- Open access
- Published: 22 June 2022
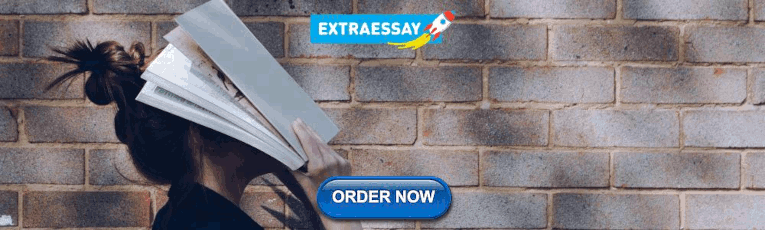
How can big data analytics be used for healthcare organization management? Literary framework and future research from a systematic review
- Nicola Cozzoli 1 ,
- Fiorella Pia Salvatore ORCID: orcid.org/0000-0001-6294-3360 1 ,
- Nicola Faccilongo 1 &
- Michele Milone 1
BMC Health Services Research volume 22 , Article number: 809 ( 2022 ) Cite this article
18k Accesses
23 Citations
17 Altmetric
Metrics details
Multiple attempts aimed at highlighting the relationship between big data analytics and benefits for healthcare organizations have been raised in the literature. The big data impact on health organization management is still not clear due to the relationship’s multi-disciplinary nature. This study aims to answer three research questions: a) What is the state of art of big data analytics adopted by healthcare organizations? b) What about the benefits for both health managers and healthcare organizations? c) What about future directions on big data analytics research in healthcare?
Through a systematic literature review the impact of big data analytics on healthcare management has been examined. The study aims to map extant literature and present a framework for future scholars to further build on, and executives to be guided by.
The positive relationship between big data analytics and healthcare organization management has emerged. To find out common elements in the studies reviewed, 16 studies have been selected and clustered into 4 research areas: 1) Potentialities of big data analytics. 2) Resource management. 3) Big data analytics and management of health surveillance systems. 4) Big data analytics and technology for healthcare organization.
Conclusions
In conclusion is identified how the big data analytics solutions are considered a milestone for managerial studies applied to healthcare organizations, although scientific research needs to investigate standardization and integration of the devices as well as the protocol in data analysis to improve the performance of the healthcare organization.
Peer Review reports
Big data is transforming and will transform the healthcare organizations in the near future [ 1 , 2 ]. Scientific literature in the managerial context applied to healthcare organizations, consider the Big Data Analytics (BDA) a fundamental tool, so much so that it has attracted the attention of the scientific community and stakeholders [ 3 ]. However, a premise should be made: data by themselves explain little, thus, to be useful in the healthcare organization management, firstly it is necessary to validate their quality, and secondly, find the right correlations. In other words, the data should be processed, analyzed, and interpreted with the appropriate tools [ 4 , 5 ].
Technological applications in healthcare BDA-related are rapidly increasing [ 6 ] and will increasingly characterize managers’ decision-making process. For example, IBM’s Watson project [ 7 ] is a "super-computer" that has scoured through several million scientific articles over the last twenty years and uses artificial intelligence tools (e.g., Machine Learning) to correlate disease symptoms and predict possible diagnostic scenarios. This case helps to understand how and to what extent BDA could really support healthcare managers to improve their decision processes, while increasing the performance of the healthcare organization.
Nowadays, the amount of data is no longer an issue. Internet traffic reports from Cisco and other network operators have estimated the entire digital universe to be 44 zettabytes and 463 exabytes will be the daily information could be generated by 2025. A new era took place in which the processes of production and management of human knowledge will no longer be the exclusive preserve of humans; machines will also play their part as knowledge producers [ 8 ]. From pharmaceutical companies to healthcare organizations, this enormous potential of data products, combined with IoT applications and AI tools [ 9 , 10 , 11 ], will play a significant role in the near future. Today, the medical applications based on IoT allow the monitoring of clinical data through the production of data generated by special devices (e.g., wearable devices) [ 12 ], remotely accessible by a physician rather than by caregivers [ 13 ].
The market size is a useful indicator of how much the healthcare organizations are turning their attention to new management models based on the use of big data. By 2025, the big data market in healthcare will touch $70 billion with a record 568% growth in 10 years. The use of such a tool not only represents a complex challenge [ 14 ], but also opens opportunities for all those involved in the healthcare supply chain who manage decision-making processes. Moreover, if on the one hand this technology will influence the definition of new managerial strategies within healthcare organizations, on the other hand, it will have positive repercussions on the effectiveness and efficiency of healthcare processes [ 15 ]. Indeed, the big data technology is used by healthcare managers to get, for example, information related to the list of doctors and nurses, the list of drugs with their expiration date, etc., in order to have tools for facilitating decision-making processes, improving the quality of services provided, and, at the same time, rationalizing the use of resources, by facilitating the management of the healthcare organization as a whole.
The BDA satisfies multiple needs that, on the one hand, influence the quality of the healthcare organization’s performance and, on the other hand, are useful in directing management strategies to improve the supply of healthcare services. Below there are some strategies, which aim to:
Provide specific services to patients, from diagnostics to preventive medicine passing through therapeutic adherence.
Detect the onset and spread of diseases in advance.
Observe parameters inherent to hospital quality standards, promoting control and prevention actions.
Modify treatment techniques.
Facilitate research and development in pharmacology, reducing the time to market of drugs.
Facilitate research and development of new and specific medical devices.
The main aim of this research is, therefore, to provide both an integrative framework on the state of art, and perspectives on how the BDA can be useful for the management of the healthcare organization. Considering the results, food-for-thought on how this technological and cultural revolution will affect the modus operandi of healthcare organizations will be launched.
Through an overview of recent scientific studies, this research aims to raise awareness among both practitioners and managers about BDA tools applied to healthcare management to address more effectively and efficiently the challenges imposed by an increasing demand for healthcare services.
In this regard, the study provides a systematic literature review (SLR) to explore the effect of BDA on the healthcare management by analyzing articles from the Scopus database during a period of 5 years (2016 – 2021).
Furthermore, the result through a content analysis, aspires to be a privileged starting point to find out potential barriers and opportunities provided by BDA-based management systems for smarter healthcare organization. Specifically, the study answers different research questions (RQs) as different levels of analysis have been performed. By analyzing the relationship between BDA-based management systems and the benefits delivered to the organizations, the research could not be conducted without exploring the state of art of BDA tools deployed in the field of healthcare. Thus, starting from this background the discussion on the future perspectives on BDA development in the healthcare organizations appears as a need.
Theoretical framework
Why use BDA and how to exploit its potential for healthcare organization management? This is the main question asked by managers and decision makers working in the healthcare sector. In recent years there have been multiple attempts in the literature aimed at highlighting the relationship between implementation of BDA and benefits for healthcare organizations, in terms of both resource efficiency and process management.
In 2017, a study by Wang and Hajli [ 16 ] has proposed a model founded on Resource-Based Theory and BDA Capabilities (BDAC) to explain the relationship between BDA, benefits, and value creation for healthcare organizations. As stated by Srinivasan and Swink [ 17 ], BDAC refers to “ organizational facility with tools, techniques, and processes that enable a firm to process, organize, visualize, and analyze data, thereby producing insights that enable data-driven operational planning, decision-making, and execution ”. In the healthcare organization, BDAC represents the ability to collect, store, analyze, and process huge volume variety, and velocity of health data come from various sources to improve data-driven decisions [ 18 , 19 ]. Indeed, the study of Wang and Hajli [ 16 ], validated on an empirical basis by 109 cases of BDA tools implementation in 63 healthcare organizations, has demonstrated how specific "path-to-value" can be identified. By varying degrees of relevance of the identified pathways, it has been shown that alongside the challenges of implementing certain BDA tools, there are corresponding specific benefits for healthcare organizations. Preliminarily, the study has defined the ability to analyze big data through the concept of Information Lifecycle Management (ILM) [ 20 ]. In this perspective, the capabilities of the BDA in healthcare organizations are configured as the abilities to process health data from diverse sources and provide significant information to healthcare managers. Thorough BDA, managers can detect timely indicators and identify business strategies, which allow them to put in place perspective plans, efficient strategies, and programs to increase the performance of organizations.
Researchers have found that BDA capabilities primarily stem from the implementation of various tools and features. Specifically, in order of importance, BDA capabilities are firstly triggered by processing tools (e.g., OLAP, machine learning, NLP), followed by aggregation tools (e.g., data warehouse tools), and, secondly, by data visualization tools and capabilities (e.g., visual dashboards/systems, reporting systems/interfaces).
Among the potentials triggered by the implementation of BDA in the healthcare organization, the analytical one was the main capability, that is the ability to process clinical data characterized by immense volume, variety (from text to graph), and speed (from batch to streaming), using descriptive analysis techniques [ 21 , 22 ]. In this regard, it is important to note that BDA-based management systems are the only ones capable of analyzing semi-structured or unstructured data. This represents a crucial element for revealing correlation patterns that are difficult to determine with traditional management systems [ 23 ]. Furthermore, the launch of these systems in a healthcare organization ensures the ability to effectively manage outputs regarding care process and service in order to constantly improve the performance of the organization. In summary, the characteristics of BDA-based management systems implemented in a healthcare organization, are:
predictive analytics capability, i.e., the ability to explore data and identify useful correlations, patterns and trends, and extrapolate them to predict what is likely to occur in the future [ 24 , 25 ];
interoperability capability, i.e., the ability to integrate data and processes to support management, collaboration, and sharing across different healthcare departments, managers, and facilities [ 26 ], and finally,
traceability capability, i.e., the ability to integrate and track all patient history data from different IT facilities and different healthcare units.
In terms of expected benefits from the BDA implementation, the study of Wang and Hajli [ 16 ] has showed that the most important ones are obtained from improved operational activities, such as improved quality and accuracy of healthcare decisions, rapid processing of issues, and the ability to enable treatments proactively before patients’ conditions worsen. Next, in terms of relevance, they were the benefits related to IT infrastructure, such as standardization and reduced costs for redundant infrastructure and the ability to quickly transfer data between different IT systems. Substantially, they have delivered a useful business model that healthcare managers can draw on to evaluate the specific leverages they need to activate in relation to the implementation of the BDA-based management systems. In addition to highlighting the undoubted benefits, the authors clearly show how specific BDA tools can facilitate the decision-making processes of healthcare managers and make them faster and more effective.
In another study carried out to identify BDA benefits and supports, and to drive organizational strategies, Wang, Kung, and Byrd [ 19 ], through the analysis of 26 case studies related to the BDA applications in the healthcare organization, have identified five "capabilities" of BDA: analytic capability for care patterns, unstructured data analytical capability, decision support, predictive, and traceability capabilities [ 19 ]. The study is remarkably interesting because in addition to mapping precise benefits, it also recommends specific strategies considering the BDA implementation for healthcare organizations. These strategies are useful for achieving effective results by leveraging the potential of BDA.
The first successful strategy is to implement governance based on the use of big data, starting with a definition of objectives, procedures, and key performance indicators (KPIs). Once again, one of the discriminating factors for success in implementing such a strategy remains the integration of information systems and the standardization of data protocols that often come from heterogeneous sources already existing in healthcare organizations. The second strategy is related to developing a culture of data sharing. The third one considers the training of healthcare managers, who cannot ignore knowledge related to BDA, for example on the use of data mining and business intelligence tools. The fourth strategy is related to the storage of big data, often available in heterogeneous formats, and is identified in the transition from the more expensive traditional storage systems (NAS) to more efficient and effective systems such as cloud computing solutions. The last strategic driver involves pathways related to the implementation of predictive BDA models. The mastery of KPIs, interactive visualization and data aggregation tools such as dashboards and reports should be acquired instruments for healthcare managers and in general for healthcare organizations oriented to BDA driven process management strategies.
More recent studies focus attention on the management practices supply chain in healthcare. In the study performed by Yu et al. [ 27 ], the authors, interviewing senior executives in Chinese hospitals, show on both a theoretical and empirical basis, how BDAC positively impacts the three dimensions of hospital supply chain integration (SCI) (inter-functional integration, hospital-patient integration and hospital-supplier integration) and how SCI, in turn, contributes to improve the operational flexibility [ 27 ]. By “operational flexibility” in the healthcare organization, it is meant the ability of a ward to adapt its operating procedures in relation to unforeseen circumstances while meeting the needs of patients [ 28 , 29 ].
The scholars have delivered an important contribution in demonstrating the relationship between BDAC, SCI, and operational flexibility from multiple perspectives, by providing useful management guidance for healthcare executives and managers involved in the supply chain. By analyzing and processing medical and managerial data with advanced analytical techniques, Chinese healthcare organizations were able to facilitate decision-making process with timely and appropriate actions, for example, tracking people's movements during the lockdown caused by the Coronavirus, understanding ongoing health trends, and managing pharmaceutical supplies [ 30 , 31 ].
This theoretical framework provides a key to interpreting the benefits offered by good practices deriving from the use of the BDA in the healthcare organization.
At the same time, the rigorous scientific method allows the validation of empirical experiences in relation to clear theoretical references. In the next paragraph projects that demonstrate what is stated in the literature are shown.
Practical framework
N(ursing) + Care App is an mHealth application that supports the work of frontline health workers (FHW) in developing countries [ 32 ]. The system is designed to collect not only patient data, but also diagnostic images. It is also given the opportunity to add recommended doctors based on the advice of FHWs in case the patient needs to follow a specific hospital visit.
For healthcare managers, predicting the number of emergency department accesses is a critical issue which complicates the optimization of the human resource management. To this end, Intel, and Assistance Publique-Hôpitaux de Paris (AP-HP), the largest hospital university in Europe, leveraging datasets from multiple sources, worked together to build a cloud-based solution to predict the number of patient visits to emergency rooms and hospital admissions. This predictive analytics tool, will enable healthcare managers at AP-HP hospitals to know the number of emergency room visits and hospital admissions at 15 days in order to reduce wait times, optimize human resource (HR) levels based on anticipated needs, accurately plan patient loads, including by pathology, and overall improve the quality and efficiency of services provided by the healthcare organization [ 33 ].
Chronic conditions, if not kept under control through a rigorous program of therapeutic adherence, can become a source of both more serious physical problems for patients and economic burdens for healthcare organizations. Another project that actively introduced BDA tools into healthcare management was carried out by the European Commission to launch production of the drug Enerzair Breezhaler . It was the first drug for the treatment of asthma co-packaged and co-prescribed with the Propeller digital platform. The app sends a reminder to comply with therapeutic adherence and maintains a record of the data, which the patient shares with him or her physician. Studies have demonstrated that the Propeller platform increases the degree of asthma control by up to 63%, therapeutic adherence by up to 58% [ 34 ], and reduces asthma emergency department visits and hospital admissions by up to 57% [ 35 ].
The practical framework described, aided by some empirical experience, only partially reveals the potential offered by BDA. The diffusion of BDA-based management systems in the healthcare organization will trigger a virtuous circle, allowing soon to accumulate increasingly accurate medical data. By exploiting the most advanced AI technologies, BDA will support predictive analysis, allow physicians to make more accurate and faster diagnostic pathways and managers to use results. It will help health practitioners in the decision-making process, optimize the use of resources with a consequent costs reduction and, overall, improve the quality of services provided by healthcare organizations.
The main aim of this study is to update the state of art about the BDA-based management systems adopted in the healthcare organization, underlining management advantages for both the organizations and managers. BDA has the potential to reduce the cost of care, prevent disease outbreaks, and improve the patients’ quality of life. Through its ability to process and cross-reference massive amounts of both management, and clinical information, BDA promises to be an effective support tool for both healthcare managers and patients.
To achieve this aim, a Systematic Literature Review (SLR) was performed. This method identifies, evaluates, and summarizes the updates that raise from the literature about the BDA tools used to improve both the healthcare organizations performance and patients’ quality of life. The method takes inspiration from the protocol used by Khanra S., et al. [ 36 ] which considers inclusion and exclusion criteria.
The present study aims to add a contribute to the literature by addressing three RQs:
What is the state of art of BDA adopted by healthcare organizations?
What about the benefits for both health managers and healthcare organization?
What about future directions on BDA research in healthcare?
To answer the RQs, as widespread electronic database Scopus has been selected. To obtain an international validity of studies, the research only considers papers in English. Utilizing the Boolean operator “AND”, the following keywords have been searched: “big data analytics” AND “healthcare” AND “management”. As inclusion criteria, only papers published from 2016 to 2021 have been considered. As subject areas, “medicine” and “business, management and accounting” have been selected. Instead, as exclusion criteria, article in press and the following documents type: “review”, “book”, “conference review”, “letter” and “note” have not been taken into account. Also, to avoid a dispersal of the study, conference proceedings have been excluded. Following the searching protocol, 34 results have been obtained (Fig. 1 ).
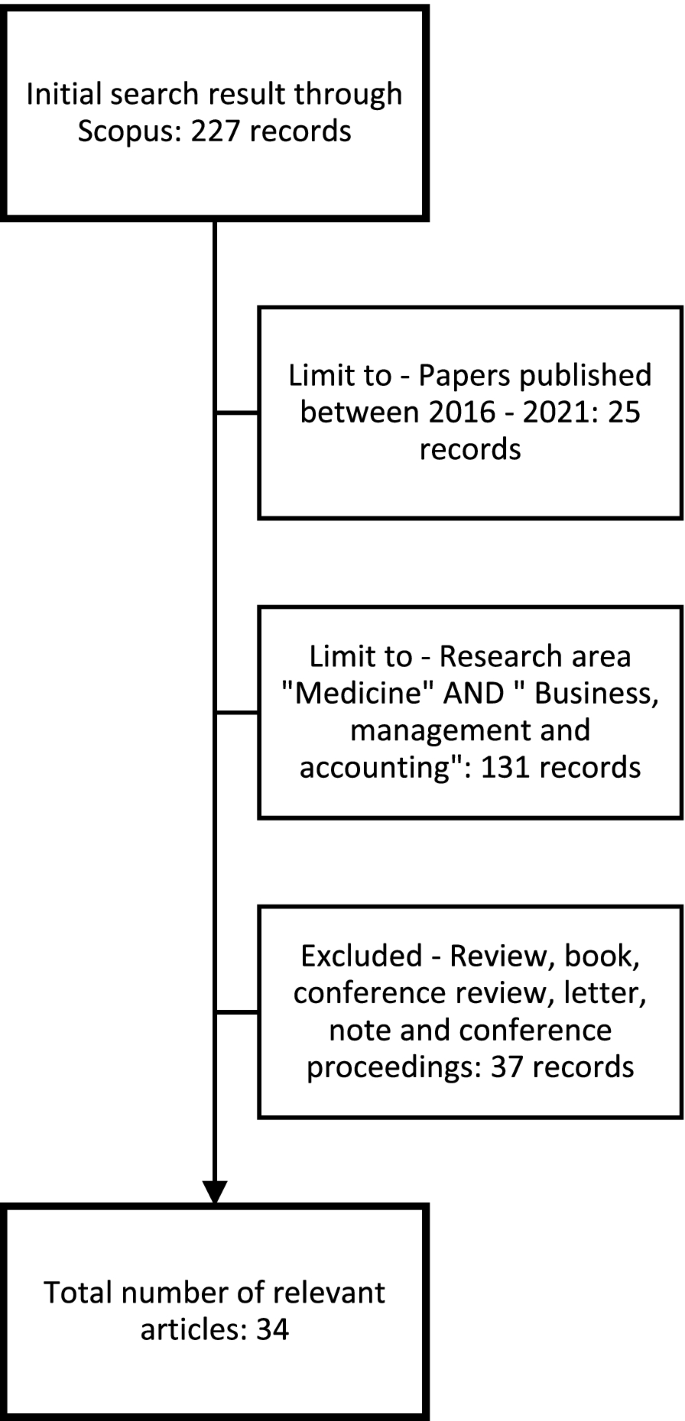
Workflow of articles selection
An excel spreadsheet was used to perform the extraction procedures while the statistical analyses were carried out using the software STATA 16 ©. The list of the extracted papers investigated with the content analysis can be found in the Appendix.
The work proceeds through a descriptive analysis. After that, a content analysis has been performed to identify the most relevant characteristics of the BDA-based management systems, underlining the positive impact for the healthcare organizations, without neglecting to outline the trends for the future scenarios and research directions.
According to the SLR, the iterative process shown in the Fig. 1 , has allowed to delete the duplicates and match the results with the RQs.
As shown in Fig. 1 the initial search on Scopus database has delivered 227 results. By limiting research to papers published between 2016 and 2021, 11% of records have been removed. At the second stage, by selecting the subject areas, the screening has allowed to exclude 131 records; thus, the 57.7% of the results initially selected. The last step of the process has conducted to exclude document types such as Review, Book, Conference Review, Letter, and Note. In other words, 37 records were excluded, representing 16.3% of the sample. At the end of the screening process, 34 articles were selected, representing about 15% of the sample.
In the descriptive analysis the time distribution of the studies from 2016 to 2021 is included. It is important to note the increasing of publication trend from 2017 to 2019. This output confirms a growing interest in the research field of BDA applied to healthcare organizations (Fig. 2 ).
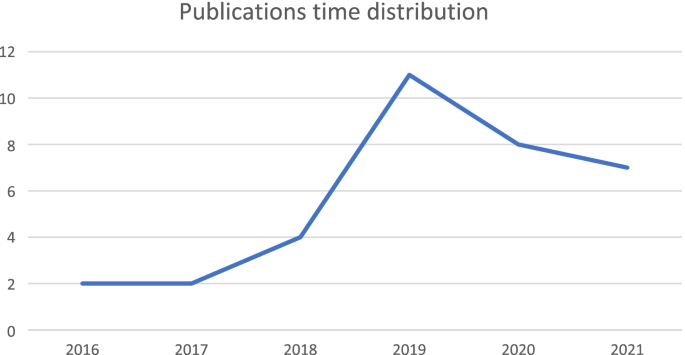
Trend of research steams
The trend of research steams considers a sample of 34 scientific contributions as they come from the screening process above described. Although 6% of the total sample was collected in the years 2016 and 2017, it is only indicative of the growing trend of scientific studies on BDA in healthcare sector. The overall incidence in 2018 was 12% but the turning point was reached in 2019 as 32% of the studies collected in the sample were reached. This outcome could be read considering the Covid-19 pandemic outbreak which has been a representative testing ground for BDA tools by helping managers and decision-makers to plan healthcare managerial strategies.
In this context, the use of the BDA by Chinese healthcare organizations for tracking people's flow during the lockdown, represents an important case study that has registered the peak in the time flow of research. By looking at 2020 and 2021 data, which represent respectively 24% and 21% of the total scientific contributions, the growing trend seems to be confirmed by validating the rising interest in BDA research seen as a planning tool for healthcare processes.
The pie-chart shows the scientific production by country. It is necessary to specify that Scopus database clusters the studies by home country author’s organization, therefore the same study could be referred to more than one country and thus belong to more than one cluster.
The geographical locations of the studies showed in the Fig. 3 outlining India, UK, and USA as more than one third of the total scientific producers. It is well known that IT companies as Google, Apple, Amazon, and Microsoft are investing considerable resources on BDA tools for healthcare. China and India contribute together with 22% of the scientific articles. Big data technology has played a key role in virus tracking during the pandemic crisis. The "Internet Plus Healthcare", a big data center in Zhongwei (China), provides cloud services to both healthcare institutions and IT companies. In Yinchuan (China), an industrial park for big data acts as a catalyst for IT company involved in healthcare sector. India confirms to be one of the heavily adopter countries of artificial intelligence, big data analytics, and IoT technologies. Although India must face the challenge to provide basic healthcare services in a predominantly rural country, start-ups with BDA skills in healthcare are springing up.
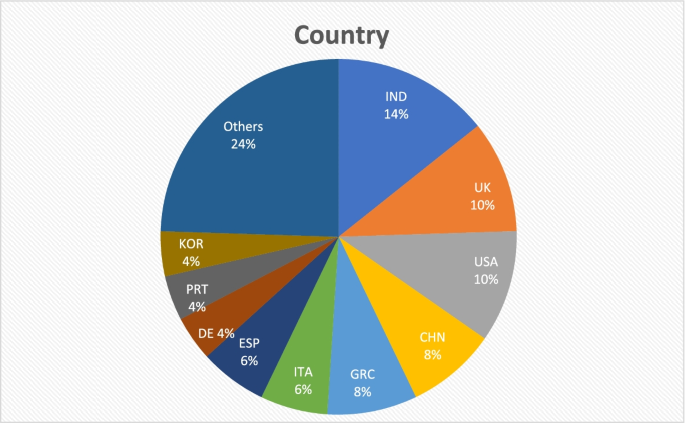
Geographical locations of the studies
It is also important underlining the performance of the European countries. UK, Greece, Italy, Spain, Germany, and Portugal support the research with almost 40% of the studies published, confirming that Europe will be a driving force for the BDA research in the next future. The development of a European Health Data Space (EHDS) is an ambitious project of the European Commission. It will lead member states to share an efficient infrastructure for both exchange and management health data by providing citizens with equal treatment, free access to clinical data, and quality healthcare services.
In the area “Others” all the other countries contributing marginally to research have been included.
The next step of the study is focused on a content analysis to show the experiences of applying BDA in healthcare organizations.
Starting from the 34 articles selected for the descriptive analysis, to identify in detail the core issue of the study, a second screening was performed. 18 articles were excluded because weakly focused on the research objective which concerns specifically how BDA can be used for healthcare organization management. Thus, after an in-depth reading of abstracts and full papers, the scholars have identified 16 papers closer targeted on the mentioned research objective. The 16 studies selected through a content analysis were clustered into 4 research areas (RAs) as showed in the following table (Table 1 ). The clustering procedure identifies 4 relevant topics: Potentialities of BDA (RA1), Resource management (RA2), BDA and management of health surveillance system (RA3), BDA technology for healthcare organization (RA4). The proposed clustering has been though to give an easy-to-go research map and to support the healthcare managers.
RA1: potentialities of BDA
Wang and Hajli [ 16 ] define BDA potentialities in the healthcare context as “ the ability to acquire, store, process and analyze large amounts of health data in various forms, and deliver meaningful information to users, which allows them to discover business values and insights in a timely fashion ”. The relationship between BDA and the benefits for the healthcare organizations it has been well expressed by the theory of the “path to value chain” [ 16 ]. This path represents an important contribution to the exploration of business value, not only for drawing the generic and well-established connection between big data capabilities [ 19 ] and the benefits, but also for empirically showing how capabilities can be developed and what benefits can be achieved in the healthcare organizations. Another study included in this area, explores the key role of BDA capabilities in developing healthcare supply chain integrations and its impact on hospital flexibility [ 27 ]. Specifically, the BDA has a fundamental role in developing healthcare integration supply chain and the operational flexibility. Considering the health and economic crises caused by the Covid-19, this dimension of BDA has been an especially important leverage for managers to improve operational flexibility of the healthcare organizations. The ability to provide predictive models and real-time insights, is a powerful prospective of the BDA for helping healthcare professionals and managers in decision-making process. In this regard, the literature presents several applications of big data in healthcare that support the data collection, management, and integration of data in healthcare organizations [ 37 ]. Moreover, BDA enables the integration of massive datasets, supporting decisions of manager and monitoring the managerial aspects of healthcare organizations. Building a decision-making process based on BDA, firstly means identifying the big data keys that can implement ad-hoc strategies to improve efficiency along the healthcare value chain. To this end, the research carried out by Sousa et al., [ 37 ] underlines the benefits that BDA can give to the decision-making process, through predictive models and real-time analytics, assisting in the collection, management, and integration of data in healthcare organizations.
To date, thanks to an integrated and interconnected ecosystem, is becoming possible to provide personalized healthcare services, collect an enormous quantity of both clinical and biometrics data and, thus, implement BDA instruments. Nevertheless, to take a real advantage from these tools and turn them into useful decision support systems (DSS), is necessary for R&D to be focused on data filtering mechanisms in order to obtain good-quality reliable information [ 38 ]. The healthcare models based on BDA and implementation of new healthcare programs, enable both medical and managerial decision support for the healthcare services provision. New types of interactions with and among users of the healthcare ecosystem will produce in the next future a wide variety of complex data, thus, the main challenges refer to information processing and analytics.
In light of the above, the RA1 includes studies for which the quality of data and the need for high performance filtering mechanisms are becoming keys factor for the success of BDA-based management systems in the healthcare organizations. For example, the study carried out by Maglaveras et al., [ 38 ], included in this area, explores new R&D pathways in biomedical information processing and management, as well as to the design of new intelligent decision support systems.
RA2: resource management
Another important research direction emerged from the literature review, concerns positive impact of the BDA on the resource management. Insufficient policy for managing medical materials waste, energy use and environmental burden, restricts the resources conservation. The BDA is extremely useful in this aspect; it could provide in the next future an important contribution to implement the circular economy processes and to support sustainable development initiatives in the healthcare organizations [ 39 ]. To this end, the study developed by Kazançoğlu et al. [ 39 ], underline the importance of circularity and sustainability concepts to mitigate the sector’s negative impacts on the environment. Furthermore, the study identifies the barriers related to circular economy in the healthcare organization and provides solutions to these barriers by implementing BDA-based management systems. Lastly, the authors, have developed a managerial, policy and theoretical framework to support healthcare managers to launch sustainable initiatives in the context of healthcare organization.
The impact on the performance has been also investigated by studies that have linked benefits of BDA and artificial intelligence with green supply chain integration process [ 40 ]. Digital learning is more becoming a “moderator” of the green supply chain process with a significant positive impact on environmental performance of the healthcare organization. BDA-AI technologies will lead to improvement of the environmental process integration and green supply chain collaboration and, consequently, will support the managers’ decisions involved in the supply processes. This study also provides an important reference framework for logistics/supply chain managers who want to implement BDA-AI technologies for supporting green supply processes and enhancing environmental performance of the healthcare organization [ 40 ].
Nowadays, many scholars are focusing on BDA-driven decision support systems to sustain the healthcare managers [ 41 ]. These types of BDA-based analytical tools will provide a useful quantitative support for managers of healthcare organizations. The authors have reported design and technical details of the system implementations using case studies. They have developed a toolkit which represents a framework reference for resources management, allowing to create strategic models and obtain analytical results for evidence-based decisions and managerial evaluations.
In this RA, two other important topics investigated by BDA are: high quality healthcare service, and healthcare costs. Optimize the supply chain activities is an imperative to keep lower the healthcare costs. The data generated by medical equipment and devices can be successfully used in forecasting, decision-making process, and to make more efficient the healthcare supply chain management [ 42 ]. The study carried out by Alotaibi et al. [ 42 ], thus, presents a review on the use of big data in healthcare organizations underling opportunities and challenges deriving from the application of BDA-based management systems within the organizations.
As already asserted, a good implementation of BDA in the healthcare organization will play a fundamental role in improving the clinical outcomes management, giving helpful insights for decision makers and managers, in order to avoiding diseases, reducing healthcare expenses, and improving the performance of the healthcare organization [ 43 ]. However, to achieve these ambitious outcomes the research will face a crucial challenge: how to rationalize, make easily usable, and at affordable costs, heterogeneous data coming from diverse sources. The research developed by Kundella and Gobinath [ 43 ] represents an important contribute to explore key challenges, techniques, technologies, privacy issues, security algorithms and future directions of the use of BDA in the healthcare organization.
RA3: BDA and management of health surveillance system
The rise of BDA promises to solve many healthcare challenges in the developing countries. The BDA applied to healthcare organization help managers to rationalize the resources, and health system to better delivery treatments to the patients [ 44 ]. In this regard, the government of Zambia is thinking to implement BDA solutions to provide more effective and efficient healthcare services. A well-managed health surveillance system represents an important driver to improve the quality of life and reduce the medical waste, especially in developing countries where the lack of resources is severe and limits economic development. For all these reasons, Europe is investing on BDA initiatives in public health and in the oncology sectors, to generate new knowledge, improve clinical care and make more efficient the management of the public health surveillance system [ 45 ]. The BDA capability for identifying specific population pattern, managing high volume of data and turn it into real (or near real) time insights, contributes to identify it as a powerful tool to support the managers for the decision-making processes. Despite this, implementing a BDA-based management systems within the healthcare organizations requires investment in the human capital, strong collaboration with stakeholders, and data integration with and among the healthcare units. To this end, Gunapal et al., [ 46 ] has highlighted that Singapore has setup a Regional Health System (RHS) database to facilitate BDA for proactive population health management (PHM) and health services research [ 46 ]. The structure of the healthcare database has been built collecting data from four database coming from three RHSs: National Healthcare Group (NHG), Tan Tock Seng Hospital (TTSH), National University Hospital (NUH) and Alexandra Hospital (AH). The result has been a database including information useful for the healthcare managers which incorporates data on patient demographics, chronic disease, and healthcare utilization information. These characteristics facilitate the identification of specific patients’ paths linked by past healthcare utilization and chronic disease information. Converging information into a single database helps to understand the cross-utilization of healthcare services across the three RHSs. A such approach allows to setup the RHSs structure for initiative-taking population health management (PHM) and to improve the performance of healthcare organizations [ 46 ].
RA 4: BDA technology for healthcare organization
The wearable devices and different kind of sensors, able to collect clinical data, in combination with BDA, will constitute the basis of personalized medicine and will be crucial tools to improve the performance of healthcare organizations [ 47 ]. The scientific research has to face the important challenge to adapt data acquisition, storage, transmission and analytics to healthcare demand. Thus, the healthcare data should be categorized, homogenized, and implemented into specific models by adapting machine-learning techniques to the nature of the healthcare organization.
A fruitful field of interest for the application of BDA in healthcare organization is the diagnostic imaging. To take out maximum benefits from it and to be useful for managers of healthcare organizations, it is necessary to implement digital platforms and applications [ 48 ]. Indeed, the simple production of a large amount of data does not automatically translate to an advantage for the healthcare performance. Specific applications are required to favor the correct and advantageous management of diagnostic images [ 48 ]. The link between BDA and IoT technologies, as instrument to incorporate the accessibility, capacity to customize, and practical conveyance of clinical data, emerged as another research direction investigated by the papers included in this RA. These tools allow: (1) the healthcare organizations to decrease expenses; (2) the people to self regulates treatments; (3) practitioners to take as quickly as possible decisions in remote way and keep constant contact with patients [ 49 ].
In light of these results, it is possible to state that IoT, big data, and artificial intelligence as machine-learning algorithms, are three of the most significative innovations in the healthcare organization. These types of organizations are implementing home-centric data collection networks and intelligent BDA systems based on machine learning technologies. For example, a high-level implementation of these systems has been efficiently implemented in Cartagena, Colombia, for hypertensive patients by using an e-Health sensor and Amazon Web Services components [ 50 ]. The authors stress the importance of using the combination of IoT, big data, and artificial intelligence as tools to obtain better health outcomes for the communities and improved performance for healthcare organization. The new generation of machine-learning algorithms can use standardized data sets generated by these sources to improve the effectiveness of public health interventions [ 50 ]. To this end, as pointed out by numerous studies in the field of BDA applied on healthcare organizations, it becomes crucial for the next future research to concentrate R&D efforts towards full standardized dataset protocols.
As highlighted by the results, in Europe, as well as in the rest of the world, a significant trend is emerging among healthcare organizations in adopting BDA-based management systems [ 45 ]. Among the clustering process performed, the common element in the studies reviewed is the positive relationship between BDA tools and achievable benefits for healthcare organizations.
As emerged by the RAs, some studies explore business value for healthcare organizations and the concept of potentialities of BDA (RA1) to explain the evidence of precise path-to-value chains leading to specific benefits [ 16 ]. These perspectives provide useful guidelines for healthcare managers who want to consider implementing BDA tools in their organizations. Some authors in particular focus on the role of BDA capabilities in the development of hospital supply chain integration and operational flexibility, demonstrating a positive relationship between the two dimensions [ 27 ]. During the Covid-19 outbreak, it became clearer how important operational flexibility is to healthcare organizations. The scholars also underline how BDA can impact to the efficiency of the decision-making processes in healthcare organizations, through predictive models and real-time analytics, helping health professionals in the collection, management, and analysis [ 37 ].
In general, BDA-based management systems make personalized care programs possible. However, considering the enormous amount and heterogeneity of information available nowadays, it emerges the necessity to address R&D pathways towards data filtering mechanisms and engineering new intelligent decision support systems within the healthcare organizations [ 38 ].
Circular economy (CE) and sustainability concepts are becoming important key drivers in healthcare organizations to reduce negative impact on the environment (RA2). Some study directions look at BDA as tool to provide solution for barriers related to CE and support sustainable development initiatives in the healthcare organizations [ 39 ]. Empirical studies have demonstrated the benefits of BDA-AI in the supply chain integration process and its impact on environmental performance. By assessing a sample of 168 French hospitals, Benzidia et al. [ 40 ], has observed that the use of BDA-AI technologies has a significant impact on environmental process integration and green supply chain. In particular, this study provides important insights for healthcare managers, who wish to implement BDA-AI technologies for sustaining green supply processes and improving environmental performance [ 40 ]. BDA and web technologies can successfully help managers to redesign healthcare processes making them more effective and efficient. Since healthcare spending is constantly growing in the world’s major regions, there is urgent need to redesign processes optimizing supply chain activities such that high-quality services could be provided at lower costs [ 42 ]. Although BDA-based management systems promise to fulfil this role in the healthcare organization, more in-depth studies are required. Due to heterogeneity of information sources, one of future research direction should deeply investigate the protocol standardization and integration in data analyzing as well as techniques and technologies used, security algorithms of BDA in the healthcare and medical data [ 43 ].
In developing countries, as well as in the rest of the world, the management of health surveillance is a sensitive issue (RA3). Therefore, authors have studied main key factors that hind BDA access in the healthcare organization [ 44 ]. Technology, staff, data management and health policies have been identified as some of decisive variables [ 44 ]. Due to increasing of the ageing population and the related disability, healthcare organizations will face hard challenges soon. To this end, big data can also help healthcare managers to detect patterns and to turn high volumes of data into usable knowledges. In this context investments in technological infrastructures are needed as well as in the human capital [ 45 ]. China is proving, with a large scale of investment, to be a pioneer country in the adoption of BDA-based management systems in the healthcare organization [ 46 ].
The rising of AI, IoT, machine learning [ 49 , 50 , 51 ], and sensors technology, as well as embedded systems able to communicate each other, have boosted the adoption of BDA with valuable benefits for the healthcare organization (RA4). These technologies will play a fundamental role on big data management to improve the performances of the healthcare organizations. Some authors have underlined privacy issues related to healthcare data and the necessity to make sensor data homogeneous and tagged. Furthermore, implementation of clinical records into models and adaptation of machine-learning techniques is required [ 47 ]. Future R&D in this field should be focused on the developing of digital platforms and specific applications based on BDA also for managing diagnostic images [ 48 ].
By exploring the relationship between BDA-based management systems and the benefits delivered to the healthcare organizations, this study replies to 3 RQs: 1) What is the state of art of BDA adopted by healthcare organizations, 2) What are the benefits for both health managers and healthcare organizations and 3) What are the future directions on BDA research in healthcare.
To answer the RQs the SLR has started from an investigation on the recent literature BDA about the BDA in healthcare organizations. Descriptive analysis has been performed on a sample of 34 studies coming from all over the world. The second stage shows a detailed content analysis on 16 studies which better answer to research question about the relationship between benefits for the healthcare organization and BDA solutions.
By analyzing the successful BDA strategies in healthcare context, some authors focus their attention on the BDA potentialities applied in the healthcare organizations [ 16 , 37 ]. Indeed, the research highlights how analytical tools through personal health systems support public health management systems and how BDA suggests new pathways to support healthcare managers in decision-making process.
In the literature, other scholars highlight the positive impact of BDA on resource management. The BDA solutions are analyzed as tools to sustain CE initiatives [ 38 , 39 ] as well as to enable green supply chain process integration and improve hospital performance [ 40 ]. By exploiting KPIs coming from BDA solutions, some researchers present innovative models for planning public health policy [ 41 ]. In this context, the studies consider BDA cloud computing solutions and social media data analytics for supporting the performance of healthcare supply chain management [ 42 , 43 ]. Furthermore, researchers from all around the world are showing particular interest on BDA for health surveillance system management [ 44 , 45 , 46 ].
According to the recent literature, BDA is transforming the healthcare organizations. The SLR has showed how the BDA solutions are now quite considered a milestone for managerial studies applied to healthcare organizations. The Coronavirus pandemic has been a good test run for using BDA to design healthcare policy strategies. Although an extensive literature on BDA to support healthcare management is being produced, the classification into four RAs proposed is an attempt to examine precise key research directions. About that, the limitations of the present research can be detected as the difficulty to review a field of literature constantly evolving. To date, the amount of data is no longer an issue. To be useful in the healthcare context, is necessary to validate their quality and then find the right correlations. In other words, the data should be processed, analyzed, and interpreted correctly. For this reason, emerges the need to address research pathways towards filtering mechanisms, by converting data from big to smart, and engineering new decision support systems within the healthcare organizations [ 38 ].
The content analysis carried out in this research has shown that studies are addressed to find out new models for both predictive and personalized medicine by exploiting BDA technologies [ 47 ]. The researchers underline the added value of using BDA both in the medical diagnostic process [ 48 ] and jointly with IT technologies such as IOT and machine learning [ 49 , 51 ].
Thus, considering the results obtained, it is possible to state that BDA can effectively help healthcare managers to detect common patterns and turn high volumes of data into usable knowledges. Investments on human capital become a priority to exploit the potential of BDA [ 45 ].
To achieve these objectives the future research should provide usable insights and standardized procedures for training healthcare managers and practitioners. AI, machines learning, as well as management strategies, will also play their part as knowledge producers in the healthcare organization. Privacy issues related to healthcare data and also the necessity to make sensor data homogeneous, are becoming crucial research topics to be faced. Finally, due to the heterogeneity of information sources, the future direction of research should investigate the standardization and integration of the protocol in data analysis, as well as the techniques useful for the managerial sector to implement increasingly BDA-based management systems in future healthcare organizations [ 43 ].
Nowadays the challenge for healthcare organizations is the development of useful applications BDA-based. According with the circular economy view, the future research directions should be addressed considering the relationship between digitalization and management resources consumption. The data centralization combined with a BDA approach can effectively support circular economy processes in healthcare supply chain by reducing waste and resource consumptions.
Exploiting the BDA’s capabilities will also be a key factor in forecasting and monitoring outbreaks. Future studies will need to focus on developing more efficient models for sharing data in order to improve the performance of healthcare organizations around the world.
Availability of data and materials
The datasets analyzed during the current study are not publicly available due to data relating to scientific journal names and authors but are available from the corresponding author on reasonable request.
Wang L, Alexander CA. Big data in medical applications and health care. Curr Res Med. 2015;6:1–8.
Article Google Scholar
Aceto G, Persico V, Pescape A. Industry 4.0 and health: internet of things, big data, and cloud computing for healthcare 4.0. J Ind Inf Integr. 2020;18:100129.
Google Scholar
Galetsi P, Katsaliaki K, Kumar S. Values, challenges and future directions of big data analytics in healthcare: A systematic review. Soc Sci Med. 2019;241:112533.
Article CAS PubMed Google Scholar
Obermeyer Z, Emanuel EJ. Predicting the future — big data, machine learning, and clinical medicine. New Engl J Med. 2016;375:1216–9.
Article PubMed Google Scholar
Kumar Y, Sood K, Kaul S, Vasuja R, et al. Big data analytics and its benefits in healthcare. In: Kulkarni J, et al., editors. Big data analytics in healthcare, studies in big data 66. Cham: Springer; 2020. p. 3–21.
Raghupati W, Raghupathi V. Big data analytics in healthcare: promise and potential. Health Inf Sci Syst Vol. 2014;2(1):1–10.
Jain DA, Kumar V, Khanduja D, Sharma K, Bateja R. A detailed study of big data in healthcare: case study of Brenda and IBM Watson. Int J Recent Technol Eng. 2019;7:8–12.
Tremolada, L. (2019), “Quanti dati sono generati in un giorno?” Il Sole24Ore , May 26, 2019, available at: https://www.infodata.ilsole24ore.com/2019/05/14/quanti-dati-sono-generati-in-un-giorno/?refresh_ce=1 (Accessed 17 Feb 2022).
Srivastava P.K., Rakshit P. Cutting edge IoT Technology for Smart Indian Pharma. In: International Conference on Advance Computing and Innovative Technologies in Engineering, (ICACITE) 2021. Greater Noida: Institute of Electrical and Electronics Engineers Inc.; 2021. p. 360–2.
Rayan R.A, Tsagkaris C, Zafar I. IoT for better mobile health applications. In: Kumar P, editor. A fusion of artificial intelligence and internet of things for emerging cyber systemsand internet of things for emerging cyber systems. Cham: Springer; 2022. p. 1–13.
Chung K, Park RC. Chatbot-based healthcare service with a knowledge base for cloud computing. Cluster Comput. 2019;22:1925–37.
Ali F, El-Sappagh S, Islam SMR, Ali A, Attique M, Imran M, Kwak KS. An intelligent healthcare monitoring framework using wearable sensors and social networking data. Fut Generation Comput Syst. 2021;114:23–43.
Yousefi S, Derakhshan F, Karimipour H. Applications of big data analytics and machine learning in the internet of things. In: Choo KK, Dehghantanha A, editors. Handbook of big data privacy. Cham: Springer; 2020. p. 77–108.
Chapter Google Scholar
Mehta N, Pandit A, Kulkarni M. Elements of healthcare big data analytics. In: Big data analytics in healthcare, studies in big data 66. Cham: Springer; 2018.
Han Y, Lie RK, Guo R. The internet hospital as a telehealth model in China: systematic search and content analysis. J Med Int Res. 2020;22:e17995.
Wang Y Hajli, N.,. Exploring the path to big data analytics success in healthcare. J Bus Res. 2017;70:287–99.
Srinivasan R, Swink M. An investigation of visibility and flexibility as complements to supply chain analytics: an organizational information processing theory perspective. Prod Oper Manage. 2018;27:1849–67.
Wang Y, Byrd TA. Business analytics-enabled decision-making effectiveness through knowledge absorptive capacity in health care. J Knowl Manage. 2017;21:517–39.
Wang Y, Kung LA, Byrd TA. Big data analytics: Understanding its capabilities and potential benefits for healthcare organizations. Technol Forecast Soc Change. 2018;126:3–13.
Jagadish HV, Gehrke J, Labrinidis A, Papakonstantinou Y, Patel JM, Ramakrishnan R, Shahabi C. Big data and its technical challenges. Commun ACM. 2014;57:86–94.
Seddon PB, Constantinidis D, Dod H. How does business analytics contribute to business value? In: Information Systems Journal, Proceeding of Thirty Third International Conference on Information Systems. Orlando: Wiley Publishing Ltd; 2012. p. 237–69.
Cao G, Duan Y, Li G. Linking business analytics to decision making effectiveness: a path model analysis. IEEE Trans Eng Manage. 2015;62:384–95.
Watson HJ. Tutorial: big data analytics: concepts, technologies, and applications. Commun Assoc Inf Syst. 2014;34:1247–68.
Negash S. Business intelligence. Commun Assoc Inf Syst. 2004;13:177–95.
Hurwitz J, Nugent A, Hapler F, Kaufman M. Big data for dummies. Hoboken: Wiley; 2013.
Sadeghi P, Benyoucef M, Kuziemsky CE. A mashup-based framework for multimulti-level healthcare interoperability. Inf Syst Front. 2012;14:57–72.
Yu W, Zhao G, Liu Q, Song Y. Role of big data analytics capability in developing integrated hospital supply chains and operational flexibility: An organizational information processing theory perspective. Technol Forecast Soc Change. 2021;163:120417.
Butler TW, Leong GK, Everett LN. The operations management role in hospital strategic planning. J Oper Manag. 1996;14:137–56.
Slack N, Brandon-Jones A, Johnston R. Operations management. 8th ed. Harlow: Pearson; 2016.
Liu, J., (2020), “Deployment of health IT in China’s fight against the COVID-19 pandemic”, available at: https://www.itnonline.com/article/deployment-health-it-china%E2%80%99s-fight-against-covid-19-pandemic (Accessed 20 Dec 2021).
Ting DS, Wei LC, Dzau V, Wong TY. Digital technology and COVID-19. Nat Med. 2020;26:459–61.
Article CAS PubMed PubMed Central Google Scholar
Rajasekera J, Mishal A.V., Mori Y, et al. Innovative mHealth solution for reliable patient data empowering rural healthcare in developing countries. In: Kulkarni A, et al., editors. Big data analytics in healthcare. Studies in big data, vol 66,. Cham: Springer; 2020. p. 83–103.
Ambert, K., Beaune, S., Chaibi, A., Briard, L., Bhattacharjee, A., Bharadwaj, V., Sumanth, K., Crowe, K. (2016), “French Hospital Uses Trusted Analytics Platform to Predict Emergency Department Visits and Hospital Admissions”, available at: https://www.intel.com/content/dam/www/public/us/en/documents/white-papers/french-hospital-analytics-predict-admissions-paper.pdf , (Accessed 13 Mar 2022).
Van Sickle D, Barrett M, Humblet O, Henderson K, Hogg C. Randomized, controlled study of the impact of a mobile health tool on asthma SABA use, control and adherence. Eur Respir J . 2016;48(Suppl. 60):1018.
Merchant R, Szefler SJ, Bender BG, Tuffli M, Barrett MA, Gondalia R, Kaye L, Van Sickle D, Stempel DA. Impact of a digital health intervention on asthma resource utilization. World Allergy Org J. 2018;411:28.
Khanra S, Dhir A, Islam N, Mäntymäki M. Big data analytics in healthcare: a systematic literature review. Enterprise Inf Syst. 2020;14:878–912.
Sousa MJ, Pesqueira AM, Lemos C, Sousa M, Rocha Á. Decision-making based on big data analytics for people management in healthcare organizations. J Med Syst. 2019;43:290.
Maglaveras N, Kilintzis V, Koutkias V, Chouvarda I. Integrated care and connected health approaches leveraging personalised health through big data analytics. Stud Health Technol Inf. 2016;224:117–22.
Kazançoğlu Y, Sağnak M, Lafcı Ç, Luthra S, Kumar A, Taçoğlu C. Big Data-enabled solutions framework to overcoming the barriers to circular economy initiatives in healthcare sector. Int J Environ Res Public Health. 2021;18:7513.
Article PubMed PubMed Central Google Scholar
Benzidia S, Makaoui N, Bentahar O. The impact of big data analytics and artificial intelligence on green supply chain process integration and hospital environmental performance. Technol Forecast Soc Change. 2021;165:120557.
Moutselos K, Maglogiannis I. Evidence-based public health policy models development and evaluation using big data analytics and web technologies. Med Arch (Sarajevo, Bosnia and Herzegovina). 2020;74:47–53.
Alotaibi S, Mehmood R, Katib I, Chlamtac I. The role of big data and twitter data analytics in healthcare supply chain management. In: Mehmood R, See S, Katib I, editors. Smart infrastructure and applications. Cham: EAI/Springer Innovations in Communication and Computing, Springer; 2020. p. 267–79.
Kundella S, Gobinath R. A survey on big data analytics in medical and healthcare using cloud computing. Int J Sci Technol Res. 2019;8:1061–5.
Chellah RC, Kunda D. An assessment of factors that affect the implementation of big data analytics in the Zambian health sector for strategic planning and predictive analysis: a case of Copperbelt province. Int J Electron Healthc. 2020;11:101–22.
Pastorino R, De Vito C, Migliara G, Glocker K, Binenbaum I, Ricciardi W, Boccia S. Benefits and challenges of big data in healthcare: an overview of the European initiatives. Eur J Public Health. 2019;29:23–7.
Gunapal PPG, Kannapiran P, Teow KL, Zhu Z, You AX, Saxena N, Singh V, Tham L, Choo PWJ, Chong P-N, Sim JHJ, Wong JEL. Setting up a regional health system database for seamless population health management in Singapore. Proc Singapore Healthc. 2016;25:27–34.
Clim A, Zota RD, Tinica G. Big data in home healthcare: A new frontier in personalized medicine. Medical emergency services and prediction of hypertension risks. Int J Healthc Manage. 2019;12:241–9.
Aiello M, Cavaliere C, D’Albore A, Salvatore M. The challenges of diagnostic imaging in the era of big data. J Clin Med. 2019;8:316.
Article PubMed Central Google Scholar
Bharathi MJ, Rajavarman VN. A survey on big data management in health care using IOT. Int J Recent Technol Eng. 2019;7:196–8.
Lai A, Rossignoli F, Stacchezzini R. How integrated reporting meets the investors and other stakeholders’information needs . (In Vrontis D., Weber Y., Tsoukatos E.) Global and national business theories and practice: bridging the past with the future. Cyprus: EuroMed Press; 2017.
Martinez F.E.L, Núñez-Valdez E.R, et al. Big data and machine learning: a way to improve outcomes in population health management. In: González García C, et al., editors. Protocols and applications for the industrial internet of things. Hershey: IGI Global; 2018. p. 225–39.
Download references
Acknowledgements
Not applicable.
The research was carried out without funding.
Author information
Authors and affiliations.
Department of Economics, University of Foggia, Via Caggese n.1, Foggia, Italy
Nicola Cozzoli, Fiorella Pia Salvatore, Nicola Faccilongo & Michele Milone
You can also search for this author in PubMed Google Scholar
Contributions
NC and FPS designed and conducted the empirical study, wrote and revised the manuscript. NC and FPS carried out the analysis and wrote the results, discussion and conclusions. NC, FPS, NF, and MM revised the manuscript. All authors read the manuscript and approved the final version.
Corresponding author
Correspondence to Fiorella Pia Salvatore .
Ethics declarations
Ethics approval and consent to participate, consent for publication, competing interests.
The authors declare that they have no competing interests.
Additional information
Publisher's note.
Springer Nature remains neutral with regard to jurisdictional claims in published maps and institutional affiliations.
Supplementary Information
Additional file 1..
List of articles.
Rights and permissions
Open Access This article is licensed under a Creative Commons Attribution 4.0 International License, which permits use, sharing, adaptation, distribution and reproduction in any medium or format, as long as you give appropriate credit to the original author(s) and the source, provide a link to the Creative Commons licence, and indicate if changes were made. The images or other third party material in this article are included in the article's Creative Commons licence, unless indicated otherwise in a credit line to the material. If material is not included in the article's Creative Commons licence and your intended use is not permitted by statutory regulation or exceeds the permitted use, you will need to obtain permission directly from the copyright holder. To view a copy of this licence, visit http://creativecommons.org/licenses/by/4.0/ . The Creative Commons Public Domain Dedication waiver ( http://creativecommons.org/publicdomain/zero/1.0/ ) applies to the data made available in this article, unless otherwise stated in a credit line to the data.
Reprints and permissions
About this article
Cite this article.
Cozzoli, N., Salvatore, F.P., Faccilongo, N. et al. How can big data analytics be used for healthcare organization management? Literary framework and future research from a systematic review. BMC Health Serv Res 22 , 809 (2022). https://doi.org/10.1186/s12913-022-08167-z
Download citation
Received : 02 March 2022
Accepted : 06 June 2022
Published : 22 June 2022
DOI : https://doi.org/10.1186/s12913-022-08167-z
Share this article
Anyone you share the following link with will be able to read this content:
Sorry, a shareable link is not currently available for this article.
Provided by the Springer Nature SharedIt content-sharing initiative
- Healthcare management
- Healthcare organization
- Healthcare governance
- Big data analytics
BMC Health Services Research
ISSN: 1472-6963
- General enquiries: [email protected]
Thank you for visiting nature.com. You are using a browser version with limited support for CSS. To obtain the best experience, we recommend you use a more up to date browser (or turn off compatibility mode in Internet Explorer). In the meantime, to ensure continued support, we are displaying the site without styles and JavaScript.
- View all journals
- My Account Login
- Explore content
- About the journal
- Publish with us
- Sign up for alerts
- Open access
- Published: 26 December 2022
Systematic analysis of healthcare big data analytics for efficient care and disease diagnosing
- Sulaiman Khan 1 ,
- Habib Ullah Khan 1 &
- Shah Nazir 2
Scientific Reports volume 12 , Article number: 22377 ( 2022 ) Cite this article
6526 Accesses
11 Citations
7 Altmetric
Metrics details
- Biotechnology
- Computational biology and bioinformatics
Big data has revolutionized the world by providing tremendous opportunities for a variety of applications. It contains a gigantic amount of data, especially a plethora of data types that has been significantly useful in diverse research domains. In healthcare domain, the researchers use computational devices to extract enriched relevant information from this data and develop smart applications to solve real-life problems in a timely fashion. Electronic health (eHealth) and mobile health (mHealth) facilities alongwith the availability of new computational models have enabled the doctors and researchers to extract relevant information and visualize the healthcare big data in a new spectrum. Digital transformation of healthcare systems by using of information system, medical technology, handheld and smart wearable devices has posed many challenges to researchers and caretakers in the form of storage, minimizing treatment cost, and processing time (to extract enriched information, and minimize error rates to make optimum decisions). In this research work, the existing literature is analysed and assessed, to identify gaps that result in affecting the overall performance of the available healthcare applications. Also, it aims to suggest enhanced solutions to address these gaps. In this comprehensive systematic research work, the existing literature reported during 2011 to 2021, is thoroughly analysed for identifying the efforts made to facilitate the doctors and practitioners for diagnosing diseases using healthcare big data analytics. A set of rresearch questions are formulated to analyse the relevant articles for identifying the key features and optimum management solutions, and laterally use these analyses to achieve effective outcomes. The results of this systematic mapping conclude that despite of hard efforts made in the domains of healthcare big data analytics, the newer hybrid machine learning based systems and cloud computing-based models should be adapted to reduce treatment cost, simulation time and achieve improved quality of care. This systematic mapping will also result in enhancing the capabilities of doctors, practitioners, researchers, and policymakers to use this study as evidence for future research.
Similar content being viewed by others
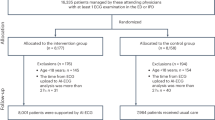
AI-enabled electrocardiography alert intervention and all-cause mortality: a pragmatic randomized clinical trial
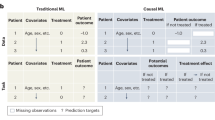
Causal machine learning for predicting treatment outcomes
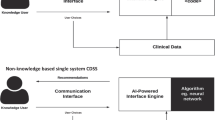
An overview of clinical decision support systems: benefits, risks, and strategies for success
Introduction.
Healthcare around the world is under high pressure due to limiting financial resources, over-population, and disease burden. In this modern technological age the healthcare paradigm is shifting from traditional, one-size-fits-all approach to a focus on personalized individual care 1 . Additionally, the healthcare data is varying both in type and amount. The healthcare providers are not only dealing with patient’s historical, physical and namely information, but they also deal with imaging information, labs, and other digital and analogue information consists of ECG, MRI etc. This data is voluminous, varying in type and formats, and of differing structure. These are the capabilities of Big Data to handle not only different types of and forms of data, but can handle 10 V structure including volume, variety, venue, varifocal, varmint, vocabulary, validity, volatility, veracity and velocity. Thus, the doctors facing an increasing burden of rising patient numbers coupled with progressively less time to spend with each patient. In other words, we are facing more patients, more data, and less time.
Big data has significantly attracted the researchers to explore different research fields including healthcare, banking, imaging, smart cities, internet of things (IoT) based smart applications, tracking and transportation system etc. 2 . Software engineers constantly develops new applications for patient’s health and well-being. Both government and non-government organizations develop infrastructure using big data analytics for improved decision making capabilities of both doctors and managers 3 . It was recorded that 80% increase in big data is due to cloud sources, big data analytics, mobile technology and social media technologies 4 . A number of research articles proposed using big data analytics in varying domains especially in healthcare such as Kumar et al. 5 proposed a cognitive technology-based healthcare evaluations system using big data analytics. Chen et al. 6 presented an intelligent healthcare application for brain hemorrhage detection using Big Data analytics and machine learning (ML) techniques. Smart health appointment system is developed by Liang and Zhao using big data analytics is 7 .
Some researchers explored big data analytics in healthcare domain in different ways. They presented survey papers and review papers to understand the meanings of big data analytics in healthcare such as Galetsi and Kasaliasi performed a review of healthcare big data analytics 8 while Lindell defined big data analytics in terms of accounting and business perspectives 9 . Alharthi proposed a review article on healthcare challenges facing in Saudi Arabia by performing analysis of the available literature 10 . Lee et al. 11 presented a survey paper to explore the applications and challenges of healthcare big data analytics. From the literature it is concluded that multiple new applications are developed for big data analysis. Review and survey papers are presented to outline the published literature, but most of these papers are region specific or limited to a few numbers of papers. On the other side systematic review process formulate multiple research question and identifies keywords to explore the available literature from different angles. Systematic analysis of the available literature is presented in many fields like PMIPv6 domain 12 , in smart homes 13 , navigation assistants 14 , and many others, but there is no significant work reported on systematic analysis for healthcare big data domain to find the gaps in the available literature and suggest future research directions.
The inspirational point that led us to pursue this systematic analysis was the pervasive and ubiquitous nature of big data. Efficient management and timely execution are the dire needs of big data, to extract enriched information regarding a certain problem of interest 15 . Many factors involved behind this systematic research work, but the most eminent reasons are:
The exiting research reported on big data does not provide significant information about the key features that should be considered to integrate both structured and unstructured big data in healthcare domain. The pervasiveness of big data features challenging the researchers in pursuing research in this specialized domain. The underlying research on finding the key features will not only help in integrating big data in healthcare domain, but it will also assist in findings new gateways for future research directions.
Digital transformation of healthcare systems after the integration of information system, medical technology and other imaging systems have posed a big barrier for the research community in the form of a vast amount of information to deal with. While the over-population, limited data access, and disease burdens have restricted the doctors and practitioners to check more patients in a limited time. So, finding a suitable model that can efficiently process healthcare big data to extract information for a certain disease symptoms will not only helps the practitioners to suggest accurate medication and check more patients in timely manners, but it will open future research directions for the industrialists and policymakers to develop optimal healthcare big data processing models.
Accurate disease diagnosing by processing of gigantic amount of data, especially a plethora of types of data, within an interested processing domain is a key concern for both researchers and practitioners. Developing an efficient model that can accurately diagnose a certain by classifying images or other historical details of patients will not only helps the doctors to diagnose disease in timely manner and suggest medicine accordingly, but it will encourage the researchers and developers to develop an accurate disease identification model.
The remaining research paper of the paper is organized as follows. Section 2 of the paper outlines the related work reported in the proposed field. Section 3 presents the research framework followed for this systematic research work. Quality assessment is detailed in Sect. 4 . Section 5 outlines the discussion on findings of the proposed systematic research work. Section 6 provides the limitations of this systematic study traced by the conclusion and future work in Sect. 7 of the paper.
Literature review
From the last few decades, we experienced an unprecedented transformation of traditional healthcare systems to digital and portable healthcare applications with the help of information systems, medical technology and other imaging resources 16 . Big data are radically changing the healthcare system by encouraging the healthcare organizations to embrace extraction of relevant information from imaginary data and other clinical records. This information will produce high throughput in terms of accurate disease diagnosing, plummeting treatment cost increase availability. In data visualization context the term ‘big data’, is firstly introduced in 1997 17 , posed an ambitious and exceptional challenge for both policy-makers and doctors with special emphasis on personalized medicine. Nonetheless, data gathering moves faster than both data analysis and data processing, emphasizing the widening gap between the rapid technological progress in data acquisition and the comparatively slow functional characterization of healthcare information. In this regard, the historical information (phonotypical and other genomic information) of an individual patient form electronic health records (EHR) are becoming of critical importance. Figure 1 represents the primary sources of big data.
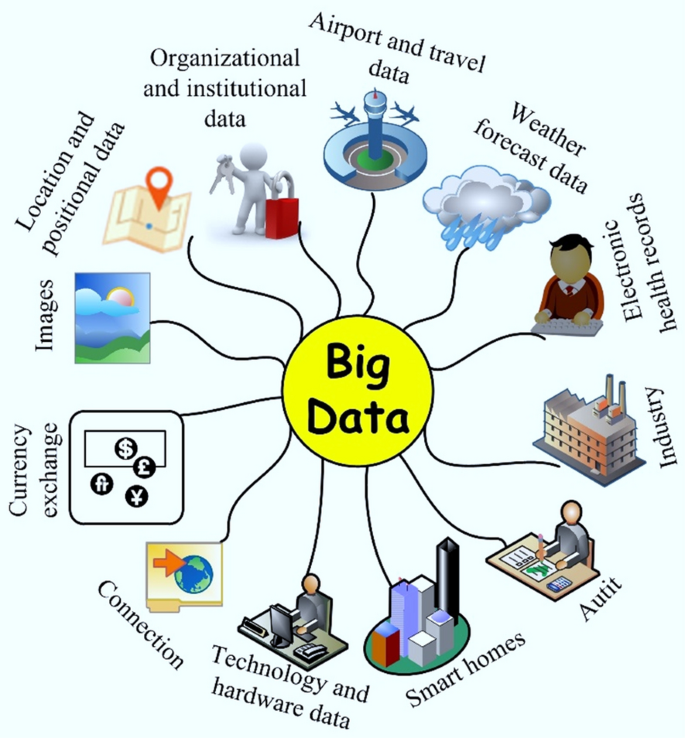
Main steps of the research protocol.
Significant research work has been reported in the domains of healthcare big data analytics. To process this vast amount of information in timely manner and identify someone’s health condition based on his her is more difficult. Researchers proposed numerous applications to address this problem such as; Syed et al. 18 proposed a machine learning-based healthcare system for providing remote healthcare services to both diseased and healthy population using big data analytics and IoT devices. Venkatesh et al. 19 developed heart disease prediction model using big data analytics and Naïve Bayes classification technique. Kaur et al. 20 suggested a machine learning (ML) based healthcare application for disease diagnosing and data privacy restrictions. This model works by considering different aspects like activity monitoring, granular access control and mask encryption. Some researchers presented review and survey papers to outline the recent published work in a specific directions such as Patel and Gandhi reviewed the literature for identifying the machine learning approaches proposed for healthcare big data analytics 21 . Rumbold et al. 22 reviewed the literature for find the research work reported for diabetic diagnosing using big data analytics.
From the above discussions, it is worth mentioning that most of the researchers and industrialists gave significant attention towards the development of new computational models or surveyed the literature in a specific research direction (heart disease detection, diabetes detection, storage and security analysis etc.), but no significant research work is reported to systematically analyze the literature with different perspectives. To address this problem, this research work presents a systematic literature review (SLR) work to analyze the literature reported in healthcare big data analytics domain. This systematic analysis will not only find the gaps in the available literature but it will also suggest new directions of future research to explore.
Research framework
Systematic literature reviews and meta-analysis has gained significant attention and became increasingly important in healthcare domain. Clinicians, developers and researchers follow SLR studies to get updated about new knowledge reported in their fields 23 , 24 , and they are often followed as a starting point for preparing basic records. Granting agencies mostly requires SLR studies to ensure justification of further research 25 , and even some healthcare journals follows this direction 26 . Keeping these SLR applications in mind the proposed systematic analysis is performed following the guidelines presented by Moher et al. 27 (PRISMA) and Kitchenham et al. 28 . This SLR work accumulates the most relevant research work from primary sources. These papers are then evaluated and analyzed to grab the best results for the selected research problem. Figure 2 represents the results after following the PRISMA guidelines. This systematic analysis are performed using the following preliminary steps:
Identification of research questions to systematically analyze the proposed field from different perspectives.
Selection of relevant keywords and queries to download the most relevant research articles.
Selection of peer-reviewed online databases to download relevant research articles published in healthcare big data domain during the period ranging from 2011 – 2021.
Perform inclusion and exclusion process based on title, abstract and the contents presented in the article to remove duplicate records.
Assess the finalized relevant articles for identifying gaps in the available literature and suggest new research directions to explore.
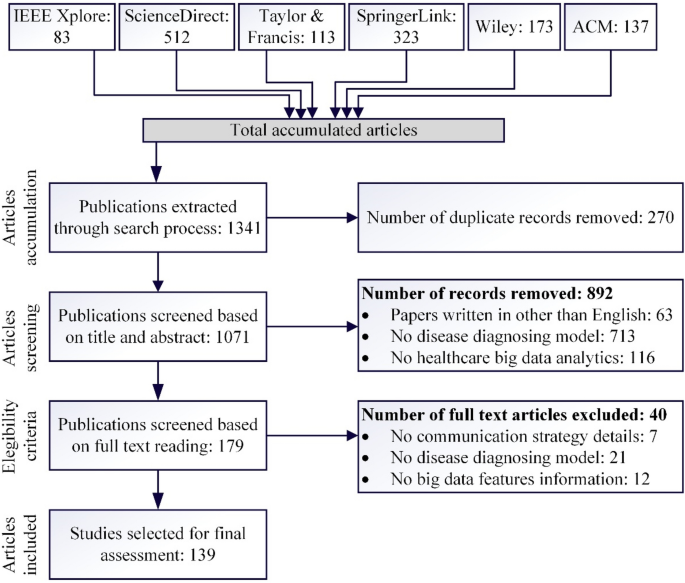
PRISMA process model for articles accumulation, screening, and final selection.
Research questions
Selecting a well-constructed research question(s) is essential for a successful review process. We formulate a set of five research questions based on the Goal Questions Metrics approach proposed by Van Solingen et al. 29 . The formulated research questions are depicted in Table 1 below.
Search strategy
Search strategy is the key step in any systematic research work because this is the step that ensures the most relevant article for the analysis and the assessment process. To define a well-organized search strategy a search string is developed using the formulated relevant keywords. For the accumulation of most relevant articles for a certain research problem, only keywords are not sufficient. These keywords are concatenated in different strings for searching articles in multiple online repositories 30 . Inspired from the SLR work of Achimugu et al. 31 , in software requirement domain, our search strategy consists of four main steps includes identification of keywords relevant to selected research problem, formulation of search string based on the keywords, and selection of online repositories to accumulate relevant articles to the problem selected.
Selection of keywords
List of keywords are defined for each research question to download all relevant articles. Some researchers defined a generic query 32 and starts downloading articles. Although it is simple for the accumulation of articles from online database but mostly it tends to skip some most relevant articles. So, the correct option is to define keywords for each research question. In fact, it is a hectic job, but it ensures the retrieval of each relevant article from online databases regarding a certain research problem.
Formulation of search string
Search strings (queries) are formulated using the keywords identified from the selected research questions. The search string is tested in online databases and was modified according to retrieve each relevant articles from these databases. Inspired from the guidelines proposed by Wohlin 33 , following are the key steps undertaken to develop an optimal search string:
Identification of key terms from the formulated topic and research questions
Selection of alternate words or synonyms for key terms
Use “OR” operator for alternating words or synonyms during query formation
Link all major terms with Boolean “AND” operator to validate every single keyword.
Following all these preliminary steps a generic query/search-string is developed that is depicted in Table 2 . This generic query is further refined for each research question as depicted in Table 3 to retrieve each relevant article.
Selection of online repositories
After identifying keywords and formulating search strings the next step is to download relevant articles specific to the interested research problem. For the accumulation of relevant articles six well-known and peer-reviewed online repositories are selected, as depicted in Table 3 .
Articles accumulation and final database development
For relevant articles accumulation and final database development we followed the guidelines suggested by Kable et al. 34 . After specifying the research questions, identifying keywords, and formulating search queries, and selecting online repositories, the next key step is to develop a relevant articles database for the analysis and assessment purposes that includes three prime steps: (1) identification of inclusion/exclusion criteria for a certain research article(s), and (2) Relevant articles database development. These steps are discussed in detail below.
Inclusion and exclusion criteria
After selecting online database and starts the articles downloading process, the most tedious task that the author (s) facing, is the decision about whether a certain paper should be included in the final database or not? To overcome this problem an inclusion and exclusion criteria is defined for the inclusion of a certain article in the final set of articles. Table 4 represents the inclusion and exclusion criteria followed for this systematic research work.
A manual process is followed by the authors for the inclusion and exclusion of a certain article. These articles are evaluated based on title, abstract and information provided in the overall paper. If more than half authors agree upon the inclusion of a certain article based on these parameters (title, abstract, and contents presented in the article), then that paper was counted in the final database otherwise rejected. A total of 134 relevant primary studies are selected for the final assessment process. To ensure no skip of relevant article snowballing is applied to retrieve each relevant article.
Snowballing To extract each relevant primary article snowballing is applied in the proposed research work 33 . In this systematic analysis both types of snowballing backward and forward snowballing is applied to ensure extraction of each relevant primary article. 145 relevant articles retrieved after applying snowballing process. These articles are then filtered by title and resulted for 53 relevant articles. After further processing by abstract resulted into 19 articles, and at last when filtered by contents presented in the paper resulted into only 5 relevant articles. This overall process is depicted in Fig. 3 . After adding these articles to the accumulated relevant articles, a total of 139 articles added to the final database.
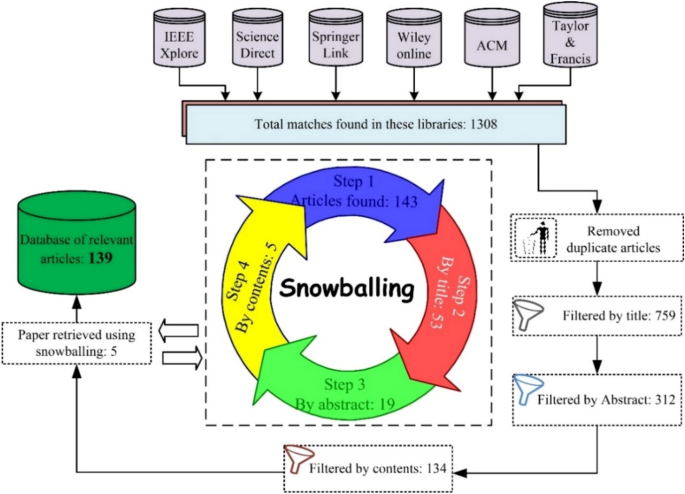
Extraction of each relevant article using snowballing.
Relevant articles database development
After accumulating each primary article reported in the proposed field, a database of relevant articles is developed for the assessment and analysis work, to find the current available trends in healthcare big data analytical domain and investigate the gaps in these research articles to open new gates for future research work. A total of 139 relevant articles are added to the final database. The overall contribution of the selected online repositories in the relevant articles database development is depicted in Fig. 4 .
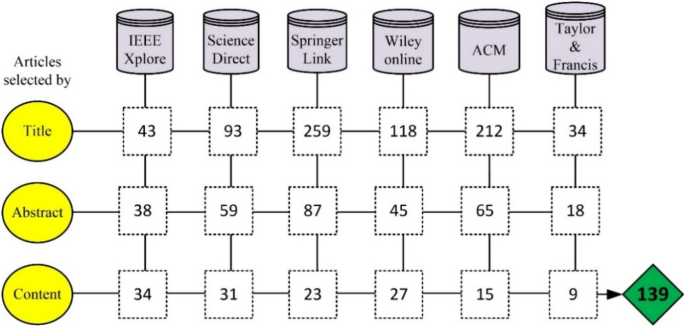
Distribution of primary studies.
From Fig. 4 , it is concluded that IEEE Xplore and Science Direct contributing the more that reflects the interest of research community to present their work with.
After developing a database of relevant articles, it is evaluated using different parameters like type of article (conference proceedings, journal article, book chapter etc.), publication year, and contribution of individual library. Figure 5 represents the information regarding the total contribution of articles by type in the final database.
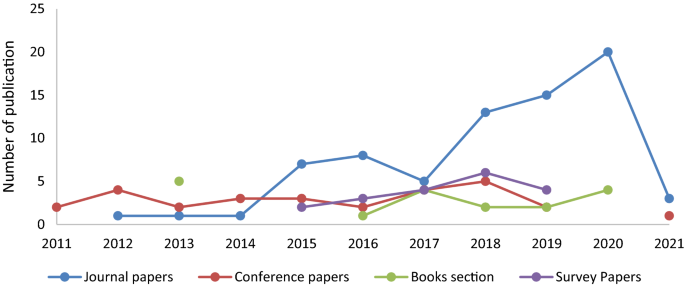
Evolution of final database by type of article and year.
Figure 5 concludes that the researchers paid significant attention towards the development of new healthcare systems instead of finding the gaps in the available systems and develop enhanced solutions accordingly. This enhanced solution can accurately identify and diagnose a certain disease based on patient’s historical medical information. A small amount of work is reported using review articles, survey papers, but no systematic mechanism is followed to analyse the work in specific range of years followed by a set of research questions. The same problem can also be seen from Fig. 6 where highest percentage contribution is shown more comparative to book sections, conference papers etc.
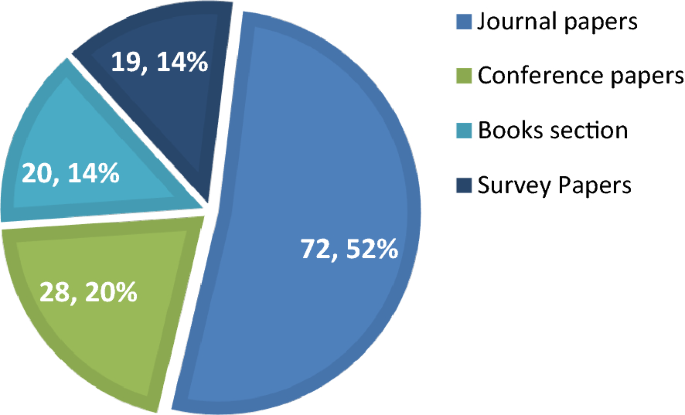
Percentage contribution by type of paper.
Figure 7 depicts the percentage contribution of each library in the proposed assessment work.
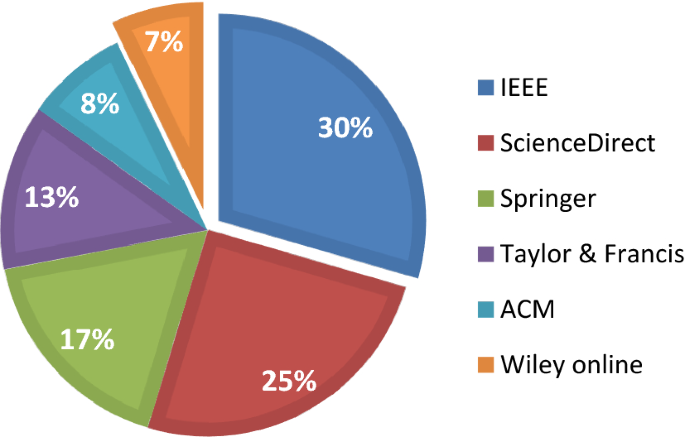
Percentage contribution of each library.
Figure 8 represents the annual distribution of articles selected for the analysis and assessment purposes. Form Fig. 8 it is evident, that with passage of time number of articles increases, and that shows the maturity and interest of the researchers in this specific domain.
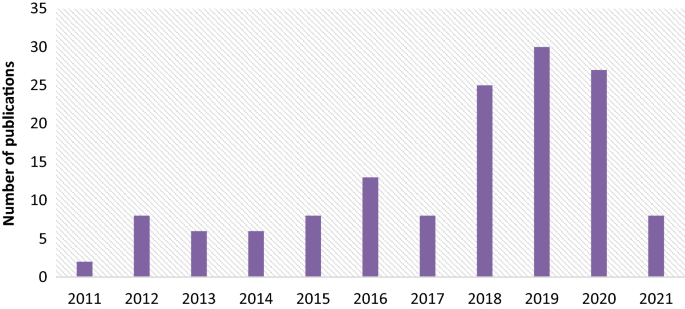
Annual distribution of articles.
From Fig. 8 , it is concluded that IEEE Xplore contributing the more in the final database of relevant articles that shows the trend of researchers to present healthcare relevant works in the IEEE journals. Figure 9 represents the total number of journal articles, survey papers, conference papers, and book sections in the selected relevant articles database.
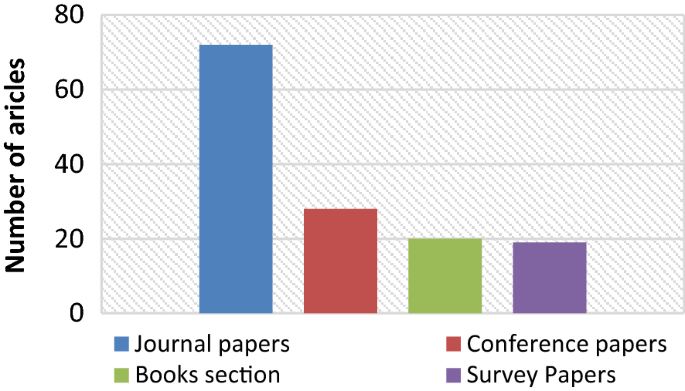
Evolution of database by number of articles by type.
From Fig. 9 it is concluded that significant attention is given towards the development of new healthcare models. This shows the maturity of the proposed field. Dealing with such a mature field and extracting useful information is hectic job for the researchers. A systematic analysis of this research field is needed to provide an overview of the work reported during a specific range of years. This analysis will not only save precious time of the researchers, but it will also open gates for the future research work in this field.
Table 5 represents the annual contribution of studies in the final relevant database.
Overall information regarding type of paper, publication year and number of records is depicted in Fig. 10 below.
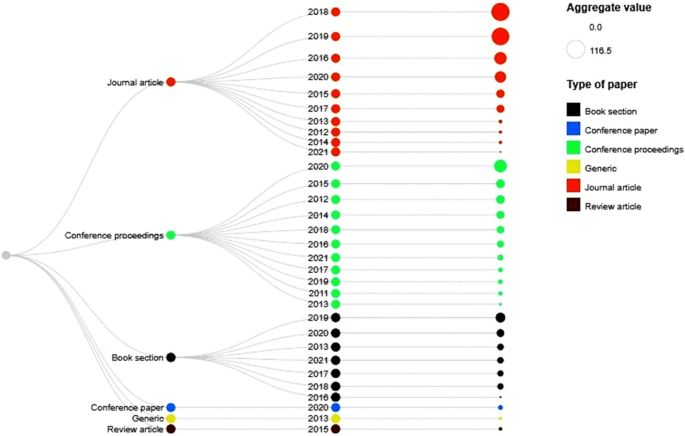
Evolution of final database.
Quality assesment
After executing exclusion and inclusion process, all the relevant articles in the database are manually assessed by authors to check the relevancy of each article with the selected research problem. A quality criterion is defined to check every research article against the formulated research questions. This quality criteria is defined in Table 6 .
Weighted values are assigned against each quality criteria to check the relevancy of an article with a certain research question. These weighted values and description is depicted in Fig. 11 .
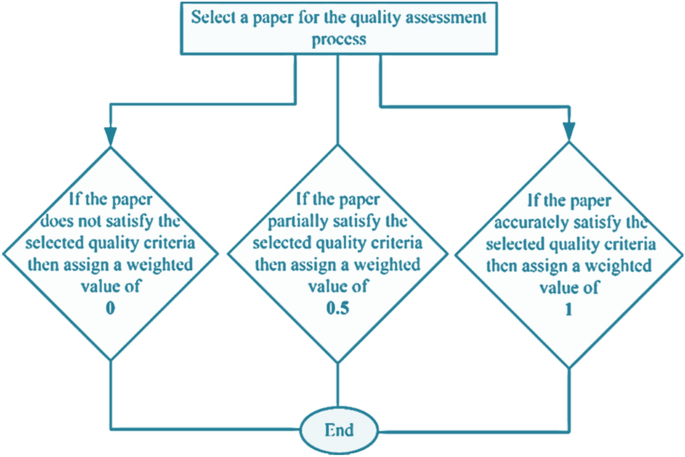
Quality criteria for the proposed SLR work.
After the assessment process, the relevancy of each article is decided based on its aggregated weighting score. If the score is greater than 3 it represents the most relevancy of an article to the selected research topic. Figure 12 represents the aggregate score values of each article based on the defined quality assessment criteria.
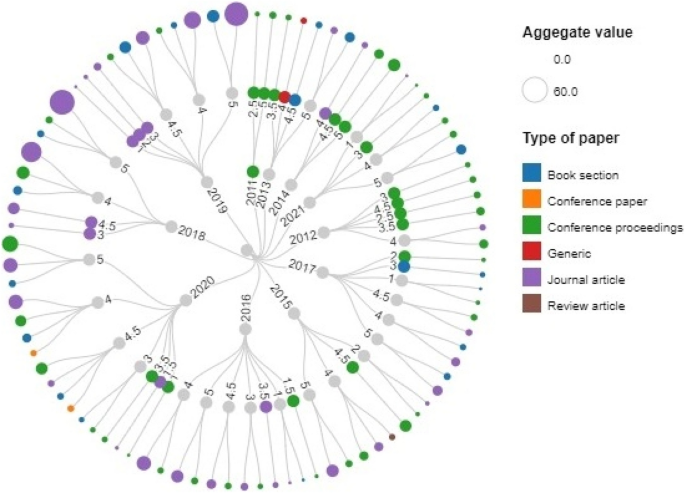
Quality assessment process.
Results and discussion
After executing the quality assessment work, the next key step of an SLR work is, to analyse all the relevant article to identify different techniques proposed for efficient communication between patient and practitioner, accurate feature extraction from healthcare big data and implement it in practical use.
This section of the paper performs a descriptive analysis of each article based on five research questions. In this systematic review process, a total of 139 research articles published during the period ranging from 2011 to 2021.
Healthcare big data
The researcher and data analysts suggested no contextual name for “big data” in healthcare, but for implementation and interpretation purposes they divided it into 5 V architecture. Figure 13 depicts a 5 V architecture of big data.
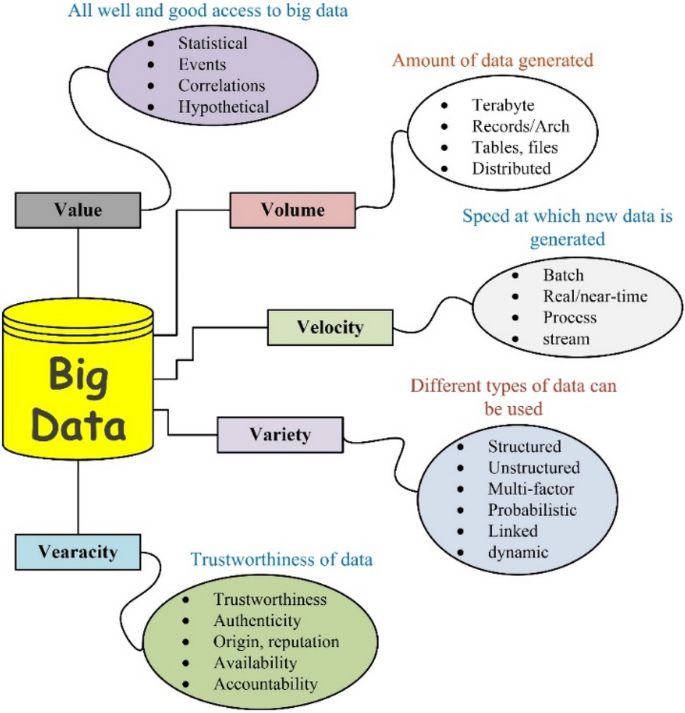
Big Data 5Vs 15 .
The exponential increase in IoT-based smart devices and information systems resulted a plethora of information in healthcare domain. This information increases exponentially on daily basis. These smart IoT based healthcare devices produces a huge of data. An alternated term “Big Data” is selected for this gigantic amount of data. This is the data for which scale, diversity, and complexities require innovative structure, variables, design, and analytics for efficient utilization and management, accurate data extraction and visualization, and to grab hidden stored information regarding a specific problem of interest. Main idea behind the implementation of healthcare big data analytics is to retrieve enriched information from huge amount of data using different machine leering and data mining techniques 191 . These techniques help in improving quality of care, reducing cost of care, and helps the practitioners to suggest medicines based on clinical historical information.
RQ1. What are the key features adapted to integrate the structured and unstructured data in healthcare big data domain?
Big data comprises a huge amount of data to be processed, especially a plethora of types of data to process and extract enriched information regarding a problem of interest. Several features are assessed and analyzed especially in healthcare domain, to integrate both structural and non-structural data. Multiple researchers analyzed semantic based big data features for big data integration purposes while some researchers proposed behavior and structural based features for patient monitoring and activity management purposes 151 , 192 . While some performed real-time analysis using a group of people for data integrating and clustering purposes. Table 7 enlists the research work published for the structural and non-structural data integration purposes.
After analysing the available literature in Table 8 , it was concluded that mostly semantic based, structure-based, and real-time activity-based features are considered for the information extraction and organization purposes. If we consider geometric based feature and adapt clustering mechanism for data organization purposes, then this will not only integrate both structural and non-structural data efficiently, but it will improve the simulation capabilities of different applications.
RQ2. What are different techniques proposed to provide an easy and timely data-access interface for doctors?
Digital transformation of healthcare systems by using of information system, medical technology, handheld and smart wearable devices has posed many challenges for both the researchers and caretakers in the form of storage, dropping the cost of care and processing time (to extract relevant information for refining quality of care and reduce waste and error rates). Prime goal of healthcare big data analytics is, to process this vast amount of data using machine learning and other processing models to extract certain problem relevant information and use it for human well beings 195 . Several supervised and unsupervised classification techniques are followed for the said purposes. ML-based architectures and big data analytical techniques are integrated in healthcare domain for efficient information retrieval and exchange purposes, risk analysis, optimum decision-support system in clinics, and suggesting precise medicines using genomic information 196 . Table 8 represent the literature reported for the providence of an easy and timely data-access interface for the practitioners.
RQ3. What are different ways to improve communication between the doctor and patient?
Healthcare around the world is under high pressure due to limiting financial resources, over-population, and disease burden. In this modern technological age, the healthcare paradigm is shifting from traditional, one-size-fits-all approach to a focus on personalized individual care 1 . Additionally, the healthcare data is varying both in type and amount. The healthcare providers are not only dealing with patient’s historical, physical and namely information, but they also deal with imaging information, labs, and other digital and analogue information consists of ECG, MRI etc. This data is voluminous, varying in type and formats, and of differing structure. These are the capabilities of Big Data to handle not only different types of and forms of data, but can handle 5 V structure including volume, variety, value, veracity, and velocity. Thus, the doctors facing an increasing burden of rising patient numbers coupled with progressively less time to spend with each patient. In other words, we are dealing with more patients, more data, and less time.
Different techniques are proposed in the literature to provide an easy and timely communication interface for both doctors and patients. Table 9 depicts different information exchange tools/techniques reported in the literature.
RQ4. What are different types of classification models proposed for accurate disease diagnosing using patient historical information?
This research question aims to outline different disease diagnosing models proposed in the literature using healthcare big data. Around the world diverse approaches are proposed by researchers for healthcare big data analysis to ensure accurate disease diagnosing capabilities, provide healthcare facilities at doorstep, development of eHealth and mHealth applications, and many others. Multiple statistical and ML-based approaches proposed for accurate diagnosing purposes. Figure 14 represents multiple techniques proposed for automatic disease diagnosing purposes using healthcare big data domain.
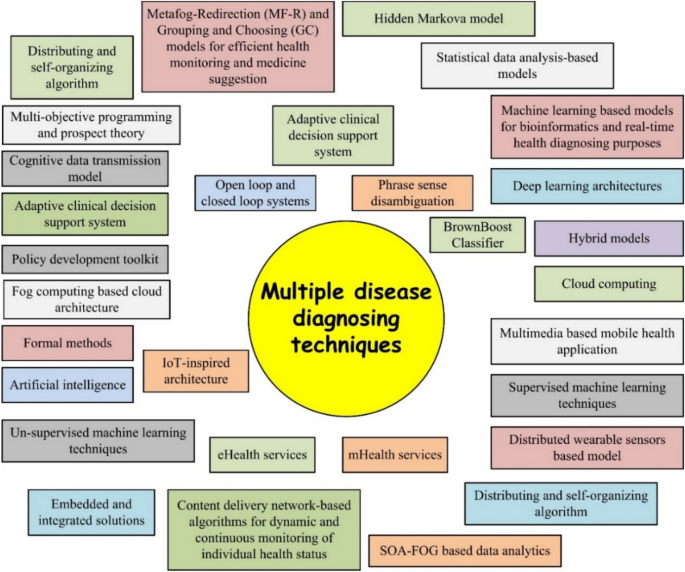
Multiple disease diagnosing techniques proposed in the literature.
All these techniques perform the diagnosing process using semantic-based features or structural based features. But no attention is given towards geometric feature extraction techniques that are prominent in extracting enriched information from data and results in high identification rates. Also, no advanced hybrid neural network and shallow architectures are proposed for the automatic diagnosing purposes. Keeping these gaps in mind, an optimum eHealth application can be developed by applying these hybrid techniques.
RQ5. What are different applications of big data analytics in healthcare domain?
Big data analytics has revolutionized our lives by presenting many state of the art applications in various domains ranging from eHealth to mHealth, weather forecasting to climate changes, traffic management to object detection, and many others. This research question mainly focusing on enlisting different applications of big data analytics in Table 10 .
Limitations
This article has a number of limitations. Some of these limitations are listed below.
For this systematic analysis articles are only accumulated from six different peer-reviewed libraries (ACM, SpringerLink, Taylor & Francis, Science Direct = IEEE Xplore, and Wiley online library), but there exist a number of multi-disciplinary databases for articles accumulation purposes.
This systematic analysis covers a specific range of years (2011 –2021), while a number of articles are reporting on daily basis.
Articles are accumulated from online libraries using search queries, so if a paper has no matching words to the query, then it was skipped during search process.
Google Scholar is skipped during the articles accumulation phase to shorten the searching time. Also, it gives access to both peer-reviewed and non-peer-reviewed journals and we only focused on peer-reviewed journals for the relevant articles.
Being a systematic literature work it can be broadened to grab the knowledge about other varying topics such as healthcare data commercialization, health sociology etc.
Besides these limitations we hope that this systematic research work will be an inspiration for future research in the recommended fields and will open gates for both industrialists and policymakers.
Conclusion and future work
In this research article, the existing research reported during 2011 to 2021 is thoroughly analysed for the efforts made by researchers to help caretakers and clinicians to make authentic decisions in disease diagnosing and suggest medicines accordingly. Based on the research problem and underlying requirements, the researchers proposed several feature extraction, identification, and remote communication frameworks to develop doctor and patient communication in a timely fashion. These real-time or nearer to real-time applications mostly use big data analytics and computational devices. This research work identified several key features and optimum management designs proposed in healthcare big data analytical domain to achieve effective outcomes in disease diagnosing. The results of this systematic work suggests that advanced hybrid machine learning-based models and cloud computing application should be adapted to reduce treatment cost, simulation time, and achieve improved quality of care. The findings of this research work will not only help the policymakers to encourage the researchers and practitioners to develop advanced disease diagnosing models, but it will also assist in presenting an improved quality of treatment mechanism for patients.
Advanced hybrid machine learning architectures for cognitive computing are considered as the future toolbox for the data-driven analysis of healthcare big data. Also, geometric-based features must be considered for feature extraction purposes instead of semantic and structural-based features. These geometric-based feature extraction techniques will not only reduce the simulation time, but it will also improve the identification and disease diagnosing capabilities of smart health devices. Additionally, these features can help in accurate identification of Alzheimer, tumours in PET or MRI images using upgraded machine learning and big data analytics. Cluster-based mechanism should be considered for data organization purposes to improve big data timely-access and easy-management capabilities. Promoting research in these areas will be crucial for future innovation in healthcare domain.
Data availability
The data used and/or analyzed during the current study available from the corresponding author on reasonable request.
Rahman, F. & Slepian, M. J. Application of big-data in healthcare analytics—Prospects and challenges. In 2016 IEEE-EMBS International Conference on Biomedical and Health Informatics (BHI) 13–16 (2016).
Khan, N. et al. Big data: Survey, technologies, opportunities, and challenges. Sci. World J. 2014 , 1–18 (2014).
Google Scholar
Groves, P., Kayyali, B., Knott, D. & Van Kuiken, S. The ‘big data ‘revolution in healthcare. In McKinsey Quarterly (2013).
Andreu-Perez, J., Poon, C. C., Merrifield, R. D., Wong, S. T. & Yang, G.-Z. Big data for health. IEEE J. Biomed. Health Inform. 19 , 1193–1208 (2015).
Article Google Scholar
Kumar, M. A., Vimala, R. & Britto, K. A. A cognitive technology based healthcare monitoring system and medical data transmission. Measurement 146 , 322–332 (2019).
Article ADS Google Scholar
Chen, H., Khan, S., Kou, B., Nazir, S., Liu, W. & Hussain, A. A smart machine learning model for the detection of brain hemorrhage diagnosis based internet of things in smart cities. Complexity 2020 (2020).
Liang, Y. & Zhao, L. Intelligent hospital appointment system based on health data bank. Procedia Comput. Sci. 159 , 1880–1889 (2019).
Galetsi, P. & Katsaliaki, K. A review of the literature on big data analytics in healthcare. J. Oper. Res. Soc. 1–19 (2019).
Lindell, J. What are big data and analytics?. In Analytics and Big Data for Accountants (2018).
Alharthi, H. Healthcare predictive analytics: An overview with a focus on Saudi Arabia. J. Infect. Public Health 11 , 749–756 (2018).
Lee, C. et al. "Big healthcare data analytics: Challenges and applications. In Handbook of Large-Scale Distributed Computing in Smart Healthcare 11–41 (Springer, 2017).
Chapter Google Scholar
Hussain, A., Nazir, S., Khan, S. & Ullah, A. Analysis of PMIPv6 extensions for identifying and assessing the efforts made for solving the issues in the PMIPv6 domain: A systematic review. Comput. Netw. 179 , 107366 (2020).
Khan, H.-U. et al. Systematic analysis of safety and security risks in smart homes. Comput. Mater. Contin. 68 , 1409–1428 (2021).
Khan, S., Nazir, S. & Khan, H.-U. Analysis of navigation assistants for blind and visually impaired people: A systematic review. IEEE Access 9 , 26712–26734 (2021).
Nazir, S. et al. A comprehensive analysis of healthcare big data management, analytics and scientific programming. IEEE Access 8 , 95714–95733 (2020).
Kitchin, R. Big Data, new epistemologies and paradigm shifts. Big Data Soc. 1 , 2053951714528481 (2014).
Cox, M. & Ellsworth, D. Application-controlled demand paging for out-of-core visualization. In Proceedings. Visualization’97 (Cat. No. 97CB36155) 235–244 (1997).
Syed, L., Jabeen, S., Manimala, S. & Elsayed, H. A. Data science algorithms and techniques for smart healthcare using IoT and big data analytics. In Smart Techniques for a Smarter Planet 211–241 (Springer, 2019).
Venkatesh, R., Balasubramanian, C. & Kaliappan, M. Development of big data predictive analytics model for disease prediction using machine learning technique. J. Med. Syst. 43 , 272 (2019).
Article CAS Google Scholar
Kaur, P., Sharma, M. & Mittal, M. Big data and machine learning based secure healthcare framework. Procedia Comput. Sci. 132 , 1049–1059 (2018).
Patel, H. B. & Gandhi, S. A review on big data analytics in healthcare using machine learning approaches. In 2018 2nd International Conference on Trends in Electronics and Informatics (ICOEI) 84–90 (2018).
Rumbold, J. M. M., O’Kane, M., Philip, N. & Pierscionek, B. K. Big Data and diabetes: The applications of Big Data for diabetes care now and in the future. Diabetic Med. (2019).
Oxman, A. D. et al. Users’ guides to the medical literature: VI. How to use an overview. JAMA 272 , 1367–1371 (1994).
Swingler, G. H., Volmink, J. & Ioannidis, J. P. Number of published systematic reviews and global burden of disease: database analysis. BMJ 327 , 1083–1084 (2003).
Research, C. I. O. H. Randomized controlled trials registration/application checklist (12/2006). Available at: http://www.cihr-irsc.gc.ca/e/documents/rct_reg_e.pdf . Accessed 22 June 2009.
Young, C. & Horton, R. Putting clinical trials into context. Lancet 366 , 107–107 (2005).
P. Group, Moher, D., Liberati, A., Tetzlaff, J. & Altman, D. G. Preferred reporting items for systematic reviews and meta-analyses: The PRISMA statement. PLoS Med. 6 , e1000097 (2009).
Kitchenham, B. & Charters, S. Guidelines for performing systematic literature reviews in software engineering (2007).
Van Solingen, R., Basili, V., Caldiera, G. & Rombach, H. D. Goal question metric (gqm) approach. Encycl. Softw. Eng. (2002).
Brereton, P., Kitchenham, B. A., Budgen, D., Turner, M. & Khalil, M. Lessons from applying the systematic literature review process within the software engineering domain. J. Syst. Softw. 80 , 571–583 (2007).
Achimugu, P., Selamat, A., Ibrahim, R. & Mahrin, M. N. R. A systematic literature review of software requirements prioritization research. Inf. Softw. Technol. 56 , 568–585 (2014).
Nazir, S., Ali, Y., Ullah, N. & García-Magariño, I. Internet of things for healthcare using effects of mobile computing: A systematic literature review. Wirel. Commun. Mobile Comput. 109 , 5931315 (2019).
Wohlin, C. Guidelines for snowballing in systematic literature studies and a replication in software engineering. In Proceedings of the 18th International Conference on Evaluation and Assessment in Software Engineering 1–10 (2014).
Kable, A. K., Pich, J. & Maslin-Prothero, S. E. A structured approach to documenting a search strategy for publication: A 12 step guideline for authors. Nurse Educ. Today 32 , 878–886 (2012).
Helmer, A., Kretschmer, F., Müller, F., Eichelberg, M., Deparade, R., Tegtbur, U. et al. Integration of medical models in personal health records using the example of rehabilitation training for cardiopulmonary patients. In 2011 4th International Conference on Biomedical Engineering and Informatics (BMEI) 1887–1892 (2011).
Tian, M. Integrated feature based medical image retrieval. In 2011 International Conference on Control, Automation and Systems Engineering (CASE) 1–3 (2011).
Chaves, R., Ramírez, J., Górriz, J. M., Illán, I. A. & Salas-Gonzalez, D. FDG and PIB biomarker PET analysis for the Alzheimer’s disease detection using Association Rules. In 2012 IEEE Nuclear Science Symposium and Medical Imaging Conference Record (NSS/MIC) 2576–2579 (2012).
Chute, C. G. Obstacles and options for big-data applications in biomedicine: The role of standards and normalizations. In 2012 IEEE International Conference on Bioinformatics and Biomedicine (2012).
Goel, A. & Chandra, N. A prototype model for secure storage of medical images and method for detail analysis of patient records with PACS. In 2012 International Conference on Communication Systems and Network Technologies 167–170 (2012).
Huang, H. & Hsiao, I. Use of anatomical information in a Bayesian reconstruction with an edge-preserving median prior. In 2012 IEEE Nuclear Science Symposium and Medical Imaging Conference Record (NSS/MIC) 3321–3323 (2012).
López, C. M., Welkenhuysen, M., Musa, S., Eberle, W., Bartic, C., Puers, R. et al. Towards a noise prediction model for in vivo neural recording. In 2012 Annual International Conference of the IEEE Engineering in Medicine and Biology Society 759–762 (2012).
Ng, H., Chuang, C. & Hsu, C. Extraction and analysis of structural features of lateral ventricle in brain medical images. In 2012 Sixth International Conference on Genetic and Evolutionary Computing 35–38 (2012).
Patel, A. B., Birla, M. & Nair, U. Addressing big data problem using Hadoop and Map Reduce. In 2012 Nirma University International Conference on Engineering (NUiCONE) 1–5 (2012).
Zheng, G., Yu, L., Feng, Y., Han, Z., Chen, L., Zhang, S. et al. Seizure prediction model based on method of common spatial patterns and support vector machine. In 2012 IEEE International Conference on Information Science and Technology 29–34 (2012).
Li, L., Bagheri, S., Goote, H., Hasan, A. & Hazard, G. Risk adjustment of patient expenditures: A big data analytics approach. In 2013 IEEE International Conference on Big Data 12–14 (2013).
Loshin, D. Chapter 8—Developing big data applications. In Big Data Analytics (ed. Loshin, D.) 73–81 (Morgan Kaufmann, 2013).
Chapter MATH Google Scholar
Loshin, D. Chapter 9—NoSQL data management for big data. In Big Data Analytics (ed. Loshin, D.) 83–90 (Morgan Kaufmann, 2013).
Loshin, D. Chapter 1—Market and business drivers for big data analytics. In Big Data Analytics (ed. Loshin, D.) 1–9 (Morgan Kaufmann, 2013).
MATH Google Scholar
Purkayastha, S. & Braa, J. Big data analytics for developing countries–Using the cloud for operational BI in health. Electron. J. Inf. Syst. Dev. Ctries. 59 , 1–17 (2013).
Lin, C.-H., Huang, L.-C., Chou, S.-C. T., Liu, C.-H., Cheng, H.-F. & Chiang, I. J. Temporal event tracing on big healthcare data analytics. In 2014 IEEE International Congress on Big Data 281–287 (2014)
Martínez, J. G., Ramos-Becerril, F. J., Leija, L., López, F., García, U., Vera, A. et al. Development of an electronic equipment for the pre medical diagnose in the progress of diabetic foot disease. In 2014 International Conference on Control, Decision and Information Technologies (CoDIT) 679–683 (2014).
Mian, M., Teredesai, A., Hazel, D., Pokuri, S. & Uppala, K. Work in progress—In-memory analysis for healthcare big data. In 2014 IEEE International Congress on Big Data 778–779 (2014).
Panahiazar, M., Taslimitehrani, V., Jadhav, A. & Pathak, J. Empowering personalized medicine with big data and semantic web technology: Promises, challenges, and use cases. In 2014 IEEE International Conference on Big Data (Big Data) 790–795 (2014).
Vargheese, R. Dynamic protection for critical health care systems using cisco CWS: Unleashing the power of big data analytics. In 2014 Fifth International Conference on Computing for Geospatial Research and Application 77–81 (2014).
Archenaa, J. & Anita, E. A. M. A survey of big data analytics in healthcare and government. Procedia Comput. Sci. 50 , 408–413 (2015).
Boman, M. & Sanches, P. Sensemaking in intelligent health data analytics. KI Künstliche Intell. 29 , 143–152 (2015).
Chong, D. & Shi, H. Big data analytics: A literature review. J. Manag. Anal. 2 , 175–201 (2015).
Dantanarayana, G., Sahama, T. & Wikramanayake, G. Quality of information for quality of life: Healthcare big data analytics. In 2015 Fifteenth International Conference on Advances in ICT for Emerging Regions (ICTer) 281–281 (2015).
Gomathi, S. & Narayani, V. Implementing big data analytics to predict systemic lupus erythematosus. In 2015 International Conference on Innovations in Information, Embedded and Communication Systems (ICIIECS) 1–5 (2015).
Hussain, S. & Lee, S. Semantic transformation model for clinical documents in big data to support healthcare analytics. In 2015 Tenth International Conference on Digital Information Management (ICDIM) 99–102 (2015).
Kuo, M., Chrimes, D., Moa, B. & Hu, W. Design and construction of a big data analytics framework for health applications. In 2015 IEEE International Conference on Smart City/SocialCom/SustainCom (SmartCity) 631–636 (2015).
Mehmood, R. & Graham, G. Big data logistics: A health-care transport capacity sharing model. Procedia Comput. Sci. 64 , 1107–1114 (2015).
Raj, P., Raman, A., Nagaraj, D. & Duggirala, S. Big data analytics for healthcare. In High-Performance Big-Data Analytics Computer Communications and Networks 1525–1525 (Springer, Cham, 2015).
Viceconti, M., Hunter, P. & Hose, R. Big data, big knowledge: Big data for personalized healthcare. IEEE J. Biomed. Health Inform. 19 , 1209–1215 (2015).
Wang, M. D. Biomedical big data analytics for patient-centric and outcome-driven precision health. In 2015 IEEE 39th Annual Computer Software and Applications Conference 1–2 (2015).
Batarseh, F. A. & Latif, E. A. Assessing the quality of service using big data analytics: With application to healthcare. Big Data Res. 4 , 13–24 (2016).
Buzzi, M. C. et al. Facebook: A new tool for collecting health data?. Multimed. Tools Appl. 76 , 10677–10700 (2016).
Chauhan, R., Jangade, R. & Mudunuru, V. K. A cloud based environment for big data analytics in healthcare. In International Conference on Soft Computing and Pattern Recognition 315–321 (2016).
Stefano, A. D., Corte, A. L., Lió, P. & Scatá, M. Bio-inspired ICT for big data management in healthcare. In Intelligent Agents in Data-intensive Computing 1–26 (Springer, 2016).
Gupta, S. & Tripathi, P. An emerging trend of big data analytics with health insurance in India. In 2016 International Conference on Innovation and Challenges in Cyber Security (ICICCS-INBUSH) 64–69 (2016).
Haas, M. et al. Big data to smart data in Alzheimer’s disease: Real-world examples of advanced modeling and simulation. Alzheimers Dement. 12 , 1022–1030 (2016).
Jiang, P. et al. An intelligent information forwarder for healthcare big data systems with distributed wearable sensors. IEEE Syst. J. 10 , 1147–1159 (2016).
Kankanhalli, A., Hahn, J., Tan, S. & Gao, G. Big data and analytics in healthcare: Introduction to the special section. Inf. Syst. Front. 18 , 233–235 (2016).
Kashyap, H., Ahmed, H. A., Hoque, N., Roy, S. & Bhattacharyya, D. K. Big data analytics in bioinformatics: Architectures, techniques, tools and issues. Netw. Model. Anal. Health Inform. Bioinform. 5 , 28 (2016).
Lv, Z., Chirivella, J. & Gagliardo, P. Bigdata oriented multimedia mobile health applications. J. Med. Syst. 40 , 120 (2016).
Pandey, M. K. & Subbiah, K. A novel storage architecture for facilitating efficient analytics of health informatics big data in cloud. In 2016 IEEE International Conference on Computer and Information Technology (CIT) 578–585 (2016).
Plachkinova, M., Vo, A., Bhaskar, R. & Hilton, B. A conceptual framework for quality healthcare accessibility: A scalable approach for big data technologies. Inf. Syst. Front. 20 , 289–302 (2016).
Rallapalli, S., Gondkar, R. R. & Ketavarapu, U. P. K. Impact of processing and analyzing healthcare big data on cloud computing environment by implementing hadoop cluster. Procedia Comput. Sci. 85 , 16–22 (2016).
Sakr, S. & Elgammal, A. Towards a comprehensive data analytics framework for smart healthcare services. Big Data Res. 4 , 44–58 (2016).
Xu, B. et al. Healthcare data analytics: Using a metadata annotation approach for integrating electronic hospital records. J. Manag. Anal. 3 , 136–151 (2016).
Tresp, V. et al. Going digital: A survey on digitalization and large-scale data analytics in healthcare. Proc. IEEE 104 , 2180–2206 (2016).
Straton, N., Hansen, K., Mukkamala, R. R., Hussain, A., Gronli, T., Langberg, H. et al. Big social data analytics for public health: Facebook engagement and performance. In 2016 IEEE 18th International Conference on e-Health Networking, Applications and Services (Healthcom) 1–6 (2016).
Abouelmehdi, K., Beni-Hssane, A., Khaloufi, H. & Saadi, M. Big data security and privacy in healthcare: A review. Procedia Comput. Sci. 113 , 73–80 (2017).
Alonso, S. G., de la Torre, Diez I., Rodrigues, J. J., Hamrioui, S. & Lopez-Coronado, M. A systematic review of techniques and sources of big data in the healthcare sector. J. Med. Syst. 41 , 183 (2017).
Anjum, A. et al. Big data analytics in healthcare: A cloud-based framework for generating insights. In Cloud Computing 153–170 (Springer, 2017).
Barik, R. K., Dubey, H. & Mankodiya, K. SOA-FOG: Secure service-oriented edge computing architecture for smart health big data analytics. In 2017 IEEE Global Conference on Signal and Information Processing (GlobalSIP) 477–481 (2017).
Cano, I., Tenyi, A., Vela, E., Miralles, F. & Roca, J. Perspectives on big data applications of health information. Curr. Opin. Syst. Biol. 3 , 36–42 (2017).
A. Di Meglio and M. Manca, "From Big Data to Big Insights: The Role of Platforms in Healthcare IT," in New Perspectives in Medical Records, ed: Springer, 2017, pp. 33–47.
Manogaran, G. et al. Big data analytics in healthcare Internet of Things. In Innovative Healthcare Systems for the 21st Century 263–284 (Springer, 2017).
Plageras, A. P., Stergiou, C., Kokkonis, G., Psannis, K. E., Ishibashi, Y., Kim, B. et al. Efficient large-scale medical data (eHealth Big Data) analytics in Internet of Things. In 2017 IEEE 19th Conference on Business Informatics (CBI) 21–27 (2017).
Pramanik, M. I., Lau, R. Y. K., Demirkan, H. & Azad, M. A. K. Smart health: Big data enabled health paradigm within smart cities. Expert Syst. Appl. 87 , 370–383 (2017).
Spanoudakis, G., Katrakazas, P., Koutsouris, D., Kikidis, D., Bibas, A. & Pontopidan, N. H. Public health policy for management of hearing impairments based on big data analytics: EVOTION at genesis. In 2017 IEEE 17th International Conference on Bioinformatics and Bioengineering (BIBE) 525–530 (2017).
Wu, J., Li, H., Liu, L. & Zheng, H. Adoption of big data and analytics in mobile healthcare market: An economic perspective. Electron. Commer. Res. Appl. 22 , 24–41 (2017).
Aceto, G., Persico, V. & Pescape, A. The role of Information and Communication Technologies in healthcare: Taxonomies, perspectives, and challenges. J. Netw. Comput. Appl. 107 , 125–154 (2018).
Antoniou, C., Dimitriou, L. & Pereira, F. Mobility Patterns, Big Data and Transport Analytics: Tools and Applications for Modeling (Elsevier, 2018).
Bates, D. W., Heitmueller, A., Kakad, M. & Saria, S. Why policymakers should care about “big data” in healthcare. Health Policy Technol. 7 , 211–216 (2018).
Choi, T.-M., Wallace, S. W. & Wang, Y. Big data analytics in operations management. Prod. Oper. Manag. 27 , 1868–1883 (2018).
Forestiero, A. & Papuzzo, G. Distributed algorithm for big data analytics in healthcare. In 2018 IEEE/WIC/ACM International Conference on Web Intelligence (WI) 776–779 (2018).
Ganesh, S. & Talukder, A. K. Formal methods, artificial intelligence, big-data analytics, and knowledge engineering in medical care to reduce disease burden and health disparities. In International Conference on Big Data Analytics 307–321 (2018).
Giacalone, M., Cusatelli, C. & Santarcangelo, V. Big data compliance for innovative clinical models. Big Data Res. 12 , 35–40 (2018).
Guha, S. & Kumar, S. Emergence of big data research in operations management, information systems, and healthcare: Past contributions and future roadmap. Prod. Oper. Manag. 27 , 1724–1735 (2018).
Gupta, V., Singh Gill, H., Singh, P. & Kaur, R. An energy efficient fog-cloud based architecture for healthcare. J. Stat. Manag. Syst. 21 , 529–537 (2018).
Hopp, W. J., Li, J. & Wang, G. Big data and the precision medicine revolution. Prod. Oper. Manag. 27 , 1647–1664 (2018).
Huang, H. K. Big data in PACS-based multimedia medical imaging informatics. In PACS Based Multimedia Imaging Informatics (ed Huang, H.) 575–589 (2018).
Istepanian, R. S. H. & Al-Anzi, T. m-Health 2.0: New perspectives on mobile health, machine learning and big data analytics. Methods 151 , 34–40 (2018).
Khaloufi, H., Abouelmehdi, K., Beni-hssane, A. & Saadi, M. Security model for big healthcare data lifecycle. Procedia Comput. Sci. 141 , 294–301 (2018).
Krittanawong, C., Johnson, K. W., Hershman, S. G. & Tang, W. H. W. Big data, artificial intelligence, and cardiovascular precision medicine. Expert Rev. Precis. Med. Drug Dev. 3 , 305–317 (2018).
Ma, X., Wang, Z., Zhou, S., Wen, H. & Zhang, Y. Intelligent healthcare systems assisted by data analytics and mobile computing. In 2018 14th International Wireless Communications & Mobile Computing Conference (IWCMC) 1317–1322 (2018).
Manogaran, G. et al. A new architecture of Internet of Things and big data ecosystem for secured smart healthcare monitoring and alerting system. Future Gener. Comput. Syst. 82 , 375–387 (2018).
Mehta, N. & Pandit, A. Concurrence of big data analytics and healthcare: A systematic review. Int. J. Med. Inform. 114 , 57–65 (2018).
Miller, J. B. Big data and biomedical informatics: Preparing for the modernization of clinical neuropsychology. Clin. Neuropsychol. 33 , 287–304 (2018).
Moutselos, K., Kyriazis, D. & Maglogiannis, I. A web based modular environment for assisting health policy making utilizing big data analytics. In 2018 9th International Conference on Information, Intelligence, Systems and Applications (IISA) 1–5 (2018).
Nair, L. R., Shetty, S. D. & Shetty, S. D. Applying spark based machine learning model on streaming big data for health status prediction. Comput. Electr. Eng. 65 , 393–399 (2018).
Pashazadeh, A. & Navimipour, N. J. Big data handling mechanisms in the healthcare applications: A comprehensive and systematic literature review. J. Biomed. Inform. 82 , 47–62 (2018).
Ravishankar Rao, A., Clarke, D. & Vargas, M. Building an open health data analytics platform: A case study examining relationships and trends in seniority and performance in healthcare providers. J. Healthc. Inform. Res. 2 , 44–70 (2018).
Sahoo, P. K., Mohapatra, S. K. & Wu, S.-L. SLA based healthcare big data analysis and computing in cloud network. J. Parallel Distrib. Comput. 119 , 121–135 (2018).
Sarkar, B. K. & Sana, S. S. A conceptual distributed framework for improved and secured healthcare system. Int. J. Healthc. Manag. 1–13 (2018).
Sebaa, A., Chikh, F., Nouicer, A. & Tari, A. Medical big data warehouse: architecture and system design, a case study: Improving healthcare resources distribution. J. Med. Syst. 42 , 59 (2018).
Shafqat, S., Kishwer, S., Rasool, R. U., Qadir, J., Amjad, T. & Ahmad, H. F. Big data analytics enhanced healthcare systems: A review. J. Supercomput.
Sivaparthipan, C. B., Karthikeyan, N. & Karthik, S. Designing statistical assessment healthcare information system for diabetics analysis using big data. Multimed. Tools Appl.
Tang, V. et al. An adaptive clinical decision support system for serving the elderly with chronic diseases in healthcare industry. Expert. Syst. 36 , e12369 (2018).
Wang, Y., Kung, L. & Byrd, T. A. Big data analytics: Understanding its capabilities and potential benefits for healthcare organizations. Technol. Forecast. Soc. Change 126 , 3–13 (2018).
Agrawal, A. & Choudhary, A. Health services data: Big data analytics for deriving predictive healthcare insights. In Health Services Evaluation 3–18 (2019).
Ahmed, M., Choudhury, S. & Al-Turjman, F. Big data analytics for intelligent internet of things. In Artificial Intelligence in IoT 107–127 (Springer, 2019).
Ahmed, Z. & Liang, B. T. Systematically dealing practical issues associated to healthcare data analytics. In Future of Information and Communication Conference 599–613 (2019).
Bora, D. J. Chapter 3—Big data analytics in healthcare: A critical analysis. In Big Data Analytics for Intelligent Healthcare Management (eds Dey, N. et al. ) 43–57 (Academic Press, 2019).
Chanchaichujit, J., Tan, A., Meng, F. & Eaimkhong, S. Internet of Things (IoT) and big data analytics in healthcare. In Healthcare 4.0 17–36 (Springer, 2019).
Cirillo, D. & Valencia, A. Big data analytics for personalized medicine. Curr. Opin. Biotechnol. 58 , 161–167 (2019).
Dey, N., Das, H., Naik, B. & Behera, H. S. Big Data Analytics for Intelligent Healthcare Management (Academic Press, 2019).
Din, S. & Paul, A. Smart health monitoring and management system: Toward autonomous wearable sensing for Internet of Things using big data analytics. Future Gener. Comput. Syst. 91 , 611–619 (2019).
Galetsi, P., Katsaliaki, K. & Kumar, S. Values, challenges and future directions of big data analytics in healthcare: A systematic review. Soc. Sci. Med. 241 , 112533 (2019).
Guo, C. & Chen, J. Big data analytics in healthcare: data-driven methods for typical treatment pattern mining. J. Syst. Sci. Syst. Eng. 28 , 694–714 (2019).
Hussain, S. et al. Semantic preservation of standardized healthcare documents in big data. Int. J. Med. Inform. 129 , 133–145 (2019).
Mehta, N., Pandit, A. & Shukla, S. Transforming healthcare with big data analytics and artificial intelligence: A systematic mapping study. J. Biomed. Inform. 100 , 103311 (2019).
Muniasamy, A., Tabassam, S., Hussain, M. A., Sultana, H., Muniasamy, V. & Bhatnagar, R. Deep learning for predictive analytics in healthcare. In International Conference on Advanced Machine Learning Technologies and Applications 32–42 (2019).
Palanisamy, V. & Thirunavukarasu, R. Implications of big data analytics in developing healthcare frameworks–A review. J. King Saud Univ. Comput. Inf. Sci. 31 , 415–425 (2019).
Rajabion, L., Shaltooki, A. A., Taghikhah, M., Ghasemi, A. & Badfar, A. Healthcare big data processing mechanisms: The role of cloud computing. Int. J. Inf. Manag. 49 , 271–289 (2019).
Ramasamy, V., Gomathy, B. & Verma, R. K. Smart HIV/AIDS digital system using big data analytics. In Progress in Advanced Computing and Intelligent Engineering 415–421 (Springer, 2019).
Razzak, M. I., Imran, M. & Xu, G. Big data analytics for preventive medicine. Neural Comput. Appl.
Reiz, A. N., de la Hoz, M. A. & García, M. S. Big data analysis and machine learning in intensive care units. Med. Intensiva 43 , 416–426 (2019).
Saheb, T. & Izadi, L. Paradigm of IoT big data analytics in the healthcare industry: A review of scientific literature and mapping of research trends. Telematics Inform. 41 , 70–85 (2019).
Sahoo, A. K. et al. Chapter 9—Intelligence-based health recommendation system using big data analytics. In Big Data Analytics for Intelligent Healthcare Management (eds Dey, N. et al. ) 227–246 (Academic Press, 2019).
Shahbaz, M., Gao, C., Zhai, L., Shahzad, F. & Hu, Y. Investigating the adoption of big data analytics in healthcare: The moderating role of resistance to change. J. Big Data 6 , 6 (2019).
Sivaparthipan, C. B. et al. Innovative and efficient method of robotics for helping the Parkinson’s disease patient using IoT in big data analytics. Trans. Emerg. Telecommun. Technol. 31 , e3838 (2019).
Sousa, M. J., Pesqueira, A. N. M., Lemos, C., Sousa, M. & Rocha, Ãl. Decision-making based on big data analytics for people management in healthcare organizations. J. Med. Syst. 43 , 290 (2019).
Strang, K. D. Problems with research methods in medical device big data analytics. Int. J. Data Sci. Anal.
Thomas, J., Kneale, D., McKenzie, J. E., Brennan, S. E. & Bhaumik, S. Determining the scope of the review and the questions it will address. In Cochrane Handbook for Systematic Reviews of Interventions 13–31 (2019).
Wang, Y., Kung, L., Gupta, S. & Ozdemir, S. Leveraging big data analytics to improve quality of care in healthcare organizations: A configurational perspective. Br. J. Manag. 30 , 362–388 (2019).
Zetino, J. & Mendoza, N. Big data and its utility in social work: Learning from the big data revolution in business and healthcare. Soc. Work Public Health 34 , 409–417 (2019).
Nazir, S., Nawaz, M., Adnan, A., Shahzad, S. & Asadi, S. Big data features, applications, and analytics in cardiology—A systematic literature review. IEEE Access 7 , 143742–143771 (2019).
Shah, G., Shah, A. & Shah, M. Panacea of challenges in real-world application of big data analytics in healthcare sector. J. Data Inf. Manag. 1 , 107–116 (2019).
Galetsi, P., Katsaliaki, K. & Kumar, S. Big data analytics in health sector: Theoretical framework, techniques and prospects. Int. J. Inf. Manag. 50 , 206–216 (2020).
Iyengar, S. P., Acharya, H. & Kadam, M. Big data analytics in healthcare using spreadsheets. In Big Data Analytics in Healthcare 155–187 (Springer, 2002).
Kumar, S. A. & Venkatesulu, M. BrownBoost classifier-based bloom hash data storage for healthcare big data analytics. In Information and Communication Technology for Sustainable Development 53–69 (Springer, 2020).
Kumar, Y., Sood, K., Kaul, S. & Vasuja, R. Big data analytics and its benefits in healthcare. In Big Data Analytics in Healthcare 3–21 (Springer, 2020).
Naqishbandi, T. A. & Ayyanathan, N. Clinical big data predictive analytics transforming healthcare:-An integrated framework for promise towards value based healthcare. In Advances in Decision Sciences 545–561 (Springer, 2020).
Lambay, M. A. & Mohideen, S. P. Big data analytics for healthcare recommendation systems. In 2020 International Conference on System, Computation, Automation and Networking (ICSCAN) 1–6 (2020).
Katarya, R. & Jain, S. Exploration of big data analytics in healthcare analytics. In 2020 4th International Conference on Computer, Communication and Signal Processing (ICCCSP) 1–4 (2020).
Javid, T., Faris, M., Beenish, H. & Fahad, M. Cybersecurity and data privacy in the cloudlet for preliminary healthcare big data analytics. In 2020 International Conference on Computing and Information Technology (ICCIT-1441) 1–4 (2020).
Leung, C. K., Chen, Y., Hoi, C. S. H., Shang, S. & Cuzzocrea, A. Machine learning and OLAP on big COVID-19 data. In 2020 IEEE International Conference on Big Data (Big Data) 5118–5127 (2020).
Akhtar, U., Lee, J. W., Bilal, H. S. M., Ali, T., Khan, W. A. & Lee, S. The impact of big data in healthcare analytics. In 2020 International Conference on Information Networking (ICOIN) 61–63 (2020).
Mung, P. S. & Phyu, S. Effective analytics on healthcare big data using ensemble learning. In 2020 IEEE Conference on Computer Applications (ICCA) 1–4 (2002).
Georgakopoulos, S. V., Gallos, P. & Plagianakos, V. P. Using big data analytics to detect fraud in healthcare provision. In 2020 IEEE 5th Middle East and Africa Conference on Biomedical Engineering (MECBME) 1–3 (2020).
Leung, C. K., Chen, Y., Shang, S. & Deng, D. Big data science on COVID-19 Data. In 2020 IEEE 14th International Conference on Big Data Science and Engineering (BigDataSE) 14–21 (2020).
Juddoo, S. & George, C. A Qualitative assessment of machine learning support for detecting data completeness and accuracy issues to improve data analytics in big data for the healthcare industry. In 2020 3rd International Conference on Emerging Trends in Electrical, Electronic and Communications Engineering (ELECOM) 58–66 (2020).
Chauhan, R. & Yafi, E. Big data analytics for prediction modelling in healthcare databases. In 2021 15th International Conference on Ubiquitous Information Management and Communication (IMCOM) 1–5 (2021).
Islam, M., Karim, R., Khatun, M. A. & Reza, S. A research on big data analytics in healthcare industry. In 2020 International Conference on Information Science and Communications Technologies (ICISCT) 1–5 (2020).
Leung, C. K., Chen, Y., Hoi, C. S. H., Shang, S., Wen, Y. & Cuzzocrea, A. Big data visualization and visual analytics of COVID-19 data. In 2020 24th International Conference Information Visualisation (IV) 415–420 (2020).
Balaji, S. & Prasathkumar, V. Dynamic changes by big data in health care. In 2020 International Conference on Computer Communication and Informatics (ICCCI) 1–4 (2020).
Alahmar, A. & Benlamri, R. Optimizing hospital resources using big data analytics with standardized e-clinical pathways. In 2020 IEEE Intl Conf on Dependable, Autonomic and Secure Computing, Intl Conf on Pervasive Intelligence and Computing, Intl Conf on Cloud and Big Data Computing, Intl Conf on Cyber Science and Technology Congress (DASC/PiCom/CBDCom/CyberSciTech) 650–657 (2020).
Sadineni, P. K. Developing a model to enhance the quality of health informatics using big data. In 2020 Fourth International Conference on I-SMAC (IoT in Social, Mobile, Analytics and Cloud) (I-SMAC) 1267–1272 (2020).
Pramanik, M. I. et al. Healthcare informatics and analytics in big data. Expert Syst. Appl. 152 , 113388 (2020).
Ravikumaran, P., Vimala Devi, K., Kartheeban, K. & Narayanan Prasanth, N. Health data analytics: Framework & review on tool & technology. Mater. Today Proc. (2020).
Ramesh, T. & Santhi, V. Exploring big data analytics in health care. Int. J. Intell. Netw. 1 , 135–140 (2020).
Galetsi, P. & Katsaliaki, K. A review of the literature on big data analytics in healthcare. J. Oper. Res. Soc. 71 , 1511–1529 (2020).
Mehta, N., Pandit, A. & Kulkarni, M. Elements of healthcare big data analytics. In Big Data Analytics in Healthcare 23–43 (Springer, 2020).
Ehwerhemuepha, L. et al. HealtheDataLab–a cloud computing solution for data science and advanced analytics in healthcare with application to predicting multi-center pediatric readmissions. BMC Med. Inform. Decis. Mak. 20 , 1–12 (2020).
Sivasangari, A., Lakshmanan, L., Ajitha, P., Deepa, D. & Jabez, J. Big data analytics for 5G-enabled IoT healthcare. In Blockchain for 5G-Enabled IoT 261.
Ma, S. & Huai, J. Approximate computation for big data analytics. SIGWEB Newsl. (2021).
Uzunbaz, S. & Aref, W. G. Shared execution techniques for business data analytics over big data streams. In Presented at the 32nd International Conference on Scientific and Statistical Database Management, Vienna, Austria (2020).
Chalumporn, G. & Hewett, R. Health data analytics with an opportunistic big data algorithm. In Presented at the Proceedings of the 11th International Conference on Advances in Information Technology, Bangkok, Thailand (2020).
Minami, T. & Ohura, Y. Small data analysis for bigger data analysis. In Presented at the 2021 Workshop on Algorithm and Big Data, Fuzhou, China (2021).
Chakraborty, C. & Rathi, M. Chapter 2—Smart healthcare systems using big data. In Demystifying Big Data, Machine Learning, and Deep Learning for Healthcare Analytics (eds Kautish, P. N. S. & Peng, S.-L.) 17–32 (Academic Press, 2021).
Ilmudeen, A. Chapter 3—Big data-based frameworks for healthcare systems. In Demystifying Big Data, Machine Learning, and Deep Learning for Healthcare Analytics (eds Kautish, P. N. S. & Peng, S.-L.) 33–56 (Academic Press, 2021).
Mendhe, C. H., Henderson, N., Srivastava, G. & Mago, V. A scalable platform to collect, store, visualize, and analyze big data in real time. IEEE Trans. Comput. Soc. Syst. 8 , 260–269 (2021).
Sivabalaselvamani, D., Selvakarthi, D., Yogapriya, J., Thiruvenkatasuresh, M. P., Maruthappa, M. & Chandra, A. S. Artificial Intelligence in data-driven analytics for the personalized healthcare. In 2021 International Conference on Computer Communication and Informatics (ICCCI) 1–5 (2021)
Harb, H., Mansour, A., Nasser, A., Cruz, E. M. & de la Torre Diez, I. A sensor-based data analytics for patient monitoring in connected healthcare applications. IEEE Sens. J. 21 , 974–984 (2021).
Article ADS CAS Google Scholar
Jones, J. & Jones, J. Optimizing healthcare. In 2020 IEEE International Conference on E-health Networking, Application & Services (HEALTHCOM) 1–6 (2021).
Hassan, S., Dhali, M., Zaman, F. & Tanveer, M. Big data and predictive analytics in healthcare in Bangladesh: Regulatory challenges. Heliyon 7 , e07179 (2021).
Khan, S. et al. KNN and ANN-based recognition of handwritten pashto letters using zoning features. Mach. Learn. 9 , 570–577 (2018).
Pant, D., Kumar, V., Kishore, J. & Pal, R. Healthcare data modeling in R. In 2017 1st International Conference on Intelligent Systems and Information Management (ICISIM) 230–233 (2017).
Brennan, P. F. & Bakken, S. Nursing needs big data and big data needs nursing. J. Nurs. Scholarsh. 47 , 477–484 (2015).
Sreedevi, A. G., Nitya Harshitha, T., Sugumaran, V. & Shankar, P. Application of cognitive computing in healthcare, cybersecurity, big data and IoT: A literature review. Inform. Process. Manag. 59 , 102888 (2022).
Sinha, A., Hripcsak, G. & Markatou, M. Large datasets in biomedicine: A discussion of salient analytic issues. J. Am. Med. Inform. Assoc. JAMIA 16 , 759–767 (2009).
Alonso-Betanzos, A. & Bolón-Canedo, V. Big-Data analysis, cluster analysis, and machine-learning approaches (2018).
Dayal, M. & Singh, N. Indian health care analysis using big data programming tool. Procedia Comput. Sci. 89 , 521–527 (2016).
Jayaraman, P. P., Forkan, A. R. M., Morshed, A., Haghighi, P. D. & Kang, Y.-B. Healthcare 4.0: A review of frontiers in digital health. WIREs Data Min. Knowl. Discov. 10 , e1350 (2018).
Gallos, P. et al. CrowdHEALTH: Big data analytics and holistic health records. Stud. Health Technol. Inform. 258 , 255–256 (2019).
Wang, L., Ranjan, R., Kołodziej, J., Zomaya, A. & Alem, L. Software tools and techniques for big data computing in healthcare clouds. Future Gener. Comput. Syst. 43–44 , 38–39 (2015).
Kiourtis, A. et al. An autoscaling platform supporting graph data modelling big data analytics. Stud. Health Technol. Inform. 295 , 376–379 (2022).
Download references
Acknowledgements
This research work is performed by Department of Accounting and Information Systems, Collage of Business and Economics, Qatar University in collaboration with the Department of Computer Science, University of Swabi, Swabi, Pakistan.
Open Access funding provided by the Qatar National Library. This research was funded by Qatar University Internal Grant under Grant No. IRCC-2021–010. The findings achieved herein are solely the responsibility of the authors.
Author information
Authors and affiliations.
Department of Accounting and Information Systems, College of Business and Economics, Qatar University, Doha, Qatar
Sulaiman Khan & Habib Ullah Khan
Department of Computer Science, University of Swabi, Swabi, Pakistan
You can also search for this author in PubMed Google Scholar
Contributions
S.K. wrote the original draft of the paper. He also revised the draft based on the reviewers suggestions. Dr. H.U.K. developed the experimental setup for the proposed systematic research work. Dr. S.N. performed articles accumulation and database development process.
Corresponding author
Correspondence to Habib Ullah Khan .
Ethics declarations
Competing interests.
The authors declare no competing interests.
Additional information
Publisher's note.
Springer Nature remains neutral with regard to jurisdictional claims in published maps and institutional affiliations.
Rights and permissions
Open Access This article is licensed under a Creative Commons Attribution 4.0 International License, which permits use, sharing, adaptation, distribution and reproduction in any medium or format, as long as you give appropriate credit to the original author(s) and the source, provide a link to the Creative Commons licence, and indicate if changes were made. The images or other third party material in this article are included in the article's Creative Commons licence, unless indicated otherwise in a credit line to the material. If material is not included in the article's Creative Commons licence and your intended use is not permitted by statutory regulation or exceeds the permitted use, you will need to obtain permission directly from the copyright holder. To view a copy of this licence, visit http://creativecommons.org/licenses/by/4.0/ .
Reprints and permissions
About this article
Cite this article.
Khan, S., Khan, H.U. & Nazir, S. Systematic analysis of healthcare big data analytics for efficient care and disease diagnosing. Sci Rep 12 , 22377 (2022). https://doi.org/10.1038/s41598-022-26090-5
Download citation
Received : 09 September 2022
Accepted : 09 December 2022
Published : 26 December 2022
DOI : https://doi.org/10.1038/s41598-022-26090-5
Share this article
Anyone you share the following link with will be able to read this content:
Sorry, a shareable link is not currently available for this article.
Provided by the Springer Nature SharedIt content-sharing initiative
This article is cited by
The usage of population and disease registries as pre-screening tools for clinical trials, a systematic review.
- Juliette Foucher
- Louisa Azizi
- Caroline Ingre
Systematic Reviews (2024)
By submitting a comment you agree to abide by our Terms and Community Guidelines . If you find something abusive or that does not comply with our terms or guidelines please flag it as inappropriate.
Quick links
- Explore articles by subject
- Guide to authors
- Editorial policies
Sign up for the Nature Briefing: Translational Research newsletter — top stories in biotechnology, drug discovery and pharma.

The big-data revolution in US health care: Accelerating value and innovation
A big-data revolution is under way in health care. Start with the vastly increased supply of information. Over the last decade, pharmaceutical companies have been aggregating years of research and development data into medical databases, while payors and providers have digitized their patient records. Meanwhile, the US federal government and other public stakeholders have been opening their vast stores of health-care knowledge, including data from clinical trials and information on patients covered under public insurance programs. In parallel, recent technical advances have made it easier to collect and analyze information from multiple sources—a major benefit in health care, since data for a single patient may come from various payors, hospitals, laboratories, and physician offices.
Fiscal concerns, perhaps more than any other factor, are driving the demand for big-data applications. After more than 20 years of steady increases, health-care expenses now represent 17.6 percent of GDP—nearly $600 billion more than the expected benchmark for a nation of the United States’s size and wealth. 1 1. This estimate is based on a measure developed by McKinsey, “estimated spending according to wealth,” which is derived from a regression analysis of income and spending data from other countries in the Organisation of Economic Co-operation and Development. It allows us to estimate how much a given country would be expected to spend on health care based on per capita GDP. To discourage overutilization, many payors have shifted from fee-for-service compensation, which rewards physicians for treatment volume, to risk-sharing arrangements that prioritize outcomes. Under the new schemes, when treatments deliver the desired results, provider compensation may be less than before. Payors are also entering similar agreements with pharmaceutical companies and basing reimbursement on a drug’s ability to improve patient health. In this new environment, health-care stakeholders have greater incentives to compile and exchange information.
While health-care costs may be paramount in big data’s rise, clinical trends also play a role. Physicians have traditionally used their judgment when making treatment decisions, but in the last few years there has been a move toward evidence-based medicine, which involves systematically reviewing clinical data and making treatment decisions based on the best available information. Aggregating individual data sets into big-data algorithms often provides the most robust evidence, since nuances in subpopulations (such as the presence of patients with gluten allergies) may be so rare that they are not readily apparent in small samples.
Although the health-care industry has lagged behind sectors like retail and banking in the use of big data—partly because of concerns about patient confidentiality—it could soon catch up. First movers in the data sphere are already achieving positive results, which is prompting other stakeholders to take action, lest they be left behind. These developments are encouraging, but they also raise an important question: is the health-care industry prepared to capture big data’s full potential, or are there roadblocks that will hamper its use? (In a related video, McKinsey director Nicolaus Henke explains how analytics is transforming the practice of medicine.)
A new value framework
Health-care stakeholders are well versed in capturing value and have developed many levers to assist with this goal. But traditional tools do not always take complete advantage of the insights that big data can provide. Unit-price discounts, for instance, are based primarily on contracting and negotiating leverage. And like most other well-established health-care value levers, they focus solely on reducing costs rather than improving patient outcomes. Although these tools will continue to play an important role, stakeholders will only benefit from big data if they take a more holistic, patient-centered approach to value, one that focuses equally on health-care spending and treatment outcomes. We have created five pathways to assist them in redefining value and identifying tools that are appropriate for the new era. They focus on the following concepts:
- Right living. Patients must be encouraged to play an active role in their own health by making the right choices about diet, exercise, preventive care, and other lifestyle factors.
- Right care. Patients must receive the most timely, appropriate treatment available. In addition to relying heavily on protocols, right care requires a coordinated approach, with all caregivers having access to the same information and working toward the same goal to avoid duplication of effort and suboptimal treatment strategies.
- Right provider. Any professionals who treat patients must have strong performance records and be capable of achieving the best outcomes. They should also be selected based on their skill sets and abilities rather than their job titles. For instance, nurses or physicians’ assistants may perform many tasks that do not require a doctor.
- Right value. Providers and payors should continually look for ways to improve value while preserving or improving health-care quality. For example, they could develop a system in which provider reimbursement is tied to patient outcomes or undertake programs designed to eliminate wasteful spending.
- Right innovation. Stakeholders must focus on identifying new therapies and approaches to health-care delivery. They should also try to improve the innovation engines themselves—for instance, by advancing medicine and boosting R&D productivity.
The value pathways evolve as new data become available, fostering a feedback loop. The concept of right care, for instance, could change if new data suggest that the standard protocol for a particular disease does not produce optimal results. And a change in one pathway could spur changes in others, since they are interdependent. An investigation into right value, for example, could reveal that patients are most likely to suffer costly complications after an appendectomy if their physician performs few of these operations. This finding could influence opinions not only about value but about the right provider to perform an appendectomy.
The pathways in action
Some health-care leaders have already captured value from big data by focusing on the concepts outlined in our pathways or have set the groundwork for doing so. Consider a few examples:
- Kaiser Permanente has fully implemented a new computer system, HealthConnect, to ensure data exchange across all medical facilities and promote the use of electronic health records. The integrated system has improved outcomes in cardiovascular disease and achieved an estimated $1 billion in savings from reduced office visits and lab tests.
- Blue Shield of California, in partnership with NantHealth, is improving health-care delivery and patient outcomes by developing an integrated technology system that will allow doctors, hospitals, and health plans to deliver evidence-based care that is more coordinated and personalized. This will help improve performance in a number of areas, including prevention and care coordination.
- AstraZeneca established a four-year partnership with WellPoint’s data and analytics subsidiary, HealthCore, to conduct real-world studies to determine the most effective and economical treatments for some chronic illnesses and common diseases. AstraZeneca will use HealthCore data, together with its own clinical-trial data, to guide R&D investment decisions. The company is also in talks with payors about providing coverage for drugs already on the market, again using HealthCore data as evidence.
During a recent scan of the industry, we found that interest in big data is not confined to traditional players. Since 2010, more than 200 new businesses have developed innovative health-care applications. About 40 percent of these were aimed at direct health interventions or predictive capabilities. That’s a powerful new frontier for health-data applications, which historically focused more on data management and retrospective data analysis (exhibit).
Many innovative US health-care data applications move beyond retroactive reporting to interventions and predictive capabilities.
Some devices take patient monitoring to a new level. For instance, Asthmapolis has created a GPS-enabled tracker that records inhaler usage by asthmatics. The information is ported to a central database and used to identify individual, group, and population-based trends. The data are then merged with Centers for Disease Control and Prevention information about known asthma catalysts (such as high pollen counts in the Northeast or volcanic fog in Hawaii). Together, the information helps physicians develop personalized treatment plans and spot prevention opportunities.
Another company, Ginger.io, offers a mobile application in which patients with select conditions agree, in conjunction with their providers, to be tracked through their mobile phones and assisted with behavioral-health therapies. The app records data about calls, texts, geographic location, and even physical movements. Patients also respond to surveys delivered over their smartphones. The Ginger.io application integrates patient data with public research on behavioral health from the National Institutes of Health and other sources. The insights obtained can be revealing—for instance, a lack of movement or other activity could signal that a patient feels physically unwell, and irregular sleep patterns (revealed through late-night calls or texts) may signal that an anxiety attack is imminent.
Improvement at scale: What is the potential?
To determine the opportunity of the new value pathways, we evaluated a range of health-care initiatives and assessed their potential impact as total annual cost savings, holding outcomes constant, using a 2011 baseline. If these early successes were scaled up to create systemwide impact, we estimate that the pathways could account for $300 billion to $450 billion in reduced health-care spending, or 12 to 17 percent of the $2.6 trillion baseline in US health-care costs.
Even a few simple interventions can have an enormous impact when scaled up. In the “right living” pathway, for instance, we estimate that aspirin use by those at risk for coronary heart disease, combined with early cholesterol screening and smoking cessation, could reduce the total cost of their care by more than $30 billion. While these actions have been encouraged for some time, big data now enables faster identification of high-risk patients, more effective interventions, and closer monitoring.
Our estimate of $300 billion to $450 billion in reduced health-care spending could be conservative, as many insights and innovations are still ahead. We have yet to fully understand subpopulation efficacy of cancer therapies and the predictive indicators of relapse, for example, and we believe the big-data revolution will uncover many new learning opportunities in these areas.
A few caveats
Although we are optimistic about big data’s potential to transform health care, some structural issues may pose obstacles. The move away from fee-for-service care—already well under way—must continue. Similarly, traditional medical-management techniques must change, since they pit payors and providers against each other, framing benefit plans with respect to what is and is not covered rather than what is and is not most effective. And all stakeholders must recognize the value of big data and be willing to act on its insights, a fundamental mind-set shift for many and one that may prove difficult to achieve. Patients will not benefit from research on exercise, for example, if they persist in their sedentary lifestyles. And physicians may not improve patient outcomes if they refuse to follow treatment protocols based on big data and instead rely solely on their own judgment.
Privacy issues will continue to be a major concern. Although new computer programs can readily remove names and other personal information from records being transported into large databases, stakeholders across the industry must be vigilant and watch for potential problems as more information becomes public.
Finally, health care will need to learn from other data-driven revolutions. All too often, players have taken advantage of data transparency by pursuing objectives that create value only for themselves, and this could also occur in the health-care sector. For instance, owners of MRI machines, looking to amortize fixed costs across more patients, might choose to use big data only to identify underserved patients and disease areas. If they convincingly market their services, patients may receive unnecessary MRIs—a situation that would increase costs without necessarily improving outcomes.
Big-data initiatives have the potential to transform health care. Stakeholders that are committed to innovation, willing to build their capabilities, and open to a new view of value will likely be the first to reap the rewards of big data and help patients achieve better outcomes.
Download the full report, The ‘big data’ revolution in healthcare: Accelerating value and innovation (PDF–1.4MB).
Basel Kayyali is a principal in McKinsey’s New Jersey office, where Steve Van Kuiken is a director; David Knott is a director in the New York office.
The authors would like to thank Peter Groves for his extensive contribution to this article.
Explore a career with us
Related articles.
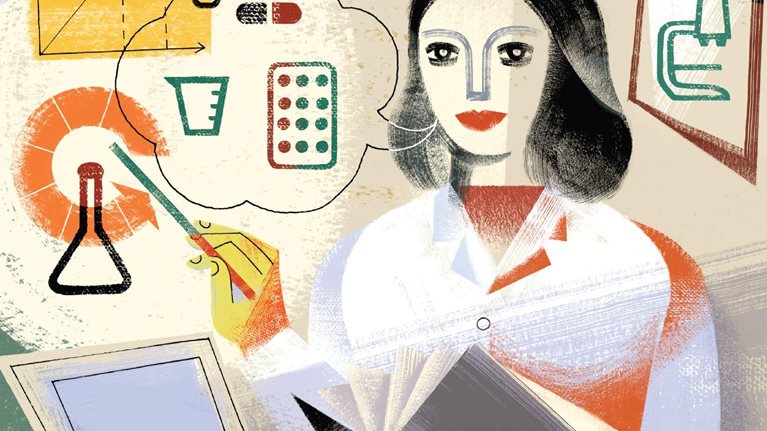
How big data can revolutionize pharmaceutical R&D
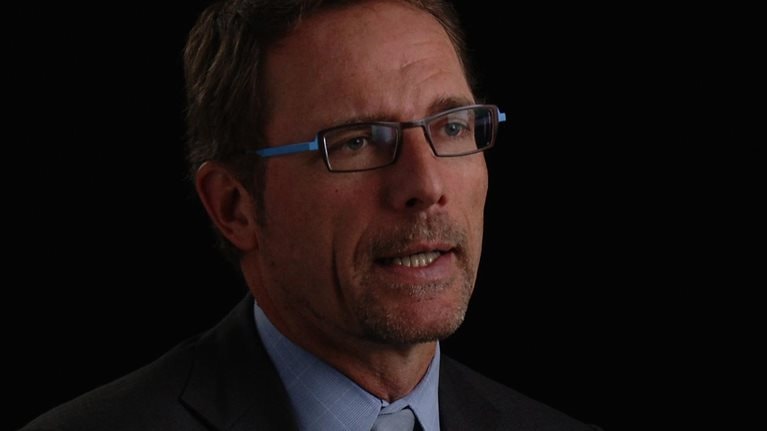
Making data analytics work: Three key challenges
24 Examples Of Big Data Analytics In Healthcare That Can Save People
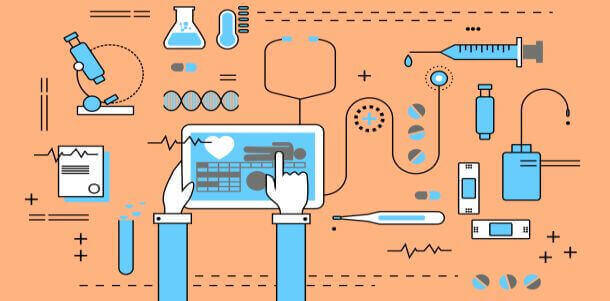
Table of Contents
1) What Is Big Data In Healthcare?
2) Top Big Data Applications In Healthcare
3) How To Use Big Data In Healthcare
4) Why Use Big Data Analytics In Healthcare
5) Obstacles Of Big Data In Healthcare
Big data has changed how we manage, analyze, and leverage data across industries. One of the most notable areas where data analysis is making big changes is healthcare.
In fact, healthcare analytics has the potential to reduce costs of treatment, predict outbreaks of epidemics, avoid preventable diseases, and improve the quality of life in general. The average human lifespan is increasing across the world population, which poses new challenges to today’s treatment delivery methods. Health professionals, just like business entrepreneurs, are capable of collecting massive amounts of data and looking for the best strategies to use these numbers.
In this article, we’re going to address the need for big data in healthcare and hospital big data: why and how can it help? What are the obstacles to its adoption? We will then look at 24 big data examples in healthcare that already exist and that medical-based institutions can benefit from.
But first, let’s examine the core concept and role of big data in healthcare.
What Is Big Data In Healthcare?

Big data in healthcare is a term used to describe massive volumes of information created by the adoption of digital technologies that collect patients' records and help in managing hospital performance; otherwise too large and complex for traditional technologies.
The application of big data analytics in healthcare has a lot of positive and also life-saving outcomes. In essence, big-style data refers to the vast quantities of information created by the digitization of everything that gets consolidated and analyzed by specific technologies. Applied to healthcare, it will use specific health data of a population (or of a particular individual) and potentially help to prevent epidemics, cure diseases, cut down costs, etc.
Now that we live longer, treatment models have changed, and many of these changes are namely driven by data. Doctors want to understand as much as they can about a person and, as early in their life as possible, to pick up warning signs of serious illness as they arise – treating any disease at an early stage is far more simple and less expensive. By utilizing key performance indicators in healthcare and healthcare data analytics, prevention is better than cure, and managing to draw a comprehensive picture of someone will let insurance provide a tailored package. This is the industry’s attempt to tackle the siloes problems a patient’s data has: everywhere are collected bits and bites of it and archived in hospitals, clinics, surgeries, etc., with the impossibility of communicating properly.
That said, the amount of sources from which health professionals can gain insights from their patients keeps growing. This data is normally coming in different formats and sizes, which presents a challenge to the user. However, the current focus is no longer on how “big” the data is but on how smartly it is managed. With the help of the right technology, data can be extracted from the following sources of the healthcare industry in a smart and fast way:
- Patients portals
- Research studies
- Wearable devices
- Search engines
- Generic databases
- Government agencies
- Payer records
- Staffing schedules
- Patient waiting room
Indeed, for years gathering huge amounts of data for medical use has been costly and time-consuming. With today’s always-improving technologies, it becomes easier not only to collect such data but also to create comprehensive healthcare reports and convert them into relevant critical insights that can then be used to provide better care. This is the purpose of healthcare data analysis: using data-driven findings to predict and solve a problem before it is too late, but also assess methods and treatments faster, keep better track of inventory, involve patients more in their own health, and empower them with the tools to do so.
24 Big Data Applications In Healthcare

Now that you understand the importance of big data in the healthcare industry let’s explore 24 real-world applications that demonstrate how an analytical approach can improve processes, enhance patient care, and, ultimately, save lives.
1) Patients Predictions For Improved Staffing
For our first example of big data in healthcare, we will look at one classic problem that any shift manager faces: how many people do I put on staff at any given period? If you put on too many workers, you run the risk of having unnecessary labor costs add up. With too few workers, you can have poor customer service outcomes – which can be fatal for patients in that industry.

Big data is helping to solve this problem, at least at a few hospitals in Paris. A white paper by Intel details how four hospitals that are part of the Assistance Publique-Hôpitaux de Paris have been using data from a variety of sources to come up with daily and hourly predictions of how many patients are expected to be at each facility.
One of the key data sets is 10 years’ worth of hospital admissions records, which data scientists crunched using “time series analysis” techniques. These analyses allowed the researchers to see relevant patterns in admission rates. Then, they could use machine learning to find the most accurate algorithms that predicted future admissions trends.
Summing up the product of all this work, the data science team developed a web-based user interface that forecasts patient loads and helps in planning resource allocation by utilizing online data visualization that reaches the goal of improving the overall patients' care.
2) Electronic Health Records (EHRs)
It’s the most widespread application of big data in medicine. Every person has their own digital record, which includes demographics, medical history, allergies, laboratory test results, etc. Records are shared via secure information systems and are available for providers from both the public and private sectors. Every record is comprised of one modifiable file, which means that doctors can implement changes over time with no paperwork and no danger of data replication.
EHRs can also trigger warnings and reminders when a patient should get a new lab test or track prescriptions to see if he or she has been following doctors’ orders.
Although EHR is a great idea, many countries still struggle to implement them fully. U.S. has made a major leap, with 94% of hospitals adopting EHRs according to this HITECH research, but the EU still lags behind. However, an ambitious directive drafted by the European Commission is supposed to change it .
Kaiser Permanente is leading the way in the U.S. and could provide a model for the EU to follow. They’ve fully implemented a system called HealthConnect that shares data across all of their facilities and makes it easier to use EHRs. A McKinsey report on big data healthcare analytics states that “The integrated system has improved outcomes in cardiovascular disease and achieved an estimated $1 billion in savings from reduced office visits and lab tests.”
3) Real-Time Alerting
Other examples of data analytics in healthcare share one crucial functionality – real-time alerting. In hospitals, Clinical Decision Support (CDS) software analyzes medical data on the spot, providing health practitioners with advice as they make prescriptive decisions.
However, doctors want patients to stay away from hospitals to avoid costly in-house treatments. This is already trending as one of the business intelligence buzzwords in 2021 and has the potential to become part of a new strategy. Wearables will collect patients’ health data continuously and send this data to the cloud.
Additionally, this information will be accessed to the database on the state of health of the general public, which will allow doctors to compare this data in a socio-economic context and modify the delivery strategies accordingly. Institutions and care managers will use sophisticated tools to monitor this massive data stream and react every time the results will be disturbing.
For example, if a patient’s blood pressure increases alarmingly, the system will send a live alert to the doctor, who will then take action to reach the patient and administer measures to lower the pressure.
Another example is that of Asthmapolis, which has started to use inhalers with GPS-enabled trackers in order to identify asthma trends both on an individual level and looking at larger populations. This data is being used in conjunction with data from the CDC in order to develop better treatment plans for asthmatics.
4) Enhancing Patient Engagement
Many consumers – and hence, potential patients – already have an interest in smart devices that record every step they take, their heart rates, sleeping habits, etc., on a permanent basis. All this vital information can be coupled with other trackable data to identify potential health risks lurking . Chronic insomnia and an elevated heart rate can signal a risk for future heart disease, for instance. Patients are directly involved in the monitoring of their own health, and incentives from health insurance can push them to lead a healthy lifestyle (e.g., giving money back to people using smartwatches).
Another way to do so comes with new wearables under development, tracking specific health trends and relaying them to the cloud where physicians can monitor them. Patients suffering from asthma or blood pressure could benefit from it, become a bit more independent and reduce unnecessary visits to the doctor.
5) Prevent Opioid Abuse In The US
Our fifth example of big data healthcare is tackling a serious problem in the US. Here’s a sobering fact: as of this year, overdoses from misused opioids have caused more accidental deaths in the U.S. than road accidents, which were previously the most common cause of accidental death.
Analysis expert Bernard Marr writes about the problem in a Forbes article . The situation has gotten so dire that Canada has declared opioid abuse to be a “national health crisis,” and President Obama earmarked $1.1 billion dollars for developing solutions to the issue while he was in office.
Once again, an application of big data analytics in healthcare might be the answer everyone is looking for: data scientists at Blue Cross Blue Shield have started working with analytical experts at Fuzzy Logix to tackle the problem. Using years of insurance and pharmacy data, Fuzzy Logix analysts have been able to identify 742 risk factors that predict with a high degree of accuracy whether someone is at risk for abusing opioids.

To be fair, reaching out to people identified as “high risk” and preventing them from developing a drug issue is a delicate undertaking. However, this project still offers a lot of hope for mitigating an issue that is destroying the lives of many people and costing the system a lot of money.
6) Using Health Data For Informed Strategic Planning
The use of big data in healthcare allows for strategic planning thanks to better insights into people’s motivations. Care managers can analyze check-up results among people in different demographic groups and identify what factors discourage people from taking up treatment.
The University of Florida made use of Google Maps and free public health data to prepare heat maps targeted at multiple issues, such as population growth and chronic diseases. Subsequently, academics compared this data with the availability of medical services in most heated areas. The insights gleaned from this allowed them to review their delivery strategy and add more care units to the most problematic areas.
7) Big Data Might Just Cure Cancer
Another interesting example of the use of big data in healthcare is the Cancer Moonshot program. Before the end of his second term, President Obama came up with this program that had the goal of accomplishing 10 years’ worth of progress toward curing cancer in half that time.
Medical researchers can use large amounts of data on treatment plans and recovery rates of cancer patients in order to find trends and treatments that have the highest rates of success in the real world. For example, researchers can examine tumor samples in biobanks that are linked up with patient treatment records. Using this data, researchers can see things like how certain mutations and cancer proteins interact with different treatments and find trends that will lead to better outcomes.
This data can also lead to unexpected benefits, such as finding that Desipramine, which is an antidepressant, has the ability to help cure certain types of lung cancer .
However, in order to make these kinds of insights more available, patient databases from different institutions, such as hospitals, universities, and nonprofits, need to be linked up. Then, for example, researchers could access patient biopsy reports from other institutions. One of the potential big data use cases in healthcare would be genetically sequencing cancer tissue samples from clinical trial patients and making these data available to the wider cancer database.
But, there are a lot of obstacles in the way, including:
- Incompatible data systems. This is perhaps the biggest technical challenge, as making these data sets able to interface with each other is quite a feat.
- Patient confidentiality issues. There are differing laws state by state which govern what patient information can be released with or without consent, and all of these would have to be navigated.
- Simply put, institutions that have put a lot of resources and money into developing their own cancer dataset may not be eager to share it with others, even though it could lead to a cure much more quickly.
However, as an article by Fast Company states, there are precedents to navigating these types of problems and roadblocks while accelerating progress toward curing cancer using the strength of data analysis.
8) Predictive Analytics In Healthcare
We have already recognized predictive analysis as one of the biggest business intelligence trends for two years in a row, but the potential applications reach far beyond business and much further into the future. Optum Labs, a US research collaborative, has collected EHRs of over 30 million patients to create a database for predictive analysis tools that will improve the delivery of care.
The goal of healthcare online business intelligence is to help doctors make data-driven decisions within seconds and improve patients’ treatment. This is particularly useful in the case of patients with complex medical histories suffering from multiple conditions. New BI solutions and tools would also be able to predict, for example, who is at risk of diabetes and thereby be advised to make use of additional screenings or weight management.
9) Reduce Fraud And Enhance Security
Some studies have shown that 93% of healthcare organizations have experienced a data breach. The reason is simple: personal data is extremely valuable and profitable on the black market. And any breach would have dramatic consequences. With that in mind, many organizations started to use analytics to help prevent security threats by identifying changes in network traffic or any other behavior that reflects a cyber-attack. Of course, big data has inherent security issues, and many think that using it will make organizations more vulnerable than they already are. But advances in security, such as encryption technology, firewalls, anti-virus software, etc., answer the need for more security, and the benefits brought largely overtake the risks.
Likewise, it can help prevent fraud and inaccurate claims in a systemic, repeatable way. Analytical tools help to streamline the processing of insurance claims, enabling patients to get better returns on their claims and caregivers to be paid faster. For instance, the Centers for Medicare and Medicaid Services said they saved over $210.7 million in fraud in just a year.
10) Telemedicine
Telemedicine has been present on the market for over 40 years, but only today, with the arrival of online video conferences, smartphones, wireless devices, and wearables, has it been able to come into full bloom. The term refers to the delivery of remote clinical services using technology.
It is used for primary consultations and initial diagnosis, remote patient monitoring, and medical education for health professionals. Some more specific uses include telesurgery – doctors can perform operations with the use of robots and high-speed real-time data delivery without physically being in the same location as a patient.
Clinicians use telemedicine to provide personalized treatment plans and prevent hospitalization or re-admission. Such use of healthcare data analytics can be linked to the use of predictive analytics, as seen previously. It allows clinicians to predict acute medical events in advance and prevent the deterioration of patients’ conditions.
By keeping patients away from hospitals, telemedicine helps to reduce costs and improve the quality of service. Patients can avoid waiting in lines, and doctors don’t waste time on unnecessary consultations and paperwork. Telemedicine also improves the availability of care as patients’ states can be monitored and consulted anywhere and anytime.
11) Integrating Big-Style Data With Medical Imaging
Medical imaging is vital, and each year in the US, about 600 million imaging procedures are performed. Analyzing and storing these images manually is expensive both in terms of time and money, as radiologists need to examine each image individually, while hospitals need to store them for several years.
Medical imaging provider Carestream explains how big data analytics for healthcare could change how images are read: algorithms developed analyzing hundreds of thousands of images could identify specific patterns in the pixels and convert them into a number to help the physician with the diagnosis. They even go further, saying that it could be possible that radiologists will no longer need to look at the images but instead analyze the outcomes of the algorithms that will inevitably study and remember more images than they could in a lifetime. This would undoubtedly impact the role of radiologists, their education, and the required skillset.
12) A Way To Prevent Unnecessary ER Visits
Saving time, money, and energy using big data analytics for healthcare is necessary. What if we told you that over the course of 3 years, one woman visited the ER on more than 900 occasions? That situation is a reality in Oakland, California, where a woman who suffers from mental illness and substance abuse went to a variety of local hospitals on an almost daily basis.
This woman’s issues were exacerbated by the lack of shared medical records between local emergency rooms, increasing the cost to taxpayers and hospitals and making it harder for this woman to get good care. As Tracy Schrider, who coordinates the care management program at Alta Bates Summit Medical Center in Oakland, stated in a Kaiser Health News article :
“Everybody meant well. But she was being referred to three different substance abuse clinics and two different mental health clinics, and she had two case management workers both working on housing. It was not only bad for the patient, it was also a waste of precious resources for both hospitals.”
In order to prevent future situations like this from happening, Alameda County hospitals came together to create a program called PreManage ED, which shares patient records between emergency departments.
This system lets the ER staff know things like:
- If the patient they are treating has already had certain tests done at other hospitals, what the results of those tests are.
- If the patient in question already has a case manager at another hospital, prevent unnecessary assignments.
- What advice has already been given to the patient so that a coherent message to the patient can be maintained by providers?
This is another great example where the application of healthcare data analysis is useful and needed. In the past, hospitals without PreManage ED would repeat tests over and over, and even if they could see that a test had been done at another hospital, they would have to go old school and request or send long fax just to get the information they needed.
13) Smart Staffing & Personnel Management
Without a cohesive, engaged workforce, patient care will dwindle, service rates will drop, and mistakes will happen. But with big data tools in healthcare, it’s possible to streamline your staff administration activities in a wealth of key areas. By working with the right HR analytics , it’s possible for time-stretched medical institutions to optimize staffing while forecasting operating room demands, streamlining patient care as a result.
Too often, there is a significant lack of fluidity in healthcare institutions, with staff distributed in the wrong areas at the wrong time. This imbalance of personnel administration could mean a particular department is either too overcrowded with staff or lacking staff when it matters most, which can develop risks of lower motivation for work and increases the absenteeism rate. An HR dashboard , in this case, may help:

**click to enlarge**
Through data-driven analytics, it’s possible to predict when you might need staff in particular departments at peak times while distributing skilled personnel to other areas within the institution during quieter periods.
Moreover, medical data analysis will empower senior staff or operatives to offer the right level of support when needed, improve strategic planning, and make vital staff and personnel management processes as efficient as possible.
14) Learning & Development
Expanding on our previous point, in a hospital or medical institution, the skills, confidence, and abilities of your staff can mean the difference between life and death. Naturally, doctors and surgeons are highly skilled in their areas of expertise. But most medical institutions have a range of people working under one roof, from porters and admin clerks to cardiac specialists and brain surgeons.
In healthcare, soft skills are almost important as certifications . To keep the institution running at optimum capacity, you have to encourage continual learning and development. By keeping track of employee performance across the board while keeping a note of training data, you can use healthcare data analysis to gain insight into who needs support or training and when. If everyone is able to evolve with the changes around them, you will save more lives — and medical data analytics will help you do just that.
15) Advanced Risk & Disease Control
Big data and healthcare analytics are essential for tackling the hospitalization risk for specific patients with chronic diseases. It can also help prevent deterioration.
By drilling down into insights such as medication type, symptoms, and the frequency of medical visits, among many others, it’s possible for healthcare institutions to provide accurate preventative care and, ultimately, reduce hospital admissions. Not only will this level of risk calculation result in reduced spending on in-house patient care, but it will also ensure that space and resources are available for those who need it most. This is a clearcut example of how analytics in healthcare can improve and save people’s lives.
As a result, big data for healthcare can improve the quality of patient care while making the organization more economically streamlined in every key area.
16) Suicide & Self-Harm Prevention
Globally, almost 800,000 people die from suicide every year. Plus, 17% of the world’s population will self-harm during their lifetime. These numbers are alarming. But while this is a very difficult area to tackle, big data uses in healthcare are helping to make a positive change concerning suicide and self-harm. As entities that see a wealth of patients every single day, healthcare institutions can use data analysis to identify individuals that might be likely to harm themselves.
In a 2018 study from KP and the Mental Health Research Network, a mix of EHR data and a standard depression questionnaire identified individuals who had an enhanced risk of a suicide attempt with great accuracy. Utilizing a predictions algorithm, the team found that suicide attempts and successes were 200 times more likely among the top 1% of patients flagged according to specific datasets. Speaking on the subject, Gregory E. Simon, MD, MPH, a senior investigator at Kaiser Permanente Washington Health Research Institute, explained:
“We demonstrated that we can use electronic health record data in combination with other tools to accurately identify people at high risk for suicide attempt or suicide death.”
This essential use case for big data in the healthcare industry really is a testament to the fact that medical analytics can save lives.
“If somebody tortures the data enough (open or not), it will confess anything.” – Paolo Magrassi, former vice president, research director, Gartner.
17) Improved Supply Chain Management
If a medical institution’s supply chain is weakened or fragmented, everything else is likely to suffer, from patient care and treatment to long-term finances and beyond. That said, the next in our big data in healthcare examples focuses on the value of analytics to keep the supply chain fluent and efficient from end to end.
Leveraging analytical tools to track the supply chain performance metrics and make accurate, data-driven decisions concerning operations as well as spending can save hospitals up to $10 million per year .
Both descriptive and predictive models can enhance decisions for negotiating prices, reducing the variation in supplies, and optimizing the ordering process as a whole. By doing so, medical institutions can thrive in the long term while delivering vital treatment to patients without potentially disastrous delays, snags, or bottlenecks.
18) Financial facility management
As you’ve learned by now, data plays a crucial role in today’s society. It has permeated industries across the world to assist in the development of new technologies and innovations. This is especially true in the business landscape, where data-driven initiatives have become a requirement rather than a choice. This is no different in the healthcare industry, which leads us to our next big data application.
So far, we’ve covered multiple applications related to patients and medical innovation. That said, all of these breakthroughs would not be possible if the finances of the different health organizations wouldn’t be on check. Big data in healthcare provides the necessary tools and knowledge to ensure optimal financial health across facilities, especially for big hospital networks that manage multiple facilities simultaneously.
When we talk about the financial side of the healthcare industry, we’ll encounter similar KPIs as any other organization, such as net profit, cash flow, etc. However, there are some financial indicators that are unique to this sector. We are talking about drug costs, claims denial rates, reimbursement rates, and much more. Keeping track of all those indicators is not an easy task; however, organizations that invest in software to collect, arrange and analyze their data will ensure their finances are in check and the facility remains profitable while providing the best care.
The example below is a hospital network dashboard generated with datapine’s dashboard designer . The template provides a strategic overview of a private hospital network with insights into multiple facilities. With these insights in hand, decision-makers can make informed decisions and spot improvement opportunities to boost their financial efficiency.

19) Developing New Therapies & Innovations
The next in our healthcare analytics examples centers on working for a brighter, bolder future in the medical industry. Big data analysis in healthcare has the power to assist in new therapy and innovative drug discoveries. By utilizing a mix of historical, real-time, and predictive metrics as well as a cohesive mix of data visualization techniques , healthcare experts can identify potential strengths and weaknesses in trials or processes.
Moreover, through data-driven genetic information analysis as well as reactionary predictions in patients, big data analytics in healthcare can play a pivotal role in the development of groundbreaking new drugs and forward-thinking therapies. Data analytics in healthcare can streamline, innovate, provide security, and save lives. It gives confidence and clarity, and it is the way forward.
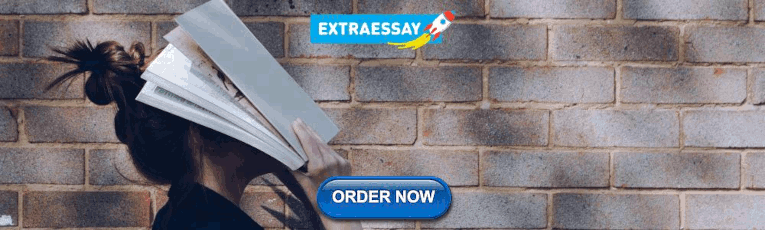
20) Help To Manage And Track Mass Diseases
Since it started at the beginning of the year 2020, the COVID-19 pandemic has impacted millions and millions of people around the world. The widespread nature of this virus presented a challenge to the health industry, which found itself trying to learn from it and control it at the same time. In that context, big data played a fundamental role in the response given to this growing disease that made the world stop for years.
Supported by advanced data management technologies, health experts were able to track in real-time how COVID was spreading, how fast it mutated under different conditions, as well as the effect it was having on the different world economies. This is done by analyzing massive data sets coming from diverse sources, such as medical records as well as individual human behaviors. For instance, looking at how many of them are staying at home, getting on the train, or going to school, as this highly influences how fast the virus spreads.
Paired to this, technologies such as AI allowed various medical imaging modalities, such as X-rays, tomography, ultrasounds, and others, to provide an earlier diagnosis to patients and prevent the spread of the disease. In fact, in 2020, the EU backed a software called InferRead to facilitate this process. Essentially, this AI-powered software “analyses images of the lungs taken by a CT scanner, identifies the signs of coronavirus, and assesses the lesions. The process would typically require a careful study by an experienced doctor, yet the machine needs only a few seconds”. This level of technology allowed hospitals around Europe to prevent the spread of the virus and, consequently, flatten the curve.
21) Improved Drug Prescription Processes
As seen throughout our list of examples, the vast amount of data that care professionals have at their fingertips has helped revolutionize patient care, disease control, and many others. That said, it is not a surprise that this trend has also spread into the pharmaceutical industry, where it promises to maximize the value of medicines and improve the quality of prescribing. This leads us to our next big data application in healthcare.
One of the companies applying it is Express Scripts, a third-party organization that manages medicines coverage for clients who provide health insurance plans. According to a publication from the Pharmaceutical Journal, the US-based company collected data from 83 million patients, which included everything from patients' clinical and behavioral characteristics, such as someone picking up a prescription, to Tweets directed at the company. With this data in hand, Express Scripts can predict important scenarios, such as who is likely to become addicted to a certain medication or who is not adhering to its treatment. If the last happens, the company immediately sends personalized interventions and support to make sure people adhere to their prescribed treatment. As a result, Express Scripts stated that they cut the non-adherence rate of hepatitis C patients from 8,3% to 4,8%.
22) Prevent Human Error
Fraud in the health industry can be considered anything from erroneous billings to inefficiencies that result in wasteful tests or even adding incorrect information to a person's medical record. In fact, the National Health Care Anti-Fraud Association estimates that the financial losses due to healthcare fraud could reach $300 billion in the US alone. This means that around 10% of the total health spending is wasted due to fraud or human error. However, money is not even the biggest concern when it comes to human error and fraud, it's patients’ lives that are put at risk. Prescribing someone with the wrong medicine or treatment can result in long-lasting consequences or even death.
To avoid this, companies leverage big data and forecasting to identify and prevent fraud or human error quickly. For example, by analyzing massive amounts of prescription patterns, experts are able to detect prescription errors before they occur. The same happens with dosage ranges, tests, and other procedures. In time, this not only allows doctors and caregivers to trust in technology for their decision-making but also saves facilities a big amount of money while providing the best care.
23) Alerting heart problems through personal devices
In 2017, Apple and Stanford Medicine joined forces to carry out one-of-a-kind research called “Apple Heart Study.” They studied the heart rate sensor data of 400.000 Apple Watch users to see if wearable devices could help in detecting irregular heart rates to prevent fatal complications, especially atrial fibrillation (AFib).
AFib is among the leading causes of strokes across the world. Costing the life of approximately 130.000 people in the US every year, with another 750.000 hospitalizations. That’s because patients that are suffering from AFib often don’t experience any symptoms, so the condition can go unnoticed until it is fatal.
According to Jeff Williams, Apple’s COO, the motivation to carry out this study was their own customer's testimonies. At the time, he said: “Every week, we receive incredible customer letters about how Apple Watch has affected their lives, including learning that they have AFib. These stories inspire us, and we're determined to do more to help people understand their health”
Apple and Standford Medicine created an app where any Apple Watch users in the US that were over the age of 22 could join the study. If an irregular heart rate were identified, the user would get a notification and a free doctor consultation with an electrocardiogram (ECG) patch for additional monitoring. After 8 months of testing using top-notch technology, in 2019, the results of the study were published.
Stanford Medicine found that only 0.5% of participants had irregular heart rate notifications, a positive result overall. Of the participants that did receive the notification, 84% were diagnosed with atrial fibrillation at the time of the notification. 34% of the participants that received a notification and followed up with an ECG patch were finally diagnosed with AFib. This last result was not surprising, as AFib can often go undetected.
Lastly, the study showed that comparisons between Apple Watch pulse detection and ECG patches pulse detection have a 71% positive predictive value. Showing that these devices can help people take charge of their health and, in some cases, save lives. A great testament to the power of big data in healthcare.
24) Bluetooth helps asthma patients
According to the WHO, Asthma affected an estimated 262 million people in 2019 and caused 455 000 deaths globally. People who suffer from asthma can be triggered even by invisible particles in the air, making it a complicated disease to carry.
With those concerning numbers in mind, a Wisconsin-based digital health company called Propeller Health launched a Bluetooth-powered inhaler that can be connected to a person’s smartphone to collect data about the frequency of asthma attacks and the environmental conditions in which the person was located.
Once the inhaler is pressed, data about the time and location of the patient’s asthma attack is sent to an app on the phone via a Bluetooth sensor. Doctors can use this data later on to analyze the frequency of the attacks as well as any other factors such as location and weather. Plus, the inhaler is also set to help patients with their medication schedules since taking medication correctly and when expected can significantly decrease the chances of ending up in the emergency room for an attack.
In fact, research carried out by Propeller Health showed that 330 patients who had attached the Bluetooth sensor to their inhaler experienced 60% fewer asthma-related emergency hospital visits. The company also found that collecting data like this for patients that live in the same town or region can prove even more valuable. Another test of their Bluetooth inhaler sensor showed that in Louisville, Kentucky, asthma attacks were mostly caused by proximity to railroads and utilities. Plus, they found multiple asthma triggers in public areas. The data collected by the sensor is highly valuable to the city as health authorities can use it to prevent asthma from becoming a major issue for their population.
How To Use Big Data In Healthcare?
All in all, we’ve noticed three key trends through these 24 examples of healthcare analytics: the patient experience will improve dramatically, including quality of treatment and satisfaction levels; the overall health of the population can also be enhanced on a sustainable basis, and operational costs can be reduced significantly.
Let’s have a look now at concrete examples of big data in healthcare:
a) Big Data In Healthcare Applied On A Hospital Dashboard
This healthcare dashboard below provides you with the overview needed as a hospital director or as a facility manager. Gathering in one central point all the data on every division of the hospital, the attendance, its nature, the costs incurred, etc., you have the big picture of your facility, which will be of great help to run it smoothly.

You can see here the most important metrics concerning various aspects: the number of patients that were welcomed in your facility, how long they stayed and where, how much it cost to treat them, and the average waiting time in emergency rooms. Such a holistic view helps top administrators to identify potential bottlenecks, spot trends and patterns over time, and in general, assess the situation. This is key in order to make better-informed decisions that will improve the overall operations performance, with the goal of treating patients better and having the right staffing resources.
b) Big Data Healthcare Application On Patients' Care
Another real-world application of healthcare big data analytics, our dynamic patient KPI dashboard , is a visually-balanced tool designed to enhance service levels as well as treatment accuracy across departments.

By offering a perfect storm or patience-centric information in one central location, medical institutions can create harmony between departments while streamlining care processes in a wealth of vital areas. For instance, bed occupancy rate metrics offer a window of insight into where resources might be required while tracking canceled or missed appointments will give senior executives the data they need to reduce costly patient no-shows.
Here, you will find everything you need to enhance your level of patient care both in real-time and in the long term. This is a visual innovation that has the power to improve every type of medical institution, big or small.
Why We Need Big Data Analytics In Healthcare
By looking at our list of most insightful medical big data applications, you should have a notion of how positive the use of analytics can be for this industry. If this is not clear yet, here we will summarize the main points of importance by listing a few benefits of big data in healthcare.
As mentioned, there’s a huge need for big data in healthcare, especially due to rising costs in nations like the United States. As a McKinsey report states: “After more than 20 years of steady increases, healthcare expenses now represent 17.6 percent of GDP — nearly $600 billion more than the expected benchmark for a nation of the United States’s size and wealth.” This quote leads us to our first benefit.
Reducing costs
As stated above, costs are much higher than they should be, and they have been rising for the past 20 years. Clearly, we are in need of some smart, data-driven thinking in this area. And current incentives are changing as well: many insurance companies are switching from fee-for-service plans (which reward using expensive and sometimes unnecessary treatments and treating large amounts of patients quickly) to plans that prioritize patient outcomes
As the authors of the popular Freakonomics books have argued, financial incentives matter – and incentives that prioritize patients' health over treating large amounts of patients are a good thing. Why does this matter?
Well, in the previous scheme, healthcare providers had no direct incentive to share patient information with one another, which made it harder to utilize the power of analytics. Now that more of them are getting paid based on patient outcomes, they have a financial incentive to share data that can be used to improve the lives of patients while cutting costs for insurance companies.
Reducing medical errors
Physician decisions are becoming more and more evidence-based, meaning that they rely on large swathes of research and clinical data as opposed to solely their schooling and professional opinion. That said, the risk of human error is always a latent threat. Even though doctors are highly trained professionals, they are still human, and the risk of selecting the wrong medication or treatment can potentially risk a person’s life. With the use of big data and the tools that we mentioned throughout this post, professionals can be easily alerted when the wrong medication, test, treatment, or other has been provided and remediate it immediately. In time, this can significantly reduce the rates of medical errors and improve the facility’s reputation.
As in many other industries, data gathering and management are getting bigger, and professionals need help in the matter. This new treatment attitude means there is a greater demand for big data analytics in healthcare facilities than ever before, and the rise of SaaS BI tools is also answering that need.
Optimizing organizational and personnel management
While using data to ensure you are providing the best care to patients is fundamental, there are also other operational areas in which it can assist the health industry. Part of providing quality care is ensuring the facility works optimally, and this can also be achieved with the help of big data.
By using the right BI software , professionals can gather and analyze real-time data about the performance of their organization in areas such as operations and finances, as well as personnel management. For instance, predictive analytics technologies can provide relevant information regarding admission rates. These insights can help define staffing schedules to cover demand as well as inventory for medical supplies. This way, care facilities can stay one step ahead and ensure that patients are getting the best experience possible.
Getting this level of insight in such an intuitive way allows managers to redirect resources where they are most needed and optimize areas that are not performing well to ensure the best return on investment possible.
Drive innovation and growth
Our last benefit is one that should be the clearest from the list of applications we provided earlier. The use of big data in the care industry enables professionals to test new technologies, drugs, and treatments to improve the quality of care given to patients and battle diseases that were once thought of as unbeatable.
Thanks to wearable devices that can tell your heart rate, Bluetooth asthma inhalers that gather insights to prevent attacks, and much more, doctors are able to use data to understand how common diseases work and how certain external factors might be affecting entire communities. Through that, they are able to provide personalized quality care to each and every person that goes into a hospital.
There is no denying that the power of big data analytics is saving lives. That being said, the process of managing data requires a lot of effort, and with that comes challenges, which we will discuss below.
Obstacles To A Widespread Big Data Healthcare
The points mentioned above are just a few of the countless benefits of big data in healthcare. That said, with benefits also come limitations. In order to provide a full picture of the topic, we will now list a few obstacles and healthcare data challenges that organizations can face when implementing analytics into their processes.
Data integration and storage: One of the biggest hurdles standing in the way of using big data in medicine is how medical data is spread across many sources governed by different states, hospitals, and administrative departments. The integration of these data sources would require developing a new infrastructure where all data providers collaborate with each other.
Data sharing: Equally important is implementing new online reporting software and business intelligence strategy that will allow all relevant users to be connected with the data. Healthcare needs to catch up with other industries that have already moved from standard regression-based methods to more future-oriented ones like predictive analytics, machine learning, and graph analytics. This is done with the help of modern reporting tools such as a dashboard creator that allows anyone to perform advanced analytics with just a few clicks easily.
Security and privacy: Security and privacy are constant concerns are one of the biggest challenges of big data in healthcare. Daily, hospitals and care centers deal with sensitive patient data that needs to be carefully protected. Taking that into consideration with the fact that the data is coming from many different sources, security can present a challenge for these types of organizations. To avoid this, it is critical to follow law regulations, conduct regular audits to ensure everything is going well, and train employees on data protection best practices.
Data literacy: Using big data and analytics in healthcare involves many processes and tools to collect, clean, process, manage, and analyze the huge amounts of data available. This requires a level of knowledge and skills that can present a limitation for average users that are not acquainted with these processes. However, while data literacy might have been one of the big disadvantages of big data in healthcare, it is no longer the case. In the past years, various self service BI tools have been developed with the purpose of opening the analytical doors to any type of use without the need for any technical knowledge.
So, even if these analytical services are not your cup of tea, you are a potential patient, and so you should care about new healthcare analytics applications. Besides, it’s good to take a look around sometimes and see how other industries cope with it. They can inspire you to adapt and adopt some good ideas.
24 Big Data Examples In Healthcare - A Summary
The industry is changing, and like any other, big-style data is starting to transform it – but there is still a lot of work to be done. The sector slowly adopts the new technologies that will push it into the future, helping it to make better-informed decisions, improve operations, etc. In a nutshell, here’s a short list of the examples we have gone over in this article. With healthcare data analytics, you can:
- Predict the daily patients' income to tailor staffing accordingly
- Use Electronic Health Records (EHRs)
- Use real-time alerting for instant care
- Help in preventing opioid abuse in the US
- Enhance patient engagement in their own health
- Use health data for a better-informed strategic planning
- Research more extensively to cure cancer
- Use predictive analytics
- Reduce fraud and enhance data security
- Practice telemedicine
- Integrate medical imaging for a broader diagnosis
- Prevent unnecessary ER visits
- Smart staffing & personnel management
- Learning & development
- Advanced risk & disease management
- Suicide & self-harm prevention
- Improved supply chain management
- Financial facility management
- Developing new therapies & innovations
- Track and control mass diseases
- Improve the prescription process
- Mitigate human error
- Apple can alert people about heart problems
- Bluetooth helps asthma patients
“Most of the world will make decisions by either guessing or using their gut. They will be either lucky or wrong.” – Suhail Doshi, chief executive officer, Mixpanel.
These 24 real-world examples of data analytics in healthcare prove that medical applications can save lives and should be a top priority of experts across the field. Even now, data-driven analytics facilitates early identification as well as intervention in illnesses while streamlining institutions for swifter, safer, and more accurate patient care. As technology evolves, these invaluable functions can only get stronger – the future of healthcare is here, and it lies in data.
Want to take your healthcare institution to the next level? Start building your own analysis and reports, and improve your healthcare data management with datapine's 14-day free trial !
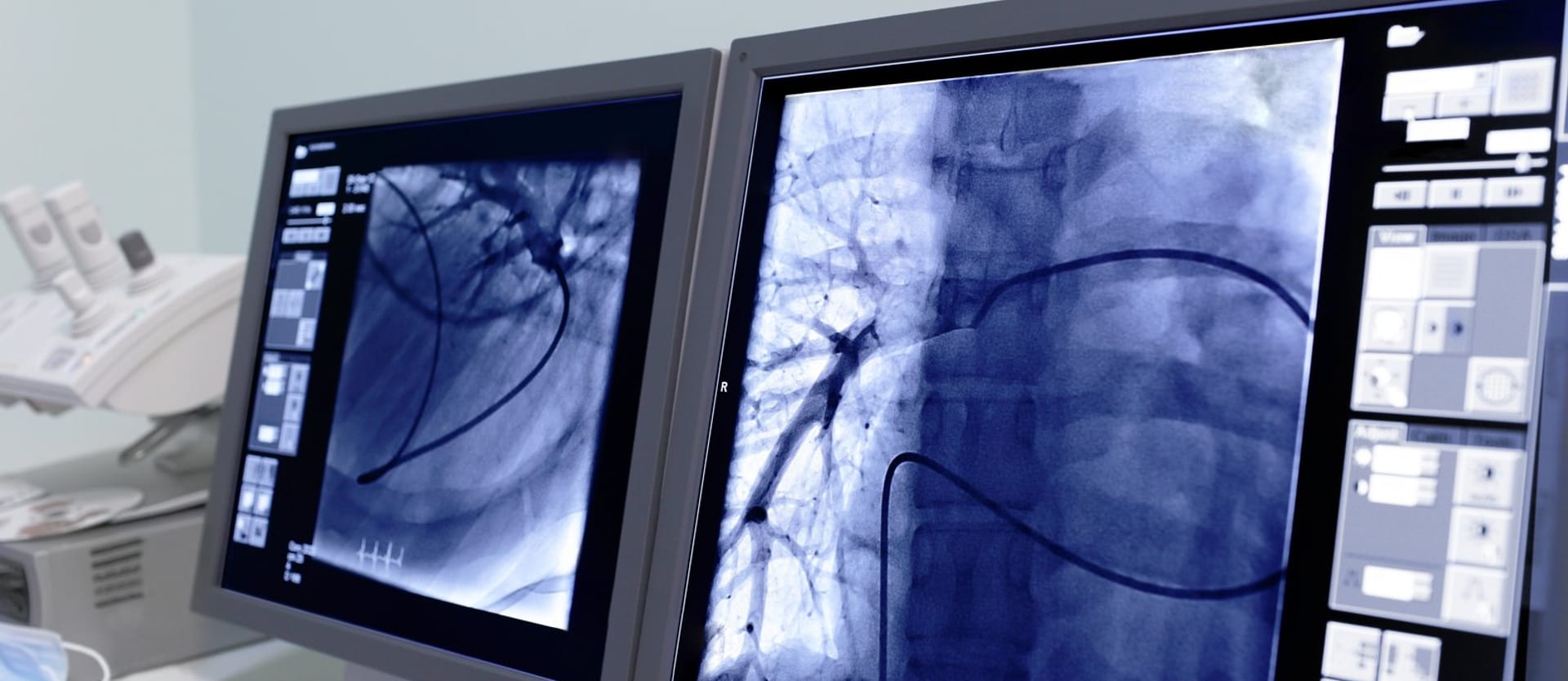
Data Analytics in Healthcare: 7 Real-World Examples and Use Cases
- Data Science , Healthcare
- 31 Aug, 2020
- No comments Share
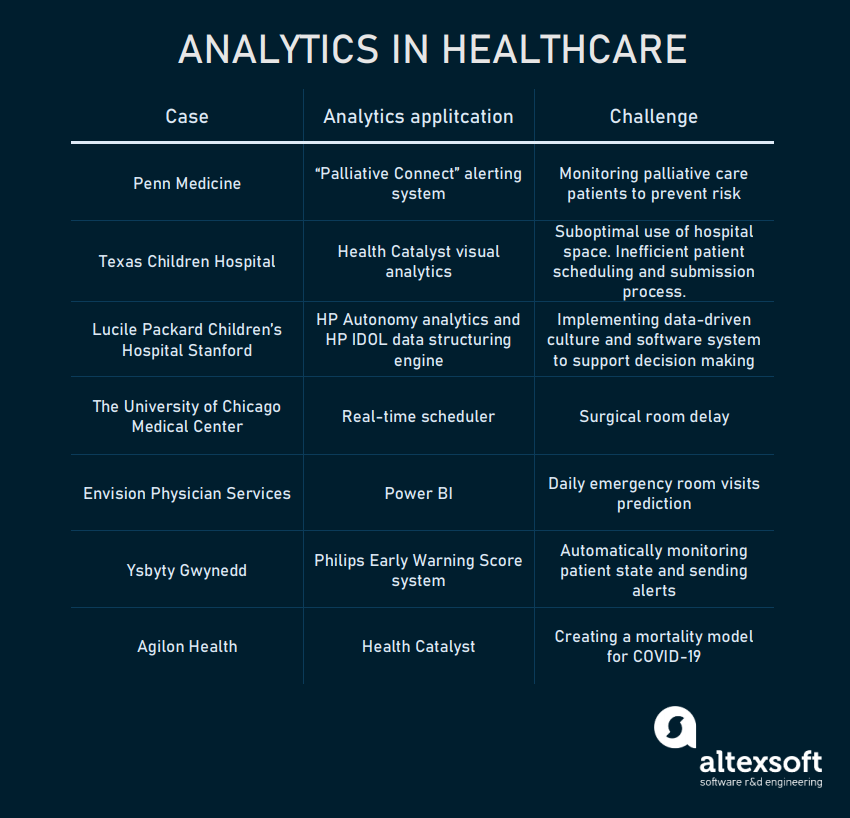
Analytics application cases in healthcare
Predicting palliative care patients risk: Penn Medicine
Optimization of clinical space usage: texas children's hospital.
- An online scheduling tool was leveraged to allow self-scheduling through the web.
- The hospital also established a template for allocating scheduling time in four-hour blocks. Appointments of different duration were allocated to different time blocks. All the unfilled appointments were distributed in a 72-hour time zone to close the gap.
- Weekend appointments and extended hospital hours were added.
- An annual revenue increased by $8.3 million with 53 thousand appointments respectively
- 30 thousand online schedules
- 39 percent patient satisfaction rate growth
Applying machine learning to predict operation duration and disease risk probability: Lucile Packard Children’s Hospital Stanford
- Identify patients at clinical decline risk
- Prevent central line-associated bloodstream infections
- Predict surgical operation duration
Operation room delay reduction: The University of Chicago Medical Center
Daily emergency room visits prediction: envision physician services, monitoring patient state deterioration: ysbyty gwynedd, leveraging data to create covid-19 mortality model: agilon health.
- create a COVID-19 model for approximately 125,000 individuals that were assigned with risk scores.
- increase one partner location’s telehealth appointments from none in the first week to 2,200 in weeks 12 and 13, aligning with social distancing and overall pandemic policies.
What are the other opportunities of data analytics in healthcare?
Big data in healthcare - the promises, challenges and opportunities from a research perspective: A case study with a model database
Affiliations.
- 1 Regenstrief Center for Healthcare Engineering, Purdue University, West Lafayette, Indiana, USA.
- 2 Children's Health Services Research Group, Department of Pediatrics, Indiana University School of Medicine, Indianapolis, USA.
- PMID: 29854102
- PMCID: PMC5977694
Recent advances in data collection during routine health care in the form of Electronic Health Records (EHR), medical device data (e.g., infusion pump informatics, physiological monitoring data, and insurance claims data, among others, as well as biological and experimental data, have created tremendous opportunities for biological discoveries for clinical application. However, even with all the advancement in technologies and their promises for discoveries, very few research findings have been translated to clinical knowledge, or more importantly, to clinical practice. In this paper, we identify and present the initial work addressing the relevant challenges in three broad categories: data, accessibility, and translation. These issues are discussed in the context of a widely used detailed database from an intensive care unit, Medical Information Mart for Intensive Care (MIMIC III) database.
- Confidentiality
- Data Collection
- Databases, Factual*
- Electronic Health Records*
- Health Information Interoperability
- Intensive Care Units*
Advertisement
Medical Big Data Warehouse: Architecture and System Design, a Case Study: Improving Healthcare Resources Distribution
- Transactional Processing Systems
- Published: 19 February 2018
- Volume 42 , article number 59 , ( 2018 )
Cite this article
- Abderrazak Sebaa ORCID: orcid.org/0000-0002-8742-1240 1 ,
- Fatima Chikh 2 ,
- Amina Nouicer 1 &
- AbdelKamel Tari 1
3344 Accesses
38 Citations
1 Altmetric
Explore all metrics
The huge increases in medical devices and clinical applications which generate enormous data have raised a big issue in managing, processing, and mining this massive amount of data. Indeed, traditional data warehousing frameworks can not be effective when managing the volume, variety, and velocity of current medical applications. As a result, several data warehouses face many issues over medical data and many challenges need to be addressed. New solutions have emerged and Hadoop is one of the best examples, it can be used to process these streams of medical data. However, without an efficient system design and architecture, these performances will not be significant and valuable for medical managers. In this paper, we provide a short review of the literature about research issues of traditional data warehouses and we present some important Hadoop-based data warehouses. In addition, a Hadoop-based architecture and a conceptual data model for designing medical Big Data warehouse are given. In our case study, we provide implementation detail of big data warehouse based on the proposed architecture and data model in the Apache Hadoop platform to ensure an optimal allocation of health resources.
This is a preview of subscription content, log in via an institution to check access.
Access this article
Price includes VAT (Russian Federation)
Instant access to the full article PDF.
Rent this article via DeepDyve
Institutional subscriptions
Similar content being viewed by others
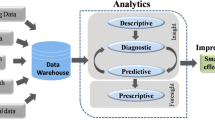
Big data in healthcare: management, analysis and future prospects
Trends and Future Perspective Challenges in Big Data
Big Data Analytics: Applications, Prospects and Challenges
Kuo, M.H., Sahama, T., Kushniruk, A.W., Borycki, E.M., and Grunwell, D.K., Health big data analytics: Current perspectives, challenges and potential solutions. Int. J. Big Data Intell. 1(1–2):114–126, 2014. https://doi.org/10.1504/IJBDI.2014.063835 .
Article Google Scholar
Cuzzocrea, A., Warehousing and Protecting Big Data: State-Of-The-Art-Analysis, Methodologies, Future Challenges. In Proceedings of the International Conference on Internet of things and Cloud Computing (p. 14). ACM, 2016. https://doi.org/10.1145/2896387.2900335
White, T., Hadoop: The definitive guide (third edition). O’Reilly, 2012. ISBN: 978-1-449-322252-0.
Sumathi, S., and Esakkirajan, S., Fundamentals of relational database management systems (Vol. 47). Springer, 2007. ISBN: 978 3 540 48397 7.
Ewen, E.F., Medsker, C.E., and Dusterhoft, L.E., Data warehousing in an integrated health system: building the business case. In Proceedings of the 1st ACM international workshop on Data warehousing and OLAP (pp. 47–53). ACM, 1998. https://doi.org/10.1145/294260.294271
Pedersen, T.B., and Jensen, C.S., Research issues in clinical data warehousing. In Scientific and Statistical Database Management. Proceedings. Tenth international conference on (pp. 43–52). IEEE, 1998. https://doi.org/10.1109/SSDM.1998.688110
Guérin, E., Moussouni, F., Courselaud, B., and Loréal, O., UML modeling of Gedaw: A gene expression data warehouse specialised in the liver. In The 3rd French bioinformatics conference proceeding: JOBIM 2002 (pp. 319–334), Saint-Malo, France, 2002.
Banek, M., Tjoa, A.M., and Stolba, N., Integrating different grain levels in a medical data warehouse federation. In International Conference on Data Warehousing and Knowledge Discovery (pp. 185–194). Springer Berlin Heidelberg, 2006. https://doi.org/10.1007/11823728_18
Kerkri, E.M., Quantin, C., Allaert, F.A., Cottin, Y., Charve, P., Jouanot, F., and Yétongnon, K., An approach for integrating heterogeneous information sources in a medical data warehouse. J. Med. Syst. 25(3):167–176, 2001. https://doi.org/10.1023/A:1010728915998 .
Article CAS PubMed Google Scholar
Pavalam, S.M., Jawahar, M., and Akorli, F.K., Data warehouse based Architecture for Electronic Health Records for Rwanda. In Education and Management Technology (ICEMT) International Conference on (pp. 253–255). IEEE, 2010. https://doi.org/10.1109/ICEMT.2010.5657660
Sebaa, A., Nouicer, A., Tari, A., Ramtani, T., and Ouhab, A., Decision support system for health care resources allocation. Electron. Physician . 9(6):4661–4668, 2017. https://doi.org/10.19082/4661 .
Article PubMed PubMed Central Google Scholar
Sebaa, A., Nouicer, A., Tari, A., Ramtani, T., and Ouhab, A., Decision support system for Health Care Resources allocation. Abstracts Book of ICHSMT’16- International Conference on Health Sciences and Medical Technologies; 2016 Sep 27-29; Tlemcen, Algeria. Mehr publishing. p. 8, 2016. ISBN: 978-600-96661-0-2.
Sebaa, A., Tari, A., Ramtani, T., and Ouhab, A., DW RHSB: A framework for optimal allocation of health resources. Int. J. Comput. Sci. Commun Inf. Technol . 2(1):12–17, 2015.
Google Scholar
Wang, L., and Alexander, C.A., Big data in medical applications and health care. Am. Med. J. 6(1):1, 2015. https://doi.org/10.3844/amjsp.2015.1.8 .
Cuzzocrea, A., Song, I.Y., and Davis, K.C., Analytics over large-scale multidimensional data: the big data revolution. In Proceedings of the ACM 14th international workshop on Data Warehousing and OLAP. pp. 101–104. ACM, 2011. https://doi.org/10.1145/2064676.2064695
Sebaa, A., Nouicer, N., Chikh, F., and Tari, A., Big Data Technologies to Improve Medical Data Warehousing. In Proceedings of 2nd international conference on Big Data, Cloud and Applications. ACM, 2017. https://doi.org/10.1145/3090354.3090376
Yao, Q., Tian, Y., Li, P.F., Tian, L.L., Qian, Y.M., and Li, J.S., Design and development of a medical big data processing system based on Hadoop. J. Med. Syst. 39(3):23, 2015. https://doi.org/10.1007/s10916-015-0220-8 .
Article PubMed Google Scholar
Istephan, S., and Siadat, M.R., Unstructured medical image query using big data–an epilepsy case study. J. Biomed. Inform. 59:218–226, 2016. https://doi.org/10.1016/j.jbi.2015.12.005 .
Aji, A., Wang, F., Vo, H., Lee, R., Liu, Q., Zhang, X., and Saltz, J., Hadoop GIS: a high performance spatial data warehousing system over Map-Reduce. VLDB Endowment . 6(11):1009–1020, 2013. https://doi.org/10.14778/2536222.2536227 .
Saravanakumar, N.M., Eswari, T., Sampath, P., and Lavanya, S., Predictive methodology for diabetic data analysis in big data. In 2nd ISBCC. Procedia Computer Science . 50:203–208, 2015. https://doi.org/10.1016/j.procs.2015.04.069 .
Rodger, J.A., Discovery of medical big data analytics: Improving the prediction of traumatic brain injury survival rates by data mining patient informatics processing software hybrid Hadoop hive. Informatics in Medicine Unlocked . 1:17–26, 2015. https://doi.org/10.1016/j.imu.2016.01.002 .
Sundvall, E., Wei-Kleiner, F., Freire, S.M., and Lambrix, P., Querying archetype-based electronic health records using Hadoop and Dewey encoding of openEHR models. Stud. Health Technol. Inform. 235:406, 2017. https://doi.org/10.3233/978-1-61499-753-5-406 .
PubMed Google Scholar
Raja, P.V., and Sivasankar, E., Modern Framework for Distributed Healthcare Data Analytics Based on Hadoop. In Information and Communication Technology-EurAsia Conference (pp. 348–355). Springer Berlin Heidelberg, 2014. https://doi.org/10.1007/978-3-642-55032-4_34
Yang, C.T., Liu, J.C., Chen, S.T., and Lu, H.W., Implementation of a big data accessing and processing platform for medical records in cloud. J. Med. Syst. 41(10):149, 2017. https://doi.org/10.1007/s10916-017-0777-5 .
Sebaa, A., Chick, F., Nouicer, A., and Tari, A., Research in big data warehousing using Hadoop. J. Inform. Syst. Eng. Manag. 2(2), 2017. https://doi.org/10.20897/jisem.201710 .
Dean, J., and Ghemawat, S., MapReduce: A flexible data processing tool. CACM . 53(1):72–77, 2010. https://doi.org/10.1145/1629175.1629198 .
Wu, S., Li, F., Mehrotra, S., and Ooi, B.C., Query optimization for massively parallel data processing. In Proceedings of the 2nd ACM Symposium on Cloud Computing (p. 12). ACM, 2011. https://doi.org/10.1145/2038916.2038928
Apache Hadoop: http://hadoop.apache.org/ , Viewed in 02/2015.
Taylor, R.C., An overview of the Hadoop/MapReduce/HBase framework and its current applications in bioinformatics. BMC bioinform. 11(12):S1, 2010. https://doi.org/10.1186/1471-2105-11-S12-S1 .
Apache Hive: https://hive.apache.org/ , Viewed in 02/2015.
Liu, X., Thomsen, C., and Pedersen, T.B., ETLMR: a highly scalable dimensional ETL framework based on mapreduce. In Transactions on Large-Scale Data-and Knowledge-Centered Systems VIII (pp. 1–31). Springer Berlin Heidelberg, 2013. https://doi.org/10.1007/978-3-642-37574-3_1
Gao, S., Li, L., Li, W., Janowicz, K., and Zhang, Y., Constructing gazetteers from volunteered big geo-data based on Hadoop. Comput. Environ. Urban . Syst. 61:172–186, 2017. https://doi.org/10.1016/j.compenvurbsys.2014.02.004 .
Thusoo, A., Sarma, J.S., Jain, N., Shao, Z., Chakka, P., Anthony, S., et al., Hive: A warehousing solution over a map-reduce framework. Proc. VLDB Endowment . 2(2):1626–1629, 2009. https://doi.org/10.14778/1687553.1687609 .
Ross, J., The use of economic evaluation in health care: Australian decision makers' perceptions. Health Policy . 31(2):103–110, 1995. https://doi.org/10.1016/0168-8510(94)00671-7 .
ANDI: National Agency for Investment Development of Algeria, http://www.andi.dz/index.php/en/secteur-de-sante , Viewed in 02/2015.
Download references
Acknowledgements
This work was partially supported by the Ministry of Higher Education and Scientific Research of Algeria and the University of Bejaia, under the project CNEPRU (Ref. B*00620140066/2015-2018).
Author information
Authors and affiliations.
LIMED Laboratory, Faculty of Exact Sciences, University of Bejaia, Bejaia, Algeria
Abderrazak Sebaa, Amina Nouicer & AbdelKamel Tari
Department of Computer Science, Faculty of Exact Sciences, University of Bejaia, Bejaia, Algeria
Fatima Chikh
You can also search for this author in PubMed Google Scholar
Corresponding author
Correspondence to Abderrazak Sebaa .
Ethics declarations
Conflict of interest.
Authors declare that they have no conflict of interest.
Ethical Approval
This article does not contain any studies with human participants or animals performed by any of the authors.
Additional information
This article is part of the Topical Collection on Transactional Processing Systems
Rights and permissions
Reprints and permissions
About this article
Sebaa, A., Chikh, F., Nouicer, A. et al. Medical Big Data Warehouse: Architecture and System Design, a Case Study: Improving Healthcare Resources Distribution. J Med Syst 42 , 59 (2018). https://doi.org/10.1007/s10916-018-0894-9
Download citation
Received : 23 September 2016
Accepted : 08 January 2018
Published : 19 February 2018
DOI : https://doi.org/10.1007/s10916-018-0894-9
Share this article
Anyone you share the following link with will be able to read this content:
Sorry, a shareable link is not currently available for this article.
Provided by the Springer Nature SharedIt content-sharing initiative
- Data warehouse
- Decision support
- Medical resources allocation
- Find a journal
- Publish with us
- Track your research
Healthcare industry case study
No sector is changing or growing faster than digital healthcare. A recent digital healthcare CAGR forecast by PMI estimates 17.4% growth over the next decade. See how Iron Mountain is helping healthcare organizations meet their IT goals.
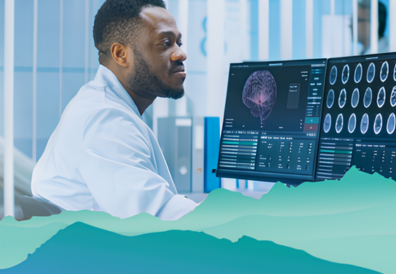
- New distributed applications
- Big data and high density power
- Scalability
- Sustainability
- AI & Hybrid Cloud
- Scalable power
- Secure storage
- Diverse connectivity ecosystems
- Global compliance
- Renewable power and recycling
Industry Challenges
No sector is changing or growing faster than digital healthcare. Both the challenge and the opportunities are huge for what a Deloitte Healthcare Leader has described as “predictive, preventative, personalized and participatory medicine” - all built with digital technologies. A recent digital healthcare CAGR forecast by PMI estimates 17.4% growth over the next decade, taking total market size from $283 BN in 2024 to $1406 BN in 2034.
Healthcare’s digital infrastructure has traditionally been both centralized and specialized. To enable resilience, support new dispersed networks and research and drive consumer-style multi-device health apps, providers now need an enterprise-style infrastructure. AI also has a growing role: healthcare is one of the areas in which generative AI-driven solutions are already mainstream, pushing innovation and improving patient outcomes worldwide.
Iron Mountain is proud to serve some of the world’s leading healthcare businesses. We work with more than 2,000 hospitals and 45,000 healthcare customers across our global storage and data center footprint, curating close to a billion patient records and providing the infrastructure for many of the sector’s most successful new applications.
Featured services & solutions
Data centers.
Iron Mountain is a global data center company that provides tailored, sustainable, secure, carrier and cloud-neutral colocation solutions.
Customer data center solutions
From cloud data centers to federal data centers to healthcare data centers and more, we have the data center solutions for your unique industry.
Elevate the power of your work
Get a FREE consultation today!
Get Started
Related resources
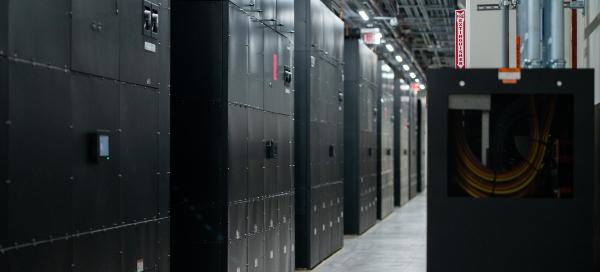
What you need to know about decommissioning data centers
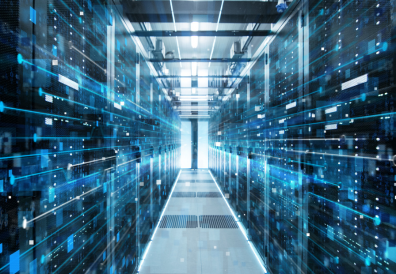
Data sanitization enables more sustainable data center ITAD
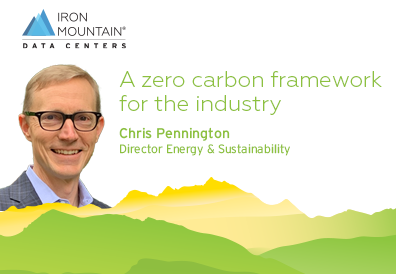
A Zero Carbon Framework for the Industry
- Open access
- Published: 06 January 2022
The use of Big Data Analytics in healthcare
- Kornelia Batko ORCID: orcid.org/0000-0001-6561-3826 1 &
- Andrzej Ślęzak 2
Journal of Big Data volume 9 , Article number: 3 ( 2022 ) Cite this article
72k Accesses
103 Citations
28 Altmetric
Metrics details
The introduction of Big Data Analytics (BDA) in healthcare will allow to use new technologies both in treatment of patients and health management. The paper aims at analyzing the possibilities of using Big Data Analytics in healthcare. The research is based on a critical analysis of the literature, as well as the presentation of selected results of direct research on the use of Big Data Analytics in medical facilities. The direct research was carried out based on research questionnaire and conducted on a sample of 217 medical facilities in Poland. Literature studies have shown that the use of Big Data Analytics can bring many benefits to medical facilities, while direct research has shown that medical facilities in Poland are moving towards data-based healthcare because they use structured and unstructured data, reach for analytics in the administrative, business and clinical area. The research positively confirmed that medical facilities are working on both structural data and unstructured data. The following kinds and sources of data can be distinguished: from databases, transaction data, unstructured content of emails and documents, data from devices and sensors. However, the use of data from social media is lower as in their activity they reach for analytics, not only in the administrative and business but also in the clinical area. It clearly shows that the decisions made in medical facilities are highly data-driven. The results of the study confirm what has been analyzed in the literature that medical facilities are moving towards data-based healthcare, together with its benefits.
Introduction
The main contribution of this paper is to present an analytical overview of using structured and unstructured data (Big Data) analytics in medical facilities in Poland. Medical facilities use both structured and unstructured data in their practice. Structured data has a predetermined schema, it is extensive, freeform, and comes in variety of forms [ 27 ]. In contrast, unstructured data, referred to as Big Data (BD), does not fit into the typical data processing format. Big Data is a massive amount of data sets that cannot be stored, processed, or analyzed using traditional tools. It remains stored but not analyzed. Due to the lack of a well-defined schema, it is difficult to search and analyze such data and, therefore, it requires a specific technology and method to transform it into value [ 20 , 68 ]. Integrating data stored in both structured and unstructured formats can add significant value to an organization [ 27 ]. Organizations must approach unstructured data in a different way. Therefore, the potential is seen in Big Data Analytics (BDA). Big Data Analytics are techniques and tools used to analyze and extract information from Big Data. The results of Big Data analysis can be used to predict the future. They also help in creating trends about the past. When it comes to healthcare, it allows to analyze large datasets from thousands of patients, identifying clusters and correlation between datasets, as well as developing predictive models using data mining techniques [ 60 ].
This paper is the first study to consolidate and characterize the use of Big Data from different perspectives. The first part consists of a brief literature review of studies on Big Data (BD) and Big Data Analytics (BDA), while the second part presents results of direct research aimed at diagnosing the use of big data analyses in medical facilities in Poland.
Healthcare is a complex system with varied stakeholders: patients, doctors, hospitals, pharmaceutical companies and healthcare decision-makers. This sector is also limited by strict rules and regulations. However, worldwide one may observe a departure from the traditional doctor-patient approach. The doctor becomes a partner and the patient is involved in the therapeutic process [ 14 ]. Healthcare is no longer focused solely on the treatment of patients. The priority for decision-makers should be to promote proper health attitudes and prevent diseases that can be avoided [ 81 ]. This became visible and important especially during the Covid-19 pandemic [ 44 ].
The next challenges that healthcare will have to face is the growing number of elderly people and a decline in fertility. Fertility rates in the country are found below the reproductive minimum necessary to keep the population stable [ 10 ]. The reflection of both effects, namely the increase in age and lower fertility rates, are demographic load indicators, which is constantly growing. Forecasts show that providing healthcare in the form it is provided today will become impossible in the next 20 years [ 70 ]. It is especially visible now during the Covid-19 pandemic when healthcare faced quite a challenge related to the analysis of huge data amounts and the need to identify trends and predict the spread of the coronavirus. The pandemic showed it even more that patients should have access to information about their health condition, the possibility of digital analysis of this data and access to reliable medical support online. Health monitoring and cooperation with doctors in order to prevent diseases can actually revolutionize the healthcare system. One of the most important aspects of the change necessary in healthcare is putting the patient in the center of the system.
Technology is not enough to achieve these goals. Therefore, changes should be made not only at the technological level but also in the management and design of complete healthcare processes and what is more, they should affect the business models of service providers. The use of Big Data Analytics is becoming more and more common in enterprises [ 17 , 54 ]. However, medical enterprises still cannot keep up with the information needs of patients, clinicians, administrators and the creator’s policy. The adoption of a Big Data approach would allow the implementation of personalized and precise medicine based on personalized information, delivered in real time and tailored to individual patients.
To achieve this goal, it is necessary to implement systems that will be able to learn quickly about the data generated by people within clinical care and everyday life. This will enable data-driven decision making, receiving better personalized predictions about prognosis and responses to treatments; a deeper understanding of the complex factors and their interactions that influence health at the patient level, the health system and society, enhanced approaches to detecting safety problems with drugs and devices, as well as more effective methods of comparing prevention, diagnostic, and treatment options [ 40 ].
In the literature, there is a lot of research showing what opportunities can be offered to companies by big data analysis and what data can be analyzed. However, there are few studies showing how data analysis in the area of healthcare is performed, what data is used by medical facilities and what analyses and in which areas they carry out. This paper aims to fill this gap by presenting the results of research carried out in medical facilities in Poland. The goal is to analyze the possibilities of using Big Data Analytics in healthcare, especially in Polish conditions. In particular, the paper is aimed at determining what data is processed by medical facilities in Poland, what analyses they perform and in what areas, and how they assess their analytical maturity. In order to achieve this goal, a critical analysis of the literature was performed, and the direct research was based on a research questionnaire conducted on a sample of 217 medical facilities in Poland. It was hypothesized that medical facilities in Poland are working on both structured and unstructured data and moving towards data-based healthcare and its benefits. Examining the maturity of healthcare facilities in the use of Big Data and Big Data Analytics is crucial in determining the potential future benefits that the healthcare sector can gain from Big Data Analytics. There is also a pressing need to predicate whether, in the coming years, healthcare will be able to cope with the threats and challenges it faces.
This paper is divided into eight parts. The first is the introduction which provides background and the general problem statement of this research. In the second part, this paper discusses considerations on use of Big Data and Big Data Analytics in Healthcare, and then, in the third part, it moves on to challenges and potential benefits of using Big Data Analytics in healthcare. The next part involves the explanation of the proposed method. The result of direct research and discussion are presented in the fifth part, while the following part of the paper is the conclusion. The seventh part of the paper presents practical implications. The final section of the paper provides limitations and directions for future research.
Considerations on use Big Data and Big Data Analytics in the healthcare
In recent years one can observe a constantly increasing demand for solutions offering effective analytical tools. This trend is also noticeable in the analysis of large volumes of data (Big Data, BD). Organizations are looking for ways to use the power of Big Data to improve their decision making, competitive advantage or business performance [ 7 , 54 ]. Big Data is considered to offer potential solutions to public and private organizations, however, still not much is known about the outcome of the practical use of Big Data in different types of organizations [ 24 ].
As already mentioned, in recent years, healthcare management worldwide has been changed from a disease-centered model to a patient-centered model, even in value-based healthcare delivery model [ 68 ]. In order to meet the requirements of this model and provide effective patient-centered care, it is necessary to manage and analyze healthcare Big Data.
The issue often raised when it comes to the use of data in healthcare is the appropriate use of Big Data. Healthcare has always generated huge amounts of data and nowadays, the introduction of electronic medical records, as well as the huge amount of data sent by various types of sensors or generated by patients in social media causes data streams to constantly grow. Also, the medical industry generates significant amounts of data, including clinical records, medical images, genomic data and health behaviors. Proper use of the data will allow healthcare organizations to support clinical decision-making, disease surveillance, and public health management. The challenge posed by clinical data processing involves not only the quantity of data but also the difficulty in processing it.
In the literature one can find many different definitions of Big Data. This concept has evolved in recent years, however, it is still not clearly understood. Nevertheless, despite the range and differences in definitions, Big Data can be treated as a: large amount of digital data, large data sets, tool, technology or phenomenon (cultural or technological.
Big Data can be considered as massive and continually generated digital datasets that are produced via interactions with online technologies [ 53 ]. Big Data can be defined as datasets that are of such large sizes that they pose challenges in traditional storage and analysis techniques [ 28 ]. A similar opinion about Big Data was presented by Ohlhorst who sees Big Data as extremely large data sets, possible neither to manage nor to analyze with traditional data processing tools [ 57 ]. In his opinion, the bigger the data set, the more difficult it is to gain any value from it.
In turn, Knapp perceived Big Data as tools, processes and procedures that allow an organization to create, manipulate and manage very large data sets and storage facilities [ 38 ]. From this point of view, Big Data is identified as a tool to gather information from different databases and processes, allowing users to manage large amounts of data.
Similar perception of the term ‘Big Data’ is shown by Carter. According to him, Big Data technologies refer to a new generation of technologies and architectures, designed to economically extract value from very large volumes of a wide variety of data by enabling high velocity capture, discovery and/or analysis [ 13 ].
Jordan combines these two approaches by identifying Big Data as a complex system, as it needs data bases for data to be stored in, programs and tools to be managed, as well as expertise and personnel able to retrieve useful information and visualization to be understood [ 37 ].
Following the definition of Laney for Big Data, it can be state that: it is large amount of data generated in very fast motion and it contains a lot of content [ 43 ]. Such data comes from unstructured sources, such as stream of clicks on the web, social networks (Twitter, blogs, Facebook), video recordings from the shops, recording of calls in a call center, real time information from various kinds of sensors, RFID, GPS devices, mobile phones and other devices that identify and monitor something [ 8 ]. Big Data is a powerful digital data silo, raw, collected with all sorts of sources, unstructured and difficult, or even impossible, to analyze using conventional techniques used so far to relational databases.
While describing Big Data, it cannot be overlooked that the term refers more to a phenomenon than to specific technology. Therefore, instead of defining this phenomenon, trying to describe them, more authors are describing Big Data by giving them characteristics included a collection of V’s related to its nature [ 2 , 3 , 23 , 25 , 58 ]:
Volume (refers to the amount of data and is one of the biggest challenges in Big Data Analytics),
Velocity (speed with which new data is generated, the challenge is to be able to manage data effectively and in real time),
Variety (heterogeneity of data, many different types of healthcare data, the challenge is to derive insights by looking at all available heterogenous data in a holistic manner),
Variability (inconsistency of data, the challenge is to correct the interpretation of data that can vary significantly depending on the context),
Veracity (how trustworthy the data is, quality of the data),
Visualization (ability to interpret data and resulting insights, challenging for Big Data due to its other features as described above).
Value (the goal of Big Data Analytics is to discover the hidden knowledge from huge amounts of data).
Big Data is defined as an information asset with high volume, velocity, and variety, which requires specific technology and method for its transformation into value [ 21 , 77 ]. Big Data is also a collection of information about high-volume, high volatility or high diversity, requiring new forms of processing in order to support decision-making, discovering new phenomena and process optimization [ 5 , 7 ]. Big Data is too large for traditional data-processing systems and software tools to capture, store, manage and analyze, therefore it requires new technologies [ 28 , 50 , 61 ] to manage (capture, aggregate, process) its volume, velocity and variety [ 9 ].
Undoubtedly, Big Data differs from the data sources used so far by organizations. Therefore, organizations must approach this type of unstructured data in a different way. First of all, organizations must start to see data as flows and not stocks—this entails the need to implement the so-called streaming analytics [ 48 ]. The mentioned features make it necessary to use new IT tools that allow the fullest use of new data [ 58 ]. The Big Data idea, inseparable from the huge increase in data available to various organizations or individuals, creates opportunities for access to valuable analyses, conclusions and enables making more accurate decisions [ 6 , 11 , 59 ].
The Big Data concept is constantly evolving and currently it does not focus on huge amounts of data, but rather on the process of creating value from this data [ 52 ]. Big Data is collected from various sources that have different data properties and are processed by different organizational units, resulting in creation of a Big Data chain [ 36 ]. The aim of the organizations is to manage, process and analyze Big Data. In the healthcare sector, Big Data streams consist of various types of data, namely [ 8 , 51 ]:
clinical data, i.e. data obtained from electronic medical records, data from hospital information systems, image centers, laboratories, pharmacies and other organizations providing health services, patient generated health data, physician’s free-text notes, genomic data, physiological monitoring data [ 4 ],
biometric data provided from various types of devices that monitor weight, pressure, glucose level, etc.,
financial data, constituting a full record of economic operations reflecting the conducted activity,
data from scientific research activities, i.e. results of research, including drug research, design of medical devices and new methods of treatment,
data provided by patients, including description of preferences, level of satisfaction, information from systems for self-monitoring of their activity: exercises, sleep, meals consumed, etc.
data from social media.
These data are provided not only by patients but also by organizations and institutions, as well as by various types of monitoring devices, sensors or instruments [ 16 ]. Data that has been generated so far in the healthcare sector is stored in both paper and digital form. Thus, the essence and the specificity of the process of Big Data analyses means that organizations need to face new technological and organizational challenges [ 67 ]. The healthcare sector has always generated huge amounts of data and this is connected, among others, with the need to store medical records of patients. However, the problem with Big Data in healthcare is not limited to an overwhelming volume but also an unprecedented diversity in terms of types, data formats and speed with which it should be analyzed in order to provide the necessary information on an ongoing basis [ 3 ]. It is also difficult to apply traditional tools and methods for management of unstructured data [ 67 ]. Due to the diversity and quantity of data sources that are growing all the time, advanced analytical tools and technologies, as well as Big Data analysis methods which can meet and exceed the possibilities of managing healthcare data, are needed [ 3 , 68 ].
Therefore, the potential is seen in Big Data analyses, especially in the aspect of improving the quality of medical care, saving lives or reducing costs [ 30 ]. Extracting from this tangle of given association rules, patterns and trends will allow health service providers and other stakeholders in the healthcare sector to offer more accurate and more insightful diagnoses of patients, personalized treatment, monitoring of the patients, preventive medicine, support of medical research and health population, as well as better quality of medical services and patient care while, at the same time, the ability to reduce costs (Fig. 1 ).
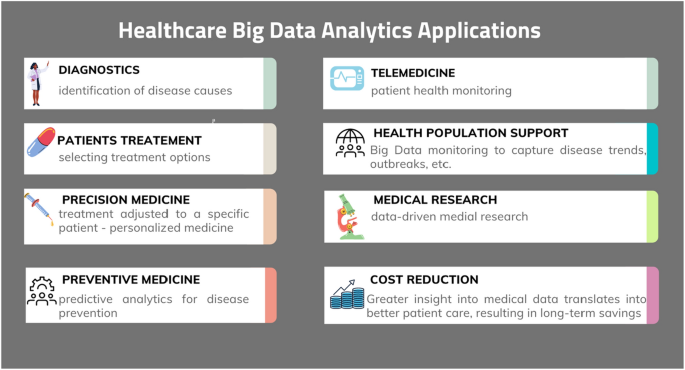
(Source: Own elaboration)
Healthcare Big Data Analytics applications
The main challenge with Big Data is how to handle such a large amount of information and use it to make data-driven decisions in plenty of areas [ 64 ]. In the context of healthcare data, another major challenge is to adjust big data storage, analysis, presentation of analysis results and inference basing on them in a clinical setting. Data analytics systems implemented in healthcare are designed to describe, integrate and present complex data in an appropriate way so that it can be understood better (Fig. 2 ). This would improve the efficiency of acquiring, storing, analyzing and visualizing big data from healthcare [ 71 ].
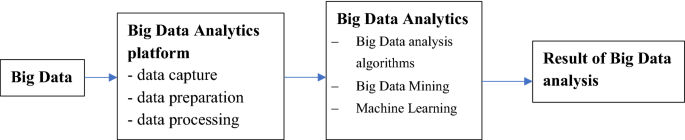
Process of Big Data Analytics
The result of data processing with the use of Big Data Analytics is appropriate data storytelling which may contribute to making decisions with both lower risk and data support. This, in turn, can benefit healthcare stakeholders. To take advantage of the potential massive amounts of data in healthcare and to ensure that the right intervention to the right patient is properly timed, personalized, and potentially beneficial to all components of the healthcare system such as the payer, patient, and management, analytics of large datasets must connect communities involved in data analytics and healthcare informatics [ 49 ]. Big Data Analytics can provide insight into clinical data and thus facilitate informed decision-making about the diagnosis and treatment of patients, prevention of diseases or others. Big Data Analytics can also improve the efficiency of healthcare organizations by realizing the data potential [ 3 , 62 ].
Big Data Analytics in medicine and healthcare refers to the integration and analysis of a large amount of complex heterogeneous data, such as various omics (genomics, epigenomics, transcriptomics, proteomics, metabolomics, interactomics, pharmacogenetics, deasomics), biomedical data, talemedicine data (sensors, medical equipment data) and electronic health records data [ 46 , 65 ].
When analyzing the phenomenon of Big Data in the healthcare sector, it should be noted that it can be considered from the point of view of three areas: epidemiological, clinical and business.
From a clinical point of view, the Big Data analysis aims to improve the health and condition of patients, enable long-term predictions about their health status and implementation of appropriate therapeutic procedures. Ultimately, the use of data analysis in medicine is to allow the adaptation of therapy to a specific patient, that is personalized medicine (precision, personalized medicine).
From an epidemiological point of view, it is desirable to obtain an accurate prognosis of morbidity in order to implement preventive programs in advance.
In the business context, Big Data analysis may enable offering personalized packages of commercial services or determining the probability of individual disease and infection occurrence. It is worth noting that Big Data means not only the collection and processing of data but, most of all, the inference and visualization of data necessary to obtain specific business benefits.
In order to introduce new management methods and new solutions in terms of effectiveness and transparency, it becomes necessary to make data more accessible, digital, searchable, as well as analyzed and visualized.
Erickson and Rothberg state that the information and data do not reveal their full value until insights are drawn from them. Data becomes useful when it enhances decision making and decision making is enhanced only when analytical techniques are used and an element of human interaction is applied [ 22 ].
Thus, healthcare has experienced much progress in usage and analysis of data. A large-scale digitalization and transparency in this sector is a key statement of almost all countries governments policies. For centuries, the treatment of patients was based on the judgment of doctors who made treatment decisions. In recent years, however, Evidence-Based Medicine has become more and more important as a result of it being related to the systematic analysis of clinical data and decision-making treatment based on the best available information [ 42 ]. In the healthcare sector, Big Data Analytics is expected to improve the quality of life and reduce operational costs [ 72 , 82 ]. Big Data Analytics enables organizations to improve and increase their understanding of the information contained in data. It also helps identify data that provides insightful insights for current as well as future decisions [ 28 ].
Big Data Analytics refers to technologies that are grounded mostly in data mining: text mining, web mining, process mining, audio and video analytics, statistical analysis, network analytics, social media analytics and web analytics [ 16 , 25 , 31 ]. Different data mining techniques can be applied on heterogeneous healthcare data sets, such as: anomaly detection, clustering, classification, association rules as well as summarization and visualization of those Big Data sets [ 65 ]. Modern data analytics techniques explore and leverage unique data characteristics even from high-speed data streams and sensor data [ 15 , 16 , 31 , 55 ]. Big Data can be used, for example, for better diagnosis in the context of comprehensive patient data, disease prevention and telemedicine (in particular when using real-time alerts for immediate care), monitoring patients at home, preventing unnecessary hospital visits, integrating medical imaging for a wider diagnosis, creating predictive analytics, reducing fraud and improving data security, better strategic planning and increasing patients’ involvement in their own health.
Big Data Analytics in healthcare can be divided into [ 33 , 73 , 74 ]:
descriptive analytics in healthcare is used to understand past and current healthcare decisions, converting data into useful information for understanding and analyzing healthcare decisions, outcomes and quality, as well as making informed decisions [ 33 ]. It can be used to create reports (i.e. about patients’ hospitalizations, physicians’ performance, utilization management), visualization, customized reports, drill down tables, or running queries on the basis of historical data.
predictive analytics operates on past performance in an effort to predict the future by examining historical or summarized health data, detecting patterns of relationships in these data, and then extrapolating these relationships to forecast. It can be used to i.e. predict the response of different patient groups to different drugs (dosages) or reactions (clinical trials), anticipate risk and find relationships in health data and detect hidden patterns [ 62 ]. In this way, it is possible to predict the epidemic spread, anticipate service contracts and plan healthcare resources. Predictive analytics is used in proper diagnosis and for appropriate treatments to be given to patients suffering from certain diseases [ 39 ].
prescriptive analytics—occurs when health problems involve too many choices or alternatives. It uses health and medical knowledge in addition to data or information. Prescriptive analytics is used in many areas of healthcare, including drug prescriptions and treatment alternatives. Personalized medicine and evidence-based medicine are both supported by prescriptive analytics.
discovery analytics—utilizes knowledge about knowledge to discover new “inventions” like drugs (drug discovery), previously unknown diseases and medical conditions, alternative treatments, etc.
Although the models and tools used in descriptive, predictive, prescriptive, and discovery analytics are different, many applications involve all four of them [ 62 ]. Big Data Analytics in healthcare can help enable personalized medicine by identifying optimal patient-specific treatments. This can influence the improvement of life standards, reduce waste of healthcare resources and save costs of healthcare [ 56 , 63 , 71 ]. The introduction of large data analysis gives new analytical possibilities in terms of scope, flexibility and visualization. Techniques such as data mining (computational pattern discovery process in large data sets) facilitate inductive reasoning and analysis of exploratory data, enabling scientists to identify data patterns that are independent of specific hypotheses. As a result, predictive analysis and real-time analysis becomes possible, making it easier for medical staff to start early treatments and reduce potential morbidity and mortality. In addition, document analysis, statistical modeling, discovering patterns and topics in document collections and data in the EHR, as well as an inductive approach can help identify and discover relationships between health phenomena.
Advanced analytical techniques can be used for a large amount of existing (but not yet analytical) data on patient health and related medical data to achieve a better understanding of the information and results obtained, as well as to design optimal clinical pathways [ 62 ]. Big Data Analytics in healthcare integrates analysis of several scientific areas such as bioinformatics, medical imaging, sensor informatics, medical informatics and health informatics [ 65 ]. Big Data Analytics in healthcare allows to analyze large datasets from thousands of patients, identifying clusters and correlation between datasets, as well as developing predictive models using data mining techniques [ 65 ]. Discussing all the techniques used for Big Data Analytics goes beyond the scope of a single article [ 25 ].
The success of Big Data analysis and its accuracy depend heavily on the tools and techniques used to analyze the ability to provide reliable, up-to-date and meaningful information to various stakeholders [ 12 ]. It is believed that the implementation of big data analytics by healthcare organizations could bring many benefits in the upcoming years, including lowering health care costs, better diagnosis and prediction of diseases and their spread, improving patient care and developing protocols to prevent re-hospitalization, optimizing staff, optimizing equipment, forecasting the need for hospital beds, operating rooms, treatments, and improving the drug supply chain [ 71 ].
Challenges and potential benefits of using Big Data Analytics in healthcare
Modern analytics gives possibilities not only to have insight in historical data, but also to have information necessary to generate insight into what may happen in the future. Even when it comes to prediction of evidence-based actions. The emphasis on reform has prompted payers and suppliers to pursue data analysis to reduce risk, detect fraud, improve efficiency and save lives. Everyone—payers, providers, even patients—are focusing on doing more with fewer resources. Thus, some areas in which enhanced data and analytics can yield the greatest results include various healthcare stakeholders (Table 1 ).
Healthcare organizations see the opportunity to grow through investments in Big Data Analytics. In recent years, by collecting medical data of patients, converting them into Big Data and applying appropriate algorithms, reliable information has been generated that helps patients, physicians and stakeholders in the health sector to identify values and opportunities [ 31 ]. It is worth noting that there are many changes and challenges in the structure of the healthcare sector. Digitization and effective use of Big Data in healthcare can bring benefits to every stakeholder in this sector. A single doctor would benefit the same as the entire healthcare system. Potential opportunities to achieve benefits and effects from Big Data in healthcare can be divided into four groups [ 8 ]:
Improving the quality of healthcare services:
assessment of diagnoses made by doctors and the manner of treatment of diseases indicated by them based on the decision support system working on Big Data collections,
detection of more effective, from a medical point of view, and more cost-effective ways to diagnose and treat patients,
analysis of large volumes of data to reach practical information useful for identifying needs, introducing new health services, preventing and overcoming crises,
prediction of the incidence of diseases,
detecting trends that lead to an improvement in health and lifestyle of the society,
analysis of the human genome for the introduction of personalized treatment.
Supporting the work of medical personnel
doctors’ comparison of current medical cases to cases from the past for better diagnosis and treatment adjustment,
detection of diseases at earlier stages when they can be more easily and quickly cured,
detecting epidemiological risks and improving control of pathogenic spots and reaction rates,
identification of patients who are predicted to have the highest risk of specific, life-threatening diseases by collating data on the history of the most common diseases, in healing people with reports entering insurance companies,
health management of each patient individually (personalized medicine) and health management of the whole society,
capturing and analyzing large amounts of data from hospitals and homes in real time, life monitoring devices to monitor safety and predict adverse events,
analysis of patient profiles to identify people for whom prevention should be applied, lifestyle change or preventive care approach,
the ability to predict the occurrence of specific diseases or worsening of patients’ results,
predicting disease progression and its determinants, estimating the risk of complications,
detecting drug interactions and their side effects.
Supporting scientific and research activity
supporting work on new drugs and clinical trials thanks to the possibility of analyzing “all data” instead of selecting a test sample,
the ability to identify patients with specific, biological features that will take part in specialized clinical trials,
selecting a group of patients for which the tested drug is likely to have the desired effect and no side effects,
using modeling and predictive analysis to design better drugs and devices.
Business and management
reduction of costs and counteracting abuse and counseling practices,
faster and more effective identification of incorrect or unauthorized financial operations in order to prevent abuse and eliminate errors,
increase in profitability by detecting patients generating high costs or identifying doctors whose work, procedures and treatment methods cost the most and offering them solutions that reduce the amount of money spent,
identification of unnecessary medical activities and procedures, e.g. duplicate tests.
According to research conducted by Wang, Kung and Byrd, Big Data Analytics benefits can be classified into five categories: IT infrastructure benefits (reducing system redundancy, avoiding unnecessary IT costs, transferring data quickly among healthcare IT systems, better use of healthcare systems, processing standardization among various healthcare IT systems, reducing IT maintenance costs regarding data storage), operational benefits (improving the quality and accuracy of clinical decisions, processing a large number of health records in seconds, reducing the time of patient travel, immediate access to clinical data to analyze, shortening the time of diagnostic test, reductions in surgery-related hospitalizations, exploring inconceivable new research avenues), organizational benefits (detecting interoperability problems much more quickly than traditional manual methods, improving cross-functional communication and collaboration among administrative staffs, researchers, clinicians and IT staffs, enabling data sharing with other institutions and adding new services, content sources and research partners), managerial benefits (gaining quick insights about changing healthcare trends in the market, providing members of the board and heads of department with sound decision-support information on the daily clinical setting, optimizing business growth-related decisions) and strategic benefits (providing a big picture view of treatment delivery for meeting future need, creating high competitive healthcare services) [ 73 ].
The above specification does not constitute a full list of potential areas of use of Big Data Analysis in healthcare because the possibilities of using analysis are practically unlimited. In addition, advanced analytical tools allow to analyze data from all possible sources and conduct cross-analyses to provide better data insights [ 26 ]. For example, a cross-analysis can refer to a combination of patient characteristics, as well as costs and care results that can help identify the best, in medical terms, and the most cost-effective treatment or treatments and this may allow a better adjustment of the service provider’s offer [ 62 ].
In turn, the analysis of patient profiles (e.g. segmentation and predictive modeling) allows identification of people who should be subject to prophylaxis, prevention or should change their lifestyle [ 8 ]. Shortened list of benefits for Big Data Analytics in healthcare is presented in paper [ 3 ] and consists of: better performance, day-to-day guides, detection of diseases in early stages, making predictive analytics, cost effectiveness, Evidence Based Medicine and effectiveness in patient treatment.
Summarizing, healthcare big data represents a huge potential for the transformation of healthcare: improvement of patients’ results, prediction of outbreaks of epidemics, valuable insights, avoidance of preventable diseases, reduction of the cost of healthcare delivery and improvement of the quality of life in general [ 1 ]. Big Data also generates many challenges such as difficulties in data capture, data storage, data analysis and data visualization [ 15 ]. The main challenges are connected with the issues of: data structure (Big Data should be user-friendly, transparent, and menu-driven but it is fragmented, dispersed, rarely standardized and difficult to aggregate and analyze), security (data security, privacy and sensitivity of healthcare data, there are significant concerns related to confidentiality), data standardization (data is stored in formats that are not compatible with all applications and technologies), storage and transfers (especially costs associated with securing, storing, and transferring unstructured data), managerial skills, such as data governance, lack of appropriate analytical skills and problems with Real-Time Analytics (health care is to be able to utilize Big Data in real time) [ 4 , 34 , 41 ].
The research is based on a critical analysis of the literature, as well as the presentation of selected results of direct research on the use of Big Data Analytics in medical facilities in Poland.
Presented research results are part of a larger questionnaire form on Big Data Analytics. The direct research was based on an interview questionnaire which contained 100 questions with 5-point Likert scale (1—strongly disagree, 2—I rather disagree, 3—I do not agree, nor disagree, 4—I rather agree, 5—I definitely agree) and 4 metrics questions. The study was conducted in December 2018 on a sample of 217 medical facilities (110 private, 107 public). The research was conducted by a specialized market research agency: Center for Research and Expertise of the University of Economics in Katowice.
When it comes to direct research, the selected entities included entities financed from public sources—the National Health Fund (23.5%), and entities operating commercially (11.5%). In the surveyed group of entities, more than a half (64.9%) are hybrid financed, both from public and commercial sources. The diversity of the research sample also applies to the size of the entities, defined by the number of employees. Taking into account proportions of the surveyed entities, it should be noted that in the sector structure, medium-sized (10–50 employees—34% of the sample) and large (51–250 employees—27%) entities dominate. The research was of all-Poland nature, and the entities included in the research sample come from all of the voivodships. The largest group were entities from Łódzkie (32%), Śląskie (18%) and Mazowieckie (18%) voivodships, as these voivodships have the largest number of medical institutions. Other regions of the country were represented by single units. The selection of the research sample was random—layered. As part of medical facilities database, groups of private and public medical facilities have been identified and the ones to which the questionnaire was targeted were drawn from each of these groups. The analyses were performed using the GNU PSPP 0.10.2 software.
The aim of the study was to determine whether medical facilities in Poland use Big Data Analytics and if so, in which areas. Characteristics of the research sample is presented in Table 2 .
The research is non-exhaustive due to the incomplete and uneven regional distribution of the samples, overrepresented in three voivodeships (Łódzkie, Mazowieckie and Śląskie). The size of the research sample (217 entities) allows the authors of the paper to formulate specific conclusions on the use of Big Data in the process of its management.
For the purpose of this paper, the following research hypotheses were formulated: (1) medical facilities in Poland are working on both structured and unstructured data (2) medical facilities in Poland are moving towards data-based healthcare and its benefits.
The paper poses the following research questions and statements that coincide with the selected questions from the research questionnaire:
From what sources do medical facilities obtain data? What types of data are used by the particular organization, whether structured or unstructured, and to what extent?
From what sources do medical facilities obtain data?
In which area organizations are using data and analytical systems (clinical or business)?
Is data analytics performed based on historical data or are predictive analyses also performed?
Determining whether administrative and medical staff receive complete, accurate and reliable data in a timely manner?
Determining whether real-time analyses are performed to support the particular organization’s activities.
Results and discussion
On the basis of the literature analysis and research study, a set of questions and statements related to the researched area was formulated. The results from the surveys show that medical facilities use a variety of data sources in their operations. These sources are both structured and unstructured data (Table 3 ).
According to the data provided by the respondents, considering the first statement made in the questionnaire, almost half of the medical institutions (47.58%) agreed that they rather collect and use structured data (e.g. databases and data warehouses, reports to external entities) and 10.57% entirely agree with this statement. As much as 23.35% of representatives of medical institutions stated “I agree or disagree”. Other medical facilities do not collect and use structured data (7.93%) and 6.17% strongly disagree with the first statement. Also, the median calculated based on the obtained results (median: 4), proves that medical facilities in Poland collect and use structured data (Table 4 ).
In turn, 28.19% of the medical institutions agreed that they rather collect and use unstructured data and as much as 9.25% entirely agree with this statement. The number of representatives of medical institutions that stated “I agree or disagree” was 27.31%. Other medical facilities do not collect and use structured data (17.18%) and 13.66% strongly disagree with the first statement. In the case of unstructured data the median is 3, which means that the collection and use of this type of data by medical facilities in Poland is lower.
In the further part of the analysis, it was checked whether the size of the medical facility and form of ownership have an impact on whether it analyzes unstructured data (Tables 4 and 5 ). In order to find this out, correlation coefficients were calculated.
Based on the calculations, it can be concluded that there is a small statistically monotonic correlation between the size of the medical facility and its collection and use of structured data (p < 0.001; τ = 0.16). This means that the use of structured data is slightly increasing in larger medical facilities. The size of the medical facility is more important according to use of unstructured data (p < 0.001; τ = 0.23) (Table 4 .).
To determine whether the form of medical facility ownership affects data collection, the Mann–Whitney U test was used. The calculations show that the form of ownership does not affect what data the organization collects and uses (Table 5 ).
Detailed information on the sources of from which medical facilities collect and use data is presented in the Table 6 .
The questionnaire results show that medical facilities are especially using information published in databases, reports to external units and transaction data, but they also use unstructured data from e-mails, medical devices, sensors, phone calls, audio and video data (Table 6 ). Data from social media, RFID and geolocation data are used to a small extent. Similar findings are concluded in the literature studies.
From the analysis of the answers given by the respondents, more than half of the medical facilities have integrated hospital system (HIS) implemented. As much as 43.61% use integrated hospital system and 16.30% use it extensively (Table 7 ). 19.38% of exanimated medical facilities do not use it at all. Moreover, most of the examined medical facilities (34.80% use it, 32.16% use extensively) conduct medical documentation in an electronic form, which gives an opportunity to use data analytics. Only 4.85% of medical facilities don’t use it at all.
Other problems that needed to be investigated were: whether medical facilities in Poland use data analytics? If so, in what form and in what areas? (Table 8 ). The analysis of answers given by the respondents about the potential of data analytics in medical facilities shows that a similar number of medical facilities use data analytics in administration and business (31.72% agreed with the statement no. 5 and 12.33% strongly agreed) as in the clinical area (33.04% agreed with the statement no. 6 and 12.33% strongly agreed). When considering decision-making issues, 35.24% agree with the statement "the organization uses data and analytical systems to support business decisions” and 8.37% of respondents strongly agree. Almost 40.09% agree with the statement that “the organization uses data and analytical systems to support clinical decisions (in the field of diagnostics and therapy)” and 15.42% of respondents strongly agree. Exanimated medical facilities use in their activity analytics based both on historical data (33.48% agree with statement 7 and 12.78% strongly agree) and predictive analytics (33.04% agrees with the statement number 8 and 15.86% strongly agree). Detailed results are presented in Table 8 .
Medical facilities focus on development in the field of data processing, as they confirm that they conduct analytical planning processes systematically and analyze new opportunities for strategic use of analytics in business and clinical activities (38.33% rather agree and 10.57% strongly agree with this statement). The situation is different with real-time data analysis, here, the situation is not so optimistic. Only 28.19% rather agree and 14.10% strongly agree with the statement that real-time analyses are performed to support an organization’s activities.
When considering whether a facility’s performance in the clinical area depends on the form of ownership, it can be concluded that taking the average and the Mann–Whitney U test depends. A higher degree of use of analyses in the clinical area can be observed in public institutions.
Whether a medical facility performs a descriptive or predictive analysis do not depend on the form of ownership (p > 0.05). It can be concluded that when analyzing the mean and median, they are higher in public facilities, than in private ones. What is more, the Mann–Whitney U test shows that these variables are dependent from each other (p < 0.05) (Table 9 ).
When considering whether a facility’s performance in the clinical area depends on its size, it can be concluded that taking the Kendall’s Tau (τ) it depends (p < 0.001; τ = 0.22), and the correlation is weak but statistically important. This means that the use of data and analytical systems to support clinical decisions (in the field of diagnostics and therapy) increases with the increase of size of the medical facility. A similar relationship, but even less powerful, can be found in the use of descriptive and predictive analyses (Table 10 ).
Considering the results of research in the area of analytical maturity of medical facilities, 8.81% of medical facilities stated that they are at the first level of maturity, i.e. an organization has developed analytical skills and does not perform analyses. As much as 13.66% of medical facilities confirmed that they have poor analytical skills, while 38.33% of the medical facility has located itself at level 3, meaning that “there is a lot to do in analytics”. On the other hand, 28.19% believe that analytical capabilities are well developed and 6.61% stated that analytics are at the highest level and the analytical capabilities are very well developed. Detailed data is presented in Table 11 . Average amounts to 3.11 and Median to 3.
The results of the research have enabled the formulation of following conclusions. Medical facilities in Poland are working on both structured and unstructured data. This data comes from databases, transactions, unstructured content of emails and documents, devices and sensors. However, the use of data from social media is smaller. In their activity, they reach for analytics in the administrative and business, as well as in the clinical area. Also, the decisions made are largely data-driven.
In summary, analysis of the literature that the benefits that medical facilities can get using Big Data Analytics in their activities relate primarily to patients, physicians and medical facilities. It can be confirmed that: patients will be better informed, will receive treatments that will work for them, will have prescribed medications that work for them and not be given unnecessary medications [ 78 ]. Physician roles will likely change to more of a consultant than decision maker. They will advise, warn, and help individual patients and have more time to form positive and lasting relationships with their patients in order to help people. Medical facilities will see changes as well, for example in fewer unnecessary hospitalizations, resulting initially in less revenue, but after the market adjusts, also the accomplishment [ 78 ]. The use of Big Data Analytics can literally revolutionize the way healthcare is practiced for better health and disease reduction.
The analysis of the latest data reveals that data analytics increase the accuracy of diagnoses. Physicians can use predictive algorithms to help them make more accurate diagnoses [ 45 ]. Moreover, it could be helpful in preventive medicine and public health because with early intervention, many diseases can be prevented or ameliorated [ 29 ]. Predictive analytics also allows to identify risk factors for a given patient, and with this knowledge patients will be able to change their lives what, in turn, may contribute to the fact that population disease patterns may dramatically change, resulting in savings in medical costs. Moreover, personalized medicine is the best solution for an individual patient seeking treatment. It can help doctors decide the exact treatments for those individuals. Better diagnoses and more targeted treatments will naturally lead to increases in good outcomes and fewer resources used, including doctors’ time.
The quantitative analysis of the research carried out and presented in this article made it possible to determine whether medical facilities in Poland use Big Data Analytics and if so, in which areas. Thanks to the results obtained it was possible to formulate the following conclusions. Medical facilities are working on both structured and unstructured data, which comes from databases, transactions, unstructured content of emails and documents, devices and sensors. According to analytics, they reach for analytics in the administrative and business, as well as in the clinical area. It clearly showed that the decisions made are largely data-driven. The results of the study confirm what has been analyzed in the literature. Medical facilities are moving towards data-based healthcare and its benefits.
In conclusion, Big Data Analytics has the potential for positive impact and global implications in healthcare. Future research on the use of Big Data in medical facilities will concern the definition of strategies adopted by medical facilities to promote and implement such solutions, as well as the benefits they gain from the use of Big Data analysis and how the perspectives in this area are seen.
Practical implications
This work sought to narrow the gap that exists in analyzing the possibility of using Big Data Analytics in healthcare. Showing how medical facilities in Poland are doing in this respect is an element that is part of global research carried out in this area, including [ 29 , 32 , 60 ].
Limitations and future directions
The research described in this article does not fully exhaust the questions related to the use of Big Data Analytics in Polish healthcare facilities. Only some of the dimensions characterizing the use of data by medical facilities in Poland have been examined. In order to get the full picture, it would be necessary to examine the results of using structured and unstructured data analytics in healthcare. Future research may examine the benefits that medical institutions achieve as a result of the analysis of structured and unstructured data in the clinical and management areas and what limitations they encounter in these areas. For this purpose, it is planned to conduct in-depth interviews with chosen medical facilities in Poland. These facilities could give additional data for empirical analyses based more on their suggestions. Further research should also include medical institutions from beyond the borders of Poland, enabling international comparative analyses.
Future research in the healthcare field has virtually endless possibilities. These regard the use of Big Data Analytics to diagnose specific conditions [ 47 , 66 , 69 , 76 ], propose an approach that can be used in other healthcare applications and create mechanisms to identify “patients like me” [ 75 , 80 ]. Big Data Analytics could also be used for studies related to the spread of pandemics, the efficacy of covid treatment [ 18 , 79 ], or psychology and psychiatry studies, e.g. emotion recognition [ 35 ].
Availability of data and materials
The datasets for this study are available on request to the corresponding author.
Abouelmehdi K, Beni-Hessane A, Khaloufi H. Big healthcare data: preserving security and privacy. J Big Data. 2018. https://doi.org/10.1186/s40537-017-0110-7 .
Article Google Scholar
Agrawal A, Choudhary A. Health services data: big data analytics for deriving predictive healthcare insights. Health Serv Eval. 2019. https://doi.org/10.1007/978-1-4899-7673-4_2-1 .
Al Mayahi S, Al-Badi A, Tarhini A. Exploring the potential benefits of big data analytics in providing smart healthcare. In: Miraz MH, Excell P, Ware A, Ali M, Soomro S, editors. Emerging technologies in computing—first international conference, iCETiC 2018, proceedings (Lecture Notes of the Institute for Computer Sciences, Social-Informatics and Telecommunications Engineering, LNICST). Cham: Springer; 2018. p. 247–58. https://doi.org/10.1007/978-3-319-95450-9_21 .
Bainbridge M. Big data challenges for clinical and precision medicine. In: Househ M, Kushniruk A, Borycki E, editors. Big data, big challenges: a healthcare perspective: background, issues, solutions and research directions. Cham: Springer; 2019. p. 17–31.
Google Scholar
Bartuś K, Batko K, Lorek P. Business intelligence systems: barriers during implementation. In: Jabłoński M, editor. Strategic performance management new concept and contemporary trends. New York: Nova Science Publishers; 2017. p. 299–327. ISBN: 978-1-53612-681-5.
Bartuś K, Batko K, Lorek P. Diagnoza wykorzystania big data w organizacjach-wybrane wyniki badań. Informatyka Ekonomiczna. 2017;3(45):9–20.
Bartuś K, Batko K, Lorek P. Wykorzystanie rozwiązań business intelligence, competitive intelligence i big data w przedsiębiorstwach województwa śląskiego. Przegląd Organizacji. 2018;2:33–9.
Batko K. Możliwości wykorzystania Big Data w ochronie zdrowia. Roczniki Kolegium Analiz Ekonomicznych. 2016;42:267–82.
Bi Z, Cochran D. Big data analytics with applications. J Manag Anal. 2014;1(4):249–65. https://doi.org/10.1080/23270012.2014.992985 .
Boerma T, Requejo J, Victora CG, Amouzou A, Asha G, Agyepong I, Borghi J. Countdown to 2030: tracking progress towards universal coverage for reproductive, maternal, newborn, and child health. Lancet. 2018;391(10129):1538–48.
Bollier D, Firestone CM. The promise and peril of big data. Washington, D.C: Aspen Institute, Communications and Society Program; 2010. p. 1–66.
Bose R. Competitive intelligence process and tools for intelligence analysis. Ind Manag Data Syst. 2008;108(4):510–28.
Carter P. Big data analytics: future architectures, skills and roadmaps for the CIO: in white paper, IDC sponsored by SAS. 2011. p. 1–16.
Castro EM, Van Regenmortel T, Vanhaecht K, Sermeus W, Van Hecke A. Patient empowerment, patient participation and patient-centeredness in hospital care: a concept analysis based on a literature review. Patient Educ Couns. 2016;99(12):1923–39.
Chen H, Chiang RH, Storey VC. Business intelligence and analytics: from big data to big impact. MIS Q. 2012;36(4):1165–88.
Chen CP, Zhang CY. Data-intensive applications, challenges, techniques and technologies: a survey on big data. Inf Sci. 2014;275:314–47.
Chomiak-Orsa I, Mrozek B. Główne perspektywy wykorzystania big data w mediach społecznościowych. Informatyka Ekonomiczna. 2017;3(45):44–54.
Corsi A, de Souza FF, Pagani RN, et al. Big data analytics as a tool for fighting pandemics: a systematic review of literature. J Ambient Intell Hum Comput. 2021;12:9163–80. https://doi.org/10.1007/s12652-020-02617-4 .
Davenport TH, Harris JG. Competing on analytics, the new science of winning. Boston: Harvard Business School Publishing Corporation; 2007.
Davenport TH. Big data at work: dispelling the myths, uncovering the opportunities. Boston: Harvard Business School Publishing; 2014.
De Cnudde S, Martens D. Loyal to your city? A data mining analysis of a public service loyalty program. Decis Support Syst. 2015;73:74–84.
Erickson S, Rothberg H. Data, information, and intelligence. In: Rodriguez E, editor. The analytics process. Boca Raton: Auerbach Publications; 2017. p. 111–26.
Fang H, Zhang Z, Wang CJ, Daneshmand M, Wang C, Wang H. A survey of big data research. IEEE Netw. 2015;29(5):6–9.
Fredriksson C. Organizational knowledge creation with big data. A case study of the concept and practical use of big data in a local government context. 2016. https://www.abo.fi/fakultet/media/22103/fredriksson.pdf .
Gandomi A, Haider M. Beyond the hype: big data concepts, methods, and analytics. Int J Inf Manag. 2015;35(2):137–44.
Groves P, Kayyali B, Knott D, Van Kuiken S. The ‘big data’ revolution in healthcare. Accelerating value and innovation. 2015. http://www.pharmatalents.es/assets/files/Big_Data_Revolution.pdf (Reading: 10.04.2019).
Gupta V, Rathmore N. Deriving business intelligence from unstructured data. Int J Inf Comput Technol. 2013;3(9):971–6.
Gupta V, Singh VK, Ghose U, Mukhija P. A quantitative and text-based characterization of big data research. J Intell Fuzzy Syst. 2019;36:4659–75.
Hampel HOBS, O’Bryant SE, Castrillo JI, Ritchie C, Rojkova K, Broich K, Escott-Price V. PRECISION MEDICINE-the golden gate for detection, treatment and prevention of Alzheimer’s disease. J Prev Alzheimer’s Dis. 2016;3(4):243.
Harerimana GB, Jang J, Kim W, Park HK. Health big data analytics: a technology survey. IEEE Access. 2018;6:65661–78. https://doi.org/10.1109/ACCESS.2018.2878254 .
Hu H, Wen Y, Chua TS, Li X. Toward scalable systems for big data analytics: a technology tutorial. IEEE Access. 2014;2:652–87.
Hussain S, Hussain M, Afzal M, Hussain J, Bang J, Seung H, Lee S. Semantic preservation of standardized healthcare documents in big data. Int J Med Inform. 2019;129:133–45. https://doi.org/10.1016/j.ijmedinf.2019.05.024 .
Islam MS, Hasan MM, Wang X, Germack H. A systematic review on healthcare analytics: application and theoretical perspective of data mining. In: Healthcare. Basel: Multidisciplinary Digital Publishing Institute; 2018. p. 54.
Ismail A, Shehab A, El-Henawy IM. Healthcare analysis in smart big data analytics: reviews, challenges and recommendations. In: Security in smart cities: models, applications, and challenges. Cham: Springer; 2019. p. 27–45.
Jain N, Gupta V, Shubham S, et al. Understanding cartoon emotion using integrated deep neural network on large dataset. Neural Comput Appl. 2021. https://doi.org/10.1007/s00521-021-06003-9 .
Janssen M, van der Voort H, Wahyudi A. Factors influencing big data decision-making quality. J Bus Res. 2017;70:338–45.
Jordan SR. Beneficence and the expert bureaucracy. Public Integr. 2014;16(4):375–94. https://doi.org/10.2753/PIN1099-9922160404 .
Knapp MM. Big data. J Electron Resourc Med Libr. 2013;10(4):215–22.
Koti MS, Alamma BH. Predictive analytics techniques using big data for healthcare databases. In: Smart intelligent computing and applications. New York: Springer; 2019. p. 679–86.
Krumholz HM. Big data and new knowledge in medicine: the thinking, training, and tools needed for a learning health system. Health Aff. 2014;33(7):1163–70.
Kruse CS, Goswamy R, Raval YJ, Marawi S. Challenges and opportunities of big data in healthcare: a systematic review. JMIR Med Inform. 2016;4(4):e38.
Kyoungyoung J, Gang HK. Potentiality of big data in the medical sector: focus on how to reshape the healthcare system. Healthc Inform Res. 2013;19(2):79–85.
Laney D. Application delivery strategies 2011. http://blogs.gartner.com/doug-laney/files/2012/01/ad949-3D-Data-Management-Controlling-Data-Volume-Velocity-and-Variety.pdf .
Lee IK, Wang CC, Lin MC, Kung CT, Lan KC, Lee CT. Effective strategies to prevent coronavirus disease-2019 (COVID-19) outbreak in hospital. J Hosp Infect. 2020;105(1):102.
Lerner I, Veil R, Nguyen DP, Luu VP, Jantzen R. Revolution in health care: how will data science impact doctor-patient relationships? Front Public Health. 2018;6:99.
Lytras MD, Papadopoulou P, editors. Applying big data analytics in bioinformatics and medicine. IGI Global: Hershey; 2017.
Ma K, et al. Big data in multiple sclerosis: development of a web-based longitudinal study viewer in an imaging informatics-based eFolder system for complex data analysis and management. In: Proceedings volume 9418, medical imaging 2015: PACS and imaging informatics: next generation and innovations. 2015. p. 941809. https://doi.org/10.1117/12.2082650 .
Mach-Król M. Analiza i strategia big data w organizacjach. In: Studia i Materiały Polskiego Stowarzyszenia Zarządzania Wiedzą. 2015;74:43–55.
Madsen LB. Data-driven healthcare: how analytics and BI are transforming the industry. Hoboken: Wiley; 2014.
Manyika J, Chui M, Brown B, Bughin J, Dobbs R, Roxburgh C, Hung BA. Big data: the next frontier for innovation, competition, and productivity. Washington: McKinsey Global Institute; 2011.
Marconi K, Dobra M, Thompson C. The use of big data in healthcare. In: Liebowitz J, editor. Big data and business analytics. Boca Raton: CRC Press; 2012. p. 229–48.
Mehta N, Pandit A. Concurrence of big data analytics and healthcare: a systematic review. Int J Med Inform. 2018;114:57–65.
Michel M, Lupton D. Toward a manifesto for the ‘public understanding of big data.’ Public Underst Sci. 2016;25(1):104–16. https://doi.org/10.1177/0963662515609005 .
Mikalef P, Krogstie J. Big data analytics as an enabler of process innovation capabilities: a configurational approach. In: International conference on business process management. Cham: Springer; 2018. p. 426–41.
Mohammadi M, Al-Fuqaha A, Sorour S, Guizani M. Deep learning for IoT big data and streaming analytics: a survey. IEEE Commun Surv Tutor. 2018;20(4):2923–60.
Nambiar R, Bhardwaj R, Sethi A, Vargheese R. A look at challenges and opportunities of big data analytics in healthcare. In: 2013 IEEE international conference on big data; 2013. p. 17–22.
Ohlhorst F. Big data analytics: turning big data into big money, vol. 65. Hoboken: Wiley; 2012.
Olszak C, Mach-Król M. A conceptual framework for assessing an organization’s readiness to adopt big data. Sustainability. 2018;10(10):3734.
Olszak CM. Toward better understanding and use of business intelligence in organizations. Inf Syst Manag. 2016;33(2):105–23.
Palanisamy V, Thirunavukarasu R. Implications of big data analytics in developing healthcare frameworks—a review. J King Saud Univ Comput Inf Sci. 2017;31(4):415–25.
Provost F, Fawcett T. Data science and its relationship to big data and data-driven decisionmaking. Big Data. 2013;1(1):51–9.
Raghupathi W, Raghupathi V. An overview of health analytics. J Health Med Inform. 2013;4:132. https://doi.org/10.4172/2157-7420.1000132 .
Raghupathi W, Raghupathi V. Big data analytics in healthcare: promise and potential. Health Inf Sci Syst. 2014;2(1):3.
Ratia M, Myllärniemi J. Beyond IC 4.0: the future potential of BI-tool utilization in the private healthcare, conference: proceedings IFKAD, 2018 at: Delft, The Netherlands.
Ristevski B, Chen M. Big data analytics in medicine and healthcare. J Integr Bioinform. 2018. https://doi.org/10.1515/jib-2017-0030 .
Rumsfeld JS, Joynt KE, Maddox TM. Big data analytics to improve cardiovascular care: promise and challenges. Nat Rev Cardiol. 2016;13(6):350–9. https://doi.org/10.1038/nrcardio.2016.42 .
Schmarzo B. Big data: understanding how data powers big business. Indianapolis: Wiley; 2013.
Senthilkumar SA, Rai BK, Meshram AA, Gunasekaran A, Chandrakumarmangalam S. Big data in healthcare management: a review of literature. Am J Theor Appl Bus. 2018;4:57–69.
Shubham S, Jain N, Gupta V, et al. Identify glomeruli in human kidney tissue images using a deep learning approach. Soft Comput. 2021. https://doi.org/10.1007/s00500-021-06143-z .
Thuemmler C. The case for health 4.0. In: Thuemmler C, Bai C, editors. Health 4.0: how virtualization and big data are revolutionizing healthcare. New York: Springer; 2017.
Tsai CW, Lai CF, Chao HC, et al. Big data analytics: a survey. J Big Data. 2015;2:21. https://doi.org/10.1186/s40537-015-0030-3 .
Wamba SF, Gunasekaran A, Akter S, Ji-fan RS, Dubey R, Childe SJ. Big data analytics and firm performance: effects of dynamic capabilities. J Bus Res. 2017;70:356–65.
Wang Y, Byrd TA. Business analytics-enabled decision-making effectiveness through knowledge absorptive capacity in health care. J Knowl Manag. 2017;21(3):517–39.
Wang Y, Kung L, Wang W, Yu C, Cegielski CG. An integrated big data analytics-enabled transformation model: application to healthcare. Inf Manag. 2018;55(1):64–79.
Wicks P, et al. Scaling PatientsLikeMe via a “generalized platform” for members with chronic illness: web-based survey study of benefits arising. J Med Internet Res. 2018;20(5):e175.
Willems SM, et al. The potential use of big data in oncology. Oral Oncol. 2019;98:8–12. https://doi.org/10.1016/j.oraloncology.2019.09.003 .
Williams N, Ferdinand NP, Croft R. Project management maturity in the age of big data. Int J Manag Proj Bus. 2014;7(2):311–7.
Winters-Miner LA. Seven ways predictive analytics can improve healthcare. Medical predictive analytics have the potential to revolutionize healthcare around the world. 2014. https://www.elsevier.com/connect/seven-ways-predictive-analytics-can-improve-healthcare (Reading: 15.04.2019).
Wu J, et al. Application of big data technology for COVID-19 prevention and control in China: lessons and recommendations. J Med Internet Res. 2020;22(10): e21980.
Yan L, Peng J, Tan Y. Network dynamics: how can we find patients like us? Inf Syst Res. 2015;26(3):496–512.
Yang JJ, Li J, Mulder J, Wang Y, Chen S, Wu H, Pan H. Emerging information technologies for enhanced healthcare. Comput Ind. 2015;69:3–11.
Zhang Q, Yang LT, Chen Z, Li P. A survey on deep learning for big data. Inf Fusion. 2018;42:146–57.
Download references
Acknowledgements
We would like to thank those who have touched our science paths.
This research was fully funded as statutory activity—subsidy of Ministry of Science and Higher Education granted for Technical University of Czestochowa on maintaining research potential in 2018. Research Number: BS/PB–622/3020/2014/P. Publication fee for the paper was financed by the University of Economics in Katowice.
Author information
Authors and affiliations.
Department of Business Informatics, University of Economics in Katowice, Katowice, Poland
Kornelia Batko
Department of Biomedical Processes and Systems, Institute of Health and Nutrition Sciences, Częstochowa University of Technology, Częstochowa, Poland
Andrzej Ślęzak
You can also search for this author in PubMed Google Scholar
Contributions
KB proposed the concept of research and its design. The manuscript was prepared by KB with the consultation of AŚ. AŚ reviewed the manuscript for getting its fine shape. KB prepared the manuscript in the contexts such as definition of intellectual content, literature search, data acquisition, data analysis, and so on. AŚ obtained research funding. Both authors read and approved the final manuscript.
Corresponding author
Correspondence to Kornelia Batko .
Ethics declarations
Ethics approval and consent to participate.
Not applicable.
Consent for publication
Competing interests.
The author declares no conflict of interest.
Additional information
Publisher's note.
Springer Nature remains neutral with regard to jurisdictional claims in published maps and institutional affiliations.
Rights and permissions
Open Access This article is licensed under a Creative Commons Attribution 4.0 International License, which permits use, sharing, adaptation, distribution and reproduction in any medium or format, as long as you give appropriate credit to the original author(s) and the source, provide a link to the Creative Commons licence, and indicate if changes were made. The images or other third party material in this article are included in the article's Creative Commons licence, unless indicated otherwise in a credit line to the material. If material is not included in the article's Creative Commons licence and your intended use is not permitted by statutory regulation or exceeds the permitted use, you will need to obtain permission directly from the copyright holder. To view a copy of this licence, visit http://creativecommons.org/licenses/by/4.0/ .
Reprints and permissions
About this article
Cite this article.
Batko, K., Ślęzak, A. The use of Big Data Analytics in healthcare. J Big Data 9 , 3 (2022). https://doi.org/10.1186/s40537-021-00553-4
Download citation
Received : 28 August 2021
Accepted : 19 December 2021
Published : 06 January 2022
DOI : https://doi.org/10.1186/s40537-021-00553-4
Share this article
Anyone you share the following link with will be able to read this content:
Sorry, a shareable link is not currently available for this article.
Provided by the Springer Nature SharedIt content-sharing initiative
- Big Data Analytics
- Data-driven healthcare
Study: 47% of data is underutilized in healthcare decision-making
Healthcare leaders agree that data is essential to improve care quality and boost workforce productivity
BOSTON — May 7, 2024 — New research published today by Arcadia ( arcadia.io ), a leading data platform for healthcare, found that although four out of five healthcare leaders believe most of their data is accurate, 47% of healthcare data, on average, is underutilized when making clinical and business decisions. 1 The research highlights a pressing challenge within the healthcare industry: despite the proliferation of AI, a vast amount of data remains untapped, resulting in missed opportunities to enhance patient outcomes, improve productivity, and reduce costs.
“The research demonstrates technology’s potential to breathe new life into healthcare organizations’ underutilized data,” said Dr. Kate Behan , MD, FACP, Chief Medical Officer at Arcadia. “Data analytics platforms are necessary tools and essential enablers for healthcare organizations to turn dormant data into actionable insights that address workforce challenges, reduced revenues, and complex market dynamics.”
The study , conducted by Arcadia in partnership with the Healthcare Information and Management Systems Society (HIMSS), examines how hospitals of various types and sizes use the 137 terabytes of data they produce every day. 2 Key findings include:
- Data supports high-quality care: More than half of healthcare leaders view data as crucial for improving care quality, and one in four said data is necessary to power care management and patient engagement strategies. 1 28% of healthcare leaders said data platforms enable their organizations to enhance health outcomes and patient satisfaction. 1 The findings reinforce the criticality of data to accelerate the transition to value-based care and achieve The Centers for Medicare and Medicaid Services’ goal to transition all traditional Medicare beneficiaries into a value-based care arrangement by 2030.
- Actional information improves productivity and efficiency: 95% of healthcare leaders grasp the opportunity for data and analytics to enable clinicians to improve their productivity, 30% said data platforms lead to cost savings through better resource allocation and workforce management, and 25% said they enable better decision-making. 1 Healthcare organizations navigating workforce shortages, care team burnout, and higher operating expenses can leverage data to create strategies to do more with fewer resources while still delivering quality patient care and supporting their staff. 3
- AI fuels innovation: 84% of leaders have plans to integrate artificial intelligence, machine learning, or large language models with their data platform, and 55% plan to aggregate unstructured data, such as images, audio, or PDFs, to reduce time-consuming manual review and unlock insights, like an undocumented condition, to better inform care delivery. One healthcare organization used Arcadia's clinical co-pilot, Arcadia SageAI™ , to reduce the time needed to prepare for a patient visit by more than 20%. 4
- Technology makes data more useable: Healthcare leaders named enhancing data literacy (58%), using AI (47%), and addressing productivity challenges (34%) as key priorities to make data more usable. 1 Moreover, 76% of large organizations (those with 15,000+ employees) said implementing a comprehensive enterprise data solution is essential. 1 Larger organizations have the greatest need to connect disparate data sources to derive comprehensive insights and actions across the enterprise. 5
- Accelerating data recency remains an opportunity: 61% of organizations refresh their data at least daily for business intelligence analytics, but when building and running artificial intelligence models, that number drops to 32%. 1 Only 47% of organizations leverage daily refreshed data for generating lists of care gaps and just 39% for patient stratification and building cohorts. 1 Activating data in real-time provides care teams with immediate access to the most current patient information, which ensures providers make decisions using the freshest insights. 6
“Our findings show that healthcare leaders view data analytics platforms as critical tools to confront growing workforce shortages, financial pressures, and the shift towards value-based care,” said Michael Meucci , President and CEO of Arcadia. “Investing in a strong data analytics platform can further transform these challenges into opportunities and progress towards a more efficient and effective healthcare system.”
Download the report to discover how healthcare leaders plan to deploy a data analytics platform to power future innovations within their organizations.
1 HIMSS Market Insights Survey, Data Analytics Platforms , 2024
2 “ 4 ways data is improving healthcare ,” World Economic Forum, 2019
3 “ The use of Big Data Analytics in healthcare ,” Journal of Big Data, 2022
4 Arcadia product performance measured in Q1 2024
5 “ Sharing Data, Saving Lives: The Hospital Agenda for Interoperability ,” American Hospital Association, 2019
6 “ How Healthcare is Leveraging Real-World Data to Improve Outcomes ,” Health IT Analytics, 2020
TechRepublic
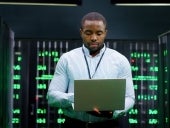
8 Best Data Science Tools and Software
Apache Spark and Hadoop, Microsoft Power BI, Jupyter Notebook and Alteryx are among the top data science tools for finding business insights. Compare their features, pros and cons.
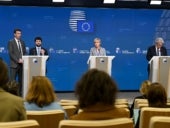
EU’s AI Act: Europe’s New Rules for Artificial Intelligence
Europe's AI legislation, adopted March 13, attempts to strike a tricky balance between promoting innovation and protecting citizens' rights.
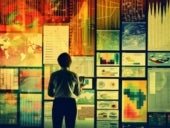
10 Best Predictive Analytics Tools and Software for 2024
Tableau, TIBCO Data Science, IBM and Sisense are among the best software for predictive analytics. Explore their features, pricing, pros and cons to find the best option for your organization.
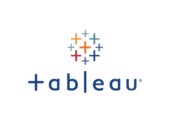
Tableau Review: Features, Pricing, Pros and Cons
Tableau has three pricing tiers that cater to all kinds of data teams, with capabilities like accelerators and real-time analytics. And if Tableau doesn’t meet your needs, it has a few alternatives worth noting.
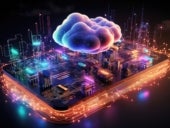
Top 6 Enterprise Data Storage Solutions for 2024
Amazon, IDrive, IBM, Google, NetApp and Wasabi offer some of the top enterprise data storage solutions. Explore their features and benefits, and find the right solution for your organization's needs.
Latest Articles
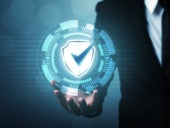
How Can Businesses Defend Themselves Against Common Cyberthreats?
TechRepublic consolidated expert advice on how businesses can defend themselves against the most common cyberthreats, including zero-days, ransomware and deepfakes.
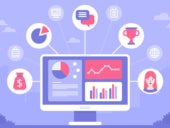
Top 10 CRM Features and Functionalities
Discover the top CRM features for business success. Explore a curated list of key capabilities to consider when choosing the right CRM solution, including marketing tools, activity tracking and more.
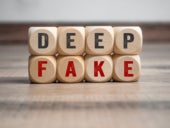
Combatting Deepfakes in Australia: Content Credentials is the Start
The production of deepfakes is accelerating at more than 1,500% in Australia, forcing organisations to create and adopt standards like Content Credentials.
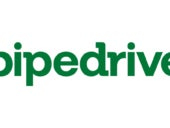
The Top 5 Pipedrive Alternatives for 2024
Discover the top alternatives to Pipedrive. Explore a curated list of CRM platforms with similar features, pricing and pros and cons to find the best fit for your business.
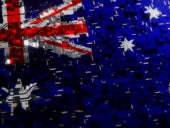
The Australian Government’s Manufacturing Objectives Rely on IT Capabilities
The intent of the Future Made in Australia Act is to build manufacturing capabilities across all sectors, which will likely lead to more demand for IT skills and services.
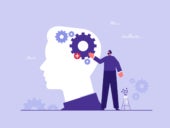
Udemy Report: Which IT Skills Are Most in Demand in Q1 2024?
Informatica PowerCenter, Microsoft Playwright and Oracle Database SQL top Udemy’s list of most popular tech courses.
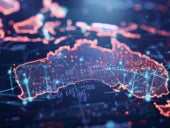
Gartner: 4 Bleeding-Edge Technologies in Australia
Gartner recently identified emerging tech that will impact enterprise leaders in APAC. Here’s what IT leaders in Australia need to know about these innovative technologies.
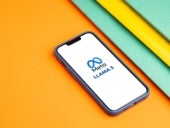
Llama 3 Cheat Sheet: A Complete Guide for 2024
Learn how to access Meta’s new AI model Llama 3, which sets itself apart by being open to use under a license agreement.
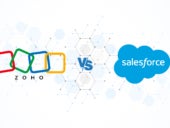
Zoho vs Salesforce (2024): Which CRM Is Better?
Look at Zoho CRM and Salesforce side-by-side to compare the cost per functionality and top pros and of each provider to determine which is better for your business needs.
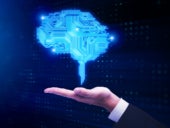
9 Innovative Use Cases of AI in Australian Businesses in 2024
Australian businesses are beginning to effectively grapple with AI and build solutions specific to their needs. Here are notable use cases of businesses using AI.
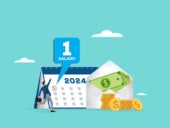
How Are APAC Tech Salaries Faring in 2024?
The year 2024 is bringing a return to stable tech salary growth in APAC, with AI and data jobs leading the way. This follows downward salary pressure in 2023, after steep increases in previous years.
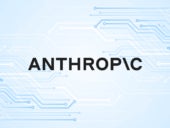
Anthropic Releases Claude Team Enterprise AI Plan and iOS App
The enterprise plan seeks to fill a need for generative AI tools for small and medium businesses. Plus, a Claude app is now on iOS.
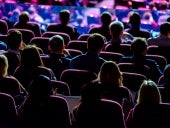
Top Tech Conferences & Events to Add to Your Calendar in 2024
A great way to stay current with the latest technology trends and innovations is by attending conferences. Read and bookmark our 2024 tech events guide.
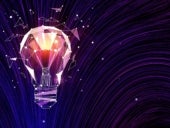
TechRepublic Premium Editorial Calendar: Policies, Checklists, Hiring Kits and Glossaries for Download
TechRepublic Premium content helps you solve your toughest IT issues and jump-start your career or next project.
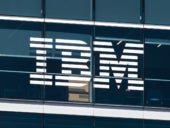
IBM Acquires HashiCorp for $6.4 Billion, Expanding Hybrid Cloud Offerings
The deal is intended to strengthen IBM’s hybrid and multicloud offerings and generative AI deployment.
Create a TechRepublic Account
Get the web's best business technology news, tutorials, reviews, trends, and analysis—in your inbox. Let's start with the basics.
* - indicates required fields
Sign in to TechRepublic
Lost your password? Request a new password
Reset Password
Please enter your email adress. You will receive an email message with instructions on how to reset your password.
Check your email for a password reset link. If you didn't receive an email don't forgot to check your spam folder, otherwise contact support .
Welcome. Tell us a little bit about you.
This will help us provide you with customized content.
Want to receive more TechRepublic news?
You're all set.
Thanks for signing up! Keep an eye out for a confirmation email from our team. To ensure any newsletters you subscribed to hit your inbox, make sure to add [email protected] to your contacts list.
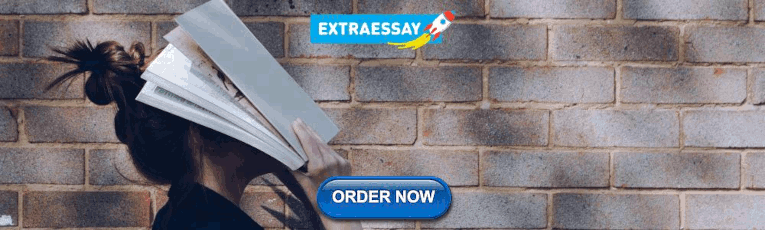
COMMENTS
'Big data' is massive amounts of information that can work wonders. It has become a topic of special interest for the past two decades because of a great potential that is hidden in it. Various public and private sector industries generate, store, and analyze big data with an aim to improve the services they provide. In the healthcare industry, various sources for big data include hospital ...
Despite these challenges, several new technological improvements are allowing healthcare big data to be converted to useful, actionable information. By leveraging appropriate software tools, big data is informing the movement toward value-based healthcare and is opening the door to remarkable advancements, even while reducing costs. With the wealth of information that healthcare data analytics ...
2. Promises. In the era of genomics, the volume of data being captured from biological experiments and routine health care procedures is growing at an unprecedented pace 4.This data trove has brought new promises for discovery in health care research and breakthrough treatments as well as new challenges in technology, management, and dissemination of knowledge.
The use of Big Data in healthcare, in fact, can contribute at different levels as reported by the Study on Big Data in Public Health, Telemedicine and Healthcare of the European Commission: 9 (i) ... Collaborations are of extremely high importance especially in the case of paediatric or other rare types of cancer, where the data collected for ...
Adibuzzaman M, DeLaurentis P, Hill J, Benneyworth BD (2018) Big data in healthcare—the promises, challenges and opportunities from a research perspective: a case study with a model database ...
The cost pressure in the US system is not a new phenomenon, since healthcare expenses have been rising rapidly over the last two decades. By 2009, they represented 17.6 percent of GDP—nearly $600 billion more than the expected benchmark for a nation of the United States' size and wealth.
Big data analytics in healthcare, studies in big data 66. Cham: Springer; 2020. p. 3-21. Raghupati W, Raghupathi V. Big data analytics in healthcare: promise and potential. Health Inf Sci Syst Vol. 2014;2(1):1-10. Jain DA, Kumar V, Khanduja D, Sharma K, Bateja R. A detailed study of big data in healthcare: case study of Brenda and IBM Watson.
Systematic literature reviews and meta-analysis has gained significant attention and became increasingly important in healthcare domain. Clinicians, developers and researchers follow SLR studies ...
The enormous data sets made possible by big data are essential to digital health because they fuel innovation and lead to better results for patients. 1.1 Big data analytics (BDA) for EHRs. The analysis of EHRs and other sources of health-related data is one of the key uses of big data in digital health . EHRs include a wealth of information ...
A big-data revolution is under way in health care. Start with the vastly increased supply of information. Over the last decade, pharmaceutical companies have been aggregating years of research and development data into medical databases, while payors and providers have digitized their patient records. Meanwhile, the US federal government and ...
The use of Big Data Analytics can literally revolutionize the way healthcare is practiced for better health and disease reduction. The analysis of the latest data reveals that data analytics increase the accuracy of diagnoses. Physicians can use predictive algorithms to help them make more accurate diagnoses [ 45 ].
The promise of Big Data in healthcare depends on the ability to extract meaningful information from real-world, large-scale resources that may pave the way to scientific discoveries in the pathogenesis, diagnosis, prevention, treatment, and prognosis of diseases, and eventually revolutionize clinical medicine and public health [ 11, 12, 13 ].
Studies in the sample were published in 20 peer-reviewed journals and 17 conference proceedings (see Exhibit A). Among the 13 publishers that contributed to the sample of the current study, the leading sources are IEEE Access (3 studies), Big Data (2 studies), and Information and Management (2 studies). IEEE (17 studies) is the most prominent ...
By Jessica Kent. December 10, 2020 - Researchers at Johns Hopkins Bloomberg School of Public Health have developed a series of case studies for public health issues that will enable healthcare leaders to use big data analytics tools in their work. The Open Case Studies project offers an interactive online hub made up of ten case studies that ...
3) Real-Time Alerting. Other examples of data analytics in healthcare share one crucial functionality - real-time alerting. In hospitals, Clinical Decision Support (CDS) software analyzes medical data on the spot, providing health practitioners with advice as they make prescriptive decisions.
Big data has the potential to create significant value in health care by improving outcomes while lowering costs. ... Unstructured medical image query using big data - An epilepsy case study ...
Data Analytics in Healthcare: 7 Real-World Examples and Use Cases. There are few things in the world requiring such precision as clinical decision-making. The adoption of technologies supports healthcare organizations on different levels: from population monitoring, health records, diagnostics, and clinical decisions, to drug procurement, and ...
Abstract and Figures. A case studies brief by Cenacle Research presenting a variety of Healthcare Analytics solutions crafted to the needs of: + Individuals (Patients) Personalized Healthcare ...
Big data in healthcare - the promises, challenges and opportunities from a research perspective: A case study with a model database AMIA Annu Symp Proc . 2018 Apr 16:2017:384-392. eCollection 2017.
We conducted a literature review using the Scopus database over the period 2010-2020. The article selection process involved five steps: the planning and identification of studies, the evaluation of articles, the extraction of results, the summary, and the dissemination of the audit results. We included 93 documents.
The most important use case for big data in health care is, of course, electronic health records (EHRs). The use of EHRs is supported by the HITECH act and governed by HIPAA rules and standards. Efficient use and analysis of EHRs can improve care coordination and reduce healthcare costs as sharing data between doctors and care providers can ...
In addition, a Hadoop-based architecture and a conceptual data model for designing medical Big Data warehouse are given. In our case study, we provide implementation detail of big data warehouse based on the proposed architecture and data model in the Apache Hadoop platform to ensure an optimal allocation of health resources.
A recent digital healthcare CAGR forecast by PMI estimates 17.4% growth over the next decade, taking total market size from $283 BN in 2024 to $1406 BN in 2034. Healthcare's digital infrastructure has traditionally been both centralized and specialized. To enable resilience, support new dispersed networks and research and drive consumer-style ...
The introduction of Big Data Analytics (BDA) in healthcare will allow to use new technologies both in treatment of patients and health management. The paper aims at analyzing the possibilities of using Big Data Analytics in healthcare. The research is based on a critical analysis of the literature, as well as the presentation of selected results of direct research on the use of Big Data ...
BOSTON — May 7, 2024 — New research published today by Arcadia ( arcadia.io ), a leading data platform for healthcare, found that although four out of five healthcare leaders believe most of their data is accurate, 47% of healthcare data, on average, is underutilized when making clinical and business decisions. 1 The research highlights a ...
Big Data Big Data Tableau Review: Features, Pricing, Pros and Cons . Tableau has three pricing tiers that cater to all kinds of data teams, with capabilities like accelerators and real-time analytics.