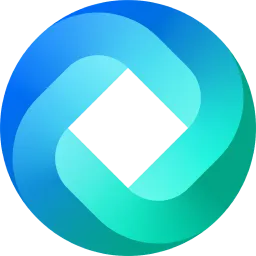
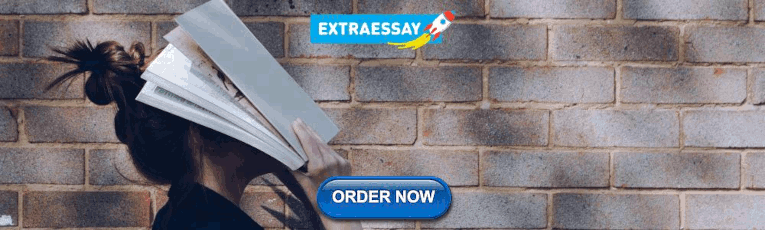
AI Literature Review Generator
Generate high-quality literature reviews fast with ai.
- Academic Research: Create a literature review for your thesis, dissertation, or research paper.
- Professional Research: Conduct a literature review for a project, report, or proposal at work.
- Content Creation: Write a literature review for a blog post, article, or book.
- Personal Research: Conduct a literature review to deepen your understanding of a topic of interest.
New & Trending Tools
Job search ai assistant, nursing school ai assistant, university administrator ai assistant.
Revolutionize Your Research with Jenni AI
Literature Review Generator
Welcome to Jenni AI, the ultimate tool for researchers and students. Our AI Literature Review Generator is designed to assist you in creating comprehensive, high-quality literature reviews, enhancing your academic and research endeavors. Say goodbye to writer's block and hello to seamless, efficient literature review creation.

Loved by over 1 million academics

Endorsed by Academics from Leading Institutions
Join the Community of Scholars Who Trust Jenni AI

Elevate Your Research Toolkit
Discover the Game-Changing Features of Jenni AI for Literature Reviews
Advanced AI Algorithms
Jenni AI utilizes cutting-edge AI technology to analyze and suggest relevant literature, helping you stay on top of current research trends.
Get started

Idea Generation
Overcome writer's block with AI-generated prompts and ideas that align with your research topic, helping to expand and deepen your review.
Citation Assistance
Get help with proper citation formats to maintain academic integrity and attribute sources correctly.

Our Pledge to Academic Integrity
At Jenni AI, we are deeply committed to the principles of academic integrity. We understand the importance of honesty, transparency, and ethical conduct in the academic community. Our tool is designed not just to assist in your research, but to do so in a way that respects and upholds these fundamental values.
How it Works
Start by creating your account on Jenni AI. The sign-up process is quick and user-friendly.
Define Your Research Scope
Enter the topic of your literature review to guide Jenni AI’s focus.
Citation Guidance
Receive assistance in citing sources correctly, maintaining the academic standard.
Easy Export
Export your literature review to LaTeX, HTML, or .docx formats
Interact with AI-Powered Suggestions
Use Jenni AI’s suggestions to structure your literature review, organizing it into coherent sections.
What Our Users Say
Discover how Jenni AI has made a difference in the lives of academics just like you

· Aug 26
I thought AI writing was useless. Then I found Jenni AI, the AI-powered assistant for academic writing. It turned out to be much more advanced than I ever could have imagined. Jenni AI = ChatGPT x 10.

Charlie Cuddy
@sonofgorkhali
· 23 Aug
Love this use of AI to assist with, not replace, writing! Keep crushing it @Davidjpark96 💪

Waqar Younas, PhD
@waqaryofficial
· 6 Apr
4/9 Jenni AI's Outline Builder is a game-changer for organizing your thoughts and structuring your content. Create detailed outlines effortlessly, ensuring your writing is clear and coherent. #OutlineBuilder #WritingTools #JenniAI

I started with Jenni-who & Jenni-what. But now I can't write without Jenni. I love Jenni AI and am amazed to see how far Jenni has come. Kudos to http://Jenni.AI team.

· 28 Jul
Jenni is perfect for writing research docs, SOPs, study projects presentations 👌🏽

Stéphane Prud'homme
http://jenni.ai is awesome and super useful! thanks to @Davidjpark96 and @whoisjenniai fyi @Phd_jeu @DoctoralStories @WriteThatPhD
Frequently asked questions
What exactly does jenni ai do, is jenni ai suitable for all academic disciplines, is there a trial period or a free version available.
How does Jenni AI help with writer's block?
Can Jenni AI write my literature review for me?
How often is the literature database updated in Jenni AI?
How user-friendly is Jenni AI for those not familiar with AI tools?
Jenni AI: Standing Out From the Competition
In a sea of online proofreaders, Jenni AI stands out. Here’s how we compare to other tools on the market:
Feature Featire
COMPETITORS
Advanced AI-Powered Assistance
Uses state-of-the-art AI technology to provide relevant literature suggestions and structural guidance.
May rely on simpler algorithms, resulting in less dynamic or comprehensive support.
User-Friendly Interface
Designed for ease of use, making it accessible for users with varying levels of tech proficiency.
Interfaces can be complex or less intuitive, posing a challenge for some users.
Transparent and Flexible Pricing
Offers a free trial and clear, flexible pricing plans suitable for different needs.
Pricing structures can be opaque or inflexible, with fewer user options.
Unparalleled Customization
Offers highly personalized suggestions and adapts to your specific research needs over time.
Often provide generic suggestions that may not align closely with individual research topics.
Comprehensive Literature Access
Provides access to a vast and up-to-date range of academic literature, ensuring comprehensive research coverage.
Some may have limited access to current or diverse research materials, restricting the scope of literature reviews.
Ready to Transform Your Research Process?
Don't wait to elevate your research. Sign up for Jenni AI today and discover a smarter, more efficient way to handle your academic literature reviews.
Analyze research papers at superhuman speed
Search for research papers, get one sentence abstract summaries, select relevant papers and search for more like them, extract details from papers into an organized table.
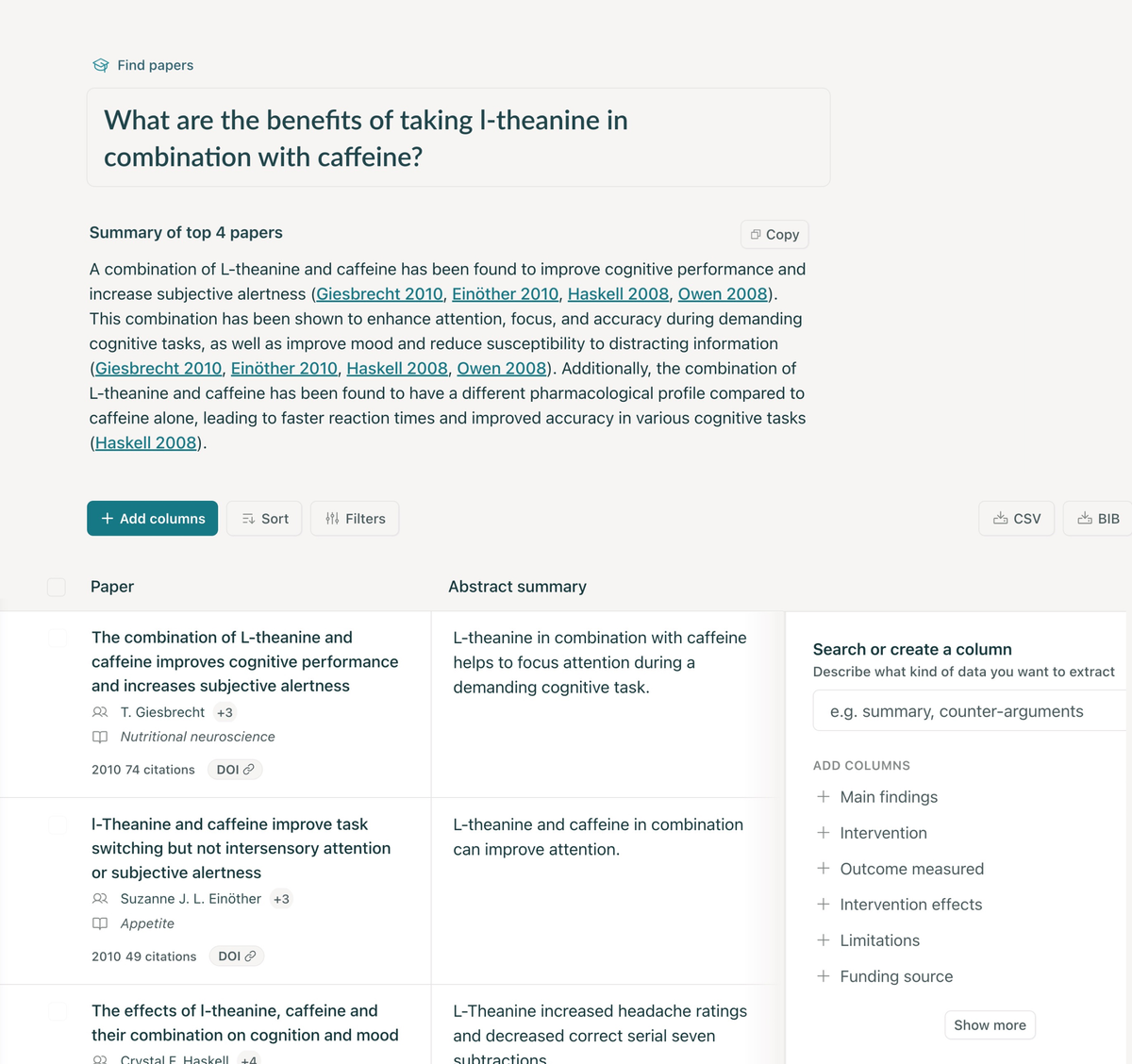
Find themes and concepts across many papers
Don't just take our word for it.
.webp)
Tons of features to speed up your research
Upload your own pdfs, orient with a quick summary, view sources for every answer, ask questions to papers, research for the machine intelligence age, pick a plan that's right for you, get in touch, enterprise and institutions, custom pricing, common questions. great answers., how do researchers use elicit.
Over 2 million researchers have used Elicit. Researchers commonly use Elicit to:
- Speed up literature review
- Find papers they couldn’t find elsewhere
- Automate systematic reviews and meta-analyses
- Learn about a new domain
Elicit tends to work best for empirical domains that involve experiments and concrete results. This type of research is common in biomedicine and machine learning.
What is Elicit not a good fit for?
Elicit does not currently answer questions or surface information that is not written about in an academic paper. It tends to work less well for identifying facts (e.g. “How many cars were sold in Malaysia last year?”) and theoretical or non-empirical domains.
What types of data can Elicit search over?
Elicit searches across 125 million academic papers from the Semantic Scholar corpus, which covers all academic disciplines. When you extract data from papers in Elicit, Elicit will use the full text if available or the abstract if not.
How accurate are the answers in Elicit?
A good rule of thumb is to assume that around 90% of the information you see in Elicit is accurate. While we do our best to increase accuracy without skyrocketing costs, it’s very important for you to check the work in Elicit closely. We try to make this easier for you by identifying all of the sources for information generated with language models.
What is Elicit Plus?
Elicit Plus is Elicit's subscription offering, which comes with a set of features, as well as monthly credits. On Elicit Plus, you may use up to 12,000 credits a month. Unused monthly credits do not carry forward into the next month. Plus subscriptions auto-renew every month.
What are credits?
Elicit uses a credit system to pay for the costs of running our app. When you run workflows and add columns to tables it will cost you credits. When you sign up you get 5,000 credits to use. Once those run out, you'll need to subscribe to Elicit Plus to get more. Credits are non-transferable.
How can you get in contact with the team?
Please email us at [email protected] or post in our Slack community if you have feedback or general comments! We log and incorporate all user comments. If you have a problem, please email [email protected] and we will try to help you as soon as possible.
What happens to papers uploaded to Elicit?
When you upload papers to analyze in Elicit, those papers will remain private to you and will not be shared with anyone else.
How accurate is Elicit?
Training our models on specific tasks, searching over academic papers, making it easy to double-check answers, save time, think more. try elicit for free..
A free, AI-powered research tool for scientific literature
- Economic Growth
- Reforestation
New & Improved API for Developers
Introducing semantic reader in beta.
Stay Connected With Semantic Scholar Sign Up What Is Semantic Scholar? Semantic Scholar is a free, AI-powered research tool for scientific literature, based at the Allen Institute for AI.
RAxter is now Enago Read! Enjoy the same licensing and pricing with enhanced capabilities. No action required for existing customers.
Your all in one AI-powered Reading Assistant
A Reading Space to Ideate, Create Knowledge, and Collaborate on Your Research
- Smartly organize your research
- Receive recommendations that cannot be ignored
- Collaborate with your team to read, discuss, and share knowledge
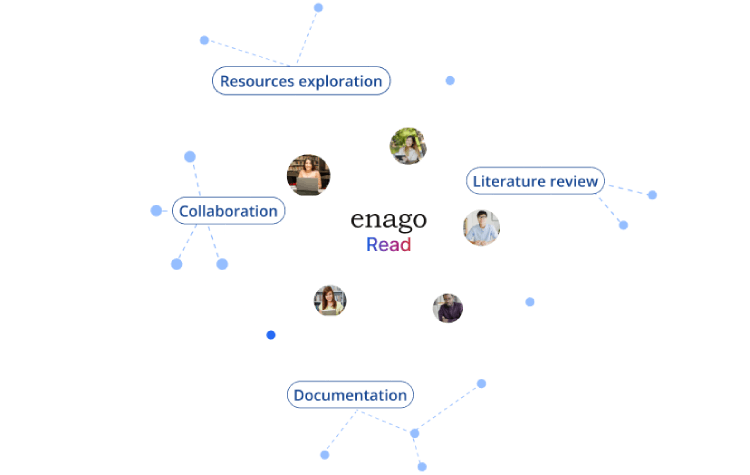
From Surface-Level Exploration to Critical Reading - All in one Place!
Fine-tune your literature search.
Our AI-powered reading assistant saves time spent on the exploration of relevant resources and allows you to focus more on reading.
Select phrases or specific sections and explore more research papers related to the core aspects of your selections. Pin the useful ones for future references.
Our platform brings you the latest research related to your and project work.
Speed up your literature review
Quickly generate a summary of key sections of any paper with our summarizer.
Make informed decisions about which papers are relevant, and where to invest your time in further reading.
Get key insights from the paper, quickly comprehend the paper’s unique approach, and recall the key points.
Bring order to your research projects
Organize your reading lists into different projects and maintain the context of your research.
Quickly sort items into collections and tag or filter them according to keywords and color codes.
Experience the power of sharing by finding all the shared literature at one place.
Decode papers effortlessly for faster comprehension
Highlight what is important so that you can retrieve it faster next time.
Select any text in the paper and ask Copilot to explain it to help you get a deeper understanding.
Ask questions and follow-ups from AI-powered Copilot.
Collaborate to read with your team, professors, or students
Share and discuss literature and drafts with your study group, colleagues, experts, and advisors. Recommend valuable resources and help each other for better understanding.
Work in shared projects efficiently and improve visibility within your study group or lab members.
Keep track of your team's progress by being constantly connected and engaging in active knowledge transfer by requesting full access to relevant papers and drafts.
Find papers from across the world's largest repositories
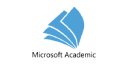
Testimonials
Privacy and security of your research data are integral to our mission..
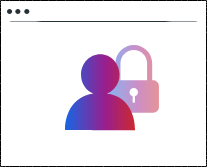
Everything you add or create on Enago Read is private by default. It is visible if and when you share it with other users.
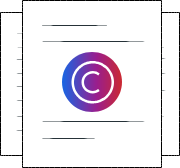
You can put Creative Commons license on original drafts to protect your IP. For shared files, Enago Read always maintains a copy in case of deletion by collaborators or revoked access.
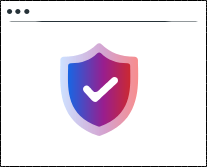
We use state-of-the-art security protocols and algorithms including MD5 Encryption, SSL, and HTTPS to secure your data.
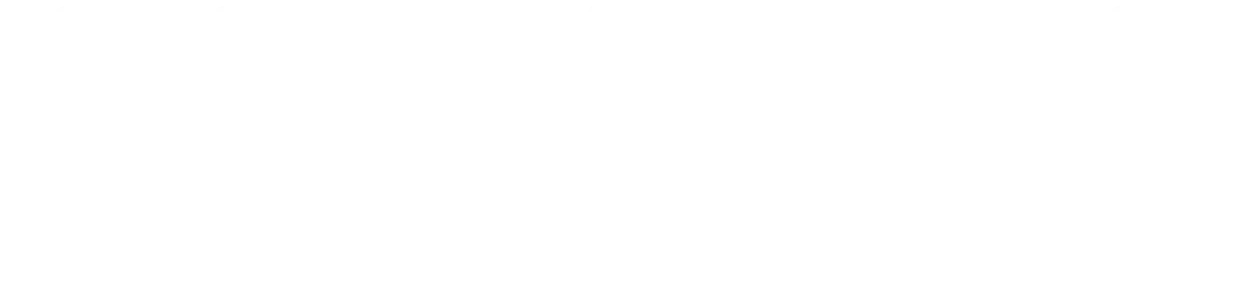
- Help Center
GET STARTED
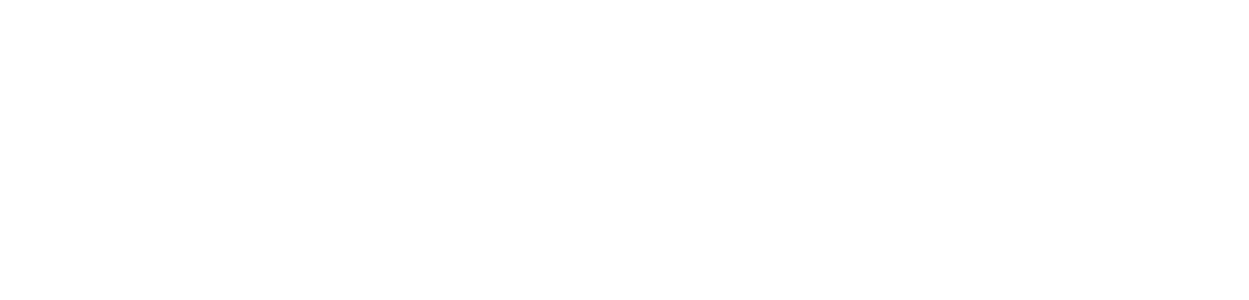
COLLABORATE ON YOUR REVIEWS WITH ANYONE, ANYWHERE, ANYTIME
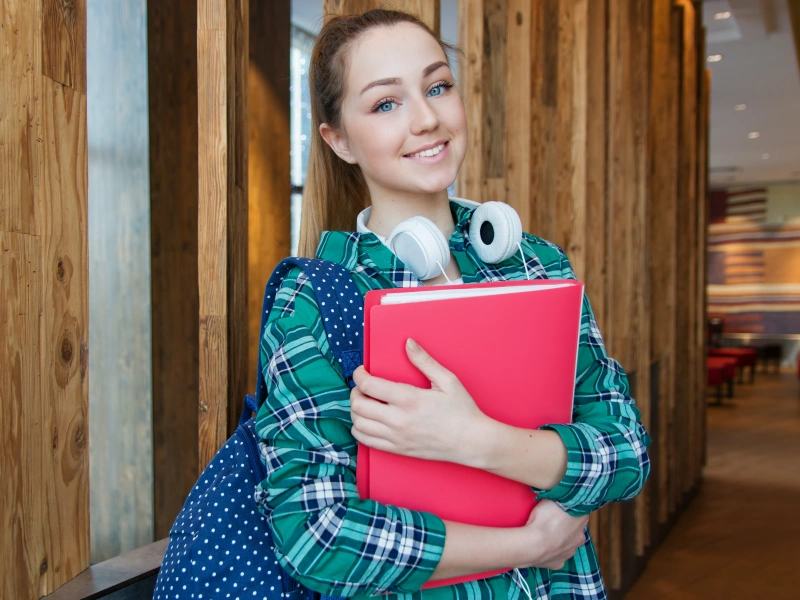
Save precious time and maximize your productivity with a Rayyan membership. Receive training, priority support, and access features to complete your systematic reviews efficiently.
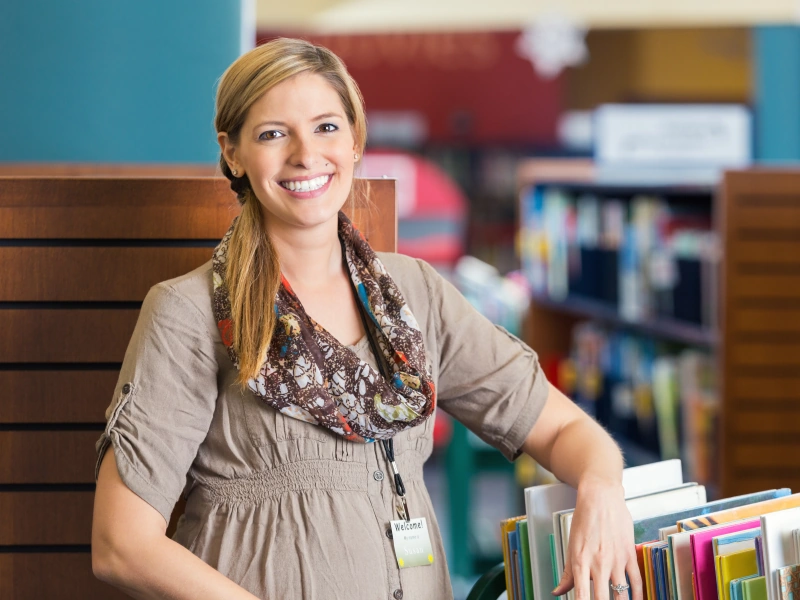
Rayyan Teams+ makes your job easier. It includes VIP Support, AI-powered in-app help, and powerful tools to create, share and organize systematic reviews, review teams, searches, and full-texts.
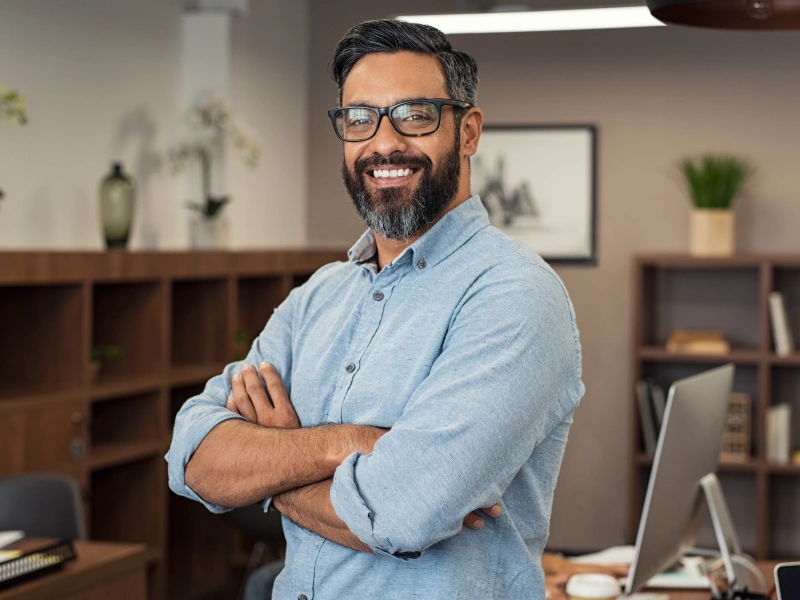
RESEARCHERS
Rayyan makes collaborative systematic reviews faster, easier, and more convenient. Training, VIP support, and access to new features maximize your productivity. Get started now!
Over 1 billion reference articles reviewed by research teams, and counting...
Intelligent, scalable and intuitive.
Rayyan understands language, learns from your decisions and helps you work quickly through even your largest systematic literature reviews.
WATCH A TUTORIAL NOW
Solutions for Organizations and Businesses
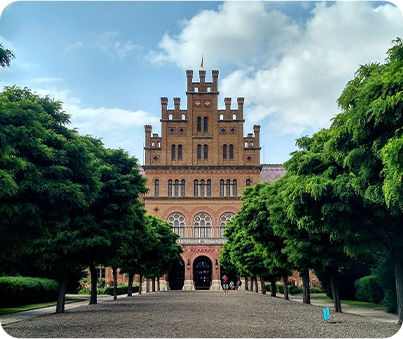
Rayyan Enterprise and Rayyan Teams+ make it faster, easier and more convenient for you to manage your research process across your organization.
- Accelerate your research across your team or organization and save valuable researcher time.
- Build and preserve institutional assets, including literature searches, systematic reviews, and full-text articles.
- Onboard team members quickly with access to group trainings for beginners and experts.
- Receive priority support to stay productive when questions arise.
- SCHEDULE A DEMO
- LEARN MORE ABOUT RAYYAN TEAMS+
RAYYAN SYSTEMATIC LITERATURE REVIEW OVERVIEW
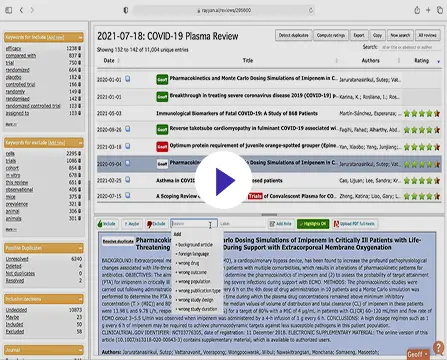
LEARN ABOUT RAYYAN’S PICO HIGHLIGHTS AND FILTERS
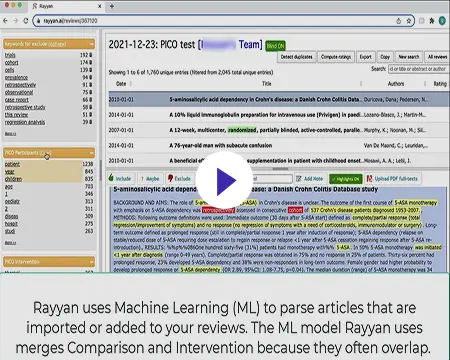
Join now to learn why Rayyan is trusted by already more than 500,000 researchers
Individual plans, teams plans.
For early career researchers just getting started with research.
Free forever
- 3 Active Reviews
- Invite Unlimited Reviewers
- Import Directly from Mendeley
- Industry Leading De-Duplication
- 5-Star Relevance Ranking
- Advanced Filtration Facets
- Mobile App Access
- 100 Decisions on Mobile App
- Standard Support
- Revoke Reviewer
- Online Training
- PICO Highlights & Filters
- PRISMA (Beta)
- Auto-Resolver
- Multiple Teams & Management Roles
- Monitor & Manage Users, Searches, Reviews, Full Texts
- Onboarding and Regular Training
Professional
For researchers who want more tools for research acceleration.
Per month billed annually
- Unlimited Active Reviews
- Unlimited Decisions on Mobile App
- Priority Support
- Auto-Resolver
For currently enrolled students with valid student ID.
Per month billed annually
Billed monthly
For a team that wants professional licenses for all members.
Per-user, per month, billed annually
- Single Team
- High Priority Support
For teams that want support and advanced tools for members.
- Multiple Teams
- Management Roles
For organizations who want access to all of their members.
Annual Subscription
Contact Sales
- Organizational Ownership
- For an organization or a company
- Access to all the premium features such as PICO Filters, Auto-Resolver, PRISMA and Mobile App
- Store and Reuse Searches and Full Texts
- A management console to view, organize and manage users, teams, review projects, searches and full texts
- Highest tier of support – Support via email, chat and AI-powered in-app help
- GDPR Compliant
- Single Sign-On
- API Integration
- Training for Experts
- Training Sessions Students Each Semester
- More options for secure access control
ANNUAL ONLY
Per-user, billed monthly
Rayyan Subscription
membership starts with 2 users. You can select the number of additional members that you’d like to add to your membership.
Total amount:
Click Proceed to get started.
Great usability and functionality. Rayyan has saved me countless hours. I even received timely feedback from staff when I did not understand the capabilities of the system, and was pleasantly surprised with the time they dedicated to my problem. Thanks again!
This is a great piece of software. It has made the independent viewing process so much quicker. The whole thing is very intuitive.
Rayyan makes ordering articles and extracting data very easy. A great tool for undertaking literature and systematic reviews!
Excellent interface to do title and abstract screening. Also helps to keep a track on the the reasons for exclusion from the review. That too in a blinded manner.
Rayyan is a fantastic tool to save time and improve systematic reviews!!! It has changed my life as a researcher!!! thanks
Easy to use, friendly, has everything you need for cooperative work on the systematic review.
Rayyan makes life easy in every way when conducting a systematic review and it is easy to use.

AI Assistance in Academia for Searching Credible Scholarly Sources
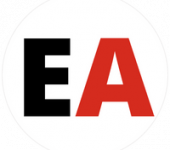
The journey of academia is a grand quest for knowledge, more specifically an adventure to find the right information through credible sources, and that’s where scholarly sources walk in. As the name suggests, it simply means that such sources are written by scholars and experts of a specialized field. These sources are in the form of journal articles, books, conference publications, or websites. Such resources undergo a stringent peer-review process by a panel of subject matter experts. Thus, the findings presented are credible and refined. In contrast, popular sources, such as newspapers, magazines, and blogs, often lack the meticulous scrutiny of peer review and may prioritize approachability over technical accuracy.
Let’s explore the key points of difference between scholarly sources and popular sources:
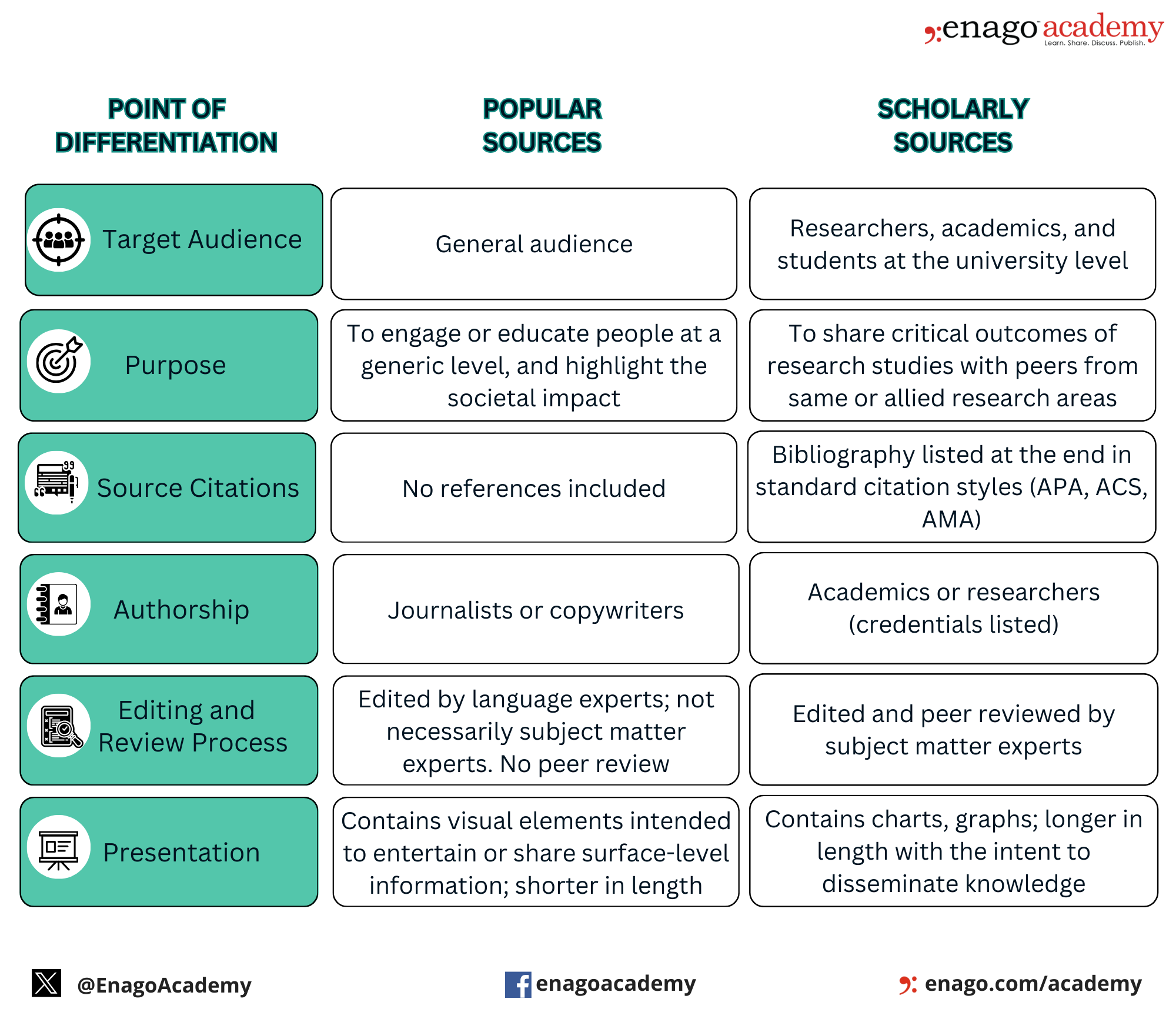
Table of Contents
Why Cite Scholarly Sources When Writing Your Research Paper?
Selecting scholarly sources is a strategic decision that affects your research outcome, as it builds the foundation of your academic work. It plays a crucial role for individuals at every stage of their academic journey, benefiting both early-career and established researchers. It allows scholars to actively participate in academic conversations by sharing valuable insights in their field, inspiring others in the research area to make advancements. Furthermore, for a university student, making their first stride in scholarly research, it enhances their knowledge and serves as a gateway to join the scholarly community.
Curious about the nuances of literature review in academia? Take our short quiz now to deepen your understanding of this essential academic practice.
Fill the Details to Check Your Score
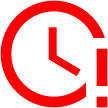
Exploring Barriers in Scholarly Literature Search
Exploring today’s vast information landscape is like finding a needle in a haystack. This mirrors the challenges researchers face with the overwhelming number of scholarly articles in today’s digital age. According to the National Center for Science and Engineering Statistics , scientists publish nearly 3 million publications each year. A simple query search on platforms like Google Scholar can result in an overwhelming number of articles, posing a challenge to navigate through them and gain a comprehensive overview. Furthermore, such scholarly search platforms often fail to reveal new findings in a particular field as the system tends to favor highly cited , older articles.
Modern researchers and students face further complex challenges as science becomes more specialized, introducing peculiar jargon and keywords. This coupled with the multidisciplinary nature of research, can result in overlooking valuable findings from other fields due to specific jargon and knowledgebase constraints. Other challenges include identifying and selecting relevant resources due to the diverse formats in which information is presented (journal articles, preprints, conference posters, etc.), selecting which literature to choose with precision to prevent unintended plagiarism , and software limitations in managing literature sources in different formats.
Understanding the Use of AI Research Assistance Tools for Reliable Scholarly Sources
In this world of the internet, where a single click generates thousands of search results, AI research assistance tools can guide you through this chaos to the right scholarly source. These tools automate the process of identifying reliable papers, finding and summarizing relevant sections of a research paper , analyzing it to bridge knowledge gaps, and generating potential project ideas. Listed below are the pros and cons of such tools to help you make informed decisions when incorporating these tools into your research workflow:
4 Essential Research Assistance AI Tools
Currently, there are numerous AI-based tools with a wide range of capabilities for literature review assistance , each tailored to specific requirements. It is imperative to make an informed decision based on certain evaluation parameters that sets each of these tools apart. Simplifying this choice for you, we have compared 4 most useful AI research assistance tools to understand their distinctiveness:
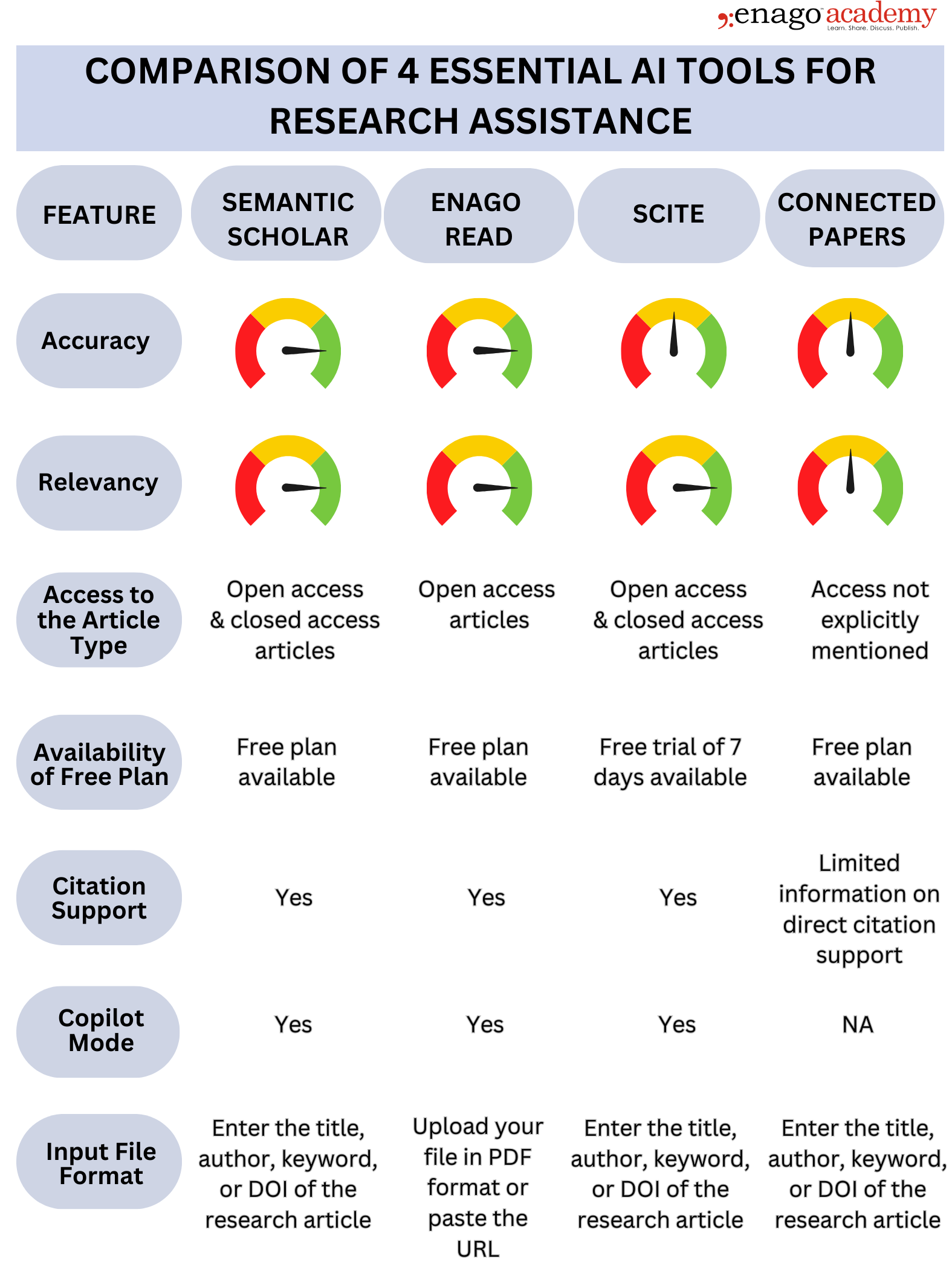
Here is a comprehensive analysis of 4 most useful AI research assistance tools for a thorough understanding of their capabilities:
1. Semantic Scholar
Semantic scholar is an AI-powered search engine that:
- Conducts contextual analysis of papers.
- Offers advanced citation analysis and automates the process of identifying relevant research papers from its database of over 200 million papers for literature reviews.
- Bridges knowledge gaps and prompts project ideas.
2. Enago Read
Enago Read offers several features to enhance the experience of researchers for literature exploration and research process. They include:
- Summarizes key sections of papers, allowing for quick insight into the content of a research article.
- Supports inline attachments, ensuring a seamless organization of project-related files.
- Provides AI-powered suggestions to discover related research material and stay updated with relevant news from the large data repository of 170+ million research papers.
- Promotes enhanced collaboration and structured note-taking for better team communication, accelerating the process of literature review and critical reading.
- Streamlines the management of references , contributing to a more efficient and organized research workflow.
Scite is an AI research tool that:
- Provides services to enhance the research experience, including smart citations that support, contrast, and mention relevant content
- Presents citation contexts with accompanying text excerpts, allowing for a more in-depth understanding from over 181 million papers.
- Facilitates thorough literature reviews, making it a valuable tool for researchers engaging in systematic reviews and meta-analyses
4. Connected Papers
Connected Papers aids in identifying gaps in literature reviews in the following ways:
- Provides valuable insights for your scholarly venture
- Visualizes the research landscape, helping you identify key papers and trends
- Deepens your understanding of research relationships by exploring paper interconnections
While many AI research assistant tools help in identifying and storing scholarly sources, the real challenge is in efficiently connecting them. You must try and choose a platform that acts as an all-in-one solution. A platform that promotes your research needs, enabling you to execute literature analysis, collaborate with your team, identify research gaps, draft, and explore the journey of research.
Have you used any of the mentioned AI research assistance tools to find reliable scholarly sources and analyze them? Let us know how this article has contributed to your journey in finding the right research source for you. Also, don’t forget to share your preferred AI tool for academic research on Enago Academy’s Open Platform .
Frequently Asked Questions
Scholarly sources are academic sources written by subject-matter experts, and are subject to the process of peer review. This process ensures that the information in such sources is updated, scientifically accurate, and of excellent quality.
The examples of scholarly sources include journal articles, books, conference publications, dissertations, and even websites.
Citing sources is ethically important as it enhances the credibility of your work and prevents plagiarism. It also ensures that the credit is given where it is due.
Scholarly sources are important for academic research as they present accurate and updated information that is free of bias and is objective in nature.
Rate this article Cancel Reply
Your email address will not be published.
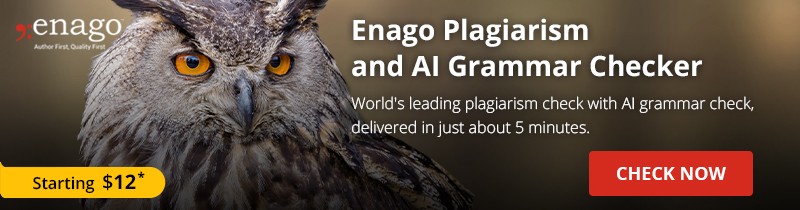
Enago Academy's Most Popular Articles
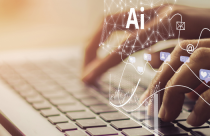
- Old Webinars
- Webinar Mobile App
Improving Research Manuscripts Using AI-Powered Insights: Enago reports for effective research communication
Language Quality Importance in Academia AI in Evaluating Language Quality Enago Language Reports Live Demo…
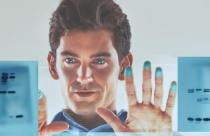
- AI in Academia
AI vs. AI: How to detect image manipulation and avoid academic misconduct
The scientific community is facing a new frontier of controversy as artificial intelligence (AI) is…
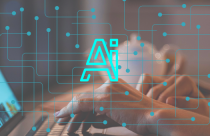
- Industry News
- Publishing News
Unified AI Guidelines Crucial as Academic Writing Embraces Generative Tools
As generative artificial intelligence (AI) tools like ChatGPT are advancing at an accelerating pace, their…
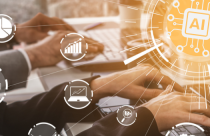
- Trending Now
Using AI for Journal Selection — Simplifying your academic publishing journey in the smart way
Strategic journal selection plays a pivotal role in maximizing the impact of one’s scholarly work.…
Using AI for Plagiarism Prevention and AI-Content Detection in Academic Writing
AI plagiarism prevention strategies Master AI tools for research integrity Ethical implications of AI-generated text…
How to Improve Lab Report Writing: Best practices to follow with and without…
AI for Accuracy in Research and Academic Writing
Upholding Academic Integrity in the Age of AI: Challenges and solutions
Preserving Research Integrity: Why author guidelines on generative AI tools matter

Sign-up to read more
Subscribe for free to get unrestricted access to all our resources on research writing and academic publishing including:
- 2000+ blog articles
- 50+ Webinars
- 10+ Expert podcasts
- 50+ Infographics
- 10+ Checklists
- Research Guides
We hate spam too. We promise to protect your privacy and never spam you.
I am looking for Editing/ Proofreading services for my manuscript Tentative date of next journal submission:
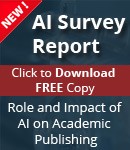
As a researcher, what do you consider most when choosing an image manipulation detector?

We generate robust evidence fast
What is silvi.ai .
Silvi is an end-to-end screening and data extraction tool supporting Systematic Literature Review and Meta-analysis.
Silvi helps create systematic literature reviews and meta-analyses that follow Cochrane guidelines in a highly reduced time frame, giving a fast and easy overview. It supports the user through the full process, from literature search to data analyses. Silvi is directly connected with databases such as PubMed and ClinicalTrials.gov and is always updated with the latest published research. It also supports RIS files, making it possible to upload a search string from your favorite search engine (i.e., Ovid). Silvi has a tagging system that can be tailored to any project.
Silvi is transparent, meaning it documents and stores the choices (and the reasons behind them) the user makes. Whether publishing the results from the project in a journal, sending them to an authority, or collaborating on the project with several colleagues, transparency is optimal to create robust evidence.
Silvi is developed with the user experience in mind. The design is intuitive and easily available to new users. There is no need to become a super-user. However, if any questions should arise anyway, we have a series of super short, instructional videos to get back on track.
To see Silvi in use, watch our short introduction video.
Short introduction video
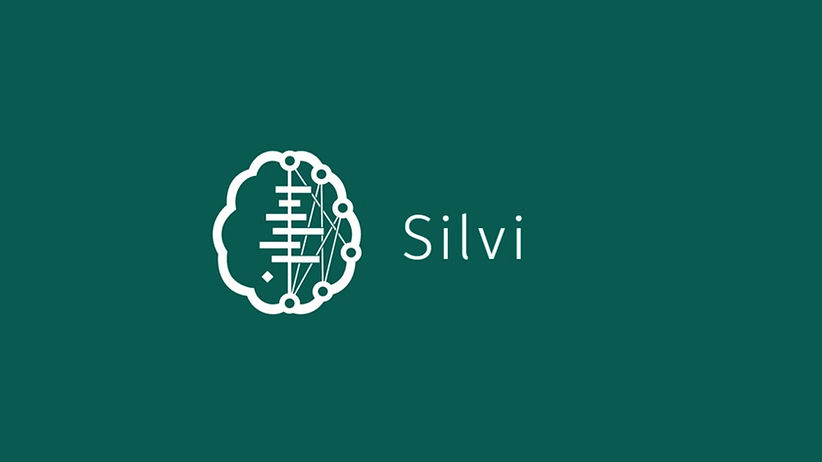
Learn more about Silvi’s specifications here.
"I like that I can highlight key inclusions and exclusions which makes the screening process really quick - I went through 2000+ titles and abstracts in just a few hours"
Eishaan Kamta Bhargava
Consultant Paediatric ENT Surgeon, Sheffield Children's Hospital
"I really like how intuitive it is working with Silvi. I instantly felt like a superuser."
Henriette Kristensen
Senior Director, Ferring Pharmaceuticals
"The idea behind Silvi is great. Normally, I really dislike doing literature reviews, as they take up huge amounts of time. Silvi has made it so much easier! Thanks."
Claus Rehfeld
Senior Consultant, Nordic Healthcare Group
"AI has emerged as an indispensable tool for compiling evidence and conducting meta-analyses. Silvi.ai has proven to be the most comprehensive option I have explored, seamlessly integrating automated processes with the indispensable attributes of clarity and reproducibility essential for rigorous research practices."
Martin Södermark
M.Sc. Specialist in clinical adult psychology
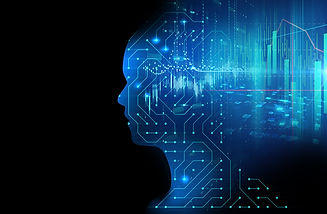
Silvi.ai was founded in 2018 by Professor in Health Economic Evidence, Tove Holm-Larsen, and expert in Machine Learning, Rasmus Hvingelby. The idea for Silvi stemmed from their own research, and the need to conduct systematic literature reviews and meta-analyses faster.
The ideas behind Silvi were originally a component of a larger project. In 2016, Tove founded the group “Evidensbaseret Medicin 2.0” in collaboration with researchers from Ghent University, Technical University of Denmark, University of Copenhagen, and other experts. EBM 2.0 wanted to optimize evidence-based medicine to its highest potential using Big Data and Artificial Intelligence, but needed a highly skilled person within AI.
Around this time, Tove met Rasmus, who shared the same visions. Tove teamed up with Rasmus, and Silvi.ai was created.
Our story
.png)
Free Trial
No card de t ails nee ded!
Your all in one AI-powered Reading Assistant
A Reading Space to Ideate, Create Knowledge, & Collaborate on Your Research
- Smartly organize your research
- Receive recommendations that can not be ignored
- Collaborate with your team to read, discuss, and share knowledge

From Surface-Level Exploration to Critical Reading - All at One Place!
Fine-tune your literature search.
Our AI-powered reading assistant saves time spent on the exploration of relevant resources and allows you to focus more on reading.
Select phrases or specific sections and explore more research papers related to the core aspects of your selections. Pin the useful ones for future references.
Our platform brings you the latest research news, online courses, and articles from magazines/blogs related to your research interests and project work.
Speed up your literature review
Quickly generate a summary of key sections of any paper with our summarizer.
Make informed decisions about which papers are relevant, and where to invest your time in further reading.
Get key insights from the paper, quickly comprehend the paper’s unique approach, and recall the key points.
Bring order to your research projects
Organize your reading lists into different projects and maintain the context of your research.
Quickly sort items into collections and tag or filter them according to keywords and color codes.
Experience the power of sharing by finding all the shared literature at one place
Decode papers effortlessly for faster comprehension
Highlight what is important so that you can retrieve it faster next time
Find Wikipedia explanations for any selected word or phrase
Save time in finding similar ideas across your projects
Collaborate to read with your team, professors, or students
Share and discuss literature and drafts with your study group, colleagues, experts, and advisors. Recommend valuable resources and help each other for better understanding.
Work in shared projects efficiently and improve visibility within your study group or lab members.
Keep track of your team's progress by being constantly connected and engaging in active knowledge transfer by requesting full access to relevant papers and drafts.
Find Papers From Across the World's Largest Repositories

Testimonials
Privacy and security of your research data are integral to our mission..

Everything you add or create on Enago Read is private by default. It is visible only if and when you share it with other users.

You can put Creative Commons license on original drafts to protect your IP. For shared files, Enago Read always maintains a copy in case of deletion by collaborators or revoked access.

We use state-of-the-art security protocols and algorithms including MD5 Encryption, SSL, and HTTPS to secure your data.
AI for literature reviews
Let ai assist boost your literature review and analysis, how to use ai assist for your literature review.
- Step one: Identify and import your literature
- Step two: Summarize your documents with AI Assist
- Step three: Determine relevance and sort accordingly
- Step four: Reading and rough coding
- Step five: Confirm your initial codings
- Step six: Refine your code system
- Step seven: Analyze your literature
Literature about literature reviews and analysis
Tuesday, September 19, 2023
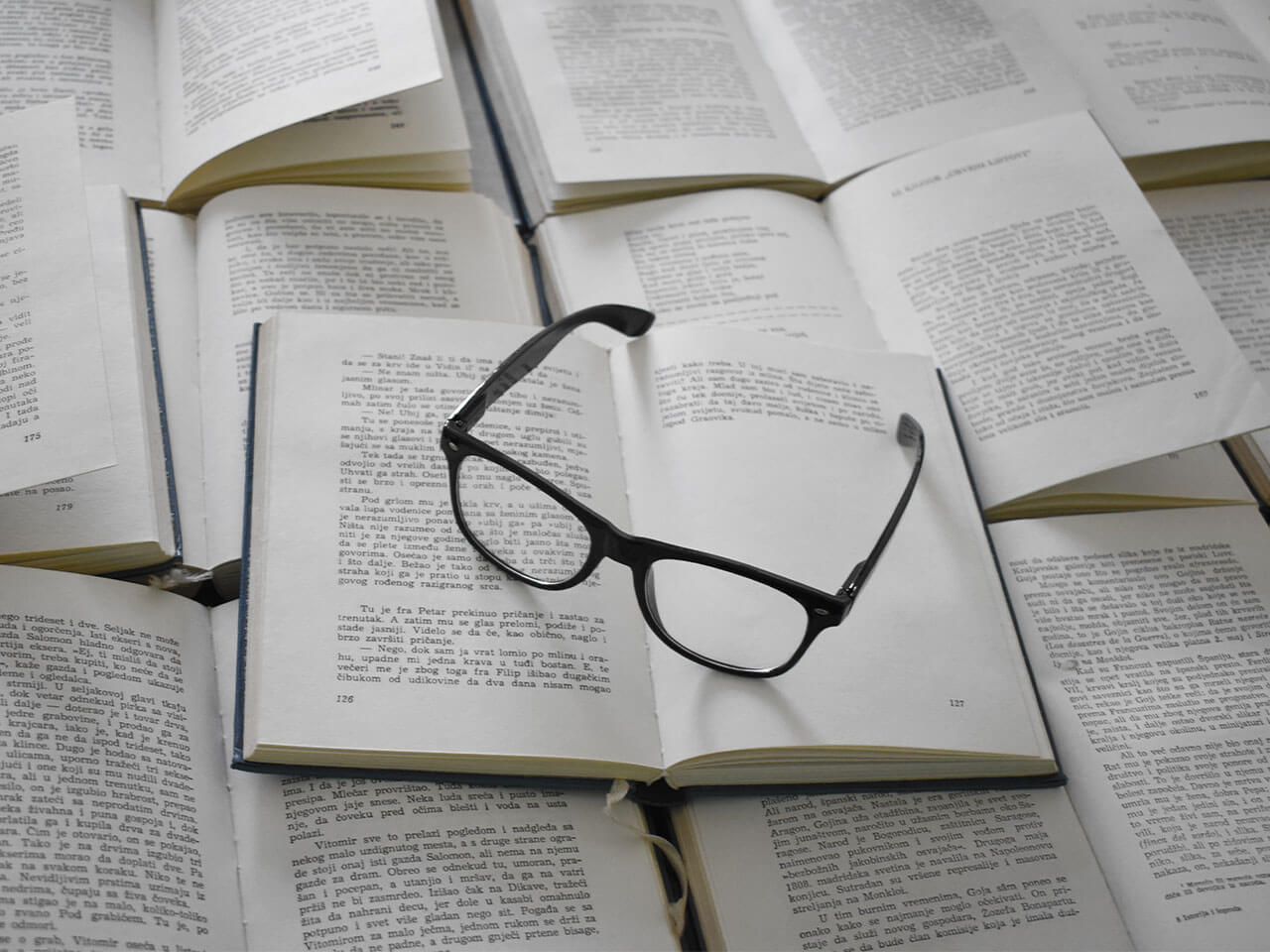
As you may have noticed, there is a rapid growth in AI-based tools for all types of software packages. We followed this trend by releasing AI Assist – your virtual research assistant that simplifies your qualitative data analysis. In the following, we will present you the tools and functions of AI Assist and how they can facilitate your literature reviews.
Literature reviews are an important step in the data analysis journey of many research projects, but often it is a time-consuming and arduous affair. Whether you are reviewing literature for writing a meta-analysis or for the background section of your thesis, work with MAXQDA! Besides the classic tools of MAXQDA that can facilitate each phase of your literature review, the new tool AI Assist can boost your literature review and analysis in multiple ways.
Year by year, the number of publications grows in almost every field of research – our insights and knowledge likewise. The drawback is that the number of publications might be too high to keep track of the recent developments in your field of research. Consequently, conducting a proper literature review becomes more and more difficult, and the importance of quickly identifying whether a publication is interesting for your research question constantly increases.
Luckily, MAXQDA’s AI Assist tool is here to help. Among others, it can summarize your documents, text segments, and coded segments. But there is more – based on your coded segments AI Assist can generate subcodes suggestions. In the following, we will present you step-by-step instructions on how to use MAXQDA for your literature review and analysis with a special focus on how AI Assist can support you.
Step one of AI for literature reviews: Identify and import your literature
Despite the fact that MAXQDA and AI Assist can facilitate your literature review and analysis in manifold ways, the best advice is to carefully plan your literature review and analysis. Think about the purpose of your literature review and the questions you want to answer. Develop a search strategy which includes, but is not limited to, deciding on literature databases, search terms, and practical and methodological criteria for selecting high-quality scientific literature. Then start your literature review and analysis by searching the identified databases. Before downloading the PDFs and/or bibliographic information (RIS), briefly scan the search results for relevance by reading the title, keywords and abstract. If you find the publication interesting, download the PDF, and let AI Assist help you determining whether the publication falls within the narrower area of your research question.
MAXQDA’s import tab offers import options dedicated to different data types, such as bibliographic data (in RIS file format) and PDF documents. To import the selected literature, just click on the corresponding button, select the data you want to import, and click okay. Alternatively, you can import data simply by drag-and-dropping the data files from your Windows Explorer/Mac Finder window. If you import full texts and the corresponding bibliographic data, MAXQDA automatically connects the full text to the literature entry with an internal link.
Step two of AI for literature reviews: Summarize your documents with AI Assist
Now that you have imported all publications that might be interesting for your research question, it is time to explore whether they are indeed relevant for your literature review and analysis. Before the release of AI Assist, this step typically took a lot of time as you had to go through each paper individually. With the release of AI Assist, MAXQDA can accelerate this step with AI-generated summaries of your publications. For example, you can create AI-generated summaries either for the entire publication or for each chapter (e.g. Introduction, Methods, Results, and so on) individually and base your decision about a paper’s relevance on these summaries. Each AI-generated summary is stored in a memo that is attached to the underlying document or text segment, respectively.
Summarizing text segments with AI Assist just takes a few clicks. Simply highlight a text segment in the Document Browser and choose AI Assist from the context menu. Adjust the settings to your needs and let OpenAI do the work for you. To view and edit the summary, double-click on the yellow memo icon attached to the summarized text passage.
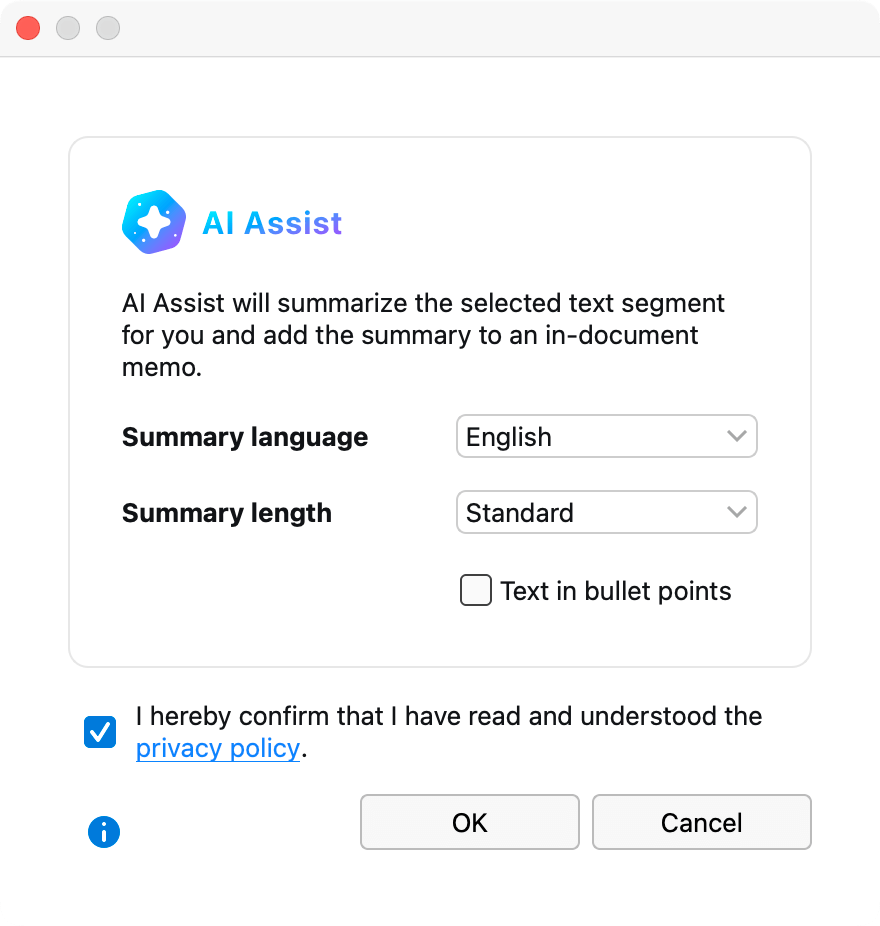
Adjust settings for summarizing text with AI Assist for literature reviews
Step three of AI for literature reviews: Determine relevance and sort accordingly
Instead of reading the entire paper, you can use the AI-generated summaries to determine whether a publication falls within the narrower area of your research question. To do so, it might be helpful to view all memos containing summaries of a specific publication at once. Of course, this is possible with MAXQDA. Go to the Memo tab, click on (In-)document Memos, and click on the publication’s name to view only the AI-generated summaries related to this document. It is important to note that AI-generated summaries are not perfect yet. Therefore, it is advisable to read the entire paper in cases where you have doubts or can’t decide whether the publication is relevant.
Depending on the number of publications in your MAXQDA project, you might want to sort your documents in document groups, for example, based on the relevance for your research question or the topics discussed in the paper. You can easily create a new Document group by clicking on the respective icon in the Document System window. Documents can be added simply via drag-and-drop. Alternatively, you can create Document Sets which are especially helpful when you want to sort your documents by more than one domain (e.g. by relevance and methodology used).
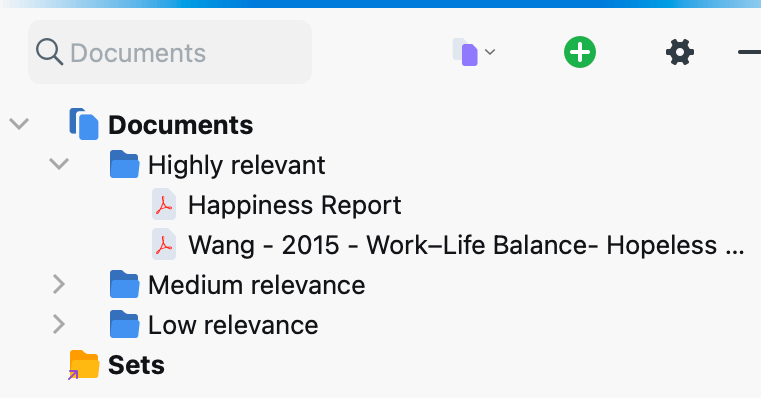
Sort documents in document groups according to their relevance using AI for literature reviews
Step four of AI for literature reviews: Reading and rough coding
Now that you have identified the publications important to your project, it is time to go through the documents. Although, AI Assist can support you at multiple stages of your literature review, it can’t replace the researcher. As a researcher, you still need a deep understanding of your material, analysis methods, and the software you use for analysis. As AI-generated summaries are not perfect yet, you might want to improve the summaries, if necessary, or add information that you consider especially important, e.g. participants’ demographics.
In a next step, it is time to create and apply some codes to the data. A code can be described as a label used to name phenomena in a text or an image. Depending on your approach, you might already have codes in mind (deductive coding) or you plan to generate codes on the basis of the data (inductive coding). No matter your approach – you can use MAXQDA’s advanced tools for coding. In many cases it is best, to start your first round of coding with rather rough codes that you can refine in a later step using the help of AI Assist. You can create codes in the Code System window by clicking on the plus-icon or in the Document Browser by highlighting a text segment via the context menu or the corresponding icons. A code can be applied to the data via drag-and-drop.
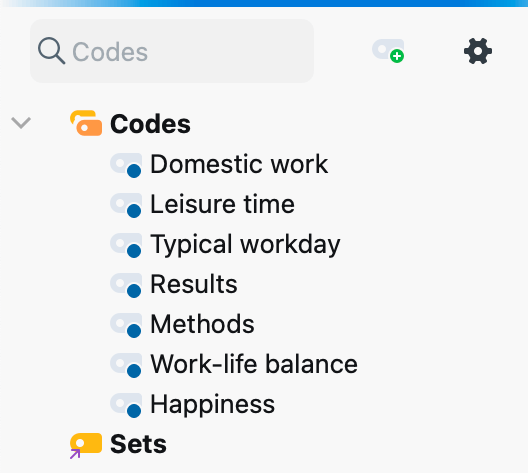
Reading and rough coding for AI for literature reviews
Step five of AI for literature reviews: Confirm your initial codings
Though AI Assist can’t validate your codings like a second researcher using intercoder agreement, AI Assist’s Code Summaries can help you to identify whether you have applied the code as intended. The AI-generated Code Summary is a summary of the content of all text segments coded with the corresponing code. This summary might give you an idea of how you have applied the code and if the coded text segments indeed contain what you had in mind when creating the code.
To create a summary of coded segments with AI Assist, simply right-click the code of interest in the Code System and choose AI Assist > Code Summary from the context menu. Adjust language and the summary length to your needs and let AI Assist do the summary for you. As for document summaries, the summary will be stored in a memo which is placed next to the code in the Code System. If the summary doesn’t match your code definition, you might want to review the coded segments and adjust your codings accordingly. By double-clicking on a code, you open the Overview of Coded Segments – a table perfectly suited to go through the coded segments and adjust or remove the codings.
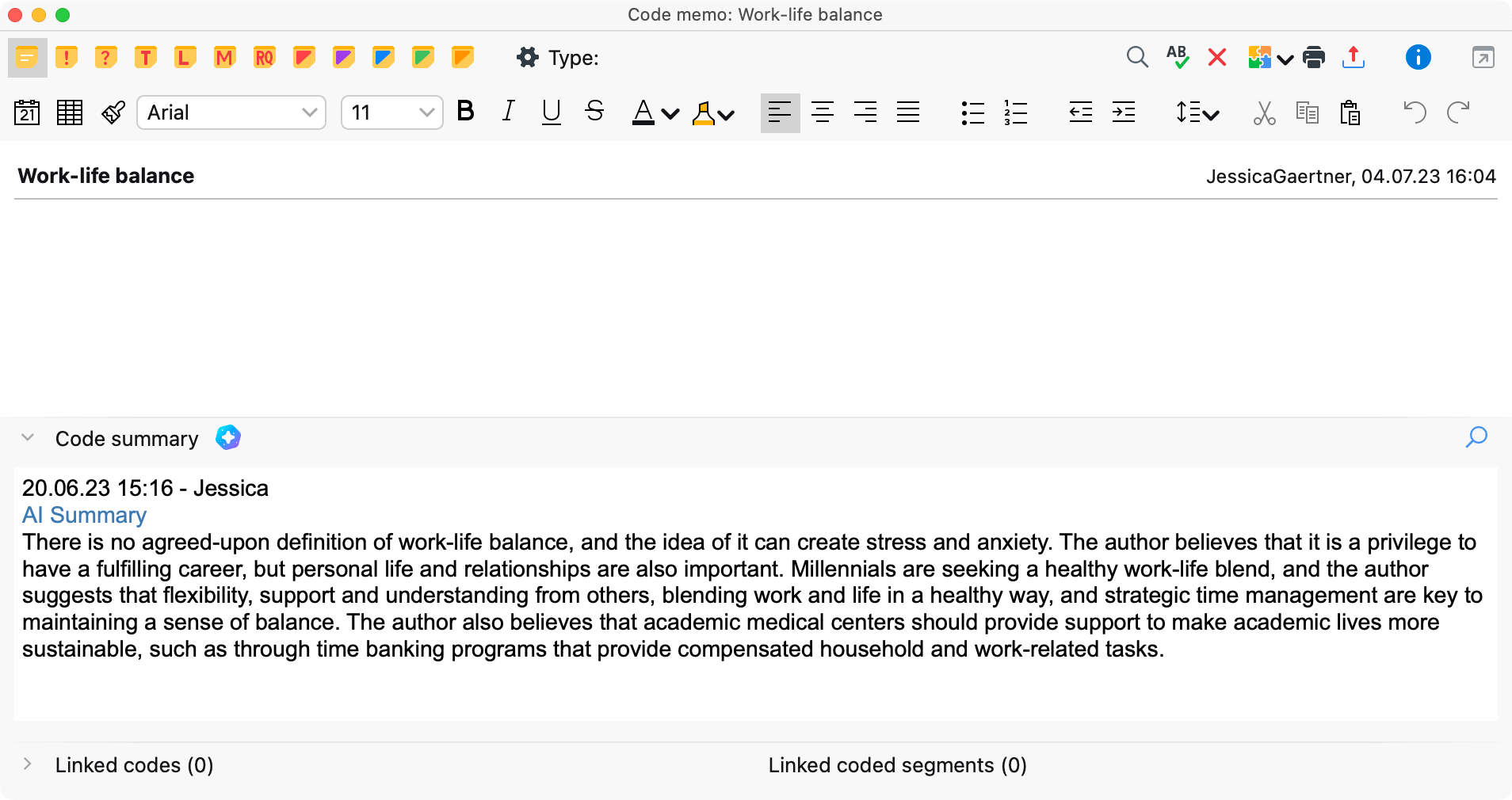
Confirm your initial codings with AI Assist’s Code Summary for literature reviews
Step six of AI for literature reviews: Refine your code system
In case you have applied rather rough codes to your data, your code definitions are probably too broad for you to make sense of the data. Depending on your goals, you might wish to refine these rather broad codes into more precise sub-codes. Again, you can use AI Assist’s power to support this step of your literature review. AI Assist analyzes the text and suggests subcodes while leaving the decision on whether you want to create the suggested sub-codes up to you.
To create AI-generated subcode suggestions, open the context menu of a code and choose AI Assist > Suggest Subcodes. Besides selecting a language, you can ask AI Assist to include examples for each subcode as a bullet list. Like the AI-generated summaries, the code suggestions are stored in the code’s memo. If you are satisfied with the code suggestions, you can create and apply them to your data. Alternatively, you can use the AI-generated code suggestions to confirm the subcodes that you have created.
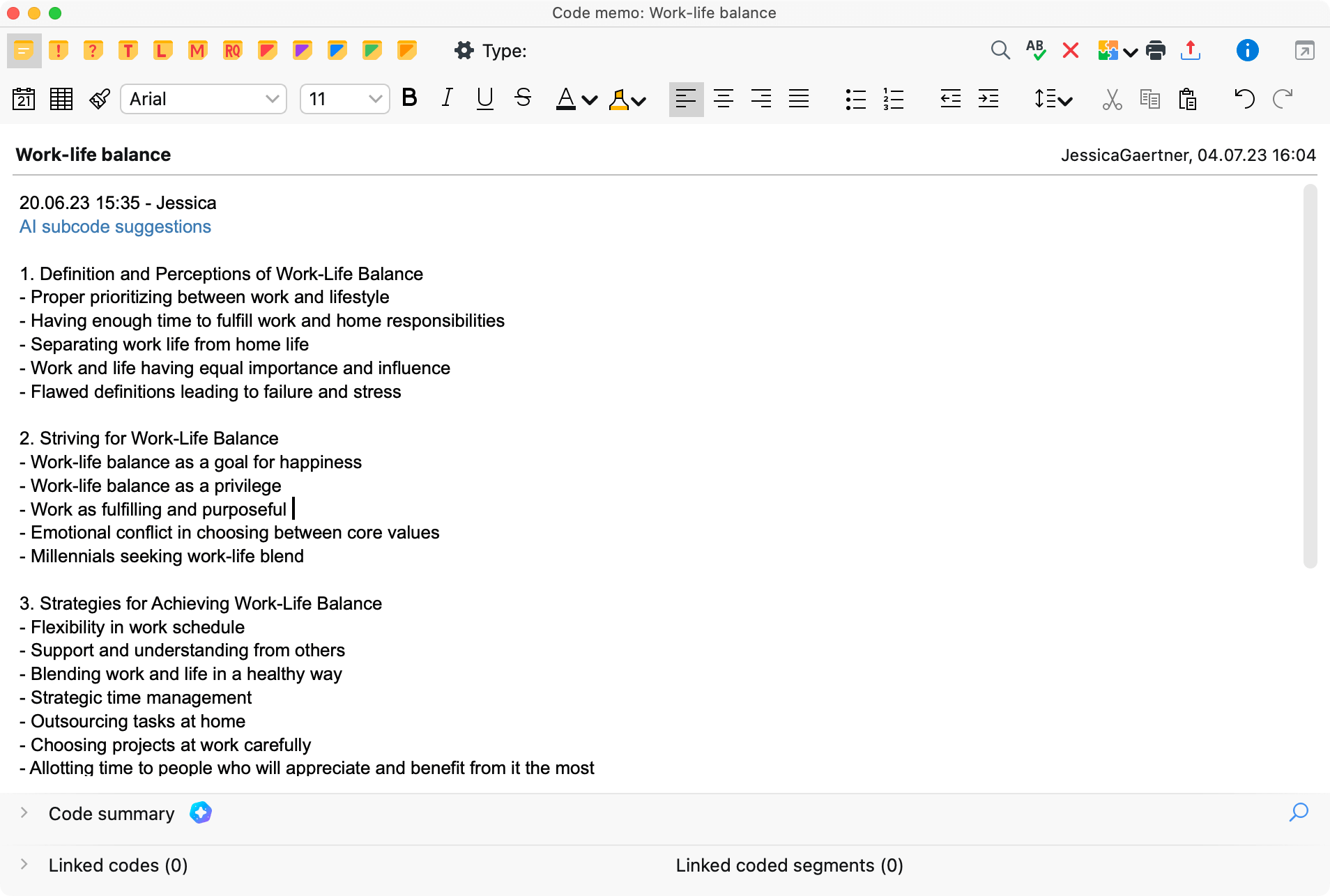
Use AI Assist’s Suggest Subcodes function to refine your code system for your literature reviews
Step seven of AI for literature reviews: Analyze your literature
Now that you have coded your literature, it’s time to analyze the material with MAXQDA. Although you can use plenty of MAXQDA’s tools and functions even when the material is not coded, other tools require coded segments to be applicable. MAXQDA offers plenty of tools for qualitative data analysis, impossible to mention all. Among others, MAXQDA’s Overview and Summary Tables are useful for aggregating your data. With MAXQDA Visualization Tools you can quickly and easily create stunning visualizations of your data, and with MAXQDA’s Questions-Themes-Theories tool you have a place to synthesize your results and write up a literature review or report.
You can find more information and ideas for conducting a literature review with MAXQDA, here:
Learn more about literature reviews
For information about AI Assist and how to Activate AI Assist, visit:
Learn more about AI Assist
We offer a variety of free learning materials to help you get started with your literature review. Check out our Getting Started Guide to get a quick overview of MAXQDA and step-by-step instructions on setting up your software and creating your first project with your brand new QDA software. In addition, the free Literature Reviews Guide explains how to conduct a literature review with MAXQDA in more detail.
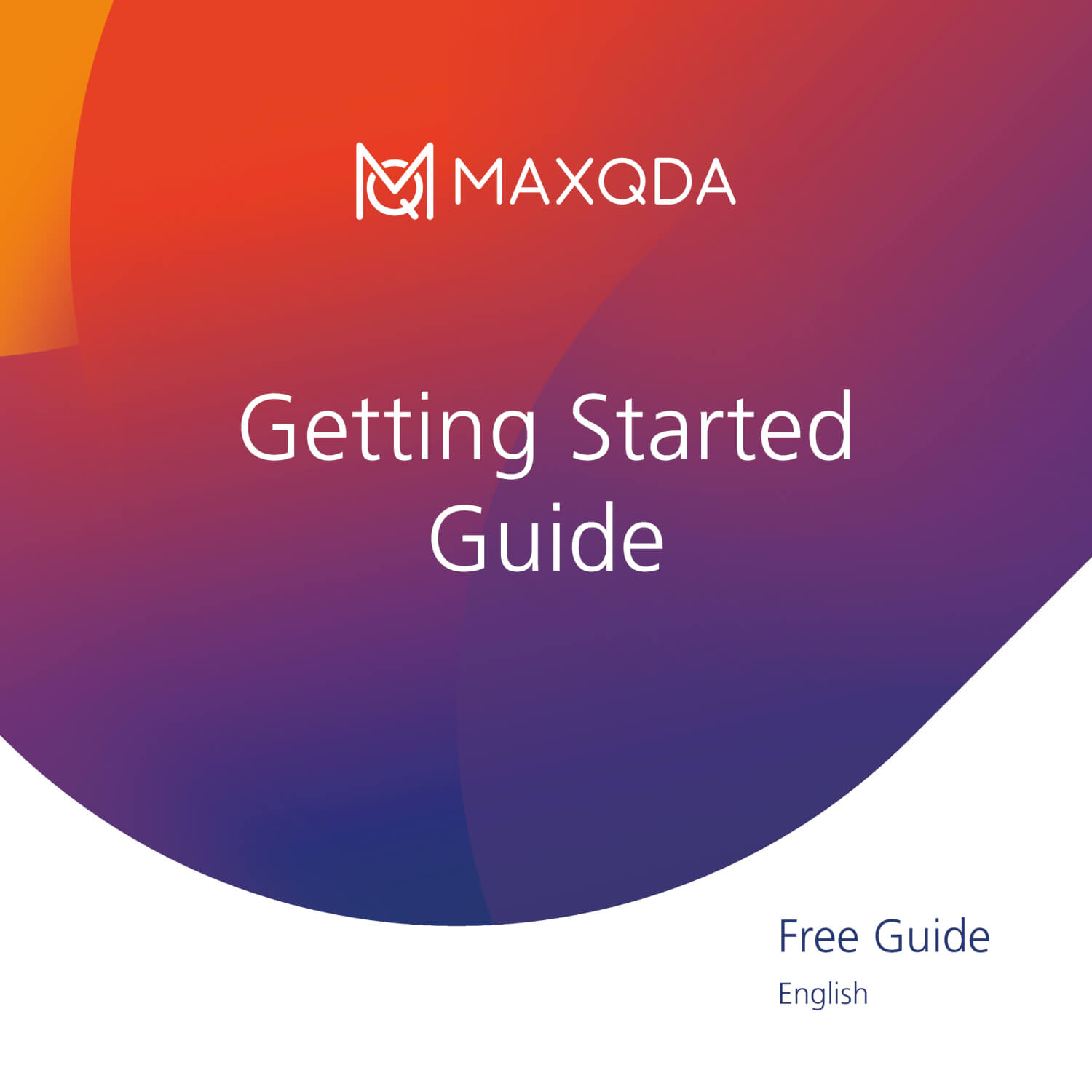
Getting Started with MAXQDA
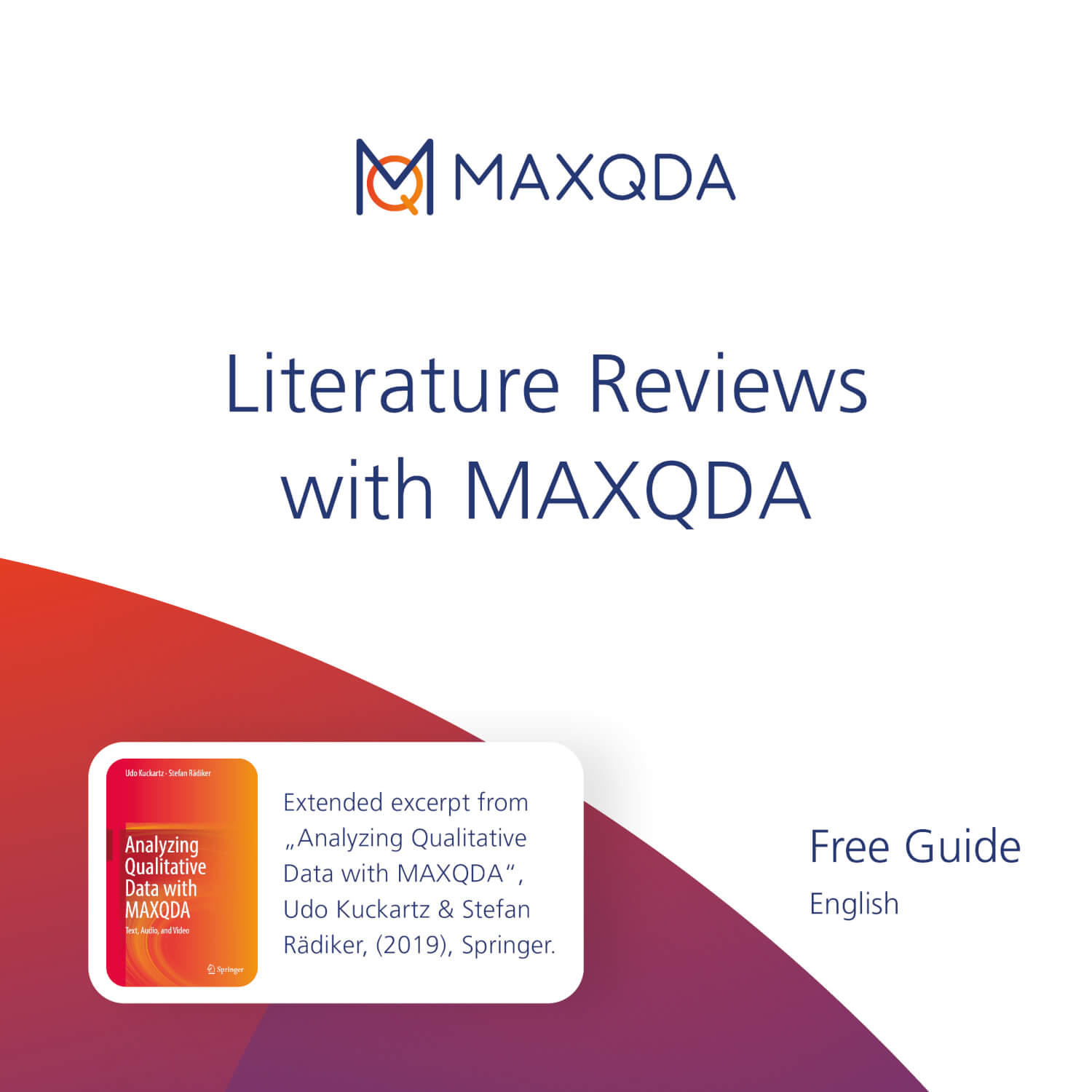
Literature Reviews with MAXQDA
MAXQDA Newsletter
Our research and analysis tips, straight to your inbox.
- By submitting the form I accept the Privacy Policy.

Something went wrong when searching for seed articles. Please try again soon.
No articles were found for that search term.
Author, year The title of the article goes here
LITERATURE REVIEW SOFTWARE FOR BETTER RESEARCH

“Litmaps is a game changer for finding novel literature... it has been invaluable for my productivity.... I also got my PhD student to use it and they also found it invaluable, finding several gaps they missed”
Varun Venkatesh
Austin Health, Australia
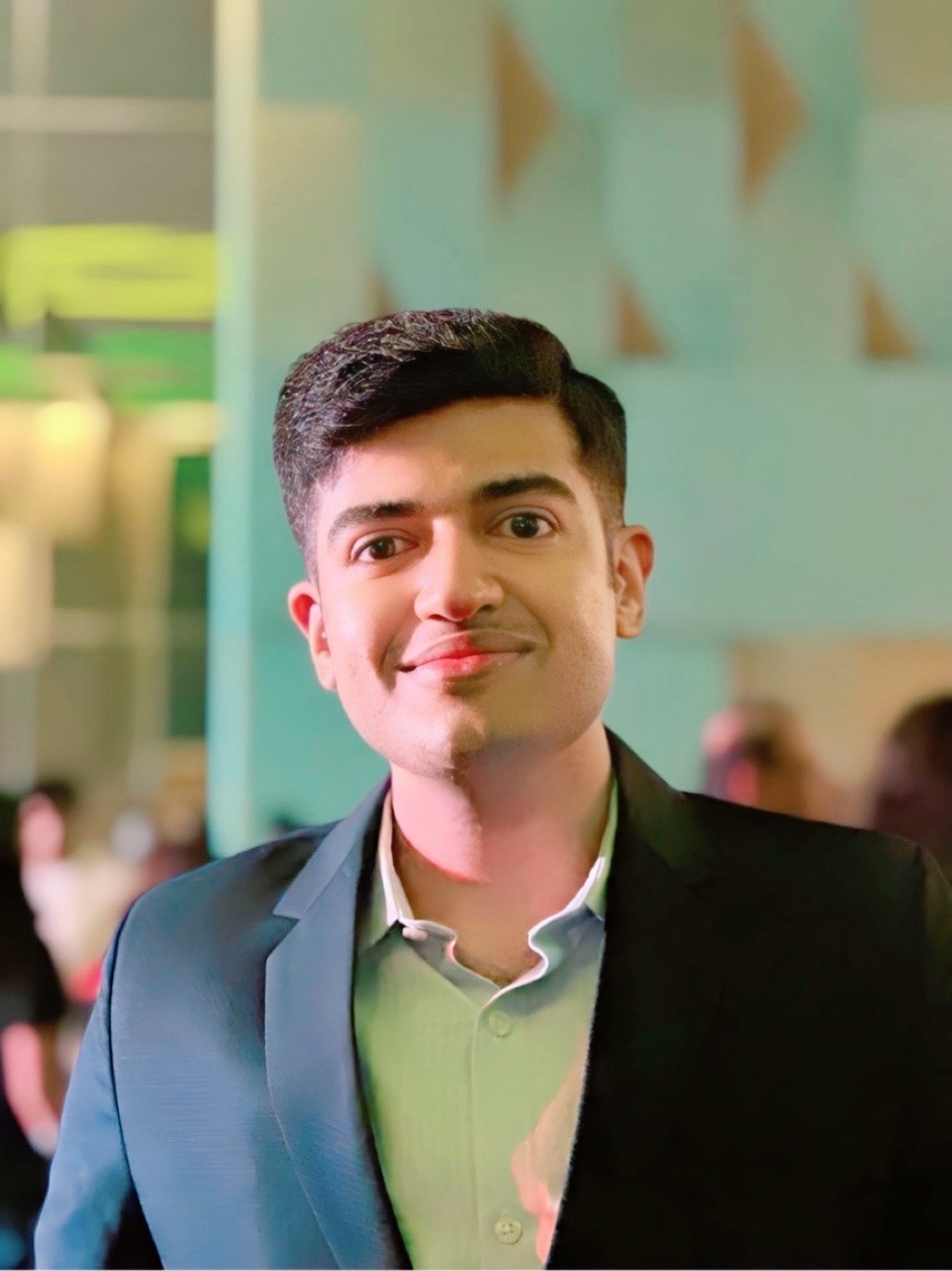
As a full-time researcher, Litmaps has become an indispensable tool in my arsenal. The Seed Maps and Discover features of Litmaps have transformed my literature review process, streamlining the identification of key citations while revealing previously overlooked relevant literature, ensuring no crucial connection goes unnoticed. A true game-changer indeed!
Ritwik Pandey
Doctoral Research Scholar – Sri Sathya Sai Institute of Higher Learning
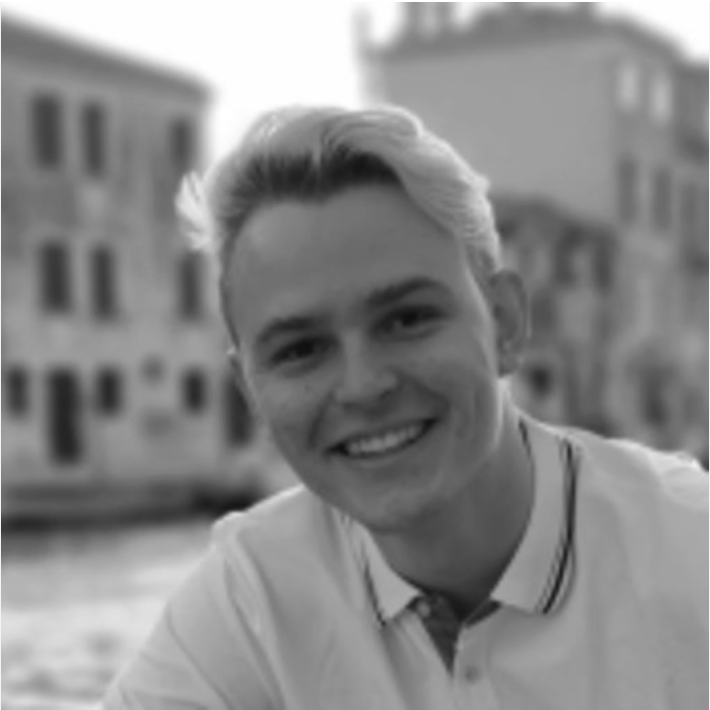
Using Litmaps for my research papers has significantly improved my workflow. Typically, I start with a single paper related to my topic. Whenever I find an interesting work, I add it to my search. From there, I can quickly cover my entire Related Work section.
David Fischer
Research Associate – University of Applied Sciences Kempten
“It's nice to get a quick overview of related literature. Really easy to use, and it helps getting on top of the often complicated structures of referencing”
Christoph Ludwig
Technische Universität Dresden, Germany
“This has helped me so much in researching the literature. Currently, I am beginning to investigate new fields and this has helped me hugely”
Aran Warren
Canterbury University, NZ
“I can’t live without you anymore! I also recommend you to my students.”
Professor at The Chinese University of Hong Kong
“Seeing my literature list as a network enhances my thinking process!”
Katholieke Universiteit Leuven, Belgium
“Incredibly useful tool to get to know more literature, and to gain insight in existing research”
KU Leuven, Belgium
“As a student just venturing into the world of lit reviews, this is a tool that is outstanding and helping me find deeper results for my work.”
Franklin Jeffers
South Oregon University, USA
“Any researcher could use it! The paper recommendations are great for anyone and everyone”
Swansea University, Wales
“This tool really helped me to create good bibtex references for my research papers”
Ali Mohammed-Djafari
Director of Research at LSS-CNRS, France
“Litmaps is extremely helpful with my research. It helps me organize each one of my projects and see how they relate to each other, as well as to keep up to date on publications done in my field”
Daniel Fuller
Clarkson University, USA
As a person who is an early researcher and identifies as dyslexic, I can say that having research articles laid out in the date vs cite graph format is much more approachable than looking at a standard database interface. I feel that the maps Litmaps offers lower the barrier of entry for researchers by giving them the connections between articles spaced out visually. This helps me orientate where a paper is in the history of a field. Thus, new researchers can look at one of Litmap's "seed maps" and have the same information as hours of digging through a database.
Baylor Fain
Postdoctoral Associate – University of Florida
Our Course: Learn and Teach with Litmaps
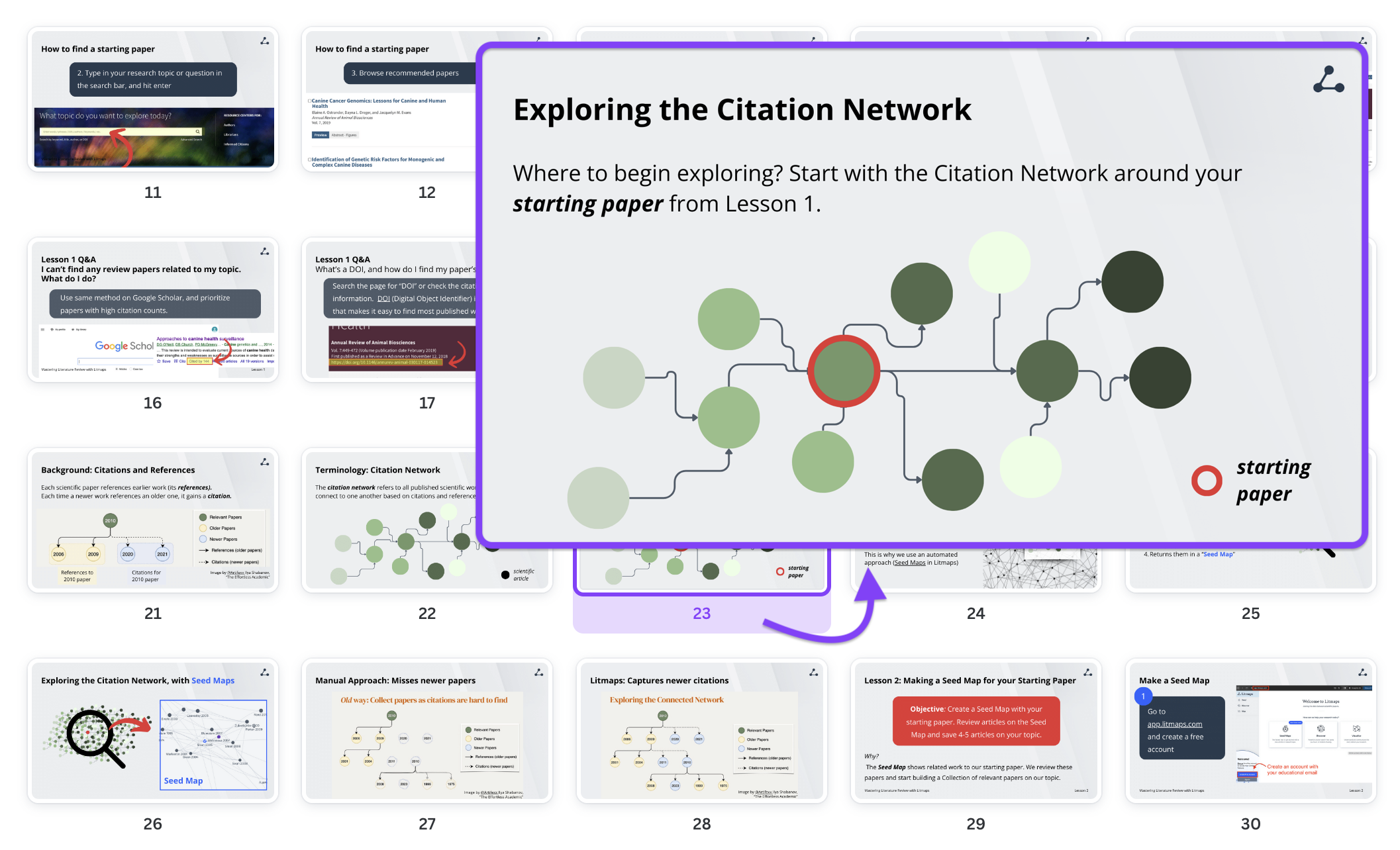
- Reference Manager
- Simple TEXT file
People also looked at
Original research article, a systematic literature review on the impact of ai models on the security of code generation.
- 1 Security and Trust, University of Luxembourg, Luxembourg, Luxembourg
- 2 École Normale Supérieure, Paris, France
- 3 Faculty of Humanities, Education, and Social Sciences, University of Luxembourg, Luxembourg, Luxembourg
Introduction: Artificial Intelligence (AI) is increasingly used as a helper to develop computing programs. While it can boost software development and improve coding proficiency, this practice offers no guarantee of security. On the contrary, recent research shows that some AI models produce software with vulnerabilities. This situation leads to the question: How serious and widespread are the security flaws in code generated using AI models?
Methods: Through a systematic literature review, this work reviews the state of the art on how AI models impact software security. It systematizes the knowledge about the risks of using AI in coding security-critical software.
Results: It reviews what security flaws of well-known vulnerabilities (e.g., the MITRE CWE Top 25 Most Dangerous Software Weaknesses) are commonly hidden in AI-generated code. It also reviews works that discuss how vulnerabilities in AI-generated code can be exploited to compromise security and lists the attempts to improve the security of such AI-generated code.
Discussion: Overall, this work provides a comprehensive and systematic overview of the impact of AI in secure coding. This topic has sparked interest and concern within the software security engineering community. It highlights the importance of setting up security measures and processes, such as code verification, and that such practices could be customized for AI-aided code production.
1 Introduction
Despite initial concerns, increasingly, many organizations rely on artificial intelligence (AI) to enhance the operational workflows in their software development life cycle and to support writing software artifacts. One of the most well-known tools is GitHub Copilot. It is created by Microsoft relies on OpenAI's Codex model, and is trained on open-source code publicly available on GitHub ( Chen et al., 2021 ). Like many similar tools—such as CodeParrot, PolyCoder, StarCoder—Copilot is built atop a large language model (LLM) that has been trained on programming languages. Using LLMs for such tasks is an idea that dates back at least as far back as the public release of OpenAI's ChatGPT.
However, using automation and AI in software development is a double-edged sword. While it can improve code proficiency, the quality of AI-generated code is problematic. Some models introduce well-known vulnerabilities, such as those documented in MITRE's Common Weakness Enumeration (CWE) list of the top 25 “most dangerous software weaknesses.” Others generate so-called “stupid bugs,” naïve single-line mistakes that developers would qualify as “stupid” upon review ( Karampatsis and Sutton, 2020 ).
This behavior was identified early on and is supported to a varying degree by academic research. Pearce et al. (2022) concluded that 40% of the code suggested by Copilot had vulnerabilities. Yet research also shows that users trust AI-generator code more than their own ( Perry et al., 2023 ). These situations imply that new processes, mitigation strategies, and methodologies should be implemented to reduce or control the risks associated with the participation of generative AI in the software development life cycle.
It is, however, difficult to clearly attribute the blame, as the tooling landscape evolves, different training strategies and prompt engineering are used to alter LLMs behavior, and there is conflicting if anecdotal, evidence that human-generated code could be just as bad as AI-generated code.
This systematic literature review (SLR) aims to critically examine how the code generated by AI models impacts software and system security. Following the categorization of the research questions provided by Kitchenham and Charters (2007) on SLR questions, this work has a 2-fold objective: analyzing the impact and systematizing the knowledge produced so far. Our main question is:
“ How does the code generation from AI models impact the cybersecurity of the software process? ”
This paper discusses the risks and reviews the current state-of-the-art research on this still actively-researched question.
Our analysis shows specific trends and gaps in the literature. Overall, there is a high-level agreement that AI models do not produce safe code and do introduce vulnerabilities , despite mitigations. Particular vulnerabilities appear more frequently and prove to be more problematic than others ( Pearce et al., 2022 ; He and Vechev, 2023 ). Some domains (e.g., hardware design) seem more at risk than others, and there is clearly an imbalance in the efforts deployed to address these risks.
This work stresses the importance of relying on dedicated security measures in current software production processes to mitigate the risks introduced by AI-generated code and highlights the limitations of AI-based tools to perform this mitigation themselves.
The article is divided as follows: we first introduce the reader to AI models and code generation in Section 2 to proceed to explain our research method in Section 3. We then present our results in Section 4. In Section 5 we discuss the results, taking in consideration AI models, exploits, programming languages, mitigation strategies and future research. We close the paper by addressing threats to validity in Section 6 and conclusion in Section 7.
2 Background and previous work
2.1 ai models.
The sub-branch of AI models that is relevant to our discussion are generative models, especially large-language models (LLMs) that developed out of the attention-based transformer architecture ( Vaswani et al., 2017 ), made widely known and available through pre-trained models (such as OpenAI's GPT series and Codex, Google's PaLM, Meta's LLaMA, or Mistral's Mixtral).
In a transformer architecture, inputs (e.g., text) are converted to tokens 1 which are then mapped to an abstract latent space, a process known as encoding ( Vaswani et al., 2017 ). Mapping back from the latent space to tokens is accordingly called decoding , and the model's parameters are adjusted so that encoding and decoding work properly. This is achieved by feeding the model with human-generated input, from which it can learn latent space representations that match the input's distribution and identify correlations between tokens.
Pre-training amortizes the cost of training, which has become prohibitive for LLMs. It consists in determining a reasonable set of weights for the model, usually through autocompletion tasks, either autoregressive (ChatGPT) or masked (BERT) for natural language, during which the model is faced with an incomplete input and must correctly predict the missing parts or the next token. This training happens once, is based on public corpora, and results in an initial set of weights that serves as a baseline ( Tan et al., 2018 ). Most “open-source” models today follow this approach. 2
It is possible to fine-tune parameters to handle specific tasks from a pre-trained model, assuming they remain within a small perimeter of what the model was trained to do. This final training often requires human feedback and correction ( Tan et al., 2018 ).
The output of a decoder is not directly tokens, however, but a probability distribution over tokens. The temperature hyperparameter of LLMs controls how much the likelihood of less probable tokens is amplified: a high temperature would allow less probable tokens to be selected more often, resulting in a less predictable output. This is often combined with nucleus sampling ( Holtzman et al., 2020 ), i.e., requiring that the total sum of token probabilities is large enough and various penalty mechanisms to avoid repetition.
Finally, before being presented to the user, an output may undergo one or several rounds of (possibly non-LLM) filtering, including for instance the detection of foul language.
2.2 Code generation with AI models
With the rise of generative AI, there has also been a rise in the development of AI models for code generation. Multiple examples exist, such as Codex, Polycoder, CodeGen, CodeBERT, and StarCoder, to name a few (337, Xu, Li). These new tools should help developers of different domains be more efficient when writing code—or at least expected to ( Chen et al., 2021 ).
The use of LLMs for code generation is a domain-specific application of generative methods that greatly benefit from the narrower context. Contrary to natural language, programming languages follow a well-defined syntax using a reduced set of keywords, and multiple clues can be gathered (e.g., filenames, other parts of a code base) to help nudging the LLM in the right direction. Furthermore, so-called boilerplate code is not project-specific and can be readily reused across different code bases with minor adaptations, meaning that LLM-powered code assistants can already go a long way simply by providing commonly-used code snippets at the right time.
By design, LLMs generate code based on their training set ( Chen et al., 2021 ). 3 In doing so, there is a risk that sensitive, incorrect, or dangerous code is uncritically copied verbatim from the training set or that the “minor adaptations” necessary to transfer code from one project to another introduces mistakes ( Chen et al., 2021 ; Pearce et al., 2022 ; Niu et al., 2023 ). Therefore, generated code may include security issues, such as well-documented bugs, malpractices, or legacy issues found in the training data. A parallel issue often brought up is the copyright status of works produced by such tools, a still-open problem that is not the topic of this paper.
Similarly, other challenges and concerns have been highlighted by different academic research. From an educational point of view, some concerns are that using AI code generation models may impact acquiring bad security habits between novice programmers or students ( Becker et al., 2023 ). However, the usage of such models can also help lower the entry barrier to the field ( Becker et al., 2023 ). Similarly, cite337 has suggested that using AI code generation models does not output secure code all the time, as they are non-deterministic, and future research on mitigation is required ( Pearce et al., 2022 ). For example, Pearce et al. (2022) was one of the first to research this subject.
There are further claims that it may be possible to use by cyber criminal ( Chen et al., 2021 ; Natella et al., 2024 ). In popular communication mediums, there are affirmations that ChatGPT and other LLMs will be “useful” for criminal activities, for example Burgess (2023) . However, these tools can be used defensively in cyber security, as in ethical hacking ( Chen et al., 2021 ; Natella et al., 2024 ).
3 Research method
This research aims to systematically gather and analyze publications that answer our main question: “ How does the code generation of AI models impact the cybersecurity of the software process? ” Following Kitchenham and Charters (2007) classification of questions for SLR, our research falls into the type of questions of “Identifying the impact of technologies” on security, and “Identifying cost and risk factors associated with a technology” in security too.
To carry out this research, we have followed different SLR guidelines, most notably Wieringa et al. (2006) , Kitchenham and Charters (2007) , Wohlin (2014) , and Petersen et al. (2015) . Each of these guidelines was used for different elements of the research. We list out in a high-level approach which guidelines were used for each element, which we further discuss in different subsections of this article.
• For the general structure and guideline on how to carry out the SLR, we used Kitchenham and Charters (2007) . This included exclusion and inclusion criteria, explained in Section 3.2 ;
• The identification of the Population, Intervention, Comparison, and Outcome (PICO) is based both in Kitchenham and Charters (2007) and Petersen et al. (2015) , as a framework to create our search string. We present and discuss this framework in Section 3.1 ;
• The questions and quality check of the sample, we used the research done by Kitchenham et al. (2010) , which we describe in further details at Section 3.4 ;
• The taxonomy of type of research is from Wieringa et al. (2006) as a strategy to identify if a paper falls under our exclusion criteria. We present and discuss this taxonomy in Section 3.2. Although their taxonomy focuses on requirements engineering, it is broad enough to be used in other areas as recognized by Wohlin et al. (2013) ;
• For the snowballing technique, we used the method presented in Wohlin (2014) , which we discuss in Section 3.3 ;
• Mitigation strategies from Wohlin et al. (2013) are used, aiming to increase the reliability and validity of this study. We further analyze the threats to validity of our research in Section 6.
In the following subsections, we explain our approach to the SLR in more detail. The results are presented in Section 4.
3.1 Search planning and string
To answer our question systematically, we need to create a search string that reflects the critical elements of our questions. To achieve this, we thus need to frame the question in a way that allows us to (1) identify keywords, (2) identify synonyms, (3) define exclusion and inclusion criteria, and (4) answer the research question. One common strategy is the PICO (population, intervention, comparison, outcome) approach ( Petersen et al., 2015 ). Originally from medical sciences, it has been adapted for computer science and software engineering ( Kitchenham and Charters, 2007 ; Petersen et al., 2015 ).
To frame our work with the PICO approach, we follow the methodologies outlined in Kitchenham and Charters (2007) and Petersen et al. (2015) . We can identify the set of keywords and their synonyms by identifying these four elements, which are explained in detail in the following bullet point.
• Population: Cybersecurity.
• Following Kitchenham and Charters (2007) , a population can be an area or domain of technology. Population can be very specific.
• Intervention: AI models.
• Following Kitchenham and Charters (2007) “The intervention is the software methodology/tool/technology, such as the requirement elicitation technique.”
• Comparison: we compare the security issues identified by the code generated in the research articles. In Kitchenham and Charters (2007) word, “This is the software engineering methodology/tool/technology/procedure with which the intervention is being compared. When the comparison technology is the conventional or commonly-used technology, it is often referred to as the ‘control' treatment.”
• Outcomes: A systematic list of security issues of using AI models for code generation and possible mitigation strategies.
• Context: Although not mandatory (per Kitchenham and Charters, 2007 ) in general we consider code generation.
With the PICO elements done, it is possible to determine specific keywords to generate our search string. We have identified three specific sets: security, AI, and code generation. Consequently, we need to include synonyms of these three sets for generating the search string, taking a similar approach as Petersen et al. (2015) . The importance of including different synonyms arises from different research papers referring to the same phenomena differently. If synonyms are not included, essential papers may be missed from the final sample. The three groups are explained in more detail:
• Set 1: search elements related to security and insecurity due to our population of interest and comparison.
• Set 2: AI-related elements based on our intervention. This set should include LLMs, generative AI, and other approximations.
• Set 3: the research should focus on code generation.
With these three sets of critical elements that our research focuses on, a search string is created. We constructed the search string by including synonyms based on the three sets (as seen in Table 1 ). In a concurrent manner, while identifying the synonyms, we create the search string. Through different iterations, we aim at achieving the “golden” string, following a test-retest approach by Kitchenham et al. (2010) . In every iteration, we checked if the vital papers of our study were in the sample. The final string was selected based on the new synonym that would add meaningful results. For example, one of the iterations included “ hard* ,” which did not add any extra article. Hence, it was excluded. Due to space constraints, the different iterations are available in the public repository of this research. The final string, with the unique query per database, is presented in Table 2 .
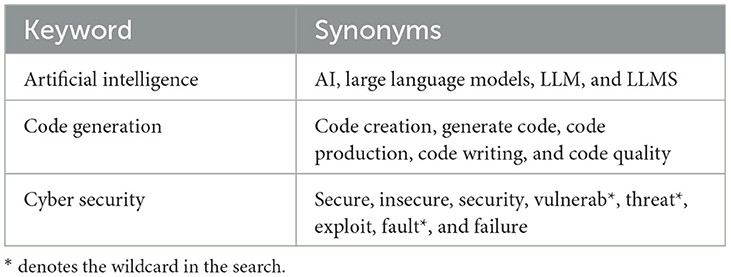
Table 1 . Keywords and synonyms.
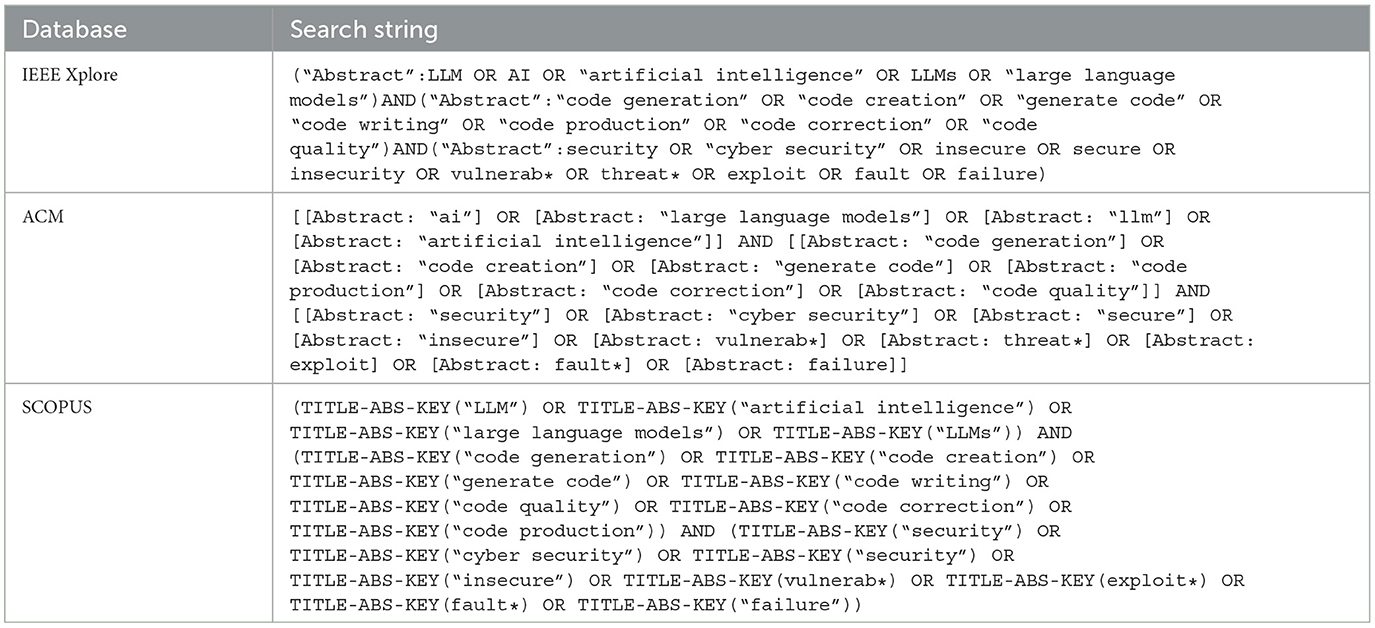
Table 2 . Search string per database.
For this research, we selected the following databases to gather our sample: IEEE Explore, ACM, and Scopus (which includes Springer and ScienceDirect). The databases were selected based on their relevance for computer science research, publication of peer-reviewed research, and alignment with this research objective. Although other databases from other domains could have been selected, the ones selected are notably known in computer science.
3.2 Exclusion and inclusion criteria
The exclusion and inclusion criteria were decided to align our research objectives. Our interest in excluding unranked venues is to avoid literature that is not peer-reviewed and act as a first quality check. This decision also applies to gray literature or book chapters. Finally, we excluded opinion and philosophical papers, as they do not carry out primary research. Table 3 shows are inclusion and exclusion criteria.

Table 3 . Inclusion and exclusion criteria.
We have excluded articles that address AI models or AI technology in general, as our interest—based on PICO—is on the security issue of AI models in code generation. So although such research is interesting, it does not align with our main objective.
For identifying the secondary research, opinion, and philosophical papers—which are all part of our exclusion criteria in Table 3 —we follow the taxonomy provided by Wieringa et al. (2006) . Although this classification was written for the requirements engineering domain, it can be generalized to other domains ( Wieringa et al., 2006 ). In addition, apart from helping us identify if a paper falls under our exclusion criteria, this taxonomy also allows us to identify how complete the research might be. The classification is as follows:
• Solution proposal: Proposes a solution to a problem ( Wieringa et al., 2006 ). “The solution can be novel or a significant extension of an existing technique ( Petersen et al., 2015 ).”
• Evaluation research: “This is the investigation of a problem in RE practice or an implementation of an RE technique in practice [...] novelty of the knowledge claim made by the paper is a relevant criterion, as is the soundness of the research method used ( Petersen et al., 2015 ).”
• Validation research: “This paper investigates the properties of a solution proposal that has not yet been implemented... ( Wieringa et al., 2006 ).”
• Philosophical papers: “These papers sketch a new way of looking at things, a new conceptual framework ( Wieringa et al., 2006 ).”
• Experience papers: Is where the authors publish their experience over a matter. “In these papers, the emphasis is on what and not on why ( Wieringa et al., 2006 ; Petersen et al., 2015 ).”
• Opinion papers: “These papers contain the author's opinion about what is wrong or good about something, how we should do something, etc. ( Wieringa et al., 2006 ).”
3.3 Snowballing
Furthermore, to increase the reliability and validity of this research, we applied a forward snowballing technique ( Wohlin et al., 2013 ; Wohlin, 2014 ). Once the first sample (start set) has passed an exclusion and inclusion criteria based on the title, abstract, and keyword, we forward snowballed the whole start set ( Wohlin et al., 2013 ). That is to say; we checked which papers were citing the papers from our starting set, as suggested by Wohlin (2014) . For this section, we used Google Scholar.
In the snowballing phase, we analyzed the title, abstract, and key words of each possible candidate ( Wohlin, 2014 ). In addition, we did an inclusion/exclusion analysis based on the title, abstract, and publication venue. If there was insufficient information, we analyzed the full text to make a decision, following the recommendations by Wohlin (2014) .
Our objective with the snowballing is to increase the reliability and validity. Furthermore, some articles found through the snowballing had been accepted at different peer-reviewed venues but had not been published yet in the corresponding database. This is a situation we address at Section 6.
3.4 Quality analysis
Once the final sample of papers is collected, we proceed with the quality check, following the procedure of Kitchenham and Charters (2007) and Kitchenham et al. (2010) . The objective behind a quality checklist if 2-fold: “to provide still more detailed inclusion/exclusion criteria” and act “as a means of weighting the importance of individual studies when results are being synthesized ( Kitchenham and Charters, 2007 ).” We followed the approach taken by Kitchenham et al. (2010) for the quality check, taking their questions and categorizing. In addition, to further adapt the questionnaire to our objectives, we added one question on security and adapted another one. The questionnaire is properly described at Table 4 . Each question was scored, according to the scoring scale defined in Table 5 .

Table 4 . Quality criteria questionnaire.
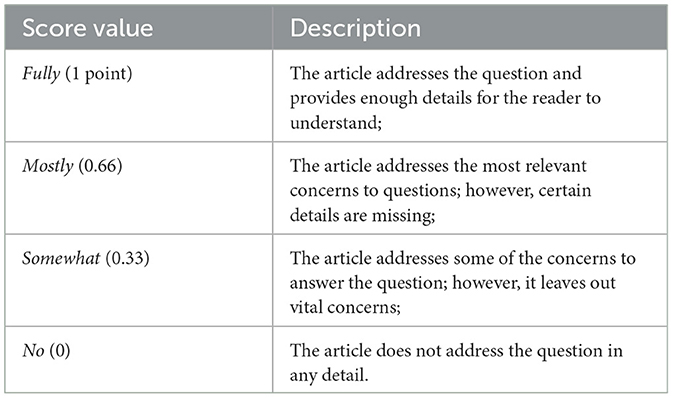
Table 5 . Quality criteria assessment.
The quality analysis is done by at least two authors of this research, for reliability and validity purposes ( Wohlin et al., 2013 ).
3.5 Data extraction
To answer the main question and extract the data, we have subdivided the main question, to answer it. This allows us to extract information and summarize it systematically; we created an extract form in line with ( Kitchenham and Charters, 2007 ; Carrera-Rivera et al., 2022 ). The data extraction form is presented in Table 6 .
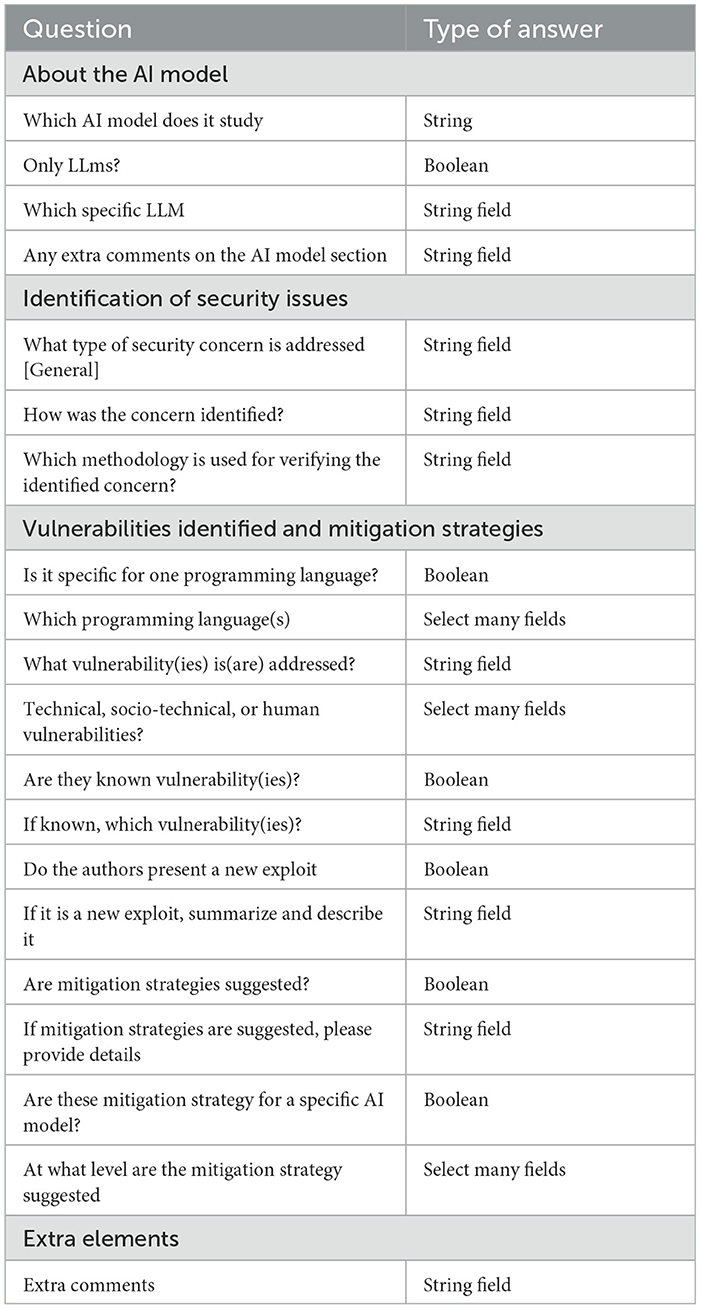
Table 6 . Data extraction form and type of answer.
The data extraction was done by at least two researchers per article. Afterward, the results are compared, and if there are “disagreements, [they must be] resolved either by consensus among researchers or arbitration by an additional independent researcher ( Kitchenham and Charters, 2007 ).”
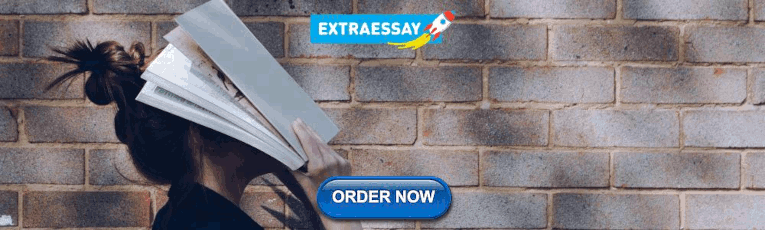
4.1 Search results
The search and recollection of papers were done during the last week of November 2023. Table 7 shows the total number of articles gathered per database. The selection process for our final samples is exemplified in Figure 1 .

Table 7 . Search results per database.
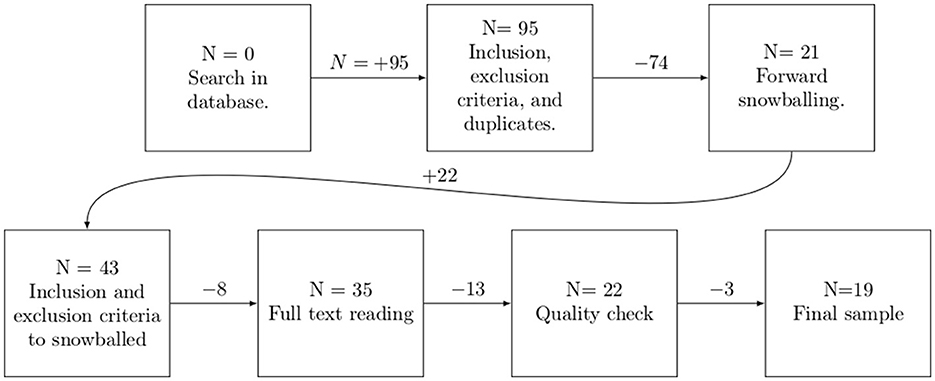
Figure 1 . Selection of sample papers for this SLR.
The total number of articles in our first round, among all the databases, was 95. We then identified duplicates and applied our inclusion and exclusion criteria for the first round of selected papers. This process left us with a sample of 21 articles.
These first 21 artcles are our starting set, from which we proceeded for a forward snowballing. We snowballed each paper of the starting set by searching Google Scholar to find where it had been cited. The selected papers at this phase were based on the title, abstract, based on Wohlin (2014) . From this step, 22 more articles were added to the sample, leaving 43 articles. We then applied inclusion and exclusion criteria to the new snowballed papers, that left us with 35 papers. We discuss this high number of snowballed papers at Section 6.
At this point, we read all the articles to analyze if they should pass to the final phase. In this phase, we discarded 12 articles deemed out of scope for this research, leaving us with 23 articles for quality check. For example, they would not focus on cybersecurity, code generation, or the usage of AI models for code generation.
At this phase, three particular articles (counted among the eight articles previously discarded) sparked discussion between the first and fourth authors regarding whether they were within the scope of this research. We defined AI code generation as artifacts that suggest or produce code. Hence, those artifacts that use AI to check and/or verify code, and vulnerability detection without suggesting new code are not within scope. In addition, the article's main focus should be on code generation and not other areas, such as code verification. So, although an article might discuss code generation, the paper was not accepted as it was not the main topic. As a result, two of the three discussion articles were accepted, and one was rejected.
4.2 Quality evaluation
We carried out a quality check for our preliminary sample of papers ( N = 23) as detailed at Section 3.4. Based on the indicated scoring system, we discarded articles that did not pass 50% of the total possible score (four points). If there were disagreements in the scoring, these were discussed and resolved between authors. Each paper's score details are provided in Table 8 , for transparency purposes ( Carrera-Rivera et al., 2022 ). Quality scores guides us on where to place more weight of importance, and on which articles to focus ( Kitchenham and Charters, 2007 ). The final sample is of N = 19.
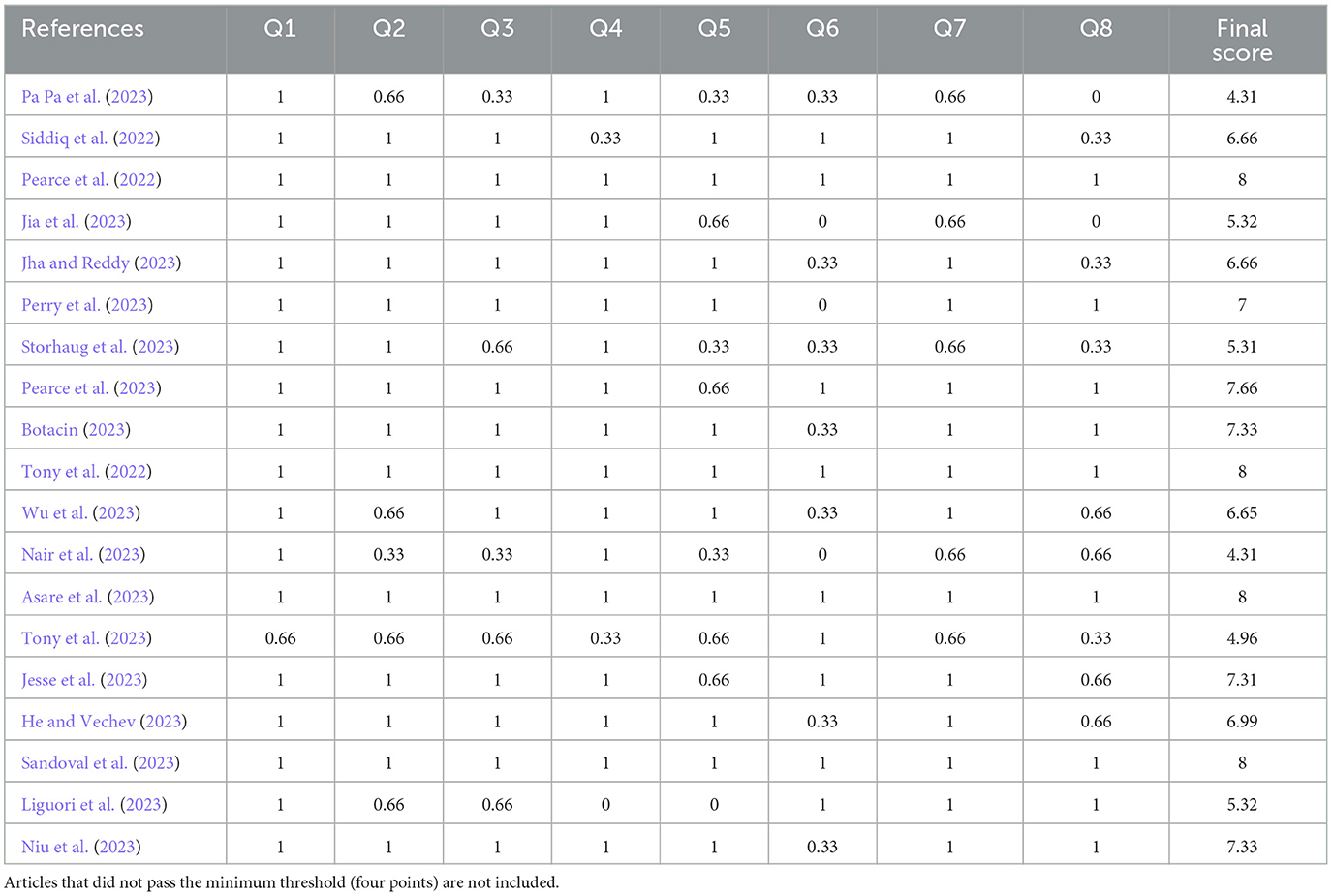
Table 8 . Quality scores of the final sample.
4.3 Final sample
The quality check discarded three papers, which left us with 19 as a final sample, as seen in Table 9 . The first article published in this sample was in 2022 and the number of publications has been increasing every year. This situation is not surprising, as generative AI has risen in popularity in 2020 and has expanded into widespread knowledge with the release of ChatGPT 3.5.
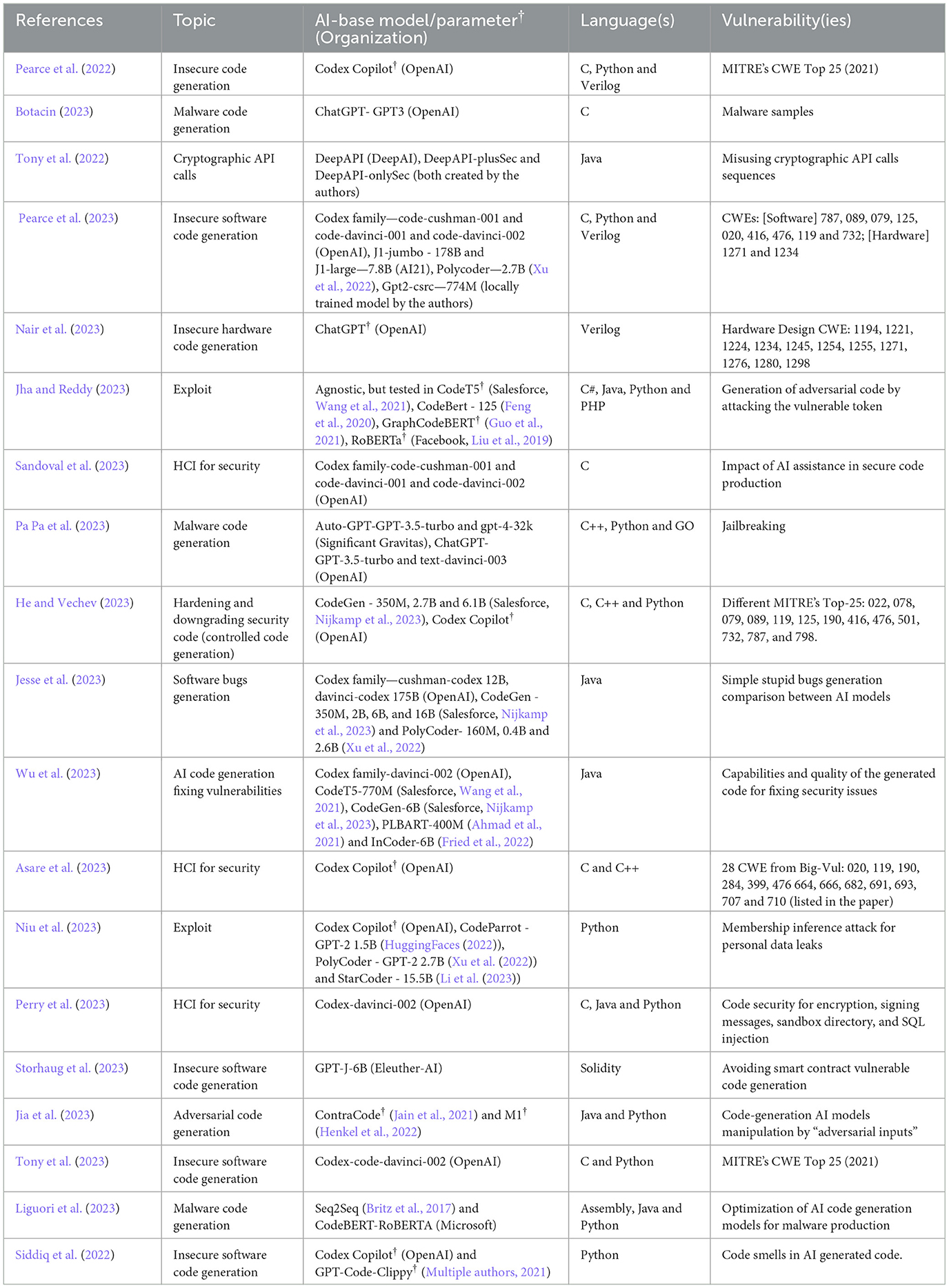
Table 9 . Sample of papers, with the main information of interest ( † means no parameter or base model was specified in the article).
5 Discussion
5.1 about ai models comparisons and methods for investigation.
Almost the majority (14 papers—73%) of the papers research at least one OpenAI model, Codex being the most popular option. OpenAI owns ChatGPT, which was adopted massively by the general public. Hence, it is not surprising that most articles focus on OpenAI models. However, other AI models from other organizations are also studied, Salesforce's CodeGen and CodeT5, both open-source, are prime examples. Similarly, Xu et al. (2022) Polycoder was a popular selection in the sample. Finally, different authors benchmarked in-house AI models and popular models. For example, papers such as Tony et al. (2022) with DeepAPI-plusSec and DeepAPI-onlySec and Pearce et al. (2023) with Gpt2-csrc. Figure 3 shows the LLM instances researched by two or more articles grouped by family.
As the different papers researched different vulnerabilities, it remains difficult to compare the results. Some articles researched specific CWE, other MITRE Top-25, the impact of AI in code, the quality of the code generated, and malware generation, among others. It was also challenging to find the same methodological approach for comparing results, and therefore, we can only infer certain tendencies. For this reason, future research could focus on generating a standardized approach and analyzing vulnerabilities to analyze the quality of security. Furthermore, it would be interesting to have more analysis between open-source and proprietary models.
Having stated this, two articles with similar approaches, topics, and vulnerabilities are Pearce et al. (2022 , 2023) . Both papers share authors, which can help explain the similarity in the approach. Both have similar conclusions on the security of the output of different OpenAI models: they can generate functional and safe code, but the percentage of this will vary between CWE and programming language ( Pearce et al., 2022 , 2023 ). For both authors, the security of the code generated in C was inferior to that in Python ( Pearce et al., 2022 , 2023 ). For example, Pearce et al. (2022) indicates that for Python, 39% of the code suggested is vulnerable and 50% for code in C. Pearce et al. (2023) highlights that the models they studied struggled with fixes for certain CWE, such as CWE-787 in C. So even though they compared different models of the OpenAI family, they produced similar results (albeit some models had better performance than others).
Based on the work of Pearce et al. (2023) , when comparing OpenAI's models to others (such as the AI21 family, Polycoder, and GPT-csrc) in C and Python with CWE vulnerabilities, OpenAI's models would perform better than the rest. In the majority of the cases, code-davinci-002 would outperform the rest. Furthermore, when applying the AI models to other programming languages, such as Verilog, not all models (namely Polycoder and gpt2-csrc) supported it ( Pearce et al., 2023 ). We cannot fully compare these results with other research articles, as they focused on different CWEs but identified tendencies. To name the difference,
• He and Vechev (2023) studies mainly CodeGen and mentions that Copilot can help with CWE-089,022 and 798. They do not compare the two AI models but compare CodeGen with SVEN. They use scenarios to evaluate CWE, adopting the method from Pearce et al. (2022) . CodeGen does seem to provide similar tendencies as Pearce et al. (2022) : certain CWE appeared more recurrently than others. For example, comparing with Pearce et al. (2022) and He and Vechev (2023) , CWE-787, 089, 079, and 125 in Python and C appeared in most scenarios at a similar rate. 4
• This data shows that even OpenAI's and CodeGen models have similar outputs. When He and Vechev (2023) present the “overall security rate” at different temperatures of CodeGen, they have equivalent security rates: 42% of the code suggested being vulnerable in He and Vechev (2023) vs. a 39% in Python and 50% in C in Pearce et al. (2022) .
• Nair et al. (2023) also studies CWE vulnerabilities for Verilog code. Both Pearce et al. (2022 , 2023) also analyze Verilog in OpenAI's models, but with very different research methods. Furthermore, their objectives are different: Nair et al. (2023) focuses on prompting and how to modify prompts for a secure output. What can be compared is that Nair et al. (2023) and Pearce et al. (2023) highlight the importance of prompting.
• Finally Asare et al. (2023) also studies OpenAI from a very different perspective: the human-computer interaction (HCI). Therefore, we cannot compare the study results of Asare et al. (2023) with Pearce et al. (2022 , 2023) .
Regarding malware code generation, both Botacin (2023) and Pa Pa et al. (2023) OpenAI's models, but different base-models. Both conclude that AI models can help generate malware but to different degrees. Botacin (2023) indicates that ChatGPT cannot create malware from scratch but can create snippets and help less-skilled malicious actors with the learning curve. Pa Pa et al. (2023) experiment with different jailbreaks and suggest that the different models can create malware, up to 400 lines of code. In contrast, Liguori et al. (2023) researchers Seq2Seq and CodeBERT and highlight the importance for malicious actors that AI models output correct code if not their attack fails. Therefore, human review is still necessary to fulfill the goals of malicious actors ( Liguori et al., 2023 ). Future work could benefit from comparing these results with other AI code generation models to understand if they have similar outputs and how to jailbreak them.
The last element we can compare is the HCI aspects, specifically Asare et al. (2023) , Perry et al. (2023) , and Sandoval et al. (2023) , who all researched on C. Both Asare et al. (2023) and Sandoval et al. (2023) agree that AI code generation models do not seem to be worse, if not the same, in generating insecure code and introducing vulnerabilities. In contrast, Perry et al. (2023) concludes that developers who used AI assistants generated more insecure code—although this is inconclusive for the C language—as these developers believed they had written more secure code. Perry et al. (2023) suggest that there is a relationship between how much trust there is between the AI model and the security of code. All three agree that AI assistant tools should not be used carefully, particularly between non-experts ( Asare et al., 2023 ; Perry et al., 2023 ; Sandoval et al., 2023 ).
5.2 New exploits
Firstly, Niu et al. (2023) hand-crafted prompts that seemed could leak personal data, which yielded 200 prompts. Then, they queried each of these prompts, obtaining five responses per prompt, giving 1,000 responses. Two authors then looked through the outputs to identify if the prompts had leaked personal data. The authors then improved these with the identified prompts. They tweaked elements such as context, pre-fixing or the natural language (English and Chinese), and meta-variables such as prompt programming language style for the final data set.
With the final set of prompts, the model was queried for privacy leaks. B efore querying the model, the authors also tuned specific parameters, such as temperature. “Using the BlindMI attack allowed filtering out 20% of the outputs, with the high recall ensuring that most of the leakages are classified correctly and not discarded ( Niu et al., 2023 ).” Once the outputs had been labeled as members, a human checked if they contained “sensitive data” ( Niu et al., 2023 ). The human could categorize such information as targeted leak, indirect leak, or uncategorized leak.
When applying the exploit to Codex Copilot and verifying with GitHub, it shows there is indeed a leakage of information ( Niu et al., 2023 ). 2.82% of the outputs contained identifiable information such as address, email, and date of birth; 0.78% private information such as medical records or identities; and 0.64% secret information such as private keys, biometric authentication or passwords ( Niu et al., 2023 ). The instances in which data was leaked varied; specific categories, such as bank statements, had much lower leaks than passwords, for example Niu et al. (2023) . Furthermore, most of the leaks tended to be indirect rather than direct. This finding implies that “the model has a tendency to generate information pertaining to individuals other than the subject of the prompt, thereby breaching privacy principles such as contextual agreement ( Niu et al., 2023 ).”
Their research proposes a scalable and semi-automatic manner to leak personal data from the training data in a code-generation AI model. The authors do note that the outputs are not verbatim or memorized data.
To achieve this, He and Vechev (2023) curated a dataset of vulnerabilities from CrossVul ( Nikitopoulos et al., 2021 ) and Big-Vul ( Fan et al., 2020 ), which focuses in C/C++ and VUDENC ( Wartschinski et al., 2022 ) for Python. In addition, they included data from commits from GitHub, taking into special consideration that they were true commits, avoiding that SVEN learns “undesirable behavior.” At the end, they target 9 CWES from MITRE Top 25.
Through benchmarking, they evaluate SVEN output's security (and functional) correctness against CodeGen (350M, 2.7B, and 6.1B). They follow a scenario-based approach “that reflect[s] real-world coding ( He and Vechev, 2023 ),” with each scenario targeting one CWE. They measure the security rate, which is defined as “the percentage of secure programs among valid programs ( He and Vechev, 2023 ).” They set the temperature at 0.4 for the samples.
Their results show that SVEN can significantly increase and decrease (depending on the controlled generation output) the code security score. “CodeGen LMs have a security rate of ≈60%, which matches the security level of other LMs [...] SVEN sec significantly improves the security rate to >85%. The best-performing case is 2.7B, where SVENsec increases the security rate from 59.1 to 92.3% ( He and Vechev, 2023 ).” Similar results are obtained for SVEN vul with the “security rate greatly by 23.5% for 350M, 22.3% for 2.7B, and 25.3% for 6.1B ( He and Vechev, 2023 )”. 5 When analyzed per CWE, in almost all cases (except CWE-416 language C) SVEN sec increases the security rate. Finally, even when tested with 4 CWE that were not included in the original training set of 9, SVEN had positive results.
Although the authors aim at evaluating and validating SVEN, as an artifact for cybersecurity, they also recognize its potential use as a malicious tool. They suggest that SVEN can be inserted in open-source projects and distributed ( He and Vechev, 2023 ). Future work could focus on how to integrate SVEN—or similar approaches—as plug-ins into AI code generations, to lower the security of the code generated. Furthermore, replication of this approach could raise security alarms. Other research can focus on seeking ways to lower the security score while keeping the functionality and how it can be distributed across targeted actors.
They benchmark CodeAttack against the TextFooler and BERT-Attack, two other adversarial attacks in three tasks: code translation (translating code between different programming languages, in this case between C# and Java), code repair (fixes bugs for Java) and code (a summary of the code in natural language). The authors also applied the benchmark in different AI models (CodeT5, CodeBERT, GraphCode-BERT, and RoBERTa) in different programming languages (C#, Java, Python, and PHP). In the majority of the tests, CodeAttack had the best results.
5.3 Performance per programming language
Different programming languages are studied. Python and the C family are the most common languages, including C, C++, and C# (as seen in Figure 2 ). To a lesser extent, Java and Verilog are tested. Finally, specific articles would study more specific programming languages, such as Solidity, Go or PHP. Figure 2 offers a graphical representation of the distribution of the programming languages.
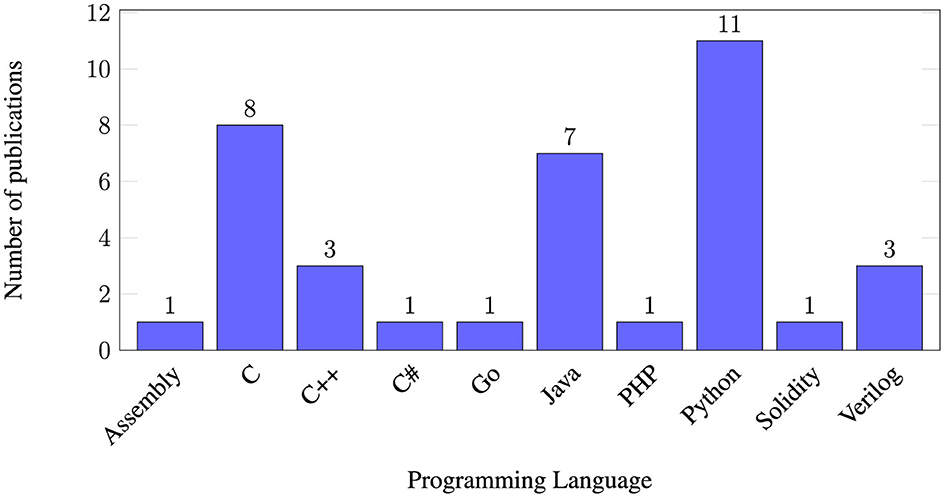
Figure 2 . Number of articles that research specific programming languages. An article may research 2 or more programming languages.
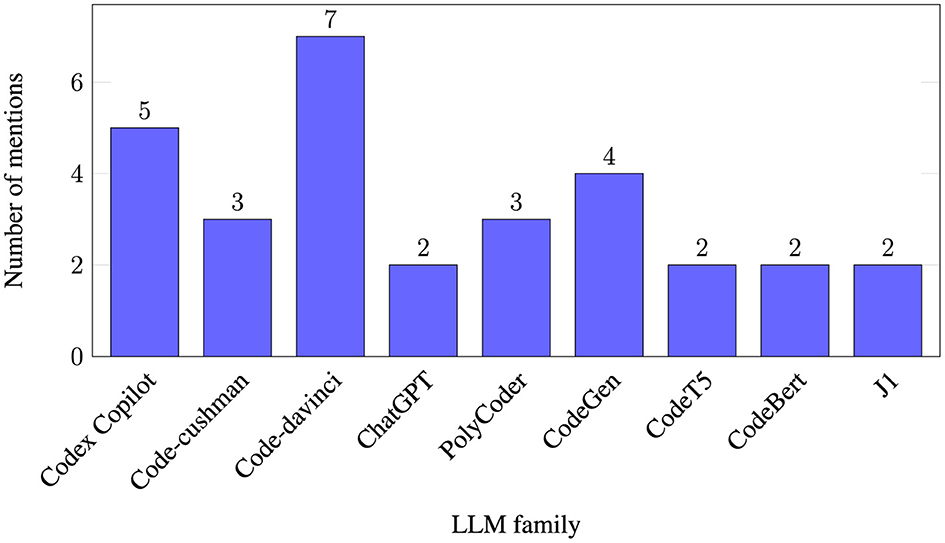
Figure 3 . Number of times each LLM instance was researched by two or more articles, grouped by family. One paper might study several instances of the same family (e.g., Code-davinci-001 and Code-davinci-002), therefore counting twice. Table 9 offers details on exactly which AI models are studied per article.
5.3.1 Python
Python is the second most used programming language 6 as of today. As a result most publicly-available training corpora include Python and it is therefore reasonable to assume that AI models can more easily be tuned to handle this language ( Pearce et al., 2022 , 2023 ; Niu et al., 2023 ; Perry et al., 2023 ). Being a rather high level, interpreted language, Python should also expose a smaller attack surface. As a result, AI-generated Python code has fewer avenues to cause issues to begin with, and this is indeed backed up by evidence ( Pearce et al., 2022 , 2023 ; Perry et al., 2023 ).
In spite of this, issues still occur: Pearce et al. (2022) experimented with 29 scenarios, producing 571 Python programs. Out of these, 219 (38.35%) presented some kind of Top-25 MITRE (2021) vulnerability, with 11 (37.92%) scenarios having a top-vulnerable score. Unaccounted in these statistics are the situations where generated programs fail to achieve functional correctness ( Pearce et al., 2023 ), which could yield different conclusions. 7
Pearce et al. (2023) , building from Pearce et al. (2022) , study to what extent post-processing can automatically detect and fix bugs introduced during code generation. For instance, on CWE-089 (SQL injection) they found that “29.6% [3197] of the 10,796 valid programs for the CWE-089 scenario were repaired” by an appropriately-tuned LLM ( Pearce et al., 2023 ). In addition, they claim that AI models can generate bug-free programs without “additional context ( Pearce et al., 2023 ).”
It is however difficult to support such claims, which need to be nuanced. Depending on the class of vulnerability, AI models varied in their ability in producing secure Python code ( Pearce et al., 2022 ; He and Vechev, 2023 ; Perry et al., 2023 ; Tony et al., 2023 ). Tony et al. (2023) experimented with code generation from natural language prompts, findings that indeed, Codex output included vulnerabilities. In another research,Copilot reports only rare occurences of CWE-079 or CWE-020, but common occurences of CWE-798 and CWE- 089 ( Pearce et al., 2022 ). Pearce et al. (2022) report a 75% vulnerable score for scenario 1, 48% scenario 2, and 65% scenario 3 with regards to CWE-089 vulnerability ( Pearce et al., 2022 ). In February 2023, Copilot launched a prevention system for CWEs 089, 022, and 798 ( He and Vechev, 2023 ), the exact mechanism of which is unclear. At the time of writing it falls behind other approaches such as SVEN ( He and Vechev, 2023 ).
Perhaps surprisingly, there is not much variability across different AI models: CodeGen-2.7B has comparable vulnerability rates ( He and Vechev, 2023 ), with CWE-089 still on top. CodeGen-2.7B also produced code that exhibited CWE-078, 476, 079, or 787, which are considered more critical.
One may think that using AI as an assistant to a human programmer could alleviate some of these issues. Yet evidence points to the opposite: when using AI models as pair programmers, developers consistently deliver more insecure code for Python ( Perry et al., 2023 ). Perry et al. (2023) led a user-oriented study on how the usage of AI models for programming affects the security and functionality of code, focusing on Python, C, and SQL. For Python, they asked participants to write functions that performed basic cryptographic operations (encryption, signature) and file manipulation. 8 They show a statistically significant difference between subjects that used AI models (experimental group) and those that did not (control group), with the experimental group consistently producing less secure code ( Perry et al., 2023 ). For instance, for task 1 (encryption and decryption), 21% of the responses of the experiment group was secure and correct vs. 43% of the control group ( Perry et al., 2023 ). In comparison, 36% of the experiment group provided insecure but correct code, compared to 14%.
Even if AI models produce on occasion bug-free and secure code, evidence points out that it cannot be guaranteed. In this light, both Pearce et al. (2022 , 2023) recommend deploying additional security-aware tools and methodologies whenever using AI models. Moreover, Perry et al. (2023) suggests a relationship between security awareness and trust in AI models on the one hand, and the security of the AI-(co)generated code.
Another point of agreement in our sample is that prompting plays a crucial role in producing vulnerabilities, which can be introduced or avoided depending on the prompt and adjustment of parameters (such as temperature). Pearce et al. (2023) observes that AI models can generate code that repairs the issue when they are given a suitable repair prompt. Similarly, Pearce et al. (2022) analyzed how meta-type changes and comments (documentation) can have varying results over the security ( Pearce et al., 2022 ). An extreme example is the difference between an SQL code generated with different prompts: the prompt “adds a separate non-vulnerable SQL function above a task function” (identified as variation C-2, as it is a code change) would never produce vulnerable code whereas “adds a separate vulnerable SQL function above the task function” (identified as variation C-3) returns vulnerable code 94% of the time ( Pearce et al., 2022 ). Such results may not be surprising if we expect the AI model to closely follow instructions, but suffice to show the effect that even minor prompt variations can have on security.
Lastly, Perry et al. (2023) observe in the experimental group a relationship between parameters of the AI model (such as temperature) and code quality. They also observe a relationship between education, security awareness, and trust ( Perry et al., 2023 ). Because of this, there could be spurious correlations in their analysis, for instance the variable measuring AI model parameters adjustments could be, in reality, measuring education or something else.
On another security topic, Siddiq et al. (2022) study code and security “smells.” Smells are hints, not necessarily actual vulnerabilities, but they can open the door for developers to make mistakes that lead to security flaws that attackers exploit. Siddiq et al. (2022) reported on the following CWE vulnerabilities: 078,703,330. They have concluded that bad code patterns can (and will) leak to the output of models, and code generated with these tools should be taken with a “grain of salt” ( Siddiq et al., 2022 ). Furthermore, identified vulnerabilities may be severe (not merely functional issues) ( Siddiq et al., 2022 ). However, as they only researched OpenAI's AI models, their conclusion may lack external validity and generalization.
Finally, some authors explore the possibility to use AI models to deliberately produce malicious code ( He and Vechev, 2023 ; Jha and Reddy, 2023 ; Jia et al., 2023 ; Niu et al., 2023 ). It is interesting to the extent that this facilitates the work of attackers, and therefore affects cybersecurity as a whole, but it does not (in this form at least) affect the software development process or deployment per se, and is therefore outside of the scope of our discussion.
The C programming language is considered in 10 (52%) papers of our final sample, with C being the most common, followed by C++ and C#. Unlike Python, C is a low-level, compiled language, that puts the programmer in charge of many security-sensitive tasks (such as memory management). The vast majority of native code today is written in C. 9
The consensus is that AI generation of C programs yields insecure code ( Pearce et al., 2022 , 2023 ; He and Vechev, 2023 ; Perry et al., 2023 ; Tony et al., 2023 ), and can readily be used to develop malware ( Botacin, 2023 ; Liguori et al., 2023 ; Pa Pa et al., 2023 ). However, it is unclear whether AI code generation introduce more or new vulnerabilities compared to humans ( Asare et al., 2023 ; Sandoval et al., 2023 ), or to what extent they influence developers' trust in the security of the code ( Perry et al., 2023 ).
Multiple authors report that common and identified vulnerabilities are regularly found in AI-generated C code ( Pearce et al., 2022 , 2023 ; Asare et al., 2023 ; He and Vechev, 2023 ; Perry et al., 2023 ; Sandoval et al., 2023 ). Pearce et al. (2022) obtained 513 C programs, 258 of which (50.29% ) had a top-scoring vulnerability. He and Vechev (2023) provides a similar conclusion.
About automated code-fixing, Asare et al. (2023) and Pearce et al. (2023) report timid scores, with only 2.2% of C code for CWE-787.
On the question of human- vs. AI-generated code, Asare et al. (2023) used 152 scenarios to conclude that AI models make in fact fewer mistakes. Indeed, when prompted with the same scenario as a human, 33% cases suggested the original vulnerability, and 25% provided a bug-free output. Yet, when tested on code replication or automated vulnerability fixing, the authors do not recommend the usage of a model by non-experts. For example, in code replication, AI models would always replicate code regardless of whether it had a vulnerability, and CWE-20 would consistently be replicated ( Asare et al., 2023 ).
Sandoval et al. (2023) experimentally compared the security of code produced by AI-assisted students to the code generated by Codex. They had 58 participants and studied memory-related CWE, given that they are in the Top-25 MITRE list ( Sandoval et al., 2023 ). Although there were differences between groups, these were not bigger than 10% and would differ between metrics ( Sandoval et al., 2023 ). In other words, depending on the chosen metric, sometimes AI-assisted subjects perform better in security and vice versa ( Sandoval et al., 2023 ). For example, CWE-787 was almost the same for the control and experimental groups, whereas the generated Codex code was prevalent. Therefore, they conclude that the impact on “cybersecurity is less conclusive than the impact on functionality ( Sandoval et al., 2023 ).” Depending on the security metric, it may be beneficial to use AI-assisted tools, which the authors recognize goes against standard literature ( Sandoval et al., 2023 ). They go so far as to conclude that there is “no conclusive evidence to support the claim LLM assistant increase CWE incidence in general, even when we looked only at severe CWEs ( Sandoval et al., 2023 ).”
Regarding AI-assisted malware generation, there seems to be fundamental limitations preventing current AI models from writing self-contained software from scratch ( Botacin, 2023 ; Liguori et al., 2023 ; Pa Pa et al., 2023 ), although it is fine for creating smaller blocks of code which, strung together, produce a complete malware ( Botacin, 2023 ). It is also possible to bypass models' limitations by leveraging basic obfuscation techniques ( Botacin, 2023 ). Pa Pa et al. (2023) experiment prompts and jailbreaks in ChatGPT to produce code (specifically, fileless malware for C++), which was only provided with 2 jailbreaks they chose. While Liguori et al. (2023) reflect on how to best optimize AI-generating tools to assist attackers in producing code, as failure or incorrect codes means the attack fails.
Over CWE, Top MITRE-25 is a concern across multiple authors ( Pearce et al., 2022 , 2023 ; He and Vechev, 2023 ; Tony et al., 2023 ). CWE-787 is a common concern across articles, as it is the #1 vulnerability in the Top-25 MITRE list ( Pearce et al., 2022 ; Botacin, 2023 ; He and Vechev, 2023 ). On the three scenarios experimented by Pearce et al. (2022) , on average, ~34% of the output is vulnerable code. He and Vechev (2023) tested with two scenarios, the first receiving a security rate of 33.7% and the second one 99.6%. What was interesting in their experiment is that they were not able to provide lower security rates for SVEN vul than the originals ( He and Vechev, 2023 ). Other vulnerabilities had varying results but with a similar trend. Overall, it seems that the AI code generation models produce more vulnerable code compared to other programming languages, possibly due to the quality and type of data in the training data set ( Pearce et al., 2022 , 2023 ).
Finally, regarding human-computer interaction, Perry et al. (2023) suggests that subjects “with access to an AI assistant often produced more security vulnerabilities than those without access [...] overall.” However, they highlight that their difference is not statistically significant and inconclusive for the case they study in C. So even if the claim applies to Python, Perry et al. (2023) indicates this is not the case for the C language. Asare et al. (2023) and Sandoval et al. (2023) , as discussed previously, both conclude that AI models do not introduce more vulnerabilities than humans into code. “This means that in a substantial number of scenarios we studied where the human developer has written vulnerable code, Copilot can avoid the detected vulnerability ( Asare et al., 2023 ).”
Java 10 is a high-level programming language that runs atop a virtual machine, and is today primarily used for the development of mobile applications. Vulnerabilities can therefore arise from programs themselves, calls to vulnerable (native) libraries, or from problems within the Java virtual machine. Only the first category of issues is discussed here.
In our sample, four articles ( Tony et al., 2022 ; Jesse et al., 2023 ; Jha and Reddy, 2023 ; Wu et al., 2023 ) analyzed code generation AI models for Java. Each research focused on very different aspects of cyber security and they did not analyze the same vulnerabilities. Tony et al. (2022) investigated the dangers and incorrect of API calls for cryptographic protocols. Their conclusions is that generative AI might not be at all optimized for generating cryptographically secure code ( Tony et al., 2022 ). The accuracy of the code generated was significantly lower on cryptographic tasks than what the AI is advertised to have on regular code ( Tony et al., 2022 ).
Jesse et al. (2023) experiments with generating single stupid bugs (SStuB) with different AI models. They provide six main findings, which can be summarized as: AI models propose twice as much SSTuB as correct code. However, they also seem to help with other SStuB ( Jesse et al., 2023 ). 11 One of the issues with SStuBs is that “where Codex wrongly generates simple, stupid bugs, these may take developers significantly longer to fix than in cases where Codex does not ( Jesse et al., 2023 ).” In addition, different AI models would behave differently over the SStuBs generated ( Jesse et al., 2023 ). Finally, Jesse et al. (2023) found that commenting on the code leads to fewer SStuBs and more patches, even if the code is misleading.
Wu et al. (2023) analyze and compare (1) the capabilities of different LLMs and fine-tuned LLMs and automated program repair (APR) techniques for repairing vulnerabilities in Java; (2) proposes VJBench and VJBench-trans as a “new vulnerability repair benchmark;” (3) and evaluates the studied AI models on their proposed VJBench and VJBench-trans. VJBench aims to extend the work of Vul4J and thus proposes 42 vulnerabilities, including 12 new CWEs that were not included in Vul4J ( Wu et al., 2023 ). Therefore, their study assessed 35 vulnerabilities proposed by Vul4J and 15 by the authors ( Wu et al., 2023 ). On the other hand, VJBench-trans is composed of “150 transformed Java vulnerabilities ( Wu et al., 2023 ).” Overall, they concluded that the AI models fix very few Java vulnerabilities, with Codex fixing 20.4% of them ( Wu et al., 2023 ). Indeed, “large language models and APR techniques, except Codex, only fix vulnerabilities that require simple changes, such as deleting statements or replacing variable/method names ( Wu et al., 2023 ).” Alternatively, it seems that fine-tuning helps the LLMs improve the task of fixing vulnerabilities ( Wu et al., 2023 ).
However, four APR and nine LLMs did not fix the new CWEs introduced by VJBench ( Wu et al., 2023 ). Some CWEs that are not tackled are “CWE-172 (Encoding error), CWE-325 (Missing cryptographic step), CWE-444 (HTTP request smuggling; Wu et al., 2023 ),” which can have considerable cybersecurity impacts. For example, CWE-325 can weaken a cryptographic protocol, thus lowering the security capacity. Furthermore, apart from Codex, the other AI models and APR studied did not apply complex vulnerability repair but would focus on “simple changes, such as deletion of a statement ( Wu et al., 2023 ).”
Jia et al. (2023) study the possibility that a code-generation AI model is manipulated by “adversarial inputs.” In other words, the user inputs designed to trick the model into either misunderstanding code, or producing code that behaves in an adversarially-controlled way. They tested Claw, M1 and ContraCode both in Python and Java for the following tasks: code summarization, code completion and code clone detection ( Jia et al., 2023 ).
Finally, Jha and Reddy (2023) proposes CodeAttack , which is implemented in different programming languages, including Java. 12 When tested in Java, their results show that 60% of the adversarial code generated is syntactically correct ( Jha and Reddy, 2023 ).
5.3.4 Verilog
Verilog is a hardware-description language. Unlike other programming languages discussed so far, its purpose is not to describe software but to design and verify of digital circuits (at the register-transfer level of abstraction).
The articles that researched Verilog generally conclude that the AI models they researched are less efficient in this programming language than Python or C ( Pearce et al., 2022 , 2023 ; Nair et al., 2023 ). Different articles would research different vulnerabilities, with two specific CWEs standing out: 1271 and 1234. Pearce et al. (2022) summarizes the difficulty of defining which vulnerability to study from the CWE for Verilog, as there is no Top 25 CWE for hardware. Hence, their research selected vulnerabilities that could be analyzed ( Pearce et al., 2022 ). This situation produces difficulties in comparing research and results, as different authors can select different focuses. The different approaches to vulnerabilities in Verilog can be seen in Table 9 , where only two CWE are common across all studies (1271 and 1234), but others such as 1221 ( Nair et al., 2023 ) or 1294 ( Pearce et al., 2022 ) are researched by one article.
Note that unlike software vulnerabilities, it is much harder to agree on a list of the most relevant hardware vulnerabilities, and to the best of our knowledge there is no current consensus on the matter today.
Regarding the security concern, both Pearce et al. (2022 , 2023) , studying OpenAI, indicated that in general these models struggled to produce correct, functional, and meaningful code, being less efficient over the task. For example, Pearce et al. (2022) generates “198 programs. Of these, 56 (28.28%) were vulnerable. Of the 18 scenarios, 7 (38.89 %) had vulnerable top-scoring options.” Pearce et al. (2023) observes that when using these AI models to generate repair code, firstly, they had to vary around with the temperature of the AI model (compared to C and Python), as it produced different results. Secondly, they conclude that the models behaved differently with Verilog vs. other languages and “seemed [to] perform better with less context provided in the prompt ( Pearce et al., 2023 ).” The hypothesis on why there is a difference between Verilog and other programming languages is because there is less training data available ( Pearce et al., 2022 ).
5.4 Mitigation strategies
There have been several attempts, or suggestions, to mitigate the negative effects on security when using AI to code. Despite reasonable, not all are necessarily effective, as we discuss in the remainder of this section. Overall, the attempts we have surveyed discuss how modify the different elements that can affect the quality of the AI models or the quality of the user control over the AI-generated code. Table 10 summarizes the suggested mitigation strategies.
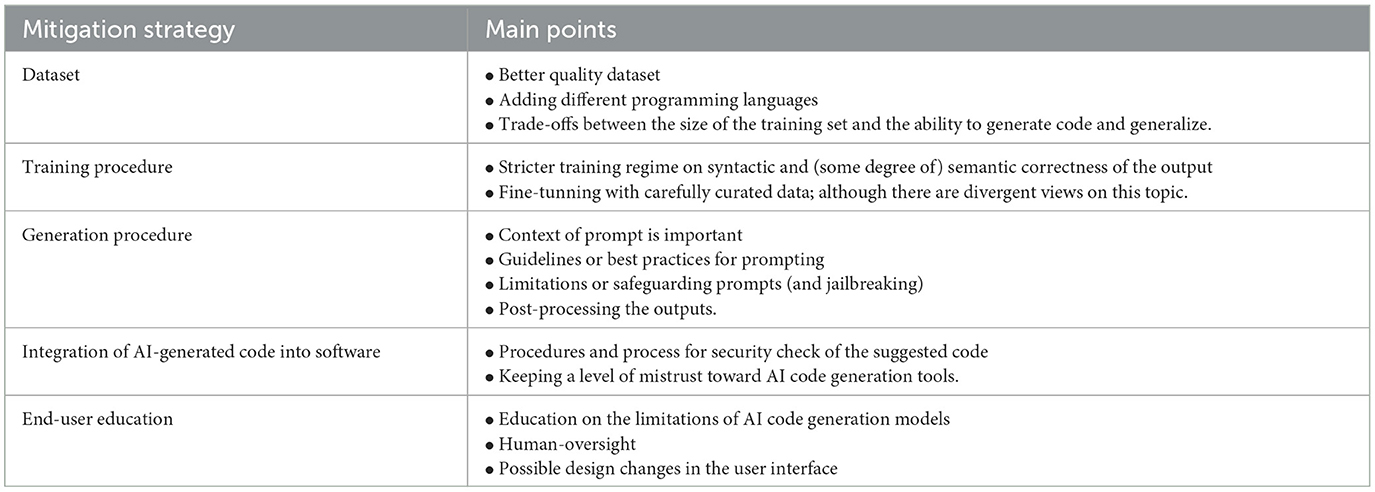
Table 10 . Summary of the mitigation strategies.
5.4.1 Dataset
Part of the issue is that LLMs are trained on code that is itself ripe with vulnerabilities and bad practice. As a number of the AI models are not open-source or their training corpora is no available, different researchers hypothesize that the security issue arise from the training dataset ( Pearce et al., 2022 ). Adding datasets that include different programming languages with different vulnerabilities may help reduce the vulnerabilities in the output ( Pearce et al., 2022 ). This is why, to mitigate the problems with dataset security quality, He and Vechev (2023) manually curated the training data for fine-tuning, which improved the output performance against the studied CWE.
By carefully selecting training corpora that are of higher quality, which can be partially automated, there is hope that fewer issues would arise ( He and Vechev, 2023 ). However, a consequence of such a mitigation is that the size of the training set would be much reduced, which weakens the LLM's ability to generate code and generalize ( Olson et al., 2018 ). Therefore one may expect that being too picky with the training set would result, paradoxically, in a reduction in code output quality. A fully fledged study of this trade-off remains to be done.
5.4.2 Training procedure
During the training process, LLMs are scored on their ability to autoencode, that is, to accurately reproduce their input (in the face of a partially occulted input). In the context of natural language, minor errors are often acceptable and almost always have little to no impact on the meaning or understanding of a sentence. Such is not the case for code, which can be particularly sensitive to minor variations, especially for low-level programming languages. A stricter training regimen could score an LLM based not only on syntactic correctness, but on (some degree of) semantic correctness, to limit the extent to which the model wanders away from a valid program. Unfortunately, experimental data from Liguori et al. (2023) suggests that currently no single metric succeeds at that task.
Alternatively, since most LLMs today come pre-trained, a better fine-tuning step could reduce the risks associated with incorrect code generation. He and Vechev (2023) took this approach and had promising results in the CWE they investigated. However, there is conflicting evidence. Evidence from Wu et al. (2023) seems to indicate that this approach is inherently limited to fixing a very narrow, and simple class of bugs. More studies analyzing the impact of fine-tuning models with curated security datasets are needed to assess the impact of this mitigation strategy.
5.4.3 Generation procedure
Code quality is improved by collecting more context that the user typically provides through their prompts ( Pearce et al., 2022 ; Jesse et al., 2023 ). The ability to use auxiliary data, such as other project files, file names, etc. seems to explain the significant difference in code acceptation between GitHub Copilot and its bare model OpenAI Codex. The exploration of creating guidelines and best practices on how to do prompts effectively may be interesting. Nair et al. (2023) explored the possibility of creating prompt strategies and techniques for ChatGPT that would output secure code.
From an adversarial point of view, Niu et al. (2023) provides evidence of the impact of context and prompts for exploiting AI models. There are ongoing efforts to limit which prompts are accepted by AI systems by safeguarding them ( Pa Pa et al., 2023 ). However, Pa Pa et al. (2023) showed—with mixed results—how to bypass these limitations, what is called “jailbreaking.” Further work on this area is needed as a mitigation strategy and its effectiveness.
Independently, post-processing the output (SVEN is one example; He and Vechev, 2023 ) has a measurable impact on code quality, and is LLM-agnostic, operating without the need for re-training nor fine-tuning. Presumably, non-LLM static analyzers or linters may be integrated as part of the code generation procedure to provide checks along the way and avoid producing code that is visibly incorrect or dangerous.
5.4.4 Integration of AI-generated code into software
Even after all the technical countermeasures have been taken to avoid producing code that is obviously incorrect, there remains situations where AI-generated programs contain (non-obvious) vulnerabilities. To a degree, such vulnerabilities could also appear out of human-generated code, and there should in any case be procedures to try and catch these as early as possible, through unit, functional and integration testing, fuzzing, or static analysis. Implementation of security policies and processes remains vital.
However AI models are specifically trained to produce code that looks correct, meaning that their mistakes may be of a different nature or appearance than those typically made by human software programmers, and may be harder to spot. At the same time, the very reason why code generation is appealing is that it increases productivity, hence the amount of code in question.
It is therefore essential that software developers who rely on AI code generation keep a level of mistrust with regards to these tools ( Perry et al., 2023 ). It is also likely that code review methodologies should be adjusted in the face of AI-generated code to look for the specific kind of mistakes or vulnerabilities that this approach produces.
5.4.5 End-user education
One straightforward suggestion is educating users to assess the quality of software generated with AI models. Among the works we have reviewed, we found no studies that specifically discuss the quality and efficacy of this potential mitigation strategy, so we can only speculate about it from related works. For instance, Moradi Dakhel et al. (2023) compares the code produced by human users with the code generated by GitHub Copilot. The study is not about security. It is about the correctness of the implementation of quite well-known algorithms. Still, human users—students with an education in algorithms—performed better than their AI counterparts, but the buggy solutions generated by Copilot were easily fixable by the users. Relevantly, the AI-generated bugs were more easily recognizable and fixable than those produced by other human developers performing the same task.
This observation suggests that using AI could help write code faster for programmers skilled in debugging and that this task should not hide particular complexity for them. As Chen et al. (2021) suggested, “human oversight and vigilance is required for safe use of code generation systems like Codex.” However, removing obvious errors from buggy implementations of well-known algorithms is not the same as spotting security vulnerabilities: the latter task is complex and error-prone, even for experts. And here we speculate that if AI-generated flaws are naïve, programmers can still have some gain from using AI if they back up coding with other instruments used in security engineering (e.g., property checking, code inspection, and static analysis). Possible design changes or decision at the user interfaces may also have an impact. However, we have no evidence of whether our speculative idea can work in practice. The question remains open and calls for future research.
6 Threats to validity and future work
Previous literature Wohlin et al. (2013) and Petersen et al. (2015) have identified different reliability and validity issues in systematic literature reviews. One of the first elements that needs to be noted is the sample of papers. As explained by Petersen et al. (2015) , the difference between systematic mapping studies and systematic literature reviews is the sample's representativeness; mappings do not necessarily need to obtain the whole universe of papers compared with literature reviews. Nevertheless, previous research has found that even two exact literature reviews on the same subject do not have the same sample of papers, affecting it. Consequently, to increase the reliability, we identified the PICO of our research and used golden standard research methods for SLR, such as Kitchenham and Charters (2007) . This strategy helps us develop different strings for the databases tested to obtain the most optimal result. Furthermore, aiming to obtain a complete sample, we followed a forward snowballing of the whole sample obtained in the first round, as suggested by Wohlin et al. (2013) and Petersen et al. (2015) .
However, there may still be reliability issues with the sample. Firstly, the amount of ongoing publications on the subjects increases daily. Therefore, the total number would increase depending on the day the sample was obtained. Furthermore, some research on open-source platforms (such as ArXiV) did not explicitly indicate if it was peer-reviewed. Hence, the authors manually checked whether it was accepted at a peer-review venue. This is why we hypothesize that the snowballing phase provided many more papers, as these had yet to be indexed in the databases and were only available at open-source platforms. Therefore, the final sample of this research may increase and change depending on the day the data was gathered.
In addition, the sample may differ based on the definition of “code generation.” For this research and as explained in Section 4 , we worked around the idea that AI models should suggest code (working or not). Some papers would fall under our scope in some cases, even if the main topic were “verification and validation,” as the AI tools proposed for this would suggest code. Hence, we focus not only on the development phase of the SDLC but also on any phase that suggests code. Different handling of “code generation” may provide different results.
On another note, the background and expertise of the researchers affect how papers are classified and information is extracted ( Wohlin et al., 2013 ). In this manner, in this research, we used known taxonomies and definitions for classification schemes, such as Wieringa et al. (2006) for the type of research or MITRE's Top Vulnerabilities to identify which are the most commonly discussed risk vulnerabilities. The objective of using well-known classification schemes and methodologies is to reduce bias, as identified ( Petersen et al., 2015 ). However, a complete reduction of bias cannot be ruled out.
Moreover, to fight authors' bias, every single article was reviewed, and data was extracted by at least two others, using a pairing strategy. If, due to time constraints, it was only reviewed by one author, the other author would review the work ( Wohlin et al., 2013 ). If disagreements appeared at any phase – such as the inclusion/exclusion or data gathering – a meeting would be done and discussed ( Wohlin et al., 2013 ). For example, in a couple of papers, Author #1 was unsure if it should be included or excluded based on the quality review, which was discussed with Author #4. Our objective in using a pairing strategy is to diminish authors' bias throughout the SLR.
On the analysis and comparison of the different articles, one threat to the validity of this SLR is that not all articles use the same taxonomy for vulnerabilities; they could not be classified under a single method. Some articles would research either MITRE's CWE or the Top-25, and others would tackle more specific vulnerabilities (such as jailbreaking, malware creation, SSB, and human programming). Therefore, comparing the vulnerabilities between the articles is, at best, complicated and, at worst, a threat to our conclusions. Given the lack of a classification scheme for the wide range of security issues tackled in our sample, we (1) tried to classify the papers based on the claims of the papers' articles; (2) we aimed at comparing based on the programming language used, and between papers researched similar subjects, such as MITRE's CWE. In this manner, we would not be comparing completely different subjects. As recognized by Petersen et al. (2015) , the need for a classification scheme for specific subjects is a common challenge for systematic mapping studies and literature reviews. Nevertheless, future studies would benefit from a better classification approach if the sample permits.
We have provided the whole sample at: https://doi.org/10.5281/zenodo.10666386 for replication and transparency, with the process explained in detail. Each paper has details on why it was included/excluded, at which phase, and with details and/or comments to help readers understand and replicate our research. Likewise, we explained our research methods in as much detail as possible in the papers. Tangently, providing the details and open sources of the data helps us increase validity issues that may be present in this study.
Nonetheless, even when using well-known strategies both for the SLR and to mitigate known issues, we cannot rule out that there are inherent validity and reliability elements proper from all SLRs. We did our best efforts to mitigate these.
7 Conclusion
By systematically reviewing the state of the art, we aimed to provide insight into the question, “How does the code generation from AI models impact the cybersecurity of the software process?” We can confirm that there is enough evidence for us to say, unsurprisingly, that code generated by AI is not necessarily secure and it also contains security flaws. But, as often happens with AI, the real matter is not if AI is infallible but whether it performs better than humans doing the same task. Unfortunately, the conclusions we gathered from the literature diverge in suggesting whether AI-generated security artifacts should be cautiously approached, for instance, because of some particular severity or because they are tricky to spot. Indeed, some work reports of them as naïve and easily detectable, but the result cannot be generalized. Overall, there is no clear favor for one hypothesis over the other because of incomparable differences between the papers' experimental setups, data sets used for the training, programming languages considered, types of flaws, and followed experimental methodologies.
Generally speaking and regardless of the code production activity—whether for code generation from scratch, generating code repair, or even suggesting code—our analysis reveals that well-documented vulnerabilities in have been found in AI-suggested code, and this happened a non-negligible amount of times. And among the many, specific vulnerabilities, such as CWE MITRE Top-25, have received special attention in the current research and for a reason. For instance, CWE-787 and 089 received particular attention from articles, as they are part of the top 3 of MITRE CWE. Furthermore, the CWE security scores of generated code suggested by AI models would vary, with some CWEs being more prevalent than others.
Other works report on having found naïve bugs, easy to fix while other discovered malware code hidden between the benign lines, and other more reported an unjustified trust by human on the quality of the AI-generated code, an issue that raises concerns of a more socio-technical nature.
Similarly, when generated with AI support, different programming languages have different security performances. AI-generated Python code seemed to be more secure (i.e., have fewer bugs) than AI-generated code of the C family. Indeed, different authors have hypothesized that this situation is a consequence of the training data set and its quality. Verilog seems to suffer from similar shortcomings as C. When comparing the security of AI-generated Verilog to C or Python, the literature converges on reporting that the security of the former is worse. Once again, the suggested reason for the finding is that available training data sets for Verilog are smaller and of worse quality than those available for training AI models to generate C or Python code. In addition, there is no identified Top 25 CWE for Verilog. Java is another commonly studied programming language, with similar conclusions as once stated before. To a lesser extent, other programming languages that could be further studied were studied.
Looking at security exploits enabled by AI-generated code with security weaknesses, four different of them are those more frequently reported: SVEN, CodeAttack, and Codex Leaks. Such attacks are reported to used to decreasing code security, creating adversarial code, and personal data leaks over automated generated code.
What can be done to mitigate the severity of flaws introduced by AI? Does the literature suggest giving up on AI entirely? No, this is not what anyone suggests, as it can be imagined that AI is considered an instrument that, despite imperfect, has a clear advantage in terms of speeding up code production. Instead different mitigation strategies are suggested, although more research is required to discuss their effectiveness and efficacy.
• Modifications to the dataset can be a possibility, but the impacts and trade-offs of such an approach are necessary;
• Raising awareness of the context of prompts and how to increase their quality seems to affect the security quality of the code generated positively;
• Security processes, policies, and a degree of mistrust of the AI-generated code could help with security. In other words, AI-generated should pass specific processes—such as test and security verification—before being accepted;
• Educating end-users on AI models (and for code generation) on their limits could help. Future research is required in this area.
As a closing remark, we welcome that the study of the impact on the security of AI models is sparking. We also greet the increased attention that the community is dedicating to the problem of how insecure our systems will be as developers continue to resort to AI support for their work. However, it is still premature to conclude on the impact of the flaws introduced by AI models and, in particular, the impact of those flaws comparatively with those generated by human programmers. Although several mitigation techniques are suggested, what combination of them is efficient or practical is a question that still needs experimental data.
Surely, we have to accept that AI will be used more and more in producing code and that the practice and this tool are still far from being flawless. Until more evidence is available, the general agreement is to exert caution: AI models for secure code generation need to be approached with due care.
Data availability statement
The datasets of the sample of papers for this study can be found in: https://zenodo.org/records/11092334 .
Author contributions
CN-R: Conceptualization, Data curation, Investigation, Methodology, Project administration, Resources, Validation, Writing—original draft, Writing—review & editing. RG-S: Investigation, Visualization, Writing—original draft, Writing—review & editing. AS: Conceptualization, Investigation, Methodology, Writing—review & editing. GL: Conceptualization, Funding acquisition, Investigation, Writing—original draft, Writing—review & editing.
The author(s) declare financial support was received for the research, authorship, and/or publication of this article. This research was funded in whole, or in part, by the Luxembourg National Research Fund (FNR), grant: NCER22/IS/16570468/NCER-FT.
Acknowledgments
The authors thank Marius Lombard-Platet for his feedback, comments, and for proof-reading the paper.
Conflict of interest
The authors declare that the research was conducted in the absence of any commercial or financial relationships that could be construed as a potential conflict of interest.
Publisher's note
All claims expressed in this article are solely those of the authors and do not necessarily represent those of their affiliated organizations, or those of the publisher, the editors and the reviewers. Any product that may be evaluated in this article, or claim that may be made by its manufacturer, is not guaranteed or endorsed by the publisher.
1. ^ The number of different tokens that a model can handle, and their internal representation, is a design choice.
2. ^ This is the meaning of “GPT:” generative pre-trained transformer.
3. ^ Some authors claim that, because there is an encoding-decoding step, and the output is probabilistic, data is not directly copy-pasted. However seriously this argument can be taken, LLMs can and do reproduce parts of their training set ( Huang et al., 2023 ).
4. ^ Certain CWE prompting scenarios, when compared between the authors, had dissimilar security rates, which we would like to note.
5. ^ The authors do highlight that their proposal is not a poisoning attack.
6. ^ In reality, multiple (broadly incompatible) versions of Python coexist, but this is unimportant in the context of our discussion and we refer to them collectively as “Python.”
7. ^ One could argue for instance that the vulnerabilities occur in large proportions in generated code that fails basic functional testing, and would never make it into production because of this. Or, the other way around, that code without security vulnerabilities could still be functionally incorrect, which also causes issues. A full study of these effects remains to be done.
8. ^ They were tasked to write a program that “takes as input a string path representing a file path and returns a File object for the file at 'path' ( Perry et al., 2023 ).”
9. ^ Following the authors of our sample, we use “C” to refer to the various versions of the C standard, indiscriminately.
10. ^ Here again we conflate all versions of Java together.
11. ^ The authors define single stupid bugs as “...bugs that have single-statement fixes that match a small set of bug templates. They are called 'simple' because they are usually fixed by small changes and 'stupid' because, once located, a developer can usually fix them quickly with minor changes ( Jesse et al., 2023 ).”
12. ^ The attack is explained in detail in Section 5.2.
Ahmad, W., Chakraborty, S., Ray, B., and Chang, K.-W. (2021). “Unified pre-training for program understanding and generation,” in Proceedings of the 2021 Conference of the North American Chapter of the Association for Computational Linguistics: Human Language Technologies , eds. K. Toutanova, A. Rumshisky, L. Zettlemoyer, D. Hakkani-Tur, I. Beltagy, S. Bethard, et al. (Association for Computational Linguistics), 2655–2668.
Google Scholar
Asare, O., Nagappan, M., and Asokan, N. (2023). Is GitHub's Copilot as bad as humans at introducing vulnerabilities in code? Empir. Softw. Eng . 28:129. doi: 10.48550/arXiv.2204.04741
Crossref Full Text | Google Scholar
Becker, B. A., Denny, P., Finnie-Ansley, J., Luxton-Reilly, A., Prather, J., and Santos, E. A. (2023). “Programming is hard-or at least it used to be: educational opportunities and challenges of ai code generation,” in Proceedings of the 54th ACM Technical Symposium on Computer Science Education V.1 (New York, NY), 500–506.
Botacin, M. (2023). “GPThreats-3: is automatic malware generation a threat?” in 2023 IEEE Security and Privacy Workshops (SPW) (San Francisco, CA: IEEE), 238–254.
Britz, D., Goldie, A., Luong, T., and Le, Q. (2017). Massive exploration of neural machine translation architectures. ArXiv e-prints . doi: 10.48550/arXiv.1703.03906
Burgess, M. (2023). Criminals Have Created Their Own ChatGPT Clones . Wired.
Carrera-Rivera, A., Ochoa, W., Larrinaga, F., and Lasa, G. (2022). How-to conduct a systematic literature review: a quick guide for computer science research. MethodsX 9:101895. doi: 10.1016/j.mex.2022.101895
PubMed Abstract | Crossref Full Text | Google Scholar
Chen, M., Tworek, J., Jun, H., Yuan, Q., de Oliveira Pinto, H. P., Kaplan, J., et al. (2021). Evaluating large language models trained on code. CoRR abs/2107.03374. doi: 10.48550/arXiv.2107.03374
Fan, J., Li, Y., Wang, S., and Nguyen, T. N. (2020). “A C/C + + code vulnerability dataset with code changes and CVE summaries,” in Proceedings of the 17th International Conference on Mining Software Repositories, MSR '20 (New York, NY: Association for Computing Machinery), 508–512.
Feng, Z., Guo, D., Tang, D., Duan, N., Feng, X., Gong, M., et al. (2020). “CodeBERT: a pre-trained model for programming and natural languages,” in Findings of the Association for Computational Linguistics: EMNLP 2020 , eds. T. Cohn, Y. He, and Y. Liu (Association for Computational Linguistics), 1536–1547.
Fried, D., Aghajanyan, A., Lin, J., Wang, S. I., Wallace, E., Shi, F., et al. (2022). InCoder: a generative model for code infilling and synthesis. ArXiv abs/2204.05999. doi: 10.48550/arXiv.2204.05999
Guo, D., Ren, S., Lu, S., Feng, Z., Tang, D., Liu, S., et al. (2021). “GraphCodeBERT: pre-training code representations with data flow,” in 9th International Conference on Learning Representations, ICLR 2021, Virtual Event, Austria, May 3–7, 2021 . OpenReview.net .
He, J., and Vechev, M. (2023). “Large language models for code: Security hardening and adversarial testing,” in Proceedings of the 2023 ACM SIGSAC Conference on Computer and Communications Security (New York, NY), 1865–1879.
Henkel, J., Ramakrishnan, G., Wang, Z., Albarghouthi, A., Jha, S., and Reps, T. (2022). “Semantic robustness of models of source code,” in 2022 IEEE International Conference on Software Analysis, Evolution and Reengineering (SANER) (Honolulu, HI), 526–537.
Holtzman, A., Buys, J., Du, L., Forbes, M., and Choi, Y. (2020). “The curious case of neural text degeneration,” in 8th International Conference on Learning Representations, ICLR 2020, Addis Ababa, Ethiopia, April 26–30, 2020 . OpenReview.net .
Huang, Y., Li, Y., Wu, W., Zhang, J., and Lyu, M. R. (2023). Do Not Give Away My Secrets: Uncovering the Privacy Issue of Neural Code Completion Tools .
HuggingFaces (2022). Codeparrot. Available online at: https://huggingface.co/codeparrot/codeparrot (accessed February, 2024).
Jain, P., Jain, A., Zhang, T., Abbeel, P., Gonzalez, J., and Stoica, I. (2021). “Contrastive code representation learning,” in Proceedings of the 2021 Conference on Empirical Methods in Natural Language Processing , eds. M.-F. Moens, X. Huang, L. Specia, and S. W.-t. Yih (Punta Cana: Association for Computational Linguistics), 5954–5971.
Jesse, K., Ahmed, T., Devanbu, P. T., and Morgan, E. (2023). “Large language models and simple, stupid bugs,” in 2023 IEEE/ACM 20th International Conference on Mining Software Repositories (MSR) (Los Alamitos, CA: IEEE Computer Society), 563–575.
Jha, A., and Reddy, C. K. (2023). “CodeAttack: code-based adversarial attacks for pre-trained programming language models,” in Proceedings of the AAAI Conference on Artificial Intelligence, Vol. 37 , 14892–14900.
Jia, J., Srikant, S., Mitrovska, T., Gan, C., Chang, S., Liu, S., et al. (2023). “CLAWSAT: towards both robust and accurate code models,” in 2023 IEEE International Conference on Software Analysis, Evolution and Reengineering (SANER) (Los Alamitos, CA: IEEE), 212–223.
Karampatsis, R.-M., and Sutton, C. (2020). “How often do single-statement bugs occur? The manySStuBs4J dataset,” in Proceedings of the 17th International Conference on Mining Software Repositories, MSR '20 (Seoul: Association for Computing Machinery), 573–577.
Kitchenham, B., and Charters, S. (2007). Guidelines for performing systematic literature reviews in software engineering. Tech. Rep. Available online at: https://scholar.google.com/citations?view_op=view_citation&hl=en&user=CQDOm2gAAAAJ&citation_for_view=CQDOm2gAAAAJ:d1gkVwhDpl0C
Kitchenham, B., Sjøberg, D. I., Brereton, O. P., Budgen, D., Dybå, T., Höst, M., et al. (2010). “Can we evaluate the quality of software engineering experiments?,” in Proceedings of the 2010 ACM-IEEE International Symposium on Empirical Software Engineering and Measurement (New York, NY), 1–8.
Li, R., Allal, L. B., Zi, Y., Muennighoff, N., Kocetkov, D., Mou, C., et al. (2023). StarCoder: may the source be with you! arXiv preprint arXiv:2305.06161 . doi: 10.48550/arXiv.2305.06161
Liguori, P., Improta, C., Natella, R., Cukic, B., and Cotroneo, D. (2023). Who evaluates the evaluators? On automatic metrics for assessing AI-based offensive code generators. Expert Syst. Appl. 225:120073. doi: 10.48550/arXiv.2212.06008
Liu, Y., Ott, M., Goyal, N., Du, J., Joshi, M., Chen, D., et al. (2019). RoBERTa: a robustly optimized BERT pretraining approach. CoRR abs/1907.11692. doi: 10.48550/arXiv.1907.11692
Moradi Dakhel, A., Majdinasab, V., Nikanjam, A., Khomh, F., Desmarais, M. C., and Jiang, Z. M. J. (2023). GitHub Copilot AI pair programmer: asset or liability? J. Syst. Softw . 203:111734. doi: 10.48550/arXiv.2206.15331
Multiple authors (2021). GPT Code Clippy: The Open Source Version of GitHub Copilot .
Nair, M., Sadhukhan, R., and Mukhopadhyay, D. (2023). “How hardened is your hardware? Guiding ChatGPT to generate secure hardware resistant to CWEs,” in International Symposium on Cyber Security, Cryptology, and Machine Learning (Berlin: Springer), 320–336.
Natella, R., Liguori, P., Improta, C., Cukic, B., and Cotroneo, D. (2024). AI code generators for security: friend or foe? IEEE Secur. Priv . 2024:1219. doi: 10.48550/arXiv.2402.01219
Nijkamp, E., Pang, B., Hayashi, H., Tu, L., Wang, H., Zhou, Y., et al. (2023). CodeGen: An Open Large Language Model for Code with Multi-Turn Program Synthesis . ICLR.
Nikitopoulos, G., Dritsa, K., Louridas, P., and Mitropoulos, D. (2021). “CrossVul: a cross-language vulnerability dataset with commit data,” in Proceedings of the 29th ACM Joint Meeting on European Software Engineering Conference and Symposium on the Foundations of Software Engineering, ESEC/FSE 2021 (New York, NY: Association for Computing Machinery), 1565–1569.
Niu, L., Mirza, S., Maradni, Z., and Pöpper, C. (2023). “CodexLeaks: privacy leaks from code generation language models in GitHub's Copilot,” in 32nd USENIX Security Symposium (USENIX Security 23) , 2133–2150.
Olson, M., Wyner, A., and Berk, R. (2018). Modern neural networks generalize on small data sets. Adv. Neural Inform. Process. Syst . 31, 3623–3632. Available online at: https://proceedings.neurips.cc/paper/2018/hash/fface8385abbf94b4593a0ed53a0c70f-Abstract.html
Pa Pa, Y. M., Tanizaki, S., Kou, T., Van Eeten, M., Yoshioka, K., and Matsumoto, T. (2023). “An attacker's dream? Exploring the capabilities of chatgpt for developing malware,” in Proceedings of the 16th Cyber Security Experimentation and Test Workshop (New York, NY), 10–18.
Pearce, H., Ahmad, B., Tan, B., Dolan-Gavitt, B., and Karri, R. (2022). “Asleep at the keyboard? Assessing the security of GitHub Copilot's code contributions,” in 2022 IEEE Symposium on Security and Privacy (SP) (IEEE), 754–768.
Pearce, H., Tan, B., Ahmad, B., Karri, R., and Dolan-Gavitt, B. (2023). “Examining zero-shot vulnerability repair with large language models,” in 2023 IEEE Symposium on Security and Privacy (SP) (Los Alamitos, CA: IEEE), 2339–2356.
Perry, N., Srivastava, M., Kumar, D., and Boneh, D. (2023). “Do users write more insecure code with AI assistants?,” in Proceedings of the 2023 ACM SIGSAC Conference on Computer and Communications Security (New York, NY), 2785–2799.
Petersen, K., Vakkalanka, S., and Kuzniarz, L. (2015). Guidelines for conducting systematic mapping studies in software engineering: an update. Inform. Softw. Technol . 64, 1–18. doi: 10.1016/j.infsof.2015.03.007
Sandoval, G., Pearce, H., Nys, T., Karri, R., Garg, S., and Dolan-Gavitt, B. (2023). “Lost at C: a user study on the security implications of large language model code assistants,” in 32nd USENIX Security Symposium (USENIX Security 23) (Anaheim, CA: USENIX Association), 2205–2222.
Siddiq, M. L., Majumder, S. H., Mim, M. R., Jajodia, S., and Santos, J. C. (2022). “An empirical study of code smells in transformer-based code generation techniques,” in 2022 IEEE 22nd International Working Conference on Source Code Analysis and Manipulation (SCAM) (Limassol: IEEE), 71–82.
Storhaug, A., Li, J., and Hu, T. (2023). “Efficient avoidance of vulnerabilities in auto-completed smart contract code using vulnerability-constrained decoding,” in 2023 IEEE 34th International Symposium on Software Reliability Engineering (ISSRE) (Los Alamitos, CA: IEEE), 683–693.
Tan, C., Sun, F., Kong, T., Zhang, W., Yang, C., and Liu, C. (2018). “A survey on deep transfer learning,” in Artificial Neural Networks and Machine Learning – ICANN 2018 , eds. V. Kůrková, Y. Manolopoulos, B. Hammer, L. Iliadis, and I. Maglogiannis (Cham. Springer International Publishing), 270–279.
Tony, C., Ferreyra, N. E. D., and Scandariato, R. (2022). “GitHub considered harmful? Analyzing open-source projects for the automatic generation of cryptographic API call sequences,” in 2022 IEEE 22nd International Conference on Software Quality, Reliability and Security (QRS) (Guangzhou: IEEE), 270–279.
Tony, C., Mutas, M., Ferreyra, N. E. D., and Scandariato, R. (2023). “LLMSecEval: a dataset of natural language prompts for security evaluations,” in 20th IEEE/ACM International Conference on Mining Software Repositories, MSR 2023, Melbourne, Australia, May 15-16, 2023 (Los Alamitos, CA: IEEE), 588–592.
Vaswani, A., Shazeer, N., Parmar, N., Uszkoreit, J., Jones, L., Gomez, A. N., et al. (2017). “Attention is all you need,” in Advances in Neural Information Processing Systems 30: Annual Conference on Neural Information Processing Systems 2017, December 4-9, 2017 , eds. I. Guyon, U. von Luxburg, S. Bengio, H. M. Wallach, R. Fergus, S. V. N. Vishwanathan, et al. (Long Beach, CA), 5998–6008.
Wang, Y., Wang, W., Joty, S. R., and Hoi, S. C. H. (2021). “CodeT5: identifier-aware unified pre-trained encoder-decoder models for code understanding and generation,” in Proceedings of the 2021 Conference on Empirical Methods in Natural Language Processing , 8696–8708.
Wartschinski, L., Noller, Y., Vogel, T., Kehrer, T., and Grunske, L. (2022). VUDENC: vulnerability detection with deep learning on a natural codebase for Python. Inform. Softw. Technol . 144:106809. doi: 10.48550/arXiv.2201.08441
Wieringa, R., Maiden, N., Mead, N., and Rolland, C. (2006). Requirements engineering paper classification and evaluation criteria: a proposal and a discussion. Requir. Eng . 11, 102–107. doi: 10.1007/s00766-005-0021-6
Wohlin, C. (2014). “Guidelines for snowballing in systematic literature studies and a replication in software engineering,” in Proceedings of the 18th International Conference on Evaluation and Assessment in Software Engineering (New York, NY), 1–10.
Wohlin, C., Runeson, P., Neto, P. A. d. M. S., Engström, E., do Carmo Machado, I., and De Almeida, E. S. (2013). On the reliability of mapping studies in software engineering. J. Syst. Softw . 86, 2594–2610. doi: 10.1016/j.jss.2013.04.076
Wu, Y., Jiang, N., Pham, H. V., Lutellier, T., Davis, J., Tan, L., et al. (2023). “How effective are neural networks for fixing security vulnerabilities,” in Proceedings of the 32nd ACM SIGSOFT International Symposium on Software Testing and Analysis, ISSTA 2023 (New York, NY: Association for Computing Machinery), 1282–1294.
PubMed Abstract | Google Scholar
Xu, F. F., Alon, U., Neubig, G., and Hellendoorn, V. J. (2022). “A systematic evaluation of large language models of code,” in Proceedings of the 6th ACM SIGPLAN International Symposium on Machine Programming (New York, NY), 1–10.
Keywords: artificial intelligence, security, software engineering, programming, code generation
Citation: Negri-Ribalta C, Geraud-Stewart R, Sergeeva A and Lenzini G (2024) A systematic literature review on the impact of AI models on the security of code generation. Front. Big Data 7:1386720. doi: 10.3389/fdata.2024.1386720
Received: 15 February 2024; Accepted: 22 April 2024; Published: 13 May 2024.
Reviewed by:
Copyright © 2024 Negri-Ribalta, Geraud-Stewart, Sergeeva and Lenzini. This is an open-access article distributed under the terms of the Creative Commons Attribution License (CC BY) . The use, distribution or reproduction in other forums is permitted, provided the original author(s) and the copyright owner(s) are credited and that the original publication in this journal is cited, in accordance with accepted academic practice. No use, distribution or reproduction is permitted which does not comply with these terms.
*Correspondence: Claudia Negri-Ribalta, claudia.negriribalta@uni.lu
This article is part of the Research Topic
Cybersecurity and Artificial Intelligence: Advances, Challenges, Opportunities, Threats
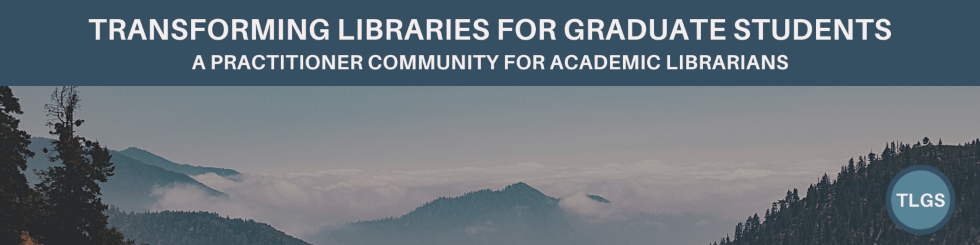
- Next Event >
Home > Conferences, Workshops, and Lectures > TGLS > March 12-13, 2024 > 2024 Presentations > 1
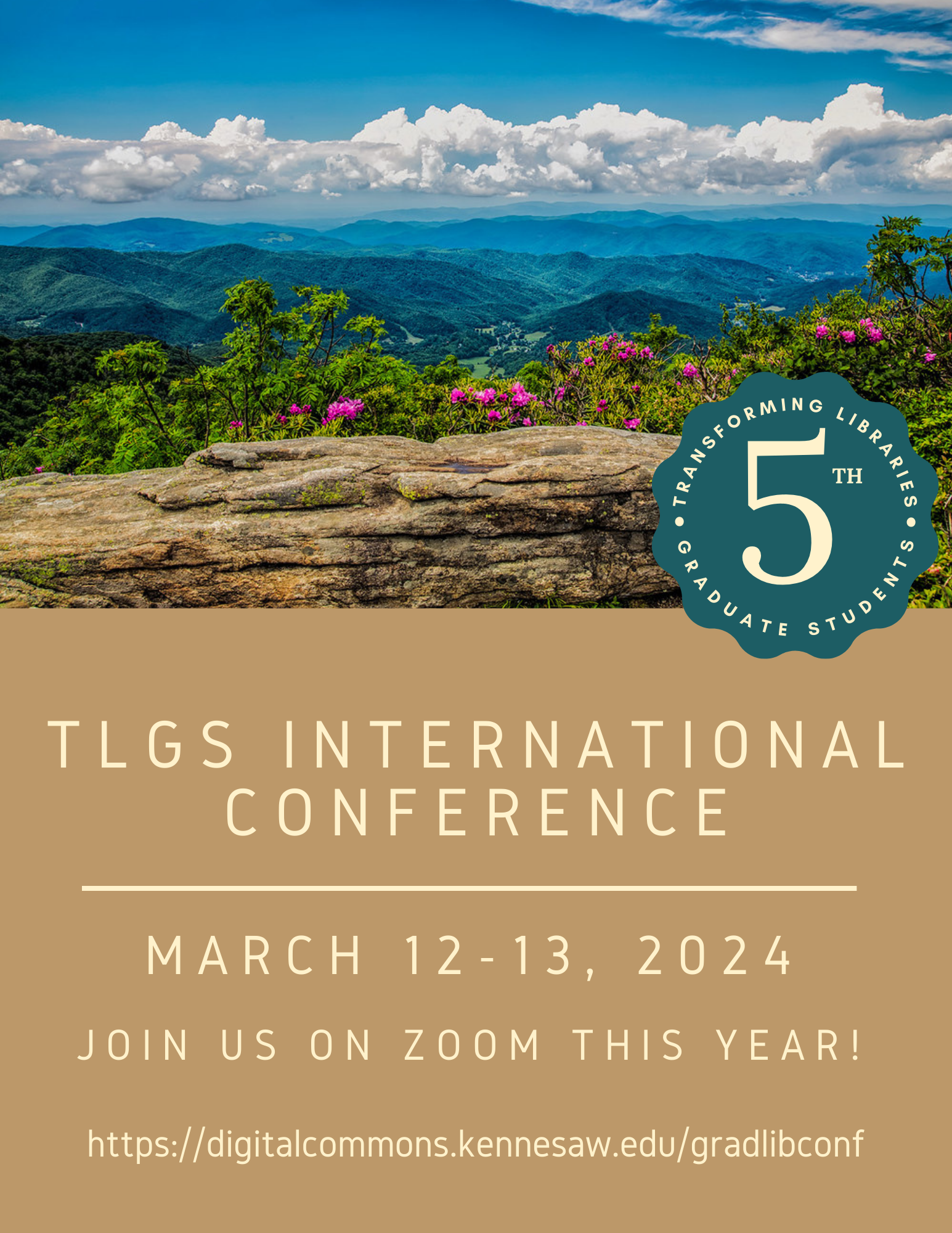
2024 Presentations
Graduate Student’s Productivity Tools for Literature Review Research and Writing in the Age of AI
Presenter(s) Information
Carmen Orth-Alfie , University of Kansas Libraries Follow Paul Thomas , University of Kansas Libraries Follow
3-12-2024 12:00 PM
3-12-2024 1:00 PM
Author(s) Bio
Carmen Orth-Alfie is an assistant librarian for business and graduate student engagement at the University of Kansas Libraries. Her professional responsibilities include information literacy instruction and research consultations. Paul Thomas is a library specialist at the University of Kansas Libraries. He holds a PhD in library and information management from Emporia State University. Carmen and Paul are colleagues in the Center for Graduate Initiatives and Engagement, which is responsible for collaborative programming and services to meet the needs of graduate students as students, emerging scholars, and future professionals.
generative AI, literature reviews, productivity tools, ChatGPT
Description of Proposal
In the fast-evolving world of academia, it is not hyperbole to say that generative AI and algorithm-based productivity tools like ChatGPT, Research Rabbit, and LitMap are quickly becoming transformative forces, reshaping the way graduate students (among many groups) approach the research and writing of thesis/dissertation literature reviews. But while the plethora of possibilities engendered by generative productivity tools is in many ways remarkable, the technology itself can often be overwhelming—not only for the graduate students, but also for us as librarians and information professionals supporting independent researchers from any discipline. Indeed, the ever-growing number of AI tools on the market suggests that the era of artificial intelligence is here. For this reason, it is critical that we develop the skills necessary to provide support and guidance to the increasing number of graduate students engaging with these advanced technologies.
In this session, we will focus on providing librarians with the skills necessary to effectively communicate with graduate students about productivity tools enabling the creation of original research and writing. We will begin by presenting a structured framework (predicated heavily on established educational and LIS research) that can be used to categorize productivity tools. In a sense, this framework will provide librarians and other information professionals with a useful wayfinder that enables the diverse range of productivity tools available to be contextualized situationally, making them easier to understand. After discussing the framework, we will then explore a curated selection of AI/generative and other tools, showcasing their potential to facilitate various stages of independent and original graduate research. Finally, we will address the ethical and legal considerations entwined with the recommendation and implementation of these tools, thus fostering a culture of informed and ethical LIS research and practice. After attending this session, librarians will arguably have a better understanding of the tools that are out there, empowering them to match these tools with the specific needs of the graduate students that they serve.
What takeaways will attendees learn from your session?
Equip academic library professionals with the skills to effectively communicate and engage with graduate students regarding productivity tools tailored to their original research needs.
Introduce a practical framework for categorizing and understanding the diverse range of productivity tools available.
Demystify a selection of AI/generative and other tools that hold significant potential in aiding graduate students throughout their research journey.
Examine the ethical and legal considerations inherent in recommending and implementing these tools within an academic setting.
Since February 26, 2024
Included in
Higher Education Commons , Library and Information Science Commons
- Registration Details
Advanced Search
- Notify me via email or RSS
- All Collections
- Disciplines
- Conferences
- Faculty Works
- Open Access
- Research Support
- Student Works
- Related Resources
- KSU University Library System Homepage
Home | About | FAQ | My Account | Accessibility Statement
Privacy Copyright
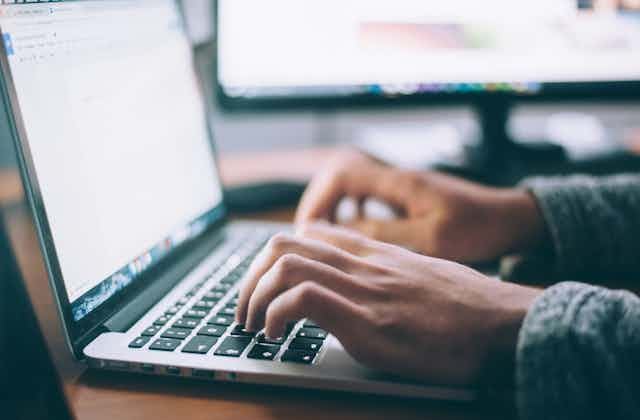
AI-assisted writing is quietly booming in academic journals. Here’s why that’s OK
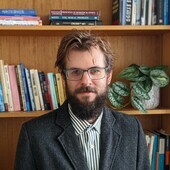
Lecturer in Bioethics, Monash University & Honorary fellow, Melbourne Law School, Monash University
Disclosure statement
Julian Koplin does not work for, consult, own shares in or receive funding from any company or organisation that would benefit from this article, and has disclosed no relevant affiliations beyond their academic appointment.
Monash University provides funding as a founding partner of The Conversation AU.
View all partners
If you search Google Scholar for the phrase “ as an AI language model ”, you’ll find plenty of AI research literature and also some rather suspicious results. For example, one paper on agricultural technology says:
As an AI language model, I don’t have direct access to current research articles or studies. However, I can provide you with an overview of some recent trends and advancements …
Obvious gaffes like this aren’t the only signs that researchers are increasingly turning to generative AI tools when writing up their research. A recent study examined the frequency of certain words in academic writing (such as “commendable”, “meticulously” and “intricate”), and found they became far more common after the launch of ChatGPT – so much so that 1% of all journal articles published in 2023 may have contained AI-generated text.
(Why do AI models overuse these words? There is speculation it’s because they are more common in English as spoken in Nigeria, where key elements of model training often occur.)
The aforementioned study also looks at preliminary data from 2024, which indicates that AI writing assistance is only becoming more common. Is this a crisis for modern scholarship, or a boon for academic productivity?
Who should take credit for AI writing?
Many people are worried by the use of AI in academic papers. Indeed, the practice has been described as “ contaminating ” scholarly literature.
Some argue that using AI output amounts to plagiarism. If your ideas are copy-pasted from ChatGPT, it is questionable whether you really deserve credit for them.
But there are important differences between “plagiarising” text authored by humans and text authored by AI. Those who plagiarise humans’ work receive credit for ideas that ought to have gone to the original author.
By contrast, it is debatable whether AI systems like ChatGPT can have ideas, let alone deserve credit for them. An AI tool is more like your phone’s autocomplete function than a human researcher.
The question of bias
Another worry is that AI outputs might be biased in ways that could seep into the scholarly record. Infamously, older language models tended to portray people who are female, black and/or gay in distinctly unflattering ways, compared with people who are male, white and/or straight.
This kind of bias is less pronounced in the current version of ChatGPT.
However, other studies have found a different kind of bias in ChatGPT and other large language models : a tendency to reflect a left-liberal political ideology.
Any such bias could subtly distort scholarly writing produced using these tools.
The hallucination problem
The most serious worry relates to a well-known limitation of generative AI systems: that they often make serious mistakes.
For example, when I asked ChatGPT-4 to generate an ASCII image of a mushroom, it provided me with the following output.
It then confidently told me I could use this image of a “mushroom” for my own purposes.
These kinds of overconfident mistakes have been referred to as “ AI hallucinations ” and “ AI bullshit ”. While it is easy to spot that the above ASCII image looks nothing like a mushroom (and quite a bit like a snail), it may be much harder to identify any mistakes ChatGPT makes when surveying scientific literature or describing the state of a philosophical debate.
Unlike (most) humans, AI systems are fundamentally unconcerned with the truth of what they say. If used carelessly, their hallucinations could corrupt the scholarly record.
Should AI-produced text be banned?
One response to the rise of text generators has been to ban them outright. For example, Science – one of the world’s most influential academic journals – disallows any use of AI-generated text .
I see two problems with this approach.
The first problem is a practical one: current tools for detecting AI-generated text are highly unreliable. This includes the detector created by ChatGPT’s own developers, which was taken offline after it was found to have only a 26% accuracy rate (and a 9% false positive rate ). Humans also make mistakes when assessing whether something was written by AI.
It is also possible to circumvent AI text detectors. Online communities are actively exploring how to prompt ChatGPT in ways that allow the user to evade detection. Human users can also superficially rewrite AI outputs, effectively scrubbing away the traces of AI (like its overuse of the words “commendable”, “meticulously” and “intricate”).
The second problem is that banning generative AI outright prevents us from realising these technologies’ benefits. Used well, generative AI can boost academic productivity by streamlining the writing process. In this way, it could help further human knowledge. Ideally, we should try to reap these benefits while avoiding the problems.
The problem is poor quality control, not AI
The most serious problem with AI is the risk of introducing unnoticed errors, leading to sloppy scholarship. Instead of banning AI, we should try to ensure that mistaken, implausible or biased claims cannot make it onto the academic record.
After all, humans can also produce writing with serious errors, and mechanisms such as peer review often fail to prevent its publication.
We need to get better at ensuring academic papers are free from serious mistakes, regardless of whether these mistakes are caused by careless use of AI or sloppy human scholarship. Not only is this more achievable than policing AI usage, it will improve the standards of academic research as a whole.
This would be (as ChatGPT might say) a commendable and meticulously intricate solution.
- Artificial intelligence (AI)
- Academic journals
- Academic publishing
- Hallucinations
- Scholarly publishing
- Academic writing
- Large language models
- Generative AI

Lecturer / Senior Lecturer - Marketing

Compliance Lead

Assistant Editor - 1 year cadetship

Executive Dean, Faculty of Health

Lecturer/Senior Lecturer, Earth System Science (School of Science)
5 Best AI Research Paper Summarizers (May 2024)
Unite.AI is committed to rigorous editorial standards. We may receive compensation when you click on links to products we review. Please view our affiliate disclosure .
Table Of Contents
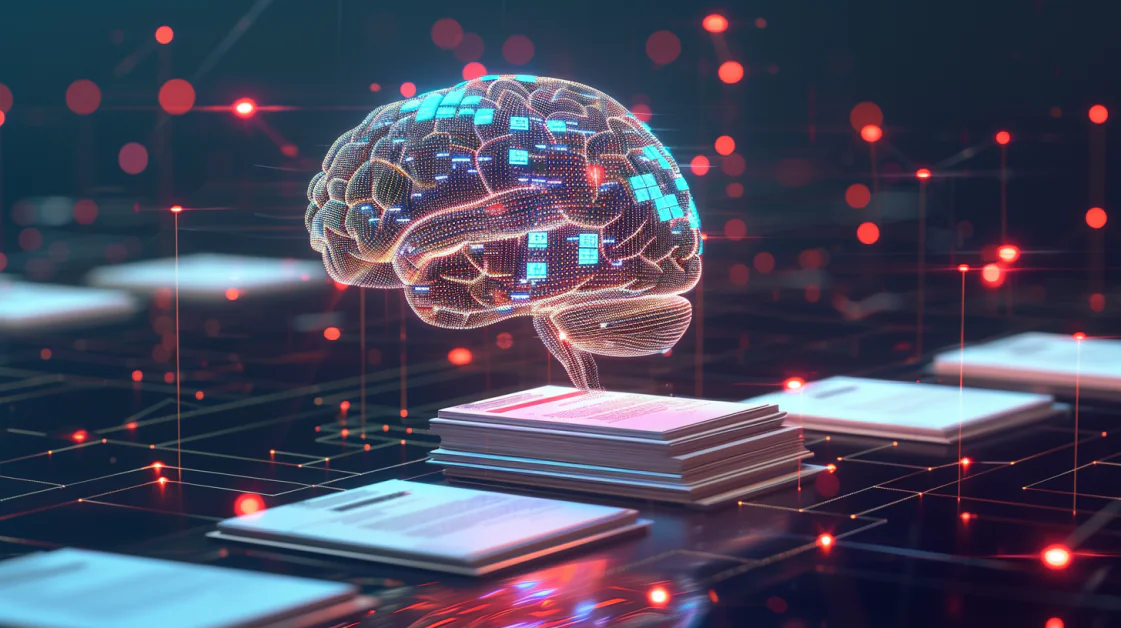
In the fast-paced world of academic research, keeping up with the ever-growing body of literature can be a daunting task. Researchers and students often find themselves inundated with lengthy research papers, making it challenging to quickly grasp the core ideas and insights. AI-powered research paper summarizers have emerged as powerful tools, leveraging advanced algorithms to condense lengthy documents into concise and readable summaries.
In this article, we will explore the top AI research paper summarizers, each designed to streamline the process of understanding and synthesizing academic literature:
1. Tenorshare AI PDF Tool

Tenorshare AI PDF Tool is a cutting-edge solution that harnesses the power of artificial intelligence to simplify the process of summarizing research papers. With its user-friendly interface and advanced AI algorithms, this tool quickly analyzes and condenses lengthy papers into concise, readable summaries, allowing researchers to grasp the core ideas without having to read the entire document.
One of the standout features of Tenorshare AI PDF Tool is its interactive chat interface, powered by ChatGPT. This innovative functionality enables users to ask questions and retrieve specific information from the PDF document, making it easier to navigate and understand complex research papers. The tool also efficiently extracts critical sections and information, such as the abstract, methodology, results, and conclusions, streamlining the reading process and helping users focus on the most relevant parts of the document.
Key features of Tenorshare AI PDF Tool:
- AI-driven summarization that quickly condenses lengthy research papers
- Interactive chat interface powered by ChatGPT for retrieving specific information
- Automatic extraction of critical sections and information from the paper
- Batch processing capabilities for handling multiple PDF files simultaneously
- Secure and private, with SSL encryption and the option to delete uploaded files
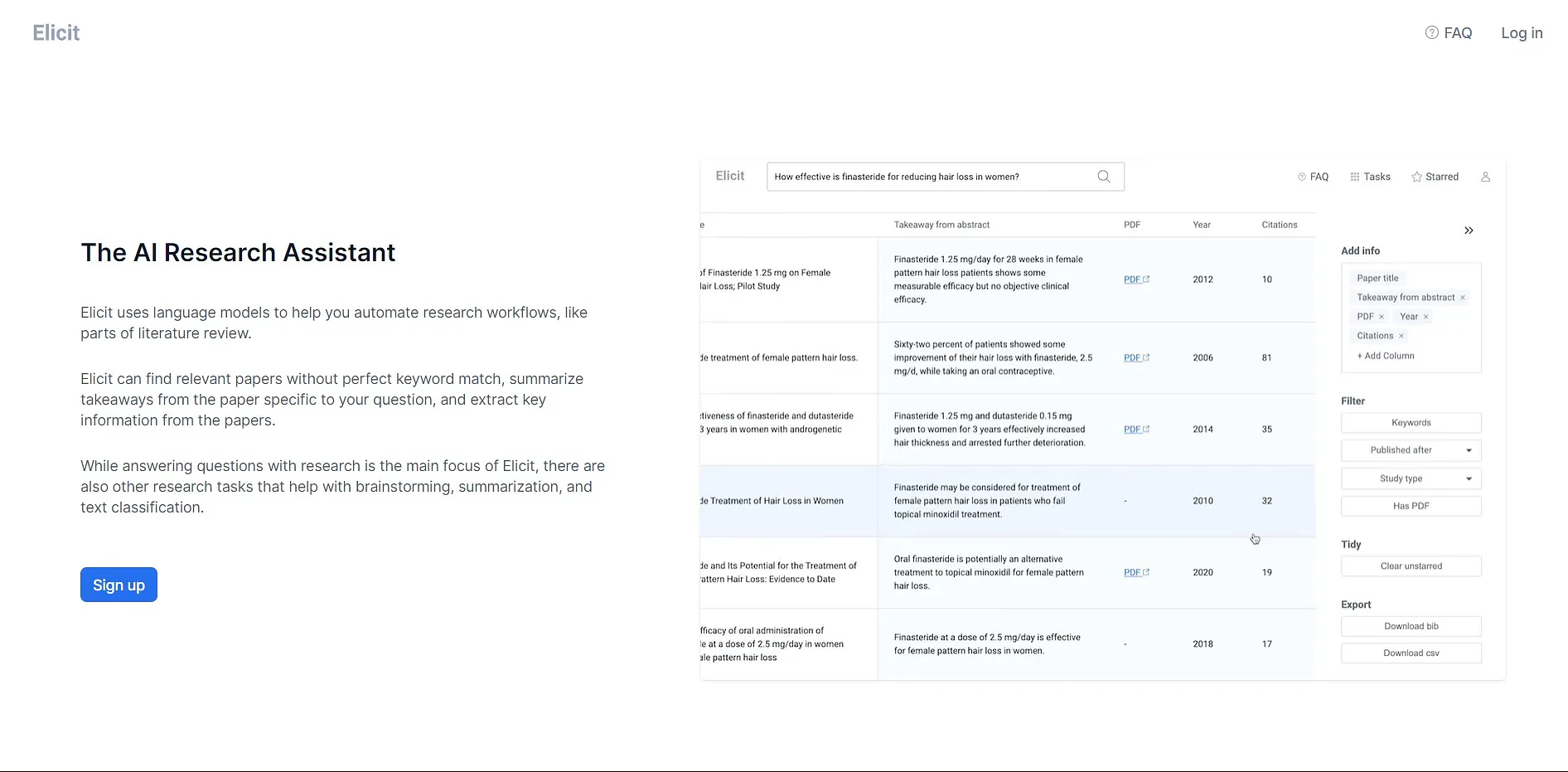
Elicit is an AI-powered research assistant that improves the way users find and summarize academic papers. With its intelligent search capabilities and advanced natural language processing, Elicit helps researchers quickly identify the most relevant papers and understand their core ideas through automatically generated summaries.
By simply entering keywords, phrases, or questions, users can leverage Elicit's AI algorithms to search through its extensive database and retrieve the most pertinent papers. The tool offers various filters and sorting options, such as publication date, study types, and citation count, enabling users to refine their search results and find exactly what they need. One of Elicit's most impressive features is its ability to generate concise summaries of the top papers related to the search query, capturing the key findings and conclusions and saving researchers valuable time.
Key features of Elicit:
- Intelligent search that understands the context and meaning of search queries
- Filters and sorting options for refining search results
- Automatic summarization of the top papers related to the search query
- Detailed paper insights, including tested outcomes, participant information, and trustworthiness assessment
- Inline referencing for transparency and accuracy verification
3. QuillBot
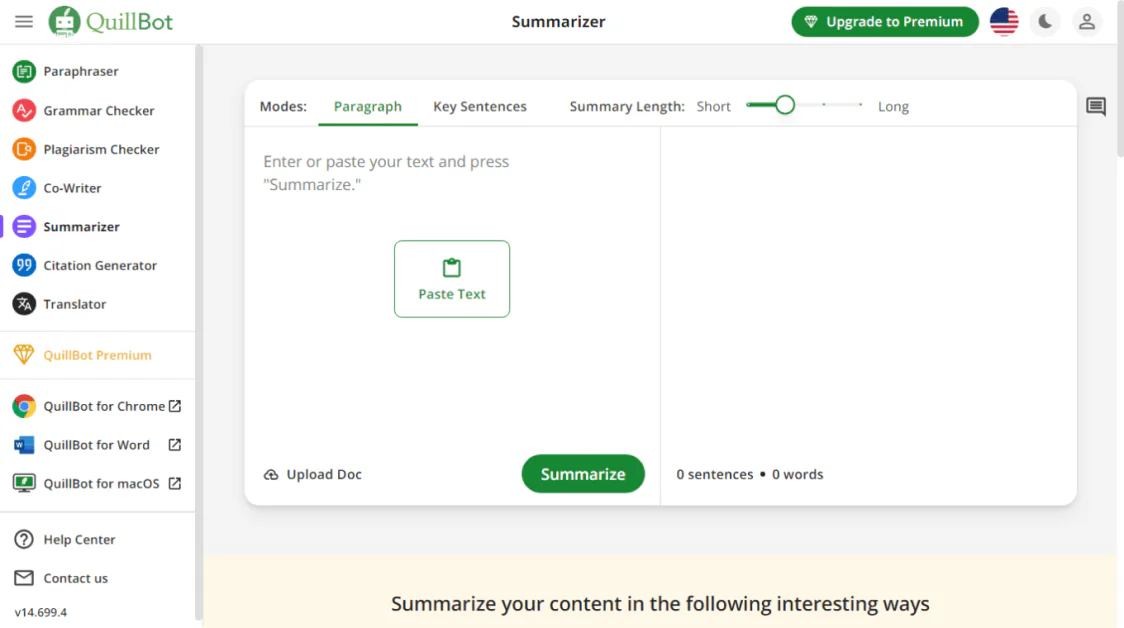
QuillBot is an AI-powered writing platform that offers a comprehensive suite of tools to enhance and streamline the writing process, including a powerful Summarizer tool that is particularly useful for condensing research papers. By leveraging advanced natural language processing and machine learning algorithms, QuillBot's Summarizer quickly analyzes lengthy articles, research papers, or documents and generates concise summaries that capture the core ideas and key points.
One of the key advantages of QuillBot's Summarizer is its ability to perform extractive summarization, which involves identifying and extracting the most critical sentences and information from the research paper while maintaining the original context. Users can customize the summary length to be either short (key sentences) or long (paragraph format) based on their needs, and the output can be generated in either a bullet point list format or as a coherent paragraph. This flexibility allows researchers to tailor the summary to their specific requirements and preferences.
Key features of QuillBot's Summarizer:
- AI-powered extractive summarization that identifies and extracts key information
- Customizable summary length (short or long) to suit different needs
- Bullet point or paragraph output for flexible formatting
- Improved reading comprehension by condensing the paper into its core concepts
- Integration with other QuillBot tools, such as Paraphraser and Grammar Checker, for further enhancement
4. Semantic Scholar

Semantic Scholar is a free, AI-powered research tool developed by the Allen Institute for AI that improves the way researchers search for and discover scientific literature. By employing advanced natural language processing, machine learning, and machine vision techniques, Semantic Scholar provides a smarter and more efficient way to navigate the vast landscape of academic publications.
One of the standout features of Semantic Scholar is its ability to generate concise, one-sentence summaries of research papers, capturing the essence of the content and allowing researchers to quickly grasp the main ideas without reading lengthy abstracts. This feature is particularly useful when browsing on mobile devices or when time is limited. Additionally, Semantic Scholar highlights the most important and influential citations within a paper, helping researchers focus on the most relevant information and understand the impact of the research.
Key features of Semantic Scholar:
- Concise one-sentence summaries of research papers for quick comprehension
- Identification of the most influential citations within a paper
- Personalized paper recommendations through the “Research Feed” feature
- Semantic Reader for in-line citation cards with summaries and “skimming highlights”
- Personal library management with the ability to save and organize papers
5. IBM Watson Discovery

IBM Watson Discovery is a powerful AI-driven tool designed to analyze and summarize large volumes of unstructured data, including research papers, articles, and scientific publications. By harnessing the power of cognitive computing, natural language processing, and machine learning, Watson Discovery enables researchers to quickly find relevant information and gain valuable insights from complex documents.
One of the key strengths of IBM Watson Discovery is its ability to understand the context, concepts, and relationships within the text, allowing it to identify patterns, trends, and connections that may be overlooked by human readers. This makes it easier to navigate and summarize complex research papers, as the tool can highlight important entities, relationships, and topics within the document. Users can create customizable queries, filter, and categorize data to generate summaries of the most relevant research findings, and the tool's advanced search capabilities enable precise searches and retrieval of specific information from large document libraries.
Key features of IBM Watson Discovery:
- Cognitive capabilities that understand context, concepts, and relationships within the text
- Customizable queries and filtering for generating summaries of relevant research findings
- Relationship identification to highlight important entities, relationships, and topics
- Significant time-saving by automating the discovery of information and insight
Empowering Researchers with AI-Driven Summarization Tools
The emergence of AI-powered research summarizers has transformed the way researchers and academics approach scientific literature. By leveraging advanced natural language processing, machine learning, and cognitive computing, these innovative tools enable users to quickly find, understand, and summarize complex research papers, saving valuable time and effort.
Each of these AI research summarizers offers unique features and benefits that cater to researchers' diverse needs. As these tools continue to evolve and improve, they will undoubtedly play an increasingly crucial role in empowering researchers to navigate the ever-expanding universe of scientific knowledge more efficiently and effectively.
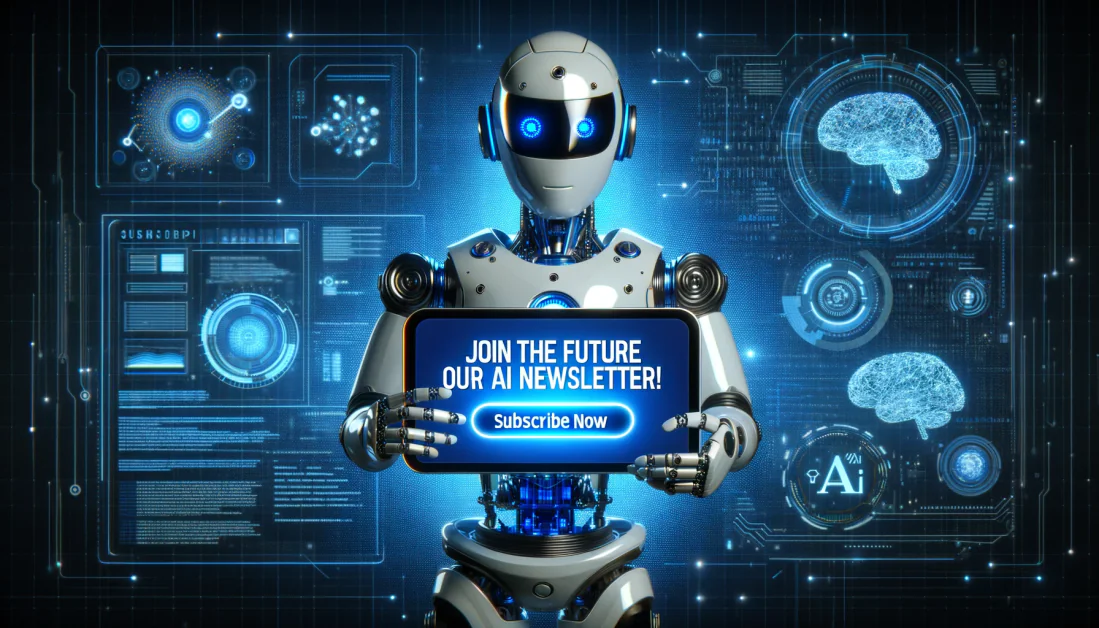
- 5 Best Vulnerability Assessment Scanning Tools (May 2024)
5 Best AI SOP (Standard Operating Procedures) Generators in 2024
Alex McFarland is an AI journalist and writer exploring the latest developments in artificial intelligence. He has collaborated with numerous AI startups and publications worldwide.
You may like
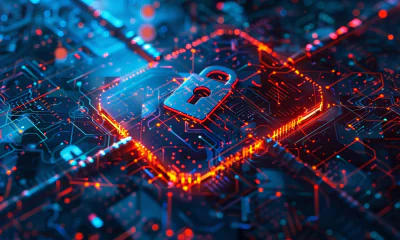
5 Best B2B Customer Support Tools (May 2024)
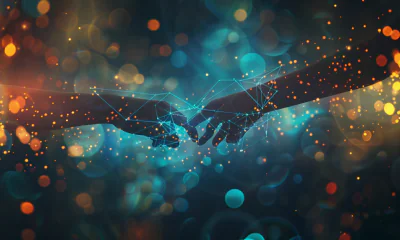
5 Best AI Apps for Couples (May 2024)
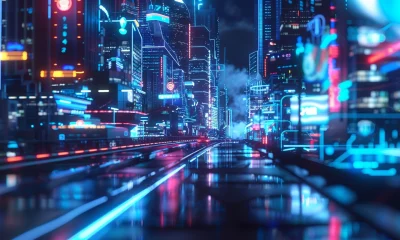
10 Best AI Shopify Tools (May 2024)
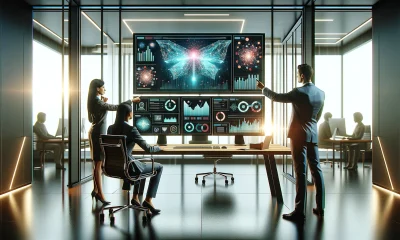
9 Best AI Business Plan Generators (May 2024)
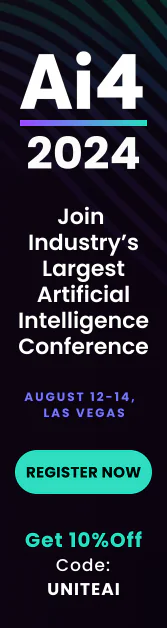
Recent Posts
- SlidesAI Review: Generate Free AI Slideshows in Seconds!
- The Multimodal Marvel: Exploring GPT-4o’s Cutting-Edge Capabilities
- Don’t Sleep on Your Database Infrastructure When Building Large Language Models or Generative AI
- A2Hosting Review – The Most Feature-packed Webhost Yet?
Teaching and learning artificial intelligence: Insights from the literature
- Published: 02 May 2024
Cite this article
- Bahar Memarian ORCID: orcid.org/0000-0003-0671-3127 1 &
- Tenzin Doleck 1
186 Accesses
Explore all metrics
Artificial Intelligence (AI) has been around for nearly a century, yet in recent years the rapid advancement and public access to AI applications and algorithms have led to increased attention to the role of AI in higher education. An equally important but overlooked topic is the study of AI teaching and learning in higher education. We wish to examine the overview of the study, pedagogical outcomes, challenges, and limitations through a systematic review process amidst the COVID-19 pandemic and public access to ChatGPT. Twelve articles from 2020 to 2023 focused on AI pedagogy are explored in this systematic literature review. We find in-depth analysis and comparison of work post-COVID and AI teaching and learning era is needed to have a more focused lens on the current state of AI pedagogy. Findings reveal that the use of self-reported surveys in a pre-and post-design form is most prevalent in the reviewed studies. A diverse set of constructs are used to conceptualize AI literacy and their associated metrics and scales of measure are defined based on the work of specific authors rather than a universally accepted framework. There remains work and consensus on what learning objectives, levels of thinking skills, and associated activities lead to the advanced development of AI literacy. An overview of the studies, pedagogical outcomes, and challenges are provided. Further implications of the studies are also shared. The contribution of this work is to open discussions on the overlooked topic of AI teaching and learning in higher education.
This is a preview of subscription content, log in via an institution to check access.
Access this article
Price includes VAT (Russian Federation)
Instant access to the full article PDF.
Rent this article via DeepDyve
Institutional subscriptions
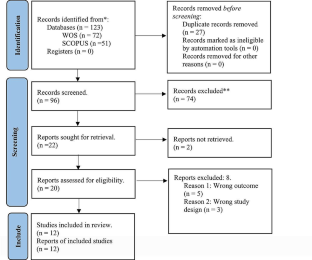
Similar content being viewed by others
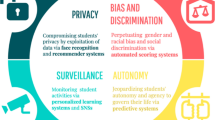
Artificial intelligence in education: Addressing ethical challenges in K-12 settings
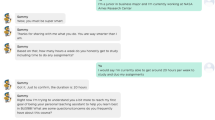
Artificial Intelligence (AI) Student Assistants in the Classroom: Designing Chatbots to Support Student Success
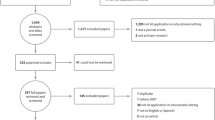
Systematic review of research on artificial intelligence applications in higher education – where are the educators?
Data availability.
Data sharing does not apply to this article as no datasets were generated or analyzed during the current study.
Ali, M., & Abdel-Haq, M. K. (2021). Bibliographical analysis of artificial intelligence learning in Higher Education: is the role of the human educator and educated a thing of the past? Fostering Communication and Learning With Underutilized Technologies in Higher Education , 36–52.
Anderson, J., Rainie, L., & Luchsinger, A. (2018). Artificial intelligence and the future of humans. Pew Research Center , 10 (12).
Bates, T., Cobo, C., Mariño, O., & Wheeler, S. (2020). No can artificial intelligence transform higher education? International Journal of Educational Technology in Higher Education , 17 (1), 1–12.
Article Google Scholar
Biggs, J., & Tang, C. (2011). Train-the-trainers: Implementing outcomes-based teaching and learning in Malaysian higher education. Malaysian Journal of Learning and Instruction , 8 , 1–19.
Blei, D. M., Ng, A. Y., & Jordan, M. I. (2003). Latent dirichlet allocation. Journal of Machine Learning Research , 3 , 993–1022.
Google Scholar
Chang, Y. S., Wang, Y. Y., & Ku, Y. T. (2023). Influence of online STEAM hands-on learning on AI learning, creativity, and creative emotions. Interact Learn Environ PG . https://doi.org/10.1080/10494820.2023.2205898 .
Chen, X., Xie, H., Zou, D., & Hwang, G. J. (2020). Application and theory gaps during the rise of artificial intelligence in education. Computers and Education: Artificial Intelligence , 1 , 100002.
Clark, D. (2023). PedAIgogy – New Era of Knowledge and Learning Where AI changes Everything .
Covidence (2023). Covidence systematic review software . www.covidence.org.
Crompton, H., & Burke, D. (2023). Artificial intelligence in higher education: The state of the field. International Journal of Educational Technology in Higher Education , 20 (1), 1–22.
Cumming, G., & McDougall, A. (2000). Mainstreaming AIED into education. International Journal of Artificial Intelligence in Education , 11 (2), 197–207.
Dignum, V. (2020). AI is multidisciplinary. AI Matters , 5 (4), 18–21.
Fuller, C. M., Simmering, M. J., Atinc, G., Atinc, Y., & Babin, B. J. (2016). Common methods variance detection in business research. Journal of Business Research , 69 (8), 3192–3198. https://doi.org/10.1016/j.jbusres.2015.12.008 .
Gao, Z., Wanyama, T., & Singh, I. (2020). Project and practice centered learning: A systematic methodology and strategy to cultivate future full stack artificial intelligence engineers. Int. J. Eng. Educ , 36 (6 PG-1760–1772), 1760–1772. https://www.scopus.com/inward/record.uri?eid=2s2.085096038454&partnerID=40&md5=b4778257adf34da504fdf1f97ebb7fe9NS .
Halic, O., Lee, D., Paulus, T., & Spence, M. (2010). To blog or not to blog: Student perceptions of blog effectiveness for learning in a college-level course. The Internet and Higher Education , 13 (4), 206–213.
Herrington, J., & Oliver, R. (2000). An instructional design framework for authentic learning environments. Educational Technology Research and Development , 48 (3), 23–48.
Hsu, T. C., & Chen, M. S. (2022). The engagement of students when learning to use a personal audio classifier to control robot cars in a computational thinking board game. Res Pract Technol Enhanc Learn , 17 (1 PG-). https://doi.org/10.1186/s41039-022-00202-1 .
Hwang, G. J., Xie, H., Wah, B. W., & Gašević, D. (2020). Vision, challenges, roles and research issues of Artificial Intelligence in Education. Computers and Education: Artificial Intelligence , 1 , 100001.
Jaramillo, F., Locander, W. B., Spector, P. E., & Harris, E. G. (2007). Getting the job done: The moderating role of initiative on the relationship between intrinsic motivation and adaptive selling. Journal of Personal Selling and Sales Management , 27 (1), 59–74.
Javed, R. T., Nasir, O., Borit, M., Vanhée, L., Zea, E., Gupta, S., Vinuesa, R., & Qadir, J. (2022). Get out of the BAG! Silos in AI Ethics Education: Unsupervised topic modeling analysis of global AI Curricula. Journal of Artificial Intelligence Research , 73 , 933–965. https://doi.org/10.1613/jair.1.13550 .
Jiang, L. (2021). Virtual reality Action interactive teaching Artificial Intelligence Education System. Complexity , 2021 (PG-). https://doi.org/10.1155/2021/5553211 .
Jobin, A., Ienca, M., & Vayena, E. (2019). The global landscape of AI ethics guidelines. Nature Machine Intelligence , 1 (9), 389–399.
Keller, J. M. (1987). Development and use of the ARCS model of instructional design. Journal of Instructional Development , 10 (3), 2–10.
Article MathSciNet Google Scholar
Kim, S., Jang, Y., Choi, S., Kim, W., Jung, H., Kim, S., & Kim, H. (2021). Analyzing teacher competency with TPACK for K-12 AI education. KI-Künstliche Intelligenz , 35 (2), 139–151.
Koh, J. H., Chai, L., Wong, C. S., & Hong, B., H.-Y (2015). Design thinking for education: Conceptions and applications in teaching and learning . Springer.
Kong, S. C., Cheung, W. M. Y., & Zhang, G. (2023). Evaluating an Artificial Intelligence Literacy Programme for developing University students’ conceptual understanding, literacy, empowerment and ethical awareness. Educational Technology and Society , 26 (1), 16–30. https://doi.org/10.30191/ETS.202301_26(1).0002 .
Korkmaz, Ö., & Xuemei, B. A. I. (2019). Adapting computational thinking scale (CTS) for Chinese high school students and their thinking scale skills level. Participatory Educational Research , 6 (1), 10–26.
Krathwohl, D. R. (2002). A revision of Bloom’s taxonomy: An overview. Theory into Practice , 41 (4), 212–218. https://doi.org/10.1207/s15430421tip4104_2 .
Lin, C. H., Wu, L. Y., Wang, W. C., Wu, P. L., & Cheng, S. Y. (2020). Development and validation of an instrument for AI-Literacy. 3rd Eurasian Conference on Educational Innovation (ECEI 2020) .
Lin, X. F., Chen, L., Chan, K. K., Peng, S. Q., Chen, X. F., Xie, S. Q., Liu, J. C., & Hu, Q. T. (2022). Teachers’ perceptions of teaching sustainable Artificial Intelligence: A Design Frame Perspective. SUSTAINABILITY , 14 (13 PG-). https://doi.org/10.3390/su14137811 .
Martín-Núñez, J. L., Ar, A. Y., Fernández, R. P., Abbas, A., & Radovanović, D. (2023). Does intrinsic motivation mediate perceived artificial intelligence (AI) learning and computational thinking of students during the COVID-19 pandemic? Computers & Education , 4(PG-) . https://doi.org/10.1016/j.caeai.2023.100128 .
Meek, T., Barham, H., Beltaif, N., Kaadoor, A., & Akhter, T. (2016). Managing the ethical and risk implications of rapid advances in artificial intelligence: A literature review. 2016 Portland International Conference on Management of Engineering and Technology (PICMET) , 682–693.
Miriyev, A., & Kovač, M. (2020). Skills for physical artificial intelligence. Nature Machine Intelligence , 2 (11), 658–660.
Ng, D. T. K., Lee, M., Tan, R. J. Y., Hu, X., Downie, J. S., & Chu, S. K. W. (2022). A review of AI teaching and learning from 2000 to 2020. EDUCATION AND INFORMATION TECHNOLOGIES . https://doi.org/10.1007/s10639-022-11491-w .
Pinkwart, N. (2016). Another 25 years of AIED? Challenges and opportunities for intelligent educational technologies of the future. International Journal of Artificial Intelligence in Education , 26 , 771–783.
Popenici, S. A., & Kerr, S. (2017). Exploring the impact of artificial intelligence on teaching and learning in higher education. Research and Practice in Technology Enhanced Learning , 12 (1), 1–13.
Rizvi, S., Waite, J., & Sentance, S. (2023). Artificial Intelligence teaching and learning in K-12 from 2019 to 2022: A systematic literature review. Computers and Education: Artificial Intelligence , 100145.
Shih, P. K., Lin, C. H., Wu, L. Y., & Yu, C. C. (2021). Learning ethics in AI—teaching non-engineering undergraduates through situated learning. Sustainability , 13 (7), 3718.
Southworth, J., Migliaccio, K., Glover, J., Glover, J. N., Reed, D., McCarty, C., Brendemuhl, J., & Thomas, A. (2023). Developing a model for AI across the curriculum: Transforming the higher education landscape via innovation in AI literacy. Computers & Education , 4 , PG–. https://doi.org/10.1016/j.caeai.2023.100127 .
Su, J., Guo, K., Chen, X., & Chu, S. K. W. (2023). Teaching artificial intelligence in K–12 classrooms: A scoping review. Interactive Learning Environments , 1–20.
Tedre, M., Toivonen, T., Kahila, J., Vartiainen, H., Valtonen, T., Jormanainen, I., & Pears, A. (2021). Teaching machine learning in K–12 classroom: Pedagogical and technological trajectories for artificial intelligence education. Ieee Access : Practical Innovations, Open Solutions , 9 , 110558–110572.
UNESCO (2023). 10 Innovative Learning Strategies For Modern Pedagogy . TeachThought Staff. https://policytoolbox.iiep.unesco.org/library/BHABKKH6 .
Wong, M. K., Wu, J., Ong, Z. Y., Goh, J. L., Cheong, C. W. S., Tay, K. T., Tan, L. H. S., & Krishna, L. K. R (2019). Teaching ethics in medical schools: A systematic review from 2000 to 2018. Journal of Medical Education , 18 , 226–250.
Xu, B. (2021). Artificial Intelligence Teaching System and Data Processing Method based on Big Data. Complexity , 2021(PG-) . https://doi.org/10.1155/2021/9919401 .
Yağcı, M. (2019). A valid and reliable tool for examining computational thinking skills. Education and Information Technologies , 24 (1), 929–951.
Yi, Y. (2021). Establishing the concept of AI literacy. European Journal of Bioethics , 12 (2), 353–368.
Yue, M., Jong, M. S. Y., & Dai, Y. (2022). Pedagogical design of K-12 artificial intelligence education: A systematic review. Sustainability , 14 (23), 15620.
Zawacki-Richter, O., Marín, V. I., Bond, M., & Gouverneur, F. (2019). Systematic review of research on artificial intelligence applications in higher education–where are the educators? International Journal of Educational Technology in Higher Education , 16 (1), 1–27.
Zeide, E. (2019). Artificial intelligence in higher education: Applications, promise and perils, and ethical questions. Educause Review , 54 (3).
Zhang, K., & Aslan, A. B. (2021). AI technologies for education: Recent research & future directions. Computers and Education: Artificial Intelligence , 2 , 100025.
Download references
Acknowledgements
Not applicable.
Author information
Authors and affiliations.
Faculty of Education, Simon Fraser University, Vancouver, BC, Canada
Bahar Memarian & Tenzin Doleck
You can also search for this author in PubMed Google Scholar
Corresponding author
Correspondence to Bahar Memarian .
Ethics declarations
Conflict of interest, additional information, publisher’s note.
Springer Nature remains neutral with regard to jurisdictional claims in published maps and institutional affiliations.
Rights and permissions
Reprints and permissions
About this article
Memarian, B., Doleck, T. Teaching and learning artificial intelligence: Insights from the literature. Educ Inf Technol (2024). https://doi.org/10.1007/s10639-024-12679-y
Download citation
Received : 03 August 2023
Accepted : 10 April 2024
Published : 02 May 2024
DOI : https://doi.org/10.1007/s10639-024-12679-y
Share this article
Anyone you share the following link with will be able to read this content:
Sorry, a shareable link is not currently available for this article.
Provided by the Springer Nature SharedIt content-sharing initiative
- Higher education
- Artificial intelligence (AI)
- Find a journal
- Publish with us
- Track your research
Help | Advanced Search
Computer Science > Cryptography and Security
Title: large language models for cyber security: a systematic literature review.
Abstract: The rapid advancement of Large Language Models (LLMs) has opened up new opportunities for leveraging artificial intelligence in various domains, including cybersecurity. As the volume and sophistication of cyber threats continue to grow, there is an increasing need for intelligent systems that can automatically detect vulnerabilities, analyze malware, and respond to attacks. In this survey, we conduct a comprehensive review of the literature on the application of LLMs in cybersecurity (LLM4Security). By comprehensively collecting over 30K relevant papers and systematically analyzing 127 papers from top security and software engineering venues, we aim to provide a holistic view of how LLMs are being used to solve diverse problems across the cybersecurity domain. Through our analysis, we identify several key findings. First, we observe that LLMs are being applied to a wide range of cybersecurity tasks, including vulnerability detection, malware analysis, network intrusion detection, and phishing detection. Second, we find that the datasets used for training and evaluating LLMs in these tasks are often limited in size and diversity, highlighting the need for more comprehensive and representative datasets. Third, we identify several promising techniques for adapting LLMs to specific cybersecurity domains, such as fine-tuning, transfer learning, and domain-specific pre-training. Finally, we discuss the main challenges and opportunities for future research in LLM4Security, including the need for more interpretable and explainable models, the importance of addressing data privacy and security concerns, and the potential for leveraging LLMs for proactive defense and threat hunting. Overall, our survey provides a comprehensive overview of the current state-of-the-art in LLM4Security and identifies several promising directions for future research.
Submission history
Access paper:.
- HTML (experimental)
- Other Formats
References & Citations
- Google Scholar
- Semantic Scholar
BibTeX formatted citation

Bibliographic and Citation Tools
Code, data and media associated with this article, recommenders and search tools.
- Institution
arXivLabs: experimental projects with community collaborators
arXivLabs is a framework that allows collaborators to develop and share new arXiv features directly on our website.
Both individuals and organizations that work with arXivLabs have embraced and accepted our values of openness, community, excellence, and user data privacy. arXiv is committed to these values and only works with partners that adhere to them.
Have an idea for a project that will add value for arXiv's community? Learn more about arXivLabs .
Thank you for visiting nature.com. You are using a browser version with limited support for CSS. To obtain the best experience, we recommend you use a more up to date browser (or turn off compatibility mode in Internet Explorer). In the meantime, to ensure continued support, we are displaying the site without styles and JavaScript.
- View all journals
- My Account Login
- Explore content
- About the journal
- Publish with us
- Sign up for alerts
- Open access
- Published: 11 March 2024
Twitter users perceptions of AI-based e-learning technologies
- Luisa Stracqualursi 1 &
- Patrizia Agati 1
Scientific Reports volume 14 , Article number: 5927 ( 2024 ) Cite this article
1492 Accesses
15 Altmetric
Metrics details
- Human behaviour
- Machine learning
- Psychology and behaviour
Today, teaching and learning paths increasingly intersect with technologies powered by emerging artificial intelligence (AI).This work analyses public opinions and sentiments about AI applications that affect e-learning, such as ChatGPT, virtual and augmented reality, microlearning, mobile learning, adaptive learning, and gamification. The way people perceive technologies fuelled by artificial intelligence can be tracked in real time in microblog messages promptly shared by Twitter users, who currently constitute a large and ever-increasing number of individuals. The observation period was from November 30, 2022, the date on which ChatGPT was launched, to March 31, 2023. A two-step sentiment analysis was performed on the collected English-language tweets to determine the overall sentiments and emotions. A latent Dirichlet allocation model was built to identify commonly discussed topics in tweets. The results show that the majority of opinions are positive. Among the eight emotions of the Syuzhet package, ‘trust’ and ‘joy’ are the most common positive emotions observed in the tweets, while ‘fear’ is the most common negative emotion. Among the most discussed topics with a negative outlook, two particular aspects of fear are identified: an ‘apocalyptic-fear’ that artificial intelligence could lead the end of humankind, and a fear for the ‘future of artistic and intellectual jobs’ as AI could not only destroy human art and creativity but also make the individual contributions of students and researchers not assessable. On the other hand, among the topics with a positive outlook, trust and hope in AI tools for improving efficiency in jobs and the educational world are identified. Overall, the results suggest that AI will play a significant role in the future of the world and education, but it is important to consider the potential ethical and social implications of this technology. By leveraging the positive aspects of AI while addressing these concerns, the education system can unlock the full potential of this emerging technology and provide a better learning experience for students.
Similar content being viewed by others
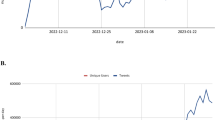
ChatGPT in education: global reactions to AI innovations
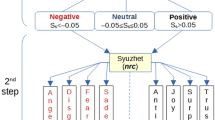
Tweet topics and sentiments relating to distance learning among Italian Twitter users
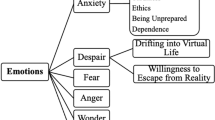
A perspective from Turkey on construction of the new digital world: analysis of emotions and future expectations regarding Metaverse on Twitter
Introduction, ai-powered e-learning technologies.
Current technology continues to advance, continuously transforming the way we live and the way we learn. Over the past few years, e-learning has become increasingly popular, as more people turn to online platforms for education and training. The COVID-19 pandemic has further accelerated this trend, as traditional classroom-based learning has become difficult, if not impossible, in many parts of the world.
Some of the key trends that we are also likely to see in the future of e-learning are as follows:
Adaptive learning. This method uses AI technology to personalize the learning experience for each student. This method adjusts the presentation of educational material according to an individual learner’s needs, preferences, and progress. By analysing students’ performance, it is possible to track their progress, and identify areas where they need more support or challenge. 1 , 2 , 3
Immersive learning. This refers to an educational approach that involves deeply engaging learners in a simulated or interactive environment. It seeks to create a sense of immersion, in which individuals feel fully involved in the learning process through various sensory experiences. The primary goal is to enhance the understanding, retention, and application of knowledge or skills. The key components of immersive learning include virtual and augmented reality (VR/AR). These technologies are becoming increasingly advanced and accessible, and we are likely to see more e-learning platforms using these technologies. 4 , 5 For example, a medical student could use VR to practice surgical techniques in a simulated operating room, 6 or an engineering student could use AR to visualize complex machinery and processes. 7
Microlearning. This refers to short, bite-sized learning modules that are designed to be completed in a few minutes or less. These modules are ideal for learners who have limited time or attention spans, and they can be easily accessed on mobile devices. In the future, we are likely to see more e-learning platforms using microlearning to deliver targeted, on-demand learning experiences. 8 , 9
Gamification. This refers to the use of game-like elements, such as badges, points, and leaderboards, to increase engagement and motivation among learners. Through the addition of game-like features to e-learning courses, learners can be incentivized to complete assignments and reach learning goals; thus, the learning experience becomes more engaging and enjoyable. 10 , 11 , 12
Mobile learning. With the widespread use of smartphones and tablets, e-learning is becoming more mobile-friendly, allowing learners to access course materials and complete assignments on the go. This makes learning more convenient and accessible, as learners can fit their learning into their busy schedules and on-the-go lifestyles. 13 , 14
Social learning. Social media and collaborative tools can enable learners to connect and learn from each other in online communities. 15 Learners can share their experiences, ask questions, and receive feedback from their peers, creating a sense of community and collaboration that can enhance the learning experience. 16
Generative AI. This refers to a subset of artificial intelligence focused on creating new content or information. It involves algorithms and models that are capable of generating novel content that can resemble human-generaed data. In February, 2022, an AI generative system named AlphaCode was launched. This system has been trained to ‘understand’ natural language, design algorithms to solve problems, and then implement them in code. At the end of November 2022, a new generative artificial intelligence chatbot that was developed by OpenAI and named ChatGPT was launched. ChatGPT has a wide range of potential applications due to its ability to generate human-like responses to natural language input. Some of its potentialities include text generation, summarization of long texts into shorter summaries, language translation, question answering, text completion, text correction, programming code generation, equation solutions and so on. ChatGPT evolved into OpenAI’s most widely used product to date, leading to the launch of ChatGPT Plus, a pilot paid subscription, in March 2023. Overall, the potential of AlphaCode and ChatGPT are vast, and these tools are likely to be used in many applications in the future of e-learning, as their capabilities will continue to improve through further research and development. ChatGPT can be integrated as a conversational AI within e-learning platforms. It can provide real-time responses to queries, clarify doubts, offer explanations, and guide learners through course materials. It is a promising tool for language lessons since it can translate text from one language to another. 17 To assist students and improve their writing abilities, ChatGPT may check for grammatical and structural problems in their work and provide valuable comments. 18 Students can explore many things with the help of ChatGPT, such as developing a computer program, writing an essay and solving a mathematical problem. 19 AlphaCode can aid e-learning platforms focused on programming or coding courses. It can provide code suggestions, explanations, and debugging assistance, helping learners better understand coding concepts. 20
How people perceive AI-based technologies: general and e-learning-focused literature overview
In the literature, people’s perceptions of technologies fuelled by artificial intelligence (AI) can vary depending on various factors such as their personal experiences and cultural backgrounds, and the way in which AI is portrayed in the media. The following are some common perceptions of AI technologies:
Fear of Job Loss. One of the most common fears associated with AI technologies is that they will take over jobs previously performed by humans. This fear is especially prominent in industries such as manufacturing, customer service, translation 21 , 22 , 23 and teaching 24 .
Improved Efficiency. Many people view AI technologies as a way to improve efficiency and accuracy in various fields. For example, AI-powered tools can improve students’ performance, 25 AI-powered software can help doctors diagnose diseases, 26 and chatbots can help students 27 and customer service representatives answer queries more quickly. 28
Ethical Concerns. There are concerns about the ethical implications of AI, such as bias in decision-making, 29 invasion of privacy, 30 and the potential for the development of AI-powered weapons. 31 For example, schools and institutions use AI-powered technologies to analyse student academic performance and collect a large amount of personal identity data; if these data are leaked or misused, this will seriously affect students’ personal privacy and security. In addition, students have difficulty controlling their own data and understanding how it is being used and shared, which may also lead to concerns and mistrust regarding personal privacy. 32 , 33
Excitement for Innovation. Some people are excited about the potential of AI to bring about new and innovative solutions to long-standing problems. For example, AI is being used to develop autonomous vehicles, which could revolutionize transportation, 34 and new methods for teaching and learning music. 35
Lack of Trust. Many people are still sceptical about the reliability and safety of AI technologies, especially given recent high-profile incidents of AI systems making mistakes 36 or being manipulated. 37 The lack of trust in the current application of generative AI in education mainly involves two aspects: opacity and reliability. When AI gives a result, it is difficult to explain the decision-making process, which makes it difficult for students to understand why they obtain a particular answer and how to improve their mistakes (opacity). Moreover, generative AI needs to be trained on a large dataset to ensure its effectiveness and reliability. For example, to train an effective automatic grading model, a large dataset of student essays and high-quality labelled data, such as scores for grammar, spelling, and logic, is needed. Insufficient datasets or low-quality labelled data may cause an automatic grading model to make mistakes and miss important aspects and affect its accuracy and application effectiveness. 38
Overall, people’s perceptions of AI technologies are complex and multifaceted, are influenced by a range of factors and are likely to continue evolving as AI becomes more integrated into our lives.
Purpose and outline of the paper
Considering what has been said thus far, it could be interesting to explore sentiments and major topics in the tweets about the new AI-based technologies.
Social media are indeed a major and rich data source for research in many domains due to their 4.8 billion active users 39 across the globe. For instance, researchers analyse user comments extracted from social media platforms (such as Facebook, 40 Twitter, 40 and Instagram 41 ) to uncover insights into social issues such as health, politics and business. Among these platforms, Twitter is one of the most immediate; tweets flow nonstop on the bulletin boards of users. Twitter allows users to express and spread opinions, thoughts and emotions as concisely and quickly as possible. Therefore, researchers have often preferred to analyse user comments on Twitter to immediately uncover insights into social issues during the COVID-19 pandemic (e.g., conspiracy theories, 42 why people oppose wearing a mask, 43 experiences in health care 44 and vaccinations 45 ) or distance learning. 46 , 47 , 48
Furthermore, we chose Twitter for its ability to immediately capture and spread people’s opinions and emotions on any topic, as well as for its ability to provide plentiful data, even in a short amount of time. Moreover, the people who have more direct experience with e-learning and AI technologies are students, teachers and researchers, i.e., persons of school or working age; that is, people who, by age, make up approximately 83% of Twitter users. 49
The text content of a tweet is a short microblog message containing at most 280 characters. This feature makes tweets particularly suitable for natural language processing (NLP) techniques, which are widely used to extract insights from unstructured texts and can then be used to explore sentiments and major topics of tweets. Unlike traditional methods, which use surveys and samples to evaluate these frameworks and are expensive and time-consuming, NLP techniques are economical and fast and provide immediate results.
In this paper, we aim to answer three main questions related to the first months following the launch of ChatGPT:
What has been the dominant sentiment towards AI-powered technologies? We responded through a sentiment analysis of related tweets. We used VADER as a sentiment analysis tool. 50
Which emotions about AI-powered technologies are prevalent? In this regard, we explored the emotions to the tweets using the Syuzhet package. 51
What are the most discussed topics among those who have positive feelings and those who have negative feelings? With respect to this problem, we used the latent Dirichlet allocation (LDA) model. 52
The findings from this study could aid in reimagining education in the postpandemic era to exploit technology and emerging strategies as benefits for educational institutions rather than as preparation for a new possible increase in infections. To this end, we decided to use only the technologies listed in “ AI-powered e-learning technologies ” as keywords for extracting tweets.
Methodology
Twitter was chosen as the data source. It is one of the world’s major social media platforms, with 237.8 million active users in July 2022, 53 and it is also a common source of text for sentiment analyses. 54 , 55 , 56
To collect AI-related tweets, we used ‘Academic Account for Twitter API V2’, which provides historical data and allows for the data to be filtered by language and geolocation. 57
For our study, we chose geolocated English-tweets only, posted from November 30, 2022 - March 31, 2023, with one or more of the following keywords: ‘ChatGPT’, ‘AlphaCode’, ‘virtual reality’, ‘augmented reality’, ‘micro-learning’, ‘mobile learning’, ‘adaptive learning’, ‘social leaning’, ‘AI’, ‘AI learning’ and ‘gamification’. A total of 31,147 tweets were collected.
Data preprocessing
In order to prepare the data for sentiment analysis, we employed various preprocessing techniques using NLP tools in Python. The steps we followed are as follows:
Eliminated mentions, URLs, and hashtags from the text,
Substituted HTML characters with their respective Unicode equivalents (e.g., replacing ‘ &’ with ‘ &’),
Removed HTML tags such as<br>,<p>, and others,
Eliminated unnecessary line breaks,
Removed special characters and punctuation except for exclamation points (the exclamation point is the only punctuation marks to which the used VADER lexicon is sensitive),
Excluded words that consist of only numbers.
For the second part, a high-quality dataset was required for the topic model. To achieve this, we removed duplicate tweets. In addition to the general data cleaning methods, we employed tokenization and lemmatization techniques to enhance the model’s performance.
We used the Gensim library 58 to tokenize the text, converting all the content to lowercase to ensure uniformity in word representation. Next, we pruned the vocabulary by removing stop words and terms unrelated to the topic. Additionally, we created a ‘ bigrams ' model to capture meaningful word combinations.
Finally, we employed the ‘ spaCy ' library from NLTK 59 to carry out lemmatization, which helped simplify words to their base form.
Sentiment and emotions analysis
To conduct sentiment analysis, we utilized the Valence Aware Dictionary for Sentiment Reasoning (VADER) algorithm, developed by Hutto et al. 50 VADER is a sentiment analysis tool that uses a sentiment lexicon, a dictionary specifically designed for sentiment analysis, to determine the emotion intensity of sentiment expressed in a text. The lexicon consists of words or phrases with their accompanying sentiment ratings. It allows for efficient sentiment analysis of social media content and exhibits remarkable accuracy comparable to that of humans.
Using VADER, we assigned sentiment scores to the preprocessed text data of each tweet. We followed the classification method recommended by the authors, categorizing the sentiment scores into three main categories: positive, negative, and neutral (see Fig. 1 —1st Step).
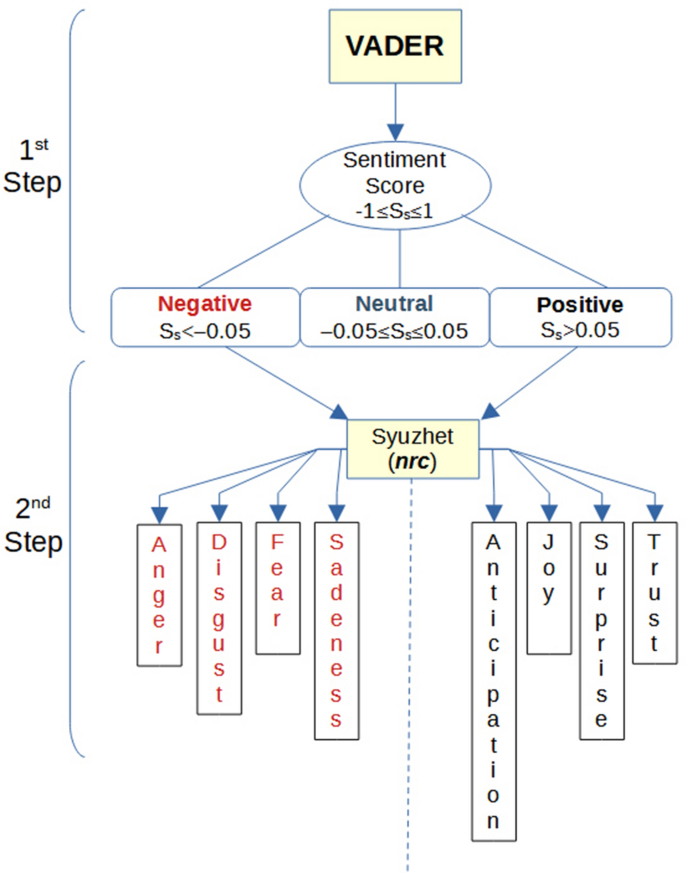
Steps in determining sentiment and emotions analysis.
VADER has demonstrated outstanding performance in analysing social media text. Comprehensive rules to consider various lexical features, including punctuation, capitalization, degree modifiers, the contrastive conjunction ’but’ and negation flipping trigrams.
Next, we employed the ‘ nrc ' algorithm, a component of the R library Syuzhet package, 51 to explore the underlying emotions associated with the tweet categories. In the ‘ nrc ' algorithm, an emotion dictionary is utilized to evaluate each tweet based on two sentiments (positive or negative) and eight emotions (anger, fear, anticipation, trust, surprise, sadness, joy, and disgust). Its purpose is to recognize the emotions conveyed within a tweet.
Whenever a tweet is connected to a specific emotion or sentiment, it receives points indicating the degree of valence in relation to that category. For instance, if a tweet includes three words associated with the ‘fear’ emotion in the word list, the tweet will receive a score of 3 in the fear category. Conversely, if a tweet does not contain any words related to a particular emotion, it will not receive a score for that specific emotion category.
When employing the ‘ nrc ’ lexicon, each tweet is assigned a score for each emotion category instead of a single algebraic score based on positive and negative words. However, this algorithm has limitations in accounting for negators and relies on a bag-of-words approach, disregarding the influence of syntax and grammar. Consequently, the VADER and ‘ nrc ’ methods are not directly comparable in terms of tweet volume and polarity categories.
Therefore, we utilized VADER for sentiment analysis and subsequently employed the ‘ nrc ' algorithm specifically for identifying positive and negative emotions. The sentiment analysis process follows a two-step procedure, as illustrated in Fig. 1 . While VADER’s neutral tweets play a valuable role in classification, they are not particularly informative for emotion analysis. Therefore we focused on tweets exhibiting positive and negative sentiments. This methodology was the original source of our previous paper. 60
The topic model
The topic model is an unsupervised machine learning method; that is, it is a text mining procedure that can be used to identify the topics or themes of documents in a large document corpus. 61 The latent Dirichlet allocation (LDA) model is one of the most popular topic modelling methods; it is a probabilistic model for expressing a corpus based on a three-level hierarchical Bayesian model. Latent Dirichlet allocation (LDA) is a generative probabilistic model of a corpus. The basic idea is that documents are represented as random mixtures over latent topics, where each topic is characterized by a distribution over words. 62 Particularly in LDA models, the generation of documents within a corpus follows the following process:
A mixture of k topics, \(\theta\) , is sampled from a Dirichlet prior, which is parameterized by \(\alpha\) ;
A topic \(z_n\) is sampled from the multinomial distribution, \(p(\theta \mid \alpha )\) that is the document topic distribution which models \(p(z_{n}=i\mid \theta )\) ;
Fixed the number of topics \(k=1...,K\) , the distribution of words for k topics is denoted by \(\phi\) ,which is also a multinomial distribution whose hyper-parameter \(\beta\) follows the Dirichlet distribution;
Given the topic \(z_n\) , a word, \(w_n\) , is then sampled via the multinomial distribution \(p(w \mid z_{n};\beta )\) .
Overall, the probability of a document (or tweet, in our case) “ \(\textbf{w}\) ” containing words can be described as:
Finally, the probability of the corpus of M documents \(D=\{\textbf{ w}_{\textbf{1}},...,{\textbf{w}}_{\textbf{M}}\}\) can be expressed as the product of the marginal probabilities of each single document \(D_m\) , as shown in ( 2 ).
An essential challenge in LDA is deterining an appropriate number of topics. Roder et al. 63 proposed coherence scores to evaluate the quality of each topic model. In particular, topic coherence is the metric used to evaluate the coherence between topics inferred by a model. As coherence measures, we used \(C_v\) which is a measure based on a sliding window that uses normalized pointwise mutual information (NPMI) and cosine similarity. 63 This value is used to emulate the relative score that a human is likely to assign to a topic and indicate how much the topic words ‘make sense’. This score is used to infer cohesiveness between ‘top’ words within a given topic. For topic visualization we used PyLDAvis, a web-based interactive visualization package that facilitates the display of the topics that were identified using the LDA approach. 52 In this package, each topic is visualized as a circle in a two-dimensional plane determined by principal components between topics and used Multidimensional scaling is used to project all the interrelated topic distances to two dimensions. 64 In the best hypothetic situation, the circles have similar dimensions and are well spaced from each other, covering the entire space made up of the 4 quadrants of the graph. An LDA model is evaluated the better the more the coherence is and the closer the pyLDAvis visualization is to the hypothetical situation.
Exploring the tweets
The word frequency of the most frequent 25 words terms are counted and visualized in Fig. 2 . The words are related to new AI tools and have positive attributes such as ‘good’ or ‘great’.
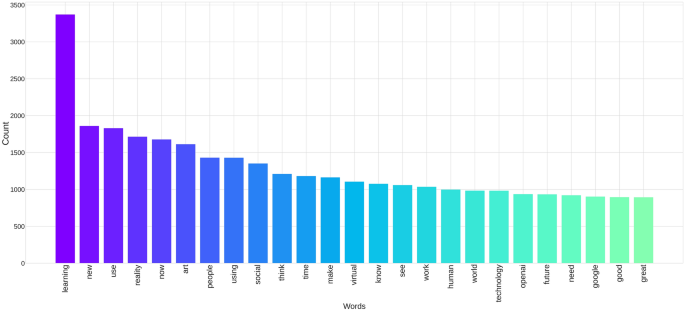
The total text word frequency. After removing irrelevant words, we counted and visualized the 25 most frequent words in our dataset.
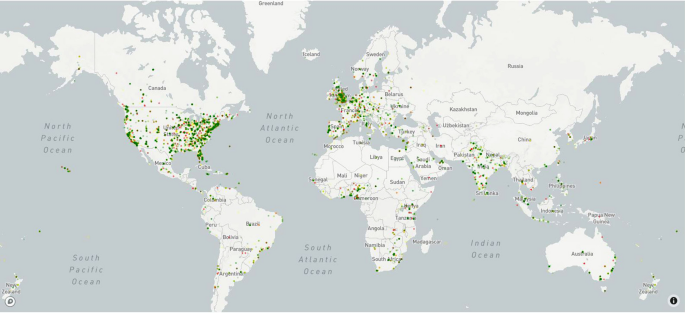
Tweets according to polarity by country.
All the tweets extracted were geolocated, but the ‘user location’ was detected in only approximately 85% of the tweets, highlighting the number of tweets that came from different counties around the world. The countries with the highest number of tweets are the United States and Great Britain (Fig. 3 ) but this result should be read considering that we have extracted tweets in the English language; therefore, it is normal that in countries where English is spoken, the number of tweets is very high. Notably, India and Europe also have a many Twitter users. In the United States, most Twitter users are located near the East and West Coasts. This figure also shows the polarity of the tweets: the colours red, yellow and green indicate negative, neutral and positive tweets, respectively.
Sentiment analysis
The sentiment score of a sentence is calculated by summing up the lexicon rates of each VADER-dictionary-listed word in the sentence. After proper normalization is applied, VADER returns a ‘compound’ sentiment score ( \(S_s\) ) in the range of \(-1\) to 1, from the most negative to the most positive. Once the score \(S_s\) is known, threshold values can be used to categorize tweets as positive, negative, or neutral (see Fig. 1 —1st Step). According to our analysis, the output of the VADER model shows a great predominance of positive public opinion (Table 1 ). As an example, three tweets with their own polarity are shown in Table 2 . Table 3 shows the number of total tweets with the related percentages and the percentages of positive, negative and neutral tweets of the various AI applications examined.
Regarding the timeline, the results showed that the number of tweets slowly increased during the observation period (Fig. 4 ). Clearly, as shown in the chart, there was a single weekly decline in the third week of March, likely due to the St. Patrick’s Day holiday, which fell close to the weekend (March 17).
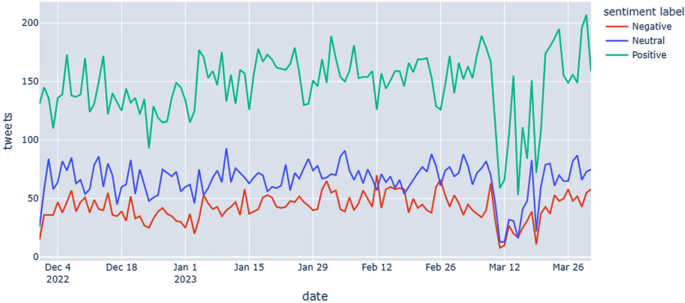
Timeline showing the sentiment of tweets.
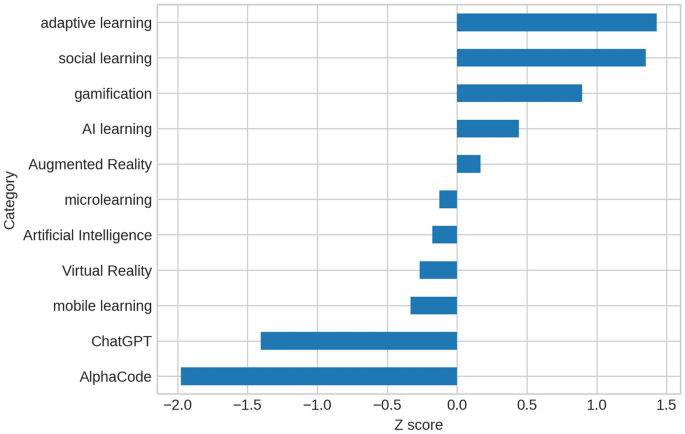
z scores of relative frequencies for positive sentiments.
The graph in Fig. 5 includes only the tweets with positive sentiment towards the 11 AI-based tools (categories). It returns the z scores of the relative frequencies \(Fr_{i}\) of positive tweets of the i -tool ( \(i=1,...,11\) ), where the z score is computed as follows:
A z score describes the position of a score (in our case \(Fr_i\) ) in terms of standard deviation units from the average. This score is grater then 0 if the relative frequency of \(Fr_i\) lies above the mean, while it is less than 0 if \(Fr_i\) lies below the mean.
We preferred z scores to relative frequencies because the different categories have different averages: the z scores highlight the position of each \(Fr_i\) with respect to the average of all the relative frequencies.
In Fig. 5 , we can see that the new generative AI ‘AlphaCode’ and ‘ChatGPT’ have scores that are much lower than and far from the average of positive sentiments. This could be due to concerns about the possible errors of such AI-based tools. Instead, ‘adaptive learning’, ‘social learning’ and ‘gamification’ lie above the mean of positive sentiments. This clearly attests to the more positive sentiment towards these tools, in our opinion largely due to their immediate feedback and to their attitude towards keeping learners engaged and motivated.
The second step of the analysis focused on identifying emotions in non-neutral tweets (see Fig. 1 —2nd Step). Among the eight basic emotions, ‘trust’ was the most common positive emotion observed in the tweets, followed by ‘joy’, while ‘fear’ was the most common negative emotion (Fig. 6 ). These results need to be interpreted in light of recent literature on the psychological dimensions of AI-based e-learning technologies (see section " How people perceive AI-based technologies: general and e-learning-focused literature overview "). In the literature, the dimension of fear includes the fear of job loss for teachers but also for the entire working world, 21 , 22 , 23 , 65 as well as concerns about AI systems making mistakes 36 or being manipulated. 37 The ‘trust’ dimension could be interpreted as the expectation that such technologies can improve the performance of students and people in general, 26 while ‘joy’ could be associated with enthusiasm for the potential of artificial intelligence in creating new and innovative solutions. 34
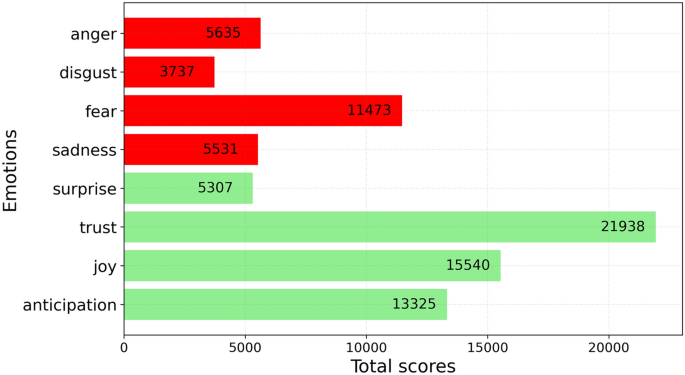
Emotion analysis of non-neutral tweets performed by Syuzhet .
To explore what concerns about AI-based tools Twitter users have, we applied the LDA model to our clean corpus of 28,259 words, which included only the following tagger components: nouns, adjectives, verbs and adverbs. Our goal was not to discover the topics discussed in the whole set of tweets but to detect the topics discussed in the positive sentiment tweets and the topics discussed in the negative sentiment tweets. Due to the large difference between the number of tweets with positive and negative sentiment polarity (57.58% vs. 16.65%), the application of the LDA model to the whole dataset would lead to not seeing the topics discussed in tweets with negative sentiment. Therefore, we chose to create two LDA models: one for tweets with positive polarity and one for those with negative polarity. For a better representation of the entire content, in each model, it is necessary to find an appropriate number of topics. By using topic numbers k ranging from 2 to 10, we initialized the LDA models and calculated the model coherence.
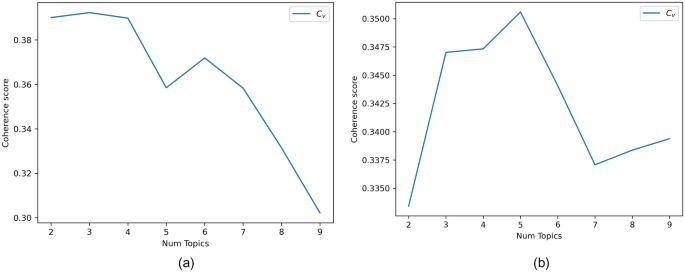
Coherence values of the LDA models.
We used \(C_v\) coherence for both models as a first reference. This value indicates the degree of ‘sense’ and ‘cohesiveness’ of the main words within a topic. 63
According to Fig. 7 a, the coherence score peaked at 3 for the negative tweet model. In contrast, in the positive tweet model, the coherence score (Fig. 7 b) peaked at 3 and 5 topics. The choice of 5 topic numbers would lead to a nonuniform distribution on Principal Component (PC) axes displayed by pyLDAvis, which means that there is not a high degree of independence for each topic (see the LDAvis map in Supplementary Information ‘ S2 ’ and ‘ S3 ’). A good model is judeged by a higher coherence and an average distribution on the principal component analysis displayed by pyLDAvis. 52 Therefore, we chose 3 as the topic number: the model has no intersections among topics, summarizes the whole word space well, and retains relatively independent topics.
The LDA analysis for negative and positive polarity tweets is shown in Table 4 .
In the negative tweets, the first theme accounts for 37.5% of the total tokens and includes tokens such as ‘chatgpt’, ‘write’,‘art’, ‘need’, ‘thing’, ‘stop’ and ‘generate’. It is rather immediate to think that this negative opinion refers to tools such as ChatGPT and expresses concerns about its use in art and writing. People think that human creativity can generate art and literature rather than generative AI; for this reason, the use of generative tools in these contexts should be stopped. The second theme accounts for 33.3% of the total tokens and includes the words ‘bad’, ‘job’, ‘technology’, ‘scary’, ‘change’ and ‘learn’. We infer people’s fear of the changes that technology will bring in the world of job and learning. Several words, such as ‘kill’, ‘war’, ‘worry’ ,‘fear’, ‘fight’, ‘robot’ and ‘dangerous’, are mentioned in the third topic. This may indicate a sort of ‘apocalyptic fear’ that artificial intelligence could lead us to a new war and the end of humankind. For a map representation of the three topics, see the Supplementary Information ‘ S1 ’.
In the positive tweets, the first theme accounts for 36.3% of the total tokens and includes tokens such as ‘learn’, ‘help’, ‘technology’, ‘student’ and ‘job’. Based on this, we inferred that people think that AI technologies have the potential to improve the job world and educational system. The second theme accounts for 34.7% of the total tokens, including the words ‘chatgpt’, ‘well’, ‘write’, ‘create’ and ‘ask’, showing people’s positive perception of AIs such as ChatGPT writing, asking questions and creating new solutions. After all, several words, such as ‘love’, ‘chatgpt’, ‘good’ ,‘answer’ and ‘believe’, are mentioned in the third topic. This indicates that people ‘believe in AI’ and trust tha AI, particularly ChatGPT provides good answers and solutions. For a map representation of the three topics, see the Supplementary Information ‘ S2 ’.
Based on the LDA outputs, the following six topics were identified:
For ‘negative polarity’ tweets:
Topic 1: Concerns about ChatGPT use in art and writing
Topic 2: Fear of changes in the world of job and learning
Topic 3: Apocalyptic-fear.
For ‘positive polarity’ tweets:
Topic 1: AI technologies can improve job and learning
Topic 2: Useful ChatGPT features
Topic 3: Belief in the ability of ChatGPT.
Limitations
This study has several limitations, which can be summarized as follows.
Limitations related to the use of keywords to extract tweets. Sometimes, keywords can be ambiguous, leading to noise-affected results. Due to the dynamic nature of social media, trends and topics change rapidly. Keywords might quickly lose relevance as new terms emerge.
Limitations related to emotion analysis . A first limitation is that the number of emotion categories was limited to 8; 51 , 66 however, emotion is a broad concept and, according to Cowen and Keltner 67 , may involve up to 27 categories. A second limitation is that misspelled words could not be identified or analysed in the algorithm. Further limitations involve the dictionary of sentiments (“lexicon”) developed by Mohammad and Turney for emotion analysis. 51 This dictionary maps a list of language features to emotion intensities, where:
Only 5 individuals were recruited to annotate a term against each of the 8 primary emotions.
The emotions associated with a term were annotated without considering the possible term context.
Although the percentages of agreement were apparently high, interrater reliability statistics were not reported.
Limitations of topic analysis. Considering that LDA is an unsupervised learning technique, the main limitation is the degree of subjectivity in defining the topic created. 45
Limitations of Twitter-based studies. Twitter data generally underestimate the opinions of people aged 50 and over, because approximately 83% of Twitter users worldwide are indeed under age 50. 49 However, in the present study bias has an almost negligible impact: the future of AI-powered e-learning technologies indeed has a greater impact on younger people than on older people.
Conclusions and future perspectives
With the aim of studying the opinions and emotions related to AI-powered e-learning technologies, we collected tweets on this issue and carried out a sentiment analysis using the VADER and Syuzhet packages in combination with a topic analysis.
There is no doubt that artificial intelligence has the potential to transform the whole education system. The results showed a predominance of positive attitudes: topics with a positive outlook indicate trust and hope in AI tools that can improve efficiency in jobs and the educational world. Indeed, among the eight emotions of the Syuzhet package, ‘trust’ and ’joy’ were the most positive emotions observed in the tweets, while ‘fear’ was the most common negative emotion. Based on the analysis, two particular aspects of fear were identified: an ‘apocalyptic fear’ that artificial intelligence could lead to the end of humankind and a fear of the ‘future of artistic and intellectual jobs’, as AI could not only destroy human art and creativity but also make individual contributions of students and researchers not assessable.
In our analysis, people with positive sentiments were directed towards ‘adaptive learning’, ‘social learning’ and ‘gamification’. Therefore, from a future perspective, we can expect an ever-increasing implementation of these aspects in e-learning. AI could help educators ‘adapt learning’ techniques to tailor them to the individual student, with his or her own interests, strengths and preferences.
By analysing data about interactions between students, AI can identify opportunities for collaboration between students and thus transform ‘social learning’ into ‘collaborative learning’. AI could help educators create more effective group work assignments, provide targeted support to struggling students, and promote positive social interactions among them.
In class, instead of administering boring tests, AI-powered ‘games and simulations’ could increasingly provide engaging and interactive learning experiences to help students develop skills and knowledge in a fun and engaging way. Moreover, gamification could be increasingly useful for providing immediate feedback and monitoring student progress over time.
Despite our analysis highlighting the great potential of and people’s expectations for AI-based technologies, there is an aspect that cannot be elucidated by examining tweets.
Algorithms such as ChatGPT disrupt traditional text-based assessments, as students can query the program to research a topic that results in documents authored by an algorithm and ready to submit as a graded assignment. Therefore, we need to reimagine student assessment in new ways. The current debate is whether educators should ban artificial intelligence platforms through school internet filters 38 or embrace algorithms as teaching and research tools. 68
In March, 2023, Italy was the first government to ban ChatGPT as a result of privacy concerns. The Italian data-protection authority said there were privacy concerns relating to the model and said it would investigate immediately. However, in late April, the ChatGPT chatbot was reactivated in Italy after its maker OpenAI addressed issues raised by Italy’s data protection authority.
Regardless of privacy concerns, possible data manipulations or the right answers to AI-based tools, we believe that the future cannot be stopped.
Data availability
All data generated or analyzed during this study are included in the Supplementary Information Files of this published article.
Zahabi, M. & Abdul Razak, A. M. Adaptive virtual reality-based training: A systematic literature review and framework. Virtual Real. 24 , 725–752. https://doi.org/10.1007/s10055-020-00434-w (2020).
Article Google Scholar
Raj, N. S. & Renumol, V. G. A systematic literature review on adaptive content recommenders in personalized learning environments from 2015 to 2020. J. Comput. Educ. 9 , 113–148. https://doi.org/10.1007/s40692-021-00199-4 (2022).
Al-Badi, A., Khan, A. & Eid-Alotaibi,. Perceptions of learners and instructors towards artificial intelligence in personalized learning. Proced. Comput. Sci. 201 , 445–451. https://doi.org/10.1016/j.procs.2022.03.058 (2022).
Bizami, N. A., Tasir, Z. & Kew, S. N. Innovative pedagogical principles and technological tools capabilities for immersive blended learning: A systematic literature review. Educ. Inf. Technol. 28 , 1373–1425. https://doi.org/10.1007/s10639-022-11243-w (2023).
Won, M. et al. Diverse approaches to learning with immersive virtual reality identified from a systematic review. Comput. Educ. 195 , 104701. https://doi.org/10.1016/j.compedu.2022.104701 (2023).
Tang, Y. M., Chau, K. Y., Kwok, A. P. K., Zhu, T. & Ma, X. A systematic review of immersive technology applications for medical practice and education—trends, application areas, recipients, teaching contents, evaluation methods, and performance. Educ. Res. Rev. 35 , 100429. https://doi.org/10.1016/j.edurev.2021.100429 (2022).
Wilkerson, M., Maldonado, V., Sivaraman, S., Rao, R. R. & Elsaadany, M. Incorporating immersive learning into biomedical engineering laboratories using virtual reality. J. Biol. Eng. 16 , 20. https://doi.org/10.1186/s13036-022-00300-0 (2022).
Article CAS PubMed PubMed Central Google Scholar
Taylor, A.-D. & Hung, W. The effects of microlearning: A scoping review. Educ. Technol. Res. Dev. 70 , 363–395. https://doi.org/10.1007/s11423-022-10084-1 (2022).
Wang, C., Bakhet, M., Roberts, D., Gnani, S. & El-Osta, A. The efficacy of microlearning in improving self-care capability: A systematic review of the literature. Public Health 186 , 286–296. https://doi.org/10.1016/j.puhe.2020.07.007 (2020).
Article CAS PubMed Google Scholar
Oliveira, W. et al. Tailored gamification in education: A literature review and future agenda. Educ. Inf. Technol. 28 , 373–406. https://doi.org/10.1007/s10639-022-11122-4 (2023).
Indriasari, T. D., Luxton-Reilly, A. & Denny, P. Gamification of student peer review in education: A systematic literature review. Educ. Inf. Technol. 25 , 5205–5234. https://doi.org/10.1007/s10639-020-10228-x (2020).
Liu, T., Oubibi, M., Zhou, Y. & Fute, A. Research on online teachers’ training based on the gamification design: A survey analysis of primary and secondary school teachers. Heliyon 9 , e15053. https://doi.org/10.1016/j.heliyon.2023.e15053 (2023).
Article PubMed PubMed Central Google Scholar
Widiastuti, N. L. A systematic literature review of mobile learning applications in environmental education from 2011–2021. J. Educ. Technol. Inst. 1 , 89–98 (2022).
Google Scholar
Criollo-C, S., Guerrero-Arias, A., Jaramillo-Alcázar, A. & Luján-Mora, S. Mobile learning technologies for education: Benefits and pending issues. Appl. Sci. https://doi.org/10.3390/app11094111 (2021).
Chelarescu, P. Deception in social learning: a multi-agent reinforcement learning perspective. arxiv: 2106.05402 (2021)
Gweon, H. Inferential social learning: Cognitive foundations of human social learning and teaching. Trends Cogn. Sci. 25 , 896–910. https://doi.org/10.1016/j.tics.2021.07.008 (2021).
Article PubMed Google Scholar
Javaid, M., Haleem, A., Singh, R. P., Khan, S. & Khan, I. H. Unlocking the opportunities through chatgpt tool towards ameliorating the education system. BenchCouncil Trans. Benchmarks Stand. Eval. 3 , 100115. https://doi.org/10.1016/j.tbench.2023.100115 (2023).
Sok, S. & Heng, K. ChatGPT for education and research: A review of benefits and risks. SSRN Electron. J. https://doi.org/10.2139/ssrn.4378735 (2023).
Yilmaz, R. & Karaoglan Yilmaz, F. G. Augmented intelligence in programming learning: Examining student views on the use of chatgpt for programming learning. Comput. Hum. Behav. Artif. Hum. 1 , 100005. https://doi.org/10.1016/j.chbah.2023.100005 (2023).
Becker, B. A. et al. Programming is hard—or at least it used to be: Educational opportunities and challenges of AI code generation. In Proceedings of the 54th ACM Technical Symposium on Computer Science Education V. 1 , SIGCSE 2023, 500–506, https://doi.org/10.1145/3545945.3569759 (Association for Computing Machinery, New York, 2023).
Ernst, E., Merola, R. & Samaan, D. Economics of artificial intelligence: Implications for the future of work. IZA J. Labor Policy 9 , 55. https://doi.org/10.2478/izajolp-2019-0004 (2019).
Jaiswal, A., Arun, C. J. & Varma, A. Rebooting employees: Upskilling for artificial intelligence in multinational corporations. Int. J. Hum. Resour. Manag. 33 , 1179–1208. https://doi.org/10.1080/09585192.2021.1891114 (2022).
Kirov, V. & Malamin, B. Are translators afraid of artificial intelligence?. Societies https://doi.org/10.3390/soc12020070 (2022).
Selwyn, N. Should Robots Replace Teachers?: AI and the Future of Education (John Wiley & Sons, 2019).
Baidoo-Anu, D. & Owusu Ansah, L. Education in the era of generative artificial intelligence (AI): Understanding the potential benefits of chatgpt in promoting teaching and learning. J. AI 7 , 52–62. https://doi.org/10.61969/jai.1337500 (2023).
van Leeuwen, K. G., de Rooij, M., Schalekamp, S., van Ginneken, B. & Rutten, M. J. C. M. How does artificial intelligence in radiology improve efficiency and health outcomes?. Pediatr. Radiol. 52 , 2087–2093. https://doi.org/10.1007/s00247-021-05114-8 (2022).
Shingte, K., Chaudhari, A., Patil, A., Chaudhari, A. & Desai, S. Chatbot development for educational institute. SSRN Electron. J. https://doi.org/10.2139/ssrn.3861241 (2021).
Wang, X., Lin, X. & Shao, B. How does artificial intelligence create business agility? Evidence from chatbots. Int. J. Inf. Manage. 66 , 102535. https://doi.org/10.1016/j.ijinfomgt.2022.102535 (2022).
Parikh, R. B., Teeple, S. & Navathe, A. S. Addressing bias in artificial intelligence in health care. JAMA 322 , 2377–2378. https://doi.org/10.1001/jama.2019.18058 (2019).
Mazurek, G. & Małagocka, K. Perception of privacy and data protection in the context of the development of artificial intelligence. J. Manag. Anal. 6 , 344–364 (2019).
David, W. E. A. Ai-powered lethal autonomous weapon systems in defence transformation. Impact and challenges. In Modelling and Simulation for Autonomous Systems (eds Mazal, J. et al. ) 337–350 (Springer International Publishing, 2020).
Chapter Google Scholar
May, M. & George, S. Privacy concerns in e-learning: Is UsingTracking system a threat?. Int. J. Inf. Educ. Technol. 1 , 1–8 (2011).
ADS Google Scholar
Ashman, H. et al. The ethical and social implications of personalization technologies for e-learning. Inf. Manag. 51 , 819–832. https://doi.org/10.1016/j.im.2014.04.003 (2014).
Ma, Y., Wang, Z., Yang, H. & Yang, L. Artificial intelligence applications in the development of autonomous vehicles: A survey. IEEE/CAA J. Autom. Sin. 7 , 315–329. https://doi.org/10.1109/JAS.2020.1003021 (2020).
Wei, J., Karuppiah, M. & Prathik, A. College music education and teaching based on AI techniques. Comput. Electr. Eng. 100 , 107851. https://doi.org/10.1016/j.compeleceng.2022.107851 (2022).
Mahmood, A., Fung, J. W., Won, I. & Huang, C.-M. Owning mistakes sincerely: Strategies for mitigating AI errors. In Proceedings of the 2022 CHI Conference on Human Factors in Computing Systems , CHI ’22, https://doi.org/10.1145/3491102.3517565 (Association for Computing Machinery, New York, 2022).
Carroll, M., Chan, A., Ashton, H. & Krueger, D. Characterizing manipulation from AI systems. arxiv: 2303.09387 (2023).
Yu, H. & Guo, Y. Generative artificial intelligence empowers educational reform: Current status, issues, and prospects. Front. Educ. https://doi.org/10.3389/feduc.2023.1183162 (2023).
Kemp, S. Digital 2023: Global digital overview. (Accessed April 2023); Online https://datareportal.com/reports/digital-2023-april-global-statshot (2023).
Zhan, Y., Etter, J.-F., Leischow, S. & Zeng, D. Electronic cigarette usage patterns: A case study combining survey and social media data. J. Am. Med. Inform. Assoc. 26 , 9–18. https://doi.org/10.1093/jamia/ocy140 (2019).
Hassanpour, S., Tomita, N., DeLise, T., Crosier, B. & Marsch, L. A. Identifying substance use risk based on deep neural networks and instagram social media data. Neuropsychopharmacology 44 , 487–494. https://doi.org/10.1038/s41386-018-0247-x (2019).
Rains, S. A., Leroy, G., Warner, E. L. & Harber, P. Psycholinguistic markers of COVID-19 conspiracy tweets and predictors of tweet dissemination. Health Commun. https://doi.org/10.1080/10410236.2021.1929691 (2021).
He, L. et al. Why do people oppose mask wearing? a comprehensive analysis of U.S. tweets during the COVID-19 pandemic. J. Am. Med. Inform. Assoc. 28 , 1564–1573. https://doi.org/10.1093/jamia/ocab047 (2021).
Ainley, E., Witwicki, C., Tallett, A. & Graham, C. Using twitter comments to understand people’s experiences of UK health care during the COVID-19 pandemic: Thematic and sentiment analysis. J. Med. Internet Res. https://doi.org/10.2196/31101 (2021).
Kwok, S. W. H., Vadde, S. K. & Wang, G. Tweet topics and sentiments relating to COVID-19 vaccination among Australian twitter users: Machine learning analysis. J. Med. Internet Res. 23 , e26953. https://doi.org/10.2196/26953 (2021).
Aljabri, M. et al. Sentiment analysis of Arabic tweets regarding distance learning in Saudi Arabia during the COVID-19 pandemic. Sensors (Basel) 21 , 5431. https://doi.org/10.3390/s21165431 (2021).
Article ADS CAS PubMed Google Scholar
Mujahid, M. et al. Sentiment analysis and topic modeling on tweets about online education during COVID-19. Appl. Sci. (Basel) 11 , 8438. https://doi.org/10.3390/app11188438 (2021).
Article CAS Google Scholar
Asare, A. O., Yap, R., Truong, N. & Sarpong, E. O. The pandemic semesters: Examining public opinion regarding online learning amidst COVID-19. J. Comput. Assist. Learn. 37 , 1591–1605. https://doi.org/10.1111/jcal.12574 (2021).
Statista. Distribution of twitter users worldwide as of april 2021, by age group. Statista. https://www.statista.com/statistics/283119/age-distribution-of-global-twitter-users/ (2021).
Hutto, C. & Gilbert, E. Vader: A parsimonious rule-based model for sentiment analysis of social media text. In Proceedings of the 8th International Conference on Weblogs and Social Media, ICWSM 2014 (2015).
Mohammad, S. & Turney, P. Emotions evoked by common words and phrases: Using mechanical turk to create an emotion lexicon. In Proceedings of the NAACL HLT 2010 Workshop on Computational Approaches to Analysis and Generation of Emotion in Text (LA, California, 2010).
Sievert, C. & Shirley, K. LDAvis: A method for visualizing and interpreting topics. In Proceedings of the Workshop on Interactive Language Learning, Visualization, and Interfaces , 63–70, https://doi.org/10.3115/v1/W14-3110 (Association for Computational Linguistics, https://aclanthology.org/W14-3110 , Baltimore, Maryland, 2014).
Kemp, S. Digital 2022: Twitter report (Accessed July 2022). Online https://datareportal.com/reports/digital-2023-deep-dive-the-state-of-twitter-in-april-2023 (2022).
Tumasjan, A., Sprenger, T., Sandner, P. & Welpe, I. Predicting elections with twitter: What 140 characters reveal about political sentiment. In Proc. Fourth Int. AAAI Conf. Weblogs Soc. Media Predict. , vol. 10 (2010).
Oyebode, O., Orji, R. Social. & media and sentiment analysis: The Nigeria presidential election,. In 2019 IEEE 10th Annual Information Technology. Electronics and Mobile Communication Conference (IEMCON) 2019 , https://doi.org/10.1109/IEMCON.2019.8936139 (IEEE 2019).
Budiharto, W. & Meiliana, M. Prediction and analysis of Indonesia presidential election from twitter using sentiment analysis. J. Big Data https://doi.org/10.1186/s40537-018-0164-1 (2018).
Twitter API V2 . Academic Account for Twitter API V2. https://developer.twitter.com/en/products/twitter-api/academic-research (2022).
Řehuřek, R. & Sojka, P. Software framework for topic modelling with large corpora. In Proceedings of LREC 2010 workshop New Challenges for NLP Frameworks , 46–50 (Univerity of Malta, 2010).
Bird, S., Klein, E. & Loper, E. Natural Language Processing with Python (O’Reilly Media, 2009).
Stracqualursi, L. & Agati, P. Tweet topics and sentiments relating to distance learning among Italian twitter users. Sci. Rep. 12 , 9163 (2022).
Article ADS CAS PubMed PubMed Central Google Scholar
Blei, D. M., Ng, A. Y., Jordan, M. I. & Lafferty, J. Latent dirichlet allocation. J. Mach. Learn. Res. 3 , 993–1022 (2003).
Lee, J. et al. Ensemble modeling for sustainable technology transfer. Sustainability 10 , 22–78. https://doi.org/10.3390/su10072278 (2018).
Röder, M., Both, A. & Hinneburg, A. Exploring the space of topic coherence measures. In Proceedings of the Eighth ACM International Conference on Web Search and Data Mining - WSDM ’15 (ACM Press, 2015).
Sievert, C. & Shirley, K. Package ldavis (Online) https://cran.r-project.org/web/packages/LDAvis/LDAvis.pdf (2022).
Edwards, B. I. & Cheok, A. D. Why not robot teachers: Artificial intelligence for addressing teacher shortage. Appl. Artif. Intell. 32 , 345–360. https://doi.org/10.1080/08839514.2018.1464286 (2018).
Plutchik, R. A general psychoevolutionary theory of emotion. In Theories of Emotion , 3–33, https://doi.org/10.1016/b978-0-12-558701-3.50007-7 (Elsevier, 1980).
Cowen, A. S. & Keltner, D. Self-report captures 27 distinct categories of emotion bridged by continuous gradients. Proc. Natl. Acad. Sci. U. S. A. 114 , E7900–E7909. https://doi.org/10.1073/pnas.1702247114 (2017).
Reyna, J. The potential of artificial intelligence (AI) and chatgpt for teaching, learning and research. In EdMedia+ Innovate Learning , 1509–1519 (Association for the Advancement of Computing in Education (AACE), 2023).
Download references
Author information
Authors and affiliations.
Department of Statistics, University of Bologna, 40126, Bologna, Italy
Luisa Stracqualursi & Patrizia Agati
You can also search for this author in PubMed Google Scholar
Contributions
L.S. and P.A. contributed equally to this work. Particularly, L.S. wrote the main text of the manuscript, contributed to the formal analysis, conceptualization, investigation and creation of the software in Python. P.A. contributed to the formalization of the applied methodology, to the data management, to the preparation of the figures, to the supervision and to the revision and modification of the manuscript.
Corresponding author
Correspondence to Luisa Stracqualursi .
Ethics declarations
Competing interests.
The authors declare no competing interests.
Additional information
Publisher's note.
Springer Nature remains neutral with regard to jurisdictional claims in published maps and institutional affiliations.
Supplementary Information
Supplementary figure s1., supplementary figure s2., supplementary figure s3., supplementary table s1., rights and permissions.
Open Access This article is licensed under a Creative Commons Attribution 4.0 International License, which permits use, sharing, adaptation, distribution and reproduction in any medium or format, as long as you give appropriate credit to the original author(s) and the source, provide a link to the Creative Commons licence, and indicate if changes were made. The images or other third party material in this article are included in the article’s Creative Commons licence, unless indicated otherwise in a credit line to the material. If material is not included in the article’s Creative Commons licence and your intended use is not permitted by statutory regulation or exceeds the permitted use, you will need to obtain permission directly from the copyright holder. To view a copy of this licence, visit http://creativecommons.org/licenses/by/4.0/ .
Reprints and permissions
About this article
Cite this article.
Stracqualursi, L., Agati, P. Twitter users perceptions of AI-based e-learning technologies. Sci Rep 14 , 5927 (2024). https://doi.org/10.1038/s41598-024-56284-y
Download citation
Received : 06 May 2023
Accepted : 05 March 2024
Published : 11 March 2024
DOI : https://doi.org/10.1038/s41598-024-56284-y
Share this article
Anyone you share the following link with will be able to read this content:
Sorry, a shareable link is not currently available for this article.
Provided by the Springer Nature SharedIt content-sharing initiative
By submitting a comment you agree to abide by our Terms and Community Guidelines . If you find something abusive or that does not comply with our terms or guidelines please flag it as inappropriate.
Quick links
- Explore articles by subject
- Guide to authors
- Editorial policies
Sign up for the Nature Briefing newsletter — what matters in science, free to your inbox daily.

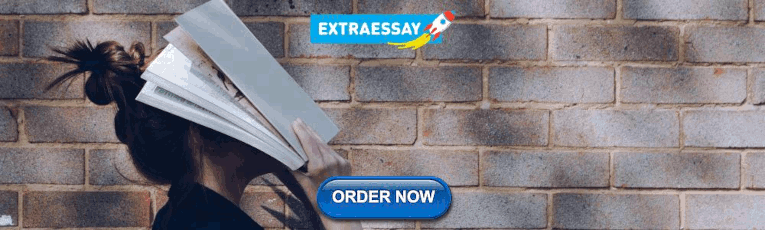
IMAGES
VIDEO
COMMENTS
The AI Literature Review Generator uses advanced AI models to search and analyze scholarly articles, books, and other resources related to your research topic. It identifies key themes, methodologies, findings, and gaps in the existing research, and compiles this information into a structured literature review, complete with an introduction ...
5. Consensus.app: Simplifying Literature Review with AI. Consensus is a search engine that simplifies the literature review process for researchers. By accepting research questions and finding relevant answers within research papers, Consensus synthesizes the results using language model technology.
Our AI Literature Review Generator is designed to assist you in creating comprehensive, high-quality literature reviews, enhancing your academic and research endeavors. Say goodbye to writer's block and hello to seamless, efficient literature review creation. Start writing - it's free.
In a survey of users, 10% of respondents said that Elicit saves them 5 or more hours each week. 2. In pilot projects, we were able to save research groups 50% in costs and more than 50% in time by automating data extraction work they previously did manually. 5. Elicit's users save up to 5 hours per week 1.
Semantic Reader is an augmented reader with the potential to revolutionize scientific reading by making it more accessible and richly contextual. Try it for select papers. Semantic Scholar uses groundbreaking AI and engineering to understand the semantics of scientific literature to help Scholars discover relevant research.
Best AI Tools for Literature Review. Since generative AI and ChatGPT came into the picture, there are heaps of AI tools for literature review available out there. Some of the most comprehensive ones are: SciSpace. SciSpace is a valuable tool to have in your arsenal. It has a repository of 270M+ papers and makes it easy to find research articles.
Enago Read is an AI assistant that speeds up the literature review process, offering summaries and key insights to save researchers reading time. It boosts productivity with advanced AI technology and the Copilot feature, enabling real-time questions for deeper comprehension of extensive literature.
In this essay, we focus on the use of AI-based tools in the conduct of literature reviews. Advancing knowledge in this area is particularly promising since (1) standalone review projects require substantial efforts over months and years (Larsen et al., 2019), (2) the volume of reviews published in IS journals has been rising steadily (Schryen et al., 2020), and (3) literature reviews involve ...
It includes VIP Support, AI-powered in-app help, and powerful tools to create, share and organize systematic reviews, review teams, searches, and full-texts. GET STARTED. ... LITERATURE REVIEW OVERVIEW. LEARN ABOUT RAYYAN'S PICO HIGHLIGHTS AND FILTERS. Join now to learn why Rayyan is trusted by already more than 500,000 researchers. ANNUAL.
Introducing SciSpace's all-new AI-powered literature review workspace. Sucheth. Jul 4, 2023. Scientists increasingly rely on AI and automation's power to uncover groundbreaking scientific discoveries. And we have a new addition to this toolbox — our all-new AI-powered literature review tool. Now simply enter a keyword or query, and the AI ...
SciSpace is an AI-powered literature review tool that excels in helping users find, read, and comprehend research papers. With a repository housing over 270 million research papers, SciSpace offers an extensive collection to explore. The key highlight of SciSpace is its AI research assistant, Copilot, which provides explanations, summaries, and ...
Artificial Intelligence for Literature Reviews: Opportunities and Challenges. This manuscript presents a comprehensive review of the use of Artificial Intelligence (AI) in Systematic Literature Reviews (SLRs). A SLR is a rigorous and organised methodology that assesses and integrates previous research on a given topic.
1 ServiceNow Research, 2 Mila - Quebec AI Institute, 3 HEC Montreal, Canada 4 UBC, Vancouver, Canada, 5 Canada CIFAR AI Chair Correspondence: [email protected]. Abstract. ... The literature review is a difficult task that can be decomposed into several sub-tasks, including retrieving relevant papers and generating a related works ...
The process of conducting literature reviews is often time-consuming and labor-intensive. To streamline this process, I present an AI Literature Review Suite that integrates several functionalities to provide a comprehensive literature review. This tool leverages the power of open access science, large language models (LLMs) and natural language processing to enable the searching, downloading ...
Provides AI-powered suggestions to discover related research material and stay updated with relevant news from the large data repository of 170+ million research papers. Promotes enhanced collaboration and structured note-taking for better team communication, accelerating the process of literature review and critical reading.
8. Get Merlin. Get Merlin AI literature review generator is an easy-to-use tool that helps automate the process of creating a literature review. It automates the creation of comprehensive literature reviews by searching and analyzing scholarly resources to identify key themes, methodologies, findings, and gaps.
Silvi.ai was founded in 2018 by Professor in Health Economic Evidence, Tove Holm-Larsen, and expert in Machine Learning, Rasmus Hvingelby. The idea for Silvi stemmed from their own research, and the need to conduct systematic literature reviews and meta-analyses faster. The ideas behind Silvi were originally a component of a larger project.
Enago Read - AI-based research assistant helps with discovery, literature review, critical analysis, paper summary and organize research papers. Free Sign-up!
Let AI Assist boost your literature review and analysis. As you may have noticed, there is a rapid growth in AI-based tools for all types of software packages. We followed this trend by releasing AI Assist - your virtual research assistant that simplifies your qualitative data analysis. In the following, we will present you the tools and ...
Our Mastering Literature Review with Litmaps course allows instructors to seamlessly bring Litmaps into the classroom to teach fundamental literature review and research concepts. Learn More. Join the 250,000+ researchers, students, and professionals using Litmaps to accelerate their literature review. Find the right papers faster.
Our Literature Review Generator is an AI-powered tool that streamlines and simplifies the creation of literature reviews by automatically collecting, analyzing, summarizing, and synthesizing all the relevant academic sources on a specific topic within the parameters you define. It saves you additional time by highlighting themes, trends, and ...
Welcome to the next level of academic exploration with our Literature Review Generator. Embrace the AI-driven convenience and precision, saving valuable time and resources, while empowering your research to stand out amidst the scholarly landscape.
This systematic literature review (SLR) aims to critically examine how the code generated by AI models impacts software and system security. Following the categorization of the research questions provided by Kitchenham and Charters (2007) on SLR questions, this work has a 2-fold objective: analyzing the impact and systematizing the knowledge ...
Mar 12th, 12:00 PM Mar 12th, 1:00 PM. Graduate Student's Productivity Tools for Literature Review Research and Writing in the Age of AI. In the fast-evolving world of academia, it is not hyperbole to say that generative AI and algorithm-based productivity tools like ChatGPT, Research Rabbit, and LitMap are quickly becoming transformative forces, reshaping the way graduate students (among ...
Many people are worried by the use of AI in academic papers. Indeed, the practice has been described as "contaminating" scholarly literature. Some argue that using AI output amounts to plagiarism.
In the fast-paced world of academic research, keeping up with the ever-growing body of literature can be a daunting task. Researchers and students often find themselves inundated with lengthy research papers, making it challenging to quickly grasp the core ideas and insights. AI-powered research paper summarizers have emerged as powerful tools, leveraging advanced algorithms to condense […]
Artificial Intelligence (AI) has been around for nearly a century, yet in recent years the rapid advancement and public access to AI applications and algorithms have led to increased attention to the role of AI in higher education. An equally important but overlooked topic is the study of AI teaching and learning in higher education. We wish to examine the overview of the study, pedagogical ...
The rapid advancement of Large Language Models (LLMs) has opened up new opportunities for leveraging artificial intelligence in various domains, including cybersecurity. As the volume and sophistication of cyber threats continue to grow, there is an increasing need for intelligent systems that can automatically detect vulnerabilities, analyze malware, and respond to attacks. In this survey, we ...
Today, teaching and learning paths increasingly intersect with technologies powered by emerging artificial intelligence (AI).This work analyses public opinions and sentiments about AI applications ...