Attracting and recruiting quality talent: firm perspectives
Rajagiri Management Journal
ISSN : 0972-9968
Article publication date: 26 August 2020
Issue publication date: 13 October 2020
Understanding matching patterns and determinants of attracting quality talents is an under-researched area, especially from a firm perspective. Firm’s recruitment strategies have an impact on the sorting patterns in the labour market which remains undetermined. This paper aims to explore the drivers of attracting and recruiting quality talents. Also, the role of policies including the national labour laws, industry norms and localised firm policies have on hiring practices and drivers in a developing country.
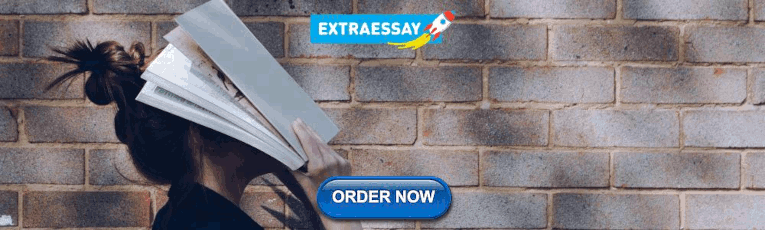
Design/methodology/approach
This study is underpinned by network theory, equity theory, social exchange theory and resource-based theory. The authors leveraged on a mixed methodology that is a structured questionnaire administered to 200 firm representatives in Lagos and interviews with key informants from the demand side for labour.
The study revealed that firms can leverage on salary, brand name, referral, job security as core factors in attracting and recruiting quality talents. Also, digitisation is a key strategy leveraged on attracting and recruiting quality talents. Techniques such as the use of social media, traditional media, online interviews, physical interviews have proven to help in selecting quality talents.
Originality/value
Specifically, the paper throws light on how firms use different recruitment channels for hiring workers, and how the use of these channels affects the quality of matches. Furthermore, the role of social networks, wages and benefits for firm recruitment and matching efficiency was well highlighted.
- Recruitment
- Labour market
- Quality talents
Adeosun, O.T. and OHIANI, A.S. (2020), "Attracting and recruiting quality talent: firm perspectives", Rajagiri Management Journal , Vol. 14 No. 2, pp. 107-120. https://doi.org/10.1108/RAMJ-05-2020-0016
Emerald Publishing Limited
Copyright © 2020, Oluyemi Theophilus Adeosun and Adeku Salihu OHIANI.
Published in Rajagiri Management Journal . Published by Emerald Publishing Limited. This article is published under the Creative Commons Attribution (CC BY 4.0) license. Anyone may reproduce, distribute, translate and create derivative works of this article (for both commercial and non-commercial purposes), subject to full attribution to the original publication and authors. The full terms of this license may be seen at http://creativecommons.org/licences/by/4.0/legalcode
1. Introduction
Attention has recently given to understand how organisations source for their workforce, because employees are widely recognised as valuable assets that organisations can leverage upon for survival and attaining competitive advantage. Firms are constrained with a number of challenges in achieving their production objectives ( Deakins and Bensemann, 2019 ; Fowowe, 2017 ). With an increasing rate of unemployment in sub Saharan Africa ( Abraham and Nosa, 2018 ; Ihensekhien and Aisien, 2019 ), and a focus on decent employment ( Moen et al. , 2020 ), the effort of all stakeholders in the labour market is worth exploring. Understanding matching patterns and determinants of attracting quality talents is an under researched area especially from the firm perspective ( Ghavidel et al. , 2019 ). Firms recruitment strategies have impacts on the sorting patterns in the labour market which remains undetermined ( Hensvik and Skans, 2016 ; Nekoei and Weber, 2017 ). Furthermore, recruiting quality talent enhances productivity and performance which every firm strives to achieve ( Cascio, 2014 ; Maheshwari et al. , 2017 ). Lagos has about 6,000 registered firms cutting across various industries ( Nigeria Companies Directory (NCD), 2020 ). The number of available talents is approximately 9 million youths as at 2020 ( World Population Review, 2020 ).
In developed countries, there are generally openly known industry and role compensation packages ( Adom, 2018 ; Clemens et al. , 2017 ). Different roles have a range of rate per hour or per year. This is however not the same for most sectors in Lagos, Nigeria. Firms which are unable to attract the best talents have issues with productivity and output. Firms in Lagos compete with global companies for their local talent and can easily loose the talent to countries like Canada, Australia and other countries open to immigrant of skilled talents ( Marsh and Oyelere, 2018 ; Chand and Tung, 2019 ). Lagos has a high influx of fresh graduates and also concentration of experienced professionals. The competition for exceptional talent is fierce, and they typically not always on the lookout for job opportunities at least actively ( Mahony, 2019 ; Kireru, 2019 ). Some of these exceptional talents too already are engaged in freelancing and private practice ( Perampalam et al. , 2017 ; Schwartz, 2018 ), some calibers of professionals are social media savvy; others are no, and not be easily visible for opportunities. While the professionals typically talk and engage with each other and share information around compensation and benefits; therefore, the firms are not always this cooperative as they compete for the scare talents ( Chunyan et al. , 2015 ).
Lagos is the “industrial and commercial hub” of Nigeria and the most industrialised state in the country ( Ikpe and Torriti, 2018 ). Lagos accounts for 80% of the value-added growth in Nigeria's manufacturing sector and generates 65% of Nigeria's value added tax (VAT) (Central Bank of Nigeria 2017). Lagos is also the financial capital of the country. It has more than 200 well capitalised and efficiently managed banking and financial institutions ( Juliana and Okoye, 2016 ; Adewale, 2018 ). Lagos accommodates the largest number of businesses among all the states in the federation and 11.5% of small businesses in Nigeria (Small Medium and Development Agency of Nigeria, 2013). Lagos has a large population of about 17.5 million as of 2017 ( National Bureau of Statistics report, 2018 ) which translates into a huge market for goods and services. It is home to the Apapa and the Tin Can Island seaport that handles 70% of total national cargo freight in Nigeria; this has increased her need for recruitment of quality talents by firms operating in the state.
With an average graduate churn out of 600,000 by tertiary institutions in Nigeria and the graduate unemployment put at 25.4 m or 38.1% ( NBS, 2019 , Q2), it looks like sourcing for employees should be easy. However, many employers of labour lament that they find it difficult to fill vacant roles ( Eneji et al. , 2013 ; Longe, 2017 ). Getting a quality candidate out of a large pool is difficult due to the quality of candidates and lack of workplace readiness ( Aloysius, 2019 ; Silva and Crisp, 2020 ). Consequently, multiple layers of assessment and screening are put in place at a great cost by firms to sieve through the crowd and pick out the best and brightest. On the other hand, the exceptional graduates who are few are conscious of their worth and value and hence highly mobile ( Lievens and Slaughter, 2016 ; Hu, 2017 ; Okolie and Irabor, 2017 ). This is particularly true of the younger generation called Millennial. This is part of the challenge firm faces in attracting and retaining quality talents.
Therefore, to be at the forefront in retaining talents, firm brand need to display the capability of projecting the image and personality of the organisation as an attractive employer of labour, exposing employee to its guiding principles, ideals, code of conduct as a way of attracting, engaging and retaining quality talents ( Drury, 2016 ; Dabirian et al. , 2017 ). Also, Chabra and Sharma (2014) opined that determinants of workplace attractiveness such as compensation, career opportunities, nature of the job and corporate culture should be communicated by the employer brand to enable organisations to attract, engage and retain talents.
This research therefore explores the drivers of attracting and recruiting quality talents, and the roles that national labour laws, industry norms and localised firm policies have on hiring practices and drivers in developing country. Specifically, this paper tends to throw more light on the following research questions: how do firms use different recruitment channels for hiring workers, and how does the use of these channels affect the quality of matches? What is the role of social networks for firm recruitment and for matching efficiency? To what extent do firms use wages and other benefits to improve their hiring process? Are recruited talents productive and capable of improving performance?
2. Literature review
2.1 employee recruitment.
Recruitment has a long history, most likely to pre-historic times because people tend to consume the services of others to achieve what they cannot singlehandedly accomplish ( Adetunji and Ogbonna, 2013 ). The process of searching for the best candidates and attracting them to consider job offers is regarded as recruitment ( Cole, 2005 ; Bhupendra and Swati, 2015 ). However, when an organisation ended up either erroneously or intentionally hiring the wrong candidates for its jobs, then it becomes a liability or a huge cost that might negatively affect the business activities of such a firm ( Ekwoaba et al. , 2015 ). This has therefore made recruitment process a human resource strategy that can determine the fortune of an organisation, because, getting high-quality talents and competent workforce is a key source of sustainable competitive advantage ( Hiltrop, 1999 ; Bondarouk et al. , 2014 ). Acquiring and retaining superior talent through appropriate recruitment process is critical to firm’s image ( Oginni and Ogunyomi, 2012 ; Bouton, 2015 ). It is a valuable weapon for an organisation that has workforce of good quality talent ( Tlaiss et al. , 2017 ).
2.2 Employee recruitment process
Recruiting talented workforce is critical to a firm’s success and superior performance, but to hire the most talented, effective recruitment process needs to be considered ( Breaugh, 2009 ). It is argued by this author that no best way to hire best talented, but the situation at a point determines the best method to apply. However, Figure 1 demonstrates a recruitment process as proposed by Breaugh (2009) .
The model in Figure 1 indicates that, for an organisation to hire talent to fill a gap, the first thing to do is to strategically formulate recruitment objectives. This could be carried out by seeking inputs from those that have vital information about the skills required and the particular unit that is in need of such talent. The second phase of the model maintains that there should be a coherent strategy to fill the gap through specific plan of actions ( Breaugh, 2009 ). The third phase of the model is about exploring all possible channels through which the needed talent can be acquired, either internally or externally. Most human resources managers are concerned about whether their outcomes meet their original objectives, but unfortunately, some many firms do not formally appraise recruitment efforts ( Breaugh, 2009 ; Carlson et al. , 2002 ). Measuring and evaluating results in the fourth phase of the model involves comparing the current result with the previous data either from within or information from universities, recruiting agencies, consulting firms or any other external source. The model is cyclical in nature because firms continuously engage in hiring talent to fill gaps as long as they still exist.
2.3 Sources of recruitment
Recruitment can be carried out either by the organisation itself or contracting it out to an external body through outsourcing. However, selecting the most effective recruitment source is situational and it has its attendant benefits and challenges according to Arthur (2004) . Some of the recruitment sources, their benefits and challenges are demonstrated in Table 1 .
2.4 Social media
According to Kaplan and Haenlein (2010) , social media is an internet-based platform that allows users to generate and exchange ideas, contents and values. For this reason, social media has increasingly played a crucial role in human resources management in general and hiring quality talent in particular ( Landers and Schmidt, 2016 ). Landers and Schmidt (2016) opine that social network sites contain three major features such as allow users to generate a public personal profile; specify a list of connections with others using sites like Facebook, Twitter and LinkedIn; and observe and explore content from within and connections of others to achieve a purpose. Adopting social media platforms for recruitment has its benefits and challenges. Most opinion in the practice of social media-assisted employee hiring is informal, unsystematic, muddled and anecdotal ( Bondarouk et al. , 2013 ; Landers and Schmidt, 2016 ). However, social recruiting approach can offer speed, efficiency and the firm’s ability to target and attract suitable candidates, and as well provide useful and additional information about a potential candidate or job seeker ( Emanuela, 2018 ).
The internet has offered many companies the opportunity of reducing the burden of recruitment and they have keyed into it in their recruitment process ( Mindia and Hoque, 2018 ). Against the huge time and resource required in screening qualified candidates, given a large pool of applicant. Software have now been a veritable tool in the screening of applicant ( Omolawal, 2015 ). The internet provides an avenue for online aptitude test and assessment which is becoming more popular and widely acceptable in the selection and requirement of quality talents ( Randall, 2016 ; Ujah-Ogbuagu, 2019 ). Interview process is aided with video conferencing, reducing cost of transport and other associated risk, offer letter can be gotten through the internet, e-signing allows acceptance or rejection of an offer online without much stress ( Petre et al. , 2016 ).
In another vain, the development of database which allows job seeker upload their curriculum vitae provides an avenue for recruiters to search for candidates that suit vacant roles, it also allows those who are willing to change job to apply without being very active in job search ( Fiandrino et al. , 2016 ; Leach et al. , 2017 ). Among software being used are HR software, Application Tracking System (ATS), web 2.0 Artificial Intelligence (AI) among many others. Albert (2019) and Son et al. (2019) . The use of internet further positively improves the corporate image and profile of firms, reduction in recruitment cost, administrative burden are some of the benefit of using the internet as a recruiting platform ( Fred and Kinange, 2018 ).
3. Theoretical framework
This study is underpinned by network theory, equity theory, social exchange theory and resource-based theory. Network theory is proposed by Johanson and Mattsson (1988) in with the postulation that every business venture makes use of available networks to acquire resources both human and material to achieve its goals and objectives. The network theory is useful in this study to address the issue of how firms in Lagos relate and network with the business environment to make use of the available recruiting channels through social networking for them to source and recruit quality talents. However, networking alone may not be able to resolve the issue because employees will like to take the jobs that seem fair to them according to equity theory as postulated by Adams (1963) . Organisations sometimes find it difficult to recruit and select employees with the right qualifications to help realise goals, but the appropriate recruitment and selection is that which gives every applicant an equal opportunity ( Deshpande and Golhar, 1994 ; Atkinson and Storey, 1994 ).
Every organisation is a social entity that takes inputs from the environment and in returns, sends outputs back to the same environment. For this reason, firms engage in social exchange. Social exchange theory has gained much interest in the literature and has been applied in understanding workplace behaviour ( Cropanzano and Mitchell, 2005 ), such as psychological contracts ( Rousseau, 1995 ) and networks ( Brass et al. , 2004 ). One of the major arguments of this theory is that, it is an exchange of values or actions between individuals ( Homans, 1961 ); therefore, the extent to which firms use wages and other benefits in their hiring process may attract quality talents that will serve as valuable assets. Human resource is a valuable assert for an organisation, because resource based theory has maintained that competitive advantages can be attained by the firm’s resources that are rare ( Barney , 1991, 2001 ).
4. Data, methods and descriptive analysis
4.1 methods.
The paper leveraged on mixed methodology. We are motivated to use this research method because of its potential to confirm research findings through triangulation, gather richer data, and to initiate new modes of thinking ( Rossman and Wilson, 1985 ; Denscombe, 2008 ). We adopted both survey and also interviews with key informants ( Marshall,1996 ). The key informant technique was adopted to obtain quality insights and information data within a short period and also practical solutions ( Marshall,1996 ). The key informants were identified upfront abd were selected based on the fact that they are opinion and thought leaders in the Human Resource space. Twenty key informants were identified and interviewed successfully.
We used structured interview with open-ended and closed-ended questions and interviews with key informants from the demand side for labour. We conducted the primary survey within the first quarter of 2020, administering 200 questionnaires in a five-point Likert scale form to human resource managers who advise the organisations management on people matters including recruitment and attraction of new employees; representatives of employers of labour from the organised private sector and government agencies based in Lagos.
For the sample size, we leverage on the 250 population of the Nigerian Employers Consultative Association.
We arrived at the sample size (150) by applying the Yamane formula for determining the sample size given by: n=N/(1+Ne^ 2) where
n = corrected sample size
N = population size and e = Margin of error (MoE)
e = 0.05 based on the research condition ( Yamane, 1967 ; Singh and Masuku, 2013 ).
However, we reached out to 200 people to accommodate for no response or invalid responses and to have a more robust in-depth. The study used descriptive statistics ( Boodhoo and Purmessur, 2009 ) to provide inference from data gathered. Descriptive statistics was used as data obtained are qualitative, in a form of likert scale question.
The population cuts across all the diferent sectors and industry as a cross-sectional approach was taken to the survey using simple random sampling to provide equal opportunities to all Human Resource managers across all sectors within the membership base. The survey was administed using google survey to the participants who were informed of the purpose of the survey. Of the 200 questionnaires, 168 was returned while 18 was incomplete, 150 was valid and therefore used in the course of the study. We proceed to use descriptive analysis to generate insights from the data generated.
4.2 Data analyses
4.2.1 result presentation..
In attracting suitable talents by firm, several factors contribute to the choice of candidate selected.
In carrying out this study, firms in the private sector form the majority, constituting 79% of the sample; this is because private firms carry out recruitment processes more when compared to the private sector. Hence, they have better contribution than the public sector. From the respondents, most firms hire senior members of the organisation from outside the firm with the belief that they will be able to inject fresh ideas that will aid productivity and performance ( Table 2 ).
The impact of digitisation is evident on the recruitment process of the firms; most of them took advantage of technology to drive their selection, training and placement processes. From the sample, 46% do not state salary when placing advertisement, stating that it mostly gives rise to higher application and makes it difficult to select those who are passionate about the job, while a higher salary scale attracts many candidates, a lower salary scale can further put candidates off including capable candidates. However, 29% include salary range in calling for application. Overall, firms outline benefit attached to a vacant position when seeking qualified candidates.
In attracting quality talents, firms leverage on some certain factors, among such factor is salary, brand name, flexible working conditions among other. Table 3 highlights the effect of such factors in attracting and recruiting quality talents.
Expert in the human capital believe strongly that firm can leverage on salary, brand name, referral, job security as core factors in attracting and recruiting quality talents. Among the respondents, there are divergent opinions about job title as a core factor in attracting and recruiting quality talents, for instance, 36% agreed, 41% disagreed, while 22% are uncertain of the importance of job title as a factor to leverage on in attracting and recruiting quality talents. Outsourcing and recruiting agencies are seen as a viable factor to leverage on in attracting and recruiting quality talents most of the respondents agreed to their impact. There is also a mix feeling about flexible working opportunities as a factor to leverage on in attracting and recruiting quality talents. In the bid to attract quality talents, job security play a vital role, employee are always looking for jobs that can guarantee them long time job security; therefore, firm with job security leverage on such goodwill to attract talent unlike firms that do not guarantee job security.
Firm overtime develops certain criteria which form porting of the selection process. Indeed, many of such criteria have barred many from being considered in the selection process. Table 4 gives a breakdown of the position of the respondents when asked about their thought on such criteria in selection process. While most of the respondents are in favour of Bachelor of Science over Higher National Diploma, as a factor to consider in the selection process, many are also of the opinion that the class of degree obtained in various institution of learning is also a strong criterion to consider. They favour first-class candidate and those with second-class upper over those with lower grades such as second-class lover, third class and pass.
When it comes to school attended by candidates, about 57% of the respondents believe there should not be discrimination between those who went to private, federal and state schools. Furthermore, age and gender of candidate should not be a barrier to their being selected. However, some respondents are of the opinion that there are some certain positions that cannot be managed by a person of lower age or gender.
Aside from leveraging on some key factors in attracting quality talents, techniques applied in the selection process was considered. Techniques such as the use of social media, traditional media (television, newspapers and radio), online interview, physical interview, etc., were considered. Respondents were asked to evaluate the impact of the technique in attracting and recruiting quality talents, the result is presented in Table 5 .
Recruiters believe that the social media platform is a good technique to attract suitable candidates. Uncertainty was expressed in their opinion on traditional media such as newspaper, television and radio, they believe the impact may not be measurable and may lead to pool of unskilled applicant; furthermore, talent targeted at may not see such advert. Respondents are in agreement with using professional and personal networks to attract quality talents, it is believed that professionals are able to identify and recommend talents that are capable of filling vacant positions. In face of ever improving technology, online interviews and assessment are been embraced by recruiters and found to be a good technique to attract quality talent, physical assessment of talents have also proven to be a good technique in attracting quality talents.
4.2.2 Qualitative data analysis-interview.
In the face of modern trend in the labour space, respondents were asked to suggest modifications that can be adopted or suitable for firms to attract and recruit quality talent. The expert feedback generally aligned with the feedback from the administered questionaires. Some of their responses are standardisation of benefits within industries and salary disclosure in all advertisements; review of labour laws to cover electronic recruitment, remote working and emerging work situations. Also more investment by firm management in technology tools such as artificial intelligence and digitalisation should be embraced at work place. Furthermore, candidates are advised to be more tech savvy and familisare themselves with mordern apps and gadgets as traditional recruitment would soon be the exception rather than the rule. Firms also use technology to drive feedback to applicants and carry them along on their interview status.
In the bid to improve organisational policies with respect to recruitment, suggestions were made by the respondents, such suggestions are as follows: Organisations are encouraged to leverage more on technology, do first level interviews electronically before candidates are brought in for physical assessment; video teleconference can be adopted this will reduce cost of physical travel expenses and risk associated with travelling; social media and technology play a vital role in modern recruitment; hence, it positives can be adopted in recruitment process; improved disclosure and data protection; there should be more background check even during and after recruitment to ensure credibility and prevent impersonafication or cheating by the candidates. The government should also improve generally on infrastructure such as electricity and internet bandwidth that technology depends on as the absence of this is sometimes a source of frustration to the firms and candidates.
5. Conclusion and policy implications
The study aims to examine the firm perspective in the recruitment process, what they look forward to in their selection and recruitment processes. Overall, firms have high expectations from candidates seeking to fill vacant positions at various points in time. It was found out in this study that firms can leverage on salary, brand name, referral, job security as core factors in attracting and recruiting quality talents. Digitisation is regarded as a key ingredient in attracting and recruiting quality talents. Techniques such as the use of social media, traditional media, online interview, physical interview have proven to help in selecting quality talents. Evidently, the study found relationship between the network theory which gives an overview of the interactions within an organisation and the views of the respondent. Many human resources manager leverage on relationship with colleagues and social networks in the bid to attract quality talents. Findings also aligned with the equity theory, fairness to employee, employee compensation, etc., are key in attracting and retaining quality talents.
It is recommended that organisations are encouraged to invest more in technology and digitalisation so they can leverage more on information technology and the social media platforms to aid in attracting quality talents, and adequate background checks should be done on candidates to curb falsification of certificates and identity. Staff and management involved in recruitment should be trained in how to manage remote interviews and engage with candidates appropriately. The organisation interfaces via social media should be enhance and populated with information that will attract the right kind of candidates. In addition, video teleconference can be adopted; there should be eradication of age restrictions on vacancies. Online assessment can be done to ease stress on the candidates and save time in filling vacancies. Furthermore, the labour laws should be reviewed to support electronic recruitment, remote working and electronic docuementations of employees and contract enforcement. Greater transparency in wage and benefit information disemination should encourage to reduce exploitation of employees at all levels.
Employee recruitment process
Sources of recruitment
Extent to which respondent agree or disagree with below techniques in attracting and recruiting quality talents
Source: Author computation 2020
Abraham , I.O. and Nosa , A.L. ( 2018 ), “ Unemployment and output growth: evidence from upper middle-income countries in Sub-Saharan Africa ”, American Economic and Social Review , Vol. 3 No. 1 , pp. 32 - 43 .
Adams , J.S. ( 1963 ), “ Toward an understanding of equity ”, The Journal of Abnormal and Social Psychology , Vol. 67 No. 5 , pp. 422 - 434 .
Adetunji , O.J. and Ogbonna , I.G. ( 2013 ), “ Corporate social responsibility as a recruitment strategy by organisations ”, International Review of Management and Business Research , Vol. 2 No. 2 , pp. 313 - 319 .
Adewale , O.P. ( 2018 ), “ Value added tax (vat) and economic development of nigeria: a case study of Lagos state metropolis ”, doctoral dissertation, department of accounting, faculty of management science, National Open University of Nigeria .
Adom , A.Y. ( 2018 ), “ The role of commission-based pay on employee retention in the Ghanaian banking industry: the case of GCB bank limited ”, Africa’s Public Service Delivery and Performance Review , Vol. 6 No. 1 , pp. 1 - 5 .
Albert , E.T. ( 2019 ), “ AI in talent acquisition: a review of AI-applications used in recruitment and selection ”, Strategic HR Review , Vol. 18 No. 5 , pp. 14 - 22 .
Aloysius , O.I. ( 2019 ), “ Exploring university and industry perceptual meaning of employability skills among Nigerian graduates ”, International Journal of Educational Science and Research (IJESR) , Vol. 9 No. 1 , pp. 21 - 36 .
Arthur , D. ( 2004 ), Fundamentals of Human Resources Management , 4th ed. , American Management Association , New York, NY .
Atkinson , J. and Storey , D. ( 1994 ), Small Firms and Employment, Employment in the Small Firm and the Labour Market , Routledge , London .
Barney , J.B. ( 1991 ), “ Firm resources and sustained competitive advantage ”, Journal of Management , Vol. 17 No. 1 , pp. 99 - 120 .
Barney , J.B. ( 2001 ), “ Resource-based theories of competitive advantage: a ten-year retrospective on the resource-based view ”, Journal of Management , Vol. 27 No. 6 , pp. 643 - 650 .
Bhupendra , S.H. and Swati , G. ( 2015 ), “ Opportunities and challenges of e-recruitment ”, Journal of Management Engineering and Information Technology , Vol. 2 No. 2 , pp. 1 - 4 .
Bondarouk , T. , Marsman , E. and Rekers , M. ( 2014 ), “ HRM, technology and innovation: new HRM competences for old business challenges? ”, Human Resource Management, Social Innovation and Technology Advanced Series in Management , Vol. 14 , pp. 179 - 215 .
Bondarouk , T. , Ruël , H. , Axinia , E. and Arama , R. ( 2013 ), “ What is the future of employer branding through social media? Results of the delphi study into the perceptions of HR professionals and academics ”, Social Media in Human Resources Management Advanced Series in Management , pp. 23 - 57 .
Boodhoo , R. and Purmessur , R.D. ( 2009 ), “ Justifications for qualitative research in organisations: a step forward ”, The Journal of Online Education , pp. 1 - 7 .
Bouton , K. ( 2015 ), “ Recruiting for cultural fit ”, Harvard Business Review , available at: https://hbr.org/2015/07/recruiting-for-cultural-fit
Brass , D.J. , Galaskiewicz , J. , Greve , H.R. and Tsai , W. ( 2004 ), “ Taking stock of networks and organizations: a multilevel perspective ”, Academy of Management Journal , Vol. 47 No. 6 , pp. 795 - 817 .
Breaugh , J.A. ( 2009 ), Recruiting and Attracting Talent: A Guide to Understanding and Managing the Recruitment Process , Strategic Human Resource Management Foundation .
Carlson , K.D. , Connerley , M.L. and Mechan , R.L. ( 2002 ), “ Recruitment evaluation: the case for assessing the quality of applicants attracted ”, Personnel Psychology , Vol. 55 No. 2 , pp. 461 - 490 . PP.
Cascio , W.F. ( 2014 ), “ Leveraging employer branding, performance management and human resource development to enhance employee retention ”, Human Resource Development International , Vol. 17 No. 2 , pp. 121 - 128 .
Chabra , N.L. and Sharma , S. ( 2014 ), “ Employer branding: strategy for improving workplace attractiveness ”, International Journal of Organizational Analysis , Vol. 22 No. 1 , pp. 48 - 60 .[Mismatch ]
Chand , M. and Tung , R.L. ( 2019 ), “ Skilled immigration to fill talent gaps: a comparison of the immigration policies of the United States, Canada, and Australia ”, Journal of International Business Policy , Vol. 2 No. 4 , pp. 333 - 355 .
Chunyan , X. , Bagozzi , R.P. and Meland , K.V. ( 2015 ), “ The impact of reputation and identity congruence on employer brand attractiveness ”, Marketing Intelligence and Planning , Vol. 33 No. 2 , pp. 1 - 35 .
Clemens , J. , Kahn , L.B. and Meer , J. ( 2017 ), Wage Regulation, Compensation Packages, and Worker Welfare , University of CA at San Diego, Economics Department , Clemens .
Cole , G.A. ( 2005 ), Personnel and Human Resources Management , 5th ed. , Thompson Learning , London .
Cropanzano , R. and Mitchell , M.S. ( 2005 ), “ Social exchange theory: an interdisciplinary review ”, Journal of Management , Vol. 31 No. 6 , pp. 874 - 900 .
Dabirian , A. , Kietzmann , J. and Diba , H. ( 2017 ), “ A great place to work!Understanding crowdsourced employer branding ”, Business Horizons , Vol. 60 No. 2 .
Deakins , D. and Bensemann , J. ( 2019 ), “ Achieving innovation in a lean environment: how innovative small firms overcome resource constraints ”, International Journal of Innovation Management , Vol. 23 No. 4 , pp. 1 - 36 .
Denscombe , M. ( 2008 ), “ Communities of practice: a research paradigm for the mixed methods approach ”, Journal of Mixed Methods Research , Vol. 2 No. 3 , pp. 270 - 283 .
Deshpande , S. and Golhar , D.Y. ( 1994 ), “ HRM practices in large and small manufacturing firms: a comparative study ”, Journal of Small Business Management , Vol. 32 No. 2 , pp. 49 - 56 . P
Drury , P. ( 2016 ), “ Employer branding: your not-so-secret weapon in the competition for young workers ”, Human Resource Management International Digest , Vol. 24 No. 3 , pp. 29 - 31 .
Ekwoaba , J.O. , Ikeije , U.U. and Ufoma , N. ( 2015 ), “ The impact of recruitment and selection criteria on organizational performance ”, Global Journal of Human Resource Management , Vol. 3 No. 2 , pp. 22 - 33 .
Emanuela , T.A. ( 2018 ), “ The impact of social media on recruitment ”, Ovidius” University Annals, Economic Sciences Series , Vol. 18 No. 2 , pp. 537 - 541 .
Eneji , M.A. , Mai-Lafia , D. and Weiping , S. ( 2013 ), “ Socio-economic impact of graduate unemployment on Nigeria and the vision 20:2020 ”, International Journal of Development and Sustainability , Vol. 2 No. 1 , pp. 148 - 176 .
Fiandrino , C. , Kantarci , B. , Anjomshoa , F. , Kliazovich , D. , Bouvry , P. and Matthews , J. ( 2016 ), “ Sociability-driven user recruitment in mobile crowdsensing internet of things platforms ”, 2016 IEEE Global Communications Conference (GLOBECOM) , IEEE , pp. 1 - 6 .
Fowowe , B. ( 2017 ), “ Access to finance and firm performance: evidence from African countries ”, Review of Development Finance , Vol. 7 No. 1 , pp. 6 - 17 .
Fred , M.O. and Kinange , U.M. ( 2018 ), “ Effectiveness of e-recruitment in organization development ”, Management and Economic Journal , pp. 294 - 301 .
Ghavidel , B.M. , Nasirpour , K. and Asgari , A. ( 2019 ), “ Identifying and explaining the dimensions of teacher talent management using the grounded theory ”, International Journal of Schooling , Vol. 1 No. 2 , pp. 23 - 36 .
Hensvik , L. and Skans , O.N. ( 2016 ), “ Social networks, employee selection, and labor market outcomes ”, Journal of Labor Economics , Vol. 34 No. 4 , pp. 825 - 867 .
Hiltrop , J.M. ( 1999 ), “ The quest for the best: human resource practices to attract and retain talent ”, European Management Journal , Vol. 17 No. 4 , pp. 422 - 430 .
Homans , G.C. ( 1961 ), ‘Social Behavior’: Its Elementary Forms , Harcourt Brace , New York, NY .
Hu , L. ( 2017 ), “ Job accessibility and employment outcomes: which income groups benefit the most? ”, Transportation , Vol. 44 No. 6 , pp. 1421 - 1443 .
Ihensekhien , O.A. and Aisien , L.N. ( 2019 ), “ Growth-youth unemployment nexus in upper middle income countries in Sub-Saharan Africa ”, Turkish Economic Review , Vol. 6 No. 1 , pp. 62 - 76 .
Ikpe , E. and Torriti , J. ( 2018 ), “ A means to an industrialisation end? Demand side management in Nigeria ”, Energy Policy , Vol. 115 , pp. 207 - 215 .
Johanson , J. and Mattsson , L.G. ( 1988 ), “ Internationalisation in industrial systems-a network approach ”, in Hood , N. and Vahlne , J-E. (Eds), Strategies in Global Competition , Croom Helm , pp. 303 - 321 .
Juliana , M.I. and Okoye , N.E. ( 2016 ), “ Assessment of the contribution of value added tax to the Nigerian economy ”, International Journal of Social Sciences and Management Research , Vol. 2 No. 1 , pp. 45 - 56 .
Kaplan , A.M. and Haenlein , M. ( 2010 ), “ Users of the world, unite! the challenges and opportunities of social media ”, Business Horizons , Vol. 53 No. 1 , pp. 59 - 68 .
Kireru , C. ( 2019 ), “ Role of integrated talent management processes in competitive advantage of telecommunication firms in Nairobi city county, Kenya ”, Doctoral dissertation, JKUAT COHRED .
Landers , R.N. and Schmidt , G.B. ( 2016 ), “ Social media in employee selection and recruitment: an overview ”, in Landers , R.N. and Schmidt , G.B. (Eds), Social Media in Employee Selection and Recruitment: Theory, Practice, and Current Challenges , Springer , London , pp. 3 - 14 .
Leach , L.S. , Butterworth , P. , Poyser , C. , Batterham , P.J. and Farrer , L.M. ( 2017 ), “ Online recruitment: feasibility, cost, and representativeness in a study of postpartum women ”, Journal of Medical Internet Research , Vol. 19 No. 3 , p. e61 .
Lievens , F. and Slaughter , J.E. ( 2016 ), “ Employer image and employer branding: what we know and what we need to know ”, Annual Review of Organizational Psychology and Organizational Behavior Contents , Vol. 3 No. 1 .
Longe , O. ( 2017 ), “ Graduate unemployment in Nigeria: causes, consequences and remediable approaches ”, American International Journal of Contemporary Research , Vol. 7 No. 4 , pp. 63 - 73 .
Maheshwari , V. , Gunesh , P. , Lodorfos , G. and Konstantopoulou , A. ( 2017 ), “ Exploring HR practitioners’ perspective on employer branding and its role in organizational attractiveness and talent management ”, International Journal of Organizational Analysis , Vol. 25 No. 5 , pp. 1 - 24 .
Mahony , G. ( 2019 ), “ Time for a ceasefire in the war for talent ”, HR Future, 2019 , pp. 22 - 23 .
Marsh , R.R. and Oyelere , R.U. ( 2018 ), “ Global migration of talent: drain, gain, and transnational impacts ”, International Scholarships in Higher Education , Palgrave Macmillan , Cham , pp. 209 - 234 .
Marshall , M.N. ( 1996 ), “ The key informant technique ”, Family Practice , Vol. 13 No. 1 , pp. 92 - 97 .
Mindia , P.M. and Hoque , M.K. ( 2018 ), “ Effects of e-recruitment and internet on recruitment process: an empirical study on multinational companies of Bangladesh ”, International Journal of Scientific Research and Management ( Management) , Vol. 6 No. 1 , pp. 1 - 6 .
Moen , B.E. , Nyarubeli , I.P. , Tungu , A.M. , Ngowi , A.V. , Kumie , A. , Deressa , W. and Mamuya , S.H.D. ( 2020 ), “ The challenge of obtaining a decent work environment in Sub-Saharan Africa ”, Africa and the Sustainable Development Goals , Springer , Cham , pp. 155 - 164 .
National Bureau of Statistics report ( 2018 ), available at: www.nigerianstat.gov.ng/
NBS ( 2019 ), Q2 report , available at: https://nigerianstat.gov.ng/elibrary
Nekoei , A. and Weber , A. ( 2017 ), “ Does extending unemployment benefits improve job quality? ”, American Economic Review , Vol. 107 No. 2 , pp. 527 - 561 .
Nigeria Companies Directory (NCD) ( 2020 ), available at: www.6000profiles.com/States/Lagos%20State.htm
Oginni , B.O. and Ogunyomi , P.O. ( 2012 ), “ The roles of employment processes in organizational image in insurance organistions in Nigeria: employees and community perspectives ”, Australian Journal of Business and Management Research , Vol. 2 No. 5 , pp. 52 - 57 .
Okolie , U.C. and Irabor , I.E. ( 2017 ), “ E-recruitment: practices, opportunities and challenges ”, European Journal of Business and Management , Vol. 9 No. 11 , pp. 116 - 122 .
Omolawal , S.A. ( 2015 ), “ E- recruitment: practices, benefits and challenges ”, Journal of the Institute of Personnel Management of Nigeria , Vol. 7 No. 2 , pp. 76 - 86 .
Perampalam , S. Galpaya , H. and Senanayake , L. ( 2017 ), “ Understanding the factors influencing the take-off of online freelancing in Sri Lanka ”, Available at SSRN 3097564 .
Petre , A. , Osoian , C. and Zaharie , M. ( 2016 ), “ Applicants' perceptions on online recruitment ”, Managerial Challenges of the Contemporary Society. Proceedings , Vol. 9 No. 1 , p. 63 .
Randall , R. ( 2016 ), “ Aptitude test ”, Encyclopedia of Human Resource Management , Edward Elgar Publishing .
Rossman , G.B. and Wilson , B.L. ( 1985 ), “ Numbers and words: combining quantitative and qualitative methods in a single large-scale evaluation study ”, Evaluation Review , Vol. 9 No. 5 , pp. 627 - 643 .
Rousseau , D.M. ( 1995 ), Psychological Contracts in Organizations: Understanding Written and Unwritten Agreements , Sage , Thousand Oaks, CA .
Schwartz , D. ( 2018 ), “ Embedded in the crowd: creative freelancers, crowdsourced work, and occupational community ”, Work and Occupations , Vol. 45 No. 3 , pp. 247 - 282 .
Silva , P.C. and Crisp , B.F. ( 2020 ), “ The impact of cuing candidate quality on female candidates ”, Electoral Studies , Vol. 64 , pp. 102 - 127 .
Singh , A.S. and Masuku , M.B. ( 2013 ), “ Fundamental of applied research and sampling techniques ”, International Journal of Medical Research and Applied Science , Vol. 2 No. 4 , pp. 124 - 132 .
Son , M. , Lee , H. and Chang , H. ( 2019 ), “ Artificial intelligence-based business communication: application for recruitment and selection ”, Business Communication Research and Practice , Vol. 2 No. 2 , pp. 84 - 92 .
Tlaiss , H.A. , Martin , P. and Hofaidhllaoui , M. ( 2017 ), “ Talent retention: evidence from a multinational firm in France ”, Employee Relations , Vol. 39 No. 4 , pp. 426 - 455 .
Ujah-Ogbuagu , B.C. ( 2019 ), “ A competence-based recruitment system using mean deviation ”, IUP Journal of Information Technology , Vol. 15 No. 2 , pp. 1 - 5 .
World Population Review ( 2020 ), available at: https://worldpopulationreview.com/world-cities/lagospopulation .
Yamane , T. ( 1967 ), Statistics: An Introductory Analysis , 2nd ed. , Harper and Row , New York, NY .
Further reading
Small Medium Enterprises Development Agency of Nigeria ( 2007 ), “ Annual report ”, (SMEDAN), Nigeria , available at: www.smedan.gov.ng/images/SMEDANandNBS2013
Acknowledgements
The authors acknowledge everyone who have and will still contribute to the great success of this research work. Special thanks to Owolabi kayode, Esther Ezemba, Charles Orakwe and Dr Omolara Morounkeji Faboya for their support and contribution towards the research.
Funding details : The authors received no direct funding for this research work.
Declaration of interest statement : The authors declare no conflict of interest.
Corresponding author
Related articles, we’re listening — tell us what you think, something didn’t work….
Report bugs here
All feedback is valuable
Please share your general feedback
Join us on our journey
Platform update page.
Visit emeraldpublishing.com/platformupdate to discover the latest news and updates
Questions & More Information
Answers to the most commonly asked questions here
- Publisher Home
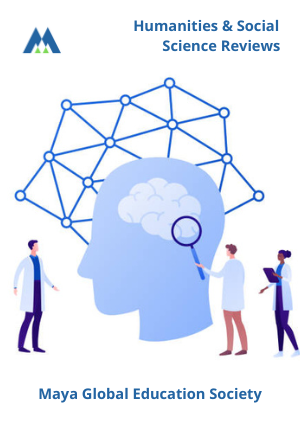
Vol. 7 No. 2 (2019): March
Authors retain the copyright without restrictions for their published content in this journal. HSSR is a SHERPA ROMEO Green Journal .
Publishing License

A SYSTEMATIC REVIEW OF LITERATURE ON RECRUITMENT AND SELECTION PROCESS
Corresponding author(s) : kanagavalli g..
Humanities & Social Sciences Reviews , Vol. 7 No. 2 (2019): March Article Published : March 5, 2019
- Authors Details
Purpose of the study: The main purpose of this study is to provide a new, macro-level model of strategic staffing to bridge the gap in the knowledge regarding how practices within recruitment and selection systems can work to provide a competitive advantage among various sectors. This study identifies the various methods of recruitment and selection process through a systematic review of literature, which would be the right fit for attracting and selecting employees in an organization.
Design/methodology/approach: Content analysis method is adopted to review the literature and subcategories were formed to analyze the research. Literature was collected from 40 articles of a reputed journal from 2010 to 2018.
Main findings: The review of literature revealed that the recruitment and selection process is carried out in organizations by adopting latest technologies like online portals, outsourcing, job fair, campus interviews, and mobile recruitment applications. The representation of this practice is to find the best candidate for an organization. Besides adopting the latest technology, consideration of the expatriate factor would lead to an effective way of recruitment practices in finding out the right candidate for the right job and thus create a healthier work environment. The expatriate factors have not been considered well in the Indian context, but have been given importance in the global context in the process of recruitment and selection.
Social Implications: Highlighting the significance of various recruitment practices results in the selection of the right person in the right job, which enhances a healthier working environment in organizations, in turn rendering high quality products and services to the society.
Originality of the study: Prior research has studied various factors that influence internal recruitment, external recruitment, and selection process. This study is an attempt to analyze the expatriate factors and other factors through the content analysis method.
Full Article
Download citation.
Anna B, Holm. (2012). E-recruitment: Towards an Ubiquitous Recruitment Process and Candidate Relationship Management. Electronic Human Resource Management: Transformation of HRM, Vol.26, Issues: 3, pp. 241-259. DOI: https://doi.org/10.1177/239700221202600303
Annette scherpenzeel., and Vera Toepoel. (2012). Recruiting a probability sample for an online panel: Effects of contact mode, incentives, and information, The Public Opinion Quarterly, Vol. 76, No. 3 ,pp. 470-490 DOI: https://doi.org/10.1093/poq/nfs037
Chungyalpa., & Wand Karishma,T. (2016), Best Practices and Emerging Trends in Recruitment and Selection. Journal of Entrepreneurship and organization Management, Vol . 5. Issue: 2
Cornelius, J. König, Eva Jöri., and PatriziaKnüsel. (2011), The Amazing Diversity of Thought: A Qualitative Study on How Human Resource Practitioners Perceive Selection Procedures. Journal of Business and Psychology, Vol. 26, No. 4. pp. 437-452 DOI: https://doi.org/10.1007/s10869-010-9199-9
Dennis Campbell..(2012) .Employee Selection as a Control System. Journal of Accounting Research, Vol. 50, No. 4. pp. 931-966 DOI: https://doi.org/10.1111/j.1475-679X.2012.00457.x
Don Boyd., Hump Lankford., Susanna Loeb., Matthew Ronfeldt., and JimWyckoff. (2010). The Role of Teacher Quality in Retention and Hiring: Using Applications to Transfer to Uncover Preferences of Teachers and Schools. Journal of Policy Analysis and Management, Vol. 30, No. 1. pp. 88-110 DOI: https://doi.org/10.1002/pam.20545
Dr. DilipAher., and Dr. Ghanshyam D, Giri. (2018). A study of Recruitment and Selection process with Special reference to manufacturing industries in Pimpri- chinchwadmidc. Elk Asia pacific journal of human resource management and organizational behavior, Volume 4, Issue 1
Eunmi Mun. (2010). Sex Typing of Jobs in Hiring: Evidence from Japan. Oxford University Press, Vol. 88, Issues: 5, pp. 1999-2026 DOI: https://doi.org/10.1353/sof.2010.0046
Helen D, Arnold, (2013). The Affordable Care Act and International Recruitment and Migration of Nursing Professionals .Indiana Journal of Global Legal Studies, Vol. 20, No. 2 .pp. 1373-1391 DOI: https://doi.org/10.2979/indjglolegstu.20.2.1373
Jasjit Singh., and Ajay Agrawal. (2011). Recruiting for Ideas: How Firms Exploit the Prior Inventions of New Hires. Management Science, Vol. 57, No. 1, pp. 129-150 DOI: https://doi.org/10.1287/mnsc.1100.1253
Jed DeVaro., and Hodaka Morita. (2013).Internal Promotion and External Recruitment: a Theoretical and Empirical Analysis. Journal of Labor Economics, Vol. 31, No. 2. pp. 227-269 DOI: https://doi.org/10.1086/667814
Katherine Calogero. (2011). Become A fan of Government Procurement on Facebook: How The Federal government's acquisition workforce can use social networking websites to recruit New Employees. Public Contract Law Journal, Vol. 40, No. 3. pp. 807-828
Lauren a, River. (2012), Diversity within Reach: Recruitment versus Hiring in Elite Firms. The Annals of the American Academy of Political and Social Science, Vol. 639, pp. 71-90 DOI: https://doi.org/10.1177/0002716211421112
Leonard Bright., and Cole Blease Graham ,Jr.(2015).Why Does Interest in Government Careers Decline Among Public Affairs Graduate Students? .Journal of Public Affairs Education, Vol. 21, No. 4. pp. 575-594 DOI: https://doi.org/10.1080/15236803.2015.12002221
Lieselotte Blommaert., Marcel Coenders., and Frank van Tubergen. (2014), Discrimination of Arabic-Named Applicants in the Netherlands: An Internet-Based Field Experiment Examining Different Phases in Online Recruitment Procedures. Social Forces, Vol. 92, No. 3 ,pp. 957-982 DOI: https://doi.org/10.1093/sf/sot124
Linda Colley.(2011).The Passing of Youth: How Removal of Traditional Youth Recruitment Policies Contributed to the Ageing of Public Service Workforces. Labour History, No. 101.pp. 177-193 DOI: https://doi.org/10.5263/labourhistory.101.0177
MarÃa Fernanda GarcÃa, Richard, A. Posthuma and Manuel Quiñones. (2010). How Benefit Information and Demographics Influence Employee Recruiting in Mexico. Journal of Business and Psychology, Vol. 25, No. 3 ,pp. 523-531 DOI: https://doi.org/10.1007/s10869-010-9175-4
Matthew Bidwell. (2011). Paying More to Get Less: The Effects of External Hiring versus Internal Mobility. Administrative Science Quarterly, Vol. 56, No. 3. pp. 369-407 DOI: https://doi.org/10.1177/0001839211433562
Melody E, Valdini., and Christopher Shortell. (2016).Women's Representation in the Highest Court: A Comparative Analysis of the Appointment of Female Justices. Political Research Quarterly, Vol. 69, No. 4. pp. 865-876 DOI: https://doi.org/10.1177/1065912916668411
Mir Mohammed Nurul Absar.(2012). Recruitment & Selection Practices in Manufacturing Firms in Bangladesh. Indian Journal of Industrial Relations, Vol. 47, No. 3 .pp. 436-449
Paul T, Knudson. (2012). Regional Industrial Recruitment in Upstate New York. State & Local Government Review, Vol. 44, No. 1.pp. 21-32 DOI: https://doi.org/10.1177/0160323X11420885
Samita Sen. (2010). Commercial recruiting and Informal Intermediation: debate over the sardari system in Assam tea plantations, 1860–1900.Modern Asian Studies, Vol. 44, No. 1, pp. 3-28 DOI: https://doi.org/10.1017/S0026749X09990047
Shamima Ahmed and Allison Adams. (June 2010). Web Recruiting in Government Organizations: A Case Study of the Northern Kentucky/Greater Cincinnati Metropolitan Region. Public Performance & Management Review, Vol. 33, No. 4. pp. 653-670 DOI: https://doi.org/10.2753/PMR1530-9576330406
Shereen Hussein., Jill Manthorpe., and Martin Stevens. (2010). People in Places: A Qualitative Exploration of Recruitment Agencies' Perspectives on the Employment of International Social Workers in the UK. The British Journal of Social Work, Vol. 40, No. 3, pp. 1000-1016 DOI: https://doi.org/10.1093/bjsw/bcn131
Silja K, Bruland., Grete Rusten., and Silja Kristiansen Bruland. (2012). Sourcing strategies, channels and geographies in the International Recruitment of a highly skilled work-force. Erdkunde, Bd. 66, H. 1 .pp. 1-11 DOI: https://doi.org/10.3112/erdkunde.2012.01.01
Subhash C, Kundu., and Neha Gahlawat. (2015). Recruitment and Selection Techniques used in Corporate Sector: A Comparative Study of Indian and Multinational Companies. Journal of Organization & Human Behavior, Volume 4, Issue 4 DOI: https://doi.org/10.21863/johb/2015.4.4.022
Sylvie Monchatre., and Peter Hamilton.(2014). Coming to Terms with Diversity: Recruitment between Market Forces and Employee Mobilization. Revue française de sociologie (English Edition), Vol. 55, No. 1.pp. 33-60
Thomas Hegghammer.(2013).The recruiter's dilemma: Signaling and rebel recruitment tactics. Journal of Peace Research, Vol. 50, No. 1.pp. 3-16 DOI: https://doi.org/10.1177/0022343312452287
Tracy M, Kantrowitz., Craig R, Dawson., and Michael S. Fetzer.(2011). Computer Adaptive Testing (CAT): A Faster, Smarter, and More Secure Approach to Pre-Employment Testing .Journal of Business and Psychology, Vol. 26, No. 2. pp. 227-232 DOI: https://doi.org/10.1007/s10869-011-9228-3
VesaPeltokorpi., and EeroVaara.(2014). Knowledge transfer in multinational corporations: Productive and counterproductive effects of language-sensitive recruitment. Journal of International Business Studies, Vol. 45, No. 5. Special Issue: TheMultifaceted Role of Language in International Business: Unpacking the Forms, Functionsand Features of a Critical Challenge to MNC Theory and Performance,pp. 600-622 DOI: https://doi.org/10.1057/jibs.2014.1
Vipin Nadda., and Pankaj Tyagi. (2017). Effectiveness and Challenges of Recruitment process outsourcing (RPO) in the Indian Hotel Sector. Tourism and Hospitality Management, Volume 12, pp.223 - 234
Vladimir Hlasny. (2011).Discriminatory Practices at South Korean Firms Quantitative Analysis Based on Job Application Forms .European Journal of East Asian Studies, Vol. 10, No. 1 .pp. 85-113 DOI: https://doi.org/10.1163/156805811X592522
Yas A, Alsultanny., Mona F, Alotaibi.(2015). Evaluating the Factors Affecting on Intension to Use of E-Recruitment.. American Journal of Information Science and Computer Engineering Vol. 1, No. 5, 2015, pp. 324-331. http://www.aiscience.org/journal/ajisce
Yashar Fadhil Mohammed Harky. (2018). The Significance of Recruitment and Selection on Organizational Performance: The Case of Private owned Organizations in Erbil, North of Iraq. International Journal of Contemporary Research and Review, Volume 09,Issue 02
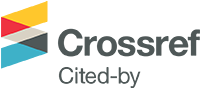
Table Of Contents
literature Review: Artificial Intelligence Impact on the Recruitment ProcessA LITERATURE REVIEW: ARTIFICIAL INTELLIGENCE IMPACT ON THE RECRUITMENT PROCESS
This paper aim is to review the implementation of artificial intelligence (AI) in the Human Resources Management (HRM) recruitment processes. A systematic review was adopted in which academic papers, magazine articles as well as high rated websites with related fields were checked. The findings of this study should contribute to the general understanding of the impact of AI on the HRM recruitment process. It was impossible to track and cover all topics related to the subject. However, the research methodology used seems to be reasonable and acceptable as it covers a good number of articles which are related to the core subject area. The results and findings were almost clear that using AI is advantages in the area of recruitment as technology can serve best in this area. Moreover, time, efforts, and boring daily tasks are transformed to be computerized which makes a good space for humans to focus on more important subjects related to boosting performance and development. Acquiring automation and cognitive insights as well as cognitive engagement in the recruitment process would make it possible for systems to work similarly to the human brain in terms of data analysis and the ability to build an effective systematic engagement to process the data in an unbiased, efficient and fast way.
- Related Documents
INFORMATION AND COGNITIVE TECHNOLOGY FOR STUDYING THE IMPACT OF INVESTMENTS ON THE RESULTS OF AGRICULTURAL ACTIVITIES (COGNITIVE STRUCTURING OF THE SUBJECT AREA)
Speculation in the cryptocurrency market.
The article analyses the impact of speculation on the cryptocurrency market. In the introduction, the author interprets the meaning of the speculation and a speculator’s way of thinking. The literature review was broken down into two aspects. The first is to analyse the literature on the subject in terms of the cryptocurrency market. The second is an analysis of the sentiment index, which becomes the primary research method. The analysis of the sentiment value shows a high correlation between the impact of sentiment on the value of cryptocurrencies. Further recommendations are to analyse the sentiment with regard to listed companies (Polish and international) and stock indices (including the forex market).
Leadership style and employee readiness: Basic factors of leadership efficiency
The scope of this study is based on the Situational Leadership Theory according to the model created by Hersey and Blanchard, predominantly defined by two research sub-fields: leadership style and readiness of employees. This model distinguishes four different leadership styles, while the subject area of readiness of employees comprises two components: readiness of employees based on the competency component and readiness of employees based on a psychological (motivational) component. The subject matter of the study is to identify leadership style as a new variable which will be utilized for determining the interdependence with leadership efficiency levels and readiness of employees. Leadership efficiency is determined by the degree of compatibility between the dominant style of leadership and the level of readiness of employees. In addition to the main objective of the research, aimed at determining leadership efficiency, significant research findings come as a result of specific objectives. That is impact of the dominant leadership style on readiness of employees and leadership results, based on each specific component. The sample will be a random sample from a finite population of units, with repeated sampling and known probability sampling. A sample of size n=100, with a proportion of 0.10, is based on a list of top 100 biggest companies in the Republic of Serbia in 2019. The application of Principal Component Analysis aims to identify new variables in the form of regression coefficient results, while the application of the hierarchical regression model will help determine the impact of each leadership style on readiness of employees. The study findings confirm the basic hypotheses of the aforementioned model, with regard to the results of leadership efficiency, as well as its impact of each leadership style on readiness of employees.
Don’t write, just mark: the validity of assessing student ability via their computerized peer-marking of an essay rather than their creation of an essay
This paper reports on a case study that evaluates the validity of assessing students via a computerized peer-marking process, rather than on their production of an essay in a particular subject area. The study assesses the higher-order skills shown by a student in marking and providing consistent feedback on an essay. In order to evaluate the suitability of this method of assessment in judging a student’s ability, their results in performing this peer-marking process are correlated against their results in a number of computerized multiple-choice exercises and also the production of an essay in a cognate area of the subject being undertaken. The results overall show a correlation of the expected results in all three areas of assessment being undertaken, rated by the final grades of the students undertaking the assessment. The results produced by quantifying the quality of the marking and commenting of the students is found to map well to the overall expectations of the results produced for the cohort of students. It is also shown that the higher performing students achieve a greater improvement in their overall marks by performing the marking process than those students of a lower quality. This appears to support previous claims that awarding a ‘mark for marking’ rewards the demonstration of higher order skills of assessment. Finally, note is made of the impact that such an assessment method can have upon eradicating the possibility of plagiarism.DOI: 10.1080/0968776042000259573
Personal development and self-actualization of different employee generations-important key to the organizations success
The presented paper presents the results of the project VEGA No. 1/0721/20 "Identification of priorities for sustainable human resources management for disadvantaged employees in the context of Industry 4.0". It focuses on the importance of personal development and self-realization for employees in industrial enterprises in the Slovak Republic about generation groups. The aim was to identify the preferences of employees in the subject area and their fulfillment by employers. The following research methods were used to process the paper: a comparative analysis of literary sources, questionnaire survey, statistical evaluation of results using IBM SPSS 22.0 (Statistical Package for the Social Sciences), and interpretation of research results in industrial enterprises in Slovakia.
Analysing the impact of artificial intelligence on the competitiveness of tourism firms: a modified total interpretive structural modeling (m-TISM) approach
PurposeThis study explores the variables that drive the impact of artificial intelligence (AI) on the competitiveness of a tourism firm. The relationship between the variables is established using the modified total interpretive structural modelling (m-TISM) methodology. The factors are identified through literature review and expert opinion. This study investigates the hierarchical relationship between these variables.Design/methodology/approachThe modified total interpretive structural modelling (m-TISM) method is used to develop a hierarchical interrelationship among variables that display direct and indirect impact. The competitiveness of a tourism firm is measured by investigating the effect of variables on the firm's financial performance.FindingsThe study identifies ten key factors essential for analysing the impact of AI on a firm's competitiveness. The m-TISM methodology gave us the hierarchical relationship between the factors and their interpretation. A theoretical TISM model has been constructed based on the hierarchy and relationship of the elements. The elements that fall in Level V are “AI Skilled Workforce”, “Infrastructure” and “Policies and Regulations”. Level IV includes the elements “AI Readiness”, “AI-Enabled Technologies” and “Digital Platforms”. Elements that fall under Level III are “Productivity” and “AI Innovation”. Level II and Level I comprise “Tourist Satisfaction” and “Financial Performance”, respectively. The levels indicate the elements' hierarchical level, with Level I the highest and Level V the lowest.Research limitations/implicationsTourism and AI scholars can analyse the given variables by including the transitive links and incorporate new variables depending upon future research. The m-TISM model constructed from literature review and expert opinion can act as a theoretical base for future studies to be conducted by researchers.Practical implicationsManagement/Practitioners can focus on the available characteristics and capitalise on them while working on the factors lacking in their organisation to enhance their competitiveness. Entrepreneurs starting their own business can utilise the elements in understanding the ecosystem of strengthening a firm's competitiveness. They can work to improve on the aspects which are crucial and trigger the impact on competitiveness. The government and management can devise policies and strategies that encompass the essential factors that positively impact the competitiveness of the firms. The approach can then be looked at with a holistic approach to cater to the other related components of the tourism industry.Originality/valueThis study is the first of its kind to use the modified TISM methodology to understand the impact of AI on the competitiveness of tourism firms.
A Conceptual Model of Manufacturing Focus
Despite the importance given to manufacturing focus in the literature, the subject area continues to suffer from three interrelated problems: lack of agreement with regard to the meaning of “focus” the absence of a conceptual framework for integrating the existing body of research; and uncertainty with regard to the appropriate direction of future research. These problems should be addressed if the focus literature is to continue to mature, and if the true role of focus is to be understood in the light of the “newer” strategic imperatives, such as time‐based competition and flexible manufacturing. Introduces a conceptual model of focus specifically designed in response to these problems. The conceptual model identifies three distinct dimensions of focus, and relates these to the competitive factors facing manufacturing organizations. It is designed specifically to serve as a tool with which researchers and managers can discuss the impact of focus at the firm level. A review of key works in manufacturing focus is also included to justify the structure of the model, and to show how the model integrates previous conceptual and empirical research on focus.
AngloGold Ashanti: the dawning of a new age
Title AngloGold Ashanti: the dawning of a new age. Subject area Change management, leadership, human resources management and organisational behaviour. Study level/applicability Business and organisational behaviour students at a Master's level of study. Case overview This case study explores the challenges facing one of the jewels of corporate South Africa, AngloGold Ashanti, as it seeks to introduce and implement vast changes across its global operations within a pre-established time frame. It explores those factors that impact the introduction and implementation of a successful and sustainable change initiative at AngloGold Ashanti. Expected learning outcomes By understanding the manner in which the company's new business improvement initiative is sought to be introduced at the organisation, students are better able to understand the role of leadership and the impact of change on a global workforce. Students will gain an appreciation of how to manage the change process, key actions that ought to be taken by all levels of management and staff, pitfalls that should be avoided and challenges that need to be addressed to ensure that the organisation can emerge stronger and take its rightful place in the competitive global arena. Supplementary materials Teaching notes are available; please consult your librarian for access.
Artificial Intelligence and the Development of Smart Tourism
The main intention of this manuscript is to fill this gap by evaluating the impacts of artificial intelligence on the development of smart tourism. The study will start with discussing previous literature on smart tourism and contributions of artificial intelligence on the development of tourism based on earlier academic findings and practical implications. For methodology, the paper will follow literature review as a guideline and the findings will be critically evaluated. Besides that, the study will suggest a model whereby the impact of artificial intelligence on smart tourism will be quantitatively analyzed. In the suggested model, related economic, technological, social and environmental variables including total revenue created by smart tourism using artificial intelligence, contributions of technological improvement, the degree of social inclusion to tourism activities for disadvantaged individuals (such as elderly, people with disabilities, pregnant women etc.) with respect to the artificial intelligence applications, and the degree of environmental degradation parallel to the shift to smart tourism.
Development of human capital in the context of improving processes in the enterprise
Human is the key resource of the organization, and proper human resources management, manifested primarily by matching knowledge, skills and attitudes of employees to the strategic goals of the company should lead to their transformation into human capital and the synergy effect of combining the qualities and strengths of employees intensifying the frequency of the innovative concepts. The main purpose of the article is to present the results of research conducted on a sample of 70 enterprises regarding the analysis of the extent of enterprises’ use of the potential accumulated within human capital to improve the implemented processes, including quality management. The research covered issues related to the impact of the functioning of the human resources management system on the company efficiency, the main factors supporting and hindering the functioning of this system, as well as problems and difficulties occurring both at the stage of implementing the system and its subsequent operation. The basic research tool was a questionnaire consisting of 23 questions of a closed nature supported by interviews conducted with representatives of the management. The article also includes a literature review on selected aspects of shaping and managing human capital and increasing the professional potential of employees as a result of systemic management of their competences.
Export Citation Format
Share document.

An official website of the United States government
The .gov means it’s official. Federal government websites often end in .gov or .mil. Before sharing sensitive information, make sure you’re on a federal government site.
The site is secure. The https:// ensures that you are connecting to the official website and that any information you provide is encrypted and transmitted securely.
- Publications
- Account settings
Preview improvements coming to the PMC website in October 2024. Learn More or Try it out now .
- Advanced Search
- Journal List
- Elsevier - PMC COVID-19 Collection

Employees recruitment: A prescriptive analytics approach via machine learning and mathematical programming
Dana pessach.
a Department of Industrial Engineering, Tel-Aviv University, Israel
Gonen Singer
b Faculty of Engineering, Bar-Ilan University, Israel
Dan Avrahami
Hila chalutz ben-gal.
c Department of Industrial Engineering and Management, Afeka College of Engineering, Israel
Erez Shmueli
Irad ben-gal.
In this paper, we propose a comprehensive analytics framework that can serve as a decision support tool for HR recruiters in real-world settings in order to improve hiring and placement decisions. The proposed framework follows two main phases: a local prediction scheme for recruitments' success at the level of a single job placement, and a mathematical model that provides a global recruitment optimization scheme for the organization, taking into account multilevel considerations. In the first phase, a key property of the proposed prediction approach is the interpretability of the machine learning (ML) model, which in this case is obtained by applying the Variable-Order Bayesian Network (VOBN) model to the recruitment data. Specifically, we used a uniquely large dataset that contains recruitment records of hundreds of thousands of employees over a decade and represents a wide range of heterogeneous populations. Our analysis shows that the VOBN model can provide both high accuracy and interpretability insights to HR professionals. Moreover, we show that using the interpretable VOBN can lead to unexpected and sometimes counter-intuitive insights that might otherwise be overlooked by recruiters who rely on conventional methods.
We demonstrate that it is feasible to predict the successful placement of a candidate in a specific position at a pre-hire stage and utilize predictions to devise a global optimization model. Our results show that in comparison to actual recruitment decisions, the devised framework is capable of providing a balanced recruitment plan while improving both diversity and recruitment success rates, despite the inherent trade-off between the two.
- • A model for pre-hire prediction of employees' recruitment success is proposed.
- • A hybrid framework using ML and a global mathematical optimization is proposed.
- • The model is evaluated using a uniquely large and heterogeneous real-world dataset.
- • The model obtains high accuracy and interpretability as a practical tool for HR.
- • Results show high recruitment success even when diversity is enhanced by the model.
1. Introduction
One of the most challenging and strategic organizational processes is to efficiently hire suitable workforce. A comprehensive study by the Boston Consulting Group has shown that the recruitment function has the most significant impact on companies' revenue growth and profit margins compared to any other function in the field of human resources (HR) [ 1 ]. Indeed, poor recruitment decisions may lead not only to low-performing employees but also to increased turnover. Turnover may have a direct impact stemming from employee replacement costs (e.g., interviews and rehiring costs, training and productivity loss, overtime of other employees), as well as indirect effects, such as poor service to clients or a decline in employee morale [ 2 ]. Thus, improving organizational recruitment processes by hiring the most suitable candidates has a significant impact on organizational performance [ 3 , 4 ].
In this study, we propose a data analytics approach, which can be used as a decision support tool for recruiters in real-world settings to improve hiring decisions of candidates to specific positions or jobs. The proposed approach comprises two components: a local prediction model for recruitment success per candidate and job type, and a global optimization model of the recruitment process.
The first part of this study is based on interpretability ML modeling, which provides meaningful insights into the potential recruitments related to the candidate's background features as well as the planned job placement. The output of these models is the probabilities of successful recruitment per employee and job. The second part in this research is based on a mathematical modeling formulation at an organizational level that takes into account multi-objective considerations and optimize the recruitment process over many candidates and jobs by using the success probability outputs of the ML models.
Previous efforts have been invested in trying to predict recruiters' decisions (e.g., [ 5 , 6 ]). Such prediction models, if accurate enough, may eventually replace the human recruiter and save a considerable amount of resources. Note, however, that recruiters' decisions are inherently subjective, and human intuition plays an important part in recruitments and placements. Hence, using interpretability modeling tools that can enrich and guide recruiters' decisions by insight seems to be a relevant approach, which recently gained popularity and is also known as explainable artificial intelligence (XAI) (see, for example, [ 7 ]). Another line of work has focused on the post-hire prediction of turnover or performance (e.g., [ 8 ]). While such measures are somewhat more objective, post-hire prediction efforts might be too late in certain cases to act upon. Therefore, in this paper, we focus on the pre-hire prediction of performance and turnover as a combined objective measure.
A key property of our approach is the interpretability of predictions, providing a useful explanation of how they are obtained. Apart from the accuracy of the prediction model, users' trust in the model is often directly impacted by how much they can understand and anticipate its behavior [ 9 ]. Understanding why the model behaves the way it does may increase users' trust and their potential to act upon its recommendations. This is especially true in decisions that involve human beings' intuition, such as in the case of employees' recruitment and job placement.
To address the prediction task described above, we propose applying the interpretable Variable-Order Bayesian Network (VOBN) model [ 10 , 11 ]. In contrast to other interpretable models such as decision trees, which often suffer from high variance and overfit to the training set, the VOBN model provides an inherent modeling flexibility that reduces such effects. Therefore, it often results in an improved generalization and predictive ability over various test sets. Finally, we show that the VOBN model is also flexible enough for mining significant patterns and insights in HR data.
Nevertheless, recruitment requires not only hiring the highest-potential workforce, but also meeting other organizational objectives. For example, there is a necessity to meet the demand for employees in different departments, the facilitation of diversity in teams and the allocation of the workforce among different departments in a balanced manner. Each of these dimensions may also include numerous points of view: the local point of view of each separate candidate-position pair, the positional point of view and the organizational or regulatory point of view. Given that there are requirements of various stakeholders in the organization, there is a need to balance the trade-offs in this multi-objective scenario. Hence, in the second part of this research, we address the recruitment problem with a global perspective by accounting for the various dimensions and points of view.
We evaluate the proposed method using a unique dataset obtained from a large nonprofit service organization that is highly diversified over roles, accountabilities and job descriptions, with heterogeneous population of employees with diverse backgrounds, geographic locations and levels of socioeconomic status.
The dataset includes a rich feature set of hundreds of thousands of employment cases collected over a decade and represents a wide range of heterogeneous populations. These characteristics enable us to test potentially biased recruitment policies and placement decisions that traditionally may not be tested due to the absence of sufficient data on such large groups in the population.
The results of our evaluation reveal that the proposed prediction approach can perform well in terms of both accuracy and interpretability, despite the inherent trade-off that often exists between the two [ 9 , 12 ]. In addition, we demonstrate how our interpretable approach can be used to extract meaningful insights that may support and benefit the recruiters' decision process. These extracted insights are sometimes counter-intuitive and shed light on the limitations of existing approaches and on the recruiters' intuition, which is limited and biased at times.
Moreover, we demonstrate that it is feasible to predict a successful placement of a candidate to a specific position at a pre-hire stage with a relatively high prediction performance (AUC = 0.73) and then utilize these predictions to devise a global optimization model. Our results show that using the proposed mathematical programming model, we are able to increase diversity (by 40%) while maintaining a high level of recruitment success (decreased by only 1%). Moreover, the results show an improvement of both diversity and recruitment success rates compared to recruiters' actual selections, although these objectives are generally found to be in conflict. The proposed approach can provide recruiters and organizations alike, with an applicable decision support tool for hiring successful candidates while improving organizational recruitment and placement processes and procedures.
This paper is structured as follows. Section 2 reviews the relevant literature. Section 3 describes the proposed analytics framework and the experimental settings. Section 4 describes the results, and finally, Section 5 summarizes and provides some concluding remarks.
2. Background and literature review
We organize the relevant literature review as follows. We first survey the related studies that address predictive analytics in HR and classify them along three core dimensions: functional, data and method. We then review the related topics from the HR literature.
2.1. Functional dimension
In recent years, several preliminary studies have focused on predicting recruiters' decisions [ 5 , 6 , [13] , [14] , [15] ]. However, imitating the recruiter's decision may not necessarily be the best approach, since they are often affected by highly subjective and potentially inaccurate judgments that preserve, rather than improve, hiring biases. Consequently, there is a need for an objective measure of the actual success of employee recruitment and performance, as well as providing meaningful insights to the recruiters themselves.
Other recent studies have focused on objective measures of successful recruitment based on employee past performance. Some of these studies examined the post-hire prediction of turnover or performance with predictors collected over the employment period [ 8 , [16] , [17] , [18] , [19] , [20] , [21] , [22] , [23] ]. Note that the prediction of turnover or performance using post-hire data (such as absenteeism, punctuality and performance reviews) may be useful as part of some retention activities but may lead to a late discovery of recruitment errors and may often be too late to act upon [ 8 , 24 ].
In contrast, the potential benefit of the early pre-hire foresight of longer-term employee success may be much higher, saving more financial and social costs. Few studies have addressed the pre-hire prediction of recruitment success using performance assessments [ [25] , [26] , [27] ] separately from turnover assessments [ 25 , 28 ].
Measuring performance may incorporate one aspect of the success of an employee; however, high-performers will not necessarily remain in the organization. Moreover, turnover alone may only partially indicate recruitment success — as often happens in practice, low-performers may not leave the organization due to organizational policies to minimize layoffs and promote high internal mobility.
No previous study has referenced the combination of turnover and performance into one measure that represents an objective measure of recruitment success (see Fig. 1 for a taxonomy of the functional dimension). Thus, in this study, we focus on the case of pre-hire predictions of recruitment success using a combined measure. In the rest of this review, we focus mostly on the case of pre-hire predictions of recruitment success. Note that our methodology approaches hiring from the point of view of recruiters, as opposed to other methodologies that examine the perspective of candidates (for example, how they browse or select relevant job positions [ [29] , [30] , [31] ]).

Literature review based on the functional dimension.
2.2. Data dimension
One of the challenges of using machine learning (ML) techniques in HR is the deficiency of empirical data. A noticeable number of studies have examined rather small datasets, in terms of both the number of candidates, as well as the number of features (e.g. [ 8 , 15 , 23 , 26 , 32 ]).
Within the line of studies that have addressed pre-hire prediction, studies traditionally included a rather narrow set of samples (such as [ [25] , [26] , [27] ]). However, in most cases, a small dataset fails to adequately portray the characteristics of the population, yielding the challenge to adequately train a reliable model based on such a small dataset. Narrow datasets often result in low support values of subpopulations, meaning that very few samples are associated with each predicted (or rule-based) subpopulation, resulting in low statistical significance. This challenge is even more noticeable with the growth in the number of features.
Some studies have also involved a limited set of features. For example, Li et al. [ 26 ] and Bach et al. [ 27 ] use only psychological assessments of personality and cognitive abilities, whereas Mehta et al. [ 28 ] use resume data only. Chien and Chen [ 25 ] use only a few features, such as age, gender, marital status, educational background, work experience, and recruitment channels. Mehta et al. [ 28 ] conclude that features that capture candidate attributes, such as leadership, may contribute significantly to the analysis and that different models should be evaluated for different jobs. They indicate that a broader set of features and samples may enhance both prediction results and root cause analysis.
Lack of sufficient empirical data is reflected not only in the absolute amount of data (features, candidates) but also in the available data on populations that are usually not recruited and often are not even interviewed. It is evident that to extract significant insights using the potential of machine learning techniques on HR data, data should include a range of differing applicants [ 33 ]. Hence, data collected from a large organization that promotes a wide social diversity policy and hires a wide range of heterogeneous populations would be beneficial in showing new understandings and counter-intuitive results.
In contrast to many of the abovementioned papers, in our study, we use a large dataset with hundreds of thousands of employees from a wide range of heterogeneous populations, containing >100 features. This unique dataset allows us to extract relatively deeper rules and insights based on a wider feature set and with high significance predictions of successful or unsuccessful recruitments.
2.3. Method dimension
Preliminary studies in HR analytics often used conventional statistical tools such as descriptive statistics, hypothesis testing, analysis of variance, regression and correlation analysis [ 27 , [34] , [35] , [36] , [37] ]. Bollinger et al. [ 37 ] used a t-test to determine the factors that affect recruiters' decisions and integrated them into their aggregated score. Then, this single-score measure was used as a correlated measure to recruiters' surveyed opinions. Samuel and Chipunza [ 35 ] used the Chi-square test to identify which post-hire employment factors impact organizational turnover. Bach et al. [ 27 ] used multiple regression analysis to test which personality traits and cognitive ability features have an impact on employee performance. However, their regression models obtain a low fit ( R 2 = 0.054, R 2 = 0.088).
More recent studies have started to use machine learning techniques for HR analytics. Some of them have implemented models that provide interpretable insights (e.g., [ 19 , 21 , 25 , 38 ]) and others have implemented non-interpretable models that provide solely the predictions or their ranked scores (e.g., [ 8 , 16 , 28 , 39 ]; further literature is detailed in recent surveys, e.g., [ 18 , 33 ]). In the rest of this section, we mainly focus on papers that addressed the pre-hire prediction of recruitment success using ML tools.
Chien and Chen [ 25 ] used the CHAID decision tree to extract rules for three different problems with separate classification targets: employee performance levels, turnover in the first three months of employment, and turnover in the first year of employment. They extracted several rules based on the demographic data of a rather moderately sized dataset of 3825 applicants, using all data as the training set (without using validation or test set, which can lead to overfitting). They suggested implementing some strategies based on the one-time findings from the obtained decision trees, such as recruiting from first-tier universities. However, they indicate that the HR staff found the extracted rules to be difficult to implement. The researches suggest performing an in-depth analysis to further clarify the root causes of turnover and implementing processes to effectively improve orgranizational retention rate. The small dataset used in their research could be the reason for the limitations of the extracted rules.
Li et al. [ 26 ] used a support vector machine (SVM) model to predict the performance of seven test candidates using a training set of 32 employees and focused on their personality test features. Mehta et al. [ 28 ] showed the results of a random forest classifier on a dataset containing resumes of candidates. However, they did not use an interpretable model to provide recruitment insights for the organization.
It should be noted that the suggested modeling approach in this study is intended to be used by HR professionals in order to facilitate improved interaction with candidates. Thus, there is significant importance to the provision of an interpretable model that can be well comprehended by HR professionals. The model evaluation should consider the interpretability as well as the accuracy of the model [ 9 , 12 ].
Another challenge that the proposed approach must take into account is complexity. In the recruitment-success classification problem under consideration, the complexity arises from a large set of features in the HR dataset (with >150 features). Each feature has several or more possible values, resulting in a large combinatorial space of potential feature interactions. Specifically, the dataset includes many categorical features, such as education certificates, test results, background details and potential assigned positions. In fact, extracting rules (i.e., patterns of feature values), even with a small number of features, may result in an extremely large space of potential combinations [ 10 ].
This study investigates several interpretable machine learning algorithms for predicting recruitment and placement success. The proposed method, which has not been used before for this objective, performs well in terms of both interpretability and accuracy, despite the inherent trade-off between the two [ 9 , 12 ]. The results of this research are expected to provide recruiters and organizations alike, with a useful modeling approach that generates insights for supporting recruitment and placement plans.
Moreover, the above reviewed studies provide local prediction scores, rankings or rules but do not provide a global prescriptive method that takes into account the position or the organizational point of view as a whole. To conclude, a prescriptive solution, rather than only a predictive methodology, is required for implementation in an actual organizational environment.
2.4. HR practices and HR analytics
Employees are considered one of the most important assets for modern organizations; hence, many efforts are invested in improving their success in the workplace. This has led to the rise of fields such as human resources (HR) analytics (which includes other related topics, such as “workforce analytics”, “people analytics”, and “human capital analytics” [ 40 ]). A recent review [ 40 ] maps the different tasks of HR practices to HR analytics tools and discusses how these tools can influence the organizational return on investment (ROI). The review shows that HR predictive analytics in workforce planning and recruitment have the highest effect on organizational ROI (similar conclusions are shown in a report by the Boston Consulting Group in [ 1 ]). Interestingly, as opposed to recruitment and workforce planning, other HR tasks, such as “industry analysis”, “job analysis” and “performance management”, have low expected ROI. Tasks such as “training”, “compensation” and “retention” have medium expected ROI [ 40 ].
These findings correspond with our approach of a pre-hire in-advance design of the recruitment plan, which is expected to have more impact than a post-hoc approach. Post-hire information includes information such as: employee engagement, organizational commitment, organizational support and HR practices applied for retention [ [41] , [42] , [43] , [44] ]. This information surely affects employees' success and could improve the prediction accuracy if included in the model, but it may be too late to act upon this information while inducing much higher expenses. Nevertheless, there is already much hinted evidence in pre-recruitment information that can help predict success, even before it is known how the recruited individual engages with the organization. Hence, it is highly beneficial to focus on early pre-hire predictions that have the highest effect on organizational ROI.
An additional important organizational aspect to examine is diversity. A report by McKinsey & Company shows that diversity leads to better profits and that diverse companies may outperform others [ 45 , 46 ]. Therefore, there are economic incentives for enhancing diversity, not solely social or legal incentives.
Literature reveals that there is some criticism with regards to the use of HR analytics for business and commercial use [ [47] , [48] , [49] ]. Gelbard et al. (2017) [ 41 ] state that one of the main reasons for the rather scarce adoption of HR analytics approaches among organizations is the use of “black-box” methods and a lack of actionable items. As shown in [ 40 ], indeed, the focus of most human resources studies is mostly descriptive or predictive, and fewer are focused on prescriptive methodologies; however, a prescriptive solution can benefit organizations greatly [ 18 ]. For further information about the literature in the field of HR analytics, we refer the reader to recent reviews in [ [40] , [41] , [42] , [43] , [44] ].
In this paper, we aim to provide a prescriptive methodology that includes interpretable insights and an optimization tool for recruitment planning and execution. This tool can be used as a decision support tool for HR professionals, since it not only provides actionable items but also allows for the incorporation of their valuable knowledge and experience into the model.
3. Methods and data
The goal of this study is to develop an analytic framework that can be implemented as a decision support tool for HR recruiters in real-world settings to efficiently hire suitable candidates and place them in the organization. The proposed methodology comprises two main components: i) a local prediction scheme for the recruitments' success with a technique for extracting meaningful insights based on the trained ML model and ii) a robust mathematical model that provides a global optimization of the recruitment process, taking into account multilevel considerations.
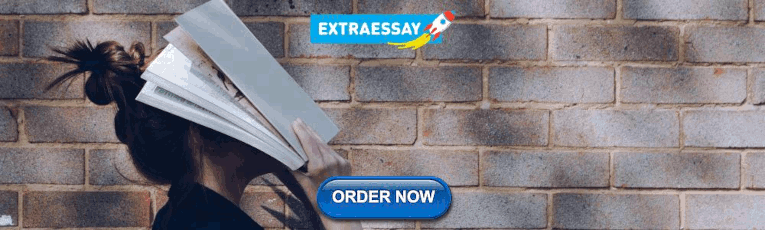
3.1. Local recruitment perspective
The first phase of this study is essentially aimed at predicting the fit of an employee to a specific position he or she is hired for. In this part of the study, we focus on using machine learning models for the pre-hire prediction of recruitment success and for the extraction of interpretable insights. The recruitment success measure is based on a combination of turnover and an objective performance indicator. This approach has several advantages in comparison to traditional methods: i) the target measure is objective; ii) it takes into account both turnover and performance; and iii) it focuses on the pre-hire prediction of recruitment success.
The use of an objective target measure, as opposed to other evaluations, allows for the examination of existing recruitment policies as well as the extraction of actionable and sometimes intriguing and unexpected insights. Objective performance is affected by the circumstances leading to a position change within the organization.
For classification and prediction of successful and unsuccessful recruitments and placements, as well as for mining significant patterns, we use a Variable-Order Bayesian Network model (VOBN) proposed by Ben-Gal et al. [ 10 ] and Singer and Ben-Gal [ 11 ]. Further details on the model used and its implementation in the recruitment process can be found in Appendix A . We evaluate the model against other interpretable and non-interpretable machine learning algorithms applied to the real-world recruitment dataset. We show that although the VOBN model has not been used before for the task of predicting recruitment success, it performs very well in terms of both interpretability and accuracy.
We use the trained VOBN model to identify context-based patterns that can support the organization in the recruitment process. As opposed to some black box models, the VOBN model can be used to extract rules and actions for the recruiters without any machine learning background, providing both scores and specific insights on factors and root causes that affect the success of recruitments.
In this phase, we focus on insights and interpretability (that are further discussed in Section 4 and Section 5 ), while in the second phase, we use the predicted probabilities for successful recruitments as inputs into a global recruitment optimization scheme that addresses more global parameters and objectives of the recruitment decisions at an organizational level.
3.2. Global recruitment optimization perspective
Recruitment success at an organizational level requires not only hiring the highest-potential workforce in a greedy manner but also optimizing the process to meet more general objectives. For example, a greedy allocation of candidates to jobs, such that the first candidates are allocated to the most promising job in terms of allocation success, can result in a sub-optimal situation in which certain jobs in the organization will be poorly allocated. Other high-level goals that could be considered are meeting the need for employees at a certain proportion, facilitating the diversity of teams, or properly balancing the workforce among different departments. Each of these dimensions may also include numerous viewpoints, e.g., successful recruitment from the candidate viewpoint, successful allocation from the job viewpoint, and an overall organizational regulatory viewpoint. In this section, we mainly focus on a global optimization perspective that takes into consideration multiple goals of various organizational stakeholders.
In the first phase, we pursued interpretability via extracted patterns, through which HR professionals can locally act. In this phase, however, we aim at higher prediction accuracy rather than interpretability for the purpose of designing a more global optimization strategy. To this end, the model with the best prediction results (even if non-interpretable) can be used to predict the probability of success of each candidate for each of the intended positions. These predictions can then be used to address a more global recruitment plan that controls more parameters of the recruitment decisions.
3.2.1. Global optimization implementation
The considered problem spans multiple dimensions , satisfying different requirements as follows: i) demand – minimizing the difference between the required workforce demand and the actual number of recruited employees; ii) accuracy – maximizing the sum of the probabilities of the successful recruitment of employees in the organization; iii) diversity – balancing diverse groups of employees to maintain a heterogeneous work environment.
Note that when facing a recruitment challenge at an organizational level, it is important to ensure that each of the above dimensions is balanced across the various business units and positions in the organization. For example, when aiming to minimize the total number of non-filled open positions in the organization, the solution has to also account for fulfilling the demand over all the open positions in a balanced manner.
3.2.1.1. Mathematical programming formulation
We consider the global recruitment task as an optimization problem and propose a mathematical programming formulation to solve it. The proposed formulation incorporates the objectives that were described above. We use the following parameters as input for the problem: the set of candidates E ; the set of positions J ; the binary qualification of candidate i to position j , represented by q ij (it equals 1 if candidate i is qualified for position j and 0 otherwise); the predicted probability of candidate i to succeed in position j , denoted by P ij which is the output of the learning model such as VOBN or GBM; and the number of open jobs in position j , denoted by N j .
Since different positions may have different values associated with successful recruitment, our formulation introduces V j as an input parameter that represents the value of successful recruitment to position j , it equals 1 if all the jobs are considered evenly, or can be set propositionally to the compensation value of that position relatively to other positions. To support diversity, this formulation includes, in addition, the following input parameters: T denotes different types or classes of candidates ( T may represent, for example, the association with diverse groups of the population); the association of candidate i to a class of type t , denoted by b it (it equals 1 if candidate i belongs to class t and 0 otherwise); and the minimal proportion of candidates of type t for position j , denoted by PR jt . A summary of the notations, including the input parameters, the indices, and the model's decision variables, is presented in Table 1 . Additionally, we use a more simplified and less constrained formulation for benchmark purposes.
Formulation notations.
The first formulation ( Formulation 1 ) is used for benchmark purposes and is a rather simple adjustment to the assignment problem [ 50 ], in which the objective function (1.1) maximizes the sum of the predicted probabilities of assignments. Constraint set (1.2) ensures that no candidate is recruited to more than one position. Constraint set (1.3) requires that the number of recruitments for position j will not exceed N j . Constraint set (1.4) ensures that only qualified candidates are recruited for positions. The next set of constraints (1.5) limits the set of possible values for X ij (whether to assign candidate i to position j ) to 0 or 1.
A simple linear programming based on the classic assignment problem solution.
Formulation 1 raises several challenges that we wish to address. For example, positions that have a very low probability of succeeding might not receive any recruitments (hence, not considering the positional point of view of our demand requirement). Another challenge is that employees might not be evenly distributed among positions. In Formulation 2 below, we propose one way to address these requirements by adding a cost to the deviation from the recruitment demand (can be proportional to the loss due to this position staying unfulfilled).
Formulation 2 introduces the decision variable Y j , which represents the difference between the required and recruited employees to the position while Y max , is set the maximal allowed position shortage (constraints sets (2.5) and (2.6)). Accordingly, we then modify the objective function (2.1) to penalize the maximum deviation from the number of open positions ( B Y max ), where B is a parameter that balances accuracy and demand objectives.
Hence, this penalty approach leads to a better distribution of the employee shortage among positions. Note that we choose to use the demand as a “soft” constraint and penalize shortages in the objective function, rather than forcing a specific level of demand satisfaction. This enables a larger feasible solution space and allows for achieving higher demand satisfaction by minimizing shortages in the objective function.
Formulation 2 also introduces diversity constraints into the model. Constraints (2.7) require that Z jt will determine the number of candidates of type t that are assigned to position j . Constraints (2.8) require that the proportion of candidates of type t assigned to position j will be at least PR jt . Table 2 presents the requirements that both Formulation 1 , Formulation 2 address in terms of the dimensions and viewpoints presented above.
Proposed linear programming with diversity and penalty on maximal position shortage.
Dimensions addressed by Formulation 1 , Formulation 2 .
3.2.2. Motivating and illustrative example
We first solve the model over a sample of the real-world dataset as a motivating and illustrative example. We then continue to implement the solution using a larger real-world dataset and perform an analysis of the trade-off between the objectives, as shown in Section 4.4 . As a first step, we use small sample data from the real-world dataset to illustrate the properties of the formulations. The data for the example are shown in Fig. 2 . It includes four positions (columns), sixteen candidates (rows), two types of candidates that need to be balanced (e.g., based on their background), and the predicted success probability for each pair of candidate and position (shades of green represent high probability and shades of red represent low probabilities). In addition, we assume a demand of 6 employees for each position.

Predicted probabilities of success of assigning sixteen candidates of two types of populations to four positions. The entries are color-coded by the success probability values, green - high probability, red - low probability. (For interpretation of the references to color in this figure legend, the reader is referred to the web version of this article.)
For example, it is clear from the table that if the only objective is to maximize the sum of success probabilities of candidates for each position separately, position 379 will be filled by candidates from the group of type 2 only.
Fig. 3 illustrates four different solutions to the problem: (1) solution to Formulation 1 , (2) solution to Formulation 2 with PR = 0, (3) solution to Formulation 2 with PR = 0.1, and (4) solution to Formulation 2 with PR = 0.3. The rows represent the different candidates, and the columns represent the positions. Within each position, an assignment of a candidate to that position is marked with color. Table 3 shows several aggregated properties of the different solutions.

Assignment of candidates to positions by four different solutions. For example, solution 1 (marked in red) suggests the following: i) recruiting 4 candidates to position 1409; ii) recruiting 6 candidates to position 1509; iii) recruiting 6 candidates to position 379 (note that none of them are of type 1); and iv) not recruiting any of the candidates to position 40 (For interpretation of the references to color in this figure legend, the reader is referred to the web version of this article.).
Illustrative example results. Entropy is used as a suitable measure for diversity in the case of more than two candidate type.
We observe the following: i) Accuracy: the predicted success probability decreases with more constrained models (i.e., models with more constraints) as a result of the shift from global to local objectives; ii) Demand: (1) formulations that penalize deviation from the required demand (Solutions 2–4) avoid cases of positions in shortage of assignments, and (2) solutions that incorporate the penalty on the maximum shortage (Solutions 2–4) manage to better balance the demand satisfaction among positions; and iii) Diversity: (1) adding diversification constraints to the formulations (Solutions 3 and 4) results in higher diversity without significantly compromising accuracy, and (2) solutions that impose a high diversity requirement (Solution 4) may result in higher demand shortage. Similar results are expected over larger recruitment experiment.
3.3. Dataset description and target definition
The input dataset for this research includes hundreds of thousands of employment cases (approximately 700,000 cases) of employees who were recruited to the organization over the span of a decade (hired between the years 2000–2010). The pre-hire features in the dataset include age, gender, family and marital status, residence, nationality details, background record, education and grades, interviews and test scores (including leadership scores and language scores), professional preferences questionnaires, family details (when available), “lifestyle” data (when available), and details about the positions. Table 4 presents the main categories of the 164 features in the dataset.
Feature summary (after data preparation procedures).
In the preprocessing phase, 21 data tables were consolidated to mask sensitive private data and personal identification; on this dataset we also performed feature enrichment processes and addressed missing data and outliers. Specifically, in the feature enrichment process, we identified several interesting hierarchies of position groups and background data. In addition, we used residence-related data to deduce the socioeconomic levels of the candidates, using statistical data from the Central Bureau of Statistics. Missing values were tagged in the dataset by zeros, since these values mainly represented a lack of a specific test result or interview attribute. The reason to avoid a certain test or question for a specific candidate was not random nor uniform but rather based on the candidate's profile. For example, candidates who seemed to be less relevant to a specific job type were not asked to complete a related questionnaire or did not go through a specific interview segment. As such, these zeros indicate a specific categorical decision, which could be overlooked had we used the mean values (e.g., the mean of the results of certain tests, to impute them). The data records of candidates with many missing values were removed entirely; however, only <1% of the records were removed in total. Additional dimensionality reduction procedures were performed in accordance with each of the applied machine learning algorithms (see details in Section 4 ).
The class feature definition for successful and unsuccessful recruitments was conducted by utilizing the following process: based on HR department records, the reasons for employee turnover were analyzed and accordingly divided into two groups: successful recruitments (e.g., the employee left for “natural” reasons, such as leaving the job after a sufficient time period) and unsuccessful recruitments (e.g., job termination after a short amount of time or due to poor performance). Position and placement changes were classified as negative (e.g., “misfit”) or positive (e.g. “promotion” or “job enrichment processes”).
To conclude, the combination of turnover and position changes was used as a combined measure for labeling successful vs. unsuccessful recruitments, as seen in Table 5 . To clarify, the fifth row in the table represents instances that were excluded from the analysis as their period of employment was not long enough to determine if they were successful or not. To maintain consistency, the a-priori distributions of the target class in both the training and testing datasets include 30% of the unsuccessful recruits and 70% of the successful recruitments.
Target definitions by HR department.
Recall that the dataset was acquired from a large nonprofit service organization that is highly diversified over roles, accountabilities and job descriptions with a heterogeneous population. These characteristics allow for testing potentially biased recruitment policies and decisions that traditionally may not be tested due to the absence of sufficient data on certain groups or lack of information on different personal properties. Specifically, it enables us to focus on various groups in the population and to show some counter-intuitive understandings based on data, which is not commonly available.
With respect to data selection, we aimed to focus on early pre-hire predictions; thus, the features that were integrated as predictors in the model included only the available pre-hire data, i.e., data from before the recruitment day. The motivation for such data selection was based on several reasons. First, the recruitment day is an important decision point in which it is easier for the organization to take action —for example, the early identification of a possible misfit may save a great deal of financial and social costs. Second, such data selection enables the identification of actionable recommendations for preventive actions. For example, there is little interest in the revelation of turnover among employees who were absent for a long period of time immediately before they resigned (these causes are obvious and self-evident and also occur too late to be acted upon).
Note that although post-hire data was available (i.e., data about each employee through his employment period), we utilize only the pre-recruitment data. This approach allows us to achieve the goal of improving the recruitment process and providing insights that may be integrated within recruitment decision processes.
3.4. Prediction model and evaluation measure
The classification algorithms were trained on 70% of the candidates (first 8 years in the dataset). In the test stage, we used the trained classification models to predict the recruitment success of the remaining 30% of the candidates and validate our predictions with the ground truth. Note that we used time-dependent partitioning for training and testing to reassure the applicability of the model in the real world and show that the model can still be valid even when the organization changes.
In this process, we examined five interpretable machine learning algorithms and four non-interpretable algorithms. We evaluated the results of the prediction models by relying on the AUC (area under ROC curve) measure. According to the literature, e.g., Chawla [ 51 ], when the dataset is imbalanced (e.g., when the target variable includes large differences between the frequencies of different class values), an appropriate performance measure is the ROC curve and the AUC measure.
4.1. Model evaluation
The study results are presented in Table 6 below. Comparing various interpretable and non-interpretable models, the best AUC score obtained by an interpretable model was obtained using the VOBN algorithm [ 10 , 11 ], with an AUC = 0.705 on the test set. The best results by a non-interpretable model, were obtained by the gradient boosting machine (GBM) algorithm with an AUC = 0.73. Thus, for interpretability purposes, we suggest selecting the VOBN model, whereas for solely aiming at prediction, we suggest using the GBM model.
Evaluation of models. a
A conventional approach to handle multiple (conflicting) objectives is to use a Pareto-optimality approach [ 52 ]. The model's AUC and its interpretability can be considered as two conflicting objectives that should be addressed by a Pareto-optimality approach. In this sense, the VOBN and the GBM algorithms are both “Pareto optimal”. Specifically, the GBM should be selected if the objective is mainly prediction (although non-interpretable), while the VOBN model should be prioritized if the model interpretability is important, despite a relatively small decrease in the AUC score. Such interpretability not only enhances the understanding of key features in the prediction model but also provides root cause analysis and insights into the recruitment process. Following the evaluation of the different models, the VOBN and GBM models were used for further experimentation and analysis — the former for identifying interpretable patterns and the latter for a global optimization approach.
4.2. Identified patterns
Patterns in this use case can be thought of as regularities in the dataset that characterize subpopulations of candidates with common characteristics. A pattern is often described by a set of rules that can be used to cluster subpopulations into different categories. The VOBN, as an interpretable descriptive model, enables the extraction of patterns that can be mapped into insights for the recruitment process, as seen in the next example. The VOBN model has generated more than a thousand patterns that went through a filtering process based on the following: their statistical validity (i.e., statistical significance and support set that indicates how many cases they refer to) and the change they imply on the recruitment's success probability with respect to other subpopulations. The final set of implemented patterns contained few dozens of patterns (a number that also depends on the ability of the recruiters to implement it in their routine procedures), including the ones used by the HR department and the ones presented in the next examples. These patterns were selected by a prioritization process that included the following steps: i ) selecting patterns that contain at least one variable that can be controlled by the HR department, such as a threshold on a test result (otherwise the pattern is non-actionable); ii ) selecting patterns in which the controlled variables separates well the population into subgroups resulting in different success probability outcomes; iii ) prioritizing patterns that represent “counter-intuitive” phenomena that were not known to the recruiters; and iv ) prioritizing patterns with larger number of instances in the leaves and with a larger turnover percentage.
The following are several examples of patterns, some of which are counter-intuitive and were extracted from the data by the VOBN algorithm.
Correlation of a high analytical score in a pre-placement test with the dropout rate in a specific administrator position over different subpopulations.
As shown in Fig. 4 , an interesting pattern is found related to the correlation of a high analytical score in a pre-placement test on the position dropout rate of certain administrator positions. As seen in the left figure, the position dropout rate falls only slightly (from 42.5% to 39.3%) when the candidate obtains a higher analytical score. However, as seen from the pattern in the right figure, for men with low leadership skills scores and low language scores, the dropout rate increases significantly (from 58.1% to 68.3%, with p -value<0.001) if the candidate has a high analytical score. A possible explanation can be related to the fact that a high analytical ability has an over-qualifying effect on these specific candidates.

High analytical score effect on administrator position dropout rate over various subpopulations.
Skowronski [ 53 ] reviews the connections between over-qualification and turnover as well as performance. The paper proposes several practices for the pre-hire and post-hire management of overqualified employees and suggests considering perceived over-qualification rather than merely objective over-qualification. In the case of the considered pattern, it is likely for an employee to feel overqualified and less motivated if he or she is highly skilled but not able to demonstrate his or her competence due to language and communication gaps.
To overcome the above difficulties, recruiters should investigate which jobs' properties might decrease the probability of successful recruitment and adjust the specific job requirements to accommodate for wider populations of employees. They may also devise unique programs for different populations that includes for example language, communication and technical training.
The effect of competencies on the position dropout rate for a specific field-support position.
In general, the data show that candidates with high competencies are less likely to leave their position than are candidates with low competencies (15% vs. 30% position dropout rate, respectively, with p -value<0.001). However, for specific field-support positions, this effect is reversed. Fig. 5 illustrates how candidates for specific support positions who have high competencies follow a significantly higher position dropout rate than do candidates with low competencies (43% vs. 21% position dropout rate, respectively, with p-value<0.001). Here, the recruiters should again be aware of the reversed relation in the case of this field-support position.

The effect of competencies on the position dropout rate for all positions and for a specific field support position.
This considered pattern also shows that the dropout rate for low-competency employees has decreased when they are assigned to a specific support position. This is somewhat unexpected since it implies that an organization should strive for the heterogeneity and diversity of its employees rather than recruiting only the most highly scored individuals. This notion is also supported in a report by McKinsey & Company that interestingly showed that diversity leads to better profits among organizations [ 45 , 46 ]. Let us note again that this output is due to the analysis of a unique dataset of a large nonprofit service organization that hires diverse populations with different backgrounds and skills.
Correlation between low personal interview scores and low management skill levels with position dropout rates in specific business units for male candidates.
The pattern under consideration shows that the effect on the position dropout rate for male candidates with low scores in a specific section in the personal interview, combined with low management-skills score, is business-unit dependent, as shown in Fig. 6 . For males, these low scores are associated with a position dropout rate of 37%, compared to an average dropout rate of 29% for all male candidates. However, this observation changes significantly among different business units, as seen in the figure.

The relationship between poor-skill levels and position dropout rates for male candidates in different business units.
In business unit A, the position dropout rate for all males is 39% (1928 out of 4916), while candidates with low scores have a considerably higher position dropout rate of 60% (394 out of 662). In business unit B, the opposite effect is observed: the dropout rate for male candidates with low scores is 23% (4113 out of 17,600), which is slightly lower than the rate for males, with an average score of 26% (7648 out of 29,049). All these differences have p -values lower than 0.001.
As mentioned above, these findings support previous observations in the literature that call for the diversification and heterogeneity of workers [ 45 , 46 ]. Moreover, these findings emphasize the advantage of using data-driven methods to allocate people with diversified backgrounds and skills to specific positions (involving complex hidden patterns, related in this example to gender, business units, managerial and personal skills as well as specific test scores), in which they have a higher potential for success and good performance. Using the proposed approach, organizations should detect the characteristics of specific positions that are found to be statistically related to the allocation success of candidates from various backgrounds. These recruitment and allocation insights should be implemented accordingly, as long as they follow the required regulations for transparency, fairness and explainability (e.g., see GDPR: The EU's General Data Protection Regulation).
Cultural background effect on position dropout for a specific office administrative position.
The model identified a unique pattern that is related to a specific administrative office position. It turns out that for this office position, allocating a subpopulation of people with a specific common background results in a significantly lower dropout rate (23% instead of 44%, with p -value<0.001).
Note that without a granular pattern-detection model, such as the one proposed here, it would be extremely difficult to identify such significant correlations between this office position and the specific cultural background. As seen in Fig. 7 , the average effect of the cultural background over all the positions is minor (indicating a 5% difference only). However, for this considered administrative office position, the effect on the dropout rate is marginal, i.e., more than four times greater (a 21% difference).

The potential effect of the candidates' cultural background (A or B) on the dropout rate for all positions and for a specific administrative office position.
Organizations and researchers should investigate why some subpopulations of candidates who share common characteristics outperform or underperform in specific jobs or scenarios. Accordingly, they should find more opportunities to include (rather than exclude) specific populations as well as to adjust other organizational practices to support successful recruitment, considering the data-driven patterns discovered. In this context, it worth noting that the literature already recognized, for example, that some subpopulations of immigrants who share common cultural assets and social norms are sometimes better equipped than others to succeed in specific scenarios and vice versa (see [ 53 , 54 ]).
The effect of oral language score on turnover differs by specific subpopulation.
The effect of an oral language score on turnover is heavily dependent on the chosen subpopulation. Fig. 8 shows that when analyzing this factor over all the employees in the organization, the turnover rate associated with a low oral language score results in a significantly higher position dropout rate (31% vs. 9%, with p -value<0.001) and thus a lift of 3.2. However, when considering a subpopulation of women in administrative positions from a specific cultural background and a certain educational path, the turnover lift grows approximately to 15.5 (77% vs. 5%, with p-value<0.001). This pattern addresses a rather privileged group of women according to their cultural and educational background, and although expected to succeed in their placement (with a 6% turnover only), there is a noticeable language deficiency that affects their ability to succeed in specific jobs.

The effect of the oral language score on turnover changes for specific subpopulations of candidates.
It is interesting to compare the relative contribution of features when considering a large population to that of a specific subpopulation. Note that the feature importance of language according to Table 4 is relatively low; however, for a specific subpopulation, there is a greater impact of language skills. This notion is also closely related to Simpson's Paradox [ 55 ], which shows that an observed trend in subgroups may behave quite differently (even reversely) when these subgroups are aggregated and analyzed together.
4.3. Results application
When recruiters are looking for candidates to be placed in certain positions, they can take advantage of many patterns, such as those shown above. First, they can check that all the relevant data being used by the algorithm are collected and analyzed for all candidates. In addition, they can decide to send some of the candidates to undergo additional testing shown to be informatively correlated with the dropout rates. Then, they can apply the obtained patterns that were discovered by the algorithm to improve the recruitment and placement processes.
Finally, the obtained patterns can be used to reveal insights about factors that contribute to the recruitment success of specific positions. This in turn can provide feedback to the organization and can be used to adjust the position definition, such that it increases employment satisfaction and the overall recruitment success probability.
It is important to note that these insights must be considered in the proper ethical and legal contexts following specific regulations (e.g., GDPR). Organizations should investigate the reasons as to why some candidates underperform under certain scenarios and find opportunities to include, rather than exclude, diverse populations while adjusting organizational practices when the discovered patterns are considered. In the next section, we discuss a proposed global optimization formulation that can be used to enhance diversity in the organization while maintaining high success placement rates.
4.4. Data-driven global optimization results
In this section, we show an analysis of the proposed global optimization model. The analysis incorporates the data of real candidates, positions and demand and includes the predicted success probabilities derived from the prediction for a yearly planning program of our organization. We then perform a sensitivity analysis of the results and compare them to the recruiters' actual decisions.
The best prediction was obtained using the GBM algorithm [ 56 ], with AUC = 0.73 (see Table 6 ). We analyzed the robustness of the model by using different time-based partitions for training and testing and noted that the AUC value remained stable. Let us note that the results may be improved using post-hire data; however, as mentioned above, in this study, we focus on recruitment, as performing a prediction in a later post-hire phase may be too late to act upon, leading to much higher expenses.
The problem includes 30 position-types and all the candidates recruited to these position-types during a period of one year (10,329 candidates). As in the previous section, we compared different formulations of the problem to the actual assignment of the recruiters in the organization (see Table 7 and Fig. 9 ) and analyzed the trade-offs between different objectives. We expect to have similar results and to be able to show an improvement (in terms of both accuracy and diversity) compared to the actual allocation that was performed by the recruiters.
Results for a yearly plan.

Pareto efficiency for a yearly plan of the real-world scenario.
The results above indicate the following 1 :
- • We expect that more complicated diversity requirements will lead to a reduced predicted probability of success. However, it can be observed that in the suggested formulations (Solutions 2.4–2.6 in Table 7 ), there was a significant improvement in both objectives in comparison to the actual assignment.
- o Average entropy - measuring individual positional diversity (the higher it is, the better).
- o Mean difference - measuring balanced scoring between candidate groups (the lower it is, the better).
- o Standard deviation of average probabilities for positions - measuring balanced average scoring between positions (the lower the standard deviation is, the higher the balance between positions).
To combine the local and global procedures, it is possible to integrate additional limitations or preferences in the model that were induced from interpretable insights or from other sources, such as legal or regulatory requirements. One approach for doing so is to incorporate additional constraints to the model. Another possible approach is to indicate entries in the probability matrix, such as in the Big M Method [ 57 ]. For a future study, we suggest devising a model that will explicitly require the reduction of the imbalance between positions.
4.4.1. Arc deletion heuristic
We note that a more demanding and complex diversity requirement entails longer runtime, which ranges from a few minutes for PR = 0 to 51 min with PR = 0.1 up to several hours with a higher diversity requirement. However, the bottom line is that the size of a relevant assignment problem, even for a large organization with thousands of workers, is fully feasible with the proposed approach (specifically with the suggested heuristic described below).
In order to reduce the computational complexity of the model, we suggest a simple heuristic for the deletion of arcs. The heuristic deletes arcs that have a predicted success probability under a certain threshold (in our experimentation we use a threshold of P ij < 0.45). We found that such a deletion allows one to reduce computational complexity while having only a minor effect on the obtained accuracy. In particular, after introducing arc deletion, the observed gaps to the best solution (without an arc deletion) were at most 1% but resulted in runtimes shorter by half or less with respect to the original runtimes. Note that deleting arcs, although improving runtimes, may compromise demand satisfaction in cases in which there are many candidates with an allocation probability which is lower than the threshold, for a certain position.
5. Conclusions
The objective of this study is to develop a hybrid decision support tool for HR professionals in the operations of recruitment and placement. The proposed methodology consists of two main components. The first is the definition of the problem as a machine learning problem with objective recruitment success as the target variable for specific candidate-position recruitments. The second is the development of a method based on mathematical modeling, which provides a global prescriptive hiring policy at an organizational level rather than a local one.
In the first phase, the machine learning model predicts the probabilities of successful recruitments and placements by taking into account various turnover scenarios and pre-recruitment data. The proposed approach is objective, based on an integrated performance indicator as opposed to some other evaluation schemes from the HR literature. It allows for an examination of current recruitment policies and the extraction of interpretable and actionable pattern-based insights.
In the second phase, the methodology considers the multi-stakeholder environment of the recruitment problem, including multisided balance and diversity in the process. We show that using the proposed mathematical programming model, even with the requirements of balanced demand and diversity, one is able to maintain a high level of accuracy and while improving the multiple objectives, compared to the actual selection of the recruiters. Implementing the presented approach as a decision support tool can increase the impact of recruiters and maximize organizational return on investment.
The utilized dataset in this study is unique and includes the data of hundreds of thousands of employees over a decade. The data represent a wide range of heterogeneous populations represented in a big-data repository. These characteristics allow us to analyze various recruitment policies and decisions that traditionally could not be tested due to the absence of proper data for such research studies.
The proposed methodology can be acted upon directly by HR professionals, without a need for deeper technical or machine learning knowledge, and can be implemented as a support software tool for recruiters and HR managers. A detailed study on the contribution of the proposed approach with respect to existing HR theories is beyond the scope of this paper and can be found in [ 40 , 58 ].
We recognize that a prediction model that stands alone may be inherently biased; hence, in this work, we approach this potential bias through several measures: i) an objective target measure; ii) a large dataset incorporating a large range of differing applicants; iii) a mathematical programming model that enhances diversity and balance; and iv) a proposition to use a combined decision of both the recruiter and the used algorithm.
For future research, we suggest examining various directions of post-hire feature analysis and studying how these factors affect recruitment performance in comparison to the baseline literature as well as to a pre-hire analysis only. In light of the explainable patterns discovered in relevant candidate profiles, organizations may also devise and adjust personalized practices, such as specific training programs, awareness workshops, compensation and benefit plans, definitions of job duties, work-life balance policies, management and communication campaigns, and the overall organizational culture [ 44 ].
CRediT authorship contribution statement
All authors conceived of the presented ideas, developed the theory, performed the computations, discussed the results and took part in writing the paper. All authors read and approved the final manuscript. Dana Pessach: Conceptualization, Methodology, Formal analysis, Validation, Writing - original draft, Writing - review & editing. Gonen Singer: Conceptualization, Methodology, Formal analysis, Validation, Writing - original draft, Writing - review & editing. Dan Avrahami: Conceptualization, Methodology, Formal analysis, Validation, Writing - original draft, Writing - review & editing. Hila Chalutz Ben-Gal: Conceptualization, Methodology, Formal analysis, Validation, Writing - original draft, Writing - review & editing. Erez Shmueli: Conceptualization, Methodology, Formal analysis, Validation, Writing - original draft, Writing - review & editing. Irad Ben-Gal: Conceptualization, Methodology, Formal analysis, Validation, Writing - original draft, Writing - review & editing.
Declaration of competing interest
Acknowledgements.
This paper was partially supported by the Koret Foundation Grant for Smart Cities and Digital Living 2030.
Biographies
Dana Pessach is a PhD candidate at the Big Data Lab at Tel-Aviv University. Her research focuses on applying computational methods to social sciences and contributing to the development of new methods to deal with real-life problems. She is additionally a member of the steering committee at LAMBDA, the artificial intelligence lab at Tel-Aviv University, and a freelance consultant to start-ups and entrepreneurs in the fields of Machine Learning and Artificial Intelligence. As part of her role she leads research projects in domains such as: HR analytics using Machine Learning methods, financial data technologies, health data analysis and smart-cities. Her teaching experience includes several topics for undergraduate programs such as: operations research, robotics, mobile application development, modeling and 3D printing, social network analysis and computer vision.
Gonen Singer , Ph.D., is a Senior Lecturer of Industrial Engineering and Information Systems at Bar-Ilan University. Before joining Bar Ilan, Gonen was a Senior Lecturer at AFEKA-Tel-Aviv Academic College of Engineering. He joined to the Department of Industrial Engineering and Management at AFEKA, shortly after its establishment at 2008 and was appointed as Head of the Department in the years 2009–2015. Gonen Singer has extensive expertise in machine learning techniques and stochastic optimal control and their application to real-world problems in different areas, such as retail, manufacturing and education and has around 50 Scientific and professional publications. Gonen Singer served as a principal investigator in 10 Research Grants since 2011 that were obtained from various sources.
Dan Avrahami holds an M.Sc. from LAMBDA, the artificial intelligence lab at Tel-Aviv University, where he also taught Information Systems Engineering. In his HR analytics research, he explored the application of machine learning in general, and a generalization of Bayesian Networks in particular, to improve employees' recruitment and placement. Dan is a data scientist and machine learning researcher with experience implementing various machine learning methods on challenging data domains. He has a solid background as a software engineer and database administrator.
Hila Chalutz Ben-Gal is a Senior Lecturer at the Department of Industrial Engineering and Management, Tel Aviv Afeka College of Engineering, Israel. She received her BA degree (with honors) from the Hebrew University in Jerusalem, Master's Degree from Brandeis University, Boston, MA, USA, and her Ph.D from Haifa University, Israel. Her research interests include HR Analytics, and the changing nature of organizations in the digital era, with a focus on organizational design and the future of work. She conducts field research and consulting work in a variety of organizational settings. Some of her current projects include HR analytics and turnover forecasting, career moves detection in on-line labor markets and the changing nature of work due to COVID-19 pandemic. During 2015–17 she was a Visiting Scholar at the ChartLAB at the University of San Francisco in California, USA.
Erez Shmueli is a senior lecturer and the head of the Big Data Lab at the department of Industrial Engineering at Tel-Aviv University and a research affiliate at the MIT Media Lab. He received his BA degree (with honors) in Computer Science from the Open University of Israel and MSc and PhD degrees in Information Systems Engineering from Ben-Gurion University of the Negev and spent two years as a post-doctoral associate at the MIT Media Lab. His research interests include Big Data, Complex Networks, Computational Social Science, Machine Learning, Recommender Systems, Database Systems, Information Security and Privacy. His professional experience includes being a programmer and a team leader in the Israeli Air-Force and, a project manager in Deutsche Telekom Laboratories at Ben-Gurion University of the Negev, a co-founder of two startups (Babator and SafeMode), and a consultant (among the rest to Microsoft and the municipality of Ashdod).
Irad Ben-Gal is a full professor and the Head of the Laboratory for AI, Machine learning, Business & Data Analytics (LAMBDA) at the Engineering Faculty in Tel Aviv University. He received his M.Sc. (1996) and Ph.D. (1999) from the Boston University. His research interests include machine learning, applied probability and statistical control, involving R&D collaborations with companies such as Oracle, Intel, GM, AT&T, Applied Materials and Nokia. He wrote three books, published >120 journal and peer-reviewed conference papers and patents, supervised dozens of graduate students and received numerous awards for his work. He co-heads the TAU/Stanford “Digital Living 2030” research program that focuses on data science challenges of modern digital life. The program involves scientists at TAU and Stanford, where he held a visiting professor position, teaching “analytics in action” to graduate students. Irad is the co-founder of CB4 (“See Before”), a startup backed by Sequoia Capital that provides predictive analytics solutions to retail organizations.
1 The experiments were solved using the R Rglpk package (see [ 73 , 74 ]) for solution with the presolver option on ( presolve = True ) and were executed on a Windows Server based 64-bit with two 6-core CPU processors with 1.9GHz and 128 GB memory.
Appendix A. Use of VOBN for recruitment success prediction
In this study, we propose to use flexible and generalized version of the Bayesian Network (BN) models [ 59 ], called Variable Order Bayesian Networks (VOBN) model as proposed by [ 10 , 11 ]. Similar to the BN it is an interpretable model that can be used to describe the relationship among various features, however, as opposed to BN possible connection between features does not imply necessarily that all the feature values of the conditioning features affect the conditioned feature. The possibility to construct such a flexible learning model that is not necessarily balanced over the entire feature space and at the same time can reveal those specific value-dependent patterns is found to be of outmost importance in the case of HR recruitment applications. For example, for a certain position, the probability of a successful recruitment might depend only on a specific language test score (e.g., a test score above 95) that is correlated with a specific managerial background, while all the other scores and background levels do not affect the recruitment success and should be therefore ignored or “lumped” together.
The following walk through example demonstrates the VOBN algorithm and implementation for predicting the turnover rate of female candidates who were hired to perform administrative roles. Detailed discussion on the VOBN algorithm can be found in [ 10 ].
Stage 1: Bayesian Network construction
First, the algorithm builds a Bayesian Network for the available features and the target variable, which in this case is the turnover rate. It uses the mutual information between the features as a dependence measure, and constructs the maximum likelihood graph structure, by placing feature with high mutual information next to each other. Next, it locates the target variable in the Bayesian Network and the features leading to it. In Fig. 10 one can see a portion of the Bayesian Network generated for the candidates' dataset. It shows that the conditioned distribution of the turnover rate, depends directly on the Oral Language Score feature, which depends on the feature Educational Background , which depends on the Birth Country etc.

Portion of the Bayesian Network constructed for the dataset.
For an alternative algorithm which uses a Bayesian network instead of the Bayesian tree see [ 10 ].
Stage 2: variable order Markov (VOM) context tree construction
After the Bayesian network (or a Bayesian tree in this example) is constructed, the algorithm constructs a complete and balanced tree of depth L – a fixed-order Markov tree of depth L , using the features from the Bayesian Network. It sets R to be the minimal frequency of samples in a leaf, for statistically significance evaluation. It chooses an initial depth L for the context tree, such that there are on average at least R examples in each leaf, to enable sufficient number of leaves with minimal frequency after the pruning stage (see stage 3). In the walk-through example the depth of the tree is set to L = 3 to obtain an average of R = 100 samples per leaf. We use the order found by the Bayesian network, as an input for the context tree construction.
Stage 3: context tree pruning
In order to obtain a minimal context tree, which capture most of the information in the features, and allows statistical significance, two pruning rules are applied as follows.

VOM Context Tree of the walk thorough example Pruned nodes are marked with a dashed line.
- ii) Pruning rule 2 – The algorithm compares the information obtained from the descendant leaf, defined by series of features sb , to the information obtained from the parent node, defined by series of features s . It then prunes the descendant node if the difference is smaller than a predefined penalty value for making the tree bigger – this penalty is called the pruning threshold . Hence, a node that has a turnover distribution similar to the distribution of the parent's node is pruned, since it doesn't add enough information. In this example, the algorithm estimates the turnover probability for each of the nodes, according to the frequencies of turnover cases.
In Eq. (1) the algorithm computes ΔN ( sb ) - the (ideal) code length difference between each descendant leaf, denoted by the pattern sb and its parent node, marked by the pattern s . b is the last split feature and its value of the descendent leaf, and s is the pattern defined by all previous split features and their values till the parent node. For example, in Fig. 11 , descendant leaf can be sb = { Low oral language score, Educational background A, Birth Country I }, while its parent node is denoted by s = { Low oral language score, Educational background A }.
P ^ x sb is the conditional probability for obtaining the value x in the descendant node sb , and n(x|sb) denotes the number of samples with the value x in the descendant node sb , X is the finite set of values of the variable target. In our case, these are the turnoverTrue and the turnoverFalse values. If the difference is smaller than a pre-selected pruning threshold , the leaf is pruned, as defined in Eq. (2) .
In order to reduce over-fit and simplify the context tree, without losing much information, the algorithm prunes the context tree, which leaves nodes that contributes significantly to the turnover classification task and contains enough samples to allow statistical significance. In order to achieve this requirement, it requires that ΔN ( sb ) will satisfy Eq. (2) .
where C is a pruning constant tuned to the considered process requirements (with default of C = 2 as suggested in [ 60 ]). d is the number of values the target variable can obtain, in our case d = 2 (since the target variable includes only two values: turnoverTrue, turnoverFalse) and t is the number of features defined by the pattern sb of the examined node.
We will now show an example for the calculations of Eqs. (1) , (2) using the tree shown on Fig. 11 . When we calculate the (ideal) code length difference for the bottom descendent left leaf {6}, defined by the patterns sb = {Low oral language score, Educational background B, Birth country II}, compared to its parent node defined by s = {Low oral language score, Educational background B} using Eq. (1) we obtain the following result:
In order for this descendent leaf not to be pruned, Eq. (2) must hold, i.e.,
Since ΔN (8.27) is below the threshold in our case, 12, then leaf is pruned.
Similarly, the algorithm prunes leaf {2} in the tree shown on Fig. 11 , with the pattern High Oral Language Score since its turnover rate (6%) is similar to the turnover rate of its parent node - in this case the root node {1} (7%).
The summary of the used notations in this section is presented in Table 8 .
Stage 4: patterns identifications
The pruned context tree is left with a smaller number of leaves, each represents a pattern related to a specific sub-population, whose turnover rate is distinguishably different than the parent sub-population. In the context tree in Fig. 11 , the following patterns are found for women in administrative roles with low oral language score:
- 1. Candidates from educational background B – 25% turnover rate.
- 2. Candidates from educational background A who were born in country II – 29% turnover rate.
- 3. Candidates from educational background A who were born in country I – 77% turnover rate.
Strength and Weaknesses of the VOBN Model in Recruitment Analysis
VOBN provides an important extension with respect to both Bayesian Network and Decision Tree models. In Decision Tree models, leaves represent class labels, nodes represent features and branches represent conjunctions of features that lead to those class labels. When the target variable takes a discrete set of values these trees are often called Classification Trees, while for a continuous target variable they are called Regression Trees. Decision Trees can generate a set of rules directing how to classify the target variable based on the associated features values, yet in a tree-like structure, thus where each node (feature) has only a single parent node. As opposed to decision trees, VOBN constructs a more general graph structure, where several nodes can be the parents of other nodes, thus representing a more general dependencies structure among different features in the model (these structures can then be mapped to a simpler tree-like rules, as done in this study). This generalization is important in the considered recruitment and placement application, since complex dependency patterns that involve several features and their interactions (e.g., background, performance, motivation etc.) can lead to different placements and recruitment recommendations that can result in a higher performance, as seen in Table 6 .
The VOBN not only generalize Decision Trees but also generalizes the conventional Bayesian Network (BN) model. In BN modeling each variable (feature) depends on a fixed subset of random variables that are locally connected to it, however, in VOBN models these subsets may vary based on the specific realization of their observed variables. For example, a complex dependency between a Language Score and a Leadership Score features to the placement success in a specific position, might exists only for specific score values, while for other score values such a dependency is practically insignificant. This generalization lead to a reduction in the number of the model parameters, resulting in a better training and performance. The observed realizations in the VOBN are often called the contexts and, hence, VOBN models are also known as Context-Specific Bayesian networks.
In summary, compared to Decision Trees and conventional Bayesian Networks, often the classification performance of the VOBN is better, based on its higher flexibility in learning and expressing complex conditions and patterns among subsets of feature values. In the considered domain of HR analytics, this flexibility implies that the context dependency (based on the variable ordering) may be represented differently for each of the considered positions. Additionally, the VOBN handles better the variance-bias tradeoff, compared to decision trees, which often suffers from over-fitting [ 10 , 61 ] and may cause high variance. The VOBN models have previously shown good performance in analyzing various datasets (some of which publicly available), including DNA sequence classification [ 10 , 62 , 63 ], transportation and production monitoring [ 11 , 64 , 65 ]. The VOBN has two main limitations. First, the dataset has to contain relatively large amount of data in order to construct the initial network structure. Second, the features introduced into the model should be discretized in a preprocess stage. In this study we used a large HR dataset, in which most of the features contain discrete values, hence yielding high performance of the VOBN.
It is worth noticing that this machine learning model has two main distinctions from traditional hypothesis-testing and regression models. First, the latter focuses on features that are highly correlated with trends across the entire aggregated sample, whereas the analysis by the VOBN model allows for identifying patterns in specific sub-groups. This notion is also closely related to Simpson's Paradox [ 55 ], which shows that an observed trend in subgroups may behave quite differently when these subgroups are aggregated and analyzed together. Second, hypothesis-testing requires in-advance assumptions about the interactions among features, whereas machine learning models do not require such assumptions, and allow for discovering insights that were not assumed ahead. For further mathematical and experimental details on the construction of the VOBN model, please see [ 10 , 11 ].
Digital Job Searching and Recruitment Platforms: A Semi-systematic Literature Review
- Conference paper
- First Online: 29 August 2023
- Cite this conference paper
- Chiara Signore 15 ,
- Bice Della Piana 15 &
- Francesco Di Vincenzo 15
Part of the book series: Lecture Notes in Networks and Systems ((LNNS,volume 769))
Included in the following conference series:
- International Conference in Methodologies and intelligent Systems for Techhnology Enhanced Learning
291 Accesses
1 Citations
The purpose of this paper is to shed light on the new E-recruitment trend that is pervading the lives of job seekers, included students, and job offers. A semi-systematic literature review on digital job searching and recruiting platform in the last five years was conducted with the aim to develop a preliminary conceptual framework. Following a replicable research process, a final sample of 37 publications was located in five subdimensions - Web Application Framework, Use of Artificial Intelligence technologies, Use of Blockchain Technologies, Type of User, User Experience - grouped by two dimensions of analysis: “Technical implementation of the platform”, “Platform usability analysis”. From our findings it emerges that the first one received strong attention, specifically with regards to subdimensions Web Application Framework and Use of the Artificial Intelligence Technologies; the subdimension Use of the Blockchain Technologies started to attract scholarly attention only from 2020. The second dimension of analysis has received a fair amount of attention over the last five years, but it seems that in 2021 the sub-dimension Type of User is perceived as the most attractive from scholars from different field of studies.
The contribution of this work is twofold. Firstly, it tries to shed lights on the main characteristics of the studies about the job searching and recruiting platforms as derived from the publications included in our review identifying appropriate dimensions and sub-dimensions of analysis that could be useful to analyze these platforms in the future. Secondly, for each sub-dimensions we identified the major challenges that authors have set out to address. This specific aspect will be helpful to identify the future research agenda for the topic investigated.
This is a preview of subscription content, log in via an institution to check access.
Access this chapter
- Available as PDF
- Read on any device
- Instant download
- Own it forever
- Available as EPUB and PDF
- Compact, lightweight edition
- Dispatched in 3 to 5 business days
- Free shipping worldwide - see info
Tax calculation will be finalised at checkout
Purchases are for personal use only
Institutional subscriptions
Similar content being viewed by others
E-Recruitment and the Impact of Digital Age on Recruitment: A Critical Literature Review
Innovating e-Recruitment Services: An Italian Case Study
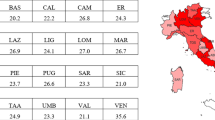
Online job ads in Italy: a regional analysis of ICT professionals
Adamovic, M.: Organizational justice research: a review, synthesis, and research agenda. Eur. Manag. Rev. (2023)
Google Scholar
Afnan, T., Rabaan, H., Jones, K.M.L., Dombrowski, L.: Asymmetries in online job-seeking: a case study of Muslim-American women. Proc. ACM Hum.-Comput. Interact. 5 (CSCW2), 1–29 (2021). https://doi.org/10.1145/3479548
Ahamad, F.: Impact of online job search and job reviews on job decision. In: Paper Presented at the WSDM 2020 - Proceedings of the 13th International Conference on Web Search and Data Mining, pp. 909–910 (2020). https://doi.org/10.1145/3336191.3372184
Alben, L.: Defining the Criteria for Effective Interaction Design, vol. 5 (1996)
Awaji, B., Solaiman, E., Marshall, L.: Blockchain-based trusted achievement record system design. In: Paper Presented at the ACM International Conference Proceeding Series, pp. 46–51 (2020). https://doi.org/10.1145/3411681.3411689
McElrath, B.: Braiding the blockchain. Presentation at Scaling Bitcoin Hong Kong, 7 December 2015 (2015). https://scalingbitcoin.org/hongkong2015/presentations/DAY2/2breakingthechain1-mcelrath.pdf
Basri, W., Siam, M.R.: E-recruitment adoption strategy in the universities of Saudi Arabia. Eur. J. Bus. Manag. 8 (33), 32–43 (2016)
Behaneck, M.: Employment websites: personnel by mouse click. [Online-Jobborsen: Personal per Mausklick]. Betonwerk Und Fertigteil-Technik/Concrete Plant Precast Technol. 84 (1), 42–51 (2019)
Brown, J., Matsa, D.A.: Locked in by leverage: job search during the housing crisis. J. Financ. Econ. 136 (3), 623–648 (2020). https://doi.org/10.1016/j.jfineco.2019.11.001
Article Google Scholar
Cao, H., Do, D., Tran, V., Cao, T., Song, Y.: Synonym prediction for Vietnamese occupational skills (2022). https://doi.org/10.1007/978-3-031-08530-7_29
Chamakiotis, P., Panteli, N., Davison, R.M.: Reimagining e-leadership for reconfigured virtual teams due to Covid-19. Int. J. Inf. Manag. 60 , 102381 (2021)
Choi, K.: Gender differences in the use of social contacts in the job search process. In: Paper Presented at the 81st Annual Meeting of the Academy of Management 2021: Bringing the Manager Back in Management, AoM 2021 (2021). https://doi.org/10.5465/AMBPP.2021.181
Chou, C., Lu, T.: A hybrid-feedback recommender system for employment websites. J. Ambient Intell. Human. Comput. (2020). https://doi.org/10.1007/s12652-020-01772-y
Chou, Y., Wongso, F.R., Chao, C., Yu, H.: An AI mock-interview platform for interview performance analysis. In: Paper Presented at the 2022 10th International Conference on Information and Education Technology, ICIET 2022, pp. 37–41 (2022). https://doi.org/10.1109/ICIET55102.2022.9778999
Dabić, M., Obradović, T., Vlačić, B., Sahasranamam, S., Paul, J.: Frugal innovations: a multidisciplinary review & agenda for future research. J. Bus. Res. 142 , 914–929 (2022)
Dagnino, G.B., Picone, P.M., Ferrigno, G.: Temporary competitive advantage: a state-of-the-art literature review and research directions. Int. J. Manag. Rev. 23 (1), 85–115 (2021)
Della Piana, B., Griffith, R., Milosevic, M., Scymcyk, J.M.: Reviewing culture–innovation relationships: trends and themes from selected management journals. J. Int. Counc. Small Bus. 4 , 1–29 (2022)
Dillahunt, T.R., Israni, A., Lu, A.J.: Examining the use of online platforms for employment: a survey of U.S. job seekers. In: Paper Presented at the Conference on Human Factors in Computing Systems - Proceedings (2021). https://doi.org/10.1145/3411764.3445350
Dubovitskaya, A., Mazzola, L., Denzler, A.: Towards a trusted support platform for the job placement task (2020). https://doi.org/10.1007/978-3-030-48340-1_16
Gasparėnienė, L., Matulienė, S., Žemaitis, E.: Opportunities of job search through social media platforms and its development in Lithuania. Bus.: Theory Pract. 22 (2), 330–339 (2021). https://doi.org/10.3846/btp.2021.11055
Grant, M.J., Booth, A.: A typology of reviews: an analysis of 14 review types and associated methodologies. Health Inf. Libr. J. 26 (2), 91–108 (2009)
Gusdorf, M.: Recruitment and selection: hiring the right person (2008)
Hassan, H.M., Galal-Edeen, G.H.: From usability to user experience. In: 2017 International Conference on Intelligent Informatics and Biomedical Sciences (ICIIBMS), Okinawa, Japan, pp. 216–222 (2017)
Hamdane, A., Belhaj, N., El Hamdaoui, H., Aissaoui, K., El Bekkali, M., El Houda Chaoui, N.: Big data based architecture to bringing together graduates and recruiters: case of Moroccan University. Indonesian J. Electr. Eng. Comput. Sci. 26 (3), 1701–1709 (2022). https://doi.org/10.11591/ijeecs.v26.i3.pp1701-1709
Hassenzahl, M., Law, E.L.C., Hvannberg, E.T.: User Experience-towards a unified view. Ux Ws Nordichi 6 , 1–3 (2006)
Heggo, I.A., Abdelbaki, N.: Hybrid information filtering engine for personalized job recommender system (2018). https://doi.org/10.1007/978-3-319-74690-6_54
Henry, C., Lewis, K.V.: The art of dramatic construction: enhancing the context dimension in women’s entrepreneurship research. J. Bus. Res. 155 , 113440 (2023)
Javed, Z., Qazi, H., Khoja, S.A.: An ontology-based knowledge management model for e-recruitment utilizing MOOCs data. In: Paper Presented at the 2019 8th International Conference on Information and Communication Technologies, ICICT 2019, pp. 124–128 (2019). https://doi.org/10.1109/ICICT47744.2019.9001911
Kamaru Zaman, E.A., Ahmad Kamal, A.F., Mohamed, A., Ahmad, A., Raja Mohd Zamri, R.A.Z.: Staff employment platform (StEP) using job profiling analytics (2019). https://doi.org/10.1007/978-981-13-3441-2_30
Kaur, D., Kaur, R.: Does electronic word-of-mouth influence e-recruitment adoption? A mediation analysis using the PLS-SEM approach. Manag. Res. Rev. 46 (2), 223–244 (2023). https://doi.org/10.1108/MRR-04-2021-0322
Kim, J., Heo, W.: Artificial intelligence video interviewing for employment: perspectives from applicants, companies, developer and academicians. Inf. Technol. People 35 (3), 861–878 (2022). https://doi.org/10.1108/ITP-04-2019-0173
Kişi, N.: Exploratory research on the use of blockchain technology in recruitment. Sustainability 14 (16), 10098 (2022)
Kolekar, A.: SKYNET: a platform for maximizing career opportunities. In: Paper Presented at the 2021 International Conference on Nascent Technologies in Engineering, ICNET 2021 - Proceedings (2021). https://doi.org/10.1109/ICNTE51185.2021.9487581
Lee, D., Ahn, C.: Industrial human resource management optimization based on skills and characteristics. Comput. Ind. Eng. 144 , 106463 (2020). https://doi.org/10.1016/j.cie.2020.106463
Li, L., Peltsverger, S., Zheng, J., Le, L., Handlin, M.: Retrieving and classifying LinkedIn job titles for alumni career analysis. In: Paper Presented at the SIGITE 2021 - Proceedings of the 22nd Annual Conference on Information Technology Education, pp. 85–90 (2021). https://doi.org/10.1145/3450329.3476858
Lakhani, A.: Recommendations for recruiters with sentiment detection. In: Paper Presented at the CEUR Workshop Proceedings, vol. 2967 (2021)
Liu, H., Ge, Y.: Job and employee embeddings: a joint deep learning approach. IEEE Trans. Knowl. Data Eng. 35 , 1–12 (2022). https://doi.org/10.1109/TKDE.2022.3180593
Ma, H., Xu, Y., Ma, W., Lin, Z., Jiang, K.: A multi-field feature interaction convolutional neural network for resume recommendation. In: Paper Presented at the 2020 International Symposium on Autonomous Systems, ISAS 2020, pp. 186–191 (2020). https://doi.org/10.1109/ISAS49493.2020.9378849
Martins, N., Dominique-Ferreira, S., Lopes, C.: Design and development of a digital platform for seasonal jobs: improving the hiring process. J. Glob. Sch. Mark. Sci.: Bridg. Asia World 32 (3), 452–469 (2022). https://doi.org/10.1080/21639159.2020.1808851
Mat Saad, M.F., Listyo Nugro, A.W., Thinakaran, R., Baijed, M.: A review of artificial intelligence based platform in human resource recruitment process. In: Paper Presented at the 2021 6th IEEE International Conference on Recent Advances and Innovations in Engineering, ICRAIE 2021 (2022). https://doi.org/10.1109/ICRAIE52900.2021.9704023
Mehboob, M., Ali, M.S., Ul Islam, S., Sarmad Ali, S.: Evaluating automatic CV shortlisting tool for job recruitment based on machine learning techniques. In: Paper Presented at the Proceedings of the 2022 Mohammad Ali Jinnah University International Conference on Computing, MAJICC 2022 (2022). https://doi.org/10.1109/MAJICC56935.2022.9994112
Mehta, M., et al.: A service-oriented human capital management recommendation platform. In: Paper Presented at the SysCon 2019 - 13th Annual IEEE International Systems Conference, Proceedings (2019). https://doi.org/10.1109/SYSCON.2019.8836842
Norman, D., Miller, J., Henderson, A.: What you see, some of what’s in the future, and how we go about doing it: HI at Apple Computer. In: Conference Companion on Human Factors in Computing Systems, p. 155 (1995)
Okolie, U.C., Irabor, I.E.: E-recruitment: practices, opportunities and challenges. Eur. J. Bus. Manag. 9 (11), 116–122 (2017)
Saini, A., Rusu, F., Johnston, A.: PrivateJobMatch: a privacy-oriented deferred multi-match recommender system for stable employment. In: Paper Presented at the RecSys 2019 - 13th ACM Conference on Recommender Systems, pp. 87–95 (2019). https://doi.org/10.1145/3298689.3346983
Sarath, C., Sandhya, G.: A qualitative approach to study the perception of job seekers towards digital job portals. Int. J. Bus. Innov. Res. 29 (1), 32–46 (2022). https://doi.org/10.1504/ijbir.2022.125668
Salehi, B., Kazimipour, B., Baldwin, T.: Differences in language use: insights from job and talent search. In: Paper Presented at the ACM International Conference Proceeding Series (2019). https://doi.org/10.1145/3372124.3372127
Shan, T.C., Hua, W.W.: Taxonomy of Java web application frameworks. In: 2006 IEEE International Conference on e-Business Engineering (ICEBE 2006), Shanghai, China, pp. 378–385 (2006). https://doi.org/10.1109/ICEBE.2006.98
Smaldone, F., Ippolito, A., Lagger, J., Pellicano, M.: Employability skills: profiling data scientists in the digital labour market. Eur. Manag. J. 40 (5), 671–684 (2022). https://doi.org/10.1016/j.emj.2022.05.005
Snyder, H.: Literature review as a research methodology: an overview and guidelines. J. Bus. Res. 104 , 333–339 (2019)
Verschuere, B., Brandsen, T., Pestoff, V.: Co-production: the state of the art in research and the future agenda. VOLUNTAS: Int. J. Voluntary Nonprofit Org. 23 , 1083–1101 (2012)
Wickramasinghe, H.C.P., Thebuwana, T.D., Wijesinghe, G.K.H.S., Dissanayake, U.N., Kodagoda, N., Suriyawansa, K.: Digital platform to empower the self-employment in Sri Lanka. In: Paper Presented at the Proceedings of 6th International Conference on Information Technology Research: Digital Resilience and Reinvention, ICITR 2021 (2021). https://doi.org/10.1109/ICITR54349.2021.9657410
Xu, C., Mewburn, I., Grant, W.J., Suominen, H.: PostAc®: a visual interactive search, exploration, and analysis platform for Ph.D. intensive job postings. In: Paper Presented at the ACL 2019 - 57th Annual Meeting of the Association for Computational Linguistics, Proceedings of System Demonstrations, pp. 43–48 (2019)
Yujun, Y., Yimei, Y., Wang, Z., Wei, L., Liyun, L., Debin, H.: Research on high-quality employment of college students based on big data technology and artificial intelligence. In: Paper Presented at the 2022 19th International Computer Conference on Wavelet Active Media Technology and Information Processing, ICCWAMTIP 2022 (2022). https://doi.org/10.1109/ICCWAMTIP56608.2022.10016596
Yazdanian, R., West, R., Dillenbourg, P.: Keeping up with the trends: analyzing the dynamics of online learning and hiring platforms in the software programming domain. Int. J. Artif. Intell. Educ. 31 (4), 896–939 (2021). https://doi.org/10.1007/s40593-020-00231-1
Vermeeren, A.P., Law, E.L.C., Roto, V., Obrist, M., Hoonhout, J., Väänänen-Vainio-Mattila, K.: User experience evaluation methods: current state and development needs. In: Proceedings of the 6th Nordic Conference on Human-Computer Interaction: Extending Boundaries, pp. 521–530 (2010)
Zhang, Z., Luo, Y., Wen, Y., Zhang, X.: CycleResume: a cycle learning framework with Hybrid attention for Fine-grained talent-job fit (2022). https://doi.org/10.1007/978-3-031-20503-3_21
Zhang, W., Hu, F., Xie, Q.: Design, development and application of big data platform on provision of job information search services for students in vocational college. In: Paper presented at the Proceedings - 2018 7th International Conference of Educational Innovation through Technology, EITT 2018, pp. 180–183 (2018). https://doi.org/10.1109/EITT.2018.00043
Zhou, Z., Zhou, X., Li, M., Song, Y., Zhang, T., Yan, R.: Personalized query suggestion with searching dynamic flow for online recruitment. In: Paper Presented at the International Conference on Information and Knowledge Management, Proceedings, pp. 2773–2783 (2022). https://doi.org/10.1145/3511808.3557416
Download references
Author information
Authors and affiliations.
3CLab-Cross Cultural Competence Learning and Education, Department of Management and Innovation Systems, University of Salerno, Via Giovanni Paolo II, 132, Fisciano, Italy
Chiara Signore, Bice Della Piana & Francesco Di Vincenzo
You can also search for this author in PubMed Google Scholar
Corresponding author
Correspondence to Chiara Signore .
Editor information
Editors and affiliations.
Faculty of Mathematics, Physics and Informatics, Comenius University, Bratislava, Slovakia
Zuzana Kubincová
University of L'Aquila, L'Aquila, Italy
Federica Caruso
Universitetet i Tromsø (UiT) – Norges arktiske universitet, Tromsø, Norway
Tae-eun Kim
Technical University of Sofia , Sofia, Bulgaria
Malinka Ivanova
Department of Life, Health and Environmental Sciences, University of L'Aquila, Coppito, Italy
Loreto Lancia
Department of Computer Science, University of Salerno, FISCIANO, Salerno, Italy
Maria Angela Pellegrino
Rights and permissions
Reprints and permissions
Copyright information
© 2023 The Author(s), under exclusive license to Springer Nature Switzerland AG
About this paper
Cite this paper.
Signore, C., Della Piana, B., Di Vincenzo, F. (2023). Digital Job Searching and Recruitment Platforms: A Semi-systematic Literature Review. In: Kubincová, Z., Caruso, F., Kim, Te., Ivanova, M., Lancia, L., Pellegrino, M.A. (eds) Methodologies and Intelligent Systems for Technology Enhanced Learning, Workshops - 13th International Conference. MIS4TEL 2023. Lecture Notes in Networks and Systems, vol 769. Springer, Cham. https://doi.org/10.1007/978-3-031-42134-1_31
Download citation
DOI : https://doi.org/10.1007/978-3-031-42134-1_31
Published : 29 August 2023
Publisher Name : Springer, Cham
Print ISBN : 978-3-031-42133-4
Online ISBN : 978-3-031-42134-1
eBook Packages : Intelligent Technologies and Robotics Intelligent Technologies and Robotics (R0)
Share this paper
Anyone you share the following link with will be able to read this content:
Sorry, a shareable link is not currently available for this article.
Provided by the Springer Nature SharedIt content-sharing initiative
- Publish with us
Policies and ethics
- Find a journal
- Track your research
Academia.edu no longer supports Internet Explorer.
To browse Academia.edu and the wider internet faster and more securely, please take a few seconds to upgrade your browser .
Enter the email address you signed up with and we'll email you a reset link.
- We're Hiring!
- Help Center
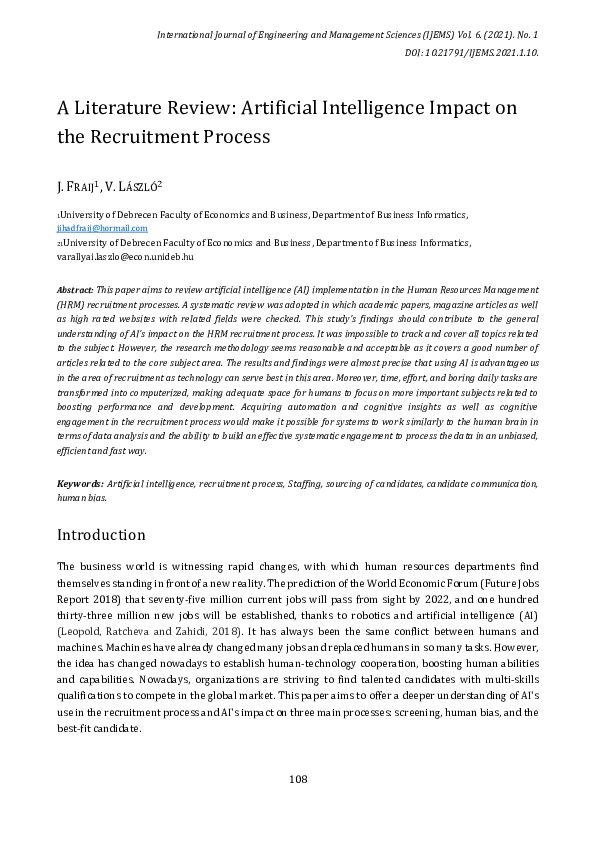
literature Review: Artificial Intelligence Impact on the Recruitment ProcessA LITERATURE REVIEW: ARTIFICIAL INTELLIGENCE IMPACT ON THE RECRUITMENT PROCESS

2021, International Journal of Engineering and Management Sciences
This paper aim is to review the implementation of artificial intelligence (AI) in the Human Resources Management (HRM) recruitment processes. A systematic review was adopted in which academic papers, magazine articles as well as high rated websites with related fields were checked. The findings of this study should contribute to the general understanding of the impact of AI on the HRM recruitment process. It was impossible to track and cover all topics related to the subject. However, the research methodology used seems to be reasonable and acceptable as it covers a good number of articles which are related to the core subject area. The results and findings were almost clear that using AI is advantages in the area of recruitment as technology can serve best in this area. Moreover, time, efforts, and boring daily tasks are transformed to be computerized which makes a good space for humans to focus on more important subjects related to boosting performance and development. Acquiring a...
Related Papers
International Journal of Scientific & Technology Research
Nishad Nawaz
This paper aims to review applications of artificial intelligence (AI) in the recruitment process and their practical implications as reported by the systemic review of 7 referred articles, 5 magazines articles and 20 websites viewpoints of the topic and related areas were considered. The study findings contribute to a more holistic view of the topic and related area's and thus complement the study of AI based recruitment process. The study may not have taken a complete coverage of all articles in the area of artificial intelligence role in recruitment process. Yet, based on the selection on the chosen research methodology it seems reasonable to assume that the review process covered a large published in web portals / reports / academic journals. As per the best of author knowledge, there is no systematic literature review on artificial intelligence role in recruitment process has previously been published in academic journals and biased on selected papers a number of research questions are planned aimed at guiding and suggest some promising avenues for future research.
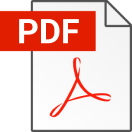
Zenodo (CERN European Organization for Nuclear Research)
Dr. Ansha Gupta
International Journal of Advanced Trends in Computer Science and Engineering
The paper discussed on artificial intelligence (AI) inference in human resource management, especially in the recruitment process. The study aim is to know artificial intelligence swapping human involvement in the recruitment process in selected software companies in India. To get valid data, the study designed structure questionnaire for different designations of 138 HR professionals and also used convenience sampling, coefficients beta (β), cronbach's alpha, t test and descriptive statistics test were used. The study found that artificial intelligence has a positive on human replacement in the recruitment process. Meanwhile, the study is expected to help studied organizations to formulate recruitment strategies and policy interferences to align to develop its effective recruitment process to recruit qualified talent into team to encounter competitive business and to develop sustainable environment.
Supriya Poduval
As the Indian corporate sector is moving towards digitization, one observes an immense potential for the utilization of artificial intelligence in the human resource department, especially in recruitment. This research paper will be analyzing the future applications of Artificial Intelligence (AI) in the recruitment process and also, how this technology can/will reshape the 'human resources department' (HRD) holistically. The analysis will also be done in order to explore the strength and weakness of the existing corporate sector, to be more precise, the HRD dynamics in accepting AI. The research is done with a future orientation kept in mind, wherein the forecasting is done more on the basis of the current requirements of AI.
Artificial Intelligence is used for various business processes including hiring employees. The purpose of this article is to assess the flip-side of Artificial Intelligence applied in recruitment software. Its insufficiency of delivering the expected results in terms of the right match for the hiring, difficulty in language processing. The paper reviews the literature available to understand the principles of Socio-technical systems design requirements and on Artificial Intelligence's usage in the recruitment process. The research is qualitative, has followed the phenomenology approach, and uses the interview technique for understanding the opinions of the users. The results reveal that, though AI in recruitment provides ease in searching the candidate's barriers in language and recognition, low turn-in ratio, incorrect recommendations due to Data inadequacy, skepticism among HR professionals due to lack of human intelligence, need for budgets for acquiring and training are ...
Andrea Rezzani
The problem I intend to address in my industrial PhD is the lack of data regarding the acceptance and experience of users involved in the transformation of recruitment and selection processes as a result of the hypothetical, but now quite realistic, application of Artificial Intelligence. The program aims to bring advanced research into an industrial environment within an IT company. It aims to explore theoretical issues about the level of acceptance towards AI technologies and human/artificial factors involved in decision making, including associated biases and ethical concerns. Here, I present an overview of the literature conducted during the first year of my doctoral program and a preliminary study regarding candidates' perceptions of initiating a computer-based selection process. Finally, I discuss some future research that I would like to develop over the course of my upcoming studies.
Asian Journal of Social Sciences and Management Studies
Faiyaz Iqbal
Advances in human resources management and organizational development book series
Filiz Mızrak
Proceedings of the 6th International Scientific and Practical Conference «Recent Scientific Investigation»
ismail karakulle
Technological developments in today's world have forced organizations to keep up with change, as in all areas of life. Organizations need to adapt to technological developments in order to ensure their sustainability and gain competitive advantage. Artificial intelligence applications have become the main priorities of organizations in order to keep up with technological developments and distinguish themselves from their competitors[1]. Human resource management has a strategic importance for organizations. Although the technology used by the organizations, the goods and services they produce can be imitated by their competitors, one of the most important resource that cannot be imitated is human resources. This situation increases the importance that organizations attach to human resources management. With the effect of globalization, organizations do not continue their activities limited only to the certain region or geography they are in. In this respect, the qualified and competent human resources of the organizations located in many different regions and geographies can be obtained by the competitors of the organizations. Organizations that aware of the importance of the technology and want to achieve their future goals without being behind the times benefit from many innovations provided by artificial intelligence. The use of artificial intelligence in human resources management provides many advantages to organizations. Such as selection of the suitable employees for the right position, the workload of the employees is reduced, allowing them to gain new gains for their qualifications and competencies. Employing talented employees enables fewer mistakes to be made in the work processes and reduces the additional cost that the organization will bear. It also contributes to the minimization of prejudiced attitudes and behaviors in decision processes by ensuring the integration of workflows between different units within the organization[2]. Thanks to artificial intelligence algorithms in the recruitment processes, it analyzes the resumes of the candidates from the candidate pool of the organization, scores their experiences, knowledge and experience, qualifications and provides data to human resources managers in a short time[1]. Thus, the supply of human resources necessary for the organization is effectively ensured. Organizations provide various trainings in order to improve the competencies of their employees and to increase their organizational performance. Giving the wrong training means loss of time and cost for the organization. With artificial intelligence applications, the trainings received by the employees in the past are analyzed and the most appropriate training program is revealed for newly hired employees[3]. The use of artificial intelligence enables the minimization of problems in human resources management and the creation of employee experience. The main goal of artificial intelligence is to provide convenience to employees in their work and to enable them to be competent and efficient. Artificial intelligence applications determine the competencies of the employees and provide support to the employees in the organization to draw a roadmap in their career preferences[4]. Artificial intelligence plays a critical role in providing competitive advantage for organizations. The usage areas of artificial intelligence in human resources management will be used more widely in the future compatible with the technological advances. According to the Eightfold AI’s report, HR leaders have been already using artificial intelligence for human resources functions like employee records management, payroll processing and benefits administration, recruitment and hiring process, performance management and onboarding new employees[5].
IAEME Publication
An industry or a business or a firm requires quality personnel for accomplishment of objectives framed by them in order to survive in this competitive era. They all are in the beginning of fourth industrial revolution. To remain competitive in this digital world all search for bright, potential and dynamic employees. Organizations with an effective recruitment strategy will be able to employ suitable individual in order to manage the digital world and developing business environment. So the recruitment strategy is the prime factor for every organization in hiring skilled employees who could be more efficient and effective in accomplishing the job objectives. The recruitment strategy as it is a major function of organization apparently takes help of data analysis for decision making process. The data analysis is known as " Artificial Intelligence " which plays a crucial role in recruitment decision. Artificial intelligence in a most basic terminology and is a human develop intelligent machines. AI will work and react like human and its ultimate goal is to facilitate computers to carry out the work as normally done by people. AI leads with an incredible speed and accuracy. The major objective of this paper is to study how Artificial Intelligence influences the recruitment strategy. The study also throws light on the techniques used by companies in AI while recruiting. This study is entirely done based upon secondary sources of information like conceptual papers, various peer reviewed journal articles, books and websites are used to further explore the concept.
RELATED PAPERS
Luiz Guilherme Marinoni
Hilmi S. Salem (Prof. Dr.)
Teologia Brasileira / Bereianos | Apologética e Teologia Reformada
Franklin Ferreira
Journal of Personality
Stewart Donaldson
Heikki Jalli
ELIDA HAFNI
Land Use Policy
Murat Yücekaya
달림포차 광양오피【 DȺLPØChȺ 4ㆍnEt 】광양휴게텔≞광양오피
dmz okocewakaf
Matheus Rumetna
Peter Ullrich
Asian Journal of Surgery
Hirofumi Fujii
International Journal of Basic Science in Medicine
Majid Darroudi
Journal of Medicinal Chemistry
Gaurav Bharadwaj
Sports Orthopaedics and Traumatology Sport-Orthopädie - Sport-Traumatologie
Brenda Laky
neonbox huruf timbul tegal
Cecillia Juneta
G Graaf, de
Archives of Clinical Infectious Diseases
Saham Ansari
Sustainability
MARIA DE LOS DOLORES VALADEZ SIERRA
Amilcar Torrão Filho
Eloi Magalhaes
Aboriginal History
Lyndall Ryan
Journal of physics
Ika Apriyani
Santé Publique
Nathalie Baunot
RELATED TOPICS
- We're Hiring!
- Help Center
- Find new research papers in:
- Health Sciences
- Earth Sciences
- Cognitive Science
- Mathematics
- Computer Science
- Academia ©2024
Ohio State navigation bar
- BuckeyeLink
- Search Ohio State

Recruitment Updates to Enhance Manager and Candidate Experiences
As we continually work to improve the experience for hiring teams and job applicants, the latest Workday changes are designed to optimize our processes and elevate the services provided by the Human Resources talent acquisition team. These changes improve how we screen applicants and welcome new employees.
Applicant Screening
Starting May 9, talent acquisition consultants/recruiters – a community of skilled, trained colleagues who support the experience for hiring teams and job seekers – will initially screen applicants in Workday prior to hiring teams being able to view applicants. Hiring teams (i.e., manager, manager liaison, hiring team and search committee security role) will only view applicants after the TA Consultant has completed the initial screening process.
Recruiters will identify applicants who meet the position and salary requirements and present a more qualified pool for the hiring team to review. This frees up the hiring team to focus on reviewing applicants aligned with the qualifications for the job. It also helps to ensure compliance with hiring regulations and tracking of candidates for audit purposes.
Offer Letters
Offer letters have been updated to better reflect the culture and brand of Ohio State and highlight important information for new employees.
Candidate Notifications
The disposition process will soon notify applicants who are not selected for a position during the process, not just when a final candidate is selected, giving them real-time updates in Workday.
If you have questions about the recruiting process, please work with your talent acquisition consultant or contact HR Connection .
Share this page

HR Focus News
Staff professional development funds, ohio state listening survey, job posting process and review changes integrated with workday, new benefits and pay dashboard in workday, recent hr news, bright horizon benefits support family and personal wellbeing, outstanding buckeyes recognized for impactful focus on diversity and inclusion, ohio state voted columbest top employer, nepotism policy revision, paid time off policy minor revision.
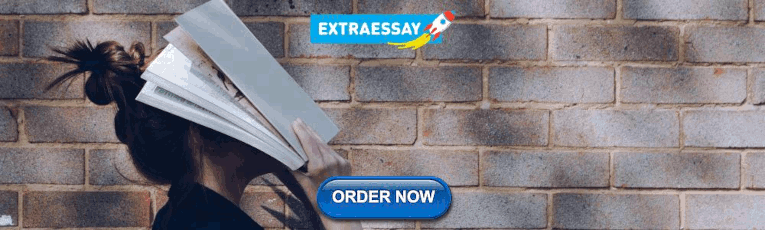
IMAGES
VIDEO
COMMENTS
Keywords: Recruitment and Selection, Literature Review, Content Analysis, Strategic Staffing, Competitive Advantage. ... Recruitment and selection is the process of bringing human resources into ...
Summary of key research findings in recruitment and selection. A systematic, fully comprehensive literature review of extent selection and recruitment literature is beyond the scope of this paper - rather, we focus our effort on recent meta-analyses as well as conceptual and literature review papers to identify the meta-trends in the recruitment and selection research.
recruitment and selection practices to help refresh concepts and understanding for researchers on the topic. 2. Literature Review 2.1. Recruitment Recruitment is a decision of human resource management planning regarding the number of employees needed, when needed, as well as the criteria for what is needed in an organisation.
Strategic human resource management (SHRM) research increasingly focuses on the performance effects of human resource (HR) systems rather than individual HR practices (Combs, Liu, Hall, & Ketchen, 2006).Researchers tend to agree that the focus should be on systems because employees are simultaneously exposed to an interrelated set of HR practices rather than single practices one at a time, and ...
Literature review 2.1 Employee recruitment. ... Many human resources manager leverage on relationship with colleagues and social networks in the bid to attract quality talents. Findings also aligned with the equity theory, fairness to employee, employee compensation, etc., are key in attracting and retaining quality talents. ...
Literature was collected from 40 articles of a reputed journal from 2010 to 2018. Main findings: The review of literature revealed that the recruitment and selection process is carried out in organizations by adopting latest technologies like online portals, outsourcing, job fair, campus interviews, and mobile recruitment applications.
Over the last thirty years, the amount of research on recruitment topics has increased dramatically. Despite this increase, recent reviews of the recruitment literature often have had a somewhat pessimistic tone. Reviewers have concluded that we still do not know a great deal about why recruitment activities have the effects they do.
Keywords: E-recruitment Conceptualization Literature review Grounded Theory Methodology 1 Introduction E-recruitment has many labels that include; internet recruitment, online recruitment, web-recruitment and many others. Unlike traditional recruitment, e-recruitment makes use of information technology to handle the recruitment processes.
Keywords: Recruitment and Selection, Literature Review, Content Analysis, ... Recruitment and selection is the process of getting human resources into the organizations' departments, sections, and ...
3.1 E-recruitment as a Technology Tool. E-recruitment as a technology tool is a conceptualization of e-recruitment as a technical artefact [].This means is demonstrated by Faliagka et al. [] who presented a tool to automate the ranking of applicants in recruitment.3.2 E-recruitment as a System. Studies that view e-recruitment as a system conceptually divide e-recruitment into independent but ...
To achieve this objective, this study performs a systematic literature review and content analysis of 93 papers from 75 journals. The main results of the research show that digital trends resulting from Industry 4.0 affect the field of HRM in 13 different themes, promoting trends and challenges for HRM, the workforce, and organizations.
This paper aim is to review the implementation of artificial intelligence (AI) in the Human Resources Management (HRM) recruitment processes. A systematic review was adopted in which academic papers, magazine articles as well as high rated websites with related fields were checked.
A systematic, fully comprehensive literature review of extent selection and recruitment literature is beyond the scope of this paper - rather, we focus our effort on recent meta-analyses as well as conceptual and literature review papers to identify the meta-trends in the recruitment and selection research.
A recent review maps the different tasks of HR practices to HR analytics tools and discusses how these tools can influence the organizational return on investment (ROI). The review shows that HR predictive analytics in workforce planning and recruitment have the highest effect on organizational ROI (similar conclusions are shown in a report by ...
This systematic literature review addresses the intersection of AI and HRM, elucidating AI-driven tools and techniques that optimize recruitment, p rformance man gement, and employee engagement. oot d in the recogn tio that conv ntional HRM approach s can be time-intensive and bias d, AI's integration promises to enhance decision-making ...
The purpose of this paper is to shed light on the new E-recruitment trend that is pervading the lives of job seekers, included students, and job offers. A semi-systematic literature review on digital job searching and recruiting platform in the last five years was conducted with the aim to develop a preliminary conceptual framework. Following a ...
The Human Resources (HR) concept has undergone significant changes in how it is viewed as a capability in modern industry. The study of HR is fraught with disagreement regarding its origin as well as laden with discourse on the implications for contemporary management. Drucker (1954) created the term "human resources" in his seminal work . The
Abstract. Research on human resources (HR) indicates that many biases (e.g., halo effect, confirmation bias, stereotyping bias) affect decisions taken by HR employees. However, it remains unclear whether HR employees are aware of their susceptibility to bias. To improve understanding, this study examines the "bias blind spot" phenomenon ...
This paper aim is to review the implementation of artificial intelligence (AI) in the Human Resources Management (HRM) recruitment processes. A systematic review was adopted in which academic papers, magazine articles as well as high rated websites ... (Nawaz, 2019b) stated that the AI title in the recruitment process lakes literature review ...
Recruitment Updates to Enhance Manager and Candidate Experiences. ... applicants who meet the position and salary requirements and present a more qualified pool for the hiring team to review. This frees up the hiring team to focus on reviewing applicants aligned with the qualifications for the job. ... Human Resources. 1590 North High St ...
Review Vacancy. Date Posted 05/15/24. Applications Due 06/05/24. Vacancy ID 158675. Basics; Schedule; Location; ... Be eligible for transfer via Section 70.4 of the Civil Service Law to a Human Resources Specialist Trainee 1. To be eligible for Section 70.4 transfer, the candidate must have a Bachelor's degree and status on either the PCO or ...
Duties Description Performs and supervises the performance of various human resources activities such as staffing services, which consist of recruitment, examination, and placement activities; position classification and compensation; and employee services such as employee benefit, health and safety, and employee recognition to build and maintain a workforce that is effective in accomplishing ...