- Bipolar Disorder
- Therapy Center
- When To See a Therapist
- Types of Therapy
- Best Online Therapy
- Best Couples Therapy
- Best Family Therapy
- Managing Stress
- Sleep and Dreaming
- Understanding Emotions
- Self-Improvement
- Healthy Relationships
- Student Resources
- Personality Types
- Guided Meditations
- Verywell Mind Insights
- 2024 Verywell Mind 25
- Mental Health in the Classroom
- Editorial Process
- Meet Our Review Board
- Crisis Support
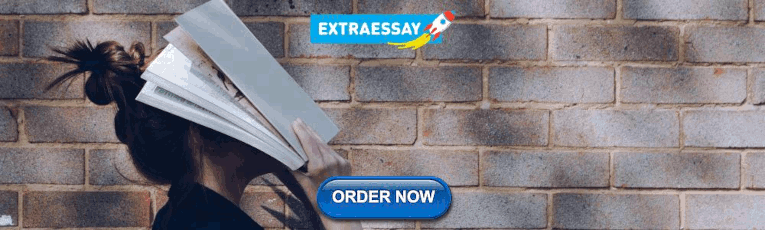
How to Write a Great Hypothesis
Hypothesis Definition, Format, Examples, and Tips
Kendra Cherry, MS, is a psychosocial rehabilitation specialist, psychology educator, and author of the "Everything Psychology Book."
:max_bytes(150000):strip_icc():format(webp)/IMG_9791-89504ab694d54b66bbd72cb84ffb860e.jpg)
Amy Morin, LCSW, is a psychotherapist and international bestselling author. Her books, including "13 Things Mentally Strong People Don't Do," have been translated into more than 40 languages. Her TEDx talk, "The Secret of Becoming Mentally Strong," is one of the most viewed talks of all time.
:max_bytes(150000):strip_icc():format(webp)/VW-MIND-Amy-2b338105f1ee493f94d7e333e410fa76.jpg)
Verywell / Alex Dos Diaz
- The Scientific Method
Hypothesis Format
Falsifiability of a hypothesis.
- Operationalization
Hypothesis Types
Hypotheses examples.
- Collecting Data
A hypothesis is a tentative statement about the relationship between two or more variables. It is a specific, testable prediction about what you expect to happen in a study. It is a preliminary answer to your question that helps guide the research process.
Consider a study designed to examine the relationship between sleep deprivation and test performance. The hypothesis might be: "This study is designed to assess the hypothesis that sleep-deprived people will perform worse on a test than individuals who are not sleep-deprived."
At a Glance
A hypothesis is crucial to scientific research because it offers a clear direction for what the researchers are looking to find. This allows them to design experiments to test their predictions and add to our scientific knowledge about the world. This article explores how a hypothesis is used in psychology research, how to write a good hypothesis, and the different types of hypotheses you might use.
The Hypothesis in the Scientific Method
In the scientific method , whether it involves research in psychology, biology, or some other area, a hypothesis represents what the researchers think will happen in an experiment. The scientific method involves the following steps:
- Forming a question
- Performing background research
- Creating a hypothesis
- Designing an experiment
- Collecting data
- Analyzing the results
- Drawing conclusions
- Communicating the results
The hypothesis is a prediction, but it involves more than a guess. Most of the time, the hypothesis begins with a question which is then explored through background research. At this point, researchers then begin to develop a testable hypothesis.
Unless you are creating an exploratory study, your hypothesis should always explain what you expect to happen.
In a study exploring the effects of a particular drug, the hypothesis might be that researchers expect the drug to have some type of effect on the symptoms of a specific illness. In psychology, the hypothesis might focus on how a certain aspect of the environment might influence a particular behavior.
Remember, a hypothesis does not have to be correct. While the hypothesis predicts what the researchers expect to see, the goal of the research is to determine whether this guess is right or wrong. When conducting an experiment, researchers might explore numerous factors to determine which ones might contribute to the ultimate outcome.
In many cases, researchers may find that the results of an experiment do not support the original hypothesis. When writing up these results, the researchers might suggest other options that should be explored in future studies.
In many cases, researchers might draw a hypothesis from a specific theory or build on previous research. For example, prior research has shown that stress can impact the immune system. So a researcher might hypothesize: "People with high-stress levels will be more likely to contract a common cold after being exposed to the virus than people who have low-stress levels."
In other instances, researchers might look at commonly held beliefs or folk wisdom. "Birds of a feather flock together" is one example of folk adage that a psychologist might try to investigate. The researcher might pose a specific hypothesis that "People tend to select romantic partners who are similar to them in interests and educational level."
Elements of a Good Hypothesis
So how do you write a good hypothesis? When trying to come up with a hypothesis for your research or experiments, ask yourself the following questions:
- Is your hypothesis based on your research on a topic?
- Can your hypothesis be tested?
- Does your hypothesis include independent and dependent variables?
Before you come up with a specific hypothesis, spend some time doing background research. Once you have completed a literature review, start thinking about potential questions you still have. Pay attention to the discussion section in the journal articles you read . Many authors will suggest questions that still need to be explored.
How to Formulate a Good Hypothesis
To form a hypothesis, you should take these steps:
- Collect as many observations about a topic or problem as you can.
- Evaluate these observations and look for possible causes of the problem.
- Create a list of possible explanations that you might want to explore.
- After you have developed some possible hypotheses, think of ways that you could confirm or disprove each hypothesis through experimentation. This is known as falsifiability.
In the scientific method , falsifiability is an important part of any valid hypothesis. In order to test a claim scientifically, it must be possible that the claim could be proven false.
Students sometimes confuse the idea of falsifiability with the idea that it means that something is false, which is not the case. What falsifiability means is that if something was false, then it is possible to demonstrate that it is false.
One of the hallmarks of pseudoscience is that it makes claims that cannot be refuted or proven false.
The Importance of Operational Definitions
A variable is a factor or element that can be changed and manipulated in ways that are observable and measurable. However, the researcher must also define how the variable will be manipulated and measured in the study.
Operational definitions are specific definitions for all relevant factors in a study. This process helps make vague or ambiguous concepts detailed and measurable.
For example, a researcher might operationally define the variable " test anxiety " as the results of a self-report measure of anxiety experienced during an exam. A "study habits" variable might be defined by the amount of studying that actually occurs as measured by time.
These precise descriptions are important because many things can be measured in various ways. Clearly defining these variables and how they are measured helps ensure that other researchers can replicate your results.
Replicability
One of the basic principles of any type of scientific research is that the results must be replicable.
Replication means repeating an experiment in the same way to produce the same results. By clearly detailing the specifics of how the variables were measured and manipulated, other researchers can better understand the results and repeat the study if needed.
Some variables are more difficult than others to define. For example, how would you operationally define a variable such as aggression ? For obvious ethical reasons, researchers cannot create a situation in which a person behaves aggressively toward others.
To measure this variable, the researcher must devise a measurement that assesses aggressive behavior without harming others. The researcher might utilize a simulated task to measure aggressiveness in this situation.
Hypothesis Checklist
- Does your hypothesis focus on something that you can actually test?
- Does your hypothesis include both an independent and dependent variable?
- Can you manipulate the variables?
- Can your hypothesis be tested without violating ethical standards?
The hypothesis you use will depend on what you are investigating and hoping to find. Some of the main types of hypotheses that you might use include:
- Simple hypothesis : This type of hypothesis suggests there is a relationship between one independent variable and one dependent variable.
- Complex hypothesis : This type suggests a relationship between three or more variables, such as two independent and dependent variables.
- Null hypothesis : This hypothesis suggests no relationship exists between two or more variables.
- Alternative hypothesis : This hypothesis states the opposite of the null hypothesis.
- Statistical hypothesis : This hypothesis uses statistical analysis to evaluate a representative population sample and then generalizes the findings to the larger group.
- Logical hypothesis : This hypothesis assumes a relationship between variables without collecting data or evidence.
A hypothesis often follows a basic format of "If {this happens} then {this will happen}." One way to structure your hypothesis is to describe what will happen to the dependent variable if you change the independent variable .
The basic format might be: "If {these changes are made to a certain independent variable}, then we will observe {a change in a specific dependent variable}."
A few examples of simple hypotheses:
- "Students who eat breakfast will perform better on a math exam than students who do not eat breakfast."
- "Students who experience test anxiety before an English exam will get lower scores than students who do not experience test anxiety."
- "Motorists who talk on the phone while driving will be more likely to make errors on a driving course than those who do not talk on the phone."
- "Children who receive a new reading intervention will have higher reading scores than students who do not receive the intervention."
Examples of a complex hypothesis include:
- "People with high-sugar diets and sedentary activity levels are more likely to develop depression."
- "Younger people who are regularly exposed to green, outdoor areas have better subjective well-being than older adults who have limited exposure to green spaces."
Examples of a null hypothesis include:
- "There is no difference in anxiety levels between people who take St. John's wort supplements and those who do not."
- "There is no difference in scores on a memory recall task between children and adults."
- "There is no difference in aggression levels between children who play first-person shooter games and those who do not."
Examples of an alternative hypothesis:
- "People who take St. John's wort supplements will have less anxiety than those who do not."
- "Adults will perform better on a memory task than children."
- "Children who play first-person shooter games will show higher levels of aggression than children who do not."
Collecting Data on Your Hypothesis
Once a researcher has formed a testable hypothesis, the next step is to select a research design and start collecting data. The research method depends largely on exactly what they are studying. There are two basic types of research methods: descriptive research and experimental research.
Descriptive Research Methods
Descriptive research such as case studies , naturalistic observations , and surveys are often used when conducting an experiment is difficult or impossible. These methods are best used to describe different aspects of a behavior or psychological phenomenon.
Once a researcher has collected data using descriptive methods, a correlational study can examine how the variables are related. This research method might be used to investigate a hypothesis that is difficult to test experimentally.
Experimental Research Methods
Experimental methods are used to demonstrate causal relationships between variables. In an experiment, the researcher systematically manipulates a variable of interest (known as the independent variable) and measures the effect on another variable (known as the dependent variable).
Unlike correlational studies, which can only be used to determine if there is a relationship between two variables, experimental methods can be used to determine the actual nature of the relationship—whether changes in one variable actually cause another to change.
The hypothesis is a critical part of any scientific exploration. It represents what researchers expect to find in a study or experiment. In situations where the hypothesis is unsupported by the research, the research still has value. Such research helps us better understand how different aspects of the natural world relate to one another. It also helps us develop new hypotheses that can then be tested in the future.
Thompson WH, Skau S. On the scope of scientific hypotheses . R Soc Open Sci . 2023;10(8):230607. doi:10.1098/rsos.230607
Taran S, Adhikari NKJ, Fan E. Falsifiability in medicine: what clinicians can learn from Karl Popper [published correction appears in Intensive Care Med. 2021 Jun 17;:]. Intensive Care Med . 2021;47(9):1054-1056. doi:10.1007/s00134-021-06432-z
Eyler AA. Research Methods for Public Health . 1st ed. Springer Publishing Company; 2020. doi:10.1891/9780826182067.0004
Nosek BA, Errington TM. What is replication ? PLoS Biol . 2020;18(3):e3000691. doi:10.1371/journal.pbio.3000691
Aggarwal R, Ranganathan P. Study designs: Part 2 - Descriptive studies . Perspect Clin Res . 2019;10(1):34-36. doi:10.4103/picr.PICR_154_18
Nevid J. Psychology: Concepts and Applications. Wadworth, 2013.
By Kendra Cherry, MSEd Kendra Cherry, MS, is a psychosocial rehabilitation specialist, psychology educator, and author of the "Everything Psychology Book."
Research Hypothesis In Psychology: Types, & Examples
Saul Mcleod, PhD
Editor-in-Chief for Simply Psychology
BSc (Hons) Psychology, MRes, PhD, University of Manchester
Saul Mcleod, PhD., is a qualified psychology teacher with over 18 years of experience in further and higher education. He has been published in peer-reviewed journals, including the Journal of Clinical Psychology.
Learn about our Editorial Process
Olivia Guy-Evans, MSc
Associate Editor for Simply Psychology
BSc (Hons) Psychology, MSc Psychology of Education
Olivia Guy-Evans is a writer and associate editor for Simply Psychology. She has previously worked in healthcare and educational sectors.
On This Page:
A research hypothesis, in its plural form “hypotheses,” is a specific, testable prediction about the anticipated results of a study, established at its outset. It is a key component of the scientific method .
Hypotheses connect theory to data and guide the research process towards expanding scientific understanding
Some key points about hypotheses:
- A hypothesis expresses an expected pattern or relationship. It connects the variables under investigation.
- It is stated in clear, precise terms before any data collection or analysis occurs. This makes the hypothesis testable.
- A hypothesis must be falsifiable. It should be possible, even if unlikely in practice, to collect data that disconfirms rather than supports the hypothesis.
- Hypotheses guide research. Scientists design studies to explicitly evaluate hypotheses about how nature works.
- For a hypothesis to be valid, it must be testable against empirical evidence. The evidence can then confirm or disprove the testable predictions.
- Hypotheses are informed by background knowledge and observation, but go beyond what is already known to propose an explanation of how or why something occurs.
Predictions typically arise from a thorough knowledge of the research literature, curiosity about real-world problems or implications, and integrating this to advance theory. They build on existing literature while providing new insight.
Types of Research Hypotheses
Alternative hypothesis.
The research hypothesis is often called the alternative or experimental hypothesis in experimental research.
It typically suggests a potential relationship between two key variables: the independent variable, which the researcher manipulates, and the dependent variable, which is measured based on those changes.
The alternative hypothesis states a relationship exists between the two variables being studied (one variable affects the other).
A hypothesis is a testable statement or prediction about the relationship between two or more variables. It is a key component of the scientific method. Some key points about hypotheses:
- Important hypotheses lead to predictions that can be tested empirically. The evidence can then confirm or disprove the testable predictions.
In summary, a hypothesis is a precise, testable statement of what researchers expect to happen in a study and why. Hypotheses connect theory to data and guide the research process towards expanding scientific understanding.
An experimental hypothesis predicts what change(s) will occur in the dependent variable when the independent variable is manipulated.
It states that the results are not due to chance and are significant in supporting the theory being investigated.
The alternative hypothesis can be directional, indicating a specific direction of the effect, or non-directional, suggesting a difference without specifying its nature. It’s what researchers aim to support or demonstrate through their study.
Null Hypothesis
The null hypothesis states no relationship exists between the two variables being studied (one variable does not affect the other). There will be no changes in the dependent variable due to manipulating the independent variable.
It states results are due to chance and are not significant in supporting the idea being investigated.
The null hypothesis, positing no effect or relationship, is a foundational contrast to the research hypothesis in scientific inquiry. It establishes a baseline for statistical testing, promoting objectivity by initiating research from a neutral stance.
Many statistical methods are tailored to test the null hypothesis, determining the likelihood of observed results if no true effect exists.
This dual-hypothesis approach provides clarity, ensuring that research intentions are explicit, and fosters consistency across scientific studies, enhancing the standardization and interpretability of research outcomes.
Nondirectional Hypothesis
A non-directional hypothesis, also known as a two-tailed hypothesis, predicts that there is a difference or relationship between two variables but does not specify the direction of this relationship.
It merely indicates that a change or effect will occur without predicting which group will have higher or lower values.
For example, “There is a difference in performance between Group A and Group B” is a non-directional hypothesis.
Directional Hypothesis
A directional (one-tailed) hypothesis predicts the nature of the effect of the independent variable on the dependent variable. It predicts in which direction the change will take place. (i.e., greater, smaller, less, more)
It specifies whether one variable is greater, lesser, or different from another, rather than just indicating that there’s a difference without specifying its nature.
For example, “Exercise increases weight loss” is a directional hypothesis.
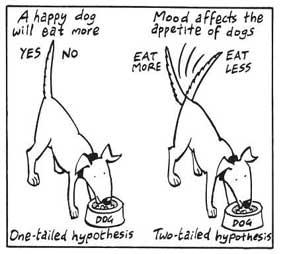
Falsifiability
The Falsification Principle, proposed by Karl Popper , is a way of demarcating science from non-science. It suggests that for a theory or hypothesis to be considered scientific, it must be testable and irrefutable.
Falsifiability emphasizes that scientific claims shouldn’t just be confirmable but should also have the potential to be proven wrong.
It means that there should exist some potential evidence or experiment that could prove the proposition false.
However many confirming instances exist for a theory, it only takes one counter observation to falsify it. For example, the hypothesis that “all swans are white,” can be falsified by observing a black swan.
For Popper, science should attempt to disprove a theory rather than attempt to continually provide evidence to support a research hypothesis.
Can a Hypothesis be Proven?
Hypotheses make probabilistic predictions. They state the expected outcome if a particular relationship exists. However, a study result supporting a hypothesis does not definitively prove it is true.
All studies have limitations. There may be unknown confounding factors or issues that limit the certainty of conclusions. Additional studies may yield different results.
In science, hypotheses can realistically only be supported with some degree of confidence, not proven. The process of science is to incrementally accumulate evidence for and against hypothesized relationships in an ongoing pursuit of better models and explanations that best fit the empirical data. But hypotheses remain open to revision and rejection if that is where the evidence leads.
- Disproving a hypothesis is definitive. Solid disconfirmatory evidence will falsify a hypothesis and require altering or discarding it based on the evidence.
- However, confirming evidence is always open to revision. Other explanations may account for the same results, and additional or contradictory evidence may emerge over time.
We can never 100% prove the alternative hypothesis. Instead, we see if we can disprove, or reject the null hypothesis.
If we reject the null hypothesis, this doesn’t mean that our alternative hypothesis is correct but does support the alternative/experimental hypothesis.
Upon analysis of the results, an alternative hypothesis can be rejected or supported, but it can never be proven to be correct. We must avoid any reference to results proving a theory as this implies 100% certainty, and there is always a chance that evidence may exist which could refute a theory.
How to Write a Hypothesis
- Identify variables . The researcher manipulates the independent variable and the dependent variable is the measured outcome.
- Operationalized the variables being investigated . Operationalization of a hypothesis refers to the process of making the variables physically measurable or testable, e.g. if you are about to study aggression, you might count the number of punches given by participants.
- Decide on a direction for your prediction . If there is evidence in the literature to support a specific effect of the independent variable on the dependent variable, write a directional (one-tailed) hypothesis. If there are limited or ambiguous findings in the literature regarding the effect of the independent variable on the dependent variable, write a non-directional (two-tailed) hypothesis.
- Make it Testable : Ensure your hypothesis can be tested through experimentation or observation. It should be possible to prove it false (principle of falsifiability).
- Clear & concise language . A strong hypothesis is concise (typically one to two sentences long), and formulated using clear and straightforward language, ensuring it’s easily understood and testable.
Consider a hypothesis many teachers might subscribe to: students work better on Monday morning than on Friday afternoon (IV=Day, DV= Standard of work).
Now, if we decide to study this by giving the same group of students a lesson on a Monday morning and a Friday afternoon and then measuring their immediate recall of the material covered in each session, we would end up with the following:
- The alternative hypothesis states that students will recall significantly more information on a Monday morning than on a Friday afternoon.
- The null hypothesis states that there will be no significant difference in the amount recalled on a Monday morning compared to a Friday afternoon. Any difference will be due to chance or confounding factors.
More Examples
- Memory : Participants exposed to classical music during study sessions will recall more items from a list than those who studied in silence.
- Social Psychology : Individuals who frequently engage in social media use will report higher levels of perceived social isolation compared to those who use it infrequently.
- Developmental Psychology : Children who engage in regular imaginative play have better problem-solving skills than those who don’t.
- Clinical Psychology : Cognitive-behavioral therapy will be more effective in reducing symptoms of anxiety over a 6-month period compared to traditional talk therapy.
- Cognitive Psychology : Individuals who multitask between various electronic devices will have shorter attention spans on focused tasks than those who single-task.
- Health Psychology : Patients who practice mindfulness meditation will experience lower levels of chronic pain compared to those who don’t meditate.
- Organizational Psychology : Employees in open-plan offices will report higher levels of stress than those in private offices.
- Behavioral Psychology : Rats rewarded with food after pressing a lever will press it more frequently than rats who receive no reward.

Related Articles
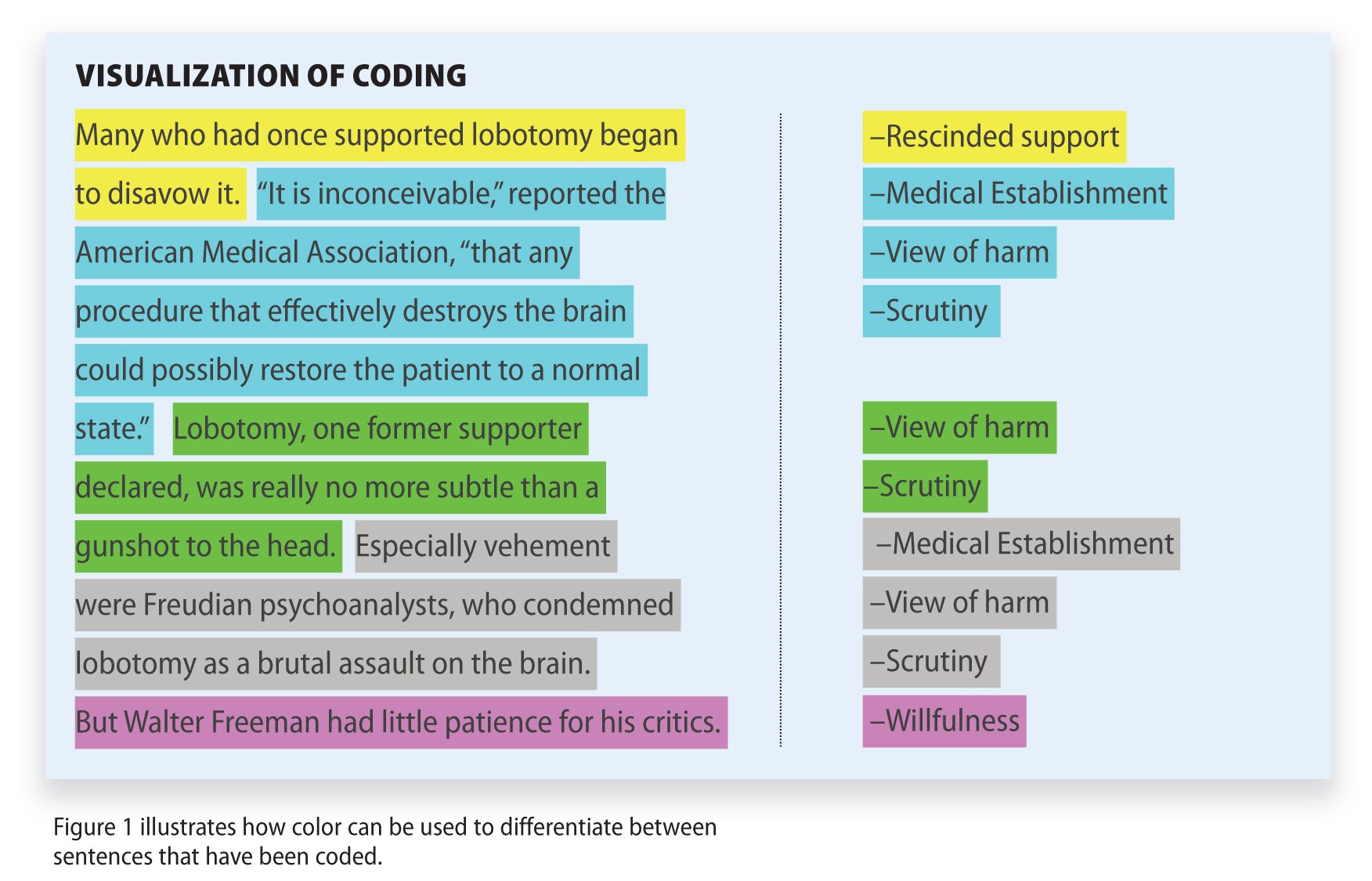
Research Methodology
Qualitative Data Coding
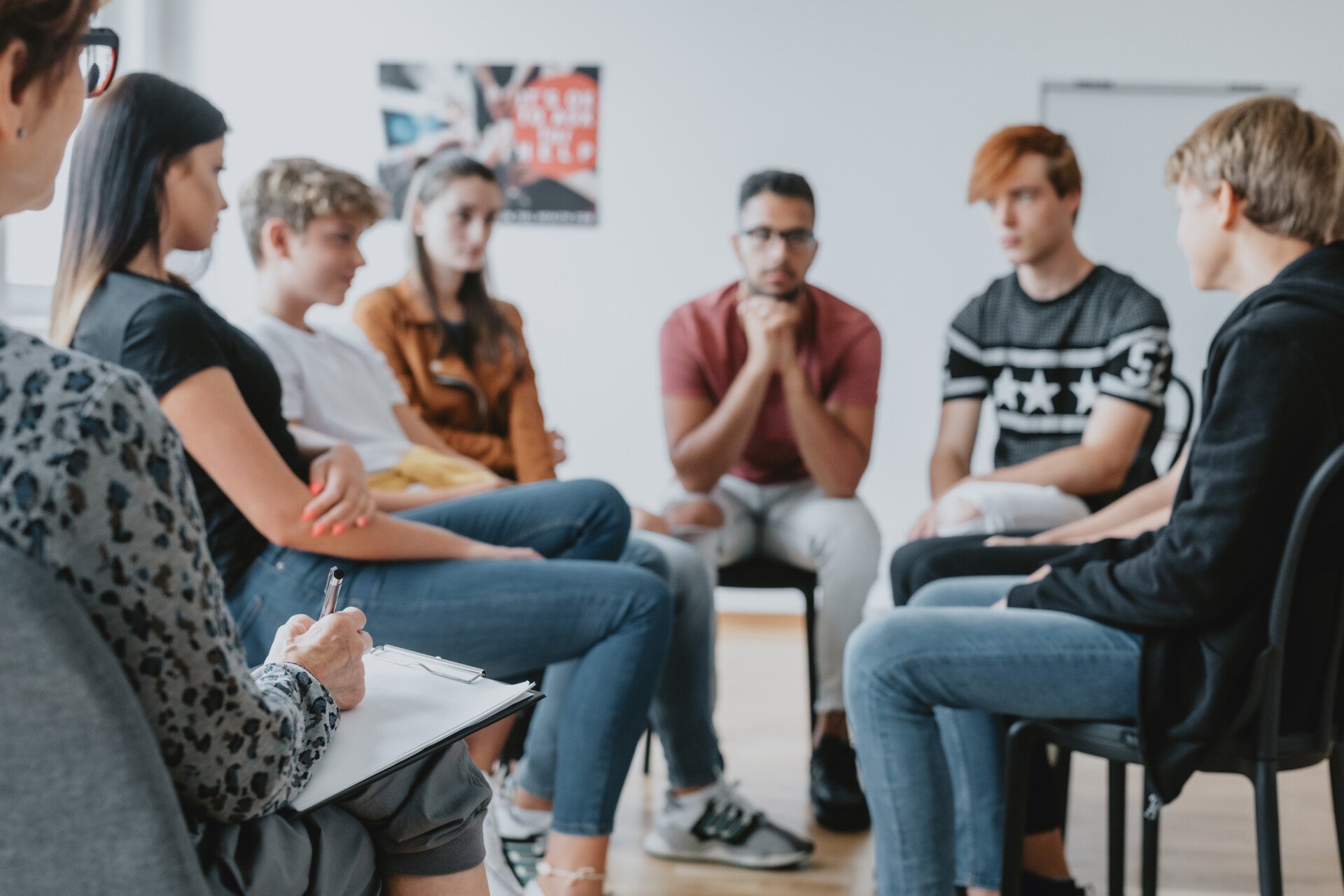
What Is a Focus Group?
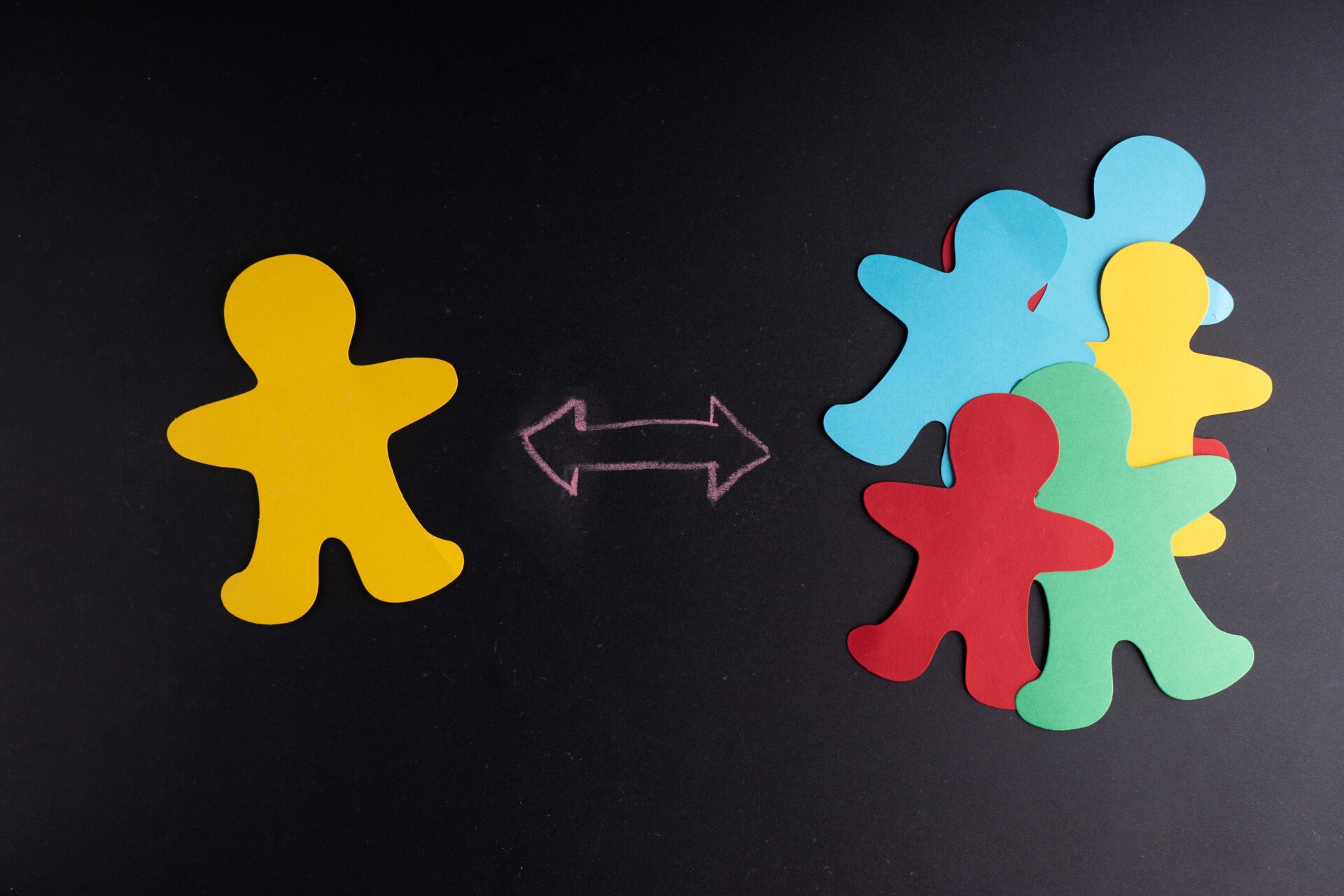
Cross-Cultural Research Methodology In Psychology
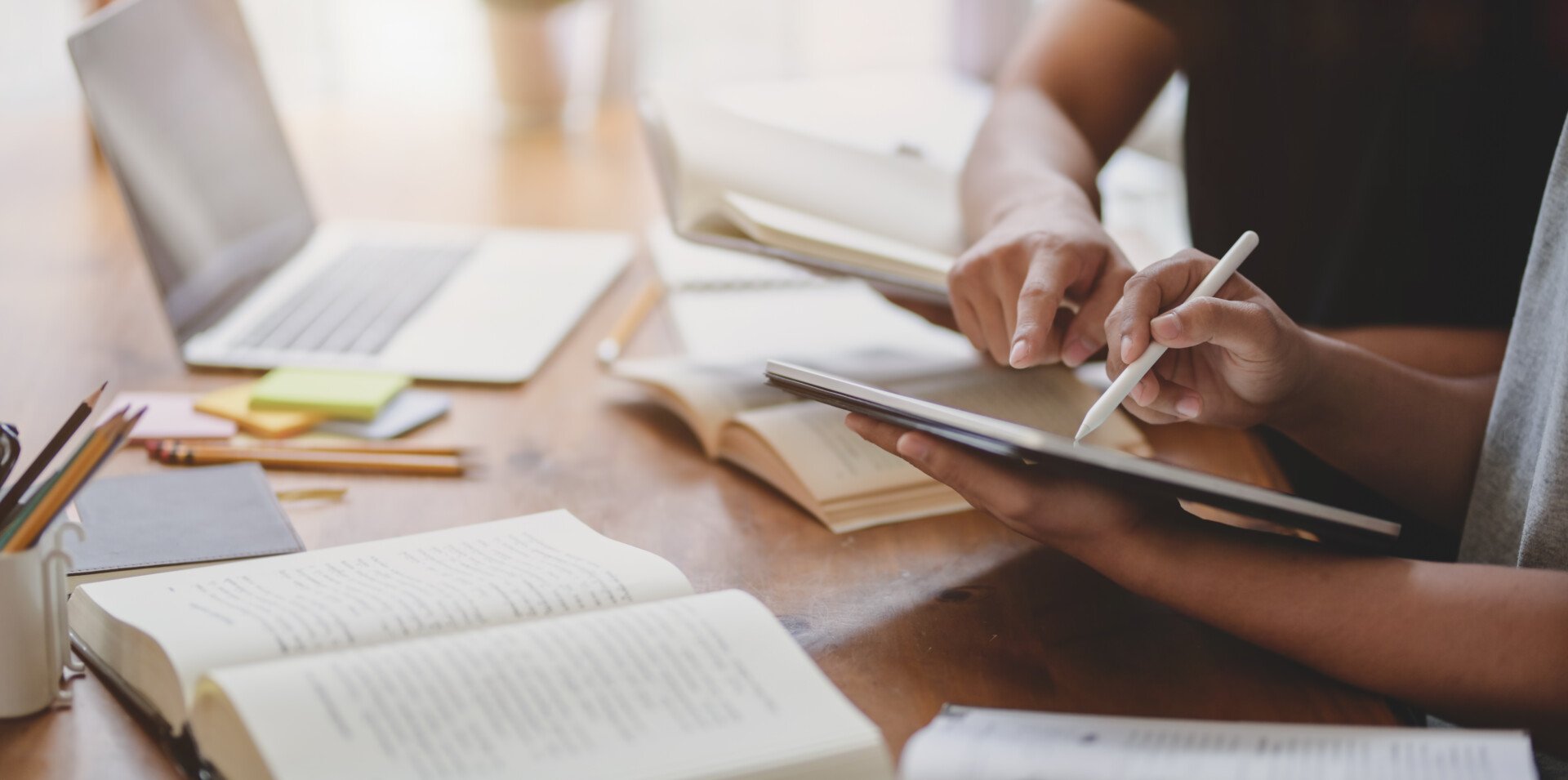
What Is Internal Validity In Research?
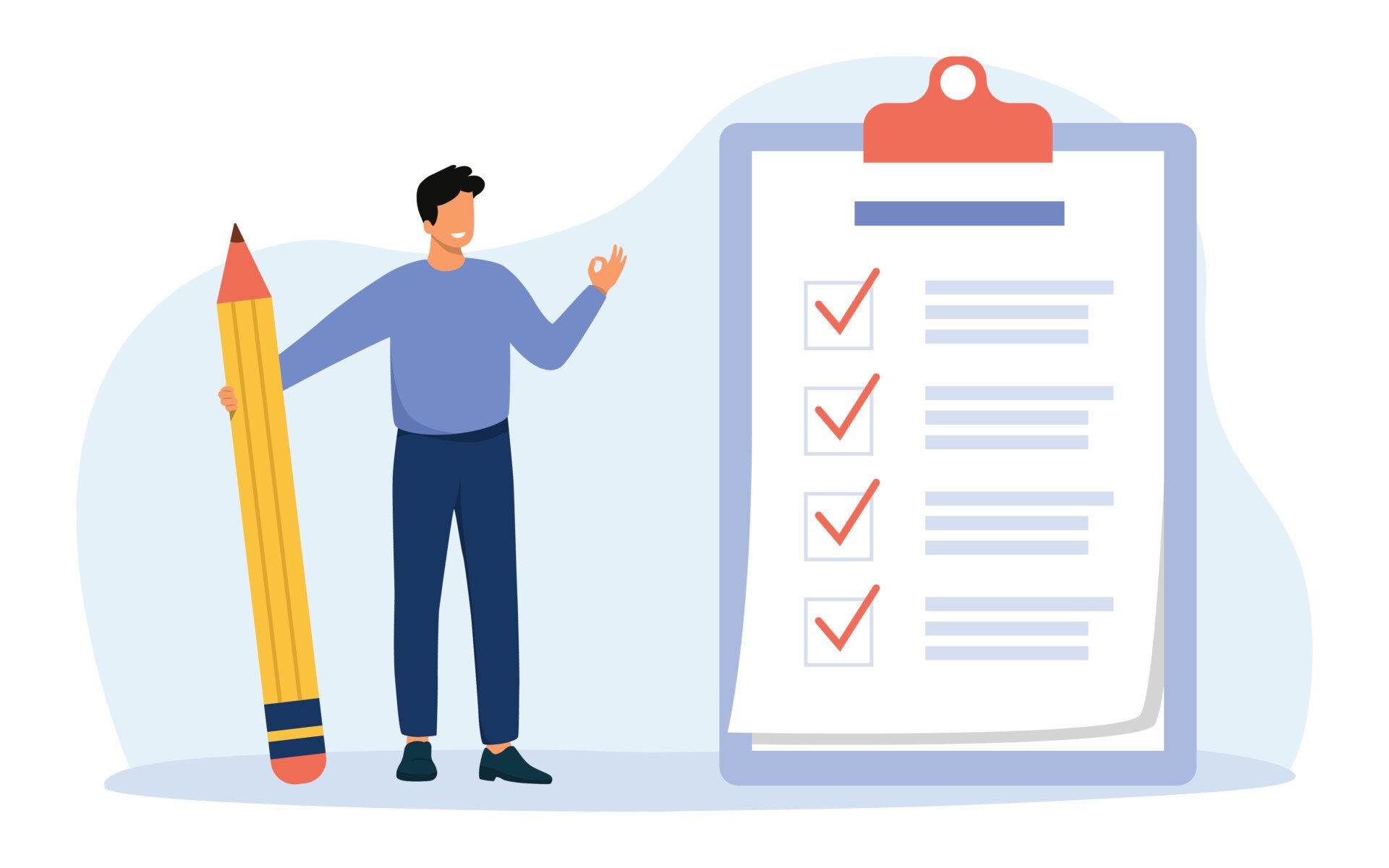
Research Methodology , Statistics
What Is Face Validity In Research? Importance & How To Measure
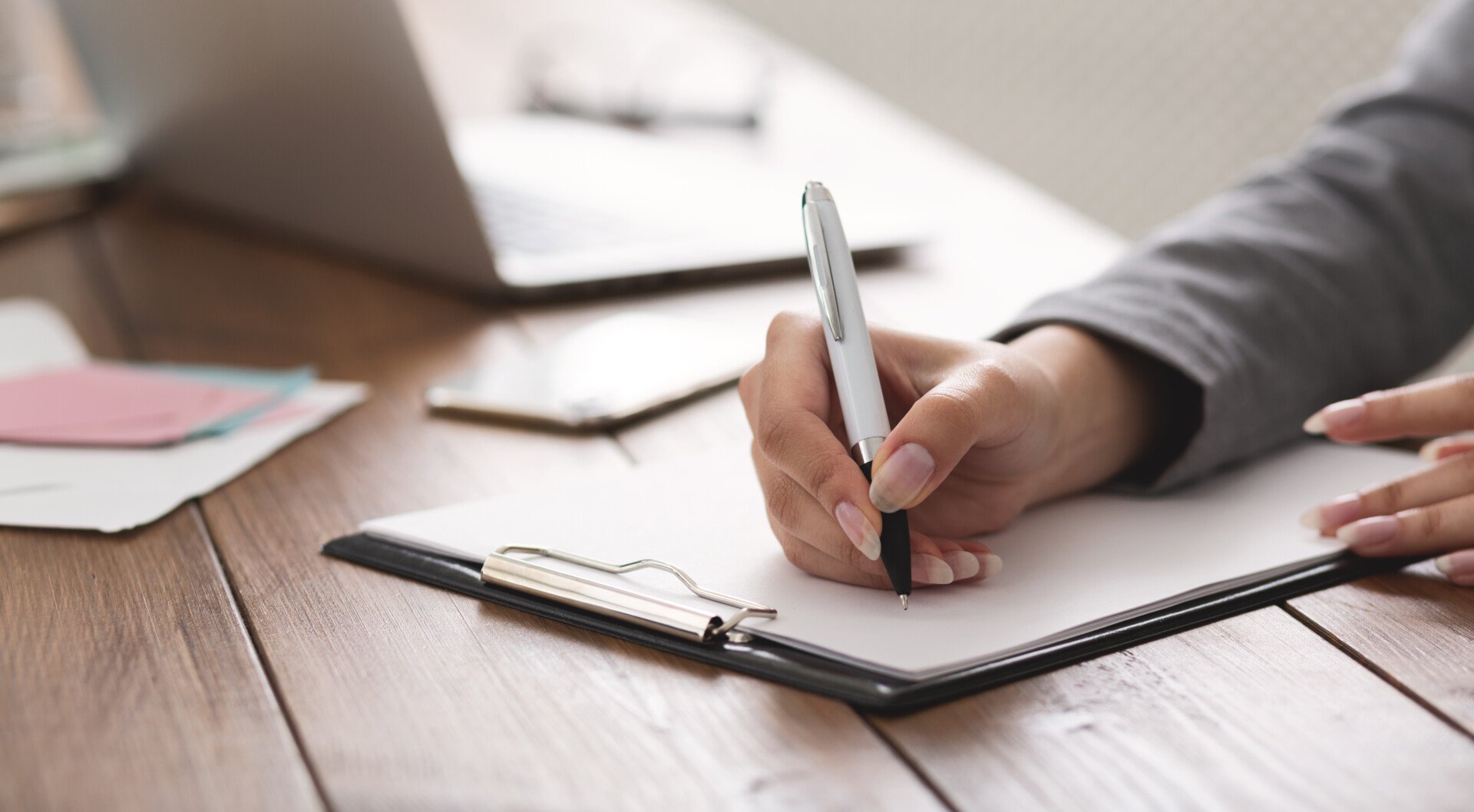
Criterion Validity: Definition & Examples

- Science Notes Posts
- Contact Science Notes
- Todd Helmenstine Biography
- Anne Helmenstine Biography
- Free Printable Periodic Tables (PDF and PNG)
- Periodic Table Wallpapers
- Interactive Periodic Table
- Periodic Table Posters
- How to Grow Crystals
- Chemistry Projects
- Fire and Flames Projects
- Holiday Science
- Chemistry Problems With Answers
- Physics Problems
- Unit Conversion Example Problems
- Chemistry Worksheets
- Biology Worksheets
- Periodic Table Worksheets
- Physical Science Worksheets
- Science Lab Worksheets
- My Amazon Books
Hypothesis Examples

A hypothesis is a prediction of the outcome of a test. It forms the basis for designing an experiment in the scientific method . A good hypothesis is testable, meaning it makes a prediction you can check with observation or experimentation. Here are different hypothesis examples.
Null Hypothesis Examples
The null hypothesis (H 0 ) is also known as the zero-difference or no-difference hypothesis. It predicts that changing one variable ( independent variable ) will have no effect on the variable being measured ( dependent variable ). Here are null hypothesis examples:
- Plant growth is unaffected by temperature.
- If you increase temperature, then solubility of salt will increase.
- Incidence of skin cancer is unrelated to ultraviolet light exposure.
- All brands of light bulb last equally long.
- Cats have no preference for the color of cat food.
- All daisies have the same number of petals.
Sometimes the null hypothesis shows there is a suspected correlation between two variables. For example, if you think plant growth is affected by temperature, you state the null hypothesis: “Plant growth is not affected by temperature.” Why do you do this, rather than say “If you change temperature, plant growth will be affected”? The answer is because it’s easier applying a statistical test that shows, with a high level of confidence, a null hypothesis is correct or incorrect.
Research Hypothesis Examples
A research hypothesis (H 1 ) is a type of hypothesis used to design an experiment. This type of hypothesis is often written as an if-then statement because it’s easy identifying the independent and dependent variables and seeing how one affects the other. If-then statements explore cause and effect. In other cases, the hypothesis shows a correlation between two variables. Here are some research hypothesis examples:
- If you leave the lights on, then it takes longer for people to fall asleep.
- If you refrigerate apples, they last longer before going bad.
- If you keep the curtains closed, then you need less electricity to heat or cool the house (the electric bill is lower).
- If you leave a bucket of water uncovered, then it evaporates more quickly.
- Goldfish lose their color if they are not exposed to light.
- Workers who take vacations are more productive than those who never take time off.
Is It Okay to Disprove a Hypothesis?
Yes! You may even choose to write your hypothesis in such a way that it can be disproved because it’s easier to prove a statement is wrong than to prove it is right. In other cases, if your prediction is incorrect, that doesn’t mean the science is bad. Revising a hypothesis is common. It demonstrates you learned something you did not know before you conducted the experiment.
Test yourself with a Scientific Method Quiz .
- Mellenbergh, G.J. (2008). Chapter 8: Research designs: Testing of research hypotheses. In H.J. Adèr & G.J. Mellenbergh (eds.), Advising on Research Methods: A Consultant’s Companion . Huizen, The Netherlands: Johannes van Kessel Publishing.
- Popper, Karl R. (1959). The Logic of Scientific Discovery . Hutchinson & Co. ISBN 3-1614-8410-X.
- Schick, Theodore; Vaughn, Lewis (2002). How to think about weird things: critical thinking for a New Age . Boston: McGraw-Hill Higher Education. ISBN 0-7674-2048-9.
- Tobi, Hilde; Kampen, Jarl K. (2018). “Research design: the methodology for interdisciplinary research framework”. Quality & Quantity . 52 (3): 1209–1225. doi: 10.1007/s11135-017-0513-8
Related Posts
- Urgent Support
Engineering Graduate Studies
Hypothesis and Experimental Design
Jump to: Activity Examples | Resources
Two important elements of The Scientific Method that will help you design your research approach more efficiently are “Generating Hypotheses” and “Designing Controlled Experiments” to test these hypotheses. A well-designed experiment that you deeply understand will save time and resources and facilitate easier data analysis/interpretation. Many people reading this may be working on a project that focuses on designing a product, or discovery research where the hypothesis it is not immediately obvious. We encourage you to read on however as the exercise of generating a hypothesis will likely help you think about the assumptions you are making in your research and the physical principles your work builds upon.
These activities will help you …
- Begin formulating an appropriate hypothesis related to your research.
- Apply a systematic process for designing experiments.
What is a Hypothesis?
A hypothesis is an “educated guess/prediction” or “ proposed explanation ” of how a system will behave based on the available evidence . A hypothesis is a starting point for further investigation and testing because a hypothesis makes a prediction about the behavior of a measurable outcome of an experiment. A hypothesis should be:
- Testable – you can design an experiment to test it
- Falsifiable – it can be proven wrong (note it cannot be “proved”)
- Useful – the outcome must give valuable information
A useful hypothesis may relate to the underlying question of your research. For example:
“We hypothesize that therapy resistant cell populations will be enriched in hypoxic microenvironments. “
“We hypothesize that increasing the number of boreholes simulated in 3D geological models minimizes the variation of the geological model results.”
Some research projects do not have an obvious hypothesis to test, but the design strategy/concept chosen is based on an underlying assumption about how the system being designed works (i.e. the hypothesis). For example:
“We hypothesize that decreasing the baking temperature of the photoresist layer will reduce thermal expansion and device cracking”
In this case the researcher is troubleshooting poor device quality and is proposing to vary different fabrication parameters (one being baking temperature). Understanding the assumptions (working hypotheses) of why different variables might improve device quality is useful as it provides a basis to prioritize what variables to focus on first. The core goal of this research is not to test a specific hypothesis, but using the scientific method to troubleshoot a design challenge will enable the researcher to understand the parameters that control the behavior of different designs and to identify a design that is successful more efficiently.
In all the examples above, the hypothesis helps to guide the design of a useful and interpretable experiment with appropriate controls that rule out alternative explanations of the experimental observation. Hypotheses are therefore likely essential and useful parts of all research projects.
Suggested Activity – Create a Hypothesis for Your Research
Estimated time: 30 mins
- Write down the parameters you are varying or testing in your experimental system or model and how you think the behaviour of the system is going to vary with these parameters.
- (Alternative) If your project goal is to design a device, write down the parameters you believe control whether the device will work.
- (Alternative) If your project goal involves optimizing a process, write down the underlying physics or chemistry controlling the process you are studying.
- With these parameters in mind, write down the key assumption(s) you are making about how your system works.
- Try to formulate each one of these assumptions into a hypothesis that might be useful for your research project. If you have multiple aims each one may have a separate hypothesis. Make sure the hypothesis meets each of the three key elements above.
- Share your hypothesis with a peer or your supervisor to discuss if this is a good hypothesis – is it testable? Does it make a useful prediction? Does it capture the key underlying assumptions your research is based upon?
Remember that writing a good research hypothesis is challenging and will take a lot of careful thought about the underlying science that governs your system.
Designing Experiments
Designing experiments appropriately is very important to avoid wasting resources (time!) and to ensure results can be interpreted correctly. It is often very useful to discuss the design of your planned experiments in your meetings with your supervisor to get feedback before you start doing experiments. This will also ensure you and your supervisor have a consistent understanding of experimental design and that all the appropriate controls required to interpret your data have been considered.
The factors that must be considered when you design experiments is going to depend on your specific area of research. S ome important things to think about when designing experiments include:
Rationale: What is the purpose of this experiment? Is this the best experiment I can do? Does my experiment answer any question ? Does this experiment help answer the question I am trying to ask? What hypothesis am I trying to test?
Will my experiment be interpretable? What controls can I use to distinguish my results from other potential explanations? Can I add a control to distinguish between explanations? Can I add a control to further test my hypothesis?
Is my experiment/model rigorous? What is the sensitivity of the method I am using and can it measure accurately what I want to measure? What outcomes (metrics) will I measure and is this measurement appropriate? How many replicates (technical replicates versus independent replicates) will I do? Am I only changing the variable that I am testing? What am I keeping constant? What statistical tests do I plan to carry out and what considerations are needed? Is my statistical design appropriate (power analysis, sufficient replicates)?
What logistics do I need to consider? Are the equipment/resources I need available? Do I need additional training or equipment access? Are there important safety or ethical issues/permits to consider? Are pilot experiments needed to assess feasibility and what would these be? What is my planned experimental protocol and are there important timing issues to consider? What experimental outputs and parameters need to be documented throughout experiment?
This list is not exhaustive and you should consider what is missing for your particular situation.
Suggested Activity – Design an Experiment Using a Template
Estimated time: 45 min
- Explore the excel template for experimental design ( Resource 1 ) or modelling ( Resource 2 ). A template like this is very useful for keeping track of protocols as well as improving the reproducibility of your experiments. Note this template is simply a starting point to get you thinking systematically and should be adapted to best suit your needs.
- Fill out the template for an experiment or modelling project you are planning to complete soon.
- Consider how you can modify this template to be more applicable to your specific project.
- Using the template document, explain your experimental design/model design to a peer or your supervisor. Let them ask questions to understand your design and provide feedback. Alternatively, if there is a part of your design that you are unclear about this is a great starting point for a targeted and efficient discussion with your supervisor.
- Revise your design based on feedback.
Activity Examples
© 2024 Faculty of Applied Science and Engineering
- U of T Home
- Accessibility
- Student Data Practices
- Website Feedback
Have a language expert improve your writing
Run a free plagiarism check in 10 minutes, automatically generate references for free.
- Knowledge Base
- Methodology
- A Quick Guide to Experimental Design | 5 Steps & Examples
A Quick Guide to Experimental Design | 5 Steps & Examples
Published on 11 April 2022 by Rebecca Bevans . Revised on 5 December 2022.
Experiments are used to study causal relationships . You manipulate one or more independent variables and measure their effect on one or more dependent variables.
Experimental design means creating a set of procedures to systematically test a hypothesis . A good experimental design requires a strong understanding of the system you are studying.
There are five key steps in designing an experiment:
- Consider your variables and how they are related
- Write a specific, testable hypothesis
- Design experimental treatments to manipulate your independent variable
- Assign subjects to groups, either between-subjects or within-subjects
- Plan how you will measure your dependent variable
For valid conclusions, you also need to select a representative sample and control any extraneous variables that might influence your results. If if random assignment of participants to control and treatment groups is impossible, unethical, or highly difficult, consider an observational study instead.
Table of contents
Step 1: define your variables, step 2: write your hypothesis, step 3: design your experimental treatments, step 4: assign your subjects to treatment groups, step 5: measure your dependent variable, frequently asked questions about experimental design.
You should begin with a specific research question . We will work with two research question examples, one from health sciences and one from ecology:
To translate your research question into an experimental hypothesis, you need to define the main variables and make predictions about how they are related.
Start by simply listing the independent and dependent variables .
Then you need to think about possible extraneous and confounding variables and consider how you might control them in your experiment.
Finally, you can put these variables together into a diagram. Use arrows to show the possible relationships between variables and include signs to show the expected direction of the relationships.
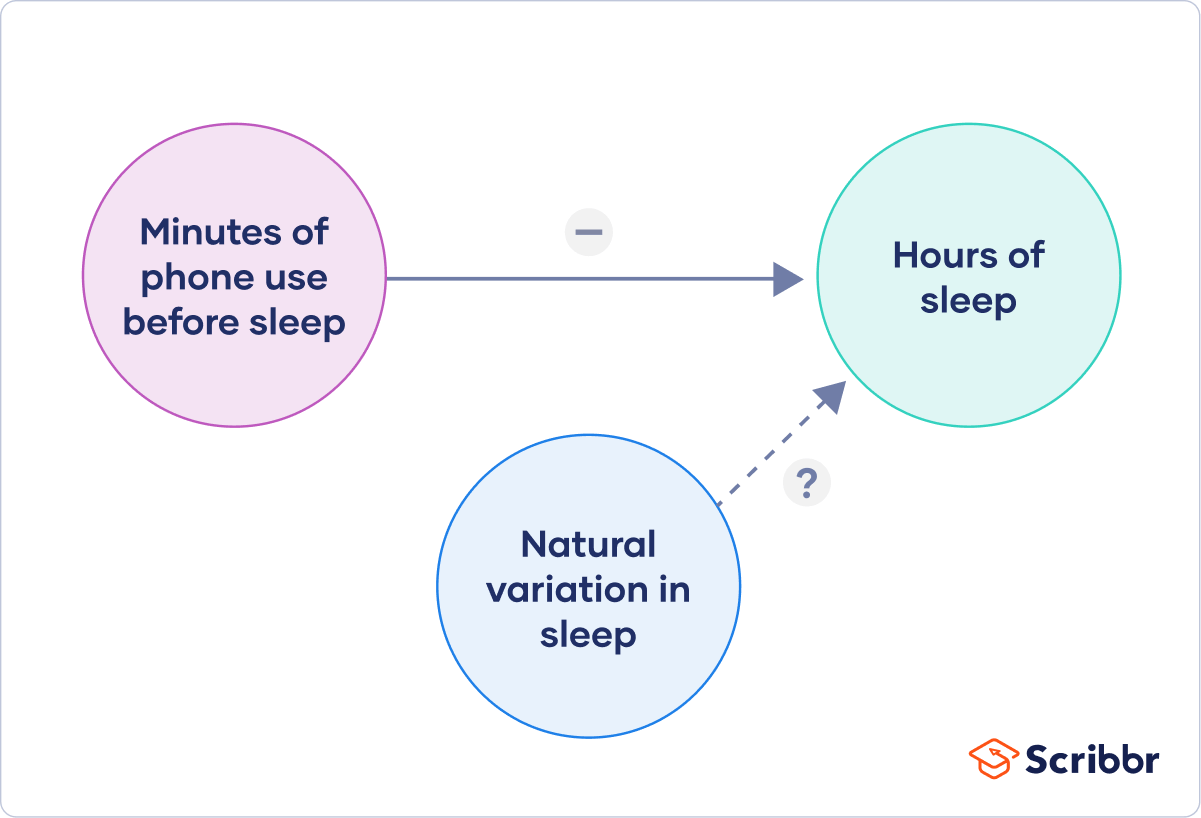
Here we predict that increasing temperature will increase soil respiration and decrease soil moisture, while decreasing soil moisture will lead to decreased soil respiration.
Prevent plagiarism, run a free check.
Now that you have a strong conceptual understanding of the system you are studying, you should be able to write a specific, testable hypothesis that addresses your research question.
The next steps will describe how to design a controlled experiment . In a controlled experiment, you must be able to:
- Systematically and precisely manipulate the independent variable(s).
- Precisely measure the dependent variable(s).
- Control any potential confounding variables.
If your study system doesn’t match these criteria, there are other types of research you can use to answer your research question.
How you manipulate the independent variable can affect the experiment’s external validity – that is, the extent to which the results can be generalised and applied to the broader world.
First, you may need to decide how widely to vary your independent variable.
- just slightly above the natural range for your study region.
- over a wider range of temperatures to mimic future warming.
- over an extreme range that is beyond any possible natural variation.
Second, you may need to choose how finely to vary your independent variable. Sometimes this choice is made for you by your experimental system, but often you will need to decide, and this will affect how much you can infer from your results.
- a categorical variable : either as binary (yes/no) or as levels of a factor (no phone use, low phone use, high phone use).
- a continuous variable (minutes of phone use measured every night).
How you apply your experimental treatments to your test subjects is crucial for obtaining valid and reliable results.
First, you need to consider the study size : how many individuals will be included in the experiment? In general, the more subjects you include, the greater your experiment’s statistical power , which determines how much confidence you can have in your results.
Then you need to randomly assign your subjects to treatment groups . Each group receives a different level of the treatment (e.g. no phone use, low phone use, high phone use).
You should also include a control group , which receives no treatment. The control group tells us what would have happened to your test subjects without any experimental intervention.
When assigning your subjects to groups, there are two main choices you need to make:
- A completely randomised design vs a randomised block design .
- A between-subjects design vs a within-subjects design .
Randomisation
An experiment can be completely randomised or randomised within blocks (aka strata):
- In a completely randomised design , every subject is assigned to a treatment group at random.
- In a randomised block design (aka stratified random design), subjects are first grouped according to a characteristic they share, and then randomly assigned to treatments within those groups.
Sometimes randomisation isn’t practical or ethical , so researchers create partially-random or even non-random designs. An experimental design where treatments aren’t randomly assigned is called a quasi-experimental design .
Between-subjects vs within-subjects
In a between-subjects design (also known as an independent measures design or classic ANOVA design), individuals receive only one of the possible levels of an experimental treatment.
In medical or social research, you might also use matched pairs within your between-subjects design to make sure that each treatment group contains the same variety of test subjects in the same proportions.
In a within-subjects design (also known as a repeated measures design), every individual receives each of the experimental treatments consecutively, and their responses to each treatment are measured.
Within-subjects or repeated measures can also refer to an experimental design where an effect emerges over time, and individual responses are measured over time in order to measure this effect as it emerges.
Counterbalancing (randomising or reversing the order of treatments among subjects) is often used in within-subjects designs to ensure that the order of treatment application doesn’t influence the results of the experiment.
Finally, you need to decide how you’ll collect data on your dependent variable outcomes. You should aim for reliable and valid measurements that minimise bias or error.
Some variables, like temperature, can be objectively measured with scientific instruments. Others may need to be operationalised to turn them into measurable observations.
- Ask participants to record what time they go to sleep and get up each day.
- Ask participants to wear a sleep tracker.
How precisely you measure your dependent variable also affects the kinds of statistical analysis you can use on your data.
Experiments are always context-dependent, and a good experimental design will take into account all of the unique considerations of your study system to produce information that is both valid and relevant to your research question.
Experimental designs are a set of procedures that you plan in order to examine the relationship between variables that interest you.
To design a successful experiment, first identify:
- A testable hypothesis
- One or more independent variables that you will manipulate
- One or more dependent variables that you will measure
When designing the experiment, first decide:
- How your variable(s) will be manipulated
- How you will control for any potential confounding or lurking variables
- How many subjects you will include
- How you will assign treatments to your subjects
The key difference between observational studies and experiments is that, done correctly, an observational study will never influence the responses or behaviours of participants. Experimental designs will have a treatment condition applied to at least a portion of participants.
A confounding variable , also called a confounder or confounding factor, is a third variable in a study examining a potential cause-and-effect relationship.
A confounding variable is related to both the supposed cause and the supposed effect of the study. It can be difficult to separate the true effect of the independent variable from the effect of the confounding variable.
In your research design , it’s important to identify potential confounding variables and plan how you will reduce their impact.
In a between-subjects design , every participant experiences only one condition, and researchers assess group differences between participants in various conditions.
In a within-subjects design , each participant experiences all conditions, and researchers test the same participants repeatedly for differences between conditions.
The word ‘between’ means that you’re comparing different conditions between groups, while the word ‘within’ means you’re comparing different conditions within the same group.
Cite this Scribbr article
If you want to cite this source, you can copy and paste the citation or click the ‘Cite this Scribbr article’ button to automatically add the citation to our free Reference Generator.
Bevans, R. (2022, December 05). A Quick Guide to Experimental Design | 5 Steps & Examples. Scribbr. Retrieved 27 May 2024, from https://www.scribbr.co.uk/research-methods/guide-to-experimental-design/
Is this article helpful?
Rebecca Bevans
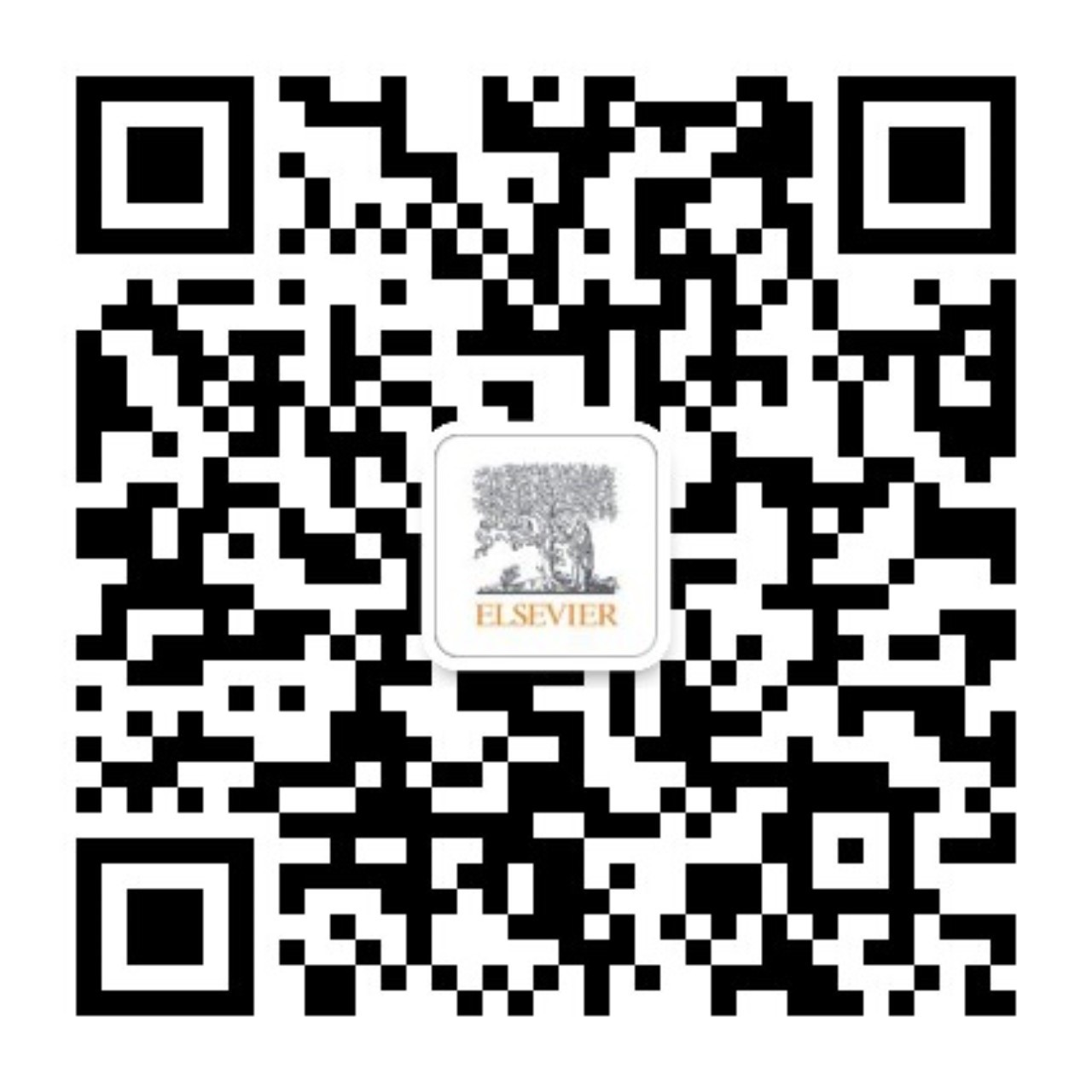
- Manuscript Preparation
What is and How to Write a Good Hypothesis in Research?
- 4 minute read
- 318.6K views
Table of Contents
One of the most important aspects of conducting research is constructing a strong hypothesis. But what makes a hypothesis in research effective? In this article, we’ll look at the difference between a hypothesis and a research question, as well as the elements of a good hypothesis in research. We’ll also include some examples of effective hypotheses, and what pitfalls to avoid.
What is a Hypothesis in Research?
Simply put, a hypothesis is a research question that also includes the predicted or expected result of the research. Without a hypothesis, there can be no basis for a scientific or research experiment. As such, it is critical that you carefully construct your hypothesis by being deliberate and thorough, even before you set pen to paper. Unless your hypothesis is clearly and carefully constructed, any flaw can have an adverse, and even grave, effect on the quality of your experiment and its subsequent results.
Research Question vs Hypothesis
It’s easy to confuse research questions with hypotheses, and vice versa. While they’re both critical to the Scientific Method, they have very specific differences. Primarily, a research question, just like a hypothesis, is focused and concise. But a hypothesis includes a prediction based on the proposed research, and is designed to forecast the relationship of and between two (or more) variables. Research questions are open-ended, and invite debate and discussion, while hypotheses are closed, e.g. “The relationship between A and B will be C.”
A hypothesis is generally used if your research topic is fairly well established, and you are relatively certain about the relationship between the variables that will be presented in your research. Since a hypothesis is ideally suited for experimental studies, it will, by its very existence, affect the design of your experiment. The research question is typically used for new topics that have not yet been researched extensively. Here, the relationship between different variables is less known. There is no prediction made, but there may be variables explored. The research question can be casual in nature, simply trying to understand if a relationship even exists, descriptive or comparative.
How to Write Hypothesis in Research
Writing an effective hypothesis starts before you even begin to type. Like any task, preparation is key, so you start first by conducting research yourself, and reading all you can about the topic that you plan to research. From there, you’ll gain the knowledge you need to understand where your focus within the topic will lie.
Remember that a hypothesis is a prediction of the relationship that exists between two or more variables. Your job is to write a hypothesis, and design the research, to “prove” whether or not your prediction is correct. A common pitfall is to use judgments that are subjective and inappropriate for the construction of a hypothesis. It’s important to keep the focus and language of your hypothesis objective.
An effective hypothesis in research is clearly and concisely written, and any terms or definitions clarified and defined. Specific language must also be used to avoid any generalities or assumptions.
Use the following points as a checklist to evaluate the effectiveness of your research hypothesis:
- Predicts the relationship and outcome
- Simple and concise – avoid wordiness
- Clear with no ambiguity or assumptions about the readers’ knowledge
- Observable and testable results
- Relevant and specific to the research question or problem
Research Hypothesis Example
Perhaps the best way to evaluate whether or not your hypothesis is effective is to compare it to those of your colleagues in the field. There is no need to reinvent the wheel when it comes to writing a powerful research hypothesis. As you’re reading and preparing your hypothesis, you’ll also read other hypotheses. These can help guide you on what works, and what doesn’t, when it comes to writing a strong research hypothesis.
Here are a few generic examples to get you started.
Eating an apple each day, after the age of 60, will result in a reduction of frequency of physician visits.
Budget airlines are more likely to receive more customer complaints. A budget airline is defined as an airline that offers lower fares and fewer amenities than a traditional full-service airline. (Note that the term “budget airline” is included in the hypothesis.
Workplaces that offer flexible working hours report higher levels of employee job satisfaction than workplaces with fixed hours.
Each of the above examples are specific, observable and measurable, and the statement of prediction can be verified or shown to be false by utilizing standard experimental practices. It should be noted, however, that often your hypothesis will change as your research progresses.
Language Editing Plus
Elsevier’s Language Editing Plus service can help ensure that your research hypothesis is well-designed, and articulates your research and conclusions. Our most comprehensive editing package, you can count on a thorough language review by native-English speakers who are PhDs or PhD candidates. We’ll check for effective logic and flow of your manuscript, as well as document formatting for your chosen journal, reference checks, and much more.
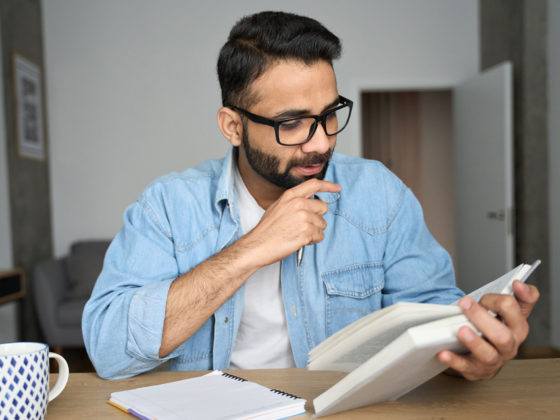
- Research Process
Systematic Literature Review or Literature Review?
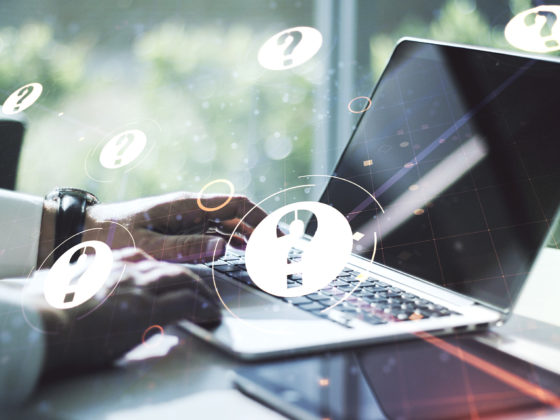
What is a Problem Statement? [with examples]
You may also like.
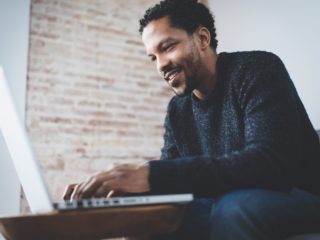
Page-Turner Articles are More Than Just Good Arguments: Be Mindful of Tone and Structure!
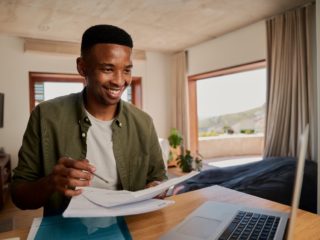
A Must-see for Researchers! How to Ensure Inclusivity in Your Scientific Writing
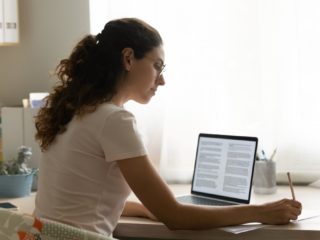
Make Hook, Line, and Sinker: The Art of Crafting Engaging Introductions
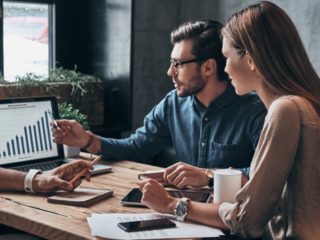
Can Describing Study Limitations Improve the Quality of Your Paper?
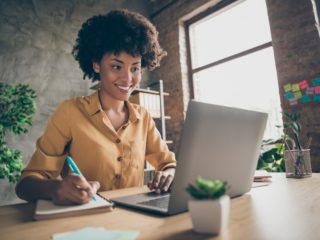
A Guide to Crafting Shorter, Impactful Sentences in Academic Writing
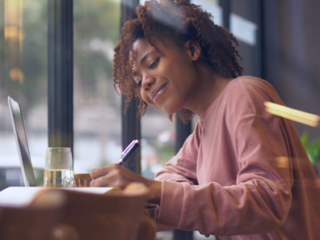
6 Steps to Write an Excellent Discussion in Your Manuscript
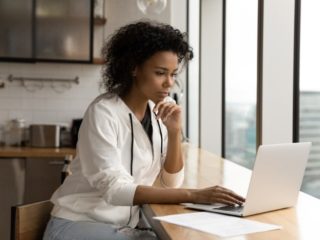
How to Write Clear and Crisp Civil Engineering Papers? Here are 5 Key Tips to Consider
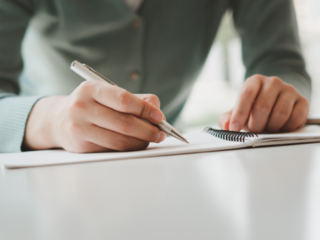
The Clear Path to An Impactful Paper: ②
Input your search keywords and press Enter.

15 Hypothesis Examples
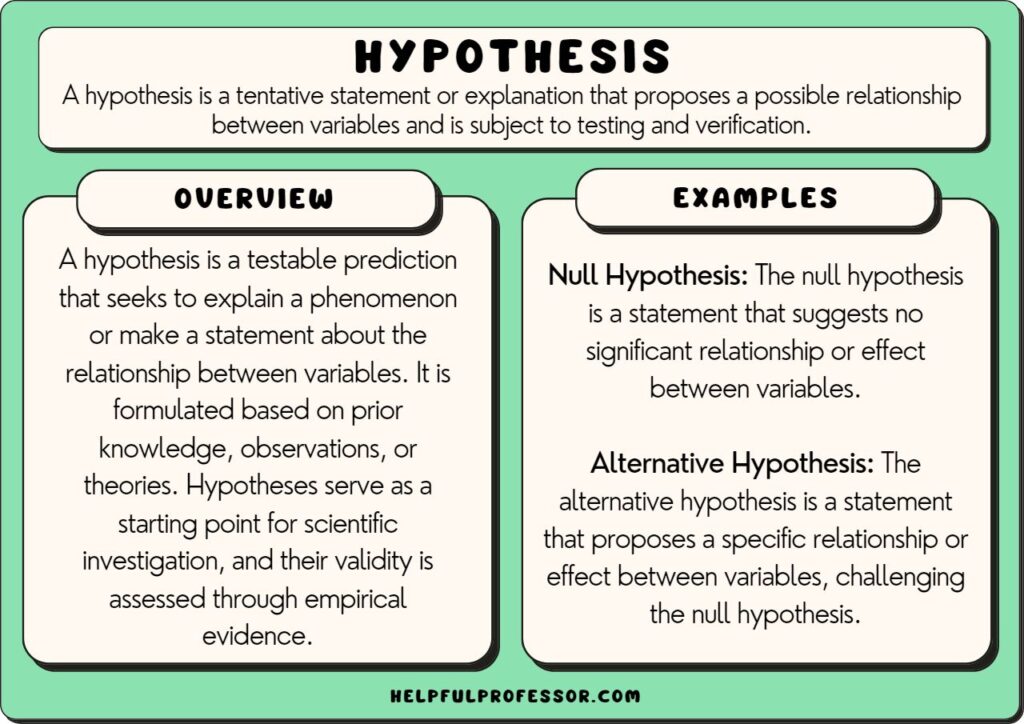
A hypothesis is defined as a testable prediction , and is used primarily in scientific experiments as a potential or predicted outcome that scientists attempt to prove or disprove (Atkinson et al., 2021; Tan, 2022).
In my types of hypothesis article, I outlined 13 different hypotheses, including the directional hypothesis (which makes a prediction about an effect of a treatment will be positive or negative) and the associative hypothesis (which makes a prediction about the association between two variables).
This article will dive into some interesting examples of hypotheses and examine potential ways you might test each one.
Hypothesis Examples
1. “inadequate sleep decreases memory retention”.
Field: Psychology
Type: Causal Hypothesis A causal hypothesis explores the effect of one variable on another. This example posits that a lack of adequate sleep causes decreased memory retention. In other words, if you are not getting enough sleep, your ability to remember and recall information may suffer.
How to Test:
To test this hypothesis, you might devise an experiment whereby your participants are divided into two groups: one receives an average of 8 hours of sleep per night for a week, while the other gets less than the recommended sleep amount.
During this time, all participants would daily study and recall new, specific information. You’d then measure memory retention of this information for both groups using standard memory tests and compare the results.
Should the group with less sleep have statistically significant poorer memory scores, the hypothesis would be supported.
Ensuring the integrity of the experiment requires taking into account factors such as individual health differences, stress levels, and daily nutrition.
Relevant Study: Sleep loss, learning capacity and academic performance (Curcio, Ferrara & De Gennaro, 2006)
2. “Increase in Temperature Leads to Increase in Kinetic Energy”
Field: Physics
Type: Deductive Hypothesis The deductive hypothesis applies the logic of deductive reasoning – it moves from a general premise to a more specific conclusion. This specific hypothesis assumes that as temperature increases, the kinetic energy of particles also increases – that is, when you heat something up, its particles move around more rapidly.
This hypothesis could be examined by heating a gas in a controlled environment and capturing the movement of its particles as a function of temperature.
You’d gradually increase the temperature and measure the kinetic energy of the gas particles with each increment. If the kinetic energy consistently rises with the temperature, your hypothesis gets supporting evidence.
Variables such as pressure and volume of the gas would need to be held constant to ensure validity of results.
3. “Children Raised in Bilingual Homes Develop Better Cognitive Skills”
Field: Psychology/Linguistics
Type: Comparative Hypothesis The comparative hypothesis posits a difference between two or more groups based on certain variables. In this context, you might propose that children raised in bilingual homes have superior cognitive skills compared to those raised in monolingual homes.
Testing this hypothesis could involve identifying two groups of children: those raised in bilingual homes, and those raised in monolingual homes.
Cognitive skills in both groups would be evaluated using a standard cognitive ability test at different stages of development. The examination would be repeated over a significant time period for consistency.
If the group raised in bilingual homes persistently scores higher than the other, the hypothesis would thereby be supported.
The challenge for the researcher would be controlling for other variables that could impact cognitive development, such as socio-economic status, education level of parents, and parenting styles.
Relevant Study: The cognitive benefits of being bilingual (Marian & Shook, 2012)
4. “High-Fiber Diet Leads to Lower Incidences of Cardiovascular Diseases”
Field: Medicine/Nutrition
Type: Alternative Hypothesis The alternative hypothesis suggests an alternative to a null hypothesis. In this context, the implied null hypothesis could be that diet has no effect on cardiovascular health, which the alternative hypothesis contradicts by suggesting that a high-fiber diet leads to fewer instances of cardiovascular diseases.
To test this hypothesis, a longitudinal study could be conducted on two groups of participants; one adheres to a high-fiber diet, while the other follows a diet low in fiber.
After a fixed period, the cardiovascular health of participants in both groups could be analyzed and compared. If the group following a high-fiber diet has a lower number of recorded cases of cardiovascular diseases, it would provide evidence supporting the hypothesis.
Control measures should be implemented to exclude the influence of other lifestyle and genetic factors that contribute to cardiovascular health.
Relevant Study: Dietary fiber, inflammation, and cardiovascular disease (King, 2005)
5. “Gravity Influences the Directional Growth of Plants”
Field: Agronomy / Botany
Type: Explanatory Hypothesis An explanatory hypothesis attempts to explain a phenomenon. In this case, the hypothesis proposes that gravity affects how plants direct their growth – both above-ground (toward sunlight) and below-ground (towards water and other resources).
The testing could be conducted by growing plants in a rotating cylinder to create artificial gravity.
Observations on the direction of growth, over a specified period, can provide insights into the influencing factors. If plants consistently direct their growth in a manner that indicates the influence of gravitational pull, the hypothesis is substantiated.
It is crucial to ensure that other growth-influencing factors, such as light and water, are uniformly distributed so that only gravity influences the directional growth.
6. “The Implementation of Gamified Learning Improves Students’ Motivation”
Field: Education
Type: Relational Hypothesis The relational hypothesis describes the relation between two variables. Here, the hypothesis is that the implementation of gamified learning has a positive effect on the motivation of students.
To validate this proposition, two sets of classes could be compared: one that implements a learning approach with game-based elements, and another that follows a traditional learning approach.
The students’ motivation levels could be gauged by monitoring their engagement, performance, and feedback over a considerable timeframe.
If the students engaged in the gamified learning context present higher levels of motivation and achievement, the hypothesis would be supported.
Control measures ought to be put into place to account for individual differences, including prior knowledge and attitudes towards learning.
Relevant Study: Does educational gamification improve students’ motivation? (Chapman & Rich, 2018)
7. “Mathematics Anxiety Negatively Affects Performance”
Field: Educational Psychology
Type: Research Hypothesis The research hypothesis involves making a prediction that will be tested. In this case, the hypothesis proposes that a student’s anxiety about math can negatively influence their performance in math-related tasks.
To assess this hypothesis, researchers must first measure the mathematics anxiety levels of a sample of students using a validated instrument, such as the Mathematics Anxiety Rating Scale.
Then, the students’ performance in mathematics would be evaluated through standard testing. If there’s a negative correlation between the levels of math anxiety and math performance (meaning as anxiety increases, performance decreases), the hypothesis would be supported.
It would be crucial to control for relevant factors such as overall academic performance and previous mathematical achievement.
8. “Disruption of Natural Sleep Cycle Impairs Worker Productivity”
Field: Organizational Psychology
Type: Operational Hypothesis The operational hypothesis involves defining the variables in measurable terms. In this example, the hypothesis posits that disrupting the natural sleep cycle, for instance through shift work or irregular working hours, can lessen productivity among workers.
To test this hypothesis, you could collect data from workers who maintain regular working hours and those with irregular schedules.
Measuring productivity could involve examining the worker’s ability to complete tasks, the quality of their work, and their efficiency.
If workers with interrupted sleep cycles demonstrate lower productivity compared to those with regular sleep patterns, it would lend support to the hypothesis.
Consideration should be given to potential confounding variables such as job type, worker age, and overall health.
9. “Regular Physical Activity Reduces the Risk of Depression”
Field: Health Psychology
Type: Predictive Hypothesis A predictive hypothesis involves making a prediction about the outcome of a study based on the observed relationship between variables. In this case, it is hypothesized that individuals who engage in regular physical activity are less likely to suffer from depression.
Longitudinal studies would suit to test this hypothesis, tracking participants’ levels of physical activity and their mental health status over time.
The level of physical activity could be self-reported or monitored, while mental health status could be assessed using standard diagnostic tools or surveys.
If data analysis shows that participants maintaining regular physical activity have a lower incidence of depression, this would endorse the hypothesis.
However, care should be taken to control other lifestyle and behavioral factors that could intervene with the results.
Relevant Study: Regular physical exercise and its association with depression (Kim, 2022)
10. “Regular Meditation Enhances Emotional Stability”
Type: Empirical Hypothesis In the empirical hypothesis, predictions are based on amassed empirical evidence . This particular hypothesis theorizes that frequent meditation leads to improved emotional stability, resonating with numerous studies linking meditation to a variety of psychological benefits.
Earlier studies reported some correlations, but to test this hypothesis directly, you’d organize an experiment where one group meditates regularly over a set period while a control group doesn’t.
Both groups’ emotional stability levels would be measured at the start and end of the experiment using a validated emotional stability assessment.
If regular meditators display noticeable improvements in emotional stability compared to the control group, the hypothesis gains credit.
You’d have to ensure a similar emotional baseline for all participants at the start to avoid skewed results.
11. “Children Exposed to Reading at an Early Age Show Superior Academic Progress”
Type: Directional Hypothesis The directional hypothesis predicts the direction of an expected relationship between variables. Here, the hypothesis anticipates that early exposure to reading positively affects a child’s academic advancement.
A longitudinal study tracking children’s reading habits from an early age and their consequent academic performance could validate this hypothesis.
Parents could report their children’s exposure to reading at home, while standardized school exam results would provide a measure of academic achievement.
If the children exposed to early reading consistently perform better acadically, it gives weight to the hypothesis.
However, it would be important to control for variables that might impact academic performance, such as socioeconomic background, parental education level, and school quality.
12. “Adopting Energy-efficient Technologies Reduces Carbon Footprint of Industries”
Field: Environmental Science
Type: Descriptive Hypothesis A descriptive hypothesis predicts the existence of an association or pattern related to variables. In this scenario, the hypothesis suggests that industries adopting energy-efficient technologies will resultantly show a reduced carbon footprint.
Global industries making use of energy-efficient technologies could track their carbon emissions over time. At the same time, others not implementing such technologies continue their regular tracking.
After a defined time, the carbon emission data of both groups could be compared. If industries that adopted energy-efficient technologies demonstrate a notable reduction in their carbon footprints, the hypothesis would hold strong.
In the experiment, you would exclude variations brought by factors such as industry type, size, and location.
13. “Reduced Screen Time Improves Sleep Quality”
Type: Simple Hypothesis The simple hypothesis is a prediction about the relationship between two variables, excluding any other variables from consideration. This example posits that by reducing time spent on devices like smartphones and computers, an individual should experience improved sleep quality.
A sample group would need to reduce their daily screen time for a pre-determined period. Sleep quality before and after the reduction could be measured using self-report sleep diaries and objective measures like actigraphy, monitoring movement and wakefulness during sleep.
If the data shows that sleep quality improved post the screen time reduction, the hypothesis would be validated.
Other aspects affecting sleep quality, like caffeine intake, should be controlled during the experiment.
Relevant Study: Screen time use impacts low‐income preschool children’s sleep quality, tiredness, and ability to fall asleep (Waller et al., 2021)
14. Engaging in Brain-Training Games Improves Cognitive Functioning in Elderly
Field: Gerontology
Type: Inductive Hypothesis Inductive hypotheses are based on observations leading to broader generalizations and theories. In this context, the hypothesis deduces from observed instances that engaging in brain-training games can help improve cognitive functioning in the elderly.
A longitudinal study could be conducted where an experimental group of elderly people partakes in regular brain-training games.
Their cognitive functioning could be assessed at the start of the study and at regular intervals using standard neuropsychological tests.
If the group engaging in brain-training games shows better cognitive functioning scores over time compared to a control group not playing these games, the hypothesis would be supported.
15. Farming Practices Influence Soil Erosion Rates
Type: Null Hypothesis A null hypothesis is a negative statement assuming no relationship or difference between variables. The hypothesis in this context asserts there’s no effect of different farming practices on the rates of soil erosion.
Comparing soil erosion rates in areas with different farming practices over a considerable timeframe could help test this hypothesis.
If, statistically, the farming practices do not lead to differences in soil erosion rates, the null hypothesis is accepted.
However, if marked variation appears, the null hypothesis is rejected, meaning farming practices do influence soil erosion rates. It would be crucial to control for external factors like weather, soil type, and natural vegetation.
The variety of hypotheses mentioned above underscores the diversity of research constructs inherent in different fields, each with its unique purpose and way of testing.
While researchers may develop hypotheses primarily as tools to define and narrow the focus of the study, these hypotheses also serve as valuable guiding forces for the data collection and analysis procedures, making the research process more efficient and direction-focused.
Hypotheses serve as a compass for any form of academic research. The diverse examples provided, from Psychology to Educational Studies, Environmental Science to Gerontology, clearly demonstrate how certain hypotheses suit specific fields more aptly than others.
It is important to underline that although these varied hypotheses differ in their structure and methods of testing, each endorses the fundamental value of empiricism in research. Evidence-based decision making remains at the heart of scholarly inquiry, regardless of the research field, thus aligning all hypotheses to the core purpose of scientific investigation.
Testing hypotheses is an essential part of the scientific method . By doing so, researchers can either confirm their predictions, giving further validity to an existing theory, or they might uncover new insights that could potentially shift the field’s understanding of a particular phenomenon. In either case, hypotheses serve as the stepping stones for scientific exploration and discovery.
Atkinson, P., Delamont, S., Cernat, A., Sakshaug, J. W., & Williams, R. A. (2021). SAGE research methods foundations . SAGE Publications Ltd.
Curcio, G., Ferrara, M., & De Gennaro, L. (2006). Sleep loss, learning capacity and academic performance. Sleep medicine reviews , 10 (5), 323-337.
Kim, J. H. (2022). Regular physical exercise and its association with depression: A population-based study short title: Exercise and depression. Psychiatry Research , 309 , 114406.
King, D. E. (2005). Dietary fiber, inflammation, and cardiovascular disease. Molecular nutrition & food research , 49 (6), 594-600.
Marian, V., & Shook, A. (2012, September). The cognitive benefits of being bilingual. In Cerebrum: the Dana forum on brain science (Vol. 2012). Dana Foundation.
Tan, W. C. K. (2022). Research Methods: A Practical Guide For Students And Researchers (Second Edition) . World Scientific Publishing Company.
Waller, N. A., Zhang, N., Cocci, A. H., D’Agostino, C., Wesolek‐Greenson, S., Wheelock, K., … & Resnicow, K. (2021). Screen time use impacts low‐income preschool children’s sleep quality, tiredness, and ability to fall asleep. Child: care, health and development, 47 (5), 618-626.
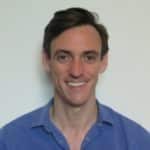
Chris Drew (PhD)
Dr. Chris Drew is the founder of the Helpful Professor. He holds a PhD in education and has published over 20 articles in scholarly journals. He is the former editor of the Journal of Learning Development in Higher Education. [Image Descriptor: Photo of Chris]
- Chris Drew (PhD) https://helpfulprofessor.com/author/chris-drew-phd/ Social Exchange Theory: Definition and Examples
- Chris Drew (PhD) https://helpfulprofessor.com/author/chris-drew-phd/ 10 Cognitive Dissonance Examples
- Chris Drew (PhD) https://helpfulprofessor.com/author/chris-drew-phd/ 10 Elaborative Rehearsal Examples
- Chris Drew (PhD) https://helpfulprofessor.com/author/chris-drew-phd/ Maintenance Rehearsal - Definition & Examples
Leave a Comment Cancel Reply
Your email address will not be published. Required fields are marked *

An official website of the United States government
The .gov means it’s official. Federal government websites often end in .gov or .mil. Before sharing sensitive information, make sure you’re on a federal government site.
The site is secure. The https:// ensures that you are connecting to the official website and that any information you provide is encrypted and transmitted securely.
- Publications
- Account settings
Preview improvements coming to the PMC website in October 2024. Learn More or Try it out now .
- Advanced Search
- Journal List
- J Korean Med Sci
- v.37(16); 2022 Apr 25

A Practical Guide to Writing Quantitative and Qualitative Research Questions and Hypotheses in Scholarly Articles
Edward barroga.
1 Department of General Education, Graduate School of Nursing Science, St. Luke’s International University, Tokyo, Japan.
Glafera Janet Matanguihan
2 Department of Biological Sciences, Messiah University, Mechanicsburg, PA, USA.
The development of research questions and the subsequent hypotheses are prerequisites to defining the main research purpose and specific objectives of a study. Consequently, these objectives determine the study design and research outcome. The development of research questions is a process based on knowledge of current trends, cutting-edge studies, and technological advances in the research field. Excellent research questions are focused and require a comprehensive literature search and in-depth understanding of the problem being investigated. Initially, research questions may be written as descriptive questions which could be developed into inferential questions. These questions must be specific and concise to provide a clear foundation for developing hypotheses. Hypotheses are more formal predictions about the research outcomes. These specify the possible results that may or may not be expected regarding the relationship between groups. Thus, research questions and hypotheses clarify the main purpose and specific objectives of the study, which in turn dictate the design of the study, its direction, and outcome. Studies developed from good research questions and hypotheses will have trustworthy outcomes with wide-ranging social and health implications.
INTRODUCTION
Scientific research is usually initiated by posing evidenced-based research questions which are then explicitly restated as hypotheses. 1 , 2 The hypotheses provide directions to guide the study, solutions, explanations, and expected results. 3 , 4 Both research questions and hypotheses are essentially formulated based on conventional theories and real-world processes, which allow the inception of novel studies and the ethical testing of ideas. 5 , 6
It is crucial to have knowledge of both quantitative and qualitative research 2 as both types of research involve writing research questions and hypotheses. 7 However, these crucial elements of research are sometimes overlooked; if not overlooked, then framed without the forethought and meticulous attention it needs. Planning and careful consideration are needed when developing quantitative or qualitative research, particularly when conceptualizing research questions and hypotheses. 4
There is a continuing need to support researchers in the creation of innovative research questions and hypotheses, as well as for journal articles that carefully review these elements. 1 When research questions and hypotheses are not carefully thought of, unethical studies and poor outcomes usually ensue. Carefully formulated research questions and hypotheses define well-founded objectives, which in turn determine the appropriate design, course, and outcome of the study. This article then aims to discuss in detail the various aspects of crafting research questions and hypotheses, with the goal of guiding researchers as they develop their own. Examples from the authors and peer-reviewed scientific articles in the healthcare field are provided to illustrate key points.
DEFINITIONS AND RELATIONSHIP OF RESEARCH QUESTIONS AND HYPOTHESES
A research question is what a study aims to answer after data analysis and interpretation. The answer is written in length in the discussion section of the paper. Thus, the research question gives a preview of the different parts and variables of the study meant to address the problem posed in the research question. 1 An excellent research question clarifies the research writing while facilitating understanding of the research topic, objective, scope, and limitations of the study. 5
On the other hand, a research hypothesis is an educated statement of an expected outcome. This statement is based on background research and current knowledge. 8 , 9 The research hypothesis makes a specific prediction about a new phenomenon 10 or a formal statement on the expected relationship between an independent variable and a dependent variable. 3 , 11 It provides a tentative answer to the research question to be tested or explored. 4
Hypotheses employ reasoning to predict a theory-based outcome. 10 These can also be developed from theories by focusing on components of theories that have not yet been observed. 10 The validity of hypotheses is often based on the testability of the prediction made in a reproducible experiment. 8
Conversely, hypotheses can also be rephrased as research questions. Several hypotheses based on existing theories and knowledge may be needed to answer a research question. Developing ethical research questions and hypotheses creates a research design that has logical relationships among variables. These relationships serve as a solid foundation for the conduct of the study. 4 , 11 Haphazardly constructed research questions can result in poorly formulated hypotheses and improper study designs, leading to unreliable results. Thus, the formulations of relevant research questions and verifiable hypotheses are crucial when beginning research. 12
CHARACTERISTICS OF GOOD RESEARCH QUESTIONS AND HYPOTHESES
Excellent research questions are specific and focused. These integrate collective data and observations to confirm or refute the subsequent hypotheses. Well-constructed hypotheses are based on previous reports and verify the research context. These are realistic, in-depth, sufficiently complex, and reproducible. More importantly, these hypotheses can be addressed and tested. 13
There are several characteristics of well-developed hypotheses. Good hypotheses are 1) empirically testable 7 , 10 , 11 , 13 ; 2) backed by preliminary evidence 9 ; 3) testable by ethical research 7 , 9 ; 4) based on original ideas 9 ; 5) have evidenced-based logical reasoning 10 ; and 6) can be predicted. 11 Good hypotheses can infer ethical and positive implications, indicating the presence of a relationship or effect relevant to the research theme. 7 , 11 These are initially developed from a general theory and branch into specific hypotheses by deductive reasoning. In the absence of a theory to base the hypotheses, inductive reasoning based on specific observations or findings form more general hypotheses. 10
TYPES OF RESEARCH QUESTIONS AND HYPOTHESES
Research questions and hypotheses are developed according to the type of research, which can be broadly classified into quantitative and qualitative research. We provide a summary of the types of research questions and hypotheses under quantitative and qualitative research categories in Table 1 .
Research questions in quantitative research
In quantitative research, research questions inquire about the relationships among variables being investigated and are usually framed at the start of the study. These are precise and typically linked to the subject population, dependent and independent variables, and research design. 1 Research questions may also attempt to describe the behavior of a population in relation to one or more variables, or describe the characteristics of variables to be measured ( descriptive research questions ). 1 , 5 , 14 These questions may also aim to discover differences between groups within the context of an outcome variable ( comparative research questions ), 1 , 5 , 14 or elucidate trends and interactions among variables ( relationship research questions ). 1 , 5 We provide examples of descriptive, comparative, and relationship research questions in quantitative research in Table 2 .
Hypotheses in quantitative research
In quantitative research, hypotheses predict the expected relationships among variables. 15 Relationships among variables that can be predicted include 1) between a single dependent variable and a single independent variable ( simple hypothesis ) or 2) between two or more independent and dependent variables ( complex hypothesis ). 4 , 11 Hypotheses may also specify the expected direction to be followed and imply an intellectual commitment to a particular outcome ( directional hypothesis ) 4 . On the other hand, hypotheses may not predict the exact direction and are used in the absence of a theory, or when findings contradict previous studies ( non-directional hypothesis ). 4 In addition, hypotheses can 1) define interdependency between variables ( associative hypothesis ), 4 2) propose an effect on the dependent variable from manipulation of the independent variable ( causal hypothesis ), 4 3) state a negative relationship between two variables ( null hypothesis ), 4 , 11 , 15 4) replace the working hypothesis if rejected ( alternative hypothesis ), 15 explain the relationship of phenomena to possibly generate a theory ( working hypothesis ), 11 5) involve quantifiable variables that can be tested statistically ( statistical hypothesis ), 11 6) or express a relationship whose interlinks can be verified logically ( logical hypothesis ). 11 We provide examples of simple, complex, directional, non-directional, associative, causal, null, alternative, working, statistical, and logical hypotheses in quantitative research, as well as the definition of quantitative hypothesis-testing research in Table 3 .
Research questions in qualitative research
Unlike research questions in quantitative research, research questions in qualitative research are usually continuously reviewed and reformulated. The central question and associated subquestions are stated more than the hypotheses. 15 The central question broadly explores a complex set of factors surrounding the central phenomenon, aiming to present the varied perspectives of participants. 15
There are varied goals for which qualitative research questions are developed. These questions can function in several ways, such as to 1) identify and describe existing conditions ( contextual research question s); 2) describe a phenomenon ( descriptive research questions ); 3) assess the effectiveness of existing methods, protocols, theories, or procedures ( evaluation research questions ); 4) examine a phenomenon or analyze the reasons or relationships between subjects or phenomena ( explanatory research questions ); or 5) focus on unknown aspects of a particular topic ( exploratory research questions ). 5 In addition, some qualitative research questions provide new ideas for the development of theories and actions ( generative research questions ) or advance specific ideologies of a position ( ideological research questions ). 1 Other qualitative research questions may build on a body of existing literature and become working guidelines ( ethnographic research questions ). Research questions may also be broadly stated without specific reference to the existing literature or a typology of questions ( phenomenological research questions ), may be directed towards generating a theory of some process ( grounded theory questions ), or may address a description of the case and the emerging themes ( qualitative case study questions ). 15 We provide examples of contextual, descriptive, evaluation, explanatory, exploratory, generative, ideological, ethnographic, phenomenological, grounded theory, and qualitative case study research questions in qualitative research in Table 4 , and the definition of qualitative hypothesis-generating research in Table 5 .
Qualitative studies usually pose at least one central research question and several subquestions starting with How or What . These research questions use exploratory verbs such as explore or describe . These also focus on one central phenomenon of interest, and may mention the participants and research site. 15
Hypotheses in qualitative research
Hypotheses in qualitative research are stated in the form of a clear statement concerning the problem to be investigated. Unlike in quantitative research where hypotheses are usually developed to be tested, qualitative research can lead to both hypothesis-testing and hypothesis-generating outcomes. 2 When studies require both quantitative and qualitative research questions, this suggests an integrative process between both research methods wherein a single mixed-methods research question can be developed. 1
FRAMEWORKS FOR DEVELOPING RESEARCH QUESTIONS AND HYPOTHESES
Research questions followed by hypotheses should be developed before the start of the study. 1 , 12 , 14 It is crucial to develop feasible research questions on a topic that is interesting to both the researcher and the scientific community. This can be achieved by a meticulous review of previous and current studies to establish a novel topic. Specific areas are subsequently focused on to generate ethical research questions. The relevance of the research questions is evaluated in terms of clarity of the resulting data, specificity of the methodology, objectivity of the outcome, depth of the research, and impact of the study. 1 , 5 These aspects constitute the FINER criteria (i.e., Feasible, Interesting, Novel, Ethical, and Relevant). 1 Clarity and effectiveness are achieved if research questions meet the FINER criteria. In addition to the FINER criteria, Ratan et al. described focus, complexity, novelty, feasibility, and measurability for evaluating the effectiveness of research questions. 14
The PICOT and PEO frameworks are also used when developing research questions. 1 The following elements are addressed in these frameworks, PICOT: P-population/patients/problem, I-intervention or indicator being studied, C-comparison group, O-outcome of interest, and T-timeframe of the study; PEO: P-population being studied, E-exposure to preexisting conditions, and O-outcome of interest. 1 Research questions are also considered good if these meet the “FINERMAPS” framework: Feasible, Interesting, Novel, Ethical, Relevant, Manageable, Appropriate, Potential value/publishable, and Systematic. 14
As we indicated earlier, research questions and hypotheses that are not carefully formulated result in unethical studies or poor outcomes. To illustrate this, we provide some examples of ambiguous research question and hypotheses that result in unclear and weak research objectives in quantitative research ( Table 6 ) 16 and qualitative research ( Table 7 ) 17 , and how to transform these ambiguous research question(s) and hypothesis(es) into clear and good statements.
a These statements were composed for comparison and illustrative purposes only.
b These statements are direct quotes from Higashihara and Horiuchi. 16
a This statement is a direct quote from Shimoda et al. 17
The other statements were composed for comparison and illustrative purposes only.
CONSTRUCTING RESEARCH QUESTIONS AND HYPOTHESES
To construct effective research questions and hypotheses, it is very important to 1) clarify the background and 2) identify the research problem at the outset of the research, within a specific timeframe. 9 Then, 3) review or conduct preliminary research to collect all available knowledge about the possible research questions by studying theories and previous studies. 18 Afterwards, 4) construct research questions to investigate the research problem. Identify variables to be accessed from the research questions 4 and make operational definitions of constructs from the research problem and questions. Thereafter, 5) construct specific deductive or inductive predictions in the form of hypotheses. 4 Finally, 6) state the study aims . This general flow for constructing effective research questions and hypotheses prior to conducting research is shown in Fig. 1 .

Research questions are used more frequently in qualitative research than objectives or hypotheses. 3 These questions seek to discover, understand, explore or describe experiences by asking “What” or “How.” The questions are open-ended to elicit a description rather than to relate variables or compare groups. The questions are continually reviewed, reformulated, and changed during the qualitative study. 3 Research questions are also used more frequently in survey projects than hypotheses in experiments in quantitative research to compare variables and their relationships.
Hypotheses are constructed based on the variables identified and as an if-then statement, following the template, ‘If a specific action is taken, then a certain outcome is expected.’ At this stage, some ideas regarding expectations from the research to be conducted must be drawn. 18 Then, the variables to be manipulated (independent) and influenced (dependent) are defined. 4 Thereafter, the hypothesis is stated and refined, and reproducible data tailored to the hypothesis are identified, collected, and analyzed. 4 The hypotheses must be testable and specific, 18 and should describe the variables and their relationships, the specific group being studied, and the predicted research outcome. 18 Hypotheses construction involves a testable proposition to be deduced from theory, and independent and dependent variables to be separated and measured separately. 3 Therefore, good hypotheses must be based on good research questions constructed at the start of a study or trial. 12
In summary, research questions are constructed after establishing the background of the study. Hypotheses are then developed based on the research questions. Thus, it is crucial to have excellent research questions to generate superior hypotheses. In turn, these would determine the research objectives and the design of the study, and ultimately, the outcome of the research. 12 Algorithms for building research questions and hypotheses are shown in Fig. 2 for quantitative research and in Fig. 3 for qualitative research.

EXAMPLES OF RESEARCH QUESTIONS FROM PUBLISHED ARTICLES
- EXAMPLE 1. Descriptive research question (quantitative research)
- - Presents research variables to be assessed (distinct phenotypes and subphenotypes)
- “BACKGROUND: Since COVID-19 was identified, its clinical and biological heterogeneity has been recognized. Identifying COVID-19 phenotypes might help guide basic, clinical, and translational research efforts.
- RESEARCH QUESTION: Does the clinical spectrum of patients with COVID-19 contain distinct phenotypes and subphenotypes? ” 19
- EXAMPLE 2. Relationship research question (quantitative research)
- - Shows interactions between dependent variable (static postural control) and independent variable (peripheral visual field loss)
- “Background: Integration of visual, vestibular, and proprioceptive sensations contributes to postural control. People with peripheral visual field loss have serious postural instability. However, the directional specificity of postural stability and sensory reweighting caused by gradual peripheral visual field loss remain unclear.
- Research question: What are the effects of peripheral visual field loss on static postural control ?” 20
- EXAMPLE 3. Comparative research question (quantitative research)
- - Clarifies the difference among groups with an outcome variable (patients enrolled in COMPERA with moderate PH or severe PH in COPD) and another group without the outcome variable (patients with idiopathic pulmonary arterial hypertension (IPAH))
- “BACKGROUND: Pulmonary hypertension (PH) in COPD is a poorly investigated clinical condition.
- RESEARCH QUESTION: Which factors determine the outcome of PH in COPD?
- STUDY DESIGN AND METHODS: We analyzed the characteristics and outcome of patients enrolled in the Comparative, Prospective Registry of Newly Initiated Therapies for Pulmonary Hypertension (COMPERA) with moderate or severe PH in COPD as defined during the 6th PH World Symposium who received medical therapy for PH and compared them with patients with idiopathic pulmonary arterial hypertension (IPAH) .” 21
- EXAMPLE 4. Exploratory research question (qualitative research)
- - Explores areas that have not been fully investigated (perspectives of families and children who receive care in clinic-based child obesity treatment) to have a deeper understanding of the research problem
- “Problem: Interventions for children with obesity lead to only modest improvements in BMI and long-term outcomes, and data are limited on the perspectives of families of children with obesity in clinic-based treatment. This scoping review seeks to answer the question: What is known about the perspectives of families and children who receive care in clinic-based child obesity treatment? This review aims to explore the scope of perspectives reported by families of children with obesity who have received individualized outpatient clinic-based obesity treatment.” 22
- EXAMPLE 5. Relationship research question (quantitative research)
- - Defines interactions between dependent variable (use of ankle strategies) and independent variable (changes in muscle tone)
- “Background: To maintain an upright standing posture against external disturbances, the human body mainly employs two types of postural control strategies: “ankle strategy” and “hip strategy.” While it has been reported that the magnitude of the disturbance alters the use of postural control strategies, it has not been elucidated how the level of muscle tone, one of the crucial parameters of bodily function, determines the use of each strategy. We have previously confirmed using forward dynamics simulations of human musculoskeletal models that an increased muscle tone promotes the use of ankle strategies. The objective of the present study was to experimentally evaluate a hypothesis: an increased muscle tone promotes the use of ankle strategies. Research question: Do changes in the muscle tone affect the use of ankle strategies ?” 23
EXAMPLES OF HYPOTHESES IN PUBLISHED ARTICLES
- EXAMPLE 1. Working hypothesis (quantitative research)
- - A hypothesis that is initially accepted for further research to produce a feasible theory
- “As fever may have benefit in shortening the duration of viral illness, it is plausible to hypothesize that the antipyretic efficacy of ibuprofen may be hindering the benefits of a fever response when taken during the early stages of COVID-19 illness .” 24
- “In conclusion, it is plausible to hypothesize that the antipyretic efficacy of ibuprofen may be hindering the benefits of a fever response . The difference in perceived safety of these agents in COVID-19 illness could be related to the more potent efficacy to reduce fever with ibuprofen compared to acetaminophen. Compelling data on the benefit of fever warrant further research and review to determine when to treat or withhold ibuprofen for early stage fever for COVID-19 and other related viral illnesses .” 24
- EXAMPLE 2. Exploratory hypothesis (qualitative research)
- - Explores particular areas deeper to clarify subjective experience and develop a formal hypothesis potentially testable in a future quantitative approach
- “We hypothesized that when thinking about a past experience of help-seeking, a self distancing prompt would cause increased help-seeking intentions and more favorable help-seeking outcome expectations .” 25
- “Conclusion
- Although a priori hypotheses were not supported, further research is warranted as results indicate the potential for using self-distancing approaches to increasing help-seeking among some people with depressive symptomatology.” 25
- EXAMPLE 3. Hypothesis-generating research to establish a framework for hypothesis testing (qualitative research)
- “We hypothesize that compassionate care is beneficial for patients (better outcomes), healthcare systems and payers (lower costs), and healthcare providers (lower burnout). ” 26
- Compassionomics is the branch of knowledge and scientific study of the effects of compassionate healthcare. Our main hypotheses are that compassionate healthcare is beneficial for (1) patients, by improving clinical outcomes, (2) healthcare systems and payers, by supporting financial sustainability, and (3) HCPs, by lowering burnout and promoting resilience and well-being. The purpose of this paper is to establish a scientific framework for testing the hypotheses above . If these hypotheses are confirmed through rigorous research, compassionomics will belong in the science of evidence-based medicine, with major implications for all healthcare domains.” 26
- EXAMPLE 4. Statistical hypothesis (quantitative research)
- - An assumption is made about the relationship among several population characteristics ( gender differences in sociodemographic and clinical characteristics of adults with ADHD ). Validity is tested by statistical experiment or analysis ( chi-square test, Students t-test, and logistic regression analysis)
- “Our research investigated gender differences in sociodemographic and clinical characteristics of adults with ADHD in a Japanese clinical sample. Due to unique Japanese cultural ideals and expectations of women's behavior that are in opposition to ADHD symptoms, we hypothesized that women with ADHD experience more difficulties and present more dysfunctions than men . We tested the following hypotheses: first, women with ADHD have more comorbidities than men with ADHD; second, women with ADHD experience more social hardships than men, such as having less full-time employment and being more likely to be divorced.” 27
- “Statistical Analysis
- ( text omitted ) Between-gender comparisons were made using the chi-squared test for categorical variables and Students t-test for continuous variables…( text omitted ). A logistic regression analysis was performed for employment status, marital status, and comorbidity to evaluate the independent effects of gender on these dependent variables.” 27
EXAMPLES OF HYPOTHESIS AS WRITTEN IN PUBLISHED ARTICLES IN RELATION TO OTHER PARTS
- EXAMPLE 1. Background, hypotheses, and aims are provided
- “Pregnant women need skilled care during pregnancy and childbirth, but that skilled care is often delayed in some countries …( text omitted ). The focused antenatal care (FANC) model of WHO recommends that nurses provide information or counseling to all pregnant women …( text omitted ). Job aids are visual support materials that provide the right kind of information using graphics and words in a simple and yet effective manner. When nurses are not highly trained or have many work details to attend to, these job aids can serve as a content reminder for the nurses and can be used for educating their patients (Jennings, Yebadokpo, Affo, & Agbogbe, 2010) ( text omitted ). Importantly, additional evidence is needed to confirm how job aids can further improve the quality of ANC counseling by health workers in maternal care …( text omitted )” 28
- “ This has led us to hypothesize that the quality of ANC counseling would be better if supported by job aids. Consequently, a better quality of ANC counseling is expected to produce higher levels of awareness concerning the danger signs of pregnancy and a more favorable impression of the caring behavior of nurses .” 28
- “This study aimed to examine the differences in the responses of pregnant women to a job aid-supported intervention during ANC visit in terms of 1) their understanding of the danger signs of pregnancy and 2) their impression of the caring behaviors of nurses to pregnant women in rural Tanzania.” 28
- EXAMPLE 2. Background, hypotheses, and aims are provided
- “We conducted a two-arm randomized controlled trial (RCT) to evaluate and compare changes in salivary cortisol and oxytocin levels of first-time pregnant women between experimental and control groups. The women in the experimental group touched and held an infant for 30 min (experimental intervention protocol), whereas those in the control group watched a DVD movie of an infant (control intervention protocol). The primary outcome was salivary cortisol level and the secondary outcome was salivary oxytocin level.” 29
- “ We hypothesize that at 30 min after touching and holding an infant, the salivary cortisol level will significantly decrease and the salivary oxytocin level will increase in the experimental group compared with the control group .” 29
- EXAMPLE 3. Background, aim, and hypothesis are provided
- “In countries where the maternal mortality ratio remains high, antenatal education to increase Birth Preparedness and Complication Readiness (BPCR) is considered one of the top priorities [1]. BPCR includes birth plans during the antenatal period, such as the birthplace, birth attendant, transportation, health facility for complications, expenses, and birth materials, as well as family coordination to achieve such birth plans. In Tanzania, although increasing, only about half of all pregnant women attend an antenatal clinic more than four times [4]. Moreover, the information provided during antenatal care (ANC) is insufficient. In the resource-poor settings, antenatal group education is a potential approach because of the limited time for individual counseling at antenatal clinics.” 30
- “This study aimed to evaluate an antenatal group education program among pregnant women and their families with respect to birth-preparedness and maternal and infant outcomes in rural villages of Tanzania.” 30
- “ The study hypothesis was if Tanzanian pregnant women and their families received a family-oriented antenatal group education, they would (1) have a higher level of BPCR, (2) attend antenatal clinic four or more times, (3) give birth in a health facility, (4) have less complications of women at birth, and (5) have less complications and deaths of infants than those who did not receive the education .” 30
Research questions and hypotheses are crucial components to any type of research, whether quantitative or qualitative. These questions should be developed at the very beginning of the study. Excellent research questions lead to superior hypotheses, which, like a compass, set the direction of research, and can often determine the successful conduct of the study. Many research studies have floundered because the development of research questions and subsequent hypotheses was not given the thought and meticulous attention needed. The development of research questions and hypotheses is an iterative process based on extensive knowledge of the literature and insightful grasp of the knowledge gap. Focused, concise, and specific research questions provide a strong foundation for constructing hypotheses which serve as formal predictions about the research outcomes. Research questions and hypotheses are crucial elements of research that should not be overlooked. They should be carefully thought of and constructed when planning research. This avoids unethical studies and poor outcomes by defining well-founded objectives that determine the design, course, and outcome of the study.
Disclosure: The authors have no potential conflicts of interest to disclose.
Author Contributions:
- Conceptualization: Barroga E, Matanguihan GJ.
- Methodology: Barroga E, Matanguihan GJ.
- Writing - original draft: Barroga E, Matanguihan GJ.
- Writing - review & editing: Barroga E, Matanguihan GJ.

Experimental Hypothesis
Ai generator.
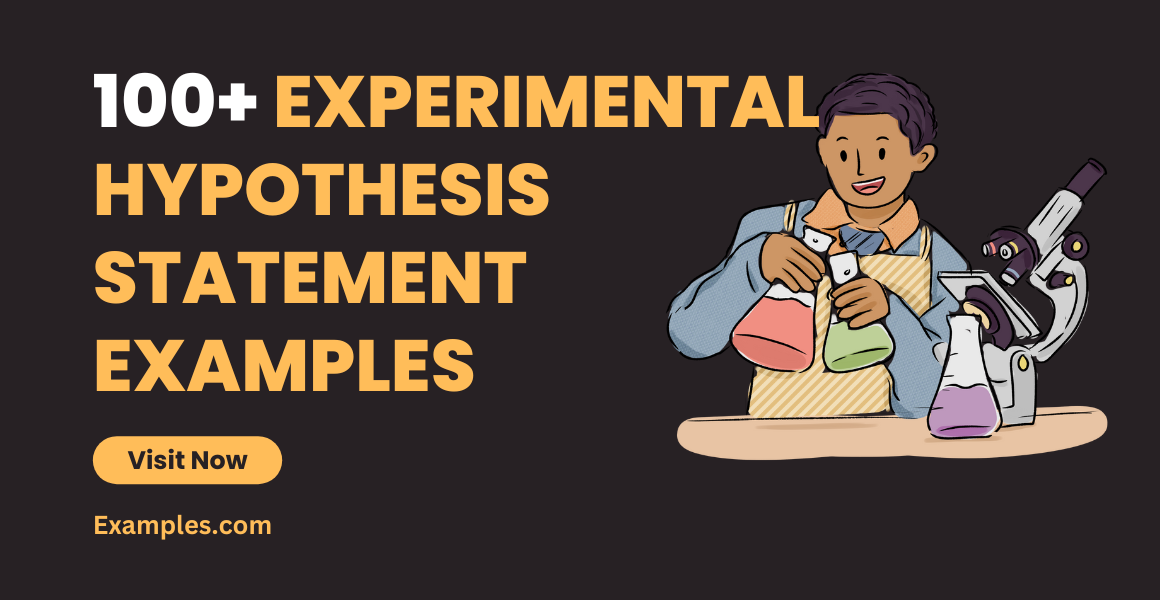
Hypotheses stand at the very heart of experiments, guiding the inquisitive minds towards deeper understanding and discoveries. Crafting a well-framed experimental hypothesis is both an art and a science. Dive into our comprehensive guide, filled with intriguing thesis statement examples and tips, to master the art of formulating compelling experimental hypotheses that not only pique curiosity but also pave the way for meaningful investigations.
What is Experimental Hypothesis?
An experimental hypothesis, often referred to simply as a hypothesis, is a precise, testable statement about the expected outcome of an experiment or a research study. It predicts a relationship between two or more variables that can be tested through controlled experiments. Essentially, it provides a clear direction for an experiment by making a claim about a potential cause-and-effect relationship.
What is an example of an Experimental Hypothesis Statement?
Suppose a researcher believes that consuming a particular herbal tea might improve memory in adults over 60 years of age. The experimental hypothesis could be stated as:
“If adults over 60 years of age drink the specified herbal tea daily, then their memory performance on standardized tests will improve when compared to those who do not consume the tea.”
This hypothesis clearly presents a predicted relationship between the independent variable (herbal tea consumption) and the dependent variable (memory performance on standardized tests). It’s testable, specific, and gives direction to the research.
100 Experimental Hypothesis Statement Examples
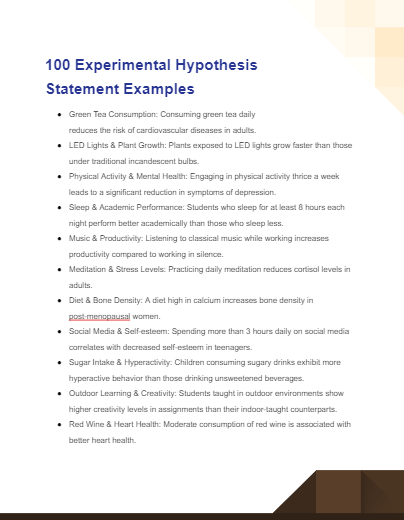
Size: 223 KB
Experimental hypotheses serve as predictive cornerstones in the vast realm of scientific inquiry. They articulate potential cause-and-effect relationships between variables, guiding researchers in their quest for knowledge. Below, you’ll discover an array of experimental hypotheses, each shedding light on a different dimension of our intricate world.
- Green Tea Consumption : Consuming green tea daily reduces the risk of cardiovascular diseases in adults.
- LED Lights & Plant Growth : Plants exposed to LED lights grow faster than those under traditional incandescent bulbs.
- Physical Activity & Mental Health : Engaging in physical activity thrice a week leads to a significant reduction in symptoms of depression.
- Sleep & Academic Performance : Students who sleep for at least 8 hours each night perform better academically than those who sleep less.
- Music & Productivity : Listening to classical music while working increases productivity compared to working in silence.
- Meditation & Stress Levels : Practicing daily meditation reduces cortisol levels in adults.
- Diet & Bone Density : A diet high in calcium increases bone density in post-menopausal women.
- Social Media & Self-esteem : Spending more than 3 hours daily on social media correlates with decreased self-esteem in teenagers.
- Sugar Intake & Hyperactivity : Children consuming sugary drinks exhibit more hyperactive behavior than those drinking unsweetened beverages.
- Outdoor Learning & Creativity : Students taught in outdoor environments show higher creativity levels in assignments than their indoor-taught counterparts.
- Red Wine & Heart Health : Moderate consumption of red wine is associated with better heart health.
- Chocolates & Mood : Eating dark chocolate can lead to a short-term mood boost in adults.
- Air Pollution & Respiratory Diseases : Living in areas with high air pollution increases the risk of developing respiratory diseases.
- Pet Ownership & Loneliness : Individuals owning pets report lower feelings of loneliness compared to non-pet owners.
- Bilingualism & Cognitive Abilities : Bilingual children score higher in cognitive ability tests than monolingual children.
- Digital Games & Problem-solving Skills : Playing strategic digital games enhances problem-solving skills in teenagers.
- Yoga & Flexibility : Regular yoga practice results in increased flexibility and muscle strength.
- High Protein Diet & Weight Loss : Consuming a high-protein diet leads to faster weight loss compared to a low-protein diet.
- Forest Bathing & Well-being : Engaging in forest bathing activities reduces anxiety and promotes a sense of well-being.
- Reading Habits & Vocabulary : Children who read books daily have a more extensive vocabulary than those who don’t.
- Coffee & Alertness : Regular coffee drinkers exhibit higher alertness levels than non-coffee drinkers in early morning hours.
- Urban Living & Anxiety : Individuals living in urban environments experience higher anxiety levels than those in rural settings.
- Art Classes & Emotional Expression : Children attending weekly art classes display a more profound ability for emotional expression than those who don’t attend such classes.
- E-books vs. Printed Books : Students reading from printed books have better retention than those reading from e-books.
- Sunscreen & Skin Health : Regular use of sunscreen reduces the risk of skin damage due to UV rays.
- High-Fiber Diet & Digestion : Consuming a high-fiber diet leads to improved digestion and reduced instances of constipation.
- Homework & Academic Performance : Students who consistently complete their homework score higher on standardized tests.
- Virtual Reality & Pain Management : Using virtual reality during minor surgical procedures reduces the perceived pain in patients.
- Music Lessons & Mathematical Ability : Children taking music lessons perform better in math tests compared to those who don’t.
- Nature Walks & Concentration : Taking a short walk in nature improves concentration and reduces cognitive fatigue.
- Red Meat Consumption & Heart Health : Regular consumption of red meat is associated with a higher risk of heart-related diseases.
- Office Plants & Productivity : Offices with indoor plants see higher productivity and lower stress levels among employees.
- Dairy Intake & Bone Strength : Children who consume dairy products regularly have stronger bones than those who avoid dairy.
- Video Conferencing & Team Cohesion : Teams that use video conferencing for meetings feel more cohesive than teams relying only on voice calls.
- Organic Foods & Health Benefits : Consuming organic foods leads to a lower intake of pesticides and may offer health benefits over conventionally grown foods.
- Laughter & Immune System : Engaging in activities that induce laughter boosts the immune system.
- Travel Experiences & Cultural Tolerance : Individuals who travel internationally are more culturally tolerant than those who don’t travel.
- Weight Training & Metabolism : Engaging in weight training activities thrice a week boosts metabolism in adults.
- Night-time Screen Exposure & Sleep Quality : Exposure to screens before bedtime reduces the quality and duration of sleep.
- Group Studies & Learning Retention : Students who engage in group studies retain information better than those studying alone.
- Aquatic Therapy & Joint Pain : Engaging in aquatic therapy reduces joint pain more effectively than traditional physiotherapy.
- Language Learning Apps & Proficiency : Individuals using language learning apps daily achieve greater proficiency than those learning through traditional methods.
- Aerobic Exercise & Memory : Regular aerobic exercise enhances memory and cognitive functions in older adults.
- Pet Therapy & Hospital Recovery : Patients exposed to pet therapy during hospital stays report faster recovery rates and lower stress levels.
- Gardening & Mental Well-being : Individuals engaging in gardening activities experience improved mental well-being and lower depression symptoms.
- Vegetarian Diet & Cholesterol Levels : Adopting a vegetarian diet leads to lower cholesterol levels compared to omnivorous diets.
- Mindfulness Practices & Attention Span : Incorporating daily mindfulness practices increases attention span in school-going children.
- Online Tutorials & Skill Development : Individuals using online tutorials for skill development learn faster than those attending conventional classes.
- Dark Mode Screens & Eye Strain : Using dark mode on digital devices reduces eye strain and improves sleep quality.
- Open Office Spaces & Collaboration : Employees in open office spaces collaborate more frequently than those in closed offices.
- Herbal Teas & Relaxation : Consuming herbal teas like chamomile and lavender promotes relaxation and reduces anxiety symptoms.
- Dance Classes & Body Coordination : Attending regular dance classes improves body coordination and balance.
- Tree-rich Areas & Air Quality : Regions with a higher density of trees have better air quality and lower pollution levels.
- Probiotics & Gut Health : Regular intake of probiotics leads to a healthier gut and reduced digestive issues.
- Journaling & Emotional Regulation : Individuals who practice daily journaling exhibit better emotional regulation.
- Solar Panels & Energy Efficiency : Homes equipped with solar panels consume less non-renewable energy and have lower electricity bills.
- Remote Work & Job Satisfaction : Employees who work remotely report higher job satisfaction levels than those working on-site.
- Car Pooling & Carbon Emissions : Carpooling significantly reduces individual carbon emissions compared to solo driving.
- Therapeutic Music & Hospital Recovery : Playing therapeutic music in hospital wards accelerates the recovery process of patients.
- Active Commuting & Physical Health : Individuals who engage in active commuting (walking or cycling to work) have better physical health than those using vehicles.
- Robot-assisted Surgery & Recovery Time : Patients undergoing robot-assisted surgeries experience shorter recovery times than those having traditional surgeries.
- Podcast Learning & Retention : Individuals learning through podcasts retain more information than those learning through printed materials.
- Green Roofs & Building Temperature : Buildings with green roofs maintain a more consistent interior temperature, reducing the need for heating or cooling.
- Local Produce & Nutritional Value : Consuming local produce leads to a higher intake of nutrients than consuming imported produce.
- Intermittent Fasting & Weight Management : Individuals practicing intermittent fasting experience better weight management than those following traditional diet plans.
- 3D Printing & Customization : Products manufactured using 3D printing techniques offer better customization options than traditionally manufactured products.
- Ergonomic Workstations & Employee Health : Employees using ergonomic workstations report fewer musculoskeletal issues.
- Flipped Classrooms & Student Engagement : Students in flipped classroom settings are more engaged and participate more actively than in traditional classrooms.
- Elderly & VR Exercises : Elderly individuals engaging in VR-assisted exercises show improved balance and reduced fall risk.
- Ceramic Coating & Vehicle Protection : Vehicles with ceramic coatings have better protection against environmental damages than those without.
- Augmented Reality & Shopping Experience : Shoppers using augmented reality apps report a more satisfying shopping experience.
- Meditation & Blood Pressure : Individuals practicing daily meditation have lower blood pressure levels than those who don’t meditate.
- Afforestation & Biodiversity : Areas undergoing afforestation see a significant increase in local biodiversity.
- Subscription Models & Customer Loyalty : Businesses using subscription models achieve higher customer loyalty and retention rates.
- Farm-to-Table & Food Freshness : Restaurants following a farm-to-table approach serve fresher and more flavorful dishes.
- Paperless Offices & Efficiency : Offices operating on a paperless model report higher efficiency and reduced costs.
- Sensory Play & Child Development : Children exposed to sensory play activities exhibit enhanced cognitive and motor skills.
- Desalination & Water Quality : Water from desalination processes is of comparable quality to freshwater sources.
- Biofuels & Engine Performance : Engines running on biofuels show similar performance to those using traditional fuels but with reduced emissions.
- Recycled Products & Environmental Impact : Products manufactured from recycled materials significantly reduce environmental impact compared to those made from new resources.
- Urban Gardens & Mental Health : Urban residents who participate in community gardening experience improved mental health compared to those who don’t.
- LED Lighting & Energy Consumption : Buildings that use LED lighting consume significantly less energy than those using conventional bulbs.
- Online Therapy & Accessibility : Patients using online therapy platforms attend sessions more consistently than those having face-to-face appointments.
- Digital Games & Cognitive Skills : Children who play educational digital games enhance their cognitive skills faster than those who play non-educational games.
- Hydroponics & Plant Growth : Plants grown hydroponically grow faster and yield more than plants grown in traditional soil.
- Noise-cancelling Headphones & Productivity : Employees using noise-cancelling headphones in open offices experience higher productivity levels.
- Plant-based Diets & Heart Health : Individuals on plant-based diets have a lower risk of developing heart diseases than those on omnivorous diets.
- E-books & Reading Comprehension : Readers using e-books retain information similarly to those reading traditional printed books.
- Yoga & Stress Reduction : Regular practice of yoga leads to a significant reduction in stress and anxiety levels.
- Wearable Fitness Trackers & Physical Activity : Individuals using wearable fitness trackers engage in more physical activity than those without.
- Aquaponics & Agricultural Efficiency : Aquaponic systems yield higher crop production compared to traditional farming methods in the same space.
- Artificial Intelligence & Medical Diagnosis : AI-powered diagnostic tools detect certain medical conditions with similar accuracy as trained medical professionals.
- Biodegradable Packaging & Waste Management : Products using biodegradable packaging contribute to a significant reduction in landfill waste.
- Virtual Reality & Training Efficiency : Employees trained using virtual reality simulations are better prepared than those using traditional training methods.
- Organic Farming & Soil Health : Farmlands managed using organic farming practices have healthier soil with better nutrient retention.
- Mobile Banking & Financial Accessibility : Mobile banking services provide greater financial accessibility to rural populations than traditional banks.
- Green Tea & Metabolism : Regular consumption of green tea boosts metabolism and assists in weight management.
- Prefabricated Houses & Construction Time : Prefabricated houses require significantly less construction time than traditionally built homes.
- Telecommuting & Job Satisfaction : Employees offered the option of telecommuting experience higher job satisfaction and reduced turnover rates.
- Bilingual Education & Cognitive Flexibility : Students in bilingual education programs develop greater cognitive flexibility and problem-solving skills than monolingual peers.
Experimental Hypothesis Statement Examples in Research
Experimental hypotheses in research are central predictions that guide the design of an investigation. These statements propose specific outcomes based on manipulated variables, enabling a clear and focused exploration.
- AI Algorithms & Job Recruitment : In recruitment processes, AI algorithms reduce bias more effectively than traditional human-led processes.
- Smart Cities & Crime Rate : The implementation of smart city technologies correlates with a reduction in urban crime rates.
- Nano-coatings & Product Lifespan : Products coated with nano-materials exhibit a prolonged lifespan compared to those without.
- Blockchain & Supply Chain Efficiency : The integration of blockchain technology into supply chains results in higher transparency and efficiency.
- Microfinance & Poverty Alleviation : Microfinance initiatives lead to increased financial independence among low-income households in developing countries.
Test Hypothesis Statement Examples
Test hypotheses are specific predictions made before testing a particular concept or intervention. They play a critical role in narrowing down the scope of the experiment, ensuring clarity and direction.
- Carbon Fiber & Structural Integrity : Structures made with carbon fiber reinforcements will bear more weight than traditional structures.
- Ergonomic Chairs & Back Pain : Employees provided with ergonomic chairs will report fewer instances of back pain.
- Cloud Computing & Data Retrieval Speed : Cloud computing solutions provide faster data retrieval times than local server storage.
- Solar Windows & Energy Efficiency : Buildings fitted with solar windows produce a significant percentage of their energy needs.
- Drip Irrigation & Crop Yield : Farmlands utilizing drip irrigation have a higher crop yield compared to those using traditional irrigation methods.
Experimental Hypothesis Statement Examples in Biology
In the realm of biology, experimental hypotheses dive into the intricacies of living organisms, predicting outcomes based on biological principles and observations.
- CRISPR & Genetic Disorders : The CRISPR technology can reduce the occurrence of specific genetic disorders.
- Probiotics & Gut Health : Regular consumption of probiotics leads to improved gut flora balance.
- Phytoplankton & Ocean Acidity : An increase in phytoplankton concentration correlates with reduced ocean acidity.
- Stem Cells & Tissue Regeneration : The introduction of specific stem cells accelerates the regeneration of damaged tissues.
- Biodiversity & Ecosystem Resilience : Ecosystems with higher biodiversity demonstrate greater resilience against environmental disruptions.
Alternative Experimental Hypothesis Statement Examples
Alternative hypotheses predict that there will be an effect as a result of the experiment. These statements contrast with the null hypothesis, which predicts no effect.
- Night-time Screen Exposure & Sleep Quality : Exposure to screens before bedtime negatively affects the quality of sleep.
- Afforestation & Air Quality : Areas with afforestation initiatives will have improved air quality.
- Hydrogel & Wound Healing : Hydrogel applications on open wounds accelerate the healing process.
- Intermittent Fasting & Metabolic Rate : Engaging in intermittent fasting enhances the metabolic rate.
- VR Learning & Retention Rate : Students taught through VR experiences retain information more effectively than through traditional teaching methods.
Experimental Hypothesis Statement Examples in Psychology
Experimental Psychology hypotheses often delve into behavioral, cognitive, or emotional outcomes based on specific interventions or observations.
- Music Therapy & Trauma Recovery : Trauma victims undergoing music therapy experience faster emotional recovery.
- Meditation & Attention Span : Regular meditation increases an individual’s attention span.
- Nature Exposure & Stress Reduction : Regular exposure to natural environments significantly reduces stress levels.
- Positive Reinforcement & Skill Acquisition : Using positive reinforcement techniques accelerates the rate of skill acquisition in children.
- Social Media & Self-esteem : Prolonged usage of social media platforms correlates with reduced self-esteem in teenagers.
Experimental Hypothesis Statement Examples in Testing Research
In testing research, experimental hypotheses focus on the outcomes of specific tests or interventions, aiding in the understanding of tool efficacy and methodological impacts.
- Dynamic Testing & Learning Agility : Students undergoing dynamic testing demonstrate higher learning agility.
- A/B Testing & Website Engagement : A/B testing on website layouts leads to enhanced user engagement.
- VR Simulation & Skill Transfer : Training professionals using VR simulations results in better skill transfer to real-world scenarios.
- Remote User Testing & Software Usability : Software products tested through remote user testing yield higher usability scores.
- Mobile Eye-tracking & User Experience (UX) : The use of mobile eye-tracking in UX research provides deeper insights into user preferences and behaviors.
How do you find the experimental hypothesis?
The experimental hypothesis is the prediction or statement that suggests a relationship between two or more variables in an experiment. Finding or determining it requires clear identification of the research question and a clear understanding of the variables involved.
- Identify Your Research Question : Before you can come up with a hypothesis, you need to know what you’re aiming to study. What’s the primary purpose of your research?
- Determine the Key Variables : Understand what you’ll manipulate (independent variable) and what you’ll measure (dependent variable).
- Formulate a Relationship : Your experimental hypothesis should indicate a relationship or effect between the variables.
- Ensure It’s Testable : Make sure your hypothesis is something you can test using the tools, methods, and techniques at your disposal.
Is research hypothesis same as experimental hypothesis?
Both terms are frequently used interchangeably, but they have distinct meanings:
- Research Hypothesis : A generalized statement regarding an anticipated relationship between variables, or an anticipated outcome. It’s a broad statement that gives an overview of what the researcher aims to achieve in the study.
- Experimental Hypothesis : More specific than a research hypothesis, the experimental hypothesis predicts the relationship between two or more variables in an experiment. It’s a precise statement indicating the expected outcome of an experiment, often framed in terms of how one variable will affect another.
In essence, while all experimental hypotheses are research hypotheses, not all research hypotheses are experimental.
How do you write an experimental hypothesis? – A Step by Step Guide
- Understand the Problem : Begin by thoroughly understanding the research problem or question.
- Identify the Variables : Clearly identify the independent and dependent variables. The independent variable is what you will change or manipulate. The dependent variable is what you’ll observe or measure as a result.
- Predict the Outcome : Based on existing literature, prior knowledge, or logical reasoning, predict the outcome or effect.
- Write a Clear, Concise Statement : Your hypothesis should be a simple, clear statement that reflects the expected relationship between variables. For example: “Increasing the amount of sunlight plants receive (independent variable) will increase their rate of growth (dependent variable).”
- Make it Testable : Ensure your hypothesis is formulated in a way that allows for empirical testing.
Tips for Writing Experimental Hypothesis
- Stay Specific : Avoid vague terms and ensure that your hypothesis specifies the relationship between your variables.
- Be Concise : Your hypothesis should be a clear and succinct statement, not a paragraph.
- Ground it in Literature : Base your hypothesis on existing research to ensure its relevance and feasibility.
- Avoid Bias : Ensure your hypothesis is written objectively without indicating a preferred outcome.
- Iterate : Your initial hypothesis might evolve as you delve deeper into your research. It’s okay to refine it for clarity or accuracy.
- Seek Feedback : Before finalizing, discuss your hypothesis with peers, mentors, or experts in the field for additional insights or potential pitfalls.
Remember, a well-crafted hypothesis is not just about being right or wrong; it’s about creating a solid foundation upon which to build your research.
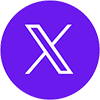
Text prompt
- Instructive
- Professional
10 Examples of Public speaking
20 Examples of Gas lighting
- Experimental Research Designs: Types, Examples & Methods

Experimental research is the most familiar type of research design for individuals in the physical sciences and a host of other fields. This is mainly because experimental research is a classical scientific experiment, similar to those performed in high school science classes.
Imagine taking 2 samples of the same plant and exposing one of them to sunlight, while the other is kept away from sunlight. Let the plant exposed to sunlight be called sample A, while the latter is called sample B.
If after the duration of the research, we find out that sample A grows and sample B dies, even though they are both regularly wetted and given the same treatment. Therefore, we can conclude that sunlight will aid growth in all similar plants.
What is Experimental Research?
Experimental research is a scientific approach to research, where one or more independent variables are manipulated and applied to one or more dependent variables to measure their effect on the latter. The effect of the independent variables on the dependent variables is usually observed and recorded over some time, to aid researchers in drawing a reasonable conclusion regarding the relationship between these 2 variable types.
The experimental research method is widely used in physical and social sciences, psychology, and education. It is based on the comparison between two or more groups with a straightforward logic, which may, however, be difficult to execute.
Mostly related to a laboratory test procedure, experimental research designs involve collecting quantitative data and performing statistical analysis on them during research. Therefore, making it an example of quantitative research method .
What are The Types of Experimental Research Design?
The types of experimental research design are determined by the way the researcher assigns subjects to different conditions and groups. They are of 3 types, namely; pre-experimental, quasi-experimental, and true experimental research.
Pre-experimental Research Design
In pre-experimental research design, either a group or various dependent groups are observed for the effect of the application of an independent variable which is presumed to cause change. It is the simplest form of experimental research design and is treated with no control group.
Although very practical, experimental research is lacking in several areas of the true-experimental criteria. The pre-experimental research design is further divided into three types
- One-shot Case Study Research Design
In this type of experimental study, only one dependent group or variable is considered. The study is carried out after some treatment which was presumed to cause change, making it a posttest study.
- One-group Pretest-posttest Research Design:
This research design combines both posttest and pretest study by carrying out a test on a single group before the treatment is administered and after the treatment is administered. With the former being administered at the beginning of treatment and later at the end.
- Static-group Comparison:
In a static-group comparison study, 2 or more groups are placed under observation, where only one of the groups is subjected to some treatment while the other groups are held static. All the groups are post-tested, and the observed differences between the groups are assumed to be a result of the treatment.
Quasi-experimental Research Design
The word “quasi” means partial, half, or pseudo. Therefore, the quasi-experimental research bearing a resemblance to the true experimental research, but not the same. In quasi-experiments, the participants are not randomly assigned, and as such, they are used in settings where randomization is difficult or impossible.
This is very common in educational research, where administrators are unwilling to allow the random selection of students for experimental samples.
Some examples of quasi-experimental research design include; the time series, no equivalent control group design, and the counterbalanced design.
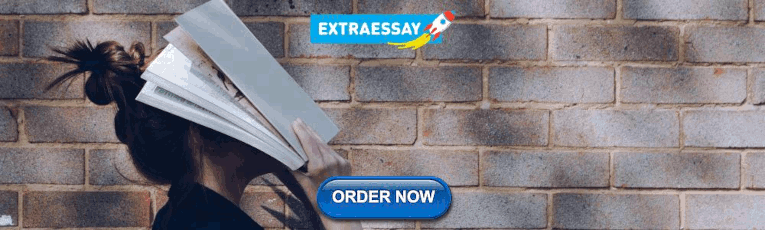
True Experimental Research Design
The true experimental research design relies on statistical analysis to approve or disprove a hypothesis. It is the most accurate type of experimental design and may be carried out with or without a pretest on at least 2 randomly assigned dependent subjects.
The true experimental research design must contain a control group, a variable that can be manipulated by the researcher, and the distribution must be random. The classification of true experimental design include:
- The posttest-only Control Group Design: In this design, subjects are randomly selected and assigned to the 2 groups (control and experimental), and only the experimental group is treated. After close observation, both groups are post-tested, and a conclusion is drawn from the difference between these groups.
- The pretest-posttest Control Group Design: For this control group design, subjects are randomly assigned to the 2 groups, both are presented, but only the experimental group is treated. After close observation, both groups are post-tested to measure the degree of change in each group.
- Solomon four-group Design: This is the combination of the pretest-only and the pretest-posttest control groups. In this case, the randomly selected subjects are placed into 4 groups.
The first two of these groups are tested using the posttest-only method, while the other two are tested using the pretest-posttest method.
Examples of Experimental Research
Experimental research examples are different, depending on the type of experimental research design that is being considered. The most basic example of experimental research is laboratory experiments, which may differ in nature depending on the subject of research.
Administering Exams After The End of Semester
During the semester, students in a class are lectured on particular courses and an exam is administered at the end of the semester. In this case, the students are the subjects or dependent variables while the lectures are the independent variables treated on the subjects.
Only one group of carefully selected subjects are considered in this research, making it a pre-experimental research design example. We will also notice that tests are only carried out at the end of the semester, and not at the beginning.
Further making it easy for us to conclude that it is a one-shot case study research.
Employee Skill Evaluation
Before employing a job seeker, organizations conduct tests that are used to screen out less qualified candidates from the pool of qualified applicants. This way, organizations can determine an employee’s skill set at the point of employment.
In the course of employment, organizations also carry out employee training to improve employee productivity and generally grow the organization. Further evaluation is carried out at the end of each training to test the impact of the training on employee skills, and test for improvement.
Here, the subject is the employee, while the treatment is the training conducted. This is a pretest-posttest control group experimental research example.
Evaluation of Teaching Method
Let us consider an academic institution that wants to evaluate the teaching method of 2 teachers to determine which is best. Imagine a case whereby the students assigned to each teacher is carefully selected probably due to personal request by parents or due to stubbornness and smartness.
This is a no equivalent group design example because the samples are not equal. By evaluating the effectiveness of each teacher’s teaching method this way, we may conclude after a post-test has been carried out.
However, this may be influenced by factors like the natural sweetness of a student. For example, a very smart student will grab more easily than his or her peers irrespective of the method of teaching.
What are the Characteristics of Experimental Research?
Experimental research contains dependent, independent and extraneous variables. The dependent variables are the variables being treated or manipulated and are sometimes called the subject of the research.
The independent variables are the experimental treatment being exerted on the dependent variables. Extraneous variables, on the other hand, are other factors affecting the experiment that may also contribute to the change.
The setting is where the experiment is carried out. Many experiments are carried out in the laboratory, where control can be exerted on the extraneous variables, thereby eliminating them.
Other experiments are carried out in a less controllable setting. The choice of setting used in research depends on the nature of the experiment being carried out.
- Multivariable
Experimental research may include multiple independent variables, e.g. time, skills, test scores, etc.
Why Use Experimental Research Design?
Experimental research design can be majorly used in physical sciences, social sciences, education, and psychology. It is used to make predictions and draw conclusions on a subject matter.
Some uses of experimental research design are highlighted below.
- Medicine: Experimental research is used to provide the proper treatment for diseases. In most cases, rather than directly using patients as the research subject, researchers take a sample of the bacteria from the patient’s body and are treated with the developed antibacterial
The changes observed during this period are recorded and evaluated to determine its effectiveness. This process can be carried out using different experimental research methods.
- Education: Asides from science subjects like Chemistry and Physics which involves teaching students how to perform experimental research, it can also be used in improving the standard of an academic institution. This includes testing students’ knowledge on different topics, coming up with better teaching methods, and the implementation of other programs that will aid student learning.
- Human Behavior: Social scientists are the ones who mostly use experimental research to test human behaviour. For example, consider 2 people randomly chosen to be the subject of the social interaction research where one person is placed in a room without human interaction for 1 year.
The other person is placed in a room with a few other people, enjoying human interaction. There will be a difference in their behaviour at the end of the experiment.
- UI/UX: During the product development phase, one of the major aims of the product team is to create a great user experience with the product. Therefore, before launching the final product design, potential are brought in to interact with the product.
For example, when finding it difficult to choose how to position a button or feature on the app interface, a random sample of product testers are allowed to test the 2 samples and how the button positioning influences the user interaction is recorded.
What are the Disadvantages of Experimental Research?
- It is highly prone to human error due to its dependency on variable control which may not be properly implemented. These errors could eliminate the validity of the experiment and the research being conducted.
- Exerting control of extraneous variables may create unrealistic situations. Eliminating real-life variables will result in inaccurate conclusions. This may also result in researchers controlling the variables to suit his or her personal preferences.
- It is a time-consuming process. So much time is spent on testing dependent variables and waiting for the effect of the manipulation of dependent variables to manifest.
- It is expensive.
- It is very risky and may have ethical complications that cannot be ignored. This is common in medical research, where failed trials may lead to a patient’s death or a deteriorating health condition.
- Experimental research results are not descriptive.
- Response bias can also be supplied by the subject of the conversation.
- Human responses in experimental research can be difficult to measure.
What are the Data Collection Methods in Experimental Research?
Data collection methods in experimental research are the different ways in which data can be collected for experimental research. They are used in different cases, depending on the type of research being carried out.
1. Observational Study
This type of study is carried out over a long period. It measures and observes the variables of interest without changing existing conditions.
When researching the effect of social interaction on human behavior, the subjects who are placed in 2 different environments are observed throughout the research. No matter the kind of absurd behavior that is exhibited by the subject during this period, its condition will not be changed.
This may be a very risky thing to do in medical cases because it may lead to death or worse medical conditions.
2. Simulations
This procedure uses mathematical, physical, or computer models to replicate a real-life process or situation. It is frequently used when the actual situation is too expensive, dangerous, or impractical to replicate in real life.
This method is commonly used in engineering and operational research for learning purposes and sometimes as a tool to estimate possible outcomes of real research. Some common situation software are Simulink, MATLAB, and Simul8.
Not all kinds of experimental research can be carried out using simulation as a data collection tool . It is very impractical for a lot of laboratory-based research that involves chemical processes.
A survey is a tool used to gather relevant data about the characteristics of a population and is one of the most common data collection tools. A survey consists of a group of questions prepared by the researcher, to be answered by the research subject.
Surveys can be shared with the respondents both physically and electronically. When collecting data through surveys, the kind of data collected depends on the respondent, and researchers have limited control over it.
Formplus is the best tool for collecting experimental data using survey s. It has relevant features that will aid the data collection process and can also be used in other aspects of experimental research.
Differences between Experimental and Non-Experimental Research
1. In experimental research, the researcher can control and manipulate the environment of the research, including the predictor variable which can be changed. On the other hand, non-experimental research cannot be controlled or manipulated by the researcher at will.
This is because it takes place in a real-life setting, where extraneous variables cannot be eliminated. Therefore, it is more difficult to conclude non-experimental studies, even though they are much more flexible and allow for a greater range of study fields.
2. The relationship between cause and effect cannot be established in non-experimental research, while it can be established in experimental research. This may be because many extraneous variables also influence the changes in the research subject, making it difficult to point at a particular variable as the cause of a particular change
3. Independent variables are not introduced, withdrawn, or manipulated in non-experimental designs, but the same may not be said about experimental research.
Conclusion
Experimental research designs are often considered to be the standard in research designs. This is partly due to the common misconception that research is equivalent to scientific experiments—a component of experimental research design.
In this research design, one or more subjects or dependent variables are randomly assigned to different treatments (i.e. independent variables manipulated by the researcher) and the results are observed to conclude. One of the uniqueness of experimental research is in its ability to control the effect of extraneous variables.
Experimental research is suitable for research whose goal is to examine cause-effect relationships, e.g. explanatory research. It can be conducted in the laboratory or field settings, depending on the aim of the research that is being carried out.
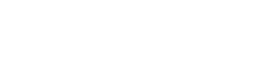
Connect to Formplus, Get Started Now - It's Free!
- examples of experimental research
- experimental research methods
- types of experimental research
- busayo.longe
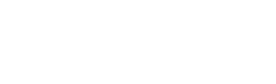
You may also like:
Response vs Explanatory Variables: Definition & Examples
In this article, we’ll be comparing the two types of variables, what they both mean and see some of their real-life applications in research

Experimental Vs Non-Experimental Research: 15 Key Differences
Differences between experimental and non experimental research on definitions, types, examples, data collection tools, uses, advantages etc.
Simpson’s Paradox & How to Avoid it in Experimental Research
In this article, we are going to look at Simpson’s Paradox from its historical point and later, we’ll consider its effect in...
What is Experimenter Bias? Definition, Types & Mitigation
In this article, we will look into the concept of experimental bias and how it can be identified in your research
Formplus - For Seamless Data Collection
Collect data the right way with a versatile data collection tool. try formplus and transform your work productivity today..

Experimental Research Design — 6 mistakes you should never make!
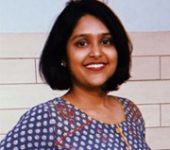
Since school days’ students perform scientific experiments that provide results that define and prove the laws and theorems in science. These experiments are laid on a strong foundation of experimental research designs.
An experimental research design helps researchers execute their research objectives with more clarity and transparency.
In this article, we will not only discuss the key aspects of experimental research designs but also the issues to avoid and problems to resolve while designing your research study.
Table of Contents
What Is Experimental Research Design?
Experimental research design is a framework of protocols and procedures created to conduct experimental research with a scientific approach using two sets of variables. Herein, the first set of variables acts as a constant, used to measure the differences of the second set. The best example of experimental research methods is quantitative research .
Experimental research helps a researcher gather the necessary data for making better research decisions and determining the facts of a research study.
When Can a Researcher Conduct Experimental Research?
A researcher can conduct experimental research in the following situations —
- When time is an important factor in establishing a relationship between the cause and effect.
- When there is an invariable or never-changing behavior between the cause and effect.
- Finally, when the researcher wishes to understand the importance of the cause and effect.
Importance of Experimental Research Design
To publish significant results, choosing a quality research design forms the foundation to build the research study. Moreover, effective research design helps establish quality decision-making procedures, structures the research to lead to easier data analysis, and addresses the main research question. Therefore, it is essential to cater undivided attention and time to create an experimental research design before beginning the practical experiment.
By creating a research design, a researcher is also giving oneself time to organize the research, set up relevant boundaries for the study, and increase the reliability of the results. Through all these efforts, one could also avoid inconclusive results. If any part of the research design is flawed, it will reflect on the quality of the results derived.
Types of Experimental Research Designs
Based on the methods used to collect data in experimental studies, the experimental research designs are of three primary types:
1. Pre-experimental Research Design
A research study could conduct pre-experimental research design when a group or many groups are under observation after implementing factors of cause and effect of the research. The pre-experimental design will help researchers understand whether further investigation is necessary for the groups under observation.
Pre-experimental research is of three types —
- One-shot Case Study Research Design
- One-group Pretest-posttest Research Design
- Static-group Comparison
2. True Experimental Research Design
A true experimental research design relies on statistical analysis to prove or disprove a researcher’s hypothesis. It is one of the most accurate forms of research because it provides specific scientific evidence. Furthermore, out of all the types of experimental designs, only a true experimental design can establish a cause-effect relationship within a group. However, in a true experiment, a researcher must satisfy these three factors —
- There is a control group that is not subjected to changes and an experimental group that will experience the changed variables
- A variable that can be manipulated by the researcher
- Random distribution of the variables
This type of experimental research is commonly observed in the physical sciences.
3. Quasi-experimental Research Design
The word “Quasi” means similarity. A quasi-experimental design is similar to a true experimental design. However, the difference between the two is the assignment of the control group. In this research design, an independent variable is manipulated, but the participants of a group are not randomly assigned. This type of research design is used in field settings where random assignment is either irrelevant or not required.
The classification of the research subjects, conditions, or groups determines the type of research design to be used.
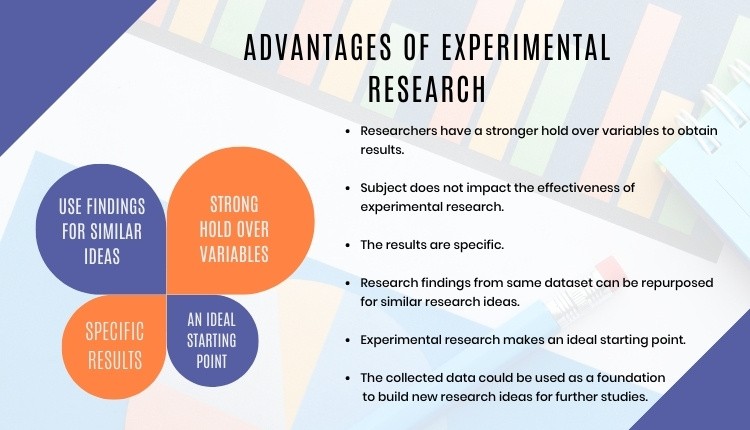
Advantages of Experimental Research
Experimental research allows you to test your idea in a controlled environment before taking the research to clinical trials. Moreover, it provides the best method to test your theory because of the following advantages:
- Researchers have firm control over variables to obtain results.
- The subject does not impact the effectiveness of experimental research. Anyone can implement it for research purposes.
- The results are specific.
- Post results analysis, research findings from the same dataset can be repurposed for similar research ideas.
- Researchers can identify the cause and effect of the hypothesis and further analyze this relationship to determine in-depth ideas.
- Experimental research makes an ideal starting point. The collected data could be used as a foundation to build new research ideas for further studies.
6 Mistakes to Avoid While Designing Your Research
There is no order to this list, and any one of these issues can seriously compromise the quality of your research. You could refer to the list as a checklist of what to avoid while designing your research.
1. Invalid Theoretical Framework
Usually, researchers miss out on checking if their hypothesis is logical to be tested. If your research design does not have basic assumptions or postulates, then it is fundamentally flawed and you need to rework on your research framework.
2. Inadequate Literature Study
Without a comprehensive research literature review , it is difficult to identify and fill the knowledge and information gaps. Furthermore, you need to clearly state how your research will contribute to the research field, either by adding value to the pertinent literature or challenging previous findings and assumptions.
3. Insufficient or Incorrect Statistical Analysis
Statistical results are one of the most trusted scientific evidence. The ultimate goal of a research experiment is to gain valid and sustainable evidence. Therefore, incorrect statistical analysis could affect the quality of any quantitative research.
4. Undefined Research Problem
This is one of the most basic aspects of research design. The research problem statement must be clear and to do that, you must set the framework for the development of research questions that address the core problems.
5. Research Limitations
Every study has some type of limitations . You should anticipate and incorporate those limitations into your conclusion, as well as the basic research design. Include a statement in your manuscript about any perceived limitations, and how you considered them while designing your experiment and drawing the conclusion.
6. Ethical Implications
The most important yet less talked about topic is the ethical issue. Your research design must include ways to minimize any risk for your participants and also address the research problem or question at hand. If you cannot manage the ethical norms along with your research study, your research objectives and validity could be questioned.
Experimental Research Design Example
In an experimental design, a researcher gathers plant samples and then randomly assigns half the samples to photosynthesize in sunlight and the other half to be kept in a dark box without sunlight, while controlling all the other variables (nutrients, water, soil, etc.)
By comparing their outcomes in biochemical tests, the researcher can confirm that the changes in the plants were due to the sunlight and not the other variables.
Experimental research is often the final form of a study conducted in the research process which is considered to provide conclusive and specific results. But it is not meant for every research. It involves a lot of resources, time, and money and is not easy to conduct, unless a foundation of research is built. Yet it is widely used in research institutes and commercial industries, for its most conclusive results in the scientific approach.
Have you worked on research designs? How was your experience creating an experimental design? What difficulties did you face? Do write to us or comment below and share your insights on experimental research designs!
Frequently Asked Questions
Randomization is important in an experimental research because it ensures unbiased results of the experiment. It also measures the cause-effect relationship on a particular group of interest.
Experimental research design lay the foundation of a research and structures the research to establish quality decision making process.
There are 3 types of experimental research designs. These are pre-experimental research design, true experimental research design, and quasi experimental research design.
The difference between an experimental and a quasi-experimental design are: 1. The assignment of the control group in quasi experimental research is non-random, unlike true experimental design, which is randomly assigned. 2. Experimental research group always has a control group; on the other hand, it may not be always present in quasi experimental research.
Experimental research establishes a cause-effect relationship by testing a theory or hypothesis using experimental groups or control variables. In contrast, descriptive research describes a study or a topic by defining the variables under it and answering the questions related to the same.

good and valuable
Very very good
Good presentation.
Rate this article Cancel Reply
Your email address will not be published.
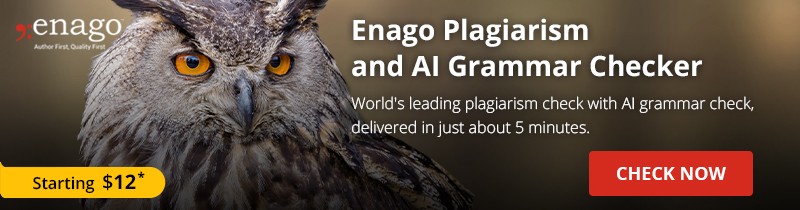
Enago Academy's Most Popular Articles
![examples of hypothesis in experimental research What is Academic Integrity and How to Uphold it [FREE CHECKLIST]](https://www.enago.com/academy/wp-content/uploads/2024/05/FeatureImages-59-210x136.png)
Ensuring Academic Integrity and Transparency in Academic Research: A comprehensive checklist for researchers
Academic integrity is the foundation upon which the credibility and value of scientific findings are…
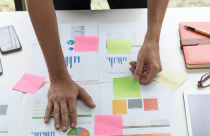
- Publishing Research
- Reporting Research
How to Optimize Your Research Process: A step-by-step guide
For researchers across disciplines, the path to uncovering novel findings and insights is often filled…
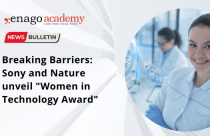
- Industry News
- Trending Now
Breaking Barriers: Sony and Nature unveil “Women in Technology Award”
Sony Group Corporation and the prestigious scientific journal Nature have collaborated to launch the inaugural…
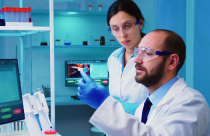
Achieving Research Excellence: Checklist for good research practices
Academia is built on the foundation of trustworthy and high-quality research, supported by the pillars…
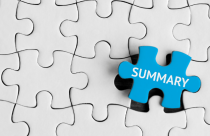
- Promoting Research
Plain Language Summary — Communicating your research to bridge the academic-lay gap
Science can be complex, but does that mean it should not be accessible to the…
Choosing the Right Analytical Approach: Thematic analysis vs. content analysis for…
Comparing Cross Sectional and Longitudinal Studies: 5 steps for choosing the right…
Research Recommendations – Guiding policy-makers for evidence-based decision making

Sign-up to read more
Subscribe for free to get unrestricted access to all our resources on research writing and academic publishing including:
- 2000+ blog articles
- 50+ Webinars
- 10+ Expert podcasts
- 50+ Infographics
- 10+ Checklists
- Research Guides
We hate spam too. We promise to protect your privacy and never spam you.
I am looking for Editing/ Proofreading services for my manuscript Tentative date of next journal submission:
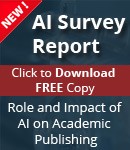
As a researcher, what do you consider most when choosing an image manipulation detector?
- Privacy Policy

Home » Experimental Design – Types, Methods, Guide
Experimental Design – Types, Methods, Guide
Table of Contents

Experimental Design
Experimental design is a process of planning and conducting scientific experiments to investigate a hypothesis or research question. It involves carefully designing an experiment that can test the hypothesis, and controlling for other variables that may influence the results.
Experimental design typically includes identifying the variables that will be manipulated or measured, defining the sample or population to be studied, selecting an appropriate method of sampling, choosing a method for data collection and analysis, and determining the appropriate statistical tests to use.
Types of Experimental Design
Here are the different types of experimental design:
Completely Randomized Design
In this design, participants are randomly assigned to one of two or more groups, and each group is exposed to a different treatment or condition.
Randomized Block Design
This design involves dividing participants into blocks based on a specific characteristic, such as age or gender, and then randomly assigning participants within each block to one of two or more treatment groups.
Factorial Design
In a factorial design, participants are randomly assigned to one of several groups, each of which receives a different combination of two or more independent variables.
Repeated Measures Design
In this design, each participant is exposed to all of the different treatments or conditions, either in a random order or in a predetermined order.
Crossover Design
This design involves randomly assigning participants to one of two or more treatment groups, with each group receiving one treatment during the first phase of the study and then switching to a different treatment during the second phase.
Split-plot Design
In this design, the researcher manipulates one or more variables at different levels and uses a randomized block design to control for other variables.
Nested Design
This design involves grouping participants within larger units, such as schools or households, and then randomly assigning these units to different treatment groups.
Laboratory Experiment
Laboratory experiments are conducted under controlled conditions, which allows for greater precision and accuracy. However, because laboratory conditions are not always representative of real-world conditions, the results of these experiments may not be generalizable to the population at large.
Field Experiment
Field experiments are conducted in naturalistic settings and allow for more realistic observations. However, because field experiments are not as controlled as laboratory experiments, they may be subject to more sources of error.
Experimental Design Methods
Experimental design methods refer to the techniques and procedures used to design and conduct experiments in scientific research. Here are some common experimental design methods:
Randomization
This involves randomly assigning participants to different groups or treatments to ensure that any observed differences between groups are due to the treatment and not to other factors.
Control Group
The use of a control group is an important experimental design method that involves having a group of participants that do not receive the treatment or intervention being studied. The control group is used as a baseline to compare the effects of the treatment group.
Blinding involves keeping participants, researchers, or both unaware of which treatment group participants are in, in order to reduce the risk of bias in the results.
Counterbalancing
This involves systematically varying the order in which participants receive treatments or interventions in order to control for order effects.
Replication
Replication involves conducting the same experiment with different samples or under different conditions to increase the reliability and validity of the results.
This experimental design method involves manipulating multiple independent variables simultaneously to investigate their combined effects on the dependent variable.
This involves dividing participants into subgroups or blocks based on specific characteristics, such as age or gender, in order to reduce the risk of confounding variables.
Data Collection Method
Experimental design data collection methods are techniques and procedures used to collect data in experimental research. Here are some common experimental design data collection methods:
Direct Observation
This method involves observing and recording the behavior or phenomenon of interest in real time. It may involve the use of structured or unstructured observation, and may be conducted in a laboratory or naturalistic setting.
Self-report Measures
Self-report measures involve asking participants to report their thoughts, feelings, or behaviors using questionnaires, surveys, or interviews. These measures may be administered in person or online.
Behavioral Measures
Behavioral measures involve measuring participants’ behavior directly, such as through reaction time tasks or performance tests. These measures may be administered using specialized equipment or software.
Physiological Measures
Physiological measures involve measuring participants’ physiological responses, such as heart rate, blood pressure, or brain activity, using specialized equipment. These measures may be invasive or non-invasive, and may be administered in a laboratory or clinical setting.
Archival Data
Archival data involves using existing records or data, such as medical records, administrative records, or historical documents, as a source of information. These data may be collected from public or private sources.
Computerized Measures
Computerized measures involve using software or computer programs to collect data on participants’ behavior or responses. These measures may include reaction time tasks, cognitive tests, or other types of computer-based assessments.
Video Recording
Video recording involves recording participants’ behavior or interactions using cameras or other recording equipment. This method can be used to capture detailed information about participants’ behavior or to analyze social interactions.
Data Analysis Method
Experimental design data analysis methods refer to the statistical techniques and procedures used to analyze data collected in experimental research. Here are some common experimental design data analysis methods:
Descriptive Statistics
Descriptive statistics are used to summarize and describe the data collected in the study. This includes measures such as mean, median, mode, range, and standard deviation.
Inferential Statistics
Inferential statistics are used to make inferences or generalizations about a larger population based on the data collected in the study. This includes hypothesis testing and estimation.
Analysis of Variance (ANOVA)
ANOVA is a statistical technique used to compare means across two or more groups in order to determine whether there are significant differences between the groups. There are several types of ANOVA, including one-way ANOVA, two-way ANOVA, and repeated measures ANOVA.
Regression Analysis
Regression analysis is used to model the relationship between two or more variables in order to determine the strength and direction of the relationship. There are several types of regression analysis, including linear regression, logistic regression, and multiple regression.
Factor Analysis
Factor analysis is used to identify underlying factors or dimensions in a set of variables. This can be used to reduce the complexity of the data and identify patterns in the data.
Structural Equation Modeling (SEM)
SEM is a statistical technique used to model complex relationships between variables. It can be used to test complex theories and models of causality.
Cluster Analysis
Cluster analysis is used to group similar cases or observations together based on similarities or differences in their characteristics.
Time Series Analysis
Time series analysis is used to analyze data collected over time in order to identify trends, patterns, or changes in the data.
Multilevel Modeling
Multilevel modeling is used to analyze data that is nested within multiple levels, such as students nested within schools or employees nested within companies.
Applications of Experimental Design
Experimental design is a versatile research methodology that can be applied in many fields. Here are some applications of experimental design:
- Medical Research: Experimental design is commonly used to test new treatments or medications for various medical conditions. This includes clinical trials to evaluate the safety and effectiveness of new drugs or medical devices.
- Agriculture : Experimental design is used to test new crop varieties, fertilizers, and other agricultural practices. This includes randomized field trials to evaluate the effects of different treatments on crop yield, quality, and pest resistance.
- Environmental science: Experimental design is used to study the effects of environmental factors, such as pollution or climate change, on ecosystems and wildlife. This includes controlled experiments to study the effects of pollutants on plant growth or animal behavior.
- Psychology : Experimental design is used to study human behavior and cognitive processes. This includes experiments to test the effects of different interventions, such as therapy or medication, on mental health outcomes.
- Engineering : Experimental design is used to test new materials, designs, and manufacturing processes in engineering applications. This includes laboratory experiments to test the strength and durability of new materials, or field experiments to test the performance of new technologies.
- Education : Experimental design is used to evaluate the effectiveness of teaching methods, educational interventions, and programs. This includes randomized controlled trials to compare different teaching methods or evaluate the impact of educational programs on student outcomes.
- Marketing : Experimental design is used to test the effectiveness of marketing campaigns, pricing strategies, and product designs. This includes experiments to test the impact of different marketing messages or pricing schemes on consumer behavior.
Examples of Experimental Design
Here are some examples of experimental design in different fields:
- Example in Medical research : A study that investigates the effectiveness of a new drug treatment for a particular condition. Patients are randomly assigned to either a treatment group or a control group, with the treatment group receiving the new drug and the control group receiving a placebo. The outcomes, such as improvement in symptoms or side effects, are measured and compared between the two groups.
- Example in Education research: A study that examines the impact of a new teaching method on student learning outcomes. Students are randomly assigned to either a group that receives the new teaching method or a group that receives the traditional teaching method. Student achievement is measured before and after the intervention, and the results are compared between the two groups.
- Example in Environmental science: A study that tests the effectiveness of a new method for reducing pollution in a river. Two sections of the river are selected, with one section treated with the new method and the other section left untreated. The water quality is measured before and after the intervention, and the results are compared between the two sections.
- Example in Marketing research: A study that investigates the impact of a new advertising campaign on consumer behavior. Participants are randomly assigned to either a group that is exposed to the new campaign or a group that is not. Their behavior, such as purchasing or product awareness, is measured and compared between the two groups.
- Example in Social psychology: A study that examines the effect of a new social intervention on reducing prejudice towards a marginalized group. Participants are randomly assigned to either a group that receives the intervention or a control group that does not. Their attitudes and behavior towards the marginalized group are measured before and after the intervention, and the results are compared between the two groups.
When to use Experimental Research Design
Experimental research design should be used when a researcher wants to establish a cause-and-effect relationship between variables. It is particularly useful when studying the impact of an intervention or treatment on a particular outcome.
Here are some situations where experimental research design may be appropriate:
- When studying the effects of a new drug or medical treatment: Experimental research design is commonly used in medical research to test the effectiveness and safety of new drugs or medical treatments. By randomly assigning patients to treatment and control groups, researchers can determine whether the treatment is effective in improving health outcomes.
- When evaluating the effectiveness of an educational intervention: An experimental research design can be used to evaluate the impact of a new teaching method or educational program on student learning outcomes. By randomly assigning students to treatment and control groups, researchers can determine whether the intervention is effective in improving academic performance.
- When testing the effectiveness of a marketing campaign: An experimental research design can be used to test the effectiveness of different marketing messages or strategies. By randomly assigning participants to treatment and control groups, researchers can determine whether the marketing campaign is effective in changing consumer behavior.
- When studying the effects of an environmental intervention: Experimental research design can be used to study the impact of environmental interventions, such as pollution reduction programs or conservation efforts. By randomly assigning locations or areas to treatment and control groups, researchers can determine whether the intervention is effective in improving environmental outcomes.
- When testing the effects of a new technology: An experimental research design can be used to test the effectiveness and safety of new technologies or engineering designs. By randomly assigning participants or locations to treatment and control groups, researchers can determine whether the new technology is effective in achieving its intended purpose.
How to Conduct Experimental Research
Here are the steps to conduct Experimental Research:
- Identify a Research Question : Start by identifying a research question that you want to answer through the experiment. The question should be clear, specific, and testable.
- Develop a Hypothesis: Based on your research question, develop a hypothesis that predicts the relationship between the independent and dependent variables. The hypothesis should be clear and testable.
- Design the Experiment : Determine the type of experimental design you will use, such as a between-subjects design or a within-subjects design. Also, decide on the experimental conditions, such as the number of independent variables, the levels of the independent variable, and the dependent variable to be measured.
- Select Participants: Select the participants who will take part in the experiment. They should be representative of the population you are interested in studying.
- Randomly Assign Participants to Groups: If you are using a between-subjects design, randomly assign participants to groups to control for individual differences.
- Conduct the Experiment : Conduct the experiment by manipulating the independent variable(s) and measuring the dependent variable(s) across the different conditions.
- Analyze the Data: Analyze the data using appropriate statistical methods to determine if there is a significant effect of the independent variable(s) on the dependent variable(s).
- Draw Conclusions: Based on the data analysis, draw conclusions about the relationship between the independent and dependent variables. If the results support the hypothesis, then it is accepted. If the results do not support the hypothesis, then it is rejected.
- Communicate the Results: Finally, communicate the results of the experiment through a research report or presentation. Include the purpose of the study, the methods used, the results obtained, and the conclusions drawn.
Purpose of Experimental Design
The purpose of experimental design is to control and manipulate one or more independent variables to determine their effect on a dependent variable. Experimental design allows researchers to systematically investigate causal relationships between variables, and to establish cause-and-effect relationships between the independent and dependent variables. Through experimental design, researchers can test hypotheses and make inferences about the population from which the sample was drawn.
Experimental design provides a structured approach to designing and conducting experiments, ensuring that the results are reliable and valid. By carefully controlling for extraneous variables that may affect the outcome of the study, experimental design allows researchers to isolate the effect of the independent variable(s) on the dependent variable(s), and to minimize the influence of other factors that may confound the results.
Experimental design also allows researchers to generalize their findings to the larger population from which the sample was drawn. By randomly selecting participants and using statistical techniques to analyze the data, researchers can make inferences about the larger population with a high degree of confidence.
Overall, the purpose of experimental design is to provide a rigorous, systematic, and scientific method for testing hypotheses and establishing cause-and-effect relationships between variables. Experimental design is a powerful tool for advancing scientific knowledge and informing evidence-based practice in various fields, including psychology, biology, medicine, engineering, and social sciences.
Advantages of Experimental Design
Experimental design offers several advantages in research. Here are some of the main advantages:
- Control over extraneous variables: Experimental design allows researchers to control for extraneous variables that may affect the outcome of the study. By manipulating the independent variable and holding all other variables constant, researchers can isolate the effect of the independent variable on the dependent variable.
- Establishing causality: Experimental design allows researchers to establish causality by manipulating the independent variable and observing its effect on the dependent variable. This allows researchers to determine whether changes in the independent variable cause changes in the dependent variable.
- Replication : Experimental design allows researchers to replicate their experiments to ensure that the findings are consistent and reliable. Replication is important for establishing the validity and generalizability of the findings.
- Random assignment: Experimental design often involves randomly assigning participants to conditions. This helps to ensure that individual differences between participants are evenly distributed across conditions, which increases the internal validity of the study.
- Precision : Experimental design allows researchers to measure variables with precision, which can increase the accuracy and reliability of the data.
- Generalizability : If the study is well-designed, experimental design can increase the generalizability of the findings. By controlling for extraneous variables and using random assignment, researchers can increase the likelihood that the findings will apply to other populations and contexts.
Limitations of Experimental Design
Experimental design has some limitations that researchers should be aware of. Here are some of the main limitations:
- Artificiality : Experimental design often involves creating artificial situations that may not reflect real-world situations. This can limit the external validity of the findings, or the extent to which the findings can be generalized to real-world settings.
- Ethical concerns: Some experimental designs may raise ethical concerns, particularly if they involve manipulating variables that could cause harm to participants or if they involve deception.
- Participant bias : Participants in experimental studies may modify their behavior in response to the experiment, which can lead to participant bias.
- Limited generalizability: The conditions of the experiment may not reflect the complexities of real-world situations. As a result, the findings may not be applicable to all populations and contexts.
- Cost and time : Experimental design can be expensive and time-consuming, particularly if the experiment requires specialized equipment or if the sample size is large.
- Researcher bias : Researchers may unintentionally bias the results of the experiment if they have expectations or preferences for certain outcomes.
- Lack of feasibility : Experimental design may not be feasible in some cases, particularly if the research question involves variables that cannot be manipulated or controlled.
About the author
Muhammad Hassan
Researcher, Academic Writer, Web developer
You may also like

Questionnaire – Definition, Types, and Examples

Case Study – Methods, Examples and Guide

Observational Research – Methods and Guide

Quantitative Research – Methods, Types and...

Qualitative Research Methods

Explanatory Research – Types, Methods, Guide
Thank you for visiting nature.com. You are using a browser version with limited support for CSS. To obtain the best experience, we recommend you use a more up to date browser (or turn off compatibility mode in Internet Explorer). In the meantime, to ensure continued support, we are displaying the site without styles and JavaScript.
- View all journals
- My Account Login
- Explore content
- About the journal
- Publish with us
- Sign up for alerts
- Open access
- Published: 27 May 2024
Biodiversity increases resistance of grasslands against plant invasions under multiple environmental changes
- Cai Cheng ORCID: orcid.org/0000-0002-7979-7790 1 , 2 ,
- Zekang Liu ORCID: orcid.org/0000-0003-1391-6352 2 ,
- Wei Song 2 ,
- Xue Chen 2 ,
- Zhijie Zhang ORCID: orcid.org/0000-0003-0463-2665 3 ,
- Bo Li ORCID: orcid.org/0000-0002-0439-5666 4 ,
- Mark van Kleunen ORCID: orcid.org/0000-0002-2861-3701 3 , 5 &
- Jihua Wu ORCID: orcid.org/0000-0001-8623-8519 1
Nature Communications volume 15 , Article number: 4506 ( 2024 ) Cite this article
Metrics details
- Biodiversity
- Climate-change ecology
- Invasive species
Biodiversity often helps communities resist invasion. However, it is unclear whether this diversity–invasion relationship holds true under environmental changes. Here, we conduct a meta-analysis of 1010 observations from 25 grassland studies in which plant species richness is manipulated together with one or more environmental change factors to test invasibility (measured by biomass or cover of invaders). We find that biodiversity increases resistance to invaders across various environmental conditions. However, the positive biodiversity effect on invasion resistance is strengthened under experimental warming, whereas it is weakened under experimentally imposed drought. When multiple factors are imposed simultaneously, the positive biodiversity effect is strengthened. Overall, we show that biodiversity helps grassland communities resist plant invasions under multiple environmental changes. Therefore, investment in the protection and restoration of native biodiversity is not only important for prevention of invasions under current conditions but also under continued global environmental change.
Similar content being viewed by others
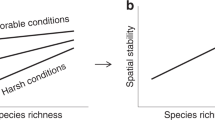
Global evidence of positive biodiversity effects on spatial ecosystem stability in natural grasslands
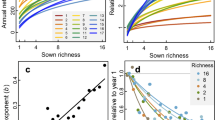
Biodiversity–stability relationships strengthen over time in a long-term grassland experiment
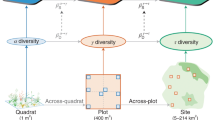
Consistent stabilizing effects of plant diversity across spatial scales and climatic gradients
Introduction.
The Anthropocene has seen a rapid increase in invasions by alien species as well as by range-expanding native species 1 , 2 , 3 . Such invasions may pose a major threat to biodiversity, the economy, and human well-being 4 , 5 . There are many factors that affect the likelihood of species invasions, including background climatic conditions, the magnitude and type of anthropogenic environmental change, and biotic features of the community (e.g. the types and diversity of native species), all of which can interact 6 , 7 . Among the many hypotheses in invasion biology addressing these factors 8 , much attention has been paid to the biotic resistance hypothesis 9 , 10 , which predicts that more diverse communities should be more resistant to species invasions.
Empirical support for the biotic resistance hypothesis has been mixed. While some large-scale observational studies show compelling evidence for negative relationships between native diversity and invasion 7 , 11 , these observational studies have limited ability to infer causality. This is because both native residents and invaders respond to variation in the environment and to each other 12 , 13 . Indeed, across larger spatial focal units (i.e. regions), there are often positive correlations between native and alien species richness because both groups of species respond in similar ways to the environmental conditions in the regions 14 , 15 , 16 , even if there are negative relationships at smaller spatial scales 12 , 17 . Given the limited causal inference of observational studies, the most definitive way to examine the relationship between diversity and invasion is through experiments that manipulate the diversity (e.g. species richness) of the resident community and measure its resistance to invasion (e.g. biomass or cover of invaders) 18 . Indeed, many such experiments corroborate the positive relationship between diversity and invasion resistance 19 , 20 , 21 , 22 . However, there is considerable variability in the strength of the relationship 23 , 24 , and a number of exceptions also occur 25 , 26 . Likely, this variation in the strength of the relationship between diversity and invasion resistance is caused by variations in environmental conditions 27 , 28 , 29 .
Earth’s ecosystems are exposed to numerous environmental change factors 30 , such as climate change, eutrophication, overgrazing and pesticide use, all of which can have profound consequences for resident biota and invaders. According to the stress-gradient hypothesis, species interactions could switch from strong competition in favorable environments to weak competition or even facilitation in stressful environments 28 , 31 . Therefore, we might expect a stronger biodiversity effect on invasion resistance when the communities face stressful factors (i.e. impairing the overall performance of plants) as these should enhance positive interactions between native species. In contrast, the biodiversity effect would be weakened by favorable factors (i.e. benefiting the overall performance of plants). In addition to the effect of environmental change factors on interactions between native species, the relationship between diversity and invasion resistance could also be influenced by different responses of alien and native species to environmental change factors 32 . However, it remains unclear whether and how the different environmental change factors affect the relationship between diversity and invasion resistance.
In addition to the type of environmental change factors, the number of simultaneously acting factors may also influence the relationship between diversity and invasion resistance. While different factors can additively influence the relationship between diversity and invasion resistance 33 , they could also act synergistically or antagonistically 34 , 35 . Even though the joint effects of multiple factors on either resident biota or invaders have been reported 36 , 37 , we still lack information about how the relationship between diversity and invasion resistance responds to multiple simultaneously acting factors. This gap may result from the complex and large experimental designs that are needed when multiple levels of biodiversity are crossed with numerous environmental change factors. However, a recent study reported that increasing the number of simultaneously acting factors caused increasingly stressful environments 38 , suggesting that there might be a stronger biodiversity effect on invasion resistance in the face of multiple simultaneous factors. Knowledge about the effect of multiple factors on the relationship between diversity and invasion resistance is necessary to boost our confidence that promoting native biodiversity in order to reduce invasions is a viable option under realistic global change scenarios.
Typically, biodiversity helps resist invasion primarily through enhanced competitive suppression (e.g. due to higher productivity) of resident species on invaders, mainly via complementarity and selection effects 20 . Complementarity effects occur when more diverse communities have more species that more fully occupy the available niche space, thereby pre-empting opportunities for invaders. Selection effects occur when more diverse communities have a higher probability of containing species that have greater competitive ability against invaders. As such, the biodiversity effect on invasion resistance will be influenced by multiple experimental factors. For example, a greater number of resident plant species, a longer duration of the experiment and smaller experimental units should result in stronger complementarity effects, and should reduce the niche space available for invaders 39 . Furthermore, the biodiversity effect on invasion resistance could also be influenced by the type of invaders. Several biodiversity experiments refer to invaders as any species that has not been planted in a given experimental unit 40 . Among these invaders, species that are residents in other experimental units (i.e. internal invaders) should, due to a priority effect, be more likely to invade than novel external invaders —particular alien ones— that are not part of the experiment’s resident species pool.
Here, we conduct a meta-analysis on 1010 observations from 25 grassland studies in which plant species richness is experimentally manipulated together with one or more environmental change factors. These factors include warming, drought, elevated atmospheric CO 2 , eutrophication, pesticide use, grazing by domestic animals, human-caused fire, physical disturbance, and combinations of two or three of these factors. Our main objective is to assess whether and how the type and number of environmental change factors affect the biodiversity effect on invasion resistance. We measure invasion resistance of the resident community by the performance (biomass or percent cover) of all invaders of an experimental unit. However, when possible, we also distinguish for each experimental unit between internal invaders and external invaders. For the latter, we also distinguish between native and alien invaders (non-native to the experiment site). We here hypothesize that: (1) plant diversity increases the resistance of grasslands against invaders, with the strongest resistance to alien external invaders, (2) the biodiversity effect on invasion resistance is positively correlated with the effect on resident productivity and becomes stronger with increasing resident species richness and experimental duration, and with smaller sizes of the experimental units, (3) the biodiversity effect on invasion resistance is strengthened by stressful factors (e.g. drought, grazing and fire) but weakened by favorable factors (e.g. warming, elevated atmospheric CO 2 and eutrophication), and (4) the biodiversity effect on invasion resistance is strengthened by multiple simultaneous factors. By testing these hypotheses, our study provides evidence that plant diversity increases the resistance of grasslands against plant invasions. This is also the case under environmental changes, although the magnitude of the positive biodiversity effect increases or decreases, depending on the type and number of environmental change factors.
Averaged across all studies, we found a significantly positive effect of biodiversity on invasion resistance, both under ambient conditions and in the presence of environmental change factors (Fig. 1a ; Supplementary Fig. 1a ). The positive biodiversity effect on invasion resistance was strengthened by warming ( Q M = 8.77, p = 0.003) and weakened by drought ( Q M = 7.06, p = 0.008), but was not significantly affected by the other factors (Fig. 1a ). This was also reflected by significant effect sizes of ∆NBE —difference in the net biodiversity effect (NBE) between manipulated and ambient conditions— under warming (mean = 0.82, 95% CI = [0.05, 1.59]) and drought (mean = –0.50, 95% CI = [–0.77, –0.22]) (Fig. 1b ). Although most of the other factors individually did not alter the positive biodiversity effect on invasion resistance, it was strengthened when multiple factors were imposed simultaneously (two co-acting factors: mean ∆NBE = 0.16, 95% CI = [0.05, 0.27]; three co-acting factors: mean ∆NBE = 0.64, 95% CI = [0.37, 0.92]) (Fig. 1b ).
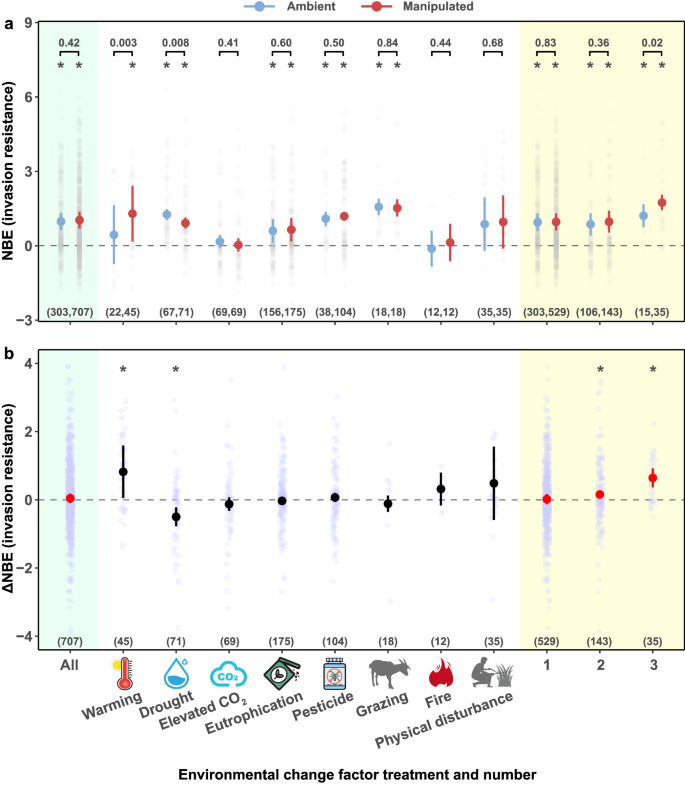
The net biodiversity effect (NBE) on invasion resistance ( a ) and the difference in NBE between ambient and manipulated environmental conditions (∆NBE) ( b ). Positive values of NBE indicate higher invasion resistance of resident mixtures in comparison with that of resident monocultures, whereas negative values indicate the opposite. Positive values of ∆NBE indicate stronger biodiversity effects under manipulated environmental conditions in comparison with ambient conditions, and vice versa. In panel a, the numbers above the brackets are the p -values of the Q M tests for the effect of environmental manipulation (ambient vs. manipulated) on NBE. The numbers in brackets show the number of effect sizes. Points with error bars are the estimated means with corrected 95% confidence intervals. Confidence intervals not overlapping with the dashed line (i.e. 0) indicate statistical significance, as indicated by asterisks. Green shading indicates the analysis on all environmental change factors and yellow shading indicates the analysis on different numbers of factors. Symbols of environmental change factors are created by Yue Chen.
The strengthened biodiversity effect on invasion resistance under warming conditions was also indicated by the finding that warming had a negative effect on invasion resistance of monocultures but not on invasion resistance of mixtures (Supplementary Figs. 2 , 3a ). Similarly, the weakened biodiversity effect on invasion resistance under drought conditions was consistent with the finding of a positive effect of drought on invasion resistance of monocultures and the absence of such an effect in mixtures (Supplementary Figs. 2 , 3b ). While eutrophication did not alter the biodiversity effect on invasion resistance, it decreased the invasion resistance of both monocultures and mixtures (Supplementary Figs. 2 , 3c ). In addition, while three co-acting factors increased invasion resistance, the effect was stronger for mixtures than monocultures (Supplementary Figs. 2 , 3d ), which was consistent with the strengthened biodiversity effect on invasion resistance when there were three co-acting factors.
For the subset of studies in which we could distinguish between internal invaders and external invaders (either native or alien), we found that the positive effect of biodiversity on invasion resistance was strongest for external aliens (Supplementary Fig. 4 ; Supplementary Table 1 ). However, we found no significant interaction between environmental manipulation and invader type (Supplementary Table 1 ), suggesting that the biodiversity effect on the resistance to internal and external invaders was consistent across environmental conditions.
We found that the biodiversity effect on invasion resistance was positively associated with the effect on resident productivity across various environmental conditions (Fig. 2 ). Moreover, the biodiversity effect on invasion resistance increased with the number of resident species in the mixture (Fig. 3 ), but had overall weak relationships with experimental duration and experimental unit size when the different factors were analyzed (Supplementary Figs. 5 – 7 ).
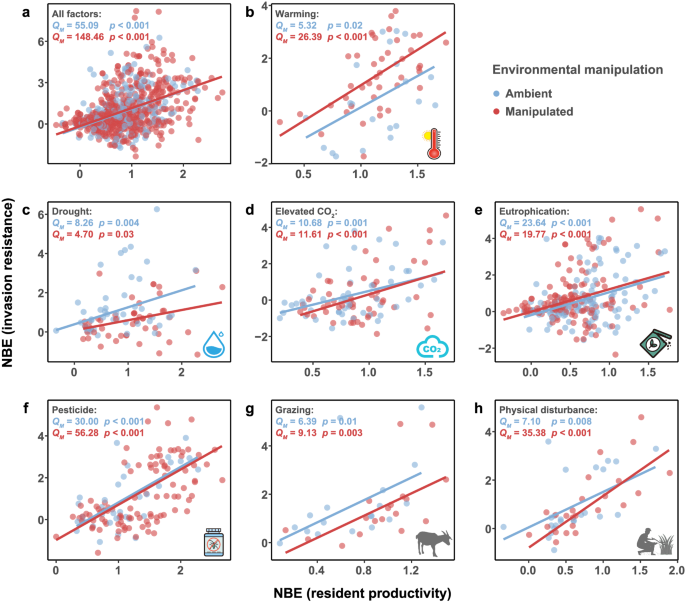
Relationships were tested using the Q M tests for datasets of all environmental change factors ( a ), warming ( b ), drought ( c ), elevated CO 2 ( d ), eutrophication ( e ), pesticide ( f ), grazing ( g ) and physical disturbance ( h ). Positive values of NBE indicate higher invasion resistance or productivity of resident mixtures in comparison to resident monocultures, whereas negative values indicate the opposite. Blue indicates the ambient condition and red indicates the manipulated environmental condition. Symbols of environmental change factors are created by Yue Chen.
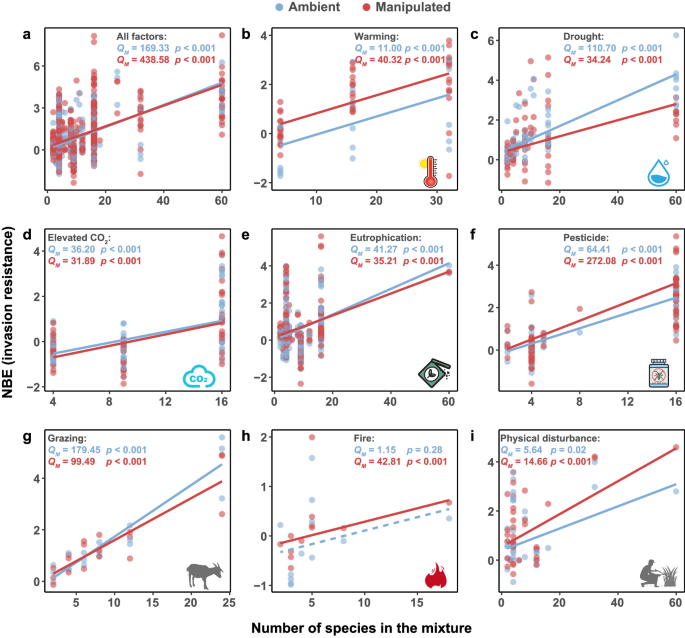
Relationships were tested using the Q M tests for datasets of all environmental change factors ( a ), warming ( b ), drought ( c ), elevated CO 2 ( d ), eutrophication ( e ), pesticide ( f ), grazing ( g ), fire ( h ) and physical disturbance ( i ). Positive values of NBE indicate higher invasion resistance of resident mixtures in comparison to resident monocultures, whereas negative values indicate the opposite. Blue indicates the ambient condition and red indicates the manipulated environmental condition. Symbols of environmental change factors are created by Yue Chen.
Several environmental change factors had significant net effects on the productivity of resident monocultures (Fig. 4a ). Specifically, monoculture productivity was increased on average by warming and eutrophication, indicating that these were favorable environmental conditions, but decreased by grazing and three co-acting factors, indicating that these were stressful environmental conditions. Across environmental change factors, invasion-resistance ∆NBE increased when factors caused stressful environmental conditions ( Q M = 6.29, p = 0.01; Fig. 4b ).
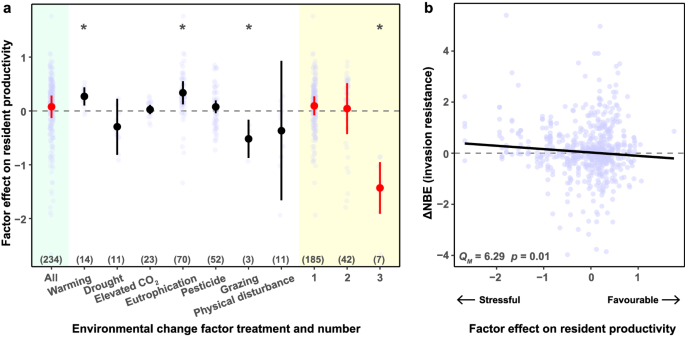
In panel a , positive values of the factor effect on resident productivity indicate that environmental change factors increase the productivity of resident monocultures and thus provide a favorable condition, and negative values indicate that environmental change factors decrease the productivity of resident monocultures and thus provide a stressful condition. The numbers in brackets show the number of effect sizes. Points with error bars are the estimated means with corrected 95% confidence intervals. Confidence intervals not overlapping with the dashed line (i.e. 0) indicate statistical significance, as indicated by asterisks. Green shading indicates the analysis on all environmental change factors and yellow shading indicates the analysis on different numbers of factors. In panel b , the relationship between ∆NBE and the factor effect on resident productivity was tested using the Q M test.
While the positive relationship between diversity and invasion resistance was proposed more than 60 years ago 9 , and has been well corroborated by experimental studies in grassland systems 20 , 21 , 22 , evidence for neutral and even negative relationships has also been reported 25 , 26 . Our meta-analysis of 25 factorial grassland experiments showed that these seemingly conflicting patterns can, at least partially, be explained by the dependence of the relationship between diversity and invasion resistance on environmental conditions. Specifically, while our results generally supported the hypothesis that plant diversity promotes invasion resistance of grassland communities, we found that the type and number of environmental change factors could modulate the strength of the positive biodiversity effect on invasion resistance.
We found that across all environmental change factors, invasion-resistance ΔNBE increased when factors caused stressful environments, which is in line with the stress-gradient hypothesis that predicts stronger biodiversity effects in more stressful environments 28 , 31 . However, there were exceptions for particular factors. For example, although warming resulted in a favorable environment, it strengthened the biodiversity effect on invasion resistance. As there was no significant influence of warming on the biodiversity effect on resident productivity (Supplementary Fig. 8 ), this result may be because alien plant species benefit more from elevated temperatures than native plant species 32 , thereby decreasing invasion resistance of monocultures under warming conditions (Supplementary Fig. 2a ). A recent study also showed that plant diversity buffered elevated temperature in grasslands 41 , which could reduce the positive impact of warming on invaders and resulted in a strengthened biodiversity effect on invasion resistance under warming conditions (Supplementary Fig. 3a ). Drought, on the other hand, resulted in a stressful environment, but nevertheless weakened the biodiversity effect on invasion resistance. However, our finding that drought strengthened the biodiversity effect on resident productivity is consistent with the prediction of the stress-gradient hypothesis (Supplementary Fig. 8 ). This discrepancy may be because alien plant species suffered more from drought than native plant species 32 , thereby increasing invasion resistance of monocultures under drought conditions (Supplementary Fig. 2a ). In contrast, diverse plant communities have denser canopies that reduce solar radiation at the soil level and thereby reduce evaporation 42 , 43 . This could buffer the negative impact of drought on invaders and result in a weakened biodiversity effect on invasion resistance under drought conditions (Supplementary Fig. 3b ). Taken together, our results suggest that warming and drought altered the biodiversity effect on invasion resistance through changes in invasion resistance of monocultures, which aligns with previous studies reporting that biodiversity contributes to the stability of ecosystem functions in grassland systems 44 , 45 .
Most studies on the consequences of environmental change factors for biodiversity effects have focused on single factors 39 , 46 . Here, however, we were able to examine the joint effects of co-acting factors and found that the positive biodiversity effect on invasion resistance became stronger as the number of factors increased. This result is consistent with a recent meta-analysis reporting that plant communities were more likely to be altered when facing at least three global change factors simultaneously 47 . Our finding that three co-acting factors strengthened the biodiversity effect on invasion resistance is also consistent with the prediction of the stress-gradient hypothesis. While each of the individual factors resulted in either stressful, favorable or unaltered environments, three co-acting factors caused a stressful environment (Fig. 4a ). This aligns with a recent study reporting that synergistic interactions between co-acting factors significantly decreased the performance of a herbaceous plant (i.e. resulted in a stressful environment) 38 . Although three co-acting factors increased the invasion resistance of both monocultures and mixtures, they had larger impacts on the invasion resistance of mixtures than of monocultures (Supplementary Figs. 2 , 3d ). This suggests that environmental change factors acted synergistically and increased complementarity effects in mixtures 48 , 49 , which increased resistance against invasion.
Our finding that biodiversity effects on invasion resistance and resident productivity were positively associated suggests that plant diversity effects on resident productivity —and the associated greater competitive ability— may be a mechanism by which resident communities resist invasion. This is also supported by the results from a number of individual studies in grassland communities 20 , 24 , 39 . However, as it has been found that biodiversity effects frequently increase over time, primarily through an increase of complementary effects 50 , 51 , we surprisingly found only weak relationships between the biodiversity effect on invasion resistance and experimental duration. Given the larger maximum experimental duration in our meta-analysis (~24 years) compared to other grassland (~15 years) 50 and forest (~8 years) 51 studies, this discrepancy is likely explained by the negligible role of complementarity effects in our study. This is indicated by the fact that there was little transgressive resistance (an indicator of complementarity effects) of biodiversity to invasion (Supplementary Fig. 9 ), suggesting that the observed biodiversity effect was primarily due to selection effects. This result aligns with previous meta-analyses demonstrating that in most experiments, the most diverse communities did not achieve greater biomass than the single most productive species 52 , 53 .
Our findings may have implications for grassland management aimed at reducing plant invasions under continued global environmental change. First, our result that mixtures, in contrast to monocultures, did not experience a negative effect of warming on invasion resistance (i.e. resulting in a stronger biodiversity effect on invasion resistance), suggests that biodiversity has a buffering effect. This implies that maintaining and enhancing native plant diversity should be a priority to prevent invasion by alien species in an increasingly warmer world. Although drought had positive effects on invasion resistance of monocultures, this was not the case for mixtures. Nevertheless, the relationship between biodiversity and invasion resistance was still positive under drought, indicating that biodiversity is also important under drought. While eutrophication did not alter the biodiversity effect on invasion resistance, its negative impacts on invasion resistance, irrespective of the diversity of resident communities, suggest that grassland managers should reduce the use of fertilizer that may promote plant invasions. Furthermore, our result that plant diversity strengthened the positive effect of three co-acting factors on invasion resistance (i.e. had a stronger biodiversity effect on invasion resistance), suggests that enhancing plant diversity should be prioritized to increase resistance of grasslands against invasion in a changing world in which plant communities may be exposed to multiple factors simultaneously.
Our meta-analysis has several caveats. First, like many meta-analyses, we found evidence for publication bias in our dataset (Supplementary Fig. 10 ), likely because studies with low precision that found a negative relationship between diversity and invasion resistance —which contradicts the expected positive relationship— are difficult to publish. Nevertheless, because our study was primarily focused on how environmental change factors modulate the strength of biodiversity effects, this bias should not influence the main conclusions drawn from our study. Indeed, our sensitivity analysis indicated that the publication bias was unlikely to influence the robustness of our conclusions (Supplementary Fig. 11 ). Second, our main finding that biodiversity consistently increased invasion resistance under environmental change factors is based on the performance of all invaders. Despite this, our results could also have implications for biodiversity conservation in an increasingly invaded world, because our subset analysis showed that alien invaders were the most strongly resisted by biodiversity, and that the biodiversity effect on the resistance to different types of invaders was consistent across environmental conditions. Third, while our search aimed to include all taxa and ecosystem types, we mainly found suitable data on the relationship between plant diversity and invasion resistance in grassland systems. Whether our findings are applicable to other ecosystems (e.g. forests) and other taxa (e.g. microbes and phytoplankton) remains unclear and should be explored further in future studies. Finally, the number of experiments included in our meta-analysis was relatively small, which was especially evident for particular factors (i.e. elevated CO 2 and grazing). We acknowledge that this could result from the complex and large experimental designs that are required to simultaneously manipulate biodiversity, invasion and environmental change factors. Nevertheless, the studies that made these three types of manipulations suggest that more attention should be paid to the relationship between diversity and invasion resistance in a rapidly changing world. Furthermore, amongst the studies we analyzed, the number of simultaneously applied factors and their combination was limited. This calls for experiments that incorporate more combinations of co-acting factors to explore potential generality and/or variation in higher-order interactions of factors on the relationship between diversity and invasion resistance.
Data compilation
We compiled a dataset that included factorial experiments that manipulated species richness together with at least one of several environmental change factors. We followed the PRISMA protocol 54 to identify, select and synthesize studies (Supplementary Fig. 12 ). Specifically, we searched the ISI Web of Science database, with no restriction on publication year, using the following search terms: (species richness OR diversity OR biodiversity) AND (invasion resistance OR biotic resistance OR invasibility) AND (global change* OR climate change* OR anthropogenic stressor* OR warm* OR temperatur* OR heat* OR drought OR water* OR precipitation OR rain* OR carbon dioxide OR CO 2 OR nutrient* OR fertiliz* OR fertilis* OR eutroph* OR pollution OR biocid* OR pesticid* OR fungicid* OR insecticide* OR herbicid* OR bacteriacid* OR nematicide* OR graz* OR herbivor* OR trampl* OR disturb* OR mow* OR clip* OR burn* OR fire*) AND (manipulat* OR treat* OR experiment*). We also searched for additional studies that were included in previous meta-analyses on the relationship between diversity and invasion resistance 15 , 17 , 39 , as well as the online repositories of two large biodiversity experiments: the Jena experiment in Germany ( https://jexis.idiv.de/ ) and the Cedar Creek experiment in the United States ( https://www.cedarcreek.umn.edu/research/data ).
We conducted the initial search on 10 August 2023, yielding a sample of 2096 publications. Of these, 43 duplicates were discarded, resulting in 2053 publications after the first phase of screening. After the removal of publications that based on the titles and abstracts were review or modeling studies, we assessed the remaining 1652 papers for eligibility of inclusion in our analysis using the following criteria: (1) the study must have manipulated the number of species in the resident community directly (i.e. observational studies were excluded); (2) the study must have compared mixtures with monocultures under both ambient and manipulated environmental conditions; (3) the study must provide the mean, statistical variation (standard deviation, standard error or 95% confidence intervals), and sample sizes for the performance of invaders (including both alien and native species) in different treatments. Together with five studies obtained from the online repositories of the Jena and Cedar Creek experiments, we found a total of 25 studies that met these criteria (Supplementary Data 1 ). All of these studies focused on herbaceous plant communities grown under natural or semi-natural conditions, except for two that were conducted in the greenhouse (excluding these two greenhouse studies did not qualitatively affect our conclusions; Supplementary Fig. 13 ). Environmental change factors included warming ( N = 2), drought ( N = 5), elevated atmospheric CO 2 ( N = 1), eutrophication ( N = 14), pesticide use (e.g. fungicide and insecticide) ( N = 4), grazing by domestic animals ( N = 1), human-caused fire ( N = 2), physical disturbance (e.g. mowing and trampling) ( N = 5), and combinations of two ( N = 7) or three ( N = 1) of these factors.
We used the performance —measured as biomass or percent cover— of all invaders as a proxy of invasion resistance of the resident community. Specifically, a lower performance of invaders indicates a higher invasion resistance of the resident community. If information about the identity of the invader was provided, we also distinguished between internal invaders of an experimental unit that were residents of other units of the experiment and external invaders that were not part of the experiment’s resident species pool. For the latter, we also distinguished between native and alien invaders (non-native to the location where the experiment was done). When several performance metrics were reported in the same study (e.g. cover and biomass), we used only the biomass of invaders because the majority of the studies (17 of 25) only reported biomass data. We also found that excluding the four studies that only reported cover data did not qualitatively affect our conclusions (Supplementary Figs. 14 – 16 ). We extracted the mean, statistical variation, and sample size for the performance metrics of invaders directly from data appendices, the text or tables, or from the figures using GetData Graph Digitizer (version 2.20, Russian Federation). When the relevant data were not provided in the publication, we contacted the corresponding author to obtain them. In total, we compiled a dataset consisting of 1010 observations on the performance of invaders at different levels of resident diversity. In addition to the performance of invaders, we also extracted data on the productivity (biomass or cover) of the resident community, resident species richness (1–60), experimental unit size (0.01–47.5 m 2 ) and experimental duration (0.25–24 years) wherever possible.
Effect size calculation
We calculated the effect size of NBE on invasion resistance, at each diversity level of the resident community under both ambient and manipulated environmental conditions, using the natural log of the response ratio 55 :
where \({X}_{{{{{\mathrm{mono}}}}}}\) and \({X}_{{{{{\mathrm{mix}}}}}}\) are the mean performance of invaders grown in resident monocultures and mixtures, respectively. Positive values of NBE indicate a higher invasion resistance of resident mixtures than in resident monocultures, whereas negative values indicate the opposite. The variance of NBE, \({v}_{{{{{\mathrm{NBE}}}}}}\) , was calculated as 55 :
where \(S\) is the standard deviation and \(n\) is the sample size; and the subscripts ‘mono’ and ‘mix’ refer to resident monocultures and mixtures, respectively. For 16 studies with data on resident productivity, we also calculated resident-productivity NBE using Eq. ( 1 ), but replaced invaders with the resident community.
To quantify the response of the biodiversity effect to environmental change factors, we calculated the difference in invasion-resistance NBE between ambient and manipulated environmental conditions (∆NBE), pairwise for each diversity level of the resident community, using the following equation 46 :
where the subscripts ‘A’ and ‘M’ refer to ambient and manipulated environmental conditions, respectively. Positive values of ∆NBE indicate stronger biodiversity effects under manipulated environmental conditions than under ambient conditions, while negative values indicate the opposite. The variance of ∆NBE, \({v}_{{\Delta}{{{{\mathrm{NBE}}}}}}\) , was calculated as 46 :
We quantified the effect of environmental change factors on invasion resistance and its variance in resident monocultures and mixtures, respectively, using the following equations 55 :
where \({X}_{{{{{\mathrm{A}}}}}}\) and \({X}_{{{{{\mathrm{M}}}}}}\) are the mean performance of invaders under ambient and manipulated environmental conditions, respectively. Positive values of the factor effect on invasion resistance indicate that environmental change factors increase invasion resistance, whereas negative values indicate the opposite.
To explore whether environmental change factors result in stressful or favorable environments, we quantified the effect of environmental change factors on the productivity of resident monocultures using the following equation 55 :
where \({Y}_{{{{{\mathrm{mono}}}}},{{\mbox{A}}}}\) and \({Y}_{{{{{\mathrm{mono}}}}},{{\mbox{M}}}}\) are the mean productivity of resident monocultures under ambient and manipulated environmental conditions, respectively. Positive values of the factor effect on resident productivity indicate that the environmental change factor increases the productivity of resident monocultures and thus is a favorable condition for plant growth, while negative values indicate that the environmental change factor decreases the productivity of resident monocultures and thus provides a stressful condition 46 . We only considered data for monocultures because biodiversity might buffer the effect of environmental change factors in the mixtures 46 .
Statistical analyses
Because biodiversity effects are scale-dependent and sensitive to species richness and duration of the experiment 56 , 57 , we used meta-regression models that included resident species richness, experimental duration and experimental unit size as covariates to test the effect of environmental manipulation (ambient vs. manipulated) on NBE and to derive the mean effect size of ΔNBE. We first performed these analyses for all environmental change factors and then for different types or numbers of factors. For the subset of studies with data on the invader type (internal invader, native external invader, alien external invader), we included the interaction between environmental manipulation and invader type in meta-regression models to explore whether invader type influences the effect of environmental change factors. Since we calculated the effect size of NBE by comparing multiple diversity levels to the same monoculture control, we accounted for this non-independence by computing the variance-covariance matrix of effect sizes 58 . The inverse of the sampling variance of the variance-covariance matrix was then used to weight the precision of effect sizes. To further account for possible non-independence of observations from the same study and for between-observation errors, we included observations nested in “study” as random factors in models 59 .
To test whether the biodiversity effect on invasion resistance is associated with the effect on resident productivity, resident species richness, experimental duration and experimental unit size, we used meta-regression models that included these moderators under ambient and manipulated environmental conditions, respectively. We used meta-regression models that included experimental duration and experimental unit size as covariates to test the effect of environmental change factors on invasion resistance or productivity of resident monocultures. To test the effect of environmental change factors on invasion resistance of resident mixtures, we also included resident species richness as covariate in meta-regression models. Furthermore, we used meta-regression models that included species richness, experimental duration and experimental unit size as covariates to test the relationship between invasion-resistance ∆NBE and the factor effect on resident productivity.
Finally, we tested publication bias in two ways 60 : (1) visual inspection for asymmetry in the funnel plot of the residuals from the meta-regression models, and (2) testing funnel asymmetry using Egger’s regression by including sampling standard error as a moderator in the meta-regression models (a significant sampling standard error indicates asymmetry in the funnel). When publication bias was detected, we conduced sensitivity analysis to identify potential outliers based on the Cook’s distance 61 and then conducted the analyses after removing outliers.
We performed all statistical analyses in R 4.1.3 62 . Meta-regression analyses were performed using the ‘rma.mv’ function in the ‘metafor’ package (version 4.1–0) 63 . We conducted the Q M test to determine the significance ( p < 0.05) of moderators using the ‘anova’ function in the ‘metafor’ package. We estimated the mean effect size of the biodiversity effect or the factor effect from the meta-regression models and corrected the 95% CI using the Bonferroni method with the ‘emmeans’ package (version 1.8.4–1) 64 . We considered the mean effect size to be significant if the corrected 95% CI did not overlap zero. We tested pairwise differences in the mean effect size of the biodiversity effect among invader types using the ‘multcomp’ package (version 1.4–22) 65 .
Reporting summary
Further information on research design is available in the Nature Portfolio Reporting Summary linked to this article.
Data availability
All raw data are archived in Figshare at https://doi.org/10.6084/m9.figshare.24953433 66 . Source data are provided with this paper.
Code availability
All codes are archived in Figshare at https://doi.org/10.6084/m9.figshare.24953433 66 .
Essl, F. et al. A conceptual framework for range-expanding species that track human-induced environmental change. BioScience 69 , 908–919 (2019).
Article Google Scholar
van Kleunen, M. et al. Global exchange and accumulation of non-native plants. Nature 525 , 100–103 (2015).
Article ADS PubMed Google Scholar
Seebens, H. et al. No saturation in the accumulation of alien species worldwide. Nat. Commun. 8 , 14435 (2017).
Article ADS CAS PubMed PubMed Central Google Scholar
Diagne, C. et al. High and rising economic costs of biological invasions worldwide. Nature 592 , 571–576 (2021).
Article ADS CAS PubMed Google Scholar
Simberloff, D. et al. Impacts of biological invasions: what’s what and the way forward. Trends Ecol. Evol. 28 , 58–66 (2013).
Article PubMed Google Scholar
Bellard, C., Leroy, B., Thuiller, W., Rysman, J. F. & Courchamp, F. Major drivers of invasion risks throughout the world. Ecosphere 7 , e01241 (2016).
Delavaux, C. S. et al. Native diversity buffers against severity of non-native tree invasions. Nature 621 , 773–781 (2023).
Enders, M. et al. A conceptual map of invasion biology: integrating hypotheses into a consensus network. Glob. Ecol. Biogeogr. 29 , 978–991 (2020).
Article PubMed PubMed Central Google Scholar
Elton, C. S. The Ecology of Invasions by Animals and Plants . (Springer, New York, 1958).
Levine, J. M., Adler, P. B. & Yelenik, S. G. A meta-analysis of biotic resistance to exotic plant invasions. Ecol. Lett. 7 , 975–989 (2004).
Beaury, E. M., Finn, J. T., Corbin, J. D., Barr, V. & Bradley, B. A. Biotic resistance to invasion is ubiquitous across ecosystems of the United States. Ecol. Lett. 23 , 476–482 (2020).
Davies, K. F. et al. Spatial heterogeneity explains the scale dependence of the native-exotic diversity relationship. Ecology 86 , 1602–1610 (2005).
Fridley, J. D. et al. The invasion paradox: Reconciling pattern and process in species invasions. Ecology 88 , 3–17 (2007).
Article CAS PubMed Google Scholar
Burns, K. C. Native–exotic richness relationships: a biogeographic approach using turnover in island plant populations. Ecology 97 , 2932–2938 (2016).
Peng, S., Kinlock, N. L., Gurevitch, G. & Peng, S. Correlation of native and exotic species richness: a global meta-analysis finds no invasion paradox across scales. Ecology 100 , e02552 (2019).
Stohlgren, T. J., Barnett, D. T. & Kartesz, J. T. The rich get richer: patterns of plant invasions in the United States. Front. Ecol. Environ. 1 , 11–14 (2003).
Smith, N. S. & Côté, I. M. Multiple drivers of contrasting diversity–invasibility relationships at fine spatial grains. Ecology 100 , e02573 (2019).
Tilman, D., Isbell, F. & Cowles, J. M. Biodiversity and ecosystem functioning. Annu. Rev. Ecol. Evol. Syst. 45 , 471–493 (2014).
Cheng, C. et al. Genotype diversity enhances invasion resistance of native plants via soil biotic feedbacks. Ecol. Lett. 27 , e14384 (2024).
Fargione, J. E. & Tilman, D. Diversity decreases invasion via both sampling and complementarity effects. Ecol. Lett. 8 , 604–611 (2005).
Kennedy, T. A. et al. Biodiversity as a barrier to ecological invasion. Nature 417 , 636–638 (2002).
van Ruijven, J., De Deyn, G. B. & Berendse, F. Diversity reduces invasibility in experimental plant communities: the role of plant species. Ecol. Lett. 6 , 910–918 (2003).
Wei, G. W. & van Kleunen, M. Soil heterogeneity tends to promote the growth of naturalized aliens when competing with native plant communities. J. Ecol. 110 , 1161–1173 (2022).
Zheng, Y. L. et al. Species composition, functional and phylogenetic distances correlate with success of invasive Chromolaena odorata in an experimental test. Ecol. Lett. 21 , 1211–1220 (2018).
El-barougy, R. et al. Richness, phylogenetic diversity, and abundance all have positive effects on invader performance in an arid ecosystem. Ecosphere 11 , e03045 (2020).
Emery, S. M. & Gross, K. L. Dominant species identity regulates invasibility of old-field plant communities. Oikos 115 , 549–558 (2006).
Article ADS Google Scholar
Von Holle, B. Environmental stress alters native-nonnative relationships at the community scale. Biol. Invasions 15 , 417–427 (2013).
Steudel, B. et al. Biodiversity effects on ecosystem functioning change along environmental stress gradients. Ecol. Lett. 15 , 1397–1405 (2012).
Stotz, G. C., Pec, G. J. & Cahill, J. F. Is biotic resistance to invaders dependent upon local environmental conditions or primary productivity? A meta-analysis. Basic Appl. Ecol. 17 , 377–387 (2016).
Ripple, W. J. et al. World scientists’ warning to humanity: A second notice. BioScience 67 , 1026–1028 (2017).
He, Q., Bertness, M. D. & Altieri, A. H. Global shifts towards positive species interactions with increasing environmental stress. Ecol. Lett. 16 , 695–706 (2013).
Liu, Y. et al. Do invasive alien plants benefit more from global environmental change than native plants? Glob. Change Biol. 23 , 3363–3370 (2017).
Heckman, R. W., Halliday, F. W., Wilfahrt, P. A. & Mitchell, C. E. Effects of native diversity, soil nutrients, and natural enemies on exotic invasion in experimental plant communities. Ecology 98 , 1409–1418 (2017).
de Gea, A. B., Hautier, Y. & Geisen, S. Interactive effects of global change drivers as determinants of the link between soil biodiversity and ecosystem functioning. Glob. Change Biol. 29 , 296–307 (2023).
Rillig, M. C. et al. The role of multiple global change factors in driving soil functions and microbial biodiversity. Science 366 , 886–890 (2019).
Qiu, S. et al. Changes in multiple environmental factors additively enhance the dominance of an exotic plant with a novel trade-off pattern. J. Ecol. 108 , 1989–1999 (2020).
Speißer, B., Wilschut, R. A. & van Kleunen, M. Number of simultaneously acting global change factors affects composition, diversity and productivity of grassland plant communities. Nat. Commun. 13 , 7811 (2022).
Article ADS PubMed PubMed Central Google Scholar
Zandalinas, S. I. et al. The impact of multifactorial stress combination on plant growth and survival. New Phytol 230 , 1034–1048 (2021).
Article CAS PubMed PubMed Central Google Scholar
Li, S. P. et al. Functional traits explain the consistent resistance of biodiversity to plant invasion under nitrogen enrichment. Ecol. Lett. 25 , 778–789 (2022).
Hector, A., Dobson, K., Minns, A., Bazeley-White, E. & Lawton, J. H. Community diversity and invasion resistance: An experimental test in a grassland ecosystem and a review of comparable studies. Ecol. Res. 16 , 819–831 (2001).
Huang, Y. et al. Enhanced stability of grassland soil temperature by plant diversity. Nat. Geosci. 17 , 44–50 (2024).
Fischer, C. et al. Plant species richness and functional groups have different effects on soil water content in a decade-long grassland experiment. J. Ecol. 107 , 127–141 (2019).
Thakur, M. P. et al. Plant diversity drives soil microbial biomass carbon in grasslands irrespective of global environmental change factors. Glob. Change Biol. 21 , 4076–4085 (2015).
Isbell, F. et al. Biodiversity increases the resistance of ecosystem productivity to climate extremes. Nature 526 , 574–577 (2015).
Tilman, D., Reich, P. B. & Knops, J. M. H. Biodiversity and ecosystem stability in a decade-long grassland experiment. Nature 441 , 629–632 (2006).
Hong, P. et al. Biodiversity promotes ecosystem functioning despite environmental change. Ecol. Lett. 25 , 555–569 (2022).
Komatsu, K. J. et al. Global change effects on plant communities are magnified by time and the number of global change factors imposed. Proc. Natl. Acad. Sci. USA. 116 , 17867–17873 (2019).
Benkwitt, C. E., Wilson, S. K. & Graham, N. A. J. Biodiversity increases ecosystem functions despite multiple stressors on coral reefs. Nat. Ecol. Evol. 4 , 919–926 (2020).
Orr, J. A., Luijckx, P., Arnoldi, J., Jackson, A. L. & Piggott, J. J. Rapid evolution generates synergism between multiple stressors: Linking theory and an evolution experiment. Glob. Change Biol. 28 , 1740–1752 (2022).
Article CAS Google Scholar
Reich, P. B. et al. Impacts of biodiversity loss escalate through time as redundancy fades. Science 336 , 589–592 (2012).
Huang, Y. et al. Impacts of species richness on productivity in a large-scale subtropical forest experiment. Science 362 , 80–83 (2018).
Cardinale, B. J. et al. Effects of biodiversity on the functioning of trophic groups and ecosystems. Nature 443 , 989–992 (2006).
Li, C. et al. The productive performance of intercropping. Proc. Natl. Acad. Sci. USA. 120 , e2201886120 (2023).
Moher, D. et al. Preferred reporting items for systematic reviews and meta-analyses: The PRISMA statement. PLos Med 6 , e1000097 (2009).
Borenstein, M., Hedges, L. V., Higgins, J. P. T. & Rothstein, H. R. Introduction to Meta-analysis (Wiley, New York, 2009).
Chase, J. M. et al. Embracing scale-dependence to achieve a deeper understanding of biodiversity and its change across communities. Ecol. Lett. 21 , 1737–1751 (2018).
Spake, R. et al. Implications of scale dependence for cross-study syntheses of biodiversity differences. Ecol. Lett. 24 , 374–390 (2021).
Lajeunesse, M. J. On the meta-analysis of response ratios for studies with correlated and multi-group designs. Ecology 92 , 2049–2055 (2011).
Zhang, Z., Liu, Y., Yuan, L., Weber, E. & van Kleunen, M. Effect of allelopathy on plant performance: a meta-analysis. Ecol. Lett. 24 , 348–362 (2021).
Bishop, J. & Nakagawa, S. Quantifying crop pollinator dependence and its heterogeneity using multi-level meta-analysis. J. Appl. Ecol. 58 , 1030–1042 (2021).
Viechtbauer, W. & Cheung, M. W. L. Outlier and influence diagnostics for meta-analysis. Res. Synth. Methods 1 , 112–125 (2010).
R Core Team. R: A language and environment for statistical computing (R Foundation for Statistical Computing, Vienna, 2022).
Viechtbauer, W. Conducting meta-analyses in R with the metafor package. J. Stat. Softw. 36 , 1–48 (2010).
Lenth, R. V. et al. emmeans: Estimated marginal means, aka least-squares means. R package version 1.8.4-1. https://cran.r-project.org/package=emmeans (2023).
Hothorn, T., Bretz, F. & Westfall, P. Simultaneous inference in general parametric models. Biom. J. 50 , 346–363 (2008).
Article MathSciNet PubMed Google Scholar
Cheng, C. et al. Biodiversity increases resistance of grasslands against plant invasions under multiple environmental changes. figshare https://doi.org/10.6084/m9.figshare.24953433 (2024).
Download references
Acknowledgements
The study was funded by National Key Research and Development Program of China (2022YFC2601100), National Natural Science Foundation of China (32030067), Department of Science and Technology of Yunnan Province (202405AS350011) and Talent Scientific Fund of Lanzhou University awarded to J.W. and B.L. We thank Yue Chen for drawing the symbols of environmental change factors. We also thank the authors who generously shared their data. The data on the Jena Experiment were obtained by C.C. from the Jena Experiment database ( https://jexis.idiv.de/ ) in August 2023. We thank Anja Vogel, Alexandra Weigelt and Anne Ebeling for making this data set available. The Jena Experiment is a research unit funded by the Deutsche Forschungsgemeinschaft (FOR 456/1451/5000).
Author information
Authors and affiliations.
State Key Laboratory of Herbage Improvement and Grassland Agro-Ecosystems, College of Ecology, Lanzhou University, Lanzhou, 730000, China
Cai Cheng & Jihua Wu
Ministry of Education Key Laboratory for Biodiversity Science and Ecological Engineering, National Observations and Research Station of Wetland Ecosystems of the Yangtze Estuary, Institute of Biodiversity Science and Institute of Eco-Chongming, School of Life Sciences, Fudan University, Shanghai, 200438, China
Cai Cheng, Zekang Liu, Wei Song & Xue Chen
Department of Biology, University of Konstanz, Konstanz, 78464, Germany
Zhijie Zhang & Mark van Kleunen
Ministry of Education Key Laboratory for Transboundary Ecosecurity of Southwest China, Yunnan Key Laboratory of Plant Reproductive Adaptation and Evolutionary Ecology and Centre for Invasion Biology, Institute of Biodiversity, School of Ecology and Environmental Science, Yunnan University, Kunming, 650504, China
Zhejiang Provincial Key Laboratory of Plant Evolutionary Ecology and Conservation, Taizhou University, Taizhou, 318000, China
Mark van Kleunen
You can also search for this author in PubMed Google Scholar
Contributions
J.W. conceived the study. C.C. led the data collection, with help from Z.L., W.S., and X.C. C.C. analyzed the data and wrote the manuscript, with substantial input from M.v.K., J.W., B.L., and Z.Z.
Corresponding author
Correspondence to Jihua Wu .
Ethics declarations
Competing interests.
The authors declare no competing interests.
Peer review
Peer review information.
Nature Communications thanks Camille Delavaux and Lotte Korell for their contribution to the peer review of this work. A peer review file is available.
Additional information
Publisher’s note Springer Nature remains neutral with regard to jurisdictional claims in published maps and institutional affiliations.
Supplementary information
Supplementary information, peer review file, description of additional supplementary files, supplementary data 1, reporting summary, source data, source data, rights and permissions.
Open Access This article is licensed under a Creative Commons Attribution 4.0 International License, which permits use, sharing, adaptation, distribution and reproduction in any medium or format, as long as you give appropriate credit to the original author(s) and the source, provide a link to the Creative Commons licence, and indicate if changes were made. The images or other third party material in this article are included in the article’s Creative Commons licence, unless indicated otherwise in a credit line to the material. If material is not included in the article’s Creative Commons licence and your intended use is not permitted by statutory regulation or exceeds the permitted use, you will need to obtain permission directly from the copyright holder. To view a copy of this licence, visit http://creativecommons.org/licenses/by/4.0/ .
Reprints and permissions
About this article
Cite this article.
Cheng, C., Liu, Z., Song, W. et al. Biodiversity increases resistance of grasslands against plant invasions under multiple environmental changes. Nat Commun 15 , 4506 (2024). https://doi.org/10.1038/s41467-024-48876-z
Download citation
Received : 07 January 2024
Accepted : 15 May 2024
Published : 27 May 2024
DOI : https://doi.org/10.1038/s41467-024-48876-z
Share this article
Anyone you share the following link with will be able to read this content:
Sorry, a shareable link is not currently available for this article.
Provided by the Springer Nature SharedIt content-sharing initiative
By submitting a comment you agree to abide by our Terms and Community Guidelines . If you find something abusive or that does not comply with our terms or guidelines please flag it as inappropriate.
Quick links
- Explore articles by subject
- Guide to authors
- Editorial policies
Sign up for the Nature Briefing newsletter — what matters in science, free to your inbox daily.

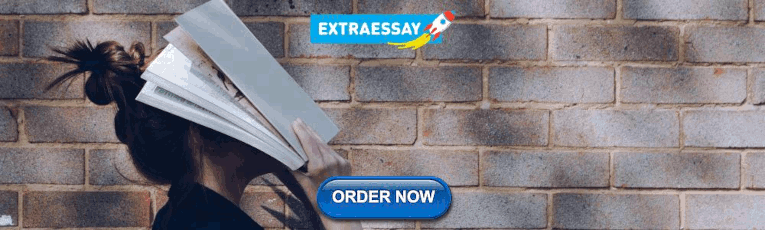
IMAGES
VIDEO
COMMENTS
Developing a hypothesis (with example) Step 1. Ask a question. Writing a hypothesis begins with a research question that you want to answer. The question should be focused, specific, and researchable within the constraints of your project. Example: Research question.
A hypothesis is a tentative statement about the relationship between two or more variables. It is a specific, testable prediction about what you expect to happen in a study. It is a preliminary answer to your question that helps guide the research process. Consider a study designed to examine the relationship between sleep deprivation and test ...
Examples. A research hypothesis, in its plural form "hypotheses," is a specific, testable prediction about the anticipated results of a study, established at its outset. It is a key component of the scientific method. Hypotheses connect theory to data and guide the research process towards expanding scientific understanding.
Simple hypothesis. A simple hypothesis is a statement made to reflect the relation between exactly two variables. One independent and one dependent. Consider the example, "Smoking is a prominent cause of lung cancer." The dependent variable, lung cancer, is dependent on the independent variable, smoking. 4.
Here are some good research hypothesis examples: "The use of a specific type of therapy will lead to a reduction in symptoms of depression in individuals with a history of major depressive disorder.". "Providing educational interventions on healthy eating habits will result in weight loss in overweight individuals.".
Step 5: Phrase your hypothesis in three ways. To identify the variables, you can write a simple prediction in if … then form. The first part of the sentence states the independent variable and the second part states the dependent variable. If a first-year student starts attending more lectures, then their exam scores will improve.
Here are some research hypothesis examples: If you leave the lights on, then it takes longer for people to fall asleep. If you refrigerate apples, they last longer before going bad. If you keep the curtains closed, then you need less electricity to heat or cool the house (the electric bill is lower). If you leave a bucket of water uncovered ...
A hypothesis is a starting point for further investigation and testing because a hypothesis makes a prediction about the behavior of a measurable outcome of an experiment. A hypothesis should be: Testable - you can design an experiment to test it. Falsifiable - it can be proven wrong (note it cannot be "proved")
Definition: Hypothesis is an educated guess or proposed explanation for a phenomenon, based on some initial observations or data. It is a tentative statement that can be tested and potentially proven or disproven through further investigation and experimentation. Hypothesis is often used in scientific research to guide the design of experiments ...
A good experimental design requires a strong understanding of the system you are studying. There are five key steps in designing an experiment: Consider your variables and how they are related. Write a specific, testable hypothesis. Design experimental treatments to manipulate your independent variable.
An effective hypothesis in research is clearly and concisely written, and any terms or definitions clarified and defined. Specific language must also be used to avoid any generalities or assumptions. Use the following points as a checklist to evaluate the effectiveness of your research hypothesis: Predicts the relationship and outcome.
The steps to write a research hypothesis are: 1. Stating the problem: Ensure that the hypothesis defines the research problem. 2. Writing a hypothesis as an 'if-then' statement: Include the action and the expected outcome of your study by following a 'if-then' structure. 3.
15 Hypothesis Examples. A hypothesis is defined as a testable prediction, and is used primarily in scientific experiments as a potential or predicted outcome that scientists attempt to prove or disprove (Atkinson et al., 2021; Tan, 2022). In my types of hypothesis article, I outlined 13 different hypotheses, including the directional hypothesis ...
EXAMPLE 3. Hypothesis-generating research to establish a framework for hypothesis testing (qualitative research) ... (RCT) to evaluate and compare changes in salivary cortisol and oxytocin levels of first-time pregnant women between experimental and control groups. The women in the experimental group touched and held an infant for 30 min ...
This section will review several examples of experimental hypotheses. One example is a scientist looking to investigate the relationship between exposure to UV radiation and incidence of brain ...
An experimental hypothesis, often referred to simply as a hypothesis, is a precise, testable statement about the expected outcome of an experiment or a research study. It predicts a relationship between two or more variables that can be tested through controlled experiments.
Experimental research serves as a fundamental scientific method aimed at unraveling. cause-and-effect relationships between variables across various disciplines. This. paper delineates the key ...
The true experimental research design relies on statistical analysis to approve or disprove a hypothesis. It is the most accurate type of experimental design and may be carried out with or without a pretest on at least 2 randomly assigned dependent subjects. ... Examples of Experimental Research. Experimental research examples are different ...
A true experimental research design relies on statistical analysis to prove or disprove a researcher's hypothesis. It is one of the most accurate forms of research because it provides specific scientific evidence. Furthermore, out of all the types of experimental designs, only a true experimental design can establish a cause-effect ...
Experimental Design. Experimental design is a process of planning and conducting scientific experiments to investigate a hypothesis or research question. It involves carefully designing an experiment that can test the hypothesis, and controlling for other variables that may influence the results. Experimental design typically includes ...
For example, a greater number of resident plant species, a longer duration of the experiment and smaller experimental units should result in stronger complementarity effects, and should reduce the ...