- Search Menu
- Sign in through your institution
- Advanced Articles
- Editor's Choice
- Author Guidelines
- Publish with us
- Submission Site
- Open Access
- Self-Archiving Policy
- About Clean Energy
- About the National Institute of Clean and Low-Carbon Energy
- Editorial Board
- Instructions for Reviewers
- Advertising & Corporate Services
- Journals Career Network
- Journals on Oxford Academic
- Books on Oxford Academic

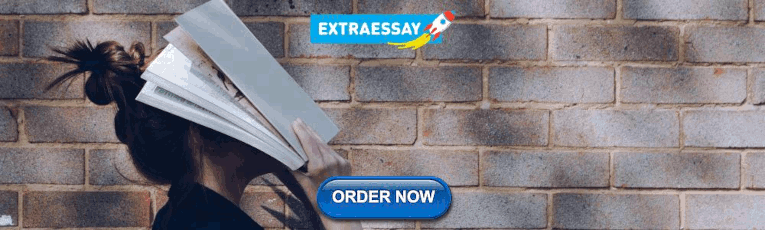
Article Contents
Introduction, 1 methods and challenges addressed, 2 ai techniques, 3 application of ai in the power sector, 4 conclusions, 5 future scope, authors’ contributions, conflict of interest statement, data availability.
- < Previous
Applications of artificial intelligence in power system operation, control and planning: a review

- Article contents
- Figures & tables
- Supplementary Data
Utkarsh Pandey, Anshumaan Pathak, Adesh Kumar, Surajit Mondal, Applications of artificial intelligence in power system operation, control and planning: a review, Clean Energy , Volume 7, Issue 6, December 2023, Pages 1199–1218, https://doi.org/10.1093/ce/zkad061
- Permissions Icon Permissions
As different artificial intelligence (AI) techniques continue to evolve, power systems are undergoing significant technological changes with the primary goal of reducing computational time, decreasing utility and consumer costs and ensuring the reliable operation of an electrical power system. AI techniques compute large amounts of data at a faster speed than numerical optimization methods with higher processing speeds. With these features, AI techniques can further automate and increase the performance of power systems. This paper presents a comprehensive overview of diverse AI techniques that can be applied in power system operation, control and planning, aiming to facilitate their various applications. We explained how AI can be used to resolve system frequency changes, maintain the voltage profile to minimize transmission losses, reduce the fault rate and minimize reactive current in distributed systems to increase the power factor and improve the voltage profile.

The demand for advanced research and technology has steadily increased in the sector of electric grids [ 1 ]. Automation and intelligent technology have become widely used in response to development demands over time. Traditional research methods are quickly becoming insufficient to enable data scientists and researchers to keep up with any global challenges that artificial intelligence (AI) may be able to assist us in solving and uncovering important insights among the billions of pieces of data scattered throughout power systems. AI can handle large amounts of data and utilize them to make power system operations, control and planning more efficient. The use of AI technology in power systems has been investigated and debated in related areas and has resulted in more study material and certain outcomes, which are reviewed in this paper. The demand for advanced research and technology has constantly risen in the sector of electricity grids. The application of AI technology to the automation of power system control can improve the efficiency of electrical automation management, mitigate the risk of accidents and ensure smooth operation of the power system over an extended period [ 2 ]. Evaluating the use of AI technology in power systems requires a comprehensive analysis of existing research in the field of artificial intelligence and its related industries.
The power system is a network consisting of three components: generation, distribution and transmission. In the power system, energy sources (such as coal, sunlight, wind, nuclear reactions and diesel) are transformed into electrical energy [ 3 ]. There are different power systems, such as solar power systems, wind power systems, thermal power plants [ 4 ], nuclear power plants, geothermal power plants, etc. All power systems have different structures and equipment for the generation of electricity [ 5 ]. The basic structure of a power system includes:
(i) generating substation;
(ii) transmission substation;
(iii) sub-transmission substation;
(iv) distribution substation.
In power system problem-solving, conventional approaches such as practical numerical optimization methods (e.g. lambda iteration and Newton–Rapson methods) have been used. Optimization problems are non-linear and, with the various constraints included, these optimization problems become slow and complex. So, several AI techniques are discussed here to solve many optimization issues with less computation time. Furthermore, experiments were conducted to identify which backpropagation algorithm would give the most efficient and reliable network training [ 6 ]. The systematic approach was introduced [ 7 ] for developing a recurrent neural network (RNN) that could effectively predict the dynamic behaviour of a pilot-scale entrained-flow gasifier. The RNN was trained using a data set consisting of input and output data collected from a dynamic read-only memory (ROM) system established in a previous study. To evaluate the accuracy of the RNN, comparisons were made with computational fluid dynamics models and experimental data obtained from the pilot-scale gasifier. The findings demonstrated that the RNN surpassed the performance of the dynamic ROM model, showing strong predictive capabilities to capture the transient behaviour of the gasifier. Additionally, this was achieved while significantly reducing the computational resources required. Power systems are one of the main study topics for the advanced development of AI. The use of AI algorithms in power plants has been under continual investigation since the advent of the expert system technique [ 8 ]. However, in most situations, issues such as long cycle times, complex computation and difficulty in learning arise with classic AI methods. In recent years, efficiency has increased significantly with the continued advancement of AI algorithms. The multi-source model of heterogeneous large data has gradually developed, the data volume continues to grow, and new possibilities and problems are being created with the use of AI in power systems. AI encompasses several technologies such as expert systems, pattern recognition, genetic algorithms (GAs) and neural networks. By incorporating AI into the automation of power system control, it has the potential to enhance the efficiency of electrical automation management, mitigate the risk of accidents and ensure long-term smooth operation of the power system. Machine learning (ML) has also found extensive applications in predicting the properties of rechargeable battery materials, particularly electrolyte and electrode materials, as well as the development of novel materials, according to relevant research. The breadth of ML applications will grow steadily as ML technology advances and new unique issues emerge in the research of rechargeable battery material [ 9 ]. Although ML has shown considerable potential in modelling complex systems, its implementation introduces new challenges. These challenges include difficulties associated with accessing relevant and reliable data sets, and addressing inaccuracies in model predictions needs to be addressed before ML can be widely deployed. To effectively implement ML on a large scale, it is crucial to have compelling evidence of its effectiveness in diverse areas such as manufacturing processes, energy generation, storage and management. Furthermore, the availability of commercial software and a skilled workforce specialized in the relevant domains is essential [ 10 ]. ML has proven to be beneficial in creating data-driven models that accurately correlate material properties with catalytic performance, including activity, selectivity and stability. As a result, there have been advancements in the development of effective design and screening criteria for solid-state catalysts with desired properties [ 11 ]. Nevertheless, there are still difficulties in applying existing ML algorithms to accurately predict catalyst performance or devise strategies for designing high-performance catalysts. The review emphasizes recent advancements in ML applied to solid heterogeneous catalysis, as well as the limitations and constraints faced by ML in this field, and also discusses some of the prospects for using ML effectively in the design of solid heterogeneous catalysts. Successful uses of ML in short-term hydrothermal scheduling will strengthen the link between real operations and issue formulation, and prepare the hydropower sector for autonomy by identifying the need for and availability of autonomous systems now and in the future. In this research, a review of the state of the art of ML applications for the hydroelectric sector was offered [ 12 ]. Digital technologies have a significant impact on energy market services and the safety of residents and energy consumers, particularly in smart homes. Sustainable smart home networks can improve energy efficiency, utilize local renewable energy, decarbonize heating and cooling systems, and promote responsible electric vehicle charging [ 13 ]. The next decade is crucial for achieving ambitious global CO 2 reduction targets and the decarbonization of buildings is a major challenge. Water-efficient development and resilient homes are essential for coping with impacts of climate change. Research on sustainability and energy efficiency is vital to improve the quality of life in the face of climate change [ 14 ]. Several important aspects were highlighted [ 15 ] with respect to the current state and prospects of smart homes. It was recognized that despite the increasing prevalence of smart homes and the growing familiarity with them, there were still significant obstacles that researchers must address to achieve widespread adoption [ 16 ].
One of the technical hurdles highlighted is the diversity of manufacturers and devices, each with varying charging systems, frequencies and communication methods [ 17 ]. This fragmentation can hinder interoperability and compatibility between devices and systems. The greater acceptance of smart home technologies poses a significant challenge. This review highlights the crucial task of convincing consumers about the safety and reliability of these technologies. An approach [ 18 ] was presented to develop prediction models that were capable of identifying faults and malfunctions in power equipment, demonstrating their effectiveness in predicting the progression of degradation phenomena. The challenges were discussed [ 19 ] associated with predicting the technical condition index of the equipment and determining the probability of its current state having defects. This research contributes significantly to the advancement of predictive analytics tools in the industry, enabling proactive maintenance of equipment. ML and data-driven approaches exhibit significant promise in the field of predictive analysis within power systems, especially in the context of smart grids. These methods can efficiently analyse the vast amounts of data collected from smart meters and other devices in real time, facilitating optimized energy flow in an increasingly renewable-energy-focused landscape [ 20 ]. They offer advantages such as improved accuracy, cost reduction and improved efficiency. However, certain challenges must be overcome, such as ensuring the availability of high-quality data and managing the potential risk of information overload [ 21 ].
The articles selected for review are based on different parameters and selection criteria. The shortlist is based on parameters such as duration, analysis, comparison and applications, as listed in Table 1 . The challenges investigated for power system operations, control and planning in the article are as follows and a diagram visualizing the domains of the power sector along with the AI techniques used and their application is presented as Fig. 1 . Power system operation [ 22 ] includes the total power requirement that must reliably meet the real-time generation, including transmission losses. The problems involved in this task are economic load dispatch (ELD), power flow, unit commitment and generator maintenance schedule.
Selection criteria for shortlisted research papers

Visual depiction of power sector domains, their application and AI techniques used
The complex and large design of the power system is presented [ 23 ] and interferences in the power system are a problem. When a large interference occurs, control tasks are needed to find the disturbed area, control the impact caused and bring the process to normal form. Heuristic solutions are non-linear and hence are not designed to deal with fast-occurring disturbances. Therefore, many control optimization techniques such as voltage control (VC), power system stability control and load frequency control are discussed to address this problem.
Power system planning has an arrangement of a power system that is complex and large with many parts such as flexible alternating current transmission system (FACTS) devices and distribution systems. The major goal of least-cost planning is to optimize the components required to deliver enough power at a minimal cost. Many factors such as FACTS placement and demand are given importance in the expansion of power system planning. Reactive power optimization, distribution system planning (DSP) [ 24 ] and capacitor placement are the optimization problems considered in this task [ 25 ].
2.1 Artificial neural network
In AI, a set of inputs is transformed into an output using a network of neurons. A neuron produces a single power by simply operating its input in the same way as a processor [ 26 ]. The working group of neurons and the pattern of their connections may be utilized to build computers with real-world issues in model recognition and pattern categorization. As the human brain processes are replicated, input signals are processed using mathematical operations utilizing artificial neurons.
The network consists of neurons organized in layers and connected to ensure information input–output flows [ 27 ]. By using what is known as the activation function, in layer ‘i’, each neuron is linked to the ‘i+1’ layer of all neurons. The input signals for a specific neuron originate from all neurons in the prior layer and their excitation power changes to govern the degree of signal reaching each neuron [ 28 ].
In several scientific disciplines, such as medical diagnosis, voice, pattern recognition, etc., artificial neural networks (ANNs) are utilized. The ANN is a computing system partly based on biological neural networks, expressed by linked nodes (artificial neurons), correctly structured in layers that are found in human or animal brains. All artificial neurons are linked and are able, employing their connections (synapses) to send signals, generally real values, which result in an output computed according to the original input, depending on the sizes allocated to all neurons [ 29 ].
ANNs are recognized as data-mining approaches capable of modelling several independent characteristics with dependent functions in non-linear functions. ANNs may predict a future value of a dependent variable after training with a comparable sample, replicating the learning process of a human brain [ 30 ].
In turn, a difference in signal strength affects the activation of the neuron and, as a result, signals that are transmitted to other neurons as shown in Fig. 2 .

Simplified diagram of the artificial neural network
Input layer—distribute other units but does not process the data.
Hidden layer—the ability to map the non-linear problems is provided through hidden layers.
Output later—the output units encode the value to be assigned to this instance.
2.2 Adaptive neuro-fuzzy interference system
The adaptive neuro-fuzzy interference system (ANFIS) creates an input/output data set whose membership function parameters are modified with the minimum square method type or the backpropagation algorithm by itself, using a fuzzy interference system [ 31 ]. This modification has helped the fuzzy system learn from the data it models. By applying hybrid learning, ANFIS utilizes a systematic approach to determine the optimal distribution of membership functions, enabling effective mapping of the relationship between input and output data [ 32 ]. The ANFIS architecture combines ANNs with fuzzy logic, making the modelling process more structured and less dependent on expert knowledge. This inference system is constructed using five layers in its basic form. Each ANFIS layer has several nodes defined in the layer specification using the node function. The current layer inputs from the preceding layer nodes are collected. The structure of the ANFIS is shown in Fig. 3 [ 33 ].

Simplified diagram of an artificial neuro-fuzzy interference system
The fuzzy-inference method involves organizing empirical information in a professional manner, which presents challenges in quantifying it through membership functions (MFs) and fuzzy rule bases [ 34 ]. Additionally, neural networks possess learning capabilities. From top to bottom, they are very adaptable in their system set-up and have great parallel processing and fault tolerance. The theories for neural network neuro-fuzzy systems are actively explored in several areas [ 35 ].
The utilization of a neuro-fuzzy system, which emulates human learning and decision-making abilities, can lead to varying model performances compared with traditional mathematical approaches. The process of rule generation and grouping in a neuro-fuzzy inference system, adapted to the specific model, can be approached through a grid-based methodology, known as the ANFIS.
2.3 Fuzzy logic
To identify the fuzzy set from which the value comes and the degree of membership within that set, fuzzy logic systems base their choices on input in terms of variables generated from the member functions [ 36 ]. The variables are then combined with IF–THEN language requirements (fuzzy logic rules) and a fluid implication is used to answer each rule [ 37 ]. The response of each rule is weighted according to the confidence or degree of the inputs of each rule and the central part of answers is computed to provide a suitable output and achieve the compositional rule of deference. Now, the design of fuzzy logic systems is not a systematic approach. The easiest way is to subjectively define member functions and rules with a human-operated system or an existing controller and then test the design for the right output. If the design fails the testing, the MFs and/or rules should be changed. Recent investigation directions involve the creation of fuzzy logic systems that can learn from experience.
Currently, only published findings can create and modify fuzzy control rules based on experience [ 38 ]. Among them can be Scharf’s self-organizing robotic control system [ 39 ] by using a performance matrix to change the rule matrix and alter the rules that constitute the management strategy. Another intriguing example is the Sugeno fuzzy vehicle, which can be trained to turn and park itself. Instead of a membership function, the effect of a rule is viewed as a linear equation of the process state variables. Through optimization of least-squares performance indices using a weighted linear regression system, the challenge is simplified to a parameter estimate. Although these approaches provide promising outcomes, they are subjective, somewhat heuristic and depend on trial and error for the choice of MFs. Thus, the ability to learn neural networks can offer a more promising approach to fuzzy logic systems [ 40 ]. As shown in Fig. 4 , the fuzzy logic system consists of four parts: knowledge base, fuzzification, inference and defuzzification. On the basis of the fuzzy constants provided, the process converts given inputs to the fuzzification stage. Based on the knowledge base, the inference is made. Then, in the defuzzification stage, every fuzzy output is mapped to complex output MFs [ 41 , 42 ].

Fuzzy logic system
2.4 Ant colony optimization
Ants exhibit a behaviour in which they remember and follow a specific path between their colony and a food source. They achieve this by leaving pheromone trails during their food search [ 43 ]. When other ants come across these pheromone trails, they start to follow them. Because the increased presence of the chemical on the path has the effect of attracting more ants to follow it, the ants will emphasize the pheromone trail. To find the best solution to the problem studied, ant colony optimization (ACO) builds multiple iterative solutions. The objectives of [ 44 ] were to evaluate the features of the search area for problems and to use this knowledge to address the solution process. The solution–construction process is a sequential decision-making process due to parametric stochastic decisions. An ACO algorithm depends on a sequence of learning of the parameters used in decision-making to reach a global policy that provides optimum solutions for a particular situation [ 45 ]. The parameters of the learning object are considered pheromones and are called variables of the pheromones.
An ACO method contains a stochastic local search technique to organize the routing pathways that artificial ants can determine. These ants co-operate together through indirect information exchange to create the best and shortest route. The concept of the ACO is taken from the food search characteristic of the true colony in an intelligent optimization algorithm and how the ants work together in this difficult job. It can be expected that the ACO finds the quickest route from nest to food according to the biological study of the ants. The ant pheromone distribution technique is termed staggered, in which information is shared with other ants indirectly. Pheromone updates are the basis of the ACO algorithm. These pheromone updates depend on the pheromone and the number of ants that work best. Natural ants can determine the quickest route based on their best knowledge and a strong pheromone trace. The shortest path is inversely proportional to the amount of pheromone and length of the path using an ACO method. The following is a step-by-step explanation of the algorithm replicating these properties [ 46 ]. The pseudocode for ACO is shown in Table 2 .
Pseudocode of the ant colony optimization algorithm
Set pheromone pathways: The algorithm starts by setting the initial pheromone pathways in the search space of the problem. These pathways act as a guide for ants to navigate and find solutions.
Generate a random ant population: Next, the algorithm generates a population of random solutions (ants) to start searching for the optimal solution.
Choose the optimal position: Each ant then uses a combination of pheromone information and heuristics to determine the next step (position) to take. The objective is to find the position that maximizes the target function.
Get the finest search ant: After all ants have completed their search, the algorithm selects the best ant, i.e. the one with the highest value of the target function.
Restore the trail of pheromone: The pheromone trail of the best ant is then updated to reinforce its path, encouraging other ants to follow it.
Check the end condition: The algorithm repeats the above steps until a stopping criterion is met.
End: The algorithm concludes when it satisfies the stopping condition and provides the best solution discovered.
2.5 Artificial bee colony optimization
The artificial bee colony (ABC) optimization imitates bee behaviour. A colony of bees is made up of onlookers, scouts and worker bees [ 47 ]. Artificial bees are flown in this system in a multidimensional search room and, depending on the experience they have gained and based on their next partner experience, the used bees pick their food sources and bees to change positions. Scout bees pick their food sources at random without any experience. Each food source avoids the probable solution to the problem under discussion [ 48 ]. The number of bees employed is as high as the food sources, each being a site, which is currently being used or as many solutions as individuals [ 49 ]. This procedure is continued until the ABC optimization meets a stop criterion.
ABC_Optimization (n, m, k)
population <- initialize (n, m, k)
global_best <- assign_random_food_source(population[m])
while! stop_criteria_met ()
for bee in population
fitness <- calculate_fitness (bee. food_source)
if fitness > global_best. fitness
global_best <- bee. food_source
for the bee in population
bee. update_food_source (global_best, bee. next_partner)
update_food_source (global_best, next_partner)
prob <- random_probability ()
if prob < experience
food_source <- global_best
else if prob < experience + next_partner. experience
food_source <- next_partner. food_source
food_source <- random_food_source ()
Initialization phase:
Initialize the x i j solution population in the j domain parameter. The exact description may be used for that purpose:
where x m a x j is the upper bound of the parameter j and x m i n j is the lower bound of the parameter j.
Worker bee phase:
Each worker bee uses a formula to identify and assess a food source v i j representative of a location such as a food source in her x i j memory. Each worker offers information about their food source to onlookers who select a food source website based on information collected from their bees while they wait at the hive according to Equation (2) :
If x k is a randomly picked solution, j is a parameter randomly selected and β i j is a random integer within the [–a, a] range. A greedy selection between v i and x i is applied after the production of a new solution v i .
Onlooker bee phase:
There is a reference previously to the proportion of the amount of a food source to its location in the solution. Onlookers are positioned at food sources using a selection strategy based on fitness, such as the way of selecting the roulettes wheel. New solutions x i based on pi are picked to assess the new solutions v i and new solutions v i for spectators are created. The hired bees between v i and x i receive a greedy selection.
Scout bee phase:
Former workers who lost their resources start scouting randomly for food supplies. Every colony of bees has scout bees. The scouts have little instruction when looking for food. They mostly focus on finding food. Artificial bees can find the available answers rapidly. ABC decides that the artificial scout is the bee whose food supply has been lost or whose profitability has fallen below a specific level of profitability. The control parameter that decides the class is the withdrawal criterion or the ‘limit’. After a predefined number of attempts, a worker bee leaves an unimproved solution that is a source of food. The number of tests necessary to release the answer is determined by ‘limits’.
2.6 Particle swarm optimization
Particle swarm optimization (PSO) is a population-based evolutionary computational technique that is employed to address stochastic troubleshooting. It belongs to the category of swarm intelligence and is founded on social and psychological principles. PSO provides valuable insights into engineering applications and contributes to their development [ 50 ]. Social impact and social learning make cognitive consistency possible for the person. People may resolve issues by talking to people and by changing their ideas, attitudes and behaviour; they can usually be portrayed as people moving in a socio-cognitive space towards one another. But PSO has certain inconveniences such as global convergence; unlike some other optimization algorithms, PSO does not have a guarantee of global convergence, which means that it may not find the true optimal solution. To address this drawback, a novel PSO and a chaotic PSO are designed to tackle energy-system optimization issues efficiently. The analysis of the problem of unit commitment within the regulated system leads to the examination of UCP (uniform customs and practice for documentary credits) inside the deregulated market. The overall profit, execution time and convergence criteria are compared between various approaches.
One element is the current velocity of the particle v ( t ) . Another is the optimum position Y ∗ ( t ) to approach the particle. The third factor is that the community or sub-community is optimally informed by Y ∗ ∗ ( t ) [ 51 ]. In each iteration step, the particle speed is modified to Y ∗ ( t ) and Y ∗ ∗ ( t ) . Meanwhile, the random weight is independently allocated to the V i , Y ∗ ( t ) and Y ∗ ∗ ( t ) . The speed and position are updated following Equations (3) and (4):
In the given equation, v k +1( i , j ) represents the velocity of the particle in the i -th particle and j -th dimension at iteration k + 1.
The weight factor ω determines the extent to which the previous velocity influences the new velocity.
v k ( i , j ) denotes the velocity of the particle in the i -th particle and the j -th dimension at iteration k .
C 1 and C 2 are the learning parameters that determine the influence of the personal best and global best solutions, respectively.
r and 1 and r and 2 are randomly generated numbers within the range of [0,1].
P bes t k ( i , j ) represents the personal best position of the i -th particle in the j -th dimension achieved thus far.
Y k ( I , j ) represents the current position of the i -th particle in the j -th dimension.
G b es t k signifies the global best position discovered by all particles up to the present iteration.
The flow chart for PSO is shown in Fig. 5 .

Flow chart of particle swarm optimization
2.7 Regression model
The research model [ 52 , 53 ] can be defined using Equation (5) :
where Y represents the dependent variable; this refers to the indication of respondent i ’s willingness to adopt smart home technology and their level of flexibility in terms of demand for technology j. β refers to the intercept. X 1 ij ,..., Xnij are dichotomous predictors included in the model. εij represents the random error term.
Building on Equation (5) , the level 2 model can be formulated as follows:
In Equations (5) and (6) , u 0 j ,..., u 1 j represent the random effects. W 1 j and W 2 j correspond to grand-mean centred and uncentred variables, respectively.
These equations are utilized in research to describe the relationships between the dependent variable, predictors and random effects. Equation (5) serves as the core model equation, capturing the influence of the predictors on the dependent variable while accounting for random error. Equations (5) and (6) extend the model by specifying the relationships and random effects associated with the intercept and predictor coefficients at the level 2 analysis. Collectively, these equations offer a comprehensive framework to analyse the variables that impact the acceptance of smart home technology and the adaptability of demand within the specific research context.
2.8 Regression and classification problems using AI
The RNN is a variation of the neural network frequently employed in the power systems domain to address regression and classification problems that involve sequential data. Unlike direct neural network models, the structure and operating principle of the RNN differ significantly [ 54 ]. In an RNN, the input data are fed to the model sequentially at each time step ( t ), as shown in the signal propagation diagram. At each step, the current state ( output ) is calculated by considering the current input data and the previously computed state. This iterative process continues for a fixed number of steps ( n ) until the desired output (predicted value) is achieved or until all input data ( input ) have been processed [ 55 ].
The propagation of signals in the RNN model is illustrated by the values assigned to each hidden state (hidden). These hidden states are calculated using the previous hidden state ( hidden − 1) and current input data ( input ) [ 56 ]. hiddent = (〈 w hidden , hiddent – 1〉 + 〈 w input , input 〉) Here, σ () represents the activation function (such as the sigmoid function, hyperbolic tangent or rectified linear unit (ReLU)), while w hidden and w input are the weights for the hidden and input states, respectively.
The output value at each calculation step output is obtained by taking the dot product of the weights associated with the output state and the values of the hidden state, similar to a regression equation: output = 〈w output , hiddent 〉 [ 57 ].
During training, the initial stage involves calculating the output signal, after which the error function is calculated to determine the discrepancy. For regression problems, it is common to utilize the square root of the standard deviation between the output of the RNN and the values from the response space ( y t ):
Applying the chain rule, the gradient of the error functional is calculated. The weight coefficients ( w ij ) are adjusted in a manner that reduces the functional, following the direction of decreasing values, until it reaches the minimum value or the training iterations reach the predetermined limit. It is important to note that the weights associated with the hidden state of the RNN ( w hidden ) remain unchanged after propagating the error from each output ( output ). Conversely, the coefficients w output and w input change at each step of the gradient [ 58 ].
3.1 Operation of the power system
ELD is the process of assigning the generation output to the generation unit to supply the system load fully and economically. The whole engaged generating unit produces total electricity costs to minimize energy. The main concern of the ELD problem is to reduce the overall fuel cost. This is achieved by generating electricity in a way that optimizes the use of resources and reduces the overall cost of power production in the electricity system. Multiple generators provide enough total output to meet the consumer requirements in a typical power system. The production costs of each generating unit in the electricity system are different, as the producing units are not the same distance from the loading unit. Over the years, several AI approaches have been created to address this challenge. After simulation, authors concluded that using the genetic algorithm technique to solve the ELD problem can result in a lower overall cost of electricity production, but may also result in higher emissions. However, choosing a solution with a higher cost may result in minimum emissions. Transmission losses are usually neglected when they are small. However, for long-distance transmission in large and interconnected networks, transmission losses become significant and have an impact on the optimal distribution of power generation. It is possible to operate the same multitasking system with a better voltage profile and with evolutionary calculation technology, the cost and emission value of the best compromise.
The proposed results of the simulation of the ANN emphasize that the results are indiscernible from conventional methods, although the time used by neural networks is less than conventional methods. The number of generators increases the prediction error because there is a lot of input and output data to be learned. To monitor the performance, neural networks have been modelled. The authors have performed the simulations with many generation units having ramp rate limits and prohibited operating zones as constraints and the resultant performance is compared with ANN, GA and ACO techniques, but the ABC technique gives better outputs with fast convergence. The greedy selection procedure and the timely abandonment of the used food sources contained in ABC give it this potential. The basic operations of ABC optimization prevent solutions from stopping and make the algorithm more exploitative.
3.1.2 Generator maintenance scheduling
Generator maintenance scheduling (GMS) is a complicated combinatorial optimization issue for a power provider. Mathematical approaches include traditional ways to tackle the GMS issue. To evaluate the needed objective function, a mathematical model approach employs a trial-and-error procedure. Mathematical approaches even fail to provide viable answers as in some circumstances the operator needs to rely on certain assumptions and models that may not accurately reflect real-world conditions. In some cases, operators may need to be involved to provide additional input and expertise to make informed decisions about maintenance schedules. In addition, there may be unpredictable factors, such as equipment failures or changes in demand, that cannot be accurately accounted for by mathematical models alone. Maintenance is a preventive outage program for generating units within a certain time horizon in a power system. In the event of a range of various specification generating units in the energy system and several limitations to produce a sustainable and practical solution, maintenance planning becomes a difficult challenge. The maintenance planning of the generators is done for time horizons of different lengths. Short-term maintenance plans from 1 hour to 1 day are crucial to the daily operations, engagement and operational planning of power plants. Medium-term planning is necessary for resource management between 1 day and 1 year. Long-term planning from 1 to 2 years is crucial for future planning. An examination is being conducted to resolve some AI methods, including simulated developments, neural networks and GAs. The application of the genetic algorithm through case research shows that suitable GA parameters are safeguarded, as well as issue coding and development functions. The use of integer encoding decreases the velocity of the genetic search method investigation. By using integer encoding, the algorithm needs to perform additional operations to convert solutions into integers, which can slow down the search process. Planning the generation of power remains a barrier to competent solution technology and a difficult optimization problem. The challenge in power generation planning lies in finding the optimal balance between cost and efficiency, while also considering factors such as environmental impact, reliability and security of supply. The answer to the difficulties in generation planning consists of finding the UC (unit commitment) at every point in the programming period for each generator in one power system. An electrical system must be defined in each planning interval for each power generator for the decisions and levels of output. The solution process must be addressed concurrently for binary decisions and continuous variables. Generation difficulties with scheduling are typically quite narrow and combined. Match swarm optimization approaches have been used to achieve viable schedules within a specified time. The study found that an optimization-based approach using PSO provided better results than a GA or an evolutionary strategy. Data from the actual power system were used to evaluate the performance of the different optimization techniques.
UC is properly scheduled for the ON/OFF status and the genuine generator power outputs of the system. To satisfy a high number of system limitations and decrease the overall fuel cost at every time interval, a spinning reserve is necessary (spinning reserve refers to the additional generation capacity that is available and running but not actively supplying power to the grid). UC meets the expected load requirements in advance. To implement UCs, medium-term load forecasting using ANN was used. The neural network structure was trained through learning and parameter learning. Total operational expenses under 24 hours were used for the assessment criterion. The study demonstrates the effectiveness of the proposed approach by comparing the performance of the ANN-based load forecasting model with traditional methods such as linear regression and time-series forecasting. The results show that the ANN-based load forecasting model significantly improves the accuracy of load forecasting and reduces the scheduling cost by reducing the number of units needed for scheduling. The study also highlights the importance of considering the uncertainty and variability of load demand in UC scheduling and suggests that ANN-based load forecasting models can be a useful tool for achieving more efficient and reliable scheduling in power systems. Locational marginal prices have been evaluated through a trained ANN. The findings show that the current technique gives a different UC mechanism. To develop unit commitment, the PSO technique is used. On implementation, with the increasing size, the execution time is also increasing. To accelerate the PSO, a convergence repair method is also implemented.
3.1.4 Optimal power flow
Optimal power flow (OPF) is a highly important technique to identify the optimum control parameter settings that enhance or decrease the intended target function, but also under a variety of limitations. An essential instrument to design and operate a power system is the issue of optimum power flow to identify the best parameter settings that can maximize or minimize the intended goal function within specific limitations. Voltage and reactive controls, also called OPD, are an OPF sub-problem that seeks to reduce overall transmission loss by resuming the reactive power flow. Optimal reactive power dispatch is a non-linear solution for the issue of blending integers since some control variables such as tap ratios for transformers, shunt capacitor outputs and reactors are distinct.
The alternate strategy for mitigating the problem of GA-ANN is set out in this article. A collection of ANN networks is trained offline in specified system quantities to work on a general OPF issue. To choose the appropriate ANN inputs, the k-mean clustering technique is utilized. When learning the functions correctly, ANNs can easily estimate the associated results with great precision.
The ANFIS develops the input/output data set fuzzy-inference system (FIS) that matches the membership (adjustment) parameters with a backpropagation or minimum square process type. This update allows you to learn from the fuzzy systems data IEEE 39 bus system implementations and simulated software from the power world are utilized for the formation of the ANFIS. The results indicate that the ANFIS offers solutions as accurate as conventional ones. It takes less time, though, and it works quickly. Some additional papers on the application of AI in the operation of power systems are presented in Table 3 .
Applications of artificial intelligence in the operation of a power system
3.2 Control of the power system
The main objective of a voltage controller power system is to maintain the voltage profile within a defined limit, thus minimizing transmission losses and avoiding cases of voltage instability [ 81 ]. The VC system consists of three levels of hierarchical control: AVR (automatic voltage regulator), tertiary voltage control (TerVC) and secondary voltage control (SecVC). AVR is aimed at controlling the voltage of buses that are equipped with reactive power sources (e.g. synchronous, sync, static var compensators and STATCOM (static synchronous compensator)). Actions are carried out locally at this control level. SecVC is used to monitor the voltage on a specific bus that controls a cargo bus.
In situations in which there is hardware present in the vicinity that modifies the reference point for the AVR, the control level typically operates at a slower pace compared with the AVR control level. SecVC is responsible for identifying VC regions and their correlation with individual load buses. To accommodate varying power system conditions, SecVC must demonstrate flexibility in adjusting the control regions to accommodate all grid conditions. On the other hand, TerVC determines the optimal reference value for voltage grids at each load bus. The objective is to minimize power loss, optimize reactive power and maintain a minimum load release or reservation. TerVC is usually updated every 30 minutes to 1 hour.
The backward error propagation algorithm trains the multilayered feedforward perception. The minimum singular value method analyses the static voltage collapse. The procedure uses a minimum voltage stability evaluation time once the network training is complete. For monitoring voltage collapse, complementary methodologies of neural networks and expert systems would be combined for use in the application [ 82 ].
GA is an iterative optimization technique with several solutions from the candidates (known as a population). In the case in which there is no knowledge of the problem field, then the GA starts to look for solutions from a random population. The appropriate coding (or display) must first be defined to solve the problem. A fitness function should also be defined so that every coding solution is given a figure of merit. If parents are not satisfied with the termination condition, for reproduction, they must be picked [ 83 ]. They are then joined to generate offspring through reproduction and, to refresh the population of candidate solutions, crossover and mutation operators are utilized. Typically, in a basic genetic algorithm, three operators are involved: selection, crossover and mutation. These operations are performed to generate new offspring, individuals and subsequent generations. The same process is repeated with the new generation until the desired criteria are met. The approach of this method is used to teach swarming at the beginning of PSO. In this case, 10 control variables are used for the ANN input. The neuron and its prejudice are 11. A hidden layer consists of this group of neurons and biases. Ten outputs/goals are available. These objectives are achieved by using the optimal value of PSO. The last outputs are the initializations in the time-varying non-linear particle swarm optimization (TVNL-PSO). The steps are as follows:
PSO is used to take the ANN input; the weight value is applied at random;
the ANN input and partition in a cached layer are weighed and then activated by the sigmoid binary function;
weighting of the output in the hidden layer and activation of the linear function;
to optimize the reactive power and VC by TVNL-PSO, the ANN output is transmitted as a starting initialization value.
3.2.2 Power system stability control
The stability of a power system is a feature that allows it to remain under a balance in normal operating conditions and retrieve an acceptable balance after a change. Margins of stability can be seen to decline throughout the world [ 84 ]. We highlight three of the many reasons for this:
The inhibition by economic and environmental constraints of further transmission or construction. Therefore, power systems must be operated with lower safety margins.
Restructuring of the electricity industry. The restructuring process reduces the margins of stability, as power systems do not co-operate effectively [ 85 ].
Increased complexity of power systems multiplies the compulsive properties. These include large, non-linear oscillations; frequency differences between weakly binding energy-system areas; interactions with saturated devices.
Fuzzy logic endeavours to address problems by emulating human reasoning, allowing optimal decision-making based on available information. It can also be employed to regulate the stability of un-modelled systems. To achieve improved performance, a fuzzy logic (FL) controller is combined with a PID (proportional–integral–derivative) controller. In this particular scenario, the fuzzy logic control adjusts the gains of the PID controller based on the power system.
A fuzzy logic controller primarily consists of four major parts: fuzzification, fuzzy rule base, fuzzy inference and defuzzification. FACTS have proven to be extremely promising for increasing performance under stable conditions. The most promising FACTS device is a unified power flow controller (UPFC). Three control factors can be adjusted: bus voltage, reaction line and phase angle between two buses. The power flow should be redistributed across lines while a stable state is preserved. It can also be utilized to increase damping when low frequencies are damped temporarily.
Power system stability control.
Load frequency control as defined by the controllable generator power output control in a prescribed area resolves system frequency changes, two-line loadings or interactions to maintain an interchange with other regions within the fixed limit or scheduled system frequency [ 86 ]. The traditional proportional–integral (PI) controller is the most widely used among different types of load frequency controllers. The PI controller can be easily implemented and provides a faster response, but its performance decreases when unwanted disturbances, such as load change dynamics, increase the difficulty within the system. In this paper, less computing is required for the non-linear autoregressive-moving average-L2 (NARMA-L2) control architecture. Plant output, reference and control signals are included. The controller is therefore taught to monitor the output of the reference model. The model network that updates controller settings predicts the effect of the change in plant performance. Some additional papers on the application of AI in the control of power systems are presented in Table 4 .
Applications of AI in control of a power system
3.3 Planning of power system
DSP plays a crucial role in enhancing reliability and minimizing costs for both utilities and consumers. Electric power distribution networks are a fundamental component of the electrical power system. In general, transport networks are denser and more complex than those that provide transformer stations [ 110 ]. Automating previously manual jobs increases with distribution networks becoming more complex. New tools are known as advanced automation functions that support the operation of such networks. These functions enable the network operator to effectively address issues that arise. Furthermore, the reconfiguration of distribution networks is essential to identifying optimal solutions that align with the operator’s requirements and constraints, ensuring a secure and economically optimized electricity supply.
The optimal design of a distribution network is not a fixed solution, but rather a process that involves considering various technically feasible options and using improvement tools to make the best decision based on factors such as demand, reliability of power transmission and network structure. All potential paths are initially identified with uploaded system data and then the energy-loss cost calculation applies for each identified path forward/backward sweeping load flow technique. For the distribution of power, the minimum energy-loss path is chosen. The optimal selection of the branch conductor of the radial system is done using optimization of PSO. In this case, parameters such as power loss, voltage profile and capital investment depreciation improve optimization. These parameters are used as optimization criteria to determine the optimal branch conductor that minimizes power loss, improves the voltage profile and reduces capital investment depreciation. The PSO algorithm iteratively updates the position of each particle in the search space based on its own experience and the experiences of its neighbours. The algorithm continues until a global optimum is found or a stopping criterion is met. The final solution produced by the PSO algorithm represents the optimal branch conductor that meets the optimization criteria [ 111 ]. The optimization of the PSO results in the optimal conductor and the best substation, the positioning of the optimal conductor is selected and then the optimal substation power distribution is achieved.
A multi-target algorithm was proposed that uses a fluid optimization technique to handle contradictory targets [ 112 ]. The plan formulation and the algorithm include a multi-target function that uses battery energy storage systems (BESSs) and traditional resources to select the best planning option. The microgrid BESS has been receptive to power management and improvement in power quality. The proposed algorithm is based on the fuse-based decision-making processes of the Mamdani-type FIS and Bellman–Zadeh approach.
In this paper [ 113 ], two algorithms, namely the mixed-integer linear program (MILP) and GA, are compared for the design of a radial distribution system feeder. The main objective is to minimize total investment and operational outages while maximizing system reliability. The study aims to evaluate and compare these two optimization techniques in terms of their optimality, complexity and time requirements. A unique aspect of the optimization model is the consideration of operational costs associated with failures, which are directly linked to the design of the system. The fault rate and defect cost at each loading point are updated based on the proposed configurations. It is crucial to determine which method produces superior results in terms of optimality, complexity and time efficiency.
The GA technique is used to build the algorithm for optimizing distribution networks. The fundamental concept is the growth of the genetic operator population (selection, crossover and mutation). These are used to generate a fresh population from the previous generation throughout each generation procedure. In GA, a single chromosome shows each person. This chromosome corresponds, according to the graph theory, to a radial distribution network configuration or a particular graph twist. The chromosome group is the population. Randomly, an initial group is created as a first step in the implementation of GA. Then the encoding is applied to each chromosome. In this study, only closed branches represent the network topology. A true coding strategy was used to match each gene to the edge of the coagulation tree [ 114 ].
3.3.2 Reactive power optimization
As the demand of electricity increases and new lines are built, the environment and the unforeseen power fluid in the lens are reduced, it is generated by the current scenario. Effect reactive compensation control improves voltage, reduces energy loss and improves system performance under stable and dynamic conditions in weak nodes [ 115 ]. Because the complexity of power systems is constantly increasing and the network components are constantly being loaded, abnormal operating conditions such as voltage can occur more often. Therefore, it is obvious that the power system needs adequate reactive power and VC.
In pursuit of intelligent theory development, a combination of fuzzy logic and ANNs is used to determine the control strategy for transformer taps and capacitors. However, due to the increasing complexity of control variables, rapid optimization becomes challenging [ 116 ]. To address this issue, a genetic algorithm is utilized, which tackles problems associated with incorporating regulatory time as penalty terms in the objective function and determining appropriate penalty factors that affect algorithm performance. When regulatory time is a constraint, the optimization objective focuses on minimizing the total energy loss during the dispatch period. GAs, inspired by natural selection mechanics and genetics, such as inheritance, mutation and recombination, are utilized (also referred to as crossover) to optimize the solution.
The PSO method can be used for handling FACTS devices in power systems. Various reactive power problem objectives and different solutions are addressed in the interconnected power system. Solutions and comparative analyses using the FACTS device, differential evolution (DE) and PSO algorithms are presented under various loading conditions [ 117 ]. The algorithm proposed [ 118 ] employs DE to minimize generator fuel costs on FACTS devices. Additionally, the authors discuss the hybridization of DE and PSO (DEPSO) to overcome the maximum load limit. The control of reactive flow is addressed using fuzzy sets and a fuzzy feature optimization technique is introduced to optimize reactive power. The utilization of fuzzy linear programming offers an effective approach to calculating reactive power, aiming to minimize active power loss and maximize the voltage stability margin. The paper [ 119 ] explores the combination of fuzzy and GA approaches for FACTS shunt controller placement and sizing. Lastly, the focus of [ 120 ] is on the integration of fuzzy systems with GM algorithms and the PSO algorithm to tackle the OPF problem and optimize control variables. In this paper, the authors [ 121 ] focus on fluid-based reactive and voltage controls to reduce actual loss of power.
3.3.3 Capacitor placement
There are some advantages if capacitors are placed optimally, including various factors, such as maximizing energy and reducing peak power loss through the introduction of a condenser in an electrical distribution system. In the paper, a novel adaptive modified firefly algorithm is presented to address the optimal capacitor placement problem in power systems. This optimization problem involves identifying the best positions and sizes of capacitors in a power system, in order to enhance voltage stability, minimize energy losses and improve the power factor. The proposed algorithm combines the firefly algorithm with adaptive parameter settings and introduces a unique crossover operator to enhance both convergence speed and solution quality. The authors conduct evaluations on a test system and compare the performance of the algorithm with other optimization methods. The results demonstrate the effectiveness of the proposed approach in finding optimal solutions and highlight its superiority in terms of solution quality and computational efficiency compared with other algorithms [ 122 ]. Losses occur due to reactive currents in the distribution system and are therefore minimized in the right places. Shunt capacitors are used, depending on their use. A capacitor is used to improve the voltage profile, reduce losses and increase the power factor [ 123 ].
Elbaz et al. [ 124 ] have been using ANN techniques to control both capacitor banks and voltage regulators. The ANN has many input connections and all inputs are combined to determine the output capacity. The purpose of the capacitor search algorithm was to reduce total active losses in the distribution system by utilizing the capacitor banking search to address the capacitor placement problem. The operation of the ant colony was proposed to address problems related to the installation of the capacitor. The fuzzy method uses variables such as angle, current and voltage, etc. A degree for a set and fuzzy variable is determined by MFs. This degree changes from zero to one that takes zero or one as opposed to the classical methods [ 125 ]. A fuzzy logic-based algorithm is developed to minimize line loss for the placement of condensers in a radial system. The fuzzy expert system identifies the capacitor candidate nodes by compromising the possible reduction in loss between the condenser system and the voltage level. This paper [ 126 ] presents a fluffy approach to identifying the appropriate places for capacitor placement. In the design of a seamless logic to determine the optimal placement of capacitors, two main objectives are taken into account. These objectives include (i) minimizing actual power loss and (ii) maintaining voltage within acceptable limits. Fuzzy member functions are used to model voltage and power loss indices for nodes in the transmission system [ 127 ]. The suitability of capacitor placement for each node in the distribution system is then determined. This is achieved using a set of rules within the FIS. Nodes can be fitted with capacitors of greatest appropriateness.
In [ 128 ], a method based on GAs is used to identify the optimal locations and sizes of capacitors in the distribution network. The capacitor sizes are considered discrete and known variables placed on the buses of the network. Hence, the maximum losses of the distribution system are reduced. GA technology is selected as the capacitor problem is more accurately addressed in the power grid. When the search area crosses for an optimal solution, the advantages of GA are multifunctional—that is, when a locally optimal solution is found to an engineering goal, GA adapts its search to find an optimal global solution, subject to predefined search restrictions [ 129 , 130 ]. The article shows the results of the study of the best size and location for a GA-connected system using bays in Saudi Arabia in West–East regions [ 131 , 132 ]. Two formulas are proposed for capacitor positioning: (i) cost balance condenser/loss principle and (ii) total system performance cost estimates, standard analysis and verification of annual benefits, power loss and operational tension results [ 133 , 134 ]. AI is applicable for various aspects of the power system [ 135 ]. Some additional papers on power system application of AI in planning are presented in Table 5 .
Applications of AI in the planning of a power system
Application of AI in various problems of the operation, control and planning of power systems has shown good performance over conventional methods. ABC algorithms function better than other AI techniques in the ELD problem, as shown in a comparison. The ABC algorithm has the highest-quality solutions, consistent convergence and exceptional computing efficiency. Compared with traditional mathematical models, both GA and PSO techniques are superior; the PSO technique is preferable since GA replaces humans. Unlike GA, PSO models alter humans through time and, in the following generation, all people survive. The fate of each person is continually adjusted based on the global ideal point thus far. The swarming effect in PSO allows the population of particles to move collectively in the search space, facilitating a more efficient exploration of the solution space and a faster convergence to optimum search areas compared with GA. PSO is particularly useful for optimization problems with many variables and when the solution space is complex and not well understood. Additionally, PSO requires minimal computational resources compared with GA as it does not require the evaluation and selection of multiple generations of offspring. In UC, with the increasing time, the execution time is also increasing, so the combination of many AI techniques can be progressive, as it can potentially increase the efficiency and accuracy of the optimization problem. The scalability of any heuristic optimization method is a major issue. In the load flow method, the ANFIS and ABC algorithms provide efficient and accurate solutions, and the implementation of ANNs is fast and can handle missing data effectively. In VC, rather than replacing conventional methods, the focus should be on enhancing their capabilities through the integration of AI techniques, modern control theory, fuzzy technology and ANNs, along with adaptive control and expert systems. This approach, which combines current research trends with practical experience, has great potential for practical applications. In load frequency control, the NARMA-L2 ANN network architecture provides the best output after some series of trials and improvements. Under fault conditions, it has been observed. The fuzzy-PID power output works so that the power systems are fast and stable. Due to the specialization, the fuzzy logic in condenser placement is better than other approaches. Fuzzy logic includes the relatively basic technique of calculating the necessary nodes in the distribution system to replace condensers with approximate reasoning. The results of the study indicate that the GA method can provide a globally optimal solution for reactive power optimization, particularly when an ample generation and population size is used. Furthermore, it was found that the use of the UPFC resulted in minimized losses compared with the static var compensator and thyristor-controlled series capacitor. In terms of reactive power planning, a fuzzy membership-based approach is employed in an interconnected power system to identify weak nodes and determine optimal parameter settings for FACTS devices. The feasibility of this strategy is validated through its solutions and compared with other global optimization approaches. The proposed technique is applied to a standard system under high load conditions, resulting in a stable system with reduced losses and cost savings. This approach has the potential to become a novel technology for effectively coordinating FACTS devices with other existing generators. Following the pandemic, it is expected that governments around the world will prioritize energy efficiency in buildings and smart homes. To support this, the development of open-source protocols and unified connectivity solutions is crucial. Smart home systems are focused on maximizing the energy efficiency of major household appliances, thereby contributing to overall energy balance. By constructing sustainable homes integrated with smart technologies and a combination of energy sources, significant cost savings and a reduction in carbon footprints can be achieved. While smart homes are becoming more common, there are still barriers to widespread adoption that researchers need to address. It emphasizes the potential of smart home companies and highlights technical challenges such as device compatibility. The review also discusses the importance of studying consumer attitudes and demands, and mentions the limitations of the survey methodology. Significant scientific results include an algorithm for a modern predictive analytics system, an approach to assess the condition index of the equipment and a method to determine the probability of defects using ML. The study validated the model using data from a hydroelectric power plant, demonstrating its accuracy. Future research can focus on refining the index calculation for equipment with multiple functional units and constructing predictive models for specific equipment classes. ML and data-driven techniques hold great promise in the field of power systems, especially in the context of smart grids. These methods can effectively analyse and process large volumes of data, resulting in improved accuracy and increased operational efficiency. However, some challenges need to be addressed, such as ensuring the quality of the data and interpreting the results in a meaningful way. Accurate forecasting plays a vital role in optimizing grid operations, integrating renewable energy sources and achieving cost-effective power generation. ML plays a vital role in transforming traditional grids into smart grids, improving reliability and safety. It also aids in demand-side management and enhances cybersecurity.
Evaluating energy costs and making improvements can lead to significant energy savings. Smart technologies have the potential to reduce electricity demand and environmental impact. Social acceptance of smart home systems needs to be promoted. Further research can expand sample sizes, include more diverse countries and explore smart meter readings. Future research should emphasize the importance of addressing technical, security and privacy concerns, and call for collaboration between stakeholders to enhance the smart home market. Although the developed approach offers several benefits, there is still an unresolved issue regarding the calculation of the technical condition index for equipment consisting of multiple functional units. Existing methods rely on assigning weights to each unit based on an expert evaluation to determine its importance. Additional research can focus on improving the methods for calculating the technical condition index for different types of power equipment and establishing predictive models to anticipate equipment defects in the event of functional unit failures. Future investigations should prioritize the development of more precise and dependable predictive models for power systems, taking into account the challenges related to data availability and interpretability.
Anshumaan Pathak and Utkarsh Pandey did the critical review. Surajit Mondal and Adesh Kumar supervised and reviewed the manuscript.
The authors declare that they have no known competing financial interests or personal relationships that could have appeared to influence the work reported in this paper.
The paper is a study and review article in which no specific data are referred to. No simulation software is used.
Sozontov A , Ivanova M , Gibadullin A. Implementation of artificial intelligence in the electric power industry . E3S Web of Conferences , 2019 , 114 : 01009 .
Google Scholar
Zhao X , Zhang X. Artificial intelligence applications in power system . In: Luo X (ed). Advances in Intelligent Systems Research—2016 2nd International Conference on Artificial Intelligence and Industrial Engineering (AIIE 2016). Dordrecht, Netherlands : Atlantis Press , 2016 , 158 – 161 .
Nath RP , Balaji VN. Artificial intelligence in power systems . IOSR Journal of Computer Engineering (IOSR-JCE) , 2014 . https://jcboseust.ac.in/electrical/images/notes/aitech_ug_ai_reactive_power_control.pdf ( 29 July 2023 , date last accessed).
Das S , Mukherjee M , Mondal S. Detailed energy audit of thermal power plant equipment . World Scientific News , 2015 , 22 : 70 – 90 .
Mondal S , Mondal AK , Sharma A , et al. . An overview of cleaning and prevention processes for enhancing efficiency of solar photovoltaic panels . Current Sci , 2018 , 115 : 1065 – 1077 .
Wasesa M , Tiara AR , Afrianto MA , et al. . SARIMA and artificial neural network models for forecasting electricity consumption of a microgrid based educational building . In: 2020 IEEE International Conference on Industrial Engineering and Engineering Management (IEEM), Singapore, 14–17 December 2020 , 210 – 214 .
Jang JS. ANFIS: adaptive-network-based fuzzy inference system . IEEE Trans Syst Man Cybern , 1993 , 23 : 665 – 685 .
Borges AF , Laurindo FJ , Spínola MM , et al. . The strategic use of artificial intelligence in the digital era: systematic literature review and future research directions . Int J Inf Manage , 2021 , 57 : 102225 .
Guan Y , Chaffart D , Liu G , et al. . Machine learning in solid heterogeneous catalysis: recent developments, challenges and perspectives . Chem Eng Sci , 2022 , 248 : 117224 .
Bordin C , Skjelbred HI , Kong J , et al. . Machine learning for hydropower scheduling: state of the art and future research directions . Procedia Comput Sci , 2020 , 176 : 1659 – 1668 .
Wang H , Ricardez-Sandoval LA. Dynamic optimization of a pilot-scale entrained-flow gasifier using artificial recurrent neural networks . Fuel , 2020 , 272 : 117731 .
Isnen M , Kurniawan S , Garcia-Palacios E. A-SEM: an adaptive smart energy management testbed for shiftable loads optimisation in the smart home . Measurement , 2020 , 152 : 107285 .
Bibri SE , Krogstie J. Environmentally data-driven smart sustainable cities: applied innovative solutions for energy efficiency, pollution reduction, and urban metabolism . Energy Informatics , 2020 , 3 : 1 – 59 .
Strielkowski W. Social Impacts of Smart Grids: The Future of Smart Grids and Energy Market Design . Amsterdam, Netherlands : Elsevier , 2019 .
Google Preview
Oliveira L , Mitchell V , May A. Smart home technology—comparing householder expectations at the point of installation with experiences 1 year later . Pers Ubiquitous Comput , 2020 , 24 : 613 – 626 .
Tao M , Zuo J , Liu Z , et al. . Multi-layer cloud architectural model and ontology-based security service framework for IoT-based smart homes . Future Gener Comput Syst , 2018 , 78 : 1040 – 1051 .
Shcherbatov IA , Turikov GN. Determination of power engineering equipment’s defects in predictive analytic system using machine learning algorithms . J Phys Conf Ser , 2020 , 1683 : 042056 .
Moleda M , Momot A , Mrozek D. Predictive maintenance of boiler feed water pumps using SCADA data . Sensors , 2020 , 20 : 571 .
Ren C , Xu Y. A fully data-driven method based on generative adversarial networks for power system dynamic security assessment with missing data . IEEE Trans Power Syst , 2019 , 34 : 5044 – 5052 .
Yu J , Guo Y , Sun H. Testbeds for integrated transmission and distribution networks: generation methodology and benchmarks . CSEE J Power Energy Syst , 2020 , 6 : 518 – 527 .
Gonen T. Electric Power Distribution Engineering . Boca Raton, FL, USA : CRC Press , 2015 .
Wood AJ , Wollenberg BF , Sheblé GB. Power Generation, Operation, and Control . Hoboken, NJ, USA : John Wiley & Sons , 2013 .
Kumar N , Mishra VM , Kumar A. Smart grid and nuclear power plant security by integrating cryptographic hardware chip . Nuclear Engineering and Technology , 2021 , 53 : 3327 – 3334 .
Kumar N , Mishra VM , Kumar A. Smart grid security by embedding s-box advanced encryption standard . Intelligent Automation &. Soft Comput , 2022 , 34 : 623 – 638 .
Xu G , Wang Z. Power system load flow distribution research based on adaptive neuro-fuzzy inference systems . In: 2012 Spring Congress on Engineering and Technology, Xi’an, China, 27–30 May 2012 , 1 – 4 .
Karaboga D , Akay B. A comparative study of artificial bee colony algorithm . Appl Math Comput , 2009 , 214 : 108 – 132 .
Nualhong D , Chusanapiputt S , Phomvuttisarn S , et al. . Diversity control approach to ant colony optimization for unit commitment problem . In: 2004 IEEE Region 10 Conference TENCON 2004, Chiang Mai, Thailand, 24 November 2004 , 488 – 491 .
Ho SL , Yang S , Ni G , et al. . A particle swarm optimization-based method for multiobjective design optimizations . IEEE Trans Magn , 2005 , 41 : 1756 – 1759 .
Ratnaweera A , Halgamuge SK , Watson HC. Self-organizing hierarchical particle swarm optimizer with time-varying acceleration coefficients . IEEE Trans Evol Comput , 2004 , 8 : 240 – 255 .
Kumar N , Nangia U , Sahay KB. Economic load dispatch using improved particle swarm optimization algorithms . In: 2014 6th IEEE Power India International Conference (PIICON), Delhi, India, 5–7 December 2014 , 1 – 6 .
Panta S , Premrudeepreechacharn S. Economic dispatch for power generation using artificial neural network ICPE’07 conference in Daegu, Korea . In: 2007 7th International Conference on Power Electronics, Daegu, South Korea, 22–26 October 2007 , 558 – 562 .
Rahmat NA , Musirin I. Differential evolution ant colony optimization (DEACO) technique in solving economic load dispatch problem . In: 2012 IEEE International Power Engineering and Optimization Conference, Melaka, Malaysia, 6–7 June 2012 , 263 – 268 .
Saxena A , Pandey SN , Srivastava L. Congestion management in open access—a review . International Journal of Science, Engineering and Technology Research , 2013 , 2 : 922 – 930 .
Emami H , Sadri JA. Congestion management of transmission lines in the market environment . International Research Journal of Applied and Basic Sciences , 2012 , 3 : 2572 – 2580 .
Ghosh K , Pandey U , Pathak A , et al. . Simulation of density based traffic control system using Proteus 7.1 professional . In: Mathur G , Bundele M , Tripathi A , Paprzycki M (eds). Proceedings of 3rd International Conference on Artificial Intelligence: Advances and Applications: ICAIAA 2022. Singapore: Springer Nature Singapore , 2023 , 493 – 504 .
Deb S , Goswami AK. Congestion management by generator rescheduling using artificial bee colony optimization technique . In: 2012 Annual IEEE India Conference (INDICON), Kochi, India, 7–9 December 2012 , 909 – 914 .
Choudekar P , Sinha SK , Siddiqui A. Transmission line efficiency improvement and congestion management under critical contingency condition by optimal placement of TCSC . In: 2016 7th India International Conference on Power Electronics (IICPE), Patiala, India, 17–19 November 2016 , 1 – 6 .
Wang X , McDonald JR. Modern Power System Planning . London, UK : McGraw-Hill , 1994 , 247 – 307 .
Dahal K , McDonald J. A review of generator maintenance scheduling using artificial intelligence techniques . In: Proceedings of the 32nd Universities Power Engineering Conference (UPEC ’97), Manchester, UK, 10–12 September 1997 , 787 – 790 .
Koay CA , Srinivasan D. Particle swarm optimization-based approach for generator maintenance scheduling . In: Proceedings of the 2003 IEEE Swarm Intelligence Symposium. SIS’03 (Cat. No. 03EX706), Indianapolis, IN, USA, 26 April 2003 , 167 – 173 .
Johnson RC , Happ HH , Wright WJ. Large scale hydro-thermal unit commitment: method and results . IEEE Trans Power Appar Syst , 1971 , PAS-90 : 1373 – 1384 .
Thum YM. Hierarchical linear models for multivariate outcomes . Journal of Educational and Behavioral Statistics , 1997 , 22 : 77 – 108 .
Reyes DM , de Souza RM , de Oliveira AL. A three-stage approach for modeling multiple time series applied to symbolic quartile data . Expert Syst Appl , 2022 , 187 : 115884 .
Almaghrebi A , Aljuheshi F , Rafaie M , et al. . Data-driven charging demand prediction at public charging stations using supervised machine learning regression methods . Energies , 2020 , 13 : 4231 .
Huang P , Copertaro B , Zhang X , et al. . A review of data centers as prosumers in district energy systems: renewable energy integration and waste heat reuse for district heating . Appl Energy , 2020 , 258 : 114109 .
Sun L , You F. Machine learning and data-driven techniques for the control of smart power generation systems: an uncertainty handling perspective . Engineering , 2021 , 7 : 1239 – 1247 .
Lisin E , Shuvalova D , Volkova I , et al. . Sustainable development of regional power systems and the consumption of electric energy . Sustainability , 2018 , 10 : 1111 .
Prakash S , Jain J , Hasnat S , et al. . Economic load dispatch with valve point loading effect using optimization techniques . In: Bansal P , Tushir M , Balas VE , Srivastava R (eds). Proceedings of International Conference on Artificial Intelligence and Applications: ICAIAA 2020, Singapore: Springer , 2021 , 407 – 416 .
Chowdhury AK , Mondal S , Mukherjee M , Biswas PK. Mega watt security assessment of power systems . International Letters of Chemistry, Physics and Astronomy, 2015 , 58 : 9 – 15 .
Dommel HW , Tinney WF. Optimal power flow solutions . IEEE Transactions on Power Apparatus and Systems, 1968 , PAS-87 : 1866 – 1876 .
Wakiru JM , Pintelon L , Muchiri P , et al. . A comparative analysis of maintenance strategies and data application in asset performance management for both developed and developing countries . International Journal of Quality & Reliability Management , 2022 , 39 : 961 – 983 .
Muñoz-Delgado G , Contreras J , Arroyo JM , et al. . Integrated transmission and distribution system expansion planning under uncertainty . IEEE Trans Smart Grid , 2021 , 12 : 4113 – 4125 .
Chowdhury AK , Mondal S , Alam SM , et al. . Voltage security assessment of power system . World Scientific News , 2015 , 21 : 36 – 50 .
Holen AT , Botnen A , Stoa P , et al. . Coupling between knowledge-based and algorithmic methods . Proc IEEE , 1992 , 80 : 745 – 757 .
Short MJ , Hui KC , Ekwue AO , et al. . Applications of artificial neural networks for NGC voltage collapse monitoring . International Conference On Large High Voltage Electric Systems, 1994 , 2 : 38 – 205 .
Haida T , Akimoto T. Voltage optimization using genetic algorithm . In: Proc. 3rd Symposium on Expert System Applications to Power Systems, Tokyo, Japan, 1991 , 375 – 380 .
Denny FI , Dismukes DE. Power System Operations and Electricity Markets . Boca Raton, FL, USA : CRC Press , 2017 .
Masiala M , Ghribi M , Kaddouri A. An adaptive fuzzy controller gain scheduling for power system load-frequency control . In: 2004 IEEE International Conference on Industrial Technology, IEEE ICIT’04, Vol. 3 , Hammamet, Tunisia, 8–10 December 2004 , 1515 – 1520 .
Alquthami T , Butt SE , Tahir MF , et al. . Short-term optimal scheduling of hydro-thermal power plants using artificial bee colony algorithm . Energy Rep , 2020 , 6 : 984 – 992 .
Sahay KB , Sonkar A , Kumar A. Economic load dispatch using genetic algorithm optimization technique . In: 2018 International Conference and Utility Exhibition on Green Energy for Sustainable Development (ICUE), Phuket, Thailand, 24–26 October 2018 , 1 – 5 .
Mishra SK , Mishra SK. A comparative study of solution of economic load dispatch problem in power systems in the environmental perspective . Procedia Comput Sci , 2015 , 48 : 96 – 100 .
Dixit GP , Dubey HM , Pandit M , et al. . Artificial bee colony optimization for combined economic load and emission dispatch . In: International Conference on Sustainable Energy and Intelligent Systems (SEISCON 2011), Chennai, India, 20–22 July 2011 , 340 – 345 .
Daniel L , Chaturvedi KT , Kolhe ML. Dynamic economic load dispatch using Levenberg Marquardt algorithm . Energy Procedia , 2018 , 144 : 95 – 103 .
Ruiz-Abellón MC , Fernández-Jiménez LA , Guillamón A , et al. . Integration of demand response and short-term forecasting for the management of prosumers’ demand and generation . Energies , 2020 , 13 : 11 .
Ali SS , Choi BJ. State-of-the-art artificial intelligence techniques for distributed smart grids: a review . Electronics , 2020 , 9 : 1030 .
Fu J , Nunez A , De Schutter B. A short-term preventive maintenance scheduling method for distribution networks with distributed generators and batteries . IEEE Trans Power Syst , 2020 , 36 : 2516 – 2531 .
Esmaili M , Shayanfar HA , Moslemi R. Locating series FACTS devices for multi-objective congestion management improving voltage and transient stability . Eur J Oper Res , 2014 , 236 : 763 – 773 .
Suresh K , Kumarappan N. Hybrid improved binary particle swarm optimization approach for generation maintenance scheduling problem . Swarm Evol Comput , 2013 , 9 : 69 – 89 .
Lakshminarayanan S , Kaur D. Optimal maintenance scheduling of generator units using discrete integer cuckoo search optimization algorithm . Swarm Evol Comput , 2018 , 42 : 89 – 98 .
Scalabrini Sampaio G , Vallim Filho ARDA , Santos da Silva L , et al. . Prediction of motor failure time using an artificial neural network . Sensors , 2019 , 19 : 4342 .
Fikri M , Cheddadi B , Sabri O. Predicting Moroccan real network’s power flow employing the artificial neural networks . In: 2019 Third International Conference on Intelligent Computing in Data Sciences (ICDS), Marrakech, Morocco, 28–30 October 2019 , 1 – 6 .
Rahul J , Sharma Y , Birla D. A new attempt to optimize optimal power flow-based transmission losses using genetic algorithm . In: 2012 Fourth International Conference on Computational Intelligence and Communication Networks, Mathura, India, 3–5 November 2012 , 566 – 570 .
Nakawiro W , Erlich I. A combined GA-ANN strategy for solving optimal power flow with voltage security constraint . In: 2009 Asia-Pacific Power and Energy Engineering Conference, Wuhan, China, 27–31 March 2009 , 1 – 4 .
Sumpavakup C , Srikun I , Chusanapiputt S. A solution to the optimal power flow using artificial bee colony algorithm . In: 2010 International Conference on Power System Technology, Zhejiang, China, 24–28 October 2010 , 1 – 5 .
Abdellah D , Djamel L. Power flow analysis using adaptive neuro-fuzzy inference systems . In: 2015 3rd International Renewable and Sustainable Energy Conference (IRSEC), Marrakech, Morocco, 10–13 December 2015 , 1 – 5 .
Nemati M , Braun M , Tenbohlen S. Optimization of unit commitment and economic dispatch in microgrids based on genetic algorithm and mixed integer linear programming . Appl Energy , 2018 , 210 : 944 – 963 .
Alshareef A. An application of artificial intelligent optimization techniques to dynamic unit commitment for the western area of Saudi Arabia . In: 2011 Third International Conference on Computational Intelligence, Communication Systems and Networks, Bali, Indonesia, 26–28 July 2011 , 17 – 21 .
Arora I , Kaur M. Unit commitment scheduling by employing artificial neural network-based load forecasting . In: 2016 7th India International Conference on Power Electronics (IICPE), Patiala, India, 17–19 November 2016 , 1 – 6 .
Liu Z , Li N , Zhang C. Unit commitment scheduling using a hybrid ANN and Lagrangian relaxation method . In: 2008 International Conference on Multimedia and Ubiquitous Engineering (mue 2008), Busan, South Korea, 24–25 April 2008 , 481 – 484 .
Kumar VS , Mohan MR. Solution to security constrained unit commitment problem using genetic algorithm . International Journal of Electrical Power & Energy Systems , 2010 , 32 : 117 – 125 .
Prakash S , Sinha SK. Application of artificial intelligence in load frequency control of interconnected power system . International Journal of Engineering, Science and Technology , 2011 , 3 : 264 – 275 .
Moshtagh J , Rafinia A. A new approach to high impedance fault location in three-phase underground distribution system using combination of fuzzy logic & wavelet analysis . In: 2012 11th International Conference on Environment and Electrical Engineering, Venice, Italy, 15–18 May 2012 , 90 – 97 .
Santoso NT , Tan OT. Neural-net based realtime control of capacitors installed on distribution systems . IEEE Trans. Power Delivery , 1990 , 5 : 266 – 272 .
Ng HN , Salama MMA , Chikhani AY. Capacitor allocation by approximate reasoning: fuzzy capacitor placement . IEEE Trans Power Deliv , 2000 , 15 : 393 – 398 .
Zhang H , Zhang L , Meng F. Reactive power optimization based on genetic algorithm . In: POWERCON’98. International Conference on Power System Technology. Proceedings, Vol. 2 , Beijing, China, 18–21 August 1998 , 1448 – 1453 .
Mamandur KRC , Chenoweth RD. Optimal control of reactive power flow for improvements in voltage profiles and for real power loss minimization . IEEE Transactions on Power Apparatus and Systems, 1981 , PAS-100 : 3185 – 3194 .
Kothakotla V , Kumar B. Analysis and design of robust PID controller for grid voltage control of islanded microgrid using genetic algorithm . In: 2021 5th International Conference on Intelligent Computing and Control Systems (ICICCS), Madurai, India, 6–8 May 2021 , 719 – 726 .
Wang S , Duan J , Shi D , et al. . A data-driven multi-agent autonomous voltage control framework using deep reinforcement learning . IEEE Trans Power Syst , 2020 , 35 : 4644 – 4654 .
Zidani Y , Zouggar S , Elbacha A. Steady-state analysis and voltage control of the self-excited induction generator using artificial neural network and an active filter . Iranian Journal of Science and Technology, Transactions of Electrical Engineering , 2018 , 42 : 41 – 48 .
Sumathi S. Artificial neural network application for voltage control and power flow control in power systems with UPFC . In: 2015 International Conference on Emerging Research in Electronics, Computer Science and Technology (ICERECT), Mandya, India, 17–19 December 2015 , 403 – 407 .
Kanata S , Sianipar GH , Maulidevi NU. Optimization of reactive power and voltage control in power system using hybrid artificial neural network and particle swarm optimization . In: 2018 2nd International Conference on Applied Electromagnetic Technology (AEMT), Lombok, Indonesia, 9–12 April 2018 , 67 – 72 .
Abdalla OH , Ghany AA , Fayek HH. Coordinated PID secondary voltage control of a power system based on genetic algorithm . In: 2016 Eighteenth International Middle East Power Systems Conference (MEPCON), Cairo, Egypt, 27–29 December 2016 , 214 – 219 .
Chung IY , Liu W , Cartes DA. Control parameter optimization for a microgrid system using particle swarm optimization . In: 2008 IEEE International Conference on Sustainable Energy Technologies, Singapore, 24–27 November 2008 , 837 – 842 .
Yousuf H , Zainal AY , Alshurideh M , et al. . Artificial intelligence models in power system analysis . In: Hassanien AE , Bhatnagar R , Darwish A (eds). Artificial Intelligence for Sustainable Development: Theory, Practice and Future Applications . Cham, Switzerland : Springer , 2021 , 231 – 242 .
Aakula JL , Khanduri A , Sharma A. Determining reactive power levels to improve bus voltages using PSO . In: 2020 IEEE 17th India Council International Conference (INDICON), New Delhi, India, 10–13 December 2020 , 1 – 7 .
Karthikeyan R , Pasam S , Sudheer S , et al. . Fuzzy fractional order PID based parallel cascade control system . In: Thampi S , Abraham A , Pal S , Rodriguez J (eds). Recent Advances in Intelligent Informatics. Advances in Intelligent Systems and Computing , Vol. 235 . Cham, Switzerland : Springer , 2014 , 293 – 302 .
Sallama A , Abbod M , Taylor G. Supervisory power system stability control using neuro-fuzzy system and particle swarm optimization algorithm . In: 2014 49th International Universities Power Engineering Conference (UPEC), Cluj-Napoca, Romania, 2–5 September 2014 , 1 – 6 .
Chen B , Li W , Yu P , et al. . Planning approach for cross-regional optical transmission network supporting wide-area stability control services in power system . In: 2018 International Conference on Network Infrastructure and Digital Content (IC-NIDC), Guiyang, China, 22–24 August 2018 , 105 – 109 .
Torkzadeh R , NasrAzadani H , Aliabad AD , et al. . A genetic algorithm optimized fuzzy logic controller for UPFC in order to damp of low frequency oscillations in power systems . In: 2014 22nd Iranian Conference on Electrical Engineering (ICEE), Tehran, Iran, 20–22 May 2014 , 706 – 712 .
Dutta S , Singh SP. Optimal rescheduling of generators for congestion management based on particle swarm optimization . IEEE Trans Power Syst , 2008 , 23 : 1560 – 1569 .
Nam S , Hur J. Probabilistic forecasting model of solar power outputs based on the naive Bayes classifier and kriging models . Energies , 2018 , 11 : 2982 .
Miraftabzadeh SM , Longo M , Foiadelli F , et al. . Advances in the application of machine learning techniques for power system analytics: a survey . Energies , 2021 , 14 : 4776 .
Safari A , Babaei F , Farrokhifar M. A load frequency control using a PSO-based ANN for micro-grids in the presence of electric vehicles . Int J Ambient Energy , 2021 , 42 : 688 – 700 .
Joshi M , Sharma G , Davidson IE. Load frequency control of hydro electric system using application of fuzzy with particle swarm optimization algorithm . In: 2020 International Conference on Artificial Intelligence, Big Data, Computing and Data Communication Systems (icABCD), Durban, South Africa, 6–7 August 2020 , 1 – 6 .
Nguyen GN , Jagatheesan K , Ashour AS , et al. . Ant colony optimization-based load frequency control of multi-area interconnected thermal power system with governor dead-band nonlinearity . In: Yang XS , Nagar AK , Joshi A (eds). Smart Trends in Systems, Security and Sustainability . Singapore : Springer , 2018 , 157 – 167 .
Balamurugan CR. Three area power system load frequency control using fuzzy logic controller . International Journal of Applied Power Engineering (IJAPE) , 2018 , 7 : 18 – 26 .
Otani T , Tanabe R , Koyanagi Y , et al. . Cooperative load frequency control of generator and battery using a recurrent neural network . In: TENCON 2017–2017 IEEE Region 10 Conference, Penang, Malaysia, 5–8 November 2017 , 918 – 923 .
Kumar D , Mathur HD , Bhanot S , et al. . Forecasting of solar and wind power using LSTM RNN for load frequency control in isolated microgrid . Int J Modelling Simul , 2021 , 41 : 311 – 323 .
Arora K , Kumar A , Kamboj VK , et al. . Optimization methodologies and testing on standard benchmark functions of load frequency control for interconnected multi area power system in smart grids . Mathematics , 2020 , 8 : 980 .
Vadivelu KR , Marutheswar GV. Artificial intelligence technique based reactive power planning incorporating FACTS controllers in real time power transmission system . In: 2014 IEEE 2nd International Conference on Electrical Energy Systems (ICEES), Chennai, India, 7–9 January 2014 , 26 – 31 .
Khan MA , Algarni F. A healthcare monitoring system for the diagnosis of heart disease in the IoMT cloud environment using MSSO-ANFIS . IEEE Access , 2020 , 8 : 122259 – 122269 .
Liu M , Dong M , Wu C. A new ANFIS for parameter prediction with numeric and categorical inputs . IEEE Trans Autom Sci Eng , 2010 , 7 : 645 – 653 .
Ghadiri A , Haghifam MR , Larimi SMM. Comprehensive approach for hybrid AC/DC distribution network planning using genetic algorithm . IET Generation, Transmission & Distribution , 2017 , 11 : 3892 – 3902 .
Jain SK , Bhargava A , Pal RK. Three area power system load frequency control using fuzzy logic controller . In: 2015 International Conference on Computer, Communication and Control (IC4), Indore, India, 10–12 September 2015 , 1 – 6 .
Son YS , Kim HJ , Kim JT. A video-quality control scheme using ANFIS architecture in a DASH environment . Journal of Broadcast Engineering , 2018 , 23 : 104 – 114 .
Kannadasan K , Edla DR , Yadav MH , et al. . Intelligent-ANFIS model for predicting measurement of surface roughness and geometric tolerances in three-axis CNC milling . IEEE Trans Instrum Meas , 2020 , 69 : 7683 – 7694 .
Penghui L , Ewees AA , Beyaztas BH , et al. . Metaheuristic optimization algorithms hybridized with artificial intelligence model for soil temperature prediction: novel model . IEEE Access , 2020 , 8 : 51884 – 51904 .
Tawfeek TS , Ahmed AH , Hasan S. Analytical and particle swarm optimization algorithms for optimal allocation of four different distributed generation types in radial distribution networks . Energy Procedia , 2018 , 153 : 86 – 94 .
Yeom CU , Kwak KC. Adaptive neuro-fuzzy inference system predictor with an incremental tree structure based on a context-based fuzzy clustering approach . Applied Sciences , 2020 , 10 : 8495 .
Krasopoulos CT , Beniakar ME , Kladas AG. Multicriteria PM motor design based on ANFIS evaluation of EV driving cycle efficiency . IEEE Trans Transp Electrif , 2018 , 42 : 525 – 535 .
El-Hasnony IM , Barakat SI , Mostafa . Optimized ANFIS model using hybrid metaheuristic algorithms for Parkinson’s disease prediction in IoT environment . IEEE Access , 2020 , 8 : 119252 – 119270 .
Morshedizadeh M , Kordestani M , Carriveau R , et al. . Power production prediction of wind turbines using a fusion of MLP and ANFIS networks . IET Renew Power Gener , 2018 , 12 : 1025 – 1033 .
Khosravi A , Nahavandi S , Creighton D. Prediction interval construction and optimization for adaptive neurofuzzy inference systems . IEEE Trans Fuzzy Syst , 2011 , 19 : 983 – 988 .
Elbaz K , Shen SL , Sun WJ , et al. . Prediction model of shield performance during tunneling via incorporating improved particle swarm optimization into ANFIS . IEEE Access , 2020 , 8 : 39659 – 39671 .
Dovžan D , Škrjanc I. Fuzzy space partitioning based on hyperplanes defined by eigenvectors for Takagi-Sugeno fuzzy model identification . IEEE Trans Ind Electron , 2019 , 67 : 5144 – 5153 .
Castiello C , Fanelli AM , Lucarelli M , et al. . Interpretable fuzzy partitioning of classified data with variable granularity . Appl Soft Comput , 2019 , 74 : 567 – 582 .
Alexandridis A , Chondrodima E , Sarimveis H. Radial basis function network training using a nonsymmetric partition of the input space and particle swarm optimization . IEEE Trans Neural Networks Learn Syst , 2012 , 24 : 219 – 230 .
Verstraete J. The spatial disaggregation problem: simulating reasoning using a fuzzy inference system . IEEE Trans Fuzzy Syst , 2016 , 25 : 627 – 641 .
Olamaei J , Moradi M , Kaboodi T. A new adaptive modified firefly algorithm to solve optimal capacitor placement problem . In: 18th Electric Power Distribution Conference, Kermanshah, Iran, 30 April–1 May 2013 , 1 – 6 .
Lee JS , Teng CL. An enhanced hierarchical clustering approach for mobile sensor networks using fuzzy inference systems . IEEE Internet Things J , 2017 , 4 : 1095 – 1103 .
Su ZG , Denoeux T. BPEC. Belief-peaks evidential clustering . IEEE Trans Fuzzy Syst , 2018 , 27 : 111 – 123 .
Xu P , Deng Z , Cui C , et al. . Concise fuzzy system modeling integrating soft subspace clustering and sparse learning . IEEE Trans Fuzzy Syst , 2019 , 27 : 2176 – 2189 .
Sujil A , Kumar R , Bansal RC. FCM clustering-ANFIS-based PV and wind generation forecasting agent for energy management in a smart microgrid . The Journal of Engineering , 2019 , 2019 : 4852 – 4857 .
Neamatollahi P , Naghibzadeh M , Abrishami S. Fuzzy-based clustering-task scheduling for lifetime enhancement in wireless sensor networks . IEEE Sens J , 2017 , 17 : 6837 – 6844 .
Wang Z , Xu Y. A golden section-based double population genetic algorithm applied to reactive power optimization . IOP Conference Series: Earth and Environmental Science, 2021 , 645 : 012074 .
Kahouli O , Alsaif H , Bouteraa Y , et al. . Power system reconfiguration in distribution network for improving reliability using genetic algorithm and particle swarm optimization . Applied Sciences , 2021 , 11 : 3092 .
Žarković SD , Stanković S , Shayesteh E , et al. . Reliability improvement of distribution system through distribution system planning: MILP vs. GA . In: 2019 IEEE Milan PowerTech, Milan, Italy, 23–27 June 2019, 1 – 6 .
Ahmetovic H , Saric M , Hivziefendic J. Reliability based power distribution network planning using fuzzy logic . Advances in Electrical and Electronic Engineering , 2021 , 19 : 123 – 133 .
Suresh M , Sirish TS , Subhashini TV , et al. . Load flow analysis of distribution system using artificial neural networks . In: Satapathy SC , Bhateja V , Udgata SK , Pattnaik PK (eds). Proceedings of the 5th International Conference on Frontiers in Intelligent Computing: Theory and Applications FICTA 2016, Vol. 1 . Springer , Singapore , 2017 , 515 – 524 .
Kumari M , Ranjan R , Singh VR. Optimal power distribution planning using improved particle swarm optimization . International Journal of Intelligent Systems and Applications in Engineering , 2018 , 3 : 170 – 177 .
Saha S , Mukherjee V. A novel multi-objective modified symbiotic organisms search algorithm for optimal allocation of distributed generation in radial distribution system . Neural Comput Appl , 2021 , 33 : 1751 – 1771 .
Hosseini MM , Parvania M. Artificial intelligence for resilience enhancement of power distribution systems . The Electricity Journal , 2021 , 34 : 106880 .
Lytras MD , Chui KT. The recent development of artificial intelligence for smart and sustainable energy systems and applications . Energies , 2019 , 12 : 3108 .
Gandhar S , Ohri J , Singh M. Dynamic reactive power optimization of hybrid micro grid in islanded mode using fuzzy tuned UPFC . J Inf Optim Sci , 2020 , 41 : 305 – 315 .
Tang Y , Hu W , Cao D , et al. . Artificial intelligence-aided minimum reactive power control for the DAB converter based on harmonic analysis method . IEEE Trans Power Electron , 2021 , 36 : 9704 – 9710 .
Harrye YA , Ahmed KH , Aboushady AA. Reactive power minimization of dual active bridge DC/DC converter with triple phase shift control using neural network . In: 2014 International Conference on Renewable Energy Research and Application (ICRERA), Milwaukee, WI, USA, 19–22 October 2014 , 566 – 571 .
Wang Y , Li Y. A hybrid ant colony optimization algorithm for dynamic optimization of vehicle routing problem with time windows . Applied Intelligence , 2021 , 51 : 476 – 491 .
Sharma NK , Babu DS , Choube SC. Application of particle swarm optimization technique for reactive power optimization . In: IEEE International Conference on Advances in Engineering, Science and Management (ICAESM-2012), Nagapattinam, India, 30–31 March 2020 , 88 – 93 .
Bhattacharyya B , Gupta VK. Fuzzy based evolutionary algorithm for reactive power optimization with FACTS devices . International Journal of Electrical Power & Energy Systems , 2014 , 61 : 39 – 47 .
Bharti D. Multi-point optimal placement of shunt capacitor in radial distribution network: a comparison . In: 2020 International Conference on Emerging Frontiers in Electrical and Electronic Technologies (ICEFEET), Patna, India, 10–11 July 2020 , 1 – 6 .
Roy PK , Sultana S. Optimal reconfiguration of capacitor based radial distribution system using chaotic quasi oppositional chemical reaction optimization . Microsyst Technol , 2022 , 28 : 499 – 511 .
Pimentel Filho MC , De Lacerda EGM , Junior MM. Capacitor placement using ant colony optimization and gradient . In: 2009 15th International Conference on Intelligent System Applications to Power Systems, Curitiba, Brazil, 8–12 November 2009 , 1 – 4 .
Isac SJ , Kumar KS , Kumar PV. Optimal capacitor placement in radial distribution system to minimize the loss using fuzzy logic control . In: International Conference on Smart Structures and Systems—ICSSS; 13 , Chennai, India, 28–29 March 2013 , 33 – 40 .
Reddy MD , Reddy VV. Capacitor placement using fuzzy and particle swarm optimization method for maximum annual savings . ARPN Journal of Engineering and Applied Sciences , 2008 , 3 : 25 – 30 .
Shwehdi MH , Mohamed SR , Devaraj D. Optimal capacitor placement on West–East inter-tie in Saudi Arabia using genetic algorithm . Computers & Electrical Engineering , 2018 , 68 : 156 – 169 .
Mahdavian M , Kafi MH , Movahedi A , et al. . Improve performance in electrical power distribution system by optimal capacitor placement using genetic algorithm . In: 2017 14th International Conference on Electrical Engineering/Electronics, Computer, Telecommunications, and Information Technology (ECTI-CON), Phuket, Thailand, 27–30 June 2017 , 749 – 752 .
Email alerts
Citing articles via.
- Advertising and Corporate Services
Affiliations
- Online ISSN 2515-396X
- Print ISSN 2515-4230
- Copyright © 2024 National Institute of Clean-and-Low-Carbon Energy
- About Oxford Academic
- Publish journals with us
- University press partners
- What we publish
- New features
- Open access
- Institutional account management
- Rights and permissions
- Get help with access
- Accessibility
- Advertising
- Media enquiries
- Oxford University Press
- Oxford Languages
- University of Oxford
Oxford University Press is a department of the University of Oxford. It furthers the University's objective of excellence in research, scholarship, and education by publishing worldwide
- Copyright © 2024 Oxford University Press
- Cookie settings
- Cookie policy
- Privacy policy
- Legal notice
This Feature Is Available To Subscribers Only
Sign In or Create an Account
This PDF is available to Subscribers Only
For full access to this pdf, sign in to an existing account, or purchase an annual subscription.
IEEE.org | IEEE Xplore Digital Library | IEEE Standards | IEEE Spectrum | More Sites
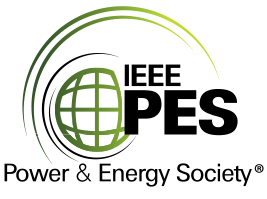
Committees & Communities
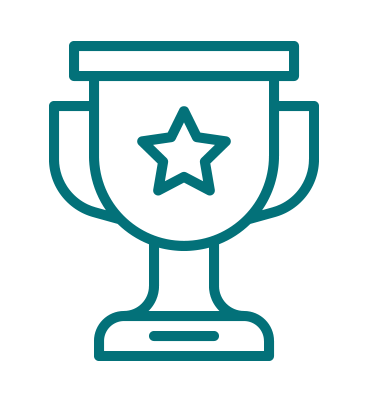
IEEE PES Awards & Scholarships
Every year we are proud to recognize leading engineers with distinct awards and honors and to offer scholarships to cultivate the next generation of power and energy professionals.
View All Awards >
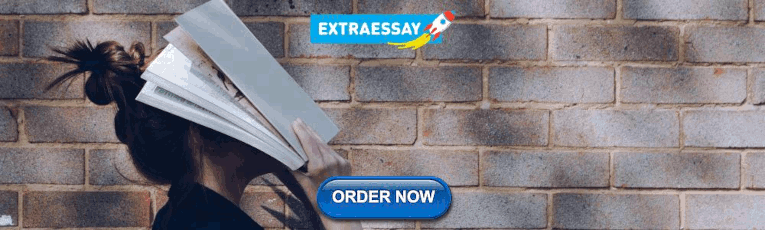
About Membership
Membership programs, member support tools.
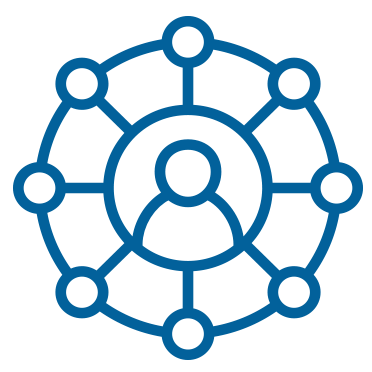
Become A PES Member
You’re invited to join the leading global network of +42k power and energy students and professionals.
Get Started Today
Technical Activities
Technical reports, trending technologies.
Latest Posts:
Risk-Informed Standard for Nuclear Power Plants
Virtual Power Plants
Lifetime Extension of Electric Power Equipment
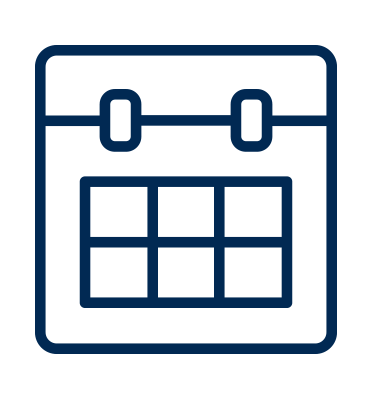
Upcoming Committee Meetings
Visit our calendar for information on upcoming technical committee meetings.
Calendar of Events >
About Conferences & Meetings
Papers, participation & proposals.
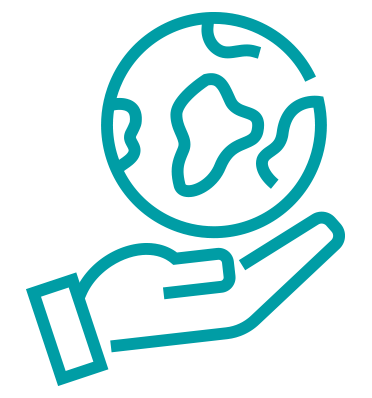
Event Sponsorship & Exhibitor Opportunities
With over 30 PES global events annually, we offer many sponsorship opportunities.
Support IEEE PES >
About Publications
Journals & transactions, education overview & resources, about pes university.
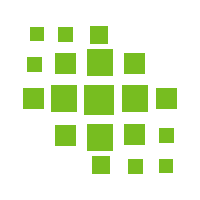
Visit the Resource Center
The largest digital library dedicated to the power and energy industry, find the resource you’ve been looking for!
New! Interactive Online Courses
A new series of interactive continuing education courses for those non-engineers and adjacent professionals working in the power industry.
View All >
IEEE Transactions on Power Systems
Home / Publications / IEEE Transactions on Power Systems
Impact Factor: 6.5
The mission of the IEEE Transactions on Power Systems is to serve the whole Power System community, including researchers, practitioners, educators and students, by publishing and disseminating insightful research results of lasting value.
IEEE Transactions on Power Systems (TPWRS) welcomes papers on the education, analysis, operation, planning, and economics of electric generation, transmission, and distribution systems for general industrial, commercial, public, and domestic consumption, including the interaction with multi-energy carriers. The focus of TPWRS is the power system from a systems viewpoint instead of components of the system. It has five (5) key areas within its scope including:
- Power System Analysis, Computing, and Economics,
- Power System Dynamic Performance,
- Power System Operations,
- Power System Planning and Implementation,
- Power Engineering Education.
TPWRS’s editorial philosophy is to promote research, innovation, and exchange for the power engineering community. We believe the purpose of paper review and publication is to stimulate further development or applications by others. Therefore, if a paper has adequate contributions, is technically sound, and does not mislead readers, it deserves a chance for publication by TPWRS. We are working to make the review processes as clear and as fair as possible, improving the experiences for both authors and reviewers.
Technical Areas & Associated Topics
Topics within the journal scope:, power system analysis, computing, and economics.
- Power system modelling
- Power system analysis (e.g., load flow formulations and implementation)
- Techno-economic aspects of congestion management and redispatch
- Development of data and scenarios for system expansion
- Transmission and distribution system analysis
- Techno-economic aspects of Risk management
- Reliability and resilience
- Uncertainty and probability
- Stochastic system and optimization applications
- Intelligent system applications
- Computing applications
Power System Dynamic Performance
- Power system dynamic modeling: components and systems
- Power system stability: phenomena, analysis, and techniques
- Power system stability controls: design and applications
- Power system dynamic measurements
- Power system interaction with conventional and renewable generators
- Dynamic security assessment: techniques and applications, risk-based methods
Power System Operations
- Emerging methods for restructured systems
- Transmission system operation and security
- Distribution system operation
- Energy control centers
- Static and dynamic state estimation
- Transmission and distribution system control
Power System Planning & Implementation
- Computational techniques and analytical methods for system planning
- Generation system resource planning
- Transmission system expansion and planning
- Distribution system expansion and planning
- Customer products and services planning and implementation
- Industry restructuring planning
- Integrated and distributed resource planning
- Techno-economic aspects of Integrated resource planning
- Load forecasting, management and aggregation
Power Engineering Education
- New instruction methods (software/internet/laboratory/combined with research)
- Virtual classrooms/laboratories
- Distance education
- Life-long learning
Examples of topics out-of-scope:
- Power quality: harmonics, flicker, voltage dips, voltage sags, etc.
- Protection: all types of protection relays, circuit breakers, fuses, protection coordination, etc.
- Fault current calculations
- Lightning protection
- Fast electromagnetic phenomena excluding inverter-driven power system stability
- Smart sensors, smart meters, AMI infrastructures
- Blockchain technologies
- Rural electrification
- Energy communities
- Platforms for local trading
- Market specific aspects of congestion management and redispatch
- Market specific aspects of risk management
- Policy, socioeconomic and macroeconomic and regulatory aspects of resource planning
- Global power system economics, principles of market organization and cost structure
Peer Review
The articles in this journal are peer reviewed in accordance with the requirements set forth in the IEEE Publication Services and Products Board Operations Manual ( https://pspb.ieee.org/images/files/files/opsmanual.pdf ). Each published article was reviewed by a minimum of two independent reviewers using a single-anonymous peer review process, where the identities of the reviewers are not known to the authors, but the reviewers know the identities of the authors. Articles will be screened for plagiarism before acceptance.
About resubmission of a rejected paper Rejected papers should not be resubmitted without being substantially revised. After revision, rejected papers can be resubmitted no sooner than 3 months after the date of the rejection. A resubmitted paper will be treated as new submission and must adhere to the same page limit as any other newly submitted paper. If the paper is rejected for the second time, the paper cannot be resubmitted any more even if further modified. The authors must also indicate in the letter to the editor how the paper has been modified. Since the resubmitted paper is not treated as a revision, the authors should not include a separate document in which they address the comments of the reviewers. All modifications should be briefly stated in the letter to the editor. If the paper was rejected from one of the PES journals and the authors have revised the paper and resubmit it to another PES journal they must also indicate in the letter to the editor which journal the paper was submitted to originally and what was the reference number of the rejected paper.
Policy on papers published at PES financially sponsored conferences: The Authors who have presented the paper(s) at the IEEE PES financially sponsored conference including PES General Meeting, PES Innovative Smart Grid Technologies (USA, Latin America, Europe, Asia), PES PowerTech and PES PowerAfrica are eligible to submit the extended version of their conference paper(s) to one of IEEE PES Transactions for consideration of publication. The extended paper requires at least 60% additional new material relative to the original conference paper and is subject to a regular review process of the respective PES Transactions. The original conference paper should be referenced in the extended journal paper and new contributions with respect to the conference paper clearly identified. The final decision for publication on the extended paper is made by the Editor-in-Chief of the respective journal.
Open Access
This publication is a hybrid journal, allowing either Traditional manuscript submission or Open Access (author-pays OA) manuscript submission.
The OA option, if selected, enables unrestricted public access to the article via IEEE Xplore. The OA option will be offered to the author at the time the manuscript is accepted. If selected, the OA fee of $2,495 must be paid before the article is published in the journal. If you have unusual circumstances about this, please contact the Editor-in-Chief. Any other applicable charges (such as the overlength page charge and/or charge for the use of color in the print format) will be billed separately once the manuscript formatting is complete but prior to the publication.
The traditional option, if selected, enables access to all qualified subscribers and purchasers via IEEE Xplore. No OA payment is required.
Table of Contents & Abstracts
Volume 39, Number 5, September 2024
Volume 39, Number 4, July 2024
Volume 39, Number 3, May 2024
Volume 39, Number 2, March 2024
Volume 39, Number 1, January 2024
Volume 38, Number 6, November 2023
Volume 38, Number 5, September 2023
Volume 38, Number 4, July 2023
Volume 38, Number 3, May 2023
Volume 38, Number 2, March 2023
Volume 38, Number 1, January 2023
Volume 37, Number 6, November 2022
Volume 37, Number 5, September 2022
Volume 37, Number 4, July 2022
Volume 37, Number 3, May 2022
Volume 37, Number 2, March 2022
Volume 37, Number 1, January 2022
Editorial Board
IEEE Transactions on Power Systems Associate Editors: Mads R. Almassalkhi, University of Vermont, Burlington, USA Farrokh Aminifar, Quanta Technology LLC, USA Turaj Amraee, K.N. Toosi University of Technology, Iran Leandro Ramos de Araujo, Federal University of Juiz de Fora, Brazil Rui Bo, Missouri University of Science and Technology, USA Siqi Bu, Hong Kong Polytechnic University, Hong Kong S.A.R., China Fei Ding, National Renewable Energy Laboratory, USA Anamika Dubey, Washington State University, USA Miao Fan, Siemens Industry Inc., USA Hêmin Golpîra, University of Kurdistan, Iran Chenghong Gu, University of Bath, UK Nikos Hatziargyriou, National Technical University of Athens, Greece Yunhe Hou, The University of Hong Kong, Hong Kong S.A.R., China Bo Hu, Chongqing University, China Lin Jiang, University of Liverpool, UK Tao Jiang, Northeast Electric Power University, China Vassilis Kekatos, Purdue University, USA Mert Korkali, University of Missouri, USA Georgios Kryonidis, Aristotle University of Thessaloniki, Greece Shunbo Lei, Chinese University of Hong Kong, Shenzhen, China Victor Levi, The University of Manchester, UK Qifeng Li, University of Central Florida, USA Zhengshuo Li, Shandong University, China Zhenzhi Lin, Zhejiang University, China Feng Liu, Tsinghua University, China Lasantha Meegahapola, RMIT University, Australia Behnam Mohammadi-Ivatloo, LUT University, Finland Hamed Mohsenian-Rad, University of California, Riverside, USA Thomas Morstyn, University of Edinburgh, UK Alexandre Nassif, LUMA Energy, Puerto Rico Michael Negnevitsky, University of Tasmania, Australia Marina Oluic, Swedish National Grid, Sweden Anamitra Pal, Arizona State University, USA Bikash C. Pal, Imperial College London, UK Hrvoje Pandžić, University of Zagreb Faculty of Electrical Engineering and Computing, Croatia Masood Parvania, University of Utah, USA Jimmy Peng, National University of Singapore, Singapore David Pozo, EC Joint Research Centre, Belgium Junjian Qi, South Dakota State University, USA Elyas Rakhshani, HESStec, Spain Nima Safari, Alberta Electric System Operator, Canada Dean Sharafi, Australian Energy Market Operator, Australia Di Shi, New Mexico State University, USA Pierluigi Siano, University of Salerno, Italy Wei Sun, University of Central Florida, USA Fei Teng, Imperial College London, UK Alfredo Vaccaro, University of Sannio, Italy Vahid Vahidinasab, Nottingham Trent University, UK François Vallee, University of Mons, Belgium Gustavo Valverde, ETH Zurich, Switzerland Gregor Verbic, University of Sydney, Australia Cheng Wang, North China Electric Power University, China Qin Wang, Electric Power Research Institute, USA Shaobu Wang, Pacific Northwest National Laboratory, USA Zhaojian Wang, Shanghai Jiao Tong University, China Wei Wei, Tsinghua University, China Qiuwei Wu, Tsinghua University, China Bolun Xu, Columbia University, USA Yan Xu, Nanyang Technological University, Singapore Yin Xu, Beijing Jiaotong University, China Ying Xue, South China University of Technology, China Koji Yamashita, University of California, Riverside, USA Ruifeng Yan, University of Queensland, Australia Ming Yang, Shandong University, China Hongcai Zhang, University of Macau, China Yichen Zhang, University of Texas at Arlington, USA Junbo Zhao, University of Connecticut, USA Jiebei Zhu, Tianjin University, China
How to become an Associate Editor for a PES Transactions
Outstanding Reviewers for 2023
Seyed Mohammad Azimi, Hamedan University of Technology Luis Badesa, Polytechnic University of Madrid University Murilo E. C. Bento, Federal University of Rio de Janeiro Siqi Bu, Hong Kong Polytechnic University Florin Capitanescu, Luxembourg Institute of Science and Technology Sheng Chen, Hohai University Yue Chen, The Chinese University of Hong Kong Ershun Du, Tsinghua University Lei Fan, University of Houston Xin Fang, Mississippi State University Mohammadreza Fathizadan, Stony Brook University Gerald Heydt, Arizona State University Jiazuo Hou, National University of Singapore Adirak Kanchanaharuthai, Rangsit University Mert Korkali, University of Missouri Peng Kou, State Key Laboratory of Electrical Insulation and Power Equipment Yang Li, Northeast Electric Power University Zhengmao Li, Nanyang Technological University Yuzhang Lin, New York University Yun Liu, South China University of Technology Zhaoxi Liu, South China University of Technology Majid Majidi, The University of Utah Nikolaos Manousakis, University of West Attica Faculty of Engineering Majid Moazzami, Islamic Azad University Najafabad Branch Marina Oluic, Swedish National Grid Anamitra Pal, Arizona State University Kai Pan, Hong Kong Polytechnic University Byungkwon Park, Soongsil University Marco Pau, Fraunhofer Institute for Energy Economics and Energy System Technology Jing Qiu, The University of Sydney Yiwei Qiu, Sichuan University Elyas Rakhshani, Delft University of Technology Guangchun Ruan, Massachusetts Institute of Technology Sahaj Saxena, Tel Aviv University Qingxin Shi, North China Electric Power University Tong Su, University of Connecticut Bendong Tan, University of Connecticut Zhenfei Tan, Shanghai Jiao Tong University Fei Teng, Imperial College London Fernanda Trindade, University of Campinas Vijay Vittal, Arizona State University Cheng Wang, North China Electric Power University Siyuan Wang, Johns Hopkins University Weiyu Wang, Changsha University of Science and Technology Yishen Wang, Smart Grid Research Institute Yu Wang, Chongqing University Linyun Xiong, Chongqing University Bolun Xu, Columbia University Xiaoyuan Xu, Shanghai Jiao Tong University Ying Xue, South China University of Technology Koji Yamashita, University of California Riverside Mingyu Yan, Huazhong University of Science and Technology Ziming Yan, Nanyang Technological University Yang Yang, National University of Singapore Zhifang Yang, Chongqing University Wei Yao, Huazhong University of Science and Technology Yiyun Yao, National Renewable Energy Laboratory Yujian Ye, Southeast University Yuxuan Yuan, Iowa State University Baosen Zhang, University of Washington Cuo Zhang, The University of Sydney Jiawei Zhang, Tsinghua University Qianzhi Zhang, The University of Alabama Yao Zhang, Xi’an Jiaotong University Jin Zhao, Trinity College Dublin Bin Zhou, Hunan University Jiebei Zhu, Tianjin University
Outstanding Associate Editors for 2023
Javier Contreras, University of Castilla-La Mancha (Outgoing Associate Editor) Xin Fang, Mississippi State University (Outgoing Associate Editor) Hêmin Golpîra, University of Kurdistan Qinglai Guo, Tsinghua University (Outgoing Associate Editor) Innocent Kamwa, Université Laval (Outgoing Associate Editor) Lasantha Meegahapola, RMIT University Federico Milano, University College Dublin Marina Oluic, Swedish National Grid Hrvoje Pandžić, University of Zagreb Faculty of Electrical Engineering and Computing Jimmy Peng, National University of Singapore Elyas Rakhshani, HESStec, Spain Alfredo Vaccaro, University of Sannio Wei Wei, Tsinghua University Rui Yao, Google/X Development LLC (Outgoing Associate Editor) Peng Zhang, Stony Brook University (Outgoing Associate Editor) Yichen Zhang, University of Texas at Arlington Junbo Zhao, University of Connecticut
Recent Improvements
Click here to access a list of recent improvements to the IEEE Transactions on Power Systems .
Over-length Page Charges
Transactions papers are limited to 10 pages at first submission. Papers exceeding 10 pages will be returned without review. Revised papers submitted in response to Revise and Resubmit decisions may exceed 10 pages. For papers submitted 1 January, 2024 or later the over-length page charges are $250 per each page in excess of the first 12 published pages. For papers submitted 1 January, 2023–31 December, 2023 the over-length page charges are $200 per each page in excess of the first 10 published pages. Papers submitted prior to 2023 will have over-length page charges of $150 per page for anything in excess of the first 8 published pages.
Useful Links
Preparation and Submission of Transactions Papers
Templates and Sample of PES Technical Papers
Subscribe to the IEEE Transactions on Power Systems
Author’s Kit
The author’s kit is available to offer guidelines for the submission for all technical work for review.
LEARN MORE
Advertise In IEEE Magazines
Editorial and digital ad opportunities are available in our leading IEEE magazines.
Open Calls For Transactions
IEEE PES Transactions distribute the latest findings to researchers, engineers, and educators in the power and energy field.
© Copyright 2024 IEEE — All rights reserved. A not-for-profit organization, IEEE is the world’s largest technical professional organization dedicated to advancing technology for the benefit of humanity.
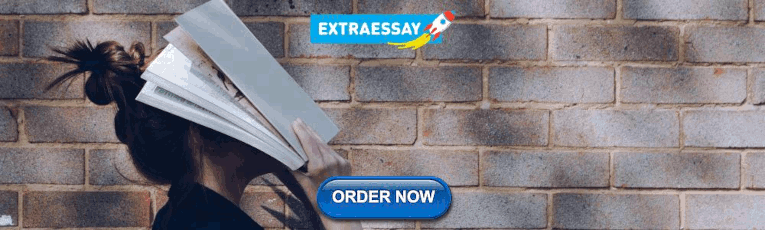
IMAGES
VIDEO
COMMENTS
Electric Power Systems Research is an international medium for the publication of original papers concerned with the generation, transmission, distribution and utilization of electrical energy.
As different artificial intelligence (AI) techniques continue to evolve, power systems are undergoing significant technological changes with the primary goal of reducing computational time, decreasing utility and consumer costs and ensuring the reliable operation of an electrical power system.
In this paper, the challenges foreseen for future power systems are identified and the most effective approaches to deal with them are reviewed.
First, this paper summarizes the device and system-level characteristics of the dual high-penetrated power systems, analyzes its impact on the classical power system stability, and discusses the emerging stability problems.
IEEE Transactions on Power Systems covers the education, analysis, operation, planning & economics of electric generation, transmission, and their distribution systems.
This research provides a detailed review of AI applications in power systems, particularly in stability, control, and protection, identifying key challenges and research gaps based on recent publications from 2017 to 2022.