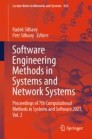
Proceedings of the Computational Methods in Systems and Software
CoMeSySo 2023: Software Engineering Methods in Systems and Network Systems pp 482–491 Cite as
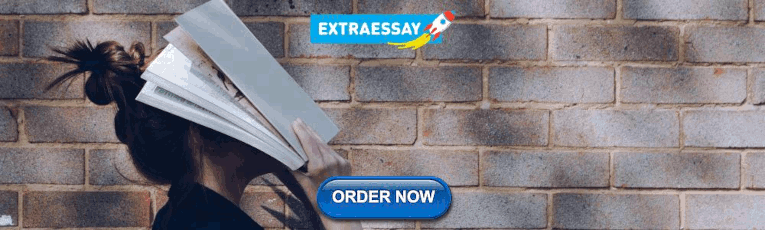
A Review Paper on Biometric Authentication and Geospatial Data – Case of Zambia
- Jeremiah Mwiinga 11 &
- Jackson Phiri 12
- Conference paper
- First Online: 28 February 2024
52 Accesses
Part of the book series: Lecture Notes in Networks and Systems ((LNNS,volume 934))
In the current global landscape, security concerns have reached unprecedented heights, necessitating innovative approaches to combat threats ranging from cybercrime to data breaches. While this paper primarily focuses on the potential of biometric authentication in enhancing security, it also explores the integration of geospatial data to bolster its efficacy further.
This is a preview of subscription content, log in via an institution .
Buying options
- Available as PDF
- Read on any device
- Instant download
- Own it forever
- Available as EPUB and PDF
- Compact, lightweight edition
- Dispatched in 3 to 5 business days
- Free shipping worldwide - see info
Tax calculation will be finalised at checkout
Purchases are for personal use only
Bolle, R.M., Connell, J.H., Pankanti, S., Ratha, N.K., Senior, A.W.: Guide to Biometrics. Springer, Cham (2015). https://doi.org/10.1007/978-1-4757-4036-3
Desai, N., Dhameliya, K., Desai, V.: Feature extraction and classification techniques for speech recognition: a review. Int. J. Emerg. Technol. Adv. Eng. 3 (12), 367–371 (2013)
Google Scholar
Kaur, M., Singh, M., Girdhar, A., Sandhu, P.S.: Fingerprint verification system using minutiae extraction technique. Int. J. Comput. Inf. Eng. 2 (10), 3405–3410 (2008)
Kirti, D., Sharma, S.: Face detection from few training examples. In: 7th International Conference on Cloud Computing Cloud Computing, Data Science & Engineering, Noida, India, pp. 629–633 (2017)
Naeem, M., Qureshi, I., Azam, F.: Face recognition techniques and approaches: a survey. Sci. Int. 27 (1), 301–305 (2015)
Malathi, S., Meena, C.: An efficient method for partial fingerprint recognition based on Local Binary Pattern. In: Proceedings of Communication Control and Computing Technologies, pp. 569–572. IEEE (2010)
Parmar, D.N., Mehta, B.B.: Face recognition methods & applications. Int. J. Comput. Technol. Appl. 4 (1), 84–86 (2013)
Singh, C.S., Manikandan, M.: Design and Implementation of face detection using ad boost algorithm. J. Theor. Appl. Inf. Technol. 65 (3), 707–714 (2014)
Download references
Author information
Authors and affiliations.
Department of Computer Science, University of Zambia, Lusaka, Zambia
Jeremiah Mwiinga
School of Natural Sciences, Department of Computer Science, University of Zambia, Lusaka, Zambia
Jackson Phiri
You can also search for this author in PubMed Google Scholar
Corresponding author
Correspondence to Jeremiah Mwiinga .
Editor information
Editors and affiliations.
Faculty of Applied Informatics, Tomas Bata University in Zlin, Zlin, Czech Republic
Radek Silhavy
Petr Silhavy
Rights and permissions
Reprints and permissions
Copyright information
© 2024 The Author(s), under exclusive license to Springer Nature Switzerland AG
About this paper
Cite this paper.
Mwiinga, J., Phiri, J. (2024). A Review Paper on Biometric Authentication and Geospatial Data – Case of Zambia. In: Silhavy, R., Silhavy, P. (eds) Software Engineering Methods in Systems and Network Systems. CoMeSySo 2023. Lecture Notes in Networks and Systems, vol 934. Springer, Cham. https://doi.org/10.1007/978-3-031-54813-0_42
Download citation
DOI : https://doi.org/10.1007/978-3-031-54813-0_42
Published : 28 February 2024
Publisher Name : Springer, Cham
Print ISBN : 978-3-031-54812-3
Online ISBN : 978-3-031-54813-0
eBook Packages : Intelligent Technologies and Robotics Intelligent Technologies and Robotics (R0)
Share this paper
Anyone you share the following link with will be able to read this content:
Sorry, a shareable link is not currently available for this article.
Provided by the Springer Nature SharedIt content-sharing initiative
- Publish with us
Policies and ethics
- Find a journal
- Track your research
Biometric Authentication Security: An Overview
Ieee account.
- Change Username/Password
- Update Address
Purchase Details
- Payment Options
- Order History
- View Purchased Documents
Profile Information
- Communications Preferences
- Profession and Education
- Technical Interests
- US & Canada: +1 800 678 4333
- Worldwide: +1 732 981 0060
- Contact & Support
- About IEEE Xplore
- Accessibility
- Terms of Use
- Nondiscrimination Policy
- Privacy & Opting Out of Cookies
A not-for-profit organization, IEEE is the world's largest technical professional organization dedicated to advancing technology for the benefit of humanity. © Copyright 2024 IEEE - All rights reserved. Use of this web site signifies your agreement to the terms and conditions.
Help | Advanced Search
Computer Science > Cryptography and Security
Title: advancements in biometric technology with artificial intelligence.
Abstract: Authentication plays a significant part in dealing with security in public and private sectors such as healthcare systems, banking system, transportation system and law and security. Biometric technology has grown quickly recently, especially in the areas of artificial intelligence and identity. Formerly, authentication process has depended on security measures like passcodes, identity fobs, and fingerprints. On the other hand, as just a consequence of these precautions, theft has increased in frequency. In response, biometric security was created, in which the identification of a person is based on features derived from the physiological and behavioral traits of a human body using biometric system. Biometric technology gadgets are available to the public as they are embedded on computer systems, electronic devices, mobile phones, and other consumer electronics. As the fraudulent is increasing demand and use of biometric electronic devices has increased. As a consequence, it may be possible to confirm a person's distinct identification. The goal of this study is to examine developments in biometric systems in the disciplines of medicine and engineering. The study will present the perspectives and different points of view of the secondary data, highlighting the need for more in-depth understanding and application of biometric technology to promote its development in the digital era. The study's findings may inspire people and businesses to more effectively incorporate biometric technologies in order to reduce the risks to data and identity security.
Submission history
Access paper:.
- Other Formats

References & Citations
- Google Scholar
- Semantic Scholar
BibTeX formatted citation

Bibliographic and Citation Tools
Code, data and media associated with this article, recommenders and search tools.
- Institution
arXivLabs: experimental projects with community collaborators
arXivLabs is a framework that allows collaborators to develop and share new arXiv features directly on our website.
Both individuals and organizations that work with arXivLabs have embraced and accepted our values of openness, community, excellence, and user data privacy. arXiv is committed to these values and only works with partners that adhere to them.
Have an idea for a project that will add value for arXiv's community? Learn more about arXivLabs .

An official website of the United States government
The .gov means it's official. Federal government websites often end in .gov or .mil. Before sharing sensitive information, make sure you're on a federal government site.
The site is secure. The https:// ensures that you are connecting to the official website and that any information you provide is encrypted and transmitted securely.
- Publications
- Account settings
- Browse Titles
NCBI Bookshelf. A service of the National Library of Medicine, National Institutes of Health.
National Research Council (US) Whither Biometrics Committee; Pato JN, Millett LI, editors. Biometric Recognition: Challenges and Opportunities. Washington (DC): National Academies Press (US); 2010.

Biometric Recognition: Challenges and Opportunities.
- Hardcopy Version at National Academies Press
5 Research Opportunities and the Future of Biometrics
The first four chapters of this report explain much about biometric systems and applications and describe many of the technical, engineering, scientific, and social challenges facing the field. This chapter covers some of the unsolved fundamental problems and research opportunities related to biometric systems, without, however, suggesting that existing systems are not useful or effective. In fact, many biometric systems have been successfully deployed. For example, hand geometry systems serve to control access to, among others, university dorms, nuclear power plants, and factories, where they record time and location. 1 Automated fingerprint identification systems (AFISs) integrate automatic and manual processes in criminal justice applications and civilian applications such as national identity systems.
An emerging technology such as biometrics typically confronts unrealistic performance expectations and is sometimes unfairly compared with approaches such as passwords that are not really alternatives. An effective biometric solution does not have to be—nor can it be—100 percent accurate or secure. For example, if there exists a 1 percent possibility of successful “buddy punching” (signing in for a friend or colleague), a hand geometry system can easily be seen as preventing 99 percent of such fraud. A particular application demands not perfection but satisfactory performance justifying the additional investments needed for the biometric system. In any given case, the system designer should understand the application well enough to achieve the target performance levels.
Nevertheless, solutions to the problem of recognizing individuals have historically been very elusive, and the effort needed to develop them has consistently been underestimated. Because humans seem to recognize familiar people easily and with great accuracy, such recognition has sometimes incorrectly been perceived as an easy task. Considering that a number of governments around the world have called for the nationwide use of biometrics in delivering crucial societal functions such as passports, there is an urgent need to act. Excepting for their application in national forensic AFISs, biometric recognition systems have never been tried at such large scales nor have they dealt with the wide use of nonforensic sensitive personal information. The current performance of some biometric systems—in particular with regard to the combination of error rate, robustness, and system security—may be inadequate for large-scale applications processing millions of users at a high throughput rate.
If there is a pressing public need for these applications, and if it is determined that biometric systems and technologies are the most appropriate way to implement them, then our understanding of the underlying science and technology must be robust enough to support the applications. 2 There is no substitute for realistic performance evaluations and sustained investment in research and development (R&D) to improve human recognition solutions and biometric systems. 3 The rest of this chapter outlines a research agenda focusing on (1) technical and engineering considerations, (2) social challenges, and (3) broader public policy considerations. The chapter concludes with a high-level overview of what constitutes a well-designed biometric system.
- TECHNOLOGY AND ENGINEERING RESEARCH OPPORTUNITIES
In recent years several research agendas for biometric technologies and systems have set important challenges for the field. 4 The issues and research opportunities raised in this chapter are meant to complement, not replace or supersede these other articulations. Indeed, the U.S. government has created or funded several interdisciplinary, academia-based research programs that provide an institutional foundation for future work. The focus of this report has been on broad systems-level considerations, particularly for large-scale applications, and the technical challenges outlined in this Chapter reflect that focus. But as these other agendas demonstrate, there are numerous opportunities for deeper understanding of these systems at almost every level. This section lays out several technical and engineering areas the committee believes would benefit from sustained research and further investigation: human factors, understanding the underlying phenomena, modality-related technical challenges, opportunities to advance testing and evaluation, statistical engineering aspects, and issues of scale.
Human Factors and Affordance
Because biometric technologies and systems are deployed for human recognition applications, understanding the subject-technology interface is paramount. A key piece of the biometric recognition process is the input of the human characteristic to be measured. With the exception of recent work at NIST-IAD 5 and at Disney, very little effort has been expended on the “affordance”—the notion that what is perceived drives the action that occurs, or, put another way, that form can drive function—of biometric systems. 6 Biometric systems should implicitly (or explicitly) suggest to the user how they are to be interacted with. 7
International standards, such as the International Organization for Standardization (ISO)/IEC 19795 series, 8 generally contain an informative annex on best practices for data collection for the modality under consideration. For example, ISO/IEC 19794-5 contains an annex on best practices for face images specifying that full frontal face poses should be used and rotation of the head should be less than +/− 5 degrees from frontal in every direction. This requirement presents an affordance challenge that has not yet been adequately addressed by the technologies—namely, how can a system be designed to suggest to the user a pose that meets this requirement? (The committee was told about one system that presented an image that, when viewed from the proper angle, was clearly visible to the user.) Similar challenges exist with every modality/application combination and will require a modality- and application-specific set of solutions.
“Quality” has been used to indicate data collected in compliance with the assumptions of the matching algorithms, such that recognition performance of the algorithm can be maximized, which means that “affordance” and data “quality” are tightly linked. System operators and administrators face their own challenges when interfacing with the systems. How should the interfaces of attended systems be designed so that the operator knows how and when to collect proper images, how to recognize when poor-quality images have been collected, and how to guide the data subject in making better presentations? Very little research in this area has been conducted, and there is opportunity for significant progress.
Distinctiveness and Stability of Underlying Phenomena
There are many open questions about the distinctiveness of the underlying biometric traits in these systems and about human distinctiveness generally. One typical assumption in the design of most biometric systems has been that characteristics, if properly collected, are sufficiently distinctive to support the application in question. This assumption has not, however, been confirmed by scientific methods for specific biometric characteristics, either by prospectively collecting and analyzing biometric samples and feature patterns or by exploiting databases of samples or feature patterns assembled for other purposes. A broad and representative sampling of the population in which distinctiveness is being evaluated should be obtained and a minimum quality specification should be set to which biometric samples should conform. More generally, the development of a scientific foundation for reliably determining the distinctive ness of various biometric traits under a variety of collection modes and environments is needed.
In other words, what is the effective limit on accuracy for a specific biometric trait in a realistic operating environment? This becomes a particularly important question at scale—that is, when the systems are expected to cope with large user populations and/or large reference databases. Even in DNA analysis, there has been controversy and uncertainty over how to estimate distinctiveness. 9 In biometric systems, “ground truth”—the collection of facts about biometric data subjects and recognition events to allow evaluation of system performance—is challenging, particularly for passive surveillance systems, where failures to acquire may be difficult to detect. 10
There are also open questions about the stability of the underlying traits—how persistent (stable) will a given individual’s biometric traits be over time? Some biometric traits, such as fingerprints, appear to be reasonably stable, but others, such as facial characteristics, can change significantly over even short periods of time. Depending on the capture and matching algorithms, changes in a trait over time may or may not have an effect on system performance and whether that person is appropriately recognized. Understanding more about the stability of common biometric traits will be important, especially if biometric systems are deployed for comparatively long (years or decades) periods of time.
All of this suggests several avenues of research that could strengthen the scientific underpinnings of the technology. There needs to be empirical analysis of base-level distinctiveness and the stability of common biometric modalities, both absolutely and under common conditions of capture, and research into what types of capture and what models and algorithms produce the most distinguishable and stable references for given modalities. Further, the scalability of various modalities under different capture and modeling conditions must be studied. The individuality of biometric identifiers, their long- and short-term physiological/pathological variations, and their relationship to the user population’s genetic makeup all merit attention as well.
Modality-Related Research
Every biometric system relies on one or more biometric modalities. The choice of modality is a key driver of how the system is architected, how it is presented to the user, and how match vs. nonmatch decisions are made. Understanding particular modalities and how best to use the modalities is critical to overall system effectiveness. Research into several interrelated areas will bring continued improvement:
- Sensors. Reducing the cost of sensor hardware; improving the signal-to-noise ratio, the ease of use and affordability, and the repeatability of measures; and extending life expectancy.
- Segmentation. Improving the reliability of identifying a region of interest when the user presents his or her biometric characteristics to the system—for example, locating the face(s) in an image or separating speech signal from ambient noise.
- Invariant representation. Finding better ways to extract invariant representation (features) from the inherently varying biometric signal—that is, what kind of digital representation should be used for a face (or fingerprint or other feature) such that the trait can be recognized despite changes in pose, illumination, expression, aging, and so on.
- Robust matching. Improving the performance of the matching algorithm in the presence of imperfect segmentation, noisy features, and inherent signal variance.
- Reference update. Developing ways to update references so that they can account for variations and the aging of reference data in long-lived systems.
- Indexing. Developing binning and partitioning schemes to speed up searches in large databases.
- Robustness in the face of adversaries. Improving robustness to attacks, including the presentation of falsified biometric traits (perhaps, for example, through automated artifact detection).
- Individuality. Exploring the distinctiveness of a particular biometric trait and its relationship to the matching performance. Does information about distinctiveness serve to increase understanding of the effective limits on matching performance, for example?
In addition to the general challenges described above, there are also challenges specific to particular biometric modalities and traits. While the following discussion does not describe all the challenges for each modality, it does offer some potentially fruitful avenues of investigation for the most common ones.
An ongoing challenge for facial recognition is segmentation—distinguishing facial features from surrounding information. Another signifi cant challenge for it is invariant representation—that is to say, finding a representation that is robust and persistent even when there are changes in pose, expression, illumination, and imaging distance, or when time has passed.
Specific challenges with respect to fingerprints include reducing the failure to enroll (FTE) and failure to acquire (FTA) rate, perhaps through the design of new sensors, artifact detection, image quality definition and enhancement, and high-resolution fingerprint matching. Fingerprint-based biometric systems could also be improved by increasing the speed of capture and minimizing contact, particularly for 10-print systems.
Iris recognition systems present R&D opportunities in the following areas: sensors; optimization of the illumination spectrum; reducing FTE and FTA rates; capturing and recognizing the iris at greater distances and with movement of the subject; and reducing the size of the hardware.
Improving speaker separation, normalizing channels, and using higher-level information (that is, beyond basic acoustic patterns) would all offer opportunities to improve voice recognition. In addition, robustness and persistence are needed in the face of language and behavioral changes and the limited number of speech samples.
Information Security Research
In many applications, biometric systems are one component of an overarching security policy and architecture. The information security community is extensive and has long experience with some of the challenges raised by biometric systems, which gives it a real opportunity for fruitful and constructive interaction with the biometrics community. Biometric systems pose two kinds of security challenges. The first is the use of biometrics to protect—provide security for—information systems. For what types of applications and in which domains is an approach incorporating biometric technologies most appropriate? This is a question for the broader information security community as well as the biometrics community and requires that we understand the goals and needs of an application to ascertain whether a biometrics-based approach is useful.
Assuming that a biometrics system is in place, the second security challenge is the security, integrity, and reliability of the system itself. 11 Information security research is needed that addresses the unique problems of biometric systems, such as preventing attacks based on the presentation of fake biometrics, the replay of previously captured biometric samples, and the concealment of biometric traits. Developing techniques for protecting biometric reference information databases to avoid their use as a source of fake biometrics is another area for such research. Decision analysis and threat modeling are other critical areas requiring research advances that will allow employing biometric systems more fully across a range of applications.
Testing and Evaluation Research
Testing and evaluation are an important component in the design, development, and deployment of biometric systems. Several areas related to the testing and evaluation of biometric systems are likely to prove fruitful. This section describes a few of them. While there has been significant work on testing and evaluating a variety of approaches, 12 it is the committee’s view that an even broader approach has merit. Moreover, while standardized evaluations of biometric systems are highly useful for development and comparison, their results may not reliably predict field performance. Methods used successfully for the study and improvement of systems in other fields (for example, controlled observation and experimentation on operational systems guided by scientific principles and statistical design and monitoring) should be used in developing, maintaining, assessing, and improving biometric systems. (See Chapter 3 for lessons that may be applicable from other domains.) The work over the last decade within the international standards community to reach agreement on fundamental concepts, such as how error rates are to be measured, has clarified the application of test methods under the usual laboratory conditions for biometric systems deployments. 13 Guidance for potential deployers of biometric systems on what is even a useful and appropriate initial set of questions to ask before getting into the details of modalities and so forth, as developed by a number of groups, has proven particularly useful. 14 And of course, in addition to the technical questions that need to be addressed, there are issues regarding how to measure cost over the life cycle of the system and how to assess potential and actual return on investment (ROI). Unfortunately, ROI analysis methodologies and case studies have been lacking in comparison to other types of assessments. See Box 5.1 for a brief discussion and example of an ROI assessment.
Return on Investment and Suitability Considerations. Determining the return on investment (ROI) for a biometric system is very much dependent on the application. It is based, among other things, on the risk the system is mitigating, the severity of the (more...)
Ultimately, determining the performance of an operational system requires an operational test, because adequately modeling all of the factors that impact human and technology performance in the laboratory is extremely difficult. Although the international standards community has made progress in developing a coherent set of best practices for technology and scenario testing, guidelines for operational testing are still under development and have been slowed by the community’s general lack of experience with these evaluations and a lack of published methods and results. 15 Designing a system and corresponding tests that can cope with ongoing data collection is a significant challenge, making it difficult for a potential user of biometric systems, such as a federal agency, to determine how well a vendor’s technology might operate in its applications and to assess progress in biometric system performance. Careful process and quality control analysis—as distinct from traditional, standardized testing of biometric systems that focuses on match performance for a test data set—at all stages of the system life cycle is essential. In addition, testing methods and results should be sufficiently open to allow disinterested parties to assess the results.
Test Data Considerations
One challenge meriting attention is test data for biometric systems. Designing large-scale systems requires large test data sets that are representative of the subject population, the collection environment, and system hardware expected in the target application. How does one determine which user population will be representative of the target application? The committee believes it is unlikely that being representative of the target application is the same as being representative of the population as a whole, because the population that should be considered will vary depending upon the ultimate application for which the system is used. Legal and privacy concerns have limited the collection and sharing of both test and operational data (for example, various data sets collected by the U.S. government) with researchers, 16 raising the question of whether biometric data can be made nonidentifiable back to its origin. 17 If it cannot, could synthetic biometric data be created and used in lieu of real biometric data? 18 If the latter is possible, does the use of synthetic (imagined) data offer any scientific validity in assessing performance of a system using real data? When test results are available, who has access to them? These and related questions merit attention from not just the T&E community but the broader biometrics communities as well.
Usability Testing
Many factors related to usability can affect system effectiveness and throughput and may also affect how well the system performs its recognition tasks. Testing and evaluation mechanisms are therefore needed that provide insight into how well a system under consideration handles a variety of user interface expectations.
Despite the recent focus of NIST’s information access division on usability testing, there is still major work to be done. One potential area of investigation is to incorporate into the design of the interface information on the expected motor control and cognitive capabilities of the user popu lations. Such information would allow the use of public health statistics to estimate the percentage of the general population (or subpopulation) that would be expected to have either cognitive or physical difficulties using the systems. By incorporating this understanding of the skills expected of users, designers and developers could tune the interfaces in ways that would increase their usability.
Usability is affected by other factors as well. For example, some unknown percentage of the population has a condition in which the fingers do not possess the usual friction ridges central to the functioning of fingerprint-based biometric system. In addition, some unknown (but believed to be nonzero) percentage of the population has either no irises or irises of unusual shape. When setting baseline error rates, it is important to have estimates of the percentage of the population lacking the required trait, because this lack interacts with the design of sensors and algorithms. It may well be that each modality will have lower error rate bounds that cannot be improved upon by better sensors, algorithms, or collection procedures. More research is needed to understand this. Such questions are related to the distinctiveness and stability of the underlying biometric traits, discussed above.
Other usability considerations relate to the ease of participation. Is the system designed to take into account user needs (such as tables on which to set their items if necessary) and physical differences (such as height and weight)? What kinds of user assistance should be provided? What accommodations could be made for people who are unfamiliar with the system, or, conversely, for people who are very familiar with it (much as toll pass transponder users can use dedicated lanes on highways)?
ROI Analysis Methodologies and Case Studies
Determining the potential ROI and identifying which system characteristics contribute is an important means of evaluating any biometrics deployment. In addition to how well a system meets its requirements, there are issues about measuring cost over the life cycle of the system and assessing potential (and actual) ROI. There are relatively few ROI analysis methodologies and case studies. The research opportunity here is to develop methods for examining likely costs and cost savings that take into account the technical life cycle as well as ongoing maintenance and usage costs.
Evaluative Frameworks for Potential Deployers
In addition to system and technology tests, there is a significant opportunity to develop an evaluative model that would guide potential procurers and users of biometric systems. Guidance for potential users of biometric systems on an appropriate initial set of questions to ask before getting into the details of modalities and so forth has proven particularly useful. 19
Testing When Data Changes
Designing a system and tests that can cope with ongoing data collection after it has been deployed is a significant challenge. The characteristics of the data may change from what was assumed during testing. This could be due to changes in the technology, changes in the user population, changes in how the system is used, or all of the above. Such potential changes to the data make it a challenge for potential users of biometrics, such as federal agencies, to readily determine how well a given vendor’s technology might operate for the agency’s applications over time. Developing testing and evaluation methodologies that can account for such potential changes or offer information on how a system’s performance might change in the event of significant changes to the data characteristics is an opportunity for further investigation.
Operational Testing
Finally, operational testing is problematic in that most existing systems do not retain the data needed to determine error and throughput rates. Each system collects and stores different data in application-specific ways. Additionally, ground truth (all of the relevant facts about all participants) cannot be known in real applications with arbitrary user populations. Privacy rights of the data subjects may prevent using collected data for testing purposes. Lastly, because system operators may not wish others to know about operational performance for reasons of security, very few operational test results have ever been published. The ISO/IEC JTC1 SC37 standards committee has been working for a number of years on basic guidance for operational testing, but progress on this standard, to be known as ISO/IEC 19795, Part 6, has been slow, reflecting the inherent difficulties in making general statements about operational tests.
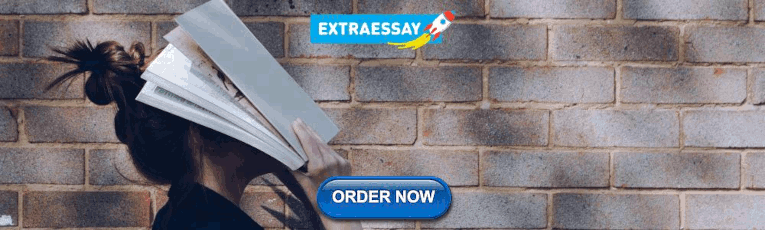
Systems-Level Statistical Engineering Research
In addition to the modality-related technical challenges outlined above, there are broad systems-level considerations to take into account. In particular, statistics and statistical engineering offer opportunities for progress and the development of design principles and model designs for operational testing of biometric systems and experimentation with modifiable system parameters. This section outlines some potential research questions in statistical engineering and biometric systems that merit attention.
Statistical approaches come into play with respect to the user populations, including cross-sectional and longitudinal studies of the variability of various biometric modalities over time, the association of biometrics with demographic and medical factors, and the effects of demographic factors and physical characteristics on failure to acquire and error rates.
Another systems-level consideration is error rates in biometric systems, including the following topics:
- The relative contribution to error of different phases and components of biometric recognition (on an algorithm-by-algorithm basis, because error rates and their causes are algorithm specific);
- The potential for algorithm-specific quality control measures to reduce error rates in varying populations of data subjects;
- The application of known statistical methods for analysis of cor- related data to estimation 20 of false match and false nonmatch rates for recognition tasks; and
- The investigation of new statistical models for the estimation as above but from biometric databases where information on replication is incomplete—that is, replication is known only for a subset, or some information about variation in replicate measurements is available from an external source.
Other areas of potential investigation include studies of statistical efficiency and cost-benefits of different approaches to choosing, acquiring, and utilizing multimodal biometrics of various sorts. Issues to be considered would include the relative algorithmic-dependent within-class and between-class variation of sample choice such as multiple instances of 2 fingerprints versus single instances of 10 fingerprints versus single instances of 2 fingerprints combined with two-dimensional facial imaging. The aim is to develop the most informative fusion methods based on application- and algorithm-dependent multivariate distributions of biometric features.
Research on Scale
There are many ways in which scale may manifest itself in biometric systems. These include the number of sensors in the system, the number of comparisons being performed for a given unit time or a given input sample, the number of users (including administrators and operators), the geographic spread of the system, the size of the potential user population, or any combination of these factors. Addressing issues of scale in biometric systems offers numerous opportunities for research.
For instance, one question is this: How does the number of persons who have references in the enrolled database affect the speed of the system and its error rates? For some applications and associated algorithmic approaches, the size of the database might not matter if typical operation involves only a one-to-one comparison—that is, one set of submitted samples being compared to one set of enrollment records. For large-scale identification and screening systems, sequentially performing a very large number of one-to-one matches is not effective; there is a need for efficiently scaling the system to control throughput and false-match error rates as the size of the database increases.
Typical approaches to scaling include (1) using multiple hardware units, (2) coarse pattern classification (for example, first classifying a fingerprint into classes such as arch, tented arch, whorl, left loop, and right loop), and (3) extensive use of exogenous data (such as gender, age, and geographical location) supplied by human operators. Although these approaches perform well in practice, they come at a price. Using hardware linearly proportional to the database size is expensive. Coarse pattern classification offers substantial scaling advantages even when single measures are available and even more advantage with multiple measures—for example, fingerprints from multiple fingers—but can add to the nonmatch error rates. Use of exogenous information creates a mechanism for intentionally avoiding identification—for example, dressing as the opposite sex or appearing older—if someone is trying to avoid being recognized by the system, not to mention potential privacy compromises.
Ideally, one would like to index biometric data patterns in some way similar to that used in conventional databases in order to benefit from lessons learned in other arenas. However, due to large intraclass variation in biometric data caused by variation in collection conditions and human anatomies and behaviors, it is not obvious how to ensure that samples from the same pattern fall into the same index bin. There have been very few published studies on reliably indexing biometric patterns. 21 Efficient indexing algorithms would need to be developed for each technology/modality combination. It is unlikely that any generic approach would be applicable to all biometric measures, although efforts to understand similarities and where lessons from one type of system can be applied to another are warranted.
False-match errors generally increase with the number of required comparisons in a large-scale identification or watch-list system. As most comparisons are false (for example, a submitted sample compared to the enrollment pattern of another person), increasing the size of the database increases the number of opportunities for a false match. However, in large-scale systems it is unlikely that a sample would be compared against every possible match in the database. Instead, just as with search algorithms generally, the set of items to compare against is winnowed according to certain criteria as quickly as possible to save time and memory. Because of the nonindependence of sequential comparisons using the same sample data, coupled with architectural and algorithm design choices that are aimed at finding any matches while sustaining throughput rate and limiting active memory, the relationship between the number of false matches and database size is a poorly understood issue meriting further investigation. 22
Although a watch-list database in a screening system is much smaller than that in a large-scale identification application, the number of continuous or active comparisons may be huge. Therefore, as in large-scale applications, the throughput and error-rate issues are also critical in screening applications. Computationally, scaling of large systems for near-real-time applications involving 1 million identities is becoming feasible, as is screening the traffic for 500 recognized identities. However, designing and building a real-time identification system involving 100 million identities is beyond our understanding. More research is needed here as well.
Social Science Research Opportunities
Biometric systems require an intimate association between people and the technologies that collect and record their biological and behavioral characteristics. This is true whether the application is overt or covert, negative claim or positive claim. It is therefore incumbent on those who conceive, design, and deploy biometric systems to consider the cultural and social contexts of these systems. Unfortunately, there are few rigorous studies of these contexts. Below is a framework for developing a portfolio of future research investigations that could help biometric systems better cope and perform within their cultural and social contexts.
Cultural and social issues arise at essentially two different levels—for the individual and for society. At the level of the individual, whether they are interacting actively or passively with a biometric system (for example, the person seeking entry to a facility), the issue is the performance of a biometric system. At the societal level, the issue is the social impact of the biometric system (for example, all are affected, either directly or indirectly, by the trade, tourism, and terrorism effects of a biometric passport).
At the level of the individual, social considerations are critical in the design, deployment and functioning of biometric systems. As we have noted, system performance may well be degraded if relevant social factors are not adequately taken into consideration. For example, religious beliefs that call for adherents to cover their faces in public make facial-recognition biometrics problematic. Thus if a biometric system is to work well for a broad range of people it must take into account behaviors resulting from such things as religion or social convention. Every biometric system has a protocol for how it is to be interacted with. The protocol may be simple or complex, uniform in application, or tailored to the individual. Obviously, however, a good protocol for a biometrics system must recognize variations in biological features. A system based on fingerprints must have ways to gracefully accommodate a person who is missing a finger or who otherwise does not have usable fingerprints.
In addition to the design issue of affordance, previously discussed, research is needed to determine effective, appropriate, and graceful protocols, processes, and devices that gain the cooperation of participants, and the protocols and devices must be acceptable to the community. In biometric systems that are essentially surveillance systems, compliance should be thought of as more than acquiescence and should extend to gracefully (perhaps without notice) promoting the types of behavior (for example, face pose and angle) that result in useful biometric measures. Full compliance represents the ideal interaction of the participant with the biometric system from the viewpoint of the system designers. Acceptability to the community refers to the endorsement, or at least the lack of active disapproval, by significant governmental and public leadership groups. In any case, community acceptability is not guaranteed. Influential parts of the community may find biometric systems overly intrusive, unfair to certain groups, or inadequately protective of the individual’s privacy.
The dimensions of individual compliance and community acceptability are discussed next. One part of the design process for particular systems or, more realistically, for a particular class of systems might be to develop data that predict how well the biometric system will perform in a target community and on factors that may make the system more acceptable to that community. Predictive aspects may just have a statistical relationship with subject compliance or community acceptability, while acceptability factors probably have a causal relationship. Developing data in these areas will provide the evidence needed to assess the relationships.
The extent and nature of participant compliance can be discovered and confirmed using either or both of two basic research strategies: field studies using ethnographic tools such as in cultural anthropology or attitude studies of using survey methods such as are common in sociology. Some of the things that might predict participant compliance include participant attitudes toward authority, their willingness to try new technology, their adherence to certain religious or cultural beliefs, and the geographic distribution of the population. Such work could, in theory, be part of the design work for biometrics systems but is generally not done, possibly because of the expense and effort involved. Research that sheds light on these issues would provide valuable information for those designing and building biometric systems.
Factors that motivate participant compliance can be discovered by experimental studies, essentially creating laboratory environments in which the factors can be controlled. This research paradigm is common in experimental psychology, but the extent to which such controlled studies might develop data that reflect factors encountered in operational applications is only speculative. Some candidate factors include self-interest, enforcement, inducement, social pressure, conviction, habit or practice, behavior of other actors, pleasantness of the experience, and attention to cultural norms. The more common approach is to survey data subjects who have just encountered an operational system to elicit their opinions, 23 but even this approach has rarely been applied.
Aspects that predict the extent and nature of community acceptability can be discovered and confirmed using either or both of two basic research strategies: field studies of similar deployments using ethnographic tools—as indicated for participant compliance above, or focus groups that are asked to discuss how they view various characteristics of a biometric system such as are common in marketing studies. The kinds of aspects that might be predictive of community acceptability include resemblance to existing well-tolerated systems, operated under the auspices of a respected institution, or a system that meets all legal requirements
One factor in motivating community acceptability is whether community concerns—for example, fairness, privacy, and confidentiality—are addressed. Using data from research in these and related areas, it should be possible to address a variety of relevant questions, such as: Where on the scale of purely voluntary to mandatory is a particular biometric system? In largely voluntary systems does cooperation vary by subgroup such as age, sex, or race? Does habituation lead to greater cooperation? How important is it that participants believe they or others will not be harmed? What factors influence such trust? What are effective and appropriate compliance mechanisms for biometric systems? Although it is not reasonable to expect designers of a specific system to conduct such research, these questions could be addressed as part of a more general research agenda.
- PUBLIC POLICY CONSIDERATIONS AND RESEARCH OPPORTUNITIES
Numerous issues come into play beyond technical and engineering considerations in government use of biometric systems. These other issues include the following:
- To what extent can the need for a biometric system be satisfied by current technical capability? Balancing mandates with maturity of systems and technology is critical. Aggressive schedules can push technology development forward but not all challenges can be addressed on short notice.
- Is there sufficient flexibility and time to support the risk management needed to develop and deploy a biometric system? Governments must avoid increasing risk through overly constrained integration and testing timelines and budgets. The risks include the possibility not only that the system will fail or be compromised but also the possibility that the system will be rejected by its users or be so cumbersome or inefficient that it is withdrawn from use.
- Should participation in the system be mandated? Such a mandate might foster a climate of distrust or social unrest.
- What is the nature of the biometrics workforce? To the extent that biometric systems and related technologies are seen as important to meet public policy goals, is there sufficient incentive to grow and maintain the needed expertise? Training and maintaining consistent biometrics workforce has been difficult. Several organizations recently announced plans to create certification programs for professionals, but consensus must be reached on what skills are required of a professional in this area. The creation of a biometrics undergraduate program at West Virginia University is a step in the right direction. (The program has a ready customer: the FBI’s Criminal Justice Information Center in Clarksburg, West Virginia.)
- The sourcing of the technology is crucial to the government’s successful deployment of technological and information systems, including biometric systems. There is an inherent danger in relying on companies with manufacturing, research, or development activities centered overseas. For biometric systems, especially, the risk is the potential for U.S. biometric data to be collected by foreign governments, inviting scrutiny of U.S. information on border control systems and other critical infrastructure by persons not cleared by the U.S. government.
The social science considerations described above may have impacts on broader public policy considerations. Systematic empirical research and factual analysis would help provide an evidence case for public policy in this area. Some key research questions that have an impact on public policy include the following:
- What lessons can be learned from environmental impact statements and privacy impact assessments that might be relevant to deciding whether social impact assessments for biometric systems are useful?
- Do existing or proposed biometric systems represent a serious potential for identity theft?
- How have authoritarian regimes made use of human recognition methods to assert their control over individuals? In what ways might biometric systems enable these sorts of uses? How could such a risk be mitigated?
- To what extent are privacy requirements, interagency control issues, and policy constraints, or the perception thereof, inhibiting the research use and sharing of existing biometric data?
- What belief sets, if any, lead to an aversion to certain biometric technologies?
A reliable and effective biometric system may be perceived as providing irrefutable proof of identity of an individual, notwithstanding the many uncertainties already mentioned, raising concerns for users. Will the information regarding biometrics-based access to resources be used to track individuals in a way that infringes on privacy or anonymity? Will biometric data be used for an unintended purpose: For example, will fingerprints provided for access control be matched against fingerprints in a criminal database? Will data be used to cross-link independent records from the same person—for example, health insurance and grocery purchases? How would a user be reassured that a biometric system is being used for the intended purpose only?
Designing information systems—not only biometric systems—whose functionality is verifiable during deployment is very difficult. One solution might be a system that meticulously records recognition decisions and the people who accessed the logged decisions using a biometric-based access control system. Such a system could automatically warn users if a suspicious pattern is seen in the system administrator’s access of users’ logs. Another solution might be biometric cryptosystems—cryptographic keys based on biometric samples. Radical approaches such as total transparency attempt to solve the privacy issues in a novel way. But there are no obviously satisfactory solutions on the horizon for the privacy problem. Additional research on the relationship between biometric (bodily) information and privacy is needed.
The privacy protections required to facilitate data collection from and about biometric systems need to be clearly established. Because many of these systems are deployed to satisfy security needs, it is reasonable to expect that performance and vulnerability data need to be protected. For best results, the data sets for such research should be very large, contain very few errors in ground truth (metadata indexing), be appropriately randomized, and represent the populations of interest to target applications. To the extent consistent with privacy and security, the results of the studies should be published in the peer-reviewed scientific literature and the biometric samples used made widely available to other researchers.
- REALIZING A WELL-DESIGNED BIOMETRIC SYSTEM
Research in the areas described above is warranted more than ever as biometric systems become widespread and are used in critical applications. This report concludes by taking a step back and presenting a vision of a well-designed biometric system that should persist even as progress is made on the challenges described earlier in this chapter.
A well-designed biometric system includes more than technology. It is a complex combination of technology, public policy, law, human processes, and social consensus. In the long term, there may be new modalities that allow recognizing human characteristics and behaviors quickly and effectively with little or no interaction on the individual’s part. Human beings may turn out to possess distinctive traits that have yet to be fully explored or that cannot be suitably represented by present technology. Some of the potential sources of suitable signals currently being pursued include inductive signature and brain waves (EKG activity). Each of these potential signal sources could bring with it a new set of societal and policy issues requiring exploration.
Even with all of these uncertainties, and even with the many intriguing open questions that merit research, the committee believes that the following framework for a well-designed biometric system will apply for the foreseeable future. Progress in such research will lead to even more well-designed systems. This framework is offered as both an evaluative tool and as a development tool.
A well-designed biometric recognition system will have (at least) the following characteristics:
- The system will be designed to take into account that no biometric characteristic is entirely stable and distinctive. In other words, it will take into account that biometric similarity represents a likely, not a definitive, recognition and and that the corresponding is true for a failure to find similarity. In particular, presumptions and burdens of proof will be designed conservatively, with due attention to the system’s inevitable imperfections.
- The policies of such a system will recognize that any claimed probabilities of correctness depend on external assumptions about dynamic presentation distributions, and that these assumptions, whether subjective or based on estimates from past internal or external data, are fallible. It will enable system operators and users to recognize that biometric information has a life cycle. Biometric information is collected or modified during, for example, enrollment, recognition, and so on. But policies should also recognize that changes in the biometric characteristics of the individual can lead to incorrect or failed recognition.
- The system will be designed so that system operators and users recognize that some inaccurate information may be created and stored in the databases linked to biometric references, and that over time information in these databases will become out of date. In particular, the reliability of information in the database is independent of the likelihood of correct recognition. The system will be designed to handle challenges to the accuracy of database information in a fair and effective way.
- Because the system’s sensors and back-end processes are not perfectly accurate, it will need to handle failures to enroll, failures to acquire samples, and other error conditions gracefully and without violating dignity, privacy, or due process rights.
- Because some individuals will attempt to force the system into failure modes in order to avoid recognition, the system’s failure modes must be just as robustly designed as the primary biometrics-based process.
- The system’s security, privacy, and legal goals must be explicit and publicly stated, and they must be designed to protect against a specific and enumerated set of risks. The system will specifically address the possibility that malicious individuals may be involved in the design and/or operation of the system itself.
- It will recognize that biometric traits are inherently not secret and will implement processes to minimize both privacy risks and risks of misrecognition arising from this fact.
- CONCLUDING REMARKS
This report lays out a broad systems view and outlines many of the subject areas with which biometrics research intersects. The committee also describes many open research problems, ranging from deep scientific questions about the nature of individuality to vexing technical and engineering challenges. It raises questions about appropriate system architecture and life-cycle design as well as questions about public policy regarding both private sector and government use of biometric systems. It notes that biometrics is an area that benefits from analyzing very large amounts of data. These and other aspects of biometrics suggest many fruitful areas and interesting problems for researchers from a range of disciplines.
A.K. Jain, R. Bolle, and S. Pankanti, eds., Biometrics: Personal Identification in Networked Society, Norwell, Mass.: Kluwer Academic Publishers (1998), as cited in A.K. Jain, S. Pankanti, S. Prabhakar, L. Hong, A. Ross, and J. Wayman, Biometrics: A Grand Challenge, Proceedings of the 18th International Conference on Pattern Recognition, Cambridge, England (2004). Available at http://biometrics .cse .msu.edu/Publications /GeneralBiometrics /Jainetal_BiometricsGrandChallenge _ICPR04.pdf .
The National Science Foundation Center for Identification Technology Research is one program taking an interdisciplinary approach to research related to biometrics. More information about the center is available at http://www .nsf.gov/eng /iip/iucrc/directory/citr.jsp .
Standardization efforts, discussed elsewhere in this report, can help facilitate the cycle of build-test-share for transitioning the technology from concept to business solution.
See, for example, A.K. Jain, S. Pankanti, S. Prabhakar, L. Hong, A. Ross, and J. Wayman, Biometrics: A Grand Challenge, Proceedings of the 18th International Conference on Pattern Recognition, Cambridge, England (2004); National Science and Technology Council, Subcommittee on Biometrics, “The National Biometrics Challenge” (2006), available at http://www .biometrics .gov/Documents/biochallengedoc.pdf ; E. Rood and A.K. Jain, Biometrics Research Agenda, report of an NSF Workshop (2003); and Mario Savastano, Philip Statham, Christiane Schmidt, Ben Schouten, and Martin Walsh, Appendix 1: Research challenges, BioVision: Roadmap for Biometrics in Europe to 2010, Astrid Albrecht, Michael Behrens, Tony Mansfield, Will McMeechan, and Marek Rejman-Greene, eds. (2003), available at http://ftp .cwi.nl/CWIreports /PNA/PNA-E0303.pdf .
M. Theofanos, B. Stanton, and C. Wolfson, Usability and biometrics: Assuring successful biometric systems, NIST Information Access Division (2008); M. Theofanos, B. Stanton, C. Sheppard, R. Micheals, J. Libert, and S. Orandi, Assessing face acquisition, NISTIR 7540, Information Access Division Information Technology Laboratory (2008); and M. Theofanos, B. Stanton, C. Sheppard, R. Micheals, Nien-Fan Zhang, J. Wydler, L. Nadel, and W. Rubin, Usability testing of height and angles of ten-print fingerprint capture, NISTIR 7504 (2008).
J.J. Gibson, The theory of affordances, in Perceiving, Acting, and Knowing , Robert Shaw and John Bransford, eds. Hillsdale, N.J.: Erlbaum Associates (1977).
This idea can be extended even to systems where the subject is unaware of the interaction but behaviors are being suggested to facilitate data collection.
“Information technology—biometric performance testing and reporting,” ISO/IEC 19795.
P.J. Bickel, Discussion of “The evaluation of forensic DNA evidence,” Proceedings of the National Academy of Sciences 94(11): 5497 (1997). Available at http://www .pnas.org/content/94/11/5497 .full?ck=nck .
Ted Dunstone and Neil Yager, Biometric System and Data Analysis: Design, Evaluation, and Data Mining , New York: Springer Science+Business Media (2008).
See NRC, Toward a Safer and More Secure Cyberspace , Washington, D.C.: The National Academies Press (2007) for an in-depth discussion of security.
NIST’s emerging National Voluntary Laboratory Accreditation Program (NVLAP) for biometrics represents progress in formalizing testing programs but does not yet provide specific testing methods required for different products and applications and does not yet address operational testing. The NVLAP Handbook 150-25 on Biometrics Testing is available at http://ts .nist.gov/Standards /Accreditation /upload/NIST-Handbook-150-25 _public_draft_v1_09-18-2008 .pdf .
Note that while these standards are aimed at a broad swath of systems, they are not seen as appropriate for governments to use for large-scale AFIS systems, where they would need to be tested for conformance to standards, compliance with system requirements, alignment with capacity and accuracy requirements, and satisfaction of availability and other traditional system parameters.
For example, the recommendations of the U.K. Biometrics Working Group in “Use of Biometrics for Identification and Authentication: Advice on Product Selection, Issue 2.0” (2002). Available at http://www .cesg.gov.uk /policy_technologies /biometrics/media/biometricsadvice.pdf .
ISO/IEC 19795-6, Biometric Performance Testing and Reporting—Part 6: Testing Methodologies for Operational Evaluation, is under development by ISO JTC1 SC37.
Government operational biometric data—that is, personally identifiable information (PII)) for research and testing are governed by the privacy impact assessments (PIAs) and system-of-record notices (SORNs) associated with the specific systems, which are required by the Privacy Act of 1974. Whether the Privacy Act provides the latitude to use operational biometric and biometric-related data for large-scale research and testing purposes (during acquisition and operation) so long as data privacy and integrity are adequately protected is subject to interpretation. Various scenarios for how such data might be shared include these: (1) using the data (such as from IAFIS or US-VISIT) internal to the agency collecting the data, (2) using such data outside the agency collecting the data (such as providing multiagency data to NIST for analysis), or (3) providing such data to a university and/or industry team for analysis, etc. Assuming the Privacy Act permits such uses, then the PIAs and SORNs would have to specify such use of the data. The NIST multimodal biometric application resource kit (MBARK) data set is an example of test data collected but not disseminated for privacy reasons. The Department of Homeland Security (DHS) has released its former IDENT database to NIST for testing (NIST reports refer to it as DHS-2), but it has not been released to general researchers.
Fingerprint images that are sufficiently similar to the original fingerprint can be reconstructed from data representations of the fingerprints. See J. Feng and A.K. Jain, FM model based fingerprint reconstruction from minutiae template, International Conference on Biometrics (2009), pp. 544-553. Available at http://biometrics .cse .msu.edu/Publications /Fingerprint/FengJain_FMModel_ICB09 .pdf .
SFINGE, a fingerprint synthesis technique, is described in Chapter 6 of D. Maltoni, D. Maio, A.K. Jain, and S. Prabhakar, Handbook of Fingerprint Recognition , 2nd edition, Springer Verlag (2009).
The NRC report Protecting Individual Privacy in the Struggle Against Terrorists: A Framework for Assessment (The National Academies Press, Washington, D.C., 2008) provides a framework for assessing program efficacy as well as impacts on privacy. The U.K. Biometrics Working Group developed another such document, which is available at http://www .cesg.gov.uk /policy_technologies /biometrics/media/biometricsadvice.pdf .
The committee notes the reluctance to use interval estimation because of lack of agreement on how to handle systematic errors except through personal probability. See ISO/IEC, Guide 98-3 (1995) Guide to the Expression of Uncertainty in Measurement .
J.L. Wayman, Multi-finger penetration rate and ROC variability for automatic fingerprint identification systems, in N. Ratha and R. Bolle, eds., Automatic Fingerprint Recognition Sys tems , New York: Springer Verlag (2003).
See, for example, J.L. Wayman, Error rate equations for the general biometric system, IEEE Robotics and Automation 6(1): 35-48, and H. Jarosz, J.-C. Fondeur, and X. Dupré, Large-scale identification system design, J.L. Wayman, A.K. Jain, D. Maltoni, and D. Maio, eds., Biometric Systems: Technology, Design, and Performance Evaluation , New York: Springer (2005).
As was done, for example, by the Orkand Corporation in Personal Identifier Project: Final Report , California Department of Motor Vehicles report DMV88-89 (1990).
- Cite this Page National Research Council (US) Whither Biometrics Committee; Pato JN, Millett LI, editors. Biometric Recognition: Challenges and Opportunities. Washington (DC): National Academies Press (US); 2010. 5, Research Opportunities and the Future of Biometrics.
- PDF version of this title (1.3M)
In this Page
Recent activity.
- Research Opportunities and the Future of Biometrics - Biometric Recognition Research Opportunities and the Future of Biometrics - Biometric Recognition
Your browsing activity is empty.
Activity recording is turned off.
Turn recording back on
Connect with NLM
National Library of Medicine 8600 Rockville Pike Bethesda, MD 20894
Web Policies FOIA HHS Vulnerability Disclosure
Help Accessibility Careers
AI Index Report
Welcome to the seventh edition of the AI Index report. The 2024 Index is our most comprehensive to date and arrives at an important moment when AI’s influence on society has never been more pronounced. This year, we have broadened our scope to more extensively cover essential trends such as technical advancements in AI, public perceptions of the technology, and the geopolitical dynamics surrounding its development. Featuring more original data than ever before, this edition introduces new estimates on AI training costs, detailed analyses of the responsible AI landscape, and an entirely new chapter dedicated to AI’s impact on science and medicine.
Read the 2024 AI Index Report
The AI Index report tracks, collates, distills, and visualizes data related to artificial intelligence (AI). Our mission is to provide unbiased, rigorously vetted, broadly sourced data in order for policymakers, researchers, executives, journalists, and the general public to develop a more thorough and nuanced understanding of the complex field of AI.
The AI Index is recognized globally as one of the most credible and authoritative sources for data and insights on artificial intelligence. Previous editions have been cited in major newspapers, including the The New York Times, Bloomberg, and The Guardian, have amassed hundreds of academic citations, and been referenced by high-level policymakers in the United States, the United Kingdom, and the European Union, among other places. This year’s edition surpasses all previous ones in size, scale, and scope, reflecting the growing significance that AI is coming to hold in all of our lives.
Steering Committee Co-Directors
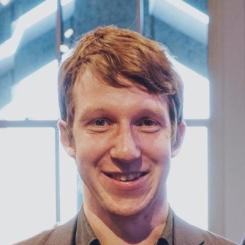
Ray Perrault
Steering committee members.
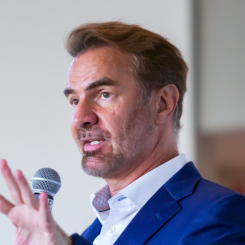
Erik Brynjolfsson
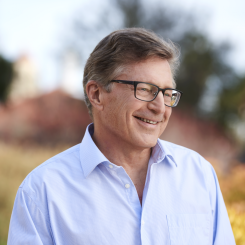
John Etchemendy
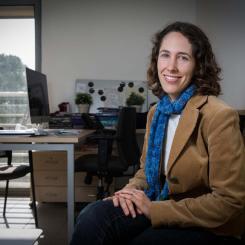
Katrina Ligett
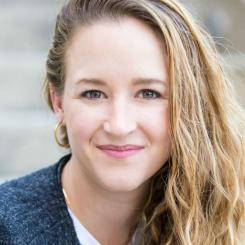
Terah Lyons
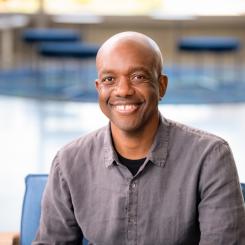
James Manyika
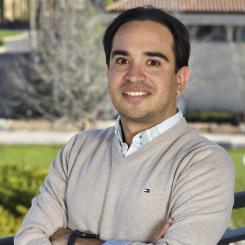
Juan Carlos Niebles
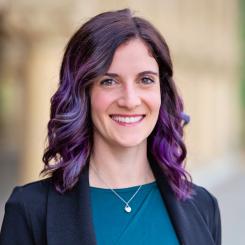
Vanessa Parli
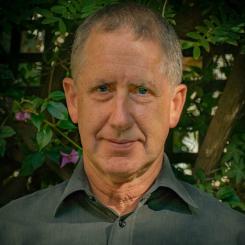
Yoav Shoham
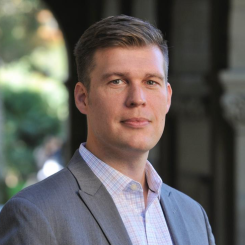
Russell Wald
Staff members.
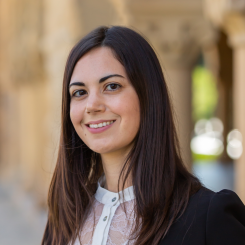
Loredana Fattorini
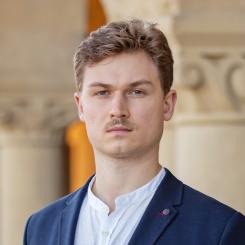
Nestor Maslej
Letter from the co-directors.
A decade ago, the best AI systems in the world were unable to classify objects in images at a human level. AI struggled with language comprehension and could not solve math problems. Today, AI systems routinely exceed human performance on standard benchmarks.
Progress accelerated in 2023. New state-of-the-art systems like GPT-4, Gemini, and Claude 3 are impressively multimodal: They can generate fluent text in dozens of languages, process audio, and even explain memes. As AI has improved, it has increasingly forced its way into our lives. Companies are racing to build AI-based products, and AI is increasingly being used by the general public. But current AI technology still has significant problems. It cannot reliably deal with facts, perform complex reasoning, or explain its conclusions.
AI faces two interrelated futures. First, technology continues to improve and is increasingly used, having major consequences for productivity and employment. It can be put to both good and bad uses. In the second future, the adoption of AI is constrained by the limitations of the technology. Regardless of which future unfolds, governments are increasingly concerned. They are stepping in to encourage the upside, such as funding university R&D and incentivizing private investment. Governments are also aiming to manage the potential downsides, such as impacts on employment, privacy concerns, misinformation, and intellectual property rights.
As AI rapidly evolves, the AI Index aims to help the AI community, policymakers, business leaders, journalists, and the general public navigate this complex landscape. It provides ongoing, objective snapshots tracking several key areas: technical progress in AI capabilities, the community and investments driving AI development and deployment, public opinion on current and potential future impacts, and policy measures taken to stimulate AI innovation while managing its risks and challenges. By comprehensively monitoring the AI ecosystem, the Index serves as an important resource for understanding this transformative technological force.
On the technical front, this year’s AI Index reports that the number of new large language models released worldwide in 2023 doubled over the previous year. Two-thirds were open-source, but the highest-performing models came from industry players with closed systems. Gemini Ultra became the first LLM to reach human-level performance on the Massive Multitask Language Understanding (MMLU) benchmark; performance on the benchmark has improved by 15 percentage points since last year. Additionally, GPT-4 achieved an impressive 0.97 mean win rate score on the comprehensive Holistic Evaluation of Language Models (HELM) benchmark, which includes MMLU among other evaluations.
Although global private investment in AI decreased for the second consecutive year, investment in generative AI skyrocketed. More Fortune 500 earnings calls mentioned AI than ever before, and new studies show that AI tangibly boosts worker productivity. On the policymaking front, global mentions of AI in legislative proceedings have never been higher. U.S. regulators passed more AI-related regulations in 2023 than ever before. Still, many expressed concerns about AI’s ability to generate deepfakes and impact elections. The public became more aware of AI, and studies suggest that they responded with nervousness.
Ray Perrault Co-director, AI Index
Our Supporting Partners
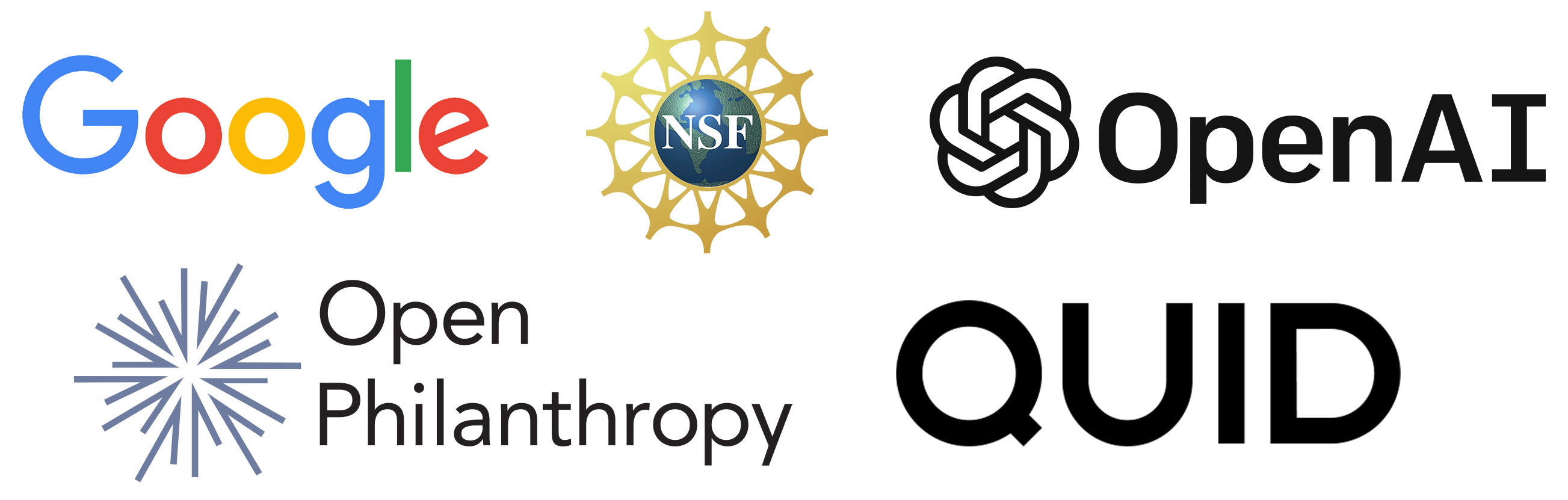
Analytics & Research Partners
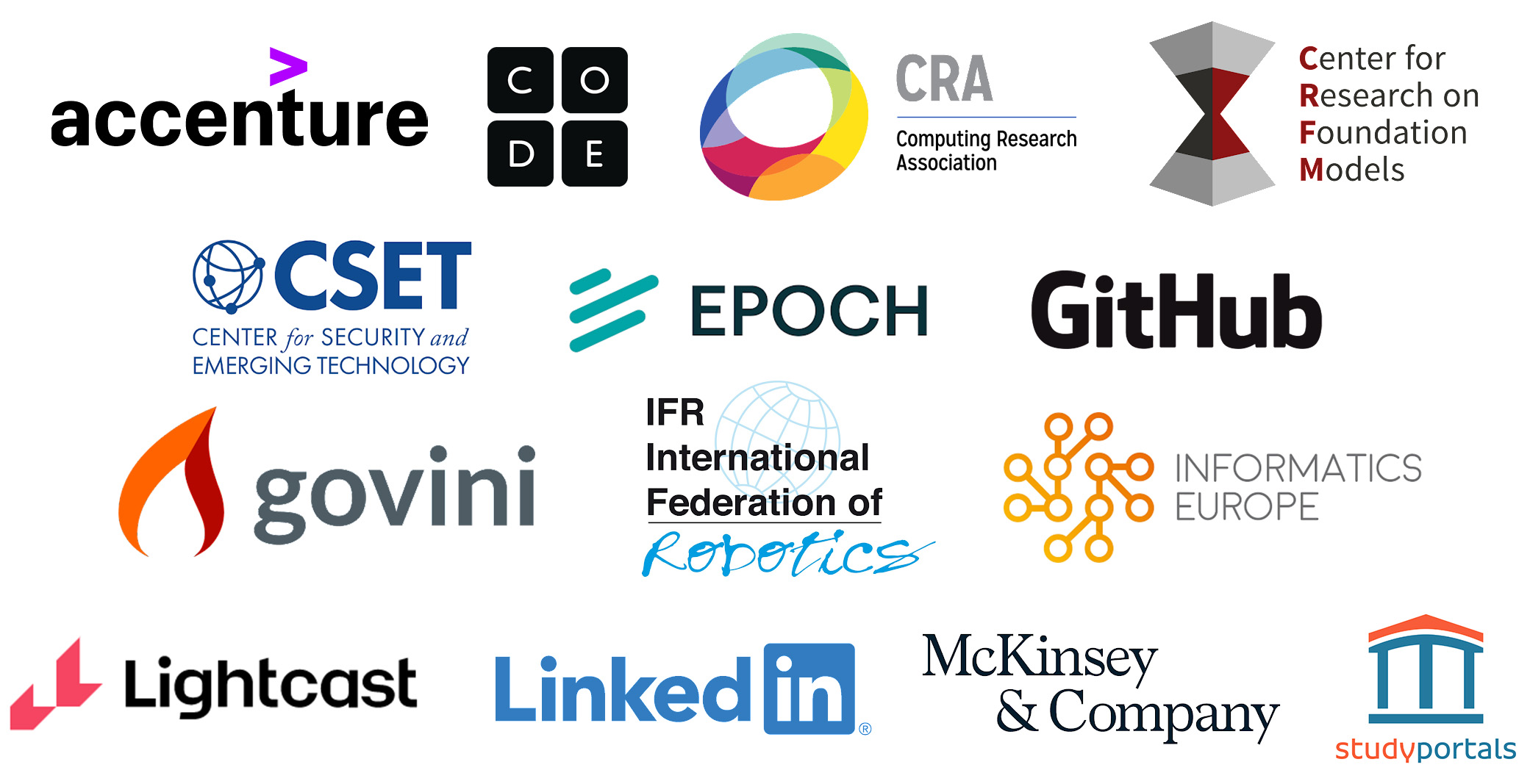
Stay up to date on the AI Index by subscribing to the Stanford HAI newsletter.
- Share full article
Advertisement
Supported by
Your Brain Waves Are Up for Sale. A New Law Wants to Change That.
In a first, a Colorado law extends privacy rights to the neural data increasingly coveted by technology companies.

By Jonathan Moens
Consumers have grown accustomed to the prospect that their personal data, such as email addresses, social contacts, browsing history and genetic ancestry, are being collected and often resold by the apps and the digital services they use.
With the advent of consumer neurotechnologies, the data being collected is becoming ever more intimate. One headband serves as a personal meditation coach by monitoring the user’s brain activity. Another purports to help treat anxiety and symptoms of depression. Another reads and interprets brain signals while the user scrolls through dating apps , presumably to provide better matches. (“‘Listen to your heart’ is not enough,” the manufacturer says on its website.)
The companies behind such technologies have access to the records of the users’ brain activity — the electrical signals underlying our thoughts, feelings and intentions.
On Wednesday, Governor Jared Polis of Colorado signed a bill that, for the first time in the United States, tries to ensure that such data remains truly private. The new law, which passed by a 61-to-1 vote in the Colorado House and a 34-to-0 vote in the Senate, expands the definition of “sensitive data” in the state’s current personal privacy law to include biological and “neural data” generated by the brain, the spinal cord and the network of nerves that relays messages throughout the body.
“Everything that we are is within our mind,” said Jared Genser, general counsel and co-founder of the Neurorights Foundation, a science group that advocated the bill’s passage. “What we think and feel, and the ability to decode that from the human brain, couldn’t be any more intrusive or personal to us.”
“We are really excited to have an actual bill signed into law that will protect people’s biological and neurological data,” said Representative Cathy Kipp, Democrat of Colorado, who introduced the bill.
Senator Mark Baisley, Republican of Colorado, who sponsored the bill in the upper chamber, said: “I’m feeling really good about Colorado leading the way in addressing this and to give it the due protections for people’s uniqueness in their privacy. I’m just really pleased about this signing.”
The law takes aim at consumer-level brain technologies. Unlike sensitive patient data obtained from medical devices in clinical settings, which are protected by federal health law, the data surrounding consumer neurotechnologies go largely unregulated, Mr. Genser said. That loophole means that companies can harvest vast troves of highly sensitive brain data, sometimes for an unspecified number of years, and share or sell the information to third parties.
Supporters of the bill expressed their concern that neural data could be used to decode a person’s thoughts and feelings or to learn sensitive facts about an individual’s mental health or physical condition, such as whether someone has epilepsy.
“We’ve never seen anything with this power before — to identify, codify people and bias against people based on their brain waves and other neural information,” said Sean Pauzauskie, a member of the board of directors of the Colorado Medical Society, who first brought the issue to Ms. Kipp’s attention. Mr. Pauzauskie was recently hired by the Neurorights Foundation as medical director.
The new law extends to biological and neural data the same protections granted under the Colorado Privacy Act to fingerprints, facial images and other sensitive, biometric data.
Among other protections, consumers have the right to access, delete and correct their data, as well as to opt out of the sale or use of the data for targeted advertising. Companies, in turn, face strict regulations regarding how they handle such data and must disclose the kinds of data they collect and their plans for it.
“Individuals ought to be able to control where that information — that personally identifiable and maybe even personally predictive information — goes,” Mr. Baisley said.
Experts say that the neurotechnology industry is poised to expand as major tech companies like Meta, Apple and Snapchat become involved.
“It’s moving quickly, but it’s about to grow exponentially,” said Nita Farahany, a professor of law and philosophy at Duke.
From 2019 to 2020, investments in neurotechnology companies rose about 60 percent globally, and in 2021 they amounted to about $30 billion, according to one market analysis . The industry drew attention in January, when Elon Musk announced on X that a brain-computer interface manufactured by Neuralink, one of his companies, had been implanted in a person for the first time. Mr. Musk has since said that the patient had made a full recovery and was now able to control a mouse solely with his thoughts and play online chess.
While eerily dystopian, some brain technologies have led to breakthrough treatments. In 2022, a completely paralyzed man was able to communicate using a computer simply by imagining his eyes moving. And last year, scientists were able to translate the brain activity of a paralyzed woman and convey her speech and facial expressions through an avatar on a computer screen.
“The things that people can do with this technology are great,” Ms. Kipp said. “But we just think that there should be some guardrails in place for people who aren’t intending to have their thoughts read and their biological data used.”
That is already happening, according to a 100-page report published on Wednesday by the Neurorights Foundation. The report analyzed 30 consumer neurotechnology companies to see how their privacy policies and user agreements squared with international privacy standards. It found that only one company restricted access to a person’s neural data in a meaningful way and that almost two-thirds could, under certain circumstances, share data with third parties. Two companies implied that they already sold such data.
“The need to protect neural data is not a tomorrow problem — it’s a today problem,” said Mr. Genser, who was among the authors of the report.
The new Colorado bill won resounding bipartisan support, but it faced fierce external opposition, Mr. Baisley said, especially from private universities.
Testifying before a Senate committee, John Seward, research compliance officer at the University of Denver, a private research university, noted that public universities were exempt from the Colorado Privacy Act of 2021. The new law puts private institutions at a disadvantage, Mr. Seward testified, because they will be limited in their ability to train students who are using “the tools of the trade in neural diagnostics and research” purely for research and teaching purposes.
“The playing field is not equal,” Mr. Seward testified.
The Colorado bill is the first of its kind to be signed into law in the United States, but Minnesota and California are pushing for similar legislation. On Tuesday, California’s Senate Judiciary Committee unanimously passed a bill that defines neural data as “sensitive personal information .” Several countries, including Chile, Brazil, Spain, Mexico and Uruguay, have either already enshrined protections on brain-related data in their state-level or national constitutions or taken steps toward doing so.
“In the long run,” Mr. Genser said, “we would like to see global standards developed,” for instance by extending existing international human rights treaties to protect neural data.
In the United States, proponents of the new Colorado law hope it will establish a precedent for other states and even create momentum for federal legislation. But the law has limitations, experts noted, and might apply only to consumer neurotechnology companies that are gathering neural data specifically to determine a person’s identity, as the new law specifies. Most of these companies collect neural data for other reasons, such as for inferring what a person might be thinking or feeling, Ms. Farahany said.
“You’re not going to worry about this Colorado bill if you’re any of those companies right now, because none of them are using them for identification purposes,” she added.
But Mr. Genser said that the Colorado Privacy Act law protects any data that qualifies as personal. Given that consumers must supply their names in order to purchase a product and agree to company privacy policies, this use falls under personal data, he said.
“Given that previously neural data from consumers wasn’t protected at all under the Colorado Privacy Act,” Mr. Genser wrote in an email, “to now have it labeled sensitive personal information with equivalent protections as biometric data is a major step forward.”
In a parallel Colorado bill , the American Civil Liberties Union and other human-rights organizations are pressing for more stringent policies surrounding collection, retention, storage and use of all biometric data, whether for identification purposes or not. If the bill passes, its legal implications would apply to neural data.
Big tech companies played a role in shaping the new law, arguing that it was overly broad and risked harming their ability to collect data not strictly related to brain activity.
TechNet, a policy network representing companies such as Apple, Meta and Open AI, successfully pushed to include language focusing the law on regulating brain data used to identify individuals. But the group failed to remove language governing data generated by “an individual’s body or bodily functions.”
“We felt like this could be very broad to a number of things that all of our members do,” said Ruthie Barko, executive director of TechNet for Colorado and the central United States.
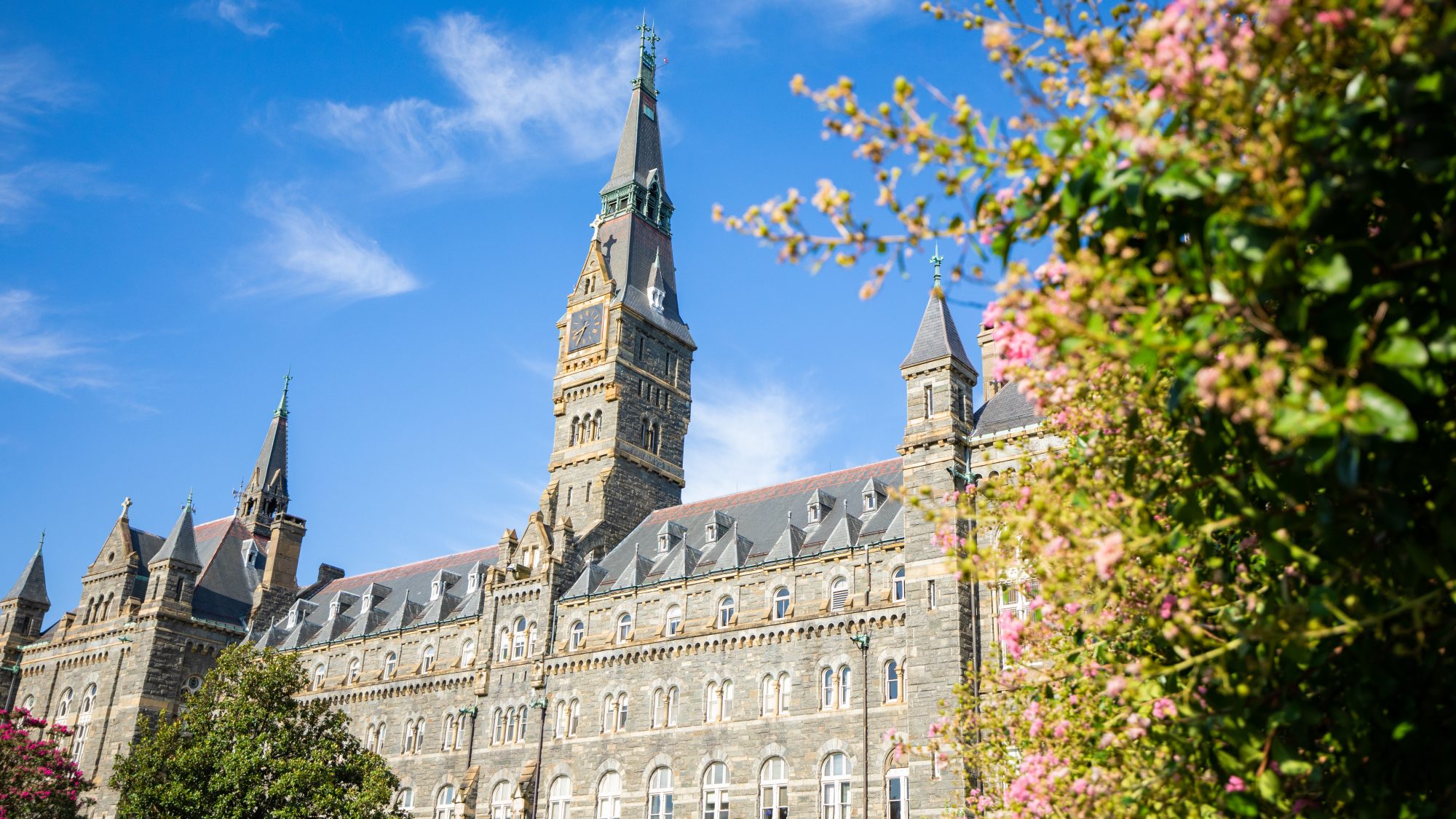
Title: Tech & Public Policy program supports research in election misinformation, improving civic tech and the hidden collection of biometric data
The Tech & Public Policy program at the McCourt School of Public Policy funds $1.5 million in interdisciplinary research projects to address critical questions about technology’s impact on society.
In partnership with Project Liberty’s Institute (formerly the McCourt Institute), the McCourt School’s Tech & Public Policy (TPP) 2024/2025 grant program focused on Technology for the Common Good: Understanding, Designing, Developing and Regulating New Technologies. TPP’s grants explore how technology might better protect values such as democracy, freedom and autonomy.
The TPP program connects threads of research, innovation and engagement to build a vibrant learning community dedicated to examining and advancing policy that addresses the challenges posed by current and future technologies. Through technologists, ethicists, legal scholars and social scientists working in collaboration, TPP grantees consider novel uses and misuses of new digital technologies, their effects on individuals and society, and new governance models to replace outdated regulatory frameworks.
The 2024-2025 Grantees
Building consensus in the digital landscape: the “viewz” platform initiative .
Nejla Asimovic, McCourt School of Public Policy, Georgetown University; Ivan Ivanek, Viewz
Consensus is essential to democratic governance and social cooperation. But polarized settings can make consensus impossible and fuel “us versus them” thinking. This project explores whether technology can be used to highlight divergences of opinion within groups to challenge narratives around homogeneity, among other questions. Using the Viewz platform, a simple online dialogue platform developed by Ivan Ivanek, the research will include a multi-country study that tests different strategies and considers the platform’s potential as a broad model for designing online discourse environments, particularly those that foster a willingness for cross-group collaboration.
Biomanipulation: The Looming Threat Year 2
Laura Donohue, Georgetown University Law Center on National Security
Left in the shadows, the emergence of biomanipulation could have enormous consequences for contemporary social and political structures. This work aims to understand and illuminate the contours and risks of the emerging field of biomanipulation for researchers, policymakers and, ultimately, end-users. She and her research team will build a patent and scientific research database and publish research papers addressing the theoretical underpinnings and technological scope of biomanipulation.
The team will also educate key stakeholders and federal and state policymakers on the risks of biomanipulation and develop policies that legislators and policymakers can use to address these risks and control the spread of biomanipulation.
Can Civic Tech Reduce Administrative Burdens and Increase Trust? An Evaluation of Two Public Interest Technology Solutions
Pamela Herd, Sebastian Jilke and Don Moynihan, McCourt School of Public Policy, Georgetown University
This work will encompass two projects that study digital innovations through collaboration with civic tech and government. Both projects focus on how digital innovations can reduce administrative burdens in the social safety net. The first asks what role Artificial Intelligence (AI) can play in supporting case worker decisions in accessing social safety net programs, and the second project asks if such innovations improve trust in government.
Redesigning the Governance Stack: New Institutional Approaches to Information Economy Harms
Paul Ohm, Julie Cohen and Meg Leta Jones, Georgetown University Law Center
This project is part of a multi-year effort to reinvent the institutions and tools the administrative state uses to govern technology and technology companies, especially given recent advances in AI. This effort will prioritize public accountability and strong public oversight. It will seek to restore and recenter the rule of law within a new institutional framework designed around algorithmically driven information-economy needs and failure modes. It will also be a “full stack” effort, encompassing the sorts of implementation details of shorter-term projects and extending to rethink fundamental principles of regulatory organization and operation.
Exploring the “Collateral Damage” Argument in Internet Censorship Resistance
Micah Sherr, College of Arts & Sciences, Georgetown University
This project proposes to be the first to consider the ethics of the mechanisms and arguments that serve as the foundation for modern censorship-resistant systems (CRSes). A common approach of CRSes is to disguise users’ attempts to access censored content as requests to allowed resources, such as someone trying to access Amazon Web Services (AWS). The entity that is censoring may choose to block access to AWS en masse which would carry potentially enormous collateral damage since all access to AWS would then be affected. The assumption that CRS designers make is that this collateral damage is too politically, economically or socially expensive for the censor, and thus, the censor will not block the CRS. This is the “collateral damage argument” that serves as the foundation of most CRS approaches. The ethics of relying on collateral damage to resist Internet censorship has not been studied in the academic literature, despite its serving as a foundation for many commonly used censorship-resistance systems. The central research focus of this project is to improve our understanding of the ethics of the collateral damage argument and quantify its potential impact.
This interdisciplinary project will tackle the problem of internet censorship from both technical and philosophical directions and focus on two main research thrusts. In thrust 1, the researchers will introduce new methods and models to improve our understanding of how current censorship-resistant systems (CRSes) apply the collateral damage argument and the associated risks to various stakeholders if CRSes’ assumptions fail to hold. Thrust 2 will present a framework for building more ethical CRSes, including the development of principles for obtaining informed consent and the construction of alternative architectures for CRSes that avoid the collateral damage argument entirely.
Generative AI, Humanness, and Misinformation in the 2024 U.S. Presidential Election
Lisa Singh, Department of Computer Science and McCourt School of Public Policy, Georgetown University; Tiago Ventura, McCourt School of Public Policy, Georgetown University; Leticia Bode, Communication, Culture, and Technology, Georgetown University
This project seeks to understand the nature of content related to the 2024 U.S. presidential election shared on social media platforms on two key dimensions — the extent to which it is true or misleading and the extent to which it is perceived as human.
Misinformation is important to identify because it could misinform people in ways that could either disenfranchise them, undermine their trust in the electoral process or change their vote choice.
While humanness is not a perfect proxy for AI-generated content, it does reflect the typical user experience with that content. A social media user encountering such content will not know whether the content is generated by AI but will be able to perceive whether it feels composed by a human.”
USDS Founding, Primary Source Archive
Emily Tavoulareas, Tech & Society and McCourt School of Public Policy, Georgetown University; Kathy Pham, Workday and Harvard Kennedy School
This project aims to create a repository of primary research related to the founding of the United States Digital Service and synthesized assets, such as publications, interviews, reports and more, that scholars can cite in the future.
Sign up for the Tech & Public Policy program newsletter here to stay updated on events and news.
- McCourt School News ,
- Tech & Public Policy
Numbers, Facts and Trends Shaping Your World
Read our research on:
Full Topic List
Regions & Countries
- Publications
- Our Methods
- Short Reads
- Tools & Resources
Read Our Research On:
Partisan divides over K-12 education in 8 charts
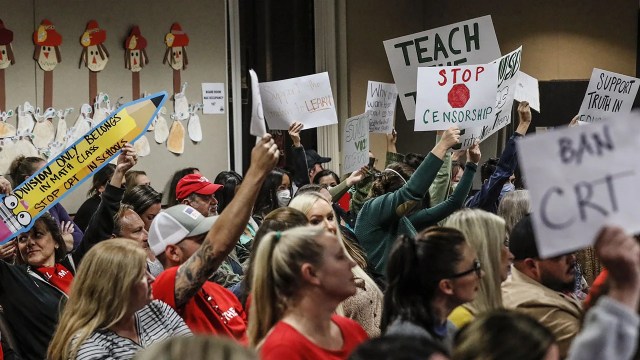
K-12 education is shaping up to be a key issue in the 2024 election cycle. Several prominent Republican leaders, including GOP presidential candidates, have sought to limit discussion of gender identity and race in schools , while the Biden administration has called for expanded protections for transgender students . The coronavirus pandemic also brought out partisan divides on many issues related to K-12 schools .
Today, the public is sharply divided along partisan lines on topics ranging from what should be taught in schools to how much influence parents should have over the curriculum. Here are eight charts that highlight partisan differences over K-12 education, based on recent surveys by Pew Research Center and external data.
Pew Research Center conducted this analysis to provide a snapshot of partisan divides in K-12 education in the run-up to the 2024 election. The analysis is based on data from various Center surveys and analyses conducted from 2021 to 2023, as well as survey data from Education Next, a research journal about education policy. Links to the methodology and questions for each survey or analysis can be found in the text of this analysis.
Most Democrats say K-12 schools are having a positive effect on the country , but a majority of Republicans say schools are having a negative effect, according to a Pew Research Center survey from October 2022. About seven-in-ten Democrats and Democratic-leaning independents (72%) said K-12 public schools were having a positive effect on the way things were going in the United States. About six-in-ten Republicans and GOP leaners (61%) said K-12 schools were having a negative effect.
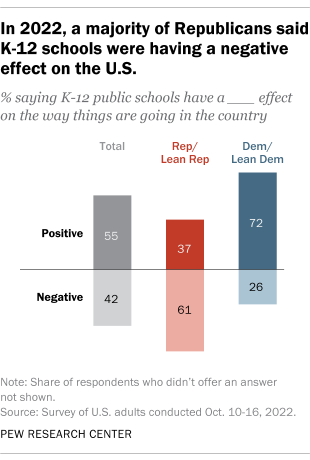
About six-in-ten Democrats (62%) have a favorable opinion of the U.S. Department of Education , while a similar share of Republicans (65%) see it negatively, according to a March 2023 survey by the Center. Democrats and Republicans were more divided over the Department of Education than most of the other 15 federal departments and agencies the Center asked about.
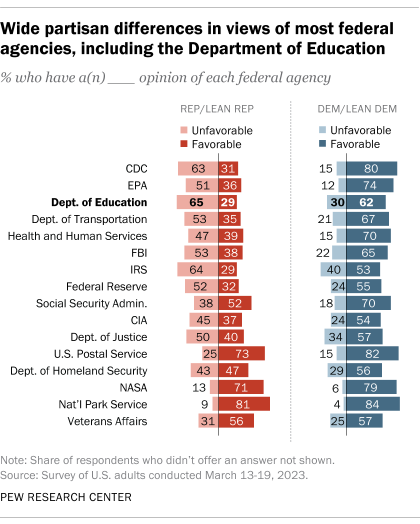
In May 2023, after the survey was conducted, Republican lawmakers scrutinized the Department of Education’s priorities during a House Committee on Education and the Workforce hearing. The lawmakers pressed U.S. Secretary of Education Miguel Cardona on topics including transgender students’ participation in sports and how race-related concepts are taught in schools, while Democratic lawmakers focused on school shootings.
Partisan opinions of K-12 principals have become more divided. In a December 2021 Center survey, about three-quarters of Democrats (76%) expressed a great deal or fair amount of confidence in K-12 principals to act in the best interests of the public. A much smaller share of Republicans (52%) said the same. And nearly half of Republicans (47%) had not too much or no confidence at all in principals, compared with about a quarter of Democrats (24%).
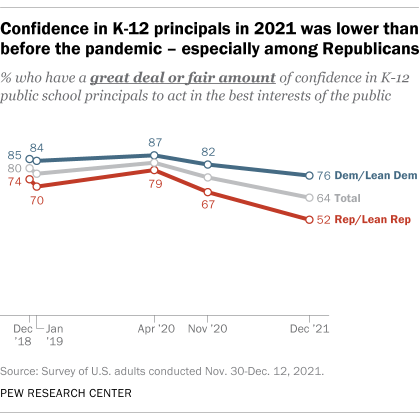
This divide grew between April 2020 and December 2021. While confidence in K-12 principals declined significantly among people in both parties during that span, it fell by 27 percentage points among Republicans, compared with an 11-point decline among Democrats.
Democrats are much more likely than Republicans to say teachers’ unions are having a positive effect on schools. In a May 2022 survey by Education Next , 60% of Democrats said this, compared with 22% of Republicans. Meanwhile, 53% of Republicans and 17% of Democrats said that teachers’ unions were having a negative effect on schools. (In this survey, too, Democrats and Republicans include independents who lean toward each party.)
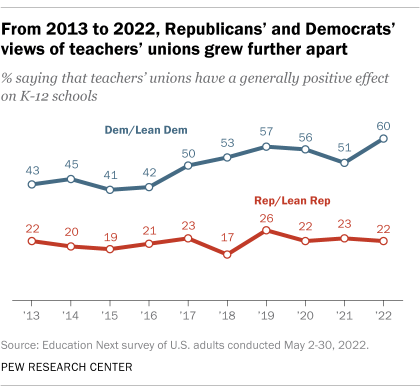
The 38-point difference between Democrats and Republicans on this question was the widest since Education Next first asked it in 2013. However, the gap has exceeded 30 points in four of the last five years for which data is available.
Republican and Democratic parents differ over how much influence they think governments, school boards and others should have on what K-12 schools teach. About half of Republican parents of K-12 students (52%) said in a fall 2022 Center survey that the federal government has too much influence on what their local public schools are teaching, compared with two-in-ten Democratic parents. Republican K-12 parents were also significantly more likely than their Democratic counterparts to say their state government (41% vs. 28%) and their local school board (30% vs. 17%) have too much influence.
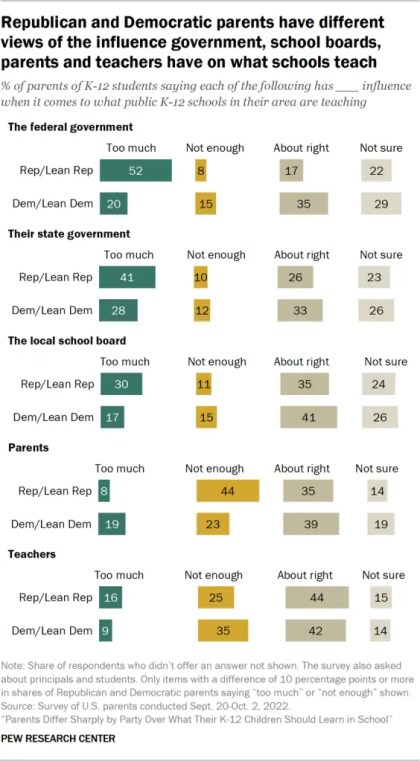
On the other hand, more than four-in-ten Republican parents (44%) said parents themselves don’t have enough influence on what their local K-12 schools teach, compared with roughly a quarter of Democratic parents (23%). A larger share of Democratic parents – about a third (35%) – said teachers don’t have enough influence on what their local schools teach, compared with a quarter of Republican parents who held this view.
Republican and Democratic parents don’t agree on what their children should learn in school about certain topics. Take slavery, for example: While about nine-in-ten parents of K-12 students overall agreed in the fall 2022 survey that their children should learn about it in school, they differed by party over the specifics. About two-thirds of Republican K-12 parents said they would prefer that their children learn that slavery is part of American history but does not affect the position of Black people in American society today. On the other hand, 70% of Democratic parents said they would prefer for their children to learn that the legacy of slavery still affects the position of Black people in American society today.
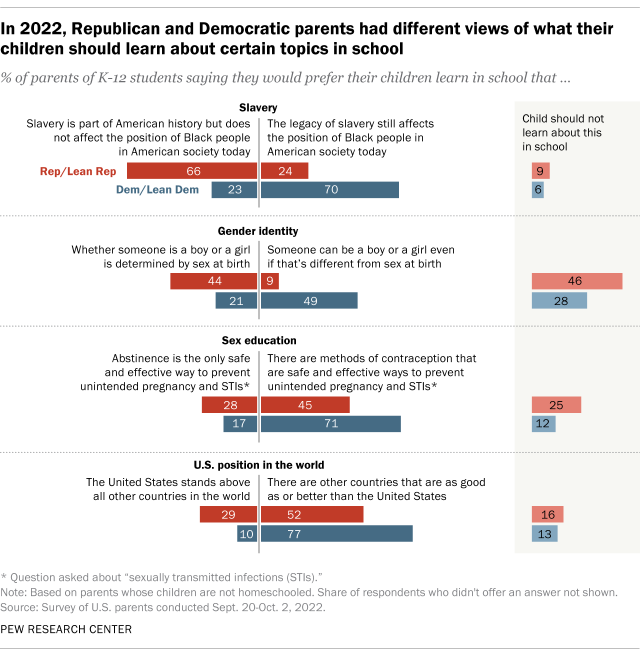
Parents are also divided along partisan lines on the topics of gender identity, sex education and America’s position relative to other countries. Notably, 46% of Republican K-12 parents said their children should not learn about gender identity at all in school, compared with 28% of Democratic parents. Those shares were much larger than the shares of Republican and Democratic parents who said that their children should not learn about the other two topics in school.
Many Republican parents see a place for religion in public schools , whereas a majority of Democratic parents do not. About six-in-ten Republican parents of K-12 students (59%) said in the same survey that public school teachers should be allowed to lead students in Christian prayers, including 29% who said this should be the case even if prayers from other religions are not offered. In contrast, 63% of Democratic parents said that public school teachers should not be allowed to lead students in any type of prayers.

In June 2022, before the Center conducted the survey, the Supreme Court ruled in favor of a football coach at a public high school who had prayed with players at midfield after games. More recently, Texas lawmakers introduced several bills in the 2023 legislative session that would expand the role of religion in K-12 public schools in the state. Those proposals included a bill that would require the Ten Commandments to be displayed in every classroom, a bill that would allow schools to replace guidance counselors with chaplains, and a bill that would allow districts to mandate time during the school day for staff and students to pray and study religious materials.
Mentions of diversity, social-emotional learning and related topics in school mission statements are more common in Democratic areas than in Republican areas. K-12 mission statements from public schools in areas where the majority of residents voted Democratic in the 2020 general election are at least twice as likely as those in Republican-voting areas to include the words “diversity,” “equity” or “inclusion,” according to an April 2023 Pew Research Center analysis .
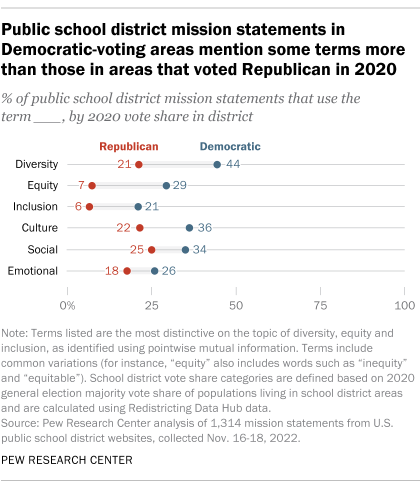
Also, about a third of mission statements in Democratic-voting areas (34%) use the word “social,” compared with a quarter of those in Republican-voting areas, and a similar gap exists for the word “emotional.” Like diversity, equity and inclusion, social-emotional learning is a contentious issue between Democrats and Republicans, even though most K-12 parents think it’s important for their children’s schools to teach these skills . Supporters argue that social-emotional learning helps address mental health needs and student well-being, but some critics consider it emotional manipulation and want it banned.
In contrast, there are broad similarities in school mission statements outside of these hot-button topics. Similar shares of mission statements in Democratic and Republican areas mention students’ future readiness, parent and community involvement, and providing a safe and healthy educational environment for students.
- Education & Politics
- Partisanship & Issues
- Politics & Policy
About 1 in 4 U.S. teachers say their school went into a gun-related lockdown in the last school year
About half of americans say public k-12 education is going in the wrong direction, what public k-12 teachers want americans to know about teaching, what’s it like to be a teacher in america today, race and lgbtq issues in k-12 schools, most popular.
1615 L St. NW, Suite 800 Washington, DC 20036 USA (+1) 202-419-4300 | Main (+1) 202-857-8562 | Fax (+1) 202-419-4372 | Media Inquiries
Research Topics
- Age & Generations
- Coronavirus (COVID-19)
- Economy & Work
- Family & Relationships
- Gender & LGBTQ
- Immigration & Migration
- International Affairs
- Internet & Technology
- Methodological Research
- News Habits & Media
- Non-U.S. Governments
- Other Topics
- Race & Ethnicity
- Email Newsletters
ABOUT PEW RESEARCH CENTER Pew Research Center is a nonpartisan fact tank that informs the public about the issues, attitudes and trends shaping the world. It conducts public opinion polling, demographic research, media content analysis and other empirical social science research. Pew Research Center does not take policy positions. It is a subsidiary of The Pew Charitable Trusts .
Copyright 2024 Pew Research Center
Terms & Conditions
Privacy Policy
Cookie Settings
Reprints, Permissions & Use Policy
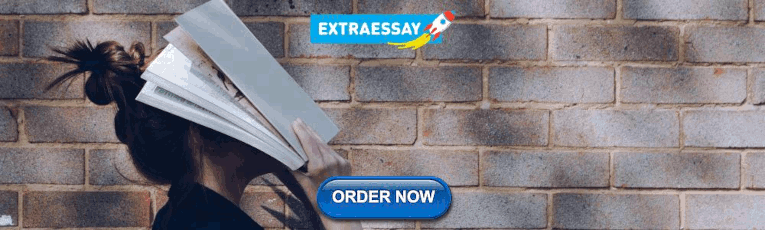
IMAGES
VIDEO
COMMENTS
Collectively grouping biometric data to create archetypes of behaviours that future 'unknown individual' consumers can be ... Finally, the paper outlines a comprehensive research agenda to guide empirical and theoretical investigations into the usage of biometric tracking from a marketing perspective, including its usage and acceptance ...
With an insight into the state-of-the-art research in biometrics for IoT security, this review paper helps advance the study in the field and assists researchers in gaining a good understanding of forward-looking issues and future research directions. Keywords: biometrics, IoT, security, access control, authentication, encryption.
A biometric authentication system has been shown to be a robust method for authentication [11]; however, it was shown that the recognition accuracy performance can be degraded due to biometric data that can be affected by the environment (e.g., illumination conditions) [15], [16] or performance factors (e.g., image quality) [17], as well as factors that may change over time [13], [18].
Abstract—Authentication plays a significant part in dealing with security in public and private sectors such as healthcare systems, banking system, transportation system and law and security. Biometric technology has grown quickly recently, especially in the areas of artificial intelligence and identity. Formerly, authentication process has ...
Biometric systems are increasingly replacing traditional password- and token-based authentication systems. Security and recognition accuracy are the two most important aspects to consider in designing a biometric system. In this paper, a comprehensive review is presented to shed light on the latest developments in the study of fingerprint-based biometrics covering these two aspects with a view ...
In this paper, we present a comprehensive review of the recent advances in biometric recognition using deep learning frameworks. For each work, we provide an overview of the key contributions, network architecture, and loss functions, developed to push state-of-the-art performance in biometric recognition. We have gathered more than 150 papers ...
Search for more papers by this author. Monalisa Sarma, Monalisa Sarma. ... This study presents a novel approach for generating unique identities from multi-modal biometric data using ensemble feature descriptors extracted from the consistent regions of fingerprint and iris images. The method employs prominent feature selection and discriminant ...
Office of Graduate Studies and Research, San José State University, San Jose, CA 95192-0025 USA ... of scientific experimentation and NIST traditions in data evaluation and reporting. We discuss ... We accept for the purposes of this paper the definition of biometrics from ISO/IEC JTC1 N3385, 16 Sept., 2009, as
I noticed some biometric research papers of biometric have explored and improved the corpo-ration of machine learning and biometric. Machine learning can greatly improve efficiency and reduce the cost of data collection. Because of the importance of data collection, some papers are directly dedicated to improving data acquisition
This paper conducts an extensive review of biometric user authentication literature, addressing three primary research questions: (1) commonly used biometric traits and their suitability for specific applications, (2) performance factors such as security, convenience, and robustness, and potential countermeasures against cyberattacks, and (3) factors affecting biometric system accuracy and po ...
1 Introduction. Biometric authentication systems, which identify users based on unique physiological or behavioral characteristics, have emerged as promising solutions to address contemporary security challenges. This paper aims to delve into the integration of biometrics and geospatial data, particularly focusing on automatic fingerprint ...
In order to overcome the difficulty of password management and improve the usability of authentication systems, biometric authentication has been widely studied and has attracted special attention in both academia and industry. Many biometric authentication systems have been researched and developed, especially for mobile devices. However, the existing biometric authentication systems still ...
Various biometric systems used to measure the unique biological and physiological characteristics of individuals to establish their identity. This paper reviews and scrutinizes the current biometrics systems used in the field of security in terms of usability, techniques, accuracy, advantages, and disadvantages. However, due to the diverse ...
This article delves into the complex ethical, legal, and societal dimensions of biometric data, underscoring the critical need for proactive legislation and corporate responsibility. The primary focus is on the unique nature of biometric data, which, due to its immutable and deeply personal characteristics, demands stringent safeguarding measures.
This systematic review aims to discuss past research on the impact of variables in the data acquisition methods of an ECG signal on the biometric recognition process. The paper is organized as follows: Section 2 presents the review methodology. Section 3 provides an overview of ECG acquisition and databases, which are discussed in Section 4.
5 Sensors used in behavioural biometrics. In mobile behavioural biometric studies, data are collected using mobile devices - phones, tablets and wearable devices. In this paper, we will not be looking at traditional biometric modalities. Table 4 Summary of the viewed research papers.
PDF | On Jan 1, 2019, Sunil Swamilingappa Harakannanavar and others published Comprehensive Study of Biometric Authentication Systems, Challenges and Future Trends | Find, read and cite all the ...
Through the. distended addition of computers and In ternet into our ordinary lives, it is essentia l to protect penetrating and p ersonal data. By. exchange PINs (or using biometrics in totalling ...
Authentication plays a significant part in dealing with security in public and private sectors such as healthcare systems, banking system, transportation system and law and security. Biometric technology has grown quickly recently, especially in the areas of artificial intelligence and identity. Formerly, authentication process has depended on security measures like passcodes, identity fobs ...
A number of research articles integrate biometrics into IoT systems to secure IoT devices and applications. Ren et al. [ 9] surveyed the technologies and challenges of applying biometrics to the IoT. Moreover, the authors provided their visions on using biometrics in areas such as big data and mobile cloud computing.
The first four chapters of this report explain much about biometric systems and applications and describe many of the technical, engineering, scientific, and social challenges facing the field. This chapter covers some of the unsolved fundamental problems and research opportunities related to biometric systems, without, however, suggesting that existing systems are not useful or effective. In ...
Fingerprint system is a keenly researched area in biometric technologies [9]. It is one of the most well-known biometrics across the globe for personal recognition and identification of ...
disciplines have expressed concern regarding research that has n ot been reproduced (e.g., [2,3,4]). The reproducibility of research is interwoven with the data used. There are aspects different associated with the reproducibility (or replicability) * of research: • Can the results be corroborated using the . same. data and the . same
Mission. The AI Index report tracks, collates, distills, and visualizes data related to artificial intelligence (AI). Our mission is to provide unbiased, rigorously vetted, broadly sourced data in order for policymakers, researchers, executives, journalists, and the general public to develop a more thorough and nuanced understanding of the complex field of AI.
On Wednesday, Governor Jared Polis of Colorado signed a bill that, for the first time in the United States, tries to ensure that such data remains truly private. The new law, which passed by a 61 ...
In partnership with Project Liberty's Institute (formerly the McCourt Institute), the McCourt School's Tech & Public Policy (TPP) 2024/2025 grant program focused on Technology for the Common Good: Understanding, Designing, Developing and Regulating New Technologies. TPP's grants explore how technology might better protect values such as democracy, freedom and autonomy.
How Pew Research Center will report on generations moving forward. Journalists, researchers and the public often look at society through the lens of generation, using terms like Millennial or Gen Z to describe groups of similarly aged people. This approach can help readers see themselves in the data and assess where we are and where we're ...
Pew Research Center conducted this analysis to provide a snapshot of partisan divides in K-12 education in the run-up to the 2024 election. The analysis is based on data from various Center surveys and analyses conducted from 2021 to 2023, as well as survey data from Education Next, a research journal about education policy.