This method is very similar to conjoint analysis, but it is much easier to implement and can be interchangeably used.
LEARN ABOUT: System Usability Scale
- Conjoint analysis: As mentioned above, conjoint analysis is similar to maxdiff analysis, only differing in its complexity and the ability to collect and analyze advance survey data. This method analyzes each parameter behind a person’s purchasing behavior. By using conjoint analysis, it is possible to understand what exactly is important to a customer and the aspects that are evaluated before purchase.
- TURF analysis: TURF analysis or Total Unduplicated Reach and Frequency analysis, is a statistical research methodology that assesses the total market reach of a product or service or a mix of both. This method is used by organizations to understand the frequency and the avenues at which their messaging reaches customers and prospective customers. This helps them tweak their go-to-market strategies.
- Gap analysis: Gap analysis uses a side-by-side matrix question type that helps measure the difference between expected performance and actual performance. This statistical method for survey data helps understand the things that have to be done to move performance from actual to planned performance.
- SWOT analysis: SWOT analysis , another widely used statistical method, organizes survey data into data that represents the strength, weaknesses, opportunities, and threats of an organization or product or service that provides a holistic picture of competition. This method helps to create effective business strategies.
- Text analysis: Text analysis is an advanced statistical method where intelligent tools make sense of and quantify or fashion qualitative and open-ended data into easily understandable data. This method is used when the survey data is unstructured.
MORE LIKE THIS
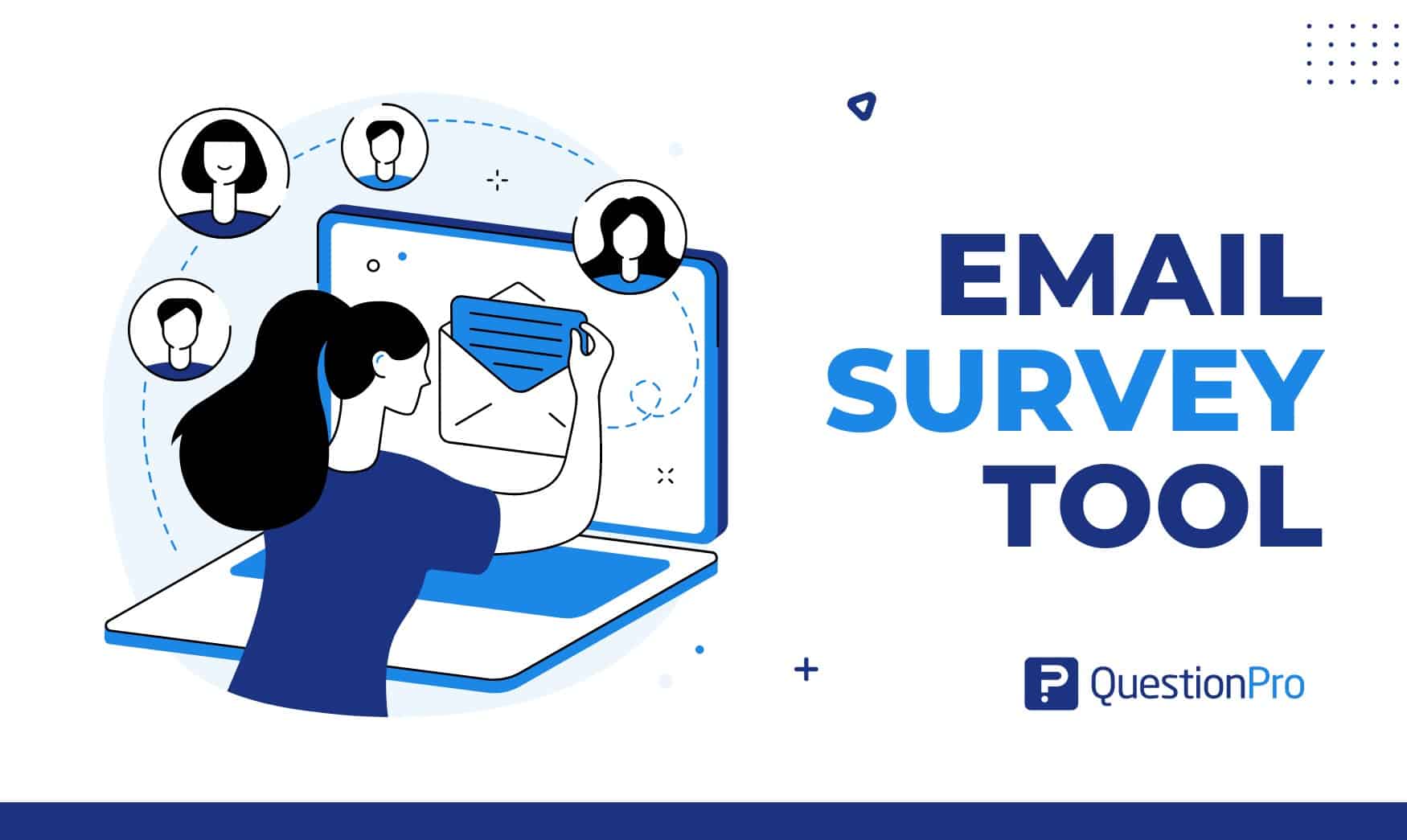
The Best Email Survey Tool to Boost Your Feedback Game
May 7, 2024
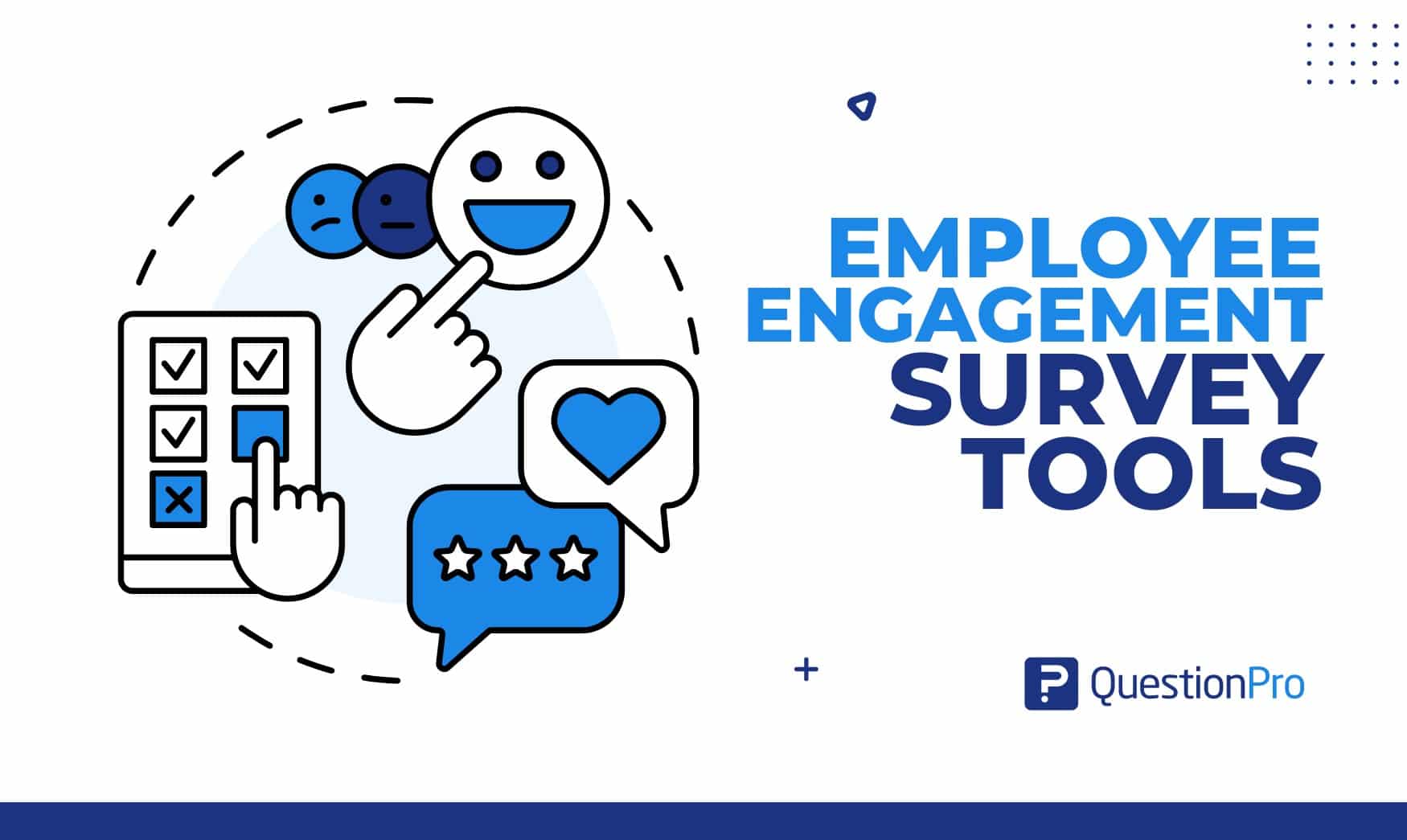
Top 10 Employee Engagement Survey Tools
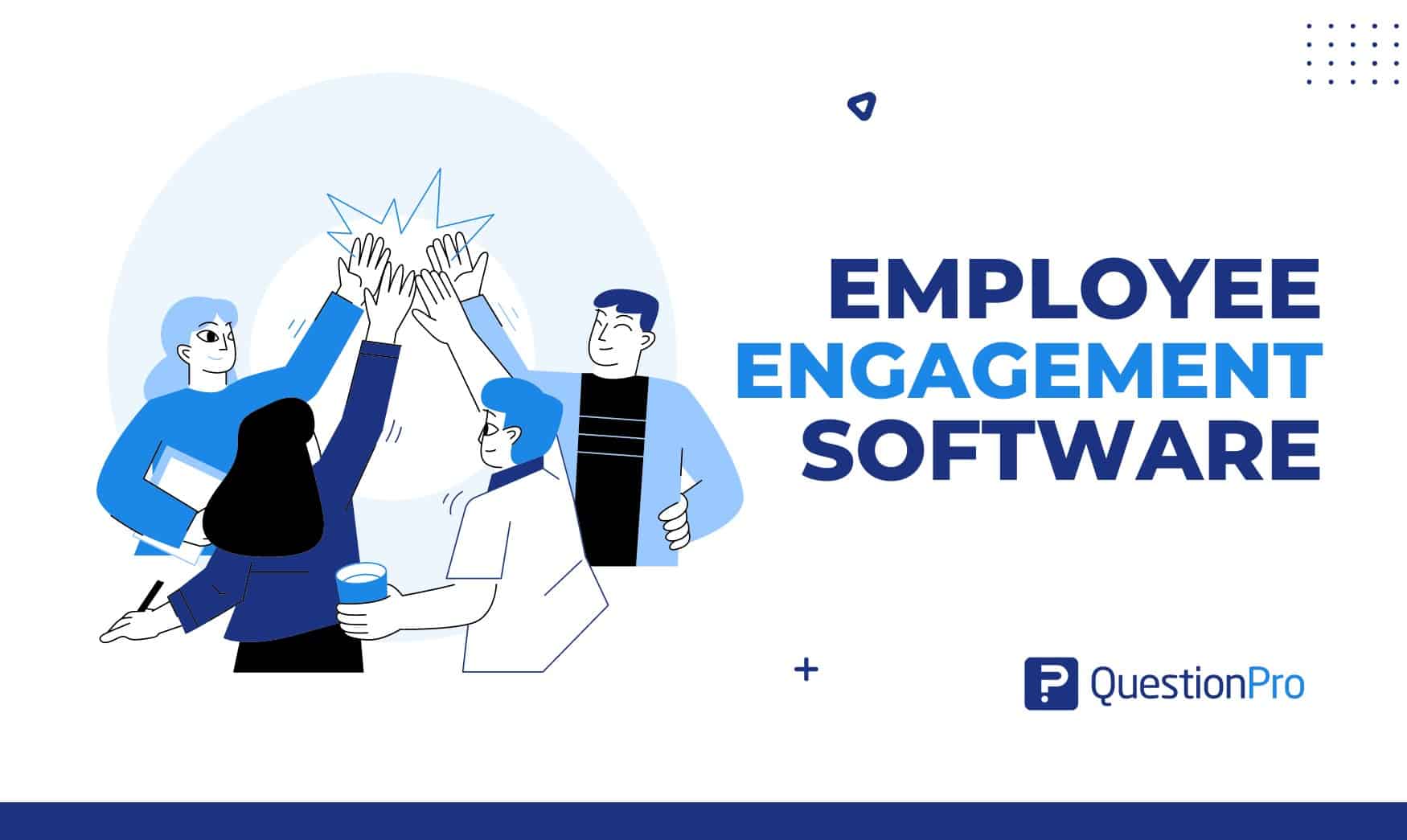
Top 20 Employee Engagement Software Solutions
May 3, 2024
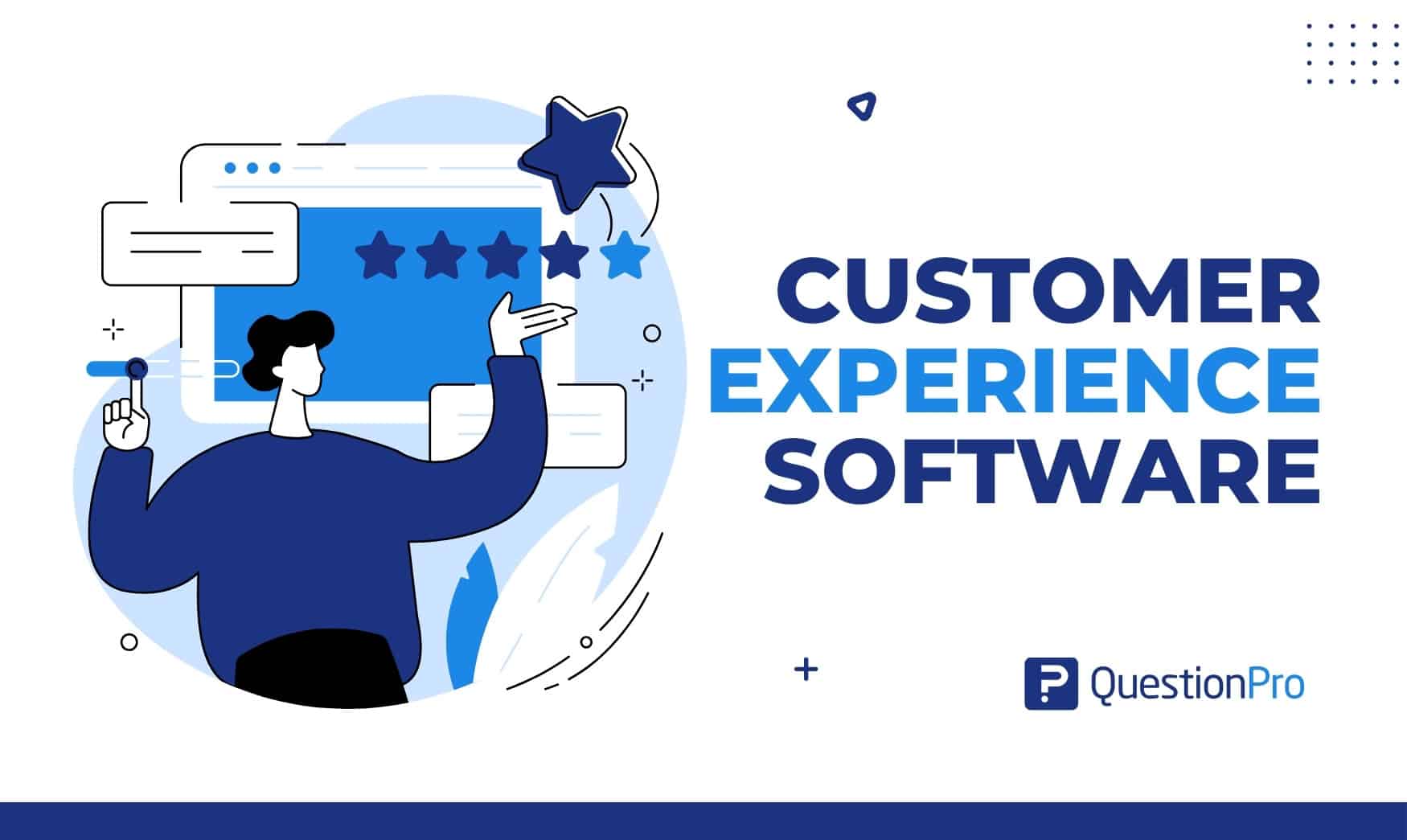
15 Best Customer Experience Software of 2024
May 2, 2024
Other categories
- Academic Research
- Artificial Intelligence
- Assessments
- Brand Awareness
- Case Studies
- Communities
- Consumer Insights
- Customer effort score
- Customer Engagement
- Customer Experience
- Customer Loyalty
- Customer Research
- Customer Satisfaction
- Employee Benefits
- Employee Engagement
- Employee Retention
- Friday Five
- General Data Protection Regulation
- Insights Hub
- Life@QuestionPro
- Market Research
- Mobile diaries
- Mobile Surveys
- New Features
- Online Communities
- Question Types
- Questionnaire
- QuestionPro Products
- Release Notes
- Research Tools and Apps
- Revenue at Risk
- Survey Templates
- Training Tips
- Uncategorized
- Video Learning Series
- What’s Coming Up
- Workforce Intelligence
Product Overview
SurveyMonkey is built to handle every use case and need. Explore our product to learn how SurveyMonkey can work for you.
SurveyMonkey
Get data-driven insights from a global leader in online surveys.
Integrations
Integrate with 100+ apps and plug-ins to get more done.
SurveyMonkey Forms
Build and customize online forms to collect info and payments.
SurveyMonkey Genius
Create better surveys and spot insights quickly with built-in AI.
Market Research Solutions
Purpose-built solutions for all of your market research needs.
Financial Services
See more industries, customer experience, human resources, see more roles.
Online Polls
Registration Forms
Employee feedback, event feedback, customer satisfaction, see more use cases.
Contact Sales
Net Promoter Score
Measure customer satisfaction and loyalty for your business.
Learn what makes customers happy and turn them into advocates.
Website Feedback
Get actionable insights to improve the user experience.
Contact Information
Collect contact information from prospects, invitees, and more.
Event Registration
Easily collect and track RSVPs for your next event.
Find out what attendees want so that you can improve your next event.
Employee Engagement
Uncover insights to boost engagement and drive better results.
Meeting Feedback
Get feedback from your attendees so you can run better meetings.
360-degree employee evaluation
Use peer feedback to help improve employee performance.
Course Evaluation
Create better courses and improve teaching methods.
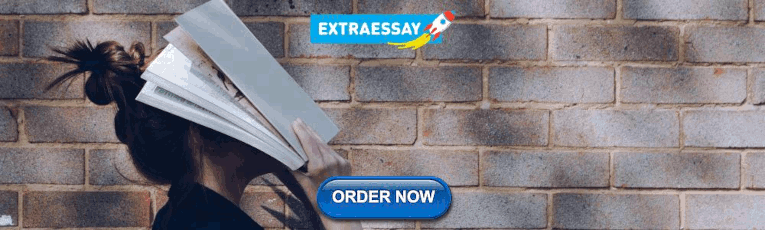
University Instructor Evaluation
Learn how students rate the course material and its presentation.
Product Testing
Find out what your customers think about your new product ideas.
See all templates
Resource center.
Best practices for using surveys and survey data
Curiosity at Work Blog
Our blog about surveys, tips for business, and more.
Help Center
Tutorials and how to guides for using SurveyMonkey.
How top brands drive growth with SurveyMonkey.
- English (US)
- English (UK)
How to analyze survey data
Learn how SurveyMonkey can help you analyze your survey data effectively, as well as create better surveys with ease.
The results are back from your online surveys. Now it’s time to tap the power of survey data analysis to make sense of the results and present them in ways that are easy to understand and act on.After you’ve collected statistical survey results and have a data analysis plan , it’s time to begin the process of calculating survey results you got back. Here’s how our survey research scientists make sense of quantitative data (versus qualitative data ). They structure their reporting around survey responses that will answer research questions. Even for the experts, it can be hard to parse the insights in raw data.
In order to reach your survey goals, you’ll want to start with relying on the survey methodology suggested by our experts. Then once you have results, you can effectively analyze them using all the data analysis tools available to you including statistical analysis, data analytics, and charts and graphs that capture your survey metrics.
Build a survey analytics team for deeper insights
Add analysts to any team plan for even bigger impact.
Survey data analysis made easy
Sound survey data analysis is key to getting the information and insights you need to make better business decisions. Yet it’s important to be aware of potential challenges that can make analysis more difficult or even skew results.
Asking too many open-ended questions can add time and complexity to your analysis because it produces qualitative results that aren’t numerically based. Meanwhile, closed-ended questions generate results that are easier to analyze. Analysis can also be hampered by asking leading or biased questions or posing questions that are confusing or too complex. Being equipped with the right tools and know-how helps assure that survey analysis is both easy and effective.
Read more about using closed-ended vs. open-ended questions .
See how SurveyMonkey makes analyzing results a breeze
With its many data analysis techniques , SurveyMonkey makes it easy for you to turn your raw data into actionable insights presented in easy-to-grasp formats. Features such as automatic charts and graphs and word clouds help bring data to life. For instance, Sentiment Analysis allows you to get an instant summary of how people feel from thousands or even millions of open text responses. You can review positive, neutral, and negative sentiments and a glance or filter by sentiment to identify areas that need attention. For even deeper insights, you can filter a question by sentiment. Imagine being able to turn all those text responses into a quantitative data set.
Word clouds let you quickly interpret open-ended responses through a visual display of the most frequently used words. You can customize the look of your word clouds in a range of ways from selecting colors or fonts for specific words to easily hiding non-relevant words.
Our wide range of features and tools can help you address analysis challenges, and quickly generate graphics and robust reports. Check out how a last-minute report request can be met in a snap through SurveyMonkey.
Ready to get started?
To begin calculating survey results more effectively, follow these 6 steps:
- Take a look at your top survey questions
Determine sample size
- Use cross tabulation to filter your results
Benchmarking, trending, and comparative data
- Crunch the numbers
- Draw conclusions
Calculating results using your top survey questions
First, let’s talk about how you’d go about calculating survey results from your top research questions. Did you feature empirical research questions? Did you consider probability sampling ? Remember that you should have outlined your top research questions when you set a goal for your survey.
For example, if you held an education conference and gave attendees a post-event feedback survey , one of your top research questions may look like this: How did the attendees rate the conference overall? Now take a look at the answers you collected for a specific survey question that speaks to that top research question:
Do you plan to attend this conference next year?
Notice that in the responses, you’ve got some percentages (71%, 18%) and some raw numbers (852, 216). The percentages are just that—the percent of people who gave a particular answer. Put another way, the percentages represent the number of people who gave each answer as a proportion of the number of people who answered the question. So, 71% of your survey respondents (852 of the 1,200 surveyed) plan on coming back next year.
This table also shows you that 18% say they are planning to return and 11% say they are not sure.
Having a good understanding of sample size is also key to making sure you are accurately and effectively analyzing your survey results. Sample size is how many people you need to take your survey and complete responses to make it statistically viable. Even if you’re a statistician, determining survey sample size can be a challenge. But SurveyMonkey takes the guesswork and complexity out of the process with its easy-to-use margin of error calculator that helps you determine how many people you need to survey to ensure your results help you avoid your margin or error.
Looking for more responses to your surveys?
Trust the panel of respondents provided by SurveyMonkey Audience—175M+ people across 130+ countries.
Cross-tabulation and filtering results
Recall that when you set a goal for your survey and developed your analysis plan, you thought about what subgroups you were going to analyze and compare. Now is when that planning pays off. For example, say you wanted to see how teachers, students, and administrators compared to one another their responses about attending next year’s conference. To figure this out, you want to dive into response rates by means of cross tabulation , or use cross tab reports, where you show the results of the conference question by subgroup:
From this table you see that a large majority of the students (86%) and teachers (80%) plan to come back next year. However, the administrators who attended your conference look different, with under half (46%) of them intending to come back! Hopefully, some of our other questions will help you figure out why this is the case and what you can do to improve the conference for administrators so more of them will return year after year.
A filter is another method of data analysis when you’re modeling data. Filtering means narrowing your focus to one particular subgroup, and filtering out the others. So, instead of comparing subgroups to one another, here we’re just looking at how one subgroup answered the question. Combining filters can give you pinpoint accuracy in your data.
For instance, you could limit your focus to just women, or just men, then re-run the crosstab by type of attendee to compare female administrators, female teachers, and female students. One thing to be wary of as you slice and dice your results: Every time you apply a filter or cross tab, your sample size decreases. To make sure your results are statistically significant, it may be helpful to use a sample size calculator .
How graphs add clarity to data analysis
Graphs can be a regular go-to tool when you aim to quickly demonstrate the results of your data analysis in a way that is easy for anyone to understand. It’s easy to create graphs with SurveyMonkey that provide clarity and context to your analysis which, in turn, makes using the data in more targeted and actionable ways.
Cross tabulations, otherwise known as crosstab reports, are useful tools for taking a deeper dive into your data. Crosstabs structure your data into a table that groups respondents based on shared background information or survey responses, allowing you to compare each group’s answers to one another. This helps you better understand each group of respondents and uncover how they differ from each other.
Let’s say on your conference feedback survey, one key question is, “Overall how satisfied were you with the conference?”
Your results show that 75% of the attendees were satisfied with the conference. That sounds pretty good. But wouldn’t you like to have some context? Something to compare it against? Is that better or worse than last year? How does it compare to other conferences?
Benchmarking can provide answers to these questions and more by readily allowing you to make comparisons to past and current data to identify trends in your industry and marketplace, and see how you stack up against them.
Well, say you did ask this question in your conference feedback survey after last year’s conference. You’d be able to make a trend comparison. Professional pollsters make poor comedians, but one favorite line is “trend is your friend.” If last year’s satisfaction rate was 60%, you increased satisfaction by 15 percentage points! What caused this increase in satisfaction? Hopefully the responses to other questions in your survey will provide some answers.
If you don’t have data from prior years’ conferences, make this the year you start collecting feedback after every conference. This is called benchmarking. You establish a benchmark or baseline number and, moving forward, you can see whether and how this has changed. You can benchmark not just attendees’ satisfaction, but other questions as well. You’ll be able to track, year after year, what attendees think of the conference. This is called longitudinal data analysis .
You can even track data for different subgroups. Say for example that satisfaction rates are increasing year over year for students and teachers, but not for administrators. You might want to look at administrators’ responses to various questions to see if you can gain insight into why they are less satisfied than other attendees.
Crunching the numbers
You know how many people said they were coming back, but how do you know if your survey has yielded answers that you can trust and answers that you can use with confidence to inform future decisions? It’s important to pay attention to the quality of your data and to understand the components of statistical significance.
In everyday conversation, the word “significant” means important or meaningful. In survey analysis and statistics, significant means “an assessment of accuracy.” This is where the inevitable “plus or minus” comes into survey work. In particular, it means that survey results are accurate within a certain confidence level and not due to random chance. Drawing an inference based on results that are inaccurate (i.e., not statistically significant) is risky. The first factor to consider in any assessment of statistical significance is the representativeness of your sample—that is, to what extent the group of people who were included in your survey “look like” the total population of people about whom you want to draw conclusions.
You have a problem if 90% of conference attendees who completed the survey were men, but only 15% of all your conference attendees were male. The more you know about the population you are interested in studying, the more confident you can be when your survey lines up with those numbers. At least when it comes to gender, you’re feeling pretty good if men make up 15% of survey respondents in this example.
If your survey sample is a random selection from a known population, statistical significance can be calculated in a straightforward manner. A primary factor here is sample size . Suppose 50 of the 1,000 people who attended your conference replied to the survey. Fifty (50) is a small sample size and results in a broad margin of error . In short, your results won’t carry much weight.
Say you asked your survey respondents how many of the 10 available sessions they attended over the course of the conference. And your results look like this:
You might want to analyze the average. As you may recall, there are three different kinds of averages: mean, median and mode.
In the table above, the average number of sessions attended is 6.1. The average reported here is the mean, the kind of average that’s probably most familiar to you. To determine the mean you add up the data and divide that by the number of figures you added. In this example, you have 100 people saying they attended one session, 50 people for four sessions, 100 people for five sessions, etc. So, you multiply all of these pairs together, sum them up, and divide by the total number of people.
The median is another kind of average. The median is the middle value, the 50% mark. In the table above, we would locate the number of sessions where 500 people were to the left of the number and 500 to the right. The median is, in this case, six sessions. This can help you eliminate the influence of outliers, which may adversely affect your data.
The last kind of average is mode. The mode is the most frequent response. In this case the answer is six. 260 survey participants attended six sessions, more than attended any other number of sessions.
Means and other types of averages can also be used if your results were based on Likert scales .
Drawing conclusions
When it comes to reporting on survey results , think about the story the data tells.
Say your conference overall got mediocre ratings. You dig deeper to find out what’s going on. The data show that attendees gave very high ratings to almost all the aspects of your conference — the sessions and classes, the social events, and the hotel—but they really disliked the city chosen for the conference. (Maybe the conference was held in Chicago in January and it was too cold for anyone to go outside!)
That is part of the story right there—great conference overall, lousy choice of locations. Miami or San Diego might be a better choice for a winter conference.
One aspect of data analysis and reporting you have to consider is causation vs. correlation.
Questions about different types of data analysis techniques
People digest and understand information in a range of different ways. Fortunately, SurveyMonkey offers a ton of different ways for you to analyze survey data so you can assess and present the information in ways that will be most useful to meet your goals and create graphs, charts, and reports that make your results easy to understand.
Here are some of the common questions that we can help you navigate as you build up your survey analysis chops:
What is longitudinal analysis?
Longitudinal data analysis (often called “trend analysis”) is basically tracking how findings for specific questions change over time. Once a benchmark is established, you can determine whether and how numbers shift. Suppose the satisfaction rate for your conference was 50% three years ago, 55% two years ago, 65% last year, and 75% this year. Congratulations are in order! Your longitudinal data analysis shows a solid, upward trend in satisfaction.
What is the difference between correlation and causation?
Causation is when one factor causes another, while correlation is when two variables move together, but one does not influence or cause the other. For example, drinking hot chocolate and wearing mittens are two variables that are correlated — they tend to go up and down together. However, one does not cause the other. In fact, they are both caused by a third factor, cold weather.
Cold weather influences both hot chocolate consumption and the likelihood of wearing mittens. Cold weather is the independent variable and hot chocolate consumption and the likelihood of wearing mittens are the dependent variables. In the case of our conference feedback survey, cold weather likely influenced attendees dissatisfaction with the conference city and the conference overall.
Finally, to further examine the relationship between variables in your survey you might need to perform a regression analysis.
What is regression analysis?
Regression analysis is an advanced method of data visualization and analysis that allows you to look at the relationship between two or more variables. There are many types of regression analysis and the one(s) a survey scientist chooses will depend on the variables he or she is examining. What all types of regression analysis have in common is that they look at the influence of one or more independent variables on a dependent variable. In analyzing our survey data we might be interested in knowing what factors most impact attendees’ satisfaction with the conference. Is it a matter of the number of sessions? The keynote speaker? The social events? The site? Using regression analysis, a survey scientist can determine whether and to what extent satisfaction with these different attributes of the conference contribute to overall satisfaction.
This, in turn, provides insight into what aspects of the conference you might want to alter next time around. Say, for example, you paid a high honorarium to get a top flight keynote speaker for your opening session. Participants gave this speaker and the conference overall high marks. Based on these two facts you might think that having a fabulous (and expensive) keynote speaker is the key to conference success. Regression analysis can help you determine if this is indeed the case. You might find that the popularity of the keynote speaker was a major driver of satisfaction with the conference. If so, next year you’ll want to get a great keynote speaker again. But say the regression shows that, while everyone liked the speaker, this did not contribute much to attendees’ satisfaction with the conference. If that is the case, the big bucks spent on the speaker might be best spent elsewhere.
If you take the time to carefully analyze the soundness of your survey data, you’ll be on your way to using the answers to help you make informed decisions.
Survey data can be one of your most powerful tools
By analyzing data in fresh, engaging and insightful ways, you can help drive your company’s growth, deepen customer relationships, and stay steps ahead of the competition. SurveyMonkey has a range of options to meet any budget.
Discover more resources
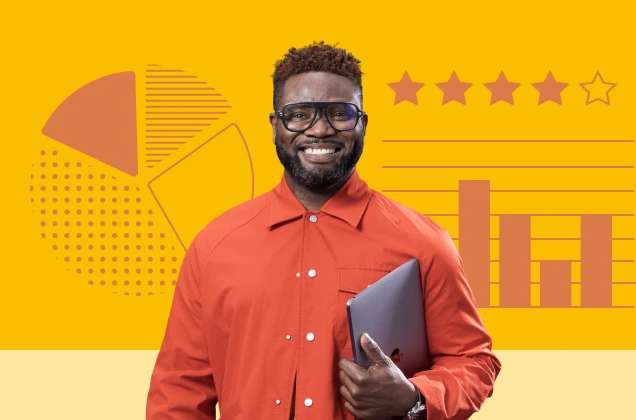
Toolkits directory
Discover our toolkits, designed to help you leverage feedback in your role or industry.
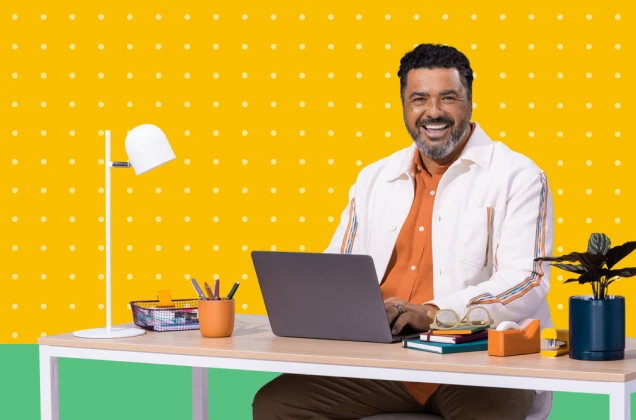
Receive requests easily with online request forms
Create and customize request forms easily to receive requests from employees, customers, and more. Use our expert-built templates to get started in minutes.
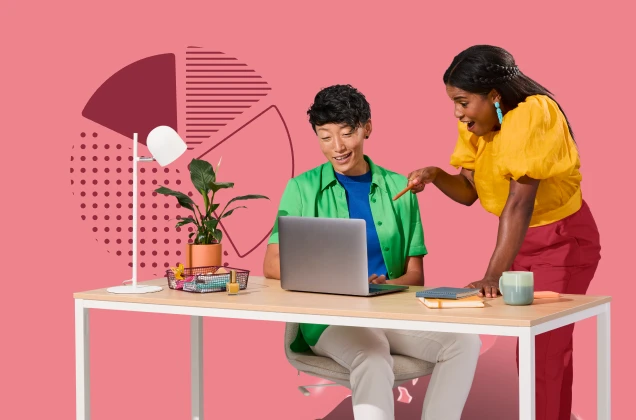
Considering a form vs. a survey? Use both to enhance your events and experiences
How do surveys and forms differ? Depends on the info you need. Here’s how to combine form data with survey feedback for seamless events and experiences.
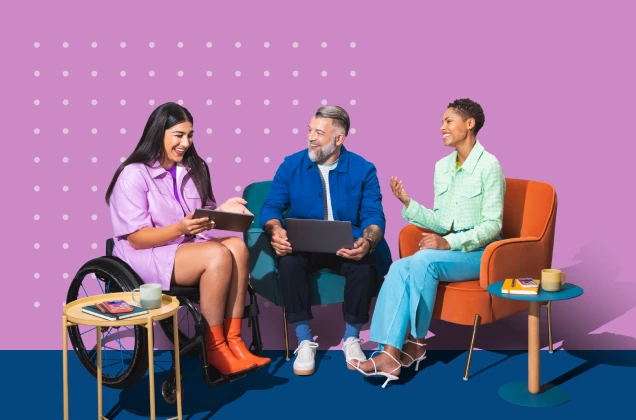
Unlocking ROI with Salesforce and SurveyMonkey
How to enrich your survey data with existing customer data in Salesforce to drive better CX and NPS, CSAT, and more.
Analyze your next survey with SurveyMonkey
What is survey data collection.
Survey data collection uses surveys to gather information from specific respondents. Survey data collection can replace or supplement other data collection types, including interviews, focus groups, and more. The data collected from surveys can be used to boost employee engagement, understand buyer behavior, and improve customer experiences.
Causation is when one factor causes another, while correlation is when two variables move together, but one does not influence or cause the other. For example, drinking hot chocolate and wearing mittens are two variables that are correlated — they tend to go up and down together. However, one does not cause the other. In fact, they are both caused by a third factor, cold weather. Cold weather influences both hot chocolate consumption and the likelihood of wearing mittens. Cold weather is the independent variable and hot chocolate consumption and the likelihood of wearing mittens are the dependent variables. In the case of our conference feedback survey, cold weather likely influenced attendees dissatisfaction with the conference city and the conference overall. Finally, to further examine the relationship between variables in your survey you might need to perform a regression analysis.
Regression analysis is an advanced method of data visualization and analysis that allows you to look at the relationship between two or more variables. There a many types of regression analysis and the one(s) a survey scientist chooses will depend on the variables he or she is examining. What all types of regression analysis have in common is that they look at the influence of one or more independent variables on a dependent variable. In analyzing our survey data we might be interested in knowing what factors most impact attendees’ satisfaction with the conference. Is it a matter of the number of sessions? The keynote speaker? The social events? The site? Using regression analysis, a survey scientist can determine whether and to what extent satisfaction with these different attributes of the conference contribute to overall satisfaction.
This, in turn, provides insight into what aspects of the conference you might want to alter next time around. Say, for example, you paid a high honorarium to get a top flight keynote speaker for your opening session. Participants gave this speaker and the conference overall high marks. Based on these two facts you might think that having a fabulous (and expensive) keynote speaker is the key to conference success. Regression analysis can help you determine if this is indeed the case. You might find that the popularity of the keynote speaker was a major driver of satisfaction with the conference. If so, next year you’ll want to get a great keynote speaker again. But say the regression shows that, while everyone liked the speaker, this did not contribute much to attendees’ satisfaction with the conference. If that is the case, the big bucks spent on the speaker might be best spent elsewhere. If you take the time to carefully analyze the soundness of your survey data, you’ll be on your way to using the answers to help you make informed decisions.
Don’t let your survey data go to waste
Take action now and start analyzing it today with our help.
App Directory
Vision and Mission
SurveyMonkey Together
Diversity, Equity & Inclusion
Health Plan Transparency in Coverage
Office Locations
Terms of Use
Privacy Notice
California Privacy Notice
Acceptable Uses Policy
Security Statement
GDPR Compliance
Email Opt-In
Accessibility
Cookies Notice
Facebook Surveys
Survey Template
Scheduling Polls
Google Forms vs. SurveyMonkey
Employee Satisfaction Surveys
Free Survey Templates
Mobile Surveys
How to Improve Customer Service
AB Test Significance Calculator
NPS Calculator
Questionnaire Templates
Event Survey
Sample Size Calculator
Writing Good Surveys
Likert Scale
Survey Analysis
360 Degree Feedback
Education Surveys
Survey Questions
NPS Calculation
Customer Satisfaction Survey Questions
Agree Disagree Questions
Create a Survey
Online Quizzes
Qualitative vs Quantitative Research
Customer Survey
Market Research Surveys
Survey Design Best Practices
Margin of Error Calculator
Questionnaire
Demographic Questions
Training Survey
Offline Survey
360 Review Template
Cookie consent
We use our own and third-party cookies to show you more relevant content based on your browsing and navigation history. Please accept or manage your cookie settings below. Here's our cookie policy
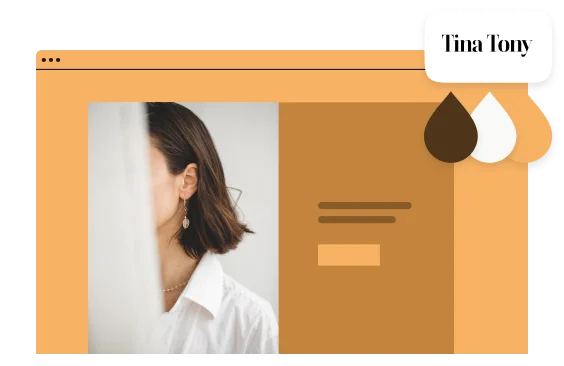
- Form Builder Signups and orders
- Survey maker Research and feedback
- Quiz Maker Trivia and product match
- Find Customers Generate more leads
- Get Feedback Discover ways to improve
- Do research Uncover trends and ideas
- Marketers Forms for marketing teams
- Product Forms for product teams
- HR Forms for HR teams
- Customer success Forms for customer success teams
- Business Forms for general business
- Form templates
- Survey templates
- Quiz templates
- Poll templates
- Order forms
- Feedback forms
- Satisfaction surveys
- Application forms
- Feedback surveys
- Evaluation forms
- Request forms
- Signup forms
- Business surveys
- Marketing surveys
- Report forms
- Customer feedback form
- Registration form
- Branding questionnaire
- 360 feedback
- Lead generation
- Contact form
- Signup sheet

- Help center Find quick answers
- Contact us Speak to someone
- Our blog Get inspired
- Our community Share and learn
- Our guides Tips and how-to
- Updates News and announcements
- Brand Our guidelines
- Partners Browse or join
- Careers Join our team
- → Opinions & Expertise
- → How to analyze survey data: Survey da...
How to analyze survey data: Survey data analysis best practices
Survey data analysis involves reviewing raw materials and transforming them into key insights. Learn how to analyze survey data and best practices here.
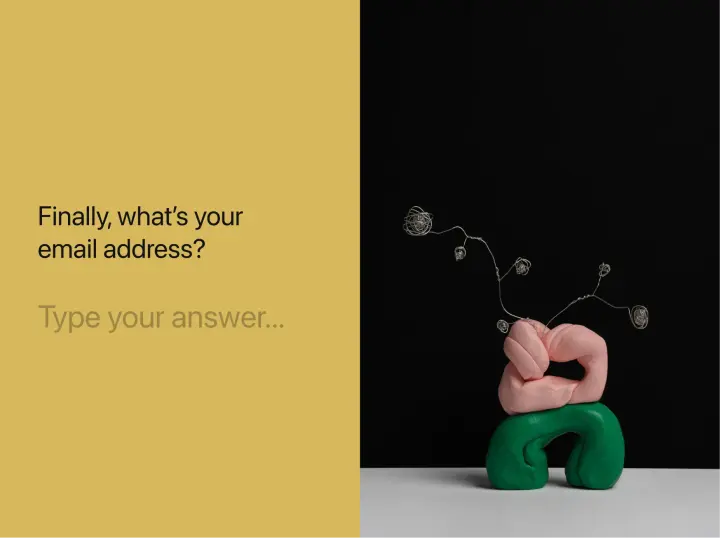
Latest posts on Opinions & Expertise
Typeform | 05.2024
Sheena Fronk | 04.2024
Lydia Kentowski | 04.2024
Norma Ventura | 04.2024
The results are in. You’ve written the questions, found the right people to ask, and got your answers back—now what?
Perfect surveys sent to insightful respondents can become entirely useless if the results aren't coherently and comprehensively analyzed..
So, don’t run and hide.
We know the phrase “survey data analysis” sounds technical and exclusive, but it’s not as scary as it seems. By following a few simple guidelines on how to analyze survey data, you can draw insights from it yourself.
What is survey data analysis?
Survey data analysis is the process of turning survey responses into compelling insights. This includes taking all of your collected data and transforming it into something actionable. Whether it’s open-ended essays, multiple-choice answers, or other questions to ask , you can take this information and uncover patterns and helpful insights.
Some survey data analysis methods include sorting data into categories and using statistical tactics to identify trends and patterns. The goal is to take these raw data responses and turn them into a clear story that answers your research questions to help you make informed decisions.
Getting started with survey data analysis
Before you get started crunching the numbers and performing a survey data analysis, there are a few pieces of information you need to gather.
First, you need to know the number of total respondents. This number will indicate how large your sample is and how much you can rely on your results. It’s always a good idea to gather people’s opinions, but if 5,000 people attended a concert and only five people answered your survey, you can’t really treat those five answers as representative of the whole group.
Secondly, you need to calculate your survey response rate . This is a straightforward percentage—calculate it by dividing the number of responses you received in total by the number of people you asked to fill out the survey. The higher your response rate and the higher your total number of respondents, the more you can trust your survey data to be representative of the sample as a whole.
How to analyze survey data
The first step when analyzing survey data is to turn your individualized responses into aggregated numbers. This sounds complicated, but really, it just means you need to do some counting.
For every question in your survey, you need to know the total number of people who answered with each response. Take a look at this example question:
By aggregating your responses, you're simply counting how many people answered a, b, c, and d, respectively. If 100 people took your survey, the aggregated results would look something like this:
In the last six months: 30
Six months to a year ago: 40
One to two years ago: 20
Over two years ago: 10
Now, if your survey was conducted through a survey host, your online survey results should be aggregated automatically, so there’ll be no need to add the numbers.
Qualitative vs. quantitative data
Once you have all of your aggregated answers, it’s time to start making some sense of the survey data.
Our brains can make sense of percentages much more quickly and easily than whole numbers. It's also far easier to compare different percentages rather than whole numbers.
Say you wrote a survey asking 5-year-olds for their favorite colors. Just saying that 67 children chose red as their favorite color means very little. However, saying that 23% of the children chose red as their favorite color, compared to 50% who chose blue, gives you a much clearer indication of the relative popularity of one color.
If you’ve asked people to write feedback or long-form answers, leave these until the end.
You don’t want the qualitative data to bias your quantitative analysis. Focus on the numbers first, and hopefully, once you have a clear idea of what the sentiment is, the qualitative answers will be able to help you understand why that might be the case.
How to cross-tabulate survey data
Cross-tabulating your data is where you can really begin to draw insights from your survey results instead of just statistics. It can help you add context to your numbers and explore how different groups of people behave or how different factors might affect a single outcome.
When you plan your survey, you'll have considered the different comparisons you'd like to make. For example, maybe you’d like to know if older people are more likely to enjoy eating olives.
Your question might be something like this:
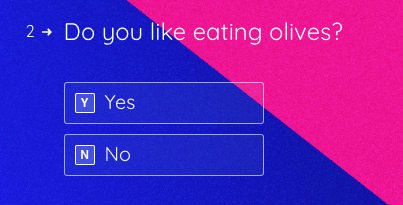
Now, in the first round of your data analysis, you might have already divided the respondents into two groups to work out the split between people who like and don't like eating olives.
So let’s say the results of this olive question were:
Like olives: 542 people (46%)
Dislike olives: 630 people (54%)
To cross-tabulate your data, you’ll need to map another variable onto this one.
We’re interested in whether tastes change with age, so let’s use that age as our second variable and ask:
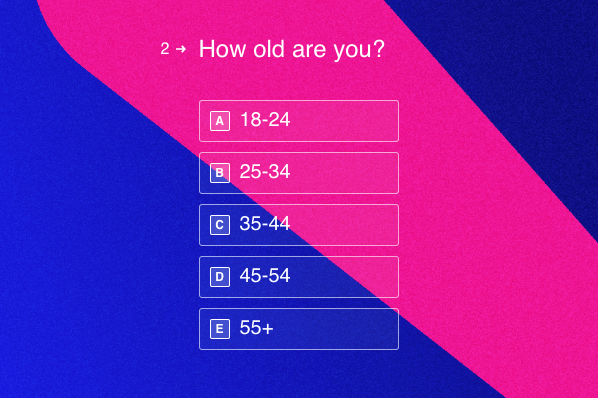
With these results, you can plug them into a Google Sheet and start to see if there are any correlations:
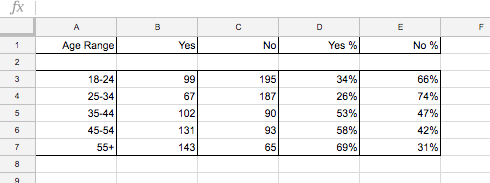
Imagine you have a client who is looking at marketing their olive brand directly to people under 35. You could ask these two questions and look at the split between olive lovers and haters just within this subgroup and see how it compares to the overall average splits.
Benchmarking survey data
Data means very little to us without context and meaning. Turning your numbers into percentages makes comparisons easier, but although proportionally, we can recognize exactly what 75% means, how can we know if that is good?
The answer is benchmarks.
Setting benchmarks is key to making sense of the data and working out what those percentages really mean.
Some of the most common benchmarking techniques involve comparisons between this survey’s results and the data from the last time the survey was collected. To do this effectively, you need to make sure that you are comparing the results of the same question from each survey .
Setting a benchmark using last year’s data is easy. You simply take the percentage splits of responses to a certain question and treat these as your starting point. Then, you can easily see if this month’s data is above or below that benchmark.
Year-over-year or month-over-month comparisons are an excellent way of tracking progress. They allow you to see whether trends are emerging or how much responses have changed in a given period. This is known as longitudinal analysis.
If this is your first time collecting data, no worries, you can still set some benchmarks. Instead of comparing your results to last month's or last year’s data, you can calculate the overall total split between responses for each question and treat this as your benchmark or baseline.
Once you begin to cross-tabulate and break your respondents down into further categories, you can compare their results to your benchmark to place their statistics in context.
If a value is higher than the average , we can say that this category is over-indexing , and if the value is lower , we can say that the category under-indexes . This gives some context to the statistics and starts letting you draw out some real insights from your survey data.
Why you need to analyze survey data
Quantitative data is extremely valuable when interpreting survey results. However, the numbers themselves are unlikely to provide a concrete answer as to why something happened or why people hold a certain opinion.
Understanding why respondents answered in the way that they did is when you can really start to address problems and make changes. This is where the real insight is born.
Sometimes, the “why” will be answered with direct questions in the survey and sometimes with multiple-choice boxes. Other times, it will be up to you as the survey analyst to determine causation, if possible. And this is where we need to be careful.
It's easy to become sucked into a trap when analyzing survey data and start to see patterns everywhere. This isn't necessarily a bad thing, as identifying a correlation between two variables is a key part of interpreting survey results. However, the danger is that we often make assumptions instead.
Assumptions about the data can be hopes or expectations, conscious or subconscious. However, realizing when we are making assumptions can help us avoid any problems further down the line and prevent us from wasting time.
Ultimately, no one wants to find out their assumptions were false after the survey analysis is complete. Similarly, you wouldn’t want a critical assumption to be false and never even realized.
Survey data analysis examples
Correlation occurs when two different variables move at the same time.
A classic example is the sale of seasonal products. During the summer, swimming pool and barbecue sales rise. When plotted on a graph, the two variables move in the same direction at the same time. However, there's no direct connection between these two variables. People buying barbecues isn't the reason the sales of swimming pools increase.
Causation, on the other hand, occurs when one factor directly causes a change in another factor.
For example, in the case of seasonal products, the weather is a key factor. As the temperature rises in the summer, so do barbecue sales. Barbecue sales here are a variable that's dependent on the weather, and there's a key link between them.
When interpreting survey results, it's easy to mistake correlation for causation. Just because two variables move at the same time, it doesn't mean that one is directly influencing the other.
This is where qualitative data comes in. If you’ve asked your respondents to fill in longer-form answers to explain why they chose a certain response, analyzing these answers can give you the insight you need to work out why.
How to report back on your survey data
When sharing your survey data analysis, remember that the story is what makes it interesting, not the numbers.
The percentages you've calculated are vital evidence for your argument, but your analysis needs a narrative to have a real impact on people's thinking.
If you can, always provide context with your statistics, either comparing them to the same survey from last year or comparing groups of people in the same year’s data. Benchmark your numbers so that your audience is immediately aware of whether what they are seeing is positive or negative.
If you are unable to provide recommended actions based on your survey data analysis, at least signpost the key areas that need attention so the relevant parties can begin to tackle the problem if necessary.
When you visualize your data, remember that while long reports can be fascinating, most people won’t read them. Whoever you are presenting to is unlikely to want to listen or read as you walk them through your survey analysis methods step-by-step, so don’t feel like you have to include every single calculation you made in your report.
Put yourself in your audience’s shoes and determine their interests and priorities. Only give them the information if it is relevant to them, they will understand it, and there's something they can do with this new information.
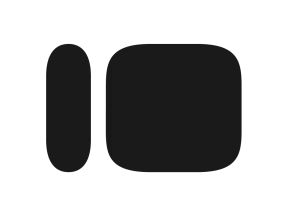
About the author
We're Typeform - a team on a mission to transform data collection by bringing you refreshingly different forms.
Liked that? Check these out:
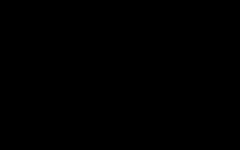
10 fascinating facts and symbolic meanings of the color black
How does the color black make you feel? From Einstein and Jay-Z, to Black Friday and the Model T—here are 10 fascinating things you should know about black.
Eric Johnson | 09.2023
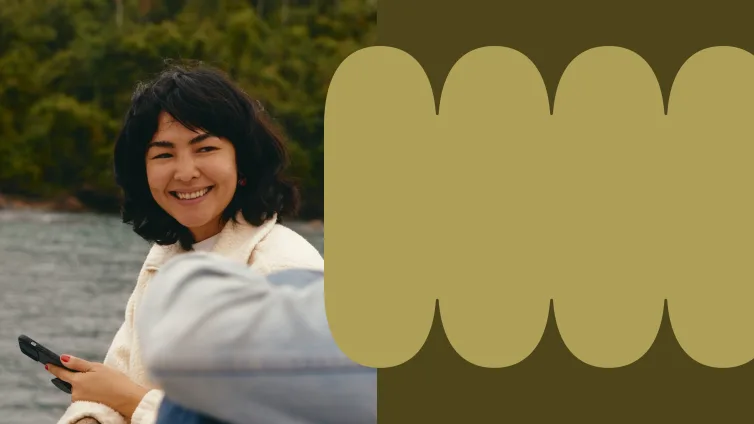
Qualitative vs Quantitative data: What's the difference and how do I collect it with forms?
Qualitative and quantitative data is key to uncovering valuable insights for your business. Understanding the purpose of both types of research, and how to go about collecting it can help you create a better respondant experience and, in turn, uncover more relevant insights.
Lydia Kentowski | 06.2023
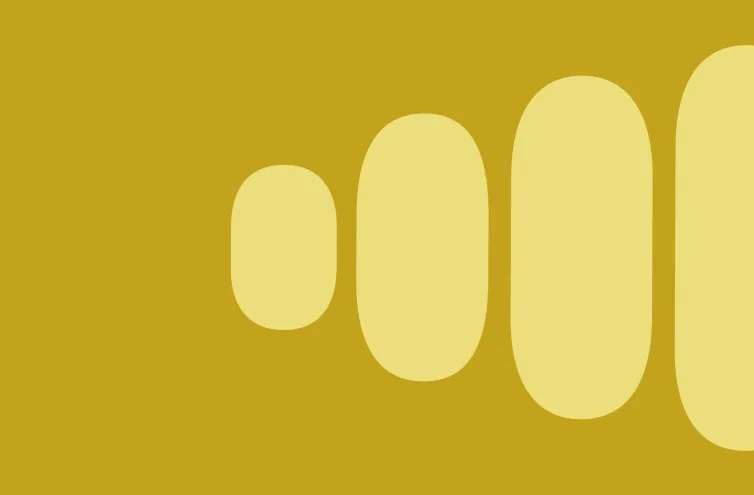
The marketer’s survival guide to the cookieless future
Third-party tracking cookies are already on their way out. But, without the mighty cookie to guide marketers' insights and strategies, what's one to do? Luckily, we've put together a concise guide to surviving the cookieless future.
Kevin Branscum | 12.2023
Thank you for visiting nature.com. You are using a browser version with limited support for CSS. To obtain the best experience, we recommend you use a more up to date browser (or turn off compatibility mode in Internet Explorer). In the meantime, to ensure continued support, we are displaying the site without styles and JavaScript.
- View all journals
- My Account Login
- Explore content
- About the journal
- Publish with us
- Sign up for alerts
- Data Descriptor
- Open access
- Published: 03 May 2024
A dataset for measuring the impact of research data and their curation
- Libby Hemphill ORCID: orcid.org/0000-0002-3793-7281 1 , 2 ,
- Andrea Thomer 3 ,
- Sara Lafia 1 ,
- Lizhou Fan 2 ,
- David Bleckley ORCID: orcid.org/0000-0001-7715-4348 1 &
- Elizabeth Moss 1
Scientific Data volume 11 , Article number: 442 ( 2024 ) Cite this article
595 Accesses
8 Altmetric
Metrics details
- Research data
- Social sciences
Science funders, publishers, and data archives make decisions about how to responsibly allocate resources to maximize the reuse potential of research data. This paper introduces a dataset developed to measure the impact of archival and data curation decisions on data reuse. The dataset describes 10,605 social science research datasets, their curation histories, and reuse contexts in 94,755 publications that cover 59 years from 1963 to 2022. The dataset was constructed from study-level metadata, citing publications, and curation records available through the Inter-university Consortium for Political and Social Research (ICPSR) at the University of Michigan. The dataset includes information about study-level attributes (e.g., PIs, funders, subject terms); usage statistics (e.g., downloads, citations); archiving decisions (e.g., curation activities, data transformations); and bibliometric attributes (e.g., journals, authors) for citing publications. This dataset provides information on factors that contribute to long-term data reuse, which can inform the design of effective evidence-based recommendations to support high-impact research data curation decisions.
Similar content being viewed by others
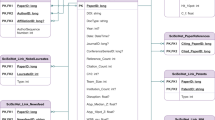
SciSciNet: A large-scale open data lake for the science of science research
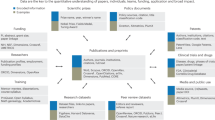
Data, measurement and empirical methods in the science of science
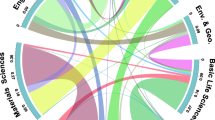
Interdisciplinarity revisited: evidence for research impact and dynamism
Background & summary.
Recent policy changes in funding agencies and academic journals have increased data sharing among researchers and between researchers and the public. Data sharing advances science and provides the transparency necessary for evaluating, replicating, and verifying results. However, many data-sharing policies do not explain what constitutes an appropriate dataset for archiving or how to determine the value of datasets to secondary users 1 , 2 , 3 . Questions about how to allocate data-sharing resources efficiently and responsibly have gone unanswered 4 , 5 , 6 . For instance, data-sharing policies recognize that not all data should be curated and preserved, but they do not articulate metrics or guidelines for determining what data are most worthy of investment.
Despite the potential for innovation and advancement that data sharing holds, the best strategies to prioritize datasets for preparation and archiving are often unclear. Some datasets are likely to have more downstream potential than others, and data curation policies and workflows should prioritize high-value data instead of being one-size-fits-all. Though prior research in library and information science has shown that the “analytic potential” of a dataset is key to its reuse value 7 , work is needed to implement conceptual data reuse frameworks 8 , 9 , 10 , 11 , 12 , 13 , 14 . In addition, publishers and data archives need guidance to develop metrics and evaluation strategies to assess the impact of datasets.
Several existing resources have been compiled to study the relationship between the reuse of scholarly products, such as datasets (Table 1 ); however, none of these resources include explicit information on how curation processes are applied to data to increase their value, maximize their accessibility, and ensure their long-term preservation. The CCex (Curation Costs Exchange) provides models of curation services along with cost-related datasets shared by contributors but does not make explicit connections between them or include reuse information 15 . Analyses on platforms such as DataCite 16 have focused on metadata completeness and record usage, but have not included related curation-level information. Analyses of GenBank 17 and FigShare 18 , 19 citation networks do not include curation information. Related studies of Github repository reuse 20 and Softcite software citation 21 reveal significant factors that impact the reuse of secondary research products but do not focus on research data. RD-Switchboard 22 and DSKG 23 are scholarly knowledge graphs linking research data to articles, patents, and grants, but largely omit social science research data and do not include curation-level factors. To our knowledge, other studies of curation work in organizations similar to ICPSR – such as GESIS 24 , Dataverse 25 , and DANS 26 – have not made their underlying data available for analysis.
This paper describes a dataset 27 compiled for the MICA project (Measuring the Impact of Curation Actions) led by investigators at ICPSR, a large social science data archive at the University of Michigan. The dataset was originally developed to study the impacts of data curation and archiving on data reuse. The MICA dataset has supported several previous publications investigating the intensity of data curation actions 28 , the relationship between data curation actions and data reuse 29 , and the structures of research communities in a data citation network 30 . Collectively, these studies help explain the return on various types of curatorial investments. The dataset that we introduce in this paper, which we refer to as the MICA dataset, has the potential to address research questions in the areas of science (e.g., knowledge production), library and information science (e.g., scholarly communication), and data archiving (e.g., reproducible workflows).
We constructed the MICA dataset 27 using records available at ICPSR, a large social science data archive at the University of Michigan. Data set creation involved: collecting and enriching metadata for articles indexed in the ICPSR Bibliography of Data-related Literature against the Dimensions AI bibliometric database; gathering usage statistics for studies from ICPSR’s administrative database; processing data curation work logs from ICPSR’s project tracking platform, Jira; and linking data in social science studies and series to citing analysis papers (Fig. 1 ).
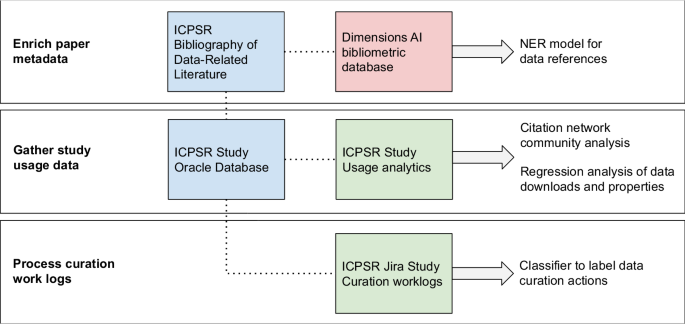
Steps to prepare MICA dataset for analysis - external sources are red, primary internal sources are blue, and internal linked sources are green.
Enrich paper metadata
The ICPSR Bibliography of Data-related Literature is a growing database of literature in which data from ICPSR studies have been used. Its creation was funded by the National Science Foundation (Award 9977984), and for the past 20 years it has been supported by ICPSR membership and multiple US federally-funded and foundation-funded topical archives at ICPSR. The Bibliography was originally launched in the year 2000 to aid in data discovery by providing a searchable database linking publications to the study data used in them. The Bibliography collects the universe of output based on the data shared in each study through, which is made available through each ICPSR study’s webpage. The Bibliography contains both peer-reviewed and grey literature, which provides evidence for measuring the impact of research data. For an item to be included in the ICPSR Bibliography, it must contain an analysis of data archived by ICPSR or contain a discussion or critique of the data collection process, study design, or methodology 31 . The Bibliography is manually curated by a team of librarians and information specialists at ICPSR who enter and validate entries. Some publications are supplied to the Bibliography by data depositors, and some citations are submitted to the Bibliography by authors who abide by ICPSR’s terms of use requiring them to submit citations to works in which they analyzed data retrieved from ICPSR. Most of the Bibliography is populated by Bibliography team members, who create custom queries for ICPSR studies performed across numerous sources, including Google Scholar, ProQuest, SSRN, and others. Each record in the Bibliography is one publication that has used one or more ICPSR studies. The version we used was captured on 2021-11-16 and included 94,755 publications.
To expand the coverage of the ICPSR Bibliography, we searched exhaustively for all ICPSR study names, unique numbers assigned to ICPSR studies, and DOIs 32 using a full-text index available through the Dimensions AI database 33 . We accessed Dimensions through a license agreement with the University of Michigan. ICPSR Bibliography librarians and information specialists manually reviewed and validated new entries that matched one or more search criteria. We then used Dimensions to gather enriched metadata and full-text links for items in the Bibliography with DOIs. We matched 43% of the items in the Bibliography to enriched Dimensions metadata including abstracts, field of research codes, concepts, and authors’ institutional information; we also obtained links to full text for 16% of Bibliography items. Based on licensing agreements, we included Dimensions identifiers and links to full text so that users with valid publisher and database access can construct an enriched publication dataset.
Gather study usage data
ICPSR maintains a relational administrative database, DBInfo, that organizes study-level metadata and information on data reuse across separate tables. Studies at ICPSR consist of one or more files collected at a single time or for a single purpose; studies in which the same variables are observed over time are grouped into series. Each study at ICPSR is assigned a DOI, and its metadata are stored in DBInfo. Study metadata follows the Data Documentation Initiative (DDI) Codebook 2.5 standard. DDI elements included in our dataset are title, ICPSR study identification number, DOI, authoring entities, description (abstract), funding agencies, subject terms assigned to the study during curation, and geographic coverage. We also created variables based on DDI elements: total variable count, the presence of survey question text in the metadata, the number of author entities, and whether an author entity was an institution. We gathered metadata for ICPSR’s 10,605 unrestricted public-use studies available as of 2021-11-16 ( https://www.icpsr.umich.edu/web/pages/membership/or/metadata/oai.html ).
To link study usage data with study-level metadata records, we joined study metadata from DBinfo on study usage information, which included total study downloads (data and documentation), individual data file downloads, and cumulative citations from the ICPSR Bibliography. We also gathered descriptive metadata for each study and its variables, which allowed us to summarize and append recoded fields onto the study-level metadata such as curation level, number and type of principle investigators, total variable count, and binary variables indicating whether the study data were made available for online analysis, whether survey question text was made searchable online, and whether the study variables were indexed for search. These characteristics describe aspects of the discoverability of the data to compare with other characteristics of the study. We used the study and series numbers included in the ICPSR Bibliography as unique identifiers to link papers to metadata and analyze the community structure of dataset co-citations in the ICPSR Bibliography 32 .
Process curation work logs
Researchers deposit data at ICPSR for curation and long-term preservation. Between 2016 and 2020, more than 3,000 research studies were deposited with ICPSR. Since 2017, ICPSR has organized curation work into a central unit that provides varied levels of curation that vary in the intensity and complexity of data enhancement that they provide. While the levels of curation are standardized as to effort (level one = less effort, level three = most effort), the specific curatorial actions undertaken for each dataset vary. The specific curation actions are captured in Jira, a work tracking program, which data curators at ICPSR use to collaborate and communicate their progress through tickets. We obtained access to a corpus of 669 completed Jira tickets corresponding to the curation of 566 unique studies between February 2017 and December 2019 28 .
To process the tickets, we focused only on their work log portions, which contained free text descriptions of work that data curators had performed on a deposited study, along with the curators’ identifiers, and timestamps. To protect the confidentiality of the data curators and the processing steps they performed, we collaborated with ICPSR’s curation unit to propose a classification scheme, which we used to train a Naive Bayes classifier and label curation actions in each work log sentence. The eight curation action labels we proposed 28 were: (1) initial review and planning, (2) data transformation, (3) metadata, (4) documentation, (5) quality checks, (6) communication, (7) other, and (8) non-curation work. We note that these categories of curation work are very specific to the curatorial processes and types of data stored at ICPSR, and may not match the curation activities at other repositories. After applying the classifier to the work log sentences, we obtained summary-level curation actions for a subset of all ICPSR studies (5%), along with the total number of hours spent on data curation for each study, and the proportion of time associated with each action during curation.
Data Records
The MICA dataset 27 connects records for each of ICPSR’s archived research studies to the research publications that use them and related curation activities available for a subset of studies (Fig. 2 ). Each of the three tables published in the dataset is available as a study archived at ICPSR. The data tables are distributed as statistical files available for use in SAS, SPSS, Stata, and R as well as delimited and ASCII text files. The dataset is organized around studies and papers as primary entities. The studies table lists ICPSR studies, their metadata attributes, and usage information; the papers table was constructed using the ICPSR Bibliography and Dimensions database; and the curation logs table summarizes the data curation steps performed on a subset of ICPSR studies.
Studies (“ICPSR_STUDIES”): 10,605 social science research datasets available through ICPSR up to 2021-11-16 with variables for ICPSR study number, digital object identifier, study name, series number, series title, authoring entities, full-text description, release date, funding agency, geographic coverage, subject terms, topical archive, curation level, single principal investigator (PI), institutional PI, the total number of PIs, total variables in data files, question text availability, study variable indexing, level of restriction, total unique users downloading study data files and codebooks, total unique users downloading data only, and total unique papers citing data through November 2021. Studies map to the papers and curation logs table through ICPSR study numbers as “STUDY”. However, not every study in this table will have records in the papers and curation logs tables.
Papers (“ICPSR_PAPERS”): 94,755 publications collected from 2000-08-11 to 2021-11-16 in the ICPSR Bibliography and enriched with metadata from the Dimensions database with variables for paper number, identifier, title, authors, publication venue, item type, publication date, input date, ICPSR series numbers used in the paper, ICPSR study numbers used in the paper, the Dimension identifier, and the Dimensions link to the publication’s full text. Papers map to the studies table through ICPSR study numbers in the “STUDY_NUMS” field. Each record represents a single publication, and because a researcher can use multiple datasets when creating a publication, each record may list multiple studies or series.
Curation logs (“ICPSR_CURATION_LOGS”): 649 curation logs for 563 ICPSR studies (although most studies in the subset had one curation log, some studies were associated with multiple logs, with a maximum of 10) curated between February 2017 and December 2019 with variables for study number, action labels assigned to work description sentences using a classifier trained on ICPSR curation logs, hours of work associated with a single log entry, and total hours of work logged for the curation ticket. Curation logs map to the study and paper tables through ICPSR study numbers as “STUDY”. Each record represents a single logged action, and future users may wish to aggregate actions to the study level before joining tables.
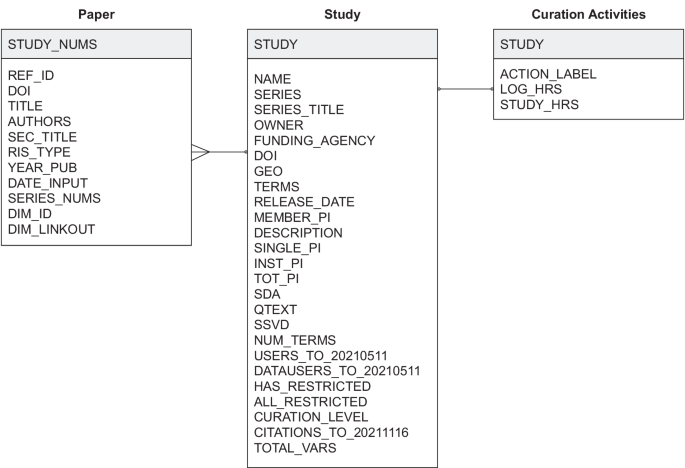
Entity-relation diagram.
Technical Validation
We report on the reliability of the dataset’s metadata in the following subsections. To support future reuse of the dataset, curation services provided through ICPSR improved data quality by checking for missing values, adding variable labels, and creating a codebook.
All 10,605 studies available through ICPSR have a DOI and a full-text description summarizing what the study is about, the purpose of the study, the main topics covered, and the questions the PIs attempted to answer when they conducted the study. Personal names (i.e., principal investigators) and organizational names (i.e., funding agencies) are standardized against an authority list maintained by ICPSR; geographic names and subject terms are also standardized and hierarchically indexed in the ICPSR Thesaurus 34 . Many of ICPSR’s studies (63%) are in a series and are distributed through the ICPSR General Archive (56%), a non-topical archive that accepts any social or behavioral science data. While study data have been available through ICPSR since 1962, the earliest digital release date recorded for a study was 1984-03-18, when ICPSR’s database was first employed, and the most recent date is 2021-10-28 when the dataset was collected.
Curation level information was recorded starting in 2017 and is available for 1,125 studies (11%); approximately 80% of studies with assigned curation levels received curation services, equally distributed between Levels 1 (least intensive), 2 (moderately intensive), and 3 (most intensive) (Fig. 3 ). Detailed descriptions of ICPSR’s curation levels are available online 35 . Additional metadata are available for a subset of 421 studies (4%), including information about whether the study has a single PI, an institutional PI, the total number of PIs involved, total variables recorded is available for online analysis, has searchable question text, has variables that are indexed for search, contains one or more restricted files, and whether the study is completely restricted. We provided additional metadata for this subset of ICPSR studies because they were released within the past five years and detailed curation and usage information were available for them. Usage statistics including total downloads and data file downloads are available for this subset of studies as well; citation statistics are available for 8,030 studies (76%). Most ICPSR studies have fewer than 500 users, as indicated by total downloads, or citations (Fig. 4 ).
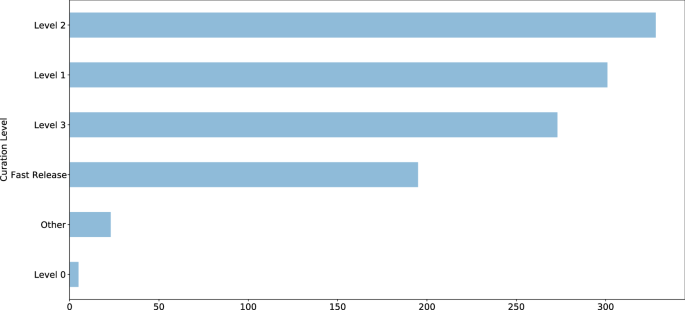
ICPSR study curation levels.
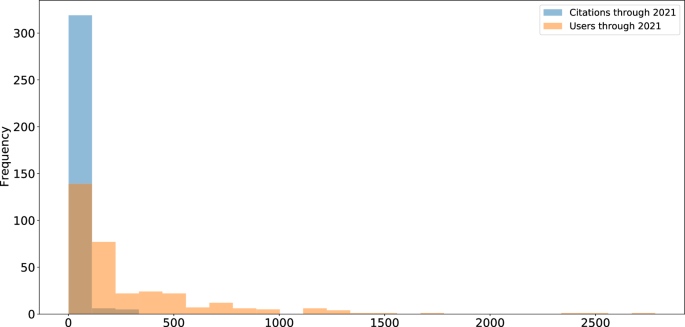
ICPSR study usage.
A subset of 43,102 publications (45%) available in the ICPSR Bibliography had a DOI. Author metadata were entered as free text, meaning that variations may exist and require additional normalization and pre-processing prior to analysis. While author information is standardized for each publication, individual names may appear in different sort orders (e.g., “Earls, Felton J.” and “Stephen W. Raudenbush”). Most of the items in the ICPSR Bibliography as of 2021-11-16 were journal articles (59%), reports (14%), conference presentations (9%), or theses (8%) (Fig. 5 ). The number of publications collected in the Bibliography has increased each decade since the inception of ICPSR in 1962 (Fig. 6 ). Most ICPSR studies (76%) have one or more citations in a publication.
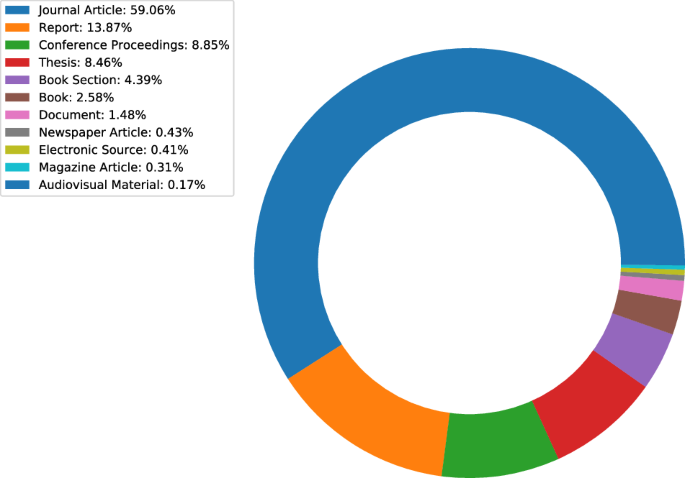
ICPSR Bibliography citation types.
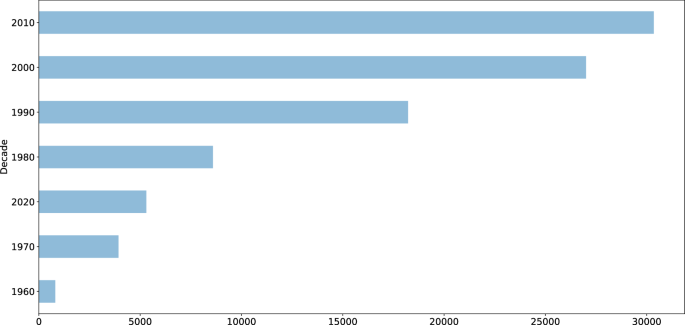
ICPSR citations by decade.
Usage Notes
The dataset consists of three tables that can be joined using the “STUDY” key as shown in Fig. 2 . The “ICPSR_PAPERS” table contains one row per paper with one or more cited studies in the “STUDY_NUMS” column. We manipulated and analyzed the tables as CSV files with the Pandas library 36 in Python and the Tidyverse packages 37 in R.
The present MICA dataset can be used independently to study the relationship between curation decisions and data reuse. Evidence of reuse for specific studies is available in several forms: usage information, including downloads and citation counts; and citation contexts within papers that cite data. Analysis may also be performed on the citation network formed between datasets and papers that use them. Finally, curation actions can be associated with properties of studies and usage histories.
This dataset has several limitations of which users should be aware. First, Jira tickets can only be used to represent the intensiveness of curation for activities undertaken since 2017, when ICPSR started using both Curation Levels and Jira. Studies published before 2017 were all curated, but documentation of the extent of that curation was not standardized and therefore could not be included in these analyses. Second, the measure of publications relies upon the authors’ clarity of data citation and the ICPSR Bibliography staff’s ability to discover citations with varying formality and clarity. Thus, there is always a chance that some secondary-data-citing publications have been left out of the bibliography. Finally, there may be some cases in which a paper in the ICSPSR bibliography did not actually obtain data from ICPSR. For example, PIs have often written about or even distributed their data prior to their archival in ICSPR. Therefore, those publications would not have cited ICPSR but they are still collected in the Bibliography as being directly related to the data that were eventually deposited at ICPSR.
In summary, the MICA dataset contains relationships between two main types of entities – papers and studies – which can be mined. The tables in the MICA dataset have supported network analysis (community structure and clique detection) 30 ; natural language processing (NER for dataset reference detection) 32 ; visualizing citation networks (to search for datasets) 38 ; and regression analysis (on curation decisions and data downloads) 29 . The data are currently being used to develop research metrics and recommendation systems for research data. Given that DOIs are provided for ICPSR studies and articles in the ICPSR Bibliography, the MICA dataset can also be used with other bibliometric databases, including DataCite, Crossref, OpenAlex, and related indexes. Subscription-based services, such as Dimensions AI, are also compatible with the MICA dataset. In some cases, these services provide abstracts or full text for papers from which data citation contexts can be extracted for semantic content analysis.
Code availability
The code 27 used to produce the MICA project dataset is available on GitHub at https://github.com/ICPSR/mica-data-descriptor and through Zenodo with the identifier https://doi.org/10.5281/zenodo.8432666 . Data manipulation and pre-processing were performed in Python. Data curation for distribution was performed in SPSS.
He, L. & Han, Z. Do usage counts of scientific data make sense? An investigation of the Dryad repository. Library Hi Tech 35 , 332–342 (2017).
Article Google Scholar
Brickley, D., Burgess, M. & Noy, N. Google dataset search: Building a search engine for datasets in an open web ecosystem. In The World Wide Web Conference - WWW ‘19 , 1365–1375 (ACM Press, San Francisco, CA, USA, 2019).
Buneman, P., Dosso, D., Lissandrini, M. & Silvello, G. Data citation and the citation graph. Quantitative Science Studies 2 , 1399–1422 (2022).
Chao, T. C. Disciplinary reach: Investigating the impact of dataset reuse in the earth sciences. Proceedings of the American Society for Information Science and Technology 48 , 1–8 (2011).
Article ADS Google Scholar
Parr, C. et al . A discussion of value metrics for data repositories in earth and environmental sciences. Data Science Journal 18 , 58 (2019).
Eschenfelder, K. R., Shankar, K. & Downey, G. The financial maintenance of social science data archives: Four case studies of long–term infrastructure work. J. Assoc. Inf. Sci. Technol. 73 , 1723–1740 (2022).
Palmer, C. L., Weber, N. M. & Cragin, M. H. The analytic potential of scientific data: Understanding re-use value. Proceedings of the American Society for Information Science and Technology 48 , 1–10 (2011).
Zimmerman, A. S. New knowledge from old data: The role of standards in the sharing and reuse of ecological data. Sci. Technol. Human Values 33 , 631–652 (2008).
Cragin, M. H., Palmer, C. L., Carlson, J. R. & Witt, M. Data sharing, small science and institutional repositories. Philosophical Transactions of the Royal Society A: Mathematical, Physical and Engineering Sciences 368 , 4023–4038 (2010).
Article ADS CAS Google Scholar
Fear, K. M. Measuring and Anticipating the Impact of Data Reuse . Ph.D. thesis, University of Michigan (2013).
Borgman, C. L., Van de Sompel, H., Scharnhorst, A., van den Berg, H. & Treloar, A. Who uses the digital data archive? An exploratory study of DANS. Proceedings of the Association for Information Science and Technology 52 , 1–4 (2015).
Pasquetto, I. V., Borgman, C. L. & Wofford, M. F. Uses and reuses of scientific data: The data creators’ advantage. Harvard Data Science Review 1 (2019).
Gregory, K., Groth, P., Scharnhorst, A. & Wyatt, S. Lost or found? Discovering data needed for research. Harvard Data Science Review (2020).
York, J. Seeking equilibrium in data reuse: A study of knowledge satisficing . Ph.D. thesis, University of Michigan (2022).
Kilbride, W. & Norris, S. Collaborating to clarify the cost of curation. New Review of Information Networking 19 , 44–48 (2014).
Robinson-Garcia, N., Mongeon, P., Jeng, W. & Costas, R. DataCite as a novel bibliometric source: Coverage, strengths and limitations. Journal of Informetrics 11 , 841–854 (2017).
Qin, J., Hemsley, J. & Bratt, S. E. The structural shift and collaboration capacity in GenBank networks: A longitudinal study. Quantitative Science Studies 3 , 174–193 (2022).
Article PubMed PubMed Central Google Scholar
Acuna, D. E., Yi, Z., Liang, L. & Zhuang, H. Predicting the usage of scientific datasets based on article, author, institution, and journal bibliometrics. In Smits, M. (ed.) Information for a Better World: Shaping the Global Future. iConference 2022 ., 42–52 (Springer International Publishing, Cham, 2022).
Zeng, T., Wu, L., Bratt, S. & Acuna, D. E. Assigning credit to scientific datasets using article citation networks. Journal of Informetrics 14 , 101013 (2020).
Koesten, L., Vougiouklis, P., Simperl, E. & Groth, P. Dataset reuse: Toward translating principles to practice. Patterns 1 , 100136 (2020).
Du, C., Cohoon, J., Lopez, P. & Howison, J. Softcite dataset: A dataset of software mentions in biomedical and economic research publications. J. Assoc. Inf. Sci. Technol. 72 , 870–884 (2021).
Aryani, A. et al . A research graph dataset for connecting research data repositories using RD-Switchboard. Sci Data 5 , 180099 (2018).
Färber, M. & Lamprecht, D. The data set knowledge graph: Creating a linked open data source for data sets. Quantitative Science Studies 2 , 1324–1355 (2021).
Perry, A. & Netscher, S. Measuring the time spent on data curation. Journal of Documentation 78 , 282–304 (2022).
Trisovic, A. et al . Advancing computational reproducibility in the Dataverse data repository platform. In Proceedings of the 3rd International Workshop on Practical Reproducible Evaluation of Computer Systems , P-RECS ‘20, 15–20, https://doi.org/10.1145/3391800.3398173 (Association for Computing Machinery, New York, NY, USA, 2020).
Borgman, C. L., Scharnhorst, A. & Golshan, M. S. Digital data archives as knowledge infrastructures: Mediating data sharing and reuse. Journal of the Association for Information Science and Technology 70 , 888–904, https://doi.org/10.1002/asi.24172 (2019).
Lafia, S. et al . MICA Data Descriptor. Zenodo https://doi.org/10.5281/zenodo.8432666 (2023).
Lafia, S., Thomer, A., Bleckley, D., Akmon, D. & Hemphill, L. Leveraging machine learning to detect data curation activities. In 2021 IEEE 17th International Conference on eScience (eScience) , 149–158, https://doi.org/10.1109/eScience51609.2021.00025 (2021).
Hemphill, L., Pienta, A., Lafia, S., Akmon, D. & Bleckley, D. How do properties of data, their curation, and their funding relate to reuse? J. Assoc. Inf. Sci. Technol. 73 , 1432–44, https://doi.org/10.1002/asi.24646 (2021).
Lafia, S., Fan, L., Thomer, A. & Hemphill, L. Subdivisions and crossroads: Identifying hidden community structures in a data archive’s citation network. Quantitative Science Studies 3 , 694–714, https://doi.org/10.1162/qss_a_00209 (2022).
ICPSR. ICPSR Bibliography of Data-related Literature: Collection Criteria. https://www.icpsr.umich.edu/web/pages/ICPSR/citations/collection-criteria.html (2023).
Lafia, S., Fan, L. & Hemphill, L. A natural language processing pipeline for detecting informal data references in academic literature. Proc. Assoc. Inf. Sci. Technol. 59 , 169–178, https://doi.org/10.1002/pra2.614 (2022).
Hook, D. W., Porter, S. J. & Herzog, C. Dimensions: Building context for search and evaluation. Frontiers in Research Metrics and Analytics 3 , 23, https://doi.org/10.3389/frma.2018.00023 (2018).
https://www.icpsr.umich.edu/web/ICPSR/thesaurus (2002). ICPSR. ICPSR Thesaurus.
https://www.icpsr.umich.edu/files/datamanagement/icpsr-curation-levels.pdf (2020). ICPSR. ICPSR Curation Levels.
McKinney, W. Data Structures for Statistical Computing in Python. In van der Walt, S. & Millman, J. (eds.) Proceedings of the 9th Python in Science Conference , 56–61 (2010).
Wickham, H. et al . Welcome to the Tidyverse. Journal of Open Source Software 4 , 1686 (2019).
Fan, L., Lafia, S., Li, L., Yang, F. & Hemphill, L. DataChat: Prototyping a conversational agent for dataset search and visualization. Proc. Assoc. Inf. Sci. Technol. 60 , 586–591 (2023).
Download references
Acknowledgements
We thank the ICPSR Bibliography staff, the ICPSR Data Curation Unit, and the ICPSR Data Stewardship Committee for their support of this research. This material is based upon work supported by the National Science Foundation under grant 1930645. This project was made possible in part by the Institute of Museum and Library Services LG-37-19-0134-19.
Author information
Authors and affiliations.
Inter-university Consortium for Political and Social Research, University of Michigan, Ann Arbor, MI, 48104, USA
Libby Hemphill, Sara Lafia, David Bleckley & Elizabeth Moss
School of Information, University of Michigan, Ann Arbor, MI, 48104, USA
Libby Hemphill & Lizhou Fan
School of Information, University of Arizona, Tucson, AZ, 85721, USA
Andrea Thomer
You can also search for this author in PubMed Google Scholar
Contributions
L.H. and A.T. conceptualized the study design, D.B., E.M., and S.L. prepared the data, S.L., L.F., and L.H. analyzed the data, and D.B. validated the data. All authors reviewed and edited the manuscript.
Corresponding author
Correspondence to Libby Hemphill .
Ethics declarations
Competing interests.
The authors declare no competing interests.
Additional information
Publisher’s note Springer Nature remains neutral with regard to jurisdictional claims in published maps and institutional affiliations.
Rights and permissions
Open Access This article is licensed under a Creative Commons Attribution 4.0 International License, which permits use, sharing, adaptation, distribution and reproduction in any medium or format, as long as you give appropriate credit to the original author(s) and the source, provide a link to the Creative Commons licence, and indicate if changes were made. The images or other third party material in this article are included in the article’s Creative Commons licence, unless indicated otherwise in a credit line to the material. If material is not included in the article’s Creative Commons licence and your intended use is not permitted by statutory regulation or exceeds the permitted use, you will need to obtain permission directly from the copyright holder. To view a copy of this licence, visit http://creativecommons.org/licenses/by/4.0/ .
Reprints and permissions
About this article
Cite this article.
Hemphill, L., Thomer, A., Lafia, S. et al. A dataset for measuring the impact of research data and their curation. Sci Data 11 , 442 (2024). https://doi.org/10.1038/s41597-024-03303-2
Download citation
Received : 16 November 2023
Accepted : 24 April 2024
Published : 03 May 2024
DOI : https://doi.org/10.1038/s41597-024-03303-2
Share this article
Anyone you share the following link with will be able to read this content:
Sorry, a shareable link is not currently available for this article.
Provided by the Springer Nature SharedIt content-sharing initiative
Quick links
- Explore articles by subject
- Guide to authors
- Editorial policies
Sign up for the Nature Briefing newsletter — what matters in science, free to your inbox daily.

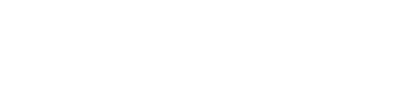
Along with Stanford news and stories, show me:
- Student information
- Faculty/Staff information
We want to provide announcements, events, leadership messages and resources that are relevant to you. Your selection is stored in a browser cookie which you can remove at any time using “Clear all personalization” below.
Today, the university is releasing the findings from qualitative data received from Stanford’s first-ever campus-wide Diversity, Equity and Inclusion (DEI) survey . The quantitative data were released in November 2021.
“I want to thank the nearly 7,000 people who took the time and expended the emotional energy to both share their stories and experiences, and help convey what we are doing well and how we can improve,” said Patrick Dunkley, vice provost for institutional equity, access, and community.
He added: “What we see in this report from the community input is consistency between the quantitative and qualitative data. The qualitative findings include firsthand accounts of discriminatory, harassing, and other harmful behavior, such as microaggression and experiences where community members felt isolated, marginalized, or excluded. We hope these accounts will serve as learning opportunities of what harmful behaviors look like, and the impact they have on members of our community so they can be avoided in the future. This information will also prove to be extremely valuable as we plot our course toward improvement.”
“Hearing the voices of our community is both powerful and distressing,” said Provost Persis Drell, who commissioned the DEI survey. “Although we understand that seeing this information may cause added pain for many members of our community, particularly given recent events on our campus and in our world, we want the entire Stanford community to understand how and where this kind of harmful behavior manifests itself in our university. Only by confronting this problem head on will we make improvements in our campus culture.”
The DEI survey
Conducted in May 2021, the DEI survey focused on the individual experiences of community members, and included questions about inclusion and belonging, as well as questions about experiences with harassing and discriminatory behavior. The survey also collected data about race/ethnicity and other identities. Nearly 15,000 students, postdocs, faculty, and staff took part in the survey. Stanford released the quantitative findings in November 2021.
The DEI survey included three open-ended questions, where community members could provide feedback to the university and share their own experiences with issues of belonging, discrimination, harassment, and other harmful behavior. The questions were as follows:
- If you would like to tell us more, please tell us about your experiences and perspectives on these and other issues that are important to you.
- We would also like to know what is working well at Stanford. What efforts, initiatives, or policies have you seen, experienced, or participated in that have had a positive impact on improving diversity, equity, and inclusion at Stanford?
- What else would you like to tell us?
A total of 6,886 responses to those questions were received. In order to preserve anonymity and privacy, a third-party contractor convened a team with extensive experience in analyzing qualitative data. The analysis involved systematically reading and coding open-ended survey responses to identify common themes across the content.
The report contains representative quotes, presented without compromising individual privacy, that illustrate major themes of the challenges that Stanford community members have experienced. The quotes provided in the report are representative of many other very similar comments.
Themes include: interpersonal interactions/harmful experiences; experiences of isolation and lack of voice; and university structures and status hierarchy.
Suggestions from the community and next steps
Many respondents to the second question – “What’s working well?” – commended Stanford leadership for making diversity, inclusion, equity, and belonging a top priority. They also identified recent successful efforts to increase diversity among the Stanford faculty and student populations. Some respondents appreciated the regular collection and release of data to improve transparency and accountability, and many cited DEI efforts that were taking place in their individual units and programs.
Respondents also offered suggestions for how to improve the current campus climate. Among those were increasing diversity across all populations; improving support for those among marginalized identities; access to more DEI education and learning opportunities; improving reporting and accountability; and a call for more centralized DEI resources.
Dunkley said, “We made a commitment when we launched the survey to use the results to inform our work going forward. Since we released the survey results in November, we have been actively engaging throughout the community educating on the importance of these data. We will now expand that engagement to working on solutions that refine or enhance efforts already under way, and we will also be exploring all new approaches.”
He said that, beginning this summer, a series of focus groups and community forums will be held to discuss the survey results and next steps.

An official website of the United States government
Here’s how you know
Official websites use .gov A .gov website belongs to an official government organization in the United States.
Secure .gov websites use HTTPS A lock ( Lock A locked padlock ) or https:// means you’ve safely connected to the .gov website. Share sensitive information only on official, secure websites.
JavaScript appears to be disabled on this computer. Please click here to see any active alerts .
Clean Watersheds Needs Survey (CWNS) – 2022 Report and Data
The Clean Watersheds Needs Survey (CWNS) provides an assessment of the capital investments necessary for states, the District of Columbia, and U.S. Territories to meet the Clean Water Act’s (CWA) water quality goals over the subsequent 20 years. These needs include projects and related infrastructure costs for wastewater publicly owned treatment works, stormwater treatment, nonpoint source control, and decentralized wastewater treatment.
The U.S. Environmental Protection Agency (EPA) has prepared the 2022 CWNS Report to Congress in compliance with CWA section 516(b)(1)(B) ( 33 U.S Code §1375 ) as well as CWA section 609, which was added by the Infrastructure Investment and Jobs Act (IIJA), P.L. 117-58, November 15, 2021. This Report summarizes the results of EPA’s 17 th survey since the CWA was enacted in 1972.
2022 CWNS Data Dashboard The dashboard features interactive maps, charts, and data tables of state, regional, and national results. Users can also download the data dictionary and CWNS data as .csv files or as an Access Database for the entire nation or for individual states.
Report to Congress
- 2022 Clean Watersheds Needs Survey Report to Congress (pdf) (9.1 MB)
- 2022 CWNS Scope and Methods (pdf) (955.9 KB) - Describes the data collection and review process, as well as the development of the Report to Congress.
- 2022 CWNS Report to Congress Summary (pdf) (1.6 MB)
Additional Resources
- 2022 CWNS State Coordinator Manual (pdf) (4.3 MB) The manual is a guide on survey policy, terminology, and data entry in the online portal.
- 2022 CWNS Cost Estimation Tool Methods (pdf) (2 MB) The methods document outlines the background, methods, and data sources associated with each of EPA’s Cost Estimation Tools. These tools were used to assign a dollar value for certain projects based on documented inputs. This document should be used in conjunction with the 2022 CWNS State Coordinator Manual for term definitions and survey policy. These estimates are not intended for any other purpose outside of the CWNS.
Summary of Report to Congress
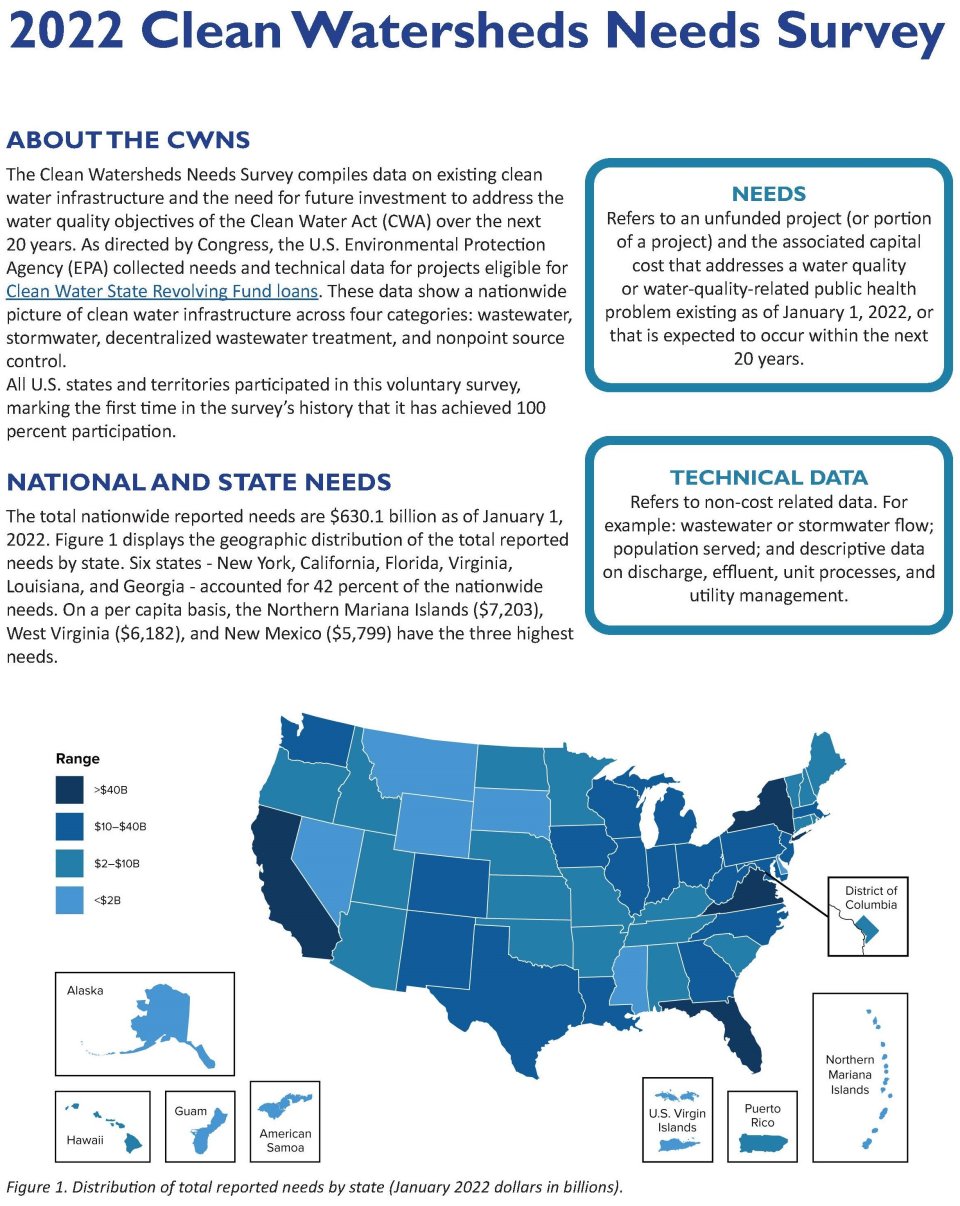
- Needs Categories
- Data Dashboard
- Reports for Earlier Years

An official website of the United States government
Here’s how you know
Official websites use .gov A .gov website belongs to an official government organization in the United States.
Secure .gov websites use HTTPS A lock ( Lock A locked padlock ) or https:// means you’ve safely connected to the .gov website. Share sensitive information only on official, secure websites.
- Researchers
- Survey Respondents
- Census.gov /
- Census Working Papers /
Exploring a Method to Produce County-Level Supplemental Poverty Measure Estimates in the American Community Survey
The goal of this paper is to release a methodology to produce county-level Supplemental Poverty Measure (SPM) estimates from the 1-year American Community Survey (ACS). This methodology would only use publicly-available data in order to be transparent and reproducible. Currently, the only sub-national SPM estimates available using 1 year of survey data are at the Public Use Microdata Area (PUMA) level and at the state level using 1-year ACS data. In this paper, county-level SPM estimates are produced from PUMA-level estimates using a county to PUMA crosswalk from the Missouri Census Data Center. There are three main types of county-PUMA relationships: counties that are exactly equal to a PUMA; counties that span multiple PUMAs; and PUMAs that span multiple counties. In the first case, the county-level estimates are exactly equal to the PUMA-level estimate. In the second case, the county-level estimate is a population-weighted average of the PUMA-level estimates. In the third case, the relationship between the official poverty measure (OPM) from the 5-year ACS at the county and PUMA level is used along with 2010 county populations to apportion the SPM population among counties within a PUMA. Several checks are performed in order to validate the PUMA to county methodology.
Related Information
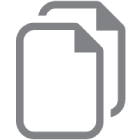
WORKING PAPER

The independent source for health policy research, polling, and news.
KFF Health Tracking Poll May 2024: The Public’s Use and Views of GLP-1 Drugs
Alex Montero , Grace Sparks , Marley Presiado , and Liz Hamel Published: May 10, 2024
- Methodology
Key Findings
- The latest KFF Health Tracking Poll finds that about one in eight adults (12%) say they have ever taken a GLP-1 agonist – an increasingly popular class of prescription drugs used for weight loss and to treat diabetes or prevent heart attacks or strokes for adults with heart disease – including 6% who say they are currently taking such a drug. The share who report ever taking these drugs rises to four in ten (43%) among adults who have been told by a doctor that they have diabetes, a quarter who have been told they have heart disease, and one in five (22%) who have been told by a doctor that they are overweight or obese in the past five years 1 . Public awareness of GLP-1 drugs has increased in the past year, with about one-third (32%) of adults now saying they have heard “a lot” about these drugs, up from 19% in July 2023.
- Most adults who have taken GLP-1 drugs say they took them to treat a chronic condition including diabetes or heart disease (62%), while about four in ten say they took them primarily to lose weight.
- About half (54%) of all adults who have taken GLP-1 drugs say it was difficult to afford the cost, including one in five (22%) who say it was “very difficult.” While most insured adults who have taken these drugs say their insurance covered at least part of the cost, even among insured adults about half (53%) say the cost was difficult to afford 2 .
- While 8% of adults ages 65 and older say they have taken a GLP-1 medication for a chronic condition, just 1% say they have ever taken a GLP-1 drug to lose weight, which may reflect Medicare’s lack of coverage for prescription drugs used for weight loss. Nearly four in ten (37%) adults ages 65 and older report being told by a doctor they are overweight or obese in the past five years.
- With Medicare currently prohibited by law from covering prescription drugs used for weight loss, six in ten adults say they think Medicare should cover the cost of these drugs when prescribed for weight loss for people who are overweight, including more than half of Democrats, independents and Republicans. Similar shares of the public continue to support Medicare coverage of these drugs for weight loss even after hearing arguments for and against this proposal.
Use, Access and Affordability of GLP-1 Drugs
KFF’s latest Health Tracking Poll examines the public’s views and use of an increasingly popular group of drugs that include Ozempic, Wegovy, Mounjaro and others that belong to a class of prescription medications known as GLP-1 agonists 3 . GLP-1 drugs have garnered an increasing amount of media attention and some notable celebrity endorsements in the U.S., with much of the focus on their use for weight loss, though many of these drugs are also prescribed to treat diabetes or reduce risk of heart attack or stroke.
A large and increasing share of the public say they have heard about GLP-1 drugs, with about eight in ten (82%) adults saying they have heard at least “a little” and about three in ten (32%) saying they have heard “a lot” about these drugs. The share of the public who report having heard about these drugs has increased since July 2023 when seven in ten adults reported having heard at least “a little” about these drugs and one in five (19%) said they had heard “a lot.”
The share who say they have heard “a lot” about these drugs rises to at least four in ten among those who have ever been told by a doctor that they have diabetes (45%) or heart disease (41%) or have been told by a doctor in the past five years that they are overweight or obese (42%) – the primary conditions these drugs are prescribed for.
Across age groups, awareness of these drugs is highest among older adults. About four in ten adults ages 50 to 64 and 65 and older say they have heard “a lot” about GLP-1 drugs, compared to about one-third of adults ages 30-49 (32%) and one in six adults ages 18-29 (17%). Notably, older adults are more likely than their younger peers to have been told by a doctor that they have diabetes or heart disease.
Adults with annual household incomes of $90,000 or greater are more likely than those with lower household incomes to say they have heard “a lot” about these drugs.
Overall, 12% of adults say they have ever used GLP-1 drugs, including 6% who say they are currently using them. The share who report ever taking these drugs rises to about four in ten (43%) among adults who have been told by a doctor that they have diabetes, a quarter (26%) of adults who have been told they have heart disease, and one in five (22%) adults who have been told by a doctor that they are overweight or obese in the past five years (some of whom also have diabetes or heart disease).
Black adults are somewhat more likely than White adults to report ever taking these drugs (18% v. 10%), while 13% of Hispanic adults say they have taken these drugs. KFF’s analysis of Centers for Disease Control (CDC) data shows that Black and Hispanic adults in the U.S. have a higher rate of obesity than White adults. For additional information on obesity rates and racial disparities, see KFF’s policy watch: What are the Implications of New Anti-Obesity Drugs for Racial Disparities?
Similar shares of adults regardless of gender, income, or health insurance coverage report taking these drugs.
Among the 12% of adults who have ever taken GLP-1 drugs, most report taking them, at least in part, to treat a chronic condition like diabetes or heart disease, with fewer saying they took them only to lose weight. Among those who have taken these drugs, six in ten (62%) say they took them to treat a chronic condition like diabetes or heart disease, including about four in ten (39%) who took them only to treat a chronic condition and one in four (23%) who say they took them to both treat a chronic condition and to lose weight. About four in ten (38%) adults who have taken these drugs report using them only to lose weight.
Among all adults, 7% say they have taken or are taking these drugs to treat a chronic condition such as diabetes or heart disease – either alone (5%) or in combination with intent of losing weight (3%) – while 5% of adults report ever taking these drugs to lose weight but not to treat a chronic condition.
About one in five (19%) adults ages 50-64 say they have ever taken GLP-1 drugs, higher than the shares reported by other age groups. Among adults ages 50-64, 15% say they have taken GLP-1 drugs to treat a chronic condition and 5% say they’ve taken them for weight loss only. Few adults under age 50 report having taken these drugs to treat chronic conditions, but similar shares of 18–29-year-olds (7%) and 30–49-year-olds (6%) report having taken them for weight loss. Among adults ages 65 and over, 8% say they have taken a GLP-1 medication for a chronic condition, while just 1% say they have taken these drugs only to lose weight, which may be a reflection of Medicare’s lack of coverage for prescription drugs used for weight loss. Nearly four in ten (37%) adults ages 65 and older report being told by a doctor they are overweight or obese in the past five years.
Alongside the relatively high cost of GLP-1 drugs in the U.S., there have been recent reports of shortages or limited availability of these drugs occurring as demand increases. Recent news reports have emphasized that some adults are seeking generic or compounded versions of these drugs through sources such as medical spas or compounding pharmacies, which may sell products claiming to be name-brand GLP-1s that have not been vetted by the F.D.A.
About eight in ten (79%) adults who have taken GLP-1 drugs report getting these drugs or a prescription for them from their primary care doctor or a specialist, while fewer report getting them from an online provider or website (11%), a medical spa or aesthetic medical center (10%), or from somewhere else (2%).
In the U.S., list prices for GLP-1 drugs can range from $936 to $1,349 before insurance coverage, rebates or coupons. Most insured adults who have taken GLP-1 drugs say their insurance covered at least part of the cost. Among adults with health insurance who report ever taking these drugs, over half (57%) say their health insurance covered part of the cost of these drugs and they paid the rest, while one in four (24%) say their health insurance covered the full cost. One in five (19%) insured adults who have taken GLP-1s say they paid for the full cost themselves.
Despite the fact that few insured adults say they paid the full cost of these drugs themselves, many report difficulty affording them. About half of adults who have taken GLP-1s say it was difficult to afford the cost of these drugs. Among those who have taken these drugs, about half (54%) – including 53% of those with health insurance – say it was either “somewhat” or “very difficult” to afford to pay for these drugs, including one in five (22%) who say it was “very difficult,” including a similar share of adults with health insurance (23%).
Public Opinion on Medicare Coverage of GLP-1s for Weight Loss
While some Medicare drug plans cover the cost of some GLP-1s such as Ozempic or Wegovy when prescribed to treat diabetes or prevent heart attacks or strokes for adults with heart disease, Medicare is currently prohibited by law from covering drugs when prescribed for weight loss – for more information, see KFF’s issue brief on Medicare coverage of GLP-1s . KFF’s latest Health Tracking Poll finds that most adults think Medicare should cover the cost of these drugs when prescribed for weight loss for people who are overweight, with support remaining largely unchanged after hearing arguments for and against this proposal.
Overall, six in ten adults (61%), including similar shares across age groups, say they think Medicare should cover the cost of these drugs when prescribed for weight loss for people who are overweight, a share that rises to about seven in ten (71%) among those who have ever taken these drugs.
While more than half of adults across partisans say Medicare should cover the cost of these drugs for weight loss, Democrats (66%) are somewhat more likely than Republicans (55%) to say this.
Attitudes on some policy proposals may change when the public hears different arguments in favor or against certain proposals. After asking whether Medicare should cover the cost of GLP-1s when prescribed for weight loss for people who are overweight, the poll presented two different arguments for and against this proposal:
Argument against: Some people say that if Medicare covers the cost of these drugs, it could increase premiums paid by people with Medicare and place financial pressure on the Medicare program and the federal budget.
Argument in favor: Others say that if Medicare covers the cost of these drugs, it could help more people afford these medications and improve health and quality of life for people who are overweight.
After being presented with these arguments, the public’s attitudes remain largely unchanged, with six in ten adults still saying they think Medicare should cover the cost of these drugs when prescribed for weight loss for people who are overweight. Attitudes also remained largely unchanged among adults 65 and older, among those who have taken GLP-1s and those who have not, and among independents and Republicans. Among Democrats, there is a slight increase in the share who say Medicare should cover the cost after hearing these arguments (71% after v. 66% before).
- Health Costs
- Tracking Poll
- Prescription Drugs
- Heart Disease
- Medicare Part D
- TOPLINE & METHODOLOGY
news release
- Poll: 1 in 8 Adults Say They’ve Taken a GLP-1 Drug, Including 4 in 10 of Those with Diabetes and 1 in 4 of Those with Heart Disease
Also of Interest
- Medicare Spending on Ozempic and Other GLP-1s Is Skyrocketing
- A New Use for Wegovy Opens the Door to Medicare Coverage for Millions of People with Obesity
- What are the Implications of New Anti-Obesity Drugs for Racial Disparities?
- How Do Prices of Drugs for Weight Loss in the U.S. Compare to Peer Nations’ Prices?
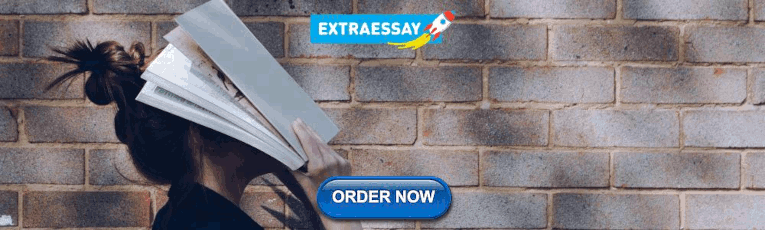
COMMENTS
Surveys are a special research tool with strengths, weaknesses, and a language all of their own. There are many different steps to designing and conducting a survey, and survey researchers have specific ways of describing what they do.This handout, based on an annual workshop offered by the Program on Survey Research at Harvard, is geared toward undergraduate honors thesis writers using survey ...
Statistical analysis is usually conducted using programs like SPSS or Stata. The same set of survey data can be subject to many analyses. Step 6: Write up the survey results. Finally, when you have collected and analyzed all the necessary data, you will write it up as part of your thesis, dissertation, or research paper.
Statistical analysis is usually conducted using programs like SPSS or Stata. The same set of survey data can be subject to many analyses. Step 6: Write up the survey results. Finally, when you have collected and analysed all the necessary data, you will write it up as part of your thesis, dissertation, or research paper.
Surveys are a powerful way to collect data for your dissertation, thesis or research project. Done right, a good survey allows you to collect large swathes of useful data with (relatively) little effort. However, if not designed well, you can run into serious issues.. Over the years, we've encountered numerous common mistakes students make when it comes to survey design.
Survey research is defined as "the collection of information from a sample of individuals through their responses to questions" ( Check & Schutt, 2012, p. 160 ). This type of research allows for a variety of methods to recruit participants, collect data, and utilize various methods of instrumentation. Survey research can use quantitative ...
Questionnaires vs. surveys. A survey is a research method where you collect and analyze data from a group of people. A questionnaire is a specific tool or instrument for collecting the data.. Designing a questionnaire means creating valid and reliable questions that address your research objectives, placing them in a useful order, and selecting an appropriate method for administration.
CGIS - K-357Presented by:Chase H. Harrison, Ph.D.Preceptor in Survey Research Surveys are a special research tool with strengths, weaknesses, and a language all of their own. There are many different steps to designing and conducting a survey, and survey researchers have specific ways of describing what they do.
You want to gather data in a form in which you can analyze it. [In this case the method is a survey administered to a group of people]. If appropriate, you should indicate what rules for interpretation or what kinds of statistical tests that you'll use. 6. Tentative Dissertation Outline. Give your committee a sense of how your thesis will be ...
Ensure that you only collect data that's relevant to your dissertation survey and leave out any questions (such as name) that can identify the respondents. Create dissertation questionnaires with SurveyPlanet. Overall, survey methodology is a great way to find research participants for your research study.
This article is a practical guide to conducting data analysis in general literature reviews. The general literature review is a synthesis and analysis of published research on a relevant clinical issue, and is a common format for academic theses at the bachelor's and master's levels in nursing, physiotherapy, occupational therapy, public health and other related fields.
The results chapter (also referred to as the findings or analysis chapter) is one of the most important chapters of your dissertation or thesis because it shows the reader what you've found in terms of the quantitative data you've collected. It presents the data using a clear text narrative, supported by tables, graphs and charts.
A literature review is a survey of scholarly sources on a specific topic. It provides an overview of current knowledge, allowing you to identify relevant theories, methods, and gaps in the existing research that you can later apply to your paper, thesis, or dissertation topic. There are five key steps to writing a literature review:
Empirical data: unique research that may be quantitative, qualitative, or mixed.. Theoretical data: secondary, scholarly sources like books and journal articles that provide theoretical context for your research.. Thesis: the culminating, multi-chapter project for a bachelor's, master's, or doctoral degree.. Qualitative data: info that cannot be measured, like observations and interviews.
The results chapter in a dissertation or thesis (or any formal academic research piece) is where you objectively and neutrally present the findings of your qualitative analysis (or analyses if you used multiple qualitative analysis methods ). This chapter can sometimes be combined with the discussion chapter (where you interpret the data and ...
Survey data is defined as the resultant data that is collected from a sample of respondents that took a survey. Learn survey data collection methods along with examples for both, types of survey data based on deployment methods and types of survey data based on the frequency at which they are administered. This article also throws light on the steps to conduct survey data analysis.
With its many data analysis techniques, SurveyMonkey makes it easy for you to turn your raw data into actionable insights presented in easy-to-grasp formats.Features such as automatic charts and graphs and word clouds help bring data to life. For instance, Sentiment Analysis allows you to get an instant summary of how people feel from thousands or even millions of open text responses.
actions, or opinions of a large group of people". three distinguishing characteristics of survey research. First, survey research is used to quantitatively describe specific. aspects of a given ...
The analysis and interpretation of data is carried out in two phases. The. first part, which is based on the results of the questionnaire, deals with a quantitative. analysis of data. The second, which is based on the results of the interview and focus group. discussions, is a qualitative interpretation.
How to analyze survey data. The first step when analyzing survey data is to turn your individualized responses into aggregated numbers. This sounds complicated, but really, it just means you need to do some counting. For every question in your survey, you need to know the total number of people who answered with each response.
This paper introduces a dataset developed to measure the impact of archival and data curation decisions on data reuse. The dataset describes 10,605 social science research datasets, their curation ...
Explain the Survey Data in your Honors Thesis Chase H. Harrison Ph.D. Program on Survey Research Harvard University Surveys Systematic method of data collection Usually use samples Designed to measure things Attitudes Behaviors Create statistics Descriptive Analytic Overview of Research Process Research Theories Survey Methods Reporting And ...
dentialize survey data from a large-scale healthcare study. To date, application of synthetic data techniques to healthcare survey data is rare. We discuss identi cation ... 1In this thesis we adopt the de nitions of privacy and con dentiality as used in Gates (2011), p. 3. 'Information privacy' is de ned as the individual's desire (claim ...
To collect the data for testing our model we have chosen to use a web-based survey. For a more detailed argumentation on the choice of the research method, we refer to Chapter 4. This thesis is organized into six chapters including this introduction. The next chapter reviews the existing literature.
The survey also collected data about race/ethnicity and other identities. Nearly 15,000 students, postdocs, faculty, and staff took part in the survey. Stanford released the quantitative findings ...
A new analysis of data from KFF's Survey on Racism, Discrimination, and Health shows Black women are more likely than other groups to report being treated unfairly by a health care provider in ...
The Clean Watersheds Needs Survey (CWNS) provides an assessment of the capital investments necessary for states, the District of Columbia, and U.S. Territories to meet the Clean Water Act's (CWA) water quality goals over the subsequent 20 years. ... The manual is a guide on survey policy, terminology, and data entry in the online portal. ...
Here are a few best practices: Your results should always be written in the past tense. While the length of this section depends on how much data you collected and analyzed, it should be written as concisely as possible. Only include results that are directly relevant to answering your research questions.
Currently, the only sub-national SPM estimates available using 1 year of survey data are at the Public Use Microdata Area (PUMA) level and at the state level using 1-year ACS data. In this paper, county-level SPM estimates are produced from PUMA-level estimates using a county to PUMA crosswalk from the Missouri Census Data Center. There are ...
Polling. KFF's latest Health Tracking Poll examines the public's views and use of an an increasingly popular class of prescription drugs used for weight loss and to treat diabetes or prevent ...