
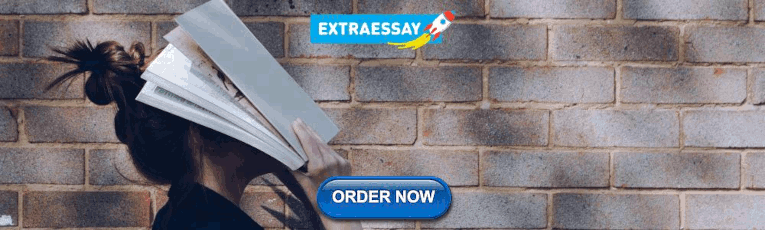
What is OLAP and OLTP, use-cases, comparison, and examples
OLTP (Online Transaction Processing) and OLAP (Online Analytical Processing) are two different types of database systems that are designed to support different types of workloads.
OLTP systems are designed to support transactional workloads, which involve inserting, updating, and deleting small amounts of data in a database. These systems are optimized for fast read and write performance, and are typically used to support real-time operational systems, such as e-commerce websites, ATM machines, and airline reservation systems.
OLAP systems, on the other hand, are designed to support analytical workloads, which involve querying large amounts of data to extract insights and perform data analysis. These systems are optimized for fast query performance and are typically used to support business intelligence and data warehousing applications.
Here are some examples of the use cases for OLTP and OLAP systems:
- E-commerce websites
- Banking systems
- Inventory management systems
- Customer relationship management (CRM) systems
- Data warehousing and business intelligence
- Financial analysis
- Marketing analysis
- Supply chain analysis
In summary, OLTP systems are used for transactional processing, while OLAP systems are used for analytical processing. OLTP systems are optimized for fast read and write performance, while OLAP systems are optimized for fast query performance.
Here is a list of some popular OLTP and OLAP tools :
OLTP (Online Transaction Processing) tools:
- Microsoft SQL Server
- Oracle (Oracle)
OLAP (Online Analytical Processing) tools:
- Apache Hive
- Apache Impala
- Amazon Redshift
- Google BigQuery
Note that some database systems, such as Oracle, can be used for both OLTP and OLAP workloads, depending on how they are configured and used.
Cloud-Based OLAP over Big Data: Application Scenarios and Performance Analysis
Ieee account.
- Change Username/Password
- Update Address
Purchase Details
- Payment Options
- Order History
- View Purchased Documents
Profile Information
- Communications Preferences
- Profession and Education
- Technical Interests
- US & Canada: +1 800 678 4333
- Worldwide: +1 732 981 0060
- Contact & Support
- About IEEE Xplore
- Accessibility
- Terms of Use
- Nondiscrimination Policy
- Privacy & Opting Out of Cookies
A not-for-profit organization, IEEE is the world's largest technical professional organization dedicated to advancing technology for the benefit of humanity. © Copyright 2024 IEEE - All rights reserved. Use of this web site signifies your agreement to the terms and conditions.
- Technologies
- Microsoft Azure
- Google Cloud
- Azure Cloud
- Records Management
- Warehousing and Storage
- Schedule a Call
OLAP: A Deep Dive into Online Analytical Processing
January 9, 2024
In the ever-evolving landscape of business intelligence, the acronym OLAP (Online Analytical Processing) stands tall as a pivotal player in data analysis and reporting. As the demand for sophisticated data-driven decision-making tools grows, businesses are increasingly turning to OLAP to unlock the potential of their data. In this comprehensive exploration, we delve into the intricacies of OLAP, its role in data warehouse architecture, and its relevance for businesses seeking to empower their higher management, chief people officers, managing directors, and country managers with actionable insights.
Key Characteristics of OLAP
Online Analytical Processing, commonly known as OLAP, is a powerful technology enabling users to analyze multidimensional data interactively. Unlike its counterpart, OLTP (Online Transaction Processing), which focuses on transactional processing, OLAP is designed for complex queries and reporting. OLAP systems organize data into multidimensional structures, facilitating efficient and dynamic analysis.
- Multidimensionality: OLAP systems organize data into dimensions and hierarchies, providing a multi-dimensional view for analysis. This allows users to navigate through data in various ways, gaining deeper insights.
- Aggregation: OLAP supports aggregation, enabling users to roll up or drill down into data at different levels of granularity. This flexibility is crucial for executives requiring high-level overviews and detailed insights.
- Interactivity: OLAP tools offer interactive analysis capabilities, allowing users to manipulate data in real-time. This feature particularly benefits decision-makers who must explore different scenarios and make informed choices quickly.
OLAP Models
Online Analytical Processing (OLAP) models form the backbone of interactive and multidimensional data analysis. In this section, we delve into the various OLAP models, each offering unique characteristics to cater to the diverse needs of businesses.
MOLAP (Multidimensional OLAP)
MOLAP systems organize data into multidimensional cubes, providing a structured and efficient approach to analysis. This model offers rapid query performance and is particularly suitable for scenarios where responsiveness is crucial.
Key Features
- Cube Structure: Data is stored in a cube format, facilitating easy navigation.
- High Performance: MOLAP systems are optimized for fast query retrieval.
- Examples: Microsoft Analysis Services, IBM Cognos TM1.
ROLAP (Relational OLAP)
ROLAP systems store data in relational databases, offering greater scalability and flexibility. This model is well-suited for businesses dealing with large datasets and complex relationships.
- Relational Storage: Data is stored in relational databases, ensuring flexibility.
- Scalability: ROLAP systems can handle vast amounts of data effectively.
- Examples: Oracle OLAP, SAP BW.
HOLAP (Hybrid OLAP)
HOLAP combines elements of both MOLAP and ROLAP, striking a balance between performance and scalability. This model integrates the advantages of multidimensional storage and relational databases.
- Hybrid Approach: HOLAP systems leverage both cube and relational storage methods.
- Optimal Performance: Balances performance considerations for diverse analytical needs.
- Examples: Microsoft SQL Server Analysis Services.
Understanding the nuances of each OLAP model is crucial for businesses seeking to align their data analysis capabilities with specific requirements and objectives. Whether prioritizing speed, scalability, or a hybrid approach, selecting the right OLAP model is integral to unlocking the full potential of multidimensional data analysis.
OLAP in Data Warehouse Architecture
In the dynamic landscape of business intelligence, a robust data warehouse OLAP architecture is the backbone that supports informed decision-making. OLAP (Online Analytical Processing) is at the heart of this architecture, a powerful tool designed to transform raw data into actionable insights.
The Data Warehouse Foundation
As of 2021, the global online analytical processing market was valued at approximately $3.8 billion , with a compound annual growth rate (CAGR) of around 8%. Before delving into OLAP, it’s essential to understand the fundamentals of a data warehouse. A data warehouse is a centralized repository consolidating data from disparate sources within an organization. This consolidation creates a unified and structured dataset, forming the bedrock for meaningful analysis. Key features of a data warehouse include:
- Centralized Storage: Data warehouses provide a single, centralized location for storing data. This eliminates data silos, ensuring that all relevant information is accessible from a unified source. This centralized storage is crucial for streamlined analysis for businesses with diverse datasets.
- Historical Data: Unlike traditional databases focusing on current data, data warehouses store historical data over time. This historical perspective allows businesses to analyze trends, track performance, and make informed decisions based on a comprehensive understanding of their data.
Enhancing Analytical Capabilities
A TDWI survey indicated that over 60% of surveyed companies have implemented OLAP in their data warehousing strategy. Once the data warehouse foundation is established, OLAP steps in to unlock the full potential of the stored data. Online analytical processing is the analytical engine facilitating interactive and dynamic data analysis. Let’s explore how OLAP seamlessly integrates into data warehouse architecture.
- Cube Creation: OLAP organizes data into multidimensional structures known as cubes. These cubes represent a comprehensive data view, incorporating multiple dimensions and hierarchies. The cube creation process involves mapping relevant dimensions to the data, allowing for more nuanced and sophisticated analysis.
- Integration with ETL Processes: The Extract, Transform, Load (ETL) process is fundamental in populating and updating a data warehouse. OLAP seamlessly integrates into these ETL processes, ensuring that the data within the warehouse is current and ready for analysis. This integration establishes a dynamic relationship between OLAP and the data warehouse, allowing real-time insights.
OLAP Models in Data Warehouse Architecture
Studies by Forrester Research highlight that organizations leveraging OLAP in their data warehousing architecture experience, on average, a 15% improvement in decision-making processes and a 20% reduction in time spent on data analysis. OLAP comes in various models, each with its strengths and use cases. Understanding these models is crucial for optimizing analytical processes within the data warehouse.
- MOLAP (Multidimensional OLAP): MOLAP systems store data in a multidimensional cube format. This storage structure is highly efficient for quick query performance, making it ideal for scenarios where rapid analysis is paramount.
- ROLAP (Relational OLAP): ROLAP systems store data in relational databases. This model offers greater scalability and flexibility, making it suitable for large-scale data warehousing scenarios.
- HOLAP (Hybrid OLAP): HOLAP combines elements of both MOLAP and ROLAP, offering a balanced approach that prioritizes performance and scalability. This model benefits organizations seeking a middle ground between speed and adaptability.
OLAP Analysis Techniques
Among businesses implementing OLAP, the distribution between MOLAP, ROLAP, and HOLAP models is approximately 40%, 35%, and 25% respectively . OLAP’s true strength lies in enabling users to analyze multidimensional data interactively. Several analysis techniques empower users to derive meaningful insights:
- Slice and Dice: OLAP allows users to “slice” the data by selecting a specific dimension and “dice” it by choosing subsets. This technique provides a granular data view, allowing detailed analysis based on specific parameters.
- Pivot: The pivot function enables users to rotate the axes of the cube, providing different perspectives of the data. This dynamic feature is valuable for decision-makers who need diverse angles for strategic decision-making.
OLAP Reporting
About 70% of large enterprises were integrating OLAP capabilities with big data analytics solutions to handle the increasing volumes of data. OLAP goes beyond analysis by facilitating the creation of comprehensive OLAP reports. Reporting features are crucial for delivering actionable insights to stakeholders across the organization.
- Customized Dashboards: OLAP tools enable the creation of customized dashboards that present key performance indicators (KPIs) in a visually appealing and easily understandable format. These dashboards provide a consolidated view of critical metrics, supporting faster decision-making.
- Ad-hoc Reporting: The flexibility of online analytical processing allows users to generate ad-hoc reports on the fly. This capability is invaluable for scenarios where immediate insights are required, empowering decision-makers with the information they need without delay.
OLAP Data Modeling
The migration to cloud-based data warehousing solutions is on the rise, with over 50% of enterprises planning or already moving their data warehousing infrastructure to the cloud. Data modeling is crucial to leveraging OLAP effectively within the data warehouse architecture. Dimensional modeling, in particular, is pivotal in optimizing OLAP analysis.
- Star Schema: One prevalent dimensional modeling technique is the star schema. In this model, a central fact table is surrounded by dimension tables. This schema simplifies querying and enhances performance by creating a structure that facilitates efficient data retrieval.
- Snowflake Schema: A snowflake schema, where dimension tables are normalized, is sometimes employed. While this approach ensures data integrity, it may require more complex queries than the star schema.
OLAP and Big Data
A case study conducted by IBM reported that organizations implementing OLAP solutions experienced, on average, a 20% improvement in return on investment (ROI) within the first year. As organizations grapple with the influx of big data, OLAP systems must adapt to handle vast datasets. The intersection of OLAP and big data opens new possibilities for scalable and high-performance analytics.
- Scalability: Scalable OLAP solutions accommodate the growing data demands faced by enterprises dealing with massive datasets. This scalability ensures that OLAP remains a reliable tool for businesses seeking to harness the potential of big data.
- Integration with Advanced Analytics: The integration of OLAP with advanced analytics tools enhances its capability to derive actionable insights from vast datasets. This synergy positions OLAP as a strategic asset for businesses looking to stay ahead in a competitive landscape.
At its core, OLAP data modeling is about organizing data to facilitate intuitive and efficient analysis. Unlike traditional relational databases optimized for transactional processing (OLTP), OLAP data modeling focuses on providing a multidimensional view of the data, allowing users to navigate through various dimensions for a comprehensive understanding.
- Centralized Metrics: Facts in OLAP data models represent the numerical data or metrics that businesses want to analyze. These could include sales figures, revenue, quantities sold, or any other measurable KPIs central to the organization.
- Organized Structures: Hierarchies define the relationships within dimensions. For example, a time dimension hierarchy could include levels like year, quarter, month, and day. Hierarchies enhance the ability to drill down or roll up through different levels of granularity.
- Quantifiable Attributes: Measures are additional quantitative attributes associated with dimensions. They provide further granularity to the analysis. For instance, within the “product” dimension, measures could include a unit price or discount percentage.
- Centralized Fact Table: The star schema is one of the most widely used OLAP data modeling techniques. In this model, a central fact table is surrounded by dimension tables, forming a star-like structure. This simplifies queries and enhances performance by reducing the number of joins needed.
- Snowflake Schema: Alternatively, the snowflake schema extends the star schema by normalizing dimension tables. While this maintains data integrity, it can result in more complex queries due to the need for additional joins.
- Collaborative Approach: Work closely with stakeholders, including higher management, to understand the metrics and dimensions critical for decision-making. This collaboration ensures the OLAP data model is tailored to meet organizational needs.
- Focus on Relevance: Identify the KPIs that align with organizational goals. This step is crucial for managing directors and executives who require high-level strategic insights.
Future Trends in OLAP
As businesses strive to stay ahead in the competitive realm of business intelligence, several future trends are poised to shape the trajectory of online analytical processing. Let’s explore some of the key trends that are likely to redefine OLAP in the coming years:
- Prescriptive Analytics: Going beyond predicting future trends, OLAP systems may evolve to offer prescriptive analytics. By recommending optimal courses of action based on historical data and machine learning algorithms, OLAP can empower decision-makers with actionable insights, guiding them toward the most favorable outcomes.
- Conversational Analytics: Future OLAP systems may incorporate advanced natural language processing (NLP) capabilities, enabling users to interact with data using plain language. This user-friendly approach makes data analysis more accessible to a broader audience, including those without a deep technical background. This trend is particularly beneficial for chief people officers and managing directors who may not have a technical background but need to derive insights from data.
- Voice-Activated Analytics: As voice-activated technologies become more prevalent, OLAP systems could integrate voice commands for data analysis. This would allow users to verbally request specific insights, enhancing the ease of interaction with data. This trend aligns with the growing importance of user experience in BI tools.
- Increased Scalability: Cloud-based OLAP solutions offer businesses increased scalability to adapt to changing data volumes and computational demands. This flexibility is crucial for managing directors overseeing expanding operations, as it allows them to scale their infrastructure as needed without the constraints of physical hardware.
- Cost-Efficiency: Cloud-based OLAP solutions often provide a cost-efficient alternative to on-premises implementations. The pay-as-you-go model eliminates the need for extensive upfront investments in hardware and infrastructure. This cost efficiency appeals to businesses looking to optimize their BI solutions while maintaining high performance.
- Immersive Analytics: Future OLAP systems may embrace immersive analytics, incorporating virtual reality (VR) or augmented reality (AR) elements for data visualization. This trend enhances the user experience, allowing decision-makers to explore data in a more interactive and three-dimensional space. This can be particularly beneficial for managing directors seeking a holistic view of complex business scenarios.
- Storytelling with Data: OLAP tools may evolve to facilitate better storytelling with data. Instead of presenting raw numbers, future systems could assist users in creating compelling narratives around the data, making it easier for higher management to communicate insights and strategies effectively.
- Real-Time Decision-Making: With the rise of edge computing, OLAP systems may integrate with edge devices to enable real-time decision-making at the network’s edge. This is particularly relevant for businesses with distributed operations, allowing them to analyze and act on data locally, reducing latency and improving responsiveness.
- Enhanced Data Security: Blockchain technology may find its way into OLAP systems to enhance data integrity and security. By providing a decentralized and tamper-proof ledger, blockchain can ensure the integrity of the data stored and analyzed by OLAP systems. This is crucial for businesses prioritizing data security and transparency, especially when dealing with sensitive information.
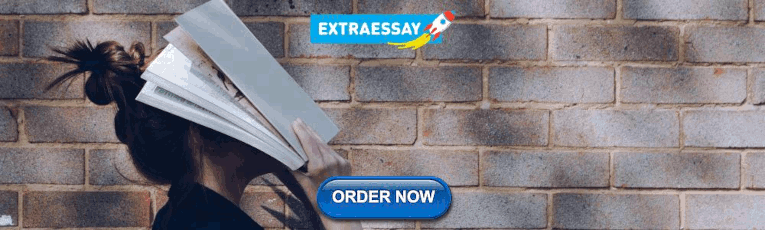
How can Brickclay Help?
Brickclay, as a leading provider of business intelligence services , is well-positioned to assist organizations in leveraging the power of OLAP for their data analysis and decision-making needs. Here’s how Brickclay can specifically help businesses, addressing the requirements of higher management, chief people officers, managing directors, and country managers:
- Customized OLAP Solutions: Brickclay specializes in tailoring OLAP solutions to meet the unique needs of businesses. Whether implementing MOLAP, ROLAP, or HOLAP models, the company ensures that the chosen OLAP system aligns seamlessly with the organization’s data structure and analytical requirements.
- Data Warehousing Expertise: With a deep understanding of data warehousing, Brickclay can assist in designing and implementing robust data warehouse architectures. This includes centralizing data from various sources, establishing efficient ETL processes, and ensuring data integrity – all essential components for effective OLAP analysis.
- Persona-Centric OLAP Implementation: Brickclay adopts a persona-centric approach to OLAP implementation. The company works closely with higher management, chief people officers, managing directors, and country managers to understand their specific analytical needs. This ensures that the OLAP system is configured to deliver actionable insights tailored to each persona’s requirements.
- Training and Support: Understanding the importance of user adoption, Brickclay provides comprehensive training sessions for employees at all levels. Whether it’s teaching executives how to perform scenario analyses or guiding HR teams through workforce analytics, the aim is to empower users to make the most of OLAP tools.
- Dashboard Development: Brickclay excels in creating intuitive and visually appealing dashboards that cater to the diverse needs of higher management, chief people officers, managing directors, and country managers. These dashboards offer quick access to key metrics, supporting informed decision-making.
- Scalable OLAP Solutions for Growth: Recognizing that businesses evolve, Brickclay ensures that the implemented OLAP solutions are scalable. This scalability is crucial for managing directors overseeing expanding operations and dealing with increasing data volumes.
- Integration with Advanced Analytics: As the landscape of business intelligence evolves, Brickclay is at the forefront of integrating OLAP with advanced analytics, including AI and machine learning. This ensures businesses can benefit from predictive analytics, gaining insights into future trends.
- Cloud-Based OLAP Solutions: Embracing the cloud computing trend, Brickclay offers expertise in implementing cloud-based OLAP solutions. This provides increased scalability and cost-efficient alternatives for businesses looking to optimize their infrastructure investments.
- Continuous Innovation and Future-Proofing: Brickclay is committed to staying ahead of the curve, keeping clients at the forefront of technological advancements in OLAP. The company actively explores emerging trends, such as enhanced natural language processing, to ensure that clients are equipped with the latest tools for data analysis.
Feel free to contact us at Brickclay for unparalleled expertise in business intelligence and personalized solutions tailored to your data needs. Contact our dedicated team today to embark on a journey of insightful decision-making. Your success starts with a conversation – connect with us now.
Like what you see ? Share with a friend.
About brickclay.
Brickclay is a digital solutions provider that empowers businesses with data-driven strategies and innovative solutions. Our team of experts specializes in digital marketing, web design and development, big data and BI. We work with businesses of all sizes and industries to deliver customized, comprehensive solutions that help them achieve their goals.
Recommended Reading
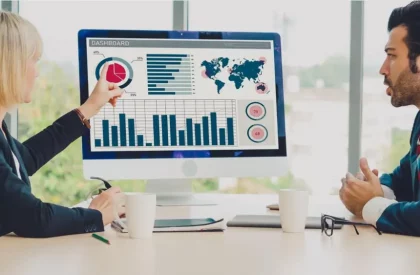
bi and data visualization
Data Reporting and Visualization Influence on Business Intelligence
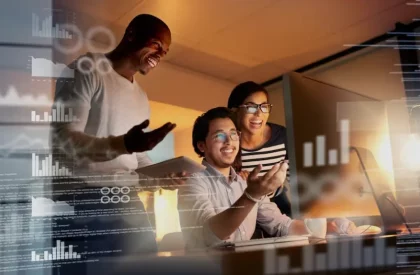
business intelligence strategy
Crafting a Data Driven Culture: Business Intelligence Strategy and Consulting
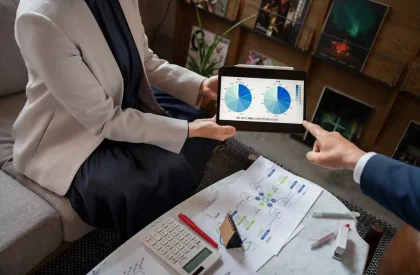
bi performance
Connecting Goals to Metrics: The Role of Performance Management in BI
Stay connected.
Get the latest blog posts delivered directly to your inbox.
Follow us for the latest updates
Have any feedback or questions.
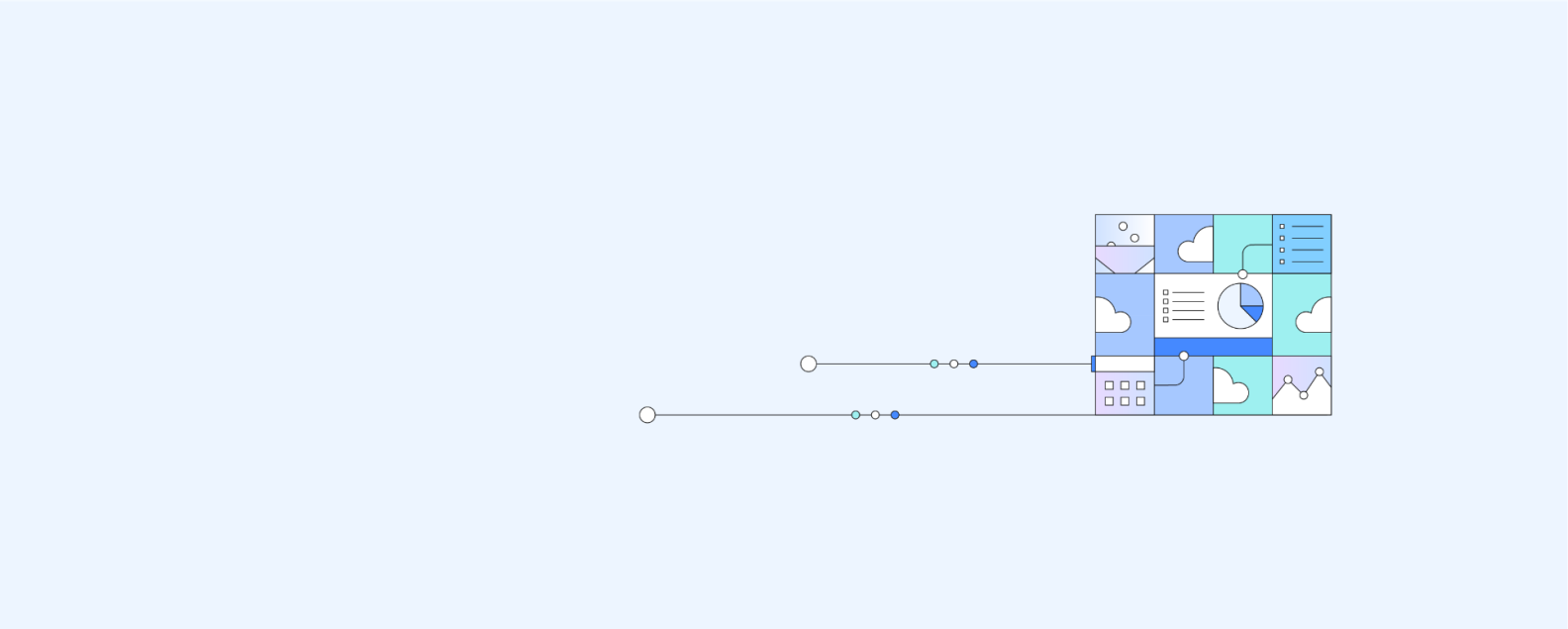
OLAP, or online analytical processing, is technology for performing high-speed complex queries or multidimensional analysis on large volumes of data in a data warehouse , data lake or other data repository. OLAP is used in business intelligence (BI) , decision support, and a variety of business forecasting and reporting applications.
Most business data have multiple dimensions—multiple categories into which the data are broken down for presentation, tracking, or analysis. For example, sales figures might have several dimensions related to location (region, country, state/province, store), time (year, month, week, day), product (clothing, men/women/children, brand, type), and more.
But in a data warehouse or data lake, data sets are stored in tables, each of which can organize data into just two of these dimensions at a time. OLAP extracts data from multiple relational data sets and reorganizes it into a multidimensional format that enables very fast processing and very insightful analysis.
Explore the free O'Reilly ebook to learn how to get started with Presto, the open source SQL engine for data analytics.
Register for the IDC report
The core of most OLAP systems, the OLAP cube is an array-based multidimensional database that makes it possible to process and analyze multiple data dimensions much more quickly and efficiently than a traditional relational database .
A relational database table is structured like a spreadsheet, storing individual records in a two-dimensional, row-by-column format. Each data “fact” in the database sits at the intersection of two dimensions–a row and a column—such as region and total sales.
SQL and relational database reporting tools can certainly query, report on, and analyze multidimensional data stored in tables, but performance slows down as the data volumes increase. And it requires a lot of work to reorganize the results to focus on different dimensions.
This is where the OLAP cube comes in. The OLAP cube extends the single table with additional layers, each adding additional dimensions—usually the next level in the “concept hierarchy” of the dimension. For example, the top layer of the cube might organize sales by region; additional layers could be country, state/province, city and even specific store.
In theory, a cube can contain an infinite number of layers. (An OLAP cube representing more than three dimensions is sometimes called a hypercube.) And smaller cubes can exist within layers—for example, each store layer could contain cubes arranging sales by salesperson and product. In practice, data analysts will create OLAP cubes containing just the layers they need, for optimal analysis and performance.
The drill-down operation converts less-detailed data into more-detailed data through one of two methods—moving down in the concept hierarchy or adding a new dimension to the cube. For example, if you view sales data for an organization’s calendar or fiscal quarter, you can drill-down to see sales for each month, moving down in the concept hierarchy of the “time” dimension.
Roll up is the opposite of the drill-down function—it aggregates data on an OLAP cube by moving up in the concept hierarchy or by reducing the number of dimensions. For example, you could move up in the concept hierarchy of the “location” dimension by viewing each country's data, rather than each city.
Slice and dice
The slice operation creates a sub-cube by selecting a single dimension from the main OLAP cube. For example, you can perform a slice by highlighting all data for the organization's first fiscal or calendar quarter (time dimension).
The dice operation isolates a sub-cube by selecting several dimensions within the main OLAP cube. For example, you could perform a dice operation by highlighting all data by an organization’s calendar or fiscal quarters (time dimension) and within the U.S. and Canada (location dimension).
The pivot function rotates the current cube view to display a new representation of the data—enabling dynamic multidimensional views of data. The OLAP pivot function is comparable to the pivot table feature in spreadsheet software, such as Microsoft Excel, but while pivot tables in Excel can be challenging, OLAP pivots are relatively easier to use (less expertise is required) and have a faster response time and query performance.
OLAP that works directly with a multidimensional OLAP cube is known as multidimensional OLAP, or MOLAP . Again, for most uses, MOLAP is the fastest and most practical type of multidimensional data analysis.
However, there are two other types of OLAP which may be preferable in certain cases:
ROLAP , or relational OLAP, is multidimensional data analysis that operates directly on data on relational tables, without first reorganizing the data into a cube.
As noted previously, SQL is a perfectly capable tool for multidimensional queries, reporting, and analysis. But the SQL queries required are complex, performance can drag, and the resulting view of the data is static—it can't be pivoted to represent a different view of the data. ROLAP is best when the ability to work directly with large amounts of data is more important than performance and flexibility.
HOLAP , or hybrid OLAP , attempts to create the optimal division of labor between relational and multidimensional databases within a single OLAP architecture. The relational tables contain larger quantities of data, and OLAP cubes are used for aggregations and speculative processing. HOLAP requires an OLAP server that supports both MOLAP and ROLAP.
A HOLAP tool can "drill through" the data cube to the relational tables, which paves the way for quick data processing and flexible access. This hybrid system can offer better scalability but can't escape the inevitable slow-down when accessing relational data sources. Also, its complex architecture typically requires more frequent updates and maintenance, as it must store and process all the data from relational databases and multidimensional databases. For this reason, HOLAP can end up being more expensive.
Online transaction processing , or OLTP , refers to data-processing methods and software focused on transaction-oriented data and applications.
The main difference between OLAP and OLTP is in the name: OLAP is analytical in nature, and OLTP is transactional.
OLAP tools are designed for multidimensional analysis of data in a data warehouse, which contains both transactional and historical data. In fact, an OLAP server is typically the middle, analytical tier of a data warehousing solution. Common uses of OLAP include data mining and other business intelligence applications, complex analytical calculations, and predictive scenarios, as well as business reporting functions like financial analysis, budgeting, and forecast planning.
OLTP is designed to support transaction-oriented applications by processing recent transactions as quickly and accurately as possible. Common uses of OLTP include ATMs, e-commerce software, credit card payment processing, online bookings, reservation systems, and record-keeping tools.
For a deep dive into the differences between these approaches, check out " OLAP vs. OLTP: What's the Difference? "
OLAP enables companies to maximize the potential of their corporate data by transforming it into the most practical format for multidimensional analysis. This, in turn, makes it easier to discern valuable business insights. However, if these systems are kept in-house, it limits the potential for scaling.
Cloud-based OLAP services are less expensive and easier to set up, making them more attractive for small businesses or startups on a budget. Enterprises can tap into the vast potential of cloud-based data warehouses that perform sophisticated analytics at unrivaled speeds because they use massively parallel processing (MPP). Therefore, companies can use OLAP at cloud speed and scale, analyzing vast amounts of data without moving it from their cloud data warehouse.
Constance Hotels, Resorts & Golf is a luxury hotel group with nine properties on islands in the Indian Ocean. However, a lack of island-to-island communications gave way to organizational silos, with business data isolated in each resort. The organization built a cloud data warehouse and analytics architecture to link all on-premises systems and tools with a central cloud-based data repository. In doing this, the company gained the group-wide insight they needed to leverage advanced, predictive analytics and implement an OLAP system.
OLAP in cloud architecture is a fast and cost-effective solution built for the future. Once the cubes are made, teams can use existing business intelligence tools to instantly connect with the OLAP model and draw interactive real-time insights from their cloud data.
IBM Db2 Warehouse on Cloud is a managed public cloud service. You can set up IBM Db2 Warehouse on premises with your own hardware or in a private cloud.
IBM DB2 Warehouse integrates and simplifies the data warehouse environment delivering dynamic warehousing and providing direct support for OLAP against the data warehouse.
Accelerate innovation and drive business outcomes by turning data into insights.
These terms are often confused for one another, so what are their key differences and how do you choose the right one for your situation?
Scale AI workloads for all your data, anywhere, with IBM watsonx.data, a fit-for-purpose data store built on an open data lakehouse architecture.
The General Ledger cubes delivered immediate benefits. ABC was using OSAS accounting software. They were not satisfied with the reports that OSAS produced, but was reluctant to invest an estimated $200K to acquire a new package and train accounting personnel to use it. Instead, they purchased an ODBC driver to export OSAS data and we built a cube to generate their reports. Today, their Balance Sheets and Profit and Loss Statements are implemented in an account rollup dimension. They can drill down from a few lines at the top to any level of detail. The drill-down feature is particularly useful in the GL Budget cube. If budget variances are detected at the highest levels, they just double-click on their OLAP pivot table to drill down until they discover the roots of the variance. The OLAP accounting reports reduced the time required to close ABC's books by 5 days. As a result, they can make critical business decisions that much faster.
Meanwhile, ABC's impressive performance attracted outside investors. A venture capital firm became the primary suitor and a team of business analysts set out to understand ABC's business. After exhaustive due diligence, the VCs decided to invest $167,000,000. They did so because ABC has a rock solid business. But, the deal might not have happened without the OLAP cubes. The OLAP cubes answered due diligence questions more quickly and in much more detail than the VC had seen in previous deals. The Billing cube that we developed at the VC's request was fundamental to their belief that future revenues would grow fast enough to support the necessary ROI.
Academia.edu no longer supports Internet Explorer.
To browse Academia.edu and the wider internet faster and more securely, please take a few seconds to upgrade your browser .
Enter the email address you signed up with and we'll email you a reset link.
- We're Hiring!
- Help Center
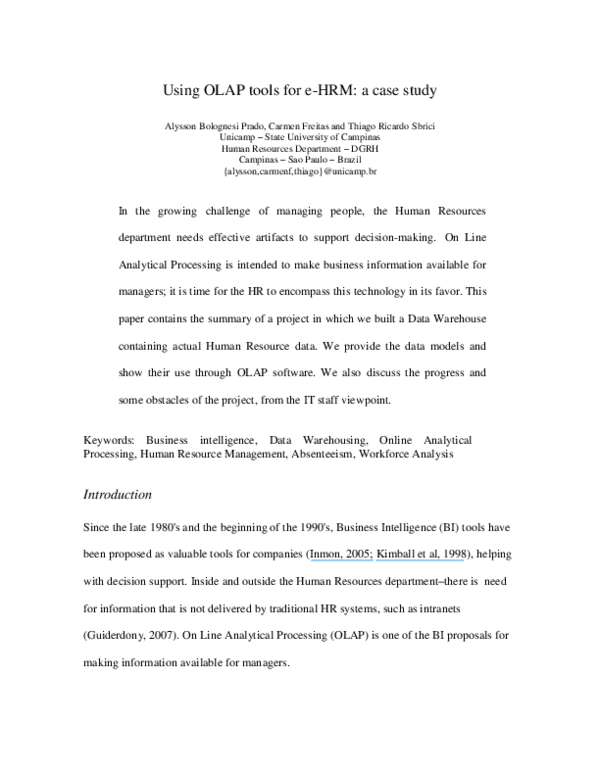
Using OLAP Tools for e-HRM: A Case Study

Related Papers
Alaa Hamoud
The organizations always need to manage their operations, process the data electronically, and find a platform that help them to support their strategic decisions. The success of the human resource department can reflect overall organizational success. The human resource department professionals try to ensure finding the right person at the right time for the job that fits the person according to skills and qualifications. This task needs a platform that supports making the right decision based on historical managerial information. The data mart is a departmental based decision support system that used departmental data to help decision-makers to support short term decisions. Human resource (HR) data mart is the base stone for building an enterprise data warehouse. The paper presents the implementation process of HR data mart starting from implementing data mart schema to online analytical processing (OLAP) reports. The data mart is implemented on retired employees' data of Basra Oil Company for over 15 years. A human resource data mart can provide a base platform to perform a different analysis operation to support the right decisions. Different OLAP reports are implemented to help analysts and decision-makers to get the answers for their questions as OLAP queries. Two categories of reports are implemented offline reports using Microsoft Excel Pivot Table 2010 and web OLAP reports using SQL Server Reporting Service 2014 (SSRS). The tools used to implement data mart vary from SQL Server Management Services (SSMS) 2014, SQL Server Integration Service 2014 (SSIS), SQL Server Analytical Service 2014 (SSAS), SQL Server Reporting Service 2014 (SSRS), SQL Server Data Tools 2013 (SSDT), and Microsoft Excel Pivot Table 2010.
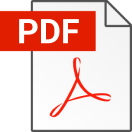
Global Trends in Human Resource Management
Yitzhak Fried
Emmanuel C Navarro
This study focused on the system development and assessment of a “Human Resource Information Management System.” It aimed to describe its developments using the Agile Software Development Method in terms of analysis, design, development, testing and evaluation. It also aimed in assessing its system qualities in terms of technicality, functionality and usability both by IT experts and HR practitioners as end-users based on the ISO/IEC 25010:2011 standards. The system was developed either for public or private organization. This study was conceptualized to further enhance a system that will consolidate the employees’ records. Its functionalities included the maintenance of employees’ records, attendance and leave monitoring, class schedules, related to work email messages notification for employees, ranking and evaluation, reduce paper management of employee’s records, generate service records, generate a pre-formatted PDS records compliant to recent Civil Service Commission format an...
IAEME PUBLICATION
IAEME Publication
In the onset of 21 st century, every day we witness change and up gradation of skills in masses. Human resources today lay stress on aligning human resource management with and organizations overall business goals and objectives. Human resources today combine itself with Artificial Intelligence to eliminate repetitive tasks, accelerate the search for talent, reduce employee attrition and improve employee engagement Human resource management is the most important component for a business or an organization to be successful. Human resource management role is to recruit, coordinate and train people in such a strategic way so as to get the maximum advantage from their work related activities. The human resource professionals are type of middleman in a business system who advises the managers and the management on issues related to assigning employees different responsibilities in an organization so that effective utilization of resources can be there. In an organization employees are often shifted from one department to another taking into consideration their skill factor. Over the past 3 decades there has been a tremendous change in Human Resource Management under the influence of new technology. HR processes have changed the way in which how companies collect, store, use and circulate information about employees. The process of recruitment has seen an exhaustive change , gone are the days when job boards and print media was used , electronic recruiting is the new trend in technology leading to qualified, diverse and motivated applicant job pool. With the help of e-recruitment HR gets more variety of employees to choose from. More than 90% of the large companies today are using more than one form of technology to advertise jobs. This current mentioned trend gives birth to a more advanced technology which helps us select the best suited applicant from the variety gathered with the help of e-recruitment i.e Artificial intelligence.
The paper presents a solution for integrating many aspects of human resource management, with extensible customization possibility (language, interface), to meets specific needs of various organizations, both regarding the organization as a whole, but also in terms of different categories of users that can use the platform. It also provides an overview and perspective on using Open Source Software in human resource management.
Sergey Zykov
Human resources management systems are having a wide audience at present. However, no truly integrate solution has been proposed yet to improve the systems concerned. Exist- ing approaches classification attempt is made in this paper. Possible approaches to extra data collection for decision- making are considered including psychological testing and fixed assets information as well as product sales data. Con-
International Journal of Human Resource Studies
Dr. Puja Sareen
Today, HR is not treated as a single function. It’s a collection of highly specialized capabilities — each with distinct objectives, tasks and needs. There is an ever-increasing pressure on Human Resource (HR) function to support strategic goals and to focus on value adding activities. Organizations have realized the growing importance of using Information Technology (IT) in leveraging their Human Resource (HR) functions. This takes the form of e-HRM (Electronic Human Resource Management). The e-HRM revolution relies on cutting-edge information technology, ranging from Internet-enabled human resources information systems (HRIS) to corporate intranets and portals. The driving forces are intensifying competition, need to manage workforce on a global level, to improve HR service delivery and to bring cost savings. e-HRM enables HR leaders to become architects in the development of competitive organizational social systems.This paper reviews the research work done in the field of e-HRM....
The days of campus selection, scanning job boards, advertising, open houses for recruitment are obsolete. Organizations are not gaining any competitive advantage or these methods covering and expressing future talent demands. To overcome this obsolete trend, "People Analytics" in the age of 'Big Data' is doing a great job of melding analytical process and methods with the large data that is available today. Big data is an ocean in which diving with predictive analytics, fishing gear virtually ensure that we will catch what you are fishing for. There is no shortage of qualified personnel in any company. But, we what we require is a world-class talent acquisition system. This is where predictive analysis system comes to our rescue. If we observe, there is a shortage of 21st-century talent acquisition strategies. Mere recruitment doesn't mean the selection of qualified and talented personnel. The employment methods, by whatever labels in vogue, are simply functional tools. It is observed that for decades, the organizational purpose of recruitment is often not fully addressed. Everything starts with the organization's purpose, goals and strategic plans. Management must first be clear, that, data and analytics can be brought together in forming a strategy. As we know, Big data is the talent ocean, Analytics is the fishing gear. Analytics help management, find the school of a It is the era to be motivated to learn some of the latest techniques and best practices of how to use different types of human resource across the enterprise. In this context, it is imperative to study the 'People Analytics' in the age of Big Data. This paper focuses on the international experiences on People Analytics from select countries and its growing relevance in HR of select companies in India.
Sergio Luján-Mora , Marek Miłosz
Advances in Science, Technology and Engineering Systems Journal
Chelsea Adora
RELATED TOPICS
- We're Hiring!
- Help Center
- Find new research papers in:
- Health Sciences
- Earth Sciences
- Cognitive Science
- Mathematics
- Computer Science
- Academia ©2024
Visualization and Analyses of Multi-Dimensional Data Sets Using OLAP- A Case Study of a Student Administration System
- Conference paper
- First Online: 01 January 2009
- Cite this conference paper
- Che-Chern Lin 4 ,
- Fun-Chi Hsu 4 ,
- Chun-Hung Wu 4 ,
- Chia-Jui Hsu 4 ,
- Chia-Hsun Wu 4 ,
- Chung-Ping Lee 4 &
- Hung-Jen Yang 4
Part of the book series: Lecture Notes in Electrical Engineering ((LNEE,volume 28))
582 Accesses
In this paper, we introduce the visualization and analyses of multidimensional data sets using on-line analytic processing (OLAP). We discuss three fact table schemas for building data cubes for displaying different views and the levels of details of date sets Five operations of OLAP are used for manipulating data cubes including slice, dice, roll-up, drill-own, and rotation operations. By the five operations one can perform extraction, aggregation, sub-dividing, changing perspective of information stored in OLAP data cubes. We present a case study to explain the above operations. In the proposed case study, a student administration system was developed. To build data cubes, we established a constellation schema consisting of three fact tables and four dimension tables. We also demonstrate the five operations on the data cubes and discuss how these operations are applied to analyze data.
This is a preview of subscription content, log in via an institution to check access.
Access this chapter
- Available as PDF
- Read on any device
- Instant download
- Own it forever
- Available as EPUB and PDF
- Compact, lightweight edition
- Dispatched in 3 to 5 business days
- Free shipping worldwide - see info
- Durable hardcover edition
Tax calculation will be finalised at checkout
Purchases are for personal use only
Institutional subscriptions
Liu Y, Sung SY, Xiong H (2000) A cube-wise balance approach for privacy preservation in data cubes. Inf Sci 176:1215–1240
Article Google Scholar
Shi Z, Huang Y, He Q, Xu L, Liu S, Qin L, Jia Z, Li J, Huang H, Zhao L (2007) MSMiner- a developing platform for OLAP. Decis Support Syst 42:2016–2028
Prat N, Akoka J, Comyn-Wattiau I (2006) A UML-based data warehouse design method. Decis Support Syst 42:1449–1473
Ahmad I, Azhar S, Lukauskis P (2004) Development of a decision support system using data warehousing to assist builders/developers in site selection. Autom Constr 13:525–542
Moon SW, Kim JS, Kwon KN (2007) Effectiveness of OLAP-based cost data management in construction cost estimate. Autom Constr 16:336–344
Roiger RJ, aGeatz MW (2003) Data mining: A tutorial-based primer. Addsion Welsley, Pearson Education, Inc., Chapter 6
Google Scholar
Download references
Author information
Authors and affiliations.
National Kaohsiung Normal University, 116 Heping First Road, Kaohsiung City, Taiwan (R.O.C.)
Che-Chern Lin, Fun-Chi Hsu, Chun-Hung Wu, Chia-Jui Hsu, Chia-Hsun Wu, Chung-Ping Lee & Hung-Jen Yang
You can also search for this author in PubMed Google Scholar
Corresponding author
Correspondence to Che-Chern Lin .
Editor information
Editors and affiliations.
Military Institutes of University, Hellenic Naval Academy, Leoforos Chatzikyriakou, Piraeus, 185 39, Greece
Nikos Mastorakis
Dept. Theoretical Electrical, Technical University of Sofia, Kl. Ohridski St. 8, Sofia, 1000, Bulgaria
Valeri Mladenov
Roumelis 6, Vrilissia, 152 35, Greece
Vassiliki T. Kontargyri
Rights and permissions
Reprints and permissions
Copyright information
© 2009 Springer Science+Business Media, LLC
About this paper
Cite this paper.
Lin, CC. et al. (2009). Visualization and Analyses of Multi-Dimensional Data Sets Using OLAP- A Case Study of a Student Administration System. In: Mastorakis, N., Mladenov, V., Kontargyri, V. (eds) Proceedings of the European Computing Conference. Lecture Notes in Electrical Engineering, vol 28. Springer, Boston, MA. https://doi.org/10.1007/978-0-387-85437-3_57
Download citation
DOI : https://doi.org/10.1007/978-0-387-85437-3_57
Published : 28 February 2009
Publisher Name : Springer, Boston, MA
Print ISBN : 978-0-387-84818-1
Online ISBN : 978-0-387-85437-3
eBook Packages : Engineering Engineering (R0)
Share this paper
Anyone you share the following link with will be able to read this content:
Sorry, a shareable link is not currently available for this article.
Provided by the Springer Nature SharedIt content-sharing initiative
- Publish with us
Policies and ethics
- Find a journal
- Track your research
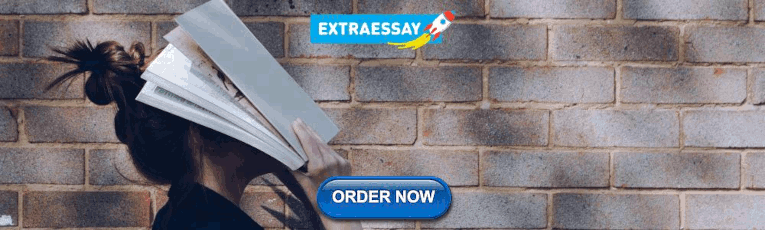
IMAGES
VIDEO
COMMENTS
Here is the list of OLAP operations: Roll-up, Drill-down, Slice and dice, Pivot (rotate). Roll-up: Roll-up performs aggregation on a data cube in any of the following ways: By climbing up a concept hierarchy for a dimension. By dimension reduction. The following diagram illustrates how roll-up works. 2.
This paper focuses the attention on a real-life case study represented by the design, the development and the practice of OLAP tools over big COVID-19 data in Canada. The OLAP tools developed in this context are further enriched by machine learning procedures that magnify the mining effect. The contribution presented in this paper also embeds an implicit methodology for OLAP over big COVID-19 ...
Supply chain analysis. In summary, OLTP systems are used for transactional processing, while OLAP systems are used for analytical processing. OLTP systems are optimized for fast read and write performance, while OLAP systems are optimized for fast query performance. Here is a list of some popular OLTP and OLAP tools:
Following our previous research results, in this paper we provide two authoritative application scenarios that build on top of OLAP*, a middleware for parallel processing of OLAP queries that truly realizes effective and efficiently OLAP over Big Data. We have provided two authoritative case studies, namely parallel OLAP data cube processing and virtual OLAP data cube design, for which we also ...
The illustration depicted in Fig. 19.1 shows a typical journey of data from an operational database, data warehousing and OLAP to data analytics. All of the previous chapters focus on the left-hand side, the transformation process from the operational database to the data warehouse, where we discuss in length the step-by-step transformation process of various cases, using extensive case studies.
OLAP and Big Data. A case study conducted by IBM reported that organizations implementing OLAP solutions experienced, on average, a 20% improvement in return on investment (ROI) within the first year. As organizations grapple with the influx of big data, OLAP systems must adapt to handle vast datasets. The intersection of OLAP and big data ...
Revising a dimension instance allows to produce consistent aggregations when an OLAP query is answered at more than one node. We then describe an implementation of a P2P system for answering OLAP queries over a network of data warehouses. We apply our proposal to a real-world case study of an insurance group. Finally, we report the results of ...
This paper describes a project in which the authors built a Data Warehouse containing actual Human Resource data. This paper provides data models and shows their use through OLAP software and their presentation to end-users using a web portal. The authors also discuss the progress, and some obstacles of the project, from the IT staff's viewpoint.
In this paper, we describe a framework for so-called exploratory OLAP over RDF sources. We propose a system that uses a multidimensional schema of the OLAP cube expressed in RDF vocabularies. Based on this information the system is able to query data sources, extract and aggregate data, and build a cube.
Modeling and tracking Covid-19 cases using Big Data analytics on HPCC system platform. The development of a model of Corona spread is described, using innovative big data analytics techniques and tools, which suggests that demand for advanced medical services might apply to only 20 % of the total infected. Expand.
Case Studies: (Bringing Concepts to Life) Imagine you're managing your personal finances using a banking app. Every day, you check your account balance, transfer funds between accounts, and pay ...
3) OLAP operations. In the multidimensional model, data are organized into. multiple dimensions. And each dimension contains multiple. levels of abstraction defined by concept hierarchies. Using ...
3.1. P2P OLAP overview. The model proposed in [2] considers each node as a peer in a cooperative query system, and allows each peer a high degree of autonomy. Each node involved in the system defines a context where it becomes the local peer, and all its dimensions and fact tables are considered local henceforth.
An attempt has been made to design of data warehouse, the proposed system constitutes an integrated platform for a thorough analysis of student's past nine years data and has been achieved with online analytical processing OLAP operations. Educational data is incremental in nature. The large amount of data in educational institutions is generated in the form of personal information, academic ...
The core of most OLAP systems, the OLAP cube is an array-based multidimensional database that makes it possible to process and analyze multiple data dimensions much more quickly and efficiently than a traditional relational database.. A relational database table is structured like a spreadsheet, storing individual records in a two-dimensional, row-by-column format.
analysis to stale and possibly irrelevant data. In this paper, we. present Lineage-based Data Store (L-Store) that combines the real-. time processing of transactional and analytical workloads ...
The Data tier aims to store multidimensional data, and it is implemented using Relational DBMS Postgres. The OLAP tier is implemented using the OLAP server Mondrian, where the cubes are defined. The Explorer tier is composed of a Cube exploratory tool, which allows the decision makers to explore the constructed cubes and visualise their instances.
If you are familiar with Excel, it suffices to say that OLAP transforms a relational database into a pivot table. 1. ABC already owned Microsoft SQL Server licenses and appreciated its ease of use and administration. 2. Microsoft has bundled Analysis Services with every copy of SQL Server since 1998.
By using OLAP for data analysis, retail businesses can gain the insights they need to understand their customers better, optimize their operations, and ultimately, drive greater success.
Several literary studies [2, 34], which address decision support systems (DSS), use the OLAP query language (OnLine Analytical Processing) for analytical real-time processing of large amounts of ...
Using OLAP tools for e-HRM: a case study Alysson Bolognesi Prado, Carmen Freitas and Thiago Ricardo Sbrici Unicamp - State University of Campinas Human Resources Department - DGRH Campinas - Sao Paulo - Brazil {alysson,carmenf,thiago}@unicamp.br In the growing challenge of managing people, the Human Resources department needs effective ...
information available from various databases. This study reports a case of implementing an OLAP tool to build complex financial reports for the use of senior management. The case illustrates the importance of the IS role of accountants with the emergence of the "systems accounting" role and the benefits of OLAP to accountants. Introduction
In this paper, we introduce the visualization and analyses of multidimensional data sets using on-line analytic processing (OLAP). We discuss three fact table schemas for building data cubes for displaying different views and the levels of details of date sets Five operations of OLAP are used for manipulating data cubes including slice, dice, roll-up, drill-own, and rotation operations.