- Speakers & Mentors
- AI services
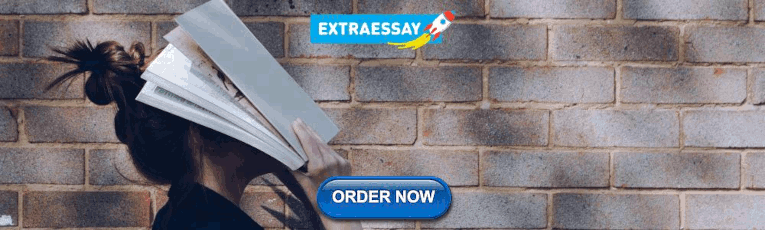
The Role and Impact of Expert Systems in AI – Enhancing Decision-Making and Problem Solving
AI-powered technologies have revolutionized the world of intelligence, enabling machines to perform tasks that once seemed only within the realm of human capability. One such technology is the expert system, a powerful tool in the field of artificial intelligence. Powered by a combination of advanced algorithms and human expertise, expert systems have the ability to analyze complex data, make informed decisions, and provide valuable insights.
An expert system is designed to mimic the problem-solving abilities of a human expert in a specific domain. By capturing and formalizing knowledge from domain experts, an expert system can provide intelligent solutions to complex problems. With their ability to process vast amounts of information and learn from past experiences, these systems are highly efficient and reliable.
The applications of expert systems are diverse and span across various industries. In medicine, expert systems can assist doctors in diagnosing diseases, recommending treatments, and predicting patient outcomes. In finance, these systems can analyze market trends, make investment recommendations, and manage risks. Additionally, expert systems are used in engineering, logistics, customer support, and many other domains where expertise is crucial for decision-making.
Examples of successful expert systems include IBM’s Watson, which gained fame by winning the Jeopardy! game show in 2011. Watson is capable of understanding natural language, processing vast amounts of unstructured data, and providing accurate answers to complex questions. Another notable example is Mycin, an expert system developed in the 1970s for diagnosing and recommending treatments for bacterial infections. Mycin demonstrated the potential of expert systems in the field of healthcare and paved the way for future advancements.
Key Advantages of Expert Systems
Artificial intelligence (AI) has revolutionized various industries, and one of its most significant applications is the development of AI-powered expert systems. These systems are knowledge-based, designed to simulate human expertise and provide accurate and reliable advice or solutions in specific domains.
1. Enhanced Decision-Making
Expert systems leverage the power of AI and knowledge-based programming to enhance decision-making processes. By employing logical reasoning capabilities and vast amounts of domain-specific knowledge, these systems can quickly analyze complex situations, evaluate various options, and recommend the most appropriate course of action. This results in faster and more accurate decision-making, as well as reduced human errors.
2. Increased Efficiency
Using expert systems eliminates the need for individuals to spend considerable time searching for information or consulting experts. These AI-powered systems can store and retrieve vast amounts of knowledge quickly, making them a valuable resource for businesses and organizations. By streamlining workflows and automating repetitive tasks, expert systems significantly increase efficiency and productivity, allowing employees to focus on more strategic and creative endeavors.
In addition, expert systems can be implemented across different platforms and accessed remotely, allowing users to benefit from their capabilities regardless of their physical location.
Overall, expert systems have the potential to revolutionize various industries and pave the way for smarter and more efficient processes. By harnessing the power of artificial intelligence and knowledge-based programming, these systems can provide accurate advice and solutions, enhancing decision-making and increasing overall productivity.
Applications of Expert Systems in Various Industries
Expert systems, also known as knowledge-based systems, are a type of AI-powered technology that emulates human expertise and intelligence. These systems are designed to simulate decision-making processes and offer customized solutions to complex problems in various industries.
Healthcare Industry
In the healthcare industry, expert systems have proven to be invaluable tools for diagnosis and treatment planning. By analyzing patient data and medical literature, these systems can provide accurate and timely recommendations for doctors, helping them make informed decisions and improve patient outcomes.
Financial Industry
The financial industry heavily relies on expert systems to automate processes and enhance decision-making. These systems can analyze vast amounts of financial data, detect patterns, and provide personalized investment advice. They can also identify potential risks and fraud, improving overall security and compliance.
Moreover, in areas like insurance underwriting, expert systems assist in assessing risk profiles and determining appropriate coverage, leading to more tailored insurance policies for customers.
Manufacturing Industry
In the manufacturing industry, expert systems are used to optimize production processes and ensure product quality. These systems can monitor equipment performance, detect anomalies, and proactively suggest maintenance actions to reduce downtime. By leveraging AI-powered technologies, manufacturers can achieve higher efficiency and cost savings.
Customer Support
Customer support is an essential area where expert systems play a crucial role. These systems can analyze customer queries, provide accurate and relevant information, and offer personalized solutions. By automating routine tasks and providing instant support, companies can enhance customer satisfaction and streamline their support operations.
AI-powered expert systems have the ability to revolutionize various industries by leveraging their knowledge-based approaches. These systems can help professionals make better decisions, improve efficiency, and enhance customer experiences. As technology continues to evolve, the applications and benefits of expert systems are expected to expand even further.
Expert Systems in Healthcare
Artificial intelligence (AI) has revolutionized many industries, including healthcare. One of the key applications of AI in healthcare is the use of expert systems, which are AI-powered knowledge-based systems designed to replicate the decision-making abilities of human experts.
Expert systems in healthcare can analyze vast amounts of patient data and medical knowledge to provide accurate diagnoses, treatment recommendations, and personalized healthcare plans. These systems use advanced AI algorithms and machine learning techniques to process and interpret the data, allowing for more efficient and effective healthcare delivery.
One of the main advantages of expert systems in healthcare is their ability to handle complex medical data and provide accurate and timely insights. These systems can analyze patient symptoms, medical histories, and laboratory results to identify potential diseases or conditions, helping healthcare professionals make faster and more accurate diagnoses.
Furthermore, expert systems can provide treatment recommendations based on evidence-based guidelines and best practices. They can suggest optimal medication regimens, dosage adjustments, and even predict potential side effects or drug interactions, aiding healthcare professionals in making informed treatment decisions.
Another benefit of expert systems in healthcare is their ability to provide personalized healthcare plans. These systems can take into account individual patient characteristics, such as age, gender, medical history, and genetic information, to create customized treatment plans tailored to each patient’s specific needs. This can lead to more effective treatments and better patient outcomes.
In summary, expert systems powered by AI intelligence are revolutionizing healthcare by providing accurate diagnoses, treatment recommendations, and personalized healthcare plans. These systems have the potential to improve healthcare delivery, enhance patient outcomes, and reduce healthcare costs. With ongoing advancements in AI technology, the future of expert systems in healthcare looks promising.
Expert Systems in Finance
Expert systems are a type of artificial intelligence (AI)-powered system that simulate the knowledge and decision-making abilities of human experts in a specific domain. In the field of finance, AI-powered expert systems have proven to be invaluable tools for analyzing and making predictions based on financial data.
These knowledge-based systems are designed to capture the expertise of financial professionals and translate it into actionable insights. They are built using a combination of rule-based algorithms and machine learning models to process vast amounts of financial data and generate accurate predictions.
One of the key advantages of expert systems in finance is their ability to quickly and efficiently analyze complex financial data. These systems can process large volumes of historical data, identify patterns, and make predictions on future market trends. This can help financial institutions make more informed decisions, manage risks, and optimize investment strategies.
Furthermore, expert systems in finance can also assist in automating various financial processes, such as credit scoring, fraud detection, and portfolio management. By leveraging AI-powered algorithms, these systems can perform these tasks more accurately and efficiently, reducing human error and improving overall operational efficiency.
Some examples of AI-powered expert systems in finance include algorithmic trading systems, robo-advisors, and credit scoring platforms. These systems use advanced machine learning algorithms to analyze market data, provide investment recommendations, and evaluate creditworthiness, respectively.
In conclusion, expert systems in finance, powered by artificial intelligence, are revolutionizing the way financial professionals analyze data, make decisions, and manage risks. With their ability to process vast amounts of information and generate accurate predictions, these systems are becoming indispensable tools in the financial industry.
Expert Systems in Manufacturing
Artificial intelligence (AI) has revolutionized various industries, and manufacturing is no exception. AI-powered expert systems have proven to be highly beneficial in streamlining and improving manufacturing processes.
What are Expert Systems?
Expert systems are a type of artificial intelligence system designed to mimic the decision-making abilities of human experts in a particular domain. These systems utilize knowledge-based techniques to analyze data, make informed decisions, and provide expert-level recommendations.
In the manufacturing industry, expert systems play a crucial role in enhancing productivity, efficiency, and quality control. They are capable of capturing and applying the knowledge and expertise of experienced manufacturing professionals to solve complex problems and make critical decisions.
Applications of Expert Systems in Manufacturing
Expert systems are widely utilized in various manufacturing processes, including:
These are just a few examples of how expert systems are transforming the manufacturing industry. By leveraging artificial intelligence and knowledge-based techniques, manufacturers can enhance decision-making processes, improve operational efficiency, and ultimately achieve greater success in today’s competitive market.
Expert Systems in Customer Service
Artificial intelligence (AI) has transformed many industries, and customer service is no exception. Companies are now leveraging AI-powered expert systems to enhance their customer support capabilities.
An expert system is an AI-powered system that emulates the decision-making process of a human expert in a particular field. It uses a knowledge base and a set of rules to provide accurate and personalized solutions to customer queries and issues.
The advantages of using expert systems in customer service are numerous. Firstly, these systems can handle a large volume of customer inquiries simultaneously, reducing the need for human agents and improving response time. Secondly, because expert systems are trained using data from past customer interactions, they are able to provide consistent and reliable answers to commonly asked questions.
Furthermore, expert systems can be programmed to learn from new data and update their knowledge base accordingly. This means that as customer preferences and needs change, the expert system can adapt and provide more relevant and up-to-date solutions.
Some common applications of expert systems in customer service include chatbots, virtual assistants, and recommendation engines. Chatbots can interact with customers in real-time, answering their questions and resolving their issues. Virtual assistants can provide personalized recommendations and suggestions based on a customer’s preferences and past interactions. Recommendation engines can analyze a customer’s browsing and purchasing history to offer relevant products and services.
In conclusion, AI-powered expert systems have revolutionized customer service by providing accurate, efficient, and personalized solutions to customer queries. By leveraging these systems, companies can enhance their customer support capabilities and improve overall customer satisfaction.
Expert Systems in Transportation
Expert systems, also known as AI-powered or knowledge-based systems, have proven to be valuable tools in the transportation industry. By leveraging artificial intelligence and expert knowledge, these systems can analyze vast amounts of data and make informed decisions to optimize transportation operations.
One of the main applications of expert systems in transportation is route optimization. By considering factors such as traffic conditions, weather conditions, and vehicle characteristics, these systems can determine the most efficient routes for transporting goods or people. This not only saves time and resources but also reduces carbon emissions and improves overall efficiency.
Another application of expert systems in transportation is predictive maintenance. By analyzing data from sensors and monitoring equipment, these systems can detect potential issues before they cause major disruptions. This allows for timely maintenance and reduces the risk of breakdowns, improving the reliability of transportation services.
Expert systems are also used in scheduling and resource allocation. By considering factors such as demand, availability, and constraints, these systems can optimize the allocation of vehicles, drivers, and other resources to meet customer demand while minimizing costs. This ensures efficient and effective transportation services.
Furthermore, expert systems can assist in decision-making processes, such as route planning, incident management, and emergency response. By analyzing real-time data and considering various factors, these systems can provide valuable insights and recommendations to help transportation professionals make informed decisions quickly and effectively.
In conclusion, expert systems powered by AI are playing a crucial role in the transportation industry. From route optimization to predictive maintenance and decision support, these systems provide intelligent solutions to improve the efficiency, reliability, and sustainability of transportation operations.
Expert Systems in Energy
Expert systems are a type of AI-powered system that utilize artificial intelligence and knowledge-based techniques to provide specialized recommendations and solutions. In the field of energy, these systems play a crucial role in optimizing energy usage, improving efficiency, and reducing costs.
Advantages of Expert Systems in Energy
- Increased Efficiency: Expert systems can analyze complex data and provide optimal solutions for energy management, leading to increased efficiency in energy usage.
- Cost Reduction: By optimizing energy usage and identifying potential areas for improvement, expert systems can help reduce energy costs in various sectors, such as manufacturing and utilities.
- Real-time Monitoring: These systems can continuously monitor energy consumption and provide real-time alerts and recommendations for energy-saving measures.
- Predictive Maintenance: Expert systems can use historical and real-time data to predict potential equipment failures, allowing for proactive maintenance and minimizing downtime.
Applications of Expert Systems in Energy
Expert systems are being used in various energy-related applications, including:
- Smart Grid Optimization: Expert systems are utilized to optimize power distribution and manage load balancing within smart grids, ensuring a stable and reliable energy supply.
- Energy Management Systems: These systems help organizations analyze and manage their energy consumption, identifying opportunities for energy efficiency and cost reduction.
- Renewable Energy Integration: Expert systems aid in the integration of renewable energy sources into existing energy grids, optimizing their usage and minimizing reliance on traditional fossil fuels.
- Energy Trading: AI-powered expert systems are used in energy trading platforms to analyze market data and make informed decisions on energy buying and selling.
- Energy Auditing: Expert systems assist in conducting energy audits by analyzing energy usage patterns, identifying areas of improvement, and recommending energy-saving measures.
In conclusion, expert systems powered by AI and knowledge-based techniques have proven to be valuable tools in the energy sector. From optimizing energy usage to enabling renewable energy integration, these systems hold great promise for a more efficient and sustainable energy future.
Expert Systems in Retail
Retail businesses can greatly benefit from the implementation of expert systems, which are knowledge-based and AI-powered tools that enable intelligent decision-making and problem-solving. With the help of artificial intelligence (AI), expert systems can analyze large amounts of data and provide valuable insights, recommendations, and personalized experiences for both customers and retailers.
One of the key advantages of expert systems in the retail industry is their ability to enhance customer service and improve the overall shopping experience. By analyzing customer preferences and purchasing history, these systems can make personalized product recommendations, offer targeted promotions, and even provide virtual assistants to assist customers in finding the right products.
Inventory Management
Expert systems can also be utilized in retail for efficient inventory management. By analyzing historical sales data, current demand, and market trends, these systems can predict demand patterns, optimize inventory levels, and prevent stockouts or overstock situations. This helps retailers minimize holding costs, improve supply chain efficiency, and ensure that they have the right products in stock at the right time.
Pricing and Promotions
Furthermore, expert systems can assist retailers in pricing and promotional strategies. By analyzing competitive pricing, customer behavior, and market dynamics, these systems can provide retailers with optimal pricing recommendations to maximize profits while remaining competitive. They can also help in creating effective promotional campaigns by identifying the right products to discount and the most suitable timing for promotions.
In conclusion, expert systems play a crucial role in the retail sector by leveraging artificial intelligence to provide intelligent solutions for various aspects of the business such as customer service, inventory management, pricing, and promotions. By utilizing these AI-powered tools, retailers can improve customer satisfaction, optimize operations, and make data-driven decisions to stay competitive in the ever-changing retail landscape.
Expert Systems in Agriculture
Agriculture is a field that greatly benefits from the implementation of expert systems, which combine the power of artificial intelligence with knowledge-based systems. These AI-powered systems are designed to emulate the decision-making abilities of human experts in the agricultural domain.
Expert systems in agriculture are capable of analyzing data from various sources, such as soil composition, weather patterns, crop types, and pests, to provide valuable insights and recommendations. By leveraging advanced algorithms and machine learning techniques, these systems can process large amounts of data and generate accurate predictions.
One of the primary advantages of expert systems in agriculture is their ability to enhance crop production and reduce the risks associated with farming. With the help of AI-powered technologies, farmers can make informed decisions about irrigation, fertilizer application, and pest control. This not only improves the overall yield but also minimizes the use of resources and increases sustainability.
Another application of expert systems in agriculture is in the field of precision farming. By collecting data from sensors and drones, coupled with machine learning algorithms, these systems can create precise maps of fields and identify areas that require specific attention. This allows farmers to optimize the use of resources and maximize the productivity of their land.
The knowledge-based nature of expert systems in agriculture ensures that the information provided is reliable and accurate. Farmers can trust these systems to provide valuable insights based on scientific principles and real-time data. This empowers farmers to make well-informed decisions, resulting in improved crop quality and increased profits.
In conclusion, expert systems in agriculture are a valuable tool in modern farming practices. By harnessing the power of artificial intelligence, these knowledge-based systems enable farmers to optimize their operations, increase productivity, and make informed decisions. With the continuous advancement of AI technologies, the potential for expert systems in agriculture is vast, offering greater precision, efficiency, and sustainability to the industry.
Knowledge-Based AI Systems
Knowledge-based AI systems, also known as expert systems, are a type of artificial intelligence (AI) system that are powered by knowledge. These systems use a combination of pre-programmed knowledge and reasoning algorithms to solve complex problems and make decisions.
How Do Knowledge-Based AI Systems Work?
In a knowledge-based AI system, expert knowledge in a specific domain is encoded into a knowledge base. This knowledge base contains rules, facts, and relationships that the system uses to evaluate and solve problems. The system uses an inference engine to apply these rules and reasoning algorithms to new input and generate solutions or recommendations.
The knowledge base is created by domain experts who input their knowledge into the system. This knowledge can be in the form of if-then rules, which specify certain conditions and corresponding actions in response to those conditions. The inference engine then uses these rules to reason about new situations and make decisions.
Advantages of Knowledge-Based AI Systems
Knowledge-based AI systems have several advantages:
- Domain-specific expertise: These systems are designed to have deep knowledge and expertise in a specific domain, making them highly effective in solving complex problems within that domain.
- Transparency: The reasoning process of knowledge-based AI systems is transparent, as the system can explain how it arrived at a particular solution or recommendation. This transparency is essential in fields where decision-making accountability is required.
- Scalability: Once the knowledge base is created, it can be easily replicated and deployed across multiple instances, allowing for scalability and widespread adoption.
Knowledge-based AI systems are widely used in various industries, including healthcare, finance, customer support, and manufacturing. These systems have proven to be valuable tools for automating complex decision-making processes, reducing errors, and improving efficiency.
In conclusion, knowledge-based AI systems, also known as expert systems, are AI-powered systems that use expertise and knowledge to solve complex problems. With their domain-specific knowledge and transparent reasoning process, these systems have become valuable tools in various industries.
Components of Knowledge-Based AI Systems
Artificial intelligence (AI) has revolutionized various industries, and one of its key branches is expert systems. These AI-powered systems are designed to mimic human expertise and decision-making processes by leveraging vast amounts of knowledge and rules. Knowledge-based AI systems are built upon two main components: the knowledge base and the inference engine.
1. Knowledge Base
The knowledge base is the core element of a knowledge-based AI system. It is a repository that stores all the information and rules necessary for solving problems or making decisions. The knowledge base is typically built using an organized structure, such as a database or a knowledge graph, to enable efficient retrieval of the relevant information.
The knowledge base consists of two types of knowledge:
2. Inference Engine
The inference engine is responsible for reasoning and making inferences based on the information stored in the knowledge base. It applies logical rules, deductive reasoning, and pattern matching techniques to draw conclusions or generate solutions to problems.
The inference engine follows a set of predefined rules and algorithms to process the available data. It uses various techniques, such as forward chaining (from facts to conclusions) and backward chaining (from goals to sub-goals), to derive new knowledge or reach a final decision.
The integration of the knowledge base and the inference engine enables knowledge-based AI systems to analyze complex problems, provide expert advice, and support decision-making processes. These systems have been successfully applied in a wide range of domains, including healthcare, finance, manufacturing, and customer service, to name a few.
In conclusion, knowledge-based AI systems combine artificial intelligence and expert knowledge to create powerful problem-solving tools. The knowledge base acts as the repository of information and rules, while the inference engine performs reasoning and decision-making tasks. Together, these components drive the effectiveness and efficiency of knowledge-based AI systems in various real-world applications.
Development of Knowledge-Based AI Systems
Knowledge-based AI systems are a crucial aspect of the artificial intelligence landscape. These systems utilize a vast amount of data and domain-specific knowledge to make informed decisions and solve complex problems. Powered by advanced algorithms and expert knowledge, they have the ability to perform tasks that would typically require human intelligence.
Development of knowledge-based AI systems involves several key steps. The first step is acquiring and organizing the knowledge required for the system. This knowledge can be obtained through various sources such as textbooks, scientific papers, and domain experts. Once the knowledge is collected, it needs to be carefully structured and stored in a format that the AI system can understand.
Next, the development team will design and implement the reasoning engine of the AI system. This engine is responsible for processing the acquired knowledge and applying it to solve problems or make decisions. Advanced algorithms and rule-based systems are commonly used in this stage to analyze the knowledge and provide accurate outputs.
One of the major challenges in developing knowledge-based AI systems is ensuring the accuracy and reliability of the information being used. This requires rigorous validation and testing procedures to identify any inconsistencies or errors in the knowledge base. Additionally, regular updates and maintenance are necessary to keep the knowledge base up to date with the latest advancements in the domain.
Once the development process is complete, the knowledge-based AI system can be deployed in various applications. These systems find applications in numerous fields such as healthcare, finance, customer support, and manufacturing. For example, in healthcare, these systems can assist in diagnosing diseases and recommending treatment plans based on a patient’s symptoms and medical history.
In conclusion, the development of knowledge-based AI systems is a complex and iterative process. By harnessing the power of artificial intelligence and expert knowledge, these systems have the potential to revolutionize various industries and improve decision-making processes.
Machine Learning in Knowledge-Based AI Systems
In the field of artificial intelligence (AI), machine learning plays a crucial role in powering knowledge-based systems. These systems, known as expert systems, utilize a combination of human expertise and machine learning algorithms to solve complex problems and make informed decisions.
Expert systems are designed to mimic human intelligence by capturing the knowledge and decision-making processes of human experts in a specific domain. They rely on a knowledge base, which contains a vast amount of domain-specific information, and a set of rules that govern how this knowledge is applied.
Advantages of Machine Learning in Expert Systems
The integration of machine learning algorithms in expert systems brings several advantages:
- Improved Decision-Making: By leveraging machine learning techniques, expert systems can learn from past experiences and continuously refine their knowledge base. This enables them to make more accurate and reliable decisions over time, leading to improved outcomes.
- Scalability: Machine learning algorithms allow expert systems to handle large amounts of data and adapt to changing circumstances. This scalability is crucial in domains where the volume of information is vast and constantly evolving.
- Efficiency: Expert systems powered by machine learning can automate complex tasks, reducing the need for human intervention. This increases operational efficiency and frees up human experts to focus on higher-level tasks.
Applications of Machine Learning in Expert Systems
Machine learning has found applications in various knowledge-based AI systems, such as:
In conclusion, machine learning plays a vital role in enhancing the capabilities of knowledge-based AI systems. Its integration allows expert systems to make better decisions, handle large volumes of data, and automate complex tasks, leading to improved outcomes and operational efficiency.
Natural Language Processing in Knowledge-Based AI Systems
One of the key components of a knowledge-based AI system is natural language processing (NLP). NLP is a branch of artificial intelligence that focuses on the interaction between computers and humans through natural language.
Knowledge-based AI systems powered by NLP have the ability to understand, interpret, and generate human language. This enables these systems to communicate with users in a way that is more intuitive and user-friendly.
NLP in knowledge-based AI systems helps to bridge the gap between human language and machine language, allowing users to interact with the system using their natural language instead of having to learn a new programming language or interface. This makes the systems more accessible to a wider range of users and allows for easier adoption and use.
With NLP, knowledge-based AI systems can perform tasks such as understanding and responding to user queries, extracting relevant information from large amounts of text, and generating human-like responses. These systems can also be trained to understand the context and nuances of language, making them capable of providing more accurate and relevant answers or suggestions.
For example, an expert system powered by NLP could be used in a healthcare setting to help doctors diagnose patients. The system could analyze the patient’s symptoms and medical history, and then ask the doctor relevant questions to gather more information. Based on this input, the system could provide a list of possible diagnoses and suggest further tests or treatments.
In summary, NLP plays a crucial role in knowledge-based AI systems by enabling them to understand and generate human language. This enhances the usability and effectiveness of these systems in a wide range of applications, from healthcare to customer service. The advancements in NLP continue to push the boundaries of what these systems can achieve, making them a valuable tool in the field of artificial intelligence.
Knowledge Representation in Knowledge-Based AI Systems
Intelligence is at the heart of AI-powered systems, and the ability to represent and use knowledge effectively is crucial in achieving intelligent behavior. In knowledge-based AI systems, knowledge representation plays a key role in organizing and structuring information for the system to reason and make decisions.
Knowledge representation involves capturing and organizing knowledge in a format that is understandable and usable by an artificial intelligence system. It provides a way to store and model information, allowing the system to access and manipulate the knowledge to solve complex problems or perform specific tasks.
AI-Powered Knowledge Representation Methods
Artificial intelligence systems use various techniques for knowledge representation, depending on the nature of the problem domain and the specific requirements of the system. Some common methods include:
- Logic-based representation: This approach uses logical formalisms, such as rules and predicates, to represent knowledge. It allows for precise reasoning and inference based on logical principles.
- Semantic networks: These represent knowledge as nodes and links, where nodes represent concepts or entities, and links represent relationships between them. Semantic networks enable the system to capture complex relationships and dependencies.
- Frames: Frames represent knowledge as structured entities with slots and fillers. Each frame represents a specific concept or object, and slots contain properties or attributes of the concept. Frames help in capturing hierarchical relationships and inheritance.
- Ontologies: Ontologies provide a formal and explicit representation of concepts and their relationships in a domain. They allow for shared and standardized knowledge representation across different systems and domains.
Applications of Knowledge-Based AI Systems
Knowledge-based AI systems find applications in various domains, including:
- Healthcare: Intelligent systems can assist in diagnosis, treatment, and monitoring of diseases by leveraging medical knowledge and patient data.
- Finance: AI-powered systems can analyze financial data and provide insights for investment decisions, risk assessment, and fraud detection.
- Customer service: Chatbots and virtual assistants use knowledge-based systems to understand and respond to customer queries and provide personalized recommendations.
- Manufacturing: AI systems can optimize production processes, predict equipment failures, and improve quality control by utilizing knowledge about manufacturing processes.
- Education: Intelligent tutoring systems use knowledge representation to provide personalized and adaptive learning experiences to students.
In conclusion, knowledge representation is a critical component in knowledge-based AI systems. It enables the system to organize, access, and manipulate knowledge to make intelligent decisions and solve complex problems in various domains.
AI-Powered Expert Systems
AI-powered expert systems combine the capabilities of artificial intelligence (AI) and knowledge-based systems to provide advanced problem-solving and decision-making abilities. These systems are designed to mimic the knowledge and expertise of human experts in specific domains and can effectively solve complex problems, provide accurate recommendations, and assist in decision-making processes.
An AI-powered expert system typically consists of two main components: a knowledge base and an inference engine. The knowledge base contains a vast amount of domain-specific information and rules that represent the expertise of human experts. The inference engine uses AI techniques, such as machine learning or natural language processing, to analyze and reason with the information in the knowledge base.
Advantages of AI-Powered Expert Systems
AI-powered expert systems offer several advantages over traditional problem-solving approaches:
- Accuracy: These systems can provide highly accurate and consistent solutions, as they are based on well-defined rules and extensive domain knowledge.
- Speed: AI-powered expert systems can quickly analyze large amounts of data and generate solutions or recommendations within seconds, saving valuable time.
- Scalability: These systems can handle a wide range of complex problems and can be easily scaled to accommodate larger datasets or more advanced domains.
- Availability: AI-powered expert systems are available 24/7 and can provide consistent and reliable assistance, eliminating the need for manual intervention.
- Cost-effective: Once developed, these systems can greatly reduce the need for human experts, leading to significant cost savings.
Applications of AI-Powered Expert Systems
AI-powered expert systems have a wide range of applications across various industries:
These are just a few examples of how AI-powered expert systems are revolutionizing industries by providing advanced problem-solving capabilities and improving decision-making processes.
Examples of AI-Powered Expert Systems
AI-powered expert systems are a type of knowledge-based system that use artificial intelligence to provide intelligent and specialized advice or solutions in specific domains. Here are a few examples of AI-powered expert systems:
MYCIN is one of the earliest and most well-known examples of an AI-powered expert system. It was developed at Stanford University in the 1970s and was designed to assist physicians in diagnosing and treating bacterial infections. MYCIN used a knowledge base of rules and an inference engine to reason and provide recommendations for antibiotics based on patient symptoms and laboratory test results.
Dendral was an AI-powered expert system developed at Stanford University in the 1960s. It was designed to analyze mass spectrometry data and identify the chemical structure of organic compounds. Dendral used a knowledge base of chemical rules and heuristics to interpret the data and generate hypotheses about the compound’s structure. It played a key role in demonstrating the potential of expert systems in scientific domains.
3. Deep Blue
Deep Blue was an AI-powered expert system developed by IBM to play chess. It famously defeated world chess champion Garry Kasparov in 1997. Deep Blue used advanced search algorithms, evaluation functions, and an extensive database of chess positions to make intelligent decisions and determine the best move in a given position. Its success demonstrated the power of AI-powered expert systems in complex strategic domains.
Watson is an AI-powered expert system developed by IBM. It gained fame by winning the television game show Jeopardy! in 2011. Watson uses natural language processing, machine learning, and an extensive knowledge base to understand and answer questions posed in natural language. It has been applied to various domains, including healthcare, finance, and customer service, demonstrating the versatility of AI-powered expert systems.
These examples illustrate the diverse range of applications for AI-powered expert systems, from medical diagnosis to scientific analysis and strategic decision-making. They showcase the potential of combining artificial intelligence and expert knowledge to solve complex problems and provide valuable insights and recommendations.
IBM Watson: An AI-Powered Expert System
IBM Watson is a highly advanced and sophisticated knowledge-based system that utilizes artificial intelligence (AI) to provide expert-level solutions and insights. With its AI-powered capabilities, Watson is capable of analyzing vast amounts of data, understanding natural language, and generating meaningful and accurate responses in real-time.
As an expert system, IBM Watson is designed to mimic human expertise and decision-making processes. It is built upon a knowledge base that includes a wide range of information from various domains, such as medicine, finance, and law. By leveraging its vast knowledge base, Watson is capable of understanding complex problems and providing expert-level recommendations.
Advantages of IBM Watson
1. Speed and Efficiency: IBM Watson can process and analyze massive amounts of data in a fraction of the time it would take a human expert to do so. This speed and efficiency enable organizations to make faster and more informed decisions.
2. Accuracy and Precision: Watson’s AI-powered algorithms and machine learning capabilities ensure that the system can provide highly accurate and precise answers. It can take into account a variety of factors and variables to generate the most relevant insights.
3. Scalability: IBM Watson’s cloud-based architecture allows for easy scalability, making it suitable for organizations of all sizes. It can handle increasing amounts of data without compromising performance or accuracy.
Applications of IBM Watson
1. Healthcare: Watson is being used in the healthcare industry to assist doctors in diagnosing and treating diseases. It can analyze patient data, medical literature, and research papers to provide personalized treatment recommendations.
2. Finance: Watson is also being used in the financial industry to analyze market data, monitor trading patterns, and make investment recommendations. Its advanced algorithms can help identify potential risks and opportunities in real-time.
3. Customer Service: Watson’s natural language processing capabilities make it an ideal solution for customer service applications. It can understand and respond to customer inquiries, provide product recommendations, and assist with problem-solving.
IBM Watson is a prime example of how AI-powered expert systems can revolutionize industries and enhance decision-making processes. Its ability to analyze vast amounts of data, understand natural language, and provide expert-level insights makes it a valuable asset for organizations across various domains. With continual advancements in AI technology, Watson’s capabilities are expected to expand even further, unlocking new possibilities and applications.
DeepMind’s AlphaGo: An AI-Powered Expert System for Go
AlphaGo , developed by DeepMind , is a groundbreaking artificial intelligence (AI) system that is powered by advanced machine learning algorithms. It is specifically designed to play the complex board game Go, which requires deep strategic thinking and mastery of intricate patterns.
AlphaGo is an example of a knowledge-based expert system that combines the power of AI and expert human knowledge to achieve remarkable results. It utilizes a combination of algorithms, neural networks, and deep learning to analyze the vast database of Go games, study different strategies, and make highly informed decisions.
Through an iterative training process, AlphaGo learns from both human gameplay and self-play, continuously improving its performance and surpassing human levels of play. By analyzing millions of positions and moves, it develops a deep understanding of the game and can anticipate its opponent’s moves ahead.
The success of AlphaGo in defeating a world champion Go player in 2016 was a major milestone in AI research, as the game was previously considered too complex for computers to master due to its vast number of possible moves. AlphaGo showcased the potential of AI-powered expert systems and their ability to tackle complex problems that require advanced decision-making and pattern recognition.
Aside from its significance in the world of Go, the techniques and algorithms used in AlphaGo have also been applied to other domains, such as drug discovery, protein folding, and autonomous driving. This demonstrates the versatility and potential of AI-powered expert systems in various fields where knowledge-based decision-making is crucial.
In summary, AlphaGo is a groundbreaking AI-powered expert system that has demonstrated the power of combining artificial intelligence with expert human knowledge. Its success in mastering the complex game of Go serves as a testament to the capabilities of AI and its potential to revolutionize various industries.
Siri: An AI-Powered Expert System for Virtual Assistance
Siri is an ai-powered expert system that utilizes knowledge-based intelligence to provide virtual assistance to users. Developed by Apple, Siri is an artificial intelligence system that has revolutionized the way we interact with our devices.
How does Siri work?
Siri operates by processing natural language and utilizing machine learning algorithms to understand user input. It then leverages its vast knowledge base to provide accurate and relevant responses to user queries. Siri’s knowledge base is continuously updated and improved by Apple to ensure that it stays up-to-date with the latest information.
Siri can perform a wide range of tasks, from setting reminders and sending messages to providing weather updates and answering general knowledge questions. It can also integrate with various apps and services on a user’s device, allowing for seamless interaction and greater convenience.
The advantages of Siri
Siri offers several benefits as an ai-powered expert system for virtual assistance.
Firstly, Siri provides a user-friendly and intuitive interface for interacting with devices. Users can simply ask Siri a question or give a command using natural language, without needing to navigate through menus or complex settings.
Secondly, Siri’s ability to understand and interpret natural language enables it to provide personalized and context-aware responses. It can adapt to individual users’ preferences and learn from their interactions to enhance the user experience over time.
Lastly, Siri’s integration with various apps and services expands its capabilities and allows for seamless multitasking. Users can use Siri to perform tasks across multiple apps and services without needing to switch between them manually.
In conclusion, Siri is a powerful ai-powered expert system that combines artificial intelligence, knowledge-based intelligence, and natural language processing to provide virtual assistance. Its ability to understand natural language, adapt to user preferences, and integrate with various apps makes it a valuable tool for enhancing productivity and convenience.
AFFINImeter: An AI-Powered Expert System for Chemical Analysis
Chemical analysis plays a crucial role in various industries, including pharmaceuticals, biotechnology, and materials science. To ensure accurate and efficient analysis, experts in these fields rely on advanced tools and techniques. One such tool is AFFINImeter, an AI-powered expert system specifically designed for chemical analysis.
AFFINImeter is a knowledge-based system that utilizes artificial intelligence to provide in-depth analysis and interpretation of complex chemical data. It combines expert knowledge with machine learning algorithms to deliver accurate and reliable results.
The AI-powered capabilities of AFFINImeter enable it to process large datasets and identify patterns that might be missed by human experts. By analyzing these patterns, the system can provide valuable insights and predictions, thereby facilitating decision-making processes.
One of the distinct advantages of AFFINImeter is its ability to handle a wide range of chemical analysis techniques, including spectroscopy, chromatography, and titration. The system’s extensive knowledge base allows it to adapt to different experimental setups and provide tailored solutions.
AFFINImeter also offers a user-friendly interface, making it accessible to both experts and non-experts in the field of chemical analysis. The system can be easily navigated, and the results are presented in a clear and intuitive manner.
In summary, AFFINImeter is an AI-powered expert system that leverages artificial intelligence and knowledge-based algorithms to provide accurate and efficient chemical analysis. Its ability to process complex data, identify patterns, and offer valuable insights makes it a valuable tool in various industries.
What is an expert system in AI?
An expert system in AI is a computer program that uses knowledge and reasoning techniques to solve complex problems in a specific domain. It is designed to emulate the decision-making ability of a human expert.
What are the advantages of using an expert system in AI?
There are several advantages of using an expert system in AI. Firstly, it can provide consistent and reliable answers as it follows a predefined set of rules and logic. Secondly, it can store and access a vast amount of knowledge and expertise. Lastly, it can be used for complex problem-solving and decision-making tasks.
What are some applications of expert systems in AI?
Expert systems in AI have a wide range of applications. Some common examples include medical diagnosis, financial forecasting, industrial process control, and troubleshooting complex systems. They can also be utilized in areas such as customer support, legal advice, and quality assurance.
Can you provide an example of an AI-powered expert system?
One example of an AI-powered expert system is IBM Watson. It is a cognitive computing system that has been used in various domains, including healthcare, finance, and customer service. Watson is capable of understanding natural language, analyzing vast amounts of data, and providing detailed answers to complex questions.
How is a knowledge-based AI system different from a traditional AI system?
A knowledge-based AI system, such as an expert system, relies on a knowledge base that contains explicit, expert knowledge about a specific domain. It uses reasoning techniques to derive conclusions and make decisions. In contrast, a traditional AI system may use machine learning algorithms to learn patterns and make decisions based on statistical analysis of data.
An expert system in AI is a computer system that uses artificial intelligence technologies to mimic the decision-making ability of a human expert in a specific domain. It is a knowledge-based system that utilizes a set of rules or inference engines to solve complex problems.
What are the advantages of using expert systems in AI?
There are several advantages of using expert systems in AI. Firstly, they can provide consistent and accurate decision-making, as they are based on known facts and rules. Secondly, they can help in capturing and preserving expert knowledge, which can be useful in training new experts. Thirdly, they can improve productivity and efficiency by automating complex tasks. Lastly, they can be easily updated to incorporate new knowledge or rules.
Related posts:
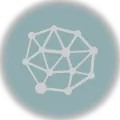
About the author
Cohere: Bridging Language and AI for a Smarter Future
Microsoft office 365 ai. microsoft copilot, understanding the distinctions between artificial intelligence and human intelligence, exploring the impact of artificial intelligence on discrimination in insurance pricing and underwriting.

Let's Talk AI
Expert Systems in AI: Pioneering Applications, Challenges, and Lasting Legacy

Artificial Intelligence (AI) has undergone remarkable evolution since its inception, with one of its early milestones being the development and application of Expert Systems.
In the 1960s and 1970s, researchers embarked on a pioneering journey to create intelligent systems capable of emulating human expertise in specific domains. This era witnessed the birth of Expert Systems, which represented a paradigm shift in AI, focusing on capturing and formalizing human knowledge to solve complex problems.
Early AI applications, particularly Expert Systems, laid the foundation for subsequent advancements in the field, leaving an indelible mark on the trajectory of artificial intelligence .
Expert Systems, characterized by rule-based reasoning and sophisticated knowledge representation, emerged as a response to the need for intelligent systems capable of decision-making and problem-solving within specific domains.
These systems leveraged the expertise of human professionals, translating it into a set of rules and logical structures. The groundbreaking work on projects like Dendral and Mycin at Stanford exemplified the potential of Expert Systems, particularly in fields such as medical diagnosis.
The evolution of these systems was propelled by the collaborative efforts of influential figures like Edward Feigenbaum , Joshua Lederberg , and Bruce Buchanan , who played pivotal roles in shaping the landscape of early AI applications.
As we delve into the history of Expert Systems, it becomes evident that these early AI applications played a vital role in expanding the horizons of artificial intelligence.
The significance of Expert Systems lies not only in their specific applications, such as medical diagnosis and industrial process control but also in their contribution to the theoretical and practical foundations of AI. This exploration into the genesis of Expert Systems sheds light on their characteristics, development, and the challenges they pose, setting the stage for a comprehensive understanding of their impact on the broader field of artificial intelligence.
In this article
Development of Expert Systems
The development of Expert Systems marks a crucial phase in the evolution of Artificial Intelligence (AI), with roots tracing back to the 1960s and 1970s. During this period, researchers aimed to create intelligent systems that could mimic human expertise and decision-making processes within specific domains.
The emergence of Expert Systems was closely tied to the Stanford Heuristic Programming Project , which sought to develop rule-based systems capable of problem-solving. This era saw the birth of pioneering projects like Dendral and Mycin , which focused on applying AI techniques to chemical analysis and medical diagnosis, respectively. These early efforts laid the foundation for rule-based reasoning, a hallmark characteristic of Expert Systems.
Key contributors to the development of Expert Systems include visionaries like Edward Feigenbaum, Joshua Lederberg, and Bruce Buchanan. Edward Feigenbaum, often regarded as the “father of expert systems,” played a pivotal role in establishing the field. His work emphasized the importance of capturing human expertise in the form of rules and representations that could be processed by computers.
The Dendral project, led by Joshua Lederberg and his team, showcased the application of rule-based reasoning in interpreting mass spectrometry data for organic compound identification. Bruce Buchanan, another influential figure, contributed significantly to the field’s theoretical foundations, emphasizing knowledge representation and inference mechanisms.
The hallmark of Expert Systems lies in their rule-based reasoning approach. These systems codify expert knowledge into a set of rules, establishing a framework for decision-making and problem-solving.
The development of inference engines, responsible for drawing logical conclusions from the established rules, played a critical role in enhancing the capabilities of Expert Systems. As these systems evolved, so did their ability to emulate human decision-making processes, making them valuable tools in various domains such as medicine, finance, and industrial control.
The development of Expert Systems during this early period not only demonstrated the feasibility of AI applications but also laid the groundwork for subsequent advancements in knowledge-based systems and artificial intelligence as a whole.
Characteristics of Expert Systems
Expert Systems, as a distinct subset of Artificial Intelligence (AI), possess distinctive characteristics that set them apart in their approach to problem-solving and decision-making.
One defining feature is their reliance on rule-based reasoning. Expert Systems codify human expertise into a set of rules that encapsulate the knowledge and decision-making processes of domain experts. These rules serve as the foundation for the system’s ability to analyze information, draw inferences, and make decisions within a specific domain.
The rule-based approach allows for a transparent and structured representation of knowledge, making it accessible to both human users and the underlying computational components.
Knowledge representation is another critical characteristic of Expert Systems. To emulate human expertise effectively, these systems employ various models and structures to represent domain-specific knowledge. This representation may include facts, heuristics, and relationships among different elements within the domain.
The choice of an appropriate knowledge representation scheme is essential for capturing the intricacies of expert knowledge and ensuring the system’s ability to reason effectively. Common knowledge representation techniques include semantic networks, frames, and production rules.
The Inference Engine serves as the computational heart of Expert Systems. This component is responsible for applying the rules and knowledge encoded in the system to make inferences and draw conclusions.
The inference engine utilizes logical reasoning to process input data, match it against the established rules, and produce meaningful outputs. It is this inferential capability that allows Expert Systems to analyze complex situations, provide explanations for their decisions, and adapt to new information.
User interface design is another noteworthy characteristic of Expert Systems. Given their application in various domains, including those outside the realm of computer science, an intuitive and user-friendly interface is crucial for effective interaction.
A well-designed user interface facilitates communication between the human user and the Expert System, allowing users to input queries, receive explanations for decisions, and understand the reasoning behind the system’s recommendations.
In summary, the characteristics of rule-based reasoning, knowledge representation, inference engines, and user interfaces collectively define Expert Systems. These features enable these systems to emulate human expertise in specific domains, making them valuable tools for decision support and problem-solving across various fields.
Early Applications of Expert Systems
The early applications of Expert Systems marked a groundbreaking phase in the utilization of artificial intelligence for problem-solving and decision-making within specific domains. These applications demonstrated the adaptability and potential impact of Expert Systems across various fields. Some notable early applications include:
Medical Diagnosis:
Dendral and Mycin Systems: Dendral, developed in the 1960s, was one of the earliest Expert Systems designed to interpret mass spectrometry data for organic compound identification. Mycin, developed in the 1970s, focused on medical diagnosis and treatment recommendations for bacterial infections. Mycin demonstrated the capability of Expert Systems to analyze patient symptoms, laboratory results, and medical knowledge to provide accurate and timely diagnoses.
Industrial Process Control:
Quality Control Applications: Expert Systems found applications in industrial settings for monitoring and controlling manufacturing processes. These systems were designed to ensure product quality, optimize production parameters, and troubleshoot issues in real-time. The ability to emulate human expertise in identifying and rectifying problems contributed to improved efficiency and reduced downtime in manufacturing.
Financial Analysis:
Investment Advisory Systems: Expert Systems were employed in the financial sector to provide investment advice based on market trends, historical data, and financial indicators. These systems assisted users in making informed decisions by leveraging the expertise of financial analysts encoded in rule-based systems. Additionally, Expert Systems were applied in risk assessment and management, aiding in the evaluation of potential financial risks.
Educational Systems:
Tutoring and Learning Systems: Expert Systems were developed to provide personalized tutoring and learning experiences. These systems could adapt to the individual needs of students, offering targeted feedback and guidance in various subjects. By incorporating expert knowledge in specific domains, these educational applications aimed to enhance the learning process and address individual learning styles.
Legal Decision Support:
Legal Expert Systems: In the legal domain, Expert Systems were utilized for legal decision support. These systems could assist lawyers and legal professionals in analyzing case law, statutes, and precedents to provide recommendations for legal strategies.
The rule-based reasoning of Expert Systems facilitated the systematic evaluation of legal scenarios.
While these early applications showcased the potential of Expert Systems, they also highlighted challenges such as the need for extensive knowledge acquisition, limited adaptability to novel situations, and difficulties in handling ambiguous information. Despite these challenges, the success of early applications laid the groundwork for the continued development and evolution of Expert Systems, influencing subsequent AI research and applications.
Challenges and Limitations
The development and deployment of Expert Systems, despite their early success and pioneering contributions to artificial intelligence, were not without challenges and limitations. Understanding these challenges is crucial for gaining insights into the strengths and weaknesses of this technology:
Knowledge Acquisition:
One of the primary challenges faced by Expert Systems was the acquisition and representation of expert knowledge. The process of extracting, formalizing, and encoding the expertise of human professionals into a knowledge base was often time-consuming and resource-intensive. The dependence on domain experts raised concerns about the completeness and accuracy of the knowledge captured, as well as the potential biases introduced during the knowledge acquisition phase.
Lack of Common Sense Reasoning:
Expert Systems struggled with common sense reasoning, meaning they often had difficulty handling situations that required contextual understanding or implicit knowledge. These systems relied heavily on explicit rules and struggled in scenarios where human intuition or common sense was essential. As a result, they were limited in their ability to adapt to unpredictable or novel situations that did not align with pre-established rules.
Limited Scope of Applicability:
The effectiveness of Expert Systems was often confined to specific, well-defined domains. These systems were experts in narrow areas but lacked the generalization capabilities seen in human intelligence. Adapting Expert Systems to new domains or expanding their knowledge base to cover a broader spectrum of topics posed significant challenges, making them less versatile compared to human experts.
Maintenance and Adaptability:
The dynamic nature of knowledge and the continuous evolution of technology and information posed challenges in maintaining and updating Expert Systems. Systems that relied on static knowledge bases struggled to keep pace with changes in their respective domains. The need for regular updates and adaptations made the maintenance of Expert Systems a complex and ongoing task.
Handling Ambiguity and Uncertainty:
Expert Systems faced difficulties in dealing with ambiguous or uncertain information. Real-world scenarios often involve incomplete or imprecise data, and the rigid rule-based nature of Expert Systems made them less adept at handling ambiguity. Developing mechanisms to incorporate and reason with uncertain information posed a significant challenge.
Ethical and Legal Considerations:
As Expert Systems began to play roles in critical areas like medical diagnosis and legal decision support, ethical and legal considerations became paramount. Issues related to accountability, transparency, and the potential consequences of relying on automated systems for decision-making raised concerns among practitioners, policymakers, and the general public.
Acknowledging these challenges and limitations prompted researchers to explore alternative AI approaches and paved the way for the evolution of more advanced technologies that could address these issues. The lessons learned from the challenges of Expert Systems continue to influence the design and development of contemporary AI systems.
Impact and Legacy
The impact and legacy of Expert Systems in the realm of artificial intelligence have been profound, shaping the trajectory of AI development and influencing subsequent generations of intelligent systems. As one of the earliest practical applications of AI, Expert Systems played a pivotal role in demonstrating the potential of machine-based decision-making and problem-solving within specific domains. The impact of Expert Systems can be understood through several key aspects:
Pioneering Role in AI Development:
Expert Systems represented a pioneering leap in the practical application of AI. Their success in domains like medical diagnosis, industrial process control, and financial analysis showcased the ability of machines to emulate human expertise and decision-making processes. This success served as a catalyst for further exploration and development of AI technologies, inspiring researchers to delve into new possibilities and applications.
Shaping Subsequent AI Research:
The early success and challenges faced by Expert Systems significantly influenced the direction of AI research. Researchers drew valuable insights from the rule-based reasoning and knowledge representation methodologies employed in Expert Systems. Lessons learned from these systems informed the development of subsequent AI techniques, leading to the integration of approaches like machine learning and neural networks.
Contributing to Knowledge-Based Systems:
Expert Systems laid the foundation for knowledge-based systems, emphasizing the importance of codifying and structuring human expertise. This legacy persists in contemporary AI applications that leverage knowledge representation techniques to enhance decision-making processes. The emphasis on explicit knowledge representation remains relevant in systems designed for explainability and transparency.
Evolution of Hybrid Approaches:
While Expert Systems had their limitations, they contributed to the evolution of hybrid AI approaches. Researchers recognized the need to integrate different AI techniques to overcome the challenges posed by rigid rule-based systems. This led to the development of hybrid systems that combine rule-based reasoning with machine learning algorithms, enhancing adaptability and performance.
Applications in Decision Support Systems:
The legacy of Expert Systems lives on in the design and implementation of decision support systems across various industries. The ability to provide reasoned explanations for decisions, interpret complex data, and offer recommendations has influenced the development of intelligent decision-support tools in areas such as healthcare, finance, and logistics.
Influence on Practical AI Applications:
The impact of Expert Systems extends to practical applications that have become integral parts of our daily lives. From voice-activated virtual assistants to personalized recommendation systems, the principles of Expert Systems continue to shape the design and functionality of contemporary AI applications.
In conclusion, the impact and legacy of Expert Systems are evident in the historical context of AI development and the ongoing evolution of intelligent systems. While Expert Systems may have faced challenges, their successes and lessons learned have left an indelible mark on the field, contributing to the diverse landscape of AI technologies we encounter today.
In conclusion, the era of Expert Systems stands as a crucial chapter in the rich history of artificial intelligence. These early applications exemplified the audacious attempt to capture and emulate human expertise, marking a transformative moment when AI transitioned from theoretical concepts to practical solutions. While Expert Systems faced challenges, particularly in knowledge acquisition, common sense reasoning, and adaptability, their impact reverberates through the corridors of AI development, influencing subsequent research and shaping the evolution of intelligent systems.
The legacy of Expert Systems extends far beyond their specific applications, contributing to the conceptual foundations of knowledge-based systems and decision-support tools. Lessons learned from their successes and limitations have spurred innovation, leading to the integration of diverse AI approaches and the development of more sophisticated systems. Expert Systems have left an enduring imprint on the landscape of artificial intelligence, serving as trailblazers that paved the way for the myriad applications and advancements witnessed in contemporary AI. As we navigate the intricate realms of AI technologies, the enduring influence of Expert Systems remains a testament to the pioneering spirit that continues to propel the field forward.
Related Questions
What is an expert system?
An expert system is a computer program or artificial intelligence application that emulates the decision-making ability of a human expert in a specific domain. It uses a knowledge base of expert-derived rules and logical reasoning to solve complex problems, make decisions, or provide recommendations within its designated area of expertise.
What is one example of an expert system?
One example of an expert system is Mycin, a pioneering AI application developed in the 1970s for medical diagnosis and treatment recommendations for bacterial infections.
What are the two types of expert systems?
The two types of expert systems are rule-based expert systems and knowledge-based expert systems. Rule-based systems operate on predefined rules, while knowledge-based systems use a broader range of symbolic representations to capture and manage expert knowledge in specific domains.
Share this:
- Share on Tumblr

[…] Read This Also: Expert Systems in AI: Pioneering Applications, Challenges, and Lasting Legacy […]
[…] Read this also: Expert Systems in AI: Pioneering Applications, Challenges, and Lasting Legacy […]
Leave a Reply Cancel reply
You may also like.

What is Advanced Speech Recognition Technology?

Empowering the Future: Unleashing the History of AI-Driven Computer Vision

Navigating the AI Winter Phenomenon: Lessons Learned from History and the Current AI Renaissance

Unleashing the Power of Early AI Languages: LISP, Prolog, AIML, and Smalltalk – A Legacy in Modern AI Development
Subscribe to our newsletter, add probo ai to your homescreen, discover more from probo ai.
Subscribe now to keep reading and get access to the full archive.
Type your email…
Continue reading
- Trending Now
- Foundational Courses
- Data Science
- Practice Problem
- Machine Learning
- System Design
- DevOps Tutorial
- Saving a machine learning Model
- Multiple Linear Regression using R
- Introduction to Artificial Neural Network | Set 2
- Pattern Recognition | Introduction
- Applications of Machine Learning
- Introduction To Machine Learning using Python
- Classifying data using Support Vector Machines(SVMs) in R
- Different Types of Clustering Algorithm
- An introduction to Machine Learning
- Gaussian Mixture Model
- ML | Using SVM to perform classification on a non-linear dataset
- ML | Locally weighted Linear Regression
- Artificial Intelligence in Robotics
- Cross Validation in Machine Learning
- Unsupervised Learning
- ML | Introduction to Data in Machine Learning
- What is Machine Learning?
- Supervised and Unsupervised learning
- ML | Understanding Data Processing
Expert Systems
Artificial Intelligence is a piece of software that simulates the behaviour and judgement of a human or an organization that has experts in a particular domain is known as an expert system. It does this by acquiring relevant knowledge from its knowledge base and interpreting it according to the user’s problem. The data in the knowledge base is added by humans that are expert in a particular domain and this software is used by a non-expert user to acquire some information. It is widely used in many areas such as medical diagnosis, accounting, coding, games etc.
An expert system is AI software that uses knowledge stored in a knowledge base to solve problems that would usually require a human expert thus preserving a human expert’s knowledge in its knowledge base. They can advise users as well as provide explanations to them about how they reached a particular conclusion or advice. Knowledge Engineering is the term used to define the process of building an Expert System and its practitioners are called Knowledge Engineers . The primary role of a knowledge engineer is to make sure that the computer possesses all the knowledge required to solve a problem. The knowledge engineer must choose one or more forms in which to represent the required knowledge as a symbolic pattern in the memory of the computer.
Example : There are many examples of an expert system. Some of them are given below –
- MYCIN – One of the earliest expert systems based on backward chaining. It can identify various bacteria that can cause severe infections and can also recommend drugs based on the person’s weight.
- DENDRAL – It was an artificial intelligence-based expert system used for chemical analysis. It used a substance’s spectrographic data to predict its molecular structure.
- R1/XCON – It could select specific software to generate a computer system wished by the user.
- PXDES – It could easily determine the type and the degree of lung cancer in a patient based on the data.
- CaDet – It is a clinical support system that could identify cancer in its early stages in patients.
- DXplain – It was also a clinical support system that could suggest a variety of diseases based on the findings of the doctor.
Components of an Expert System :

Architecture of an Expert System
- Knowledge Base – The knowledge base represents facts and rules. It consists of knowledge in a particular domain as well as rules to solve a problem, procedures and intrinsic data relevant to the domain.
- Inference Engine – The function of the inference engine is to fetch the relevant knowledge from the knowledge base, interpret it and to find a solution relevant to the user’s problem. The inference engine acquires the rules from its knowledge base and applies them to the known facts to infer new facts. Inference engines can also include an explanation and debugging abilities.
- Knowledge Acquisition and Learning Module – The function of this component is to allow the expert system to acquire more and more knowledge from various sources and store it in the knowledge base.
- User Interface – This module makes it possible for a non-expert user to interact with the expert system and find a solution to the problem.
- Explanation Module – This module helps the expert system to give the user an explanation about how the expert system reached a particular conclusion.
The Inference Engine generally uses two strategies for acquiring knowledge from the Knowledge Base, namely –
Forward Chaining
Backward Chaining
Forward Chaining – Forward Chaining is a strategic process used by the Expert System to answer the questions – What will happen next. This strategy is mostly used for managing tasks like creating a conclusion, result or effect. Example – prediction or share market movement status.
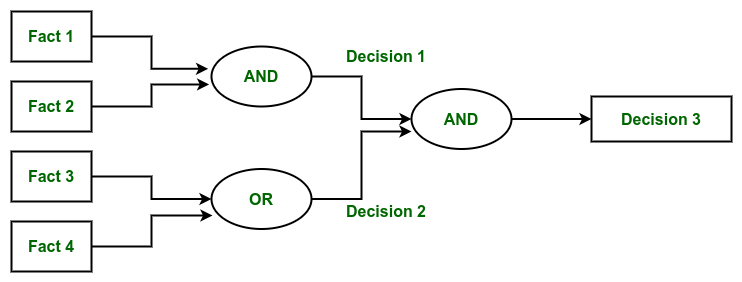
Backward Chaining – Backward Chaining is a strategy used by the Expert System to answer the questions – Why this has happened. This strategy is mostly used to find out the root cause or reason behind it, considering what has already happened. Example – diagnosis of stomach pain, blood cancer or dengue, etc.
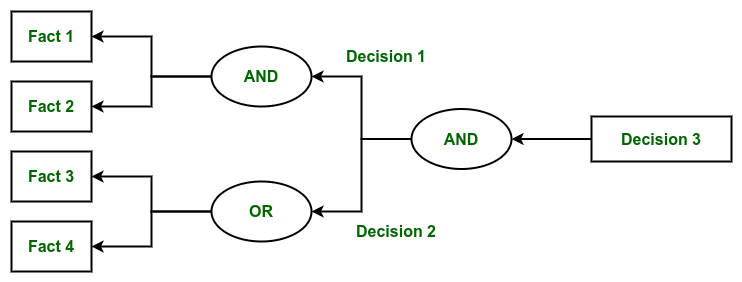
Characteristics of an Expert System :
- Human experts are perishable, but an expert system is permanent.
- It helps to distribute the expertise of a human.
- One expert system may contain knowledge from more than one human experts thus making the solutions more efficient.
- It decreases the cost of consulting an expert for various domains such as medical diagnosis.
- They use a knowledge base and inference engine.
- Expert systems can solve complex problems by deducing new facts through existing facts of knowledge, represented mostly as if-then rules rather than through conventional procedural code.
- Expert systems were among the first truly successful forms of artificial intelligence (AI) software.
Limitations :
- Do not have human-like decision-making power.
- Cannot possess human capabilities.
- Cannot produce correct result from less amount of knowledge.
- Requires excessive training.
Advantages :
- Low accessibility cost.
- Fast response.
- Not affected by emotions, unlike humans.
- Low error rate.
- Capable of explaining how they reached a solution.
Disadvantages :
- The expert system has no emotions.
- Common sense is the main issue of the expert system.
- It is developed for a specific domain.
- It needs to be updated manually. It does not learn itself.
- Not capable to explain the logic behind the decision.
Applications : The application of an expert system can be found in almost all areas of business or government. They include areas such as –
- Different types of medical diagnosis like internal medicine, blood diseases and show on.
- Diagnosis of the complex electronic and electromechanical system.
- Diagnosis of a software development project.
- Planning experiment in biology, chemistry and molecular genetics.
- Forecasting crop damage.
- Diagnosis of the diesel-electric locomotive system.
- Identification of chemical compound structure.
- Scheduling of customer order, computer resources and various manufacturing task.
- Assessment of geologic structure from dip meter logs.
- Assessment of space structure through satellite and robot.
- The design of VLSI system.
- Teaching students specialize task.
- Assessment of log including civil case evaluation, product liability etc.
Expert systems have evolved so much that they have started various debates about the fate of humanity in the face of such intelligence, with authors such as Nick Bostrom (Professor of Philosophy at Oxford University), pondering if computing power has transcended our ability to control it.
Please Login to comment...
Similar reads.
- Artificial Intelligence
- What are Tiktok AI Avatars?
- Poe Introduces A Price-per-message Revenue Model For AI Bot Creators
- Truecaller For Web Now Available For Android Users In India
- Google Introduces New AI-powered Vids App
- 30 OOPs Interview Questions and Answers (2024)
Improve your Coding Skills with Practice
What kind of Experience do you want to share?
What Are Expert Systems?
Last updated: March 18, 2024
- Artificial Intelligence
1. Introduction
Expert systems are a branch of artificial intelligence that aim to provide computerized decision-making capabilities similar to those of a human expert in a specific domain. They are designed to solve complex problems using a set of rules or algorithms that can mimic human reasoning processes.
In this tutorial, we’ll overview the expert systems, their components, working mechanisms, types, the process of building an expert system, their applications, and their advantages and limitations.
2. Overview
An expert system is an artificial intelligence system that performs a specific task with expert-like efficiency by applying predefined rules to analyze information and generate conclusions . The expert system can provide specialist advice or decision-making automation, assist in problem-solving, and help identify errors or risks.
They incorporate rules and algorithms that allow them to perform tasks and make decisions based on a knowledge base filled with expert knowledge, heuristics , and reasoning patterns. Expert systems are widely used in various industries, including healthcare, finance, engineering, and many others.
3. Components of Expert Systems
There are four main components of expert systems.
3.1. Knowledge Base
The knowledge base is the core of an expert system. It contains a set of rules, facts, and heuristics that represent the knowledge of a human expert in a specific domain. The domain expert with the required expertise and experience creates the knowledge base. It is encoded in a formal language that the expert system can understand.
3.2. Inference Engine
The inference engine is responsible for reasoning and decision-making. It applies the rules and procedures defined in the knowledge base to the input data and generates the required output. The inference engine uses various reasoning techniques, including backward and forward chaining, to deduce conclusions from the knowledge base.
3.3. User Interface
The user interface is the communication channel between the user and the expert system. It presents the questions, options, and recommendations generated by the inference engine in a user-friendly way. The user interface can be text-based or graphical, depending on the application.
3.4. Explanation Module
The explanation module is used to explain the reasoning process of the expert system. The explanation module explains how the expert system arrived at a conclusion or recommendation.
4. The Working Mechanism of Expert Systems
Expert systems work by combining the knowledge base, the inference engine, the user interface, and the explanation facility. The reasoning process begins with the user providing input data to the expert system through the user interface.
The inference engine then uses the rules and heuristics stored in the knowledge base to generate conclusions and recommendations based on the input data. The explanation facility provides the user with a detailed explanation of the reasoning process and how the expert system arrived at its conclusion:
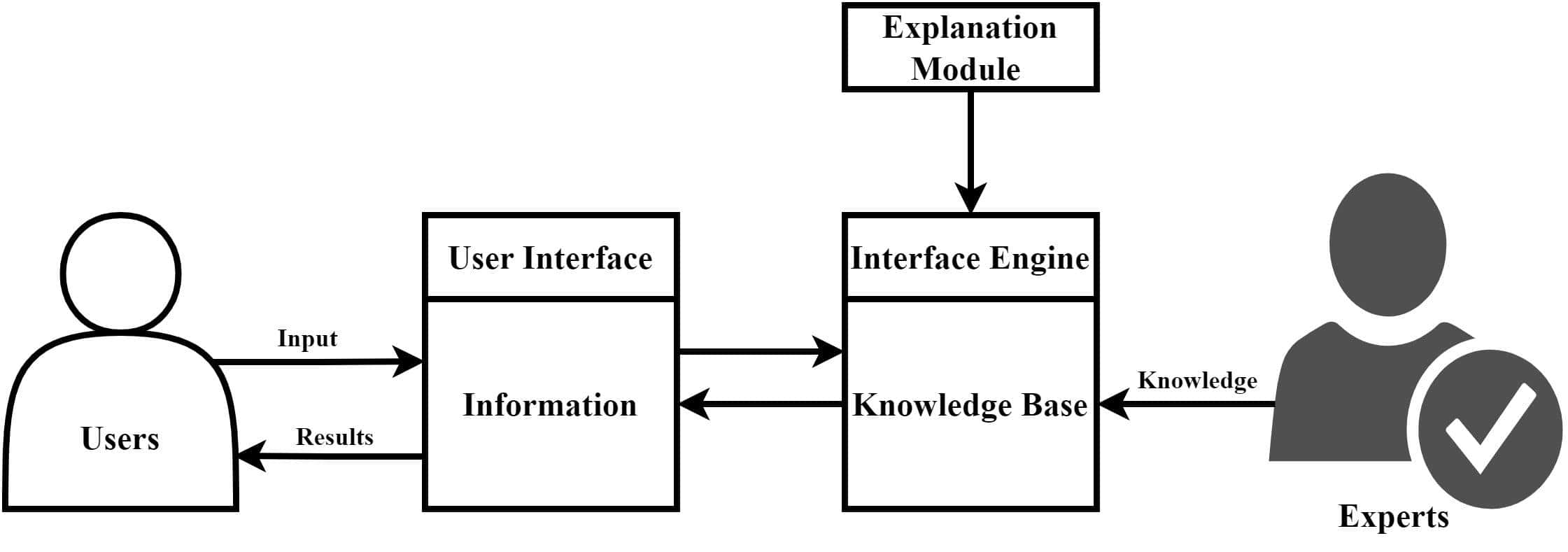
Expert systems gather and place information and facts in a knowledge base and integrate them with an inference engine. The inference engine uses two methods to acquire information from the knowledge base.
In forward chaining, the expert system reads and processes a set of facts to logically predict what will happen next. An example of this would be predicting stock market movements.
In backward chaining, the system reads and evaluates a set of information backward to reach a logical conclusion about a goal. For example, prediction in a medical diagnosis system.
5. Types of Expert Systems
There are various types of expert systems with their unique strengths and weaknesses, and they are used in various applications in multiple industries.
5.1. Rule-based Expert Systems
Rule-based expert systems are the most common type of expert system. They use a set of rules to reason about a problem and provide solutions or recommendations. These rules are created by human experts and are organized in a knowledge base.
5.2. Fuzzy Logic Expert Systems
Fuzzy logic expert systems use fuzzy logic to handle uncertainty and imprecision in data. Fuzzy logic is a mathematical framework that allows for degrees of truth instead of the traditional binary (true or false) approach. Fuzzy expert systems are used in product recommendation systems and image recognition applications.
5.3. Knowledge-based Expert Systems
Knowledge-based expert systems use a knowledge base that contains facts and rules about a specific domain. These systems are designed to mimic the problem-solving capabilities of human experts. They use a knowledge inference engine to explain the problem and provide solutions.
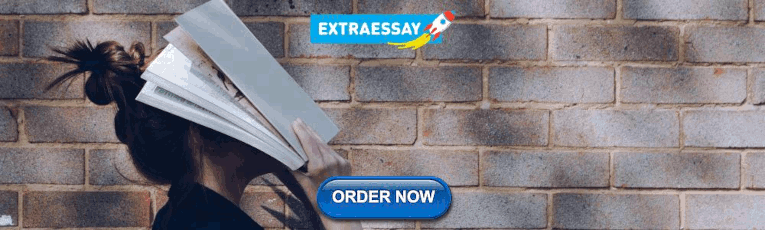
5.4. Neural Networks Expert Systems
Neural network expert systems are designed to learn from data by adjusting the weights of their connections between neurons . They are used in speech recognition, image classification, and natural language processing applications.
6. Development of Expert Systems

First, we acquire knowledge by gathering information from human experts. This process involves various techniques, including interviews, questionnaires, and observation. In the second step, we’ll transform acquired knowledge into a format the system can understand and use. This format may take various forms, such as rules, frames, semantic networks, or ontologies, depending on the nature of the knowledge and the system’s requirements.
In the third step, we’ll ensure that the knowledge in the expert system is accurate and complete. This can involve testing the expert system against known data or comparing its recommendations to those of human experts. Once validated, we’ll implement the system using an inference engine.
Finally, we maintain our expert system’s knowledge base by regularly updating it with new information. This process includes adding new rules, modifying existing ones, or removing outdated information. Overall, the knowledge engineering process is iterative, and each step may need to be revisited and refined as the development of the expert system progresses.
7. Application of Expert Systems
Expert systems have numerous applications in various industries, including healthcare, finance, engineering, and many others:
- Medical Diagnosis: The expert system can help medical professionals diagnose diseases, analyze symptoms, and provide treatment options based on medical history, symptoms, and test results. For example, MYCIN is an expert system for diagnosing bacterial infections
- Financial Planning: The expert system can assist in financial planning, such as investment strategy, retirement planning, and tax planning, based on a user’s financial situation and goals. For example, LenddoEFL is a digital financial inclusion platform that uses machine learning to evaluate the credit risk of individuals and small businesses
- Quality Control: The expert system can help identify defects or quality concerns by analyzing production data or testing results. An example is the DENDRAL expert system for chemical structure determination
- Customer Support: The expert system can help customers troubleshoot technical issues or provide product-related advice based on a user’s queries or inputs. An example expert system is XCON, used for configuring computer systems
8. Advantages and Limitations of Expert Systems
Let’s look at the advantages and limitations of expert systems:
9. Conclusion
In this tutorial, we learn about expert systems, their types, working mechanism, applications, advantages, and limitations.
Expert systems have become an integral part of many industries because they provide quick, accurate, and consistent decision-making automation . In addition, their ease of use and flexibility make them accessible to non-experts in the domain, reducing the dependence on human experts.
What are Expert Systems?

- February 12, 2024 Updated
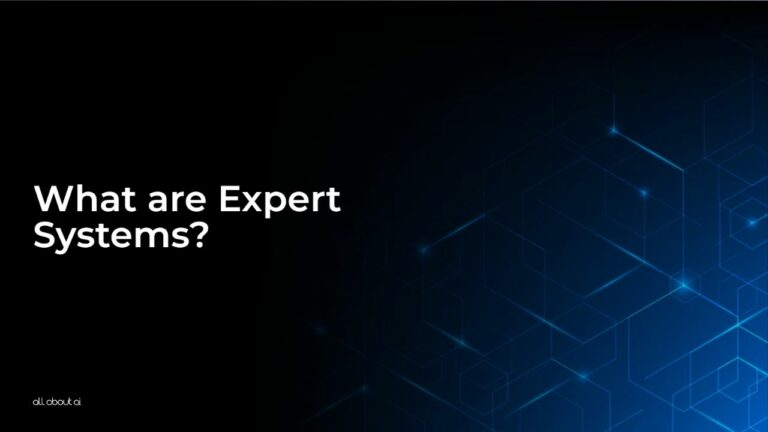
Expert systems, a branch of artificial intelligence (AI), are designed to emulate the decision-making capabilities of human experts within specific domains. These systems leverage detailed knowledge bases and sophisticated inference engines to process and analyze information, offering solutions, guidance, and advice akin to that provided by a human expert.
Their development marked a significant step forward in the quest to apply AI in solving complex problems across various fields, including medicine, finance, and environmental management.
In this article, we will discuss “What are Expert Systems?” in detail, along with its key characteristics, working procedure, and everything you want to know about it. So don’t stop! Keep reading the article written by AI Experts at All About AI .
What are Expert Systems: How AI Becomes an Expert Just Like Us!
Imagine you have a super smart robot friend who is really good at certain things, like being a doctor, a banker, or even helping to take care of the Earth. This robot friend is a type of smart computer program we call an “expert system.” It’s part of a big family of smart technologies known as artificial intelligence, or AI for short. AI is like the brain for robots and computers, helping them think and make decisions like humans do.
Expert systems are special because they can think and make decisions in specific areas really well, almost like how a real doctor knows a lot about medicine or how a banker understands money. They do this by using a big book of knowledge that has lots of information about one subject. They also have a smart engine, kind of like a detective, that looks at information, asks questions, and figures out solutions or gives advice just like a human expert would.
The Evolution of Expert Systems:
After understanding the basic concept of “What is Expert Systems?” let’s get started by reviewing its evolution. Expert systems have undergone a significant evolution since their inception in the 1970s, transitioning from academic curiosity to practical tools across multiple industries.
Early Beginnings:
The development of expert systems was initially driven by the desire to capture and replicate the decision-making process of human experts in a computerized form. This period saw the creation of foundational systems such as DENDRAL, designed for chemical analysis, and MYCIN, which focused on diagnosing bacterial infections and recommending antibiotics.
Notable Milestones:
The success of these early systems demonstrated the potential of AI to replicate and even surpass human expertise in certain domains . It paved the way for the development of more sophisticated systems capable of addressing a wider range of complex problems.
Widespread Adoption:
Throughout the 1980s and 1990s, the application of expert systems expanded significantly, finding utility in legal reasoning, financial analysis, and strategic planning, among other areas. This era solidified their role as valuable tools in enhancing decision-making processes.
Continuous Evolution:
The integration of machine learning and big data analytics has marked the latest phase in the evolution of expert systems, broadening their capabilities and applications. Today’s systems not only replicate human expertise but also learn and adapt over time, offering even more powerful and flexible solutions.
How Expert Systems Work?
After explaining “What is Expert Systems?” it’s the time to understand how do they work. Expert systems are designed to provide expert advice and make decisions in various fields, from medical diagnosis to financial analysis.

Here’s how they function:
Knowledge Base Construction:
Expert systems are built around a comprehensive knowledge base that contains specific information and rules about its domain of expertise. This database is compiled from the expertise of human specialists, encapsulating their knowledge in a structured format that the system can use.
Inference Engine Mechanism:
At the heart of an expert system is the inference engine, a crucial component that applies logical rules to the knowledge base to deduce new information or make decisions. It simulates the reasoning process of human experts by interpreting and processing the knowledge.
User Interface for Interaction:
Expert systems come equipped with a user-friendly interface that allows users to input queries and receive advice or decisions. This interface is designed to be intuitive, enabling users to interact effectively with the system without needing specialized knowledge.
Explanation and Justification:
One distinctive feature of expert systems is their ability to explain the reasoning behind their conclusions. They can provide users with the steps and rules applied during the decision-making process, offering transparency and building trust in the system’s capabilities.
Continuous Learning and Update:
While traditional expert systems do not learn from new data automatically, ongoing efforts are made to update their knowledge bases and rule sets manually to reflect new insights, discoveries, or changes in their field of expertise, ensuring their advice remains relevant and accurate.
Key Characteristics of Expert Systems:
Expert systems are distinguished by their ability to simulate the analytical capabilities of human experts.
High Performance:
They efficiently process vast amounts of data , providing solutions more rapidly than humanly possible in many cases.
Reliability:
Expert systems offer consistent and error-free decisions based on the logic and data encoded in their knowledge bases.
Adaptability:
They can be continually updated and expanded with new knowledge, allowing them to adapt to changing information and conditions.
User-Friendly Interface:
The systems are designed with interfaces that are intuitive and easy to use, making specialized knowledge accessible to non-experts.
Explainability:
By offering explanations for their decisions, expert systems demystify AI’s decision-making process, fostering user trust and understanding.
Components of an Expert System:
In this article, we will not only define “What is an Expert System?” but also define its components, because the effectiveness of an expert system hinges on the seamless integration of its components.

Knowledge Base:
Acts as the brain of the expert system, containing all the domain-specific knowledge and information needed to make decisions.
Inference Engine:
This is the logical reasoning mechanism that applies rules to the knowledge base to derive conclusions or make decisions.
User Interface:
Serves as the bridge between the user and the expert system, enabling easy communication and interaction.
Knowledge Acquisition Facility:
Tools and methodologies for updating the knowledge base are critical for maintaining the relevance and accuracy of the system.
Explanation Facility:
Provides detailed explanations of the reasoning process, enhancing the system’s transparency and user trust.
Comparing Expert and Conventional Systems:
Expert systems and conventional systems differ fundamentally in their approach to processing information and solving problems.
Problem-Solving:
Unlike conventional systems that rely on predetermined algorithms for processing, expert systems use heuristic approaches to mimic human problem-solving, making them better suited for dealing with complex and poorly structured problems.
Knowledge Encoding:
Expert systems encode knowledge in a flexible and dynamic manner, using rules and heuristics, which allows for more nuanced decision-making compared to the rigid data structures of conventional systems.
The ability to update and expand the knowledge base of expert systems makes them more adaptable to new information and changing conditions than conventional systems.
Complexity Handling:
Expert systems are designed to manage and interpret complex data and situations, utilizing logical deduction and inference in ways that conventional systems cannot.
Human Expertise vs. Expert Systems:
Now that we know “What is Expert Systems?” it’s the time to draw the comparison between human expertise and expert systems to understand the strengths and limitations of each better.
Accessibility:
Expert systems make specialized knowledge available around the clock, overcoming the physical and temporal limitations associated with human experts.
Consistency:
They provide uniform and bias -free decisions, whereas human decision-making can be inconsistent due to various factors, including fatigue and cognitive biases.
Comprehensive Analysis:
Expert systems can analyze larger datasets than human experts, offering insights that might not be immediately apparent to humans.
Lack of Intuition:
Despite their analytical capabilities, expert systems cannot replicate the intuitive and creative problem-solving abilities of humans, which can be crucial in certain contexts.
Applications of Expert Systems in Various Industries:
The versatility of expert systems is evident in their wide range of applications across different sectors.
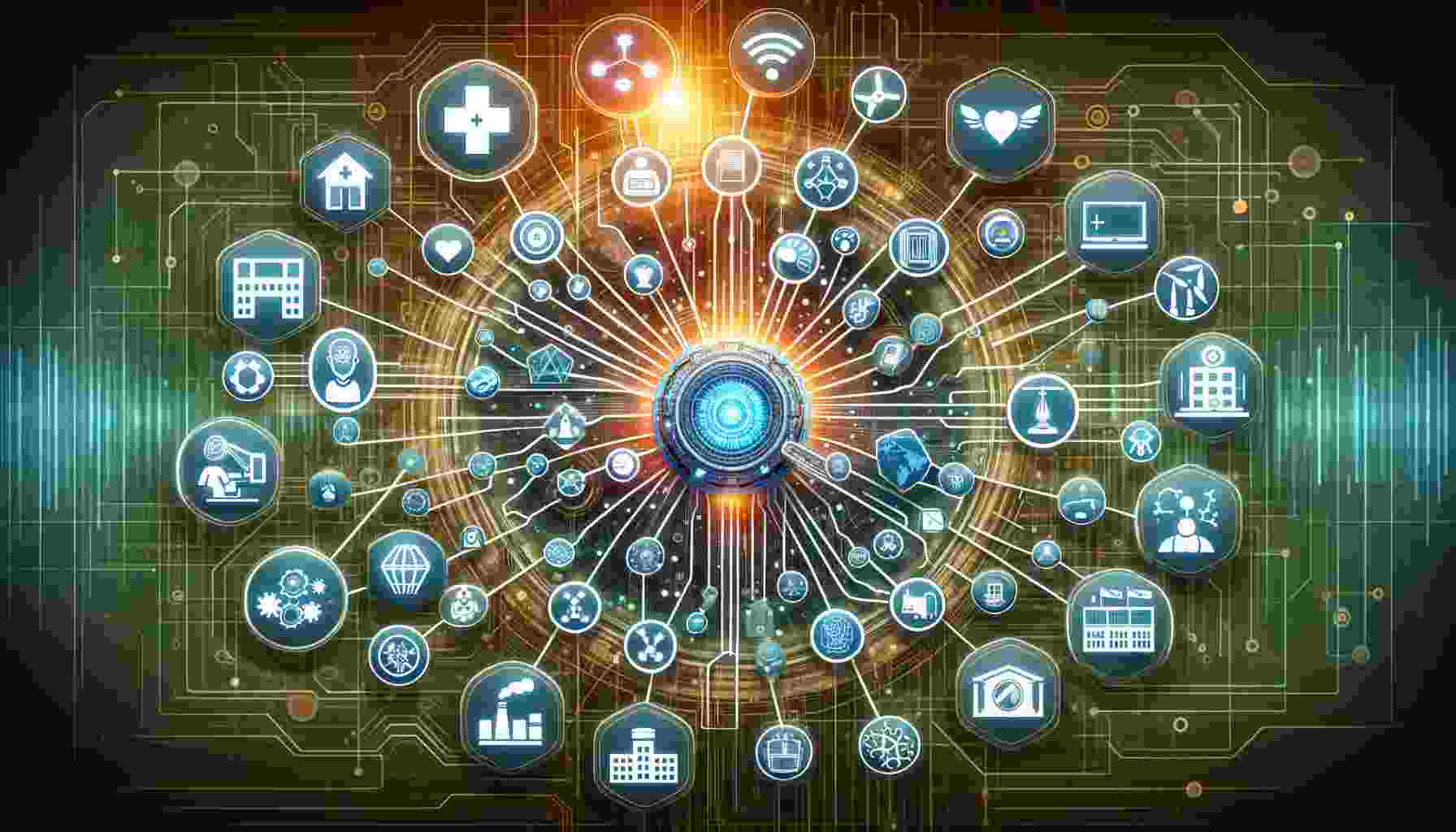
Healthcare:
From diagnosing diseases to suggesting treatment plans, expert systems play a crucial role in supporting medical professionals and improving patient outcomes.
They are used for fraud detection, investment analysis, and risk management, enhancing the efficiency and security of financial operations.
Manufacturing:
In manufacturing, expert systems contribute to quality control, production planning, and fault diagnosis, optimizing production processes and reducing downtime.
Environmental Science:
Expert systems assist in monitoring environmental conditions, predicting natural disasters, and managing natural resources, supporting sustainable environmental management practices.
They provide personalized learning experiences and tutoring, adapting to individual learners’ needs and enhancing educational outcomes.
Customer Service:
Expert systems offer automated support and personalized advice, improving customer service and satisfaction.
Legal Sector:
They assist in legal research and case analysis, streamlining legal processes and enhancing access to legal information.
Advantages of Expert Systems:
The benefits of expert systems extend across efficiency, accuracy, and decision-making.
- Accuracy: Expert systems analyze data with precision , offering accurate solutions to complex problems.
- Cost-Efficiency: By automating tasks that traditionally require human expertise, expert systems reduce operational costs and increase efficiency.
- Availability: They provide continuous access to expert-level analysis and advice, enhancing decision-making processes.
- Consistency: Expert systems ensure consistent decision-making, reducing the likelihood of human error.
- Scalability: The flexibility of expert systems allows them to be customized and scaled to meet the evolving needs of different industries.
Challenges of Expert Systems:
Despite their advantages, expert systems face several challenges that must be addressed to maximize their potential.
- Complex Development: The creation of a comprehensive and accurate knowledge base is a time-consuming and resource-intensive process.
- Maintenance: Keeping the system up-to-date with the latest knowledge and information requires ongoing effort and resources.
- Lack of Intuition: Expert systems cannot replicate the nuanced understanding and creative problem-solving capabilities of human experts.
- Ethical Considerations: The use of expert systems in decision-making processes raises ethical questions, particularly in scenarios involving moral judgments.
Future Prospects of Expert Systems:
After understanding “What is Expert Systems?” it’s the time to review its future prospect, which consist of nothing but continuous improvement and expansion.

Integration with Machine Learning:
Combining expert systems with machine learning algorithms promises to enhance their learning capabilities and adaptability.
Expanding Domains:
The application of expert systems is expected to extend into new industries and areas, further demonstrating their versatility and impact.
Enhanced User Experience:
Ongoing efforts to improve user interfaces will make expert systems more intuitive and accessible to a broader audience.
Greater Autonomy:
Future developments aim to increase the autonomy of expert systems, enabling them to make more independent decisions.
Ethical AI:
Addressing ethical considerations in the development and deployment of expert systems is crucial to ensure their responsible and beneficial use.
Want to Read More? Explore These AI Glossaries!
Immerse yourself in the realm of artificial intelligence through our meticulously assembled glossaries. No matter your skill level, there’s always something exciting to uncover!
- What is Semantics? : it refers to the process of understanding and interpreting the meaning of data.
- What is Semantic Search? : It represents a sophisticated aspect of search engines, where the focus shifts from keywords to the intent and contextual meaning behind user queries.
- What is Semi Structured Data? : It represents a blend of structured and unstructured data, containing some organizational properties without adhering to a strict schema.
- What is Sensor Fusion? : It is a vital concept in artificial intelligence (AI), which refers to the integration of sensory data from multiple sources to produce more accurate, reliable, and comprehensive information than what could be achieved by a single sensor alone.
- What is Sentiment Analysis? : It is a crucial technique in Artificial Intelligence (AI) that serves to understand people’s emotions, opinions, and attitudes expressed in text.
Here are some of the most commonly asked questions other than “What is Expert Systems?”
What is the difference between a dialogue system and a chatbot?
What are 3 examples of dialogue systems, what makes a good dialogue system, what is the dialogue system architecture, conclusion:.
Expert systems and dialogue systems represent significant advancements in the field of artificial intelligence, offering innovative solutions across a broad spectrum of industries. While expert systems emulate the decision-making abilities of human experts in specific domains, dialogue systems provide a platform for natural language communication, enhancing user interaction and service automation.
In this article, we have thoroughly discussed “What are Expert Systems?” But this is not the only term we have defined. To understand more AI-related concepts and terminologies, check out more articles in our AI Lexicon Guide .

Digital marketing enthusiast by day, nature wanderer by dusk. Dave Andre blends two decades of AI and SaaS expertise into impactful strategies for SMEs. His weekends? Lost in books on tech trends and rejuvenating on scenic trails.

What is Computer Vision?

What Is Decision Theory?

What is Decision Tree Learning?

What is Declarative Programming?
Leave a reply cancel reply.
Your email address will not be published. Required fields are marked *
Save my name, email, and website in this browser for the next time I comment.
Boost your Productivity now
- AI Tools Directory
- Submit your AI Tool
Latest Posts
- Natural Language Understanding
- Natural Language Generation
- Dialog Management
- Brain-Inspired AI
- Artificial Neural Networks
- Deep Learning
- Spiking Neural Networks
- Knowledge Representation
- Computer Vision
- Automated Reasoning
Expert Systems – an important subset of AI
- Modal Logics – an important subset of AI
The emergence of artificial intelligence (AI) has revolutionized the way we interact with technology. AI Expert Systems are a subset of AI that have important applications in both industrial and consumer settings. Expert systems use a combination of human-like reasoning and knowledge to provide advice, guidance, and automated solutions to complex problems. As a result, they are becoming increasingly popular for tasks such as medical diagnoses, fraud detection, and legal advice.
AI Expert Systems
AI expert systems are a type of artificial intelligence technology that mimics the decision-making abilities of a human expert. These systems are designed to solve complex problems by applying knowledge and reasoning to reach accurate conclusions. Expert systems have been used in various industries such as healthcare, finance, manufacturing, and engineering.
One of the significant advantages of AI expert systems is their ability to learn from past experiences and continuously improve their performance. They can analyze vast amounts of data and identify patterns that may not be visible to humans. This makes them an invaluable tool for businesses looking to streamline processes, reduce errors, and increase efficiency.
However, despite their potential benefits, there are some challenges associated with implementing AI expert systems. One major issue is the lack of transparency in how these systems make decisions. It can be challenging to understand why an AI system arrived at a particular conclusion or recommendation without visibility into its internal workings. Additionally, there are concerns about bias introduced into these systems if they are trained on biased data sets or by biased developers.
What are Expert Systems?
Expert systems are a type of artificial intelligence (AI) that uses knowledge and reasoning techniques to make decisions in specialized areas. They rely on a set of rules and algorithms to analyze data and provide solutions, mimicking the decision-making process of a human expert. Expert systems have been used in various industries such as healthcare, finance, manufacturing, and transportation.
One major advantage of expert systems is their ability to handle complex tasks efficiently without human intervention. For example, medical diagnosis can be automated using expert systems by analyzing patient symptoms and correlating them with relevant medical conditions. This reduces the time taken for diagnosis while also increasing accuracy.
However, like any other AI technology, expert systems are not perfect; they may fail when presented with new or unusual situations that do not fit into their pre-defined set of rules. As such, despite their efficiency in handling routine tasks, they still require human supervision to ensure accuracy and prevent errors. Thus, it remains important for organizations to strike a balance between automation and human oversight when deploying expert systems.
Benefits of Expert Systems
Expert systems are a type of artificial intelligence that can mimic the decision-making abilities of human experts in a particular field. These systems use machine learning algorithms to analyze and interpret data, then provide insights and recommendations based on that analysis. One major benefit of expert systems is their ability to improve decision-making accuracy and efficiency by reducing errors and eliminating bias.
In addition, expert systems can also help organizations save time and money by automating certain processes that would otherwise require human intervention. For example, an expert system could be used to automatically detect fraudulent activity in financial transactions or identify potential maintenance issues with industrial equipment before they become serious problems. This not only saves time but can also prevent costly mistakes from occurring.
Finally, expert systems can also enhance collaboration between humans and machines by providing valuable insights that may not be immediately apparent to human experts. By combining the analytical power of AI with the creativity and intuition of human workers, organizations can unlock new levels of innovation and problem-solving capabilities that were previously impossible.
How Do They Work?
AI expert systems are computer programs that simulate the decision-making ability of a human expert in a specific field. They work by utilizing knowledge-based rules and algorithms to analyze data and provide recommendations or solutions. Expert systems can be trained using machine learning techniques such as neural networks and natural language processing, allowing them to continually improve their accuracy over time.
The key components of an expert system include a knowledge base, an inference engine, and a user interface. The knowledge base contains domain-specific information and rules that the system uses to make decisions or solve problems . The inference engine processes this information using logical reasoning to reach conclusions based on the available data. Finally, the user interface presents the system’s output in an understandable format for human interaction.
Expert systems have many real-world applications across various industries such as healthcare, finance, and manufacturing. For example, in healthcare, AI expert systems can assist doctors in diagnosing diseases by analyzing patient symptoms and medical history. In finance, these systems can help financial advisors recommend investment strategies based on market trends and risk analysis. Overall, AI expert systems offer significant benefits including increased efficiency, accuracy, and cost-effectiveness compared to traditional methods of decision-making.
AI in the Modern Age
AI expert systems, also known as knowledge-based systems, are a subfield of artificial intelligence that utilizes human expertise to solve complex problems . These systems can learn from historical data and make decisions based on predefined rules or algorithms. Expert systems have been used in various industries such as finance, healthcare, and transportation to improve decision-making and provide insights into complex problems.
One example of an AI expert system is IBM’s Watson which can analyze large amounts of data to provide insights for businesses. Another use case is in medical diagnosis where expert systems can assist doctors in identifying diseases by analyzing patient symptoms and medical history. Expert systems have also been used in the financial sector for fraud detection and risk assessment.
As AI technology continues to evolve, there will be more potential applications for expert systems across various industries. However, it’s important to note that these technologies must be developed ethically and with consideration for potential biases or negative impacts on society. The future of AI expert systems relies on responsible development practices that prioritize transparency and fairness.
Challenges and Limitations
One of the biggest challenges in developing AI expert systems is ensuring that they are capable of handling complex tasks and making accurate predictions. In many cases, these systems must be able to analyze vast amounts of data in real time, which requires powerful algorithms and processing capabilities. Additionally, there is always a risk that a system may make incorrect decisions or recommendations due to inaccurate or incomplete data.
Another limitation of AI expert systems is their inability to replicate human intuition and creativity. While these systems can be programmed to follow strict rules and procedures, they cannot think outside the box or adapt to unexpected situations. This means that there will always be certain tasks that require human input and oversight.
Finally, there are ethical concerns surrounding the use of AI expert systems in certain applications. For example, some experts have raised concerns about bias in facial recognition technology or the potential for autonomous weapons to cause harm if left unchecked by human operators. As such, developers and policymakers alike need to consider not only the technical limitations but also the broader societal implications of these technologies.
Summary: Unlocking Potential
Unlocking the potential of AI expert systems is becoming increasingly important in our fast-paced and technology-driven world. These systems can automate tasks, provide personalized recommendations, and analyze vast amounts of data faster than any human could ever hope to achieve. This means that they can help businesses make better decisions and improve their overall performance.
However, it’s important to remember that these systems must be carefully designed and implemented to avoid any unintended consequences. For example, if they are given biased or incomplete data, they may generate inaccurate results that could harm individuals or organizations. Additionally, there is a risk that they may replace human workers instead of complementing their skills and expertise.
Overall, AI expert systems have the potential to revolutionize many aspects of our daily lives. By taking a thoughtful approach to their development and use, we can ensure that they bring about positive change while minimizing any negative impacts.
- Translate.Video – Video Translation was never that easy
- AI for Accounting – unleash the Power
- AI Apps are the Future of Technology
- Is AI a threat to humanity?
- Foundation Models radically change how AI is applied
- Ad generators
- Aggregators
- AI detectors
- AI Workspaces
- Art creators
- Avatar creators
- Beauty apps
- Brand design
- Communication
- Content Creation
- Content creators
- Copywriters
- Copywriting
- Customer Service
- Customer services
- Design assistants
- Email Assistant
- Email assistants
- Extended Content
- General Information
- Image editors & creators
- Marketing apps
- Paraphrasing
- Personalized videos apps
- Plagiarism checker
- Productivity
- Productivity apps
- Research apps
- Social Media
- Social media apps
- Speech-to-text apps
- Text creator apps
- Text to Video
- Text-to-Speech
- Text-to-speech apps
- Text-to-video apps
- Translation apps
- Privacy Policy
- Terms of Use
© 2024 AI Info
Open Access is an initiative that aims to make scientific research freely available to all. To date our community has made over 100 million downloads. It’s based on principles of collaboration, unobstructed discovery, and, most importantly, scientific progression. As PhD students, we found it difficult to access the research we needed, so we decided to create a new Open Access publisher that levels the playing field for scientists across the world. How? By making research easy to access, and puts the academic needs of the researchers before the business interests of publishers.
We are a community of more than 103,000 authors and editors from 3,291 institutions spanning 160 countries, including Nobel Prize winners and some of the world’s most-cited researchers. Publishing on IntechOpen allows authors to earn citations and find new collaborators, meaning more people see your work not only from your own field of study, but from other related fields too.
Brief introduction to this section that descibes Open Access especially from an IntechOpen perspective
Want to get in touch? Contact our London head office or media team here
Our team is growing all the time, so we’re always on the lookout for smart people who want to help us reshape the world of scientific publishing.
Home > Books > Enhanced Expert Systems
Introductory Chapter: Enhanced Expert System - A Long-Life Solution
Submitted: 30 January 2019 Published: 02 April 2019
DOI: 10.5772/intechopen.85704
Cite this chapter
There are two ways to cite this chapter:
From the Edited Volume
Enhanced Expert Systems
Edited by Petrică Vizureanu
To purchase hard copies of this book, please contact the representative in India: CBS Publishers & Distributors Pvt. Ltd. www.cbspd.com | [email protected]
Chapter metrics overview
1,644 Chapter Downloads
Impact of this chapter
Total Chapter Downloads on intechopen.com

Total Chapter Views on intechopen.com
Author Information
Petrică vizureanu *.
- Technical University of Iasi, Romania
*Address all correspondence to: [email protected]
1. Introduction
What is the definition of an expert system? An expert system belongs to a field of artificial intelligence, and it is a computer program or a software, which can do the same task of a human expert. It can give reliable advice in a specific area of expertise (its domain)and get new conclusions about difficult activities to examine [ 1 ]. An expert system can explain its reasoning everytime and is able to interact with a user in the same way that you might consult a human expert. Also, an expert system can be defined as a software program that can outline reasoned conclusions from an amount of knowledge in a specific domain and aims to develop “smart” programs and applications.
The human experts are not 100% reliable in different domains, which can be taken into consideration the advantages and benefits of all accomplished things, but they may disagree with each other or forget to take into account a crucial parameter before making a decision. A human expert can have unsurpassed knowledge in the field and can gain as much knowledge as possible, but be hopeless explaining that to someone else. Human experts cannot be available all the time; i.e., (i) in a small company, the expert on some area can be ill or on holiday; (ii) your doctor may not be able to see you until next week; and (iii) a human expert can move to another company (taking his expertise with him) or retire.

General description for human expert vs. expert system.

Main elements of an expert system.

Logical flow inside an expert system.

Forward-chaining technique for an expert system.

Backward-chaining technique for an expert system.
An expert system does not get tired. An expert system (properly programmed) should be 100% reliable and can combine the expertise of several experts. An expert system should be realized to explain and justify all advices it gives. Although an expert system can be expensive to develop, once it is there, its running costs should be low, so there will be economic benefits for the company. An expert system is always available. You can take it with you if you have a notebook or Internet connection, so you could consult an expert system over the Internet. Once the knowledge has been programmed/inserted into the system, it will not be lost if the human expert leaves or dies [ 2 ].
Problems with expert systems: limited domain; systems are not always up to date and do not learn, has no “common sense” and experts are needed to setup and maintain the system.
Many applications of expert systems are very well known: Prospector—used by geologists to identify sites for drilling or mining; PUFF—medical system for diagnosis of respiratory conditions; Design Advisor—gives advice to designers of processor chips; MYCIN—medical system for diagnosing blood disorders (first used in 1979); LITHIAN—gives advice to archeologists examining stone tools; and DENDRAL—used to identify the structure of chemical compounds (first used in 1965).
Main components of an expert system: (i) the knowledge base is the collection of facts and rules which describe all the knowledge about the problem domain; (ii) the inference engine is the part of the system that chooses which facts and rules to apply when trying to solve the user’s query; and (iii) the user interface is the part of the system that takes in the user’s query in a readable form and passes it to the inference engine. It then displays the results to the user [ 3 ].
An expert system behaves similar to a human expert in a field or area. Also, they can be used to solve problems in various fields or disciplines, and can assist in problem solving, but the goal is not to replace the experts, but to provide users an effective tool thereby relieving experts of routine tasks.
An expert system has some capabilities: (i) superior problem solving—only solvable problems, (ii) ability to save and apply knowledge and experience to problems, (iii) reduced time for complex problems, and (iv) looks at problems from a variety of perspectives.
An expert system has five basic components: knowledge base, inference engine, explanation component, user interface, and acquisition component.
Knowledge base contains the factual and empirical knowledge of experts in a particular subject area and all the facts, rules and procedures, which are important for problem solving.
Inference engine simulates the problem-solving strategy of a human expert, represents the logical unit by means of which conclusions are drawn from the knowledge base according to a defined problem-solving method, controls the execution, which questions to ask and in what order, and simulates the problem-solving process of human experts. Functions of the inference engine are to determine which actions are to be executed between individual parts of the expert system, how they are executed, and in what sequence, to determine how and when the rules will be processed and to control the dialog with the user [ 4 ].
Explanation component has the role to explain the problem-solving strategy to the user. The solutions must be reproducible by the user and engineer but can only be verified by the human expert: which facts were asked for? why? which facts were vital? and can go as far as, how would the conclusion change if some facts would change?
User interface employs natural language for dialogs with the user whenever possible. Questions posed such as “how should questions be answered by the user?”, “how will system responses to these questions will be formulated?”, and “what info is to be graphical?” must be easy to use, erroneous errors kept to a minimum, and questions and answers must be understandable.
Acquisition component provides support for structuring and implementation of the knowledge in the knowledge base; hence, it is very important because it allows the engineer to concentrate less on the programming. Knowledge data should be easy to enter, easy to understand methods of representing all info in knowledge base, syntax checks, and access to programming language.
People involved with expert systems are domain expert (a person who possesses the skill and knowledge to solve a specific problem in a manner superior to others), knowledge engineer (a person who designs, builds, and tests an expert system), and end-user (an individual or group who will be using the expert system).
Two main inference techniques are used: forward chaining and backward chaining.
Forward chaining is about the knowledge base searched for rules that match the known facts, and the action part of these rules is performed. The process continues until a goal is reached and puts the symptoms together to reach a conclusion that an ex. doctor has diagnosed in a patient.
Backward chaining starts from a goal, the conclusion. All the rules that contain this conclusion are then checked to determine whether the conditions of these rules have been satisfied. Ex. doctor has an end idea of what is wrong with the patient, though they must prove it by going from the diagnosis and finding the symptoms.
Expert systems have applications, in example, for automatic mapping profiles in software metrics for temporal analysis of programming learning and scoring rubrics. Also, these can be dedicated to a new knowledge representation model, providing convergence of classical operations research and modern knowledge engineering or to a mathematical model that graphically and numerically represents the probabilistic relationships between random variables.
- 1. https://torry.aberdeen.sch.uk
- 2. http://www.duncanrig.s-lanark.sch.uk
- 3. http://www.test-me.co.uk
- 4. Kalogirou SA. Artificial intelligence for the modeling and control of combustion processes: A review. Progress in Energy and Combustion Science. 2003; 29 (6):515-566
© 2019 The Author(s). Licensee IntechOpen. This chapter is distributed under the terms of the Creative Commons Attribution 3.0 License , which permits unrestricted use, distribution, and reproduction in any medium, provided the original work is properly cited.
Continue reading from the same book
Edited by Petrica Vizureanu
Published: 18 September 2019
By João Nunes, Mirko Barbosa, Luiz Silva, Kyller Gorg...
1140 downloads
By Amal Saki Malehi and Mina Jahangiri
953 downloads
By Márcia Gonçalves de Oliveira, Ádler Oliveira Silva...
1048 downloads
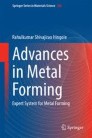
Advances in Metal Forming pp 31–39 Cite as
Fundamentals of Expert System
- Rahulkumar Shivajirao Hingole 9
- First Online: 01 January 2014
2314 Accesses
1 Citations
Part of the book series: Springer Series in Materials Science ((SSMATERIALS,volume 206))
Expert systems (ES) emerged as a branch of artificial intelligence (AI), from the effort of AI researchers to develop computer programs that could reason as humans. Many organizations have leveraged this technology to increase productivity and profits through better business decisions. ES are one of most commercially successful branches of AI. Although there have been reports of ES failures, surveys show that many companies have remained enthusiastic proponents of the technology and continue to develop important and successful applications. The detailed fundamental concepts and application expert system has been discussed in this chapter.
This is a preview of subscription content, log in via an institution .
Buying options
- Available as PDF
- Read on any device
- Instant download
- Own it forever
- Available as EPUB and PDF
- Durable hardcover edition
- Dispatched in 3 to 5 business days
- Free shipping worldwide - see info
Tax calculation will be finalised at checkout
Purchases are for personal use only
Author information
Authors and affiliations.
Department of Mechanical Engineering Padmashree, Bhivarabai Sawant Institute of Technology and Research, Wagholi, Pune, India
Rahulkumar Shivajirao Hingole
You can also search for this author in PubMed Google Scholar
Corresponding author
Correspondence to Rahulkumar Shivajirao Hingole .
Rights and permissions
Reprints and permissions
Copyright information
© 2015 Springer-Verlag Berlin Heidelberg
About this chapter
Cite this chapter.
Hingole, R.S. (2015). Fundamentals of Expert System. In: Advances in Metal Forming. Springer Series in Materials Science, vol 206. Springer, Berlin, Heidelberg. https://doi.org/10.1007/978-3-662-44497-9_3
Download citation
DOI : https://doi.org/10.1007/978-3-662-44497-9_3
Published : 09 September 2014
Publisher Name : Springer, Berlin, Heidelberg
Print ISBN : 978-3-662-44496-2
Online ISBN : 978-3-662-44497-9
eBook Packages : Engineering Engineering (R0)
Share this chapter
Anyone you share the following link with will be able to read this content:
Sorry, a shareable link is not currently available for this article.
Provided by the Springer Nature SharedIt content-sharing initiative
- Publish with us
Policies and ethics
- Find a journal
- Track your research
Change Password
Your password must have 8 characters or more and contain 3 of the following:.
- a lower case character,
- an upper case character,
- a special character
Password Changed Successfully
Your password has been changed
- Sign in / Register
Request Username
Can't sign in? Forgot your username?
Enter your email address below and we will send you your username
If the address matches an existing account you will receive an email with instructions to retrieve your username
A Detailed Characterization of the Expert Problem-Solving Process in Science and Engineering: Guidance for Teaching and Assessment
- Argenta M. Price
- Candice J. Kim
- Eric W. Burkholder
- Amy V. Fritz
- Carl E. Wieman
*Address correspondence to: Argenta M. Price ( E-mail Address: [email protected] ).
Department of Physics, Stanford University, Stanford, CA 94305
Search for more papers by this author
Graduate School of Education, Stanford University, Stanford, CA 94305
School of Medicine, Stanford University, Stanford, CA 94305
Department of Electrical Engineering, Stanford University, Stanford, CA 94305
A primary goal of science and engineering (S&E) education is to produce good problem solvers, but how to best teach and measure the quality of problem solving remains unclear. The process is complex, multifaceted, and not fully characterized. Here, we present a detailed characterization of the S&E problem-solving process as a set of specific interlinked decisions. This framework of decisions is empirically grounded and describes the entire process. To develop this, we interviewed 52 successful scientists and engineers (“experts”) spanning different disciplines, including biology and medicine. They described how they solved a typical but important problem in their work, and we analyzed the interviews in terms of decisions made. Surprisingly, we found that across all experts and fields, the solution process was framed around making a set of just 29 specific decisions. We also found that the process of making those discipline-general decisions (selecting between alternative actions) relied heavily on domain-specific predictive models that embodied the relevant disciplinary knowledge. This set of decisions provides a guide for the detailed measurement and teaching of S&E problem solving. This decision framework also provides a more specific, complete, and empirically based description of the “practices” of science.
INTRODUCTION
Many faculty members with new graduate students and many managers with employees who are recent college graduates have had similar experiences. Their advisees/employees have just completed a program of rigorous course work, often with distinction, but they seem unable to solve the real-world problems they encounter. The supervisor struggles to figure out exactly what the problem is and how they can guide the person in overcoming it. This paper is providing a way to answer those questions in the context of science and engineering (S&E). By characterizing the problem-solving process of experts, this paper investigates the “mastery” performance level and specifies an overarching learning goal for S&E students, which can be taught and measured to improve teaching.
The importance of problem solving as an educational outcome has long been recognized, but too often postsecondary S&E graduates have serious difficulties when confronted with real-world problems ( Quacquarelli Symonds, 2018 ). This reflects two long-standing educational problems with regard to problem solving: how to properly measure it, and how to effectively teach it. We theorize that the root of these difficulties is that good “problem solving” is a complex multifaceted process, and the details of that process have not been sufficiently characterized. Better characterization of the problem-solving process is necessary to allow problem solving, and more particularly, the complex set of skills and knowledge it entails, to be measured and taught more effectively. We sought to create an empirically grounded conceptual framework that would characterize the detailed structure of the full problem-solving process used by skilled practitioners when solving problems as part of their work. We also wanted a framework that would allow use and comparison across S&E disciplines. To create such a framework, we examined the operational decisions (choices among alternatives that result in subsequent actions) that these practitioners make when solving problems in their discipline.
Various aspects of problem solving have been studied across multiple domains, using a variety of methods (e.g., Newell and Simon, 1972 ; Dunbar, 2000 ; National Research Council [NRC], 2012b ; Lintern et al. , 2018 ). These ranged from expert self-reflections (e.g., Polya, 1945 ), to studies on knowledge lean tasks to discover general problem-solving heuristics (e.g., Egan and Greeno, 1974 ), to comparisons of expert and novice performances on simplified problems across a variety of disciplines (e.g., Chase and Simon, 1973 ; Chi et al. , 1981 ; Larkin and Reif, 1979 ; Ericsson et al. , 2006 , 2018 ). These studies revealed important novice–expert differences—notably, that experts are better at identifying important features and have knowledge structures that allow them to reduce demands on working memory. Studies that specifically gave the experts unfamiliar problems in their disciplines also found that, relative to novices, they had more deliberate and reflective strategies, including more extensive planning and managing of their own behavior, and they could use their knowledge base to better define the problem ( Schoenfeld, 1985 ; Wineburg, 1998 ; Singh, 2002 ). While these studies focused on discrete cognitive steps of the individual, an alternative framing of problem solving has been in terms of “ecological psychology” of “situativity,” looking at how the problem solver views and interacts with the environment in terms of affordances and constraints ( Greeno, 1994 ). “Naturalistic decision making” is a related framework that specifically examines how experts make decisions in complex, real-world, settings, with an emphasis on the importance of assessing the situation surrounding the problem at hand ( Klein, 2008 ; Mosier et al. , 2018 ).
While this work on expertise has provided important insights into the problem-solving process, its focus has been limited. Most has focused on looking for cognitive differences between experts and novices using limited and targeted tasks, such as remembering the pieces on a chessboard ( Chase and Simon, 1973 ) or identifying the important concepts represented in an introductory physics textbook problem ( Chi et al. , 1981 ). It did not attempt to explore the full process of solving, particularly for solving the type of complex problem that a scientist or engineer encounters as a member of the workforce (“authentic problems”).
There have also been many theoretical proposals as to expert problem-solving practices, but with little empirical evidence as to their completeness or accuracy (e.g., Polya, 1945 ; Heller and Reif, 1984 ; Organisation for Economic Cooperation and Development [OECD], 2019 ). The work of Dunbar (2000) is a notable exception to the lack of empirical work, as his group did examine how biologists solved problems in their work by analyzing lab meetings held by eight molecular biology research groups. His groundbreaking work focused on creativity and discovery in the research process, and he identified the importance of analogical reasoning and distributed reasoning by scientists in answering research questions and gaining new insights. Kozma et al. (2000) studied professional chemists solving problems, but their work focused only on the use of specialized representations.
The “cognitive systems engineering” approach ( Lintern et al. , 2018 ) takes a more empirically based approach looking at experts solving problems in their work, and as such tends to span aspects of both the purely cognitive and the ecological psychological theories. It uses both observations of experts in authentic work settings and retrospective interviews about how experts carried out particular work tasks. This theoretical framing and the experimental methods are similar to what we use, particularly in the “naturalistic decision making” area of research ( Mosier et al. , 2018 ). That work looks at how critical decisions are made in solving specific problems in their real-world setting. The decision process is studied primarily through retrospective interviews about challenging cases faced by experts. As described below, our methods are adapted from that work ( Crandall et al. , 2006 ), though there are some notable differences in focus and field. A particular difference is that we focused on identifying what are decisions to be made, which are more straight-forward to identify from retrospective interviews than how those decisions are made. We all have the same ultimate goal, however, to improve the training/teaching of the respective expertise.
Problem solving is central to the processes of science, engineering, and medicine, so research and educational standards about scientific thinking and the process and practices of science are also relevant to this discussion. Work by Osborne and colleagues describes six styles of scientific reasoning that can be used to explain how scientists and students approach different problems ( Kind and Osborne, 2016 ). There are also numerous educational standards and frameworks that, based on theory, lay out the skills or practices that science and engineering students are expected to master (e.g., American Association for the Advancement of Science [AAAS], 2011 ; Next Generation Science Standards Lead States, 2013 ; OECD, 2019 ; ABET, 2020 ). More specifically related to the training of problem solving, Priemer et al. (2020) synthesizes literature on problem solving and scientific reasoning to create a “STEM [science, technology, engineering, and mathematics] and computer science framework for problem solving” that lays out steps that could be involved in a students’ problem-solving efforts across STEM fields. These frameworks provide a rich groundwork, but they have several limitations: 1) They are based on theoretical ideas of the practice of science, not empirical evidence, so while each framework contains overlapping elements of the problem-solving process, it is unclear whether they capture the complete process. 2) They are focused on school science, rather than the actual problem solving that practitioners carry out and that students will need to carry out in future STEM careers. 3) They are typically underspecified, so that the steps or practices apply generally, but it is difficult to translate them into measurable learning goals for students to practice. Working to address that, Clemmons et al. (2020) recently sought to operationalize the core competencies from the Vision and Change report ( AAAS, 2011 ), establishing a set of skills that biology students should be able to master.
Our work seeks to augment this prior work by building a conceptual framework that is empirically based, grounded in how scientists and engineers solve problems in practice instead of in school. We base our framework on the decisions that need to be made during problem solving, which makes each item clearly defined for practice and assessment. In our analysis of expert problem solving, we empirically identified the entire problem-solving process. We found this includes deciding when and how to use the steps and skills defined in the work described previously but also includes additional elements. There are also questions in the literature about how generalizable across fields a particular set of practices may be. Here, we present the first empirical examination of the entire problem-solving process, and we compare that process across many different S&E disciplines.
A variety of instructional methods have been used to try and teach science and engineering problem solving, but there has been little evidence of their efficacy at improving problem solving (for a review, see NRC, 2012b ). Research explicitly on teaching problem solving has primarily focused on textbook-type exercises and utilized step-by-step strategies or heuristics. These studies have shown limited success, often getting students to follow specific procedural steps but with little gain in actually solving problems and showing some potential drawbacks ( Heller and Reif, 1984 ; Heller et al. , 1992 ; Huffman, 1997 ; Heckler, 2010 ; Kuo et al. , 2017 ). As discussed later, the framework presented here offers guidance for different and potentially more effective approaches to teaching problem solving.
These challenges can be illustrated by considering three different problems taken from courses in mechanical engineering, physics, and biology, respectively ( Figure 1 ). All of these problems are challenging, requiring considerable knowledge and effort by the student to solve correctly. Problems such as these are routinely used to both assess students’ problem-solving skills, and students are expected to learn such skills by practicing doing such problems. However, it is obvious to any expert in the respective fields, that, while these problems might be complicated and difficult to answer, they are vastly different from solving authentic problems in that field. They all have well-defined answers that can be reached by straightforward solution paths. More specifically, they do not involve needing to use judgment to make any decisions based on limited information (e.g., insufficient to specify a correct decision with certainty). The relevant concepts and information and assumptions are all stated or obvious. The failure of problems like these to capture the complexity of authentic problem solving underlies the failure of efforts to measure and teach problem solving. Recognizing this failure motivated our efforts to more completely characterize the problem-solving process of practicing scientists, engineers, and doctors.
FIGURE 1. Example problems from courses or textbooks in mechanical engineering, physics and biology. Problems from: Mechanical engineering: Wayne State mechanical engineering sample exam problems (Wayne State, n.d.), Physics: A standard physics problem in nearly every advanced quantum mechanics course, Biology: Molecular Biology of the Cell 6th edition, Chapter 7 end of chapter problems ( Alberts et al ., 2014 ).
We are building on the previous work studying expert–novice differences and problem solving but taking a different direction. We sought to create an empirically grounded framework that would characterize the detailed structure of the full problem-solving process by focusing on the operational decisions that skilled practitioners make when successfully solving authentic problems in their scientific, engineering, or medical work. We chose to identify the decisions that S&E practitioners made, because, unlike potentially nebulous skills or general problem-solving steps that might change with the discipline, decisions are sufficiently specified that they can be individually practiced by students and measured by instructors or departments. The authentic problems that we analyzed are typical problems practitioners encounter in “doing” the science or engineering entailed in their jobs. In the language of traditional problem- solving and expertise research, such authentic problems are “ill-structured” ( Simon, 1973 ) and require “adaptive expertise” ( Hatano and Inagaki, 1986 ) to solve. However, our authentic problems are considerably more complex and unstructured than what is normally considered in those literatures, because not only do they lack a clear solution path, but in many cases, it is not clear a priori that they have any solution at all. Determining that, and whether the problem needs to be redefined to be soluble, is part of the successful expert solution process. Another way in which our set of decisions goes beyond the characterization of what is involved in adaptive expertise is the prominent role of making judgments with limited information.
A common reaction of scientists and engineers to seeing the list of decisions we obtain as our primary result is, “Oh, yes, these are things I always do in solving problems. There is nothing new here.” It is comforting that these decisions all look familiar; that supports their validity. However, what is new is not that experts are making such decisions, but rather that there is a relatively small but complete set of decisions that has now been explicitly identified and that applies so generally.
We have used a much larger and broader sample of experts in this work than used in prior expert–novice studies, and we used a more stringent selection criterion. Previous empirical work has typically involved just a few experts, almost always in a single domain, and included graduate students as “experts” in some cases. Our semistructured interview sample was 31 experienced practitioners from 10 different disciplines of science, engineering, and medicine, with demonstrated competence and accomplishments well beyond those of most graduate students. Also, approximately 25 additional experts from across science, engineering, and medicine served as consultants during the planning and execution of this work.
Our research question was: What are the decisions experts make in solving authentic problems, and to what extent is this set of decisions to be made consistent both within and across disciplines?
Our approach was designed to identify the level of consistency and unique differences across disciplines. Our hypothesis was that there would be a manageable number (20–50) of decisions to be made, with a large amount of overlap of decisions made between experts within each discipline and a substantial but smaller overlap across disciplines. We believed that if we had found that every expert and/or discipline used a large and completely unique set of decisions, it would have been an interesting research result but of little further use. If our hypothesis turned out to be correct, we expected that the set of decisions obtained would have useful applications in guiding teaching and assessment, as they would show how experts in the respective disciplines applied their content knowledge to solve problems and hence provide a model for what to teach. We were not expecting to find the nearly complete degree of overlap in the decisions made across all the experts.
We first conducted 22 relatively unstructured interviews with a range of S&E experts, in which we asked about problem-solving expertise in their fields. From these interviews, we developed an initial list of decisions to be made in S&E problem solving. To refine and validate the list, we then carried out a set of 31 semistructured interviews in which S&E experts chose a specific problem from their work and described the solution process in detail. The semistructured interviews were coded for the decisions represented, either explicitly stated or implied by a choice of action. This provided a framework of decisions that characterize the problem-solving process across S&E disciplines. The research was approved by the Stanford Institutional Review Board (IRB no. 48785), and informed consent was obtained from all the participants.
This work involved interviewing many experts across different fields. We defined experts as practicing scientists, engineers, or physicians with considerable experience working as faculty at highly rated universities or having several years of experience working in moderately high-level technical positions at successful companies. We also included a few longtime postdocs and research staff in biosciences to capture more details of experimental decisions from which faculty members in those fields often were more removed. This definition of expert allows us to identify the practices of skilled professionals; we are not studying what makes only the most exceptional experts unique.
Experts were volunteers recruited through direct contact via the research team's personal and professional networks and referrals from experts in our networks. This recruitment method likely biased our sample toward people who experienced relatively similar training (most were trained in STEM disciplines at U.S. universities within the last 15–50 years). Within this limitation, we attempted to get a large range of experts by field and experience. This included people from 10 different fields (including molecular biology/biochemistry, ecology, and medicine), 11 U.S. universities, and nine different companies or government labs, and the sample was 33% female (though our engineering sample only included one female). The medical experts were volunteers from a select group of medical school faculty chosen to serve as clinical reasoning mentors for medical students at a prestigious university. We only contacted people who met our criteria for being an “expert,” and everyone who volunteered was included in the study. Most of the people who were contacted volunteered, and the only reason given for not volunteering was insufficient time. Other than their disciplinary expertise, there was little to distinguish these experts beyond the fact they were acquaintances with members of the team or acquaintances of acquaintances of team or project advisory board members. The precise number from each field was determined largely by availability of suitable experts.
We defined an “authentic problem” to be one that these experts solve in their actual jobs. Generally, this meant research projects for the science and engineering faculty, design problems for the industry engineers, and patient diagnoses for the medical doctors. Such problems are characterized by complexity, with many factors involved and no obvious solution process, and involve substantial time, effort, and resources. Such problems involve far more complexity and many more decisions, particularly decisions with limited information, than the typical problems used in previous problem-solving research or used with students in instructional settings.
Creating an Initial List of Problem-Solving Decisions
We first interviewed 22 experts ( Table 1 ), most of whom were faculty at a prestigious university, in which we asked them to discuss expertise and problem solving in their fields as it related to their own experiences. This usually resulted in their discussing examples of one or more problems they had solved. Based on the first seven interviews, plus reflections on personal experience from the research team and review of the literature on expert problem solving and teaching of scientific practices ( Ericsson et al. , 2006 ; NRC, 2012a ; Wieman, 2015 ), we created a generic list of decisions that were made in S&E problem solving. In the rest of the unstructured interviews (15), we also provided the experts with our list and asked them to comment on any additions or deletions they would suggest. Faculty who had close supervision of graduate students and industry experts who had extensively supervised inexperienced staff were particularly informative. Their observations of the way inexperienced people could fail made them sensitive to the different elements of expertise and where incorrect decisions could be made. Although we initially expected to find substantial differences across disciplines, from early in the process, we noted a high degree of overlap across the interviews in the decisions that were described.
URM (under-represented minority) included 3 African American and 2 Hispanic/Latinx. One medical faculty member was interviewed twice – in both informal and structure interviews, for a total of 53 interviews with 52 experts.
Refinement and Validation of the List of Decisions
After creating the preliminary list of decisions from the informal interviews, we conducted a separate set of more structured interviews to test and refine the list. Semistructured interviews were conducted with 31 experts from across science, engineering, and medical fields ( Table 1 ). For these interviews, we recruited experts from a range of universities and companies, though the range of institutions is still limited, given the sample size. Interviews were conducted in person or over video chat and were transcribed for analysis. In the semistructured interviews, experts were asked to choose a problem or two from their work that they could recall the details of solving and then describe the process, including all the steps and decisions they made. So that we could get a full picture of the successful problem-solving process, we decided to focus the interviews on problems that they had eventually solved successfully, though their processes inherently involved paths that needed to be revised and reconsidered. Transcripts from interviewees who agreed to have their interview transcript published are available in the supplemental data set.
Our interview protocol (see Supplemental Text) was inspired in part by the critical decision method of cognitive task analysis ( Crandall et al. , 2006 ; Lintern et al. , 2018 ), which was created for research in cognitive systems engineering and naturalistic decision making. There are some notable differences between our work and theirs, both in research goal and method. First, their goal is to improve training in specific fields by focusing on how critical decisions are made in that field during an unusual or important event; the analysis seeks to identify factors involved in making those critical decisions. We are focusing on the overall problem solving and how it compares across many different fields, which quickly led to attention on what decisions are to be made, rather than how a limited set of those decisions are made. We asked experts to describe a specific, but not necessarily unusual, problem in their work, and focused our analysis on identifying all decisions made, not reasons for making them or identifying which were most critical. The specific order of problem-solving steps was also less important to us, in part because it was clear that there was no consistent order that was followed. Second, we are looking at different types of work. Cognitive systems engineering work has primarily focused on performance in professions like firefighters, power plant operators, military technicians, and nurses. These tend to require time-sensitive critical skills that are taught with modest amounts of formal training. We are studying scientists, engineers, and doctors solving problems that require much longer and less time-critical solutions and for which the formal training occupies many years.
Given our different focus, we made several adaptations to eliminate some of the more time-consuming steps from the interview protocol, allowing us to limit the interview time to approximately 1 hour. Both protocols seek to elicit an accurate and complete reporting of the steps taken and decisions made in the process of solving a problem. Our general strategy was: 1) Have the expert explain the problem and talk step by step through the decisions involved in solving it, with relatively few interruptions from the interviewer except to keep the discussion focused on the specific problem and occasionally to ask for clarifications. 2) Ask follow-up questions to probe for more detail about particular steps and aspects of the problem-solving process. 3) Occasionally ask for general thoughts on how a novice's process might differ.
While some have questioned the reliability of information from retrospective interviews ( Nisbett and Wilson, 1977 ), we believe we avoid these concerns, because we are only identifying a decision to be made, which in this case, means identifying a well-defined action that was chosen from alternatives. This is less subjective and much more likely to be accurately recalled than is the rationale behind such a decision. See Ericsson and Simon (1980) . However, the decisions identified may still be somewhat limited—the process of deciding among possible actions might involve additional decisions in the moment, when the solution is still unknown, that we are unable to capture in the retrospective context. For the decisions we can identify, we are able to check their accuracy and completeness by comparing them with the actions taken in the conduct of the research/design. For example, consider this quote from a physician who had to re-evaluate a diagnosis, “And, in my very subjective sense, he seemed like he was being forthcoming and honest. Granted people can fool you, but he seemed like he was being forthcoming. So we had to reevaluate.” The physician then considered alternative diagnoses that could explain a test result that at first had indicated an incorrect diagnosis. While this quote does describe the (retrospective) reasoning behind a decision, we do not need to know whether that reasoning is accurately recalled. We can simply code this as “decision 18, how believable is info?” The physician followed up by considering alternative diagnoses, which in this context was coded as “26, how good is solution?” and “8, potential solutions?” This was followed by the description of the literature and additional tests conducted. These indicated actions taken that confirm the physician made a decision about the reliability of the information given by the patient.
Interview Coding
We coded the semistructured interviews in terms of decisions made, through iterative rounds of coding ( Chi, 1997 ), following a “directed content analysis approach,” which involves coding according to predefined theoretical categories and updating the codes as needed based on the data ( Hsieh and Shannon, 2005 ). Our predefined categories were the list of decisions we had developed during the informal interviews. This approach means that we limited the focus of our qualitative analysis—we were able to test and refine the list of decisions, but we did not seek to identify all possible categories of approach to selecting and solving problems. The goals of each iterative round of coding are described in the next three paragraphs. To code for decisions in general, we matched decisions from the list to statements in each interview, based on the following criteria: 1) there was an explicit statement of a decision or choice made or needing to be made; 2) there was the description of the outcome of a decision, such as listing important features of the problem (that had been decided on) or conclusions arrived at; or 3) there was a statement of actions taken that indicated a decision about the appropriate action had been made, usually from a set of alternatives. Two examples illustrate the types of comments we identified as decisions: A molecular biologist explicitly stated the decisions required to decompose a problem into subproblems (decision 11), “Which cell do we use? The gene. Which gene do we edit? Which part of that gene do we edit? How do we build the enzyme that is going to do the cutting? … And how do we read out that it worked?” An ecologist made a statement that was also coded as a decomposition decision, because it described the action taken: “So I analyze the bird data first on its own, rather than trying to smash all the taxonomic groups together because they seem really apples and oranges. And just did two kinds of analysis, one was just sort of across all of these cases, around the world.” A single statement could be coded as multiple decisions if they were occurring simultaneously in the story being recalled or were intimately interconnected in the context of that interview, as with the ecology quote, in which the last sentence leads into deciding what data analysis is needed. Inherent in nearly every one of these decisions was that there was insufficient information to know the answer with certainty, so judgment was required.
Our primary goal for the first iterative round of coding was to check whether our list was complete by checking for any decisions that were missing, as indicated by either an action taken or a stated decision that was not clearly connected to a decision on our initial list. In this round, we also clarified wording and combined decisions that we were consistently unable to differentiate during the coding. A sample of three interviews (from biology, medicine, and electrical engineering) were first coded independently by four coders (AP, EB, CK, and AF), then discussed. The decision list was modified to add decisions and update wording based on that discussion. Then the interviews were recoded with the new list and rediscussed, leading to more refinements to the list. Two additional interviews (from physics and chemical engineering) were then coded by three coders (AP, EB, and CK) and further similar refinements were made. Throughout the subsequent rounds of coding, we continued to check for missing decisions, but after the additions and adjustments made based on these five interviews, we did not identify any more missing decisions.
In our next round of coding, we focused on condensing overlapping decisions and refining wording to improve the clarity of descriptions as they applied across different disciplinary contexts and to ensure consistent interpretation by different coders. Two or three coders independently coded an additional 11 interviews, iteratively meeting to discuss codes identified in the interviews, refining wording and condensing the list to improve agreement and combine overlapping codes, and then using the updated list to code subsequent interviews. We condensed the list by combining decisions that represented the same cognitive process taking place at different times, that were discipline-specific variations on the same decision, or that were substeps involved in making a larger decision. We noticed that some decisions were frequently co-coded with others, particularly in some disciplines. But if they were identified as distinct a reasonable fraction of the time in any discipline, we listed them as separate. This provided us with a list, condensed from 42 to 29 discrete decisions (plus five additional non-decision themes that were so prevalent that they are important to describe), that gave good consistency between coders.
Finally, we used the resulting codes to tabulate which decisions occurred in each interview, simplifying our coding process to focus on deciding whether or not each decision had occurred, with an example if it did occur to back up the “yes” code, but no longer attempting to capture every time each decision was mentioned. Individual coders identified decisions mentioned in the remaining 15 interviews. Interviews that had been coded with the early versions of the list were also recoded to ensure consistency. Coders flagged any decisions they were unsure about occurring in a particular interview, and two to four coders (AP, EB, CK, and CW) met to discuss those debated codes, with most uncertainties being resolved by explanations from a team member who had more technical expertise in the field of the interview. Minor wording changes were made during this process to ensure that each description of a decision captured all instantiations of the decision across disciplines, but no significant changes to the list were needed or made.
Coding an interview in terms of decisions made and actions taken in the research often required a high level of expertise in the discipline in question. The coder had to be familiar with the conduct of research in the field in order to recognize which actions corresponded to a decision between alternatives, but our team was assembled with this requirement in mind. It included high-level expertise across five different fields of science, engineering, and medicine and substantial familiarity with several other fields.
Supplemental Table S1 shows the final tabulation of decisions identified in each interview. In the tabulation, most decisions were marked as either “yes” or “no” for each interview, though 65 out of 1054 total were marked as “implied,” for one of the following reasons: 1) for 40/65, based on the coder's knowledge of the field, it was clear that a step must have been taken to achieve an outcome or action, even though that decision was not explicitly mentioned (e.g., interviewees describe collecting certain raw data and then coming to a specific conclusion, so they must have decided how to analyze the data, even if they did not mention the analysis explicitly); 2) for 15/65, the interview context was important, in that multiple statements from different parts of the interview taken together were sufficient to conclude that the decision must have happened, though no single statement described that decision explicitly; 3) 10/65 involved a decision that was explicitly discussed as an important step in problem solving, but they did not directly state how it was related to the problem at hand, or it was stated only in response to a direct prompt from the interviewer. The proportion of decisions identified in each interview, broken down by either explicit or explicit + implied, is presented in Supplemental Tables S1 and S2. Table 2 and Figure 2 of the main text show explicit + implied decision numbers.
a See supplementary text and Table S2 for full description and examples of each decision. A set of other non-decision knowledge and skill development themes were also frequently mentioned as important to professional success: Staying up to date in the field (84%), intuition and experience (77%), interpersonal and teamwork (100%), efficiency (32%), and attitude (68%).
b Percentage of interviews in which category or decision was mentioned.
c Numbering is for reference. In practice ordering is fluid – involves extensive iteration with other possible starting points.
d Chosen predictive framework(s) will inform all other decisions.
e Reflection occurs throughout process, and often leads to iteration. Reflection on solution occurs at the end as well.
FIGURE 2. Proportion of decisions coded in interviews by field. This tabulation includes decisions 1–29, not the additional themes. Error bars represent standard deviations. Number of interviews: total = 31; physical science = 9; biological science = 8; engineering = 8; medicine = 6. Compared with the sciences, slightly fewer decisions overall were identified in the coding of engineering and medicine interviews, largely for discipline-specific reasons. See Supplemental Table S2 and associated discussion.
Two of the interviews that had not been discussed during earlier rounds of coding (one physics [AP and EB], one medicine [AP and CK]) were independently coded by two coders to check interrater reliability using the final list of decisions. The goal of our final coding was to tabulate whether or not each expert described making each decision at any point in the problem-solving process, so the level of detail we chose for coding and interrater reliability was whether or not a decision was present in the entire interview. The decisions identified in each interview were compared for the two coders. For both interviews, the raters disagreed on whether or not only one of the 29 decisions occurred. Codes of “implied” were counted as agreement if the other coder selected either “yes” or “implied.” This equates to a percent agreement of 97% for each interview (28 agree/29 total decisions per interview = 97%). As a side note, there was also one disagreement per interview on the coding of the five other themes, but those themes were not a focus of this work nor the interviews.
We identified a total set of 29 decisions to be made (plus five other themes), all of which were identified in a large fraction of the interviews across all disciplines ( Table 2 and Figure 2 ). There was a surprising degree of overlap across the different fields with all the experts mentioning similar decisions to be made. All 29 were evident by the fifth semistructured interview, and on average, each interview revealed 85% of the 29 decisions. Many decisions occurred multiple times in an interview, with the number of times varying widely, depending on the length and complexity of the problem-solving process discussed.
We focused our analysis on what decisions needed to be made, not on the experts’ processes for making those decisions: noting that a choice happened, not how they selected and chose among different alternatives. This is because, while the decisions to be made were the same across disciplines, how the experts made those decisions varied greatly by discipline and individual. The process of making the decisions relied on specialized disciplinary knowledge and experience and may vary depending on demographics or other factors that our study design (both our sample and nature of retrospective interviews) did not allow us to investigate. However, while that knowledge was distinct and specialized, we could tell that it was consistently organized according to a common structure we call a “predictive framework,” as discussed in the “ Predictive Framework ” section below. Also, while every “decision” reflected a step in the problem solving involved in the work, and the expert being interviewed was involved in making or approving the decision, that does not mean the decision process was carried out only by that individual. In many cases, the experts described the decisions made in terms of ideas and results of their teams, and the importance of interpersonal skills and teamwork was an important non-decision theme raised in all interviews.
We were particularly concerned with the correctness and completeness of the set of decisions. Although the correctness was largely established by the statements in the interviews, we also showed the list of decisions to these experts at the end of the interviews as well as to about a dozen other experts. In all cases, they all agreed that these decisions were ones they and others in their field made when solving problems. The completeness of the list of decisions was confirmed by: 1) looking carefully at all specific actions taken in the described problem-solving process and checking that each action matched a corresponding decision from the list; and 2) the high degree of consistency in the set of decisions across all the interviews and disciplines. This implies that it is unlikely that there are important decisions that we are missing, because that would require any such missing decisions to be consistently unspoken by all 31 interviewees as well as consistently unrecognized by us from the actions that were taken in the problem-solving process.
In focusing on experts’ recollections of their successful solving of problems, our study design may have missed decisions that experts only made during failed problem-solving attempts. However, almost all interviews described solution paths that were not smooth and continuous, but rather involved going down numerous dead ends. There were approaches that were tried and failed, data that turned out to be ambiguous and worthless, and so on. Identifying the failed path involved reflection decisions (23–26). Often decision 9 (is problem solvable?) would be mentioned, because it described a path that was determined to be not solvable. For example, a biologist explained, “And then I ended up just switching to a different strain that did it [crawling off the plate] less. Because it was just … hard to really get them to behave themselves. I suppose if I really needed to rely on that very particular one, I probably would have exhausted the possibilities a bit more.” Thus, we expect unsuccessful problem solving would entail a smaller subset of decisions being made, particularly lack of reflection decisions, or poor choices on the decisions, rather than making a different set of decisions.
The set of decisions represent a remarkably consistent structure underlying S&E problem solving. For the purposes of presentation, we have categorized the decisions as shown in Figure 3 , roughly based on the purposes they achieve. However, the process is far less orderly and sequential than implied by this diagram, or in fact any characterization of an orderly “scientific method.” We were struck by how variable the sequence of decisions was in the descriptions provided. For example, experts who described how they began work on a problem sometimes discussed importance and goals (1–3, what is important in field?; opportunity fits solver’s expertise?; and goals, criteria, constraints?), but others mentioned a curious observation (20, any significant anomalies?), important features of their system that led them to questions (4, important features and info?, 6, how to narrow down problem?), or other starting points. We also saw that there were flexible connections between decisions and repeated iterations—jumping back to the same type of decision multiple times in the solution process, often prompted by reflection as new information and insights were developed. The sequence and number of iterations described varied dramatically by interview, and we cannot determine to what extent this was due to legitimate differences in the problem-solving process or to how the expert recalled and chose to describe the process. This lack of a consistent starting point, with jumping and iterating between decisions, has also been identified in the naturalistic decision-making literature ( Mosier et al. , 2018 ). Finally, the experts also often described considering multiple decisions simultaneously. In some interviews, a few decisions were always described together, while in others, they were clearly separate decisions. In summary, while the specific decisions themselves are fully grounded in expert practice, the categories and order shown here are artificial simplifications for presentation purposes.
FIGURE 3. Representation of problem-solving decisions by categories. The black arrows represent a hypothetical but unrealistic order of operations, the blue arrows represent more realistic iteration paths. The decisions are grouped into categories for presentation purposes; numbers indicate the number of decisions in each category. Knowledge and skill development were commonly mentioned themes but are not decisions.
The decisions contained in the seven categories are summarized here. See Supplemental Table S2 for specific examples of each decision across multiple disciplines.
Category A. Selection and Goals of the Problem
This category involves deciding on the importance of the problem, what criteria a solution must meet, and how well it matches the capabilities, resources, and priorities of the expert. As an example, an earth scientist described the goal of her project (decision 3, goals, criteria, constraints?) to map and date the earliest volcanic rocks associated with what is now Yellowstone and explained why the project was a good fit for her group (2, opportunity fits solver’s expertise?) and her decision to pursue the project in light of the significance of this type of eruption in major extinction events (1, what is important in field?). In many cases, decisions related to framing (see category B) were mentioned before decisions in this category or were an integral part of the process for developing goals.
1. What is important in the field?
What are important questions or problems? Where is the field heading? Are there advances in the field that open new possibilities?
2. Opportunity fits solver's expertise?
If and where are there gaps/opportunities to solve in field? Given experts’ unique perspectives and capabilities, are there opportunities particularly accessible to them? (This could involve challenging the status quo, questioning assumptions in the field.)
3. Goals, criteria, constraints?
a. What are the goals, design criteria, or requirements of the problem or its solution?
b. What is the scope of the problem?
c. What constraints are there on the solution?
d. What will be the criteria on which the solution is evaluated?
Category B. Frame Problem
These decisions lead to a more concrete formulation of the solution process and potential solutions. This involves identifying the key features of the problem and deciding on predictive frameworks to use (see “ Predictive Framework ” section below), as well as narrowing down the problem, often forming specific questions or hypotheses. Many of these decisions are guided by past problem solutions with which the expert is familiar and sees as relevant. The framing decisions of a physician can be seen in his discussion of a patient with liver failure who had previously been diagnosed with HIV but had features (4, important features and info?; 5, what predictive framework?) that made the physician question the HIV diagnosis (5, what predictive framework?; 26, how good is solution?). His team then searched for possible diagnoses that could explain liver failure and lead to a false-positive HIV test (7, related problems?; 8, potential solutions?), which led to their hypothesis the patient might have Q fever (6, how to narrow down problem?; 13, what info needed?; 15, specific plan for getting info?). While each individual decision is strongly supported by the data, the categories are groupings for presentation purposes. In particular, framing (category B) and planning (see category C) decisions often blended together in interviews.
a. Which available information is relevant to problem solving and why?
b. (When appropriate) Create/find a suitable abstract representation of core ideas and information Examples: physics, equation representing process involved; chemistry, bond diagrams/potential energy surfaces; biology, diagram of pathway steps.
5. What predictive framework?
Which potential predictive frameworks to use? (Decide among possible predictive frameworks or create framework.) This includes deciding on the appropriate level of mechanism and structure that the framework needs to embody to be most useful for the problem at hand.
6. How to narrow down the problem?
How to narrow down the problem? Often involves formulating specific questions and hypotheses.
7. Related problems?
What are related problems or work seen before, and what aspects of their problem-solving process and solutions might be useful in the present context? (This may involve reviewing literature and/or reflecting on experience.)
8. Potential solutions?
What are potential solutions? (This is based on experience and fitting some criteria for solution they have for a problem having general key features identified.)
9. Is problem solvable?
Is the problem plausibly solvable and is the solution worth pursuing given the difficulties, constraints, risks, and uncertainties?
Category C. Plan the Process for Solving
These decisions establish the specifics needed to solve the problem and include: how to simplify the problem and decompose it into pieces, what specific information is needed, how to obtain that information, and what are the resources needed and priorities? Planning by an ecologist can be seen in her extensive discussion of her process of simplifying (10, approximations/simplifications to make?) a meta-analysis project about changes in migration behavior, which included deciding what types of data she needed (13, what info needed?), planning how to conduct her literature search (15, specific plan for getting info?), difficulties in analyzing the data (12, most difficult/uncertain areas?; 16, which calculations and data analysis?), and deciding to analyze different taxonomic groups separately (11, how to decompose into subproblems?). In general, decomposition often resulted in multiple iterations through the problem-solving decisions, as subsets of decisions need to be made about each decomposed aspect of a problem. Framing (category B) and planning (category C) decisions occupied much of the interviews, indicating their importance.
10. Approximations and simplifications to make?
What approximations or simplifications are appropriate? How to simplify the problem to make it easier to solve? Test possible simplifications/approximations against established criteria.
11. How to decompose into subproblems?
How to decompose the problem into more tractable subproblems? (Subproblems are independently solvable pieces with their own subgoals.)
12. Most difficult or uncertain areas?
a. What are acceptable levels of uncertainty with which to proceed at various stages?
13. What info needed?
a. What will be sufficient to test and distinguish between potential solutions?
14. Priorities?
What to prioritize among many competing considerations? What to do first and how to obtain necessary resources?
Considerations could include: What's most important? Most difficult? Addressing uncertainties? Easiest? Constraints (time, materials, etc.)? Cost? Optimization and trade-offs? Availability of resources? (facilities/materials, funding sources, personnel)
15. Specific plan for getting information?
a. What are the general requirements of a problem-solving approach, and what general approach will they pursue? (These decisions are often made early in the problem-solving process as part of framing.)
b. How to obtain needed information? Then carry out those plans. (This could involve many discipline- and problem-specific investigation possibilities such as: designing and conducting experiments, making observations, talking to experts, consulting the literature, doing calculations, building models, or using simulations.)
c. What are achievable milestones, and what are metrics for evaluating progress?
d. What are possible alternative outcomes and paths that may arise during the problem-solving process, both consistent with predictive framework and not, and what would be paths to follow for the different outcomes?
Category D. Interpret Information and Choose Solution(s)
This category includes deciding how to analyze, organize, and draw conclusions from available information, reacting to unexpected information, and deciding upon a solution. A biologist studying aging in worms described how she analyzed results from her experiments, which included representing her results in survival curves and conducting statistical analyses (16, which calculations and data analysis?; 17, how to represent and organize info?), as well as setting up blind experiments (15, specific plan for getting info?) so that she could make unbiased interpretations (18, how believable is info?) of whether a worm was alive or dead. She also described comparing results with predictions to justify the conclusion that worm aging was related to fertility (19, how does info compare to predictions?; 21, appropriate conclusions?; 22, what is best solution?). Deciding how results compared with expectations based on a predictive framework was a key decision that often preceded several other decisions.
16. Which calculations and data analysis?
What calculations and data analysis are needed? Once determined, these must then be carried out.
17. How to represent and organize information?
What is the best way to represent and organize available information to provide clarity and insights? (Usually this will involve specialized and technical representations related to key features of predictive framework.)
18. How believable is the information?
Is information valid, reliable, and believable (includes recognizing potential biases)?
19. How does information compare to predictions?
As new information comes in, particularly from experiments or calculations, how does it compare with expected results (based on the predictive framework)?
20. Any significant anomalies?
a. Does potential anomaly fit within acceptable range of predictive framework(s) (given limitations of predictive framework and underlying assumptions and approximations)?
b. Is potential anomaly an unusual statistical variation or relevant data? Is it within acceptable levels of uncertainty?
21. Appropriate conclusions?
What are appropriate conclusions based on the data? (This involves making conclusions and deciding if they are justified.)
22. What is the best solution?
a. Which of multiple candidate solutions are consistent with all available information and which can be rejected? (This could be based on comparing data with predicted results.)
b. What refinements need to be made to candidate solutions?
Category E. Reflect
Reflection decisions occur throughout the process and include deciding whether assumptions are justified, whether additional knowledge or information is needed, how well the solution approach is working, and whether potential and then final solutions are adequate. These decisions match the categories of reflection identified by Salehi (2018) . A mechanical engineer described developing a model (to inform surgical decisions) of which muscles allow the thumb to function in the most useful manner (22, what is best solution?), including reflecting on how well engineering approximations applied in the biological context (23, assumptions and simplifications appropriate?). He also described reflecting on his approach, that is, why he chose to use cadaveric models instead of mathematical models (25, how well is solving approach working?), and the limitations of his findings in that the “best” muscle identified was difficult to access surgically (26, how good is solution?; 27, broader implications?). Reflection decisions are made throughout the problem-solving process, often lead to reconsidering other decisions, and are critical for success.
23. Assumptions and simplifications appropriate?
a. Do the assumptions and simplifications made previously still look appropriate considering new information?
b Does predictive framework need to be modified?
24. Additional knowledge needed?
a. Is solver's relevant knowledge sufficient?
b. Is more information needed and, if so, what?
c. Does some information need to be checked? (Is there a need to repeat experiment or check a different source?)
25. How well is the problem-solving approach working?
How well is the problem-solving approach working, and does it need to be modified? This includes possibly modifying the goals. (One needs to reflect on one's strategy by evaluating progress toward the solution.) and reflecting on one’s strategy by evaluating progress toward the solution.
26. How good is the solution?
a. Decide by exploring possible failure modes and limitations—“try to break” solution.
b. Does it “make sense” and pass discipline-specific tests for solutions of this type of problem?
c. Does it completely meet the goals/criteria?
Category F. Implications and Communication of Results
These are decisions about the broader implications of the work, and how to communicate results most effectively. For example, a theoretical physicist developing a method to calculate the magnetic moment of the muon decided on who would be interested in his work (28, audience for communication?) and what would be the best way to present it (29, best way to present work?). He also discussed the implications of preliminary work on a simplified aspect of the problem (10, approximations and simplifications to make?) in terms of evaluating its impact on the scientific community and deciding on next steps (27, broader implications?; 29, best way to present work?). Many interviewees described that making decisions in this category affected their decisions in other categories.
27. Broader implications?
What are the broader implications of the results, including over what range of contexts does the solution apply? What outstanding problems in the field might it solve? What novel predictions can it enable? How and why might this be seen as interesting to a broader community?
28. Audience for communication?
What is the audience for communication of work, and what are their important characteristics?
29. Best way to present work?
What is the best way to present the work to have it understood, and its correctness and importance appreciated? How to make a compelling story of the work?
Category G. Ongoing Skill and Knowledge Development
Although we focused on decisions in the problem-solving process, the experts volunteered general skills and knowledge they saw as important elements of problem-solving expertise in their fields. These included teamwork and interpersonal skills (strongly emphasized), acquiring experience and intuition, and keeping abreast of new developments in their fields.
30. Stay up to date in field
a. Reviewing literature, which does involve making decisions as to which is important.
b. Learning relevant new knowledge (ideas and technology from literature, conferences, colleagues, etc.)
31. Intuition and experience
Acquiring experience and associated intuition to improve problem solving.
32. Interpersonal, teamwork
Includes navigating collaborations, team management, patient interactions, communication skills, etc., particularly as how these apply in the context of the various types of problem-solving processes.
33. Efficiency
Time management including learning to complete certain common tasks efficiently and accurately.
34. Attitude
Motivation and attitude toward the task. Factors such as interest, perseverance, dealing with stress, and confidence in decisions.
Predictive Framework
How the decisions were made was highly dependent on the discipline and problem. However, there was one element that was fundamental and common across all interviews: the early adoption of a “predictive framework” that the experts used throughout the problem-solving process. We define this framework as “a mental model of key features of the problem and the relationships between the features.” All the predictive frameworks involved some degree of simplification and approximation and an underlying level of mechanism that established the relationships between key features. The frameworks provided a structure of knowledge and facilitated the application of that knowledge to the problem at hand, allowing experts to repeatedly run “mental simulations” to make predictions for dependencies and observables and to interpret new information.
As an example, an ecologist described her predictive framework for migration, which incorporated important features such as environmental conditions and genetic differences between species and the mechanisms by which these interacted to impact the migration patterns for a species. She used this framework to guide her meta-analysis of changes in migration patterns, affecting everything from her choice of data sets to include to her interpretation of why migration patterns changed for different species. In many interviews, the frameworks used evolved as additional information was obtained, with additional features being added or underlying assumptions modified. For some problems, the relevant framework was well established and used with confidence, while for other problems, there was considerable uncertainty as to a suitable framework, so developing and testing the framework was a substantial part of the solution process.
A predictive framework contains the expert knowledge organization that has been observed in previous studies of expertise ( Egan and Greeno, 1974 ) but goes further, as here it serves as an explicit tool that guides most decisions and actions during the solving of complex problems. Mental models and mental simulations that are described in the naturalistic decision-making literature are similar, in that they are used to understand the problem and guide decisions ( Klein, 2008 ; Mosier et al. , 2018 ), but they do not necessarily contain the same level of mechanistic understanding of relationships that underlies the predictive frameworks used in science and engineering problem solving. While the use of predictive frameworks was universal, the individual frameworks themselves explicitly reflected the relevant specialized knowledge, structure, and standards of the discipline, and arguably largely define a discipline ( Wieman, 2019 ).
Discipline-Specific Variation
While the set of decisions to be made was highly consistent across disciplines, there were extensive differences within and across disciplines and work contexts, which reflected the differences in perspectives and experiences. These differences were usually evident in how experts made each of the specific decisions, but not in the choice of which decisions needed to be made. In other words, the solution methods, which included following standard accepted procedures in each field, were very different. For example, planning in some experimental sciences may involve formulating a multiyear construction and data-collection effort, while in medicine it may be deciding on a simple blood test. Some decisions, notably in categories A, D, and F, were less likely to be mentioned in particular disciplines, because of the nature of the problems. Specifically, decisions 1 (what is important in field?), 2 (opportunity fits solver’s expertise?), 27 (broader implications?), 28 (audience for communication?), and 29 (best way to present work?) were dependent on the scope of the problem being described and the expert's specific role in it. These were mentioned less frequently in interviews where the problem was assigned to the expert (most often engineering or industry) or where the importance or audience was implicit (most often in medicine). Decisions 16 (which calculations and data analysis?) and 17 (how to represent and organize info?) were particularly unlikely to be mentioned in medicine, because test results are typically provided to doctors not in the form or raw data, but rather already analyzed by a lab or other medical technology professional, so the doctors we interviewed did not need to make decisions themselves about how to analyze or represent the data. Qualitatively, we also noticed some differences between disciplines in the patterns of connections between decisions. When the problem involved development of a tool or product, most commonly the case in engineering, the interview indicated relatively rapid cycles between goals (3), framing problem/potential solutions (8), and reflection on the potential solution (26), before going through the other decisions. Biology, the experimental science most represented in our interviews, had strong links between planning (15), deciding on appropriate conclusions (21), and reflection on the solution (26). This is likely because the respective problems involved complex systems with many unknowns, so careful planning was unusually important for achieving definitive conclusions. See Supplemental Text and Supplemental Table S2 for additional notes on decisions that were mentioned at lower frequency and decisions that were likely to be interconnected, regardless of field.
This work has created a framework of decisions to characterize problem solving in science and engineering. This framework is empirically based and captures the successful problem-solving process of all experts interviewed. We see that several dozen experts across many different fields all make a common set of decisions when solving authentic problems. There are flexible linkages between decisions that are guided by reflection in a continually evolving process. We have also identified the nature of the “predictive frameworks” that S&E experts consistently use in problem solving. These predictive frameworks reveal how these experts organize their disciplinary knowledge to facilitate making decisions. Many of the decisions we identified are reflected in previous work on expertise and scientific problem solving. This is particularly true for those listed in the planning and interpreting information categories ( Egan and Greeno, 1974 ). The priority experts give to framing and planning decisions over execution compared with novices has been noted repeatedly (e.g., Chi et al. , 1988 ). Expert reflection has been discussed, but less extensively ( Chase and Simon, 1973 ), and elements of the selection and implications and communication categories have been included in policy and standards reports (e.g., AAAS, 2011 ). Thus, our framework of decisions is consistent with previous work on scientific practices and expertise, but it is more complete, specific, empirically based, and generalizable across S&E disciplines.
A limitation of this study is the small number of experts we have in total, from each discipline, and from underrepresented groups (especially lack of female representation in engineering). The lack of randomized selection of participants may also bias the sample toward experts who experienced similar academic training (STEM disciplines at U.S. universities). This means we cannot prove that there are not some experts who follow other paths in problem solving. As with any scientific model, the framework described here should be subjected to further tests and modifications as necessary. However, to our knowledge, this is a far larger sample than used in any previous study of expert problem solving. Although we see a large amount of variation both within and across disciplines in the problem-solving process, this is reflected in how experts make decisions, not in what decisions they make. The very high degree of consistency in the decisions made across the entire sample strongly suggests that we are capturing elements that are common to all experts across science and engineering. A second limitation is that decisions often overlap and co-occur in an interview, so the division between decision items is often somewhat ambiguous and could be defined somewhat differently. As noted, a number of these decisions can be interconnected, and in some fields are nearly always interconnected.
The set of decisions we have observed provides a general framework for characterizing, analyzing, and teaching S&E problem solving. These decisions likely define much of the set of cognitive skills a student needs to practice and master to perform as a skilled practitioner in S&E. This framework of decisions provides a detailed and structured way to approach the teaching and measurement of problem solving at the undergraduate, graduate, and professional training levels. For teaching, we propose using the process of “deliberate practice” ( Ericsson, 2018 ) to help students learn problem solving. Deliberate practice of problem solving would involve effective scaffolding and concentrated practice, with feedback, at making the specific decisions identified here in relevant contexts. In a course, this would likely involve only an appropriately selected set of the decisions, but a good research mentor would ensure that trainees have opportunities to practice and receive feedback on their performance on each of these 29 decisions. Future work is needed to determine whether there are additional decisions that were not identified in experts but are productive components of student problem solving and should also be practiced. Measurements of individual problem-solving expertise based on our decision list and the associated discipline-specific predictive frameworks will allow a detailed measure of an individual's discipline-specific problem-solving strengths and weaknesses relative to an established expert. This can be used to provide targeted feedback to the learner, and when aggregated across students in a program, feedback on the educational quality of the program. We are currently working on the implementation of these ideas in a variety of instructional settings and will report on that work in future publications.
As discussed in the Introduction , typical science and engineering problems fail to engage students in the complete problem-solving process. By considering which of the 29 decisions are required to answer the problem, we can more clearly articulate why. The biology problem, for example, requires students to decide on a predictive framework and access the necessary content knowledge, and they need to decide which information they need to answer the problem. However, other decisions are not required or are already made for them, such as deciding on important features and identifying anomalies. We propose that different problems, designed specifically to require students to make sets of the problem-solving decisions from our framework, will provide more effective tools for measuring, practicing, and ultimately mastering the full S&E problem-solving process.
Our preliminary work with the use of such decision-based problems for assessing problem-solving expertise is showing great promise. For several different disciplines, we have given test subjects a relevant context, requiring content knowledge covered in courses they have taken, and asked them to make decisions from the list presented here. Skilled practitioners in the relevant discipline respond in very consistent ways, while students respond very differently and show large differences that typically correlate with their different educational experiences. What apparently matters is not what content they have seen, but rather what decisions they have had practice making. Our approach was to identify the decisions made by experts, this being the task that educators want students to master. Our data do not exclude the possibility that students engage in and/or should learn other decisions as a productive part of the problem-solving process while they are learning. Future work would seek to identify decisions made at intermediate levels during the development of expertise, to identify potential learning progressions that could be used to teach problem solving more efficiently. What we have seen is consistent with previous work identifying expert–novice differences but provides a much more extensive and detailed picture of a student's strengths and weaknesses and the impacts of particular educational experiences. We have also carried out preliminary development of courses that explicitly involve students making and justifying many of these decisions in relevant contexts, followed by feedback on their decisions. Preliminary results from these courses are also encouraging. Future work will involve the more extensive development and application of decision-based measurement and teaching of problem solving.
ACKNOWLEDGMENTS
We acknowledge the many experts who agreed to be interviewed for this work, M. Flynn for contributions on expertise in mechanical engineering, and Shima Salehi for useful discussions. This work was funded by the Howard Hughes Medical Institute through an HHMI Professor grant to C.E.W.
- ABET . ( 2020 ). Criteria for accrediting engineering programs, 2020–2021 . Retrieved November 23, 2020, from www.abet.org/accreditation/accreditation-criteria/criteria-for-accrediting-engineering-programs-2020-2021 Google Scholar
- Alberts, B., Johnson, A., Lewis, J., Morgan, D., Raff, M., Roberts, K., & Walter, P. ( 2014 ). Control of gene expression . In Molecular Biology of the Cell (6th ed., pp. 436–437). New York: Garland Science. Retrieved November 12, 2020, from https://books.google.com/books?id=2xIwDwAAQBAJ Google Scholar
- American Association for the Advancement of Science . ( 2011 ). Vision and change in undergraduate biology education: A call to action . Washington, DC. Retrieved February 12, 2021, from https://visionandchange.org/finalreport Google Scholar
- Chi, M. T. H., Glaser, R., & Farr, M. J.( ( 1988 ). The nature of expertise . Hillsdale, NJ: Erlbaum. Google Scholar
- Crandall, B., Klein, G. A., & Hoffman, R. R. ( 2006 ). Working minds: A practitioner's guide to cognitive task analysis . Cambridge, MA: MIT Press. Google Scholar
- Egan, D. E., & Greeno, J. G. ( 1974 ). Theory of rule induction: Knowledge acquired in concept learning, serial pattern learning, and problem solving in L . In Gregg, W. (Ed.), Knowledge and cognition . Potomac, MD: Erlbaum. Google Scholar
- Ericsson, K. A., Charness, N., Feltovich, P. J., & Hoffman, R. R. , (Eds.) ( 2006 ). The Cambridge handbook of expertise and expert performance . Cambridge, United Kingdom: Cambridge University Press. Google Scholar
- Ericsson, K. A., Hoffman, R. R., Kozbelt, A., & Williams, A. A. , (Eds.) ( 2018 ). The Cambridge handbook of expertise and expert performance (2nd ed.). Cambridge, United Kingdom: Cambridge University Press. Google Scholar
- Hatano, G., & Inagaki, K. ( 1986 ). Two courses of expertise . In Stevenson, H. W.Azuma, H.Hakuta, K. (Eds.), A series of books in psychology. Child development and education in Japan (pp. 262–272). New York: Freeman/Times Books/Henry Holt. Google Scholar
- Klein, G. ( 2008 ). Naturalistic decision making . Human Factors , 50 (3), 456–460. Medline , Google Scholar
- Kozma, R., Chin, E., Russell, J., & Marx, N. ( 2000 ). The roles of representations and tools in the chemistry laboratory and their implications for chemistry learning . Journal of the Learning Sciences , 9 (2), 105–143. Google Scholar
- Lintern, G., Moon, B., Klein, G., & Hoffman, R. ( 2018 ). Eliciting and representing the knowledge of experts . In Ericcson, K. A.Hoffman, R. R.Kozbelt, A.Williams, A. M. (Eds.), The Cambridge handbook of expertise and expert performance (2nd ed). (pp. 165–191). Cambridge, United Kingdom: Cambridge University Press. Google Scholar
- Mosier, K., Fischer, U., Hoffman, R. R., & Klein, G. ( 2018 ). Expert professional judgments and “naturalistic decision making.” In Ericcson, K. A.Hoffman, R. R.Kozbelt, A.Williams, A. M. (Eds.), The Cambridge handbook of expertise and expert performance (2nd ed). (pp. 453–475). Cambridge, United Kingdom: Cambridge University Press. Google Scholar
- National Research Council (NRC) . ( 2012a ). A framework for K–12 science education: Practices, crosscutting concepts, and core ideas . Washington, DC: National Academies Press. Google Scholar
- Newell, A., & Simon, H. A. ( 1972 ). Human problem solving . Prentice-Hall. Google Scholar
- Next Generation Science Standards Lead States . ( 2013 ). Next Generation Science Standards: For states, by states . Washington, DC: National Academies Press. Google Scholar
- Polya, G. ( 1945 ). How to solve it: A new aspect of mathematical method . Princeton, NJ: Princeton University Press. Google Scholar
- Quacquarelli Symonds . ( 2018 ). The global skills gap in the 21st century . Retrieved July 20, 2021, from www.qs.com/portfolio-items/the-global-skills-gap-in-the-21st-century/ Google Scholar
- Salehi, S. ( 2018 ). Improving problem-solving through reflection (Doctoral dissertation) . Stanford Digital Repository, Stanford University. Retrieved February 18, 2021, from https://purl.stanford.edu/gc847wj5876 Google Scholar
- Schoenfeld, A. H. ( 1985 ). Mathematical problem solving . Orlando, FL: Academic Press. Google Scholar
- Wayne State University . ( n.d ). Mechanical engineering practice qualifying exams. Wayne State University Mechanical Engineering department . Retrieved February 23, 2021, from https://engineering.wayne.edu/me/exams/mechanics_of_materials_-_sample_pqe_problems_.pdf Google Scholar
- Wineburg, S. ( 1998 ). Reading Abraham Lincoln: An expert/expert study in the interpretation of historical texts . Cognitive Science , 22 (3), 319–346. https://doi.org/10.1016/S0364-0213(99)80043-3 Google Scholar
- Uncovering students’ problem-solving processes in game-based learning environments Computers & Education, Vol. 182
- Student understanding of kinematics: a qualitative assessment 9 May 2022 | European Journal of Engineering Education, Vol. 5
- What decisions do experts make when doing back-of-the-envelope calculations? 5 April 2022 | Physical Review Physics Education Research, Vol. 18, No. 1
- Simulation led optical design assessments: Emphasizing practical and computational considerations in an upper division physics lecture course American Journal of Physics, Vol. 90, No. 4
- Evidence-Based Principles for Worksheet Design The Physics Teacher, Vol. 59, No. 6
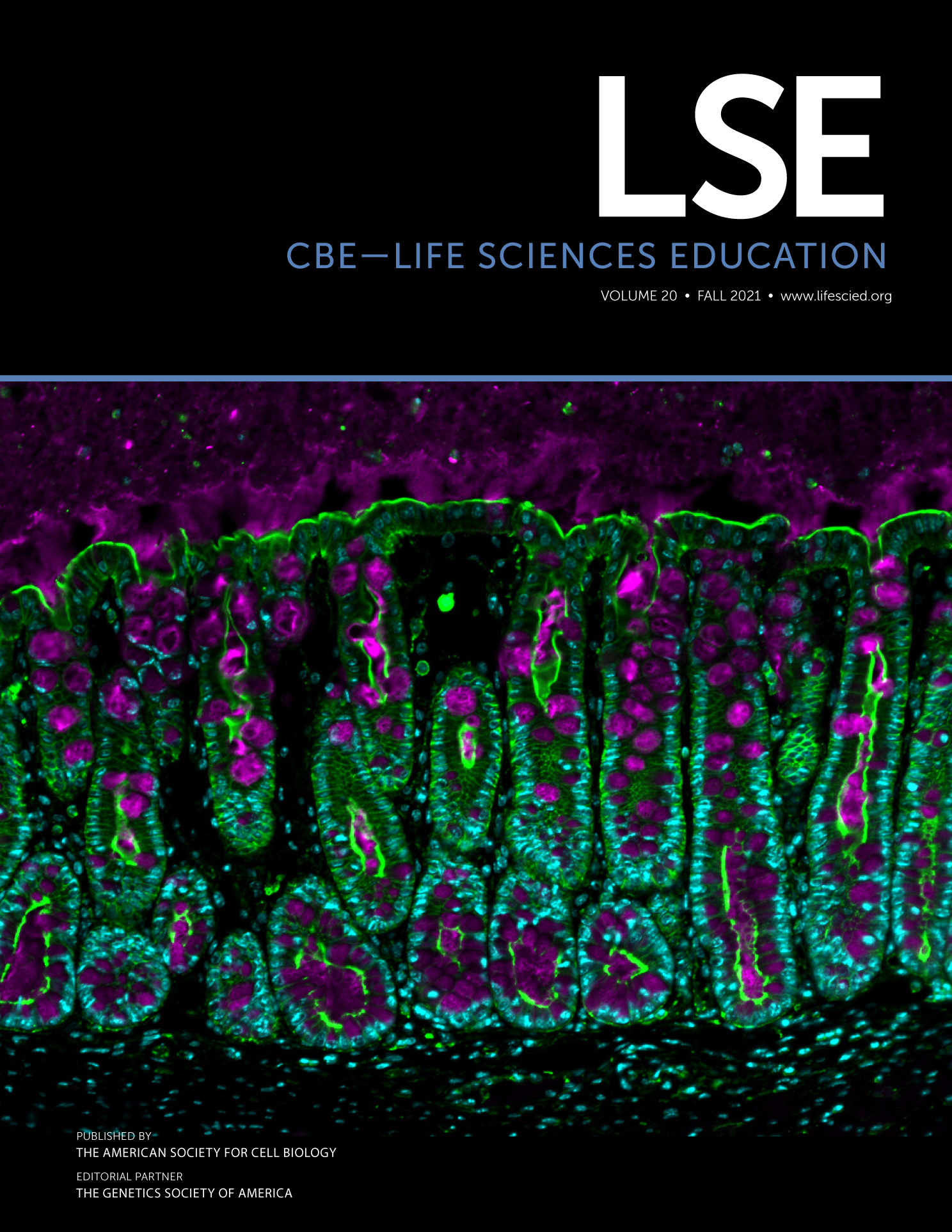
Submitted: 2 December 2020 Revised: 11 June 2021 Accepted: 23 June 2021
© 2021 A. M. Price et al. CBE—Life Sciences Education © 2021 The American Society for Cell Biology. This article is distributed by The American Society for Cell Biology under license from the author(s). It is available to the public under an Attribution–Noncommercial–Share Alike 3.0 Unported Creative Commons License (http://creativecommons.org/licenses/by-nc-sa/3.0).
To read this content please select one of the options below:
Please note you do not have access to teaching notes, expert systems in production and operations management: current applications and future prospects.
International Journal of Operations & Production Management
ISSN : 0144-3577
Article publication date: 1 December 1996
Defines an expert system (ES) as a decision‐making tool that captures perishable expertise from an expert and stores this knowledge in the computer, and also as a computer system that can solve problems using human expertise and knowledge of the system environment. Notes that expert systems have found many applications in the area of production and operations management (POM). Explains the key role of the ES as a management tool to enhance productivity, improve quality, increase profits and capture expertise in many business settings. Highlights that the field of POM has a heavy orientation towards problem solving and decision making, hence it is a very fertile area for application for ES. Evaluates the current use of ES in POM and discusses future prospects.
- Expert systems
- Operations management
Jayaraman, V. and Srivastava, R. (1996), "Expert systems in production and operations management: Current applications and future prospects", International Journal of Operations & Production Management , Vol. 16 No. 12, pp. 27-44. https://doi.org/10.1108/01443579610151742
Copyright © 1996, MCB UP Limited
Related articles
We’re listening — tell us what you think, something didn’t work….
Report bugs here
All feedback is valuable
Please share your general feedback
Join us on our journey
Platform update page.
Visit emeraldpublishing.com/platformupdate to discover the latest news and updates
Questions & More Information
Answers to the most commonly asked questions here
Clinical problem solving--the role of expert laboratory systems
Affiliation.
- 1 Repatriation General Hospital, Greenslopes, Brisbane, Australia.
- PMID: 9364433
- DOI: 10.3109/14639239709010897
The objective was to determine whether or not a laboratory based computer diagnostic program could aid the clinician in solving problems, outside his or her field of expertise, by expertly interpreting ¿Emergency Room' haematological and biochemical data and providing a list of possible diagnoses. The program, which uses Fuzzy Sets and pattern recognition as its Inference Mechanism coupled with a data base comprised of haematological and biochemical responses to disease collected over a period of 10 years in a teaching hospital, analysed data published in two leading journals--the 'Clinical Problem-Solving' section of the New England Journal of Medicine and the 'Lesson of the Week' feature of the British Medical Journal. It was found that the computer program often presented diagnoses not thought of by the clinician. With such a system, sometimes as few as three routine investigations suggested the diagnosis. The diagnostic accuracy could be improved with a more structured approach to ¿Emergency Room' laboratory investigations. It is concluded that the computer, programmed to recognize a disease by the pattern of its response to routine haematological and biochemical investigations, could contribute significantly to diagnosis.
- Clinical Laboratory Information Systems*
- Diagnosis, Computer-Assisted*
- Emergencies
- Expert Systems*
- Fuzzy Logic
- Problem Solving
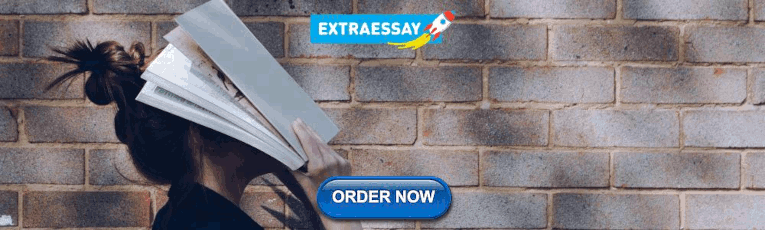
IMAGES
VIDEO
COMMENTS
An expert system is designed to mimic the problem-solving abilities of a human expert in a specific domain. By capturing and formalizing knowledge from domain experts, an expert system can provide intelligent solutions to complex problems. ... Revolutionizing Decision-Making Processes The Role of Expert Systems in Artificial Intelligence ...
As one of the earliest practical applications of AI, Expert Systems played a pivotal role in demonstrating the potential of machine-based decision-making and problem-solving within specific domains. The impact of Expert Systems can be understood through several key aspects:
The primary role of a knowledge engineer is to make sure that the computer possesses all the knowledge required to solve a problem. The knowledge engineer must choose one or more forms in which to represent the required knowledge as a symbolic pattern in the memory of the computer. ... Expert systems can solve complex problems by deducing new ...
Expert systems are a branch of artificial intelligence that aim to provide computerized decision-making capabilities similar to those of a human expert in a specific domain. They are designed to solve complex problems using a set of rules or algorithms that can mimic human reasoning processes. In this tutorial, we'll overview the expert ...
Expert systems can analyze larger datasets than human experts, offering insights that might not be immediately apparent to humans. Lack of Intuition: Despite their analytical capabilities, expert systems cannot replicate the intuitive and creative problem-solving abilities of humans, which can be crucial in certain contexts.
AI expert systems, also known as knowledge-based systems, are a subfield of artificial intelligence that utilizes human expertise to solve complex problems. These systems can learn from historical data and make decisions based on predefined rules or algorithms. Expert systems have been used in various industries such as finance, healthcare, and ...
Artificial Intelligence and its subfield Expert Systems have reached a level of maturity, particularly in recent years, and have evolved to the point that a Knowledge-Based Expert System may reach a level of performance comparable to that of a human expert in specialized problem domains like, Computer Systems, Computing, Education, Engineering, Knowledge Engineering, Geology, Medicine and Science.
An expert system has some capabilities: (i) superior problem solving—only solvable problems, (ii) ability to save and apply knowledge and experience to problems, (iii) reduced time for complex problems, and (iv) looks at problems from a variety of perspectives. An expert system has five basic components: knowledge base, inference engine ...
expert system, a computer program that uses artificial-intelligence methods to solve problems within a specialized domain that ordinarily requires human expertise. The first expert system was developed in 1965 by Edward Feigenbaum and Joshua Lederberg of Stanford University in California, U.S. Dendral, as their expert system was later known, was designed to analyze chemical compounds.
of a type called Expert Systems. An Expert System (ES) is a computer program that reasons using knowledge to solve complex problems. This overly brief caricature will be expanded upon below, but it serves to indicate an alignment of ES with AI's long term goals. Traditionally, computers solve complex problems by arithmetic calculation (not ...
The four main components of an expert system are the knowledge base, search or inference engine, knowledge acquisition system, and user interface or communication system. The basic goal of an expert is to duplicate human experts and replace them with problem-solving. There are five main types of expert systems: rule-based systems, frame-based ...
Expert systems are computer programs that exhibit some of the characteristics of expertise in human problem solving, most notably high levels of performance. Several issues are described that are relevant for the study of expertise and that have arisen in the development of the technology.
What is an Expert System? Jackson (1999) provides us with the following definition: An expert system is a computer program that represents and reasons with knowledge of some specialist subject with a view to solving problems or giving advice. To solve expert-level problems, expert systems will need efficient access to a
Nevertheless, many problem-solving systems have been constructed ... 4.2.1 THE ROLE OF EXPERT SYSTEMS When the intellectual process by which a person evaluates a situation or takes a decision is precisely modelled, it is relatively easy to program. This is the case, for example, in such areas as accountancy, scientific ...
Competence: on account of the expert knowledge, expert systems having a high problem-solving capability in their domain. 3.2.2 Rationale for a Web-Based System. The web based expert systems organize information into two bases, a knowledge base and an inference engine or rule interpreter.
The ES has an essential role in PATequation; it is responsible for providing immediate feedback to students at every step of their problem solving. ... PATequation is comprised of an Editor (Fig. 1) where students exercise problem solving and an Expert System that provides step-by-step guidance for students. In Fig. 1, on the green blackboard ...
7 Expert Systems: A Perspective from Computer Science; 8 Developing Occupational Expertise through Everyday Work Activities and Interactions; 9 Professionalism, Science, and Expert Roles: A Social Perspective; Part III Methods for Studying the Structure of Expertise; Part IV Methods for Studying the Acquisition and Maintenance of Expertise
A primary goal of science and engineering (S&E) education is to produce good problem solvers, but how to best teach and measure the quality of problem solving remains unclear. The process is complex, multifaceted, and not fully characterized. Here, we present a detailed characterization of the S&E problem-solving process as a set of specific interlinked decisions. This framework of decisions ...
Intelligent Systems Division, M/S 269-3 NASA Ames Research Center Moffett Field, CA 94035 650-604-2526, fax 4-4036. Short Title: "The Role of Method Abstraction in Work Systems Design" Number of pages (excluding title, abstract, and biographies): 55 Number of figures: 2. Workflow Agents vs. Expert Systems: Problem Solving Methods in Work ...
Defines an expert system (ES) as a decision‐making tool that captures perishable expertise from an expert and stores this knowledge in the computer, and also as a computer system that can solve problems using human expertise and knowledge of the system environment. Notes that expert systems have found many applications in the area of ...
Clinical problem solving--the role of expert laboratory systems Med Inform (Lond). Jul-Sep 1997;22(3):251-61. doi: 10.3109/14639239709010897. Author M D Innis 1 ... With such a system, sometimes as few as three routine investigations suggested the diagnosis. The diagnostic accuracy could be improved with a more structured approach to ...