
An official website of the United States government
The .gov means it’s official. Federal government websites often end in .gov or .mil. Before sharing sensitive information, make sure you’re on a federal government site.
The site is secure. The https:// ensures that you are connecting to the official website and that any information you provide is encrypted and transmitted securely.
- Publications
- Account settings
Preview improvements coming to the PMC website in October 2024. Learn More or Try it out now .
- Advanced Search
- Journal List
- J Korean Med Sci
- v.37(16); 2022 Apr 25

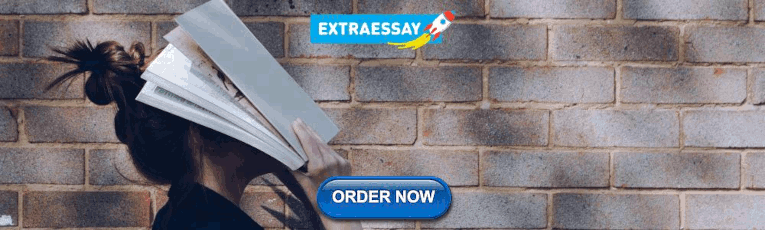
A Practical Guide to Writing Quantitative and Qualitative Research Questions and Hypotheses in Scholarly Articles
Edward barroga.
1 Department of General Education, Graduate School of Nursing Science, St. Luke’s International University, Tokyo, Japan.
Glafera Janet Matanguihan
2 Department of Biological Sciences, Messiah University, Mechanicsburg, PA, USA.
The development of research questions and the subsequent hypotheses are prerequisites to defining the main research purpose and specific objectives of a study. Consequently, these objectives determine the study design and research outcome. The development of research questions is a process based on knowledge of current trends, cutting-edge studies, and technological advances in the research field. Excellent research questions are focused and require a comprehensive literature search and in-depth understanding of the problem being investigated. Initially, research questions may be written as descriptive questions which could be developed into inferential questions. These questions must be specific and concise to provide a clear foundation for developing hypotheses. Hypotheses are more formal predictions about the research outcomes. These specify the possible results that may or may not be expected regarding the relationship between groups. Thus, research questions and hypotheses clarify the main purpose and specific objectives of the study, which in turn dictate the design of the study, its direction, and outcome. Studies developed from good research questions and hypotheses will have trustworthy outcomes with wide-ranging social and health implications.
INTRODUCTION
Scientific research is usually initiated by posing evidenced-based research questions which are then explicitly restated as hypotheses. 1 , 2 The hypotheses provide directions to guide the study, solutions, explanations, and expected results. 3 , 4 Both research questions and hypotheses are essentially formulated based on conventional theories and real-world processes, which allow the inception of novel studies and the ethical testing of ideas. 5 , 6
It is crucial to have knowledge of both quantitative and qualitative research 2 as both types of research involve writing research questions and hypotheses. 7 However, these crucial elements of research are sometimes overlooked; if not overlooked, then framed without the forethought and meticulous attention it needs. Planning and careful consideration are needed when developing quantitative or qualitative research, particularly when conceptualizing research questions and hypotheses. 4
There is a continuing need to support researchers in the creation of innovative research questions and hypotheses, as well as for journal articles that carefully review these elements. 1 When research questions and hypotheses are not carefully thought of, unethical studies and poor outcomes usually ensue. Carefully formulated research questions and hypotheses define well-founded objectives, which in turn determine the appropriate design, course, and outcome of the study. This article then aims to discuss in detail the various aspects of crafting research questions and hypotheses, with the goal of guiding researchers as they develop their own. Examples from the authors and peer-reviewed scientific articles in the healthcare field are provided to illustrate key points.
DEFINITIONS AND RELATIONSHIP OF RESEARCH QUESTIONS AND HYPOTHESES
A research question is what a study aims to answer after data analysis and interpretation. The answer is written in length in the discussion section of the paper. Thus, the research question gives a preview of the different parts and variables of the study meant to address the problem posed in the research question. 1 An excellent research question clarifies the research writing while facilitating understanding of the research topic, objective, scope, and limitations of the study. 5
On the other hand, a research hypothesis is an educated statement of an expected outcome. This statement is based on background research and current knowledge. 8 , 9 The research hypothesis makes a specific prediction about a new phenomenon 10 or a formal statement on the expected relationship between an independent variable and a dependent variable. 3 , 11 It provides a tentative answer to the research question to be tested or explored. 4
Hypotheses employ reasoning to predict a theory-based outcome. 10 These can also be developed from theories by focusing on components of theories that have not yet been observed. 10 The validity of hypotheses is often based on the testability of the prediction made in a reproducible experiment. 8
Conversely, hypotheses can also be rephrased as research questions. Several hypotheses based on existing theories and knowledge may be needed to answer a research question. Developing ethical research questions and hypotheses creates a research design that has logical relationships among variables. These relationships serve as a solid foundation for the conduct of the study. 4 , 11 Haphazardly constructed research questions can result in poorly formulated hypotheses and improper study designs, leading to unreliable results. Thus, the formulations of relevant research questions and verifiable hypotheses are crucial when beginning research. 12
CHARACTERISTICS OF GOOD RESEARCH QUESTIONS AND HYPOTHESES
Excellent research questions are specific and focused. These integrate collective data and observations to confirm or refute the subsequent hypotheses. Well-constructed hypotheses are based on previous reports and verify the research context. These are realistic, in-depth, sufficiently complex, and reproducible. More importantly, these hypotheses can be addressed and tested. 13
There are several characteristics of well-developed hypotheses. Good hypotheses are 1) empirically testable 7 , 10 , 11 , 13 ; 2) backed by preliminary evidence 9 ; 3) testable by ethical research 7 , 9 ; 4) based on original ideas 9 ; 5) have evidenced-based logical reasoning 10 ; and 6) can be predicted. 11 Good hypotheses can infer ethical and positive implications, indicating the presence of a relationship or effect relevant to the research theme. 7 , 11 These are initially developed from a general theory and branch into specific hypotheses by deductive reasoning. In the absence of a theory to base the hypotheses, inductive reasoning based on specific observations or findings form more general hypotheses. 10
TYPES OF RESEARCH QUESTIONS AND HYPOTHESES
Research questions and hypotheses are developed according to the type of research, which can be broadly classified into quantitative and qualitative research. We provide a summary of the types of research questions and hypotheses under quantitative and qualitative research categories in Table 1 .
Research questions in quantitative research
In quantitative research, research questions inquire about the relationships among variables being investigated and are usually framed at the start of the study. These are precise and typically linked to the subject population, dependent and independent variables, and research design. 1 Research questions may also attempt to describe the behavior of a population in relation to one or more variables, or describe the characteristics of variables to be measured ( descriptive research questions ). 1 , 5 , 14 These questions may also aim to discover differences between groups within the context of an outcome variable ( comparative research questions ), 1 , 5 , 14 or elucidate trends and interactions among variables ( relationship research questions ). 1 , 5 We provide examples of descriptive, comparative, and relationship research questions in quantitative research in Table 2 .
Hypotheses in quantitative research
In quantitative research, hypotheses predict the expected relationships among variables. 15 Relationships among variables that can be predicted include 1) between a single dependent variable and a single independent variable ( simple hypothesis ) or 2) between two or more independent and dependent variables ( complex hypothesis ). 4 , 11 Hypotheses may also specify the expected direction to be followed and imply an intellectual commitment to a particular outcome ( directional hypothesis ) 4 . On the other hand, hypotheses may not predict the exact direction and are used in the absence of a theory, or when findings contradict previous studies ( non-directional hypothesis ). 4 In addition, hypotheses can 1) define interdependency between variables ( associative hypothesis ), 4 2) propose an effect on the dependent variable from manipulation of the independent variable ( causal hypothesis ), 4 3) state a negative relationship between two variables ( null hypothesis ), 4 , 11 , 15 4) replace the working hypothesis if rejected ( alternative hypothesis ), 15 explain the relationship of phenomena to possibly generate a theory ( working hypothesis ), 11 5) involve quantifiable variables that can be tested statistically ( statistical hypothesis ), 11 6) or express a relationship whose interlinks can be verified logically ( logical hypothesis ). 11 We provide examples of simple, complex, directional, non-directional, associative, causal, null, alternative, working, statistical, and logical hypotheses in quantitative research, as well as the definition of quantitative hypothesis-testing research in Table 3 .
Research questions in qualitative research
Unlike research questions in quantitative research, research questions in qualitative research are usually continuously reviewed and reformulated. The central question and associated subquestions are stated more than the hypotheses. 15 The central question broadly explores a complex set of factors surrounding the central phenomenon, aiming to present the varied perspectives of participants. 15
There are varied goals for which qualitative research questions are developed. These questions can function in several ways, such as to 1) identify and describe existing conditions ( contextual research question s); 2) describe a phenomenon ( descriptive research questions ); 3) assess the effectiveness of existing methods, protocols, theories, or procedures ( evaluation research questions ); 4) examine a phenomenon or analyze the reasons or relationships between subjects or phenomena ( explanatory research questions ); or 5) focus on unknown aspects of a particular topic ( exploratory research questions ). 5 In addition, some qualitative research questions provide new ideas for the development of theories and actions ( generative research questions ) or advance specific ideologies of a position ( ideological research questions ). 1 Other qualitative research questions may build on a body of existing literature and become working guidelines ( ethnographic research questions ). Research questions may also be broadly stated without specific reference to the existing literature or a typology of questions ( phenomenological research questions ), may be directed towards generating a theory of some process ( grounded theory questions ), or may address a description of the case and the emerging themes ( qualitative case study questions ). 15 We provide examples of contextual, descriptive, evaluation, explanatory, exploratory, generative, ideological, ethnographic, phenomenological, grounded theory, and qualitative case study research questions in qualitative research in Table 4 , and the definition of qualitative hypothesis-generating research in Table 5 .
Qualitative studies usually pose at least one central research question and several subquestions starting with How or What . These research questions use exploratory verbs such as explore or describe . These also focus on one central phenomenon of interest, and may mention the participants and research site. 15
Hypotheses in qualitative research
Hypotheses in qualitative research are stated in the form of a clear statement concerning the problem to be investigated. Unlike in quantitative research where hypotheses are usually developed to be tested, qualitative research can lead to both hypothesis-testing and hypothesis-generating outcomes. 2 When studies require both quantitative and qualitative research questions, this suggests an integrative process between both research methods wherein a single mixed-methods research question can be developed. 1
FRAMEWORKS FOR DEVELOPING RESEARCH QUESTIONS AND HYPOTHESES
Research questions followed by hypotheses should be developed before the start of the study. 1 , 12 , 14 It is crucial to develop feasible research questions on a topic that is interesting to both the researcher and the scientific community. This can be achieved by a meticulous review of previous and current studies to establish a novel topic. Specific areas are subsequently focused on to generate ethical research questions. The relevance of the research questions is evaluated in terms of clarity of the resulting data, specificity of the methodology, objectivity of the outcome, depth of the research, and impact of the study. 1 , 5 These aspects constitute the FINER criteria (i.e., Feasible, Interesting, Novel, Ethical, and Relevant). 1 Clarity and effectiveness are achieved if research questions meet the FINER criteria. In addition to the FINER criteria, Ratan et al. described focus, complexity, novelty, feasibility, and measurability for evaluating the effectiveness of research questions. 14
The PICOT and PEO frameworks are also used when developing research questions. 1 The following elements are addressed in these frameworks, PICOT: P-population/patients/problem, I-intervention or indicator being studied, C-comparison group, O-outcome of interest, and T-timeframe of the study; PEO: P-population being studied, E-exposure to preexisting conditions, and O-outcome of interest. 1 Research questions are also considered good if these meet the “FINERMAPS” framework: Feasible, Interesting, Novel, Ethical, Relevant, Manageable, Appropriate, Potential value/publishable, and Systematic. 14
As we indicated earlier, research questions and hypotheses that are not carefully formulated result in unethical studies or poor outcomes. To illustrate this, we provide some examples of ambiguous research question and hypotheses that result in unclear and weak research objectives in quantitative research ( Table 6 ) 16 and qualitative research ( Table 7 ) 17 , and how to transform these ambiguous research question(s) and hypothesis(es) into clear and good statements.
a These statements were composed for comparison and illustrative purposes only.
b These statements are direct quotes from Higashihara and Horiuchi. 16
a This statement is a direct quote from Shimoda et al. 17
The other statements were composed for comparison and illustrative purposes only.
CONSTRUCTING RESEARCH QUESTIONS AND HYPOTHESES
To construct effective research questions and hypotheses, it is very important to 1) clarify the background and 2) identify the research problem at the outset of the research, within a specific timeframe. 9 Then, 3) review or conduct preliminary research to collect all available knowledge about the possible research questions by studying theories and previous studies. 18 Afterwards, 4) construct research questions to investigate the research problem. Identify variables to be accessed from the research questions 4 and make operational definitions of constructs from the research problem and questions. Thereafter, 5) construct specific deductive or inductive predictions in the form of hypotheses. 4 Finally, 6) state the study aims . This general flow for constructing effective research questions and hypotheses prior to conducting research is shown in Fig. 1 .

Research questions are used more frequently in qualitative research than objectives or hypotheses. 3 These questions seek to discover, understand, explore or describe experiences by asking “What” or “How.” The questions are open-ended to elicit a description rather than to relate variables or compare groups. The questions are continually reviewed, reformulated, and changed during the qualitative study. 3 Research questions are also used more frequently in survey projects than hypotheses in experiments in quantitative research to compare variables and their relationships.
Hypotheses are constructed based on the variables identified and as an if-then statement, following the template, ‘If a specific action is taken, then a certain outcome is expected.’ At this stage, some ideas regarding expectations from the research to be conducted must be drawn. 18 Then, the variables to be manipulated (independent) and influenced (dependent) are defined. 4 Thereafter, the hypothesis is stated and refined, and reproducible data tailored to the hypothesis are identified, collected, and analyzed. 4 The hypotheses must be testable and specific, 18 and should describe the variables and their relationships, the specific group being studied, and the predicted research outcome. 18 Hypotheses construction involves a testable proposition to be deduced from theory, and independent and dependent variables to be separated and measured separately. 3 Therefore, good hypotheses must be based on good research questions constructed at the start of a study or trial. 12
In summary, research questions are constructed after establishing the background of the study. Hypotheses are then developed based on the research questions. Thus, it is crucial to have excellent research questions to generate superior hypotheses. In turn, these would determine the research objectives and the design of the study, and ultimately, the outcome of the research. 12 Algorithms for building research questions and hypotheses are shown in Fig. 2 for quantitative research and in Fig. 3 for qualitative research.

EXAMPLES OF RESEARCH QUESTIONS FROM PUBLISHED ARTICLES
- EXAMPLE 1. Descriptive research question (quantitative research)
- - Presents research variables to be assessed (distinct phenotypes and subphenotypes)
- “BACKGROUND: Since COVID-19 was identified, its clinical and biological heterogeneity has been recognized. Identifying COVID-19 phenotypes might help guide basic, clinical, and translational research efforts.
- RESEARCH QUESTION: Does the clinical spectrum of patients with COVID-19 contain distinct phenotypes and subphenotypes? ” 19
- EXAMPLE 2. Relationship research question (quantitative research)
- - Shows interactions between dependent variable (static postural control) and independent variable (peripheral visual field loss)
- “Background: Integration of visual, vestibular, and proprioceptive sensations contributes to postural control. People with peripheral visual field loss have serious postural instability. However, the directional specificity of postural stability and sensory reweighting caused by gradual peripheral visual field loss remain unclear.
- Research question: What are the effects of peripheral visual field loss on static postural control ?” 20
- EXAMPLE 3. Comparative research question (quantitative research)
- - Clarifies the difference among groups with an outcome variable (patients enrolled in COMPERA with moderate PH or severe PH in COPD) and another group without the outcome variable (patients with idiopathic pulmonary arterial hypertension (IPAH))
- “BACKGROUND: Pulmonary hypertension (PH) in COPD is a poorly investigated clinical condition.
- RESEARCH QUESTION: Which factors determine the outcome of PH in COPD?
- STUDY DESIGN AND METHODS: We analyzed the characteristics and outcome of patients enrolled in the Comparative, Prospective Registry of Newly Initiated Therapies for Pulmonary Hypertension (COMPERA) with moderate or severe PH in COPD as defined during the 6th PH World Symposium who received medical therapy for PH and compared them with patients with idiopathic pulmonary arterial hypertension (IPAH) .” 21
- EXAMPLE 4. Exploratory research question (qualitative research)
- - Explores areas that have not been fully investigated (perspectives of families and children who receive care in clinic-based child obesity treatment) to have a deeper understanding of the research problem
- “Problem: Interventions for children with obesity lead to only modest improvements in BMI and long-term outcomes, and data are limited on the perspectives of families of children with obesity in clinic-based treatment. This scoping review seeks to answer the question: What is known about the perspectives of families and children who receive care in clinic-based child obesity treatment? This review aims to explore the scope of perspectives reported by families of children with obesity who have received individualized outpatient clinic-based obesity treatment.” 22
- EXAMPLE 5. Relationship research question (quantitative research)
- - Defines interactions between dependent variable (use of ankle strategies) and independent variable (changes in muscle tone)
- “Background: To maintain an upright standing posture against external disturbances, the human body mainly employs two types of postural control strategies: “ankle strategy” and “hip strategy.” While it has been reported that the magnitude of the disturbance alters the use of postural control strategies, it has not been elucidated how the level of muscle tone, one of the crucial parameters of bodily function, determines the use of each strategy. We have previously confirmed using forward dynamics simulations of human musculoskeletal models that an increased muscle tone promotes the use of ankle strategies. The objective of the present study was to experimentally evaluate a hypothesis: an increased muscle tone promotes the use of ankle strategies. Research question: Do changes in the muscle tone affect the use of ankle strategies ?” 23
EXAMPLES OF HYPOTHESES IN PUBLISHED ARTICLES
- EXAMPLE 1. Working hypothesis (quantitative research)
- - A hypothesis that is initially accepted for further research to produce a feasible theory
- “As fever may have benefit in shortening the duration of viral illness, it is plausible to hypothesize that the antipyretic efficacy of ibuprofen may be hindering the benefits of a fever response when taken during the early stages of COVID-19 illness .” 24
- “In conclusion, it is plausible to hypothesize that the antipyretic efficacy of ibuprofen may be hindering the benefits of a fever response . The difference in perceived safety of these agents in COVID-19 illness could be related to the more potent efficacy to reduce fever with ibuprofen compared to acetaminophen. Compelling data on the benefit of fever warrant further research and review to determine when to treat or withhold ibuprofen for early stage fever for COVID-19 and other related viral illnesses .” 24
- EXAMPLE 2. Exploratory hypothesis (qualitative research)
- - Explores particular areas deeper to clarify subjective experience and develop a formal hypothesis potentially testable in a future quantitative approach
- “We hypothesized that when thinking about a past experience of help-seeking, a self distancing prompt would cause increased help-seeking intentions and more favorable help-seeking outcome expectations .” 25
- “Conclusion
- Although a priori hypotheses were not supported, further research is warranted as results indicate the potential for using self-distancing approaches to increasing help-seeking among some people with depressive symptomatology.” 25
- EXAMPLE 3. Hypothesis-generating research to establish a framework for hypothesis testing (qualitative research)
- “We hypothesize that compassionate care is beneficial for patients (better outcomes), healthcare systems and payers (lower costs), and healthcare providers (lower burnout). ” 26
- Compassionomics is the branch of knowledge and scientific study of the effects of compassionate healthcare. Our main hypotheses are that compassionate healthcare is beneficial for (1) patients, by improving clinical outcomes, (2) healthcare systems and payers, by supporting financial sustainability, and (3) HCPs, by lowering burnout and promoting resilience and well-being. The purpose of this paper is to establish a scientific framework for testing the hypotheses above . If these hypotheses are confirmed through rigorous research, compassionomics will belong in the science of evidence-based medicine, with major implications for all healthcare domains.” 26
- EXAMPLE 4. Statistical hypothesis (quantitative research)
- - An assumption is made about the relationship among several population characteristics ( gender differences in sociodemographic and clinical characteristics of adults with ADHD ). Validity is tested by statistical experiment or analysis ( chi-square test, Students t-test, and logistic regression analysis)
- “Our research investigated gender differences in sociodemographic and clinical characteristics of adults with ADHD in a Japanese clinical sample. Due to unique Japanese cultural ideals and expectations of women's behavior that are in opposition to ADHD symptoms, we hypothesized that women with ADHD experience more difficulties and present more dysfunctions than men . We tested the following hypotheses: first, women with ADHD have more comorbidities than men with ADHD; second, women with ADHD experience more social hardships than men, such as having less full-time employment and being more likely to be divorced.” 27
- “Statistical Analysis
- ( text omitted ) Between-gender comparisons were made using the chi-squared test for categorical variables and Students t-test for continuous variables…( text omitted ). A logistic regression analysis was performed for employment status, marital status, and comorbidity to evaluate the independent effects of gender on these dependent variables.” 27
EXAMPLES OF HYPOTHESIS AS WRITTEN IN PUBLISHED ARTICLES IN RELATION TO OTHER PARTS
- EXAMPLE 1. Background, hypotheses, and aims are provided
- “Pregnant women need skilled care during pregnancy and childbirth, but that skilled care is often delayed in some countries …( text omitted ). The focused antenatal care (FANC) model of WHO recommends that nurses provide information or counseling to all pregnant women …( text omitted ). Job aids are visual support materials that provide the right kind of information using graphics and words in a simple and yet effective manner. When nurses are not highly trained or have many work details to attend to, these job aids can serve as a content reminder for the nurses and can be used for educating their patients (Jennings, Yebadokpo, Affo, & Agbogbe, 2010) ( text omitted ). Importantly, additional evidence is needed to confirm how job aids can further improve the quality of ANC counseling by health workers in maternal care …( text omitted )” 28
- “ This has led us to hypothesize that the quality of ANC counseling would be better if supported by job aids. Consequently, a better quality of ANC counseling is expected to produce higher levels of awareness concerning the danger signs of pregnancy and a more favorable impression of the caring behavior of nurses .” 28
- “This study aimed to examine the differences in the responses of pregnant women to a job aid-supported intervention during ANC visit in terms of 1) their understanding of the danger signs of pregnancy and 2) their impression of the caring behaviors of nurses to pregnant women in rural Tanzania.” 28
- EXAMPLE 2. Background, hypotheses, and aims are provided
- “We conducted a two-arm randomized controlled trial (RCT) to evaluate and compare changes in salivary cortisol and oxytocin levels of first-time pregnant women between experimental and control groups. The women in the experimental group touched and held an infant for 30 min (experimental intervention protocol), whereas those in the control group watched a DVD movie of an infant (control intervention protocol). The primary outcome was salivary cortisol level and the secondary outcome was salivary oxytocin level.” 29
- “ We hypothesize that at 30 min after touching and holding an infant, the salivary cortisol level will significantly decrease and the salivary oxytocin level will increase in the experimental group compared with the control group .” 29
- EXAMPLE 3. Background, aim, and hypothesis are provided
- “In countries where the maternal mortality ratio remains high, antenatal education to increase Birth Preparedness and Complication Readiness (BPCR) is considered one of the top priorities [1]. BPCR includes birth plans during the antenatal period, such as the birthplace, birth attendant, transportation, health facility for complications, expenses, and birth materials, as well as family coordination to achieve such birth plans. In Tanzania, although increasing, only about half of all pregnant women attend an antenatal clinic more than four times [4]. Moreover, the information provided during antenatal care (ANC) is insufficient. In the resource-poor settings, antenatal group education is a potential approach because of the limited time for individual counseling at antenatal clinics.” 30
- “This study aimed to evaluate an antenatal group education program among pregnant women and their families with respect to birth-preparedness and maternal and infant outcomes in rural villages of Tanzania.” 30
- “ The study hypothesis was if Tanzanian pregnant women and their families received a family-oriented antenatal group education, they would (1) have a higher level of BPCR, (2) attend antenatal clinic four or more times, (3) give birth in a health facility, (4) have less complications of women at birth, and (5) have less complications and deaths of infants than those who did not receive the education .” 30
Research questions and hypotheses are crucial components to any type of research, whether quantitative or qualitative. These questions should be developed at the very beginning of the study. Excellent research questions lead to superior hypotheses, which, like a compass, set the direction of research, and can often determine the successful conduct of the study. Many research studies have floundered because the development of research questions and subsequent hypotheses was not given the thought and meticulous attention needed. The development of research questions and hypotheses is an iterative process based on extensive knowledge of the literature and insightful grasp of the knowledge gap. Focused, concise, and specific research questions provide a strong foundation for constructing hypotheses which serve as formal predictions about the research outcomes. Research questions and hypotheses are crucial elements of research that should not be overlooked. They should be carefully thought of and constructed when planning research. This avoids unethical studies and poor outcomes by defining well-founded objectives that determine the design, course, and outcome of the study.
Disclosure: The authors have no potential conflicts of interest to disclose.
Author Contributions:
- Conceptualization: Barroga E, Matanguihan GJ.
- Methodology: Barroga E, Matanguihan GJ.
- Writing - original draft: Barroga E, Matanguihan GJ.
- Writing - review & editing: Barroga E, Matanguihan GJ.
- Skip to main content
- Skip to primary sidebar
- Skip to footer
- QuestionPro

- Solutions Industries Gaming Automotive Sports and events Education Government Travel & Hospitality Financial Services Healthcare Cannabis Technology Use Case NPS+ Communities Audience Contactless surveys Mobile LivePolls Member Experience GDPR Positive People Science 360 Feedback Surveys
- Resources Blog eBooks Survey Templates Case Studies Training Help center

Home Market Research
Quantitative Research: What It Is, Practices & Methods
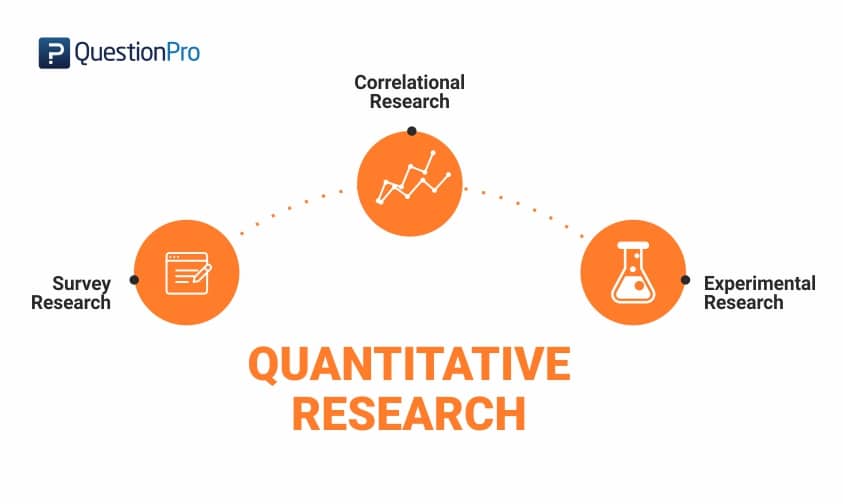
Quantitative research involves analyzing and gathering numerical data to uncover trends, calculate averages, evaluate relationships, and derive overarching insights. It’s used in various fields, including the natural and social sciences. Quantitative data analysis employs statistical techniques for processing and interpreting numeric data.
Research designs in the quantitative realm outline how data will be collected and analyzed with methods like experiments and surveys. Qualitative methods complement quantitative research by focusing on non-numerical data, adding depth to understanding. Data collection methods can be qualitative or quantitative, depending on research goals. Researchers often use a combination of both approaches to gain a comprehensive understanding of phenomena.
What is Quantitative Research?
Quantitative research is a systematic investigation of phenomena by gathering quantifiable data and performing statistical, mathematical, or computational techniques. Quantitative research collects statistically significant information from existing and potential customers using sampling methods and sending out online surveys , online polls , and questionnaires , for example.
One of the main characteristics of this type of research is that the results can be depicted in numerical form. After carefully collecting structured observations and understanding these numbers, it’s possible to predict the future of a product or service, establish causal relationships or Causal Research , and make changes accordingly. Quantitative research primarily centers on the analysis of numerical data and utilizes inferential statistics to derive conclusions that can be extrapolated to the broader population.
An example of a quantitative research study is the survey conducted to understand how long a doctor takes to tend to a patient when the patient walks into the hospital. A patient satisfaction survey can be administered to ask questions like how long a doctor takes to see a patient, how often a patient walks into a hospital, and other such questions, which are dependent variables in the research. This kind of research method is often employed in the social sciences, and it involves using mathematical frameworks and theories to effectively present data, ensuring that the results are logical, statistically sound, and unbiased.
Data collection in quantitative research uses a structured method and is typically conducted on larger samples representing the entire population. Researchers use quantitative methods to collect numerical data, which is then subjected to statistical analysis to determine statistically significant findings. This approach is valuable in both experimental research and social research, as it helps in making informed decisions and drawing reliable conclusions based on quantitative data.
Quantitative Research Characteristics
Quantitative research has several unique characteristics that make it well-suited for specific projects. Let’s explore the most crucial of these characteristics so that you can consider them when planning your next research project:
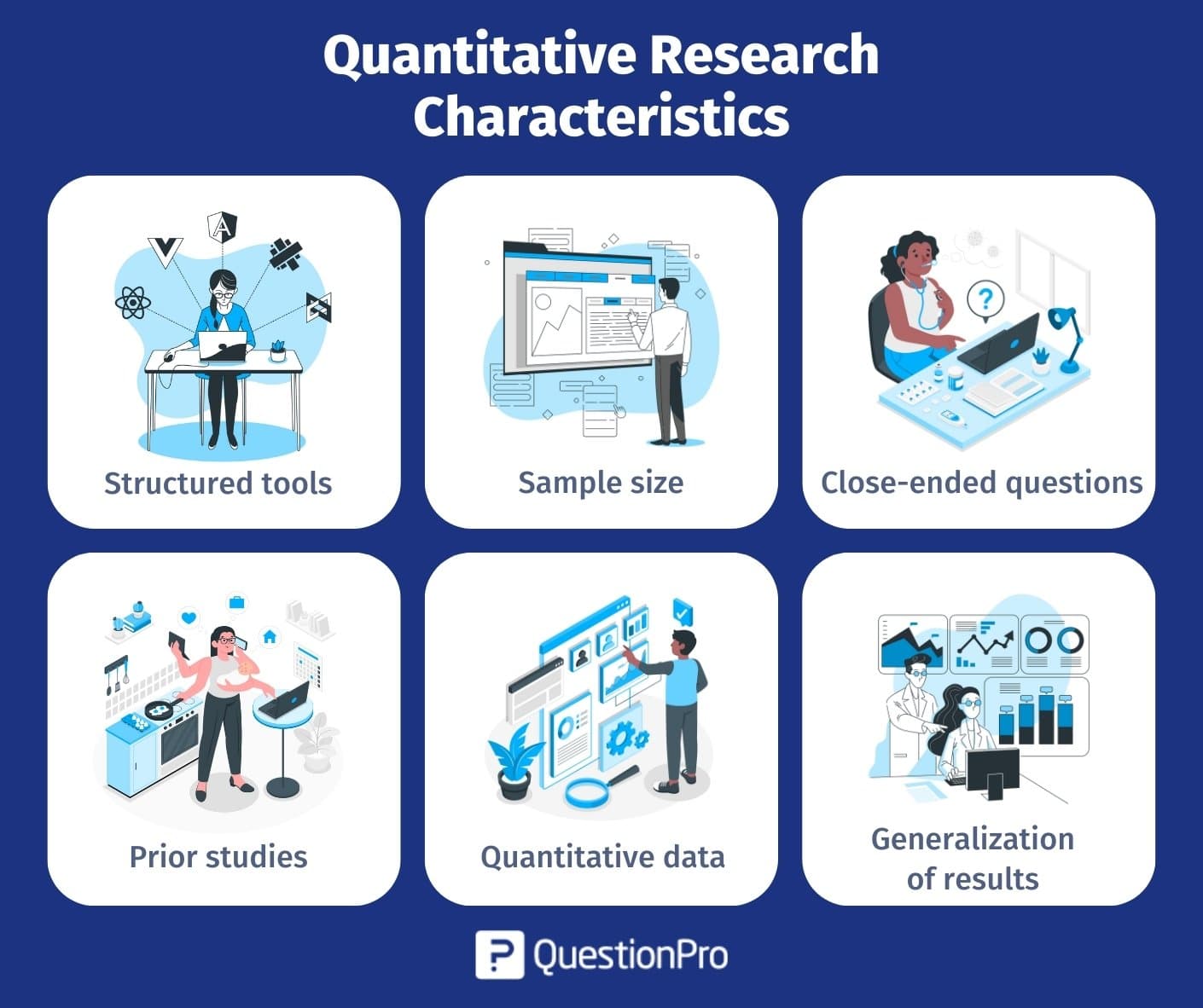
- Structured tools: Quantitative research relies on structured tools such as surveys, polls, or questionnaires to gather quantitative data . Using such structured methods helps collect in-depth and actionable numerical data from the survey respondents, making it easier to perform data analysis.
- Sample size: Quantitative research is conducted on a significant sample size representing the target market . Appropriate Survey Sampling methods, a fundamental aspect of quantitative research methods, must be employed when deriving the sample to fortify the research objective and ensure the reliability of the results.
- Close-ended questions: Closed-ended questions , specifically designed to align with the research objectives, are a cornerstone of quantitative research. These questions facilitate the collection of quantitative data and are extensively used in data collection processes.
- Prior studies: Before collecting feedback from respondents, researchers often delve into previous studies related to the research topic. This preliminary research helps frame the study effectively and ensures the data collection process is well-informed.
- Quantitative data: Typically, quantitative data is represented using tables, charts, graphs, or other numerical forms. This visual representation aids in understanding the collected data and is essential for rigorous data analysis, a key component of quantitative research methods.
- Generalization of results: One of the strengths of quantitative research is its ability to generalize results to the entire population. It means that the findings derived from a sample can be extrapolated to make informed decisions and take appropriate actions for improvement based on numerical data analysis.
Quantitative Research Methods
Quantitative research methods are systematic approaches used to gather and analyze numerical data to understand and draw conclusions about a phenomenon or population. Here are the quantitative research methods:
- Primary quantitative research methods
- Secondary quantitative research methods
Primary Quantitative Research Methods
Primary quantitative research is the most widely used method of conducting market research. The distinct feature of primary research is that the researcher focuses on collecting data directly rather than depending on data collected from previously done research. Primary quantitative research design can be broken down into three further distinctive tracks and the process flow. They are:
A. Techniques and Types of Studies
There are multiple types of primary quantitative research. They can be distinguished into the four following distinctive methods, which are:
01. Survey Research
Survey Research is fundamental for all quantitative outcome research methodologies and studies. Surveys are used to ask questions to a sample of respondents, using various types such as online polls, online surveys, paper questionnaires, web-intercept surveys , etc. Every small and big organization intends to understand what their customers think about their products and services, how well new features are faring in the market, and other such details.
By conducting survey research, an organization can ask multiple survey questions , collect data from a pool of customers, and analyze this collected data to produce numerical results. It is the first step towards collecting data for any research. You can use single ease questions . A single-ease question is a straightforward query that elicits a concise and uncomplicated response.
This type of research can be conducted with a specific target audience group and also can be conducted across multiple groups along with comparative analysis . A prerequisite for this type of research is that the sample of respondents must have randomly selected members. This way, a researcher can easily maintain the accuracy of the obtained results as a huge variety of respondents will be addressed using random selection.
Traditionally, survey research was conducted face-to-face or via phone calls. Still, with the progress made by online mediums such as email or social media, survey research has also spread to online mediums.There are two types of surveys , either of which can be chosen based on the time in hand and the kind of data required:
Cross-sectional surveys: Cross-sectional surveys are observational surveys conducted in situations where the researcher intends to collect data from a sample of the target population at a given point in time. Researchers can evaluate various variables at a particular time. Data gathered using this type of survey is from people who depict similarity in all variables except the variables which are considered for research . Throughout the survey, this one variable will stay constant.
- Cross-sectional surveys are popular with retail, SMEs, and healthcare industries. Information is garnered without modifying any parameters in the variable ecosystem.
- Multiple samples can be analyzed and compared using a cross-sectional survey research method.
- Multiple variables can be evaluated using this type of survey research.
- The only disadvantage of cross-sectional surveys is that the cause-effect relationship of variables cannot be established as it usually evaluates variables at a particular time and not across a continuous time frame.
Longitudinal surveys: Longitudinal surveys are also observational surveys , but unlike cross-sectional surveys, longitudinal surveys are conducted across various time durations to observe a change in respondent behavior and thought processes. This time can be days, months, years, or even decades. For instance, a researcher planning to analyze the change in buying habits of teenagers over 5 years will conduct longitudinal surveys.
- In cross-sectional surveys, the same variables were evaluated at a given time, and in longitudinal surveys, different variables can be analyzed at different intervals.
- Longitudinal surveys are extensively used in the field of medicine and applied sciences. Apart from these two fields, they are also used to observe a change in the market trend analysis , analyze customer satisfaction, or gain feedback on products/services.
- In situations where the sequence of events is highly essential, longitudinal surveys are used.
- Researchers say that when research subjects need to be thoroughly inspected before concluding, they rely on longitudinal surveys.
02. Correlational Research
A comparison between two entities is invariable. Correlation research is conducted to establish a relationship between two closely-knit entities and how one impacts the other, and what changes are eventually observed. This research method is carried out to give value to naturally occurring relationships, and a minimum of two different groups are required to conduct this quantitative research method successfully. Without assuming various aspects, a relationship between two groups or entities must be established.
Researchers use this quantitative research design to correlate two or more variables using mathematical analysis methods. Patterns, relationships, and trends between variables are concluded as they exist in their original setup. The impact of one of these variables on the other is observed, along with how it changes the relationship between the two variables. Researchers tend to manipulate one of the variables to attain the desired results.
Ideally, it is advised not to make conclusions merely based on correlational research. This is because it is not mandatory that if two variables are in sync that they are interrelated.
Example of Correlational Research Questions :
- The relationship between stress and depression.
- The equation between fame and money.
- The relation between activities in a third-grade class and its students.
03. Causal-comparative Research
This research method mainly depends on the factor of comparison. Also called quasi-experimental research , this quantitative research method is used by researchers to conclude the cause-effect equation between two or more variables, where one variable is dependent on the other independent variable. The independent variable is established but not manipulated, and its impact on the dependent variable is observed. These variables or groups must be formed as they exist in the natural setup. As the dependent and independent variables will always exist in a group, it is advised that the conclusions are carefully established by keeping all the factors in mind.
Causal-comparative research is not restricted to the statistical analysis of two variables but extends to analyzing how various variables or groups change under the influence of the same changes. This research is conducted irrespective of the type of relationship that exists between two or more variables. Statistical analysis plan is used to present the outcome using this quantitative research method.
Example of Causal-Comparative Research Questions:
- The impact of drugs on a teenager. The effect of good education on a freshman. The effect of substantial food provision in the villages of Africa.
04. Experimental Research
Also known as true experimentation, this research method relies on a theory. As the name suggests, experimental research is usually based on one or more theories. This theory has yet to be proven before and is merely a supposition. In experimental research, an analysis is done around proving or disproving the statement. This research method is used in natural sciences. Traditional research methods are more effective than modern techniques.
There can be multiple theories in experimental research. A theory is a statement that can be verified or refuted.
After establishing the statement, efforts are made to understand whether it is valid or invalid. This quantitative research method is mainly used in natural or social sciences as various statements must be proved right or wrong.
- Traditional research methods are more effective than modern techniques.
- Systematic teaching schedules help children who struggle to cope with the course.
- It is a boon to have responsible nursing staff for ailing parents.
B. Data Collection Methodologies
The second major step in primary quantitative research is data collection. Data collection can be divided into sampling methods and data collection using surveys and polls.
01. Data Collection Methodologies: Sampling Methods
There are two main sampling methods for quantitative research: Probability and Non-probability sampling .
Probability sampling: A theory of probability is used to filter individuals from a population and create samples in probability sampling . Participants of a sample are chosen by random selection processes. Each target audience member has an equal opportunity to be selected in the sample.
There are four main types of probability sampling:
- Simple random sampling: As the name indicates, simple random sampling is nothing but a random selection of elements for a sample. This sampling technique is implemented where the target population is considerably large.
- Stratified random sampling: In the stratified random sampling method , a large population is divided into groups (strata), and members of a sample are chosen randomly from these strata. The various segregated strata should ideally not overlap one another.
- Cluster sampling: Cluster sampling is a probability sampling method using which the main segment is divided into clusters, usually using geographic segmentation and demographic segmentation parameters.
- Systematic sampling: Systematic sampling is a technique where the starting point of the sample is chosen randomly, and all the other elements are chosen using a fixed interval. This interval is calculated by dividing the population size by the target sample size.
Non-probability sampling: Non-probability sampling is where the researcher’s knowledge and experience are used to create samples. Because of the researcher’s involvement, not all the target population members have an equal probability of being selected to be a part of a sample.
There are five non-probability sampling models:
- Convenience sampling: In convenience sampling , elements of a sample are chosen only due to one prime reason: their proximity to the researcher. These samples are quick and easy to implement as there is no other parameter of selection involved.
- Consecutive sampling: Consecutive sampling is quite similar to convenience sampling, except for the fact that researchers can choose a single element or a group of samples and conduct research consecutively over a significant period and then perform the same process with other samples.
- Quota sampling: Using quota sampling , researchers can select elements using their knowledge of target traits and personalities to form strata. Members of various strata can then be chosen to be a part of the sample as per the researcher’s understanding.
- Snowball sampling: Snowball sampling is conducted with target audiences who are difficult to contact and get information. It is popular in cases where the target audience for analysis research is rare to put together.
- Judgmental sampling: Judgmental sampling is a non-probability sampling method where samples are created only based on the researcher’s experience and research skill .
02. Data collection methodologies: Using surveys & polls
Once the sample is determined, then either surveys or polls can be distributed to collect the data for quantitative research.
Using surveys for primary quantitative research
A survey is defined as a research method used for collecting data from a pre-defined group of respondents to gain information and insights on various topics of interest. The ease of survey distribution and the wide number of people it can reach depending on the research time and objective makes it one of the most important aspects of conducting quantitative research.
Fundamental levels of measurement – nominal, ordinal, interval, and ratio scales
Four measurement scales are fundamental to creating a multiple-choice question in a survey. They are nominal, ordinal, interval, and ratio measurement scales without the fundamentals of which no multiple-choice questions can be created. Hence, it is crucial to understand these measurement levels to develop a robust survey.
Use of different question types
To conduct quantitative research, close-ended questions must be used in a survey. They can be a mix of multiple question types, including multiple-choice questions like semantic differential scale questions , rating scale questions , etc.
Survey Distribution and Survey Data Collection
In the above, we have seen the process of building a survey along with the research design to conduct primary quantitative research. Survey distribution to collect data is the other important aspect of the survey process. There are different ways of survey distribution. Some of the most commonly used methods are:
- Email: Sending a survey via email is the most widely used and effective survey distribution method. This method’s response rate is high because the respondents know your brand. You can use the QuestionPro email management feature to send out and collect survey responses.
- Buy respondents: Another effective way to distribute a survey and conduct primary quantitative research is to use a sample. Since the respondents are knowledgeable and are on the panel by their own will, responses are much higher.
- Embed survey on a website: Embedding a survey on a website increases a high number of responses as the respondent is already in close proximity to the brand when the survey pops up.
- Social distribution: Using social media to distribute the survey aids in collecting a higher number of responses from the people that are aware of the brand.
- QR code: QuestionPro QR codes store the URL for the survey. You can print/publish this code in magazines, signs, business cards, or on just about any object/medium.
- SMS survey: The SMS survey is a quick and time-effective way to collect a high number of responses.
- Offline Survey App: The QuestionPro App allows users to circulate surveys quickly, and the responses can be collected both online and offline.
Survey example
An example of a survey is a short customer satisfaction (CSAT) survey that can quickly be built and deployed to collect feedback about what the customer thinks about a brand and how satisfied and referenceable the brand is.
Using polls for primary quantitative research
Polls are a method to collect feedback using close-ended questions from a sample. The most commonly used types of polls are election polls and exit polls . Both of these are used to collect data from a large sample size but using basic question types like multiple-choice questions.
C. Data Analysis Techniques
The third aspect of primary quantitative research design is data analysis . After collecting raw data, there must be an analysis of this data to derive statistical inferences from this research. It is important to relate the results to the research objective and establish the statistical relevance of the results.
Remember to consider aspects of research that were not considered for the data collection process and report the difference between what was planned vs. what was actually executed.
It is then required to select precise Statistical Analysis Methods , such as SWOT, Conjoint, Cross-tabulation, etc., to analyze the quantitative data.
- SWOT analysis: SWOT Analysis stands for the acronym of Strengths, Weaknesses, Opportunities, and Threat analysis. Organizations use this statistical analysis technique to evaluate their performance internally and externally to develop effective strategies for improvement.
- Conjoint Analysis: Conjoint Analysis is a market analysis method to learn how individuals make complicated purchasing decisions. Trade-offs are involved in an individual’s daily activities, and these reflect their ability to decide from a complex list of product/service options.
- Cross-tabulation: Cross-tabulation is one of the preliminary statistical market analysis methods which establishes relationships, patterns, and trends within the various parameters of the research study.
- TURF Analysis: TURF Analysis , an acronym for Totally Unduplicated Reach and Frequency Analysis, is executed in situations where the reach of a favorable communication source is to be analyzed along with the frequency of this communication. It is used for understanding the potential of a target market.
Inferential statistics methods such as confidence interval, the margin of error, etc., can then be used to provide results.
Secondary Quantitative Research Methods
Secondary quantitative research or desk research is a research method that involves using already existing data or secondary data. Existing data is summarized and collated to increase the overall effectiveness of the research.
This research method involves collecting quantitative data from existing data sources like the internet, government resources, libraries, research reports, etc. Secondary quantitative research helps to validate the data collected from primary quantitative research and aid in strengthening or proving, or disproving previously collected data.
The following are five popularly used secondary quantitative research methods:
- Data available on the internet: With the high penetration of the internet and mobile devices, it has become increasingly easy to conduct quantitative research using the internet. Information about most research topics is available online, and this aids in boosting the validity of primary quantitative data.
- Government and non-government sources: Secondary quantitative research can also be conducted with the help of government and non-government sources that deal with market research reports. This data is highly reliable and in-depth and hence, can be used to increase the validity of quantitative research design.
- Public libraries: Now a sparingly used method of conducting quantitative research, it is still a reliable source of information, though. Public libraries have copies of important research that was conducted earlier. They are a storehouse of valuable information and documents from which information can be extracted.
- Educational institutions: Educational institutions conduct in-depth research on multiple topics, and hence, the reports that they publish are an important source of validation in quantitative research.
- Commercial information sources: Local newspapers, journals, magazines, radio, and TV stations are great sources to obtain data for secondary quantitative research. These commercial information sources have in-depth, first-hand information on market research, demographic segmentation, and similar subjects.
Quantitative Research Examples
Some examples of quantitative research are:
- A customer satisfaction template can be used if any organization would like to conduct a customer satisfaction (CSAT) survey . Through this kind of survey, an organization can collect quantitative data and metrics on the goodwill of the brand or organization in the customer’s mind based on multiple parameters such as product quality, pricing, customer experience, etc. This data can be collected by asking a net promoter score (NPS) question , matrix table questions, etc. that provide data in the form of numbers that can be analyzed and worked upon.
- Another example of quantitative research is an organization that conducts an event, collecting feedback from attendees about the value they see from the event. By using an event survey , the organization can collect actionable feedback about the satisfaction levels of customers during various phases of the event such as the sales, pre and post-event, the likelihood of recommending the organization to their friends and colleagues, hotel preferences for the future events and other such questions.
What are the Advantages of Quantitative Research?
There are many advantages to quantitative research. Some of the major advantages of why researchers use this method in market research are:
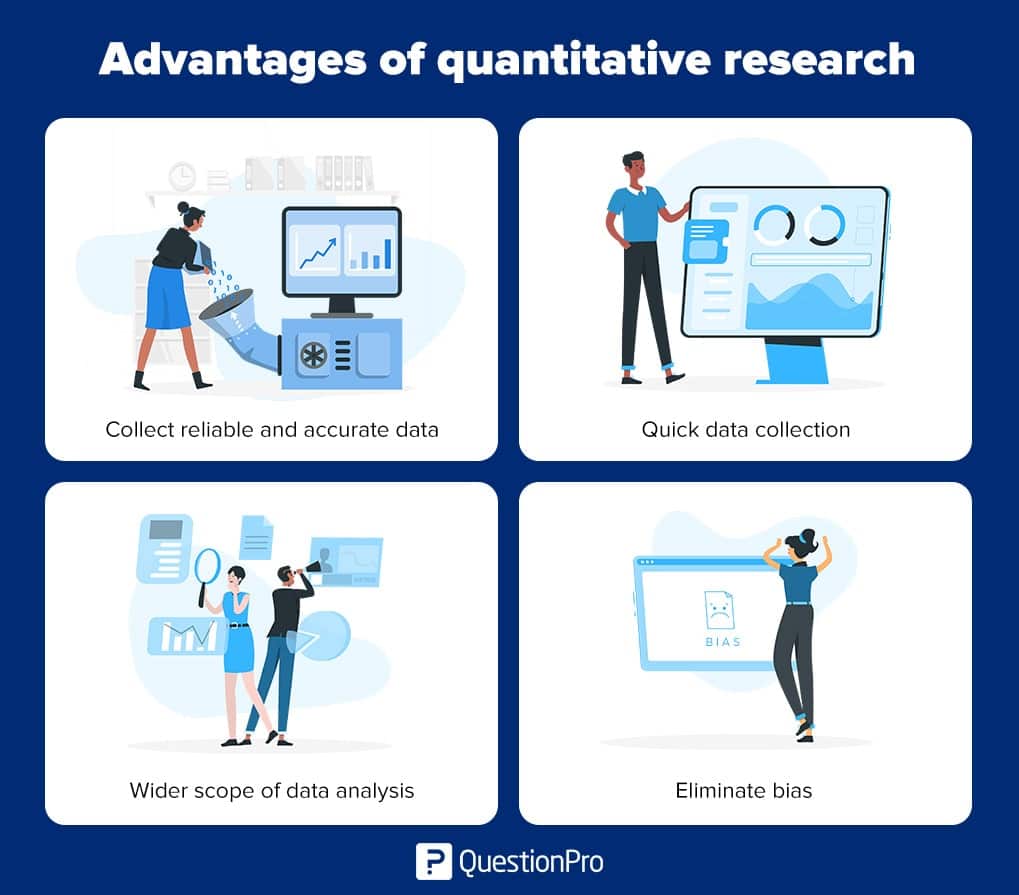
Collect Reliable and Accurate Data:
Quantitative research is a powerful method for collecting reliable and accurate quantitative data. Since data is collected, analyzed, and presented in numbers, the results obtained are incredibly reliable and objective. Numbers do not lie and offer an honest and precise picture of the conducted research without discrepancies. In situations where a researcher aims to eliminate bias and predict potential conflicts, quantitative research is the method of choice.
Quick Data Collection:
Quantitative research involves studying a group of people representing a larger population. Researchers use a survey or another quantitative research method to efficiently gather information from these participants, making the process of analyzing the data and identifying patterns faster and more manageable through the use of statistical analysis. This advantage makes quantitative research an attractive option for projects with time constraints.
Wider Scope of Data Analysis:
Quantitative research, thanks to its utilization of statistical methods, offers an extensive range of data collection and analysis. Researchers can delve into a broader spectrum of variables and relationships within the data, enabling a more thorough comprehension of the subject under investigation. This expanded scope is precious when dealing with complex research questions that require in-depth numerical analysis.
Eliminate Bias:
One of the significant advantages of quantitative research is its ability to eliminate bias. This research method leaves no room for personal comments or the biasing of results, as the findings are presented in numerical form. This objectivity makes the results fair and reliable in most cases, reducing the potential for researcher bias or subjectivity.
In summary, quantitative research involves collecting, analyzing, and presenting quantitative data using statistical analysis. It offers numerous advantages, including the collection of reliable and accurate data, quick data collection, a broader scope of data analysis, and the elimination of bias, making it a valuable approach in the field of research. When considering the benefits of quantitative research, it’s essential to recognize its strengths in contrast to qualitative methods and its role in collecting and analyzing numerical data for a more comprehensive understanding of research topics.
Best Practices to Conduct Quantitative Research
Here are some best practices for conducting quantitative research:
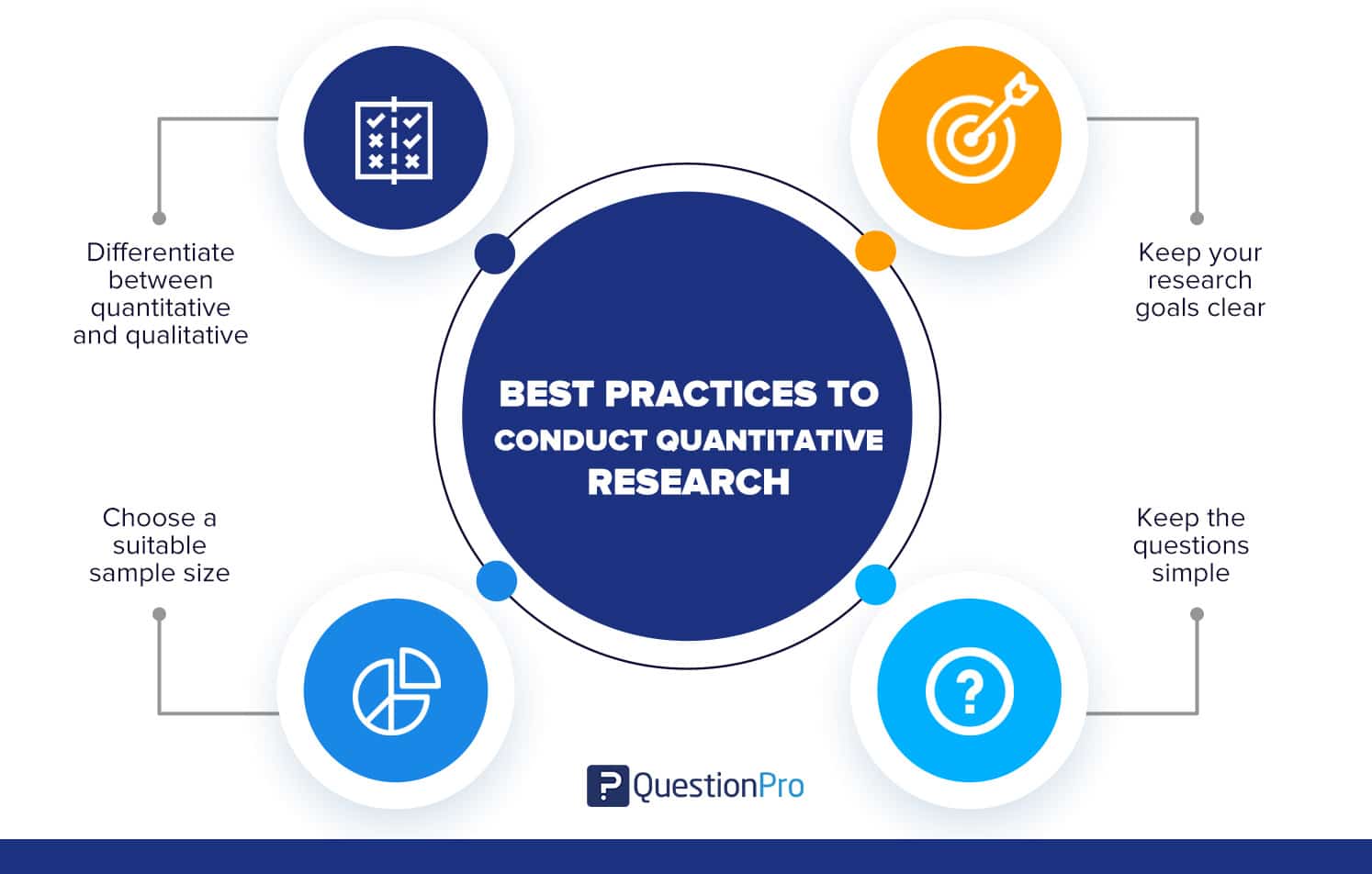
- Differentiate between quantitative and qualitative: Understand the difference between the two methodologies and apply the one that suits your needs best.
- Choose a suitable sample size: Ensure that you have a sample representative of your population and large enough to be statistically weighty.
- Keep your research goals clear and concise: Know your research goals before you begin data collection to ensure you collect the right amount and the right quantity of data.
- Keep the questions simple: Remember that you will be reaching out to a demographically wide audience. Pose simple questions for your respondents to understand easily.
Quantitative Research vs Qualitative Research
Quantitative research and qualitative research are two distinct approaches to conducting research, each with its own set of methods and objectives. Here’s a comparison of the two:
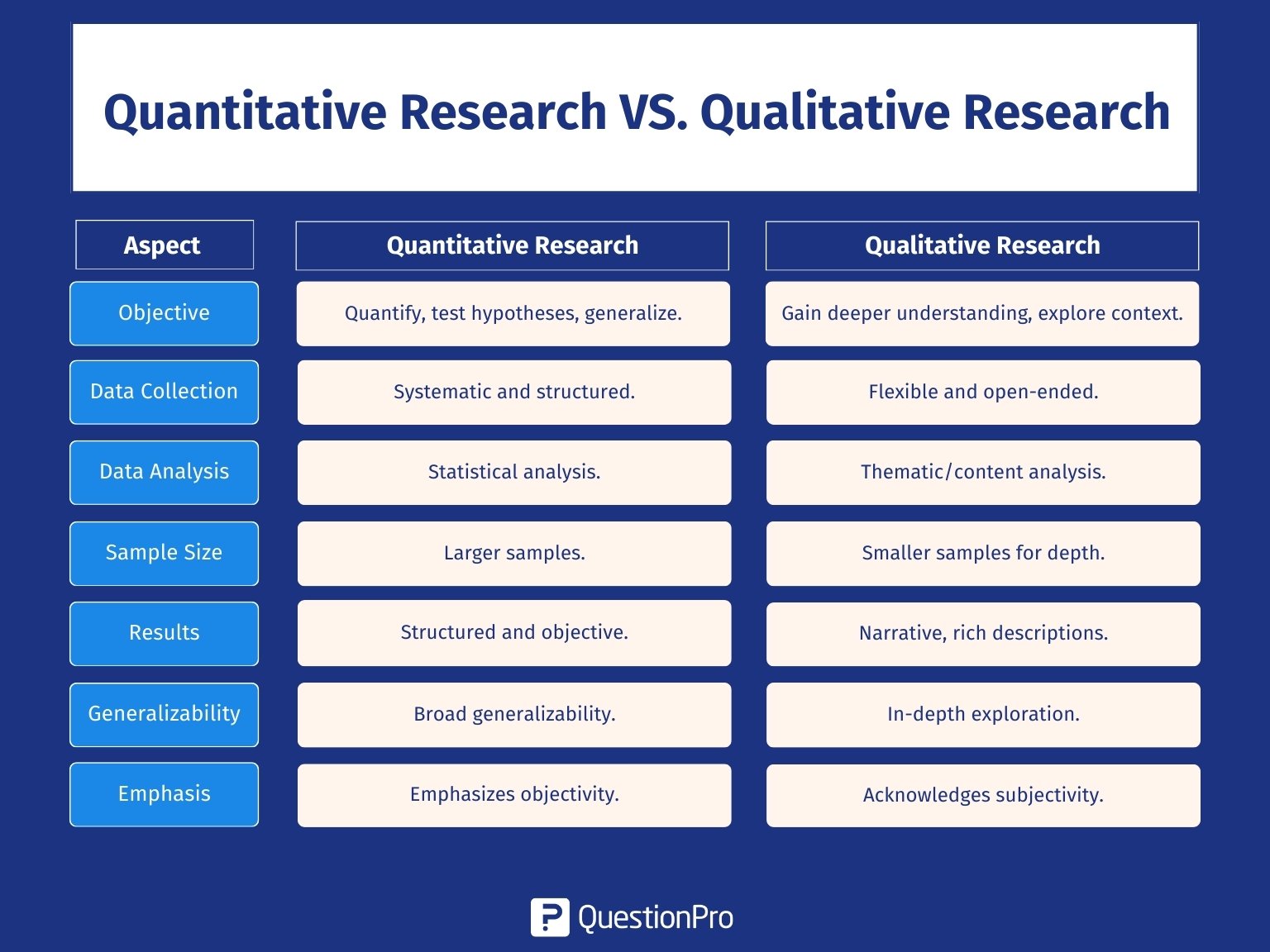
Quantitative Research
- Objective: The primary goal of quantitative research is to quantify and measure phenomena by collecting numerical data. It aims to test hypotheses, establish patterns, and generalize findings to a larger population.
- Data Collection: Quantitative research employs systematic and standardized approaches for data collection, including techniques like surveys, experiments, and observations that involve predefined variables. It is often collected from a large and representative sample.
- Data Analysis: Data is analyzed using statistical techniques, such as descriptive statistics, inferential statistics, and mathematical modeling. Researchers use statistical tests to draw conclusions and make generalizations based on numerical data.
- Sample Size: Quantitative research often involves larger sample sizes to ensure statistical significance and generalizability.
- Results: The results are typically presented in tables, charts, and statistical summaries, making them highly structured and objective.
- Generalizability: Researchers intentionally structure quantitative research to generate outcomes that can be helpful to a larger population, and they frequently seek to establish causative connections.
- Emphasis on Objectivity: Researchers aim to minimize bias and subjectivity, focusing on replicable and objective findings.
Qualitative Research
- Objective: Qualitative research seeks to gain a deeper understanding of the underlying motivations, behaviors, and experiences of individuals or groups. It explores the context and meaning of phenomena.
- Data Collection: Qualitative research employs adaptable and open-ended techniques for data collection, including methods like interviews, focus groups, observations, and content analysis. It allows participants to express their perspectives in their own words.
- Data Analysis: Data is analyzed through thematic analysis, content analysis, or grounded theory. Researchers focus on identifying patterns, themes, and insights in the data.
- Sample Size: Qualitative research typically involves smaller sample sizes due to the in-depth nature of data collection and analysis.
- Results: Findings are presented in narrative form, often in the participants’ own words. Results are subjective, context-dependent, and provide rich, detailed descriptions.
- Generalizability: Qualitative research does not aim for broad generalizability but focuses on in-depth exploration within a specific context. It provides a detailed understanding of a particular group or situation.
- Emphasis on Subjectivity: Researchers acknowledge the role of subjectivity and the researcher’s influence on the Research Process . Participant perspectives and experiences are central to the findings.
Researchers choose between quantitative and qualitative research methods based on their research objectives and the nature of the research question. Each approach has its advantages and drawbacks, and the decision between them hinges on the particular research objectives and the data needed to address research inquiries effectively.
Quantitative research is a structured way of collecting and analyzing data from various sources. Its purpose is to quantify the problem and understand its extent, seeking results that someone can project to a larger population.
Companies that use quantitative rather than qualitative research typically aim to measure magnitudes and seek objectively interpreted statistical results. So if you want to obtain quantitative data that helps you define the structured cause-and-effect relationship between the research problem and the factors, you should opt for this type of research.
At QuestionPro , we have various Best Data Collection Tools and features to conduct investigations of this type. You can create questionnaires and distribute them through our various methods. We also have sample services or various questions to guarantee the success of your study and the quality of the collected data.
Quantitative research is a systematic and structured approach to studying phenomena that involves the collection of measurable data and the application of statistical, mathematical, or computational techniques for analysis.
Quantitative research is characterized by structured tools like surveys, substantial sample sizes, closed-ended questions, reliance on prior studies, data presented numerically, and the ability to generalize findings to the broader population.
The two main methods of quantitative research are Primary quantitative research methods, involving data collection directly from sources, and Secondary quantitative research methods, which utilize existing data for analysis.
1.Surveying to measure employee engagement with numerical rating scales. 2.Analyzing sales data to identify trends in product demand and market share. 4.Examining test scores to assess the impact of a new teaching method on student performance. 4.Using website analytics to track user behavior and conversion rates for an online store.
1.Differentiate between quantitative and qualitative approaches. 2.Choose a representative sample size. 3.Define clear research goals before data collection. 4.Use simple and easily understandable survey questions.
MORE LIKE THIS
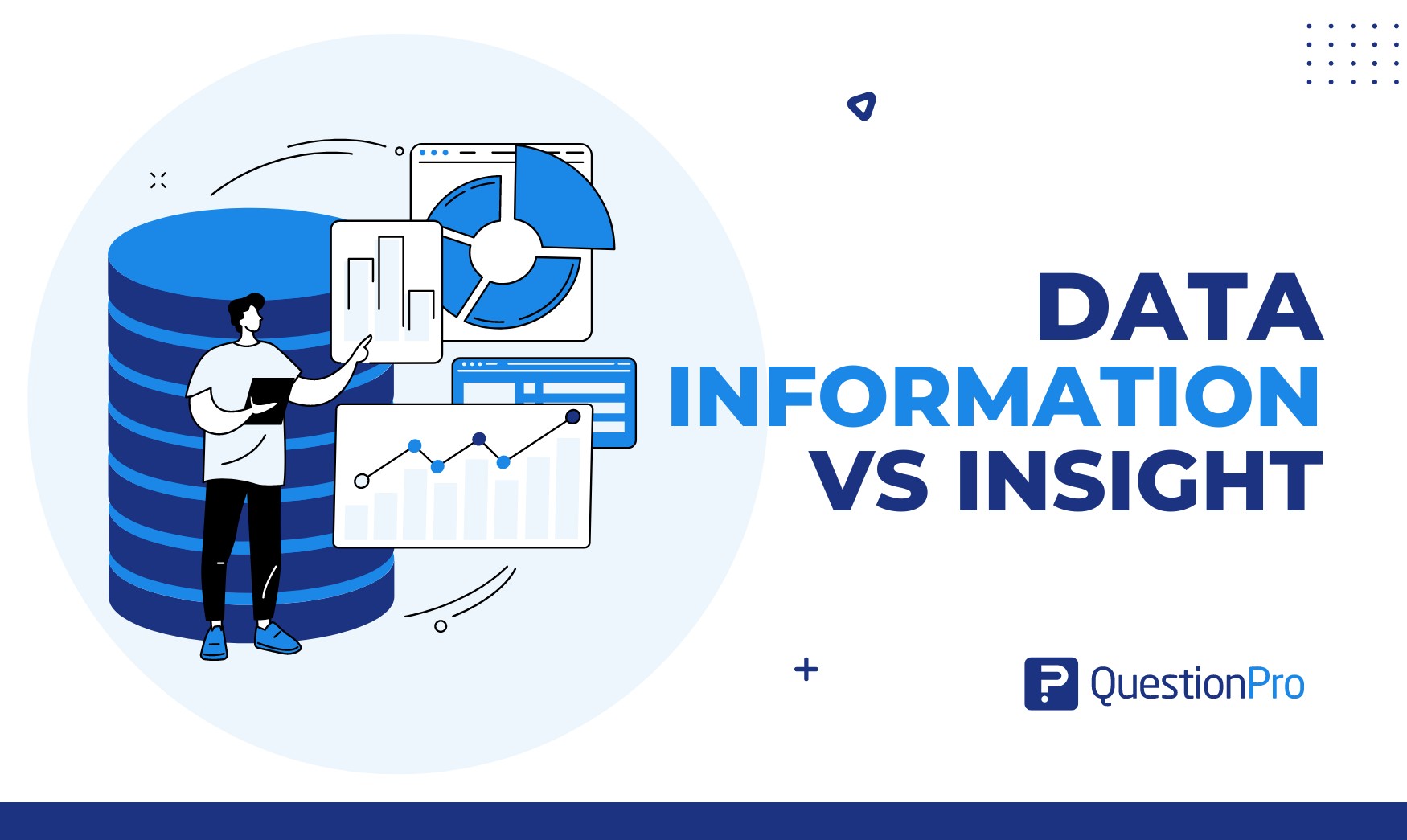
Data Information vs Insight: Essential differences
May 14, 2024
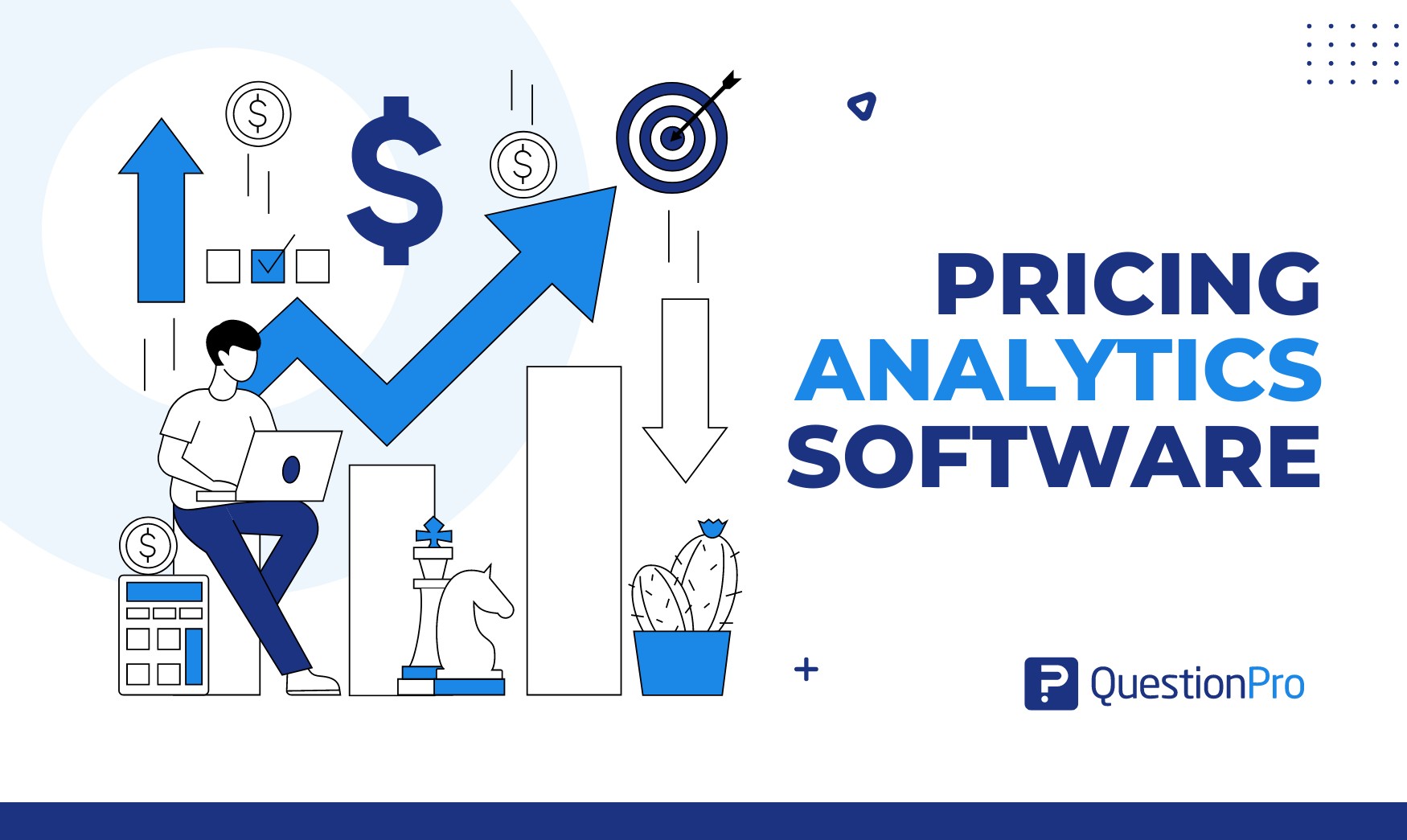
Pricing Analytics Software: Optimize Your Pricing Strategy
May 13, 2024
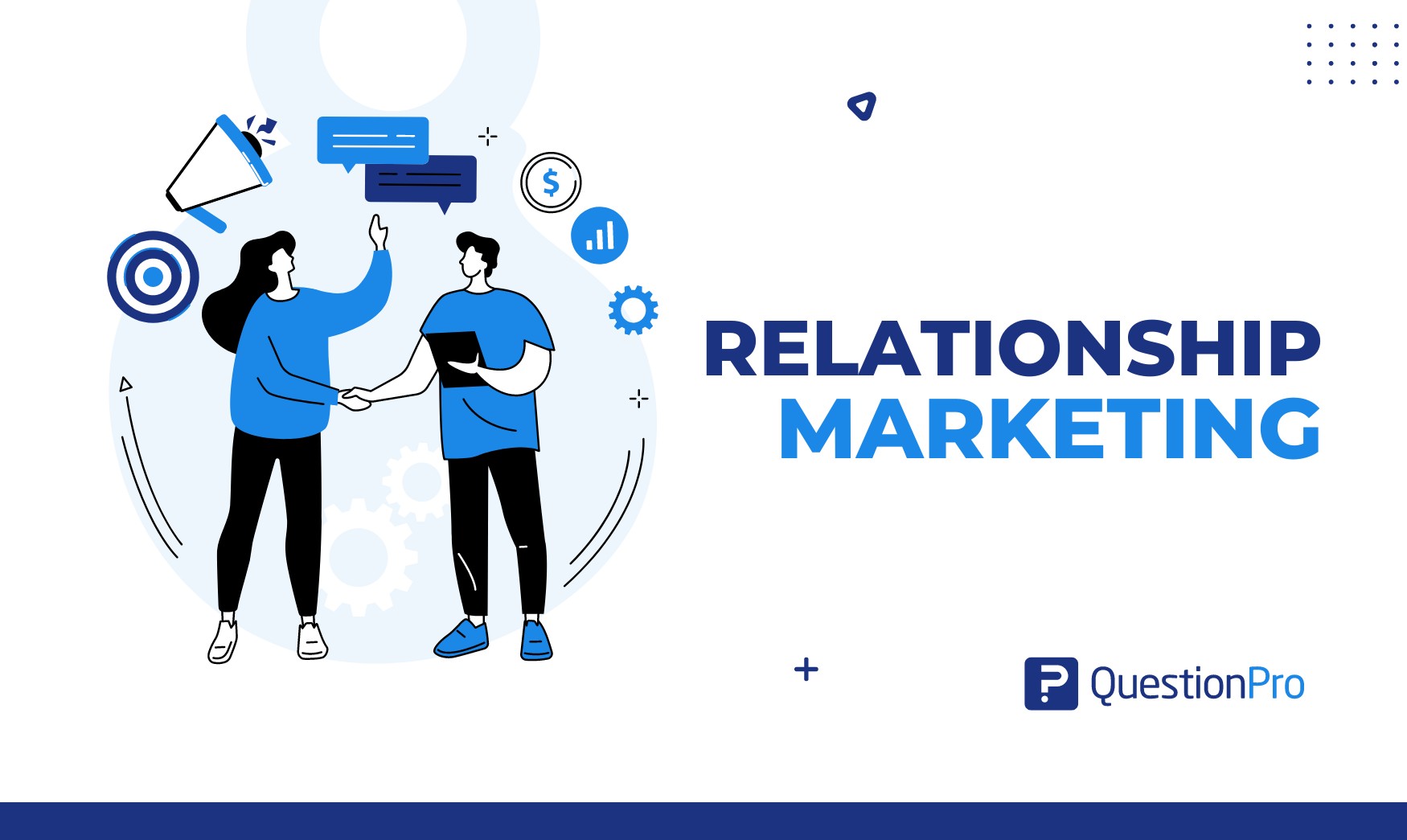
Relationship Marketing: What It Is, Examples & Top 7 Benefits
May 8, 2024
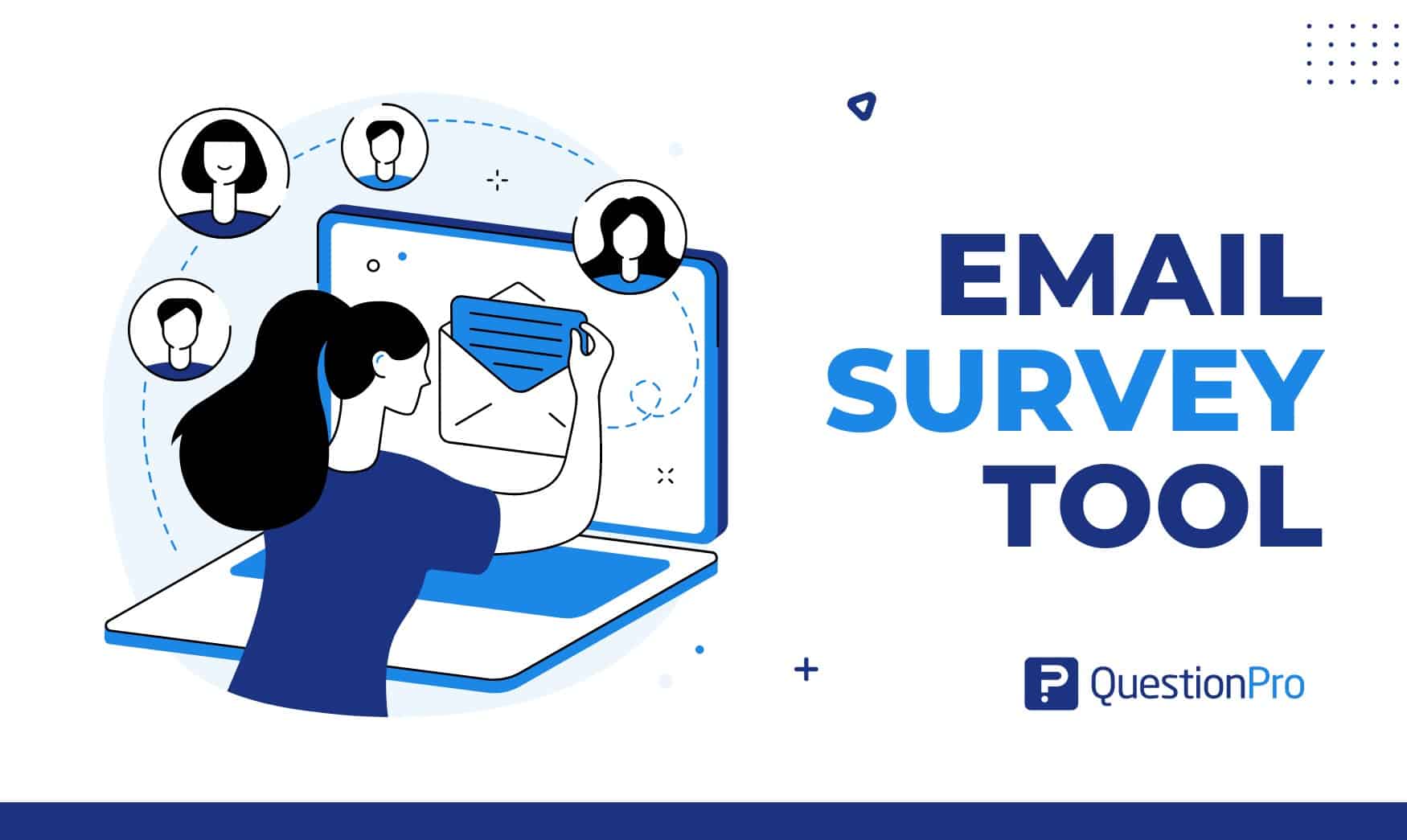
The Best Email Survey Tool to Boost Your Feedback Game
May 7, 2024
Other categories
- Academic Research
- Artificial Intelligence
- Assessments
- Brand Awareness
- Case Studies
- Communities
- Consumer Insights
- Customer effort score
- Customer Engagement
- Customer Experience
- Customer Loyalty
- Customer Research
- Customer Satisfaction
- Employee Benefits
- Employee Engagement
- Employee Retention
- Friday Five
- General Data Protection Regulation
- Insights Hub
- Life@QuestionPro
- Market Research
- Mobile diaries
- Mobile Surveys
- New Features
- Online Communities
- Question Types
- Questionnaire
- QuestionPro Products
- Release Notes
- Research Tools and Apps
- Revenue at Risk
- Survey Templates
- Training Tips
- Uncategorized
- Video Learning Series
- What’s Coming Up
- Workforce Intelligence

Quantitative and Qualitative Research
- I NEED TO . . .
What is Quantitative Research?
- What is Qualitative Research?
- Quantitative vs Qualitative
- Step 1: Accessing CINAHL
- Step 2: Create a Keyword Search
- Step 3: Create a Subject Heading Search
- Step 4: Repeat Steps 1-3 for Second Concept
- Step 5: Repeat Steps 1-3 for Quantitative Terms
- Step 6: Combining All Searches
- Step 7: Adding Limiters
- Step 8: Save Your Search!
- What Kind of Article is This?
- More Research Help This link opens in a new window
Quantitative methodology is the dominant research framework in the social sciences. It refers to a set of strategies, techniques and assumptions used to study psychological, social and economic processes through the exploration of numeric patterns . Quantitative research gathers a range of numeric data. Some of the numeric data is intrinsically quantitative (e.g. personal income), while in other cases the numeric structure is imposed (e.g. ‘On a scale from 1 to 10, how depressed did you feel last week?’). The collection of quantitative information allows researchers to conduct simple to extremely sophisticated statistical analyses that aggregate the data (e.g. averages, percentages), show relationships among the data (e.g. ‘Students with lower grade point averages tend to score lower on a depression scale’) or compare across aggregated data (e.g. the USA has a higher gross domestic product than Spain). Quantitative research includes methodologies such as questionnaires, structured observations or experiments and stands in contrast to qualitative research. Qualitative research involves the collection and analysis of narratives and/or open-ended observations through methodologies such as interviews, focus groups or ethnographies.
Coghlan, D., Brydon-Miller, M. (2014). The SAGE encyclopedia of action research (Vols. 1-2). London, : SAGE Publications Ltd doi: 10.4135/9781446294406
What is the purpose of quantitative research?
The purpose of quantitative research is to generate knowledge and create understanding about the social world. Quantitative research is used by social scientists, including communication researchers, to observe phenomena or occurrences affecting individuals. Social scientists are concerned with the study of people. Quantitative research is a way to learn about a particular group of people, known as a sample population. Using scientific inquiry, quantitative research relies on data that are observed or measured to examine questions about the sample population.
Allen, M. (2017). The SAGE encyclopedia of communication research methods (Vols. 1-4). Thousand Oaks, CA: SAGE Publications, Inc doi: 10.4135/9781483381411
How do I know if the study is a quantitative design? What type of quantitative study is it?
Quantitative Research Designs: Descriptive non-experimental, Quasi-experimental or Experimental?
Studies do not always explicitly state what kind of research design is being used. You will need to know how to decipher which design type is used. The following video will help you determine the quantitative design type.
- << Previous: I NEED TO . . .
- Next: What is Qualitative Research? >>
- Last Updated: May 13, 2024 12:01 PM
- URL: https://libguides.uta.edu/quantitative_and_qualitative_research
University of Texas Arlington Libraries 702 Planetarium Place · Arlington, TX 76019 · 817-272-3000
- Internet Privacy
- Accessibility
- Problems with a guide? Contact Us.
Have a language expert improve your writing
Run a free plagiarism check in 10 minutes, automatically generate references for free.
- Knowledge Base
- Methodology
- Qualitative vs Quantitative Research | Examples & Methods
Qualitative vs Quantitative Research | Examples & Methods
Published on 4 April 2022 by Raimo Streefkerk . Revised on 8 May 2023.
When collecting and analysing data, quantitative research deals with numbers and statistics, while qualitative research deals with words and meanings. Both are important for gaining different kinds of knowledge.
Common quantitative methods include experiments, observations recorded as numbers, and surveys with closed-ended questions. Qualitative research Qualitative research is expressed in words . It is used to understand concepts, thoughts or experiences. This type of research enables you to gather in-depth insights on topics that are not well understood.
Table of contents
The differences between quantitative and qualitative research, data collection methods, when to use qualitative vs quantitative research, how to analyse qualitative and quantitative data, frequently asked questions about qualitative and quantitative research.
Quantitative and qualitative research use different research methods to collect and analyse data, and they allow you to answer different kinds of research questions.
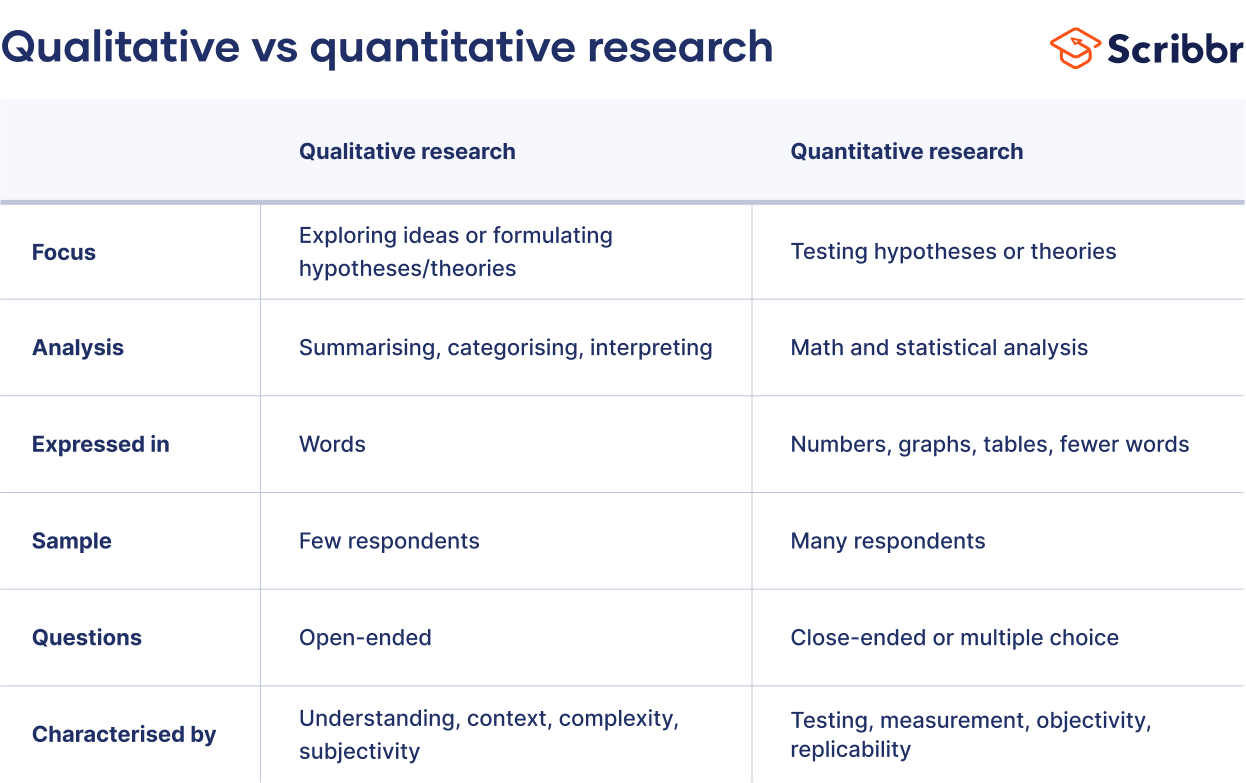
Prevent plagiarism, run a free check.
Quantitative and qualitative data can be collected using various methods. It is important to use a data collection method that will help answer your research question(s).
Many data collection methods can be either qualitative or quantitative. For example, in surveys, observations or case studies , your data can be represented as numbers (e.g. using rating scales or counting frequencies) or as words (e.g. with open-ended questions or descriptions of what you observe).
However, some methods are more commonly used in one type or the other.
Quantitative data collection methods
- Surveys : List of closed or multiple choice questions that is distributed to a sample (online, in person, or over the phone).
- Experiments : Situation in which variables are controlled and manipulated to establish cause-and-effect relationships.
- Observations: Observing subjects in a natural environment where variables can’t be controlled.
Qualitative data collection methods
- Interviews : Asking open-ended questions verbally to respondents.
- Focus groups: Discussion among a group of people about a topic to gather opinions that can be used for further research.
- Ethnography : Participating in a community or organisation for an extended period of time to closely observe culture and behavior.
- Literature review : Survey of published works by other authors.
A rule of thumb for deciding whether to use qualitative or quantitative data is:
- Use quantitative research if you want to confirm or test something (a theory or hypothesis)
- Use qualitative research if you want to understand something (concepts, thoughts, experiences)
For most research topics you can choose a qualitative, quantitative or mixed methods approach . Which type you choose depends on, among other things, whether you’re taking an inductive vs deductive research approach ; your research question(s) ; whether you’re doing experimental , correlational , or descriptive research ; and practical considerations such as time, money, availability of data, and access to respondents.
Quantitative research approach
You survey 300 students at your university and ask them questions such as: ‘on a scale from 1-5, how satisfied are your with your professors?’
You can perform statistical analysis on the data and draw conclusions such as: ‘on average students rated their professors 4.4’.
Qualitative research approach
You conduct in-depth interviews with 15 students and ask them open-ended questions such as: ‘How satisfied are you with your studies?’, ‘What is the most positive aspect of your study program?’ and ‘What can be done to improve the study program?’
Based on the answers you get you can ask follow-up questions to clarify things. You transcribe all interviews using transcription software and try to find commonalities and patterns.
Mixed methods approach
You conduct interviews to find out how satisfied students are with their studies. Through open-ended questions you learn things you never thought about before and gain new insights. Later, you use a survey to test these insights on a larger scale.
It’s also possible to start with a survey to find out the overall trends, followed by interviews to better understand the reasons behind the trends.
Qualitative or quantitative data by itself can’t prove or demonstrate anything, but has to be analysed to show its meaning in relation to the research questions. The method of analysis differs for each type of data.
Analysing quantitative data
Quantitative data is based on numbers. Simple maths or more advanced statistical analysis is used to discover commonalities or patterns in the data. The results are often reported in graphs and tables.
Applications such as Excel, SPSS, or R can be used to calculate things like:
- Average scores
- The number of times a particular answer was given
- The correlation or causation between two or more variables
- The reliability and validity of the results
Analysing qualitative data
Qualitative data is more difficult to analyse than quantitative data. It consists of text, images or videos instead of numbers.
Some common approaches to analysing qualitative data include:
- Qualitative content analysis : Tracking the occurrence, position and meaning of words or phrases
- Thematic analysis : Closely examining the data to identify the main themes and patterns
- Discourse analysis : Studying how communication works in social contexts
Quantitative research deals with numbers and statistics, while qualitative research deals with words and meanings.
Quantitative methods allow you to test a hypothesis by systematically collecting and analysing data, while qualitative methods allow you to explore ideas and experiences in depth.
In mixed methods research , you use both qualitative and quantitative data collection and analysis methods to answer your research question .
The research methods you use depend on the type of data you need to answer your research question .
- If you want to measure something or test a hypothesis , use quantitative methods . If you want to explore ideas, thoughts, and meanings, use qualitative methods .
- If you want to analyse a large amount of readily available data, use secondary data. If you want data specific to your purposes with control over how they are generated, collect primary data.
- If you want to establish cause-and-effect relationships between variables , use experimental methods. If you want to understand the characteristics of a research subject, use descriptive methods.
Data collection is the systematic process by which observations or measurements are gathered in research. It is used in many different contexts by academics, governments, businesses, and other organisations.
There are various approaches to qualitative data analysis , but they all share five steps in common:
- Prepare and organise your data.
- Review and explore your data.
- Develop a data coding system.
- Assign codes to the data.
- Identify recurring themes.
The specifics of each step depend on the focus of the analysis. Some common approaches include textual analysis , thematic analysis , and discourse analysis .
Cite this Scribbr article
If you want to cite this source, you can copy and paste the citation or click the ‘Cite this Scribbr article’ button to automatically add the citation to our free Reference Generator.
Streefkerk, R. (2023, May 08). Qualitative vs Quantitative Research | Examples & Methods. Scribbr. Retrieved 14 May 2024, from https://www.scribbr.co.uk/research-methods/quantitative-qualitative-research/
Is this article helpful?
Raimo Streefkerk
- (855) 776-7763
Training Maker
All Products
Qualaroo Insights
ProProfs.com
- Sign Up Free
Do you want a free Survey Software?
We have the #1 Online Survey Maker Software to get actionable user insights.
A Comprehensive Guide to Quantitative Research: Types, Characteristics, Methods & Examples
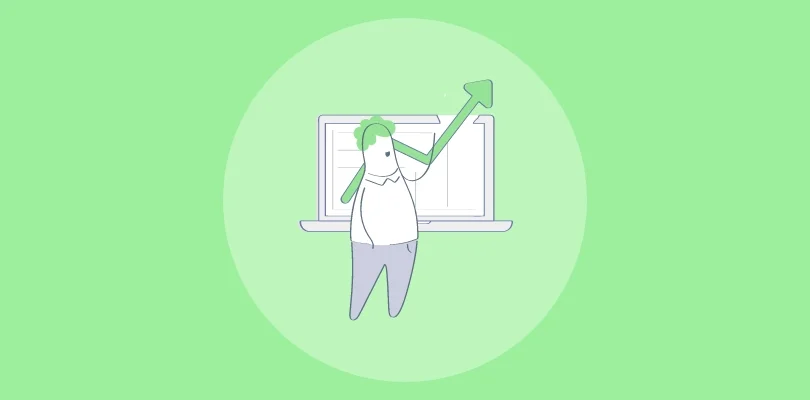
Step into the fascinating world of quantitative research, where numbers reveal extraordinary insights!
By gathering and studying data in a systematic way, quantitative research empowers us to understand our ever-changing world better. It helps understand a problem or an already-formed hypothesis by generating numerical data. The results don’t end here, as you can process these numbers to get actionable insights that aid decision-making.
You can use quantitative research to quantify opinions, behaviors, attitudes, and other definitive variables related to the market, customers, competitors, etc. The research is conducted on a larger sample population to draw predictive, average, and pattern-based insights.
Here, we delve into the intricacies of this research methodology, exploring various quantitative methods, their advantages, and real-life examples that showcase their impact and relevance.
Ready to embark on a journey of discovery and knowledge? Let’s go!
What Is Quantitative Research?
Quantitative research is a method that uses numbers and statistics to test theories about customer attitudes and behaviors. It helps researchers gather and analyze data systematically to gain valuable insights and draw evidence-based conclusions about customer preferences and trends.
Researchers use online surveys , questionnaires , polls , and quizzes to question a large number of people to obtain measurable and bias-free data.
In technical terms, quantitative research is mainly concerned with discovering facts about social phenomena while assuming a fixed and measurable reality.
Offering numbers and stats-based insights, this research methodology is a crucial part of primary research and helps understand how well an organizational decision is going to work out.
Let’s consider an example.
Suppose your qualitative analysis shows that your customers are looking for social media-based customer support . In that case, quantitative analysis will help you see how many of your customers are looking for this support.
If 10% of your customers are looking for such a service, you might or might not consider offering this feature. But, if 40% of your regular customers are seeking support via social media, then it is something you just cannot overlook.
Characteristics of Quantitative Research
Quantitative research clarifies the fuzziness of research data from qualitative research analysis. With numerical insights, you can formulate a better and more profitable business decision.
Hence, quantitative research is more readily contestable, sharpens intelligent discussion, helps you see the rival hypotheses, and dynamically contributes to the research process.
Let us have a quick look at some of its characteristics.
- Measurable Variables
The data collection methods in quantitative research are structured and contain items requiring measurable variables, such as age, number of family members, salary range, highest education, etc.
These structured data collection methods comprise polls, surveys, questionnaires, etc., and may have questions like the ones shown in the following image:
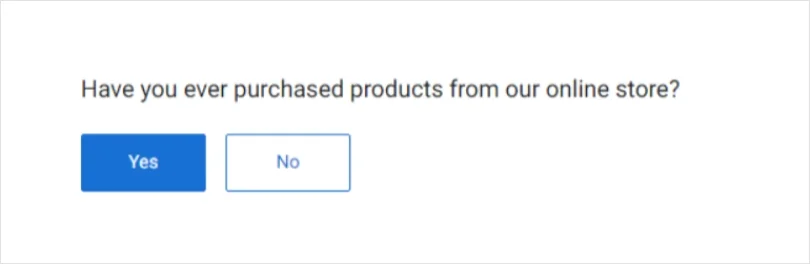
As you can see, all the variables are measurable. This ensures that the research is in-depth and provides less erroneous data for reliable, actionable insights.
- Sample Size
No matter what data analysis methods for quantitative research are being used, the sample size is kept such that it represents the target market.
As the main aim of the research methodology is to get numerical insights, the sample size should be fairly large. Depending on the survey objective and scope, it might span hundreds of thousands of people.
- Normal Population Distribution
To maintain the reliability of a quantitative research methodology, we assume that the population distribution curve is normal.
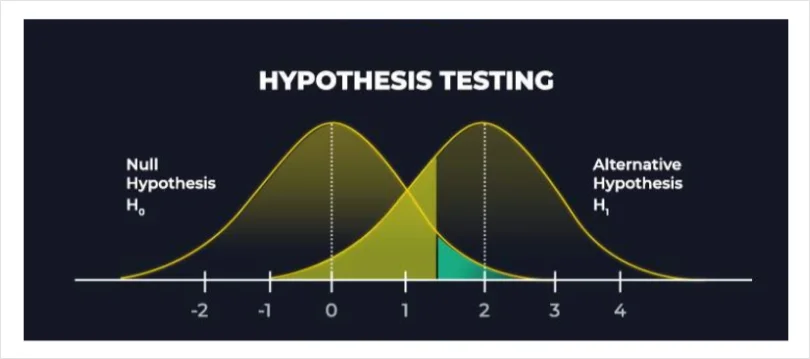
This type of population distribution curve is preferred over a non-normal distribution as the sample size is large, and the characteristics of the sample vary with its size.
This requires adhering to the random sampling principle to avoid the researcher’s bias in result interpretation. Any bias can ruin the fairness of the entire process and defeats the purpose of research.
- Well-Structured Data Representation
Data analysis in quantitative research produces highly structured results and can form well-defined graphical representations. Some common examples include tables, figures, graphs, etc., that combine large blocks of data.
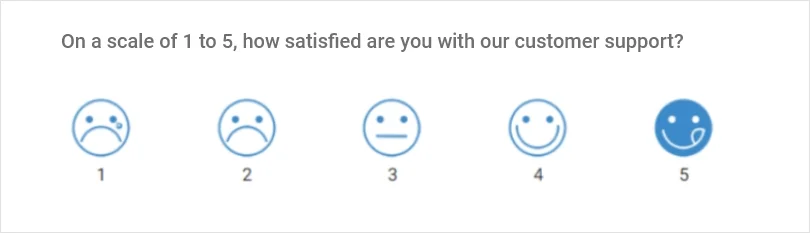
This way, you can discover hidden data trends, relationships, and differences among various measurable variables. This can help researchers understand the survey data and formulate actionable insights for decision-making.
- Predictive Outcomes
Quantitative analysis of data can also be used for estimations and prediction outcomes. You can construct if-then scenarios and analyze the data for the identification of any upcoming trends or events.
However, this requires advanced analytics and involves complex mathematical computations. So, it is mostly done via quantitative research tools that come with advanced analytics capabilities.
8 Best Practices to Conduct Quantitative Research
Here are some best practices to keep in mind while conducting quantitative research:
1. Define Research Objectives
There can be many ways to collect data via quantitative research methods that are chosen as per the research objective and scope. These methods allow you to build your own observations regarding any hypotheses – unknown, entirely new, or unexplained.
You can hypothesize a proof and build a prediction of outcomes supporting the same. You can also create a detailed stepwise plan for data collection, analysis, and testing.
Below, we explore quantitative research methods and discuss some examples to enhance your understanding of them.
2. Keep Your Questions Simple
The surveys are meant to reach people en-masse, and that includes a wide demographic range with recipients from all walks of life. Asking simple questions will ensure that they grasp what’s being asked easily.
Read More: Proven Tips to Avoid Leading and Loaded Questions in Your Survey
3. Develop a Solid Research Design
Choose an appropriate research design that aligns with your objectives, whether it’s experimental, quasi-experimental, or correlational. You also need to pay attention to the sample size and sampling technique such that it represents the target population accurately.
4. Use Reliable & Valid Instruments
It’s crucial to select or develop measurement instruments such as questionnaires, scales, or tests that have been validated and are reliable. Before proceeding with the main study, pilot-test these instruments on a small sample to assess their effectiveness and make any necessary improvements.
5. Ensure Data Quality
Implement data collection protocols to minimize errors and bias during data gathering. Double-check data entries and cleaning procedures to eliminate any inconsistencies or missing values that may affect the accuracy of your results. For instance, you might regularly cross-verify data entries to identify and correct any discrepancies.
6. Employ Appropriate Data Analysis Techniques
Select statistical methods that match the nature of your data and research questions. Whether it’s regression analysis, t-tests, ANOVA, or other techniques, using the right approach is important for drawing meaningful conclusions. Utilize software tools like SPSS or R for data analysis to ensure the accuracy and reproducibility of your findings.
7. Interpret Results Objectively
Present your findings in a clear and unbiased manner. Avoid making unwarranted causal claims, especially in correlational studies. Instead, focus on describing the relationships and patterns observed in your data.
8. Address Ethical Considerations
Prioritize ethical considerations throughout your research process. Obtain informed consent from participants, ensuring their voluntary participation and confidentiality of data. Comply with ethical guidelines and gain approval from a governing body if necessary.
Read More: How to Find Survey Participants & Respondents
Types of Quantitative Research Methods
Quantitative research is usually conducted using two methods. They are-
- Primary quantitative research methods
- Secondary quantitative research methods
1. Primary Methods
Primary quantitative research is the most popular way of conducting market research. The differentiating factor of this method is that the researcher relies on collecting data firsthand instead of relying on data collected from previous research.
There are multiple types of primary quantitative research. They can be distinguished based on three distinctive aspects, which are:
A. Techniques & Types of Studies:
- Survey Research
Surveys are the easiest, most common, and one of the most sought-after quantitative research techniques. The main aim of a survey is to widely gather and describe the characteristics of a target population or customers. Surveys are the foremost quantitative method preferred by both small and large organizations.
They help them understand their customers, products, and other brand offerings in a proper manner.
Surveys can be conducted using various methods, such as online polls, web-based surveys, paper questionnaires, phone calls, or face-to-face interviews. Survey research allows organizations to understand customer opinions, preferences, and behavior, making it crucial for market research and decision-making.
You can watch this quick video to learn more about creating surveys.
Surveys are of two types:
- Cross-Sectional Surveys Cross-sectional surveys are used to collect data from a sample of the target population at a specific point in time. Researchers evaluate various variables simultaneously to understand the relationships and patterns within the data.
- Cross-sectional surveys are popular in retail, small and medium-sized enterprises (SMEs), and healthcare industries, where they assess customer satisfaction, market trends, and product feedback.
- Longitudinal Surveys Longitudinal surveys are conducted over an extended period, observing changes in respondent behavior and thought processes.
- Researchers gather data from the same sample multiple times, enabling them to study trends and developments over time. These surveys are valuable in fields such as medicine, applied sciences, and market trend analysis.
Surveys can be distributed via various channels. Some of the most popular ones are listed below:
- Email: Sending surveys via email is a popular and effective method. People recognize your brand, leading to a higher response rate. With ProProfs Survey Maker’s in-mail survey-filling feature, you can easily send out and collect survey responses.
- Embed on a website: Boost your response rate by embedding the survey on your website. When visitors are already engaged with your brand, they are more likely to take the survey.
- Social media: Take advantage of social media platforms to distribute your survey. People familiar with your brand are likely to respond, increasing your response numbers.
- QR codes: QR codes store your survey’s URL, and you can print or publish these codes in magazines, signs, business cards, or any object to make it easy for people to access your survey.
- SMS survey: Collect a high number of responses quickly with SMS surveys. It’s a time-effective way to reach your target audience.
Read More: 24 Different Types of Survey Methods With Examples
2. Correlational Research:
Correlational research aims to establish relationships between two or more variables.
Researchers use statistical analysis to identify patterns and trends in the data, but it does not determine causality between the variables. This method helps understand how changes in one variable may impact another.
Examples of correlational research questions include studying the relationship between stress and depression, fame and money, or classroom activities and student performance.
3. Causal-Comparative Research:
Causal-comparative research, also known as quasi-experimental research, seeks to determine cause-and-effect relationships between variables.
Researchers analyze how an independent variable influences a dependent variable, but they do not manipulate the independent variable. Instead, they observe and compare different groups to draw conclusions.
Causal-comparative research is useful in situations where it’s not ethical or feasible to conduct true experiments.
Examples of questions for this type of research include analyzing the effect of training programs on employee performance, studying the influence of customer support on client retention, investigating the impact of supply chain efficiency on cost reduction, etc.
4. Experimental Research:
Experimental research is based on testing theories to validate or disprove them. Researchers conduct experiments and manipulate variables to observe their impact on the outcomes.
This type of research is prevalent in natural and social sciences, and it is a powerful method to establish cause-and-effect relationships. By randomly assigning participants to experimental and control groups, researchers can draw more confident conclusions.
Examples of experimental research include studying the effectiveness of a new drug, the impact of teaching methods on student performance, or the outcomes of a marketing campaign.
B. Data collection methodologies
After defining research objectives, the next significant step in primary quantitative research is data collection. This involves using two main methods: sampling and conducting surveys or polls.
Sampling methods:
In quantitative research, there are two primary sampling methods: Probability and Non-probability sampling.
Probability Sampling
In probability sampling, researchers use the concept of probability to create samples from a population. This method ensures that every individual in the target audience has an equal chance of being selected for the sample.
There are four main types of probability sampling:
- Simple random sampling: Here, the elements or participants of a sample are selected randomly, and this technique is used in studies that are conducted over considerably large audiences. It works well for large target populations.
- Stratified random sampling: In this method, the entire population is divided into strata or groups, and the sample members get chosen randomly from these strata only. It is always ensured that different segregated strata do not overlap with each other.
- Cluster sampling: Here, researchers divide the population into clusters, often based on geography or demographics. Then, random clusters are selected for the sample.
- Systematic sampling: In this method, only the starting point of the sample is randomly chosen. All the other participants are chosen using a fixed interval. Researchers calculate this interval by dividing the size of the study population by the target sample size.
Non-probability Sampling
Non-probability sampling is a method where the researcher’s knowledge and experience guide the selection of samples. This approach doesn’t give all members of the target population an equal chance of being included in the sample.
There are five non-probability sampling models:
- Convenience sampling: The elements or participants are chosen on the basis of their nearness to the researcher. The people in close proximity can be studied and analyzed easily and quickly, as there is no other selection criterion involved. Researchers simply choose samples based on what is most convenient for them.
- Consecutive sampling: Similar to convenience sampling, researchers select samples one after another over a significant period. They can opt for a single participant or a group of samples to conduct quantitative research in a consecutive manner for a significant period of time. Once this is over, they can conduct the research from the start.
- Quota sampling: With quota sampling, researchers use their understanding of target traits and personalities to form groups (strata). They then choose samples from each stratum based on their own judgment.
- Snowball sampling: This method is used where the target audiences are difficult to contact and interviewed for data collection. Researchers start with a few participants and then ask them to refer others, creating a snowball effect.
- Judgmental sampling: In judgmental sampling, researchers rely solely on their experience and research skills to handpick samples that they believe will be most relevant to the study.
Read More: Data Collection Methods: Definition, Types & Examples
C. Data analysis techniques
To analyze the quantitative data accurately, you’ll need to use specific statistical methods such as:
- SWOT Analysis: This stands for Strengths, Weaknesses, Opportunities, and Threats analysis. Organizations use SWOT analysis to evaluate their performance internally and externally. It helps develop effective improvement strategies.
- Conjoint Analysis: This market research method uncovers how individuals make complex purchasing decisions. It involves considering trade-offs in their daily activities when choosing from a list of product/service options.
- Cross-tabulation: A preliminary statistical market analysis method that reveals relationships, patterns, and trends within various research study parameters.
- TURF Analysis: Short for Totally Unduplicated Reach and Frequency Analysis, this method helps analyze the reach and frequency of favorable communication sources. It provides insights into the potential of a target market.
- By using these statistical techniques and inferential statistics methods like confidence intervals and margin of error, you can draw meaningful insights from your primary quantitative research that you can use in making informed decisions.
II. Secondary Quantitative Research Methods
- Secondary quantitative research, also known as desk research, is a valuable method that uses existing data, called secondary data.
- Instead of collecting new data, researchers analyze and combine already available information to enhance their research. This approach involves gathering quantitative data from various sources such as the internet, government databases, libraries, and research reports.
- Secondary quantitative research plays a crucial role in validating data collected through primary quantitative research. It helps reinforce or challenge existing findings.
Here are five commonly used secondary quantitative research methods:
A. Data Available on the Internet:
The Internet has become a vast repository of data, making it easier for researchers to access a wealth of information. Online databases, websites, and research repositories provide valuable quantitative data for researchers to analyze and validate their primary research findings.
B. Government and Non-Government Sources:
Government agencies and non-government organizations often conduct extensive research and publish reports. These reports cover a wide range of topics, providing researchers with reliable and comprehensive data for quantitative analysis.
C. Public Libraries:
While less commonly used in the digital age, public libraries still hold valuable research reports, historical data, and publications that can contribute to quantitative research.
D. Educational Institutions:
Educational institutions frequently conduct research on various subjects. Their research reports and publications can serve as valuable sources of information for researchers, validating and supporting primary quantitative research outcomes.
E. Commercial Information Sources:
Commercial sources such as local newspapers, journals, magazines, and media outlets often publish relevant data on economic trends, market research, and demographic analyses. Researchers can access this data to supplement their own findings and draw better conclusions.
Advantages of Quantitative Research Methods
Quantitative research data is often standardized and can be easily used to generalize findings for making crucial business decisions and uncover insights to supplement the qualitative research findings.
Here are some core benefits this research methodology offers.
Direct Result Comparison
As the studies can be replicated for different cultural settings and different times, even with different groups of participants, they tend to be extremely useful. Researchers can compare the results of different studies in a statistical manner and arrive at comprehensive conclusions for a broader understanding.
Replication
Researchers can repeat the study by using standardized data collection protocols over well-structured data sets. They can also apply tangible definitions of abstract concepts to arrive at different conclusions for similar research objectives with minor variations.
Large Samples
As the research data comes from large samples, the researchers can process and analyze the data via highly reliable and consistent analysis procedures. They can arrive at well-defined conclusions that can be used to make the primary research more thorough and reliable.
Hypothesis Testing
This research methodology follows standardized and established hypothesis testing procedures. So, you have to be careful while reporting and analyzing your research data , and the overall quality of results gets improved.
Proven Examples of Quantitative Research Methods
Below, we discuss two excellent examples of quantitative research methods that were used by highly distinguished business and consulting organizations. Both examples show how different types of analysis can be performed with qualitative approaches and how the analysis is done once the data is collected.
1. STEP Project Global Consortium / KPMG 2019 Global Family Business survey
This research utilized quantitative methods to identify ways that kept the family businesses sustainably profitable with time.
The study also identified the ways in which the family business behavior changed with demographic changes and had “why” and “how” questions. Their qualitative research methods allowed the KPMG team to dig deeper into the mindsets and perspectives of the business owners and uncover unexpected research avenues as well.
It was a joint effort in which STEP Project Global Consortium collected 26 cases, and KPMG collected 11 cases.
The research reached the stage of data analysis in 2020, and the analysis process spanned over 4 stages.
The results, which were also the reasons why family businesses tend to lose their strength with time, were found to be:
- Family governance
- Family business legacy
2. EY Seren Teams Research 2020
This is yet another commendable example of qualitative research where the EY Seren Team digs into the unexplored depths of human behavior and how it affected their brand or service expectations.
The research was done across 200+ sources and involved in-depth virtual interviews with people in their homes, exploring their current needs and wishes. It also involved diary studies across the entire UK customer base to analyze human behavior changes and patterns.
The study also included interviews with professionals and design leaders from a wide range of industries to explore how COVID-19 transformed their industries. Finally, quantitative surveys were conducted to gain insights into the EY community after every 15 days.
The insights and results were:
- A culture of fear, daily resilience, and hopes for a better world and a better life – these were the macro trends.
- People felt massive digitization to be a resourceful yet demanding aspect as they have to adapt every day.
- Some people wished to have a new world with lots of possibilities, and some were looking for a new purpose.
Enhance Your Quantitative Research With Cutting-Edge Software
While no single research methodology can produce 100% reliable results, you can always opt for a hybrid research method by opting for the methods that are most relevant to your objective.
This understanding comes gradually as you learn how to implement the correct combination of qualitative and quantitative research methods for your research projects. For the best results, we recommend investing in smart, efficient, and scalable research tools that come with delightful reporting and advanced analytics to make every research initiative a success.
These software tools, such as ProProfs Survey Maker, come with pre-built survey templates and question libraries and allow you to create a high-converting survey in just a few minutes.
So, choose the best research partner, create the right research plan, and gather insights that drive sustainable growth for your business.
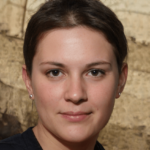
About the author
Emma David is a seasoned market research professional with 8+ years of experience. Having kick-started her journey in research, she has developed rich expertise in employee engagement, survey creation and administration, and data management. Emma believes in the power of data to shape business performance positively. She continues to help brands and businesses make strategic decisions and improve their market standing through her understanding of research methodologies.
Popular Posts in This Category
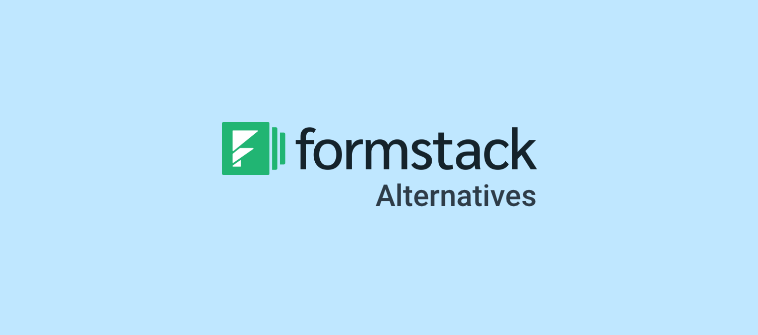
10 Best Formstack Alternatives in 2024 (Pricing + Features)
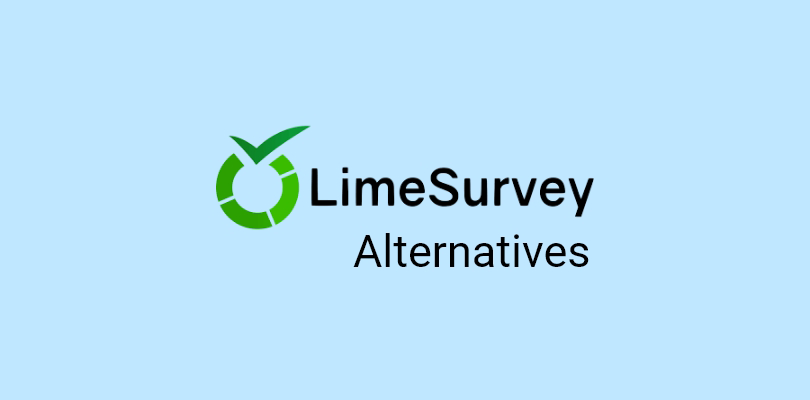
10 Best LimeSurvey Alternatives & Competitors for 2024
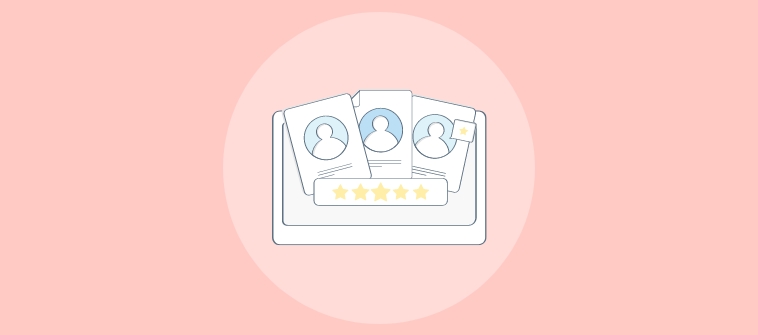
Employee Motivation Survey: 30+ Questions & Ready-to-Use Templates
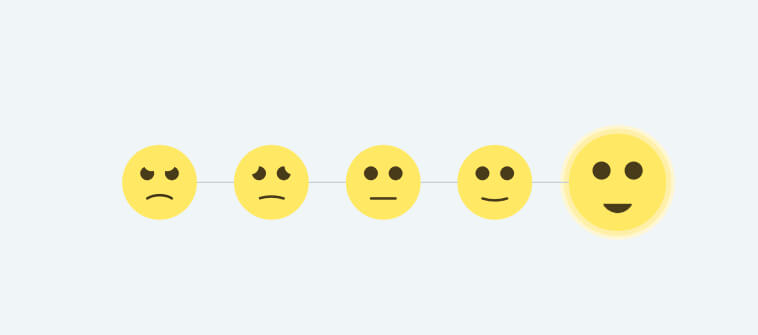
What is Customer Effort Score: An Ultimate Guide for 2024
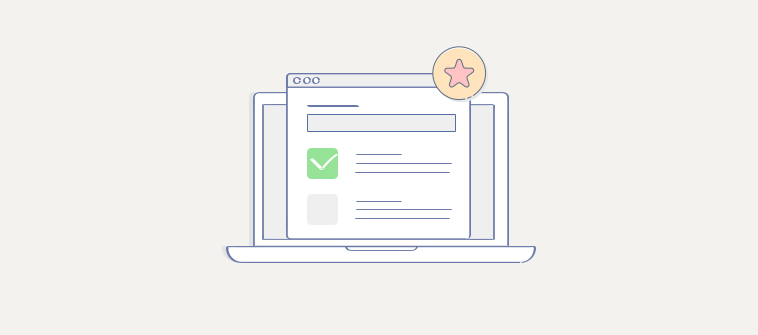
How to Eliminate Survey Bias to Improve Your Survey Responses
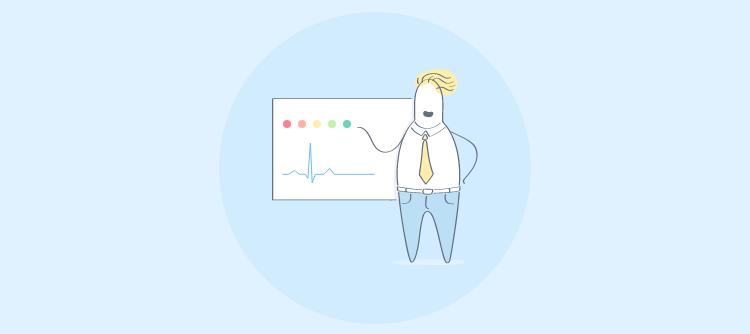
15 Effective Employee Retention Strategies (2024)
Research Methodologies
Quantitative research methodologies.
- Qualitative Research Methodologies
- Systematic Reviews
- Finding Articles by Methodology
- Design Your Research Project
Library Help
What is quantitative research.
Quantitative methodologies use statistics to analyze numerical data gathered by researchers to answer their research questions. Quantitative methods can be used to answer questions such as:
- What are the relationships between two or more variables?
- What factors are at play in an environment that might affect the behavior or development of the organisms in that environment?
Quantitative methods can also be used to test hypotheses by conducting quasi-experimental studies or designing experiments.
Independent and Dependent Variables
In quantitative research, a variable is something (an intervention technique, a pharmaceutical, a temperature, etc.) that changes. There are two kinds of variables: independent variables and dependent variables . In the simplest terms, the independent variable is whatever the researchers are using to attempt to make a change in their dependent variable.
* This is a real, repeatable experiment you can try on your plants.
Correlational
Researchers will compare two sets of numbers to try and identify a relationship (if any) between two things.
- Köse S., & Murat, M. (2021). Examination of the relationship between smartphone addiction and cyberchondria in adolescents. Archives of Psychiatric Nursing, 35(6): 563-570.
- Pilger et al. (2021). Spiritual well-being, religious/spiritual coping and quality of life among the elderly undergoing hemodialysis: a correlational study. Journal of Religion, Spirituality & Aging, 33(1): 2-15.
Descriptive
Researchers will attempt to quantify a variety of factors at play as they study a particular type of phenomenon or action. For example, researchers might use a descriptive methodology to understand the effects of climate change on the life cycle of a plant or animal.
- Lakshmi, E. (2021). Food consumption pattern and body mass index of adolescents: A descriptive study. International Journal of Nutrition, Pharmacology, Neurological Diseases, 11(4), 293–297.
- Lin, J., Singh, S., Sha, L., Tan, W., Lang, D., Gašević, D., & Chen, G. (2022). Is it a good move? Mining effective tutoring strategies from human–human tutorial dialogues. Future Generation Computer Systems, 127, 194–207.
Experimental
To understand the effects of a variable, researchers will design an experiment where they can control as many factors as possible. This can involve creating control and experimental groups. The experimental group will be exposed to the variable to study its effects. The control group provides data about what happens when the variable is absent. For example, in a study about online teaching, the control group might receive traditional face-to-face instruction while the experimental group would receive their instruction virtually.
- Jinzhang Jia, Yinuo Chen, Guangbo Che, Jinchao Zhu, Fengxiao Wang, & Peng Jia. (2021). Experimental study on the explosion characteristics of hydrogen-methane premixed gas in complex pipe networks. Scientific Reports, 11(1), 1–11.
- Sasaki, R. et al. (2021). Effects of cryotherapy applied at different temperatures on inflammatory pain during the acute phase of arthritis in rats. Physical Therapy, 101(2), 1–9.
Quasi-Experimental/Quasi-Comparative
Researchers will attempt to determine what (if any) effect a variable can have. These studies may have multiple independent variables (causes) and multiple dependent variables (effects), but this can complicate researchers' efforts to find out if A can cause B or if X, Y, and Z are also playing a role.
- Jafari, A., Alami, A., Charoghchian, E., Delshad Noghabi, A., & Nejatian, M. (2021). The impact of effective communication skills training on the status of marital burnout among married women. BMC Women’s Health, 21(1), 1-10.
- Phillips, S. W., Kim, D.-Y., Sobol, J. J., & Gayadeen, S. M. (2021). Total recall?: A quasi-experimental study of officer’s recollection in shoot - don’t shoot simulators. Police Practice and Research, 22(3), 1229–1240.
Surveys can be considered a quantitative methodology if the researchers require their respondents to choose from pre-determined responses.
- Harries et al. (2021). Effects of the COVID-19 pandemic on medical students: A multicenter quantitative study. BMC Medical Education, 21(14), 1-8.
- Call : 801.863.8840
- Text : 801.290.8123
- In-Person Help
- Email a Librarian
- Make an Appointment
- << Previous: Home
- Next: Qualitative Research Methodologies >>
- Last Updated: May 2, 2024 4:22 PM
- URL: https://uvu.libguides.com/methods
- USC Libraries
- Research Guides
Organizing Your Social Sciences Research Paper
- Quantitative Methods
- Purpose of Guide
- Design Flaws to Avoid
- Independent and Dependent Variables
- Glossary of Research Terms
- Reading Research Effectively
- Narrowing a Topic Idea
- Broadening a Topic Idea
- Extending the Timeliness of a Topic Idea
- Academic Writing Style
- Applying Critical Thinking
- Choosing a Title
- Making an Outline
- Paragraph Development
- Research Process Video Series
- Executive Summary
- The C.A.R.S. Model
- Background Information
- The Research Problem/Question
- Theoretical Framework
- Citation Tracking
- Content Alert Services
- Evaluating Sources
- Primary Sources
- Secondary Sources
- Tiertiary Sources
- Scholarly vs. Popular Publications
- Qualitative Methods
- Insiderness
- Using Non-Textual Elements
- Limitations of the Study
- Common Grammar Mistakes
- Writing Concisely
- Avoiding Plagiarism
- Footnotes or Endnotes?
- Further Readings
- Generative AI and Writing
- USC Libraries Tutorials and Other Guides
- Bibliography
Quantitative methods emphasize objective measurements and the statistical, mathematical, or numerical analysis of data collected through polls, questionnaires, and surveys, or by manipulating pre-existing statistical data using computational techniques . Quantitative research focuses on gathering numerical data and generalizing it across groups of people or to explain a particular phenomenon.
Babbie, Earl R. The Practice of Social Research . 12th ed. Belmont, CA: Wadsworth Cengage, 2010; Muijs, Daniel. Doing Quantitative Research in Education with SPSS . 2nd edition. London: SAGE Publications, 2010.
Need Help Locating Statistics?
Resources for locating data and statistics can be found here:
Statistics & Data Research Guide
Characteristics of Quantitative Research
Your goal in conducting quantitative research study is to determine the relationship between one thing [an independent variable] and another [a dependent or outcome variable] within a population. Quantitative research designs are either descriptive [subjects usually measured once] or experimental [subjects measured before and after a treatment]. A descriptive study establishes only associations between variables; an experimental study establishes causality.
Quantitative research deals in numbers, logic, and an objective stance. Quantitative research focuses on numeric and unchanging data and detailed, convergent reasoning rather than divergent reasoning [i.e., the generation of a variety of ideas about a research problem in a spontaneous, free-flowing manner].
Its main characteristics are :
- The data is usually gathered using structured research instruments.
- The results are based on larger sample sizes that are representative of the population.
- The research study can usually be replicated or repeated, given its high reliability.
- Researcher has a clearly defined research question to which objective answers are sought.
- All aspects of the study are carefully designed before data is collected.
- Data are in the form of numbers and statistics, often arranged in tables, charts, figures, or other non-textual forms.
- Project can be used to generalize concepts more widely, predict future results, or investigate causal relationships.
- Researcher uses tools, such as questionnaires or computer software, to collect numerical data.
The overarching aim of a quantitative research study is to classify features, count them, and construct statistical models in an attempt to explain what is observed.
Things to keep in mind when reporting the results of a study using quantitative methods :
- Explain the data collected and their statistical treatment as well as all relevant results in relation to the research problem you are investigating. Interpretation of results is not appropriate in this section.
- Report unanticipated events that occurred during your data collection. Explain how the actual analysis differs from the planned analysis. Explain your handling of missing data and why any missing data does not undermine the validity of your analysis.
- Explain the techniques you used to "clean" your data set.
- Choose a minimally sufficient statistical procedure ; provide a rationale for its use and a reference for it. Specify any computer programs used.
- Describe the assumptions for each procedure and the steps you took to ensure that they were not violated.
- When using inferential statistics , provide the descriptive statistics, confidence intervals, and sample sizes for each variable as well as the value of the test statistic, its direction, the degrees of freedom, and the significance level [report the actual p value].
- Avoid inferring causality , particularly in nonrandomized designs or without further experimentation.
- Use tables to provide exact values ; use figures to convey global effects. Keep figures small in size; include graphic representations of confidence intervals whenever possible.
- Always tell the reader what to look for in tables and figures .
NOTE: When using pre-existing statistical data gathered and made available by anyone other than yourself [e.g., government agency], you still must report on the methods that were used to gather the data and describe any missing data that exists and, if there is any, provide a clear explanation why the missing data does not undermine the validity of your final analysis.
Babbie, Earl R. The Practice of Social Research . 12th ed. Belmont, CA: Wadsworth Cengage, 2010; Brians, Craig Leonard et al. Empirical Political Analysis: Quantitative and Qualitative Research Methods . 8th ed. Boston, MA: Longman, 2011; McNabb, David E. Research Methods in Public Administration and Nonprofit Management: Quantitative and Qualitative Approaches . 2nd ed. Armonk, NY: M.E. Sharpe, 2008; Quantitative Research Methods. Writing@CSU. Colorado State University; Singh, Kultar. Quantitative Social Research Methods . Los Angeles, CA: Sage, 2007.
Basic Research Design for Quantitative Studies
Before designing a quantitative research study, you must decide whether it will be descriptive or experimental because this will dictate how you gather, analyze, and interpret the results. A descriptive study is governed by the following rules: subjects are generally measured once; the intention is to only establish associations between variables; and, the study may include a sample population of hundreds or thousands of subjects to ensure that a valid estimate of a generalized relationship between variables has been obtained. An experimental design includes subjects measured before and after a particular treatment, the sample population may be very small and purposefully chosen, and it is intended to establish causality between variables. Introduction The introduction to a quantitative study is usually written in the present tense and from the third person point of view. It covers the following information:
- Identifies the research problem -- as with any academic study, you must state clearly and concisely the research problem being investigated.
- Reviews the literature -- review scholarship on the topic, synthesizing key themes and, if necessary, noting studies that have used similar methods of inquiry and analysis. Note where key gaps exist and how your study helps to fill these gaps or clarifies existing knowledge.
- Describes the theoretical framework -- provide an outline of the theory or hypothesis underpinning your study. If necessary, define unfamiliar or complex terms, concepts, or ideas and provide the appropriate background information to place the research problem in proper context [e.g., historical, cultural, economic, etc.].
Methodology The methods section of a quantitative study should describe how each objective of your study will be achieved. Be sure to provide enough detail to enable the reader can make an informed assessment of the methods being used to obtain results associated with the research problem. The methods section should be presented in the past tense.
- Study population and sampling -- where did the data come from; how robust is it; note where gaps exist or what was excluded. Note the procedures used for their selection;
- Data collection – describe the tools and methods used to collect information and identify the variables being measured; describe the methods used to obtain the data; and, note if the data was pre-existing [i.e., government data] or you gathered it yourself. If you gathered it yourself, describe what type of instrument you used and why. Note that no data set is perfect--describe any limitations in methods of gathering data.
- Data analysis -- describe the procedures for processing and analyzing the data. If appropriate, describe the specific instruments of analysis used to study each research objective, including mathematical techniques and the type of computer software used to manipulate the data.
Results The finding of your study should be written objectively and in a succinct and precise format. In quantitative studies, it is common to use graphs, tables, charts, and other non-textual elements to help the reader understand the data. Make sure that non-textual elements do not stand in isolation from the text but are being used to supplement the overall description of the results and to help clarify key points being made. Further information about how to effectively present data using charts and graphs can be found here .
- Statistical analysis -- how did you analyze the data? What were the key findings from the data? The findings should be present in a logical, sequential order. Describe but do not interpret these trends or negative results; save that for the discussion section. The results should be presented in the past tense.
Discussion Discussions should be analytic, logical, and comprehensive. The discussion should meld together your findings in relation to those identified in the literature review, and placed within the context of the theoretical framework underpinning the study. The discussion should be presented in the present tense.
- Interpretation of results -- reiterate the research problem being investigated and compare and contrast the findings with the research questions underlying the study. Did they affirm predicted outcomes or did the data refute it?
- Description of trends, comparison of groups, or relationships among variables -- describe any trends that emerged from your analysis and explain all unanticipated and statistical insignificant findings.
- Discussion of implications – what is the meaning of your results? Highlight key findings based on the overall results and note findings that you believe are important. How have the results helped fill gaps in understanding the research problem?
- Limitations -- describe any limitations or unavoidable bias in your study and, if necessary, note why these limitations did not inhibit effective interpretation of the results.
Conclusion End your study by to summarizing the topic and provide a final comment and assessment of the study.
- Summary of findings – synthesize the answers to your research questions. Do not report any statistical data here; just provide a narrative summary of the key findings and describe what was learned that you did not know before conducting the study.
- Recommendations – if appropriate to the aim of the assignment, tie key findings with policy recommendations or actions to be taken in practice.
- Future research – note the need for future research linked to your study’s limitations or to any remaining gaps in the literature that were not addressed in your study.
Black, Thomas R. Doing Quantitative Research in the Social Sciences: An Integrated Approach to Research Design, Measurement and Statistics . London: Sage, 1999; Gay,L. R. and Peter Airasain. Educational Research: Competencies for Analysis and Applications . 7th edition. Upper Saddle River, NJ: Merril Prentice Hall, 2003; Hector, Anestine. An Overview of Quantitative Research in Composition and TESOL . Department of English, Indiana University of Pennsylvania; Hopkins, Will G. “Quantitative Research Design.” Sportscience 4, 1 (2000); "A Strategy for Writing Up Research Results. The Structure, Format, Content, and Style of a Journal-Style Scientific Paper." Department of Biology. Bates College; Nenty, H. Johnson. "Writing a Quantitative Research Thesis." International Journal of Educational Science 1 (2009): 19-32; Ouyang, Ronghua (John). Basic Inquiry of Quantitative Research . Kennesaw State University.
Strengths of Using Quantitative Methods
Quantitative researchers try to recognize and isolate specific variables contained within the study framework, seek correlation, relationships and causality, and attempt to control the environment in which the data is collected to avoid the risk of variables, other than the one being studied, accounting for the relationships identified.
Among the specific strengths of using quantitative methods to study social science research problems:
- Allows for a broader study, involving a greater number of subjects, and enhancing the generalization of the results;
- Allows for greater objectivity and accuracy of results. Generally, quantitative methods are designed to provide summaries of data that support generalizations about the phenomenon under study. In order to accomplish this, quantitative research usually involves few variables and many cases, and employs prescribed procedures to ensure validity and reliability;
- Applying well established standards means that the research can be replicated, and then analyzed and compared with similar studies;
- You can summarize vast sources of information and make comparisons across categories and over time; and,
- Personal bias can be avoided by keeping a 'distance' from participating subjects and using accepted computational techniques .
Babbie, Earl R. The Practice of Social Research . 12th ed. Belmont, CA: Wadsworth Cengage, 2010; Brians, Craig Leonard et al. Empirical Political Analysis: Quantitative and Qualitative Research Methods . 8th ed. Boston, MA: Longman, 2011; McNabb, David E. Research Methods in Public Administration and Nonprofit Management: Quantitative and Qualitative Approaches . 2nd ed. Armonk, NY: M.E. Sharpe, 2008; Singh, Kultar. Quantitative Social Research Methods . Los Angeles, CA: Sage, 2007.
Limitations of Using Quantitative Methods
Quantitative methods presume to have an objective approach to studying research problems, where data is controlled and measured, to address the accumulation of facts, and to determine the causes of behavior. As a consequence, the results of quantitative research may be statistically significant but are often humanly insignificant.
Some specific limitations associated with using quantitative methods to study research problems in the social sciences include:
- Quantitative data is more efficient and able to test hypotheses, but may miss contextual detail;
- Uses a static and rigid approach and so employs an inflexible process of discovery;
- The development of standard questions by researchers can lead to "structural bias" and false representation, where the data actually reflects the view of the researcher instead of the participating subject;
- Results provide less detail on behavior, attitudes, and motivation;
- Researcher may collect a much narrower and sometimes superficial dataset;
- Results are limited as they provide numerical descriptions rather than detailed narrative and generally provide less elaborate accounts of human perception;
- The research is often carried out in an unnatural, artificial environment so that a level of control can be applied to the exercise. This level of control might not normally be in place in the real world thus yielding "laboratory results" as opposed to "real world results"; and,
- Preset answers will not necessarily reflect how people really feel about a subject and, in some cases, might just be the closest match to the preconceived hypothesis.
Research Tip
Finding Examples of How to Apply Different Types of Research Methods
SAGE publications is a major publisher of studies about how to design and conduct research in the social and behavioral sciences. Their SAGE Research Methods Online and Cases database includes contents from books, articles, encyclopedias, handbooks, and videos covering social science research design and methods including the complete Little Green Book Series of Quantitative Applications in the Social Sciences and the Little Blue Book Series of Qualitative Research techniques. The database also includes case studies outlining the research methods used in real research projects. This is an excellent source for finding definitions of key terms and descriptions of research design and practice, techniques of data gathering, analysis, and reporting, and information about theories of research [e.g., grounded theory]. The database covers both qualitative and quantitative research methods as well as mixed methods approaches to conducting research.
SAGE Research Methods Online and Cases
- << Previous: Qualitative Methods
- Next: Insiderness >>
- Last Updated: May 15, 2024 9:53 AM
- URL: https://libguides.usc.edu/writingguide
Qualitative vs Quantitative Research Methods & Data Analysis
Saul Mcleod, PhD
Editor-in-Chief for Simply Psychology
BSc (Hons) Psychology, MRes, PhD, University of Manchester
Saul Mcleod, PhD., is a qualified psychology teacher with over 18 years of experience in further and higher education. He has been published in peer-reviewed journals, including the Journal of Clinical Psychology.
Learn about our Editorial Process
Olivia Guy-Evans, MSc
Associate Editor for Simply Psychology
BSc (Hons) Psychology, MSc Psychology of Education
Olivia Guy-Evans is a writer and associate editor for Simply Psychology. She has previously worked in healthcare and educational sectors.
On This Page:
What is the difference between quantitative and qualitative?
The main difference between quantitative and qualitative research is the type of data they collect and analyze.
Quantitative research collects numerical data and analyzes it using statistical methods. The aim is to produce objective, empirical data that can be measured and expressed in numerical terms. Quantitative research is often used to test hypotheses, identify patterns, and make predictions.
Qualitative research , on the other hand, collects non-numerical data such as words, images, and sounds. The focus is on exploring subjective experiences, opinions, and attitudes, often through observation and interviews.
Qualitative research aims to produce rich and detailed descriptions of the phenomenon being studied, and to uncover new insights and meanings.
Quantitative data is information about quantities, and therefore numbers, and qualitative data is descriptive, and regards phenomenon which can be observed but not measured, such as language.
What Is Qualitative Research?
Qualitative research is the process of collecting, analyzing, and interpreting non-numerical data, such as language. Qualitative research can be used to understand how an individual subjectively perceives and gives meaning to their social reality.
Qualitative data is non-numerical data, such as text, video, photographs, or audio recordings. This type of data can be collected using diary accounts or in-depth interviews and analyzed using grounded theory or thematic analysis.
Qualitative research is multimethod in focus, involving an interpretive, naturalistic approach to its subject matter. This means that qualitative researchers study things in their natural settings, attempting to make sense of, or interpret, phenomena in terms of the meanings people bring to them. Denzin and Lincoln (1994, p. 2)
Interest in qualitative data came about as the result of the dissatisfaction of some psychologists (e.g., Carl Rogers) with the scientific study of psychologists such as behaviorists (e.g., Skinner ).
Since psychologists study people, the traditional approach to science is not seen as an appropriate way of carrying out research since it fails to capture the totality of human experience and the essence of being human. Exploring participants’ experiences is known as a phenomenological approach (re: Humanism ).
Qualitative research is primarily concerned with meaning, subjectivity, and lived experience. The goal is to understand the quality and texture of people’s experiences, how they make sense of them, and the implications for their lives.
Qualitative research aims to understand the social reality of individuals, groups, and cultures as nearly as possible as participants feel or live it. Thus, people and groups are studied in their natural setting.
Some examples of qualitative research questions are provided, such as what an experience feels like, how people talk about something, how they make sense of an experience, and how events unfold for people.
Research following a qualitative approach is exploratory and seeks to explain ‘how’ and ‘why’ a particular phenomenon, or behavior, operates as it does in a particular context. It can be used to generate hypotheses and theories from the data.
Qualitative Methods
There are different types of qualitative research methods, including diary accounts, in-depth interviews , documents, focus groups , case study research , and ethnography.
The results of qualitative methods provide a deep understanding of how people perceive their social realities and in consequence, how they act within the social world.
The researcher has several methods for collecting empirical materials, ranging from the interview to direct observation, to the analysis of artifacts, documents, and cultural records, to the use of visual materials or personal experience. Denzin and Lincoln (1994, p. 14)
Here are some examples of qualitative data:
Interview transcripts : Verbatim records of what participants said during an interview or focus group. They allow researchers to identify common themes and patterns, and draw conclusions based on the data. Interview transcripts can also be useful in providing direct quotes and examples to support research findings.
Observations : The researcher typically takes detailed notes on what they observe, including any contextual information, nonverbal cues, or other relevant details. The resulting observational data can be analyzed to gain insights into social phenomena, such as human behavior, social interactions, and cultural practices.
Unstructured interviews : generate qualitative data through the use of open questions. This allows the respondent to talk in some depth, choosing their own words. This helps the researcher develop a real sense of a person’s understanding of a situation.
Diaries or journals : Written accounts of personal experiences or reflections.
Notice that qualitative data could be much more than just words or text. Photographs, videos, sound recordings, and so on, can be considered qualitative data. Visual data can be used to understand behaviors, environments, and social interactions.
Qualitative Data Analysis
Qualitative research is endlessly creative and interpretive. The researcher does not just leave the field with mountains of empirical data and then easily write up his or her findings.
Qualitative interpretations are constructed, and various techniques can be used to make sense of the data, such as content analysis, grounded theory (Glaser & Strauss, 1967), thematic analysis (Braun & Clarke, 2006), or discourse analysis.
For example, thematic analysis is a qualitative approach that involves identifying implicit or explicit ideas within the data. Themes will often emerge once the data has been coded.
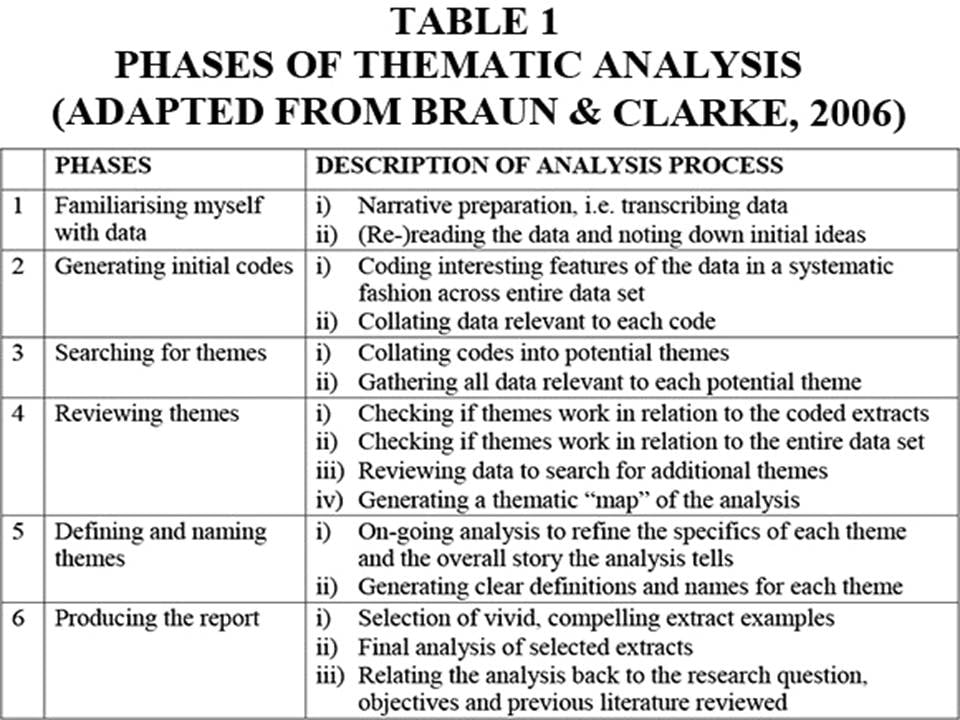
Key Features
- Events can be understood adequately only if they are seen in context. Therefore, a qualitative researcher immerses her/himself in the field, in natural surroundings. The contexts of inquiry are not contrived; they are natural. Nothing is predefined or taken for granted.
- Qualitative researchers want those who are studied to speak for themselves, to provide their perspectives in words and other actions. Therefore, qualitative research is an interactive process in which the persons studied teach the researcher about their lives.
- The qualitative researcher is an integral part of the data; without the active participation of the researcher, no data exists.
- The study’s design evolves during the research and can be adjusted or changed as it progresses. For the qualitative researcher, there is no single reality. It is subjective and exists only in reference to the observer.
- The theory is data-driven and emerges as part of the research process, evolving from the data as they are collected.
Limitations of Qualitative Research
- Because of the time and costs involved, qualitative designs do not generally draw samples from large-scale data sets.
- The problem of adequate validity or reliability is a major criticism. Because of the subjective nature of qualitative data and its origin in single contexts, it is difficult to apply conventional standards of reliability and validity. For example, because of the central role played by the researcher in the generation of data, it is not possible to replicate qualitative studies.
- Also, contexts, situations, events, conditions, and interactions cannot be replicated to any extent, nor can generalizations be made to a wider context than the one studied with confidence.
- The time required for data collection, analysis, and interpretation is lengthy. Analysis of qualitative data is difficult, and expert knowledge of an area is necessary to interpret qualitative data. Great care must be taken when doing so, for example, looking for mental illness symptoms.
Advantages of Qualitative Research
- Because of close researcher involvement, the researcher gains an insider’s view of the field. This allows the researcher to find issues that are often missed (such as subtleties and complexities) by the scientific, more positivistic inquiries.
- Qualitative descriptions can be important in suggesting possible relationships, causes, effects, and dynamic processes.
- Qualitative analysis allows for ambiguities/contradictions in the data, which reflect social reality (Denscombe, 2010).
- Qualitative research uses a descriptive, narrative style; this research might be of particular benefit to the practitioner as she or he could turn to qualitative reports to examine forms of knowledge that might otherwise be unavailable, thereby gaining new insight.
What Is Quantitative Research?
Quantitative research involves the process of objectively collecting and analyzing numerical data to describe, predict, or control variables of interest.
The goals of quantitative research are to test causal relationships between variables , make predictions, and generalize results to wider populations.
Quantitative researchers aim to establish general laws of behavior and phenomenon across different settings/contexts. Research is used to test a theory and ultimately support or reject it.
Quantitative Methods
Experiments typically yield quantitative data, as they are concerned with measuring things. However, other research methods, such as controlled observations and questionnaires , can produce both quantitative information.
For example, a rating scale or closed questions on a questionnaire would generate quantitative data as these produce either numerical data or data that can be put into categories (e.g., “yes,” “no” answers).
Experimental methods limit how research participants react to and express appropriate social behavior.
Findings are, therefore, likely to be context-bound and simply a reflection of the assumptions that the researcher brings to the investigation.
There are numerous examples of quantitative data in psychological research, including mental health. Here are a few examples:
Another example is the Experience in Close Relationships Scale (ECR), a self-report questionnaire widely used to assess adult attachment styles .
The ECR provides quantitative data that can be used to assess attachment styles and predict relationship outcomes.
Neuroimaging data : Neuroimaging techniques, such as MRI and fMRI, provide quantitative data on brain structure and function.
This data can be analyzed to identify brain regions involved in specific mental processes or disorders.
For example, the Beck Depression Inventory (BDI) is a clinician-administered questionnaire widely used to assess the severity of depressive symptoms in individuals.
The BDI consists of 21 questions, each scored on a scale of 0 to 3, with higher scores indicating more severe depressive symptoms.
Quantitative Data Analysis
Statistics help us turn quantitative data into useful information to help with decision-making. We can use statistics to summarize our data, describing patterns, relationships, and connections. Statistics can be descriptive or inferential.
Descriptive statistics help us to summarize our data. In contrast, inferential statistics are used to identify statistically significant differences between groups of data (such as intervention and control groups in a randomized control study).
- Quantitative researchers try to control extraneous variables by conducting their studies in the lab.
- The research aims for objectivity (i.e., without bias) and is separated from the data.
- The design of the study is determined before it begins.
- For the quantitative researcher, the reality is objective, exists separately from the researcher, and can be seen by anyone.
- Research is used to test a theory and ultimately support or reject it.
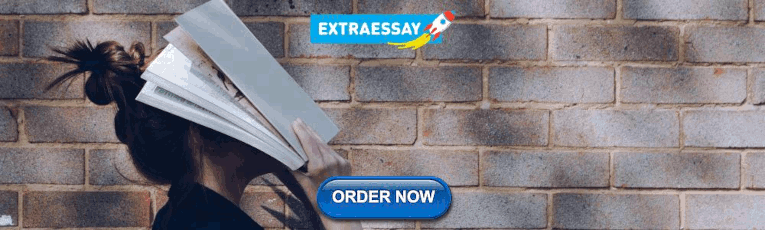
Limitations of Quantitative Research
- Context: Quantitative experiments do not take place in natural settings. In addition, they do not allow participants to explain their choices or the meaning of the questions they may have for those participants (Carr, 1994).
- Researcher expertise: Poor knowledge of the application of statistical analysis may negatively affect analysis and subsequent interpretation (Black, 1999).
- Variability of data quantity: Large sample sizes are needed for more accurate analysis. Small-scale quantitative studies may be less reliable because of the low quantity of data (Denscombe, 2010). This also affects the ability to generalize study findings to wider populations.
- Confirmation bias: The researcher might miss observing phenomena because of focus on theory or hypothesis testing rather than on the theory of hypothesis generation.
Advantages of Quantitative Research
- Scientific objectivity: Quantitative data can be interpreted with statistical analysis, and since statistics are based on the principles of mathematics, the quantitative approach is viewed as scientifically objective and rational (Carr, 1994; Denscombe, 2010).
- Useful for testing and validating already constructed theories.
- Rapid analysis: Sophisticated software removes much of the need for prolonged data analysis, especially with large volumes of data involved (Antonius, 2003).
- Replication: Quantitative data is based on measured values and can be checked by others because numerical data is less open to ambiguities of interpretation.
- Hypotheses can also be tested because of statistical analysis (Antonius, 2003).
Antonius, R. (2003). Interpreting quantitative data with SPSS . Sage.
Black, T. R. (1999). Doing quantitative research in the social sciences: An integrated approach to research design, measurement and statistics . Sage.
Braun, V. & Clarke, V. (2006). Using thematic analysis in psychology . Qualitative Research in Psychology , 3, 77–101.
Carr, L. T. (1994). The strengths and weaknesses of quantitative and qualitative research : what method for nursing? Journal of advanced nursing, 20(4) , 716-721.
Denscombe, M. (2010). The Good Research Guide: for small-scale social research. McGraw Hill.
Denzin, N., & Lincoln. Y. (1994). Handbook of Qualitative Research. Thousand Oaks, CA, US: Sage Publications Inc.
Glaser, B. G., Strauss, A. L., & Strutzel, E. (1968). The discovery of grounded theory; strategies for qualitative research. Nursing research, 17(4) , 364.
Minichiello, V. (1990). In-Depth Interviewing: Researching People. Longman Cheshire.
Punch, K. (1998). Introduction to Social Research: Quantitative and Qualitative Approaches. London: Sage
Further Information
- Designing qualitative research
- Methods of data collection and analysis
- Introduction to quantitative and qualitative research
- Checklists for improving rigour in qualitative research: a case of the tail wagging the dog?
- Qualitative research in health care: Analysing qualitative data
- Qualitative data analysis: the framework approach
- Using the framework method for the analysis of
- Qualitative data in multi-disciplinary health research
- Content Analysis
- Grounded Theory
- Thematic Analysis

Related Articles
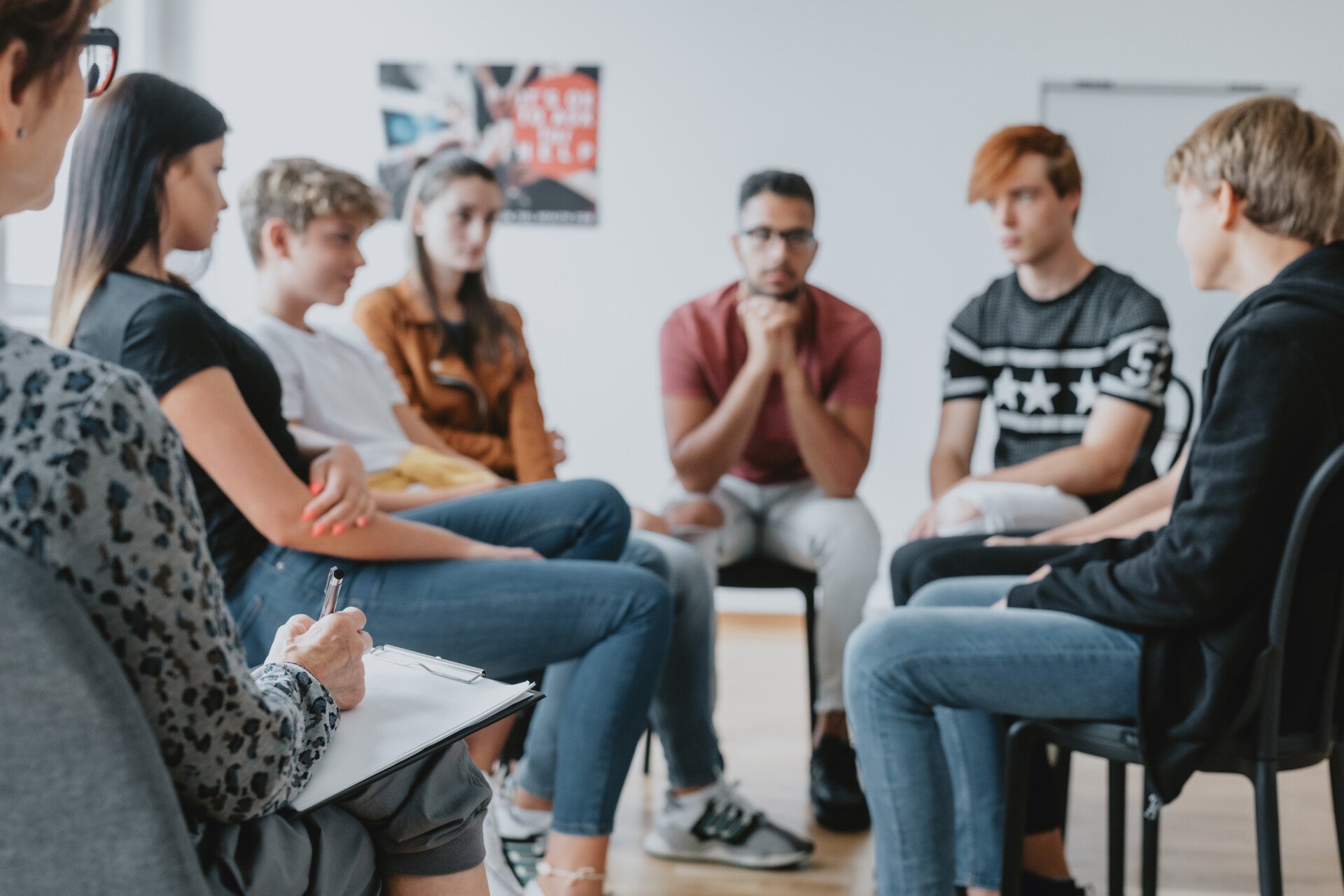
Research Methodology
What Is a Focus Group?
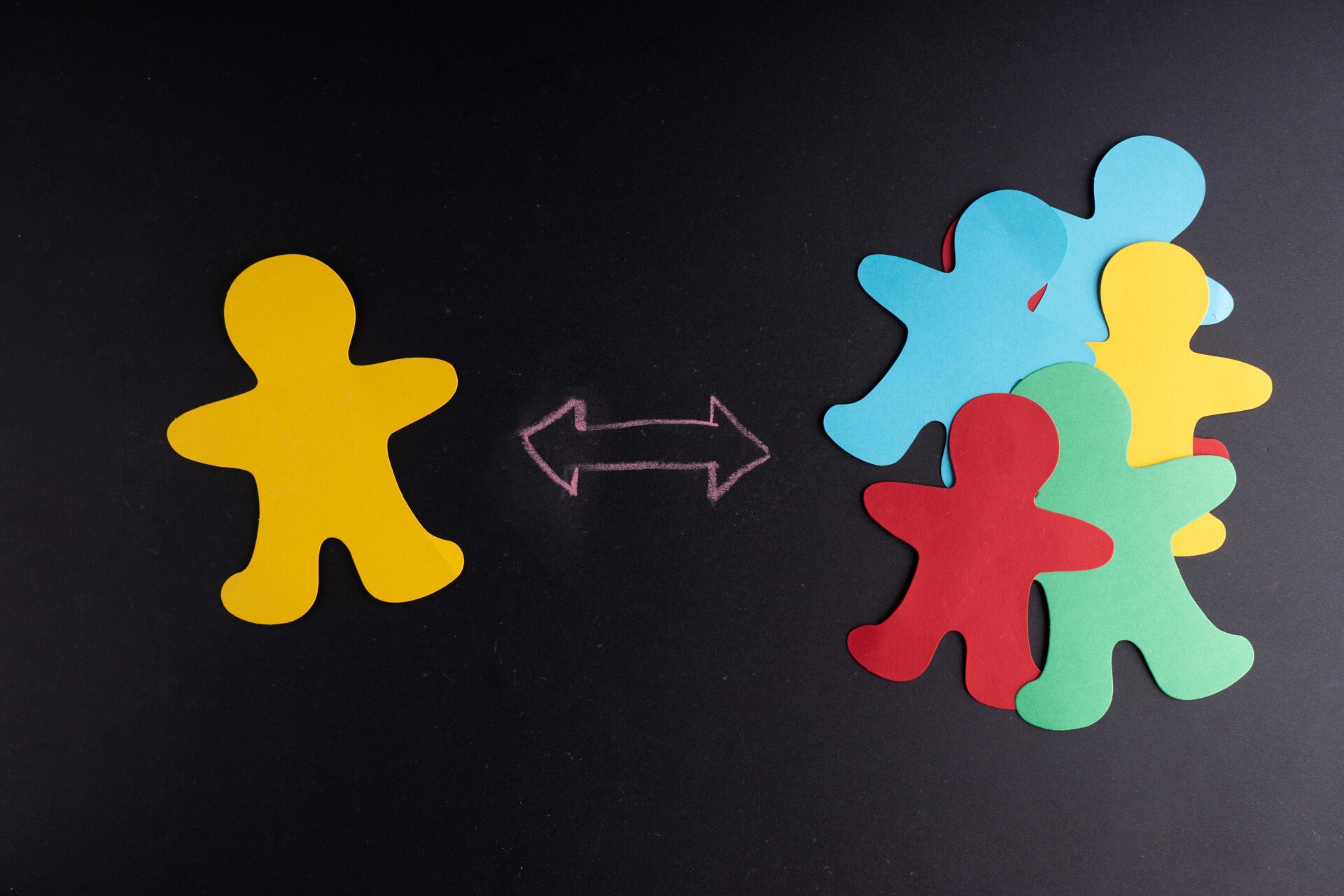
Cross-Cultural Research Methodology In Psychology
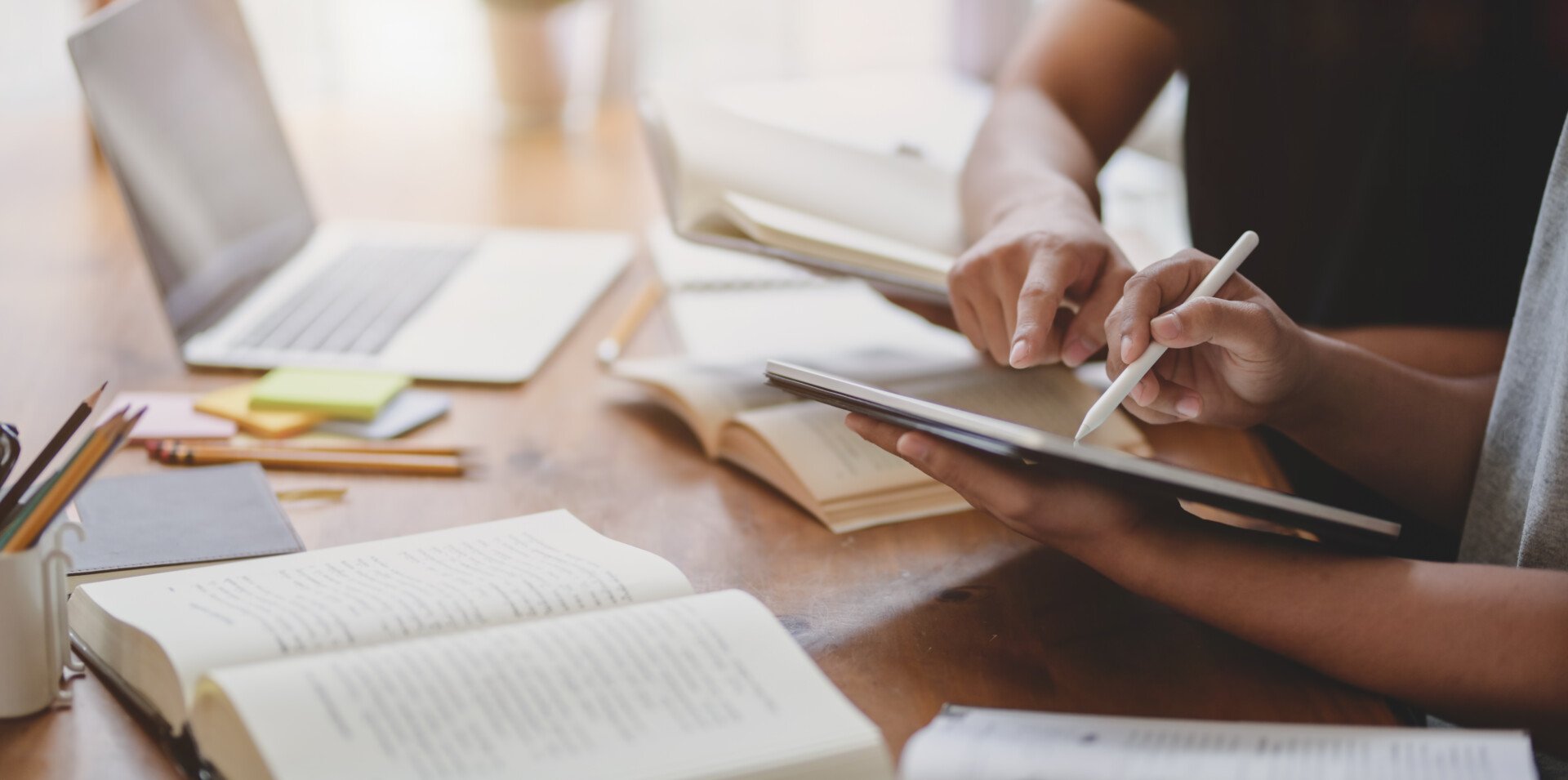
What Is Internal Validity In Research?
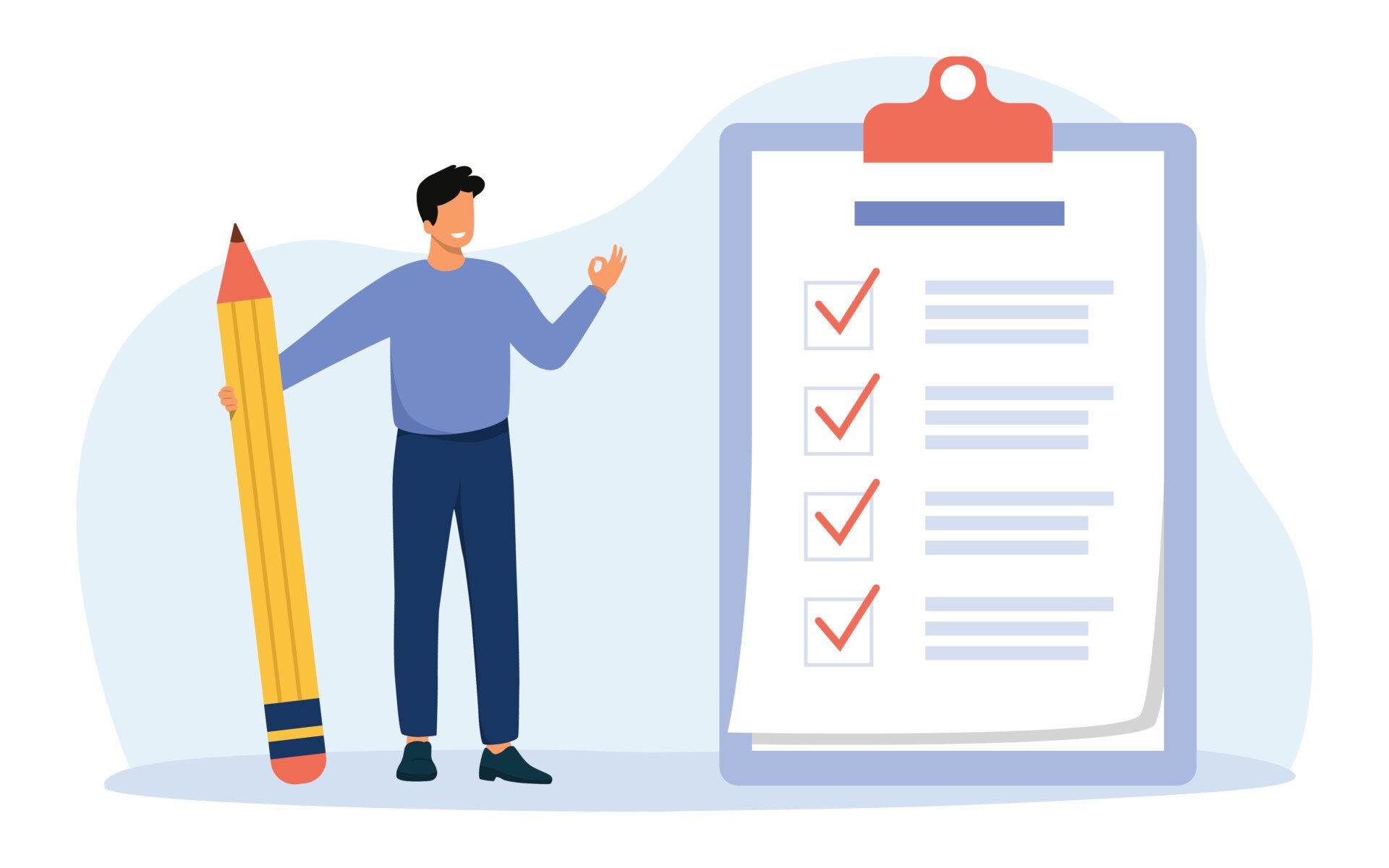
Research Methodology , Statistics
What Is Face Validity In Research? Importance & How To Measure
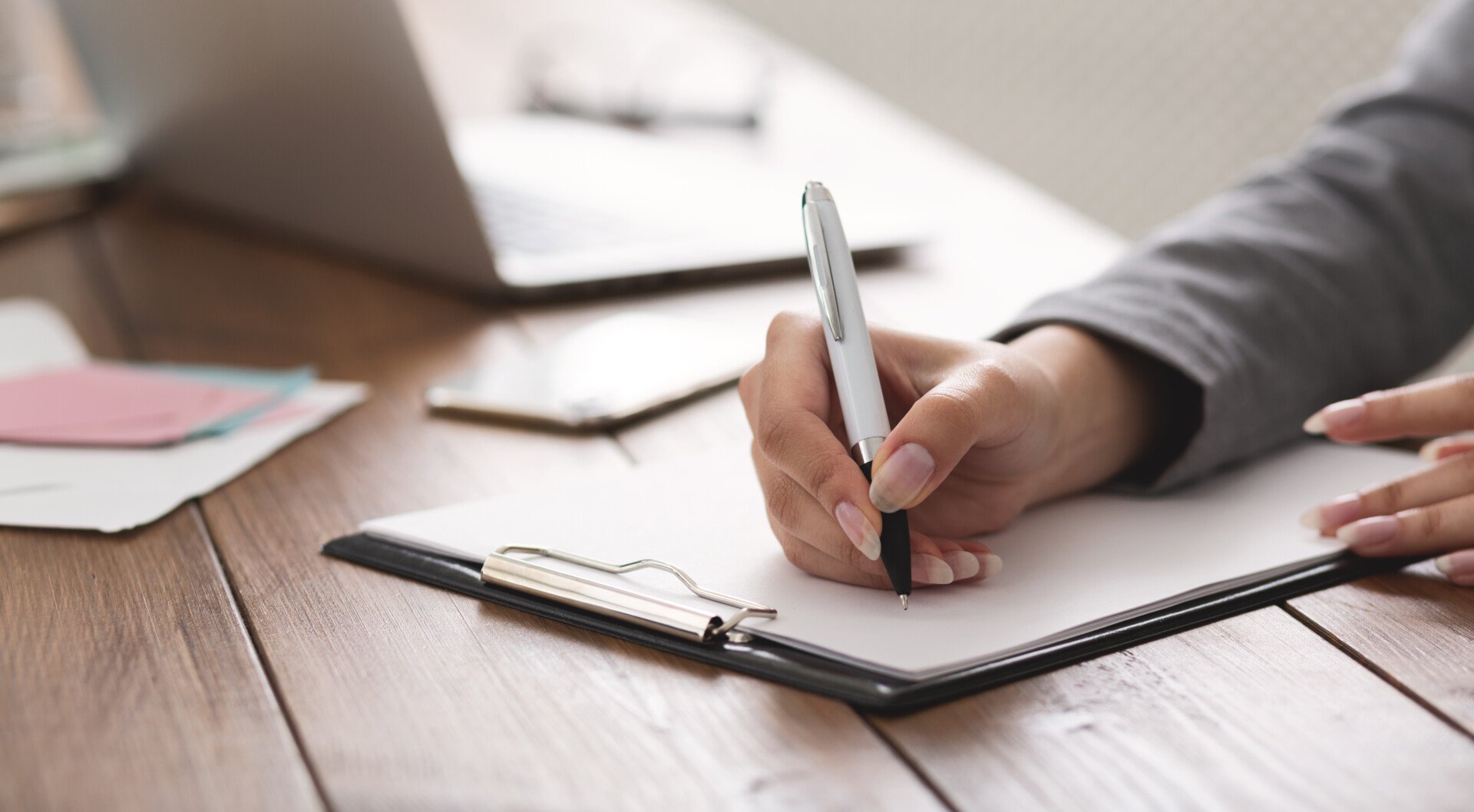
Criterion Validity: Definition & Examples
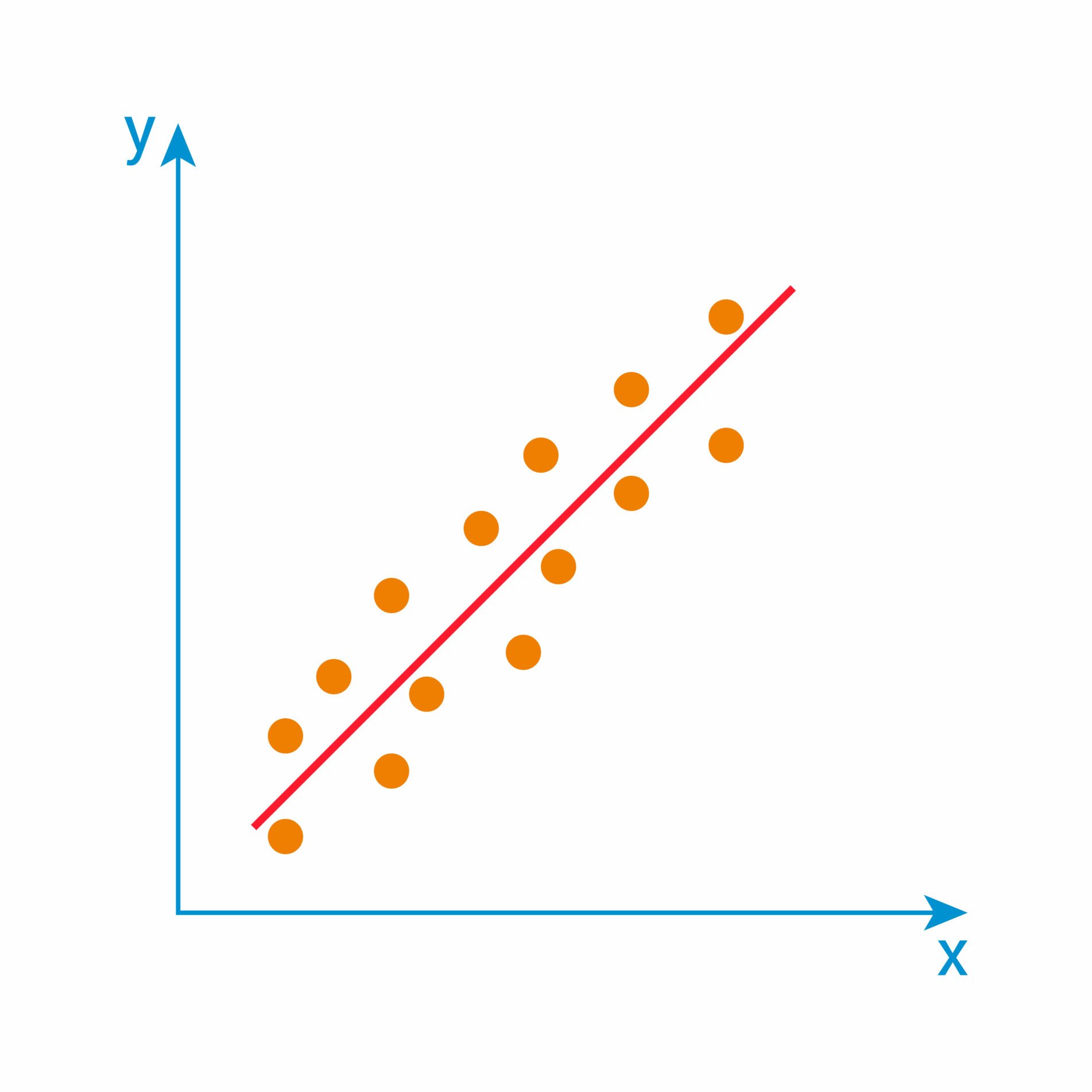
Convergent Validity: Definition and Examples
- Privacy Policy

Home » 500+ Quantitative Research Titles and Topics
500+ Quantitative Research Titles and Topics
Table of Contents

Quantitative research involves collecting and analyzing numerical data to identify patterns, trends, and relationships among variables. This method is widely used in social sciences, psychology , economics , and other fields where researchers aim to understand human behavior and phenomena through statistical analysis. If you are looking for a quantitative research topic, there are numerous areas to explore, from analyzing data on a specific population to studying the effects of a particular intervention or treatment. In this post, we will provide some ideas for quantitative research topics that may inspire you and help you narrow down your interests.
Quantitative Research Titles
Quantitative Research Titles are as follows:
Business and Economics
- “Statistical Analysis of Supply Chain Disruptions on Retail Sales”
- “Quantitative Examination of Consumer Loyalty Programs in the Fast Food Industry”
- “Predicting Stock Market Trends Using Machine Learning Algorithms”
- “Influence of Workplace Environment on Employee Productivity: A Quantitative Study”
- “Impact of Economic Policies on Small Businesses: A Regression Analysis”
- “Customer Satisfaction and Profit Margins: A Quantitative Correlation Study”
- “Analyzing the Role of Marketing in Brand Recognition: A Statistical Overview”
- “Quantitative Effects of Corporate Social Responsibility on Consumer Trust”
- “Price Elasticity of Demand for Luxury Goods: A Case Study”
- “The Relationship Between Fiscal Policy and Inflation Rates: A Time-Series Analysis”
- “Factors Influencing E-commerce Conversion Rates: A Quantitative Exploration”
- “Examining the Correlation Between Interest Rates and Consumer Spending”
- “Standardized Testing and Academic Performance: A Quantitative Evaluation”
- “Teaching Strategies and Student Learning Outcomes in Secondary Schools: A Quantitative Study”
- “The Relationship Between Extracurricular Activities and Academic Success”
- “Influence of Parental Involvement on Children’s Educational Achievements”
- “Digital Literacy in Primary Schools: A Quantitative Assessment”
- “Learning Outcomes in Blended vs. Traditional Classrooms: A Comparative Analysis”
- “Correlation Between Teacher Experience and Student Success Rates”
- “Analyzing the Impact of Classroom Technology on Reading Comprehension”
- “Gender Differences in STEM Fields: A Quantitative Analysis of Enrollment Data”
- “The Relationship Between Homework Load and Academic Burnout”
- “Assessment of Special Education Programs in Public Schools”
- “Role of Peer Tutoring in Improving Academic Performance: A Quantitative Study”
Medicine and Health Sciences
- “The Impact of Sleep Duration on Cardiovascular Health: A Cross-sectional Study”
- “Analyzing the Efficacy of Various Antidepressants: A Meta-Analysis”
- “Patient Satisfaction in Telehealth Services: A Quantitative Assessment”
- “Dietary Habits and Incidence of Heart Disease: A Quantitative Review”
- “Correlations Between Stress Levels and Immune System Functioning”
- “Smoking and Lung Function: A Quantitative Analysis”
- “Influence of Physical Activity on Mental Health in Older Adults”
- “Antibiotic Resistance Patterns in Community Hospitals: A Quantitative Study”
- “The Efficacy of Vaccination Programs in Controlling Disease Spread: A Time-Series Analysis”
- “Role of Social Determinants in Health Outcomes: A Quantitative Exploration”
- “Impact of Hospital Design on Patient Recovery Rates”
- “Quantitative Analysis of Dietary Choices and Obesity Rates in Children”
Social Sciences
- “Examining Social Inequality through Wage Distribution: A Quantitative Study”
- “Impact of Parental Divorce on Child Development: A Longitudinal Study”
- “Social Media and its Effect on Political Polarization: A Quantitative Analysis”
- “The Relationship Between Religion and Social Attitudes: A Statistical Overview”
- “Influence of Socioeconomic Status on Educational Achievement”
- “Quantifying the Effects of Community Programs on Crime Reduction”
- “Public Opinion and Immigration Policies: A Quantitative Exploration”
- “Analyzing the Gender Representation in Political Offices: A Quantitative Study”
- “Impact of Mass Media on Public Opinion: A Regression Analysis”
- “Influence of Urban Design on Social Interactions in Communities”
- “The Role of Social Support in Mental Health Outcomes: A Quantitative Analysis”
- “Examining the Relationship Between Substance Abuse and Employment Status”
Engineering and Technology
- “Performance Evaluation of Different Machine Learning Algorithms in Autonomous Vehicles”
- “Material Science: A Quantitative Analysis of Stress-Strain Properties in Various Alloys”
- “Impacts of Data Center Cooling Solutions on Energy Consumption”
- “Analyzing the Reliability of Renewable Energy Sources in Grid Management”
- “Optimization of 5G Network Performance: A Quantitative Assessment”
- “Quantifying the Effects of Aerodynamics on Fuel Efficiency in Commercial Airplanes”
- “The Relationship Between Software Complexity and Bug Frequency”
- “Machine Learning in Predictive Maintenance: A Quantitative Analysis”
- “Wearable Technologies and their Impact on Healthcare Monitoring”
- “Quantitative Assessment of Cybersecurity Measures in Financial Institutions”
- “Analysis of Noise Pollution from Urban Transportation Systems”
- “The Influence of Architectural Design on Energy Efficiency in Buildings”
Quantitative Research Topics
Quantitative Research Topics are as follows:
- The effects of social media on self-esteem among teenagers.
- A comparative study of academic achievement among students of single-sex and co-educational schools.
- The impact of gender on leadership styles in the workplace.
- The correlation between parental involvement and academic performance of students.
- The effect of mindfulness meditation on stress levels in college students.
- The relationship between employee motivation and job satisfaction.
- The effectiveness of online learning compared to traditional classroom learning.
- The correlation between sleep duration and academic performance among college students.
- The impact of exercise on mental health among adults.
- The relationship between social support and psychological well-being among cancer patients.
- The effect of caffeine consumption on sleep quality.
- A comparative study of the effectiveness of cognitive-behavioral therapy and pharmacotherapy in treating depression.
- The relationship between physical attractiveness and job opportunities.
- The correlation between smartphone addiction and academic performance among high school students.
- The impact of music on memory recall among adults.
- The effectiveness of parental control software in limiting children’s online activity.
- The relationship between social media use and body image dissatisfaction among young adults.
- The correlation between academic achievement and parental involvement among minority students.
- The impact of early childhood education on academic performance in later years.
- The effectiveness of employee training and development programs in improving organizational performance.
- The relationship between socioeconomic status and access to healthcare services.
- The correlation between social support and academic achievement among college students.
- The impact of technology on communication skills among children.
- The effectiveness of mindfulness-based stress reduction programs in reducing symptoms of anxiety and depression.
- The relationship between employee turnover and organizational culture.
- The correlation between job satisfaction and employee engagement.
- The impact of video game violence on aggressive behavior among children.
- The effectiveness of nutritional education in promoting healthy eating habits among adolescents.
- The relationship between bullying and academic performance among middle school students.
- The correlation between teacher expectations and student achievement.
- The impact of gender stereotypes on career choices among high school students.
- The effectiveness of anger management programs in reducing violent behavior.
- The relationship between social support and recovery from substance abuse.
- The correlation between parent-child communication and adolescent drug use.
- The impact of technology on family relationships.
- The effectiveness of smoking cessation programs in promoting long-term abstinence.
- The relationship between personality traits and academic achievement.
- The correlation between stress and job performance among healthcare professionals.
- The impact of online privacy concerns on social media use.
- The effectiveness of cognitive-behavioral therapy in treating anxiety disorders.
- The relationship between teacher feedback and student motivation.
- The correlation between physical activity and academic performance among elementary school students.
- The impact of parental divorce on academic achievement among children.
- The effectiveness of diversity training in improving workplace relationships.
- The relationship between childhood trauma and adult mental health.
- The correlation between parental involvement and substance abuse among adolescents.
- The impact of social media use on romantic relationships among young adults.
- The effectiveness of assertiveness training in improving communication skills.
- The relationship between parental expectations and academic achievement among high school students.
- The correlation between sleep quality and mood among adults.
- The impact of video game addiction on academic performance among college students.
- The effectiveness of group therapy in treating eating disorders.
- The relationship between job stress and job performance among teachers.
- The correlation between mindfulness and emotional regulation.
- The impact of social media use on self-esteem among college students.
- The effectiveness of parent-teacher communication in promoting academic achievement among elementary school students.
- The impact of renewable energy policies on carbon emissions
- The relationship between employee motivation and job performance
- The effectiveness of psychotherapy in treating eating disorders
- The correlation between physical activity and cognitive function in older adults
- The effect of childhood poverty on adult health outcomes
- The impact of urbanization on biodiversity conservation
- The relationship between work-life balance and employee job satisfaction
- The effectiveness of eye movement desensitization and reprocessing (EMDR) in treating trauma
- The correlation between parenting styles and child behavior
- The effect of social media on political polarization
- The impact of foreign aid on economic development
- The relationship between workplace diversity and organizational performance
- The effectiveness of dialectical behavior therapy in treating borderline personality disorder
- The correlation between childhood abuse and adult mental health outcomes
- The effect of sleep deprivation on cognitive function
- The impact of trade policies on international trade and economic growth
- The relationship between employee engagement and organizational commitment
- The effectiveness of cognitive therapy in treating postpartum depression
- The correlation between family meals and child obesity rates
- The effect of parental involvement in sports on child athletic performance
- The impact of social entrepreneurship on sustainable development
- The relationship between emotional labor and job burnout
- The effectiveness of art therapy in treating dementia
- The correlation between social media use and academic procrastination
- The effect of poverty on childhood educational attainment
- The impact of urban green spaces on mental health
- The relationship between job insecurity and employee well-being
- The effectiveness of virtual reality exposure therapy in treating anxiety disorders
- The correlation between childhood trauma and substance abuse
- The effect of screen time on children’s social skills
- The impact of trade unions on employee job satisfaction
- The relationship between cultural intelligence and cross-cultural communication
- The effectiveness of acceptance and commitment therapy in treating chronic pain
- The correlation between childhood obesity and adult health outcomes
- The effect of gender diversity on corporate performance
- The impact of environmental regulations on industry competitiveness.
- The impact of renewable energy policies on greenhouse gas emissions
- The relationship between workplace diversity and team performance
- The effectiveness of group therapy in treating substance abuse
- The correlation between parental involvement and social skills in early childhood
- The effect of technology use on sleep patterns
- The impact of government regulations on small business growth
- The relationship between job satisfaction and employee turnover
- The effectiveness of virtual reality therapy in treating anxiety disorders
- The correlation between parental involvement and academic motivation in adolescents
- The effect of social media on political engagement
- The impact of urbanization on mental health
- The relationship between corporate social responsibility and consumer trust
- The correlation between early childhood education and social-emotional development
- The effect of screen time on cognitive development in young children
- The impact of trade policies on global economic growth
- The relationship between workplace diversity and innovation
- The effectiveness of family therapy in treating eating disorders
- The correlation between parental involvement and college persistence
- The effect of social media on body image and self-esteem
- The impact of environmental regulations on business competitiveness
- The relationship between job autonomy and job satisfaction
- The effectiveness of virtual reality therapy in treating phobias
- The correlation between parental involvement and academic achievement in college
- The effect of social media on sleep quality
- The impact of immigration policies on social integration
- The relationship between workplace diversity and employee well-being
- The effectiveness of psychodynamic therapy in treating personality disorders
- The correlation between early childhood education and executive function skills
- The effect of parental involvement on STEM education outcomes
- The impact of trade policies on domestic employment rates
- The relationship between job insecurity and mental health
- The effectiveness of exposure therapy in treating PTSD
- The correlation between parental involvement and social mobility
- The effect of social media on intergroup relations
- The impact of urbanization on air pollution and respiratory health.
- The relationship between emotional intelligence and leadership effectiveness
- The effectiveness of cognitive-behavioral therapy in treating depression
- The correlation between early childhood education and language development
- The effect of parental involvement on academic achievement in STEM fields
- The impact of trade policies on income inequality
- The relationship between workplace diversity and customer satisfaction
- The effectiveness of mindfulness-based therapy in treating anxiety disorders
- The correlation between parental involvement and civic engagement in adolescents
- The effect of social media on mental health among teenagers
- The impact of public transportation policies on traffic congestion
- The relationship between job stress and job performance
- The effectiveness of group therapy in treating depression
- The correlation between early childhood education and cognitive development
- The effect of parental involvement on academic motivation in college
- The impact of environmental regulations on energy consumption
- The relationship between workplace diversity and employee engagement
- The effectiveness of art therapy in treating PTSD
- The correlation between parental involvement and academic success in vocational education
- The effect of social media on academic achievement in college
- The impact of tax policies on economic growth
- The relationship between job flexibility and work-life balance
- The effectiveness of acceptance and commitment therapy in treating anxiety disorders
- The correlation between early childhood education and social competence
- The effect of parental involvement on career readiness in high school
- The impact of immigration policies on crime rates
- The relationship between workplace diversity and employee retention
- The effectiveness of play therapy in treating trauma
- The correlation between parental involvement and academic success in online learning
- The effect of social media on body dissatisfaction among women
- The impact of urbanization on public health infrastructure
- The relationship between job satisfaction and job performance
- The effectiveness of eye movement desensitization and reprocessing therapy in treating PTSD
- The correlation between early childhood education and social skills in adolescence
- The effect of parental involvement on academic achievement in the arts
- The impact of trade policies on foreign investment
- The relationship between workplace diversity and decision-making
- The effectiveness of exposure and response prevention therapy in treating OCD
- The correlation between parental involvement and academic success in special education
- The impact of zoning laws on affordable housing
- The relationship between job design and employee motivation
- The effectiveness of cognitive rehabilitation therapy in treating traumatic brain injury
- The correlation between early childhood education and social-emotional learning
- The effect of parental involvement on academic achievement in foreign language learning
- The impact of trade policies on the environment
- The relationship between workplace diversity and creativity
- The effectiveness of emotion-focused therapy in treating relationship problems
- The correlation between parental involvement and academic success in music education
- The effect of social media on interpersonal communication skills
- The impact of public health campaigns on health behaviors
- The relationship between job resources and job stress
- The effectiveness of equine therapy in treating substance abuse
- The correlation between early childhood education and self-regulation
- The effect of parental involvement on academic achievement in physical education
- The impact of immigration policies on cultural assimilation
- The relationship between workplace diversity and conflict resolution
- The effectiveness of schema therapy in treating personality disorders
- The correlation between parental involvement and academic success in career and technical education
- The effect of social media on trust in government institutions
- The impact of urbanization on public transportation systems
- The relationship between job demands and job stress
- The correlation between early childhood education and executive functioning
- The effect of parental involvement on academic achievement in computer science
- The effectiveness of cognitive processing therapy in treating PTSD
- The correlation between parental involvement and academic success in homeschooling
- The effect of social media on cyberbullying behavior
- The impact of urbanization on air quality
- The effectiveness of dance therapy in treating anxiety disorders
- The correlation between early childhood education and math achievement
- The effect of parental involvement on academic achievement in health education
- The impact of global warming on agriculture
- The effectiveness of narrative therapy in treating depression
- The correlation between parental involvement and academic success in character education
- The effect of social media on political participation
- The impact of technology on job displacement
- The relationship between job resources and job satisfaction
- The effectiveness of art therapy in treating addiction
- The correlation between early childhood education and reading comprehension
- The effect of parental involvement on academic achievement in environmental education
- The impact of income inequality on social mobility
- The relationship between workplace diversity and organizational culture
- The effectiveness of solution-focused brief therapy in treating anxiety disorders
- The correlation between parental involvement and academic success in physical therapy education
- The effect of social media on misinformation
- The impact of green energy policies on economic growth
- The relationship between job demands and employee well-being
- The correlation between early childhood education and science achievement
- The effect of parental involvement on academic achievement in religious education
- The impact of gender diversity on corporate governance
- The relationship between workplace diversity and ethical decision-making
- The correlation between parental involvement and academic success in dental hygiene education
- The effect of social media on self-esteem among adolescents
- The impact of renewable energy policies on energy security
- The effect of parental involvement on academic achievement in social studies
- The impact of trade policies on job growth
- The relationship between workplace diversity and leadership styles
- The correlation between parental involvement and academic success in online vocational training
- The effect of social media on self-esteem among men
- The impact of urbanization on air pollution levels
- The effectiveness of music therapy in treating depression
- The correlation between early childhood education and math skills
- The effect of parental involvement on academic achievement in language arts
- The impact of immigration policies on labor market outcomes
- The effectiveness of hypnotherapy in treating phobias
- The effect of social media on political engagement among young adults
- The impact of urbanization on access to green spaces
- The relationship between job crafting and job satisfaction
- The effectiveness of exposure therapy in treating specific phobias
- The correlation between early childhood education and spatial reasoning
- The effect of parental involvement on academic achievement in business education
- The impact of trade policies on economic inequality
- The effectiveness of narrative therapy in treating PTSD
- The correlation between parental involvement and academic success in nursing education
- The effect of social media on sleep quality among adolescents
- The impact of urbanization on crime rates
- The relationship between job insecurity and turnover intentions
- The effectiveness of pet therapy in treating anxiety disorders
- The correlation between early childhood education and STEM skills
- The effect of parental involvement on academic achievement in culinary education
- The impact of immigration policies on housing affordability
- The relationship between workplace diversity and employee satisfaction
- The effectiveness of mindfulness-based stress reduction in treating chronic pain
- The correlation between parental involvement and academic success in art education
- The effect of social media on academic procrastination among college students
- The impact of urbanization on public safety services.
About the author
Muhammad Hassan
Researcher, Academic Writer, Web developer
You may also like

200+ Funny Research Topics

500+ Sports Research Topics

300+ American History Research Paper Topics

500+ Cyber Security Research Topics

500+ Environmental Research Topics

500+ Economics Research Topics
Learn / Guides / Quantitative data analysis guide
Back to guides
The ultimate guide to quantitative data analysis
Numbers help us make sense of the world. We collect quantitative data on our speed and distance as we drive, the number of hours we spend on our cell phones, and how much we save at the grocery store.
Our businesses run on numbers, too. We spend hours poring over key performance indicators (KPIs) like lead-to-client conversions, net profit margins, and bounce and churn rates.
But all of this quantitative data can feel overwhelming and confusing. Lists and spreadsheets of numbers don’t tell you much on their own—you have to conduct quantitative data analysis to understand them and make informed decisions.
Last updated
Reading time.
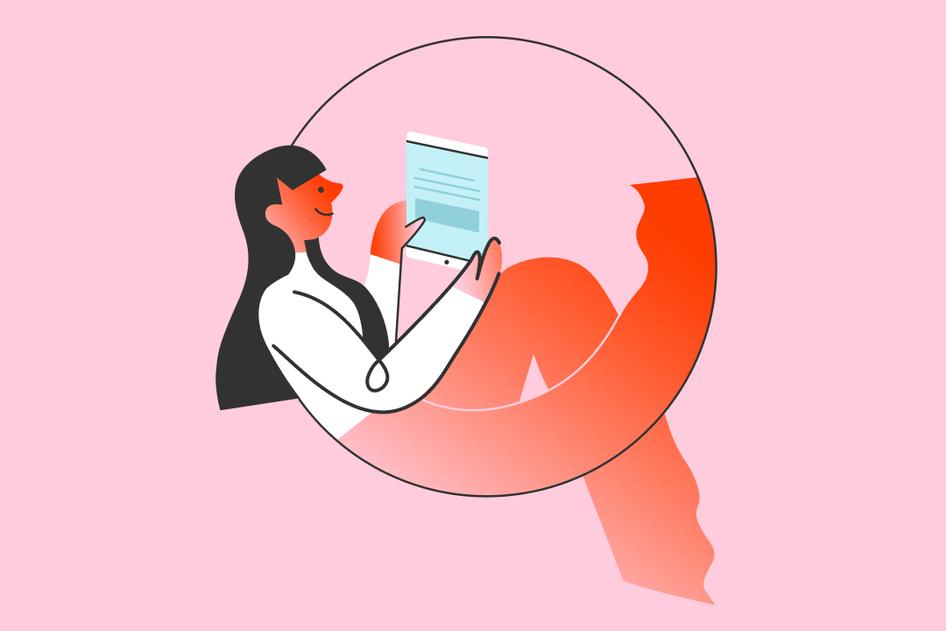
This guide explains what quantitative data analysis is and why it’s important, and gives you a four-step process to conduct a quantitative data analysis, so you know exactly what’s happening in your business and what your users need .
Collect quantitative customer data with Hotjar
Use Hotjar’s tools to gather the customer insights you need to make quantitative data analysis a breeze.
What is quantitative data analysis?
Quantitative data analysis is the process of analyzing and interpreting numerical data. It helps you make sense of information by identifying patterns, trends, and relationships between variables through mathematical calculations and statistical tests.
With quantitative data analysis, you turn spreadsheets of individual data points into meaningful insights to drive informed decisions. Columns of numbers from an experiment or survey transform into useful insights—like which marketing campaign asset your average customer prefers or which website factors are most closely connected to your bounce rate.
Without analytics, data is just noise. Analyzing data helps you make decisions which are informed and free from bias.
What quantitative data analysis is not
But as powerful as quantitative data analysis is, it’s not without its limitations. It only gives you the what, not the why . For example, it can tell you how many website visitors or conversions you have on an average day, but it can’t tell you why users visited your site or made a purchase.
For the why behind user behavior, you need qualitative data analysis , a process for making sense of qualitative research like open-ended survey responses, interview clips, or behavioral observations. By analyzing non-numerical data, you gain useful contextual insights to shape your strategy, product, and messaging.
Quantitative data analysis vs. qualitative data analysis
Let’s take an even deeper dive into the differences between quantitative data analysis and qualitative data analysis to explore what they do and when you need them.
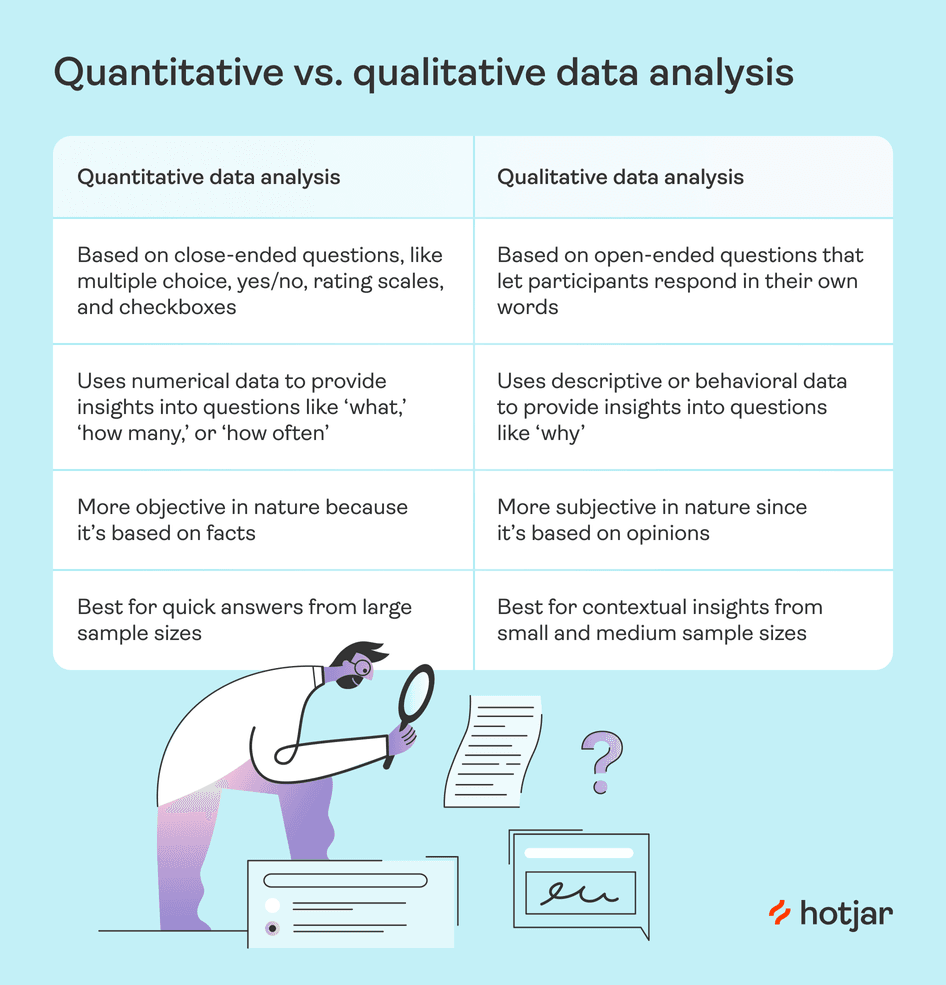
The bottom line: quantitative data analysis and qualitative data analysis are complementary processes. They work hand-in-hand to tell you what’s happening in your business and why.
💡 Pro tip: easily toggle between quantitative and qualitative data analysis with Hotjar Funnels .
The Funnels tool helps you visualize quantitative metrics like drop-off and conversion rates in your sales or conversion funnel to understand when and where users leave your website. You can break down your data even further to compare conversion performance by user segment.
Spot a potential issue? A single click takes you to relevant session recordings , where you see user behaviors like mouse movements, scrolls, and clicks. With this qualitative data to provide context, you'll better understand what you need to optimize to streamline the user experience (UX) and increase conversions .
Hotjar Funnels lets you quickly explore the story behind the quantitative data
4 benefits of quantitative data analysis
There’s a reason product, web design, and marketing teams take time to analyze metrics: the process pays off big time.
Four major benefits of quantitative data analysis include:
1. Make confident decisions
With quantitative data analysis, you know you’ve got data-driven insights to back up your decisions . For example, if you launch a concept testing survey to gauge user reactions to a new logo design, and 92% of users rate it ‘very good’—you'll feel certain when you give the designer the green light.
Since you’re relying less on intuition and more on facts, you reduce the risks of making the wrong decision. (You’ll also find it way easier to get buy-in from team members and stakeholders for your next proposed project. 🙌)
2. Reduce costs
By crunching the numbers, you can spot opportunities to reduce spend . For example, if an ad campaign has lower-than-average click-through rates , you might decide to cut your losses and invest your budget elsewhere.
Or, by analyzing ecommerce metrics , like website traffic by source, you may find you’re getting very little return on investment from a certain social media channel—and scale back spending in that area.
3. Personalize the user experience
Quantitative data analysis helps you map the customer journey , so you get a better sense of customers’ demographics, what page elements they interact with on your site, and where they drop off or convert .
These insights let you better personalize your website, product, or communication, so you can segment ads, emails, and website content for specific user personas or target groups.
4. Improve user satisfaction and delight
Quantitative data analysis lets you see where your website or product is doing well—and where it falls short for your users . For example, you might see stellar results from KPIs like time on page, but conversion rates for that page are low.
These quantitative insights encourage you to dive deeper into qualitative data to see why that’s happening—looking for moments of confusion or frustration on session recordings, for example—so you can make adjustments and optimize your conversions by improving customer satisfaction and delight.
💡Pro tip: use Net Promoter Score® (NPS) surveys to capture quantifiable customer satisfaction data that’s easy for you to analyze and interpret.
With an NPS tool like Hotjar, you can create an on-page survey to ask users how likely they are to recommend you to others on a scale from 0 to 10. (And for added context, you can ask follow-up questions about why customers selected the rating they did—rich qualitative data is always a bonus!)
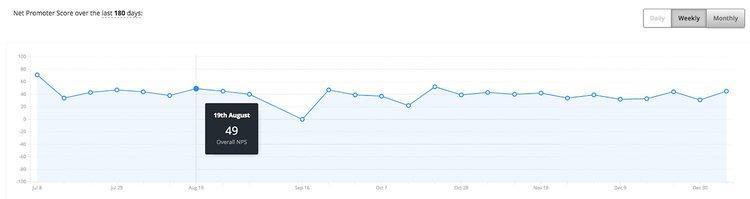
Hotjar graphs your quantitative NPS data to show changes over time
4 steps to effective quantitative data analysis
Quantitative data analysis sounds way more intimidating than it actually is. Here’s how to make sense of your company’s numbers in just four steps:
1. Collect data
Before you can actually start the analysis process, you need data to analyze. This involves conducting quantitative research and collecting numerical data from various sources, including:
Interviews or focus groups
Website analytics
Observations, from tools like heatmaps or session recordings
Questionnaires, like surveys or on-page feedback widgets
Just ensure the questions you ask in your surveys are close-ended questions—providing respondents with select choices to choose from instead of open-ended questions that allow for free responses.
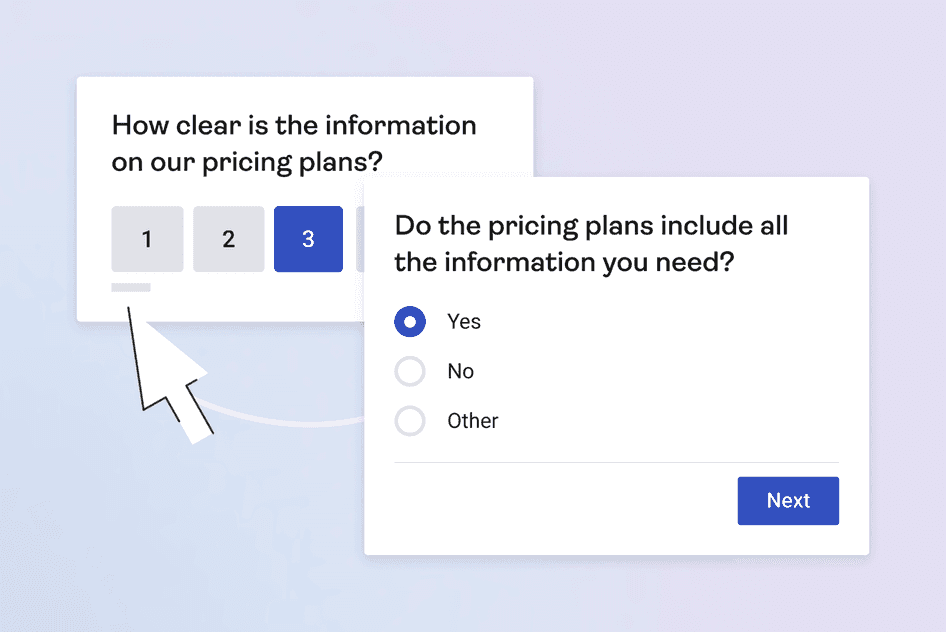
Hotjar’s pricing plans survey template provides close-ended questions
2. Clean data
Once you’ve collected your data, it’s time to clean it up. Look through your results to find errors, duplicates, and omissions. Keep an eye out for outliers, too. Outliers are data points that differ significantly from the rest of the set—and they can skew your results if you don’t remove them.
By taking the time to clean your data set, you ensure your data is accurate, consistent, and relevant before it’s time to analyze.
3. Analyze and interpret data
At this point, your data’s all cleaned up and ready for the main event. This step involves crunching the numbers to find patterns and trends via mathematical and statistical methods.
Two main branches of quantitative data analysis exist:
Descriptive analysis : methods to summarize or describe attributes of your data set. For example, you may calculate key stats like distribution and frequency, or mean, median, and mode.
Inferential analysis : methods that let you draw conclusions from statistics—like analyzing the relationship between variables or making predictions. These methods include t-tests, cross-tabulation, and factor analysis. (For more detailed explanations and how-tos, head to our guide on quantitative data analysis methods.)
Then, interpret your data to determine the best course of action. What does the data suggest you do ? For example, if your analysis shows a strong correlation between email open rate and time sent, you may explore optimal send times for each user segment.
4. Visualize and share data
Once you’ve analyzed and interpreted your data, create easy-to-read, engaging data visualizations—like charts, graphs, and tables—to present your results to team members and stakeholders. Data visualizations highlight similarities and differences between data sets and show the relationships between variables.
Software can do this part for you. For example, the Hotjar Dashboard shows all of your key metrics in one place—and automatically creates bar graphs to show how your top pages’ performance compares. And with just one click, you can navigate to the Trends tool to analyze product metrics for different segments on a single chart.
Hotjar Trends lets you compare metrics across segments
Discover rich user insights with quantitative data analysis
Conducting quantitative data analysis takes a little bit of time and know-how, but it’s much more manageable than you might think.
By choosing the right methods and following clear steps, you gain insights into product performance and customer experience —and you’ll be well on your way to making better decisions and creating more customer satisfaction and loyalty.
FAQs about quantitative data analysis
What is quantitative data analysis.
Quantitative data analysis is the process of making sense of numerical data through mathematical calculations and statistical tests. It helps you identify patterns, relationships, and trends to make better decisions.
How is quantitative data analysis different from qualitative data analysis?
Quantitative and qualitative data analysis are both essential processes for making sense of quantitative and qualitative research .
Quantitative data analysis helps you summarize and interpret numerical results from close-ended questions to understand what is happening. Qualitative data analysis helps you summarize and interpret non-numerical results, like opinions or behavior, to understand why the numbers look like they do.
If you want to make strong data-driven decisions, you need both.
What are some benefits of quantitative data analysis?
Quantitative data analysis turns numbers into rich insights. Some benefits of this process include:
Making more confident decisions
Identifying ways to cut costs
Personalizing the user experience
Improving customer satisfaction
What methods can I use to analyze quantitative data?
Quantitative data analysis has two branches: descriptive statistics and inferential statistics.
Descriptive statistics provide a snapshot of the data’s features by calculating measures like mean, median, and mode.
Inferential statistics , as the name implies, involves making inferences about what the data means. Dozens of methods exist for this branch of quantitative data analysis, but three commonly used techniques are:
Cross tabulation
Factor analysis
Language selection
- Français fr
Original quantitative research – Substance-related poisoning hospitalizations and homelessness in Canada: a descriptive study

HPCDP Journal Home

Submit a manuscript
- Information for authors
About HPCDP
- About the Journal
- Contact the Editors
- Past issues
Previous | Table of Contents | Next
Rebecca Plouffe, MPH Author reference footnote 1 Author reference footnote * ; Rochelle White, MPH Author reference footnote 2 Author reference footnote * ; Heather Orpana, PhD Author reference footnote 1 Author reference footnote 3 ; Vera Grywacheski, MPH Author reference footnote 1
https://doi.org/10.24095/hpcdp.44.5.02
This article has been peer reviewed.

Recommended Attribution
Research article by Plouffe R et al. in the HPCDP Journal licensed under a Creative Commons Attribution 4.0 International License
Rebecca Plouffe, Centre for Surveillance and Applied Research, Health Promotion and Chronic Disease Prevention Branch, Public Health Agency of Canada, 785 Carling Ave, Ottawa, ON K1A 0K9; Tel: 437-326-9306; Email: [email protected]
Plouffe R, White R, Orpana H, Grywacheski V. Substance-related poisoning hospitalizations and homelessness in Canada: a descriptive study. Health Promot Chronic Dis Prev Can. 2024;44(5):208-17. https://doi.org/10.24095/hpcdp.44.5.02
Introduction: The objective of this analysis is to describe patient demographics, the context, characteristics and outcomes of a substance-related poisoning, and the recorded mental disorder of people with housing and those experiencing homelessness.
Methods: Hospitalization data for Canada (except Quebec) from 1 April 2019 to 31 March 2020 were retrieved from the Canadian Institute for Health Information ( CIHI ) Discharge Abstract Database using ICD -10- CA codes for up to 25 diagnoses for substance-related poisonings, homelessness status and other characteristics relevant to the patient’s hospitalization. We compared the characteristics of people experiencing homelessness with those of people who were housed, and their substance-related poisoning hospitalizations, using chi-square, t tests and Fisher exact test.
Results: There was a higher proportion of males, younger individuals and people with recorded mental disorders among people experiencing homelessness hospitalized for a substance-related poisoning than among their housed counterparts. Substance-related poisonings among people experiencing homelessness were more likely to be accidental, involve opioids and stimulants (most frequently fentanyl and its analogues and heroin), result in lengthier hospitalizations and end with leaving the hospital against medical advice.
Conclusion: These findings can be used to strengthen strategies and interventions to reduce substance-related harms in priority populations, particularly those experiencing homelessness.
Keywords: opioids, overdose, fentanyl, housing, mental disorder, hospitalization
- People who are homeless were vastly overrepresented among people hospitalized for substance-related poisonings.
- In fiscal year 2019/2020, people experiencing homelessness who were hospitalized for substance-related poisonings spent, on average, about 4 days longer in hospital than people with housing.
- Almost one-quarter (23%) of the hospitalizations of people experiencing homelessness ended with the patients leaving against medical advice, compared to 8% of hospitalizations for people with housing.
- An important area for future research would be to identify ways in which hospitals can retain and treat this at-risk population.
- Research can also help inform additional prevention and harm reduction activities.
Introduction
Canada continues to experience an overdose crisis, with substance-related morbidity and mortality increasing significantly since 2016. Footnote 1 Between January 2016 and December 2020, there were 24 671 opioid-related and 11 176 stimulant-related poisoning hospitalizations in Canada (excluding Quebec). Footnote 1 Although most regions of the country have been affected, British Columbia, Alberta and Ontario continue to have the most opioid and stimulant-related poisoning hospitalizations. Footnote 1 Some subpopulations appear to be disproportionately affected by the overdose crisis, including people experiencing homelessness and housing insecurity. Footnote 2
The rates of substance use are disproportionally high among people experiencing homelessness, and they are at a greater risk of substance-related harms compared to people with housing. Footnote 3 Footnote 4 Footnote 5 Footnote 6 Footnote 7 People who are homeless are also more likely than people with housing to be diagnosed with a mental health disorder, remain hospitalized for longer, and be readmitted within 30 days following discharge. Footnote 4 Footnote 5 Footnote 6 Footnote 7 Footnote 8 Footnote 9
On average, at least 235 000 people experience homelessness in a given year in Canada, and at least 35 000 on a given night. Footnote 2 Across the country, an additional 50 000 people could be experiencing hidden homelessness every night, that is, staying temporarily with friends, relatives or others because they have no other housing option and no immediate prospect of permanent housing. Footnote 2 The number of people experiencing homelessness in Canada is very difficult to estimate, but it is thought to be increasing, possibly also as a result of job losses and evictions during and since the COVID-19 pandemic. Footnote 2 Footnote 10 Footnote 11
The objective of this analysis is to describe patterns of substance-related poisoning hospitalizations in Canada (excluding Quebec) among people with housing and people experiencing homelessness, using the Canadian Institute for Health Information ( CIHI ) Discharge Abstract Database ( DAD ) during the pre-pandemic year of 1 April 2019 to 31 March 2020. This study also examines patterns by patient demographics (sex and age); context of the poisoning (substances involved and intention of the poisoning); hospitalization characteristics and outcomes (length of stay, intensive care unit admission and discharge disposition); and recorded mental disorders.
To our knowledge, this is the first study comparing the characteristics of those experiencing homelessness and those who are housed among people hospitalized for a substance-related poisoning across Canada using this data source. The results of this study can be used to better understand the intersection of homelessness, mental health and substance-related harms and how hospital care is experienced differently by people who are homeless.
Data source
We obtained data from the DAD , which captures acute inpatient discharge records for hospitalizations across Canada, excluding Quebec. In 2019–2020, the DAD had full coverage for acute inpatient care, except from one facility that did not submit data for six periods (an estimated total of 1100 missing abstracts). Footnote 12 Data were presented for the time from 1 April 2019 to 31 March 2020. International Classification of Diseases and Related Health Problems, Tenth Revision, Canada ( ICD -10- CA ) codes were used to capture up to 25 diagnoses from the patient’s hospitalization.
Identifying the study sample
The methodology used to identify substance-related poisonings was adapted from existing CIHI methods. Footnote 13 Footnote 14 Trained coders reviewed medical records and assigned substance specific ICD -10- CA codes according to CIHI coding directives. Footnote 13 Footnote 15 Substance-related poisonings may be recorded in a patient’s chart based on toxicological analyses, self-report and/or responsiveness to treatment received (for instance, reversal of an opioid poisoning after being administered naloxone). Poisonings of interest were included if they were due to the following substances: opioids (T40.0, T40.1, T40.2, T40.20–T40.23, T40.28, T40.3, T40.4, T40.40, T40.41, T40.48, T40.6); stimulants (T40.5, T43.6); cannabis (T40.7); hallucinogens (T40.8, T40.9); alcohol (T51); other depressants (T42.3, T42.4, T42.6, T42.7); and psychotropic drugs (T43.8, T43.9).
This analysis was limited to significant poisonings, defined as cases where the poisoning influenced the duration of the time the patient spent in hospital and the treatment they received. Secondary diagnoses and unconfirmed or query diagnoses were excluded.
Additional variables
Homelessness status.
Any mention of the ICD -10- CA code Z59.0 on a patient discharge abstract was used to note confirmed or unconfirmed and suspected instances of homelessness status. Homelessness status upon admission to hospital is mandatory to code when mentioned in physician documentation or noted on routine review of the medical record.
Intention of poisoning
Intention of the poisoning was identified in line with CIHI coding standards, where coders assign an external cause ICD -10- CA code indicating whether the poisoning was accidental (X41, X42, X45), intentional (X61, X62, X65) or undetermined (Y11, Y12, Y15). Confirmed and suspected diagnoses were included in the intention analysis. Records containing one or more poisonings with a missing associated external cause code were excluded from analyses of intention.
Recorded mental disorders
Consistent with CIHI methodology, recorded mental disorders were identified using any relevant ICD -10- CA diagnoses recorded on the patient discharge abstract during their stay for the substance-related poisoning. Footnote 15 Footnote 16 It is mandatory to record the diagnosis of a mental disorder if having this disorder significantly affects the treatment received, requires treatment beyond maintenance of the pre-existing disorder or increases the length of stay in hospital by at least 24 hours.
All ICD -10- CA codes for a mental disorder on the patient discharge abstract were captured, including confirmed and suspected diagnoses. The following were included: substance-related and addictive disorders (F10–F19, F55, F63.0); schizophrenia and other psychotic disorders (F20–F25, F28, F29); mood disorders (F30–F34, F38, F39, F53.0, F53.1); anxiety disorders (F40, F41, F93.0–F93.2, F94.0); selected disorders of personality and behaviour (F60–F62, F68 [excluding F68.1], F69); and other mental disorders (F42–F45, F48.0, F48.1, F48.8, F48.9, F50–F52, F53.8, F53.9, F54, F59, F63 [excluding F63.0], F68.1, F90–F92, F93.3, F93.8, F93.9, F94.1, F94.2, F94.8, F94.9, F95, F98.0, F98.1–F98.5, F98.8, F98.9, F99, O99.3). Some examples of “other mental disorders” covered by these ICD -10- CA codes include hypochondriacal disorder, eating disorders, nonorganic sleep disorders, conduct disorders, and posttraumatic stress disorder.
Length of stay in hospital and discharge disposition
Total length of stay in hospital was calculated as the sum of the number of days a patient was in acute inpatient care and alternate level of care. Acute inpatient care length of stay describes when a patient is receiving necessary treatment for a disease or severe episode of illness for a short period; alternate level of care describes when a patient is occupying a bed, but not requiring the intensity of services provided in that care setting.
Discharge disposition refers to the status of the patient upon discharge or where the patient is discharged to, and is identified by examining the patient’s hospitalization record.
We conducted descriptive analyses of substance-related poisoning hospitalizations among people experiencing homelessness as well as among housed people (in order to have a reference category). Percentages of substance-related poisoning hospitalizations with a specific recorded mental disorder were calculated based on the denominator of the total study population; these may exceed 100% when summed because of polysubstance poisonings and diagnoses of multiple mental disorders. Counts of less than five per disaggregated category were suppressed in accordance with the CIHI privacy policy. Footnote 17
We used a Pearson chi-square test to determine significant associations between housing status and categorical variables, and a Fisher exact test when expected counts for cells were less than five. A Satterthwaite t test was used to test differences by housing status for continuous variables.
All analyses were completed using statistical package SAS Enterprise Guide version 7.1 ( SAS Institute Inc., Cary, NC , US ).
Between April 2019 and March 2020, there were 10 659 substance-related poisoning hospitalizations in Canada (excluding Quebec). Approximately 6% (623) of these were recorded among people experiencing homelessness.
Patient demographics
Among those hospitalized for substance-related poisonings, there was a higher proportion of males experiencing homelessness (71%) than females (29%), while among those with housing, slightly more females (53%) than males (47%) were hospitalized ( Table 1 ). Of those hospitalized for substance-related poisoning, the mean age of people experiencing homelessness was lower than the mean age of their housed counterparts (39.2 vs. 42.5 years; p < 0.001) ( Figure 1 ).

Hospitalization characteristics and outcomes
People who were homeless stayed for a significantly longer time in hospital for a substance-related poisoning than those with housing (11.0 vs. 6.6 days; p < 0.05) ( Table 2 ). The proportions of hospitalizations admitted into intensive care did not differ between the two population groups, but people experiencing homelessness had a higher mean length of stay in alternate level of care than those with housing (3.7 vs. 0.8 days; p < 0.05). Among individuals with housing, 8% discharged themselves from the hospital against medical advice, whereas 23% of individuals who were homeless did the same ( p < 0.001). There was no difference between the two population groups in the proportions who died while hospitalized for a substance-related poisoning.
The majority (68%) of people with housing who were hospitalized for substance-related poisoning were discharged home. In comparison, 49% of hospitalizations of people experiencing homelessness on admission and who refused shelter upon discharge were “discharged home,” suggesting that this finding should be interpreted with caution.
Substances involved in poisoning hospitalization
Opioids were the most common type of substance involved in hospitalizations for a substance-related poisoning ( Table 3 ), but to a greater extent among people experiencing homelessness than among people with housing (61% vs. 40%; p < 0.001). Stimulants, such as cocaine and methamphetamine, were also involved in a greater proportion of hospitalizations of people who were homeless (29%) compared to people with housing (29% vs. 19%; p < 0.001). In contrast, other depressants, for example, benzodiazepines and other sedatives, were more common in hospitalizations of people with housing compared to those experiencing homelessness (39% vs. 19%; p < 0.001).
Where an opioid was involved in a poisoning hospitalization, fentanyl and its analogues (34% vs. 20%; p < 0.001) and heroin (15% vs. 7%; p < 0.001) were more prevalent in higher proportions of people experiencing homelessness than of people with housing. In contrast, oxycodone, codeine and hydromorphone were significantly more prevalent in hospitalizations of people with housing.
The percentage of substance-related poisoning hospitalizations that involved one, two or three or more substances did not differ by housing status.
Intention of the poisoning
Higher proportions of substance-related poisoning hospitalizations were recorded as accidental among people who were homeless than among people with housing (62% vs. 45%; p < 0.001) ( Table 4 ). People with housing had a higher proportion of such hospitalizations recorded as intentional self-harm (46% vs. 26% for people experiencing homelessness; p < 0.001). This pattern was also observed among females and males separately, although the magnitude of the differences varied.
People experiencing homelessness who were hospitalized for substance-related poisonings had a higher proportion of mental disorders recorded during their hospital stay than those with housing (61% vs. 52%; p < 0.001) ( Table 5 ). The most commonly recorded mental disorders for both populations were substance-related and addictive disorders, although a significantly greater proportion of people who were homeless than people with housing had this diagnosis (51% vs. 25%; p < 0.001). People with housing who were hospitalized for substance-related poisonings had a higher proportion of recorded mood disorders (21% vs. 11%; p < 0.001) and of recorded anxiety disorders (9% vs. 3%; p < 0.001) than their counterparts who were experiencing homelessness.
Stratification by sex showed significant differences in the distribution of substance-related poisoning hospitalizations with various mental disorders. Females experiencing homelessness were significantly more likely to have substance-related and addictive disorders (48% vs. 21%; p < 0.001) and schizophrenia and other psychotic disorders recorded (5% vs. 2%; p < 0.05) compared to their housed counterparts. Conversely, housed females were more likely to have mood (26% vs. 15%; p < 0.001) and anxiety disorders recorded (11% vs. 6%; p < 0.05) compared to females who were homeless.
A similar trend was observed among males, with the most substantial difference between the two populations in diagnosed substance-related and addictive disorders. Among males experiencing homelessness, 53% had such a diagnosis compared to 29% of housed males ( p < 0.001). Housed males were more likely to have mood disorders (15% vs. 9%; p < 0.001) and anxiety disorders recorded (6% vs. 2%; p < 0.001) and less likely to have selected disorders of personality and behaviour (3% vs. 6%; p < 0.001) compared to males who were homeless.
Among hospitalizations for substance-related poisonings, males and younger adults were disproportionately represented among people experiencing homelessness, as compared to the housed population. Higher proportions of homelessness among men than among women have been previously reported. Footnote 2 Footnote 18 However, recent evidence suggests that many more women than men may be experiencing hidden homelessness, resulting in misclassification of housing status among females. Footnote 19 The younger mean age of people experiencing homelessness hospitalized for substance-related poisoning observed in this study likely reflects the younger age of people who are homeless. Footnote 2
We found that a higher proportion of substance-related poisoning hospitalizations among people experiencing homelessness were recorded as accidental rather than intentional self-harm, and that opioids and stimulants, notably fentanyl and its analogues and heroin, were most commonly involved in poisonings leading to hospitalizations. The large proportion of these poisonings being accidental is likely due to the increase in fentanyl and its analogues in the illicit (unregulated) drug supply. These substances have high potencies and are increasingly being combined with other controlled substances. Footnote 20 Footnote 21 Footnote 22 The people who were hospitalized may not have known that the substance they were taking also contained fentanyl and/or its analogues, they may have combined substances to alleviate withdrawal symptoms or to enhance their experience, Footnote 23 or the dose may have been higher than expected, leading to an accidental poisoning.
Hospitalizations for substance-related poisonings among people experiencing homelessness were longer than for those who were housed, with total lengths of stay averaging 11 and 6.6 days, respectively. This finding may be explained by the higher rates of infectious diseases, chronic diseases and long-term physical health conditions prevalent among people who are homeless Footnote 24 Footnote 25 as well as higher rates of mental disorders, as observed in our study. Hospitalized individuals may have also received treatment for comorbidities, resulting in increasing lengths of stay. Further, the lengths of stay for alternate level of care may have differed between the two populations because hospitals may not have options for discharging patients experiencing homelessness.
Lastly, people who were homeless were more likely than those with housing to leave the hospital against medical advice or before being formally discharged by a health care professional. This finding is consistent with previous literature that people who are discharged against medical advice are more likely to be young, male and experiencing homelessness. Footnote 26 Footnote 27 Choi et al. Footnote 26 found that people who are discharged against medical advice had higher rates of both readmission within 14 days and mortality within 12 months. This particular finding has important implications for clinical care settings looking for ways to decrease the number of patients leaving hospital against medical advice and thus reduce harms, mortality and associated costs and increase health care satisfaction.
Strengths and limitations
To our knowledge, this analysis is the first to examine characteristics of substance-related poisoning hospitalizations of people experiencing homelessness across Canada. The DAD includes inpatient acute hospitalization discharges from all provinces and territories except Quebec and therefore has substantial coverage of the population of interest in this study.
There are, however, limitations. First, this analysis only examined acute inpatient hospitalizations, and patterns of substance-related poisonings may vary across different health care settings. For example, individuals with less severe poisonings may be treated through emergency medical services or in the emergency department; not including these health care settings could lead to underestimating the overall prevalence of substance-related poisonings. Moreover, if the pattern of where people seek health care for such poisonings and who is admitted to hospital varies by housing status, these results may not adequately reflect true differences.
Also not captured were data on people who died before being admitted to hospital, which potentially focused this analysis on less severe cases or instances where help was more readily available.
The unit of analysis was hospital discharge and not at the person level or for entire episodes of care. People could have been readmitted multiple times during the study period, which would be counted as multiple hospitalizations. People with multiple admissions may have unique characteristics that are not presented in this study.
Another limitation was that identification of homelessness status may have relied on self-reported information. It is possible that some patients may not have disclosed their homelessness status, or were unable to due to disability or death, which could have resulted in their being misclassified. Similarly, it was only possible to examine housing status as a binary term, as either experiencing homelessness, or not. More nuance is required by including unstable housing, poor housing quality, overcrowding or former homelessness to fully understand the impact of housing status. It is a relatively new requirement to record homelessness status on hospital discharge records and therefore a trend analysis was not possible.
Identifying intention of the poisoning also relied on self-reported information, which can introduce bias if patients are unwilling or unable to disclose this information. Poisonings were classified as accidental unless other intentions were clearly documented, potentially leading to an overrepresentation of accidental poisonings. Throughout this analysis it was not possible to determine which poisonings were a result of pharmaceutical or illicit (or unregulated) opioids, or a combination of both, which hinders the ability to develop targeted interventions to reduce harms associated with substances from different sources.
The estimates of recorded mental disorders did not reflect the overall prevalence of mental disorders among those hospitalized for substance-related poisonings; rather, the mental disorders that were recorded were relevant to the patient’s stay in hospital.
Lastly, Canadian Armed Forces veterans are two to three times more likely to experience homelessness than the general population, and the absence of military status in these data hinders the ability to provide a comprehensive understanding of the relationships between military service, housing status and substance-related poisonings. Footnote 28
Implications
The COVID-19 pandemic has widened health disparities, particularly among hard-to-reach populations. Footnote 2 Footnote 3 Footnote 4 There has also been an increase in the number of people experiencing homelessness, as well as an increase in the number of substance-related poisonings across the country. Footnote 2 Footnote 10 Footnote 11 Although we examined a pre-pandemic period, the results of this study could be used to support actions to reduce substance-related harms by strengthening public health and social infrastructure as people continue to experience the long-term impacts associated with the COVID-19 pandemic as well as other economic impacts.
These findings highlight the need for health care professionals, researchers and policy makers to better understand the intersection of homelessness, mental illness and substance-related harms. They can also inform sectors that interact with vulnerably housed individuals. In particular, these findings demonstrate how substance-related harms and care in hospital settings may differ for people with housing compared to people experiencing homelessness, as exhibited by the high proportion of substance-related poisoning hospitalizations that ended with leaving against medical advice. This difference in care may be due to a variety of factors, such as care not meeting the needs of this population or due to a lack of trust or stigma, and may warrant further investigation to reduce barriers to care for people who are homeless.
Compared to people with housing, unhoused people hospitalized for a substance-related poisoning are more likely to be younger, male and with a recorded mental disorder. A higher proportion of substance-related poisoning hospitalizations of unhoused people were accidental and involving opioids and stimulants, particularly fentanyl and its analogues and heroin. Lastly, substance-related poisoning hospitalizations of unhoused people lasted longer and were more likely to end with leaving the hospital against medical advice.
These findings emphasize the importance of acknowledging the intersectionality of mental illness, substance use and housing status when considering options to address substance-related harms. Future studies should aim to determine how care in hospital settings and other social services can optimize support, in order to prevent further substance-related harms.
Acknowledgements
We thank the Canadian Institute for Health Information for collecting and providing the data used in this study, and Patrick Hunter and Nan Zhou from Infrastructure Canada for their input and support of this project.
Some material from this report has been previously published by the Government of Canada ; permission was obtained to reprint it. All major contributors were contacted and agreed to this publication.
This research did not receive any funding from agencies in the public, commercial or not-for-profit sectors.
Conflicts of interest
None to declare.
Authors’ contributions and statement
- RP : Investigation, data curation, methodology, formal analysis, writing – original draft.
- RW : Investigation, data curation, methodology, formal analysis, writing – review & editing.
- HO : Conceptualization, supervision, writing – review & editing.
- VG : Conceptualization, supervision, validation, writing – review & editing.
Parts of this material are based on data and information compiled and provided by the Canadian Institute for Health Information ( CIHI ). However, the analyses, conclusions, opinions and statements expressed herein are those of the authors and not necessarily those of CIHI or of the Government of Canada.
Page details
A quantitative priority-based approach using a multi-criteria decision-making model for sustainable coastal city development
- Open access
- Published: 16 May 2024
Cite this article
You have full access to this open access article
- Merve Dilman Gokkaya ORCID: orcid.org/0000-0001-6500-9944 1 ,
- Sara Demir ORCID: orcid.org/0000-0002-0813-3356 1 ,
- Onur Aksoy ORCID: orcid.org/0000-0002-8019-3724 1 &
- Nazli Deniz Ersoz ORCID: orcid.org/0000-0002-3851-2859 1
Throughout history, water availability has been an essential factor in shaping the development, culture, transportation, economic activities, and access to food in cities. The socioeconomic and cultural development of a city or country with a coast depends on the active use of the coast and the impact of the coast on urban life. In this context, for the development of coastal cities, it is necessary to determine coastal city criteria and specify their strategies in line with these criteria in order to increase coastal qualities. This research determined Bursa, located northwest of Turkey, as the study area. However, factors such as the absence of a coastal plan for the city and the inability of the coast to integrate into urban life have weakened the coastal city’s qualities of it. For this reason, this study aims to define Bursa’s coastal city criteria and determine strategies to increase the coastal city quality. The coastal city criteria developed depending on the examples of coastal cities in the world were evaluated by surveying local people and experts. The results of these surveys were digitized according to their priorities using Analytical Hierarchical Process (AHP), one of the multi-criteria decision-making (MCDM) methods. The results of this study determined the highest priority criteria among the three main and nine sub-coastal city criteria in evaluating the coastal city in Bursa. This study proposes a numerical method that develops effective and sustainable coastal design and planning strategies for Turkey and underdeveloped countries.
Avoid common mistakes on your manuscript.
1 Introduction
Historically, cities have developed along coastlines because of local economic benefits derived through trade, defense, transportation, and access to food. For this reason, cities on the coastline have generally been critically important in terms of culture, history, and economy (Sandhu et al., 2019 ). Coastal cities are the center of attraction for residents and international activities worldwide. Ports and their associated economic activities, such as shipping and activities based on ecosystem resources, such as tourism, fishing, and aquaculture, are critical activities for this attraction function (Cummins et al., 2004 ; Hegazy, 2021 ). These features offer numerous advantages to coastal communities depending on the coast’s ecological, economic, scientific, and aesthetic values (Hegazy, 2021 ).
A city that fits the definition of a coastal city has a fully integrated urban life and system. Coastal cities, acting as a bridge between the coast and the land that continues behind it, represent a highly rich geography as they contain varying ecosystems. For centuries, the presence of water in cities has been the main factor shaping the city’s life, culture, and spatial organization. Additionally, water element in the coastal cities has provided significant advantages to the city by developing economic activities and transportation infrastructure (Chinnasamy & Parikh, 2021 ). The development of the city depends on how well the coast is used and how integrated it is with the city (Barragán & De Andrés, 2015 ).
Coastal regions represent 20% of the Earth’s surface, and a large proportion of the total urban population lives in the major cities (Brommer et al., 2009 ; Hegazy, 2021 ). Due to global trade activities and internationally operating ports, the coastal population is also growing exponentially (Tibbetts, 2002 ). In many countries, populations in coastal areas are growing faster than populations in non-coastal areas. For example, in 1950, New York was the planet’s only “megacity,” defined as a coastal city of more than 10 million people. Currently, there are 17 megacities worldwide, and 14 of them (New York, Rio de Janeiro, Buenos Aires, Shanghai, Tokyo) are located in coastal areas (Tibbetts, 2002 ). However, it also raises concerns that population growth and associated activities may harm coastal and marine ecosystems (Creel, 2003 ; Pramanik et al., 2016 ).
A wide range of studies have been carried out on various issues related to coastal areas in the world. These studies highlight the features that distinguish coastal cities from other cities. To be more specific, trade/port activities, coastal tourism, and traditional economic activities (Fishing, Agriculture, Aquaculture) (Balk et al., 2009 ; Creel, 2003 ; Dimitrovski et al., 2021 ; FAO, 2002 ; Hinrichsen & Olsen, 1998 ; Ji & Wang, 2022 ; McGranahan et al., 2005 ; Timmerman & White, 1997 ; UN-Habitat, 2009 ) are the criteria evaluated within the scope of economic development. In addition, the urban development and spatial growth trends of coastal cities, population density, coastal land uses, and the central nature of coastal areas (Barragán & De Andrés, 2015 ; Creel, 2003 ; Kpienbaareh & Luginaah, 2020 ; Schneider & Woodcock, 2008 ; Yilmaz & Terzi, 2021 ) have also been evaluated as differential criteria. The transportation factor in coastal cities is typically evaluated separately as transportation within the city and sea transportation between different cities and countries (Baird, 2009 ; Boulos, 2016 ; Chen et al., 2021 ; Corbett, 2004 ; Marsh, 2012 ; Polyzos & Tsiotas, 2012 ).
Turkey is a country with affluent coastal areas and an abundance of coastal resources. Turkey’s coasts are chosen for travel and tourism due to their natural beauty and cultural and historical values (Cengiz et al., 2022 ). While the Mediterranean and Aegean coasts are developing toward tourism, the cities around the Marmara Sea are developing toward the economy and industry (Kaypak, 2012 ). Therefore, it is necessary to monitor the coastal areas by considering environmental and economic as well as transportation aspects (Dogan, 2013 ; Guneroglu, 2015 ). Maritime transport in Turkey is developing under minimal conditions as it needs to be given special importance. When road transportation was not developed, the sea provided transportation between the settlements on the coast. However, due to the development of highways, sea transportation has lost its importance rapidly (Deniz, 2016 ). Maritime Administration, which has an important place in maritime transportation in Turkey, has withdrawn from the business of passenger transportation, and national and international passenger and vehicle transportation has started to be carried out by private companies. As a result, the share of passenger transportation in maritime transportation has decreased to almost non-existence (Avci, 2005 ; Deniz, 2016 ). The lack of unique coastal planning in Turkey, the destruction of the natural coastline with various construction activities, and the development of coastal transportation only for port activities despite being surrounded by sea on three sides reduce the quality and impact of coastal cities. Turkey has the characteristics of a port with its seas, but coastal cities have remained in the background because of the lack of proper urban planning and management.
The characteristics of coastal cities have been stated in different categories in different studies (Balk et al., 2009 ; Barragán & De Andrés, 2015 ; Chen et al., 2021 ; Creel, 2003 ; Dimitrovski et al., 2021 ; Ji & Wang, 2022 ; McGranahan et al., 2005 ; Timmerman & White, 1997 ; UN-Habitat, 2009 ; Yilmaz & Terzi, 2021 ). Despite the significance of economic activities, urban development, spatial growth and transportation factors in defining the identity of coastal cities, these elements have not yet been studied in a holistic approach. Moreover, it is worth noting that despite the growing interest in coastal cities, more empirical studies need to be conducted to evaluate the criteria set forth for such regions and subsequently determine their relative importance. This gap in the literature underscores the need for further research to address this critical knowledge gap and inform decision-making in this area. In light of the existing gap in the literature, the present study aims to bridge this gap by developing a systematic appraisal mechanism to uncover a coastal city profile. Even though Bursa, the subject of the study, is situated on the coast of the Marmara Sea in Turkey, it appears that the coastal regions are not given significant attention in the city’s overall spatial planning. Thereby, this research entails the development of a comprehensive coastal city evaluation system aimed at assessing the characteristics of Bursa’s coastal city in accordance with predetermined criteria. Based on the evaluation outcomes, suitable strategies will be formulated to address the identified issues and strengthen the city’s coastal management practices. In this context, for the first time, all criteria related to the coastal city were listed under main and sub-headings. Using AHP, an MCDM method, with a practical participatory approach, each criterion was digitized and ranked according to their essential priorities. According to the emerging priorities, planning and design strategies have been proposed for the city of Bursa to increase the quality of a coastal city. Unlike other coastal city studies, this research conducted in Turkey determined coastal city criteria comprehensively. For the first time, they were digitized according to their importance priorities with a participatory approach. This research employs a fresh and creative approach, making it exceptional in its focus on the key criteria for coastal cities and the corresponding recommendations it provides. Therefore, this study can be regarded as a noteworthy model for assessing Turkey and other coastal cities worldwide, and devising effective strategies to improve their overall qualities.
2 Material and method
2.1 material: study area.
The Anatolian Peninsula, together with the Thrace Peninsula in the northwest, forms the territory of Turkey. The coast has a border length of approximately 11,000 km and a coastline of 8333 km, including the islands in Turkey. The most extended 42% of the 8333 km coastline is on the shores of the Aegean Sea, 21% in the Mediterranean, approximately 21% in the Black Sea, and 1.5 km in the Marmara. Black Sea, Marmara, Aegean, and the Mediterranean are our regions in contact with the coast, and we have 29 coastal provinces in total (Kaypak, 2012 ).
The primary material of this study was the natural and cultural landscape value of the coastline of Bursa, which was chosen as the study area. Bursa is the fourth largest city in Turkey and the third largest city on the shoreline of Turkey. Thanks to its fertile plain, rich water resources, and close location to Istanbul and other international trade centers, it has been an important commercial center from the past to the present (İlhan, 2019 ). Located on the Marmara Sea, the city of Bursa is the second largest city in Marmara after Istanbul. Consequently, many coastal structures, especially transportation coastal structures, are on the Bursa province’s shores. The length of the coastline is approximately 115 km, and three districts within the boundary of the Marmara Sea of Bursa are Gemlik, Mudanya, and Karacabey (BBB, 2015 ) (Fig. 1 ). The coastal length of Gemlik, Mudanya, and Karacabey are approximately 38 km, 42 km, and 35 km, respectively (BBB, 2015 ). However, Fig. 1 also shows that these three districts are far from the center of the Bursa.
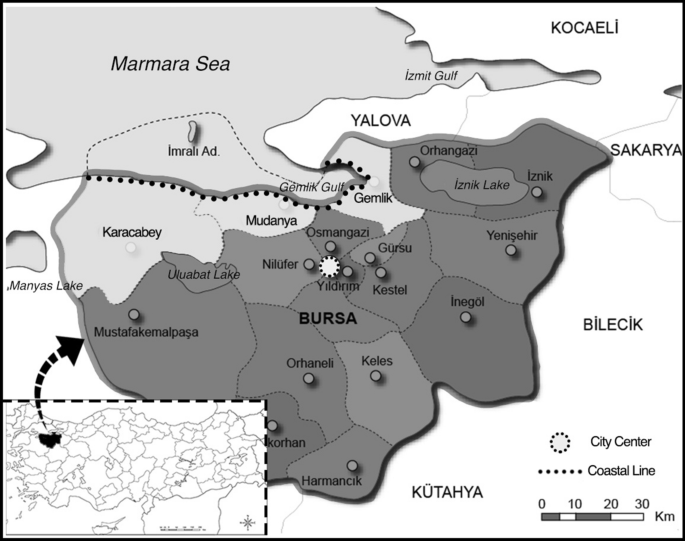
Location of study area and coastal part of the Bursa
Being an essential industrial base in the region, Gemlik continues to develop in the industrial sector with its feature of being a coastal area with the fourth largest free zone in Turkey. This situation causes the demographic structure to change and increase; dense structuring is seen on the coasts (Bulut & Murat, 2019 ). Located 28 km from Bursa city center, Mudanya is a district of Bursa with a population of 54,000. Mudanya, which has an important place in the city in terms of intercity freight and passenger transportation with its developing tourism potential, has been closely related to Bursa’s economic and cultural developments (Polat & Dostoğlu, 2007 ). The coasts of Bursa province have suitable geographical conditions for tourism and recreation. Tourism investments, which have increased in parallel with the rapidly developing tourism sector in the city, have led to a significant increase in population, especially since 1990 (Kurt, 2015 ). As a result, while the population in the coastal districts of Bursa (Gemlik, Mudanya, Karacabey) was 145,050 in 1980, it increased to 510,902 in 1990 and 700,649 in 2013. Along the coast are land uses consisting of forests, protected areas, wetlands, and sand dunes (Fig. 2 ). However, with the increasing population, many of the agricultural and forest areas within the protection zone on the coast have turned into holiday sites and second residences. Considering the tourist population added in the summer months, it becomes clear that there is significant pressure on the natural and geomorphological structure of the province’s coasts (Kurt, 2015 ). There are olive groves for agriculture in the coastal area. However, it creates severe urban pressure on olive groves with tourism-based construction activities. Furthermore, according to the accessibility situation given in Fig. 2 , only two main roads reach the coastal areas from the center of Bursa. Public transportation facilities are limited, and therefore, transportation to coastal areas is mainly provided by private vehicles.
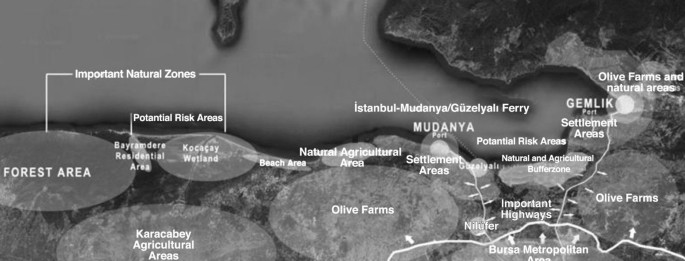
Coastal landuse and accesibility of coastal areas (Gündüz & Aytaç, 2013 )
The research process consists of four stages: determination of the current situation, (1) determination of the coastal city criteria, (2) quantification of coastal city criteria with AHP, and finally, (3) evaluation of coastal city potential and deficiencies and developing proposal strategies according to coastal city criteria to increase the coastal city potential (4) (Fig. 3 ). Each step is explained in detail in the following sections.
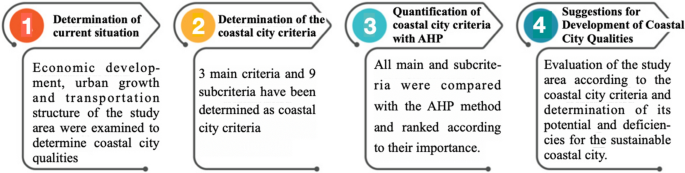
Analytical phases of research
2.2.1 Determination of current situation
Economic activities, the city’s development rate, the existing settlement order, and transportation options are the primary elements that make up the character of a coastal city. For this reason, it is of great importance to read and evaluate these elements correctly in the city to understand the nature of the coastal city. For this reason, at this stage of the study, the economic activities of Bursa and the relationship of these activities with the coast were examined. During the review, data published by official institutions (Municipality, Environment and Urban Ministry, and Chamber of Commerce and Industry) and data of BEBKA (Bursa-Eskişehir-Bilecik Development Agency) data were used. In addition, the city’s spatial organization and land use status were analyzed using Corine 2018 data. Furthermore, using 2018 population data from the Turkish Statistical Institute (TUIK), the population density of the study area has been determined. In addition, the area’s transportation network was analyzed from the regional scale to the urban scale step by step. This current situation helped to understand the relationship between the center of the Bursa and the coastal region.
2.2.2 Determination of the coastal city criteria
At this stage, literature research was conducted covering coastal cities, the development of coastal cities, their characteristics, and previous studies on coastal cities. According to Table 1 , which was created by considering the literature and the characteristics of coastal cities in different geographies, economic development (1), urban growth and spatial organizations (2) and transportation (3) were determined as three main criteria. Trade/port activities, coastal tourism, traditional economic activities (agriculture, aquaculture, fishing), coastal land use, population density, location of the city center, access to the city from other cities and countries, and inner access to the coastal zone were determined as sub-criteria of coastal cities in the decision hierarchy (Table 1 ). In the next step, these determined criteria were weighted with the AHP method, one of the MCDM methods, by taking the opinions of locals and experts. These criteria serve as a framework example for evaluating coastal city quality for other regions with coastal potential. These main and sub-criteria are explained in detail below.
2.2.2.1 Economic development
Coastal cities such as São Paulo, Buenos Aires, and Jakarta prospered after the deregulation of financial markets, and their urban centers flourished with Western-style, high-income commercial and residential gentrification (Tibbetts, 2002 ). The economic attractiveness of the coasts in terms of tourism, logistics, industry, and services leads to population growth and urban spatial growth in physical geography. (Balk et al., 2009 ; Chinnasamy & Parikh, 2021 ; Hinrichsen & Olsen, 1998 ; McGranahan et al., 2005 ; Timmerman & White, 1997 ; UN-Habitat, 2009 ).
Coastal areas worldwide are important destinations for tourism, representing the fastest-growing global economic sector. Tourism dominates the economy of some regions and small island states. As a concrete example, tourism constitutes 95 percent of the economy of the Maldives and is the country’s only source of hard currency (Creel, 2003 ). Tourism can offer some environmental benefits, such as a greater appreciation of the value of natural resources. In the Caribbean, for instance, diving tourism has helped raise awareness about the need for reef conservation, but coastal tourism can also harm the coastal zone. It can lead to unsustainable coastal development as infrastructure is built on the shoreline to accommodate tourists. Nevertheless, tourism plays an important role, especially in the economic development of coastal cities (Dimitrovski et al., 2021 ). With a long coastline, broad sea areas, diverse climates, and many coastal cities, China has rich resources for coastal tourism (Ji & Wang, 2022 ).
Marine fisheries and aquaculture (the controlled cultivation and harvesting of freshwater and marine organisms) produce almost 100 million tons of fish, shellfish, and edible plants yearly, providing a livelihood for about 35 million people, most of whom living in developing countries. However, overharvesting to meet global consumers’ growing demand for seafood can deplete many species and alter the biological structure of coastal ecosystems (Creel, 2003 ; FAO, 2002 ).
2.2.2.2 Urban growth and spatial organizations
Changes in the size, composition and distribution of human populations affect coastal regions by changing land use and land cover (Creel, 2003 ). The agglomeration in coastal cities puts the coastal regions under the pressure of urbanization. In addition, coastal cities in developed countries or with trade relationships of strong port connectivity trigger urban growth (Kpienbaareh & Luginaah, 2020 ; Schneider & Woodcock, 2008 ; Yilmaz & Terzi, 2021 ). Thus, it is essential to understand and reveal how and where urban spatial growth will occur for future urbanization policies and strategies of coastal cities. Spatial growth characteristics vary based on urban growth in developed, developing, and undeveloped countries (Kpienbaareh & Luginaah, 2020 ; Schneider & Woodcock, 2008 ). In addition to the development levels of cities, natural thresholds are one of the most critical determinants in shaping cities. Coasts and topographic thresholds are attractive natural elements driving urban growth (Yilmaz & Terzi, 2021 ). Therefore, it is also important to focus on the types and geometries of urban growth to produce sustainable spatial policies in coastal regions over time.
The average population density in coastal cities or coastal areas of cities is higher than the population density in other residential areas (Creel, 2003 ). About 50 percent of the population in North Africa and Bangladesh lives in coastal areas, and along the Nile Delta, population density reaches 500 to 1,000 people per square kilometer (Creel, 2003 ). With the denser population in coastal cities, urbanization activities are also more intense, and coastal areas are considered centers when economic advantages are taken into account (Barragán & De Andrés, 2015 ). As seen on the political maps of the world, the location of most capitals is either very central or located in coastal areas. Near port and peripheral maritime positions are often seen in countries that were former colonies of overseas powers (Barragán & De Andrés, 2015 ; Creel, 2003 ; Gottmann, 1983 ).
2.2.2.3 Transportation
Transport infrastructure drives development from the coast to the inner city areas (Baird, 2009 ; Boulos, 2016 ; Polyzos & Tsiotas, 2012 ). It acts by developing the city-port link as an effective pillar of economic growth. Access by different modes of transport, such as trains and trucks, is vital for connecting the city and the port so that passengers and freight can move further inland and into the city center beyond the port area (Boulos, 2016 ).
In the past, shipping was one of the most used ways to reach overseas countries or cities (Chen et al., 2021 ; Corbett, 2004 ). In the twenty-first century, shipping is an integral part of the global economy, although it sometimes needs to be more visible to the public. Today, although domestic transportation via maritime transportation has left its place for road and air transportation, maritime transportation in coastal cities is an important alternative with no traffic problem. The maritime transport system between countries comes to the fore in a more commercial sense (Chen et al., 2021 ; Corbett, 2004 ). The maritime transport system is a network of ships, the ports they visit, and the transport infrastructure that stretches from factories to terminals. Worldwide, approximately 44,000 offshore vessels carry more than 33 billion tons of cargo annually (Corbett, 2004 ). In addition, within the scope of cruise ship tourism, both sea transportation and tourism activities, which are economic activities, are carried out between countries (Marsh, 2012 ).
2.2.3 Quantification of coastal city criteria with AHP
The AHP method was introduced by Saaty ( 1980 ) as a model that is frequently used in MCDM problems, handles the relative importance of criteria, effortlessly combines expert decisions, and solves complex decision-making problems in a simple hierarchical order with a flexible approach (Hadipour et al., 2020 ; Zhang et al., 2022 ). This method has multiple stages: structuring the model, prioritizing the criteria, and integrating the priorities (Hadipour et al., 2020 ; Saaty, 1980 ). The AHP method converts verbal data into numerical data, is easy to understand and interpret the results, and works with a limited number (until nine criteria) of criteria (Demir et al., 2016 ; Tajer & Demir, 2022 ). For this reason, the number of criteria determined is essential for the results to be accurate and reliable.
The AHP method is frequently used as an MCDM method (AHP-DELPHI-Fuzzy AHP- TOPSIS) in coastal studies in the last 5 years such as sea level rise and flooding problems, climate change and vulnerability, coastal tourism, and land use issues (Table 2 ). However, Table 2 also shows that this method has not been used in a study to prioritize the criterias of coastal cities.
Within the scope of this study, the reason for using the AHP method is that it is the first study to evaluate the coastal city criteria, and the number of criteria (three main criteria and nine sub-criteria) is suitable for this method. For this reason, this research is both original, and the evaluation process of the results is accessible and understandable. In this context, to prioritize the criteria, a questionnaire was created so that the criteria were compared in pairs. First, the main criteria were evaluated among themselves, and then the sub-criteria were evaluated under each main criterion. At the end of the AHP analysis, each criterion received a priority value in its category by calculating its eigenvalues. Within the scope of the evaluation, the population of the neighborhoods in the central districts and coastal districts was selected to determine the number of questionnaires. Thus, a total population of 2.362.343 was obtained for the Bursa city center and coastal districts according to the Bursa 2021 census. The survey was to be carried out with at least 385 people for a confidence interval of 95%, and it was finally carried out with 391 individuals face-to-face. Those who participated in the survey were divided into 2 groups including locals and experts. In the questionnaire surveys which were conducted in one on one interview, the local participants were chosen randomly from the fields of the study and asked to allocate 10 min for the survey. The expert group consists of landscape architects, architects, city planners, and personnel working in the port and tourism sector in Gemlik and Mudanya Districts. 340 local people and 51 experts were interviewed to evaluate the main and sub-criteria. The following formula was used to determine the sample size (Ozdemir Isik & Demir 2017 ; Tajer & Demir, 2022 ):
n = Sample size, N = Population size (2.362.343), t = Table value (1.96), P = Percentage picking a choice (0.5), Q = (1 − P) Percentage unpicking a choice (0.5), d = Error margin (0.05).
The data were transferred to the Microsoft Office Excel (2020) program at the end of the survey process. The average of each data in the survey was calculated with the help of Expert Choice 11 software for studies conducted with the AHP method to be acceptable, and the consistency ratio should be less than 0.10 (Aydin et al., 2009 ; Demir & Atanur, 2019 ; Ozdemir Işik & Demir, 2017 ). In this context, the consistency rate of this analysis was obtained for the main criteria as 0.01 and sub-criteria as 0.085, 0.016, and 0.092 with an acceptable accuracy rate.
2.2.4 Suggestions for the development of coastal city qualities
This research was conducted with a participatory approach to objectively determine Bursa’s coastal city potential. Given this, in the last stage of the study, the features and potentials of the coastal city of Bursa and its limitation were determined, and offered strategies to increase the coastal city’s potential of Bursa according to the prioritized criteria.
A total of 391 people, including experts and local people, participated in this study, in which the coastal city qualities of the city of Bursa were evaluated. According to the results of this survey, all components of the AHP analysis are listed numerically according to their priorities, and the weight value of each component, together with its consistency rate (CR), is shown in Table 3 . According to the results among the main criteria, the criteria are respectively; “Economic Development” (0.41), “Urban Growth and Spatial Organizations” (0.34), and “Transportation” (0.25) were weighted (Table 3 ). According to this result, the economic development of the city shows that it should be more effective in strategies to increase the quality of the coastal city.
According to Table 3 “Trade and port activities” (0.16), which is one of the sub-criteria of “Economic development,” is determined as the highest priority criterion. In contrast, “Traditional economic activities (fishing, agriculture, aquaculture)” (0.08) is the least priority sub-criteria. This result shows that the economic activities that make a coastal city stand out are due to trade and its ports. “The location of the city center” (0.18), one of the sub-criteria under the title of “Urban growth and spatial organizations,” has been determined as the top priority criterion. Therefore, its weight value has been determined as the total top priority coastal city criterion. In the same category, “Urban population density distribution” (0.05) was chosen with the least priority. In addition, it was determined as the coastal city criterion with the least weight among all the criteria. Among the sub-criteria of “Transportation,” “Inner access to the coastal zone” (0.17) was determined as the highest priority criterion. In contrast, “Transportation from different countries to the city” (0.06) was determined as the minor priority criterion (Table 3 ).
Considering the general ranking of the coastal city criteria according to their weight values, “The location of the city center” (0.18) was determined as the most prioritized criterion (Table 3 ). This result shows that coastal areas, the main elements of coastal cities, should be close to that city’s center and the main center of all economic, urban, and social activities. Among all these criteria, “Transportation to the inner city shore” (0.17), a transportation sub-criterion, was the second highest priority criterion. In contrast, “Trade and port activities” (0.16), which is the sub-criterion of economic development, was determined as the third highest priority criterion. When the criteria with the highest weight value and therefore the first three priority criteria in Table 3 are examined, it is seen that all three belong to different main headings. Moreover, according to the graph in Fig. 4 where all criteria are listed according to their weight values, it can be said that there is a serious break after these first three priority criteria. This situation shows a need to develop strategies for these three criteria in developing coastal cities. This situation shows that the qualities of a coastal city should be developed according to economic and urban growth and transportation criteria. Among all the criteria, “Intra-urban population density distribution” (0.05) was chosen as the least important criterion. After that, “Transportation from different countries to the city” (0.06) as a transportation criterion and “Traditional economic activities (fishing, agriculture, aquaculture)” (0.08) as an economic development criterion was chosen as the least important criterion (Fig. 4 ). This result shows that the necessary infrastructure for transportation for coastal access should be developed. According to these results, the sub-criteria of the three main criteria can be both the top priority criteria of a coastal city and the minor priority criteria on the other hand. Each main criterion (economic development, urban growth, and transportation) has an impact on the development of the coastal city qualities of Bursa, and that appropriate strategies should be determined according to the sub-criteria.
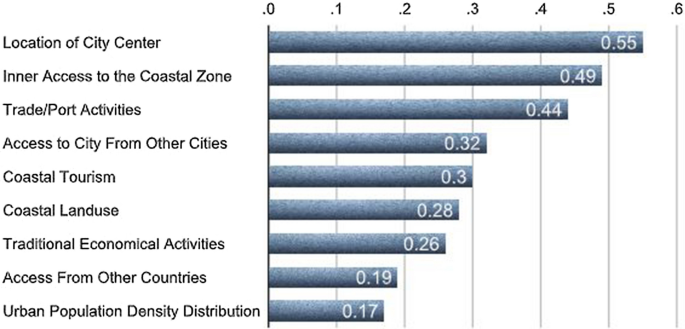
Sorting of coastal city criteria according to their weight values
4 Discussion
Within the scope of this research, coastal city criteria were determined to evaluate the coastal city qualities of Bursa, which has a coast on the Marmara Sea, and the weight values were determined by AHP, an MCDM method, and ranked according to their priorities. Since there is no research on the nature of the coastal city of the study area and there is no study on the digitization of the criteria in the studies of coastal cities in the world, the criteria were determined beforehand. Then they were digitized with AHP, which is a quantitative method. Survey results from experts and local people were used for digitization. This method was put forward with a participatory approach and was applied for the first time in Bursa and other coastal cities.
In research on coastal cities, some studies apply MCDM methods. In studies by Liu et al. ( 2019 ); Hadipour et al. ( 2020 ); Rocha et al. ( 2020 ); Sánchez-Lozano et al. ( 2020 ); Bagheri et al. ( 2021a , 2021b ); Araujo and Dias ( 2021 ); Pathan et al. ( 2022 ) and Xiao et al. ( 2022 ), Fuzzy AHP, DELPHI, Entropy Weight Methodology, SWOT and TOPSIS methods were used, primarily AHP method. On the other hand, researches mainly consist of issues such as suitability for coastal use, flood and sea level rise, assessment of vulnerability in coastal cities, water quality, coastal tourism, flood risk assessment, and cultural protection in coastal areas. In this study, the AHP method, suitable for pairwise comparison according to the number of criteria determined, creates a holistic approach to evaluating coastal cities and eliminates this gap in the literature. It has an accuracy of less than 0.1 with a consistency ratio of 0.01 for the main criteria and 0.085, 0.016, and 0.092 for the sub-criteria. This method, which is used to evaluate the coastal quality of the city of Bursa by prioritizing the digitized criteria, will be a first in coastal surveys for Bursa.
Various studies have been conducted on the relationship between the city of Bursa and the coast. In their studies, Gündüz and Aytaç, ( 2013 ), Batman et al. ( 2019 ), Bulut and Murat, ( 2019 ), Polat and Arslan ( 2019 ), Akdeniz et al. ( 2020 ), Katip ( 2020 ), Değerli and Erbaş ( 2021 ) emphasized coastal architecture, water pollution, coastal vegetation, recreation potential and coastal land uses of Bursa. However, most of the studies are qualitative studies. However, in this study, the status of Bursa as a coastal city was questioned. The criteria that make up a coastal city were determined comprehensively by examining different literature and examples worldwide. In this context, the coastal city criteria of Bursa were listed as the main and lower coastal city criteria, and each criterion was prioritized by comparing the main and sub-criteria among themselves. In this way, for the first time, the coastal city criteria for Bursa and other cities worldwide were evaluated numerically according to their priorities. In this sense, the study will serve as a base for future studies of coastal cities and contribute to evaluating coastal city qualities of cities that have a coast to a sea or ocean.
In the studies of Demir et al. ( 2016 ), Özdemir Işik and Demir ( 2017 ), Demir and Atanur ( 2019 ), Asadpourian et al. ( 2020 ), Zorlu and Yilmaz ( 2020 ); Sahani ( 2021 ) emphasized that the pairwise comparison methods they used in their research increased the questionnaire questions. However, the number of criteria determined in this study led to the formation of an appropriate number of comparison questions for the AHP method. On the other hand, one of the advantages of the survey process is that the people have an excellent command of the subject in the surveys made with the local people. However, the process progressed slowly as access to the coastal areas of Bursa was limited during the survey process. Therefore, in future studies, survey studies will be easier and more effective by increasing the transportation possibilities from the city center to the coast.
Bursa is the fourth largest city contributing to the country’s economy with its port activities. The port activities, tourism activities, and the fact that the coastal areas of the city are suitable for agriculture are important criteria that increase the quality of the coastal city. (Cengiz et al., 2022 ; Dogan, 2013 ; Guneroglu, 2015 ; Kaypak, 2012 ). However, the fact that these coastal areas are far from the city center cannot integrate the coastal areas with the city of Bursa. Within the scope of this study, with the strategies proposed for the development of Bursa according to the coastal city criteria, it will be possible to increase the city’s coastal city qualities and strengthen the connection between the center of Bursa and the coastal region. In order to ensure this integrity, urban development and transportation activities should be developed, and economic activities should support these activities.
As a result of the research, the top three coastal city criteria with the highest priority were selected, respectively, “Location of the city center” (P3), “Transport to the inner city coast” (U2), and “Trade and port activities” (E1). Since Bursa is also a historical city, the place where the first urban development of Bursa started in history is in the Osmangazi district. However, in time, with the increase in the population of the city, Yildirim and Nilüfer districts also developed and formed the metropolitan area of Bursa. The most important feature of these three districts is that they are connected to each other by different transportation lines, and the central transportation axis of the city passes between these districts. Therefore, urban integrity can be achieved by increasing the relations of the districts in the coastal part of Bursa with the city center. One crucial factor that provides the relationship between the city center and the coastal areas is the second priority criterion, the urban-to-coast transportation criterion. Due to the natural topography of Bursa, activities on transportation are limited. For this reason, a highway line for each coastal district provides transportation to the coast. However, increasing public transportation services over these lines will encourage transportation from the city center to the coast. At the same time, with the increase in transportation opportunities, the liveliness of the coast will increase, and it will be more related to the city center. Supporting these developments with the economic power coming from port activities can increase the dynamics of a coastal city. According to the results of the survey, The criteria of “Intra-urban population density distribution” (P2), “Transportation from different countries to the city” (U3), and “Traditional economic activities (fishing, agriculture, aquaculture)” (E3) were chosen as the least priority. Although it is of low value, considering these criteria in coastal cities can contribute to urban dynamics. The increase in urban population density in coastal areas may increase coastal activities. At the same time, the natural coastal structure, which is destroyed due to the pressure of urbanization, can be recovered through agriculture and aquaculture activities. It can increase the recognition of Bursa city as a coastal city by other countries by providing transportation to different geographies. Thus, it can contribute to the economic welfare of the city.
According to the AHP results, the three most and least priority criteria of the study area were selected from the three main criteria. According to this result, economic development, urban growth, and transportation should be considered together in all studies to be carried out within the scope of increasing the coastal city qualities of Bursa. However, physical and social interaction between the city center and the coast should be ensured. The transportation options of people living in the city center to the coast should be increased and facilitated. Tourism activities in coastal areas and cultural tourism activities in the city center should encourage each other. Planning should be done for coastal tourism, and the effect of tourism pressure on natural values in the coastal region should be reduced. Land use in coastal areas should be planned considering the natural, cultural, and economic potentials of Bursa. For this purpose, an urban management plan should be prepared with the relevant ministries, local governments, private sector representatives, and stakeholders. The coastal management plan should be integrated into this management plan. The development of the city center and the coastal area should be planned together. Urban development and management of the city of Bursa should always be considered with the coastal factor in mind.
5 Conclusion
Coastal cities, typically defined as areas where both land and water dynamics coexist, are among the most striking and preferred settlements in the world. The fact that a city has a coast to the ocean or the sea is the main factor that shapes all urban dynamics and economic activities of that city. Most of the developed countries in the world are coastal cities. Coastal cities are distinguished from other cities by their interaction with different settlements in terms of their urban system, economic conditions, and transportation. The main objective of this study, conducted within the context of coastal cities, was to assess the holistic criteria for coastal cities, evaluate the coastal qualities of Bursa in accordance with these criteria, and suggest strategies to enhance the coastal city experience in Bursa accordingly. In this context, criteria suitable for the study area were determined by considering the characteristics of the coastal cities in the world. In the next stage, a survey was conducted with the participation of local people and experts, and the surveys were digitized using the AHP method, which is an MCDM method.
In coastal cities, coastal areas are important for urban life and economic activities. The development of the city is increasing thanks to the coastal areas, and therefore the coastal areas are the center of the city. That said, increasing the interaction of the center and coastal areas in coastal cities and supporting access to the coast can contribute to the development and economic prosperity of that city. For the city of Bursa, the criteria of “location of the city center,” “transport to the inner city coast,” and “trade and port activities” are listed according to the degree of importance among the coastal city criteria. Within the scope of this study, it is recommended to develop appropriate planning and design strategies by focusing on these criteria in order to increase the quality of the coastal city in Bursa. In this context, it shows that for a coastal city, decisions should be made by considering the economic, urban development, and transportation characteristics of that city together and paying attention to their connections with each other.
In order to increase the coastal city quality of Bursa, a holistic urban planning and design process should be established within the framework of legal regulations and criteria priorities. In this context, a participatory working process should be established with the relevant ministries, local administrations, experts, other stakeholders, and local people. As a result, the results and methodology of this study, which integrates the AHP method with a participatory approach and determines the coastal city criteria, and is conducted for the first time within the scope of Bursa, can set an exemplary model not only for Turkey but for all cities that have the potential to become coastal cities in the world.
Data availability
All data generated or analysed during this study are included in this article.
Akdeniz, N. S., Batman, Z. P., Çanga, A. Ç., Altay, E. E., & Zencirkiran, M. (2020). Evaluation of recreation possibilities according to user requests of Mudanya district coastal line in Bursa province. Journal of Bartin Faculty of Forestry , 22 (2), 307–318.
Google Scholar
Araujo, J. C., & Dias, F. F. (2021). Multicriterial method of AHP analysis for the identification of coastal vulnerability regarding the rise of sea level: Case study in Ilha Grande Bay, Rio de Janeiro, Brazil. Natural Hazards, 107 (1), 53–72.
Article Google Scholar
Asadpourian, Z., Rahimian, M., & Gholamrezai, S. (2020). SWOT-AHP-TOWS analysis for sustainable ecotourism development in the best area in Lorestan Province. Iran. Social Indicators Research, 152 , 289–315.
Avci, S., (2005), Turkey’s transportation policies and geographical results in terms of transportation geography. In National Geography Congress 2005, Proceedings Book , pp 87–96.
Aydin, O., Oznehir, S., & Akcali, E. (2009). Selection of optimal location for ankara hospital by modelling analytic hierarchy process. Journal of Economics, Finance and Administrative Science, 14 (2), 69–86.
Bagheri, M., Zaiton Ibrahim, Z., Mansor, S., Abd Manaf, L., Akhir, M. F., Talaat, W. I. A. W., & Beiranvand Pour, A. (2021a). Application of multi-criteria decision-making model and expert choice software for coastal city vulnerability evaluation. Urban Science, 5 (4), 84.
Bagheri, M., Zaiton Ibrahim, Z., Mansor, S., Manaf, L. A., Akhir, M. F., Talaat, W. I. A. W., & Beiranvand Pour, A. (2021b). Land-use suitability assessment using Delphi and analytical hierarchy process (D-AHP) hybrid model for coastal city management: Kuala Terengganu, Peninsular Malaysia. ISPRS International Journal of Geo-Information, 10 (9), 621.
Baird, R. C. (2009). Coastal urbanization: The challenge of management lag. Management of Environmental Quality: An International Journal, 20 (4), 371–382.
Balk, D., Montgomery, M. R., McGranahan, G., Kim, D., Mara, V., Todd, M., & Dorélien, A. (2009). Mapping urban settlements and the risks of climate change in Africa, Asia and South America. Population Dynamics and Climate Change, 80 , 103.
Barragán, J. M., & De Andrés, M. (2015). Analysis and trends of the world’s coastal cities and agglomerations. Ocean & Coastal Management, 114 , 11–20.
Batman, Z. P., Özer, P., & Ayaz, E. (2019). The evaluation of ecology-based tourism potential in coastal villages in accordance with landscape values and user demands: The Bursa-Mudanya-Kumyaka case. International Journal of Sustainable Development & World Ecology, 26 (2), 166–178.
BBB., Department of Coastal Services. (2015). Bursa province integrated coastal areas plan plan explanation report . Ministry of Environment and Urbanization General Directorate of Spatial Planning.
Boulos, J. (2016). Sustainable development of coastal cities-proposal of a modelling framework to achieve sustainable city-port connectivity. Procedia-Social and Behavioral Sciences, 216 , 974–985.
Brommer, M. B., & Bochev-van der Burgh, L. M. (2009). Sustainable coastal zone management: A concept for forecasting long-term and large-scale coastal evolution. Journal of Coastal Research, 25 (1), 181–188.
Bulut, E., & Murat, T. A. Ş. (2019). Sustainability of Coastal Architectural Identity: Bursa/Gemlik. Yalvac Academy Journal, 2 (1), 45–57.
Cengiz, C., Cengiz, B., & Smardon, R. C. (2022). A bridge between coastal resilience and tourism-recreation: multifunctional benefit of boardwalk design for sustainable development in the western black Sea Region, Turkey. Water, 14 (9), 1434.
Chen, K., Su, S., Gong, Y., Xin, X., & Zeng, Q. (2021). Coastal transportation system green policy design model based on shipping network design. International Journal of Logistics Research and Applications, 27 (3), 428–449.
Chinnasamy, P., & Parikh, A. (2021). Remote sensing-based assessment of Coastal Regulation Zones in India: A case study of Mumbai, India. Environment, Development and Sustainability, 23 , 7931–7950.
Corbett, J. J. (2004). Marine transportation and energy use. Encyclopedia of Energy, 3 , 745–758.
Creel, L. (2003). Ripple effects: Population and coastal regions (pp. 1–7). Population reference bureau.
Cummins, V., Mahony, O., & Connolly, N. (2004). Review of Integrated Coastal Zone Management and principles of best practice . Environmental Research Institute University College.
Değerli, B., & Erbaş, A. E. (2021). Integrated coastal zone plans and spatial planning relations, an evaluation of a case study on İzmit Bay and Bursa province. Journal of Awareness, 6 (1), 1.
Demir, S., & Atanur, G. (2019). The prioritization of natural-historical based ecotourism strategies with multiple-criteria decision analysis in ancient UNESCO city: Iznik-Bursa case. International Journal of Sustainable Development & World Ecology, 26 (4), 329–343.
Demir, S., Esbah, H., & Akgün, A. A. (2016). Quantitative SWOT analysis for prioritizing ecotourism-planning decisions in protected areas: Igneada case. International Journal of Sustainable Development & World Ecology, 23 (5), 456–468.
Deniz, T. (2016). Changes and Current Situation in the Transportation Sector in Turkey. Eastern, Geography Journal, 21 (36), 135–156.
Dimitrovski, D., Lemmetyinen, A., Nieminen, L., & Pohjola, T. (2021). Understanding coastal and marine tourism sustainability-A multi-stakeholder analysis. Journal of Destination Marketing & Management, 19 , 100554.
Dogan, M. (2013). The industrialization process in istanbul and the development of the last 10 years from past to present. Marmara Journal of Geography, 27 , 511–550.
FAO (2002). World Fisheries and Aquaculture Atlas: 13; and FAO, The State of World Fisheries and Aquaculture, 2002 (Rome: FAO, 2003): 5.
Gottmann, J. (1983). Capital cities. Ekistics, 50 (299), 88–93.
Gündüz, S., & Aytaç, G. G. (2013). Nature based zoning for the coastal area of Bursa. A Z ITU Journal of the Faculty of Architecture, 10 (2), 51–66.
Guneroglu, A. (2015). Coastal changes and land use alteration on Northeastern part of Turkey. Ocean & Coastal Management, 118 , 225–233.
Hadipour, V., Vafaie, F., & Kerle, N. (2020). An indicator-based approach to assess social vulnerability of coastal areas to sea-level rise and flooding: A case study of Bandar Abbas city, Iran. Ocean & Coastal Management, 188 , 105077.
Hegazy, I. R. (2021). Towards sustainable urbanization of coastal cities: The case of Al-Arish City, Egypt. Ain Shams Engineering Journal, 12 (2), 2275–2284.
Hinrichsen, D., & Olsen, S. (1998). Coastal waters of the world . Island Press.
İlhan, C. (2019). Fractal geometry analysis of urban tissue morphological change: The case study of Bursa. Mimarlik Ve Yaşam, 4 (1), 117–140.
Ji, J., & Wang, D. (2022). Regional differences, dynamic evolution, and driving factors of tourism development in Chinese coastal cities. Ocean & Coastal Management, 226 , 106262.
Katip, A. (2020). Modeling of microbial contamination in the Marmara Sea, Bursa-Turkey. Journal of Environmental Biology, 41 (2), 432–438.
Kaypak, Ş. (2012). Integrated policies in the management of coastal areas. Coastal and Marine Areas of Turkey IX. National Congress.
Kpienbaareh, D., & Luginaah, I. (2020). Modeling the internal structure, dynamics and trends of urban sprawl in Ghanaian cities using remote sensing, spatial metrics and spatial analysis. African Geographical Review, 39 (3), 189–207.
Kurt, S. (2015). The Effect Of The Second Homes Constructions In Bursa Coasts On The Coastal Geomorphology. Electronic Turkish Studies , 10 (2), 641–662.
Liu, R., Wang, Y., & Qian, Z. (2019). Hybrid SWOT-AHP analysis of strategic decisions of coastal tourism: A case study of Shandong Peninsula blue economic zone. Journal of Coastal Research, 94 , 671–676.
Marsh, E. A. (2012). The effects of cruise ship tourism in coastal heritage cities: A case study of Charleston, South Carolina. Journal of Cultural Heritage Management and Sustainable Development., 2 , 190.
McGranahan, G., Marcotullio, P., Bai, X., Balk, D., Braga, T., Douglas, I., & Zlotnik, H. (2005). Urban systems. Ecosystems and Human Well-Being: Current State and Trends, 1 , 795–825.
Ozdemir Işik, B., & Demir, S. (2017). Integrated multi-criteria decision-making methods for the sustainability of historical–cultural structures on the Trabzon Coastline. Sustainability, 9 (11), 2114.
Pathan, A. I., Girish Agnihotri, P., Said, S., & Patel, D. (2022). AHP and TOPSIS based flood risk assessment-a case study of the Navsari City, Gujarat, India. Environmental Monitoring and Assessment, 194 (7), 1–37.
Polat, S., & Dostoğlu, N. (2007). On the Concept of Urban Regeneration: Samples of Kükürtlü and Mudanya in Bursa. Journal of Uludag University Faculty of Engineering , 12 (1), 61–76.
Polat, S., & Arslan, T. V. (2019). Is it possible to develop coastal public spaces with participatory design? Yıldıztepe social life center project in Mudanya. Bursa Kent Akademisi , 12 (3), 531–545.
Polyzos, S., & Tsiotas, D. (2012). The evolution and spatial dynamics of coastal cities in Greece. Urban development , pp 275–296. ISBN: 978-953-51-0442-1.
Pramanik, M. K., Biswas, S. S., Mondal, B., & Pal, R. (2016). Coastal vulnerability assessment of the predicted sea level rise in the coastal zone of Krishna-Godavari delta region, Andhra Pradesh, east coast of India. Environment, Development and Sustainability, 18 , 1635–1655.
Rocha, C., Antunes, C., & Catita, C. (2020). Coastal vulnerability assessment due to sea level rise: The case study of the Atlantic coast of mainland Portugal. Water, 12 (2), 360.
Saaty, T. L. (1980). The analytic hierarchy process (AHP). The Journal of the Operational Research Society, 41 (11), 1073–1076.
Sahani, N. (2021). Application of hybrid SWOT-AHP-FuzzyAHP model for formulation and prioritization of ecotourism strategies in Western Himalaya, India. International Journal of Geoheritage and Parks, 9 (3), 349–362.
Sánchez-Lozano, J. M., Salmerón-Vera, F. J., & Ros-Casajús, C. (2020). Prioritization of cartagena coastal military batteries to transform them into scientific, tourist and cultural places of interest: A gis-mcdm approach. Sustainability, 12 (23), 9908.
Sandhu, S. C., Kelkar, V., & Sankaran, V. (2019). Resilient coastal cities for enhancing tourism economy: Integrated planning approaches (No. 1043). ADBI Working Paper Series .
Schneider, A., & Woodcock, C. E. (2008). Compact, dispersed, fragmented, extensive? A comparison of urban growth in twenty-five global cities using remotely sensed data, pattern metrics and census information. Urban Studies, 45 (3), 659–692.
Tajer, E., & Demir, S. (2022). Ecotourism strategy of UNESCO city in Iran: Applying a new quantitative method integrated with BWM. Journal of Cleaner Production, 376 , 134284.
Tibbetts, J. (2002). Coastal cities: Living on the edge. Environmental Health Perspectives, 110 (11), A674–A681.
Timmerman, P., & White, R. (1997). Megahydropolis: Coastal cities in the context of global environmental change. Global Environmental Change, 7 (3), 205–234.
UN-Habitat (2009). Planning sustainable cities: Global report on human settlements 2009. Earthscan, London .
Xiao, K., Tamborski, J., Wang, X., Feng, X., Wang, S., Wang, Q., Lin, D., & Li, H. (2022). A coupling methodology of the analytic hierarchy process and entropy weight theory for assessing coastal water quality. Environmental Science and Pollution Research, 29 (21), 31217–31234.
Article CAS Google Scholar
Yilmaz, M., & Terzi, F. (2021). Measuring the patterns of urban spatial growth of coastal cities in developing countries by geospatial metrics. Land Use Policy, 107 , 105487.
Zhang, N., Gao, J., Xu, S., Tang, S., & Guo, M. (2022). Establishing an evaluation index system of Coastal Port shoreline resources utilization by objective indicators. Ocean & Coastal Management, 217 , 1060.
Zorlu, K., & Yilmaz, A. (2020). Determination of Strategies of Ecotourism in Protected Areas with SWOT-AHP Method: The Case of Aksaray-Ihlara Special Environmental Protection Zone (SEPZ). Coğrafya Dergisi, 40 , 247–257.
Download references
Author information
Authors and affiliations.
Forest Faculty, Landscape Architecture Department, Bursa Technical University, Bursa, Turkey
Merve Dilman Gokkaya, Sara Demir, Onur Aksoy & Nazli Deniz Ersoz
You can also search for this author in PubMed Google Scholar
Contributions
All authors contributed equally to the entire manuscript.
Corresponding author
Correspondence to Merve Dilman Gokkaya .
Ethics declarations
Conflict of interest.
No potential conflict of interest was reported by the author(s).
Ethical approval
This research complies with internationally accepted standards for research practice and reporting.
Additional information
Publisher's note.
Springer Nature remains neutral with regard to jurisdictional claims in published maps and institutional affiliations.
Rights and permissions
Open Access This article is licensed under a Creative Commons Attribution 4.0 International License, which permits use, sharing, adaptation, distribution and reproduction in any medium or format, as long as you give appropriate credit to the original author(s) and the source, provide a link to the Creative Commons licence, and indicate if changes were made. The images or other third party material in this article are included in the article's Creative Commons licence, unless indicated otherwise in a credit line to the material. If material is not included in the article's Creative Commons licence and your intended use is not permitted by statutory regulation or exceeds the permitted use, you will need to obtain permission directly from the copyright holder. To view a copy of this licence, visit http://creativecommons.org/licenses/by/4.0/ .
Reprints and permissions
About this article
Dilman Gokkaya, M., Demir, S., Aksoy, O. et al. A quantitative priority-based approach using a multi-criteria decision-making model for sustainable coastal city development. Environ Dev Sustain (2024). https://doi.org/10.1007/s10668-024-05014-5
Download citation
Received : 23 January 2024
Accepted : 04 May 2024
Published : 16 May 2024
DOI : https://doi.org/10.1007/s10668-024-05014-5
Share this article
Anyone you share the following link with will be able to read this content:
Sorry, a shareable link is not currently available for this article.
Provided by the Springer Nature SharedIt content-sharing initiative
- Coastal cities
- Coastal criteria
- Multiple-criteria decision-making (MCDM) method
- Analytic hierarchy process (AHP)
- Find a journal
- Publish with us
- Track your research

A QUANTITATIVE ASSESSMENT OF VISUAL FUNCTION FOR YOUNG AND MEDICALLY COMPLEX CHILDREN WITH CEREBRAL VISUAL IMPAIRMENT: DEVELOPMENT AND INTER-RATER RELIABILITY
- Find this author on Google Scholar
- Find this author on PubMed
- Search for this author on this site
- ORCID record for Kathleen M. Weden
- For correspondence: [email protected]
- Info/History
- Preview PDF
Background Cerebral Visual Impairment (CVI) is the most common cause of low vision in children. Standardized, quantifiable measures of visual function are needed.
Objective This study developed and evaluated a new method for quantifying visual function in young and medically complex children with CVI using remote videoconferencing.
Methods Children diagnosed with CVI who had been unable to complete clinic-based recognition acuity tests were recruited from a low-vision rehabilitation clinic(n=22)Video-based Visual Function Assessment (VFA) was implemented using videoconference technology. Three low-vision rehabilitation clinicians independently scored recordings of each child’s VFA. Interclass correlations for inter-rater reliability was analyzed using intraclass correlations (ICC). Correlations were estimated between the video-based VFA scores and both clinically obtained acuity measures and children’s cognitive age equivalence.
Results Inter-rater reliability was analyzed using intraclass correlations (ICC). Correlations were estimated between the VFA scores, clinically obtained acuity measures, and cognitive age equivalence. ICCs showed good agreement (ICC and 95% CI 0.835 (0.701-0.916)) on VFA scores across raters and agreement was comparable to that from previous, similar studies. VFA scores strongly correlated (r= -0.706, p=0.002) with clinically obtained acuity measures. VFA scores and the cognitive age equivalence were moderately correlated (r= 0.518, p=0.005), with notable variation in VFA scores for participants below a ten month cognitive age-equivalence. The variability in VFA scores among children with lowest cognitive age-equivalence may have been an artifact of the study’s scoring method, or may represent existent variability in visual function for children with the lowest cognitive age-equivalence.
Conclusions Our new VFA is a reliable, quantitative measure of visual function for young and medically complex children with CVI. Future study of the VFA intrarater reliability and validity is warranted.
Competing Interest Statement
The authors have declared no competing interest.
Funding Statement
This work was supported by the EyeSight Foundation of Alabama, Alie B. Gorrie Low Vision Research Fund and Research to Prevent Blindness. Additional support came from the National Institutes of Health [UL1 TR003096 to R.O.] and Grant T32 HS013852.
Author Declarations
I confirm all relevant ethical guidelines have been followed, and any necessary IRB and/or ethics committee approvals have been obtained.
The details of the IRB/oversight body that provided approval or exemption for the research described are given below:
IRB of the University of Alabama at Birmingham gave ethical approval for this work
I confirm that all necessary patient/participant consent has been obtained and the appropriate institutional forms have been archived, and that any patient/participant/sample identifiers included were not known to anyone (e.g., hospital staff, patients or participants themselves) outside the research group so cannot be used to identify individuals.
I understand that all clinical trials and any other prospective interventional studies must be registered with an ICMJE-approved registry, such as ClinicalTrials.gov. I confirm that any such study reported in the manuscript has been registered and the trial registration ID is provided (note: if posting a prospective study registered retrospectively, please provide a statement in the trial ID field explaining why the study was not registered in advance).
I have followed all appropriate research reporting guidelines, such as any relevant EQUATOR Network research reporting checklist(s) and other pertinent material, if applicable.
Data Availability
All data produced in the present study are available upon reasonable request to the authors
View the discussion thread.
Thank you for your interest in spreading the word about medRxiv.
NOTE: Your email address is requested solely to identify you as the sender of this article.

Citation Manager Formats
- EndNote (tagged)
- EndNote 8 (xml)
- RefWorks Tagged
- Ref Manager
- Tweet Widget
- Facebook Like
- Google Plus One
Subject Area
- Rehabilitation Medicine and Physical Therapy
- Addiction Medicine (323)
- Allergy and Immunology (627)
- Anesthesia (163)
- Cardiovascular Medicine (2367)
- Dentistry and Oral Medicine (288)
- Dermatology (206)
- Emergency Medicine (379)
- Endocrinology (including Diabetes Mellitus and Metabolic Disease) (835)
- Epidemiology (11765)
- Forensic Medicine (10)
- Gastroenterology (702)
- Genetic and Genomic Medicine (3731)
- Geriatric Medicine (348)
- Health Economics (633)
- Health Informatics (2392)
- Health Policy (929)
- Health Systems and Quality Improvement (896)
- Hematology (340)
- HIV/AIDS (780)
- Infectious Diseases (except HIV/AIDS) (13303)
- Intensive Care and Critical Care Medicine (767)
- Medical Education (365)
- Medical Ethics (104)
- Nephrology (398)
- Neurology (3493)
- Nursing (198)
- Nutrition (523)
- Obstetrics and Gynecology (673)
- Occupational and Environmental Health (662)
- Oncology (1819)
- Ophthalmology (535)
- Orthopedics (218)
- Otolaryngology (287)
- Pain Medicine (232)
- Palliative Medicine (66)
- Pathology (446)
- Pediatrics (1032)
- Pharmacology and Therapeutics (426)
- Primary Care Research (420)
- Psychiatry and Clinical Psychology (3172)
- Public and Global Health (6135)
- Radiology and Imaging (1279)
- Rehabilitation Medicine and Physical Therapy (746)
- Respiratory Medicine (825)
- Rheumatology (379)
- Sexual and Reproductive Health (372)
- Sports Medicine (322)
- Surgery (401)
- Toxicology (50)
- Transplantation (172)
- Urology (145)
- Open access
- Published: 07 May 2024
Causal association between low vitamin D and polycystic ovary syndrome: a bidirectional mendelian randomization study
- Bingrui Gao 1 ,
- Chenxi Zhang 1 ,
- Deping Wang 1 , 2 ,
- Bojuan Li 1 ,
- Zhongyan Shan 1 ,
- Weiping Teng 1 &
- Jing Li ORCID: orcid.org/0000-0002-3681-4095 1
Journal of Ovarian Research volume 17 , Article number: 95 ( 2024 ) Cite this article
280 Accesses
Metrics details
Recent studies have revealed the correlation between serum vitamin D (VD) level and polycystic ovary syndrome (PCOS), but the causality and specific mechanisms remain uncertain.
We aimed to investigate the cause-effect relationship between serum VD and PCOS, and the role of testosterone in the related pathological mechanisms.
We assessed the causality between serum VD and PCOS by using genome-wide association studies (GWAS) data in a bidirectional two-sample Mendelian randomization (TS-MR) analysis. Subsequently, a MR mediation analysis was conducted to examine the mediating action of testosterone in the causality between serum VD and PCOS. Ultimately, we integrated GWAS data with cis-expression quantitative loci (cis-eQTLs) data for gene annotation, and used the potentially related genes for functional enrichment analysis to assess the involvement of testosterone and the potential mechanisms.
TS-MR analysis showed that individuals with lower level of serum VD were more likely to develop PCOS (OR = 0.750, 95% CI: 0.587–0.959, P = 0.022). MR mediation analysis uncovered indirect causal effect of serum VD level on the risk of PCOS via testosterone (OR = 0.983, 95% CI: 0.968–0.998, P = 0.025). Functional enrichment analysis showed that several pathways may be involved in the VD-testosterone-PCOS axis, such as steroid hormone biosynthesis and autophagy process.
Our findings suggest that genetically predicted lower serum VD level may cause a higher risk of developing PCOS, which may be mediated by increased testosterone production.
Introduction
Vitamin D (VD) is an essential fat-soluble steroid hormone that is necessary for calcium-phosphate metabolism, bone homeostasis, cell differentiation, and immune system function. The prevalence of VD deficiency (VDD) in the population has gradually increased over the past few decades. VDD is associated with various diseases, including cardiovascular disease, inflammation, dyslipidemia, weight gain, and infectious diseases [ 1 , 2 ]. Furthermore, mounting studies have indicated the potential link between the serum VD status and women's reproductive health. Firstly, the biological function of VD is mediated via intracellular VD receptors (VDRs), which are distributed among various tissues, encompassing hypothalamic, pituitary tissue, endometrium, and ovary [ 3 , 4 ]. Secondly, VD participates in regulating genes associated with ovarian and placental functions [ 5 , 6 ]. All evidences suggest that the serum VD plays a potentially significant role in female reproductive health.
Polycystic ovary syndrome (PCOS) is the most common endocrine disorder that effects women of reproductive age, with a global incidence ranging 20–25% [ 7 , 8 ]. PCOS will affect woman's endometrial function and oocyte competence [ 9 , 10 ], which leads to reproductive dysfunction in PCOS patients, including infertility, miscarriage, and pregnancy complications [ 11 , 12 , 13 ]. However, the exact pathogenesis of PCOS remains unclear. Prior observational studies have elucidated the correlation between the serum VD and the risk of PCOS. A recent study revealed that serum VD concentration were lower in women diagnosed with PCOS compared to body mass index (BMI)-matched control, suggesting that regardless of BMI, PCOS is correlated with reduced VD level [ 14 ]. However, these studies can only prove that there is a correlation between them, they cannot clarify the causality between them. In addition, hyperandrogenemia stands as one of the diagnostic criteria for PCOS and impacts 60–80% of patients [ 15 ]. Female are actually more sensitive to testosterone even though it is known as a male hormone [ 16 ]. Growing evidences showed that testosterone may play an important role between the serum VD level and the risk of PCOS. Hahn et al. illustrated an association between the serum VD level and the severity of hirsutism in individuals with PCOS [ 17 ]. The research conducted by Latic et al. indicates a negative correlation between serum VD level and testosterone production in patients with PCOS [ 18 ]. However, a study by Mesinovic et al. suggested no discernible correlation between the serum VD level and androgen production in individuals with PCOS [ 19 ]. Moreover, a large observational study by Gallea et al. also showcased the association between serum VD levels, insulin, and body weight among PCOS patients but not specifically with hyperandrogenemia [ 20 ]. The reason for these different results may be due to the fact that observational studies are susceptible to confounding factors as well as various biases [ 21 ]. Therefore, it is not clear whether testosterone production mediate the relationship between serum VD level and the risk of PCOS, due to the limitations of the study methodology.
In recent years, mendelian randomization (MR) analysis is widely used as an epidemiological method in medical research. Firstly, MR analysis can minimize the impact of confounding factors and various biases on the results by simulating randomized controlled trials (RCTs) at the genetic level, and secondly, MR analysis can also determine causality and reduce the impact of reverse causality on the results of the study [ 22 ].
Thus, in this study, we use the bidirectional two-sample MR (TS-MR) analysis to investigate the cause-effect relationship between the serum VD level and the risk of PCOS. Secondly, we perform the mediation MR analysis to test the mediating role of testosterone production between serum VD level and the risk of PCOS. Finally, we used the bioinformatics analysis to assess the possible biological functions and molecular mechanisms between them.
Materials and methods
Study design of mendelian randomization study.
Our study explored the cause-effect of serum VD level as an exposure on the risk of developing PCOS as an outcome trait and the effect of testosterone as a mediator between VD and PCOS through bidirectional TS-MR analysis, multivariable MR (MVMR) and mediator MR analysis (Fig. 1 ). In order to ensure the study's validity, the study needed to meet the three following crucial assumptions [ 23 ] (Fig. 1 C):1) the correlation assumption: instrumental variables (IVs) must be robustly correlated with the exposure factors; 2) the exclusion restriction assumption: IVs are not associated with potential confounders of the exposure or the outcome; and 3) the independence assumption: IVs do not influence the outcome variables through other pathways besides the exposure factors. This study followed guidelines of STROBE-MR [ 24 ] checklist (Table S 1 ).
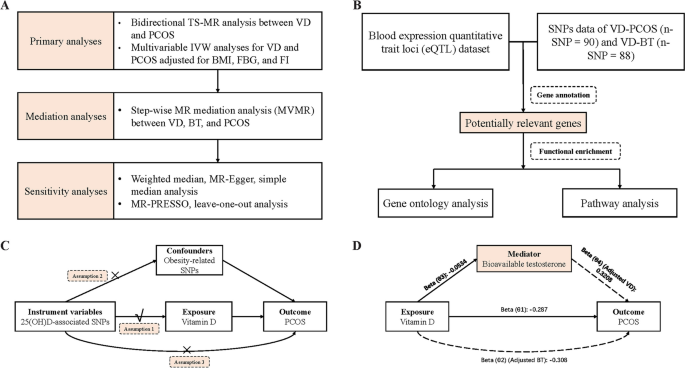
Flowchart of the study. A Flowchart of the MR study; ( B ) Flowchart of the Bioinformatics study; ( C ) Diagram of the MR assumptions of the association between VD and PCOS; ( D ) Illustrative diagram for the mediation MR analysis framework Abbreviations: MR, mendelian randomization; TS-MR, two-sample MR; VD, vitamin D; PCOS, polycystic ovary syndrome; IVW, inverse variance weighted; BMI, body mass index; FBG, fasting glucose; FI, fasting insulin; MVMR, multivariable MR; BT, bioavailable testosterone; SNPs, single-nucleotide polymorphisms
Data source and IVs selection of mendelian randomization study
We obtained data associated with VD from a large genome-wide association study (GWAS) that identified 143 loci among 417,580 participants which was conducted by Revez et al. in 2020 [ 25 ]. We accessed the summary data related to PCOS from a meta-analysis in the FinnGen and Estonian Biobank (EstBB), which included 3609 cases and 229,788 controls [ 7 ]. Summary data related to bioavailable testosterone (BT) were obtained from the UK Biobank (UKB). Data on serum fasting glucose (FBG) levels were obtained from a UKB GWAS we conducted in 340,002 British participants [ 26 ]. Summary data on circulating concentrations of fasting insulin (FI) were obtained from the MAGIC GWAS included 151,013 participants [ 27 ]. Pooled data related to BMI were acquired from a GWAS meta-analysis within the (GIANT) consortium, encompassing 681,275 participants [ 28 ]. Details of the GWAS database are summarized in Table S 2 .
In the bidirectional TS-MR analysis, Single-nucleotide polymorphisms (SNPs) with genome-wide significance ( P < 5 × 10 –8 ) were first selected. These SNPs were matched against the SNP-outcome GWAS database to exclude SNPs that could not be matched. To minimize the effects of linkage disequilibrium, we conducted a clumping process with an r 2 threshold of 0.001 and a clumping window of 10,000 kb and excluded these SNPs if present. Subsequently, we performed MR-PRESSO analysis immediately to demonstrate whether there was significant horizontal pleiotropy to exclude outlier SNPs [ 29 ]. To ensure that the IVs were not affected by confounding variables, we searched the PhenoScanner V2 [ 30 ] and deleted obesity-related SNPs associated with BMI and waist circumference (WC). Finally, 88 SNPs (VD on PCOS) and 2 SNPs (PCOS on VD) were used as IVs in the primary bidirectional TS-MR study, respectively. All SNPs exhibited an F statistic greater than 10. The variance explained for each SNP (R 2 ) was calculated using the widely-accepted formula [ 31 , 32 ]. We used the same method as above to screen the SNPs required in the MR mediation analysis. All the IVs SNPs are summarized in Table S 3 - 7 .
Statistic analysis of mendelian randomization study
Initially, the primary analysis aimed to explore the causal relationship between VD and PCOS. We used bidirectional TS-MR analysis to assess the causal relationship between VD and PCOS. In this, we used Cochran's Q test to assess the heterogeneity [ 33 ]; if there was no heterogeneity, we would use the fixed-effects inverse variance weighted (IVW) method, otherwise, we would use the random-effects IVW method [ 34 ]. Furthermore, considering that obesity, abnormal insulin levels, and abnormal glucose values are common in patients with PCOS, we adjusted genetically predicted BMI, FBG, and FI by MVMR to explore the direct causal effect between VD and PCOS. To make the results more robust.
Secondly, a stepwise MR analysis approach was used to examine whether there exist mediation effects of BT between VD and PCOS. To assess the direct causal effect between VD, BT, and PCOS, we performed an MVMR analysis using the MVMR R package [ 35 ]. Conditional F statistics were calculated for assessing the strength of the genetic instruments in MVMR analysis [ 36 ]. The product of the coefficients method [ 37 ] and the multivariate delta method [ 38 ] were used to calculate the indirect effects of VD on PCOS via mediator.
Sensitivity analysis of mendelian randomization study
The following tests were used as sensitivity analyses to assess the robustness of MR effect estimates to invalid genetic variants. Firstly, we conducted MR-Egger regression [ 39 , 40 ], weighted median [ 41 ], and weighted mode [ 42 ] methods. MR-Egger regression can detect and explain horizontal pleiotropy mainly through intercept tests [ 39 , 40 ]. Weighted median can yield impartial estimations even when over half of the information arise from flawed IVs [ 43 ]. We used weighted mode to divide SNPs into multiple subsets based on similar causal effects, and the estimates of causal effects were computed for the subset with the highest number of SNPs [ 42 ]. Secondly, the leave-one-out (LOO) analysis can test whether the results are affected by a single SNP [ 44 ]. Thirdly, as described above we performed MR-PRESSO analysis [ 29 ] to identify the presence of potential horizontal pleiotropic outliers in IVs that could lead to biased results, as well as searching for and removing obesity-related SNPs associated with BMI and WC from the PhenoScanner database [ 45 ].
All analyses were conducted using R version 4.2.0 (R Foundation for Statistical Computing, Vienna, Austria). P values were considered significant at 0.05.
Bioinformatical analysis
We used the largest whole blood expression quantitative trait loci (eQTL) dataset from the eQTLGen consortium, which includes data on cis-eQTLs for 19,250 whole blood expressed genes from 31,684 individuals [ 46 ]. We combined SNPs data of VD-PCOS ( n -SNP = 90) and VD-BT ( n -SNP = 88) with cis-eQTLs data for gene annotation, respectively. Genes with P < 5*10 –8 and FDR < 0.05 were screened as potentially relevant genes for VD-PCOS and VD-BT.
Subsequently, we used these potentially relevant genes for bioinformatics analyses, including Gene ontology (GO) and Kyoto Encyclopedia of Genes and Genomes (KEGG) analyses. GO analyses [ 47 ], including biological process (BP), molecular function (MF), and cellular composition (CC), are commonly used for large-scale functional enrichment studies. KEGG is a database that stores information about genomes, biological pathways, diseases, and drugs. We used the clusterProfiler package, org.Hs.eg.db package, and enrichplot package in the software R to perform GO and KEGG enrichment analyses of the potentially relevant genes. P < 0.05 for GO entries and KEGG pathways were considered significant.
Causal effect between serum vitamin D and polycystic ovary syndrome
In our bidirectional TS-MR analysis, the number of IVs of VD on PCOS and PCOS on VD were 90 and 2, respectively. The F-statistic values for each SNP were greater than 10 (Table S 3 ), indicating that the results were almost unaffected by weak instrumental bias. The result of fixed-effects IVW method (Cochran's Q statistic = 81.42, P = 0.704) indicated that genetically predicted higher level of VD led to a lower risk of developing PCOS after excluding obesity-associated SNPs ( n = 90 SNPs, OR = 0.750, 95% CI: 0.587–0.959, P = 0.022) (Table 1 ). MR-Egger, weighted median, and weighted mode methods all obtained similar magnitude and direction to IVW method (Table 1 ). The scatter plot demonstrates the inhibitory effect of individual SNP on PCOS (Fig. S 1 ). Since the MR-Egger P -intercept was greater than 0.05 (Table S 8 ) and the funnel plot (Fig. S 2 ) was roughly symmetrical, there was no indication of horizontal pleiotropy detected in the study. The results of the LOO analyses indicated that there were no potentially affecting SNPs in the main MR analyses (Fig. S 3 ). The result of the result of the MR-PRESSO test did not show any outlier SNPs. Nevertheless, the results of reverse TS-MR showed that genetically predicted risk of developing PCOS did not affect the VD level (fixed-IVW: n = 2 SNPs, OR = 1.004, 95% CI: 0.987–1.022, P = 0.640) (Table 1 ).
We subsequently explored the direct effect of the serum VD level on PCOS by MVMR methods, and the results of both Model 1 (adjusted BMI) and Model 2 (adjusted BMI, FBG, and FI) showed that the negative correlation between serum VD level and the risk of PCOS remained similar (Table 2 ). This confirms the robustness of the TS-MR results.
Mendelian randomization mediation analysis
After excluding the outlier SNPs and obesity-related SNPs, MVMR analysis (adjusted BT) revealed direct causal effects of serum VD level (OR: 0.735, 95% CI: 0.552–0.978; P = 0.035) on the risk of developing PCOS (Table 3 , Fig. 1 D). In the following steps of the MR mediation analysis, we found strong evidence for a causal effect of serum VD level (β: − 0.053, P = 0.026) on BT (Table 3 ). In addition to this, we also found a causal relationship between BT and PCOS (OR: 1.378, 95% CI: 1.123–1.691; P = 0.002) (Table 3 ).
Taken together, we found the potential mediation pathways between VD and PCOS: an indirect causal effect of VD on PCOS risk via BT (θ 3 × θ 4 ) (OR: 0.983, 95% CI: 0.968–0.998; P = 0.025) (Table 3 ). The pathway mediated 5.96% of the total causal effect of VD on PCOS risk. Detailed estimates of direct and indirect causal effects can be found in Table 3 .
Bioinformatics study
The results of the MR study suggested that reduced VD level may lead to the development of PCOS, and BT is a mediator between VD and PCOS, meaning that VD can ultimately influence the development of PCOS by affecting the production of testosterone. On the basis of the above studies, we collected IVs of VD-PCOS ( n -SNPs = 90) and VD-BT ( n -SNPs = 88) respectively, and combined these IVs with cis-eQTLs data for gene annotation respectively. Ultimately, 147 (VD-PCOS) and 164 (VD-BT) potentially relevant genes were annotated (Table S 9 - 10 ), respectively. We then used these genes to perform GO and KEGG analyses.
Firstly, the potentially relevant genes of VD-PCOS were analyzed for enrichment. The results of GO analysis suggested that these genes were mainly related to androgen metabolic process, superoxide metabolic process, cell body membrane, and steroid dehydrogenase activity (Fig. 2 A). The KEGG analysis was mainly enriched in the process of autophagy, steroid biosynthesis, cytochrome P450 metabolic process, and vitamin digestion and absorption process (Fig. 2 C). Subsequently, potentially relevant genes associated with VD-BT were analyzed for enrichment. The results of GO analysis suggested that these genes were mainly associated with steroid metabolism, superoxide metabolism, autophagosome membrane, nuclear androgen receptor binding, and vitamin transmembrane transporter activity (Fig. 2 B), and the KEGG analysis was mainly enriched for autophagy, steroid biosynthesis, vitamin digestion and absorption, and cholesterol metabolism process (Fig. 2 C). All information of the enrichment analysis is shown in the additional file (Table S 11 -S 12 ).
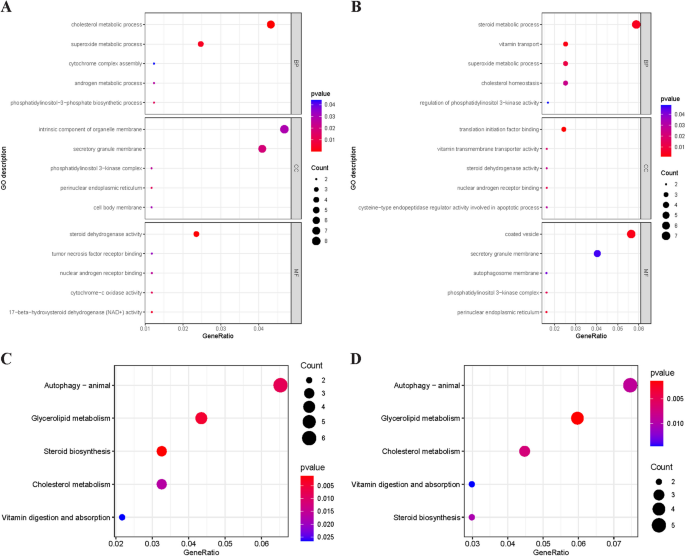
Gene Ontology and Kyoto Encyclopedia of the Genome pathway enrichment analysis of potentially relevant genes. A The GO enrichment analysis for potentially relevant genes related to VD and PCOS; ( B ) The GO enrichment analysis for potentially relevant genes related to VD and BT; ( C ). The KEGG pathway analysis for potentially relevant genes related to VD and PCOS; ( D ). The KEGG pathway analysis for potentially relevant genes related to VD and BT. Abbreviations: VD, vitamin D; PCOS, polycystic ovary syndrome; BT, bioavailable testosterone; GO, Gene Ontology; KEGG, Kyoto Encyclopedia of the Genome
In our bidirectional TS-MR analysis, we found that higher serum VD level was causally associated with a lower risk of developing PCOS (OR = 0.750, 95% CI: 0.587–0.959, P = 0.022), whereas there was little evidence for a causal effect of the risk of PCOS on the effect of serum VD level. Furthermore, our MR mediation analysis confirmed that testosterone can act as one of the mediating factors between the causality of VD and PCOS (OR = 0.983, 95% CI: 0.968–0.998, P = 0.025). The mediating effect of testosterone was 5.96%. Ultimately, we utilized potentially relevant genes for GO and KEGG enrichment analysis to assess the involvement of testosterone and the potential biological and molecular mechanisms between them.
VD, a lipid-soluble vitamin, plays a pivotal role in numerous biological processes. Primarily synthesized endogenously through exposure to sunlight, it is also acquired, albeit to a lesser extent, from dietary sources [ 48 ]. VDD is considered a globally prevalent nutritional deficiency, with various studies reporting prevalence rates of 58–91% among infertile women [ 49 ]. A cross-sectional study encompassing 625 women diagnosed with PCOS and 217 control subjects revealed that Chinese women diagnosed with PCOS exhibited notably lower level of VD compared to their healthy [ 50 ]. The result from a large observational study conducted by Krul-Poel et al. similarly demonstrated significantly diminished level of VD among women within the PCOS group [ 51 ]. Recent research has demonstrated that women with PCOS exhibit lower serum concentrations of VD compared to BMI-matched controls. This implies that the level of VD is linked to PCOS irrespective of BMI [ 14 ]. Aligned with the outcomes of these observational studies, our research indicated that higher serum VD level serves as a protective factor for the risk of PCOS. To eliminate the influence of obesity as a potential confounder on the results, we excluded obesity-related SNPs in our TS-MR analysis. Subsequently, in our MVMR analyses, we adjusted for genetically predicted BMI, FBG, and FI to explore the direct causal relationship between VD and PCOS. These stringent measures significantly enhance the credibility and robustness of our findings.
The precise mechanism through which serum VD operates on PCOS remains elusive. Hyperandrogenemia stands as a pivotal diagnostic criterion for PCOS. Numerous past studies have concentrated on exploring the correlation between serum VD and hyperandrogenemia in PCOS, yet the conclusions drawn from these studies have not reached a consensus. A study conducted by Latic N et al. revealed a negative correlation between serum VD level and testosterone in PCOS patients. Additionally, Menichini et al. demonstrated a positive impact of VD supplementation (4000 IU) on total testosterone [ 52 ]. However, a study by Mesinovic et al. suggested no discernible correlation between serum VD and androgens in individuals with PCOS [ 19 ]. Moreover, a large observational study by Gallea et al. also showcased associations between serum VD level, insulin, and body weight among PCOS patients but not specifically with hyperandrogenemia [ 20 ]. The inconsistencies observed in these findings might stem from variations in race, sample sizes, seasonal disparities, and the lifestyles of the included subjects. Our study, employing Mendelian randomization, effectively mitigated the impact of sample size, seasonal fluctuations, and diverse lifestyles on the outcomes. Furthermore, our research focused solely on individuals of European ethnicity, and we excluded BMI-related SNPs when incorporating instrumental variables, thereby significantly reducing BMI's potential confounding effect on the results. These measures ensured the robustness and reliability of our findings. Our results suggest that testosterone acts as a mediator between serum VD and PCOS, implying that serum VD may potentially contribute to the development of PCOS by influencing testosterone production.
The mechanism by which serum VD ultimately contributes to the development of PCOS by affecting testosterone remains unclear, but possible explanation has been proposed. Serum VD heightens the activity of aromatase within the ovary, thereby fostering the conversion of androgens to estrogens, ultimately culminating in diminished androgens production [ 53 ]. Kinuta et al. demonstrated a marked reduction in aromatase activity within the ovaries of VDR knockout mice in contrast to the control group [ 54 ]. In addition, we performed bioinformatics analysis to explore more possible biological mechanisms. Firstly, the results of GO and KEGG analyses of potentially related genes of VD-PCOS showed that steroid biosynthetic process, androgen metabolic process, and nuclear androgen receptor binding process were the possible biological mechanisms between the causality of the serum VD level and PCOS. These results are consistent with the results of our bidirectional TS-MR analysis, demonstrating again that the serum VD can ultimately influence the development of PCOS by modulating testosterone production. Subsequently, we subjected potentially relevant genes associated with VD-BT to bioinformatics analysis. The results suggested that autophagy process and superoxide metabolism process might be the biological mechanism between serum VD and testosterone.
There are very few studies linking autophagy to PCOS, and the results of these studies suggest that the development of PCOS is closely related to the process of autophagy [ 55 ]. Texada et al. showed that autophagy can regulate steroid production by modulating cholesterol transport in endocrine cells [ 56 ]. In addition to this, the role of VD-mediated autophagy in disease has been extensively studied, and basic study by Hu et al. showed that VD can mediate the regulation of autophagy function through gastric epithelial cell VD receptors, which ultimately affects the pathogenic effects of H. pylori [ 57 ]. However, whether VD can mediate autophagy ultimately leading to PCOS remains unknown. The results of the bioinformatics study in this study suggest that autophagy is most likely one of the important mechanisms underlying the relationship between VD and PCOS.
Our study has proved that lower serum VD level causes higher prevalence of PCOS. The latter could have oocyte competence and endometrial function impaired [ 9 , 10 ], but also cause a few adverse outcomes related to reproduction, such as infertility, miscarriage, and premature delivery [ 12 , 13 ]. It has been found that VDD could decrease the rates of ovulation and success pregnancy in the PCOS patients, leading to less live birth [ 58 ]. In addition, It has been reported that serum VD level was independent predicting factor for live birth in the PCOS patients received ovulati0on induction [ 59 ]. Yasmine et al. have reported that endometrial thickness of PCOS patients maybe improved after VD administration [ 60 ]. A recent meta-analysis has shown that VD supplementation to PCOS women could decrease the occurrence rates of early miscarriage and premature delivery [ 53 ]. The nuclear receptor of VD (VDR) and 1,25(OH)2D3 membrane binding protein are expressed in both ovarian granulosa and theca cells [ 61 , 62 ]. It has been found that VD can regulate the expression of enzymes in the VDR and ovary, ultimately regulating ovarian function [ 63 ]. One study showed that VDR mRNA was significantly less expressed in granulosa cells of the women with PCOS [ 64 ]. It may cause PCOS patients to be more sensitive to VDD. Based on the above studies and ours, serum VD level need be monitored in the female population, especially in the women of reproductive age, and timely VD administration in PCOS patients would help to improve their reproductive function and pregnancy outcomes.
Our research has several advantages. Primarily, this study confirms the direct causal relationship of the serum VD level on the risk of PCOS through the utilization of the TS-MR analysis method. This method avoids the limitation commonly found in most observational studies, thereby fortifying the reliability and validity of our finding. Secondly, we ascertain the mediating function of testosterone in the relationship between serum VD and PCOS via MR mediation analysis, thus laying the groundwork for subsequent mechanistic studies. Finally, this is the first study to combine MR studies and bioinformatics analyses together to explore causal relationship and potential functional mechanisms between serum VD level, testosterone, and the risk of PCOS, which is quite different from other studies. Nonetheless, this study also has limitations. Firstly, our study failed to capture dietary and sun exposure information that may affect serum VD level. Secondly, the use of exclusively European data in a MR analysis may not be generalizable to other ethnic populations, albeit reducing the impact of ethnicity bias on the study outcomes. Finally, the absence of relevant data prevented us from independently exploring the relationship of serum VD 2 /D 3 with the risk of PCOS, warranting further investigation.
Conclusions
In conclusion, our studies confirm the causality between lower serum VD level and higher risk of PCOS. Furthermore, testosterone may act as a mediator between serum VD and PCOS. These findings emphasize the clinical importance of testing serum VD level and timely VD supplementation as possible primary prevention and treatment of PCOS.
Availability of data and materials
No datasets were generated or analysed during the current study.
Abbreviations
- Polycystic ovary syndrome
Genome-wide association studies
Two-sample Mendelian randomization
Cis-expression quantitative loci
VD deficiency
VD receptors
Body mass index
- Mendelian randomization
Multivariable MR
Instrumental variables
Bioavailable testosterone
Fasting glucose
Fasting insulin
Single-nucleotide polymorphisms
Waist circumference
Inverse variance weighted
Leave one out
Gene ontology
Kyoto Encyclopedia of Genes and Genomes
Biological process
Molecular function
Cellular composition
Holick MF. The vitamin D deficiency pandemic: Approaches for diagnosis, treatment and prevention. Rev Endocr Metab Disord. 2017;18:153–65.
Article CAS PubMed Google Scholar
Autier P, Boniol M, Pizot C, Mullie P. Vitamin D status and ill health: a systematic review. Lancet Diabetes Endocrinol. 2014;2:76–89.
Lerchbaum E, Obermayer-Pietsch B. Vitamin D and fertility: a systematic review. Eur J Endocrinol. 2012;166:765–78.
Irani M, Merhi Z. Role of vitamin D in ovarian physiology and its implication in reproduction: a systematic review. Fertil Steril. 2014;102:460-468.e3.
Parikh G, Varadinova M, Suwandhi P, Araki T, Rosenwaks Z, Poretsky L, et al. Vitamin D regulates steroidogenesis and insulin-like growth factor binding protein-1 (IGFBP-1) production in human ovarian cells. Horm Metab Res. 2010;42:754–7.
Du H, Daftary GS, Lalwani SI, Taylor HS. Direct regulation of HOXA10 by 1,25-(OH)2D3 in human myelomonocytic cells and human endometrial stromal cells. Mol Endocrinol. 2005;19:2222–33.
Tyrmi JS, Arffman RK, Pujol-Gualdo N, Kurra V, Morin-Papunen L, Sliz E, et al. Leveraging Northern European population history: novel low-frequency variants for polycystic ovary syndrome. Hum Reprod. 2022;37:352–65.
Article PubMed Google Scholar
Bruni V, Capozzi A, Lello S. The Role of Genetics, Epigenetics and Lifestyle in Polycystic Ovary Syndrome Development: the State of the Art. Reprod Sci. 2022;29:668–79.
Palomba S, Daolio J, La Sala GB. Oocyte Competence in Women with Polycystic Ovary Syndrome. Trends Endocrinol Metab. 2017;28:186–98.
Palomba S, Piltonen TT, Giudice LC. Endometrial function in women with polycystic ovary syndrome: a comprehensive review. Hum Reprod Update. 2021;27:584–618.
Norman RJ, Dewailly D, Legro RS, Hickey TE. Polycystic ovary syndrome. Lancet. 2007;370:685–97.
Palomba S. Is fertility reduced in ovulatory women with polycystic ovary syndrome? An opinion paper Hum Reprod. 2021;36:2421–8.
CAS PubMed Google Scholar
Palomba S, De Wilde MA, Falbo A, Koster MPH, La Sala GB, Fauser BCJM. Pregnancy complications in women with polycystic ovary syndrome. Hum Reprod Update. 2015;21:575–92.
Bacopoulou F, Kolias E, Efthymiou V, Antonopoulos CN, Charmandari E. Vitamin D predictors in polycystic ovary syndrome: a meta-analysis. Eur J Clin Invest. 2017;47:746–55.
Lejman-Larysz K, Golara A, Baranowska M, Kozłowski M, Guzik P, Szydłowska I, et al. Influence of Vitamin D on the Incidence of Metabolic Syndrome and Hormonal Balance in Patients with Polycystic Ovary Syndrome. Nutrients. 2023;15:2952.
Article CAS PubMed PubMed Central Google Scholar
Durdiakova J, Ostatnikova D, Celec P. Testosterone and its metabolites–modulators of brain functions. Acta Neurobiol Exp (Warsz). 2011;71:434–54.
Hahn S, Haselhorst U, Tan S, Quadbeck B, Schmidt M, Roesler S, et al. Low serum 25-hydroxyvitamin D concentrations are associated with insulin resistance and obesity in women with polycystic ovary syndrome. Exp Clin Endocrinol Diabetes. 2006;114:577–83.
Latic N, Erben RG. Vitamin D and Cardiovascular Disease, with Emphasis on Hypertension, Atherosclerosis, and Heart Failure. Int J Mol Sci. 2020;21:6483.
Mesinovic J, Teede HJ, Shorakae S, Lambert GW, Lambert EA, Naderpoor N, et al. The Relationship between Vitamin D Metabolites and Androgens in Women with Polycystic Ovary Syndrome. Nutrients. 2020;12:1219.
Gallea M, Granzotto M, Azzolini S, Faggian D, Mozzanega B, Vettor R, et al. Insulin and body weight but not hyperandrogenism seem involved in seasonal serum 25-OH-vitamin D3 levels in subjects affected by PCOS. Gynecol Endocrinol. 2014;30:739–45.
Smith GD, Lawlor DA, Harbord R, Timpson N, Day I, Ebrahim S. Clustered environments and randomized genes: a fundamental distinction between conventional and genetic epidemiology. Cardon L, editor. PLoS Med. 2007;4:e352.
Article PubMed PubMed Central Google Scholar
Davey Smith G, Hemani G. Mendelian randomization: genetic anchors for causal inference in epidemiological studies. Hum Mol Genet. 2014;23:R89-98.
EPIC- InterAct Consortium, Burgess S, Scott RA, Timpson NJ, Davey Smith G, Thompson SG. Using published data in Mendelian randomization: a blueprint for efficient identification of causal risk factors. Eur J Epidemiol. 2015;30:543–52.
Article PubMed Central Google Scholar
Skrivankova VW, Richmond RC, Woolf BAR, Yarmolinsky J, Davies NM, Swanson SA, et al. Strengthening the Reporting of Observational Studies in Epidemiology Using Mendelian Randomization: The STROBE-MR Statement. JAMA. 2021;326:1614.
Revez JA, Lin T, Qiao Z, Xue A, Holtz Y, Zhu Z, et al. Genome-wide association study identifies 143 loci associated with 25 hydroxyvitamin D concentration. Nat Commun. 2020;11:1647.
Mbatchou J, Barnard L, Backman J, Marcketta A, Kosmicki JA, Ziyatdinov A, et al. Computationally efficient whole-genome regression for quantitative and binary traits. Nat Genet. 2021;53:1097–103.
Chen J, Spracklen CN, Marenne G, Varshney A, Corbin LJ, Luan J, et al. The trans-ancestral genomic architecture of glycemic traits. Nat Genet. 2021;53:840–60.
Yengo L, Sidorenko J, Kemper KE, Zheng Z, Wood AR, Weedon MN, et al. Meta-analysis of genome-wide association studies for height and body mass index in ∼ 700000 individuals of European ancestry. Hum Mol Genet. 2018;27:3641–9.
Verbanck M, Chen C-Y, Neale B, Do R. Detection of widespread horizontal pleiotropy in causal relationships inferred from Mendelian randomization between complex traits and diseases. Nat Genet. 2018;50:693–8.
Mortada I. Hyperuricemia, Type 2 Diabetes Mellitus, and Hypertension: an Emerging Association. Curr Hypertens Rep. 2017;19:69.
Choi HK, McCormick N, Lu N, Rai SK, Yokose C, Zhang Y. Population Impact Attributable to Modifiable Risk Factors for Hyperuricemia. Arthritis Rheumatol. 2020;72:157–65.
Nakamura K, Sakurai M, Miura K, Morikawa Y, Yoshita K, Ishizaki M, et al. Alcohol intake and the risk of hyperuricaemia: a 6-year prospective study in Japanese men. Nutr Metab Cardiovasc Dis. 2012;22:989–96.
Greco MFD, Minelli C, Sheehan NA, Thompson JR. Detecting pleiotropy in Mendelian randomisation studies with summary data and a continuous outcome. Stat Med. 2015;34:2926–40.
Article Google Scholar
Hemani G, Zheng J, Elsworth B, Wade KH, Haberland V, Baird D, et al. The MR-base platform supports systematic causal inference across the human phenome. eLife. 2018;7:e34408.
Sanderson E, Spiller W, Bowden J. Testing and correcting for weak and pleiotropic instruments in two-sample multivariable Mendelian randomization. Stat Med. 2021;40:5434–52.
Sanderson E, Davey Smith G, Windmeijer F, Bowden J. An examination of multivariable Mendelian randomization in the single-sample and two-sample summary data settings. Int J Epidemiol. 2019;48:713–27.
VanderWeele TJ. Mediation Analysis: A Practitioner’s Guide. Annu Rev Public Health. 2016;37:17–32.
MacKinnon DP, Fairchild AJ, Fritz MS. Mediation analysis. Annu Rev Psychol. 2007;58:593–614.
Bowden J, Davey Smith G, Burgess S. Mendelian randomization with invalid instruments: effect estimation and bias detection through Egger regression. Int J Epidemiol. 2015;44:512–25.
Burgess S, Thompson SG. Interpreting findings from Mendelian randomization using the MR-Egger method. Eur J Epidemiol. 2017;32:377–89.
Bowden J, Davey Smith G, Haycock PC, Burgess S. Consistent Estimation in Mendelian Randomization with Some Invalid Instruments Using a Weighted Median Estimator. Genet Epidemiol. 2016;40:304–14.
Hartwig FP, Davey Smith G, Bowden J. Robust inference in summary data Mendelian randomization via the zero modal pleiotropy assumption. Int J Epidemiol. 2017;46:1985–98.
Consistent Estimation in Mendelian Randomization with Some Invalid Instruments Using a Weighted Median Estimator - PubMed. Available from: https://pubmed.ncbi.nlm.nih.gov/27061298/ . [cited 2023 Feb 15].
Burgess S, Bowden J, Fall T, Ingelsson E, Thompson SG. Sensitivity Analyses for Robust Causal Inference from Mendelian Randomization Analyses with Multiple Genetic Variants. Epidemiology. 2017;28:30–42.
Kamat MA, Blackshaw JA, Young R, Surendran P, Burgess S, Danesh J, et al. PhenoScanner V2: an expanded tool for searching human genotype-phenotype associations. Bioinformatics. 2019;35:4851–3.
Võsa U, Claringbould A, Westra H-J, Bonder MJ, Deelen P, Zeng B, et al. Large-scale cis- and trans-eQTL analyses identify thousands of genetic loci and polygenic scores that regulate blood gene expression. Nat Genet. 2021;53:1300–10.
The Gene Ontology Consortium. Gene Ontology Consortium: going forward. Nucleic Acids Res. 2015;43:D1049–56.
Yuan C, Qian ZR, Babic A, Morales-Oyarvide V, Rubinson DA, Kraft P, et al. Prediagnostic Plasma 25-Hydroxyvitamin D and Pancreatic Cancer Survival. J Clin Oncol. 2016;34:2899–905.
Cunningham TK, Allgar V, Dargham SR, Kilpatrick E, Sathyapalan T, Maguiness S, et al. Association of Vitamin D Metabolites With Embryo Development and Fertilization in Women With and Without PCOS Undergoing Subfertility Treatment. Front Endocrinol. 2019;10:13.
Shan C, Zhu Y, Yu J, Zhang Y, Wang Y, Lu N, et al. Low Serum 25-Hydroxyvitamin D Levels Are Associated With Hyperandrogenemia in Polycystic Ovary Syndrome: A Cross-Sectional Study. Front Endocrinol. 2022;13:894935.
Krul-Poel YHM, Koenders PP, Steegers-Theunissen RP, Ten Boekel E, Wee MMT, Louwers Y, et al. Vitamin D and metabolic disturbances in polycystic ovary syndrome (PCOS): a cross-sectional study. Narayanan R, editor. PLOS One. 2018;13:e0204748.
Menichini D, Facchinetti F. Effects of vitamin D supplementation in women with polycystic ovary syndrome: a review. Gynecol Endocrinol. 2020;36:1–5.
Yang M, Shen X, Lu D, Peng J, Zhou S, Xu L, et al. Effects of vitamin D supplementation on ovulation and pregnancy in women with polycystic ovary syndrome: a systematic review and meta-analysis. Front Endocrinol. 2023;14:1148556.
Kinuta K, Tanaka H, Moriwake T, Aya K, Kato S, Seino Y. Vitamin D is an important factor in estrogen biosynthesis of both female and male gonads. Endocrinology. 2000;141:1317–24.
Kumariya S, Ubba V, Jha RK, Gayen JR. Autophagy in ovary and polycystic ovary syndrome: role, dispute and future perspective. Autophagy. 2021;17:2706–33.
Texada MJ, Malita A, Rewitz K. Autophagy regulates steroid production by mediating cholesterol trafficking in endocrine cells. Autophagy. 2019;15:1478–80.
Hu W, Zhang L, Li MX, Shen J, Liu XD, Xiao ZG, et al. Vitamin D3 activates the autolysosomal degradation function against Helicobacter pylori through the PDIA3 receptor in gastric epithelial cells. Autophagy. 2019;15:707–25.
Butts SF, Seifer DB, Koelper N, Senapati S, Sammel MD, Hoofnagle AN, et al. Vitamin D Deficiency Is Associated With Poor Ovarian Stimulation Outcome in PCOS but Not Unexplained Infertility. J Clin Endocrinol Metab. 2019;104:369–78.
Pal L, Zhang H, Williams J, Santoro NF, Diamond MP, Schlaff WD, et al. Vitamin D Status Relates to Reproductive Outcome in Women With Polycystic Ovary Syndrome: Secondary Analysis of a Multicenter Randomized Controlled Trial. J Clin Endocrinol Metab. 2016;101:3027–35.
Abuzeid Y. Impact of Vitamin D Deficiency on Reproductive Outcome in infertile anovulatory women with polycystic ovary syndrome: a systematic literature review. Curr Dev Nutr. 2020;4:nzaa067_001.
Li S, Qi J, Sun Y, Gao X, Ma J, Zhao S. An integrated RNA-Seq and network study reveals that valproate inhibited progesterone production in human granulosa cells. J Steroid Biochem Mol Biol. 2021;214:105991.
Hrabia A, Kamińska K, Socha M, Grzesiak M. Vitamin D3 Receptors and Metabolic Enzymes in Hen Reproductive Tissues. Int J Mol Sci. 2023;24:17074.
Xu J, Lawson MS, Xu F, Du Y, Tkachenko OY, Bishop CV, et al. Vitamin D3 Regulates Follicular Development and Intrafollicular Vitamin D Biosynthesis and Signaling in the Primate Ovary. Front Physiol. 2018;9:1600.
Aghadavod E, Mollaei H, Nouri M, Hamishehkar H. Evaluation of Relationship between Body Mass Index with Vitamin D Receptor Gene Expression and Vitamin D Levels of Follicular Fluid in Overweight Patients with Polycystic Ovary Syndrome. Int J Fertil Steril. 2017;11:105–11.
CAS PubMed PubMed Central Google Scholar
Download references
Acknowledgements
We would like to express our sincere gratitude to the compilers of the GWAS summary dataset for their management of the data collection and data resources.
This work was supported by the General Program of National Natural Science Foundation of China (grant number No.81771741), Distinguished Professor at Educational Department of Liaoning Province (grant number No. [2014]187) to JL.
Author information
Authors and affiliations.
Department of Endocrinology and Metabolism, The Institute of Endocrinology, NHC Key Laboratory of Diagnosis and Treatment of Thyroid Diseases, The First Affiliated Hospital of China Medical University, Shenyang, Liaoning, 110000, P.R. China
Bingrui Gao, Chenxi Zhang, Deping Wang, Bojuan Li, Zhongyan Shan, Weiping Teng & Jing Li
Department of Endocrinology and Metabolism, Hongqi Hospital Affiliated to Mudanjiang Medical College, Mudanjiang, Heilongjiang, 157011, P.R. China
Deping Wang
You can also search for this author in PubMed Google Scholar
Contributions
Designed the study: Jing Li, Bingrui Gao; Collected data: Bingrui Gao, Chenxi Zhang; Performed statistical analyses: Bingrui Gao, Deping Wang, Bojuan Li; Drafted the manuscript: Bingrui Gao; Supervised the study and reviewed the manuscript: Jing Li, Zhongyan Shan, Weiping Teng.
Corresponding author
Correspondence to Jing Li .
Ethics declarations
Ethics approval and consent to participate.
Our analysis used publicly available genome-wide association study (GWAS) summary statistics. No new data were collected, and no new ethical approval was required.
Consent for publication
Not applicable.
Competing interests
The authors declare no competing interests.
Additional information
Publisher’s note.
Springer Nature remains neutral with regard to jurisdictional claims in published maps and institutional affiliations.
Supplementary Information
Additional file 1: table s1..
STROBE-MR Checklist; Table S2. Key characteristics of participating studies; Table S3. GWAS significant SNPs used as genetic instruments for VD level on PCOS; Table S4. GWAS significant SNPs used as genetic instruments for PCOS on VD level; Table S5. GWAS significant SNPs used as genetic instruments for VD level on BT; Table S6. GWAS significant SNPs used as genetic instruments for BT on PCOS; Table S7. GWAS significant SNPs used as genetic instruments for BT and VD level on PCOS; Table S8. Heterogeneity and directional pleiotropy test using MR-Egger intercepts; Table S9. Potentially relevant genes corresponding to IVs associated with VD and PCOS; Table S10. Potentially relevant genes corresponding to IVs associated with VD and PCOS; Table S11. GO and KEGG enrichment analysis for potentially relevant genes related to VD and PCOS; Table S12. GO and KEGG enrichment analysis for potentially relevant genes related to VD and BT; Figure S1. Scatter plot of the MR estimates for the association of VD level with PCOS; Figure S2. Funnel plot reveals overall heterogeneity of the impact of VD on PCOS; Figure S3. Leave-one-out analysis of the impact of the VD on PCOS.
Rights and permissions
Open Access This article is licensed under a Creative Commons Attribution 4.0 International License, which permits use, sharing, adaptation, distribution and reproduction in any medium or format, as long as you give appropriate credit to the original author(s) and the source, provide a link to the Creative Commons licence, and indicate if changes were made. The images or other third party material in this article are included in the article's Creative Commons licence, unless indicated otherwise in a credit line to the material. If material is not included in the article's Creative Commons licence and your intended use is not permitted by statutory regulation or exceeds the permitted use, you will need to obtain permission directly from the copyright holder. To view a copy of this licence, visit http://creativecommons.org/licenses/by/4.0/ . The Creative Commons Public Domain Dedication waiver ( http://creativecommons.org/publicdomain/zero/1.0/ ) applies to the data made available in this article, unless otherwise stated in a credit line to the data.
Reprints and permissions
About this article
Cite this article.
Gao, B., Zhang, C., Wang, D. et al. Causal association between low vitamin D and polycystic ovary syndrome: a bidirectional mendelian randomization study. J Ovarian Res 17 , 95 (2024). https://doi.org/10.1186/s13048-024-01420-5
Download citation
Received : 23 February 2024
Accepted : 20 April 2024
Published : 07 May 2024
DOI : https://doi.org/10.1186/s13048-024-01420-5
Share this article
Anyone you share the following link with will be able to read this content:
Sorry, a shareable link is not currently available for this article.
Provided by the Springer Nature SharedIt content-sharing initiative
- Testosterone
Journal of Ovarian Research
ISSN: 1757-2215
- General enquiries: [email protected]
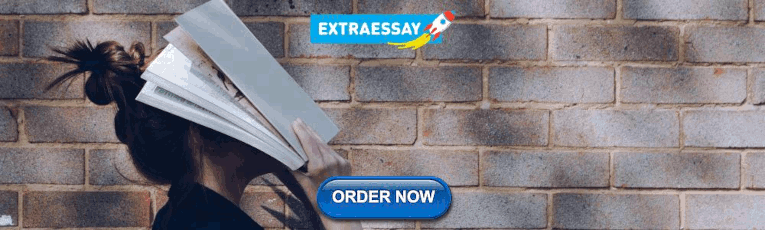
IMAGES
VIDEO
COMMENTS
Quantitative research is the opposite of qualitative research, which involves collecting and analyzing non-numerical data (e.g., text, video, or audio). Quantitative research is widely used in the natural and social sciences: biology, chemistry, psychology, economics, sociology, marketing, etc. Quantitative research question examples
Quantitative research is the process of collecting and analyzing numerical data to describe, predict, or control variables of interest. This type of research helps in testing the causal relationships between variables, making predictions, and generalizing results to wider populations. The purpose of quantitative research is to test a predefined ...
INTRODUCTION. Scientific research is usually initiated by posing evidenced-based research questions which are then explicitly restated as hypotheses.1,2 The hypotheses provide directions to guide the study, solutions, explanations, and expected results.3,4 Both research questions and hypotheses are essentially formulated based on conventional theories and real-world processes, which allow the ...
Quantitative Research. Quantitative research is a type of research that collects and analyzes numerical data to test hypotheses and answer research questions.This research typically involves a large sample size and uses statistical analysis to make inferences about a population based on the data collected.
Quantitative Research Examples. Some examples of quantitative research are: A customer satisfaction template can be used if any organization would like to conduct a customer satisfaction (CSAT) survey. Through this kind of survey, an organization can collect quantitative data and metrics on the goodwill of the brand or organization in the ...
Quantitative research is a type of research that focuses on collecting and analyzing numerical data to answer research questions. There are two main methods used to conduct quantitative research: 1. Primary Method. There are several methods of primary quantitative research, each with its own strengths and limitations.
Social scientists are concerned with the study of people. Quantitative research is a way to learn about a particular group of people, known as a sample population. Using scientific inquiry, quantitative research relies on data that are observed or measured to examine questions about the sample population. Allen, M. (2017). The SAGE encyclopedia ...
Quantitative research is the opposite of qualitative research, which involves collecting and analysing non-numerical data (e.g. text, video, or audio). Quantitative research is widely used in the natural and social sciences: biology, chemistry, psychology, economics, sociology, marketing, etc. Quantitative research question examples
This type of research can be used to establish generalisable facts about a topic. Common quantitative methods include experiments, observations recorded as numbers, and surveys with closed-ended questions. Qualitative research. Qualitative research is expressed in words. It is used to understand concepts, thoughts or experiences.
The four examples we just saw were simple hypothetical quantitative research examples. Now, let us see some real-life examples of quantitative research. Example #5. In 2015, researchers conducted an experimental study on the effect of lack of sleep on colds. The study was a two-part experiment conducted on 164 healthy individuals.
Quantitative research methods are concerned with the planning, design, and implementation of strategies to collect and analyze data. Descartes, the seventeenth-century philosopher, suggested that how the results are achieved is often more important than the results themselves, as the journey taken along the research path is a journey of discovery. . High-quality quantitative research is ...
Quantitative Research: Focus: Quantitative research focuses on numerical data, seeking to quantify variables and examine relationships between them. It aims to provide statistical evidence and generalize findings to a larger population. Measurement: Quantitative research involves standardized measurement instruments, such as surveys or questionnaires, to collect data.
Proven Examples of Quantitative Research Methods. Below, we discuss two excellent examples of quantitative research methods that were used by highly distinguished business and consulting organizations. Both examples show how different types of analysis can be performed with qualitative approaches and how the analysis is done once the data is ...
Quantitative research is a research strategy that focuses on quantifying the collection and analysis of data. It is formed from a deductive approach where emphasis is placed on the testing of theory, ... For example, Kuhn argued that within quantitative research, the results that are shown can prove to be strange. ...
me motivated and keen to study the research problem more. Thank you to my graduate advisor Cathy Levkulic. Your help was very crucial for a smooth Masters experience. Thank you, Dr. Michael Goodman for all your support during the two years of my Master's degree. I would also like to take the opportunity to appreciate all my professors.
In quantitative research, a variable is something (an intervention technique, a pharmaceutical, a temperature, etc.) that changes. There are two kinds of variables: independent variables and dependent variables.In the simplest terms, the independent variable is whatever the researchers are using to attempt to make a change in their dependent variable.
Mixed-methods research is a flexible approach, where the research design is determined by what we want to find out rather than by any predetermined epistemological position. In mixed-methods research, qualitative or quantitative components can predominate, or both can have equal status. 1.4. Units and variables.
Quantitative research designs tend to be more fixed and deductive, with variables and hypotheses clearly defined in advance of data collection. Quantitative research example If you want to test the effectiveness of an online teaching method, a quantitative approach is most suitable. You can use this type of research to measure learning outcomes ...
Quantitative methods emphasize objective measurements and the statistical, mathematical, or numerical analysis of data collected through polls, questionnaires, and surveys, or by manipulating pre-existing statistical data using computational techniques.Quantitative research focuses on gathering numerical data and generalizing it across groups of people or to explain a particular phenomenon.
Qualitative research aims to produce rich and detailed descriptions of the phenomenon being studied, and to uncover new insights and meanings. Quantitative data is information about quantities, and therefore numbers, and qualitative data is descriptive, and regards phenomenon which can be observed but not measured, such as language.
What is quantitative research? How does it work? Learn more in our in-depth guide to quantitative analysis.
Quantitative research involves collecting and analyzing numerical data to identify patterns, trends, and relationships among variables. This method is widely used in social sciences, psychology, economics, and other fields where researchers aim to understand human behavior and phenomena through statistical analysis. If you are looking for a quantitative research topic, there are numerous areas ...
What quantitative data analysis is not. But as powerful as quantitative data analysis is, it's not without its limitations. It only gives you the what, not the why. For example, it can tell you how many website visitors or conversions you have on an average day, but it can't tell you why users visited your site or made a purchase.. For the why behind user behavior, you need qualitative ...
Original quantitative research - Substance-related poisoning hospitalizations and homelessness in Canada: a descriptive study ... For example, individuals with less severe poisonings may be treated through emergency medical services or in the emergency department; not including these health care settings could lead to underestimating the ...
The coastal city criteria developed depending on the examples of coastal cities in the world were evaluated by surveying local people and experts. ... A quantitative priority-based approach using a multi-criteria decision-making model for sustainable coastal city development ... International Journal of Logistics Research and Applications, 27(3 ...
Background Cerebral Visual Impairment (CVI) is the most common cause of low vision in children. Standardized, quantifiable measures of visual function are needed. Objective This study developed and evaluated a new method for quantifying visual function in young and medically complex children with CVI using remote videoconferencing. Methods Children diagnosed with CVI who had been unable to ...
Background Recent studies have revealed the correlation between serum vitamin D (VD) level and polycystic ovary syndrome (PCOS), but the causality and specific mechanisms remain uncertain. Objective We aimed to investigate the cause-effect relationship between serum VD and PCOS, and the role of testosterone in the related pathological mechanisms. Methods We assessed the causality between serum ...