
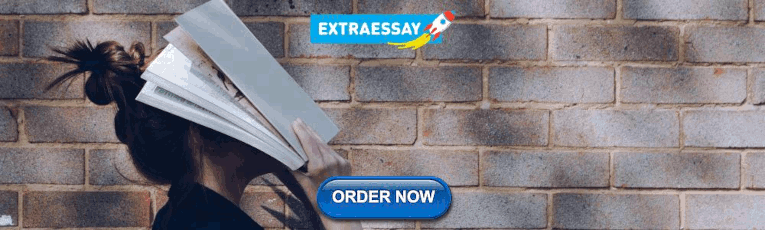
Research Variables 101
Independent variables, dependent variables, control variables and more
By: Derek Jansen (MBA) | Expert Reviewed By: Kerryn Warren (PhD) | January 2023
If you’re new to the world of research, especially scientific research, you’re bound to run into the concept of variables , sooner or later. If you’re feeling a little confused, don’t worry – you’re not the only one! Independent variables, dependent variables, confounding variables – it’s a lot of jargon. In this post, we’ll unpack the terminology surrounding research variables using straightforward language and loads of examples .
Overview: Variables In Research
What (exactly) is a variable.
The simplest way to understand a variable is as any characteristic or attribute that can experience change or vary over time or context – hence the name “variable”. For example, the dosage of a particular medicine could be classified as a variable, as the amount can vary (i.e., a higher dose or a lower dose). Similarly, gender, age or ethnicity could be considered demographic variables, because each person varies in these respects.
Within research, especially scientific research, variables form the foundation of studies, as researchers are often interested in how one variable impacts another, and the relationships between different variables. For example:
- How someone’s age impacts their sleep quality
- How different teaching methods impact learning outcomes
- How diet impacts weight (gain or loss)
As you can see, variables are often used to explain relationships between different elements and phenomena. In scientific studies, especially experimental studies, the objective is often to understand the causal relationships between variables. In other words, the role of cause and effect between variables. This is achieved by manipulating certain variables while controlling others – and then observing the outcome. But, we’ll get into that a little later…
The “Big 3” Variables
Variables can be a little intimidating for new researchers because there are a wide variety of variables, and oftentimes, there are multiple labels for the same thing. To lay a firm foundation, we’ll first look at the three main types of variables, namely:
- Independent variables (IV)
- Dependant variables (DV)
- Control variables
What is an independent variable?
Simply put, the independent variable is the “ cause ” in the relationship between two (or more) variables. In other words, when the independent variable changes, it has an impact on another variable.
For example:
- Increasing the dosage of a medication (Variable A) could result in better (or worse) health outcomes for a patient (Variable B)
- Changing a teaching method (Variable A) could impact the test scores that students earn in a standardised test (Variable B)
- Varying one’s diet (Variable A) could result in weight loss or gain (Variable B).
It’s useful to know that independent variables can go by a few different names, including, explanatory variables (because they explain an event or outcome) and predictor variables (because they predict the value of another variable). Terminology aside though, the most important takeaway is that independent variables are assumed to be the “cause” in any cause-effect relationship. As you can imagine, these types of variables are of major interest to researchers, as many studies seek to understand the causal factors behind a phenomenon.
Need a helping hand?
What is a dependent variable?
While the independent variable is the “ cause ”, the dependent variable is the “ effect ” – or rather, the affected variable . In other words, the dependent variable is the variable that is assumed to change as a result of a change in the independent variable.
Keeping with the previous example, let’s look at some dependent variables in action:
- Health outcomes (DV) could be impacted by dosage changes of a medication (IV)
- Students’ scores (DV) could be impacted by teaching methods (IV)
- Weight gain or loss (DV) could be impacted by diet (IV)
In scientific studies, researchers will typically pay very close attention to the dependent variable (or variables), carefully measuring any changes in response to hypothesised independent variables. This can be tricky in practice, as it’s not always easy to reliably measure specific phenomena or outcomes – or to be certain that the actual cause of the change is in fact the independent variable.
As the adage goes, correlation is not causation . In other words, just because two variables have a relationship doesn’t mean that it’s a causal relationship – they may just happen to vary together. For example, you could find a correlation between the number of people who own a certain brand of car and the number of people who have a certain type of job. Just because the number of people who own that brand of car and the number of people who have that type of job is correlated, it doesn’t mean that owning that brand of car causes someone to have that type of job or vice versa. The correlation could, for example, be caused by another factor such as income level or age group, which would affect both car ownership and job type.
To confidently establish a causal relationship between an independent variable and a dependent variable (i.e., X causes Y), you’ll typically need an experimental design , where you have complete control over the environmen t and the variables of interest. But even so, this doesn’t always translate into the “real world”. Simply put, what happens in the lab sometimes stays in the lab!
As an alternative to pure experimental research, correlational or “ quasi-experimental ” research (where the researcher cannot manipulate or change variables) can be done on a much larger scale more easily, allowing one to understand specific relationships in the real world. These types of studies also assume some causality between independent and dependent variables, but it’s not always clear. So, if you go this route, you need to be cautious in terms of how you describe the impact and causality between variables and be sure to acknowledge any limitations in your own research.
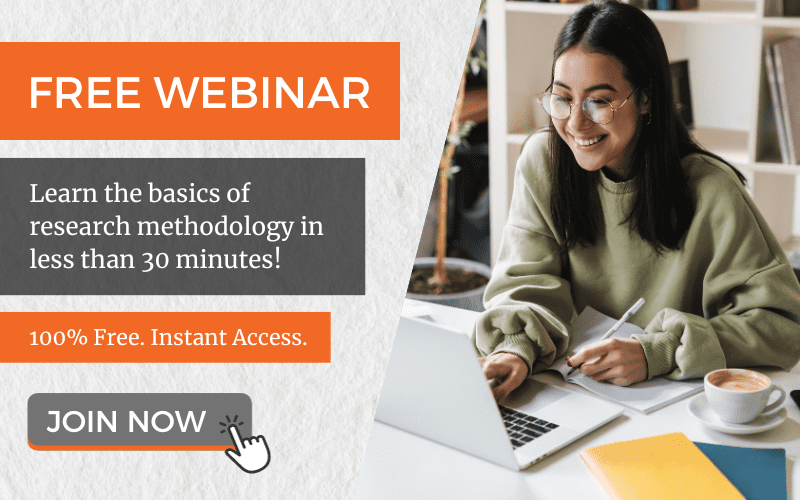
What is a control variable?
In an experimental design, a control variable (or controlled variable) is a variable that is intentionally held constant to ensure it doesn’t have an influence on any other variables. As a result, this variable remains unchanged throughout the course of the study. In other words, it’s a variable that’s not allowed to vary – tough life 🙂
As we mentioned earlier, one of the major challenges in identifying and measuring causal relationships is that it’s difficult to isolate the impact of variables other than the independent variable. Simply put, there’s always a risk that there are factors beyond the ones you’re specifically looking at that might be impacting the results of your study. So, to minimise the risk of this, researchers will attempt (as best possible) to hold other variables constant . These factors are then considered control variables.
Some examples of variables that you may need to control include:
- Temperature
- Time of day
- Noise or distractions
Which specific variables need to be controlled for will vary tremendously depending on the research project at hand, so there’s no generic list of control variables to consult. As a researcher, you’ll need to think carefully about all the factors that could vary within your research context and then consider how you’ll go about controlling them. A good starting point is to look at previous studies similar to yours and pay close attention to which variables they controlled for.
Of course, you won’t always be able to control every possible variable, and so, in many cases, you’ll just have to acknowledge their potential impact and account for them in the conclusions you draw. Every study has its limitations, so don’t get fixated or discouraged by troublesome variables. Nevertheless, always think carefully about the factors beyond what you’re focusing on – don’t make assumptions!
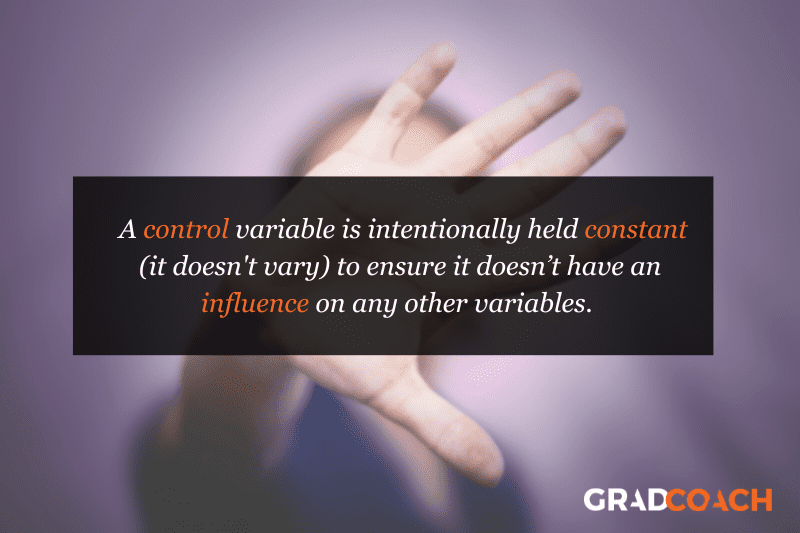
Other types of variables
As we mentioned, independent, dependent and control variables are the most common variables you’ll come across in your research, but they’re certainly not the only ones you need to be aware of. Next, we’ll look at a few “secondary” variables that you need to keep in mind as you design your research.
- Moderating variables
- Mediating variables
- Confounding variables
- Latent variables
Let’s jump into it…
What is a moderating variable?
A moderating variable is a variable that influences the strength or direction of the relationship between an independent variable and a dependent variable. In other words, moderating variables affect how much (or how little) the IV affects the DV, or whether the IV has a positive or negative relationship with the DV (i.e., moves in the same or opposite direction).
For example, in a study about the effects of sleep deprivation on academic performance, gender could be used as a moderating variable to see if there are any differences in how men and women respond to a lack of sleep. In such a case, one may find that gender has an influence on how much students’ scores suffer when they’re deprived of sleep.
It’s important to note that while moderators can have an influence on outcomes , they don’t necessarily cause them ; rather they modify or “moderate” existing relationships between other variables. This means that it’s possible for two different groups with similar characteristics, but different levels of moderation, to experience very different results from the same experiment or study design.
What is a mediating variable?
Mediating variables are often used to explain the relationship between the independent and dependent variable (s). For example, if you were researching the effects of age on job satisfaction, then education level could be considered a mediating variable, as it may explain why older people have higher job satisfaction than younger people – they may have more experience or better qualifications, which lead to greater job satisfaction.
Mediating variables also help researchers understand how different factors interact with each other to influence outcomes. For instance, if you wanted to study the effect of stress on academic performance, then coping strategies might act as a mediating factor by influencing both stress levels and academic performance simultaneously. For example, students who use effective coping strategies might be less stressed but also perform better academically due to their improved mental state.
In addition, mediating variables can provide insight into causal relationships between two variables by helping researchers determine whether changes in one factor directly cause changes in another – or whether there is an indirect relationship between them mediated by some third factor(s). For instance, if you wanted to investigate the impact of parental involvement on student achievement, you would need to consider family dynamics as a potential mediator, since it could influence both parental involvement and student achievement simultaneously.
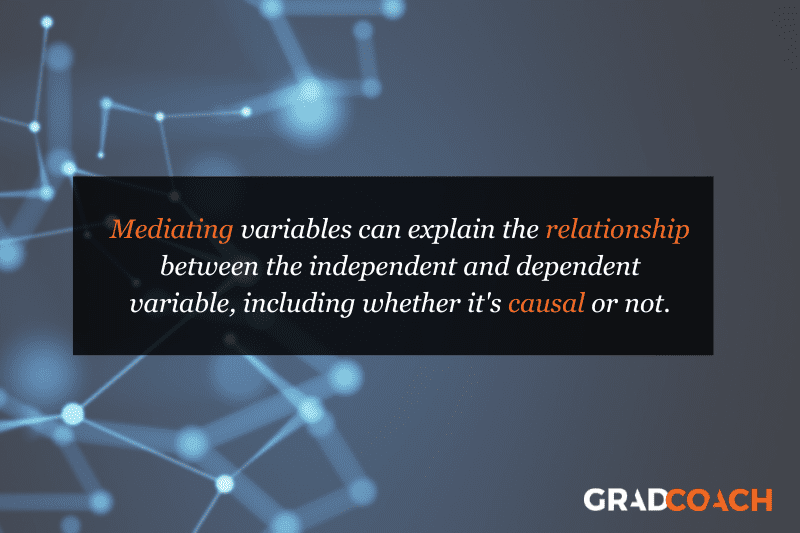
What is a confounding variable?
A confounding variable (also known as a third variable or lurking variable ) is an extraneous factor that can influence the relationship between two variables being studied. Specifically, for a variable to be considered a confounding variable, it needs to meet two criteria:
- It must be correlated with the independent variable (this can be causal or not)
- It must have a causal impact on the dependent variable (i.e., influence the DV)
Some common examples of confounding variables include demographic factors such as gender, ethnicity, socioeconomic status, age, education level, and health status. In addition to these, there are also environmental factors to consider. For example, air pollution could confound the impact of the variables of interest in a study investigating health outcomes.
Naturally, it’s important to identify as many confounding variables as possible when conducting your research, as they can heavily distort the results and lead you to draw incorrect conclusions . So, always think carefully about what factors may have a confounding effect on your variables of interest and try to manage these as best you can.
What is a latent variable?
Latent variables are unobservable factors that can influence the behaviour of individuals and explain certain outcomes within a study. They’re also known as hidden or underlying variables , and what makes them rather tricky is that they can’t be directly observed or measured . Instead, latent variables must be inferred from other observable data points such as responses to surveys or experiments.
For example, in a study of mental health, the variable “resilience” could be considered a latent variable. It can’t be directly measured , but it can be inferred from measures of mental health symptoms, stress, and coping mechanisms. The same applies to a lot of concepts we encounter every day – for example:
- Emotional intelligence
- Quality of life
- Business confidence
- Ease of use
One way in which we overcome the challenge of measuring the immeasurable is latent variable models (LVMs). An LVM is a type of statistical model that describes a relationship between observed variables and one or more unobserved (latent) variables. These models allow researchers to uncover patterns in their data which may not have been visible before, thanks to their complexity and interrelatedness with other variables. Those patterns can then inform hypotheses about cause-and-effect relationships among those same variables which were previously unknown prior to running the LVM. Powerful stuff, we say!
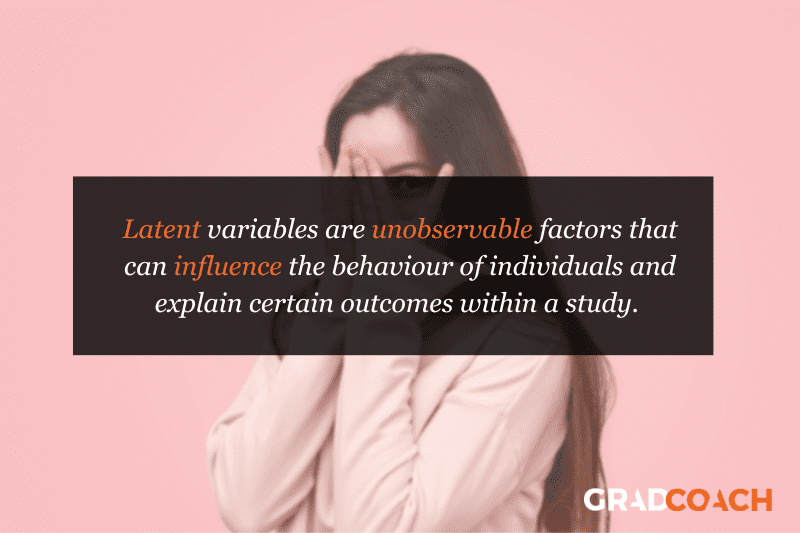
Let’s recap
In the world of scientific research, there’s no shortage of variable types, some of which have multiple names and some of which overlap with each other. In this post, we’ve covered some of the popular ones, but remember that this is not an exhaustive list .
To recap, we’ve explored:
- Independent variables (the “cause”)
- Dependent variables (the “effect”)
- Control variables (the variable that’s not allowed to vary)
If you’re still feeling a bit lost and need a helping hand with your research project, check out our 1-on-1 coaching service , where we guide you through each step of the research journey. Also, be sure to check out our free dissertation writing course and our collection of free, fully-editable chapter templates .
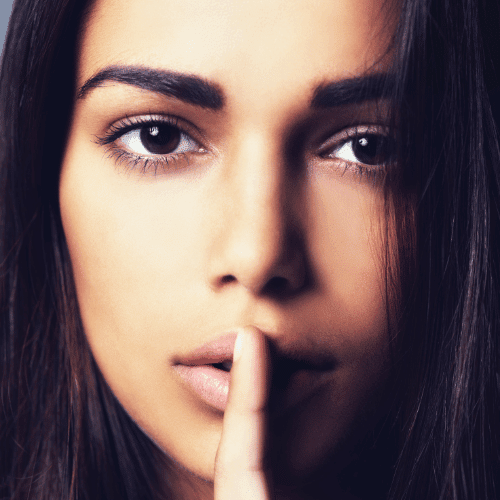
Psst... there’s more!
This post was based on one of our popular Research Bootcamps . If you're working on a research project, you'll definitely want to check this out ...
You Might Also Like:
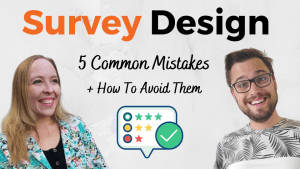
Very informative, concise and helpful. Thank you
Helping information.Thanks
practical and well-demonstrated
Very helpful and insightful
Submit a Comment Cancel reply
Your email address will not be published. Required fields are marked *
Save my name, email, and website in this browser for the next time I comment.
- Print Friendly
- Cookies & Privacy
- GETTING STARTED
- Introduction
- FUNDAMENTALS
- Acknowledgements
- Research questions & hypotheses
- Concepts, constructs & variables
- Research limitations
- Getting started
- Sampling Strategy
- Research Quality
- Research Ethics
- Data Analysis
Types of variables
Understanding the types of variables you are investigating in your dissertation is necessary for all types of quantitative research design , whether you using an experimental , quasi-experimental , relationship-based or descriptive research design. When you carry out your dissertation, you may need to measure , manipulate and/or control the variables you are investigating. In the section on Research Designs , you can learn more about the various types of quantitative research design. In this article, we present and illustrate the different types of variables you may come across in your dissertation. First, we discuss the main groups of variables: categorical variables and continuous variables . Second, we explain what dependent and independent variables are. This will provide you with one of the foundations required to tackle a dissertation based on a quantitative research design.
Categorical and continuous variables
Dependent and independent variables, ambiguities in classifying variables.
There are two groups of variables that you need to know about: categorical variables and continuous variables . We use the word groups of variables because both categorical and continuous variables include additional types of variable. However, there can also be some ambiguities when deciding whether a variable is categorical or continuous. We discuss the two groups of variable, as well as these potential ambiguities, in the sections that follow:
Categorical variables
Categorical variables are also known as qualitative (or discrete ) variables . These categorical variables can be further classified as being nominal , dichotomous or ordinal variables. Each of these types of categorical variable (i.e., nominal , dichotomous and ordinal ) has what are known as categories or levels . These categories or levels are the descriptions that you give a variable that help to explain how variables should be measured, manipulated and/or controlled. Take the following example:
Career choices of university students You are interested in the career choices of university students . You could ask university students a number of closed questions related to their career choices. For example: What is your planned occupation? What is the most important factor influencing your career choice?
The first question highlights the use of categories and the second question levels . For example:
Question 1 : What is your planned occupation? Variables with categories
Architect Attorney Biochemist Engineer Dentist Doctor Entrepreneur Social Worker Teacher ETC...
Career prospects Nature of the work Physical working conditions Salary and benefits ETC...
What is important to note about the categories in question 1 and the levels in question 2 is that these will be created by you. Ideally, you will have included these categories or levels based on some primary or secondary research. Ultimately, you choose which categories or levels to include and how many categories or levels there should be.
Each of these types of categorical variable (i.e., nominal , dichotomous and ordinal ) are described below with associated examples:
Nominal variables
The following are examples of nominal variables. These nominal variables could address questions like:
These examples highlight two core characteristics of nominal variables:
Nominal variables have two or more categories.
Nominal variables do not have an intrinsic order.
When we talk about nominal variables not having an intrinsic order , we mean that they can only have categories (e.g., black, blond, brown and red hair); not levels (e.g., a Likert scale from 1 to 5).
Dichotomous variables
The following are examples of dichotomous variables. These dichotomous variables could address questions like:
Dichotomous variables are nominal variables that have just two categories. They have a number of characteristics:
Dichotomous variables are designed to give you an either/or response
For example, you are either male or female. You either like watching television (i.e., you answer YES ) or you don't (i.e., you answer NO ).
Dichotomous variables can either be fixed or designed
For example, some variables (e.g., your sex ) can only be dichotomous (i.e., you can only be male or female ). They are therefore fixed . In other cases, dichotomous variables are designed by the researcher. For example, take the question: Do you like watching television? We have determined that the respondent can only select YES (i.e., I like watching television) or NO (i.e., I don't like watching television). However, another researcher could provide the respondent with more than two categories to this question (e.g., most of the time, sometimes , hardly ever ). Where more than two categories are used, these variables become known as nominal variables rather than dichotomous ones.
Ordinal variables
Just like nominal variables, ordinal variables have two or more categories. However, unlike nominal variables, ordinal variables can also be ordered or ranked (i.e., they have levels ). For example, take the following example of an ordinal variable:
So if you asked someone if they liked the policies of the Democratic Party and you presented them with the following three categories: Not very much , They are OK , or Yes, a lot ; you have an ordinal variable. Why? Because you have 3 categories ? namely Not very much , They are OK , and Yes, a lot ? and you can rank them from the most positive (Yes, a lot), to the middle response (They are OK), to the least positive (Not very much). However, whilst we can rank the three categories , we cannot place a value to them. For example, we cannot say that the response, They are OK , is twice as positive as the response, Not very much .
Other examples of ordinal variables are:
When it comes to Likert scales, as highlighted in the previous example, there can be some disagreement over whether these should be considered ordinal variables or continuous variables [see the section: Ambiguities in classifying variables ].
Continuous variables
Continuous variables, which are also known as quantitative variables, can be further classified a being either interval or ratio variables. Each of these types of continuous variable (i.e., interval and ratio ) has numerical properties. These numerical properties are the values by which continuous variables can be measured, manipulated and/or controlled. We illustrate the two types of continuous variable (i.e., interval and ratio ) and some associated values in the sections that follow:
Interval variables
Interval variables have a numerical value and can be measured along a continuum . Some examples of interval variables are:
However, temperature measured in degrees Celsius or Fahrenheit is NOT a ratio variable. This is because temperature measured in degrees Celsius or Fahrenheit is not a ratio variable because 0C does not mean there is no temperature.
Ratio variables
Ratio variables are interval variables that meet an additional condition: a measurement value of 0 (zero) must mean that there is none of that variable. Some examples of ratio variables are:
Sometimes, the measurement scale for data is ordinal , but the variable is treated as though it were continuous . This is more often the case when using Likert scales. When a Likert scale has five values (e.g., strongly agree, agree, neither agree nor disagree, disagree, and strongly disagree), it is treated as an ordinal variable. However, when a Likert scale has seven or more values (e.g., strongly agree, moderately agree, agree, neither agree nor disagree, disagree, moderately disagree, and strongly disagree), the variable is sometimes treated as a continuous variable. Nonetheless, this is a matter of dispute. Some researchers would argue that a Likert scale should never be treated as a continuous variable, even with seven levels/values.
Since you are responsible for setting the measurement scale for a variable, you will need to think carefully about how you characterise a variable. For example, social scientists may be more likely to consider the variable gender to be a nominal variable. This is because they view gender as having a number of categories, including male, female, bisexual and transsexual. By contrast, other researchers may simply view gender as a dichotomous variable, having just two categories: male and female. In such cases, it may be better to refer to the variable gender as sex .
A variable is not only something that you measure , but also something that you can manipulate and control for. An independent variable (sometimes called an experimental or predictor variable) is a variable that is being manipulated in an experiment in order to observe the effect this has on a dependent variable (sometimes called an outcome variable). The dependent variable is simply that; a variable that is dependent on an independent variable(s). We discuss these concepts in the example below:
For example: Imagine that a tutor asks 100 students to complete a maths test. The tutor wants to know why some students perform better than others. Whilst the tutor does not know the answer to this, she thinks that it might be because of two reasons:
Some students spend more time revising for their test; and
Some students are naturally more intelligent than others.
Therefore, the tutor decides to investigate the effect of revision time and intelligence on the test performance of the 100 students. As such, the dependent and independent variables for the study are:
The dependent variable is simply that; a variable that is dependent on an independent variable(s). In our case, the test mark (i.e. the dependent variable) that a student achieves is dependent on revision time and intelligence (i.e., the independent variables). Whilst revision time and intelligence (i.e., independent variables) may (or may not) cause a change in the test mark (i.e., the dependent variable), the reverse is implausible. In other words, whilst the number of hours a student spends revising and the higher a student's IQ score may (or may not) change the test mark that a student achieves, a change in a student's test mark has no bearing on whether a student revises more or is more intelligent. This would not make any sense.
Therefore, the aim of the tutor's investigation is to examine whether these independent variables (i.e., revision time and IQ) result in a change in the dependent variable (i.e., the students' test scores). However, it is also worth noting that whilst this is the main aim of the experiment, the tutor may also be interested to know if the independent variables (i.e., revision time and IQ) are also connected in some way.
You can find out more about the different uses of variables, especially in quantitative research designs (i.e., descriptive , experimental , quasi-experimental and relationship-based research designs), in the section on Research Designs .
Have a language expert improve your writing
Run a free plagiarism check in 10 minutes, automatically generate references for free.
- Knowledge Base
- Methodology
- Types of Variables in Research | Definitions & Examples
Types of Variables in Research | Definitions & Examples
Published on 19 September 2022 by Rebecca Bevans . Revised on 28 November 2022.
In statistical research, a variable is defined as an attribute of an object of study. Choosing which variables to measure is central to good experimental design .
You need to know which types of variables you are working with in order to choose appropriate statistical tests and interpret the results of your study.
You can usually identify the type of variable by asking two questions:
- What type of data does the variable contain?
- What part of the experiment does the variable represent?
Table of contents
Types of data: quantitative vs categorical variables, parts of the experiment: independent vs dependent variables, other common types of variables, frequently asked questions about variables.
Data is a specific measurement of a variable – it is the value you record in your data sheet. Data is generally divided into two categories:
- Quantitative data represents amounts.
- Categorical data represents groupings.
A variable that contains quantitative data is a quantitative variable ; a variable that contains categorical data is a categorical variable . Each of these types of variable can be broken down into further types.
Quantitative variables
When you collect quantitative data, the numbers you record represent real amounts that can be added, subtracted, divided, etc. There are two types of quantitative variables: discrete and continuous .
Categorical variables
Categorical variables represent groupings of some kind. They are sometimes recorded as numbers, but the numbers represent categories rather than actual amounts of things.
There are three types of categorical variables: binary , nominal , and ordinal variables.
*Note that sometimes a variable can work as more than one type! An ordinal variable can also be used as a quantitative variable if the scale is numeric and doesn’t need to be kept as discrete integers. For example, star ratings on product reviews are ordinal (1 to 5 stars), but the average star rating is quantitative.
Example data sheet
To keep track of your salt-tolerance experiment, you make a data sheet where you record information about the variables in the experiment, like salt addition and plant health.
To gather information about plant responses over time, you can fill out the same data sheet every few days until the end of the experiment. This example sheet is colour-coded according to the type of variable: nominal , continuous , ordinal , and binary .
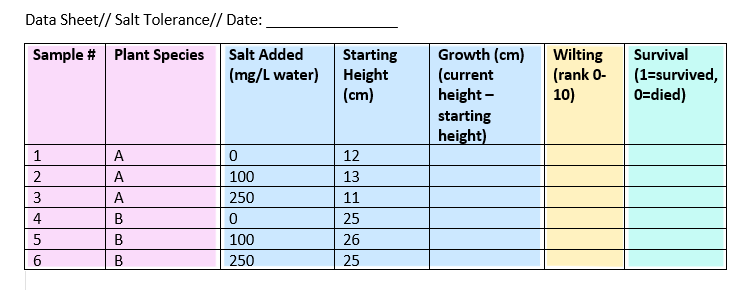
Prevent plagiarism, run a free check.
Experiments are usually designed to find out what effect one variable has on another – in our example, the effect of salt addition on plant growth.
You manipulate the independent variable (the one you think might be the cause ) and then measure the dependent variable (the one you think might be the effect ) to find out what this effect might be.
You will probably also have variables that you hold constant ( control variables ) in order to focus on your experimental treatment.
In this experiment, we have one independent and three dependent variables.
The other variables in the sheet can’t be classified as independent or dependent, but they do contain data that you will need in order to interpret your dependent and independent variables.
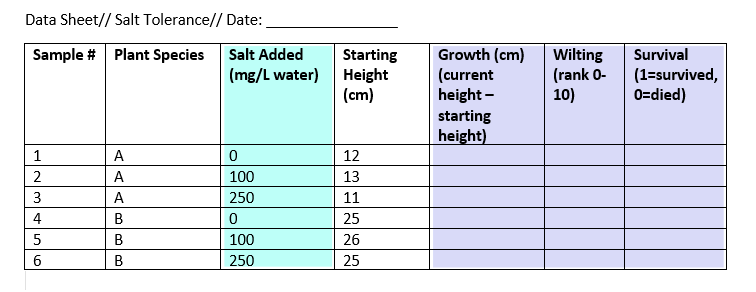
What about correlational research?
When you do correlational research , the terms ‘dependent’ and ‘independent’ don’t apply, because you are not trying to establish a cause-and-effect relationship.
However, there might be cases where one variable clearly precedes the other (for example, rainfall leads to mud, rather than the other way around). In these cases, you may call the preceding variable (i.e., the rainfall) the predictor variable and the following variable (i.e., the mud) the outcome variable .
Once you have defined your independent and dependent variables and determined whether they are categorical or quantitative, you will be able to choose the correct statistical test .
But there are many other ways of describing variables that help with interpreting your results. Some useful types of variable are listed below.
A confounding variable is closely related to both the independent and dependent variables in a study. An independent variable represents the supposed cause , while the dependent variable is the supposed effect . A confounding variable is a third variable that influences both the independent and dependent variables.
Failing to account for confounding variables can cause you to wrongly estimate the relationship between your independent and dependent variables.
Discrete and continuous variables are two types of quantitative variables :
- Discrete variables represent counts (e.g., the number of objects in a collection).
- Continuous variables represent measurable amounts (e.g., water volume or weight).
You can think of independent and dependent variables in terms of cause and effect: an independent variable is the variable you think is the cause , while a dependent variable is the effect .
In an experiment, you manipulate the independent variable and measure the outcome in the dependent variable. For example, in an experiment about the effect of nutrients on crop growth:
- The independent variable is the amount of nutrients added to the crop field.
- The dependent variable is the biomass of the crops at harvest time.
Defining your variables, and deciding how you will manipulate and measure them, is an important part of experimental design .
Cite this Scribbr article
If you want to cite this source, you can copy and paste the citation or click the ‘Cite this Scribbr article’ button to automatically add the citation to our free Reference Generator.
Bevans, R. (2022, November 28). Types of Variables in Research | Definitions & Examples. Scribbr. Retrieved 22 April 2024, from https://www.scribbr.co.uk/research-methods/variables-types/
Is this article helpful?
Rebecca Bevans
Other students also liked, a quick guide to experimental design | 5 steps & examples, quasi-experimental design | definition, types & examples, construct validity | definition, types, & examples.
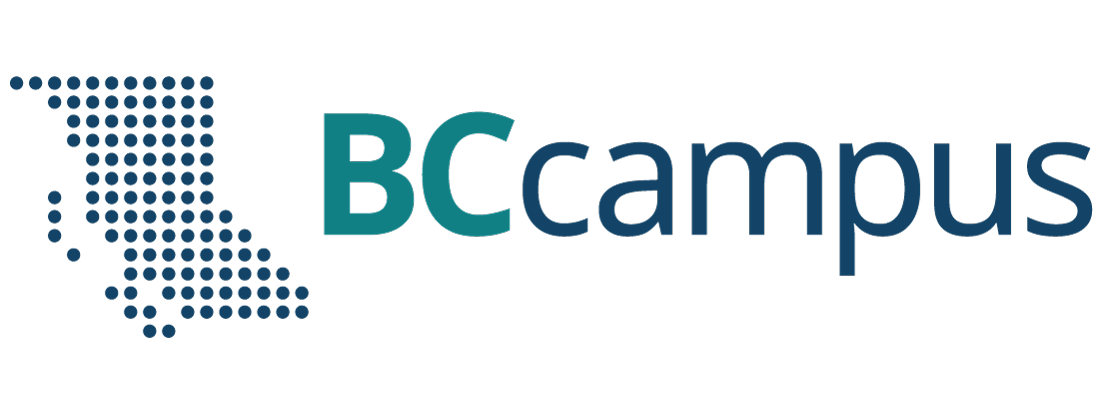
Want to create or adapt books like this? Learn more about how Pressbooks supports open publishing practices.
Chapter 8: Complex Research Designs
Multiple Dependent Variables
Learning Objectives
- Explain why researchers often include multiple dependent variables in their studies.
- Explain what a manipulation check is and when it would be included in an experiment.
Imagine that you have made the effort to find a research topic, review the research literature, formulate a question, design an experiment, obtain research ethics board (REB) approval, recruit research participants, and manipulate an independent variable. It would seem almost wasteful to measure a single dependent variable. Even if you are primarily interested in the relationship between an independent variable and one primary dependent variable, there are usually several more questions that you can answer easily by including multiple dependent variables .
Measures of Different Constructs
Often a researcher wants to know how an independent variable affects several distinct dependent variables. For example, Schnall and her colleagues were interested in how feeling disgusted affects the harshness of people’s moral judgments, but they were also curious about how disgust affects other variables, such as people’s willingness to eat in a restaurant. As another example, researcher Susan Knasko was interested in how different odours affect people’s behaviour (Knasko, 1992) [1] . She conducted an experiment in which the independent variable was whether participants were tested in a room with no odour or in one scented with lemon, lavender, or dimethyl sulfide (which has a cabbage-like smell). Although she was primarily interested in how the odours affected people’s creativity, she was also curious about how they affected people’s moods and perceived health—and it was a simple enough matter to measure these dependent variables too. Although she found that creativity was unaffected by the ambient odour, she found that people’s moods were lower in the dimethyl sulfide condition, and that their perceived health was greater in the lemon condition.
When an experiment includes multiple dependent variables, there is again a possibility of carryover effects. For example, it is possible that measuring participants’ moods before measuring their perceived health could affect their perceived health or that measuring their perceived health before their moods could affect their moods. So the order in which multiple dependent variables are measured becomes an issue. One approach is to measure them in the same order for all participants—usually with the most important one first so that it cannot be affected by measuring the others. Another approach is to counterbalance, or systematically vary, the order in which the dependent variables are measured.
Manipulation Checks
When the independent variable is a construct that can only be manipulated indirectly—such as emotions and other internal states—an additional measure of that independent variable is often included as a manipulation check . This is done to confirm that the independent variable was, in fact, successfully manipulated. For example, Schnall and her colleagues had their participants rate their level of disgust to be sure that those in the messy room actually felt more disgusted than those in the clean room. Manipulation checks are usually done at the end of the procedure to be sure that the effect of the manipulation lasted throughout the entire procedure and to avoid calling unnecessary attention to the manipulation.
Manipulation checks become especially important when the manipulation of the independent variable turns out to have no effect on the dependent variable. Imagine, for example, that you exposed participants to happy or sad movie music—intending to put them in happy or sad moods—but you found that this had no effect on the number of happy or sad childhood events they recalled. This could be because being in a happy or sad mood has no effect on memories for childhood events. But it could also be that the music was ineffective at putting participants in happy or sad moods. A manipulation check—in this case, a measure of participants’ moods—would help resolve this uncertainty. If it showed that you had successfully manipulated participants’ moods, then it would appear that there is indeed no effect of mood on memory for childhood events. But if it showed that you did not successfully manipulate participants’ moods, then it would appear that you need a more effective manipulation to answer your research question.
Measures of the Same Construct
Another common approach to including multiple dependent variables is to operationally define and measure the same construct, or closely related ones, in different ways. Imagine, for example, that a researcher conducts an experiment on the effect of daily exercise on stress. The dependent variable, stress, is a construct that can be operationally defined in different ways. For this reason, the researcher might have participants complete the paper-and-pencil Perceived Stress Scale and measure their levels of the stress hormone cortisol. This is an example of the use of converging operations. If the researcher finds that the different measures are affected by exercise in the same way, then he or she can be confident in the conclusion that exercise affects the more general construct of stress.
When multiple dependent variables are different measures of the same construct—especially if they are measured on the same scale—researchers have the option of combining them into a single measure of that construct. Recall that Schnall and her colleagues were interested in the harshness of people’s moral judgments. To measure this construct, they presented their participants with seven different scenarios describing morally questionable behaviours and asked them to rate the moral acceptability of each one. Although they could have treated each of the seven ratings as a separate dependent variable, these researchers combined them into a single dependent variable by computing their mean.
When researchers combine dependent variables in this way, they are treating them collectively as a multiple-response measure of a single construct. The advantage of this is that multiple-response measures are generally more reliable than single-response measures. However, it is important to make sure the individual dependent variables are correlated with each other by computing an internal consistency measure such as Cronbach’s α. If they are not correlated with each other, then it does not make sense to combine them into a measure of a single construct. If they have poor internal consistency, then they should be treated as separate dependent variables.
Key Takeaways
- Researchers in psychology often include multiple dependent variables in their studies. The primary reason is that this easily allows them to answer more research questions with minimal additional effort.
- When an independent variable is a construct that is manipulated indirectly, it is a good idea to include a manipulation check. This is a measure of the independent variable typically given at the end of the procedure to confirm that it was successfully manipulated.
- Multiple measures of the same construct can be analyzed separately or combined to produce a single multiple-item measure of that construct. The latter approach requires that the measures taken together have good internal consistency.
- Practice: List three independent variables for which it would be good to include a manipulation check. List three others for which a manipulation check would be unnecessary. Hint: Consider whether there is any ambiguity concerning whether the manipulation will have its intended effect.
- Practice: Imagine a study in which the independent variable is whether the room where participants are tested is warm (30°) or cool (12°). List three dependent variables that you might treat as measures of separate variables. List three more that you might combine and treat as measures of the same underlying construct.
- Knasko, S. C. (1992). Ambient odour’s effect on creativity, mood, and perceived health. Chemical Senses, 17 , 27–35. ↵
When researchers examine the relationship between a single independent variable and more than one dependent variable.
A separate measure of the construct the researcher is trying to manipulate.
Research Methods in Psychology - 2nd Canadian Edition Copyright © 2015 by Paul C. Price, Rajiv Jhangiani, & I-Chant A. Chiang is licensed under a Creative Commons Attribution-NonCommercial-ShareAlike 4.0 International License , except where otherwise noted.
Share This Book

Conceptual and Theoretical Frameworks for Thesis Studies: What you must know
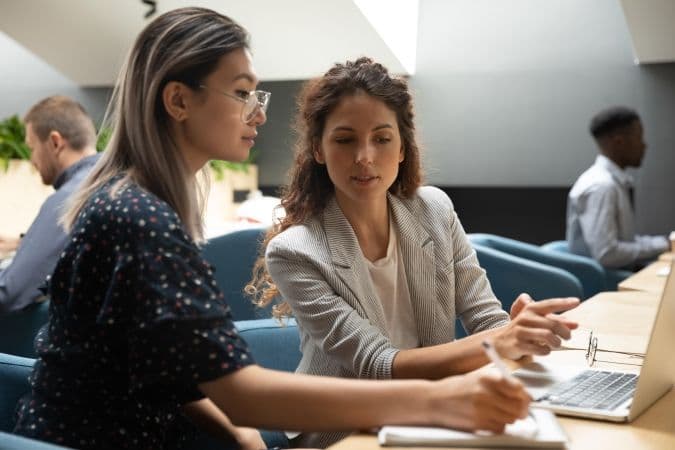
A theoretical framework is a conceptual model that provides a systematic and structured way of thinking about a research problem or question. It helps to identify key variables and the relationships between them and to guide the selection and interpretation of data. Theoretical frameworks draw on existing theories and research and can be used to develop new hypotheses or test existing ones. They provide a foundation for research design, data collection, and analysis and can help to ensure that research is relevant, rigorous, and coherent. Theoretical frameworks are common in many disciplines, including social sciences, natural sciences, and humanities, and are essential for building knowledge and advancing understanding in a field.
This article explains the importance of frameworks in a thesis study and the differences between conceptual frameworks and theoretical frameworks. It provides guidelines on how to write a thesis framework, definitions of variable types, and examples of framework types.
What is a research framework and why do I need one?
When planning your thesis study, you need to justify your research and explain its design to your readers. This is called the research framework.
When planning your thesis study, you need to justify your research and explain its design to your readers. This is called the research framework. Think of it as the foundation of a building. A good building needs a strong foundation. Similarly, your research needs to be supported by reviewing and explaining the existing knowledge in the field, describing how your research study will fit within or contribute to the existing literature (e.g., it could challenge or test an existing theory or address a knowledge gap), and informing the reader how your study design aligns with your thesis question or hypothesis.
Important components of the framework are a literature review of recent studies associated with your thesis topic as well as theories/models used in your field of research. The literature review acts as a filtering tool to select appropriate thesis questions and guide data collection, analysis, and interpretation of your findings. Think broadly! Apart from reviewing relevant published papers in your field of research, also explore theories that you have come across in your undergraduate courses, other published thesis studies, encyclopedias, and handbooks.
There are two types of research frameworks: theoretical and conceptual .
What is a conceptual framework?
A conceptual framework is a written or visual representation that explains the study variables and their relationships with each other. The starting point is a literature review of existing studies and theories about your topic.
Steps to develop a conceptual framework
- Clarify your study topic by identifying and defining key concepts in your thesis problem statement and thesis question. Essentially, your thesis should address a knowledge gap.
- Perform a literature review to provide a background to interpret and explain the study findings. Also, draw on empirical knowledge that you have gained from personal experience.
- Identify crucial variables from the literature review and your empirical knowledge, classify them as dependent or independent variables, and define them.
- Brainstorm all the possible factors that could affect each dependent variable.
- Propose relationships among the variables and determine any associations that exist between all variables.
- Use a flowchart or tree diagram to present your conceptual framework.
Types of variables
When developing a conceptual framework, you will need to identify the following:
- Independent variables
- Dependent variables
- Moderating variables
- Mediating variables
- Control variables
First, identify the independent (cause) and dependent (effect) variables in your study. Then, identify variables that influence this relationship, such as moderating variables, mediating variables, and control variables. A moderating variable changes the relationship between independent and dependent variables when its value increases or decreases. A mediating variable links independent and dependent variables to better explain the relationship between them. A control variable could potentially impact the cause-and-effect relationship but is kept constant throughout the study so that its effects on the findings/outcomes can be ruled out.
Example of a conceptual framework
You want to investigate the hours spent exercising (cause) on childhood obesity (effect).

Now, you need to consider moderating variables that affect the cause-and-effect relationship. In our example, the amount of junk food eaten would affect the level of obesity.
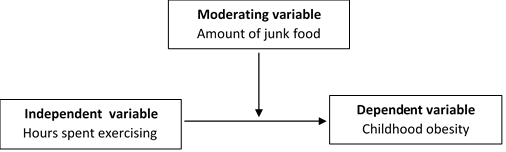
Next, you need to consider mediating variables. In our example, the maximum heart rate during exercise would affect the child’s weight.
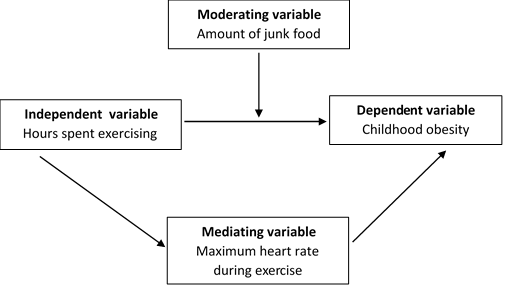
Finally, you need to consider control variables. In this example, because we do not want to investigate the role of age in obesity, we can use this as a control variable. Thus, the study subjects would be children of a specific age (e.g., aged 6–10 years).
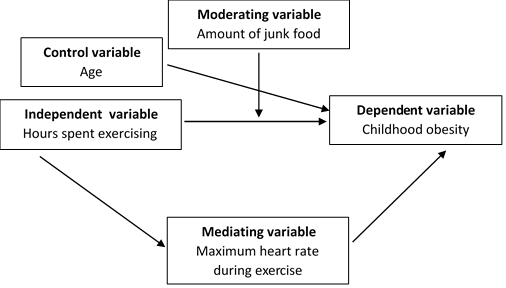
What is a theoretical framework?
A theoretical framework provides a general framework for data analysis. It defines the concepts used and explains existing theories and models in your field of research.
A theoretical framework provides a general framework for data analysis. It defines the concepts used and explains existing theories and models in your field of research. It also explains any assumptions that were used to inform your approach and your choice of specific rationales. Theoretical frameworks are often used in the fields of social sciences.
Purpose of a theoretical framework
- Test and challenge existing theories
- Establish orderly connections between observations and facts
- Predict and control situations
- Develop hypotheses
Steps to develop a theoretical framework
- Identify and define key concepts in your thesis problem statement and thesis question.
- Explain and evaluate existing theories by writing a literature review that describes the concepts, models, and theories that support your study.
- Choose the theory that best explains the relationships between the key variables in your study.
- Explain how your research study fills a knowledge gap or fits into existing studies (e.g., testing if an established theory applies to your thesis context).
- Discuss the relevance of any theoretical assumptions and limitations.
A thesis topic can be approached from a variety of angles, depending on the theories used.
- In psychology, a behavioral approach would use different methods and assumptions compared with a cognitive approach when treating anxiety.
- In literature, a book could be analyzed using different literary theories, such as Marxism or poststructuralism.
Structuring a theoretical framework
The structure of a theoretical framework is fluid, and there are no specific rules that need to be followed, as long as it is clearly and logically presented.
The theoretical framework is a natural extension of your literature review. The literature review should identify gaps in the field of your research, and reviewing existing theories will help to determine how these can be addressed. The structure of a theoretical framework is fluid, and there are no specific rules that need to be followed, as long as it is clearly and logically presented. The theoretical framework is sometimes integrated into the literature review chapter of a thesis, but it can also be included as a separate chapter, depending on the complexity of the theories.
Example of a theoretical framework
The sales staff at Company X are unmotivated and struggling to meet their monthly targets. Some members of the management team believe that this could be achieved by implementing a comprehensive product-training program, but others believe that introducing a sales commission structure will help.
Company X is not achieving their monthly sales targets
To increase monthly sales.
Research question:
How can Company X motivate their sales team to achieve its monthly sales targets?
Sub-questions:
- Why do the sales staff feel unmotivated?
- What is the relationship between motivation and monetary rewards?
- Do the sales staff feel that they have sufficient product knowledge?
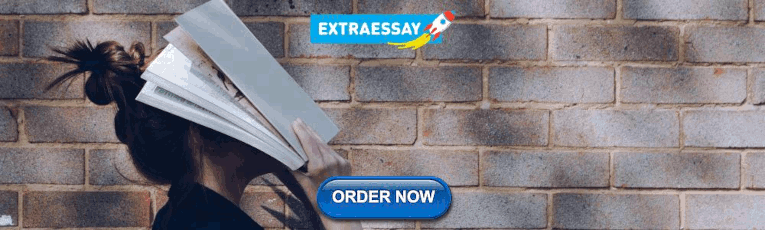
Theoretical framework:
A literature search will need to be performed to understand the background of the many different theories of motivation in psychology. For example, Maslow’s Hierarchy of Needs (basic human needs—physiological, safety, love/belonging, esteem, and self-actualization—have to be fulfilled before one can live up to their true potential), Vroom’s Theory of Expectancy (people decide upon their actions based on the outcomes they expect), and Locke’s Goal-Setting Theory (goals are a key driver of one’s behavior). These theories would need to be investigated to determine which would be the best approach to increase the motivation of the sales staff in Company X so that the monthly sales targets are met.
A robust conceptual or theoretical framework is crucial when writing a thesis/dissertation. It defines your research gap, identifies your approach, and guides the interpretation of your results.
A thesis is the most important document you will write during your academic studies. For professional thesis editing and thesis proofreading services, check out Enago's Thesis Editing service s for more information.
Editor’s pick
Get free updates.
Subscribe to our newsletter for regular insights from the research and publishing industry!
What type of framework is used in the Humanities and Social Sciences (HSS) domain? +
Theoretical frameworks are typically used in the HSS domain, while conceptual frameworks are used in the Sciences domain.
What is the difference between mediating versus moderating variables? +
The difference between mediators and moderators can be confusing. A moderating variable is unaffected by the independent variable and can increase or decrease the strength of the relationship between the independent and dependent variables. A mediating variable is affected by the independent variable and can explain the relationship between the independent and dependent variables. T he statistical correlation between the independent and dependent variables is higher when the mediating variable is excluded.
What software should I use to present my conceptual framework? +
The software program Creately provides some useful templates that can help you get started. Other recommended programs are SmartDraw , Inkscape , and diagrams.net .
- USC Libraries
- Research Guides
Organizing Your Social Sciences Research Paper
- Independent and Dependent Variables
- Purpose of Guide
- Design Flaws to Avoid
- Glossary of Research Terms
- Reading Research Effectively
- Narrowing a Topic Idea
- Broadening a Topic Idea
- Extending the Timeliness of a Topic Idea
- Academic Writing Style
- Applying Critical Thinking
- Choosing a Title
- Making an Outline
- Paragraph Development
- Research Process Video Series
- Executive Summary
- The C.A.R.S. Model
- Background Information
- The Research Problem/Question
- Theoretical Framework
- Citation Tracking
- Content Alert Services
- Evaluating Sources
- Primary Sources
- Secondary Sources
- Tiertiary Sources
- Scholarly vs. Popular Publications
- Qualitative Methods
- Quantitative Methods
- Insiderness
- Using Non-Textual Elements
- Limitations of the Study
- Common Grammar Mistakes
- Writing Concisely
- Avoiding Plagiarism
- Footnotes or Endnotes?
- Further Readings
- Generative AI and Writing
- USC Libraries Tutorials and Other Guides
- Bibliography
Definitions
Dependent Variable The variable that depends on other factors that are measured. These variables are expected to change as a result of an experimental manipulation of the independent variable or variables. It is the presumed effect.
Independent Variable The variable that is stable and unaffected by the other variables you are trying to measure. It refers to the condition of an experiment that is systematically manipulated by the investigator. It is the presumed cause.
Cramer, Duncan and Dennis Howitt. The SAGE Dictionary of Statistics . London: SAGE, 2004; Penslar, Robin Levin and Joan P. Porter. Institutional Review Board Guidebook: Introduction . Washington, DC: United States Department of Health and Human Services, 2010; "What are Dependent and Independent Variables?" Graphic Tutorial.
Identifying Dependent and Independent Variables
Don't feel bad if you are confused about what is the dependent variable and what is the independent variable in social and behavioral sciences research . However, it's important that you learn the difference because framing a study using these variables is a common approach to organizing the elements of a social sciences research study in order to discover relevant and meaningful results. Specifically, it is important for these two reasons:
- You need to understand and be able to evaluate their application in other people's research.
- You need to apply them correctly in your own research.
A variable in research simply refers to a person, place, thing, or phenomenon that you are trying to measure in some way. The best way to understand the difference between a dependent and independent variable is that the meaning of each is implied by what the words tell us about the variable you are using. You can do this with a simple exercise from the website, Graphic Tutorial. Take the sentence, "The [independent variable] causes a change in [dependent variable] and it is not possible that [dependent variable] could cause a change in [independent variable]." Insert the names of variables you are using in the sentence in the way that makes the most sense. This will help you identify each type of variable. If you're still not sure, consult with your professor before you begin to write.
Fan, Shihe. "Independent Variable." In Encyclopedia of Research Design. Neil J. Salkind, editor. (Thousand Oaks, CA: SAGE, 2010), pp. 592-594; "What are Dependent and Independent Variables?" Graphic Tutorial; Salkind, Neil J. "Dependent Variable." In Encyclopedia of Research Design , Neil J. Salkind, editor. (Thousand Oaks, CA: SAGE, 2010), pp. 348-349;
Structure and Writing Style
The process of examining a research problem in the social and behavioral sciences is often framed around methods of analysis that compare, contrast, correlate, average, or integrate relationships between or among variables . Techniques include associations, sampling, random selection, and blind selection. Designation of the dependent and independent variable involves unpacking the research problem in a way that identifies a general cause and effect and classifying these variables as either independent or dependent.
The variables should be outlined in the introduction of your paper and explained in more detail in the methods section . There are no rules about the structure and style for writing about independent or dependent variables but, as with any academic writing, clarity and being succinct is most important.
After you have described the research problem and its significance in relation to prior research, explain why you have chosen to examine the problem using a method of analysis that investigates the relationships between or among independent and dependent variables . State what it is about the research problem that lends itself to this type of analysis. For example, if you are investigating the relationship between corporate environmental sustainability efforts [the independent variable] and dependent variables associated with measuring employee satisfaction at work using a survey instrument, you would first identify each variable and then provide background information about the variables. What is meant by "environmental sustainability"? Are you looking at a particular company [e.g., General Motors] or are you investigating an industry [e.g., the meat packing industry]? Why is employee satisfaction in the workplace important? How does a company make their employees aware of sustainability efforts and why would a company even care that its employees know about these efforts?
Identify each variable for the reader and define each . In the introduction, this information can be presented in a paragraph or two when you describe how you are going to study the research problem. In the methods section, you build on the literature review of prior studies about the research problem to describe in detail background about each variable, breaking each down for measurement and analysis. For example, what activities do you examine that reflect a company's commitment to environmental sustainability? Levels of employee satisfaction can be measured by a survey that asks about things like volunteerism or a desire to stay at the company for a long time.
The structure and writing style of describing the variables and their application to analyzing the research problem should be stated and unpacked in such a way that the reader obtains a clear understanding of the relationships between the variables and why they are important. This is also important so that the study can be replicated in the future using the same variables but applied in a different way.
Fan, Shihe. "Independent Variable." In Encyclopedia of Research Design. Neil J. Salkind, editor. (Thousand Oaks, CA: SAGE, 2010), pp. 592-594; "What are Dependent and Independent Variables?" Graphic Tutorial; “Case Example for Independent and Dependent Variables.” ORI Curriculum Examples. U.S. Department of Health and Human Services, Office of Research Integrity; Salkind, Neil J. "Dependent Variable." In Encyclopedia of Research Design , Neil J. Salkind, editor. (Thousand Oaks, CA: SAGE, 2010), pp. 348-349; “Independent Variables and Dependent Variables.” Karl L. Wuensch, Department of Psychology, East Carolina University [posted email exchange]; “Variables.” Elements of Research. Dr. Camille Nebeker, San Diego State University.
- << Previous: Design Flaws to Avoid
- Next: Glossary of Research Terms >>
- Last Updated: Apr 24, 2024 10:51 AM
- URL: https://libguides.usc.edu/writingguide
- cognitive sophistication
- tolerance of diversity
- exposure to higher levels of math or science
- age (which is currently related to educational level in many countries)
- social class and other variables.
- For example, suppose you designed a treatment to help people stop smoking. Because you are really dedicated, you assigned the same individuals simultaneously to (1) a "stop smoking" nicotine patch; (2) a "quit buddy"; and (3) a discussion support group. Compared with a group in which no intervention at all occurred, your experimental group now smokes 10 fewer cigarettes per day.
- There is no relationship among two or more variables (EXAMPLE: the correlation between educational level and income is zero)
- Or that two or more populations or subpopulations are essentially the same (EXAMPLE: women and men have the same average science knowledge scores.)
- the difference between two and three children = one child.
- the difference between eight and nine children also = one child.
- the difference between completing ninth grade and tenth grade is one year of school
- the difference between completing junior and senior year of college is one year of school
- In addition to all the properties of nominal, ordinal, and interval variables, ratio variables also have a fixed/non-arbitrary zero point. Non arbitrary means that it is impossible to go below a score of zero for that variable. For example, any bottom score on IQ or aptitude tests is created by human beings and not nature. On the other hand, scientists believe they have isolated an "absolute zero." You can't get colder than that.
Research Hypothesis In Psychology: Types, & Examples
Saul Mcleod, PhD
Editor-in-Chief for Simply Psychology
BSc (Hons) Psychology, MRes, PhD, University of Manchester
Saul Mcleod, PhD., is a qualified psychology teacher with over 18 years of experience in further and higher education. He has been published in peer-reviewed journals, including the Journal of Clinical Psychology.
Learn about our Editorial Process
Olivia Guy-Evans, MSc
Associate Editor for Simply Psychology
BSc (Hons) Psychology, MSc Psychology of Education
Olivia Guy-Evans is a writer and associate editor for Simply Psychology. She has previously worked in healthcare and educational sectors.
On This Page:
A research hypothesis, in its plural form “hypotheses,” is a specific, testable prediction about the anticipated results of a study, established at its outset. It is a key component of the scientific method .
Hypotheses connect theory to data and guide the research process towards expanding scientific understanding
Some key points about hypotheses:
- A hypothesis expresses an expected pattern or relationship. It connects the variables under investigation.
- It is stated in clear, precise terms before any data collection or analysis occurs. This makes the hypothesis testable.
- A hypothesis must be falsifiable. It should be possible, even if unlikely in practice, to collect data that disconfirms rather than supports the hypothesis.
- Hypotheses guide research. Scientists design studies to explicitly evaluate hypotheses about how nature works.
- For a hypothesis to be valid, it must be testable against empirical evidence. The evidence can then confirm or disprove the testable predictions.
- Hypotheses are informed by background knowledge and observation, but go beyond what is already known to propose an explanation of how or why something occurs.
Predictions typically arise from a thorough knowledge of the research literature, curiosity about real-world problems or implications, and integrating this to advance theory. They build on existing literature while providing new insight.
Types of Research Hypotheses
Alternative hypothesis.
The research hypothesis is often called the alternative or experimental hypothesis in experimental research.
It typically suggests a potential relationship between two key variables: the independent variable, which the researcher manipulates, and the dependent variable, which is measured based on those changes.
The alternative hypothesis states a relationship exists between the two variables being studied (one variable affects the other).
A hypothesis is a testable statement or prediction about the relationship between two or more variables. It is a key component of the scientific method. Some key points about hypotheses:
- Important hypotheses lead to predictions that can be tested empirically. The evidence can then confirm or disprove the testable predictions.
In summary, a hypothesis is a precise, testable statement of what researchers expect to happen in a study and why. Hypotheses connect theory to data and guide the research process towards expanding scientific understanding.
An experimental hypothesis predicts what change(s) will occur in the dependent variable when the independent variable is manipulated.
It states that the results are not due to chance and are significant in supporting the theory being investigated.
The alternative hypothesis can be directional, indicating a specific direction of the effect, or non-directional, suggesting a difference without specifying its nature. It’s what researchers aim to support or demonstrate through their study.
Null Hypothesis
The null hypothesis states no relationship exists between the two variables being studied (one variable does not affect the other). There will be no changes in the dependent variable due to manipulating the independent variable.
It states results are due to chance and are not significant in supporting the idea being investigated.
The null hypothesis, positing no effect or relationship, is a foundational contrast to the research hypothesis in scientific inquiry. It establishes a baseline for statistical testing, promoting objectivity by initiating research from a neutral stance.
Many statistical methods are tailored to test the null hypothesis, determining the likelihood of observed results if no true effect exists.
This dual-hypothesis approach provides clarity, ensuring that research intentions are explicit, and fosters consistency across scientific studies, enhancing the standardization and interpretability of research outcomes.
Nondirectional Hypothesis
A non-directional hypothesis, also known as a two-tailed hypothesis, predicts that there is a difference or relationship between two variables but does not specify the direction of this relationship.
It merely indicates that a change or effect will occur without predicting which group will have higher or lower values.
For example, “There is a difference in performance between Group A and Group B” is a non-directional hypothesis.
Directional Hypothesis
A directional (one-tailed) hypothesis predicts the nature of the effect of the independent variable on the dependent variable. It predicts in which direction the change will take place. (i.e., greater, smaller, less, more)
It specifies whether one variable is greater, lesser, or different from another, rather than just indicating that there’s a difference without specifying its nature.
For example, “Exercise increases weight loss” is a directional hypothesis.
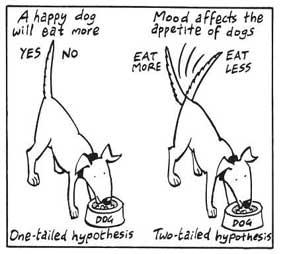
Falsifiability
The Falsification Principle, proposed by Karl Popper , is a way of demarcating science from non-science. It suggests that for a theory or hypothesis to be considered scientific, it must be testable and irrefutable.
Falsifiability emphasizes that scientific claims shouldn’t just be confirmable but should also have the potential to be proven wrong.
It means that there should exist some potential evidence or experiment that could prove the proposition false.
However many confirming instances exist for a theory, it only takes one counter observation to falsify it. For example, the hypothesis that “all swans are white,” can be falsified by observing a black swan.
For Popper, science should attempt to disprove a theory rather than attempt to continually provide evidence to support a research hypothesis.
Can a Hypothesis be Proven?
Hypotheses make probabilistic predictions. They state the expected outcome if a particular relationship exists. However, a study result supporting a hypothesis does not definitively prove it is true.
All studies have limitations. There may be unknown confounding factors or issues that limit the certainty of conclusions. Additional studies may yield different results.
In science, hypotheses can realistically only be supported with some degree of confidence, not proven. The process of science is to incrementally accumulate evidence for and against hypothesized relationships in an ongoing pursuit of better models and explanations that best fit the empirical data. But hypotheses remain open to revision and rejection if that is where the evidence leads.
- Disproving a hypothesis is definitive. Solid disconfirmatory evidence will falsify a hypothesis and require altering or discarding it based on the evidence.
- However, confirming evidence is always open to revision. Other explanations may account for the same results, and additional or contradictory evidence may emerge over time.
We can never 100% prove the alternative hypothesis. Instead, we see if we can disprove, or reject the null hypothesis.
If we reject the null hypothesis, this doesn’t mean that our alternative hypothesis is correct but does support the alternative/experimental hypothesis.
Upon analysis of the results, an alternative hypothesis can be rejected or supported, but it can never be proven to be correct. We must avoid any reference to results proving a theory as this implies 100% certainty, and there is always a chance that evidence may exist which could refute a theory.
How to Write a Hypothesis
- Identify variables . The researcher manipulates the independent variable and the dependent variable is the measured outcome.
- Operationalized the variables being investigated . Operationalization of a hypothesis refers to the process of making the variables physically measurable or testable, e.g. if you are about to study aggression, you might count the number of punches given by participants.
- Decide on a direction for your prediction . If there is evidence in the literature to support a specific effect of the independent variable on the dependent variable, write a directional (one-tailed) hypothesis. If there are limited or ambiguous findings in the literature regarding the effect of the independent variable on the dependent variable, write a non-directional (two-tailed) hypothesis.
- Make it Testable : Ensure your hypothesis can be tested through experimentation or observation. It should be possible to prove it false (principle of falsifiability).
- Clear & concise language . A strong hypothesis is concise (typically one to two sentences long), and formulated using clear and straightforward language, ensuring it’s easily understood and testable.
Consider a hypothesis many teachers might subscribe to: students work better on Monday morning than on Friday afternoon (IV=Day, DV= Standard of work).
Now, if we decide to study this by giving the same group of students a lesson on a Monday morning and a Friday afternoon and then measuring their immediate recall of the material covered in each session, we would end up with the following:
- The alternative hypothesis states that students will recall significantly more information on a Monday morning than on a Friday afternoon.
- The null hypothesis states that there will be no significant difference in the amount recalled on a Monday morning compared to a Friday afternoon. Any difference will be due to chance or confounding factors.
More Examples
- Memory : Participants exposed to classical music during study sessions will recall more items from a list than those who studied in silence.
- Social Psychology : Individuals who frequently engage in social media use will report higher levels of perceived social isolation compared to those who use it infrequently.
- Developmental Psychology : Children who engage in regular imaginative play have better problem-solving skills than those who don’t.
- Clinical Psychology : Cognitive-behavioral therapy will be more effective in reducing symptoms of anxiety over a 6-month period compared to traditional talk therapy.
- Cognitive Psychology : Individuals who multitask between various electronic devices will have shorter attention spans on focused tasks than those who single-task.
- Health Psychology : Patients who practice mindfulness meditation will experience lower levels of chronic pain compared to those who don’t meditate.
- Organizational Psychology : Employees in open-plan offices will report higher levels of stress than those in private offices.
- Behavioral Psychology : Rats rewarded with food after pressing a lever will press it more frequently than rats who receive no reward.

User Preferences
Content preview.
Arcu felis bibendum ut tristique et egestas quis:
- Ut enim ad minim veniam, quis nostrud exercitation ullamco laboris
- Duis aute irure dolor in reprehenderit in voluptate
- Excepteur sint occaecat cupidatat non proident
Keyboard Shortcuts
Relationships between two variables, introduction.
Let's get started! Here is what you will learn in this lesson.
Learning objectives for this lesson
Upon completion of this lesson, you should be able to do the following:
- Understand the relationship between the slope of the regression line and correlation,
- Comprehend the meaning of the Coefficient of Determination, R 2,
- Know how to determine which variable is a response and which is an explanatory in a regression equation,
- Understand that correlation measures the strength of a linear relationship between two variables,
- Realize how outliers can influence a regression equation, and
- Determine if variables are categorical or quantitative.
Examining Relationships Between Two Variables
Previously we considered the distribution of a single quantitative variable. Now we will study the relationship between two variables where both variables are qualitative, i.e. categorical, or quantitative. When we consider the relationship between two variables, there are three possibilities:
- Both variables are categorical. We analyze an association through a comparison of conditional probabilities and graphically represent the data using contingency tables. Examples of categorical variables are gender and class standing.
- Both variables are quantitative. To analyze this situation we consider how one variable, called a response variable, changes in relation to changes in the other variable called an explanatory variable. Graphically we use scatterplots to display two quantitative variables. Examples are age, height, weight (i.e. things that are measured).
- One variable is categorical and the other is quantitative, for instance height and gender. These are best compared by using side-by-side boxplots to display any differences or similarities in the center and variability of the quantitative variable (e.g. height) across the categories (e.g. Male and Female).
What is a Statistically Significant Relationship Between Two Variables?
How do you decide if, indeed, there is a statistically significant relationship between two variables in your study? What does the p-value output in statistical software analysis mean? This article explains the concept and provides examples with computations and video tutorial.
What does a researcher mean if he says there is a statistically significant relationship between two variables in his study? What makes the relationship statistically significant?
These questions imply that a test for correlation between two variables was made in that particular study. The specific statistical test could either be the parametric Pearson Product-Moment Correlation or the non-parametric Spearman’s Rho test.
Table of Contents
Statistical software applications to test a statistically significant relationship.
It is now easy to do computations using popular statistical software applications like the popular Statistical Package for the Social Sciences (SPSS) or Statistica and even using the data analysis function of spreadsheets like the proprietary Microsoft Excel and the open-source but less popular Gnumeric.
Once the statistical software has finished processing the data, You will get a range of correlation coefficient values along with their corresponding p-values denoted by the letter p and a decimal number for a one-tailed and two-tailed test . The p-value is the one that matters when trying to judge whether there is a statistically significant relationship between two variables.
Confusing Definition of p-value
Many of my students in the statistics course I teach are confused about the meaning of p-value. I understand this dilemma because the references I see online do not explain in easily understandable language the meaning of the p-value.
For example, Investopedia, the top resource that returns out of 83 million-plus searches on the meaning of p-value, is challenging to understand for a beginning researcher, an undergraduate, or even a graduate student for that matter. If you click the link I provided on the meaning of p-value, you will understand what I mean.
If you understand the definition provided there, then there is no need for you to read the rest of the sections. Even with my advanced graduate school education, I find the explanation vague if I would not exert an effort to try to understand it.
Hence, I explain the meaning of p-value in the next section in the best way I can in the simplest manner possible. I provide a detailed explanation of what it means in the next section.
The Meaning of p-value
What does the p-value mean? This value never exceeds 1. Why?
The computer-generated p-value represents the estimated probability of rejecting the null hypothesis (Ho) that the researcher formulated at the beginning of the study. The null hypothesis is stated in such a way that there is “ no ” difference between the two variables being tested.
Therefore, this means that as a researcher, you should be clear about what you want to test in the first place.
For example, your null hypothesis that will lend itself to statistical analysis should be written like this:
H 0 : There is no relationship between the long quiz score and the number of hours devoted by students in studying their lessons.
If the computed value is exactly 1 (p = 1.0), this means that the relationship is absolutely correlated. There is no doubt that the long quiz score and the number of hours spent by students in studying their lessons are correlated.
That p-value means a 100% probability (read simply as 100 percent sure) that the long quiz score and the number of hours devoted by the students in studying their lessons are correlated. The greater the number of hours devoted by students in learning their courses, the higher their long quiz scores. As simple as that.
Conversely, if the p-value is 0 , this means there is no correlation at all. It means that whether the students study or not, their long quiz scores are not affected at all.
Why in Reality the p-value of 1 is Not Possible
In reality, unless you are specializing in Physics, a p-value of 1 is difficult to obtain when studying human behavior. In our example, many factors or variables influence the long quiz score. Variables like the student’s intelligence quotient, the teacher’s teaching skill, the difficulty of the quiz, among others, affect the score.
Now, this means that the p-value should not be 1 or numbers greater than that. If you get a p-value of more than 1 in your computation, that’s nonsense. Your p-value, I repeat once again, should range between 1 and 0.
To illustrate, if the p-value you obtained during the computation is equal to 0.5, this means that there is a 50% chance that one variable is correlated to the other variable. In our example, we can say that there is a 50% probability that the long quiz score is correlated to the number of hours spent by students in studying their lessons.
Or to put it simply, we can say that there is a statistically significant relationship between the long quiz scores of students and the number of hours that they spend studying their lessons.
Deciding Whether the Relationship is Significant
Suppose the probability in the example given above is p = 0.05. Is it good enough to say that there is a statistically significant relationship between long quiz scores and the number of hours spent by students studying their lessons?
The answer is NO. Why?
In today’s standard rule or convention in the world of statistics, statisticians adopt a significance level denoted by alpha (α) as a pre-chosen probability for significance. The value for significance is usually set at either 0.05 (statistically significant) or 0.01 (statistically highly significant). These numbers represent 5% and 1% probability, respectively.

Comparing the computed p-value with the pre-chosen probabilities of 5% and 1% will help you decide whether the relationship between the two variables is significant or not. If, say, the p-values you obtained in your computation are 0.5, 0.4, or 0.06, you should accept the null hypothesis. That is if you set alpha at 0.05 (α = 0.05). If the value you got is below 0.05 or p < 0.05, then you should accept your alternative hypothesis.
In the above example, the alternative hypothesis that should be accepted when the p-value is less than 0.05 will be:
H 1 : There is a relationship between the long quiz score and the number of hours devoted by students in studying their lessons.
The strength of the relationship is indicated by the correlation coefficient or r values. Guilford (1956) suggested the following categories as guide:
Computation of Correlation in SPSS
If you want to learn about how to use SPSS in computing correlations, the eight-minute tutorial by Dr. Bogdan Kostic below on the correlation between Intelligence Quotient (IQ) and Grade Point Average (GPA) will guide you. He demonstrates in detail how the data are encoded in SPSS, how the labels are written, and the process of statistical test selection. In this instance, the correlation between IQ and GPA using Pearson’s product-moment correlation with the accompanying computer output. This video will further strengthen your knowledge on how to determine if there is a significant relationship between two groups of variables.
More examples and demonstrations on how to find out if there is a statistically significant relationship between variables are given in the two articles below. These articles provide example computer outputs and how these are interpreted.
How to Use Gnumeric in Comparing Two Groups of Data Heart Rate Analysis: Example of t-test using MS Excel Analysis ToolPak
More Easy-to-Follow Tips
For very easy-to-follow tips on how to select the appropriate statistical tests for your study, see my eBook on statistics at the middle of the page in Simplyeducate.me ‘s eBook store.
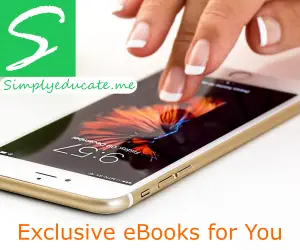
Guilford, J. P., 1956. Fundamental statistics in psychology and education. New York: McGraw-Hill. p. 145.
© 2014 May 29 P. A. Regoniel Updated 13 November 2020
Related Posts
The role of statistics in decision making, big data analytics and executive decision making.
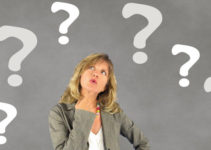
Statistical Research Questions: Five Examples for Quantitative Analysis
About the author, patrick regoniel.
Dr. Regoniel, a faculty member of the graduate school, served as consultant to various environmental research and development projects covering issues and concerns on climate change, coral reef resources and management, economic valuation of environmental and natural resources, mining, and waste management and pollution. He has extensive experience on applied statistics, systems modelling and analysis, an avid practitioner of LaTeX, and a multidisciplinary web developer. He leverages pioneering AI-powered content creation tools to produce unique and comprehensive articles in this website.
Dear Mr. Regoniel Thanks so much for this wonderful article . Pls. can U explain why the significance (alpha) level of 0.01 is higher than that of 0.05, Also in reference to the illustration which U gave in your article, as regards the alpha level of 0.05 does it mean that if I get a computed value of 0.04, then I should accept the alternative hypothesis and reject the null hypothesis
Dear Femi, I just got to read this comment. Yes, if you get a computed p value of 0.04, there is reason to believe that the relationship between two variables is significant.
Thanks for this article,It’s really helpfull.I have a question regarding my project.It is “Relationship between ocupational stress and Marital Adjustment among working women in defence”. Here,Is it necessary to do t-test as I have already calculated correlation and the sample size is 30? Please help me out from this.
Dear Namita, if you want to test for correlation, then you use either Pearson’s correlation coefficient of Spearman rho, depending on the spread of your data, meaning it depends on how the values differ from the mean in the two groups. You can read more on this concern in https://simplyeducate.me/wordpress_Y//2020/10/11/nonparametric-tests/
Thank you for the feedback Dr. Dizon. Your comment signals me to further simplify this explanation for better understanding.
The article is really very useful to me as it helps me analyze the meaning of significant relationship between two variables. However, I have yet to go over the article, read it several times to finally come with a better grasp of the idea behind relationship. Thanks anyway.
- Privacy Policy

Home » Qualitative Variable – Types and Examples
Qualitative Variable – Types and Examples
Table of Contents

Qualitative Variable
Definition:
Qualitative variable, also known as a categorical variable, is a type of variable in statistics that describes an attribute or characteristic of a data point, rather than a numerical value.
Qualitative variables are typically represented by labels or categories, such as “male” or “female,” and are often used in surveys and polls to gather information about a population’s characteristics.
Types Qualitative Variable
There are two main types of qualitative variables:
Nominal Variables
A nominal variable is a Qualitative Variable where the categories are not ordered in any particular way. For example, gender (male or female), race (Asian, Black, Hispanic, etc.), or religion (Christian, Muslim, Hindu, etc.). Nominal variables can be represented using numbers, but the numbers do not have any quantitative meaning. For example, a researcher might assign the number “1” to male and “2” to female, but these numbers do not represent a quantitative difference between the categories.
Ordinal Variables
An ordinal variable is a Qualitative Variable where the categories are ordered in some way. For example, educational level (high school, college, graduate school), income level (low, medium, high), or level of agreement (strongly agree, somewhat agree, neutral, somewhat disagree, strongly disagree). Ordinal variables can be represented using numbers, and the numbers have a quantitative meaning, but the distance between the categories is not necessarily equal. For example, the difference between “high school” and “college” may not be the same as the difference between “college” and “graduate school.”
Examples of Qualitative Variables
Here are some examples of qualitative variables:
- Gender : Male or female
- Marital status: Married, single, divorced, widowed
- Race : Asian, Black, Hispanic, White, etc.
- Religious affiliation: Christian, Muslim, Hindu, Buddhist, etc.
- Political affiliation : Democrat, Republican, Independent, etc.
- Educational level : High school, college, graduate school
- Type of employment : Full-time, part-time, self-employed, unemployed
- Type of housing: Apartment, house, condo, etc.
- Method of transportation : Car, bus, train, bike, etc.
- Language spoken: English, Spanish, French, etc.
Applications of Qualitative Variable
Qualitative variables are used in many applications in different fields, including:
- Market research : Qualitative variables are often used in market research to understand consumer behavior and preferences. For example, a company might use qualitative variables such as age, gender, and income to segment their target market and create customized marketing campaigns.
- Public opinion polling : Qualitative variables are used in public opinion polling to gather information about people’s attitudes, beliefs, and opinions. Pollsters may ask questions about political affiliation, religious affiliation, or social issues to understand public opinion on a particular topic.
- Social sciences research: Qualitative variables are commonly used in social sciences research to study human behavior, culture, and society. Researchers may use qualitative variables to categorize people based on their demographic information or cultural background, and to analyze patterns and trends in behavior or attitudes.
- Healthcare research: Qualitative variables are used in healthcare research to identify risk factors and to understand the impact of treatments on patients. Researchers may use qualitative variables such as age, gender, or medical history to identify populations at risk for certain diseases, and to evaluate the effectiveness of different treatment options.
- Education research: Qualitative variables are used in education research to study the effectiveness of different teaching methods and to identify factors that influence student learning. Researchers may use qualitative variables such as socio-economic status, educational level, or learning style to analyze patterns and trends in student performance.
When to use Qualitative Variable
Qualitative variables should be used in research when the variable being studied is categorical and does not involve numerical values. Here are some situations where qualitative variables are appropriate:
- When studying demographic characteristics: Qualitative variables are useful for studying demographic characteristics such as age, gender, ethnicity, and religion. These variables can be used to segment a population into groups and to compare differences between groups.
- When studying attitudes and beliefs : Qualitative variables can be used to study people’s attitudes and beliefs about various topics, such as politics, social issues, or religion. Researchers can use surveys or interviews to gather data on these variables.
- When studying cultural differences: Qualitative variables are often used in cross-cultural research to study differences between cultures. Researchers may use qualitative variables such as language spoken, nationality, or cultural background to identify groups for comparison.
- When studying consumer behavior : Qualitative variables can be used in market research to study consumer behavior and preferences. Researchers can use qualitative variables such as brand loyalty, product preference, or buying habits to understand consumer behavior.
- When studying patient outcomes: Qualitative variables can be used in healthcare research to study patient outcomes, such as quality of life, satisfaction with treatment, or adherence to medication. Researchers can use qualitative variables to identify factors that influence patient outcomes and to develop interventions to improve patient care.
Purpose of Qualitative Variable
The purpose of a qualitative variable is to categorize data into distinct groups based on non-numerical characteristics or attributes. The use of qualitative variables allows researchers to describe and analyze non-quantifiable phenomena, such as attitudes, beliefs, behaviors, and demographic characteristics, and to identify patterns and trends in the data. The main purposes of qualitative variables are:
- To describe and categorize : Qualitative variables are used to describe and categorize data into meaningful groups based on characteristics or attributes that are not numerical.
- To compare and contrast: Qualitative variables allow researchers to compare and contrast different groups or categories of data, such as different demographic groups or cultural backgrounds.
- To identify patterns and trends: Qualitative variables allow researchers to identify patterns and trends in data that may not be apparent with numerical data. For example, a researcher may use qualitative variables to identify cultural differences in attitudes toward healthcare.
- To develop hypotheses: Qualitative variables can be used to develop hypotheses or research questions for further study. For example, a researcher may use qualitative variables to identify risk factors for a particular disease, which can then be further studied using quantitative methods.
- To inform decision-making: Qualitative variables can provide important information to inform decision-making in fields such as healthcare, education, and business. For example, healthcare providers may use qualitative variables to identify patient preferences and needs, which can inform treatment decisions.
Characteristics of Qualitative Variable
Here are some of the characteristics of qualitative variables:
- Categorical : Qualitative variables are categorical in nature, meaning that they describe characteristics or attributes that are not numerical. They can be nominal, ordinal or binary.
- Non-numeric : Qualitative variables do not involve numerical values, but rather descriptive or categorical data such as colors, shapes, types, or names.
- Limited number of categories: Qualitative variables are often limited to a small number of categories, such as male/female, married/single/divorced, or white/black/Asian.
- Mutually exclusive categories : Categories in a qualitative variable must be mutually exclusive, meaning that each observation can only belong to one category.
- No numerical order : Unlike quantitative variables, qualitative variables do not have a numerical order or ranking. Categories are assigned based on non-numerical criteria.
- Can be used for comparison : Qualitative variables are often used for comparison purposes, such as comparing the frequency of certain behaviors or attitudes across different demographic groups.
- Can be used for classification: Qualitative variables can be used to classify data into distinct groups based on common characteristics or attributes. For example, people can be classified into different racial or ethnic groups based on their ancestry.
- Can be used for hypothesis testing : Qualitative variables can be used to test hypotheses about differences between groups or categories of data. For example, a researcher may hypothesize that men and women have different attitudes toward a particular social issue, and use a qualitative variable to test this hypothesis.
Advantages of Qualitative Variable
There are several advantages of using qualitative variables.
- Rich data: Qualitative variables can provide rich data about complex phenomena such as attitudes, behaviors, and cultural differences. This data can be useful for gaining a deep understanding of a particular issue or topic.
- Flexibility : Qualitative variables are flexible and can be used in a variety of research methods, such as interviews, focus groups, and observations. This allows researchers to choose the method that best suits their research question and participants.
- Participant perspective : Qualitative variables allow researchers to capture the participant’s perspective and experience. By using open-ended questions or prompts, researchers can gain insight into how participants perceive and interpret a particular issue.
- Depth of understanding: Qualitative variables allow for a depth of understanding that may not be possible with quantitative variables alone. Qualitative data can provide details and context that quantitative data may miss.
- Contextualization : Qualitative variables can provide contextualization, allowing researchers to understand the cultural, social, and historical factors that shape attitudes and behaviors.
- Theory development: Qualitative variables can be useful for developing new theories or refining existing ones. By gathering rich data and analyzing it using qualitative methods, researchers can identify patterns and relationships that can inform the development of new theories.
- Researcher reflexivity : Qualitative variables require the researcher to be reflexive and acknowledge their own biases and assumptions. This can help to ensure that the research is ethical and inclusive, and that the data collected is valid and reliable.
Limitations of Qualitative Variable
Some Limitations of Qualitative Variable are as follows:
- Subjectivity : Qualitative data is often collected through open-ended questions or prompts, which can lead to subjective responses that are difficult to quantify or compare. This can make it challenging to establish inter-rater reliability and can limit the generalizability of the findings.
- Limited sample size : Qualitative research often involves small sample sizes, which can limit the generalizability of the findings. While qualitative research is typically focused on gaining a deep understanding of a particular issue, the findings may not be representative of the broader population.
- Time-consuming: Qualitative research can be time-consuming, particularly when collecting and analyzing data. Researchers must spend significant amounts of time in the field, conducting interviews or focus groups, and then transcribing and analyzing the data.
- Limited control: Qualitative research often involves limited control over the research environment and the participants. This can make it challenging to ensure that the data collected is valid and reliable.
- Limited generalizability: Qualitative research is typically focused on gaining a deep understanding of a particular issue, rather than testing hypotheses or making generalizations about the broader population. As a result, the findings may be less generalizable than those obtained through quantitative research methods.
- Ethical concerns: Qualitative research often involves collecting sensitive or personal information from participants. Researchers must take care to ensure that participants are fully informed about the research, that their privacy is protected, and that they are not harmed in any way by their participation.
- Bias : Qualitative research can be subject to bias, particularly if the researcher has a vested interest in the outcome of the research. Researchers must take care to acknowledge their own biases and assumptions, and to use multiple sources of data to ensure the validity and reliability of the findings.
About the author
Muhammad Hassan
Researcher, Academic Writer, Web developer
You may also like

Control Variable – Definition, Types and Examples

Moderating Variable – Definition, Analysis...

Categorical Variable – Definition, Types and...

Independent Variable – Definition, Types and...

Ratio Variable – Definition, Purpose and Examples

Ordinal Variable – Definition, Purpose and...

An official website of the United States government
Here's how you know
The .gov means it's official. Federal government websites often end in .gov or .mil. Before sharing sensitive information, make sure you’re on a federal government site.
The site is secure. The https:// ensures that you are connecting to the official website and that any information you provide is encrypted and transmitted securely.
What the New Overtime Rule Means for Workers
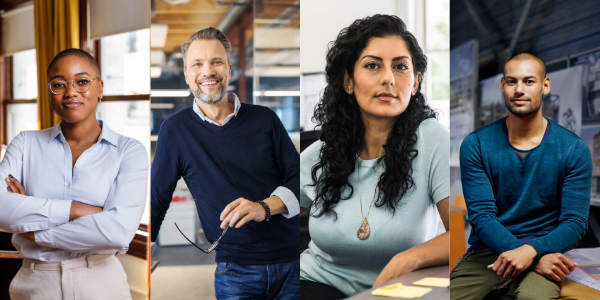
One of the basic principles of the American workplace is that a hard day’s work deserves a fair day’s pay. Simply put, every worker’s time has value. A cornerstone of that promise is the Fair Labor Standards Act ’s (FLSA) requirement that when most workers work more than 40 hours in a week, they get paid more. The Department of Labor ’s new overtime regulation is restoring and extending this promise for millions more lower-paid salaried workers in the U.S.
Overtime protections have been a critical part of the FLSA since 1938 and were established to protect workers from exploitation and to benefit workers, their families and our communities. Strong overtime protections help build America’s middle class and ensure that workers are not overworked and underpaid.
Some workers are specifically exempt from the FLSA’s minimum wage and overtime protections, including bona fide executive, administrative or professional employees. This exemption, typically referred to as the “EAP” exemption, applies when:
1. An employee is paid a salary,
2. The salary is not less than a minimum salary threshold amount, and
3. The employee primarily performs executive, administrative or professional duties.
While the department increased the minimum salary required for the EAP exemption from overtime pay every 5 to 9 years between 1938 and 1975, long periods between increases to the salary requirement after 1975 have caused an erosion of the real value of the salary threshold, lessening its effectiveness in helping to identify exempt EAP employees.
The department’s new overtime rule was developed based on almost 30 listening sessions across the country and the final rule was issued after reviewing over 33,000 written comments. We heard from a wide variety of members of the public who shared valuable insights to help us develop this Administration’s overtime rule, including from workers who told us: “I would love the opportunity to...be compensated for time worked beyond 40 hours, or alternately be given a raise,” and “I make around $40,000 a year and most week[s] work well over 40 hours (likely in the 45-50 range). This rule change would benefit me greatly and ensure that my time is paid for!” and “Please, I would love to be paid for the extra hours I work!”
The department’s final rule, which will go into effect on July 1, 2024, will increase the standard salary level that helps define and delimit which salaried workers are entitled to overtime pay protections under the FLSA.
Starting July 1, most salaried workers who earn less than $844 per week will become eligible for overtime pay under the final rule. And on Jan. 1, 2025, most salaried workers who make less than $1,128 per week will become eligible for overtime pay. As these changes occur, job duties will continue to determine overtime exemption status for most salaried employees.
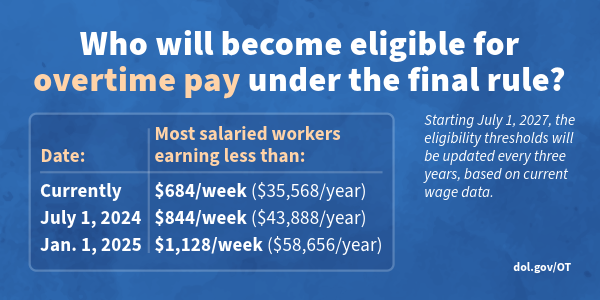
The rule will also increase the total annual compensation requirement for highly compensated employees (who are not entitled to overtime pay under the FLSA if certain requirements are met) from $107,432 per year to $132,964 per year on July 1, 2024, and then set it equal to $151,164 per year on Jan. 1, 2025.
Starting July 1, 2027, these earnings thresholds will be updated every three years so they keep pace with changes in worker salaries, ensuring that employers can adapt more easily because they’ll know when salary updates will happen and how they’ll be calculated.
The final rule will restore and extend the right to overtime pay to many salaried workers, including workers who historically were entitled to overtime pay under the FLSA because of their lower pay or the type of work they performed.
We urge workers and employers to visit our website to learn more about the final rule.
Jessica Looman is the administrator for the U.S. Department of Labor’s Wage and Hour Division. Follow the Wage and Hour Division on Twitter at @WHD_DOL and LinkedIn . Editor's note: This blog was edited to correct a typo (changing "administrator" to "administrative.")
- Wage and Hour Division (WHD)
- Fair Labor Standards Act
- overtime rule
SHARE THIS:
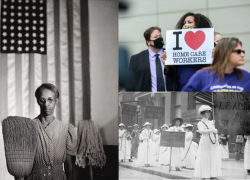
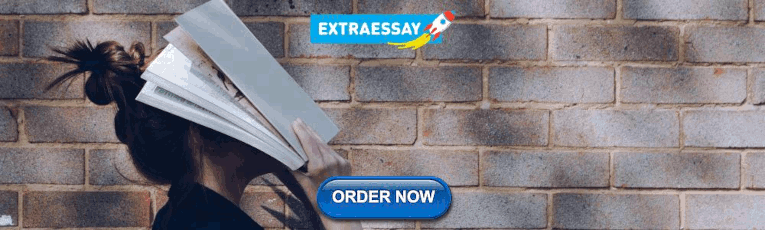
IMAGES
VIDEO
COMMENTS
Examples. Discrete variables (aka integer variables) Counts of individual items or values. Number of students in a class. Number of different tree species in a forest. Continuous variables (aka ratio variables) Measurements of continuous or non-finite values. Distance.
A confounding variable (also known as a third variable or lurking variable) is an extraneous factor that can influence the relationship between two variables being studied. Specifically, for a variable to be considered a confounding variable, it needs to meet two criteria: It must be correlated with the independent variable (this can be causal ...
Introduction. Definition of Variable. Examples of Variables in Research: 6 Phenomena. Phenomenon 1: Climate change. Phenomenon 2: Crime and violence in the streets. Phenomenon 3: Poor performance of students in college entrance exams. Phenomenon 4: Fish kill. Phenomenon 5: Poor crop growth. Phenomenon 6: How Content Goes Viral.
Developing a conceptual framework in research. Step 1: Choose your research question. Step 2: Select your independent and dependent variables. Step 3: Visualize your cause-and-effect relationship. Step 4: Identify other influencing variables. Frequently asked questions about conceptual models.
Categorical and continuous variables. There are two groups of variables that you need to know about: categorical variables and continuous variables.We use the word groups of variables because both categorical and continuous variables include additional types of variable. However, there can also be some ambiguities when deciding whether a variable is categorical or continuous.
The independent variable is the cause. Its value is independent of other variables in your study. The dependent variable is the effect. Its value depends on changes in the independent variable. Example: Independent and dependent variables. You design a study to test whether changes in room temperature have an effect on math test scores.
Categorical Variable. This is a variable that can take on a limited number of values or categories. Categorical variables can be nominal or ordinal. Nominal variables have no inherent order, while ordinal variables have a natural order. Examples of categorical variables include gender, race, and educational level.
Examples. Discrete variables (aka integer variables) Counts of individual items or values. Number of students in a class. Number of different tree species in a forest. Continuous variables (aka ratio variables) Measurements of continuous or non-finite values. Distance.
Key Takeaways. Researchers in psychology often include multiple dependent variables in their studies. The primary reason is that this easily allows them to answer more research questions with minimal additional effort. When an independent variable is a construct that is manipulated indirectly, it is a good idea to include a manipulation check.
A theoretical framework is a conceptual model that provides a systematic and structured way of thinking about a research problem or question. It helps to identify key variables and the relationships between them and to guide the selection and interpretation of data. Theoretical frameworks draw on existing theories and research and can be used ...
Based on our hypothesis there are two main variables concerning this research the first is about the independent variable the value which affects another variable (Satish, 2018), here in our ...
Dependent Variable The variable that depends on other factors that are measured. These variables are expected to change as a result of an experimental manipulation of the independent variable or variables. It is the presumed effect. Independent Variable The variable that is stable and unaffected by the other variables you are trying to measure.
Two variables may be associated but we cannot designate cause and effect. These are symmetric relationships. In asymmetric relationships ... It used to be that students had to assert null hypotheses in a thesis, dissertation, conference presentation or article. Now, we are more comfortable with students creating directional hypotheses. Many ...
The alternative hypothesis states a relationship exists between the two variables being studied (one variable affects the other). A hypothesis is a testable statement or prediction about the relationship between two or more variables. It is a key component of the scientific method. Some key points about hypotheses:
Examples of categorical variables are gender and class standing. Both variables are quantitative. To analyze this situation we consider how one variable, called a response variable, changes in relation to changes in the other variable called an explanatory variable. Graphically we use scatterplots to display two quantitative variables.
Provide limitations to identify potential weaknesses of the study. In your thesis, this will be a section in Chapter 1. Example of a limitation: The purposive sampling procedure decreases the generalizability of findings. This study will not be generalizable to all areas of nursing.
It is a number between -1 and 1 that measures the strength and direction of the relationship between two variables. Pearson correlation coefficient ( r) Correlation type. Interpretation. Example. Between 0 and 1. Positive correlation. When one variable changes, the other variable changes in the same direction. Baby length & weight:
Comparing the computed p-value with the pre-chosen probabilities of 5% and 1% will help you decide whether the relationship between the two variables is significant or not. If, say, the p-values you obtained in your computation are 0.5, 0.4, or 0.06, you should accept the null hypothesis. That is if you set alpha at 0.05 (α = 0.05).
(In this example, there are 4 independent variables (age, gender, status, and L1) and two dependent variables (proficiency and motivation). ... Please fill in this survey to help me with my thesis
Chapter 2 of this thesis has been co-written with Raj Chetty, John Friedman, Edward Glaeser, and Guido Imbens; I thank them for permission to include ... two-step instrumental variables (tsiv) estimators that include the two-stage least squares (tsls) estimator; and minimum distance estimators that include the limited information ...
Placement of the thesis statement. Step 1: Start with a question. Step 2: Write your initial answer. Step 3: Develop your answer. Step 4: Refine your thesis statement. Types of thesis statements. Other interesting articles. Frequently asked questions about thesis statements.
In this thesis, we address the variable selection problem in multivariate multiple regression models. 1.1 Modelling Multiple Outcomes Multivariate multiple regression analysis is a common statistical tool for assessing covariate effects when only one response or multiple response variables are collected in observational or experimental studies.
Also, the number of IV's is irrelevant here as both ANOVA and MANOVA can accommodate 1 IV or 2 IV's or 3 IV's, etc. (called one way, two way, three-way etc. ANOVA or MANOVA) Also, pay attention that MANOVA is a parametric test, meaning that your measurement must be continuous. it is hard to assume that measurement by any Likert-type scale with ...
Types Qualitative Variable. There are two main types of qualitative variables: Nominal Variables. A nominal variable is a Qualitative Variable where the categories are not ordered in any particular way. For example, gender (male or female), race (Asian, Black, Hispanic, etc.), or religion (Christian, Muslim, Hindu, etc.).
The Department of Labor's new overtime regulation is restoring and extending this promise for millions more lower-paid salaried workers in the U.S.
Where 0 ≤ y ≤ 1 denotes the dependent variable, Xi is the vector of explanatory variables of observation i, and G(0) is any cumulative distribution function that maps z to the (0,1) interval and G follows cumulative functional forms either logit, probit, and log log. Let γ be the HCI, (4) E y z = Z (4) Where…Z is a vector of covariates ….
Revised on June 22, 2023. A correlational research design investigates relationships between variables without the researcher controlling or manipulating any of them. A correlation reflects the strength and/or direction of the relationship between two (or more) variables. The direction of a correlation can be either positive or negative.