- Reference Manager
- Simple TEXT file
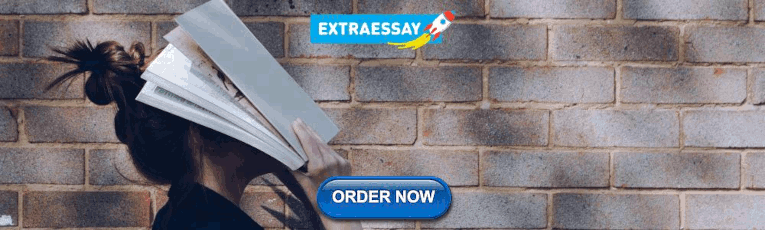
People also looked at
Original research article, linguistic variation and change in 250 years of english scientific writing: a data-driven approach.
- 1 Language Science and Technology, Saarland University, Saarbrücken, Germany
- 2 Digital Linguistics, Institut für Deutsche Sprache, Mannheim, Germany
We trace the evolution of Scientific English through the Late Modern period to modern time on the basis of a comprehensive corpus composed of the Transactions and Proceedings of the Royal Society of London, the first and longest-running English scientific journal established in 1665. Specifically, we explore the linguistic imprints of specialization and diversification in the science domain which accumulate in the formation of “scientific language” and field-specific sublanguages/registers (chemistry, biology etc.). We pursue an exploratory, data-driven approach using state-of-the-art computational language models and combine them with selected information-theoretic measures (entropy, relative entropy) for comparing models along relevant dimensions of variation (time, register). Focusing on selected linguistic variables (lexis, grammar), we show how we deploy computational language models for capturing linguistic variation and change and discuss benefits and limitations.
1. Introduction
The language of science is a socio-culturally firmly established domain of discourse that emerged in the Early Modern period (ca. 1500–1700) and fully developed in the Late Modern period (ca. 1700–1900). While considered fairly stable linguistically (cf. Görlach, 2001 ; Leech et al., 2009 ), the Late Modern period is a very prolific time when it comes to the formation of text types, with many of the registers we know today developing during that period—including the language of science (see Görlach, 2004 for a diachronic overview).
Socio-culturally, register diversification is connected to the growing complexity of modern societies, labor becoming increasingly divided with more different and increasingly specialized activities across all societal sectors 1 . Also, driven by science as well as early industry, standardization (e.g., agreements on weights and measures) and routinization of procedures become important issues. At the same time, enlightenment and the scientific and industrial revolutions support a general climate of openness and belief in technological advancement. In the domain of science, the eighteenth century is of course the epoch of encyclopedias 2 but also that of the scientific academies which promoted the scientific method and distributed scientific knowledge through their publications. The two oldest scientific journals are the French Journal des Sçavans and the Philosophical Transactions of the Royal Society of London . At the beginning of publication (both started in 1665), the journals were no more than pamphlets and included articles written in the form of letters to the editor and reviews of scientific works ( Gleick, 2010 ). Professionalization set in around the mid eighteenth century, as witnessed by the introduction of a reviewing process in the Royal Society ( Moxham and Fyfe, 2018 ; Fyfe et al., 2019 ).
While there is a fair stock of knowledge on the development of scientific language from socio-cultural and historical-pragmatic perspectives (see section 2), it is less obvious what are the underlying, more general principles of linguistic adaptation to new needs of expression in an increasingly diversified and specialized setting such as science. This provides the motivation for the present research. Using a comprehensive diachronic corpus of English scientific writing composed of the Philosophical Transactions and Proceedings of the Royal Society of London [henceforth: Royal Society Corpus (RSC); Kermes et al., 2016 ; Fischer et al., 2020 ], we trace the evolution of Scientific English looking for systematic linguistic reflexes of specialization and diversification, yielding a distinctive “scientific style” and forming diverse sublanguages (sublanguage of chemistry, physics, biology etc.). In terms of theory, our work is specifically rooted in register linguistics ( Halliday, 1985b ; Biber, 1988 ) and more broadly in theories of language use, variation and change that acknowledge the interplay of social, cognitive and formal factors (e.g., Bybee, 2007 ; Kirby et al., 2015 ; Aitchison, 2017 ; Hundt et al., 2017 ). While we zoom in on the language of science, we are ultimately driven by the more general questions about language change: What changes and how? What drives change? How does change proceed? What are the effects of change? Thus, we aim at general insights about the dynamics of language use, variation and change.
In a similar vein, the methodology we present can be applied to other domains and related analysis tasks as well as other languages. Overall, we pursue an exploratory, data-driven approach using state-of-the-art computational language models (ngram models, topic models, word embeddings) combined with selected information-theoretic measures (entropy, relative entropy) to compare models/corpora along relevant dimensions of variation (here: time and register) and to interpret the results with regard to effects on language system and use. Since the computational models we use are word-based, words act as the anchor unit of analysis. However, style is primarily indicated by lexico-grammatical usage, so we investigate both the lexical and the grammatical side of words. While we consider lexis and grammar as intricately interwoven, in line with various theories of grammar ( Halliday, 1985a ; Hunston and Francis, 2000 ; Goldberg, 2006 ), for expository purposes, we here consider the lexico-semantic and the lexico-grammatical contributions to change separately.
The remainder of the paper is organized as follows. We start with an overview of previous work in corpus and computational linguistics in modeling diachronic change with special regard to register and style (section 2). In section 3 we introduce our data set (section 3.1) and elaborate on the methods employed (section 3.3). Section 4 shows analyses of diachronic trends at the levels of lexis and grammar (section 4.1), the development of topics over time (section 4.2) and paradigmatic effects of changing language use (section 4.3). Finally, we summarize our main results and briefly assess benefits and shortcomings of the different kinds of models and measures applied to the analysis of linguistic variation and change (section 5).
2. Related Work
The present work is placed in the area of language variation and change with special regard of social and register variation and computational models of variation and change (for overviews see Aragamon, 2019 for computational register studies and Nguyen et al., 2016 for computational socio-linguistics).
Regarding the language of science, there is an abundance of linguistic-descriptive work, including diachronic aspects, providing many valuable insights (e.g., Halliday, 1988 ; Halliday and Martin, 1993 ; Atkinson, 1999 ; Banks, 2008 ; Biber and Gray, 2011 , 2016 ). However, most of the existing work is either based on text samples or starts from predefined linguistic features. Further, there are numerous studies on selected scientific domains, such as medicine or astronomy, e.g., Nevalainen (2006) ; Moskowich and Crespo (2012) and Taavitsainen and Hiltunen (2019) , which work on the basis of fairly small corpora containing hand-selected and often manually annotated material. Typically, such studies are driven from a historical socio-linguistic or pragmatic perspective and focus on selected linguistic phenomena, e.g., forms of address ( Taavitsainen and Jucker, 2003 ). For overviews on recent trends in historical pragmatics/socio-linguistics (see Jucker and Taavitsainen, 2013 ; Säily et al., 2017 ). Studies on specific domains, registers or text types provide valuable resources and insights into the socio-historical conditions of language use. Here, we build upon these insights, adding to it the perspective of general mechanisms of variation and change.
More recently, the diachronic perspective has attracted increasing attention in computational linguistics and related fields. Generally, diachronic analysis requires a methodology for comparison of linguistic productions along the time line. Such comparisons may range over whole epochs (e.g., systemic changes from early Modern English to Late Modern English), or involve short ranges (e.g., the issues of 1 year of The New York Times to detect topical trends). Applying computational language models to diachronic analysis requires a computationally valid method of comparison of language use along the time line, i.e., one that captures linguistic change if it occurs.
Different kinds of language models are suitable for this task and three major strands can be identified. First, a number of authors from fields as diverse as literary studies, history and linguistics have used simple ngram models to find trends in diachronic data using relative entropy (Kullback-Leibler Divergence, Jensen-Shannon Divergence) as a measure of comparison. For instance, Juola (2003) used Kullback-Leibler Divergence (short: KLD) to measure rate of linguistic change in 30 years of National Geographic Magazine. In more recent, large-scale analyses on the Google Ngram Corpus ( Bochkarev et al., 2014 ; Kim et al., 2014 ) analyze change in frequency distributions of words within and across languages. Specifically humanistic research questions are addressed by e.g., Hughes et al. (2012) who use relative entropy to measure stylistic influence in the evolution of literature; or Klingenstein et al. (2014) who analyze different speaking styles in criminal trials comparing violent with non-violent offenses; or Degaetano-Ortlieb and Teich (2018) applying KLD as dynamic slider over the time line of a diachronic corpus of scientific text.
Second, probabilistic topic models ( Steyvers and Griffiths, 2007 ) have become a popular means to summarize and analyze the content of text corpora, including topic shifts over time. In linguistics and the digital humanities, topic models have been applied to various analytic goals including diachronic linguistic analysis ( Blei and Lafferty, 2006 ; Hall et al., 2008 ; Yang et al., 2011 ; McFarland et al., 2013 ). Here again, a valid method of comparing model outputs along the time line has to be provided. In our work, we follow the approach proposed in Fankhauser et al. (2016) using entropy over topics as a measure to assess topical diversification over time.
Third, word embeddings have become a popular method for modeling linguistic change, with a focus on lexical semantic change (e.g., Hamilton et al., 2016 ; Dubossarsky et al., 2017 , 2019 ; Fankhauser and Kupietz, 2017 ). Word embeddings are weakly neural models that capture usage patterns of words and are used in a variety of NLP tasks. While well-suited to capture the summative effects of change (groups of words or whole vocabularies, see e.g., Grieve et al., 2016 ), the primary focus lies on lexis 3 . Other linguistic levels, e.g., grammar ( Degaetano-Ortlieb and Teich, 2016 , 2018 ; Bizzoni et al., 2019a ), collocations ( Xu and Kemp, 2015 ; Garcia and Garćia-Salido, 2019 ), or specific aspects of change, e.g., spread of change ( Eisenstein et al., 2014 ), specialization ( Bizzoni et al., 2019b ) or life-cycles of language varieties ( Danescu-Niculescu-Mizil et al., 2013 ), are only rarely considered. Once again, while word embeddings offer a specific model of language use, using them to capture diachronic change and to assess effects of change calls for adequate instruments for comparison along the time line. Here, we use the commonly applied measure of cosine distance for a general topological analysis of diachronic word embedding spaces; and we use entropy for closer inspection of specific word embeddings clusters to measure the more fine-grained paradigmatic effects of change.
In sum, in this paper we address some of the core challenges in modeling diachronic change by (a) looking at the interplay of different linguistic levels (here: lexis and grammar), (b) elaborating on the formation of style and register from a diachronic perspective, and (c) enhancing existing computational methods with explicit measures of linguistic change. Since we are driven by the goal of explanation rather than high-accuracy prediction (as in NLP tasks), qualitative interpretation by humans is an integral step. Here, micro-analytic and visual support are doubly important if one wants to explore linguistic conditions and effects of change. To support this, good instruments for human inspection and analysis of data are crucial—see, for instance, Jurish (2018) and Kaiser et al. (2019) providing visualization tools for various aspects of diachronic change, partly with interactive function ( Fankhauser et al., 2014 ; Fankhauser and Kupietz, 2017 ); or Hilpert and Perek (2015) 's application of motion charts to the analysis of meaning change. We developed a number of such visualization tools made available as web applications for inspection of the Royal Society Corpus (cf. section 3).
3. Data and Methods
The corpus used for the present analysis is the Royal Society Corpus 6.0 ( Fischer et al., 2020 ). The full version is composed of the Philosophical Transactions and Proceedings of the Royal Society from 1665 to 1996. In total, it contains 295,895,749 tokens and 47,837 documents. Here, we use a version that is open-source under a creative commons license covering the period of 1665 to 1920. In terms of periods of English, this reflects the Late Modern period (1700–1900) plus a bit of material from the last decades of the Early Modern period (before 1700) as well as a number of documents from modern English. Altogether this open version contains 78,605,737 tokens and 17,520 documents.
Note that the RSC is not balanced, later periods containing substantially more material than earlier ones (see Table 1 ), which calls for caution regarding frequency effects. Other potentially interesting features of the corpus are that the number of different authors increases over time; so does the number of papers with more than one author.
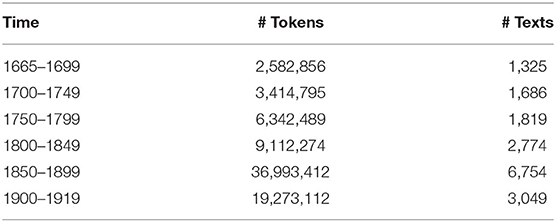
Table 1 . Size of RSC 6.0 by 50-year periods.
The documents in the corpus are marked up with meta-data including author, year of publication, text type and time period (1-, 10-, 50-year periods). The corpus is tokenized, lemmatized, annotated with part-of-speech tags and normalized (keeping both normalized and original word forms) using standard tools ( Schmid, 1995 ; Baron and Rayson, 2008 ). The corpus is made available under a Creative Commons license, downloadable and accessible via a web concordance (CQPWeb; Hardie, 2012 ) as well as interactive visualization tools 4 .
3.2. Methods
There are two important a priori considerations regarding modeling linguistic change and variation. First, one of the key concepts in language variation is use in context . Apart from extra-linguistic, situational context (e.g., field, tenor, and mode; Quirk et al., 1985 ), intra-linguistic context directly impacts on linguistic choice, both syntagmatically (as e.g., in collocations) and paradigmatically (i.e., shared context of alternative expressions). Different computational models take into account different types of context and accordingly reveal different kinds of linguistic patterns. Topic models take into account the distribution of words in document context and are suitable to capture the field of discourse (see section 3.2.2 below). Plain ngram models take into account the immediately preceding words of a given word and can reveal syntagmatic usage patterns (see section 3.2.1 below). Word embeddings take into account left and right context (e.g., ± five words) and allow clustering words together depending on similar, surrounding contexts; thus, they are suited for capturing linguistic paradigms (see section 3.2.3 below).
Second, diachronic linguistic analysis essentially consists of comparison of corpora representing language use at different time periods. Computational language models being representations of corpora, the core task consists in comparing model outputs and elicit significant differences between them. Common measures of comparing language models are perplexity and relative entropy, typically used for assessing the quality or fit of a model by estimating the difference between models in bits using a log base. Here, we use the asymmetric version of relative entropy, Kullback-Leibler Divergence, to assess differences between language models according to time. An intimately related measure is entropy. Entropy considers the richness and (un)evenness of a sample and is a common means to measure diversity, e.g., the lexical diversity of a language sample ( Thoiron, 1986 ). Here, we use entropy as a measure of diversification at two levels, the level of topics (field of discourse) and the level of paradigmatic word clusters, where greater entropy over time is interpreted as a signal of linguistic diversification and lower entropy as a signal of consolidated language use. The most basic way of exploring change in a given data set is to test whether the entropy over a simple bag-of-words model changes or not. For diversification to hold, we would expect the entropy to rise over time in the RSC, also because of the increase in size of the more recent corpus parts as well as in number of authors. As will be seen, this is not the case, entropy at this level being fairly stable (section 4.2).
3.2.1. Ngram Based Models
To obtain a more fine-grained and linguistically informed overview of the overall diachronic tendencies in the RSC than possible with token ngrams, we consider lexical and grammatical usage separately using lemmas and part-of-speech (POS) sequences as modeling units. On this basis, models of different time periods (e.g., decades) are compared with the asymmetric variant of relative entropy, Kullback-Leibler Divergence (KLD; Kullback and Leibler, 1951 ); cf. Equation (1) where A and B here denote different time periods.
KLD is a common measure for comparing probability distributions in terms of the number of additional bits needed for encoding when a non-optimal model is used. Applied to diachronic comparison, we obtain a reliable index of difference between two corpora A and B: the higher the amount of bits, the greater the diachronic difference. Also, we know which specific units/features contribute to the overall KLD score by their pointwise KLD. Thus, we can inspect particular points in time (e.g., by ranking features by pointwise KLD in 1 year) or time spans (e.g., by standard deviation across several years) to dynamically observe changes in a feature's contribution. This gives us two advantages over traditional corpus-based approaches: no predefined features are needed and results are more directly interpretable.
Apart from comparing predefined time periods with each other as is commonly done in diachronic corpus-linguistic studies (cf. Nevalainen and Traugott, 2012 for discussion), KLD can be used as a data-driven periodization technique ( Degaetano-Ortlieb and Teich, 2018 , 2019 ). KLD is dynamically pushed over the time line comparing past and future (or, as KLD is asymmetric, future vs. past). As we will show below, using KLD in this way allows detecting diachronic trends that are hard to see on a token level or with predefined, more coarse time periods. The granularity of diachronic comparison can be varied depending on the corpus and the analytic goal (year-, month-, day-based productions); again, no a priori assumptions have to be made regarding the concrete linguistic features involved in change other than selecting the linguistic level of comparison (e.g., lemmas, parts of speech). Hence, the method is generic and at the same time sensitive to the data.
3.2.2. Topic Models
To obtain a picture of the diachronic development in terms of field of discourse—a crucial component in register formation—we need to consider the usage of words in the context of whole documents. To this end, we use topic models. We follow the overall approach of applying topic models to diachronic corpora mapping topics to documents ( Blei and Lafferty, 2006 ; Steyvers and Griffiths, 2007 ; Hall et al., 2008 ; Yang et al., 2011 ; McFarland et al., 2013 ). The principle idea is to model the generation of documents with a randomized two-stage process: For every word w i in a document d select a topic z k from the document-topic distribution P ( z k | d ) and then select the word from the topic-word distribution P ( w i | z k ) . Consequently, the document-word distribution is factored as: P ( w i | d ) = ∑ k P ( w i | z k ) P ( z k | d ) . This factorization effectively reduces the dimensionality of the model for documents, improving their interpretability: Whereas P ( w i | d ) requires one dimension for each distinct word (tens of thousands) per document, P ( z k | d ) only requires one dimension for each topic (typically in the range of 20–100). To estimate the document-topic and topic-word distributions from the observable document-word distributions we use Gibbs-Sampling as implemented in MALLET 5 .
To investigate topical trends over time, we average the document-topic distributions for each year y :
where n is the number of documents per year.
For further interpretation, we cluster topics hierarchically on the basis of the distance 6 between their topic-document distributions (Equation 3).
Topics that typically co-occur in documents have similar topic-document distributions, and thus will be placed close in the cluster tree.
To assess diachronic diversification in discourse field as a central part of register formation, we measure the entropy over topics (cf. Equation 4), and the mean entropy of topic-word distributions per time period.
Note that all measures operate on relative frequencies per time period in order to control for the lack of balance in our data set (more recent periods contain considerably more data than earlier ones).
3.2.3. Word Embeddings
Word embeddings ( WE s) capture lexical paradigms, i.e., sets of words sharing similar syntagmatic contexts. Word embeddings build on the principle underlying distributional semantics that it is possible to capture important aspects of the semantics of words by modeling their context ( Harris, 1954 ; Lenci, 2008 ).
Here, we apply WE s diachronically to explore the overall development of word paradigms in our corpus with special regard to register/sublanguage formation as well as scientific style. Using the approach and tools provided by Fankhauser and Kupietz (2017) we compute WE s with a structured skip-gram approach ( Ling et al., 2015 ). This is a variant of the popular Word2Vec approach ( Mikolov et al., 2013 ). Word2Vec is a way of maximizing the likelihood of a word given its context, by training a d x V matrix where V is the vocabulary and d an arbitrary number of dimensions.
The goal of the algorithm is to maximize
where T is a text and c is the number of left and right context words to be taken into consideration. In short, the model tries to learn the probability of a word given its context, p ( w o | w i ). To this end, the model learns a set of weights that maximizes the probability of having a word in a given context. Such set of weights constitutes a word's embedding.
Usually, skip-gram considers a term's context as a bag-of-words. In Ling et al. (2015) 's variant, the order of the word context is also taken into consideration which is important to capture words with grammatical functions rather than lexical words only. For diachronic application, we calculate WE s per time period (e.g., 1-/10-/50-year periods), where the first period is randomly initialized, and each subsequent period is initialized by the model for its preceding period. Thereby, WE s are comparable across periods.
To perform analyses on our models, we then apply simple similarity measures commonly used in distributional semantics, where the similarity between two words is assessed by the cosine similarity of their vectors:
where w 1 and w 2 are the vectors of the two words taken into consideration, and | w | is a vector's norm. Alternatively, the semantic distance between words can be considered, which is the complement of their similarity:
To detect the semantic tightness or level of clustering of a group of words (how semantically similar they are), one can thus compute the average cosine similarity between all the words in a group of words:
where V (vocabulary) is the group of words taken into consideration. Reversely, it is possible to compute the average distance of a group of words from another group of words by iterating the sums on two different sets.
To detect semantic shifts over time, one of the simplest and most popular approaches is that of computing the change of the cosine similarity between a group of pre-defined words in a chronologically ordered set of WE spaces. As we will show, the WE space of the RSC as a whole expands over time. At the same time, it becomes more fragmented and specific clusters of words become more densely populated while others disappear. We base such observations on an analysis of the word embeddings' topology using cosine similarity as explained above as well as entropy. For example, since the period under investigation witnesses the systematization of several scientific disciplines, we are likely to observe a narrowing of the meaning of many individual words—mainly technical terms—which would push them further away from one another. Similarly, for specific WE clusters, we expect growth or decline, e.g., chemical terms explode in the late eighteenth century, pointing to the emergence of the field of chemistry with the associated technical language, or many Latin words disappear. Such developments can be measured by the entropy H ( P (.| w )) over a given cluster around word w , by estimating the conditional probability of words w i in the close neighborhood of word w as follows:
where w k ranges over all words (including w ) with sufficient similarity (e.g., >0.6) to w . The neighbors are weighted by their similarity to the given word, thus, a word with many near neighbors and rather uniform distribution has a large entropy, indicating a highly diversified semantic field.
4. Analyses
Our analyses are driven by two basic assumptions: register diversification (linguistic variation focused on field of discourse) and formation of “scientific style” (convergence on specific linguistic usages within the scientific domain). We carry out three kinds of analysis on the Royal Society Corpus showing these two major diachronic trends at the levels of lexis and grammar (section 4.1), development of topic over time (section 4.2) as well as paradigmatic effects (section 4.3).
4.1. Diachronic Trends in Lexis and Grammar
We trace the overall diachronic development in the RSC considering both lexical and grammatical levels. Lexis is captured by lemmas and grammar by sequences of three parts of speech (POS). Using the data-driven periodization technique described in section 3.2.1 based on KLD, we dynamically compare probability distributions of lemma unigrams and POS trigrams along the time line.
Figures 1A,B plot the temporal development for the lexical and the grammatical level, respectively. The black line visualizes relative entropy of the future modeled by the past, i.e., how well at a particular point in time the future can be modeled by a model of the past (here: 10 year slices). The gray line visualizes the reverse, i.e., how well the past is modeled by the future (again on 10-year slices). Peaks in the black line indicate changes in the future which are not captured by a model of the past, such as new terminology. Peaks in the gray line indicate differences from the opposite perspective, i.e., the future not encompassing the past, e.g., obsolete terminology. Troughs for both lines indicate convergence of future and past. A fairly persistent, low-level relative entropy indicates a period of stable language use.
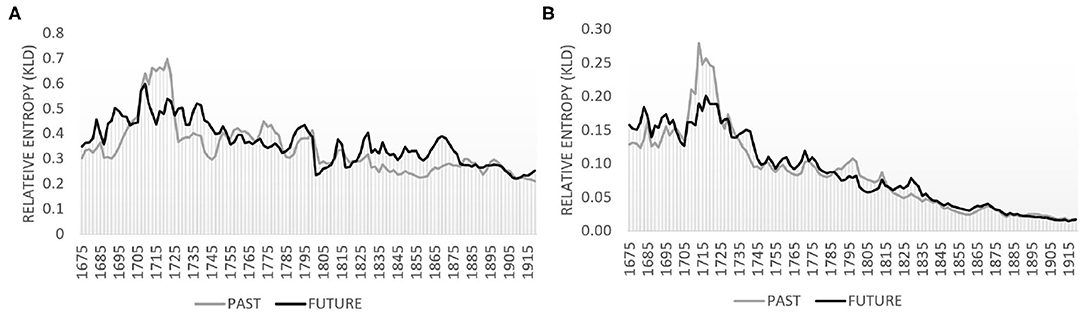
Figure 1 . Relative entropy based on lemmas and part-of-speech trigrams with 2-year slider and 10-year past and future periods. (A) Lemmas. (B) Part-of-speech trigrams.
Comparing the two graphs in Figure 1 , we observe a particularly strong decreasing tendency for the grammatical level (see Figure 1B ) and a slightly declining tendency at the lexical level with fairly pronounced oscillations of peaks and troughs ( Figure 1A ). Basically, peaks indicate innovative language use, troughs indicate converging use, the future being less and less “surprised” by the past. Thus, while grammatical usage consolidates over time, the lexical level is more volatile as it reacts directly to the pressure of expressing newly emerging things or concepts in peoples' (changing) domains of experience (here: new scientific discoveries). The downward trend at the grammatical level is a clear sign of convergence, possibly related to the formation of a scientific style; peaks at the lexical level signal innovative use and may indicate register diversification.
To investigate this in more detail, we look at specific lexical and grammatical developments. We use pointwise KLD (i.e., the contribution of individual features to overall KLD) to rank features. For example, there is a major increase in overall KLD around the 1790s at the lemma level. Considering features contributing to the highest peak in 1791 for the FUTURE model (black line), we see a whole range of words from the chemistry field around oxygen (see Figure 2 ). At the same time, we can inspect which features leave language use and contribute to an increase in KLD for the PAST model (i.e., features not well-captured by the future anymore). From Figure 3 , we observe words related to phlogiston and experiments with air contributing to the formation of the oxygen theory of combustion (represented by Lavoisier, Priestley as well as Scheele). In fact, the oxygen theory replaced Becher and Stahl's 100-years old phlogiston theory, marking a chemical revolution in the eighteenth century—it is this shift of scientific paradigm that we encounter here in the RSC.
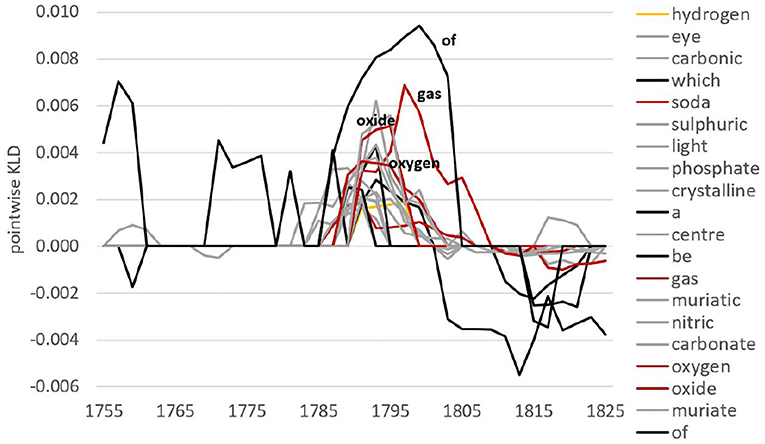
Figure 2 . Pointwise relative entropy based on lemmas for the FUTURE model in 1791.
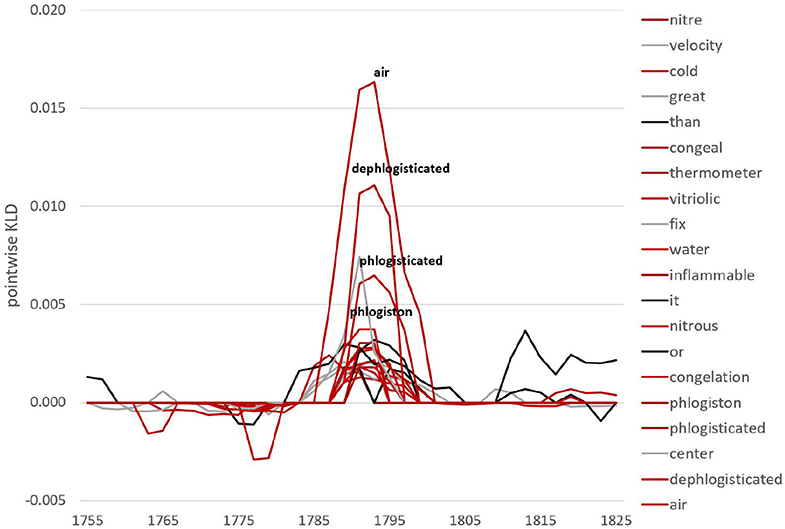
Figure 3 . Pointwise relative entropy based on lemmas for the PAST model in 1791.
At the grammatical level, after a fairly high KLD peak in the early 1700's, there is a step-wise, steady decrease with only local, smaller peaks. As an example of a typical development at the grammatical level consider the features involved in the 1771 peak (see Figure 4 ). These are passive voice and relational verb patterns (e.g., NOUN-BE-PARTICIPLE as in air is separated ; blue), nominal patterns with prepositions [e.g., indicating measurements such as the NOUN-PREPOSITION-ADJECTIVE as in the quantity of common (air) ; gray], gerunds (e.g., NOUN-PREPOSITION- ing VERB , such as method of making ; yellow), and relative clauses (e.g., DETERMINER-NOUN-RELATIVIZER , such as the air which/that ; red). While the contribution of these patterns to the overall KLD is high in 1771, it becomes zero for all of them by 1785—a clear indication of consolidation in grammatical usage pointing to the development of a uniform scientific style.
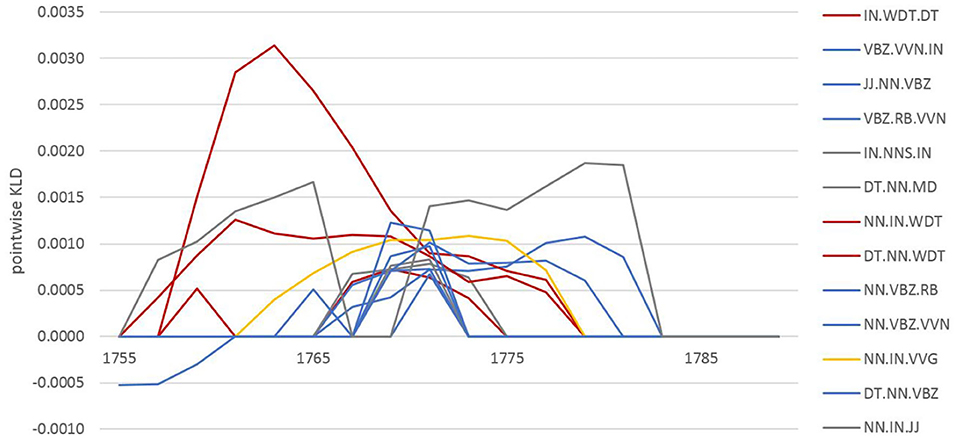
Figure 4 . Pointwise relative entropy based on POS trigrams for the PAST model in 1771.
Regarding the lexical level, to verify that the observed tendencies point to significant diversification effects, we need to explore the systematic association of words with discourse fields. For this, we turn to topic models.
4.2. Diachronic Development of Discourse Fields
To analyse the development of discourse fields over time as the core component in register diversification, we trained a topic model with 30 topics 7 . Stop words were excluded and documents were split into parts of at most 5000 tokens each to control for largely varying document lengths.
Table 2 shows four of the 30 topics with their most typical words. Note that topics do not only capture the field of discourse ( BIOLOGY 3 ) but also genre ( REPORTING ), mode ( FORMULAE ), or simply reoccurring boiler plate text ( HEADMATTER ).
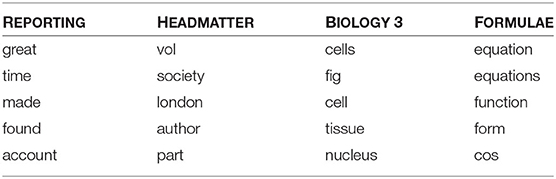
Table 2 . Top five words for selected topics.
Figure 5A displays the topic hierarchy resulting from clustering the topics based on the Pearson Distance between their topic-document distributions 8 . Labels for topics and topic clusters have been assigned manually, and redundant topics with very similar topic word distributions, such as BIOLOGY , have been numbered through.
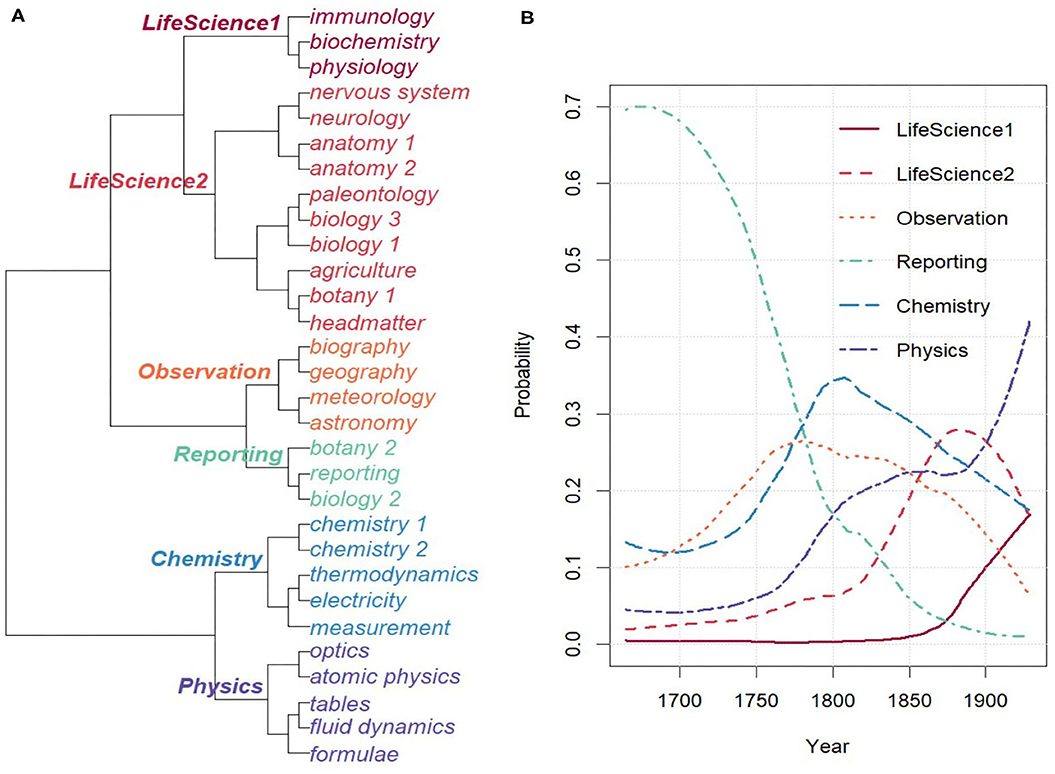
Figure 5 . Overview on topics. (A) Topic hierarchy. (B) Combined topics over time.
Figure 5B shows the probabilities of the combined topics over time. As can be seen, the first hundred years are dominated by the rather generic combined topic REPORTING , which covers around 70% of the topic space. Indeed, the underlying topic REPORTING makes for more than 50% of the topic space during the first 50 years. Starting in 1750, topics become more diversified into individual disciplines, indicating register diversification in terms of discourse field. In addition, in line with the analysis in section 3.1, we clearly see the rise of the CHEMISTRY topic around the 1790s.
As shown in Figure 6A diversification is evidenced by the clearly increasing entropy of the topic distribution over time. However, the mean entropy of the individual document-topic distributions remains remarkably stable, even though the mean number of authors per document and document length increase over time. Even the mean entropy weighted by document length (not shown) remains stable. This may be in part due to using asymmetric priors for the document-topic distributions, which generally skews them toward topics containing common words shared by many documents ( Wallach et al., 2009 ), thus stabilizing the document-topic distributions over time.
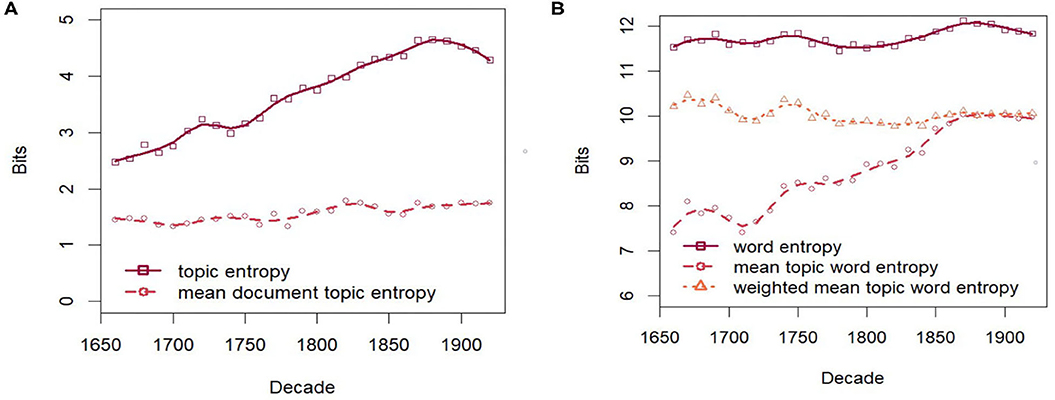
Figure 6 . Entropies over time. (A) Entropy of topics. (B) Entropy of words.
Figure 6B shows the diachronic development of entropies at the level of words. The overall entropy of the unigram language model as well as the mean entropy of the topic word distributions weighted by the topic probabilities are also remarkably stable. However, the (unweighted) mean entropy of topic word distributions clearly increases over time. Indeed, due to the fairly high correlation of 0.81 (Spearman) between topic probability and the topic word entropy, evolving topics with increasing probability also increase in their word entropy, i.e., their vocabulary becomes more diverse. Figure 7 demonstrates this for the evolving topics in the group LIFESCIENCE 2 . All topics increase over time both in probability and entropy 9 . As will be seen in section 4.3, this trend is mirrored in the analysis of paradigmatic word clusters by word embeddings.
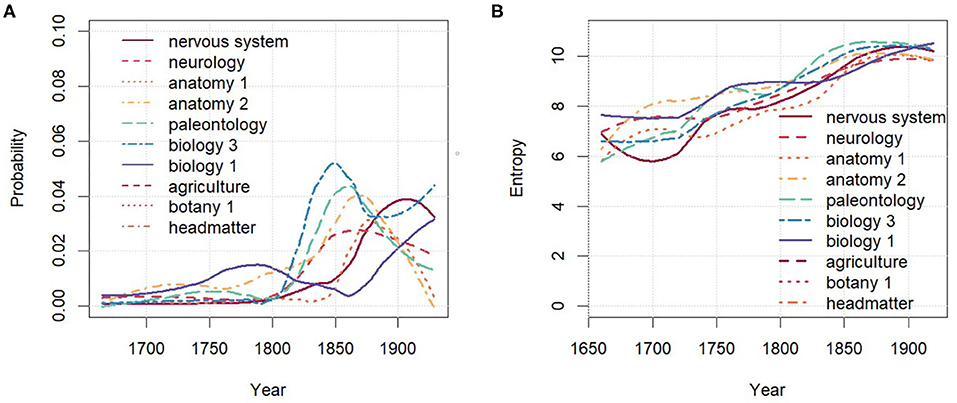
Figure 7 . LIFESCIENCE 2 over time. (A) Probability. (B) Entropy of topic word distributions.
4.3. Paradigmatic Effects
To gain insights into the paradigmatic effects of the diachronic trends detected by the preceding analyses, we need to consider word usage according to syntagmatic context. To capture grammatical aspects as well (rather than just lexical-semantic patterns), we take word forms rather than lemmas as a unit for modeling and we do not exclude function words.
Based on the word embedding model as shown in section 3.2.3, we observe that the word embedding space of the RSC grows over time both in terms of vocabulary size and in terms of average distance between words. While a growing vocabulary can be interpreted in many ways, it is more informative to look at the increase in average distance between words. Here, not every term grows apart from all other terms (in fact, many pairs of words get closer through time) but when we take two random terms the average distance between them is likely to increase—see Figure 8 : (A) shows the diachronic trend for the distance between 2,000 randomly selected pairs of words and (B) for the distance of 1,000 randomly selected words from the rest of the vocabulary. The words were selected among those terms that appear at least once in every decade. In both cases, the trend toward a growing distance is clearly visible.
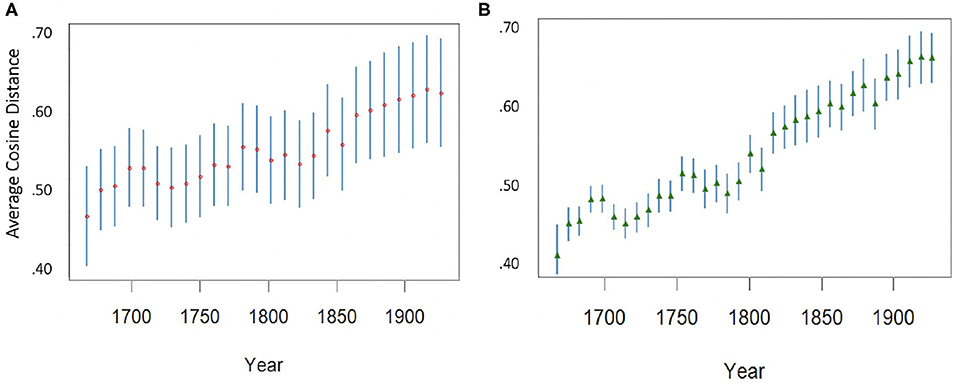
Figure 8. (A) Average distance and standard deviation of 2,000 randomly selected pairs of words. (B) Average distance from the whole vocabulary (mean and standard deviation) of 1,000 randomly selected words.
Given that WE s are based on similarity in context, this means that overall, words are used increasingly in different contexts, a clear sign of diversification in language use. For example, the usage of magnify and glorify diverges through the last centuries resulting in a meaning shift for magnify which becomes more associated with the aggrandizing effects of optical lenses while glorify remains closer to its original sense of elevating or making glorious. If we look for these two words in the WE space, what we see is, in fact, a progressive decrease of the distributional similarity between them: for example, in 1860 their cosine distance is 0.48, while in 1950 it has gone up to 0.62. The nature of their nearest neighbors also diverges: magnify increasingly shows specialized, optic-related neighborhoods ( blood-globule in 1730, object-lens in 1780, eyeglass in 1810) while the neighbors of glorify remain more mixed (mainly specific but non-technical verbs, such as bill, reread, ingratiate , with low similarity). Finally, their movement with respect to originally close neighbors is also consistent: e.g., the distance between glorify and exalt does not change between 1860 and 1920, while magnify appears to move away and back toward exalt through the decades and is more than 25 degrees further from it in 1920 than in 1670 (from 0.45 to 0.70).
To provide another example, a similar evolution is apparent for filling and saturating : their distance grows from 0.37 in 1700 to 0.65 in 1920, a difference of almost 30 degrees. In the same lapse of time, the distance between saturating and packing goes from 0.27 to 0.70. Actually, the meaning of saturating was originally closer to that of satisfying and packing : its usage as a synonym of imbuing , and its technical sense in chemistry are more recent, and have progressively drawn the word's usage apart from that of filling .
As noted above, we observe an overall expansion of the WE space. To test whether this expansion is not a simple effect of the increase of frequency and number of words in each decade, we select a set of function words which exhibit stable frequency and should not change in usage over time (e.g., the functions of the, and , and for did not change in the period considered). If the expansion we observe is due to raw frequency effects, function words should drift apart from each other at a similar rate as content words. This appears not to be the case. As shown in Table 3 , if we compare the group of function words to a group of randomly selected content words, such as verbs and nouns, we can see that the distances between the elements of such group grow much faster than the distances between function words. Purely functional words drift apart considerably less than words having a lexical meaning, indicating that the latter are probably causing most of the lexical expansion. Thus, words having a proper lexical meaning grow apart much faster on average than words having a purely functional role.
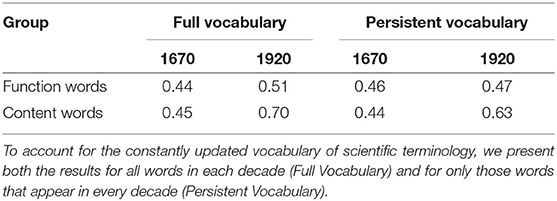
Table 3 . Average cosine distance between function words vs. 2,000 randomly selected content words in the first and last decade of RSC 6.0 Open.
This behavior is not consistent with a raw frequency effect, or with the side effects of changes in the magnitude of training data. It looks like the distributional profile of words is, on average, growing more distinct in this specific corpus. And this does not happen only for new vocabulary, created ad hoc for specific contexts: even when we factor out the changes in lexicon and we consider only those words that appear in every decade (Persistent Vocabulary in Table 3 ), the effect is still visible. This interpretation is supported when we inspect the entropy on specific WE clusters over time. We consider two cases: increasing and decreasing entropy on a cluster, the former signaling lexical diversification, the latter signaling converging linguistic usage. For instance, coming back to the field of chemistry, we observe increasing entropy in particular clusters of content words: see Figure 9 for an example, showing (A) relative frequency of selected terms denoting chemical compounds and (B) entropy on the WE cluster containing those terms (radius of cosine similarity > 0.6).
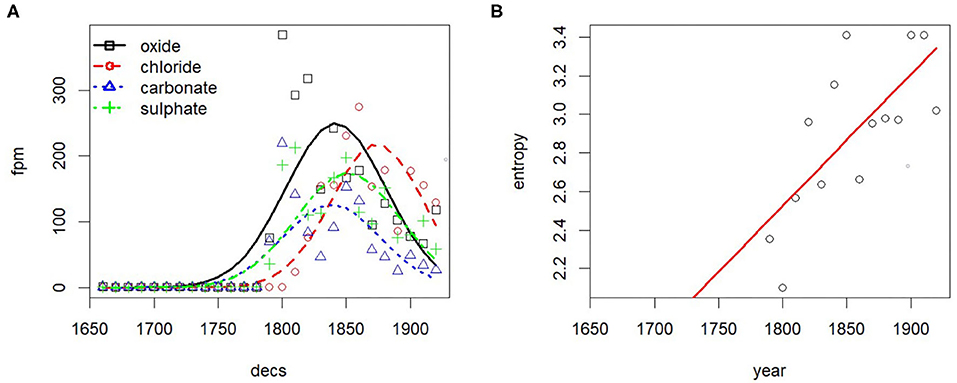
Figure 9 . Entropy increase on specific WE clusters signals terminological diversification. (A) Relative frequency. (B) Entropy.
As an example of the opposite trend, i.e., decreasing entropy, consider the use of ing -forms which diversify according to the analysis above shown for filling and saturating , i.e., they spread to different syntagmatic contexts. In the example in Figure 10 , the terms in the cluster containing assuming exhibit a skewed frequency over time with decreasing entropy, reflecting in this case stylistic convergence, i.e., the tacit agreement on using particular linguistic forms rather than others. In particular, assuming has 30 close neighbors (including supposing, assume, considering ) in the first decade, but only 13 close neighbors in the last decade, with assuming, assume dominating by frequency.
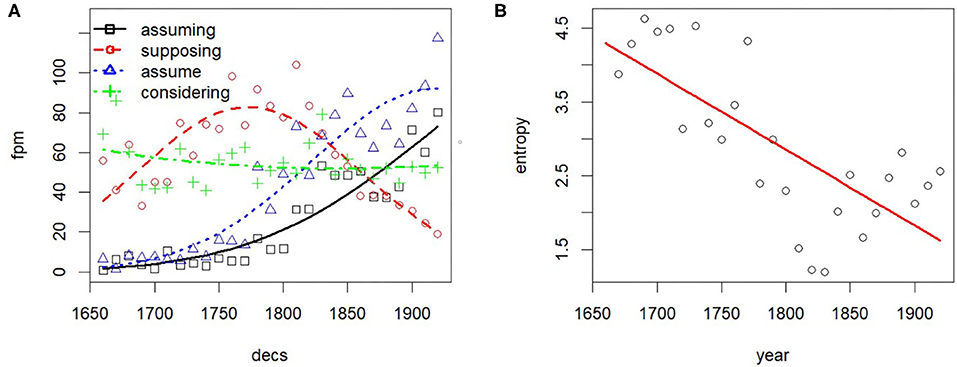
Figure 10 . Entropy decrease on specific WE clusters signals convergence in usage. (A) Relative frequency. (B) Entropy.
The effect of stylistic convergence on the reduction of the cluster entropy of assuming is visible also through a cursory look at some corpus concordances. Uses of assuming in the sense of “adopting” disappear (see example 1). Over time, assuming comes to be used increasingly at the beginning of sentences (example 2), the dominant use being the non-finite alternative to a conditional clause ( If we assume a/the/that .). In terms of frequency, the dominant choice in the cluster is assume , presumably as a short form of let us/let's assume (example 3), a usage that is often associated with mathematical reasoning.
(1) No notice is taken of any effervescence or discharge of air while it was assuming this color ( Cavendish, 1786 ).
(2) Assuming a distribution of light of the form when x is the distance along the spectrum from the center of the line, the half breadth is defined as the distance in which the intensity is reduced to half the maximum ( Strutt, 1919 ).
(3) Assume any three points a, b, c in the surface, no two of which are on one generator, [.] ( Gardiner, 1867 ).
5. Summary and Future Work
We have explored patterns of variation and change in language use in Scientific English from a diachronic perspective, focusing on the Late Modern period. Our starting assumption was that we will find both traces of diversification in terms of discourse field, thus pointing to register formation, as well as convergence in linguistic usage as indicator of an emerging scientific style. As a data set we used 250+ years of publications of the Royal Society of London [Royal Society Corpus (RSC), Version 6.0 Open].
We have elaborated a data-driven approach using three kinds of computational language models that reveal different aspects of diachronic change. Ngram models (both lemma and POS-based) point to an overall trend of consolidation in linguistic usage. But the lexical level dynamically oscillates between high peaks marking lexical innovation and lows marking stable linguistic use, where the peaks typically reflect new scientific discoveries or insights. At the grammatical level, we observe similar tendencies but at a much lower level and rate and the consolidation trend is much more obvious. Inspecting the specific grammatical patterns involved, we find that they mark what we commonly refer to as “scientific style,” such as relational and passive clauses or specific nominal patterns for hosting terminology.
To investigate further the tendencies at the level of words, we have looked at aggregations of words from two perspectives—how words group together to form topics (development of fields of discourse as the core factor in register formation) and how specific words group together to form paradigms based on their use in similar contexts. Diversification is fully born out from both perspectives with glimpses of consolidation as well. Analysis on the basis of a diachronic topic model shows that topics diversify over time, indexed by increasing entropy over topic/word distributions, a clear signal of register formation. Analysis on the basis of diachronic word embeddings reveals that the overall paradigmatic organization of the vocabulary changes quite dramatically: the lexical space expands overall and it becomes more fragmented, the latter being a clear signal of diversification in word usage. Here, bursts of innovation are shown by increasing entropy on specific word clusters, such as terms for chemical compounds, mirroring the insights from lemma-based analysis with KLD. Also, patterns of convergence (confined uses of words) as well as obsolescence (word uses leaving the language) are shown by decreasing entropy on particular word clusters, such as the cluster of ing -forms. Taken together, we encounter converging evidence of diversification at different levels of analysis; and at the same time we find signs of linguistic convergence as an overarching trend—an emerging tacit agreement on “how to say things”, a “scientific style.”
In terms of methods, we have elaborated a data-driven methodology for diachronic corpus comparison using state-of-the-art computational language models. To analyze and interpret model outputs, we have applied selected information-theoretic measures to diachronic comparison. Relative entropy used as a data-driven periodization technique provides insights into overall diachronic trends. Entropy provides a general measure of diversity and is applied here to capture diversification as well as converging language use for lexis (word embeddings) overall and discourse fields (topic models) in particular.
In future work, we will exploit more fully the results from topic modeling and the word embeddings model of the RSC. For instance, we want to systematically inspect high and low-entropy word embedding clusters to find more features marking expansion (vs. obsolescence) and diversification (vs. convergence). Also, annotating the corpus with topic labels from our diachronic topic model will allow us to investigate discipline-specific language use (e.g., chemistry) and contrast it with “general” scientific language (represented by the whole RSC) as well as study the life cycles of registers/sublanguages. Especially interesting from a sociocultural point of view would be to trace the spread of linguistic change across disciplines and authors (e.g., Did people adopt specific linguistic usages from famous scientists?). Finally, we would like to contextualize our findings from an evolutionary perspective and possibly devise predictive models of change. Our results seem to be in accordance not only with our intuitive understanding of the evolution of science but also with evolutionary studies on vocabulary formation (e.g., Smith, 2004 ) showing how populations using specialized vocabularies are more likely to develop and take over when the selective ratio is pure efficacy in information exchange.
Data Availability Statement
The Royal Society Corpus (RSC) 6.0 Open is available at: https://hdl.handle.net/21.11119/0000-0004-8E37-F (persistent handle). Word embedding models of the RSC with different parameter settings including visualization are available at: http://corpora.ids-mannheim.de/openlab/diaviz1/description.html .
Author Contributions
YB curated the analyses on word embeddings showing the diachronic expansion of the scientific semantic space and lexical-semantic specialisation. SD-O carried out the analysis on diachronic trends in lexis and grammar using data-driven periodization and elaborating on features' contribution to change. PF trained the word embeddings and the topic models and designed and implemented the entropy-based diachronic analysis. ET provided the historical background and was involved in hypothesis building and interpretation of results.
Funded by the Deutsche Forschungsgemeinschaft (DFG, German Research Foundation) - Project-ID 232722074 - SFB 1102. The authors also acknowledge support by the German Federal Ministry of Education and Research (BMBF) under grant CLARIN-D, the German Common Language Resources and Technology Infrastructure. Also, the authors are indebted to Dr. Louisiane Ferlier, digitisation project manager at Royal Society Publishing, for providing advice and access to sources.
Conflict of Interest
The authors declare that the research was conducted in the absence of any commercial or financial relationships that could be construed as a potential conflict of interest.
1. ^ An example in point are production and experimentation, which used to be carried out hand in hand in the workshops of alchemists and apothecaries but were separated later on, also physically, with experimentation becoming a scientific activity carried out in dedicated laboratories ( Burke, 2004 ; Schmidgen, 2011 ).
2. ^ For example, the publication of the famous Encyclopédie ou Dictionnaire raisonné des sciences, des arts et des métiers (1751–1765).
3. ^ For more comprehensive overviews on computational approaches to lexical semantic change see Tahmasebi et al. (2018) and on diachronic word embeddings see Kutuzov et al. (2018) .
4. ^ RSC 6.0 Open: https://hdl.handle.net/21.11119/0000-0004-8E37-F .
5. ^ http://mallet.cs.umass.edu
6. ^ We use Pearson distance, which consistently results in more intuitive hierarchies than Jensen-Shannon Divergence.
7. ^ For the corpus at hand, a smaller number of topics leads to conflated topics, a larger number to redundant topics.
8. ^ Clustering by Jensen-Shannon Divergence results in a less intuitive hierarchy.
9. ^ A similar correlation between probability and entropy can be observed in other rising topic groups.
Aitchison, J. (2017). “Psycholinguistic perspectives on language change,” in The Handbook of Historical Linguistics , eds D. Joseph and R. D. Janda (London, UK: Blackwell), 736–743. doi: 10.1002/9781405166201.ch25
CrossRef Full Text | Google Scholar
Aragamon, S. (2019). Register in computational language research. Register Stud . 1, 100–135. doi: 10.1075/rs.18015.arg
Atkinson, D. (1999). Scientific Discourse in Sociohistorical Context: The Philosophical Transactions of the Royal Society of London, 1675-1975 . New York, NY: Erlbaum. doi: 10.4324/9781410601704
Banks, D. (2008). The Development of Scientific Writing: Linguistic Features and Historical Context . London; Oakville, OM: Equinox.
Google Scholar
Baron, A., and Rayson, P. (2008). “VARD 2: a tool for dealing with spelling variation in historical corpora,” in Proceedings of the Postgraduate Conference in Corpus Linguistics (Birmingham).
Biber, D. (1988). Variation Across Speech and Writing . Cambridge: Cambridge University Press. doi: 10.1017/CBO9780511621024
Biber, D., and Gray, B. (2011). “The historical shift of scientific academic prose in English towards less explicit styles of expression: writing without verbs,” in Researching Specialized Languages , eds V. Bathia, P. Sánchez, and P. Pérez-Paredes (Amsterdam: John Benjamins), 11–24. doi: 10.1075/scl.47.04bib
Biber, D., and Gray, B. (2016). Grammatical Complexity in Academic English: Linguistic Change in Writing. Studies in English Language . Cambridge: Cambridge University Press. doi: 10.1017/CBO9780511920776
Bizzoni, Y., Degaetano-Ortlieb, S., Menzel, K., Krielke, P., and Teich, E. (2019a). “Grammar and meaning: analysing the topology of diachronic word embeddings,” in Proceedings of the 1st International Workshop on Computational Approaches to Historical Language Change (Florence: Association for Computational Linguistics), 175–185. doi: 10.18653/v1/W19-4722
Bizzoni, Y., Mosbach, M., Klakow, D., and Degaetano-Ortlieb, S. (2019b). “Some steps towards the generation of diachronic WordNets,” in Proceedings of the 22nd Nordic Conference on Computational Linguistics (NoDaLiDa'19) (Turku: ACL).
Blei, D. M., and Lafferty, J. D. (2006). “Dynamic topic models,” in Proceedings of the 23rd International Conference on Machine Learning (Pittsburgh, PA), 113–120. doi: 10.1145/1143844.1143859
PubMed Abstract | CrossRef Full Text | Google Scholar
Bochkarev, V., Solovyev, V. D., and Wichmann, S. (2014). Universals versus historical contingencies in lexical evolution. J. R. Soc. Interface 11, 1–8. doi: 10.1098/rsif.2014.0841
Burke, P. (2004). Languages and Communities in Early Modern Europe . Cambridge: CUP. doi: 10.1017/CBO9780511617362
Bybee, J. (2007). Frequency of Use and the Organization of Language . New York, NY: Oxford University Press. doi: 10.1093/acprof:oso/9780195301571.001.0001
Cavendish, H. (1786). XIII. An account of experiments made by Mr. John McNab, at Henley House, Hudson's Bay, relating to freezing mixtures. Phil. Trans. R. Soc. 76, 241–272. doi: 10.1098/rstl.1786.0013
Danescu-Niculescu-Mizil, C., West, R., Jurafsky, D., Leskovec, J., and Potts, C. (2013). “No country for old members: user lifecycle and linguistic change in online communities,” in Proceedings of the 22nd International World Wide Web Conference (WWW) (Rio de Janeiro). doi: 10.1145/2488388.2488416
Degaetano-Ortlieb, S., and Teich, E. (2016). “Information-based modeling of diachronic linguistic change: from typicality to productivity,” in Proceedings of the 10th Joint SIGHUM Workshop on Computational Linguistics for Cultural Heritage, Social Sciences, Humanities and Literature at ACL2016 , 165–173. doi: 10.18653/v1/W16-2121
Degaetano-Ortlieb, S., and Teich, E. (2018). “Using relative entropy for detection and analysis of periods of diachronic linguistic change,” in Proceedings of the 2nd Joint SIGHUM Workshop on Computational Linguistics for Cultural Heritage, Social Sciences, Humanities and Literature at COLING2018 (Santa Fe, NM), 22–33.
Degaetano-Ortlieb, S., and Teich, E. (2019). Toward an optimal code for communication: the case of scientific English. Corpus Linguist. Linguist. Theory 1–33. doi: 10.1515/cllt-2018-0088. [Epub ahead of print].
Dubossarsky, H., Hengchen, S., Tahmasebi, N., and Schlechtweg, D. (2019). “Time-out: temporal referencing for robust modeling of lexical semantic change,” in Proceedings of the 57th Meeting of the Association for Computational Linguistics (ACL2019) (Florence: ACL), 457–470. doi: 10.18653/v1/P19-1044
Dubossarsky, H., Weinshall, D., and Grossman, E. (2017). “Outta control: laws of semantic change and inherent biases in word representation models,” in Proceedings of the 2017 Conference on Empirical Methods in Natural Language Processing (Copenhagen: Association for Computational Linguistics), 1136–1145. doi: 10.18653/v1/D17-1118
Eisenstein, J., O'Connor, B., Smith, N. A., and Xing, E. P. (2014). Diffusion of lexical change in social media. PLoS ONE 9:e113114. doi: 10.1371/journal.pone.0113114
Fankhauser, P., Knappen, J., and Teich, E. (2014). “Exploring and visualizing variation in language resources,” in Proceedings of the 9th Language Resources and Evaluation Conference (LREC) (Reykjavik), 4125–4128.
Fankhauser, P., Knappen, J., and Teich, E. (2016). “Topical diversification over time in the Royal Society Corpus,” in Proceedings of Digital Humanities (DH) (Krakow).
Fankhauser, P., and Kupietz, M. (2017). “Visual correlation for detecting patterns in language change,” in Visualisierungsprozesse in den Humanities. Linguistische Perspektiven auf Prägungen, Praktiken, Positionen (VisuHu 2017) (Zürich).
Fischer, S., Knappen, J., Menzel, K., and Teich, E. (2020). “The Royal Society Corpus 6.0. Providing 300+ years of scientific writing for humanistic study,” in Proceedings of the 12th Language Resources and Evaluation Conference (LREC) (Marseille).
Fyfe, A., Squazzoni, F., Torny, D., and Dondio, P. (2019). Managing the growth of peer review at the Royal Society journals, 1865-1965. Sci. Technol. Human Values . 45, 405–429. doi: 10.1177/0162243919862868
Garcia, M., and Garćia-Salido, M. (2019). “A method to automatically identify diachronic variation in collocations,” in Proceedings of the 1st Workshop on Computational Approaches to Historical Language Change (Florence: ACL), 71–80. doi: 10.18653/v1/W19-4709
Gardiner, M. (1867). Memoir on Undevelopable Uniquadric Homographics. [Abstract]. Proc. R. Soc. Lond. 16:389–398. Available online at: www.jstor.org/stable/112537
Gleick, J. (2010). “At the beginning: More things in heaven and earth,” in Seeing Further. The Story of Science and The Royal Society , ed B. Bryson (London, UK: Harper Press), 17–36.
Goldberg, A. E. (2006). Constructions at Work. The Nature of Generalizations in Language . Oxford: OUP.
PubMed Abstract | Google Scholar
Görlach, M. (2001). Eighteenth-Century English . Heidelberg: Winter.
Görlach, M. (2004). Text Types and the History of English . Berlin, New York, NY: de Gruyter.
Grieve, J., Nini, A., and Guo, D. (2016). Analyzing lexical emergence in Modern American English online. Engl. Lang. Linguist . 20, 1–29. doi: 10.1017/S1360674316000526
Hall, D., Jurafsky, D., and Manning, C. D. (2008). “Studying the history of ideas using topic models,” in Proceedings of the Conference on Empirical Methods in Natural Language Processing (Honolulu, HI: Association for Computational Linguistics). doi: 10.3115/1613715.1613763
Halliday, M. (1985a). An Introduction to Functional Grammar . London: Edward Arnold.
Halliday, M. (1985b). Written and Spoken Language . Melbourne, VIC: Deakin University Press.
Halliday, M. (1988). “On the language of physical science,” in Registers of Written English: Situational Factors and Linguistic Features , ed M. Ghadessy (London: Pinter), 162–177.
Halliday, M., and Martin, J. (1993). Writing Science: Literacy and Discursive Power . London: Falmer Press.
Hamilton, W. L., Leskovec, J., and Jurafsky, D. (2016). “Cultural shift or linguistic drift? Comparing two computational models of semantic change,” in Proceedings of the Conference on Empirical Methods in Natural Language Processing (Austin, TX). doi: 10.18653/v1/D16-1229
Hardie, A. (2012). CQPweb – combining power, flexibility and usability in a corpus analysis tool. Int. J. Corpus Linguist . 17, 380–409. doi: 10.1075/ijcl.17.3.04har
Harris, Z. S. (1954). Distributional structure. Word 10, 146–162. doi: 10.1080/00437956.1954.11659520
Hilpert, M., and Perek, F. (2015). Meaning change in a petri dish: constructions, semantic vector spaces, and motion charts. Linguist. Vanguard 1, 339–350. doi: 10.1515/lingvan-2015-0013
Hughes, J. M., Foti, N. J., Krakauer, D. C., and Rockmore, D. N. (2012). Quantitative patterns of stylistic influence in the evolution of literature. Proc. Natl. Acad. Sci. U.S.A . 109, 7682–7686. doi: 10.1073/pnas.1115407109
Hundt, M., Mollin, S., and Pfenninger, S. E., (eds). (2017). The Changing English Language: Psycholinguistic Perspectives . Cambridge, UK: CUP. doi: 10.1017/9781316091746
Hunston, S., and Francis, G. (2000). Pattern Grammar: A Corpus-driven Approach to the Lexical Grammar of English. Pattern Grammar: A Corpus-driven Approach to the Lexical Grammar of English . Amsterdam: Benjamins. doi: 10.1075/scl.4
Jucker, A. H., and Taavitsainen, I. (2013). English Historical Pragmatics . Edinburgh: Edinburgh University Press.
Juola, P. (2003). The time course of language change. Comput. Humanit . 3, 77–96. doi: 10.1023/A:1021839220474
Jurish, B. (2018). “Diachronic collocations, genre, and DiaCollo,” in Diachronic Corpora, Genre, and Language Change , ed R. J. Whitt (Benjamins: Amsterdam), 41–64. doi: 10.1075/scl.85.03jur
Kaiser, G. A., Butt, M., Kalouli, A.-L., Kehlbeck, R., Sevastjanova, R., and Kaiser, K. (2019). “Parhistvis: visualization of parallel multilingual historical data,” in Workshop on Computational Approaches to Historical Language Change (Florence: Association for Computational Linguistics), 109–114.
Kermes, H., Degaetano-Ortlieb, S., Khamis, A., Knappen, J., and Teich, E. (2016). “The Royal Society Corpus: from uncharted data to corpus,” in Proceedings of the 10th LREC (Portoroz).
Kim, Y., Chiu, Y.-I., Hanaki, K., Hegde, D., and Petrov, S. (2014). “Temporal analysis of language through neural language models,” in Proceedings of the ACL 2014 Workshop on Language Technologies and Computational Social Science (Baltimore, MD: Association for Computational Linguistics), 61–65. doi: 10.3115/v1/W14-2517
Kirby, S., Tamariz, M., Cornish, H., and Smith, K. (2015). Compression and communication in the cultural evolution of linguistic structure. Cognition 141, 87–102. doi: 10.1016/j.cognition.2015.03.016
Klingenstein, S., Hitchcock, T., and DeDeo, S. (2014). The civilizing process in London's Old Bailey. Proc. Natl. Acad. Sci. U.S.A . 111, 9419–9424. doi: 10.1073/pnas.1405984111
Kullback, S., and Leibler, R. A. (1951). On information and sufficiency. Ann. Math. Stat . 22, 79–86. doi: 10.1214/aoms/1177729694
Kutuzov, A., Øvrelid, L., Szymanski, T., and Velldal, E. (2018). “Diachronic word embeddings and semantic shifts: a survey,” in Proceedings of the 27th International Conference on Computational Linguistics (Coling) (Sante Fe, NM: ACL), 1384–1397.
Leech, G., Hundt, M., Mair, C., and Smith, N. (2009). Change in Contemporary English: A Grammatical Study . Cambridge, UK: Cambridge University Press. doi: 10.1017/CBO9780511642210
Lenci, A. (2008). Distributional semantics in linguistic and cognitive research. Ital. J. Linguist . 20, 1–31.
Ling, W., Dyer, C., Black, A. W., and Trancoso, I. (2015). “Two/too simple adaptations of Word2Vec for syntax problems,” in Proceedings of the 2015 Conference of the North American Chapter of the Association for Computational Linguistics: Human Language Technologies (Denver, CO: Association for Computational Linguistics), 1299–1304. doi: 10.3115/v1/N15-1142
McFarland, D. A., Ramage, D., Chuang, J., Heer, J., Manning, C. D., and Jurafsky, D. (2013). Differentiating language usage through topic models. Poetics 41, 607–625. doi: 10.1016/j.poetic.2013.06.004
Mikolov, T., Sutskever, I., Chen, K., Corrado, G. S., and Dean, J. (2013). “Distributed representations of words and phrases and their compositionality,” in Advances in Neural Information Processing Systems (Lake Tahoe, NV), 3111–3119.
Moskowich, I., and Crespo, B., (eds.). (2012). Astronomy Playne and Simple: The Writing of Science between 1700 and 1900 . Amsterdam: Philadelphia, PA: John Benjamins. doi: 10.1075/z.173
Moxham, N., and Fyfe, A. (2018). The royal society and the prehistory of peer review, 1665-1965. Historical J . 61, 863–889. doi: 10.1017/S0018246X17000334
Nevalainen, T. (2006). “Historical sociolinguistics and language change,” in Handbook of the History of English , eds A. van Kemenade and B. Los (London, UK: Wiley-Blackwell), 558–588. doi: 10.1002/9780470757048.ch22
Nevalainen, T., and Traugott, E. C., (eds.). (2012). The Oxford Handbook of the History of English . New York, NY: Oxford University Press. doi: 10.1093/oxfordhb/9780199922765.001.0001
Nguyen, D., Dogruöz, A. S., Rosé, C. P., and de Jong, F. (2016). Computational sociolinguistics: a survey. Comput. Linguist . 42, 537–593. doi: 10.1162/COLI_a_00258
Quirk, R., Greenbaum, S., Leech, G., and Svartvik, J. (1985). A Comprehensive Grammar of the English Language . London: Longman.
Säily, T., Nurmi, A., Palander-Collin, M., and Auer, A., (eds.). (2017). “Exploring future paths for historical sociolinguistics,” in Advances in Historical Sociolinguistics (Amsterdam: Benjamins). doi: 10.1075/ahs.7.01sai
Schmid, H. (1995). “Improvements in Part-of-Speech Tagging with an application to German,” in Proceedings of the ACL SIGDAT-Workshop (Kyoto).
Schmidgen, H. (2011). Das Labor/The Laboratory . Europäische Geschichte Online/European History Online (EGO).
Smith, K. (2004). The evolution of vocabulary. J. Theoret. Biol . 228, 127–142. doi: 10.1016/j.jtbi.2003.12.016
Steyvers, M., and Griffiths, T. (2007). Probabilistic Topic Models . Hillsdale, NJ: Erlbaum.
Strutt, R. J. (1919). Bakerian lecture: A study of the line spectrum of sodium as excited by fluorescence. Proc. R. Soc. Lond. A 96:272–286. doi: 10.1098/rspa.1919.0054
Taavitsainen, I., and Hiltunen, T., (eds.) (2019). Late Modern English Medical Texts: Writing Medicine in the Eighteenth Century . Amsterdam: Benjamins. doi: 10.1075/z.221
Taavitsainen, I., and Jucker, A. H., (eds.). (2003). Diachronic Perspectives on Address Term Systems . Amsterdam: Benjamins. doi: 10.1075/pbns.107
Tahmasebi, N., Borin, L., and Jatowt, A. (2018). Survey of computational approaches to diachronic conceptual change. arXiv[Preprint]a.rXiv:1811.06278 .
Thoiron, P. (1986). Diversity index and entropy as measures of lexical richness. Comput. Humanit . 20, 197–202. doi: 10.1007/BF02404461
Wallach, H. M., Mimno, D. M., and McCallum, A. (2009). “Rethinking LDA: why priors matter,” in Advances in Neural Information Processing Systems 22 , eds Y. Bengio, D. Schuurmans, J. D. Lafferty, C. K. I. Williams, and A. Culotta (Vancouver, BC: Curran Associates, Inc.), 1973–1981.
Xu, Y., and Kemp, C. (2015). “A computational evaluation of two laws of semantic change,” in Proceedings of the 37th Annual Meeting of the Cognitive Science Society (CogSci) (Pasadena, CA).
Yang, T.-I., Torget, A., and Mihalcea, R. (2011). “Topic modeling on historical newspapers,” in Workshop on Language Technology for Cultural Heritage, Social Sciences, and Humanities (LaTeCH) (Portland, OR).
Keywords: linguistic change, diachronic variation in language use, register variation, evolution of Scientific English, computational language models
Citation: Bizzoni Y, Degaetano-Ortlieb S, Fankhauser P and Teich E (2020) Linguistic Variation and Change in 250 Years of English Scientific Writing: A Data-Driven Approach. Front. Artif. Intell. 3:73. doi: 10.3389/frai.2020.00073
Received: 03 February 2020; Accepted: 07 August 2020; Published: 16 September 2020.
Reviewed by:
Copyright © 2020 Bizzoni, Degaetano-Ortlieb, Fankhauser and Teich. This is an open-access article distributed under the terms of the Creative Commons Attribution License (CC BY) . The use, distribution or reproduction in other forums is permitted, provided the original author(s) and the copyright owner(s) are credited and that the original publication in this journal is cited, in accordance with accepted academic practice. No use, distribution or reproduction is permitted which does not comply with these terms.
*Correspondence: Yuri Bizzoni, yuri.bizzoni@uni-saarland.de
This article is part of the Research Topic
Computational Sociolinguistics

An official website of the United States government
The .gov means it’s official. Federal government websites often end in .gov or .mil. Before sharing sensitive information, make sure you’re on a federal government site.
The site is secure. The https:// ensures that you are connecting to the official website and that any information you provide is encrypted and transmitted securely.
- Publications
- Account settings
Preview improvements coming to the PMC website in October 2024. Learn More or Try it out now .
- Advanced Search
- Journal List

How individuals change language
Richard a. blythe.
1 SUPA, School of Physics and Astronomy, University of Edinburgh, Edinburgh, United Kingdom
William Croft
2 Department of Linguistics, University of New Mexico, Albuquerque, New Mexico, United States of America
Associated Data
Relevant data and methods are within the paper and its Supporting information files. All codes and the raw data they work with are publicly available at https://git.ecdf.ed.ac.uk/rblythe3/gram-cycles which is a non-commercial git repository hosted by the University of Edinburgh.
Languages emerge and change over time at the population level though interactions between individual speakers. It is, however, hard to directly observe how a single speaker’s linguistic innovation precipitates a population-wide change in the language, and many theoretical proposals exist. We introduce a very general mathematical model that encompasses a wide variety of individual-level linguistic behaviours and provides statistical predictions for the population-level changes that result from them. This model allows us to compare the likelihood of empirically-attested changes in definite and indefinite articles in multiple languages under different assumptions on the way in which individuals learn and use language. We find that accounts of language change that appeal primarily to errors in childhood language acquisition are very weakly supported by the historical data, whereas those that allow speakers to change incrementally across the lifespan are more plausible, particularly when combined with social network effects.
Introduction
Human language is a multiscale phenomenon. A language is shared by a large population, that is, the speech community: it is a set of linguistic conventions, characteristic of the population as a whole. Yet language originates in individuals. Individuals in a population use language to achieve specific communicative goals, and through repeated interactions there emerge the linguistic conventions of the speech community. These conventions also change over time, and as speech communities split, the linguistic conventions of the speech communities diverge, leading to variation across languages.
How does the behaviour of individual speakers lead to change in linguistic conventions and ultimately the emergence of linguistic diversity? It transpires that this is one of the most debated questions in the study of language change for at least a century [ 1 ]. A widely-held view is that the locus of language change is in child language acquisition, in particular the process of inferring a grammar that is consistent with the sentences that have been heard [ 2 – 5 ]. Where these sentences do not fully specify a grammar, a child can infer a different grammar from its parents. If enough children infer a different grammar, then the language changes as the generations succeed each other. Variations on this basic idea exist, for example, where a child may have multiple grammars representing old and new linguistic variants, with the relative weighting of the two grammars shifting across generations [ 4 ]. A competing account is the usage-based theory [ 6 – 9 ], where linguistic innovation occurs at any point in a speaker’s lifespan, and speakers vary the frequencies that they use different structures incrementally across the lifespan [ 10 – 13 ].
One reason that this question has not been resolved during the century-long debate is that direct evidence of the origin of a change that develops into a new linguistic convention is generally lacking. Research in child language acquisition has demonstrated that children are very good at acquiring and conforming to the conventions of the speech community. In fact, the primary research question in child language acquisition is how children are so successful in mastering not only general rules of language but also the many exceptions and irregularities in adult language conventions [ 14 ]. Child-based approaches argue that children find the patterns rapidly on the basis of specific innate language structures, while usage-based approaches argue that child language acquisition is incremental and general patterns are expanded gradually [ 15 ]. The fate of any innovations that are produced in the acquisition phase tends not to be investigated in this line of research. Meanwhile, sociolinguistic research on variation and change begins with a situation in which the novel variant has already been produced, and in fact the novel variant is already changing in frequency on the way to becoming a new linguistic convention. It is virtually impossible to capture the innovation as it happens; linguists are always analysing situations in which the new variant is already present.
Hence linguists have tended to rely on indirect evidence that would shed light on the role of the individual in language change. For example, it has been observed that the sound changes that are produced by children—innovations, or “errors” from the perspective of adult grammar—are not the same as the sound changes that have been documented in language history [ 16 – 21 ]. However, the innovative variation produced spontaneously by adults in both sound and grammar is of the same type that has been documented in language history [ 22 , 23 ]. These observations support the usage-based theory over the child-based theory. Also, while children are extremely good at acquiring the linguistic conventions of adults, by late adolescence they develop into the leaders propagating a novel variant through the speech community, which suggests that language change does not originate in childhood [ 10 , 13 , 24 , 25 ].
Here we take a novel approach to addressing the question of the locus of language change in the individual: we quantify and compare the plausibility of different theories of individual behaviour in producing population-level language changes and the resultant worldwide diversity of language traits. We achieve this by introducing a mathematical model that allows us to test a variety of hypotheses about how individuals ultimately bring about language change at the population level. The model is applied to diachronic and crosslinguistic data of one common type of language change, the grammatical evolution of definite and indefinite articles, such as English the and a respectively. The evolution of articles can be analysed as a cycle of states in which a language without an article may develop an article which may then disappear, allowing a simple unidirectional model of innovation and propagation of a change in a finite set of states. We draw on data of attested changes in definite and indefinite articles for 52 languages, and on the cross-linguistic distribution of article states (620 languages for definite articles, 534 languages for indefinite articles; see below for further details).
Our model allows us to access a very wide range of different individual-level processes of language learning and use which appear in different combinations, whilst remaining amenable to mathematical analysis with methods from population genetics [ 26 ]. Specifically, we can estimate the likelihood of our set of empirical language changes at the population scale, given a certain set of assumptions on the behaviour at the individual level. This then means we can determine the regions within this model space that have the strongest empirical support. As we will show below, we find that explanations of language change that appeal exclusively to childhood language learning receive considerably less support than those that allow incremental change across the lifespan. Our analysis further suggests that the complex structure of social networks—in which the degree of influence that different speakers may have over others is highly variable—may play an important role in the diffusion of linguistic innovations.
Data and methods
In this section we first set out empirical properties of changes in articles that guide us towards a statistical model of language change over historical time at the population scale. The basic picture, illustrated in Fig 1a , is one in which the population is initially at some stage of the cycle, for example, the situation where there is no definite article (stage 0). As a consequence of individual speaker innovations, an article is occasionally introduced into the population by recruiting a pre-existing word for the article function. This is indicated by diamonds in the figure. In later stages, different linguistic processes lead to a divergence in form, reduction of that form to an affix and the loss of the form. Eventually, one of the innovations propagates so that its frequency , defined as the proportion of relevant contexts in which the innovation is used, rises to 100%. Once this occurs, the next stage of the cycle has been reached and the process begins afresh. Following [ 26 ], we refer to this population-scale model as an origin-fixation model : the introduction of an innovation that successfully propagates (denoted by a circle in the figure) is referred to as origination , and the point at which it reaches a frequency of 100% is called fixation .

(a) Origin-fixation model at the population scale, showing a transition between two stages of a grammaticalisation cycle (set out in Table 1 ). Innovations are repeatedly introduced to the population; most fail (diamonds), but some successfully originate a change that propagates and goes to fixation (circles). The fixation time T F is a random variable (see text). (b) Underlying individual-based (Wright-Fisher) model. Individuals are characterised by the frequency with which they use the innovation (orange portion of pie charts). In the case shown, individuals update their innovation frequencies by retaining a fraction 1 − ϵ their existing value, and acquiring the remaining fraction ϵ through exposure to one other member of the speech community. In the figure, ϵ = 1 2 for illustrative purposes. The two levels of description are connected by averaging over the individual speaker-level innovation frequencies in the Wright-Fisher model to obtain the population-level frequency plotted for the origin-fixation model.
This population-scale process is the product of interactions between individual speakers in the population, that is, acquisition or use, or a combination of the two. These interactions are illustrated schematically in Fig 1b and will be discussed in detail in the second part of this section. The individual-based model is very similar to the Wright-Fisher model in population genetics (see e.g. [ 27 ]), and we refer to it as such. In this model, each speaker is characterised by the frequency with which they use an innovation in the relevant linguistic context. The Wright-Fisher and origin-fixation models are connected by averaging over the individual frequencies to obtain the corresponding frequency at the population level. This then provides a quantitative model for language change over historical timescales that is grounded in individual speaker interactions.
Language change at the population level
Empirical properties.
We draw on two sources of data to characterise language change at the population level: (i) a survey of documented instances of historical language change (detailed in S1 Appendix ); and (ii) the typological distribution of the current stage in the cycle across the world’s languages (as recorded in the World Atlas of Language Structures, WALS [ 28 ]). As stated in the Introduction, we focus on definite and indefinite articles for this analysis. There are a number of reasons for this. First, the evolution of articles predominantly follows a single cycle of grammaticalisation. Definite articles are predominantly derived from demonstratives such as that [ 29 ], and indefinite articles are predominantly derived from the numeral one [ 30 ]. Both articles proceed to being affixed and then disappear. Second, articles are unstable: several find articles to rank among the least stable of a large set of features [ 31 – 33 ]. This means that our historical survey includes many documented instances of multiple stages in the article grammaticalisation cycle, which in turn leads to a more sensitive likelihood-based analysis than is possible when changes are rare. Finally, this instability implies that the current distribution of stages in the cycle across languages is likely to be close to the stationary distribution, which simplifies the analysis. Although articles are at one end of the stability spectrum, we expect that similar results to those reported below would be found for more stable features: we return to this point in the Discussion.
We divide the stages of the cycle following the classification of WALS Features 37A and 38A [ 28 ]: (0) no explicit article; (1) use of that and one for definite and indefinite article meaning respectively; (2) use of a distinct word usually derived from that or one for the article; and (3) use of an affix. WALS provides the current crosslinguistic distribution of these four stages for definite and indefinite articles (see Table 1 ). One can also look at the joint distribution of the two features to establish whether they are correlated. A χ 2 test on the contingency table indicates that the features are unlikely to be independent ( p < 10 −6 ; although the conditions for the validity of the χ 2 test do not strictly apply, this level of significance was confirmed by a Monte Carlo sampling procedure).
The number of languages in each state is taken from [ 28 ].
We collected data on the documented history of articles in 52 languages from multiple sources (see S1 Appendix ), and divided their history into the same four stages. Importantly, at any given point in time, one of these conventions typically dominates; over time the dominant convention changes to the next in the sequence 0–3 above, before returning to stage 0 via loss of the article. In our analysis of the 52 languages, we find only a single instance of a stage of the cycle that was skipped. For each article and language, we can estimate the rate of change as m + 1 t , where m is the number of changes observed and t is the observation period. (Technically, this is the mean of the posterior distribution over rates when the prior is uniform and the changes assumed to occur as a Poisson process.) We plot the distribution of these rates for each article in Fig 2 . This shows that the median rate of change is roughly once every 1000 years and that the distribution is somewhat skewed towards slower rates of change. Our survey further suggests that the time taken for a change to propagate is somewhat shorter than this, perhaps of the order of 100 years. We further find that, for any given language, the number of changes in one article is not independent of the other ( χ 2 test p = 0.00058; Monte Carlo estimate p = 0.0026). In the following we present results for the two articles separately, as combining probabilities from the two analyses is not justified when measurements are correlated.

The vertical dotted line indicates the median of the distribution.
Origin-fixation model
We use the historical properties of article grammaticalisation cycles, set out above, to flesh out our statistical model of the process at the population scale. Recall from Fig 1a the picture of an initial state in which all speakers are at a given stage of the cycle (say, stage 0), and as speakers interact, instances of the next stage are repeatedly introduced. In a child-based model [ 2 , 4 , 5 ], the next convention is introduced by children in the acquisition process. In the usage-based model, by contrast, the next convention is introduced in language use by speakers of any age [ 7 , 9 , 22 ].
Under whatever mechanism one has in mind, only some of the individual innovations are replicated sufficiently often that they become used by the entire population, reaching the frequency of 100% that defines the state of fixation and therewith the onset of the next stage of the cycle [ 22 , 23 , 34 ].
We assume that the rate at which speakers introduce a specific innovation (e.g., introducing a particular form for an article) in individual instances of acquisition or use is constant over time, as is the probability that this innovation then propagates and reaches fixation. This means that at any given stage in the cycle, origination events occur at a constant rate. In mathematical terms, origination is a Poisson process with rate ω i when the population is in stage i of the cycle (and so the innovations correspond to stage i + 1).
Specifically, we take ω i = ω ¯ 4 f i , where f i is the fraction of languages currently at stage i in the cycle ( Table 1 ). This choice ensures, for any value of the parameter ω ¯ , that the stationary distribution of the origin-fixation is one in which the probability of being at stage i of the cycle is f i , and consequently matches the WALS distribution (although our conclusions do not depend on this being the case). By including the factor 4 (i.e., the number of stages in the cycle) ω ¯ can be interpreted as a mean origination rate obtained by averaging over one complete cycle. In general we will treat this rate as a free parameter (see Results , below).
Once the originating innovation has entered the population, it takes a time T F , called the fixation time to become adopted as the convention by all speakers in the population. In origin-fixation models applied to the invasion of mutant genes in a biological population [ 26 , 35 ], the origination process is generally much slower than the fixation process, and T F is typically set to zero. This is not appropriate in the application to language change: the historical survey above suggests that T F is only one order of magnitude smaller than the time between the origination of a change. Moreover, T F is unlikely to be exactly the same for each change, due to the unpredictability of human interactions and individual speech acts.
We account for this unpredictability by drawing each fixation time T F from a probability distribution. The fixation time distribution can be calculated for certain individual-based models, such as the Wright-Fisher model set out below [ 27 , 36 ]. However, the mathematical form is too complicated to be of practical use, so we approximate it by the simpler Gamma distribution. This distribution is a natural choice for a quantity that is required to be positive (like a fixation time), and whose mean and variance can be controlled independently. In fact, we will arrive at the population-scale model by setting these two quantities equal to those that derive from an underlying individual-based model. Fig 3 shows the Gamma-distribution approximation to the fixation time distribution obtained numerically for the Wright-Fisher model with and without a selection bias. Although the Gamma distribution does not fit perfectly, it captures the location and width of the peak well, and is preferable to simply assuming that T F is zero.

In (a) the Wright-Fisher model has N = 100 individuals and no selection. In (b) N = 150 and s = 0.01.
We now provide a formal mathematical definition of the origin-fixation model that is equivalent to the verbal description above. Starting from stage i of the cycle, a time T O , i at which a change to the next stage in the cycle is originated is drawn from the exponential distribution
as is appropriate for a Poisson process. Then, the time T F from origination to fixation is drawn from the Gamma distribution
At this point, stage i + 1 is entered, and origination of a change to stage i + 2 can begin (by sampling a Poisson process and Gamma-distributed fixation time, as above).
The crucial point is that once these distributions are specified, one can compute the likelihood of the observed changes in our historical survey for any desired combination of parameters ω i , T F ¯ and σ F 2 . Specifically, we ask for the probability that a language in stage i at the beginning of the observation period reaches stage j by the end of that period. The set of periods, changes, and procedure for calculating the likelihood are detailed in S1 Appendix . In the likelihood calculation, each language is treated as independent of the others: we do however consider a mother and its daughters after a split as separate languages, so that changes in the mother language are not included multiple times in the sample. It is important to note that the origin-fixation parameters are not arbitrary, but depend on the underlying behaviour of individuals. A specific choice of individual-based model will lead to specific values of the parameters ω i , T F ¯ and σ F 2 , as we establish below.
Language change at the individual level
Wright-fisher model.
We now set out a model of language behaviour at the individual level which allows us to determine parameter values for the origin-fixation model in regimes of interest. We start with the fact that all theories of language learning and use involve the linguistic behaviour of one individual in the population being adopted (in some way) by another. Looking backwards in time, one can construct a ‘genealogy’ that shows who acquired linguistic behaviour from whom, analogously to the inheritance of genetic material under biological reproduction. It is well understood in population genetics that many superficially different individual-based models of inheritance generate a common distribution of genealogies [ 37 ]. Therefore, one obtains a generic and robust description of an evolutionary process by selecting a specific individual-based model that is adapted to the context at hand. Here we construct a model of the Wright-Fisher type [ 27 ] that allows us to manipulate key properties of the individual speaker, such as how often they can change their behaviour (though learning or use, as appropriate), whether biases towards or against the innovation are operating, and which other members of the speech community they interact with.
The basic structure of this model is shown in Fig 1b . Each circle in the figure represents an individual’s linguistic behaviour at a given point in time. Each individual uses the existing convention (stage 0 in the figure) some fraction of the time, and the incoming innovation (stage 1) the remaining fraction of the time. As in the origin-fixation model, we assume that at most two linguistic variants are widely used at any given time. A variable x n specifies the relative frequency (in the range 0 to 1 inclusive) that speaker n uses the innovation. For example, the left-most speaker in the figure is using the innovation in around x 1 = 1 3 of the relevant contexts at time t . In this work, we take x n to be an average over occurrences of a particular form of the article in a general Noun Phrase construction that expresses (in)definiteness of the referent of the Noun Phrase. The forms are: no article; article identical to a source form (demonstrative for definite article, the numeral ‘one’ for indefinite article); article distinct from source form; and article attached to noun. Although this general construction may be made up of more specific subtypes of Noun Phrase constructions, there is reason to believe that a regular trajectory of change emerges from the aggregation of occurrences over subtypes [ 38 ].
In the traditional Wright-Fisher model, x n takes only the extremal values 0 or 1. In a linguistic context, this corresponds to classic child-based models [ 2 , 3 , 5 ] in which a speaker’s grammar is specified in terms of binary parameters. Other models allow for intermediate values of x n : these include variational learning [ 4 ] and usage-based [ 15 ] models.
The innovation frequencies x n are updated at a rate R for each of the N speakers in the population. We define the update rule in a way that includes the child- and usage-based models as special cases. What these have in common is that, in an interaction, each individual is exposed to the behaviour of one other speaker in the population. Each then replaces a fraction ϵ of their stored linguistic experience with a record of the variant that was perceived in this interaction. That is, x n ′ = ( 1 − ϵ ) x n + ϵ τ , where x n ′ is the updated innovation frequency, and τ = 1 if the innovation was perceived in the interaction, and τ = 0 otherwise. Fig 1b illustrates this update for the case ϵ = 1 2 .
The child-based model is obtained when ϵ = 1. The update then corresponds to a child being exposed to the behaviour of a parent, applying some learning rule to determine if the grammar of the language corresponds to the convention or the innovation, and setting x = 0 or 1 accordingly. Importantly, the learning rule can allow the child to infer a grammar that is different from that of the parent: cue-based learning [ 39 ] is one mechanism that allows for this. A general model for such mechanisms can be obtained by introducing a probability η i that, given a behaviour that is consistent with the parent holding grammar i in the cycle, the child nevertheless adopts grammar i + 1 (for example, because the sentences produced by the parent are more consistent with the next stage of the grammaticalisation cycle). In the child-based model, the appropriate choice for the update rate R would be once per generation. Under these conditions, the timescale of the cultural evolutionary process of language change is necessarily tied to that of biological evolution (although the two processes differ in other respects, for example, the number and identity of parents).
By contrast, the usage-based model allows for the cultural evolutionary dynamics to proceed more quickly than their biological counterparts, as individuals interact many times in the course of a generation. However, the impact of each interaction is likely to be smaller, implying that the parameter ϵ that quantifies this impact should be small. Fig 1b illustrates the case of ϵ = 1 2 , in which after the update (time t + Δ t ), half of the usage frequency derives from their behaviour before the interaction (light shading in the figure), and the other half (dark shading) corresponds to whether a conventional or innovative utterance was perceived in an interaction with the speaker shown by the connecting line. As in the child-based model, there is a small probability η i that a conventional behaviour is perceived as an innovation. This can represent a variety of processes that might apply in single instances of use, e.g., auditory and articulatory constraints [ 40 , 41 ] or cognitive biases [ 41 – 43 ], along with indeterminacy in inferring a phonological form [ 22 , 34 ] or meaning [ 23 , 44 ], that may favour one construction over another (see e.g. [ 7 ] for an extended discussion of innovation in language change).
To complete the description of the Wright-Fisher model, we need to specify how the interlocutor —the speaker who provides the linguistic data to the learner (or listener)—is chosen. There are two components to this: (i) a social network structure; and (ii) a possible biasing of interlocutors based on their linguistic behaviour. We describe these in turn.
The social network is set up so that speaker i has z i immediate neighbours, with z i drawn from a degree distribution p z . Thus different individuals can have different numbers of neighbours. In the absence of the bias, each neighbour is chosen as an interlocutor with equal probability in an interaction. A generic model for social networks is the power-law degree distribution p z ∝ z −(1 + ν ) in which the exponent ν controls the heterogeneity of the network. Values of ν > 2 are regarded as homogeneous, in the sense that innovations spread in the population in the same way as on a network in which all speakers have the same number of neighbours (even though there is variation). When ν < 2, the networks become increasingly heterogeneous as ν is decreased: these feature a small number of highly-connected individuals and a large number of relatively isolated individuals. Evolutionary dynamics tend to run faster on heterogeneous networks [ 45 – 47 ], and there is some evidence that human social networks are heterogeneous (1.1 < ν < 1.3, [ 48 – 50 ]). Fig 4 illustrates the distinction between homogeneous and heterogeneous random networks.

The case ν > 2 (left) corresponds to a homogeneous network in which individuals all have a similar number of neighbours. The case ν < 2 (right) is heterogeneous: the central individuals are well-connected whilst the peripheral individuals are not.
The interlocutor bias is implemented by choosing a neighbour m with a probability proportional to 1 + sx m instead of uniformly. The selection strength s serves to favour (if s > 0) or disfavour (if s < 0) the innovation, which may originate in one of a number of processes. For example, in the variational learning framework [ 4 ], there is a systematic bias towards a grammar that parses a larger number of sentences. In a sociolinguistic setting, association between a linguistic variant and a socially prestigious group may lead to a bias towards (or against) that variant [ 10 , 51 ]. The case s = 0 describes a neutral model for language change, which has been discussed in the context of new-dialect formation [ 52 , 53 ].
We emphasise that a large number of models for language learning and use that have been discussed in the literature fall into the Wright-Fisher class, even though they may differ in detail and may not be presented as such. A non-exhaustive list includes those that appeal to cue-based learning [ 39 ], Bayesian learning from one or more teachers [ 54 – 56 ], variational learning [ 4 ] and usage-based models [ 57 ]. Moreover, the Wright-Fisher model has been used as a phenomenological model for changes in word frequencies [ 58 – 60 ].
We conclude this section with a formal mathematical specification of the Wright-Fisher model. The distribution P ( x , t ) of the innovation frequency, x , at the population level, at a time t after it is originated, is generally well-described by the forward Kolmogorov equation
in which a dot and prime denote derivatives with respect to t and x , respectively [ 27 , 61 ]. The parameters T M , s and N e correspond to a memory lifetime, an innovation bias and an effective population size, respectively. We emphasise that this equation applies between successive origination events, and describes the process by which the innovation propagates (rises to x = 1) or fails (falls to x = 0). Therefore the origination rate does not appear in this equation. However, it does enter into a correction factor, set out in S1 Appendix , that accounts for the possibility that a second origination occurs before either of these endpoints is reached.
The main difference between models within the Wright-Fisher class is how T M , s and N e relate to the parameters that apply to a specific model. In the present case, which has the set of parameters specified in Table 2 , we have T M = 1/( Rϵ ), s is as specified above and N e = N ( z ¯ 2 / z 2 ¯ ) / ϵ in which z is the number of neighbours a speaker has on the social network, and the overline denotes an average over speakers [ 45 – 47 ].
The parameters in the Origin-Fixation model that characterise the dynamics at the population scale can all be expressed in terms of those relating to the behaviour of individuals (see Data and Methods ).
In S1 Appendix we demonstrate that Eq 3 applies more generally than to the specific agent-based model set out here, and furthermore that the quantities T M , s and N e have a similar interpretation. This is achieved by considering a model that has many additional features—for example, ongoing birth and death of speakers, changes in social network structure and variation in interaction rates between speakers and over time—and showing that the changes in the innovation frequency x over short time intervals are the same as those described by Eq 3 . Therefore the results we present below do not rely on this model being an accurate representation of language learning and use.
Connection to origin-fixation model
We connect the individual to the population scale by determining how the parameters in the origin-fixation model (also specified in Table 2 ) relate to those in the Wright-Fisher model. The origination rates ω i are given by the formula ω i = NRη i Q ( ϵ / N ), where N is the number of speakers in the speech community, η i is the individual innovation rate per interaction, R is the interaction rate and Q ( x 0 ) is the probability that an innovation goes to fixation starting from some frequency x 0 . In the Wright-Fisher model, this initial frequency is x 0 = ϵ / N , because exactly one speaker uses the innovation with probability ϵ . We then have
This result is obtained by solving the backward equation that corresponds to Eq 3 (see [ 27 , 36 ] and S1 Appendix ). We see that the effective population size, N e (which depends on the actual population size N , the update fraction ϵ and the social network structure) plays an important part in determining the probability that an innovation propagates. It also determines how quickly an innovation may reach fixation. Numerical methods, described in S1 Appendix with the code available at [ 62 ], are used to determine exactly how the mean and the variance in the fixation time, T F ¯ and σ F 2 , in the origin-fixation model depend on the Wright-Fisher model parameters. Here we note that the characteristic timescale is of order T M N e when the bias s is small, and of order T M ln( N e ) when it is large, which turns out to have important consequences for the plausibility of the historical data for specific models of language learning and use in our analysis below.
In summary, then, our basic approach is to use the origin-fixation model to determine the likelihood of an observed set of historical language changes. The parameters in this model are obtained from an underlying Wright-Fisher model, so that we may understand—for example—which learning rates, biases and social network structure are more or less well supported by the historical data. As we have argued, our findings do not depend on the detailed structure of the Wright-Fisher model. The crucial component is that a speaker’s behaviour can be represented by an innovation frequency x , and that this is affected by learning from or using language with other members of the speech community over time.
We now compare the likelihood of the empirically attested set of language changes (detailed in S1 Appendix ) under different assumptions on the underlying behaviour of individuals in the respective populations. An appropriate measure for likelihood comparison is the Akaike Information Criterion, corrected for small sample sizes (AIC c , [ 63 ]), as the models we consider have different structures. It is defined as
where k is the number of free parameters in the model, n is the number of observations and L is the likelihood of those n observations, as determined from the origin-fixation model. An observation is the sequence of transitions between different stages of a grammaticalisation cycle over a specified historical time period for a given language, as tabulated in S1 Appendix . The number of observations is therefore the number of languages in the sample (52 for both articles).
The difference in the AIC c value between two models, denoted ΔAIC c , gives a measure of how much the model with the lower AIC c score is preferred over the other. Models with more free parameters (higher k ) can be dispreferred even when the data likelihood increases as a result of increasing parameters. For nested models, this increase is inevitable, but for models with different structures, AIC c remains valid as it is based on general information theoretic principles [ 63 ]. Given two candidate models and a sufficiently large number of observations, e Δ AIC c / 2 provides an estimate of the probability that the model with the higher AIC c better describes the data than that with the lower value. There is some freedom to choose the value of ΔAIC c at which one discards the inferior model. In this work we take a value of around 10 (corresponding to a likelihood ratio of around 150) as indicative of the model with the higher AIC c becoming too implausible to consider further. However since there is some flexibility in this regard, we will generally show the dependence of ΔAIC c on model parameters, so one can gauge the scale of the likelihood differences between models. It is important to note that such model comparisons do not in themselves validate the superior model: for this one needs to consider goodness-of-fit measures as well [ 63 ].
We begin by establishing a baseline against which different individual-level mechanisms of language change will be compared. In this baseline model, language changes occur at the population level as a Poisson process. We emphasise from the outset that this is not an individual-based model of language change: changes in the population occur autonomously without reference to individual speakers. Nevertheless this model helps to illustrate our statistical approach and, as we discuss below, it also provides valuable insights into why particular individual-based mechanisms are found to provide more or less plausible explanations of historical language changes at the population level.
Poisson baseline
In the baseline model, we assume that a change from stage i to stage i + 1 of the cycle occurs as a Poisson process at a constant rate ω i = ω ¯ / ( 4 f i ) in each population, where f i is the fraction of the world’s languages that is currently at stage i of the cycle ( Table 1 ). This factor of f i ensures that the stationary distribution in the baseline model matches the contemporary WALS distribution. This model is equivalent to the origin-fixation model of Fig 1a , with instantaneous fixation ( T F = 0). This model has one free parameter, the mean rate of language change, ω ¯ , which is estimated by maximising the likelihood of the data.
The maximum likelihood value of ω ¯ , the corresponding AIC c , a classical p -value and two goodness-of-fit statistics are presented in Table 3 . The p -value is the probability, within the model, of all possible transitions between stages of the relevant grammaticalisation cycle over the relevant historical period for each language whose likelihood is lower than the transitions that actually occurred. This p -value can be interpreted in the usual way, with a low p -value indicating a likely departure from the model assumptions.
ω ¯ is the maximum likelihood rate of change and AIC c the corrected Akaike information criterion. p is the cumulative probability of events less likely than the observation. Overdispersion measures goodness of fit, with values closer to 1 indicating a better fit. p and overdispersion are estimated from 10 6 Monte Carlo simulations of the process.
By itself, an AIC c score (or differences between them) does not furnish any information about how well a particular model fits the data. To gain an insight into goodness-of-fit, we consider the overdispersion of two random variables X (specified below) which quantifies the extent to which observed deviations of X from their mean values X ¯ within the model are consistent with the expected deviations. For a given observation, the overdispersion is defined as O X = ( X − X ¯ ) 2 / Var ( X ) , that is, the ratio of the observed square deviation to its expected value. If the overdispersion is close to 1, the deviations are as expected, and we conclude that the distribution of X is well-predicted [ 63 ]. For a given language, the two quantities X are: (i) the total number of language changes in the historical period; and (ii) a binary variable that equals 1 if at least one change occurred, or 0 otherwise. We average over all languages in the sample to obtain the single measure that is presented in Table 3 .
The low overdispersion scores suggest that this baseline model provides a good description of changes in the indefinite article, whilst it performs less well for the definite article. A likely source of this difference is the larger number of languages whose definite article changes rapidly compared to the indefinite article, as can be seen from Fig 2 . It is further possible that assumptions made about the data (for example, that the distribution of articles is stationary, that changes in different languages are independent, or, indeed, that the fixation time can be idealised to zero) do not strictly hold. We also remark that the second overdispersion measure is less sensitive than the first: however, it turns out that this is easier to calculate for individual-based models, and we will take a large deviation of this measure from 1 as providing a strong indication of a poor fit to the data.
It is remarkable that this simple model seems to provide a reasonably good fit to the data, particularly in view of an ongoing discussion about the role of population size in language structure and change [ 64 – 67 ] (a point we return to in the Discussion). The Poisson model explicitly assumes that the phenomenological rate of change ω ¯ is constant across all populations, and that each language change is able to propagate rapidly from origination to fixation. These observations suggest that we should expect to find more plausible accounts of historical language change in individual-based models whose emergent population-level dynamics share these properties.
Child-based models of language change
We now examine the constraints on the population-level dynamics of language change that arise from assuming that language change occurs primarily through the process of childhood language acquisition (e.g., [ 2 , 4 , 39 , 54 , 56 , 68 , 69 ]). As noted above, such theories imply that the rate, R , at which a grammar can be updated is once per human generation, which we take to be once every 25 years (i.e, R = 0.04yr −1 ). In the case where learning causes children converge on a single grammar (i.e., categorical use of one of the four article variants), we take ϵ = 1. In the case of variational learners (e.g. [ 4 ]), speakers can entertain mixtures of grammars: this can be realised with ϵ < 1. We consider the categorical case first.
The literature on child-based theories rarely refers to population structure. We therefore begin by assuming that populations are homogeneous: that is, that each child learns from roughly the same number of (cultural) parents, and conversely, that each adult provides linguistic input to roughly the same number of (cultural) offspring. Under these conditions, the emergent origination rates and fixation times in each population depends on a core size that is equal to the population’s actual size (see Methods ). It is therefore necessary for us to estimate the population (speech community) size for each language over the historical period for which empirical data exist. In S1 Appendix , we set out the procedure that we use to estimate the mean population size for each language over its recorded period of change. This is then used as the core population size for that language in our analysis.
This leaves just two unconstrained parameters, the mean rate η ¯ at which innovations arise in individual instances of language learning (the “error” rate, in the child-based model), and the selective bias s in favour of the innovation. Our strategy is to choose the value of η ¯ that maximises the likelihood of the data set given all other parameter settings, and to plot ΔAIC c with respect to the Poisson baseline model as a function of the selection strength s so that we can see where the support for the child-based model is strongest. Here, we treat the individual-based model as the candidate model, so ΔAIC c = AIC c (candidate) − AIC c (baseline) is positive when the evidence supports the baseline model, and negative when the evidence supports the candidate model. The resulting plot is shown in Fig 5 , along with a corresponding plot of the second of the two overdispersion measures considered for the Poisson baseline model.

ΔAIC c (panels a–c) and binary overdispersion (c–f) for negative (a and d) and positive (b, c, d and f) selection strength s within a child-based learning paradigm. The smallest values of both measures (which indicate better fits to the data) are obtained for strong positive selection ( s > 1, highlighted in panels c and f which has a larger vertical scale). The ΔAIC c values are far away from the shaded zone where ΔAIC c ≤ 10 and the evidence in favour of the child-based model starts to become comparable with that of the baseline.
We find that across the entire range of selection strengths s , support for the child-based model is very poor. The greatest plausibility (relative to the Poisson baseline) is obtained where ΔAIC c is smallest: this happens in the limit of infinite selection strength. As can be seen from the rightmost panels of Fig 5 , the values of ΔAIC c in these regions are still rather large, reaching asymyptotes at 204 and 58.4 for definite and indefinite articles, respectively (both to 3 s.f.). This corresponds to the evidence in favour of the candidate model being 10 44 (definite) and 10 13 (indefinite) times smaller than the baseline.
However, this comparison with the Poisson baseline is not entirely fair, as this phenomenological population-level dynamics may not be accessible for any combination of parameters in the individual-based model. For this reason we must also check the goodness-of-fit via the overdispersion measure. Again we find anomalously large values, the asymptotic values being 31300 (definite) and 226 (indefinite), suggesting that the assumptions made about the underlying dynamics of language change are wildly inconsistent with the historical data. Throughout this investigation, we found that ΔAIC c correlates strongly with goodness-of-fit, and so in the rest of this work we show only ΔAIC c , and investigate whether alternative assumptions on the individual-level behaviour are capable of delivering a much smaller ΔAIC c .
To focus this investigation, it is instructive to understand why the empirical data have such a low likelihood (and therewith high ΔAIC c ) within the child-based model. As previously noted, the effective population size (which here, is the same as the actual population size) is of fundamental importance in population genetics models [ 27 ]. When the selection strength, s , is large, each individual innovation is likely to propagate, and the mean origination rate (at the population level) increases linearly with the population size. On the other hand, when the selection strength is small, the origination rate is roughly constant but fixation time T F is proportional to the population size. Since the historical average population sizes in the empirical data set range across six orders of magnitude, then either the origination rate or the fixation time exhibits this wide variation in the child-based model. The fact that the Poisson baseline, which has no dependence on population size at all, apparently provides a much better fit, suggests that individual-based models in which origination rates and fixation times vary more weakly with population size than in the child-based model should be more favoured. Variants of the child-based model in which grammars are probabilistic [ 4 ] do not fall into this class: these have ϵ < 1, which implies a fixation time N / ϵ 2 when s is small. That is, these models are more sensitive to population size than models that allow children to acquire only a single grammar.
Usage-based models of language change
In a usage-based model, a speaker’s grammar may change across their lifespan [ 15 ], in principle in response to every utterance they hear (i.e., up to around 10 7 times a year [ 70 ]). This has the potential to weaken the sensitivity to population size: if a large number of interactions between speakers is required for a change to propagate through the population, then the higher interaction frequency in the usage-based model gives the change a greater chance of going through on the attested historical timescales. However, this effect may be tempered by the fact that the change to each grammar is smaller in each interaction, which has the opposite effect.
To explore the interaction between an increased interaction rate R , and lower impact on the grammar ϵ , it is convenient to work with the memory time T M = 1/( Rϵ ), which is the expected lifetime of a single item of linguistic experience in the speaker’s mind. Considering again the case of homogeneous populations, we compare in Fig 6 the class of usage-based models with no selection ( s = 0) over the reasonable range of R at fixed memory times T M = 1/( Rϵ ) against the baseline model. Note that the dotted parts of the curves correspond to an unphysical parameter value of ϵ > 1. From these ΔAIC c plots, we see that our intuition that an increased interaction rate allows changes to go through more easily is correct. We achieve greater plausibility than the most plausible child-based model when memory times are short, specifically less than one hour. We note that we can approach the plausibility of the Poisson baseline if we allow T M to be as short as one minute.

ΔAIC c in the usage-based model as a function of interaction rate R for the definite (panels a and c) and indefinite (b and d) articles. Along each curve, the memory time T M = 1/( Rϵ ) is held constant. In panels a and b, T M ranges from 25 years (top line) to 1 hour (bottom line). Panels c and d focus on the range of interest where greater plausibility than the child-based model is achieved: the horizontal lines correspond to the s = ∞ asymptotes in Fig 5 . Dotted lines indicate where the usage-based model is unphysical ( ϵ > 1) and the shaded grey region indicates where the fit starts to become comparable to the Poisson baseline (ΔAIC c < 10).
Although shorter memory times in the individual allow for a faster rate of change in the population, the basic property of fixation times being proportional to the population size is unaffected. This is why we find that individual memory times must be very short (perhaps unreasonably so, see Discussion ) to improve on child-based models. Furthermore, there is stronger sensitivity to population size when selection is operating ( s ≠ 0), which leads to lower plausibility gains with respect to the child-based model than in the neutral case ( s = 0). This suggests that one needs to appeal beyond merely shorter memory times to explain the apparently weak effect of population size on article grammaticalisation cycles.
Social network effects
Studies of the Wright-Fisher and related models on heterogeneous networks [ 45 – 47 ] show that these can weaken the effect of population size on characteristic timescales of change. As discussed in the Wright-Fisher model section, above, we model social networks as those with a power law distribution P ( z )∼ z −(1+ ν ) . We recall that the exponent ν controls the heterogeneity of the network, with lower values of ν corresponding to greater heterogeneity: see also Fig 4 . On such networks, the mean fixation time is proportional to an effective population size N e ∼ N 2−2/ ν which is less than the actual size N if 1 < ν < 2 [ 45 – 47 ]. In the context of language change, we can think of N e as measuring the size of a core population who exert much greater influence over the periphery than vice versa. Empirical studies of large networks (like friendship networks) provide some support for this power-law distribution with an exponent ν in the range 1.1 < ν < 1.3 [ 48 – 50 ].
In Fig 7 we examine how the plausibility of both the child- and usage-based models investigated above changes when individual speakers in the model are arranged on complex network structures. This confirms our expectation that models in which timescales of change are less sensitive to population size receive greater support from the data. As previously, the usage-based model provides a more plausible description of language change than the child-based model; moreover, the range of selection strengths and memory times over which a fit comparable to that provided by the Poisson process is much larger than on homogeneous networks.

ΔAIC c for models on heterogeneous social networks for the definite (panels a, c and e) and indefinite (b, d and f) articles as a function of selection strength s . Panels a–d show the effect of different degree exponents ν on the child-based model: panel c and d zoom in on ΔAIC c ≤ 100, showing that plausibility is obtained only for the indefinite article over a limited range of s and ν . Panels e and f show the effect of memory lifetime at fixed ν = 1.2 and ϵ = 1. The horizontal line has the same meaning as in Fig 6 . The dark and light shaded regions correspond to ΔAIC c < 10 and ΔAIC c < 20, respectively, which allows one to see the sensitivity to different evidence thresholds.
We see from Fig 7 that the most plausible models in the space under consideration are those in which selection is relatively weak. This is consistent with recent observations [ 58 – 60 ] that the dynamics of word frequencies appear to be subject to the evolutionary forces of both random drift and selection (i.e., neither is so strong that it dominates the other). Moreover, a number of studies (e.g., [ 46 , 71 , 72 ]) have indicated that heterogeneity tends to lower the barrier to invasion of an infection, mutation or innovation. This possibly points towards a picture whereby the different grammatical structures that are attested cross-linguistically are somewhat similar in their fitness, but may nevertheless replace one another over time in the systematic way that is observed historically due to the manner in which human societies are structured.
The aims of this work were twofold. First, we established how specific assumptions on the way in which individuals learn and use language translate to language change at the population scale. Second, we used historical data for the latter to identify which theories and mechanisms as to how individuals change the language of their speech community have greater empirical support.
Our main result is that if we impose the constraints that arise from assuming that childhood language learning is the driver of language change, there is no combination of the remaining free parameters that provides a good fit to the empirical data. The observed changes are many orders of magnitude more likely in regions of parameter space that correspond to other theories. The reason why the support for the child-based theory is so poor lies in a strong dependence of characteristic timescales at the population level on the underlying population size. If any selective bias in favour of the innovation is weak, the time taken for a change to propagate through a large speech community (the fixation time) is very much longer than the 100 years or so that is seen historically. If selection is strong, changes propagate quickly but then the rate at which successful changes are originated varies strongly with population size. The empirical data apparently show much less sensitivity to population size than the child-based theory implies.
In fact, throughout this work, we have found that the baseline model, which has no dependence on population size, fits the historical data well. One way to construe the baseline model is as changes originating once every 1000 years or so in every population, with changes then propagating rapidly through the population. This suggests that the mechanisms that have stronger empirical support are those that have these characteristics.
We acknowledge that our analysis is based on a single pair of features (the definite and indefinite articles) that are relatively unstable and are correlated. It is due to these correlations that we treated them separately (rather than combining them together into a single likelihood measure, which would assume independence). Nevertheless, comparison of the two articles is informative about how sensitive the analysis is to the details of which languages undergo a specific sequence of changes, as this does vary between the two articles. Overall, we find that it is the overall rate of language change combined with its weak sensitivity to population size that most strongly determines the plausibility of a given individual-based theory.
It is, however, possible that the dynamics of articles are unrepresentative of grammatical features more generally, and that our conclusions therefore do not generalise. We argue that this is unlikely. Regarding overall timescales of change, it is well established, by different analyses [ 31 – 33 ], that articles rank amongst the least stable of grammatical features and that others change more slowly. Basic word order lies at the opposite end of the spectrum, and the lifetime of given word orders have been estimated as ranging from 1000–100000 years [ 73 ]. That is, these most stable structures persist for a timescale that ranges from around the same order of magnitude as articles to two orders of magnitude longer. A quick way to estimate the plausibility of the child-based theory for basic word order from our findings for articles is to consider a generational turnover that is increased by two orders of magnitude (i.e., from 25 years to around 3 months). Here we find a plausible account is possible on sufficiently heterogeneous social networks (see Fig 7 ). This implies that the child-based theory could, at best, account for only the most stable grammatical structures, and does not offer a single explanation for language change that applies across the stability spectrum. The rate of population turnover imposes a fundamental minimum rate of language change which lies above that for unstable features in the child-based account, but potentially below in the usage-based account. Therefore the latter is capable of providing a common explanation for changes across the full stability spectrum.
It is harder to establish whether the weak sensitivity to population size is a feature of other grammatical changes. A detailed record of the history of each feature of interest across many languages is required for a conclusive assessment, data that is difficult to obtain (particularly for more stable features, where greater time depth is required to see a sufficiently large number of changes). However, a number of studies that have directly examined the relationship between population size and various aspects of language structure or change [ 64 – 67 ] have tended to conclude that where there is an effect, it is weak. For example, [ 67 ] reports rates of gain and loss that scale sublinearly with the population size, consistent with the behaviour of Wright-Fisher models on heterogeneous social networks. Moreover, the fact that different methods [ 31 – 33 ] of characterising the stability of a feature with a single metric are broadly consistent suggests that they do not vary significantly over space and time. Indeed, Wichmann and Holman [ 31 ] have argued that the notion of stability is intrinsic to a feature and does not vary geographically. Given these considerations, it seems reasonable to conclude that weak population-size dependence is a generic property of language change, and not peculiar to articles.
We have identified two individual-level mechanisms that may contribute towards such a weak effect of population size on the rate of grammatical change. The first of these is provided for by usage-based accounts of language change which allow individuals to modify their behaviour across their lifespan, not just in the childhood language acquisition period. With more opportunities for individual behaviour to change per unit time, these theories allow changes to propagate through large speech communities more quickly. If the bias towards the innovation (the selection strength, s ) is close to zero and the innovation rate per interaction is also small, changes at the population scale can then occur at roughly the same rate in different speech communities.
In addition to small selection and innovation rates, this mechanism further requires a short memory lifetime in comparison to the lifetime of an individual (days or less, depending on social network structure). Taken at face value, such memory lifetimes may be considered unreasonably short. Here, we advise caution. First, a short memory does not imply that individual speakers are continually changing their behaviour: individual speakers can remain constant in their behaviour for as long as those around them do. If innovations rarely propagate, then most speakers will be exposed to existing conventions and continue to adhere to them, even though during a period of change they may alter their behaviour relatively quickly, albeit in small increments. There is some evidence that such changes can occur in older speakers as well as younger speakers, for example, in a study of Montreal French [ 12 ]. Meanwhile, research on priming [ 74 , 75 ] shows that individual linguistic utterances can affect a speaker’s behaviour in interactions in the very short term before fading away. It would be worth understanding whether such effects could effect more permanent changes, for example, when a change is in progress in a speech community, as this might then imply a shorter effective memory time at the individual level than intuition grounded in everyday experience suggests.
The second mechanism that can reduce the sensitivity of grammatical change to population size are social network effects. Specifically, heterogeneous networks, in which a small number of well-connected speakers interact with a large number of poorly-connected speakers, lead to an effective population size (and therewith a characteristic timescale for change) that increases sublinearly with population size. Since this heterogeneity is a feature of certain social networks (e.g., those relating to phone calls, movie collaborations and social media [ 48 – 50 ]), it is reasonable to assume that this is a property of human social interactions more generally. It is interesting to note that sublinear relationships between rates of change and population sizes have been reported in other empirical studies of language change [ 64 , 67 ]. Heterogeneous social networks offer one possible explanation for this phenomenon. To investigate this possibility further, it would be interesting to obtain more concrete information about the structure of linguistic interactions as well as how these stratify by age. If it were found, for example, that children’s networks are more homogeneous than adult’s, then this would point towards adults playing a key role in propagating an innovation throughout the speech community.
Although our statements about the relationship between individual behaviour and population-level change are grounded in a specific model of individual behaviour, we do not expect them to change if a different model was used. The reason for this is that any model that involves individual agents basing some or all of their future behaviour on that displayed by others (whether through learning or use) is expected to fall into the Wright-Fisher class [ 37 ]. The precise relationship between parameter values in the individual-based model and those in the population-level origin-fixation model may vary between models: however, in any two models with similar memory lifetimes, innovation biases and social network structures would be expected to have the same behaviour at the population scale. In S1 Appendix , we demonstrate this in the case of an extended model in which all properties vary between speakers, in which there is turnover in the population and social networks change over time.
This is not intended to imply that every feasible influence on language change is contained within the Wright-Fisher model used here (at least, at some level of abstraction). For example, we have excluded the possibility of a conformity bias [ 76 , 77 ], wherein speakers suppress minority variants in favour of those in the majority. Such a bias however makes it increasingly difficult for innovations to propagate as the population increases in size, and therefore would be expected to exacerbate the problems of sensitivity to population size. We have also assumed that factors influencing individual linguistic behaviour are constant over space and time. Specifically, social factors like prestige effects have been excluded, and it would be interesting in future work to establish whether these lead more readily to plausible accounts of historical language change.
Supporting information
S1 appendix, funding statement.
The author(s) received no specific funding for this work.
Data Availability
On Physical Thoughts of Language Change
Ieee account.
- Change Username/Password
- Update Address
Purchase Details
- Payment Options
- Order History
- View Purchased Documents
Profile Information
- Communications Preferences
- Profession and Education
- Technical Interests
- US & Canada: +1 800 678 4333
- Worldwide: +1 732 981 0060
- Contact & Support
- About IEEE Xplore
- Accessibility
- Terms of Use
- Nondiscrimination Policy
- Privacy & Opting Out of Cookies
A not-for-profit organization, IEEE is the world's largest technical professional organization dedicated to advancing technology for the benefit of humanity. © Copyright 2024 IEEE - All rights reserved. Use of this web site signifies your agreement to the terms and conditions.
Academia.edu no longer supports Internet Explorer.
To browse Academia.edu and the wider internet faster and more securely, please take a few seconds to upgrade your browser .
- We're Hiring!
- Help Center
Language Change
- Most Cited Papers
- Most Downloaded Papers
- Newest Papers
- Save to Library
- Last »
- Language Variation and Change Follow Following
- Historical Linguistics Follow Following
- Grammaticalization Follow Following
- Diachronic Linguistics (Or Historical Linguistics) Follow Following
- Language contact Follow Following
- Linguistics Follow Following
- Loanwords, Language contact & change Follow Following
- Contact Linguistics Follow Following
- Language Variation Follow Following
- Linguistic Typology Follow Following
Enter the email address you signed up with and we'll email you a reset link.
- Academia.edu Publishing
- We're Hiring!
- Help Center
- Find new research papers in:
- Health Sciences
- Earth Sciences
- Cognitive Science
- Mathematics
- Computer Science
- Academia ©2024
- Architecture and Design
- Asian and Pacific Studies
- Business and Economics
- Classical and Ancient Near Eastern Studies
- Computer Sciences
- Cultural Studies
- Engineering
- General Interest
- Geosciences
- Industrial Chemistry
- Islamic and Middle Eastern Studies
- Jewish Studies
- Library and Information Science, Book Studies
- Life Sciences
- Linguistics and Semiotics
- Literary Studies
- Materials Sciences
- Mathematics
- Social Sciences
- Sports and Recreation
- Theology and Religion
- Publish your article
- The role of authors
- Promoting your article
- Abstracting & indexing
- Publishing Ethics
- Why publish with De Gruyter
- How to publish with De Gruyter
- Our book series
- Our subject areas
- Your digital product at De Gruyter
- Contribute to our reference works
- Product information
- Tools & resources
- Product Information
- Promotional Materials
- Orders and Inquiries
- FAQ for Library Suppliers and Book Sellers
- Repository Policy
- Free access policy
- Open Access agreements
- Database portals
- For Authors
- Customer service
- People + Culture
- Journal Management
- How to join us
- Working at De Gruyter
- Mission & Vision
- De Gruyter Foundation
- De Gruyter Ebound
- Our Responsibility
- Partner publishers

Your purchase has been completed. Your documents are now available to view.
Research Guide on Language Change
- Edited by: Edgar C. Polomé
- X / Twitter
Please login or register with De Gruyter to order this product.
- Language: English
- Publisher: De Gruyter Mouton
- Copyright year: 1990
- Edition: Reprint 2011
- Front matter: 9
- Main content: 564
- Other: Num. figs.
- Keywords: Sprachwandel ; Literaturbericht
- Published: June 15, 2011
- ISBN: 9783110875379
- Published: December 1, 1990
- ISBN: 9783110120462
Help | Advanced Search
Computer Science > Computation and Language
Title: dijiang: efficient large language models through compact kernelization.
Abstract: In an effort to reduce the computational load of Transformers, research on linear attention has gained significant momentum. However, the improvement strategies for attention mechanisms typically necessitate extensive retraining, which is impractical for large language models with a vast array of parameters. In this paper, we present DiJiang, a novel Frequency Domain Kernelization approach that enables the transformation of a pre-trained vanilla Transformer into a linear complexity model with little training costs. By employing a weighted Quasi-Monte Carlo method for sampling, the proposed approach theoretically offers superior approximation efficiency. To further reduce the training computational complexity, our kernelization is based on Discrete Cosine Transform (DCT) operations. Extensive experiments demonstrate that the proposed method achieves comparable performance to the original Transformer, but with significantly reduced training costs and much faster inference speeds. Our DiJiang-7B achieves comparable performance with LLaMA2-7B on various benchmark while requires only about 1/50 training cost. Code is available at this https URL .
Submission history
Access paper:.
- HTML (experimental)
- Other Formats
References & Citations
- Google Scholar
- Semantic Scholar
BibTeX formatted citation

Bibliographic and Citation Tools
Code, data and media associated with this article, recommenders and search tools.
- Institution
arXivLabs: experimental projects with community collaborators
arXivLabs is a framework that allows collaborators to develop and share new arXiv features directly on our website.
Both individuals and organizations that work with arXivLabs have embraced and accepted our values of openness, community, excellence, and user data privacy. arXiv is committed to these values and only works with partners that adhere to them.
Have an idea for a project that will add value for arXiv's community? Learn more about arXivLabs .
Thank you for visiting nature.com. You are using a browser version with limited support for CSS. To obtain the best experience, we recommend you use a more up to date browser (or turn off compatibility mode in Internet Explorer). In the meantime, to ensure continued support, we are displaying the site without styles and JavaScript.
- View all journals
- My Account Login
- Explore content
- About the journal
- Publish with us
- Sign up for alerts
- Open access
- Published: 02 April 2024
Large language models could change the future of behavioral healthcare: a proposal for responsible development and evaluation
- Elizabeth C. Stade 1 , 2 , 3 ,
- Shannon Wiltsey Stirman 1 , 2 ,
- Lyle H. Ungar 4 ,
- Cody L. Boland 1 ,
- H. Andrew Schwartz 5 ,
- David B. Yaden 6 ,
- João Sedoc 7 ,
- Robert J. DeRubeis 8 ,
- Robb Willer 9 &
- Johannes C. Eichstaedt 3
npj Mental Health Research volume 3 , Article number: 12 ( 2024 ) Cite this article
113 Accesses
2 Altmetric
Metrics details
- Psychiatric disorders
Large language models (LLMs) such as Open AI’s GPT-4 (which power ChatGPT) and Google’s Gemini, built on artificial intelligence, hold immense potential to support, augment, or even eventually automate psychotherapy. Enthusiasm about such applications is mounting in the field as well as industry. These developments promise to address insufficient mental healthcare system capacity and scale individual access to personalized treatments. However, clinical psychology is an uncommonly high stakes application domain for AI systems, as responsible and evidence-based therapy requires nuanced expertise. This paper provides a roadmap for the ambitious yet responsible application of clinical LLMs in psychotherapy. First, a technical overview of clinical LLMs is presented. Second, the stages of integration of LLMs into psychotherapy are discussed while highlighting parallels to the development of autonomous vehicle technology. Third, potential applications of LLMs in clinical care, training, and research are discussed, highlighting areas of risk given the complex nature of psychotherapy. Fourth, recommendations for the responsible development and evaluation of clinical LLMs are provided, which include centering clinical science, involving robust interdisciplinary collaboration, and attending to issues like assessment, risk detection, transparency, and bias. Lastly, a vision is outlined for how LLMs might enable a new generation of studies of evidence-based interventions at scale, and how these studies may challenge assumptions about psychotherapy.
Similar content being viewed by others
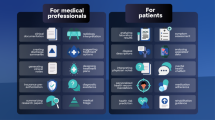
The imperative for regulatory oversight of large language models (or generative AI) in healthcare
Bertalan Meskó & Eric J. Topol
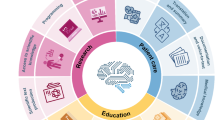
The future landscape of large language models in medicine
Jan Clusmann, Fiona R. Kolbinger, … Jakob Nikolas Kather
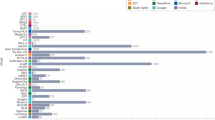
Large language models in medicine
Arun James Thirunavukarasu, Darren Shu Jeng Ting, … Daniel Shu Wei Ting
Introduction
Large language models (LLMs), built on artificial intelligence (AI) – such as Open AI’s GPT-4 (which power ChatGPT) and Google’s Gemini – are breakthrough technologies that can read, summarize, and generate text. LLMs have a wide range of abilities, including serving as conversational agents (chatbots), generating essays and stories, translating between languages, writing code, and diagnosing illness 1 . With these capacities, LLMs are influencing many fields, including education, media, software engineering, art, and medicine. They have started to be applied in the realm of behavioral healthcare, and consumers are already attempting to use LLMs for quasi-therapeutic purposes 2 .
Applications incorporating older forms of AI, including natural language processing (NLP) technology, have existed for decades 3 . For example, machine learning and NLP have been used to detect suicide risk 4 , identify the assignment of homework in psychotherapy sessions 5 , and identify patient emotions within psychotherapy 6 . Current applications of LLMs in the behavioral health field are far more nascent – they include tailoring an LLM to help peer counselors increase their expressions of empathy, which has been deployed with clients both in academic and commercial settings 2 , 7 . As another example, LLM applications have been used to identify therapists’ and clients’ behaviors in a motivational interviewing framework 8 , 9 .
Similarly, while algorithmic intelligence with NLP has been deployed in patient-facing behavioral health contexts, LLMs have not yet been heavily employed in these domains. For example, mental health chatbots Woebot and Tessa, which target depression and eating pathology respectively 10 , 11 , are rule-based and do not use LLMs (i.e., the application’s content is human-generated, and the chatbot’s responds based on predefined rules or decision trees 12 ). However, these and other existing chatbots frequently struggle to understand and respond to unanticipated user responses 10 , 13 , which likely contributes to their low engagement and high dropout rates 14 , 15 . LLMs may hold promise to fill some of these gaps, given their ability to flexibly generate human-like and context-dependent responses. A small number of patient-facing applications incorporating LLMs have been tested, including a research-based application to generate dialog for therapeutic counseling 16 , 17 , and an industry-based mental-health chatbot, Youper, which uses a mix of rule-based and generative AI 18 .
These early applications demonstrate the potential of LLMs in psychotherapy – as their use becomes more widespread, they will change many aspects of psychotherapy care delivery. However, despite the promise they may hold for this purpose, caution is warranted given the complex nature of psychopathology and psychotherapy. Psychotherapy delivery is an unusually complex, high-stakes domain vis-à-vis other LLM use cases. For example, in the productivity realm, with a “LLM co-pilot” summarizing meeting notes, the stakes are failing to maximize efficiency or helpfulness; in behavioral healthcare, the stakes may include improperly handling the risk of suicide or homicide.
While there are other applications of artificial intelligence that may involve high-stakes or life-or death decisions (e.g., self-driving cars), prediction and mitigation of risk in the case of psychotherapy is very nuanced, involving complex case conceptualization, the consideration of social and cultural contexts, and addressing unpredictable human behavior. Poor outcomes or ethical transgressions from clinical LLMs could run the risk of harming individuals, which may also be disproportionately publicized (as has occurred with other AI failures 19 ), which may damage public trust in the field of behavioral healthcare.
Therefore, developers of clinical LLMs need to act with special caution to prevent such consequences. Developing responsible clinical LLMs will be a challenging coordination problem, primarily because the technological developers who are typically responsible for product design and development lack clinical sensitivity and experience. Thus, behavioral health experts will need to play a critical role in guiding development and speaking to the potential limitations, ethical considerations, and risks of these applications.
Presented below is a discussion on the future of LLMs in behavioral healthcare from the perspective of both behavioral health providers and technologists. A brief overview of the technology underlying clinical LLMs is provided for the purposes of both educating clinical providers and to set the stage for further discussion regarding recommendations for development. The discussion then outlines various applications of LLMs to psychotherapy and provides a proposal for the cautious, phased development and evaluation of LLM-based applications for psychotherapy.
Overview of clinical LLMs
Clinical LLMs could take a wide variety of forms, spanning everything from brief interventions or circumscribed tools to augment therapy, to chatbots designed to provide psychotherapy in an autonomous manner. These applications could be patient-facing (e.g., providing psychoeducation to the patient), therapist-facing (e.g., offering options for interventions from which the therapist could select), trainee-facing (e.g., offering feedback on qualities of the trainee’s performance), or supervisor/consultant facing (e.g., summarizing supervisees’ therapy sessions in a high-level manner).
How language models work
Language models, or computational models of the probability of sequences of words, have existed for quite some time. The mathematical formulations date back to 20 and original use cases focused on compressing communication 21 and speech recognition 22 , 23 , 24 . Language modeling became a mainstay for choosing among candidate phrases in speech recognition and automatic translation systems but until recently, using such models for generating natural language found little success beyond abstract poetry 24 .
Large language models
The advent of large language models, enabled by a combination of the deep learning technique transformers 25 and increases in computing power, has opened new possibilities 26 . These models are first trained on massive amounts of data 27 , 28 using “unsupervised” learning in which the model’s task is to predict a given word in a sequence of words. The models can then be tailored to a specific task using methods, including prompting with examples or fine-tuning, some of which use no or small amounts of task-specific data (see Fig. 1 ) 28 , 29 . LLMs hold promise for clinical applications because they can parse human language and generate human-like responses, classify/score (i.e., annotate) text, and flexibly adopt conversational styles representative of different theoretical orientations.
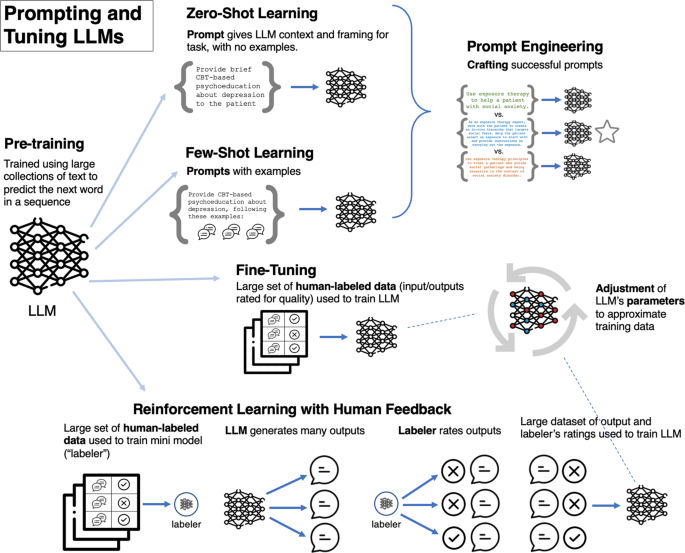
Figure was designed using image components from Flaticon.com.
LLMs and psychotherapy skills
For certain use cases, LLM show a promising ability to conduct tasks or skills needed for psychotherapy, such as conducting assessment, providing psychoeducation, or demonstrating interventions (see Fig. 2 ). Yet to date, clinical LLM products and prototypes have not demonstrated anywhere near the level of sophistication required to take the place of psychotherapy. For example, while an LLM can generate an alternative belief in the style of CBT, it remains to be seen whether it can engage in the type of turn-based, Socratic questioning that would be expected to produce cognitive change. This more generally highlights the gap that likely exists between simulating therapy skills and implementing them effectively to alleviate patient suffering. Given that psychotherapy transcripts are likely poorly represented in the training data for LLMs, and that privacy and ethical concerns make such representation challenging, prompt engineering may ultimately be the most appropriate fine-tuning approach for shaping LLM behavior in this manner.
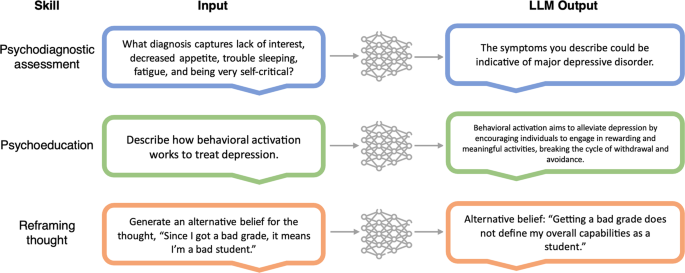
Note . Figure was designed using image component from Flaticon.com.
Clinical LLMs: stages of integration
The integration of LLMs into psychotherapy could be articulated as occurring along a continuum of stages spanning from assistive AI to fully autonomous AI (see Fig. 3 and Table 1 ). This continuum can be illustrated by models of AI integration in other fields, such as those used in the autonomous vehicle industry. For example, at one end of this continuum is the assistive AI (“machine in the loop”) stage, wherein the vehicle system has no ability to complete the primary tasks – acceleration, braking, and steering – on its own, but provides momentary assistance (e.g., automatic emergency breaking, lane departure warning) to increase driving quality or decrease burden on the driver. In the collaborative AI (“human in the loop”) stage, the vehicle system aids in the primary tasks, but requires human oversight (e.g., adaptive cruise control, lane keeping assistance). Finally, in fully autonomous AI, vehicles are self-driving and do not require human oversight. The stages of LLM integration into psychotherapy and their related functionalities are described below.
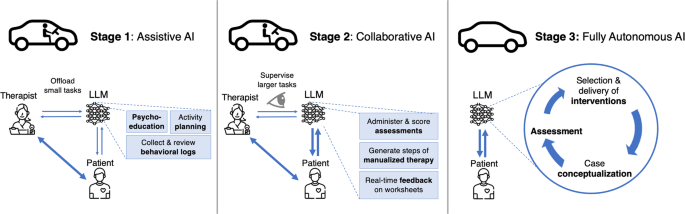
Stage 1: assistive LLMs
At the first stage in LLM integration, AI will be used as a tool to assist clinical providers and researchers with tasks that can easily be “offloaded” to AI assistants (Table 1 ; first row). As this is a preliminary step in integration, relevant tasks will be low-level, concrete, and circumscribed, such that they present a low level of risk. Examples of tasks could include assisting with collecting information for patient intakes or assessment, providing basic psychoeducation to patients, suggesting text edits for providers engaging in text-based care, and summarizing patient worksheets. Administratively, systems at this stage could also assist with clinical documentation by drafting session notes.
Stage 2: collaborative LLMs
Further along the continuum, AI systems will take the lead by providing or suggesting options for treatment planning and much of the therapy content, which humans will use their professional judgement to select from or tailor. For example, in the context of a text- or instant-message delivered structured psychotherapeutic intervention, the LLM might generate messages containing session content and assignments, which the therapist would review and adapt as needed before sending (Table 1 ; second row). A more advanced use of AI within the collaborative stage may entail a LLM providing a structured intervention in a semi-independent manner (e.g., as a chatbot), with a provider monitoring the discussion and stepping in to take control of the conversation as needed. The collaborative LLM stage has parallels to “guided self-help” approaches 30 .
Stage 3: fully autonomous LLMs
In the fully autonomous stage, AIs will achieve the greatest degree of scope and autonomy wherein a clinical LLM would perform a full range of clinical skills and interventions in an integrated manner without direct provider oversight (Table 1 ; third row). For example, an application at this stage might theoretically conduct a comprehensive assessment, select an appropriate intervention, and deliver a full course of therapy with no human intervention. In addition to clinical content, applications in this stage could integrate with the electronic health record to complete clinical documentation and report writing, schedule appointments and process billing. Fully autonomous applications offer the most scalable treatment method 30 .
Progression across the stages
Progression across the stages may not be linear; human oversight will be required to ensure that applications at greater stages of integration are safe for real world deployment. As different forms of psychopathology and their accompanying interventions vary in complexity, certain types of interventions will be simpler than others to develop as LLM applications. Interventions that are more concrete and standardized may be easier for models to deliver (and may be available sooner), such as circumscribed behavior change interventions (e.g., activity scheduling), as opposed to applications which include skills that are abstract in nature or emphasize cognitive change (e.g., Socratic questioning). Similarly, when it comes to full therapy protocols, LLM applications for interventions that are highly structured, behavioral, and protocolized (e.g., CBT for insomnia [CBT-I] or exposure therapy for specific phobia) may be available sooner than applications delivering highly flexible or personalized interventions (for example 31 ).
In theory, the final stage in the integration of LLMs into psychotherapy is fully autonomous delivery of psychotherapy which does not require human intervention or monitoring. However, it remains to be seen whether fully autonomous AI systems will reach a point at which they have been evaluated to be safe for deployment by the behavioral health community. Specific concerns include how well these systems are able to carry out case conceptualization on individuals with complex, highly comorbid symptom presentations, including accounting for current and past suicidality, substance use, safety concerns, medical comorbidities, and life circumstances and events (such as court dates and upcoming medical procedures). Similarly, it is unclear whether these systems will prove sufficiently adept at engaging patients over time 32 or accounting for and addressing contextual nuances in treatment (e.g., using exposure to treat a patient experiencing PTSD-related fear of leaving the house, who also lives in a neighborhood with high rates of crime). Furthermore, several skills which may be viewed as central to clinical work currently fall outside the purview of LLM systems, such as interpreting nonverbal behavior (e.g., fidgeting, eye-rolling), appropriately challenging a patient, addressing alliance ruptures, and making decisions about termination. Technological advances, including the approaching advent of multimodal language models that integrate text, images, video, and audio, may eventually begin to fill these gaps.
Beyond technical limitations, it remains to be decided whether complete automation is an appropriate end goal for behavioral healthcare, due to safety, legal, philosophical, and ethical concerns 33 . While some evidence indicates that humans can develop a therapeutic alliance with chatbots 34 , the long-term viability of such alliance building, and whether or not it produces undesirable downstream effects (e.g., altering an individual’s existing relationships or social skills) remains to be seen. Others have documented potentially harmful behavior of LLM chatbots, such as narcissistic tendencies 35 and expressed concerns about the potential for their undue influence on humans in addition to articulating societal risks associated with LLMs more generally 36 , 37 . The field will also need to grapple with questions of accountability and liability in the case of a fully autonomous clinical LLM application causing damage (e.g., identifying the responsible party in an incident of malpractice 38 ). For these and other reasons, some have argued against the implementation of fully autonomous systems in behavioral healthcare and healthcare more broadly 39 , 40 . Taken together, these issues and concerns may suggest that in the short and medium term, assistive or collaborative AI applications will be more appropriate for the provision of behavioral healthcare.
Applications of clinical LLMs
Given the vast nature of behavioral healthcare, there are seemingly endless applications of LLMs. Outlined below are some of the currently existing, imminently feasible, and potential long-term applications of clinical LLMs. Here we focus our discussion on applications directly related to the provision of, training in, and research on psychotherapy. As such, several important aspects of behavioral healthcare, such as initial symptom detection, psychological assessment and brief interventions (e.g., crisis counseling) are not explicitly discussed herein.
Imminent applications
Automating clinical administration tasks.
At the most basic level, LLMs have the potential to automate several time-consuming tasks associated with providing psychotherapy (Table 2 , first row). In addition to using session transcripts to summarize the session for the provider, there is potential for such models to integrate within electronic health records to aid with clinical documentation and conducting chart reviews. Clinical LLMs could also produce a handout for the patient that provides a personalized overview of the session, skills learned and assigned homework or between-session material.
Measuring treatment fidelity
A clinical LLM application could automate measurement of therapist fidelity to evidence-based practices (EBPs; Table 2 , second row), which can include measuring adherence to the treatment as designed, competence in delivering a specific therapy skill, treatment differentiation (whether multiple treatments being compared actually differ from one another), and treatment receipt (patient comprehension of, engagement with, and adherence to the therapy content) 41 , 42 . Measuring fidelity is crucial to the development, testing, dissemination, and implementation of EBPs, yet can be resource intensive and difficult to do reliably. In the future, clinical LLMs could computationally derive adherence and competence ratings, aiding research efforts and reducing therapist drift 43 . Traditional machine-learning models are already being used to assess fidelity to specific modalities 44 and other important constructs like counseling skills 45 and alliance 46 . Given their improved ability to consider context, LLMs will likely increase the accuracy with which these constructs are assessed.
Offering feedback on therapy worksheets and homework
LLM applications could also be developed deliver real-time feedback and support on patients’ between-session homework assignments (Table 2 , third row). For example, an LLM tailored to assist a patient to complete a CBT worksheet might provide clarification or aid in problem solving if the patient experiences difficulty (e.g., the patient was completing a thought log and having trouble differentiating between the thought and the emotion). This could help to “bridge the gap” between sessions and expedite patient skill development. Early evidence outside the AI realm 47 points to increasing worksheet competence as a fruitful clinical target.
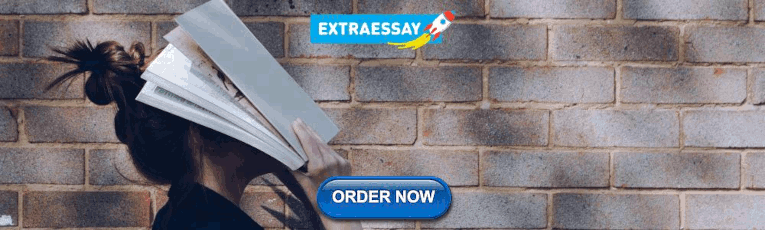
Automating aspects of supervision and training
LLMs could be used to provide feedback on psychotherapy or peer support sessions, especially for clinicians with less training and experience (i.e., peer counselors, lay health workers, psychotherapy trainees). For example, an LLM might be used to offer corrections and suggestions to the dialog of peer counselors (Table 2 , fourth row). This application has parallels to “task sharing,” a method used in the global mental health field by which nonprofessionals provide mental health care with the oversight by specialist workers to expand access to mental health services 48 . Some of this work is already underway, for example, as described above, using LLMs to support peer counselors 7 .
LLMs could also support supervision for psychotherapists learning new treatments (Table 2 , fifth row). Gold-standard methods of reviewing trainees’ work, like live observation or review of recorded sessions 49 , are time-consuming. LLMs could analyze entire therapy sessions and identify areas of improvement, offering a scalable approach for supervisors or consultants to review.
Potential long-term applications
It is important to note that many of the potential applications listed below are theoretical and have yet to be developed, let alone thoroughly evaluated. Furthermore, we use the term “clinical LLM” in recognition of the fact that when and under what circumstances the work of an LLM could be called psychotherapy is evolving and depends on how psychotherapy is defined.
Fully autonomous clinical care
As previously described, the final stage of clinical LLM development could involve an LLM that can independently conduct comprehensive behavioral healthcare. This could involve all aspects related to traditional care including conducting assessment, presenting feedback, selecting an appropriate intervention and delivering a course of therapy to the patient. This course of treatment could be delivered in ways consistent with current models of psychotherapy wherein a patient engages with a “chatbot” weekly for a prescribed amount of time, or in more flexible or alternative formats. LLMs used in this manner would ideally be trained using standardized assessment approaches and manualized therapy protocols that have large bodies of evidence.
Decision aid for existing evidence-based practices
Even without full automation, clinical LLMs could be used as a tool to guide a provider on the best course of treatment for a given patient by optimizing the delivery of existing EBPs and therapeutic techniques. In practice, this may look like a LLM that can analyze transcripts from therapy sessions and offer a provider guidance on therapeutic skills, approaches or language, either in real time, or at the end of the therapy session. Furthermore, the LLM could integrate current evidence on the tailoring of specific EBPs to the condition being treated, and to demographic or cultural factors and comorbid conditions. Developing tailored clinical LLM “advisors” based on EBPs could both enhance fidelity to treatment and maximize the possibility of patients achieving clinical improvement in light of updated clinical evidence.
Development of new therapeutic techniques and EBPs
To this point, we have discussed how LLMs could be applied to current approaches to psychotherapy using extant evidence. However, LLMs and other computational methods could greatly enhance the detection and development of new therapeutic skills and EBPs. Historically, EBPs have traditionally been developed using human-derived insights and then evaluated through years of clinical trial research. While EBPs are effective, effect sizes for psychotherapy are typically small 50 , 51 and significant proportions of patients do not respond 52 . There is a great need for more effective treatments, particularly for individuals with complex presentations or comorbid conditions. However, the traditional approach to developing and testing therapeutic interventions is slow, contributing to significant time lags in translational research 53 , and fails to deliver insights at the level of the individual.
Data-driven approaches hold the promise of revealing patterns that are not yet realized by clinicians, thus generating new approaches to psychotherapy; machine learning is already being used, for example, to predict behavioral health treatment outcomes 54 . With their ability to parse and summarize natural language, LLMs could add to existing data-driven approaches. For example, an LLM could be provided with a large historical dataset containing psychotherapy transcripts of different therapeutic orientations, outcome measures and sociodemographic information, and tasked with detecting therapeutic behaviors and techniques associated with objective outcomes (e.g., reduction in depressive symptoms). Using such a process might make it possible for an LLM to yield fine-grained insights about what makes existing therapeutic techniques work best (e.g., Which components of existing EBPs are the most potent? Are there therapist or patient characteristics that moderate the efficacy of intervention X? How does the ordering of interventions effect outcomes?) or even to isolate previously unidentified therapeutic techniques associated with improved clinical outcomes. By identifying what happens in therapy in such a fine-grained manner, LLMs could also play a role in revealing mechanisms of change, which is important for improving existing treatments and facilitating real-world implementation 55 .
However, to realize this possibility, and make sure that LLM-based advances can be integrated and vetted by the clinical community, it is necessary to steer away from the development of “black box,” LLM-identified interventions with low explainability (e.g., interpretability 56 ). To guard against interventions with low interpretability, work to finetune LLMs to improve patient outcomes could include inspectable representations of the techniques employed by the LLM. Clinicians could examine these representations and situate them in the broader psychotherapy literature, which would involve comparing them to existing psychotherapy techniques and theories. Such an approach could speed up the identification of novel mechanisms while guarding against the identification of “novel” interventions which overlap with existing techniques or constructs (thus avoiding the jangle fallacy, the erroneous assumption that two constructs with different names are necessarily distinct 57 ).
In the long run, by combining this information, it might even be possible for an LLM to “reverse-engineer” a new EBP, freed from the constraints of traditional therapeutic protocols and instead maximizing on the delivery of the constituent components shown to produce patient change (in a manner akin to modular approaches, wherein an individualized treatment plan is crafted for each patient by curating and sequencing treatment modules from an extensive menu of all available options based on the unique patient’s presentation 31 ). Eventually, a self-learning clinical LLM might deliver a broad range of psychotherapeutic interventions while measuring patient outcomes and adapting its approach on the fly in response to changes in the patient (or lack thereof).
Toward a precision medicine approach to psychotherapy
Current approaches to psychotherapy often are unable to provide guidance on the best approach to treatment when an individual has a complex presentation, which is often the rule rather than being the exception. For example, providers are likely to have greatly differing treatment plans for a patient with concurrent PTSD, substance use, chronic pain, and significant interpersonal difficulties. Models that use a data-driven approach (rather than a provider’s educated guess) to address an individual’s presenting concern alongside their comorbidities, sociodemographic factors, history, and responses to the current treatment, may ultimately offer the best chance at maximizing patient benefit. While there have been some advances in precision medicine approaches in behavioral healthcare 54 , 58 , these efforts are in their infancy and limited by sample sizes 59 .
The potential applications of clinical LLMs we have outlined above may come together to facilitate a personalized approach to behavioral healthcare, analogous to that of precision medicine. Through optimizing existing EBPs, identifying new therapeutic approaches, and better understanding mechanisms of change, LLMs (and their future descendants) may provide behavioral healthcare with an enhanced ability to identify what works best for whom and under what circumstances.
Recommendations for responsible development and evaluation of clinical LLMs
Focus first on evidence-based practices.
In the immediate future, clinical LLM applications will have the greatest chance of creating meaningful clinical impact if developed based on EBPs or a “common elements” approach (i.e., evidence-based procedures shared across treatments) 60 . Evidence-based treatments and techniques have been identified for specific psychopathologies (e.g., major depressive disorder, posttraumatic stress disorder), stressors (e.g., bereavement, job loss, divorce), and populations (e.g., LGBTQ individuals, older adults) 55 , 61 , 62 . Without an initial focus on EBPs, clinical LLM applications may fail to reflect current knowledge and may even produce harm 63 . Only once LLMs have been fully trained on EBPs can the field start to consider using LLMs in a data-driven manner, such as those outlined in the previous section on potential long-term applications.
Focus next on improvement (engagement is not enough)
Others have highlighted the importance of promoting engagement with digital mental health applications 15 , which is important for achieving an adequate “dose” of the therapeutic intervention. LLM applications hold the promise of improving engagement and retention through their ability to respond to free text, extract key concepts, and address patients’ unique context and concerns during interventions in a timely manner. However, engagement alone is not an appropriate outcome on which to train an LLM, because engagement is not expected to be sufficient for producing change. A focus on such metrics for clinical LLMs will risk losing sight of the primary goals, clinical improvement (e.g., reductions in symptoms or impairment, increases in well-being and functioning) and prevention of risks and adverse events. It will behoove the field to be wary of attempts to optimize clinical LLMs on outcomes that have an explicit relationship with a company’s profit (e.g., length of time using the application). An LLM that optimizes only for engagement (akin to YouTube recommendations) could have high rates of user retention without employing meaningful clinical interventions to reduce suffering and improve quality of life. Previous research has suggested that this may be happening with non-LLM digital mental health interventions. For instance, exposure is a technique with strong support for treating anxiety, yet it is rarely included in popular smartphone applications for anxiety 64 , perhaps because developers fear that the technique will not appeal to users, or have concerns about how exposures going poorly or increasing anxiety in the short term, which may prompt concerns about legal exposure.
Commit to rigorous yet commonsense evaluation
An evaluation approach for clinical LLMs that hierarchically prioritizes risk and safety, followed by feasibility, acceptability, and effectiveness, would be in line with existing recommendations for the evaluation of digital mental health smartphone apps 65 . The first level of evaluation could involve a demonstration that a clinical LLM produces no harm or very minimal harm that is outweighed by its benefits, similar to FDA phase I drug tests. Key risk and safety related constructs include measures of suicidality, non-suicidal self harm, and risk of harm to others.
Next, rigorous examinations of clinical LLM applications will be needed to provide empirical evidence of their utility, using head-to-head comparisons with standard treatments. Key constructs to be assessed in these empirical tests are feasibility and acceptability to the patient and the therapist as well as treatment outcomes (e.g., symptoms, impairment, clinical status, rates of relapse). Other relevant considerations include patients’ user experience with the application, measures of therapist efficiency and burnout, and cost.
Lastly, we note that given that possible benefits of clinical LLMs (including expanding access to care), it will be important for the field to adopt a commonsense approach to evaluation. While rigorous evaluation is important, the comparison conditions on which these evaluations are based should reflect real-world risk and efficacy rates, and perhaps employ a graded hierarchy with which to classify risk and error (i.e., missing a mention of suicidality is unacceptable, but getting a patient’s partner’s name wrong is nonideal but tolerable), rather than holding clinical LLM applications to a standard of perfection which humans do not achieve. Furthermore, developers will need to strike the appropriate balance of prioritizing constructs in a manner expected to be most clinically beneficial, for example, if exposure therapy is indicated for the patient, but the patient does not find this approach acceptable, the clinical LLM could recommend the intervention prioritizing effectiveness before offering second-line interventions which may be more acceptable.
Involve interdisciplinary collaboration
Interdisciplinary collaboration between clinical scientists, engineers, and technologists will be crucial in the development of clinical LLMs. While it is plausible that engineers and technologists could use available therapeutic manuals to develop clinical LLMs without the expertise of a behavioral health expert, this is ill-advised. Manuals are only a first step towards learning a specific intervention, as they do not provide guidance on how the intervention can be applied to specific individuals or presentations, or how to handle specific issues or concerns that may arise through the course of treatment.
Clinicians and clinician-scientists have expertise that bears on these issues, as well as many other aspects of the clinical LLM development process. Their involvement could include a) testing new applications to identify limitations and risks and optimize their integration into clinical practice, b) improving the ability of applications to adequately address the complexity of psychological phenomena, c) ensuring that applications are developed and implemented in an ethical manner, and d) testing and ensuring that applications don’t have iatrogenic effects, such as reinforcing behaviors that perpetuate psychopathology or distress.
Behavioral health experts could also provide guidance on how best to finetune or tailor models, including addressing the question of whether and how real patient data should be used for these purposes. For example, most proximately, behavioral health experts might assist in prompt engineering , or the designing and testing of a series of prompts which provide the LLM framing and context for delivering a specific type of treatment or clinical skill (e.g., “Use cognitive restructuring to help the patient evaluate and reappraise negative thoughts in depression”), or a desired clinical task, such as evaluating therapy sessions for fidelity (e.g., “Analyze this psychotherapy transcript and select sections in which the therapist demonstrated the particularly skillful use of CBT skills, and sections in which the therapist’s delivery of CBT skills could be improved”). Similarly, in few-shot learning , behavioral health experts could be involved in crafting example exchanges which are added to prompts. For example, treatment modality experts might generate examples of clinical skills (e.g., high-quality examples of using cognitive restructuring to address depression) or of a clinical task (e.g., examples of both high- and low-quality delivery of CBT skills). For fine-tuning , in which a large, labeled dataset is used to train the LLM, and reinforcement learning from human feedback (RLHF), in which a human-labeled dataset is used to train a smaller model which is then used for LLM “self-training,” behavioral health experts could build and curate (and ensure informed patient consent for use of) appropriate datasets (e.g., a dataset containing psychotherapy transcripts rated for fidelity to an evidence-based psychotherapy). The expertise that behavioral health experts could draw on to generate instructive examples and curate high-quality datasets holds particular value in light of recent evidence that quality of data trumps quantity of data for training well-performing models 66 .
In the service of facilitating interdisciplinary collaboration, it would benefit clinical scientists to seek out a working knowledge about LLMs, while it would benefit technologists to develop a working knowledge of therapy in general and EBPs in particular. Dedicated venues that bring together behavioral health experts and clinical psychologists for interdisciplinary collaboration and communication will aid in these efforts. Historically, venues of this type have included psychology-focused workshops at NLP conferences (e.g., the Workshop on Computational Linguistics and Clinical Psychology [CLPsych], held at the Annual Conference of the North American Chapter of the Association for Computational Linguistics [NAACL]) and technology-focused conferences or workgroups hosted by psychological organizations (e.g., APA’s Technology, Mind & Society conference; Association for Behavioral and Cognitive Therapies’ [ABCT] Technology and Behavior Change special interest group). This work has also been done at nonprofits centered on technological tools for mental health (e.g., the Society for Digital Mental Health). Beyond these venues, it may be fruitful to develop a gathering that brings together technologists, clinical scientists, and industry partners with a dedicated focus on AI/LLMs, which could routinely publish on its efforts, akin to the efforts of the World Health Organization’s Infodemic Management Conference, which has employed this approach to address misinformation 67 . Finally, given the numerous applications of AI to behavioral health, it is conceivable that a new “computational behavioral health” subfield could emerge, offering specialized training that would bridge the gap between these two domains.
Focus on trust and usability for clinicians and patients
It is important to engage therapists, policymakers, end-users, and experts in human-computer interactions to understand and improve levels of trust that will be necessary for successful and effective implementation. With respect to applications of AI to augment supervision and support for psychotherapy, therapists have expressed concern about privacy, the ability to detect subtle non-verbal cues and cultural responsiveness, and the impact on therapist confidence, but they also see benefits for training and professional growth 68 . Other research suggests that while therapists believe AI can increase access to care, allow individuals to disclose embarrassing information more comfortably, continuously refine therapeutic techniques 69 , they have concerns about privacy and the formation of a strong therapeutic bond with machine-based therapeutic interventions 70 . Involvement of individuals who will be referring their patients and using LLMs in their own practice will be essential to developing solutions they can trust and implement, and to make sure these solutions have the features that support trust and usability (simple interfaces, accurate summaries of AI-patient interactions, etc.).
Regarding how much patients will trust the AI systems, following the stages we outlined in Fig. 3 , initial AI-patient interactions will continue to be supervised by clinicians, and the therapeutic bond between the clinician and the patient will continue to be the primary relationship. During this stage, it is important that clinicians talk to the patients about their experience with the LLMs, and that the field as a whole begins to accumulate an understanding and data on how acceptable interfacing with LLMs is for what kind of patient for what kind of clinical use case, in how clinicians can scaffold the patient-LLM relationship. This data will be critical for developing collaborative LLM applications that have more autonomy, and for ensuring that the transition from assistive to collaborative stage applications is not associated with large unforeseen risk. For example, in the case of CBT for insomnia, once an assistive AI system has been iterated on to reliably collect information about patients’ sleep patterns, it is more conceivable that it could be evolved into a collaborative AI system that does a comprehensive insomnia assessment (i.e., it also collects and interprets data on patients’ clinically significant distress, impairment of functioning, and ruling out of sleep-wake disorders, like narcolepsy) 71 .
Design criteria for effective clinical LLMs
Below, we propose an initial set of desirable design qualities for clinical LLMs.
Detect risk of harm
Accurate risk detection and mandated reporting are crucial aspects that clinical LLMs must prioritize, particularly in the identification of suicidal/homicidal ideation, child/elder abuse, and intimate partner violence. Algorithms for detecting risks are under development 4 . One threat to risk detection is that current LLMs have limited context windows, meaning they only “remember” a limited amount of user input. Functionally, this means a clinical LLM application could “forget” crucial details about a patient, which could impact safety (e.g., an application “forgetting” that the patient owns firearms would threaten its ability to properly assess and intervene around suicide risk). However, context windows have been rapidly expanding with each subsequent model release, so this issue may not be a problem for long. In addition, it is already possible to augment the memory of LLMs with “vector databases,” which would have the added benefit of retaining inspectable learnings and summaries across clinical encounters 72 .
In the future, and especially given much larger context windows, clinical LLMs could prompt clinicians with ethical guidelines, legal requirements (e.g., the Tarasoff rule, which requires clinicians to warn intended victims when a patient presents a serious threat of violence), or evidence-based methods for decreasing risk (e.g., safety planning 73 ), or even provide interventions targeting risk directly to patients. This type of risk monitoring and intervention could be particularly useful in supplementing existing healthcare systems during gaps in clinician coverage like nights and weekends 4 .
b) Be “Healthy.” There is growing concern that AI chat systems can demonstrate undesirable behaviors, including expressions akin to depression or narcissism 35 , 74 . Such poorly understood, undesirable behaviors risk harming already vulnerable patients or interfering with their ability to benefit from treatment. Clinical LLM applications will need training, monitoring, auditing, and guardrails to prevent the expression of undesirable behaviors and maintain healthy interactions with users. These efforts will need to be continually evaluated and updated to prevent or address the emergence of new undesirable or clinically contraindicated behavior.
Aid in psychodiagnostic assessment
Clinical LLMs ought to integrate psychodiagnostic assessment and diagnosis, facilitating intervention selection and outcome monitoring 75 . Recent developments show promise for LLMs in the assessment realm 76 . Down the line, LLMs could be used for diagnostic interviewing (e.g., Structured Clinical Interview for the DSM-5 77 ) using chatbots or voice interfaces. Prioritizing assessment enhances diagnostic accuracy and ensures appropriate intervention, reducing the risk of harmful interventions 63 .
Be responsive and flexible
Given the frequency with which ambivalence and poor patient engagement arise in clinical encounters, clinical LLMs which use evidence-based and patient-centered methods for handling these issues (e.g., motivational enhancement techniques, shared decision making), and have options for second-line interventions for patients not interested in gold-standard treatments, will have the best chance of success.
Stop when not helping or confident
Psychologists are ethically obligated to cease treatment and offer appropriate referrals to the patient if the current course of treatment has not helped or likely will not help. Clinical LLMs can abide by this ethical standard by drawing on integrated assessment (discussed above) to assess the appropriateness of the given intervention and detect cases that need more specialized or intensive intervention.
Be fair, inclusive, and free from bias
As has been written about extensively, LLMs may perpetuate bias, including racism, sexism, and homophobia, given that they are trained on existing text 36 . These biases can contribute to both error disparities – where models are less accurate for particular groups – or outcome disparities – where models tend to over-capture demographic information 78 – which would in turn contribute to the disparities in mental health status and care already experienced by minoritized groups 79 . The integration of bias countermeasures into clinical LLM applications could serve to prevent this 78 , 80 .
Be empathetic–to an extent
Clinical LLMs will likely need to demonstrate empathy and build the therapeutic alliance in order to engage patients. Other skills used by therapists include humor, irreverence, and gentle methods of challenging the patient. Incorporating these into clinical LLMs might be beneficial, as appropriate human likeness may facilitate engagement and interaction with AI 81 . However, this needs to be balanced against associated risks, mentioned above, of incorporating human likeness in systems 36 . Whether and how much human likeness is necessary for a psychological intervention remains a question for future empirical work.
Be transparent about being AIs
Mental illness and mental health care is already stigmatized, and the application of LLMs without transparent consent can erode patient/consumer trust, which reduces trust in the behavioral health profession more generally. Some mental health startups have already faced criticism for employing generative AI in applications without disclosing this information to the end user 2 . As laid out in the White House Blueprint for an AI Bill of Rights, AI applications should be explicitly (and perhaps repeatedly/consistently) labeled as such to allow patients and consumers to “know that an automated system is being used and understand how and why it contributes to outcomes that impact them” 82 .
Unintended consequences may change the clinical profession
The development of clinical LLM applications could lead to unintended consequences, such as changes to the structure of and compensation for mental health services. AI may permit increased staffing by non-professionals or paraprofessionals, causing professional clinicians to supervise large numbers of non-professionals or even semi-autonomous LLM systems. This could reduce clinicians’ direct patient contact and perhaps increase their exposure to challenging or complicated cases not suitable for the LLM, which may lead to burnout and make clinical jobs less attractive. To address this, research could determine the appropriate number of cases for a clinician to oversee safely and guidelines could be published to disseminate these findings. The 24-hour availability of LLM-based intervention may also change consumer expectations of psychotherapy in a way that is at odds with many of the norms of psychotherapy practice (e.g., waiting for a session to discuss stressors, limited or emergency-only contact between sessions).
LLMs could pave the way for a next generation of clinical science
Beyond the imminent applications described in this paper, it is worth considering how the long-term applications of clinical LLMs might also facilitate significant advances in clinical care and clinical science.
Clinical practice
In terms of their effects on therapeutic interventions themselves, clinical LLMs might promote advances in the field by allowing for the pooling of data on what works with the most difficult cases, perhaps through the use of practice research networks 83 . At the level of health systems, they could expedite the implementation and translation of research findings into clinical practice by suggesting therapeutic strategies to psychotherapists, for instance, promoting strategies that enhance inhibitory learning during exposure therapy 84 . Lastly, clinical LLMs could increase access to care if LLM-based psychotherapy chatbots are offered as low intensity, low-cost options in stepped-care models, similar to the existing provision of computerized CBT and guided self-help 85 .
As the utilization of clinical LLMs expands, there may be a shift towards psychologists and other behavioral health experts operating at the top of their degree. Presently, a significant amount of clinician time is consumed by administrative tasks, chart review, and documentation. The shifting of responsibilities afforded by the automation of certain aspects of psychotherapy by clinical LLMs could allow clinicians to pursue leadership roles, contribute to the development, evaluation, and implementation of LLM-based care, or lead policy efforts, or simply to devote more time to direct patient care.
Clinical science
By facilitating supervision, consultation, and fidelity measurement, LLMs could expedite psychotherapist training and increase the capacity of study supervisors, thus making psychotherapy research less expensive and more efficient.
In a world in which fully autonomous LLM applications screen and assess patients, deliver high-fidelity, protocolized psychotherapy, and collect outcome measurements, psychotherapy clinical trials would be limited largely by the number of willing participants eligible for the study, rather than by the resources required to screen, assess, treat, and follow these participants. This could open the door to unprecedentedly large-N clinical trials. This would allow for well-powered, sophisticated dismantling studies to support the search for mechanisms of change in psychotherapy, which are currently only possible using individual participant level meta-analysis (for example, see ref. 86 ). Ultimately, such insights into causal mechanisms of change in psychotherapy could help to refine these treatments and potentially improve their efficacy.
Finally, the emergence of LLM treatment modalities will challenge (or confirm) fundamental assumptions about psychotherapy. Does therapeutic (human) alliance account for a majority of the variance in patient change? To what extent can an alliance be formed with a technological agent? Is lasting and meaningful therapeutic change only possible through working with a human therapist? LLMs hold the promise of empirical answers to these questions.
In summary, large language models hold promise for supporting, augmenting, or even in some cases replacing human-led psychotherapy, which may improve the quality, accessibility, consistency, and scalability of therapeutic interventions and clinical science research. However, LLMs are advancing quickly and will soon be deployed in the clinical domain, with little oversight or understanding of harms that they may produce. While cautious optimism about clinical LLM applications is warranted, it is also crucial for psychologists to approach the integration of LLMs into psychotherapy with caution and to educate the public about the potential risks and limitations of using these technologies for therapeutic purposes. Furthermore, clinical psychologists ought to actively engage with the technologists building these solutions. As the field of AI continues to evolve, it is essential that researchers and clinicians closely monitor the use of LLMs in psychotherapy and advocate for responsible and ethical use to protect the wellbeing of patients.
Data availability
Data sharing not applicable to this article as no datasets were generated or analyzed during the current study.
Bubeck, S. et al. Sparks of artificial general intelligence: Early experiments with GPT-4. Preprint at http://arxiv.org/abs/2303.12712 (2023).
Broderick, R. People are using AI for therapy, whether the tech is ready for it or not. Fast Company (2023).
Weizenbaum, J. ELIZA—a computer program for the study of natural language communication between man and machine. Commun. ACM 9 , 36–45 (1966).
Article Google Scholar
Bantilan, N., Malgaroli, M., Ray, B. & Hull, T. D. Just in time crisis response: Suicide alert system for telemedicine psychotherapy settings. Psychother. Res. 31 , 289–299 (2021).
Peretz, G., Taylor, C. B., Ruzek, J. I., Jefroykin, S. & Sadeh-Sharvit, S. Machine learning model to predict assignment of therapy homework in behavioral treatments: Algorithm development and validation. JMIR Form. Res. 7 , e45156 (2023).
Article PubMed PubMed Central Google Scholar
Tanana, M. J. et al. How do you feel? Using natural language processing to automatically rate emotion in psychotherapy. Behav. Res. Methods 53 , 2069–2082 (2021).
Sharma, A., Lin, I. W., Miner, A. S., Atkins, D. C. & Althoff, T. Human–AI collaboration enables more empathic conversations in text-based peer-to-peer mental health support. Nat. Mach. Intell . 5 , 46–57 (2023).
Chen, Z., Flemotomos, N., Imel, Z. E., Atkins, D. C. & Narayanan, S. Leveraging open data and task augmentation to automated behavioral coding of psychotherapy conversations in low-resource scenarios. Preprint at https://doi.org/10.48550/arXiv.2210.14254 (2022).
Shah, R. S. et al. Modeling motivational interviewing strategies on an online peer-to-peer counseling platform. Proc. ACM Hum.-Comput. Interact 6 , 1–24 (2022).
Chan, W. W. et al. The challenges in designing a prevention chatbot for eating disorders: Observational study. JMIR Form. Res. 6 , e28003 (2022).
Darcy, A. Why generative AI Is not yet ready for mental healthcare. Woebot Health https://woebothealth.com/why-generative-ai-is-not-yet-ready-for-mental-healthcare/ (2023).
Abd-Alrazaq, A. A. et al. An overview of the features of chatbots in mental health: A scoping review. Int. J. Med. Inf. 132 , 103978 (2019).
Lim, S. M., Shiau, C. W. C., Cheng, L. J. & Lau, Y. Chatbot-delivered psychotherapy for adults with depressive and anxiety symptoms: A systematic review and meta-regression. Behav. Ther. 53 , 334–347 (2022).
Article PubMed Google Scholar
Baumel, A., Muench, F., Edan, S. & Kane, J. M. Objective user engagement with mental health apps: Systematic search and panel-based usage analysis. J. Med. Internet Res. 21 , e14567 (2019).
Torous, J., Nicholas, J., Larsen, M. E., Firth, J. & Christensen, H. Clinical review of user engagement with mental health smartphone apps: Evidence, theory and improvements. Evid. Based Ment. Health 21 , 116–119 (2018b).
Das, A. et al. Conversational bots for psychotherapy: A study of generative transformer models using domain-specific dialogues. in Proceedings of the 21st Workshop on Biomedical Language Processing 285–297 (Association for Computational Linguistics, 2022). https://doi.org/10.18653/v1/2022.bionlp-1.27 .
Liu, H. Towards automated psychotherapy via language modeling. Preprint at http://arxiv.org/abs/2104.10661 (2021).
Hamilton, J. Why generative AI (LLM) is ready for mental healthcare. LinkedIn https://www.linkedin.com/pulse/why-generative-ai-chatgpt-ready-mental-healthcare-jose-hamilton-md/ (2023).
Shariff, A., Bonnefon, J.-F. & Rahwan, I. Psychological roadblocks to the adoption of self-driving vehicles. Nat. Hum. Behav. 1 , 694–696 (2017).
Markov, A. A. Essai d’une recherche statistique sur le texte du roman “Eugene Onegin” illustrant la liaison des epreuve en chain (‘Example of a statistical investigation of the text of “Eugene Onegin” illustrating the dependence between samples in chain’). Izvistia Imperatorskoi Akad. Nauk Bull. L’Academie Imp. Sci. St-Petersbourg 7 , 153–162 (1913).
Google Scholar
Shannon, C. E. A mathematical theory of communication. Bell Syst. Tech. J. 27 , 379–423 (1948).
Article MathSciNet Google Scholar
Baker, J. K. Stochastic modeling for automatic speech understanding. in Speech recognition: invited papers presented at the 1974 IEEE symposium (ed. Reddy, D. R.) (Academic Press, 1975).
Jelinek, F. Continuous speech recognition by statistical methods. Proc. IEEE 64 , 532–556 (1976).
Jurafsky, D. & Martin, J. H. N-gram language models. in Speech and language processing: An introduction to natural language processing, computational linguistics, and speech recognition (Pearson Prentice Hall, 2009).
Vaswani, A. et al. Attention is all you need. 31st Conf. Neural Inf. Process. Syst . (2017).
Bommasani, R. et al. On the opportunities and risks of foundation models. Preprint at http://arxiv.org/abs/2108.07258 (2022).
Gao, L. et al. The Pile: An 800GB dataset of diverse text for language modeling. Preprint at http://arxiv.org/abs/2101.00027 (2020).
Devlin, J., Chang, M.-W., Lee, K. & Toutanova, K. BERT: Pre-training of deep bidirectional transformers for language understanding. Preprint at http://arxiv.org/abs/1810.04805 (2019).
Kojima, T., Gu, S. S., Reid, M., Matsuo, Y. & Iwasawa, Y. Large language models are zero-shot reasoners. Preprint at http://arxiv.org/abs/2205.11916 (2023).
Fairburn, C. G. & Patel, V. The impact of digital technology on psychological treatments and their dissemination. Behav. Res. Ther. 88 , 19–25 (2017).
Fisher, A. J. et al. Open trial of a personalized modular treatment for mood and anxiety. Behav. Res. Ther. 116 , 69–79 (2019).
Fan, X. et al. Utilization of self-diagnosis health chatbots in real-world settings: Case study. J. Med. Internet Res. 23 , e19928 (2021).
Coghlan, S. et al. To chat or bot to chat: Ethical issues with using chatbots in mental health. Digit. Health 9 , 1–11 (2023).
Beatty, C., Malik, T., Meheli, S. & Sinha, C. Evaluating the therapeutic alliance with a free-text CBT conversational agent (Wysa): A mixed-methods study. Front. Digit. Health 4 , 847991 (2022).
Lin, B., Bouneffouf, D., Cecchi, G. & Varshney, K. R. Towards healthy AI: Large language models need therapists too. Preprint at http://arxiv.org/abs/2304.00416 (2023).
Weidinger, L. et al. Ethical and social risks of harm from language models. Preprint at http://arxiv.org/abs/2112.04359 (2021).
Bender, E. M., Gebru, T., McMillan-Major, A. & Shmitchell, S. On the dangers of stochastic parrots: Can language models be too big? In Proceedings of the 2021 ACM Conference on Fairness, Accountability, and Transparency 610–623 (ACM, 2021). https://doi.org/10.1145/3442188.3445922 .
Chamberlain, J. The risk-based approach of the European Union’s proposed artificial intelligence regulation: Some comments from a tort law perspective. Eur. J. Risk Regul. 14 , 1–13 (2023).
Norden, J. G. & Shah, N. R. What AI in health care can learn from the long road to autonomous vehicles. NEJM Catal. Innov. Care Deliv . https://doi.org/10.1056/CAT.21.0458 (2022).
Sedlakova, J. & Trachsel, M. Conversational artificial intelligence in psychotherapy: A new therapeutic tool or agent? Am. J. Bioeth. 23 , 4–13 (2023).
Gearing, R. E. et al. Major ingredients of fidelity: A review and scientific guide to improving quality of intervention research implementation. Clin. Psychol. Rev. 31 , 79–88 (2011).
Wiltsey Stirman, S. Implementing evidence-based mental-health treatments: Attending to training, fidelity, adaptation, and context. Curr. Dir. Psychol. Sci. 31 , 436–442 (2022).
Waller, G. Evidence-based treatment and therapist drift. Behav. Res. Ther. 47 , 119–127 (2009).
Flemotomos, N. et al. “Am I a good therapist?” Automated evaluation of psychotherapy skills using speech and language technologies. CoRR, Abs, 2102 (10.3758) (2021).
Zhang, X. et al. You never know what you are going to get: Large-scale assessment of therapists’ supportive counseling skill use. Psychotherapy https://doi.org/10.1037/pst0000460 (2022).
Goldberg, S. B. et al. Machine learning and natural language processing in psychotherapy research: Alliance as example use case. J. Couns. Psychol. 67 , 438–448 (2020).
Wiltsey Stirman, S. et al. A novel approach to the assessment of fidelity to a cognitive behavioral therapy for PTSD using clinical worksheets: A proof of concept with cognitive processing therapy. Behav. Ther. 52 , 656–672 (2021).
Raviola, G., Naslund, J. A., Smith, S. L. & Patel, V. Innovative models in mental health delivery systems: Task sharing care with non-specialist providers to close the mental health treatment gap. Curr. Psychiatry Rep. 21 , 44 (2019).
American Psychological Association. Guidelines for clinical supervision in health service psychology. Am. Psychol. 70 , 33–46 (2015).
Cook, S. C., Schwartz, A. C. & Kaslow, N. J. Evidence-based psychotherapy: Advantages and challenges. Neurotherapeutics 14 , 537–545 (2017).
Leichsenring, F., Steinert, C., Rabung, S. & Ioannidis, J. P. A. The efficacy of psychotherapies and pharmacotherapies for mental disorders in adults: An umbrella review and meta‐analytic evaluation of recent meta‐analyses. World Psych. 21 , 133–145 (2022).
Cuijpers, P., van Straten, A., Andersson, G. & van Oppen, P. Psychotherapy for depression in adults: A meta-analysis of comparative outcome studies. J. Consult. Clin. Psychol. 76 , 909–922 (2008).
Morris, Z. S., Wooding, S. & Grant, J. The answer is 17 years, what is the question: Understanding time lags in translational research. J. R. Soc. Med. 104 , 510–520 (2011).
Chekroud, A. M. et al. The promise of machine learning in predicting treatment outcomes in psychiatry. World Psych. 20 , 154–170 (2021).
Kazdin, A. E. Mediators and mechanisms of change in psychotherapy research. Annu. Rev. Clin. Psychol. 3 , 1–27 (2007).
Angelov, P. P., Soares, E. A., Jiang, R., Arnold, N. I. & Atkinson, P. M. Explainable artificial intelligence: An analytical review. WIREs Data Min. Knowl. Discov . 11 , (2021).
Kelley, T. L. Interpretation of Educational Measurements . (World Book, 1927).
van Bronswijk, S. C. et al. Precision medicine for long-term depression outcomes using the Personalized Advantage Index approach: Cognitive therapy or interpersonal psychotherapy? Psychol. Med. 51 , 279–289 (2021).
Scala, J. J., Ganz, A. B. & Snyder, M. P. Precision medicine approaches to mental health care. Physiology 38 , 82–98 (2023).
Article CAS Google Scholar
Chorpita, B. F., Daleiden, E. L. & Weisz, J. R. Identifying and selecting the common elements of evidence based interventions: A distillation and matching model. Ment. Health Serv. Res. 7 , 5–20 (2005).
Chambless, D. L. & Hollon, S. D. Defining empirically supported therapies. J. Consult. Clin. Psychol. 66 , 7–18 (1998).
Article CAS PubMed Google Scholar
Tolin, D. F., McKay, D., Forman, E. M., Klonsky, E. D. & Thombs, B. D. Empirically supported treatment: Recommendations for a new model. Clin. Psychol. Sci. Pract. 22 , 317–338 (2015).
Lilienfeld, S. O. Psychological treatments that cause harm. Perspect. Psychol. Sci. 2 , 53–70 (2007).
Wasil, A. R., Venturo-Conerly, K. E., Shingleton, R. M. & Weisz, J. R. A review of popular smartphone apps for depression and anxiety: Assessing the inclusion of evidence-based content. Behav. Res. Ther. 123 , 103498 (2019).
Torous, J. B. et al. A hierarchical framework for evaluation and informed decision making regarding smartphone apps for clinical care. Psychiatr. Serv. 69 , 498–500 (2018).
Gunasekar, S. et al. Textbooks are all you need. Preprint at http://arxiv.org/abs/2306.11644 (2023).
Wilhelm, E. et al. Measuring the burden of infodemics: Summary of the methods and results of the Fifth WHO Infodemic Management Conference. JMIR Infodemiology 3 , e44207 (2023).
Creed, T. A. et al. Knowledge and attitudes toward an artificial intelligence-based fidelity measurement in community cognitive behavioral therapy supervision. Adm. Policy Ment. Health Ment. Health Serv. Res. 49 , 343–356 (2022).
Aktan, M. E., Turhan, Z. & Dolu, İ. Attitudes and perspectives towards the preferences for artificial intelligence in psychotherapy. Comput. Hum. Behav. 133 , 107273 (2022).
Prescott, J. & Hanley, T. Therapists’ attitudes towards the use of AI in therapeutic practice: considering the therapeutic alliance. Ment. Health Soc. Incl. 27 , 177–185 (2023).
American Psychiatric Association. Diagnostic and Statistical Manual of Mental Disorders . (2013).
Yogatama, D., De Masson d’Autume, C. & Kong, L. Adaptive semiparametric language models. Trans. Assoc. Comput. Linguist 9 , 362–373 (2021).
Stanley, B. & Brown, G. K. Safety planning intervention: A brief intervention to mitigate suicide risk. Cogn. Behav. Pract. 19 , 256–264 (2012).
Behzadan, V., Munir, A. & Yampolskiy, R. V. A psychopathological approach to safety engineering in AI and AGI. Preprint at http://arxiv.org/abs/1805.08915 (2018).
Lambert, M. J. & Harmon, K. L. The merits of implementing routine outcome monitoring in clinical practice. Clin. Psychol. Sci. Pract . 25 , (2018).
Kjell, O. N. E., Kjell, K. & Schwartz, H. A. AI-based large language models are ready to transform psychological health assessment. Preprint at https://doi.org/10.31234/osf.io/yfd8g (2023).
First, M. B., Williams, J. B. W., Karg, R. S. & Spitzer, R. L. SCID-5-CV: Structured Clinical Interview for DSM-5 Disorders: Clinician Version . (American Psychiatric Association Publishing, 2016).
Shah, D. S., Schwartz, H. A. & Hovy, D. Predictive biases in natural language processing models: A conceptual framework and overview. in Proceedings of the 58th Annual Meeting of the Association for Computational Linguistics 5248–5264 (Association for Computational Linguistics, 2020). https://doi.org/10.18653/v1/2020.acl-main.468 .
Adams, L. M. & Miller, A. B. Mechanisms of mental-health disparities among minoritized groups: How well are the top journals in clinical psychology representing this work? Clin . Psychol. Sci. 10 , 387–416 (2022).
Viswanath, H. & Zhang, T. FairPy: A toolkit for evaluation of social biases and their mitigation in large language models. Preprint at http://arxiv.org/abs/2302.05508 (2023).
von Zitzewitz, J., Boesch, P. M., Wolf, P. & Riener, R. Quantifying the human likeness of a humanoid robot. Int. J. Soc. Robot. 5 , 263–276 (2013).
White House Office of Science and Technology Policy. Blueprint for an AI bill of rights. (2022).
Parry, G., Castonguay, L. G., Borkovec, T. D. & Wolf, A. W. Practice research networks and psychological services research in the UK and USA. in Developing and Delivering Practice-Based Evidence (eds. Barkham, M., Hardy, G. E. & Mellor-Clark, J.) 311–325 (Wiley-Blackwell, 2010). https://doi.org/10.1002/9780470687994.ch12 .
Craske, M. G., Treanor, M., Conway, C. C., Zbozinek, T. & Vervliet, B. Maximizing exposure therapy: An inhibitory learning approach. Behav. Res. Ther. 58 , 10–23 (2014).
Delgadillo, J. et al. Stratified care vs stepped care for depression: A cluster randomized clinical trial. JAMA Psychiatry 79 , 101 (2022).
Furukawa, T. A. et al. Dismantling, optimising, and personalising internet cognitive behavioural therapy for depression: A systematic review and component network meta-analysis using individual participant data. Lancet Psychiatry 8 , 500–511 (2021).
Download references
Acknowledgements
This work was supported by the National Institute of Mental Health under award numbers R01-MH125702 (PI: H.A.S) and RF1-MH128785 (PI: S.W.S.), and by the Institute for Human-Centered A.I. at Stanford University to J.C.E. The authors are grateful to Adam S. Miner and Victor Gomes who provided critical feedback on an earlier version of this manuscript.
Author information
Authors and affiliations.
Dissemination and Training Division, National Center for PTSD, VA Palo Alto Health Care System, Palo Alto, CA, USA
Elizabeth C. Stade, Shannon Wiltsey Stirman & Cody L. Boland
Department of Psychiatry and Behavioral Sciences, Stanford University, Stanford, CA, USA
Elizabeth C. Stade & Shannon Wiltsey Stirman
Institute for Human-Centered Artificial Intelligence & Department of Psychology, Stanford University, Stanford, CA, USA
Elizabeth C. Stade & Johannes C. Eichstaedt
Department of Computer and Information Science, University of Pennsylvania, Philadelphia, PA, USA
Lyle H. Ungar
Department of Computer Science, Stony Brook University, Stony Brook, NY, USA
H. Andrew Schwartz
Department of Psychiatry and Behavioral Sciences, Johns Hopkins University School of Medicine, Baltimore, MD, USA
David B. Yaden
Department of Technology, Operations, and Statistics, New York University, New York, NY, USA
Department of Psychology, University of Pennsylvania, Philadelphia, PA, USA
Robert J. DeRubeis
Department of Sociology, Stanford University, Stanford, CA, USA
Robb Willer
You can also search for this author in PubMed Google Scholar
Contributions
E.C.S., S.W.S., C.L.B., and J.C.E. wrote the main manuscript text. E.C.S., L.H.U., and J.C.E. prepared the figures. All authors reviewed the manuscript.
Corresponding authors
Correspondence to Elizabeth C. Stade or Johannes C. Eichstaedt .
Ethics declarations
Competing interests.
The authors declare the following competing interests: receiving consultation fees from Jimini Health (E.C.S., L.H.U., H.A.S., and J.C.E.).
Additional information
Publisher’s note Springer Nature remains neutral with regard to jurisdictional claims in published maps and institutional affiliations.
Rights and permissions
Open Access This article is licensed under a Creative Commons Attribution 4.0 International License, which permits use, sharing, adaptation, distribution and reproduction in any medium or format, as long as you give appropriate credit to the original author(s) and the source, provide a link to the Creative Commons licence, and indicate if changes were made. The images or other third party material in this article are included in the article’s Creative Commons licence, unless indicated otherwise in a credit line to the material. If material is not included in the article’s Creative Commons licence and your intended use is not permitted by statutory regulation or exceeds the permitted use, you will need to obtain permission directly from the copyright holder. To view a copy of this licence, visit http://creativecommons.org/licenses/by/4.0/ .
Reprints and permissions
About this article
Cite this article.
Stade, E.C., Stirman, S.W., Ungar, L.H. et al. Large language models could change the future of behavioral healthcare: a proposal for responsible development and evaluation. npj Mental Health Res 3 , 12 (2024). https://doi.org/10.1038/s44184-024-00056-z
Download citation
Received : 24 July 2023
Accepted : 30 January 2024
Published : 02 April 2024
DOI : https://doi.org/10.1038/s44184-024-00056-z
Share this article
Anyone you share the following link with will be able to read this content:
Sorry, a shareable link is not currently available for this article.
Provided by the Springer Nature SharedIt content-sharing initiative
Quick links
- Explore articles by subject
- Guide to authors
- Editorial policies
Sign up for the Nature Briefing newsletter — what matters in science, free to your inbox daily.

You are using an outdated browser. Please upgrade your browser to improve your experience.
Apple AI research: ReALM is smaller, faster than GPT-4 when parsing contextual data
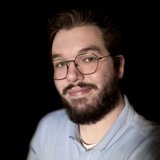
Apple is working to bring AI to Siri
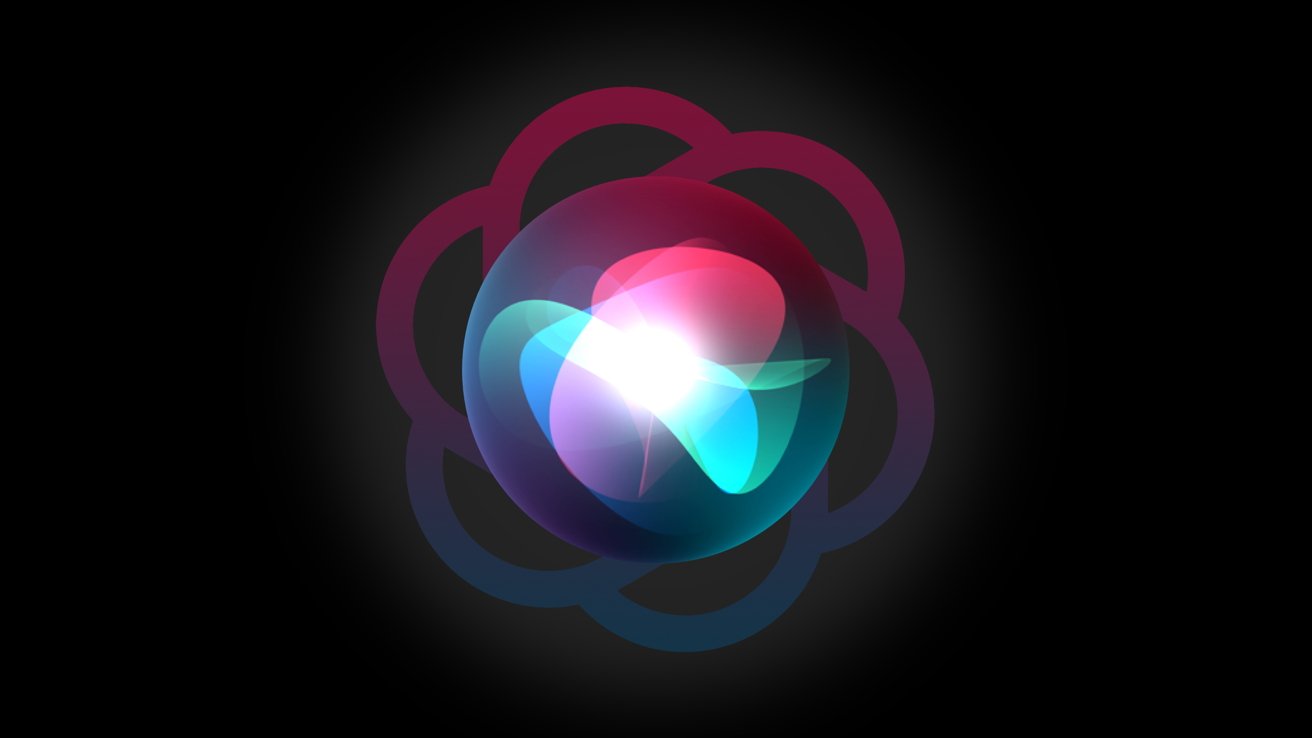
Artificial Intelligence research at Apple keeps being published as the company approaches a public launch of its AI initiatives in June during WWDC . There has been a variety of research published so far, including an image animation tool .
The latest paper was first shared by VentureBeat . The paper details something called ReALM — Reference Resolution As Language Modeling.
Having a computer program perform a task based on vague language inputs, like how a user might say "this" or "that," is called reference resolution. It's a complex issue to solve since computers can't interpret images the way humans can, but Apple may have found a streamlined resolution using LLMs.
When speaking to smart assistants like Siri , users might reference any number of contextual information to interact with, such as background tasks, on-display data, and other non-conversational entities. Traditional parsing methods rely on incredibly large models and reference materials like images, but Apple has streamlined the approach by converting everything to text.
Apple found that its smallest ReALM models performed similarly to GPT-4 with much fewer parameters, thus better suited for on-device use. Increasing the parameters used in ReALM made it substantially outperform GPT-4.
One reason for this performance boost is GPT-4's reliance on image parsing to understand on-screen information. Much of the image training data is built on natural imagery, not artificial code-based web pages filled with text, so direct OCR is less efficient.
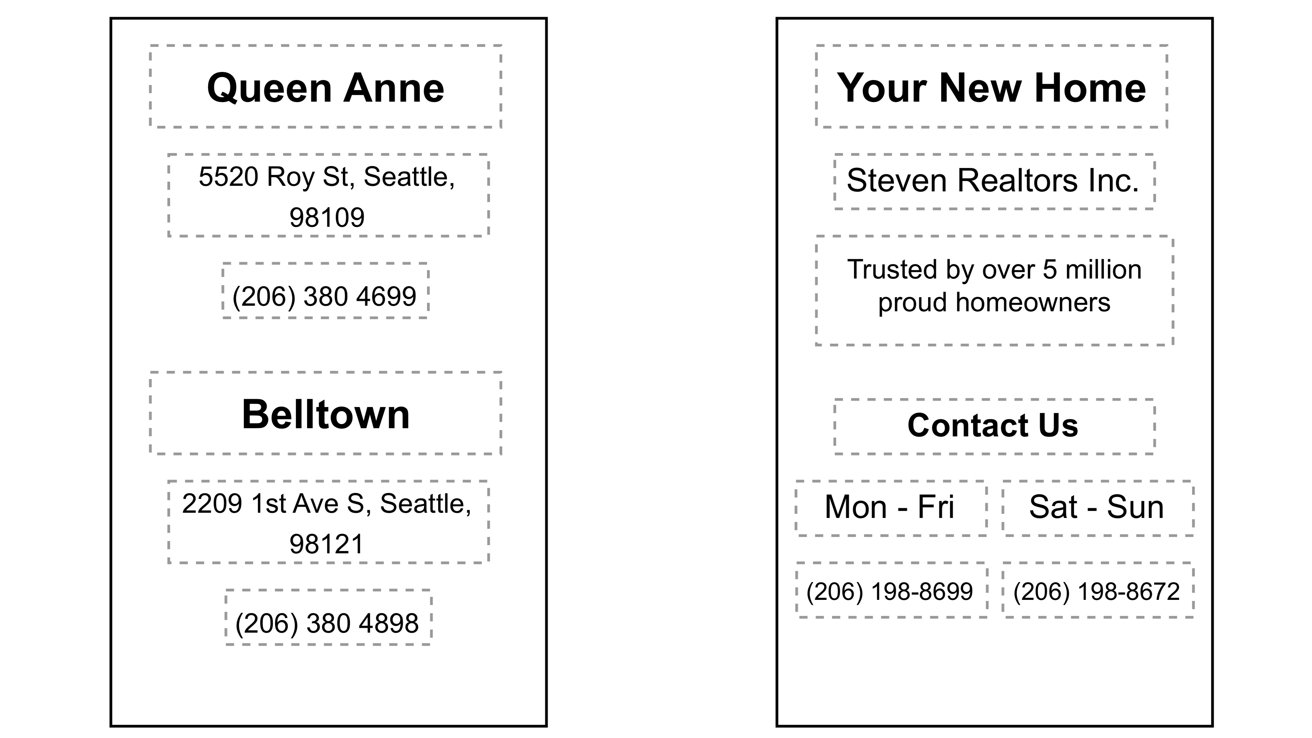
Converting an image into text allows ReALM to skip needing these advanced image recognition parameters, thus making it smaller and more efficient. Apple also avoids issues with hallucination by including the ability to constrain decoding or use simple post-processing.
For example, if you're scrolling a website and decide you'd like to call the business, simply saying "call the business" requires Siri to parse what you mean given the context. It would be able to "see" that there's a phone number on the page that is labeled as the business number and call it without further user prompt.
Apple is working to release a comprehensive AI strategy during WWDC 2024. Some rumors suggest the company will rely on smaller on-device models that preserve privacy and security, while licensing other company's LLMs for the more controversial off-device processing filled with ethical conundrums.
Top Stories
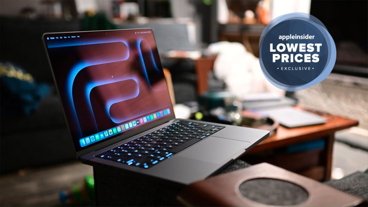
This best-selling M3 MacBook Pro 14-inch with 16GB RAM is on sale for $1,599
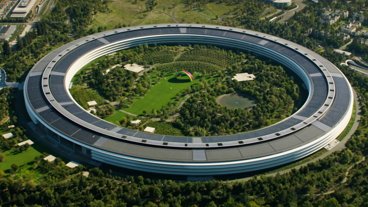
What to expect from Apple's Q2 2024 earnings on May 2
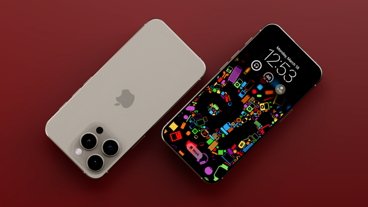
iPhone 16 dummy units show off Capture button, new camera bump
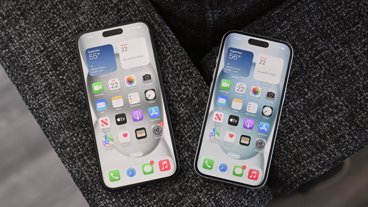
Thinnest iPhone 16 display bezels still a problem for OLED suppliers
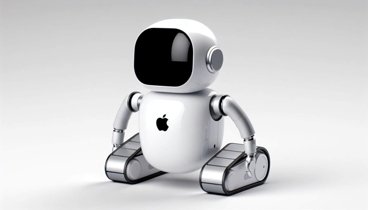
Apple's next big thing could be a home robot
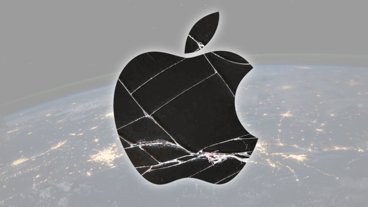
Beyond TSMC, Apple's supply chain will be disrupted by the Taiwan earthquake
Featured deals.
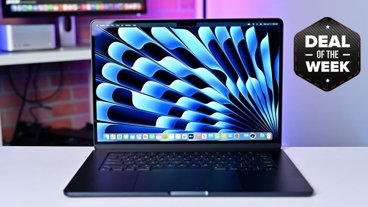
Save $400 on Apple's 15-inch MacBook Air with 24GB RAM, 2TB SSD
Latest comparisons.
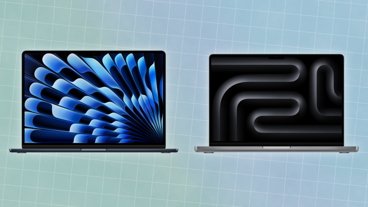
M3 15-inch MacBook Air vs M3 14-inch MacBook Pro — Ultimate buyer's guide
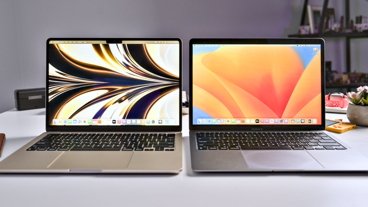
M3 MacBook Air vs M1 MacBook Air — Compared
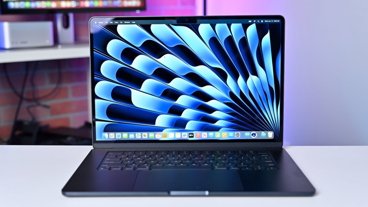
M3 MacBook Air vs M2 MacBook Air — Compared
Latest news.
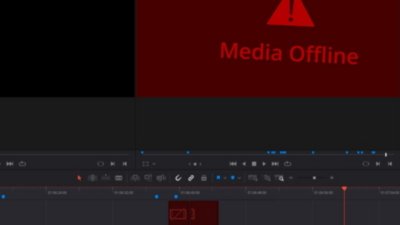
How to fix corrupted DaVinci Resolve projects
Most video creators are familiar with the dreaded "Media Offline" warning and the panic it triggers. For users of DaVinci Resolve, the fix usually takes just a few clicks, but sometimes relinking clips seems impossible, and a project appears to be gone forever.
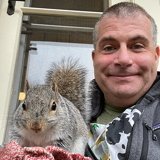
Apple lays off 600 employees, mostly from Apple Car project
After Apple killed its ambitious Apple Car project and microLED Apple Watch display dreams, the company has laid off over 600 employees.
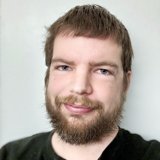
Google's Apple-friendly Find My Devices network launching in April
Apple and Google have worked together to get an interoperability standard off the ground for tracking devices, and Google's Find My Devices network is ready to launch.
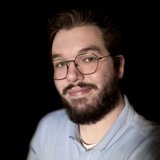
Apple offers a sneak peek into new developer channel with YouTube trailer
Apple's new developer channel on YouTube has released a short video promoting developing for Apple platforms ahead of its Worldwide Developer Conference.
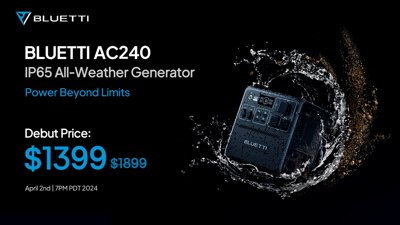
Sponsored Content
Bluetti AC240 portable power station pushes the boundaries with IP65 waterproof rating
Rugged outdoor adventures require tough equipment capable of shrugging off the elements and providing reliability when the weather turns. Bluetti's done just that with the new AC240 weatherproof portable power station, available now with an early bird discount.
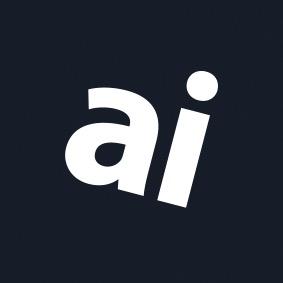
Apple will be revealing its second fiscal quarterly results on May 2. This is what to expect from the financial results and the ensuing analyst conference call.
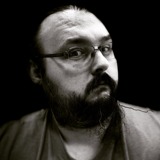
Apple begins notifying WWDC invite lottery winners
If you're a developer who applied to attend WWDC, Apple has begun notifying the lucky few selected to attend in person.
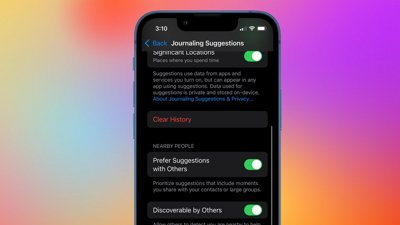
How to turn off Apple's Journal 'Discoverable by Others' setting that's enabled by default
Apple's Journal app automatically opts you into sharing your location with people around you — kind of. The truth is complicated. Here's what it specifically means, and how to opt out.
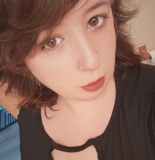
Russian antitrust regulator asks Apple about banking apps while ignoring Ukraine war
Russia's Federal Antimonopoly Service has asked Apple why Russian users cannot access full banking and payment services, while seemingly ignoring how banks in the country were sanctioned over the Ukraine war.
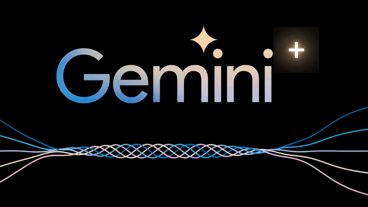
Google could charge Apple users for AI tools in iOS 18
Rumors suggest Google is looking to offer premium generative AI features just as Apple is allegedly planning an AI App Store for iOS 18.
Latest Videos
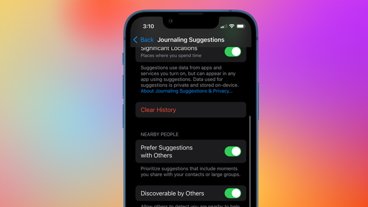
The best Thunderbolt 4 docks and hubs you can buy for your Mac
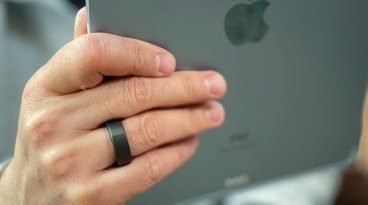
Apple Ring rumors & research - what you need to know about Apple's next wearable
Latest reviews.
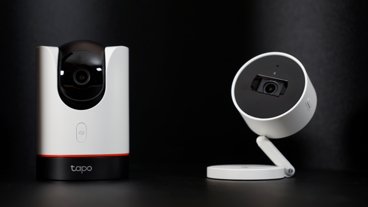
TP-Link Tapo Indoor cameras review: affordable HomeKit options with in-app AI tools
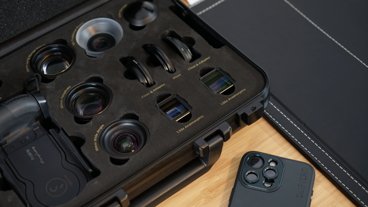
ShiftCam LensUltra Deluxe Kit review: Upgrade your iPhone photo shooting game
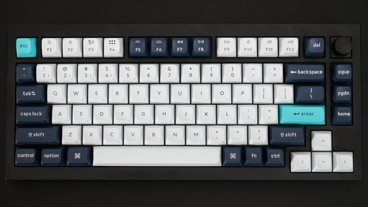
Keychron Q1 Max review: cushy, comfortable, costly

{{ title }}
{{ summary }}
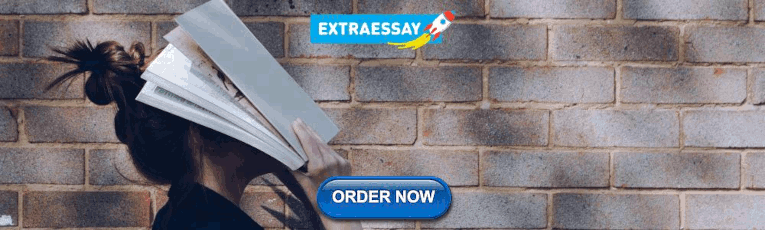
IMAGES
VIDEO
COMMENTS
Harya [13] contends that "language can change and develop because of adaptation of development and pattern change and system of society life, such as level of education, social, culture and ...
Language and national focus of papers in Language Variation and Change, 2016-2021. JofS is modestly more diverse, but still Anglo- and Western-centric: ... Overcoming the Observer's Paradox must be approached differently in research in a new language and culture. Getting a balanced sample of speakers may be impossible in a small community or ...
The influences of linguistic creativity, usage, and cognition on language change are operating at a small scale—for example, within the brain or within a community with a common language—but accumulate to generate large-scale global patterns of linguistic diversity between languages and over time. Therefore, a theory of language change and ...
As with global biodiversity, the world's language diversity is under threat. Of the approximately 7,000 documented languages, nearly half are considered endangered 1,2,3,4,5,6,7,8.In comparison ...
Nature Human Behaviour 1 , 779 ( 2017) Cite this article. Proc. Natl Acad. Sci. USA 114, E8822-E8829 (2017) Languages change over time, which makes it harder to trace their history and genealogy ...
This issue of the Journal of Historical Sociolinguistics aims to contribute to our understanding of language change in real time by presenting a group of articles particularly focused on social and sociocultural factors underlying language diversification and change. By analysing data from a varied set of languages, including Greek, English, and the Finnic and Mongolic language families, and ...
We trace the evolution of Scientific English through the Late Modern period to modern time on the basis of a comprehensive corpus composed of the Transactions and Proceedings of the Royal Society of London, the first and longest-running English scientific journal established in 1665. Specifically, we explore the linguistic imprints of specialization and diversification in the science domain ...
The paper shows that when grammatical words are involved, context is then the unit of language change. Certain changes consist in an active spreading of a form to new contexts, without changing the category or grammatical status of the form; in these cases, context must be considered the unit of language change.
This paper focuses on the micro-analysis of historical data, which allows us to investigate language use across the lifetime of individual speakers. Certain concepts, such as social network analysis or communities of practice, put individual speakers and their social embeddedness and dynamicity at the center of attention. This means that intra-speaker variation can be described and analyzed in ...
Abstract. Languages emerge and change over time at the population level though interactions between individual speakers. It is, however, hard to directly observe how a single speaker's linguistic innovation precipitates a population-wide change in the language, and many theoretical proposals exist. We introduce a very general mathematical ...
Studies in Language Change presents empirically based research that extends knowledge about changes in languages over time and historical relations among the world's languages without restriction to any particular language family or region. While not devoted explicitly to theoretical explanations, the series hopes to contribute to the advancement in understandings of language change as well ...
Founded by William Labov, Language Variation and Change is the only journal dedicated exclusively to the study of linguistic variation and the capacity to deal with systematic and inherent variation in synchronic and diachronic linguistics. Sociolinguistics involves analysing the interaction of language, culture and society; the more specific study of variation is concerned with the impact of ...
3.1The facts of Portuguese language change. In this paper, we focus on a particular change in phonological and syntactic Portuguese recently discussed by Galves & Galves (1995). Roughly, over a period of 200 years, starting from 1800, ''classical'' Portuguese (CP) underwent a change in clitic placement.
Abstract. This paper traces the changing perceptions of language in sociolinguistics. These perceptions of language are reviewed in terms of language in its verbal forms, and language in vis-à ...
Related, the use of big data could help us document how language attitudes are expressed in a wider range of contexts (e.g., social media; Durham, 2016), as well as provide us with a better understanding of how language attitudes change over time. More longitudinal research would also be helpful in this respect. While we know that specific ...
Language change is the focus of linguistic research. There are various theories on language change, among which the most important ones are Family Tree Theory, Wave Theory, Theory of Lexical Diffusion, Labov's Theory of Linguistic Variation, Overlapped Sound Change Theory, and the Punctuated Equilibrium Model. By discussing physical thoughts in these theories, this paper proposes that since ...
The paper suggests that 'language change' might hold an important key to aspects of educational reform and to the betterment of teacher education. The language we identify as contributing the most to the ineffectiveness of educational reform is the educational language impregnated by psychologised metaphors, which dominate educational ...
This study aims at analysing language change on social media which are now popular and dynamic. Combining Technological Determinism theory and Computer-mediated Discourse Analysis, it investigates the language use on focus WhatsApp groups and discovers that the medium is full of abbreviations, nonstandard spellings, emojis, and logograms.
Transactions on Machine Learning Research. Yang Xu, Yiheng Xu, Tengchao Lv, Lei Cui, Furu Wei, Guoxin Wang, Yijuan Lu, Dinei Florencio, Cha ... Joint Conference on Natural Language Processing (Volume 1: Long Papers), pages 2579-2591, Online. Association for Computational Linguistics. Yiheng Xu, Minghao Li, Lei Cui, Shaohan Huang, Furu
ReALM: Reference Resolution As Language Modeling. Joel Ruben Antony Moniz, Soundarya Krishnan, Melis Ozyildirim, Prathamesh Saraf, Halim Cagri Ates, Yuan Zhang, Hong Yu, Nidhi Rajshree. Reference resolution is an important problem, one that is essential to understand and successfully handle context of different kinds. This context includes both ...
Research Guide on Language Change. Volume 48 in the series Trends in Linguistics. Studies and Monographs [TiLSM]
We introduce Flamingo, a family of Visual Language Models (VLM) with this ability. We propose key architectural innovations to: (i) bridge powerful pretrained vision-only and language-only models, (ii) handle sequences of arbitrarily interleaved visual and textual data, and (iii) seamlessly ingest images or videos as inputs. Thanks to their ...
In an effort to reduce the computational load of Transformers, research on linear attention has gained significant momentum. However, the improvement strategies for attention mechanisms typically necessitate extensive retraining, which is impractical for large language models with a vast array of parameters. In this paper, we present DiJiang, a novel Frequency Domain Kernelization approach ...
The research will be presented at the International Conference on Learning Representations. Finding facts. Most large language models, also called transformer models, are neural networks. Loosely based on the human brain, neural networks contain billions of interconnected nodes, or neurons, that are grouped into many layers, and which encode ...
Large language models (LLMs) such as Open AI's GPT-4 (which power ChatGPT) and Google's Gemini, built on artificial intelligence, hold immense potential to support, augment, or even eventually ...
The paper details something called ReALM — Reference Resolution As Language Modeling. Having a computer program perform a task based on vague language inputs, like how a user might say "this" or ...