Science Essay

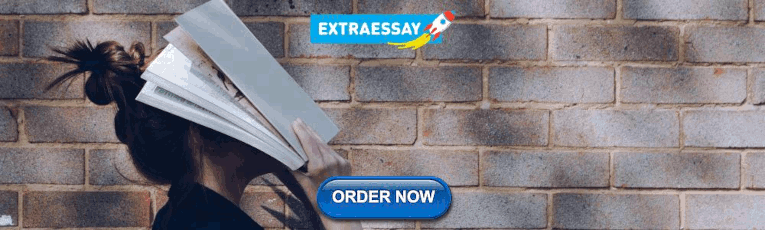
Learn How to Write an A+ Science Essay
11 min read
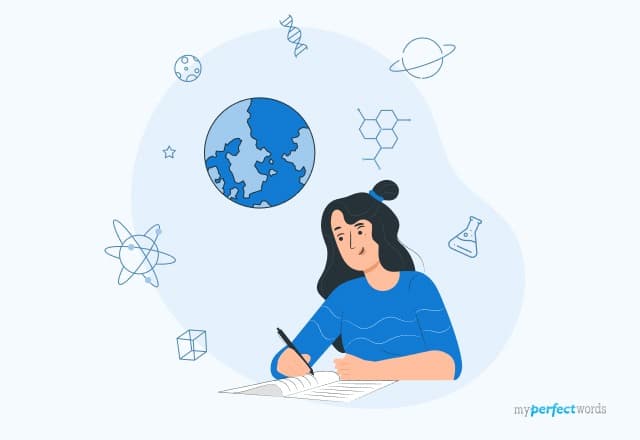
People also read
150+ Engaging Science Essay Topics To Hook Your Readers
Read 8 Impressive Science Essay Examples And Get Inspired
Science Fiction Essay: Examples & Easy Steps Guide
Essay About Science and Technology| Tips & Examples
Essay About Science in Everyday Life - Samples & Writing Tips
Check Out 5 Impressive Essay About Science Fair Examples
Did you ever imagine that essay writing was just for students in the Humanities? Well, think again!
For science students, tackling a science essay might seem challenging, as it not only demands a deep understanding of the subject but also strong writing skills.
However, fret not because we've got your back!
With the right steps and tips, you can write an engaging and informative science essay easily!
This blog will take you through all the important steps of writing a science essay, from choosing a topic to presenting the final work.
So, let's get into it!

- 1. What Is a Science Essay?
- 2. How To Write a Science Essay?
- 3. How to Structure a Science Essay?
- 4. Science Essay Examples
- 5. How to Choose the Right Science Essay Topic
- 6. Science Essay Topics
- 7. Science Essay Writing Tips
What Is a Science Essay?
A science essay is an academic paper focusing on a scientific topic from physics, chemistry, biology, or any other scientific field.
Science essays are mostly expository. That is, they require you to explain your chosen topic in detail. However, they can also be descriptive and exploratory.
A descriptive science essay aims to describe a certain scientific phenomenon according to established knowledge.
On the other hand, the exploratory science essay requires you to go beyond the current theories and explore new interpretations.
So before you set out to write your essay, always check out the instructions given by your instructor. Whether a science essay is expository or exploratory must be clear from the start. Or, if you face any difficulty, you can take help from a science essay writer as well.
Moreover, check out this video to understand scientific writing in detail.
Now that you know what it is, let's look at the steps you need to take to write a science essay.
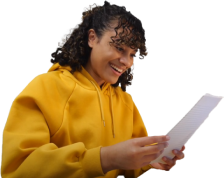
Paper Due? Why Suffer? That's our Job!
How To Write a Science Essay?
Writing a science essay is not as complex as it may seem. All you need to do is follow the right steps to create an impressive piece of work that meets the assigned criteria.
Here's what you need to do:
Choose Your Topic
A good topic forms the foundation for an engaging and well-written essay. Therefore, you should ensure that you pick something interesting or relevant to your field of study.
To choose a good topic, you can brainstorm ideas relating to the subject matter. You may also find inspiration from other science essays or articles about the same topic.
Conduct Research
Once you have chosen your topic, start researching it thoroughly to develop a strong argument or discussion in your essay.
Make sure you use reliable sources and cite them properly . You should also make notes while conducting your research so that you can reference them easily when writing the essay. Or, you can get expert assistance from an essay writing service to manage your citations.
Create an Outline
A good essay outline helps to organize the ideas in your paper. It serves as a guide throughout the writing process and ensures you don’t miss out on important points.
An outline makes it easier to write a well-structured paper that flows logically. It should be detailed enough to guide you through the entire writing process.
However, your outline should be flexible, and it's sometimes better to change it along the way to improve your structure.
Start Writing
Once you have a good outline, start writing the essay by following your plan.
The first step in writing any essay is to draft it. This means putting your thoughts down on paper in a rough form without worrying about grammar or spelling mistakes.
So begin your essay by introducing the topic, then carefully explain it using evidence and examples to support your argument.
Don't worry if your first draft isn't perfect - it's just the starting point!
Proofread & Edit
After finishing your first draft, take time to proofread and edit it for grammar and spelling mistakes.
Proofreading is the process of checking for grammatical mistakes. It should be done after you have finished writing your essay.
Editing, on the other hand, involves reviewing the structure and organization of your essay and its content. It should be done before you submit your final work.
Both proofreading and editing are essential for producing a high-quality essay. Make sure to give yourself enough time to do them properly!
After revising the essay, you should format it according to the guidelines given by your instructor. This could involve using a specific font size, page margins, or citation style.
Most science essays are written in Times New Roman font with 12-point size and double spacing. The margins should be 1 inch on all sides, and the text should be justified.
In addition, you must cite your sources properly using a recognized citation style such as APA , Chicago , or Harvard . Make sure to follow the guidelines closely so that your essay looks professional.
Following these steps will help you create an informative and well-structured science essay that meets the given criteria.
Tough Essay Due? Hire Tough Writers!
How to Structure a Science Essay?
A basic science essay structure includes an introduction, body, and conclusion.
Let's look at each of these briefly.
- Introduction
Your essay introduction should introduce your topic and provide a brief overview of what you will discuss in the essay. It should also state your thesis or main argument.
For instance, a thesis statement for a science essay could be,
"The human body is capable of incredible feats, as evidenced by the many athletes who have competed in the Olympic games."
The body of your essay will contain the bulk of your argument or discussion. It should be divided into paragraphs, each discussing a different point.
For instance, imagine you were writing about sports and the human body.
Your first paragraph can discuss the physical capabilities of the human body.
The second paragraph may be about the physical benefits of competing in sports.
Similarly, in the third paragraph, you can present one or two case studies of specific athletes to support your point.
Once you have explained all your points in the body, it’s time to conclude the essay.
Your essay conclusion should summarize the main points of your essay and leave the reader with a sense of closure.
In the conclusion, you reiterate your thesis and sum up your arguments. You can also suggest implications or potential applications of the ideas discussed in the essay.
By following this structure, you will create a well-organized essay.
Check out a few example essays to see this structure in practice.
Science Essay Examples
A great way to get inspired when writing a science essay is to look at other examples of successful essays written by others.
Here are some examples that will give you an idea of how to write your essay.
Science Essay About Genetics - Science Essay Example
Environmental Science Essay Example | PDF Sample
The Science of Nanotechnology
Science, Non-Science, and Pseudo-Science
The Science Of Science Education
Science in our Daily Lives
Short Science Essay Example
Let’s take a look at a short science essay:
Want to read more essay examples? Here, you can find more science essay examples to learn from.
How to Choose the Right Science Essay Topic
Choosing the right science essay topic is a critical first step in crafting a compelling and engaging essay. Here's a concise guide on how to make this decision wisely:
- Consider Your Interests: Start by reflecting on your personal interests within the realm of science. Selecting a topic that genuinely fascinates you will make the research and writing process more enjoyable and motivated.
- Relevance to the Course: Ensure that your chosen topic aligns with your course or assignment requirements. Read the assignment guidelines carefully to understand the scope and focus expected by your instructor.
- Current Trends and Issues: Stay updated with the latest scientific developments and trends. Opting for a topic that addresses contemporary issues not only makes your essay relevant but also demonstrates your awareness of current events in the field.
- Narrow Down the Scope: Science is vast, so narrow your topic to a manageable scope. Instead of a broad subject like "Climate Change," consider a more specific angle like "The Impact of Melting Arctic Ice on Global Sea Levels."
- Available Resources: Ensure that there are sufficient credible sources and research materials available for your chosen topic. A lack of resources can hinder your research efforts.
- Discuss with Your Instructor: If you're uncertain about your topic choice, don't hesitate to consult your instructor or professor. They can provide valuable guidance and may even suggest specific topics based on your academic goals.
Science Essay Topics
Choosing an appropriate topic for a science essay is one of the first steps in writing a successful paper.
Here are a few science essay topics to get you started:
- How space exploration affects our daily lives?
- How has technology changed our understanding of medicine?
- Are there ethical considerations to consider when conducting scientific research?
- How does climate change affect the biodiversity of different parts of the world?
- How can artificial intelligence be used in medicine?
- What impact have vaccines had on global health?
- What is the future of renewable energy?
- How do we ensure that genetically modified organisms are safe for humans and the environment?
- The influence of social media on human behavior: A social science perspective
- What are the potential risks and benefits of stem cell therapy?
Important science topics can cover anything from space exploration to chemistry and biology. So you can choose any topic according to your interests!
Need more topics? We have gathered 100+ science essay topics to help you find a great topic!
Continue reading to find some tips to help you write a successful science essay.
Science Essay Writing Tips
Once you have chosen a topic and looked at examples, it's time to start writing the science essay.
Here are some key tips for a successful essay:
- Research thoroughly
Make sure you do extensive research before you begin writing your paper. This will ensure that the facts and figures you include are accurate and supported by reliable sources.
- Use clear language
Avoid using jargon or overly technical language when writing your essay. Plain language is easier to understand and more engaging for readers.
- Referencing
Always provide references for any information you include in your essay. This will demonstrate that you acknowledge other people's work and show that the evidence you use is credible.
Make sure to follow the basic structure of an essay and organize your thoughts into clear sections. This will improve the flow and make your essay easier to read.
- Ask someone to proofread
It’s also a good idea to get someone else to proofread your work as they may spot mistakes that you have missed.
These few tips will help ensure that your science essay is well-written and informative!
You've learned the steps to writing a successful science essay and looked at some examples and topics to get you started.
Make sure you thoroughly research, use clear language, structure your thoughts, and proofread your essay. With these tips, you’re sure to write a great science essay!
Do you still need expert help writing a science essay? Our science essay writing service is here to help. With our team of professional writers, you can rest assured that your essay will be written to the highest standards.
Contact our essay service now to get started!
Also, do not forget to try our essay typer tool for quick and cost-free aid with your essays!

Write Essay Within 60 Seconds!

Betty is a freelance writer and researcher. She has a Masters in literature and enjoys providing writing services to her clients. Betty is an avid reader and loves learning new things. She has provided writing services to clients from all academic levels and related academic fields.

Paper Due? Why Suffer? That’s our Job!
Keep reading
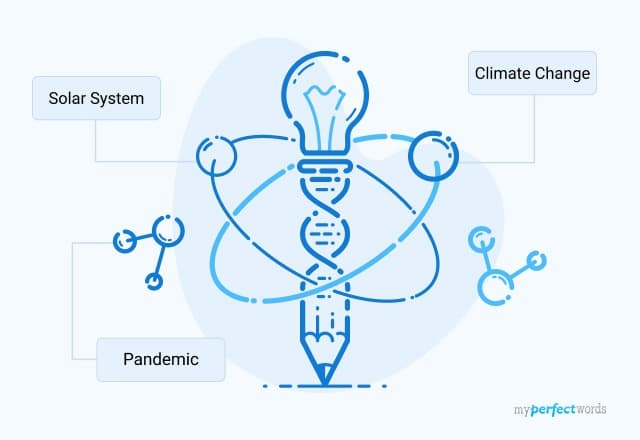

- Table of Contents
- Random Entry
- Chronological
- Editorial Information
- About the SEP
- Editorial Board
- How to Cite the SEP
- Special Characters
- Advanced Tools
- Support the SEP
- PDFs for SEP Friends
- Make a Donation
- SEPIA for Libraries
- Entry Contents
Bibliography
Academic tools.
- Friends PDF Preview
- Author and Citation Info
- Back to Top
Models in Science
Models are of central importance in many scientific contexts. The centrality of models such as inflationary models in cosmology, general-circulation models of the global climate, the double-helix model of DNA, evolutionary models in biology, agent-based models in the social sciences, and general-equilibrium models of markets in their respective domains is a case in point (the Other Internet Resources section at the end of this entry contains links to online resources that discuss these models). Scientists spend significant amounts of time building, testing, comparing, and revising models, and much journal space is dedicated to interpreting and discussing the implications of models.
As a result, models have attracted philosophers’ attention and there are now sizable bodies of literature about various aspects of scientific modeling. A tangible result of philosophical engagement with models is a proliferation of model types recognized in the philosophical literature. Probing models , phenomenological models , computational models , developmental models , explanatory models , impoverished models , testing models , idealized models , theoretical models , scale models , heuristic models , caricature models , exploratory models , didactic models , fantasy models , minimal models , toy models , imaginary models , mathematical models , mechanistic models , substitute models , iconic models , formal models , analogue models , and instrumental models are but some of the notions that are used to categorize models. While at first glance this abundance is overwhelming, it can be brought under control by recognizing that these notions pertain to different problems that arise in connection with models. Models raise questions in semantics (how, if at all, do models represent?), ontology (what kind of things are models?), epistemology (how do we learn and explain with models?), and, of course, in other domains within philosophy of science.
1. Semantics: Models and Representation
2.1 physical objects, 2.2 fictional objects and abstract objects, 2.3 set-theoretic structures, 2.4 descriptions and equations, 3.1 learning about models, 3.2 learning about target systems, 3.3 explaining with models, 3.4 understanding with models, 3.5 other cognitive functions, 4.1 models as subsidiaries to theory, 4.2 models as independent from theories, 5.1 models, realism, and laws of nature, 5.2 models and reductionism, other internet resources, related entries.
Many scientific models are representational models: they represent a selected part or aspect of the world, which is the model’s target system. Standard examples are the billiard ball model of a gas, the Bohr model of the atom, the Lotka–Volterra model of predator–prey interaction, the Mundell–Fleming model of an open economy, and the scale model of a bridge.
This raises the question what it means for a model to represent a target system. This problem is rather involved and decomposes into various subproblems. For an in-depth discussion of the issue of representation, see the entry on scientific representation . At this point, rather than addressing the issue of what it means for a model to represent, we focus on a number of different kinds of representation that play important roles in the practice of model-based science, namely scale models, analogical models, idealized models, toy models, minimal models, phenomenological models, exploratory models, and models of data. These categories are not mutually exclusive, and a given model can fall into several categories at once.
Scale models . Some models are down-sized or enlarged copies of their target systems (Black 1962). A typical example is a small wooden car that is put into a wind tunnel to explore the actual car’s aerodynamic properties. The intuition is that a scale model is a naturalistic replica or a truthful mirror image of the target; for this reason, scale models are sometimes also referred to as “true models” (Achinstein 1968: Ch. 7). However, there is no such thing as a perfectly faithful scale model; faithfulness is always restricted to some respects. The wooden scale model of the car provides a faithful portrayal of the car’s shape but not of its material. And even in the respects in which a model is a faithful representation, the relation between model-properties and target-properties is usually not straightforward. When engineers use, say, a 1:100 scale model of a ship to investigate the resistance that an actual ship experiences when moving through the water, they cannot simply measure the resistance the model experiences and then multiply it with the scale. In fact, the resistance faced by the model does not translate into the resistance faced by the actual ship in a straightforward manner (that is, one cannot simply scale the water resistance with the scale of the model: the real ship need not have one hundred times the water resistance of its 1:100 model). The two quantities stand in a complicated nonlinear relation with each other, and the exact form of that relation is often highly nontrivial and emerges as the result of a thoroughgoing study of the situation (Sterrett 2006, forthcoming; Pincock forthcoming).
Analogical models . Standard examples of analogical models include the billiard ball model of a gas, the hydraulic model of an economic system, and the dumb hole model of a black hole. At the most basic level, two things are analogous if there are certain relevant similarities between them. In a classic text, Hesse (1963) distinguishes different types of analogies according to the kinds of similarity relations into which two objects enter. A simple type of analogy is one that is based on shared properties. There is an analogy between the earth and the moon based on the fact that both are large, solid, opaque, spherical bodies that receive heat and light from the sun, revolve around their axes, and gravitate towards other bodies. But sameness of properties is not a necessary condition. An analogy between two objects can also be based on relevant similarities between their properties. In this more liberal sense, we can say that there is an analogy between sound and light because echoes are similar to reflections, loudness to brightness, pitch to color, detectability by the ear to detectability by the eye, and so on.
Analogies can also be based on the sameness or resemblance of relations between parts of two systems rather than on their monadic properties. It is in this sense that the relation of a father to his children is asserted to be analogous to the relation of the state to its citizens. The analogies mentioned so far have been what Hesse calls “material analogies”. We obtain a more formal notion of analogy when we abstract from the concrete features of the systems and only focus on their formal set-up. What the analogue model then shares with its target is not a set of features, but the same pattern of abstract relationships (i.e., the same structure, where structure is understood in a formal sense). This notion of analogy is closely related to what Hesse calls “formal analogy”. Two items are related by formal analogy if they are both interpretations of the same formal calculus. For instance, there is a formal analogy between a swinging pendulum and an oscillating electric circuit because they are both described by the same mathematical equation.
A further important distinction due to Hesse is the one between positive, negative, and neutral analogies. The positive analogy between two items consists in the properties or relations they share (both gas molecules and billiard balls have mass); the negative analogy consists in the properties they do not share (billiard balls are colored, gas molecules are not); the neutral analogy comprises the properties of which it is not known (yet) whether they belong to the positive or the negative analogy (do billiard balls and molecules have the same cross section in scattering processes?). Neutral analogies play an important role in scientific research because they give rise to questions and suggest new hypotheses. For this reason several authors have emphasized the heuristic role that analogies play in theory and model construction, as well as in creative thought (Bailer-Jones and Bailer-Jones 2002; Bailer-Jones 2009: Ch. 3; Hesse 1974; Holyoak and Thagard 1995; Kroes 1989; Psillos 1995; and the essays collected in Helman 1988). See also the entry on analogy and analogical reasoning .
It has also been discussed whether using analogical models can in some cases be confirmatory in a Bayesian sense. Hesse (1974: 208–219) argues that this is possible if the analogy is a material analogy. Bartha (2010, 2013 [2019]) disagrees and argues that analogical models cannot be confirmatory in a Bayesian sense because the information encapsulated in an analogical model is part of the relevant background knowledge, which has the consequence that the posterior probability of a hypothesis about a target system cannot change as a result of observing the analogy. Analogical models can therefore only establish the plausibility of a conclusion in the sense of justifying a non-negligible prior probability assignment (Bartha 2010: §8.5).
More recently, these questions have been discussed in the context of so-called analogue experiments, which promise to provide knowledge about an experimentally inaccessible target system (e.g., a black hole) by manipulating another system, the source system (e.g., a Bose–Einstein condensate). Dardashti, Thébault, and Winsberg (2017) and Dardashti, Hartmann et al. (2019) have argued that, given certain conditions, an analogue simulation of one system by another system can confirm claims about the target system (e.g., that black holes emit Hawking radiation). See Crowther et al. (forthcoming) for a critical discussion, and also the entry on computer simulations in science .
Idealized models . Idealized models are models that involve a deliberate simplification or distortion of something complicated with the objective of making it more tractable or understandable. Frictionless planes, point masses, completely isolated systems, omniscient and fully rational agents, and markets in perfect equilibrium are well-known examples. Idealizations are a crucial means for science to cope with systems that are too difficult to study in their full complexity (Potochnik 2017).
Philosophical debates over idealization have focused on two general kinds of idealizations: so-called Aristotelian and Galilean idealizations. Aristotelian idealization amounts to “stripping away”, in our imagination, all properties from a concrete object that we believe are not relevant to the problem at hand. There is disagreement on how this is done. Jones (2005) and Godfrey-Smith (2009) offer an analysis of abstraction in terms of truth: while an abstraction remains silent about certain features or aspects of the system, it does not say anything false and still offers a true (albeit restricted) description. This allows scientists to focus on a limited set of properties in isolation. An example is a classical-mechanics model of the planetary system, which describes the position of an object as a function of time and disregards all other properties of planets. Cartwright (1989: Ch. 5), Musgrave (1981), who uses the term “negligibility assumptions”, and Mäki (1994), who speaks of the “method of isolation”, allow abstractions to say something false, for instance by neglecting a causally relevant factor.
Galilean idealizations are ones that involve deliberate distortions: physicists build models consisting of point masses moving on frictionless planes; economists assume that agents are omniscient; biologists study isolated populations; and so on. Using simplifications of this sort whenever a situation is too difficult to tackle was characteristic of Galileo’s approach to science. For this reason it is common to refer to ‘distortive’ idealizations of this kind as “Galilean idealizations” (McMullin 1985). An example for such an idealization is a model of motion on an ice rink that assumes the ice to be frictionless, when, in reality, it has low but non-zero friction.
Galilean idealizations are sometimes characterized as controlled idealizations, i.e., as ones that allow for de-idealization by successive removal of the distorting assumptions (McMullin 1985; Weisberg 2007). Thus construed, Galilean idealizations don’t cover all distortive idealizations. Batterman (2002, 2011) and Rice (2015, 2019) discuss distortive idealizations that are ineliminable in that they cannot be removed from the model without dismantling the model altogether.
What does a model involving distortions tell us about reality? Laymon (1991) formulated a theory which understands idealizations as ideal limits: imagine a series of refinements of the actual situation which approach the postulated limit, and then require that the closer the properties of a system come to the ideal limit, the closer its behavior has to come to the behavior of the system at the limit (monotonicity). If this is the case, then scientists can study the system at the limit and carry over conclusions from that system to systems distant from the limit. But these conditions need not always hold. In fact, it can happen that the limiting system does not approach the system at the limit. If this happens, we are faced with a singular limit (Berry 2002). In such cases the system at the limit can exhibit behavior that is different from the behavior of systems distant from the limit. Limits of this kind appear in a number of contexts, most notably in the theory of phase transitions in statistical mechanics. There is, however, no agreement over the correct interpretation of such limits. Batterman (2002, 2011) sees them as indicative of emergent phenomena, while Butterfield (2011a,b) sees them as compatible with reduction (see also the entries on intertheory relations in physics and scientific reduction ).
Galilean and Aristotelian idealizations are not mutually exclusive, and many models exhibit both in that they take into account a narrow set of properties and distort them. Consider again the classical-mechanics model of the planetary system: the model only takes a narrow set of properties into account and distorts them, for instance by describing planets as ideal spheres with a rotation-symmetric mass distribution.
A concept that is closely related to idealization is approximation. In a broad sense, A can be called an approximation of B if A is somehow close to B . This, however, is too broad because it makes room for any likeness to qualify as an approximation. Rueger and Sharp (1998) limit approximations to quantitative closeness, and Portides (2007) frames it as an essentially mathematical concept. On that notion A is an approximation of B iff A is close to B in a specifiable mathematical sense, where the relevant sense of “close” will be given by the context. An example is the approximation of one curve with another one, which can be achieved by expanding a function into a power series and only keeping the first two or three terms. In different situations we approximate an equation with another one by letting a control parameter tend towards zero (Redhead 1980). This raises the question of how approximations are different from idealizations, which can also involve mathematical closeness. Norton (2012) sees the distinction between the two as referential: an approximation is an inexact description of the target while an idealization introduces a secondary system (real or fictitious) which stands for the target system (while being distinct from it). If we say that the period of the pendulum on the wall is roughly two seconds, then this is an approximation; if we reason about the real pendulum by assuming that the pendulum bob is a point mass and that the string is massless (i.e., if we assume that the pendulum is a so-called ideal pendulum), then we use an idealization. Separating idealizations and approximations in this way does not imply that there cannot be interesting relations between the two. For instance, an approximation can be justified by pointing out that it is the mathematical expression of an acceptable idealization (e.g., when we neglect a dissipative term in an equation of motion because we make the idealizing assumption that the system is frictionless).
Toy models . Toy models are extremely simplified and strongly distorted renderings of their targets, and often only represent a small number of causal or explanatory factors (Hartmann 1995; Reutlinger et al. 2018; Nguyen forthcoming). Typical examples are the Lotka–Volterra model in population ecology (Weisberg 2013) and the Schelling model of segregation in the social sciences (Sugden 2000). Toy models usually do not perform well in terms of prediction and empirical adequacy, and they seem to serve other epistemic goals (more on these in Section 3 ). This raises the question whether they should be regarded as representational at all (Luczak 2017).
Some toy models are characterized as “caricatures” (Gibbard and Varian 1978; Batterman and Rice 2014). Caricature models isolate a small number of salient characteristics of a system and distort them into an extreme case. A classic example is Akerlof’s (1970) model of the car market (“the market for lemons”), which explains the difference in price between new and used cars solely in terms of asymmetric information, thereby disregarding all other factors that may influence the prices of cars (see also Sugden 2000). However, it is controversial whether such highly idealized models can still be regarded as informative representations of their target systems. For a discussion of caricature models, in particular in economics, see Reiss (2006).
Minimal models . Minimal models are closely related to toy models in that they are also highly simplified. They are so simplified that some argue that they are non-representational: they lack any similarity, isomorphism, or resemblance relation to the world (Batterman and Rice 2014). It has been argued that many economic models are of this kind (Grüne-Yanoff 2009). Minimal economic models are also unconstrained by natural laws, and do not isolate any real factors ( ibid .). And yet, minimal models help us to learn something about the world in the sense that they function as surrogates for a real system: scientists can study the model to learn something about the target. It is, however, controversial whether minimal models can assist scientists in learning something about the world if they do not represent anything (Fumagalli 2016). Minimal models that purportedly lack any similarity or representation are also used in different parts of physics to explain the macro-scale behavior of various systems whose micro-scale behavior is extremely diverse (Batterman and Rice 2014; Rice 2018, 2019; Shech 2018). Typical examples are the features of phase transitions and the flow of fluids. Proponents of minimal models argue that what provides an explanation of the macro-scale behavior of a system in these cases is not a feature that system and model have in common, but the fact that the system and the model belong to the same universality class (a class of models that exhibit the same limiting behavior even though they show very different behavior at finite scales). It is, however, controversial whether explanations of this kind are possible without reference to at least some common features (Lange 2015; Reutlinger 2017).
Phenomenological models . Phenomenological models have been defined in different, although related, ways. A common definition takes them to be models that only represent observable properties of their targets and refrain from postulating hidden mechanisms and the like (Bokulich 2011). Another approach, due to McMullin (1968), defines phenomenological models as models that are independent of theories. This, however, seems to be too strong. Many phenomenological models, while failing to be derivable from a theory, incorporate principles and laws associated with theories. The liquid-drop model of the atomic nucleus, for instance, portrays the nucleus as a liquid drop and describes it as having several properties (surface tension and charge, among others) originating in different theories (hydrodynamics and electrodynamics, respectively). Certain aspects of these theories—although usually not the full theories—are then used to determine both the static and dynamical properties of the nucleus. Finally, it is tempting to identify phenomenological models with models of a phenomenon . Here, “phenomenon” is an umbrella term covering all relatively stable and general features of the world that are interesting from a scientific point of view. The weakening of sound as a function of the distance to the source, the decay of alpha particles, the chemical reactions that take place when a piece of limestone dissolves in an acid, the growth of a population of rabbits, and the dependence of house prices on the base rate of the Federal Reserve are phenomena in this sense. For further discussion, see Bailer-Jones (2009: Ch. 7), Bogen and Woodward (1988), and the entry on theory and observation in science .
Exploratory models . Exploratory models are models which are not proposed in the first place to learn something about a specific target system or a particular experimentally established phenomenon. Exploratory models function as the starting point of further explorations in which the model is modified and refined. Gelfert (2016) points out that exploratory models can provide proofs-of-principle and suggest how-possibly explanations (2016: Ch. 4). As an example, Gelfert mentions early models in theoretical ecology, such as the Lotka–Volterra model of predator–prey interaction, which mimic the qualitative behavior of speed-up and slow-down in population growth in an environment with limited resources (2016: 80). Such models do not give an accurate account of the behavior of any actual population, but they provide the starting point for the development of more realistic models. Massimi (2019) notes that exploratory models provide modal knowledge. Fisher (2006) sees these models as tools for the examination of the features of a given theory.
Models of data. A model of data (sometimes also “data model”) is a corrected, rectified, regimented, and in many instances idealized version of the data we gain from immediate observation, the so-called raw data (Suppes 1962). Characteristically, one first eliminates errors (e.g., removes points from the record that are due to faulty observation) and then presents the data in a “neat” way, for instance by drawing a smooth curve through a set of points. These two steps are commonly referred to as “data reduction” and “curve fitting”. When we investigate, for instance, the trajectory of a certain planet, we first eliminate points that are fallacious from the observation records and then fit a smooth curve to the remaining ones. Models of data play a crucial role in confirming theories because it is the model of data, and not the often messy and complex raw data, that theories are tested against.
The construction of a model of data can be extremely complicated. It requires sophisticated statistical techniques and raises serious methodological as well as philosophical questions. How do we decide which points on the record need to be removed? And given a clean set of data, what curve do we fit to it? The first question has been dealt with mainly within the context of the philosophy of experiment (see, for instance, Galison 1997 and Staley 2004). At the heart of the latter question lies the so-called curve-fitting problem, which is that the data themselves dictate neither the form of the fitted curve nor what statistical techniques scientists should use to construct a curve. The choice and rationalization of statistical techniques is the subject matter of the philosophy of statistics, and we refer the reader to the entry Philosophy of Statistics and to Bandyopadhyay and Forster (2011) for a discussion of these issues. Further discussions of models of data can be found in Bailer-Jones (2009: Ch. 7), Brewer and Chinn (1994), Harris (2003), Hartmann (1995), Laymon (1982), Mayo (1996, 2018), and Suppes (2007).
The gathering, processing, dissemination, analysis, interpretation, and storage of data raise many important questions beyond the relatively narrow issues pertaining to models of data. Leonelli (2016, 2019) investigates the status of data in science, argues that data should be defined not by their provenance but by their evidential function, and studies how data travel between different contexts.
2. Ontology: What Are Models?
What are models? That is, what kind of object are scientists dealing with when they work with a model? A number of authors have voiced skepticism that this question has a meaningful answer, because models do not belong to a distinctive ontological category and anything can be a model (Callender and Cohen 2006; Giere 2010; Suárez 2004; Swoyer 1991; Teller 2001). Contessa (2010) replies that this is a non sequitur . Even if, from an ontological point of view, anything can be a model and the class of things that are referred to as models contains a heterogeneous collection of different things, it does not follow that it is either impossible or pointless to develop an ontology of models. This is because even if not all models are of a particular ontological kind, one can nevertheless ask to what ontological kinds the things that are de facto used as models belong. There may be several such kinds and each kind can be analyzed in its own right. What sort of objects scientists use as models has important repercussions for how models perform relevant functions such as representation and explanation, and hence this issue cannot be dismissed as “just sociology”.
The objects that commonly serve as models indeed belong to different ontological kinds: physical objects, fictional objects, abstract objects, set-theoretic structures, descriptions, equations, or combinations of some of these, are frequently referred to as models, and some models may fall into yet other classes of things. Following Contessa’s advice, the aim then is to develop an ontology for each of these. Those with an interest in ontology may see this as a goal in its own right. It pays noting, however, that the question has reverberations beyond ontology and bears on how one understands the semantics and the epistemology of models.
Some models are physical objects. Such models are commonly referred to as “material models”. Standard examples of models of this kind are scale models of objects like bridges and ships (see Section 1 ), Watson and Crick’s metal model of DNA (Schaffner 1969), Phillips and Newlyn’s hydraulic model of an economy (Morgan and Boumans 2004), the US Army Corps of Engineers’ model of the San Francisco Bay (Weisberg 2013), Kendrew’s plasticine model of myoglobin (Frigg and Nguyen 2016), and model organisms in the life sciences (Leonelli and Ankeny 2012; Leonelli 2010; Levy and Currie 2015). All these are material objects that serve as models. Material models do not give rise to ontological difficulties over and above the well-known problems in connection with objects that metaphysicians deal with, for instance concerning the nature of properties, the identity of objects, parts and wholes, and so on.
However, many models are not material models. The Bohr model of the atom, a frictionless pendulum, or an isolated population, for instance, are in the scientist’s mind rather than in the laboratory and they do not have to be physically realized and experimented upon to serve as models. These “non-physical” models raise serious ontological questions, and how they are best analyzed is debated controversially. In the remainder of this section we review some of the suggestions that have attracted attention in the recent literature on models.
What has become known as the fiction view of models sees models as akin to the imagined objects of literary fiction—that is, as akin to fictional characters like Sherlock Holmes or fictional places like Middle Earth (Godfrey-Smith 2007). So when Bohr introduced his model of the atom he introduced a fictional object of the same kind as the object Conan Doyle introduced when he invented Sherlock Holmes. This view squares well with scientific practice, where scientists often talk about models as if they were objects and often take themselves to be describing imaginary atoms, populations, or economies. It also squares well with philosophical views that see the construction and manipulation of models as essential aspects of scientific investigation (Morgan 1999), even if models are not material objects, because these practices seem to be directed toward some kind of object.
What philosophical questions does this move solve? Fictional discourse and fictional entities face well-known philosophical questions, and one may well argue that simply likening models to fictions amounts to explaining obscurum per obscurius (for a discussion of these questions, see the entry on fictional entities ). One way to counter this objection and to motivate the fiction view of models is to point to the view’s heuristic power. In this vein Frigg (2010b) identifies five specific issues that an ontology of models has to address and then notes that these issues arise in very similar ways in the discussion about fiction (the issues are the identity conditions, property attribution, the semantics of comparative statements, truth conditions, and the epistemology of imagined objects). Likening models to fiction then has heuristic value because there is a rich literature on fiction that offers a number of solutions to these issues.
Only a small portion of the options available in the extensive literature on fictions have actually been explored in the context of scientific models. Contessa (2010) formulates what he calls the “dualist account”, according to which a model is an abstract object that stands for a possible concrete object. The Rutherford model of the atom, for instance, is an abstract object that acts as a stand-in for one of the possible systems that contain an electron orbiting around a nucleus in a well-defined orbit. Barberousse and Ludwig (2009) and Frigg (2010b) take a different route and develop an account of models as fictions based on Walton’s (1990) pretense theory of fiction. According to this view the sentences of a passage of text introducing a model should be seen as a prop in a game of make-believe, and the model is the product of an act of pretense. This is an antirealist position in that it takes talk of model “objects” to be figures of speech because ultimately there are no model objects—models only live in scientists’ imaginations. Salis (forthcoming) reformulates this view to become what she calls the “the new fiction view of models”. The core difference lies in the fact that what is considered as the model are the model descriptions and their content rather than the imaginings that they prescribe. This is a realist view of models, because descriptions exist.
The fiction view is not without critics. Giere (2009), Magnani (2012), Pincock (2012), Portides (2014), and Teller (2009) reject the fiction approach and argue, in different ways, that models should not be regarded as fictions. Weisberg (2013) argues for a middle position which sees fictions as playing a heuristic role but denies that they should be regarded as forming part of a scientific model. The common core of these criticisms is that the fiction view misconstrues the epistemic standing of models. To call something a fiction, so the charge goes, is tantamount to saying that it is false, and it is unjustified to call an entire model a fiction—and thereby claim that it fails to capture how the world is—just because the model involves certain false assumptions or fictional elements. In other words, a representation isn’t automatically counted as fiction just because it has some inaccuracies. Proponents of the fiction view agree with this point but deny that the notion of fiction should be analyzed in terms of falsity. What makes a work a fiction is not its falsity (or some ratio of false to true claims): neither is everything that is said in a novel untrue (Tolstoy’s War and Peace contains many true statements about Napoleon’s Franco-Russian War), nor does every text containing false claims qualify as fiction (false news reports are just that, they are not fictions). The defining feature of a fiction is that readers are supposed to imagine the events and characters described, not that they are false (Frigg 2010a; Salis forthcoming).
Giere (1988) advocated the view that “non-physical” models are abstract entities. However, there is little agreement on the nature of abstract objects, and Hale (1988: 86–87) lists no less than twelve different possible characterizations (for a review of the available options, see the entry on abstract objects ). In recent publications, Thomasson (2020) and Thomson-Jones (2020) develop what they call an “artifactualist view” of models, which is based on Thomasson’s (1999) theory of abstract artifacts. This view agrees with the pretense theory that the content of text that introduces a fictional character or a model should be understood as occurring in pretense, but at the same time insists that in producing such descriptions authors create abstract cultural artifacts that then exist independently of either the author or the readers. Artifactualism agrees with Platonism that abstract objects exist, but insists, contra Platonism, that abstract objects are brought into existence through a creative act and are not eternal. This allows the artifactualist to preserve the advantages of pretense theory while at the same time holding the realist view that fictional characters and models actually exist.
An influential point of view takes models to be set-theoretic structures. This position can be traced back to Suppes (1960) and is now, with slight variants, held by most proponents of the so-called semantic view of theories (for a discussion of this view, see the entry on the structure of scientific theories ). There are differences between the versions of the semantic view, but with the exception of Giere (1988) all versions agree that models are structures of one sort or another (Da Costa and French 2000).
This view of models has been criticized on various grounds. One pervasive criticism is that many types of models that play an important role in science are not structures and cannot be accommodated within the structuralist view of models, which can neither account for how these models are constructed nor for how they work in the context of investigation (Cartwright 1999; Downes 1992; Morrison 1999). Examples for such models are interpretative models and mediating models, discussed later in Section 4.2 . Another charge held against the set-theoretic approach is that set-theoretic structures by themselves cannot be representational models—at least if that requires them to share some structure with the target—because the ascription of a structure to a target system which forms part of the physical world relies on a substantive (non-structural) description of the target, which goes beyond what the structuralist approach can afford (Nguyen and Frigg forthcoming).
A time-honored position has it that a model is a stylized description of a target system. It has been argued that this is what scientists display in papers and textbooks when they present a model (Achinstein 1968; Black 1962). This view has not been subject to explicit criticism. However, some of the criticisms that have been marshaled against the so-called syntactic view of theories equally threaten a linguistic understanding of models (for a discussion of this view, see the entry on the structure of scientific theories ). First, a standard criticism of the syntactic view is that by associating a theory with a particular formulation, the view misconstrues theory identity because any change in the formulation results in a new theory (Suppe 2000). A view that associates models with descriptions would seem to be open to the same criticism. Second, models have different properties than descriptions: the Newtonian model of the solar system consists of orbiting spheres, but it makes no sense to say this about its description. Conversely, descriptions have properties that models do not have: a description can be written in English and consist of 517 words, but the same cannot be said of a model. One way around these difficulties is to associate the model with the content of a description rather than with the description itself. For a discussion of a position on models that builds on the content of a description, see Salis (forthcoming).
A contemporary version of descriptivism is Levy’s (2012, 2015) and Toon’s (2012) so-called direct-representation view. This view shares with the fiction view of models ( Section 2.2 ) the reliance on Walton’s pretense theory, but uses it in a different way. The main difference is that the views discussed earlier see modeling as introducing a vehicle of representation, the model, that is distinct from the target, and they see the problem as elucidating what kind of thing the model is. On the direct-representation view there are no models distinct from the target; there are only model-descriptions and targets, with no models in-between them. Modeling, on this view, consists in providing an imaginative description of real things. A model-description prescribes imaginings about the real system; the ideal pendulum, for instance, prescribes model-users to imagine the real spring as perfectly elastic and the bob as a point mass. This approach avoids the above problems because the identity conditions for models are given by the conditions for games of make-believe (and not by the syntax of a description) and property ascriptions take place in pretense. There are, however, questions about how this account deals with models that have no target (like models of the ether or four-sex populations), and about how models thus understood deal with idealizations. For a discussion of these points, see Frigg and Nguyen (2016), Poznic (2016), and Salis (forthcoming).
A closely related approach sees models as equations. This is a version of the view that models are descriptions, because equations are syntactic items that describe a mathematical structure. The issues that this view faces are similar to the ones we have already encountered: First, one can describe the same situation using different kinds of coordinates and as a result obtain different equations but without thereby also obtaining a different model. Second, the model and the equation have different properties. A pendulum contains a massless string, but the equation describing its motion does not; and an equation may be inhomogeneous, but the system it describes is not. It is an open question whether these issues can be avoided by appeal to a pretense account.
3. Epistemology: The Cognitive Functions of Models
One of the main reasons why models play such an important role in science is that they perform a number of cognitive functions. For example, models are vehicles for learning about the world. Significant parts of scientific investigation are carried out on models rather than on reality itself because by studying a model we can discover features of, and ascertain facts about, the system the model stands for: models allow for “surrogative reasoning” (Swoyer 1991). For instance, we study the nature of the hydrogen atom, the dynamics of a population, or the behavior of a polymer by studying their respective models. This cognitive function of models has been widely acknowledged in the literature, and some even suggest that models give rise to a new style of reasoning, “model-based reasoning”, according to which “inferences are made by means of creating models and manipulating, adapting, and evaluating them” (Nersessian 2010: 12; see also Magnani, Nersessian, and Thagard 1999; Magnani and Nersessian 2002; and Magnani and Casadio 2016).
Learning about a model happens in two places: in the construction of the model and in its manipulation (Morgan 1999). There are no fixed rules or recipes for model building and so the very activity of figuring out what fits together, and how, affords an opportunity to learn about the model. Once the model is built, we do not learn about its properties by looking at it; we have to use and manipulate the model in order to elicit its secrets.
Depending on what kind of model we are dealing with, building and manipulating a model amount to different activities demanding different methodologies. Material models seem to be straightforward because they are used in common experimental contexts (e.g., we put the model of a car in the wind tunnel and measure its air resistance). Hence, as far as learning about the model is concerned, material models do not give rise to questions that go beyond questions concerning experimentation more generally.
Not so with fictional and abstract models. What constraints are there to the construction of fictional and abstract models, and how do we manipulate them? A natural response seems to be that we do this by performing a thought experiment. Different authors (e.g., Brown 1991; Gendler 2000; Norton 1991; Reiss 2003; Sorensen 1992) have explored this line of argument, but they have reached very different and often conflicting conclusions about how thought experiments are performed and what the status of their outcomes is (for details, see the entry on thought experiments ).
An important class of models is computational in nature. For some models it is possible to derive results or solve equations of a mathematical model analytically. But quite often this is not the case. It is at this point that computers have a great impact, because they allow us to solve problems that are otherwise intractable. Hence, computational methods provide us with knowledge about (the consequences of) a model where analytical methods remain silent. Many parts of current research in both the natural and social sciences rely on computer simulations, which help scientists to explore the consequences of models that cannot be investigated otherwise. The formation and development of stars and galaxies, the dynamics of high-energy heavy-ion reactions, the evolution of life, outbreaks of wars, the progression of an economy, moral behavior, and the consequences of decision procedures in an organization are explored with computer simulations, to mention only a few examples.
Computer simulations are also heuristically important. They can suggest new theories, models, and hypotheses, for example, based on a systematic exploration of a model’s parameter space (Hartmann 1996). But computer simulations also bear methodological perils. For example, they may provide misleading results because, due to the discrete nature of the calculations carried out on a digital computer, they only allow for the exploration of a part of the full parameter space, and this subspace need not reflect every important feature of the model. The severity of this problem is somewhat mitigated by the increasing power of modern computers. But the availability of more computational power can also have adverse effects: it may encourage scientists to swiftly come up with increasingly complex but conceptually premature models, involving poorly understood assumptions or mechanisms and too many additional adjustable parameters (for a discussion of a related problem in the social sciences, see Braun and Saam 2015: Ch. 3). This can lead to an increase in empirical adequacy—which may be welcome for certain forecasting tasks—but not necessarily to a better understanding of the underlying mechanisms. As a result, the use of computer simulations can change the weight we assign to the various goals of science. Finally, the availability of computer power may seduce scientists into making calculations that do not have the degree of trustworthiness one would expect them to have. This happens, for instance, when computers are used to propagate probability distributions forward in time, which can turn out to be misleading (see Frigg et al. 2014). So it is important not to be carried away by the means that new powerful computers offer and lose sight of the actual goals of research. For a discussion of further issues in connection with computer simulations, we refer the reader to the entry on computer simulations in science .
Once we have knowledge about the model, this knowledge has to be “translated” into knowledge about the target system. It is at this point that the representational function of models becomes important again: if a model represents, then it can instruct us about reality because (at least some of) the model’s parts or aspects have corresponding parts or aspects in the world. But if learning is connected to representation and if there are different kinds of representations (analogies, idealizations, etc.), then there are also different kinds of learning. If, for instance, we have a model we take to be a realistic depiction, the transfer of knowledge from the model to the target is accomplished in a different manner than when we deal with an analogue, or a model that involves idealizing assumptions. For a discussion of the different ways in which the representational function of models can be exploited to learn about the target, we refer the reader to the entry Scientific Representation .
Some models explain. But how can they fulfill this function given that they typically involve idealizations? Do these models explain despite or because of the idealizations they involve? Does an explanatory use of models presuppose that they represent, or can non-representational models also explain? And what kind of explanation do models provide?
There is a long tradition requesting that the explanans of a scientific explanation must be true. We find this requirement in the deductive-nomological model (Hempel 1965) as well as in the more recent literature. For instance, Strevens (2008: 297) claims that “no causal account of explanation … allows nonveridical models to explain”. For further discussions, see also Colombo et al. (2015).
Authors working in this tradition deny that idealizations make a positive contribution to explanation and explore how models can explain despite being idealized. McMullin (1968, 1985) argues that a causal explanation based on an idealized model leaves out only features which are irrelevant for the respective explanatory task (see also Salmon 1984 and Piccinini and Craver 2011 for a discussion of mechanism sketches). Friedman (1974) argues that a more realistic (and hence less idealized) model explains better on the unification account. The idea is that idealizations can (at least in principle) be de-idealized (for a critical discussion of this claim in the context of the debate about scientific explanations, see Batterman 2002; Bokulich 2011; Morrison 2005, 2009; Jebeile and Kennedy 2015; and Rice 2015). Strevens (2008) argues that an explanatory causal model has to provide an accurate representation of the relevant causal relationships or processes which the model shares with the target system. The idealized assumptions of a model do not make a difference for the phenomenon under consideration and are therefore explanatorily irrelevant. In contrast, both Potochnik (2017) and Rice (2015) argue that models that explain can directly distort many difference-making causes.
According to Woodward’s (2003) theory, models are tools to find out about the causal relations that hold between certain facts or processes, and it is these relations that do the explanatory work. More specifically, explanations provide information about patterns of counterfactual dependence between the explanans and the explanandum which
enable us to see what sort of difference it would have made for the explanandum if the factors cited in the explanans had been different in various possible ways. (Woodward 2003: 11)
Accounts of causal explanation have also led to various claims about how idealized models can provide explanations, exploring to what extent idealization allows for the misrepresentation of irrelevant causal factors by the explanatory model (Elgin and Sober 2002; Strevens 2004, 2008; Potochnik 2007; Weisberg 2007, 2013). However, having the causally relevant features in common with real systems continues to play the essential role in showing how idealized models can be explanatory.
But is it really the truth of the explanans that makes the model explanatory? Other authors pursue a more radical line and argue that false models explain not only despite their falsity, but in fact because of their falsity. Cartwright (1983: 44) maintains that “the truth doesn’t explain much”. In her so-called “simulacrum account of explanation”, she suggests that we explain a phenomenon by constructing a model that fits the phenomenon into the basic framework of a grand theory (1983: Ch. 8). On this account, the model itself is the explanation we seek. This squares well with basic scientific intuitions, but it leaves us with the question of what notion of explanation is at work (see also Elgin and Sober 2002) and of what explanatory function idealizations play in model explanations (Rice 2018, 2019). Wimsatt (2007: Ch. 6) stresses the role of false models as means to arrive at true theories. Batterman and Rice (2014) argue that models explain because the details that characterize specific systems do not matter for the explanation. Bokulich (2008, 2009, 2011, 2012) pursues a similar line of reasoning and sees the explanatory power of models as being closely related to their fictional nature. Bokulich (2009) and Kennedy (2012) present non-representational accounts of model explanation (see also Jebeile and Kennedy 2015). Reiss (2012) and Woody (2004) provide general discussions of the relationship between representation and explanation.
Many authors have pointed out that understanding is one of the central goals of science (see, for instance, de Regt 2017; Elgin 2017; Khalifa 2017; Potochnik 2017). In some cases, we want to understand a certain phenomenon (e.g., why the sky is blue); in other cases, we want to understand a specific scientific theory (e.g., quantum mechanics) that accounts for a phenomenon in question. Sometimes we gain understanding of a phenomenon by understanding the corresponding theory or model. For instance, Maxwell’s theory of electromagnetism helps us understand why the sky is blue. It is, however, controversial whether understanding a phenomenon always presupposes an understanding of the corresponding theory (de Regt 2009: 26).
Although there are many different ways of gaining understanding, models and the activity of scientific modeling are of particular importance here (de Regt et al. 2009; Morrison 2009; Potochnik 2017; Rice 2016). This insight can be traced back at least to Lord Kelvin who, in his famous 1884 Baltimore Lectures on Molecular Dynamics and the Wave Theory of Light , maintained that “the test of ‘Do we or do we not understand a particular subject in physics?’ is ‘Can we make a mechanical model of it?’” (Kelvin 1884 [1987: 111]; see also Bailer-Jones 2009: Ch. 2; and de Regt 2017: Ch. 6).
But why do models play such a crucial role in the understanding of a subject matter? Elgin (2017) argues that this is not despite, but because, of models being literally false. She views false models as “felicitous falsehoods” that occupy center stage in the epistemology of science, and mentions the ideal-gas model in statistical mechanics and the Hardy–Weinberg model in genetics as examples for literally false models that are central to their respective disciplines. Understanding is holistic and it concerns a topic, a discipline, or a subject matter, rather than isolated claims or facts. Gaining understanding of a context means to have
an epistemic commitment to a comprehensive, systematically linked body of information that is grounded in fact, is duly responsive to reasons or evidence, and enables nontrivial inference, argument, and perhaps action regarding the topic the information pertains to (Elgin 2017: 44)
and models can play a crucial role in the pursuit of these epistemic commitments. For a discussion of Elgin’s account of models and understanding, see Baumberger and Brun (2017) and Frigg and Nguyen (forthcoming).
Elgin (2017), Lipton (2009), and Rice (2016) all argue that models can be used to understand independently of their ability to provide an explanation. Other authors, among them Strevens (2008, 2013), argue that understanding presupposes a scientific explanation and that
an individual has scientific understanding of a phenomenon just in case they grasp a correct scientific explanation of that phenomenon. (Strevens 2013: 510; see, however, Sullivan and Khalifa 2019)
On this account, understanding consists in a particular form of epistemic access an individual scientist has to an explanation. For Strevens this aspect is “grasping”, while for de Regt (2017) it is “intelligibility”. It is important to note that both Strevens and de Regt hold that such “subjective” aspects are a worthy topic for investigations in the philosophy of science. This contrasts with the traditional view (see, e.g., Hempel 1965) that delegates them to the realm of psychology. See Friedman (1974), Trout (2002), and Reutlinger et al. (2018) for further discussions of understanding.
Besides the functions already mentioned, it has been emphasized variously that models perform a number of other cognitive functions. Knuuttila (2005, 2011) argues that the epistemic value of models is not limited to their representational function, and develops an account that views models as epistemic artifacts which allow us to gather knowledge in diverse ways. Nersessian (1999, 2010) stresses the role of analogue models in concept-formation and other cognitive processes. Hartmann (1995) and Leplin (1980) discuss models as tools for theory construction and emphasize their heuristic and pedagogical value. Epstein (2008) lists a number of specific functions of models in the social sciences. Peschard (2011) investigates the way in which models may be used to construct other models and generate new target systems. And Isaac (2013) discusses non-explanatory uses of models which do not rely on their representational capacities.
4. Models and Theory
An important question concerns the relation between models and theories. There is a full spectrum of positions ranging from models being subordinate to theories to models being independent of theories.
To discuss the relation between models and theories in science it is helpful to briefly recapitulate the notions of a model and of a theory in logic. A theory is taken to be a (usually deductively closed) set of sentences in a formal language. A model is a structure (in the sense introduced in Section 2.3 ) that makes all sentences of a theory true when its symbols are interpreted as referring to objects, relations, or functions of a structure. The structure is a model of the theory in the sense that it is correctly described by the theory (see Bell and Machover 1977 or Hodges 1997 for details). Logical models are sometimes also referred to as “models of theory” to indicate that they are interpretations of an abstract formal system.
Models in science sometimes carry over from logic the idea of being the interpretation of an abstract calculus (Hesse 1967). This is salient in physics, where general laws—such as Newton’s equation of motion—lie at the heart of a theory. These laws are applied to a particular system—e.g., a pendulum—by choosing a special force function, making assumptions about the mass distribution of the pendulum etc. The resulting model then is an interpretation (or realization) of the general law.
It is important to keep the notions of a logical and a representational model separate (Thomson-Jones 2006): these are distinct concepts. Something can be a logical model without being a representational model, and vice versa . This, however, does not mean that something cannot be a model in both senses at once. In fact, as Hesse (1967) points out, many models in science are both logical and representational models. Newton’s model of planetary motion is a case in point: the model, consisting of two homogeneous perfect spheres located in otherwise empty space that attract each other gravitationally, is simultaneously a logical model (because it makes the axioms of Newtonian mechanics true when they are interpreted as referring to the model) and a representational model (because it represents the real sun and earth).
There are two main conceptions of scientific theories, the so-called syntactic view of theories and the so-called semantic view of theories (see the entry on the structure of scientific theories ). On both conceptions models play a subsidiary role to theories, albeit in very different ways. The syntactic view of theories (see entry section on the syntactic view ) retains the logical notions of a model and a theory. It construes a theory as a set of sentences in an axiomatized logical system, and a model as an alternative interpretation of a certain calculus (Braithwaite 1953; Campbell 1920 [1957]; Nagel 1961; Spector 1965). If, for instance, we take the mathematics used in the kinetic theory of gases and reinterpret the terms of this calculus in a way that makes them refer to billiard balls, the billiard balls are a model of the kinetic theory of gases in the sense that all sentences of the theory come out true. The model is meant to be something that we are familiar with, and it serves the purpose of making an abstract formal calculus more palpable. A given theory can have different models, and which model we choose depends both on our aims and our background knowledge. Proponents of the syntactic view disagree about the importance of models. Carnap and Hempel thought that models only serve a pedagogic or aesthetic purpose and are ultimately dispensable because all relevant information is contained in the theory (Carnap 1938; Hempel 1965; see also Bailer-Jones 1999). Nagel (1961) and Braithwaite (1953), on the other hand, emphasize the heuristic role of models, and Schaffner (1969) submits that theoretical terms get at least part of their meaning from models.
The semantic view of theories (see entry section on the semantic view ) dispenses with sentences in an axiomatized logical system and construes a theory as a family of models. On this view, a theory literally is a class, cluster, or family of models—models are the building blocks of which scientific theories are made up. Different versions of the semantic view work with different notions of a model, but, as noted in Section 2.3 , in the semantic view models are mostly construed as set-theoretic structures. For a discussion of the different options, we refer the reader to the relevant entry in this encyclopedia (linked at the beginning of this paragraph).
In both the syntactic and the semantic view of theories models are seen as subordinate to theory and as playing no role outside the context of a theory. This vision of models has been challenged in a number of ways, with authors pointing out that models enjoy various degrees of freedom from theory and function autonomously in many contexts. Independence can take many forms, and large parts of the literature on models are concerned with investigating various forms of independence.
Models as completely independent of theory . The most radical departure from a theory-centered analysis of models is the realization that there are models that are completely independent from any theory. An example of such a model is the Lotka–Volterra model. The model describes the interaction of two populations: a population of predators and one of prey animals (Weisberg 2013). The model was constructed using only relatively commonsensical assumptions about predators and prey and the mathematics of differential equations. There was no appeal to a theory of predator–prey interactions or a theory of population growth, and the model is independent of theories about its subject matter. If a model is constructed in a domain where no theory is available, then the model is sometimes referred to as a “substitute model” (Groenewold 1961), because the model substitutes a theory.
Models as a means to explore theory . Models can also be used to explore theories (Morgan and Morrison 1999). An obvious way in which this can happen is when a model is a logical model of a theory (see Section 4.1 ). A logical model is a set of objects and properties that make a formal sentence true, and so one can see in the model how the axioms of the theory play out in a particular setting and what kinds of behavior they dictate. But not all models that are used to explore theories are logical models, and models can represent features of theories in other ways. As an example, consider chaos theory. The equations of non-linear systems, such as those describing the three-body problem, have solutions that are too complex to study with paper-and-pencil methods, and even computer simulations are limited in various ways. Abstract considerations about the qualitative behavior of solutions show that there is a mechanism that has been dubbed “stretching and folding” (see the entry Chaos ). To obtain an idea of the complexity of the dynamics exhibiting stretching and folding, Smale proposed to study a simple model of the flow—now known as the “horseshoe map” (Tabor 1989)—which provides important insights into the nature of stretching and folding. Other examples of models of that kind are the Kac ring model that is used to study equilibrium properties of systems in statistical mechanics (Lavis 2008) and Norton’s dome in Newtonian mechanics (Norton 2003).
Models as complements of theories . A theory may be incompletely specified in the sense that it only imposes certain general constraints but remains silent about the details of concrete situations, which are provided by a model (Redhead 1980). A special case of this situation is when a qualitative theory is known and the model introduces quantitative measures (Apostel 1961). Redhead’s example of a theory that is underdetermined in this way is axiomatic quantum field theory, which only imposes certain general constraints on quantum fields but does not provide an account of particular fields. Harré (2004) notes that models can complement theories by providing mechanisms for processes that are left unspecified in the theory even though they are responsible for bringing about the observed phenomena.
Theories may be too complicated to handle. In such cases a model can complement a theory by providing a simplified version of the theoretical scenario that allows for a solution. Quantum chromodynamics, for instance, cannot easily be used to investigate the physics of an atomic nucleus even though it is the relevant fundamental theory. To get around this difficulty, physicists construct tractable phenomenological models (such as the MIT bag model) which effectively describe the relevant degrees of freedom of the system under consideration (Hartmann 1999, 2001). The advantage of these models is that they yield results where theories remain silent. Their drawback is that it is often not clear how to understand the relationship between the model and the theory, as the two are, strictly speaking, contradictory.
Models as preliminary theories . The notion of a model as a substitute for a theory is closely related to the notion of a developmental model . This term was coined by Leplin (1980), who pointed out how useful models were in the development of early quantum theory, and it is now used as an umbrella notion covering cases in which models are some sort of a preliminary exercise to theory.
Also closely related is the notion of a probing model (or “study model”). Models of this kind do not perform a representational function and are not expected to instruct us about anything beyond the model itself. The purpose of these models is to test new theoretical tools that are used later on to build representational models. In field theory, for instance, the so-called φ 4 -model was studied extensively, not because it was believed to represent anything real, but because it served several heuristic functions: the simplicity of the φ 4 -model allowed physicists to “get a feeling” for what quantum field theories are like and to extract some general features that this simple model shared with more complicated ones. Physicists could study complicated techniques such as renormalization in a simple setting, and it was possible to get acquainted with important mechanisms—in this case symmetry-breaking—that could later be used in different contexts (Hartmann 1995). This is true not only for physics. As Wimsatt (1987, 2007) points out, a false model in genetics can perform many useful functions, among them the following: the false model can help answering questions about more realistic models, provide an arena for answering questions about properties of more complex models, “factor out” phenomena that would not otherwise be seen, serve as a limiting case of a more general model (or two false models may define the extremes of a continuum of cases on which the real case is supposed to lie), or lead to the identification of relevant variables and the estimation of their values.
Interpretative models . Cartwright (1983, 1999) argues that models do not only aid the application of theories that are somehow incomplete; she claims that models are also involved whenever a theory with an overarching mathematical structure is applied. The main theories in physics—classical mechanics, electrodynamics, quantum mechanics, and so on—fall into this category. Theories of that kind are formulated in terms of abstract concepts that need to be concretized for the theory to provide a description of the target system, and concretizing the relevant concepts, idealized objects and processes are introduced. For instance, when applying classical mechanics, the abstract concept of force has to be replaced with a concrete force such as gravity. To obtain tractable equations, this procedure has to be applied to a simplified scenario, for instance that of two perfectly spherical and homogeneous planets in otherwise empty space, rather than to reality in its full complexity. The result is an interpretative model , which grounds the application of mathematical theories to real-world targets. Such models are independent from theory in that the theory does not determine their form, and yet they are necessary for the application of the theory to a concrete problem.
Models as mediators . The relation between models and theories can be complicated and disorderly. The contributors to a programmatic collection of essays edited by Morgan and Morrison (1999) rally around the idea that models are instruments that mediate between theories and the world. Models are “autonomous agents” in that they are independent from both theories and their target systems, and it is this independence that allows them to mediate between the two. Theories do not provide us with algorithms for the construction of a model; they are not “vending machines” into which one can insert a problem and a model pops out (Cartwright 1999). The construction of a model often requires detailed knowledge about materials, approximation schemes, and the setup, and these are not provided by the corresponding theory. Furthermore, the inner workings of a model are often driven by a number of different theories working cooperatively. In contemporary climate modeling, for instance, elements of different theories—among them fluid dynamics, thermodynamics, electromagnetism—are put to work cooperatively. What delivers the results is not the stringent application of one theory, but the voices of different theories when put to use in chorus with each other in one model.
In complex cases like the study of a laser system or the global climate, models and theories can get so entangled that it becomes unclear where a line between the two should be drawn: where does the model end and the theory begin? This is not only a problem for philosophical analysis; it also arises in scientific practice. Bailer-Jones (2002) interviewed a group of physicists about their understanding of models and their relation to theories, and reports widely diverging views: (i) there is no substantive difference between model and theory; (ii) models become theories when their degree of confirmation increases; (iii) models contain simplifications and omissions, while theories are accurate and complete; (iv) theories are more general than models, and modeling is about applying general theories to specific cases. The first suggestion seems to be too radical to do justice to many aspects of practice, where a distinction between models and theories is clearly made. The second view is in line with common parlance, where the terms “model” and “theory” are sometimes used to express someone’s attitude towards a particular hypothesis. The phrase “it’s just a model” indicates that the hypothesis at stake is asserted only tentatively or is even known to be false, while something is awarded the label “theory” if it has acquired some degree of general acceptance. However, this use of “model” is different from the uses we have seen in Sections 1 to 3 and is therefore of no use if we aim to understand the relation between scientific models and theories (and, incidentally, one can equally dismiss speculative claims as being “just a theory”). The third proposal is correct in associating models with idealizations and simplifications, but it overshoots by restricting this to models; in fact, also theories can contain idealizations and simplifications. The fourth view seems closely aligned with interpretative models and the idea that models are mediators, but being more general is a gradual notion and hence does not provide a clear-cut criterion to distinguish between theories and models.
5. Models and Other Debates in the Philosophy of Science
The debate over scientific models has important repercussions for other issues in the philosophy of science (for a historical account of the philosophical discussion about models, see Bailer-Jones 1999). Traditionally, the debates over, say, scientific realism, reductionism, and laws of nature were couched in terms of theories, because theories were seen as the main carriers of scientific knowledge. Once models are acknowledged as occupying an important place in the edifice of science, these issues have to be reconsidered with a focus on models. The question is whether, and if so how, discussions of these issues change when we shift focus from theories to models. Up to now, no comprehensive model-based account of any of these issues has emerged, but models have left important traces in the discussions of these topics.
As we have seen in Section 1 , models typically provide a distorted representation of their targets. If one sees science as primarily model-based, this could be taken to suggest an antirealist interpretation of science. Realists, however, deny that the presence of idealizations in models renders a realist approach to science impossible and point out that a good model, while not literally true, is usually at least approximately true, and/or that it can be improved by de-idealization (Laymon 1985; McMullin 1985; Nowak 1979; Brzezinski and Nowak 1992).
Apart from the usual worries about the elusiveness of the notion of approximate truth (for a discussion, see the entry on truthlikeness ), antirealists have taken issue with this reply for two (related) reasons. First, as Cartwright (1989) points out, there is no reason to assume that one can always improve a model by adding de-idealizing corrections. Second, it seems that de-idealization is not in accordance with scientific practice because it is unusual that scientists invest work in repeatedly de-idealizing an existing model. Rather, they shift to a different modeling framework once the adjustments to be made get too involved (Hartmann 1998). The various models of the atomic nucleus are a case in point: once it was realized that shell effects are important to understand various subatomic phenomena, the (collective) liquid-drop model was put aside and the (single-particle) shell model was developed to account for the corresponding findings. A further difficulty with de-idealization is that most idealizations are not “controlled”. For example, it is not clear in what way one could de-idealize the MIT bag model to eventually arrive at quantum chromodynamics, the supposedly correct underlying theory.
A further antirealist argument, the “incompatible-models argument”, takes as its starting point the observation that scientists often successfully use several incompatible models of one and the same target system for predictive purposes (Morrison 2000). These models seemingly contradict each other, as they ascribe different properties to the same target system. In nuclear physics, for instance, the liquid-drop model explores the analogy of the atomic nucleus with a (charged) fluid drop, while the shell model describes nuclear properties in terms of the properties of protons and neutrons, the constituents of an atomic nucleus. This practice appears to cause a problem for scientific realism: Realists typically hold that there is a close connection between the predictive success of a theory and its being at least approximately true. But if several models of the same system are predictively successful and if these models are mutually inconsistent, then it is difficult to maintain that they are all approximately true.
Realists can react to this argument in various ways. First, they can challenge the claim that the models in question are indeed predictively successful. If the models are not good predictors, then the argument is blocked. Second, they can defend a version of “perspectival realism” (Giere 2006; Massimi 2017; Rueger 2005). Proponents of this position (which is sometimes also called “perspectivism”) situate it somewhere between “standard” scientific realism and antirealism, and where exactly the right middle position lies is the subject matter of active debate (Massimi 2018a,b; Saatsi 2016; Teller 2018; and the contributions to Massimi and McCoy 2019). Third, realists can deny that there is a problem in the first place, because scientific models, which are always idealized and therefore strictly speaking false, are just the wrong vehicle to make a point about realism (which should be discussed in terms of theories).
A particular focal point of the realism debate are laws of nature, where the questions arise what laws are and whether they are truthfully reflected in our scientific representations. According to the two currently dominant accounts, the best-systems approach and the necessitarian approach, laws of nature are understood to be universal in scope, meaning that they apply to everything that there is in the world (for discussion of laws, see the entry on laws of nature ). This take on laws does not seem to sit well with a view that places models at the center of scientific research. What role do general laws play in science if it is models that represent what is happening in the world? And how are models and laws related?
One possible response to these questions is to argue that laws of nature govern entities and processes in a model rather than in the world. Fundamental laws, on this approach, do not state facts about the world but hold true of entities and processes in the model. This view has been advocated in different variants: Cartwright (1983) argues that all laws are ceteris paribus laws. Cartwright (1999) makes use of “capacities” (which she considers to be prior to laws) and introduces the notion of a “nomological machine”. This is
a fixed (enough) arrangement of components, or factors, with stable (enough) capacities that in the right sort of stable (enough) environment will, with repeated operation, give rise to the kind of regular behavior that we represent in our scientific laws. (1999: 50; see also the entry on ceteris paribus laws )
Giere (1999) argues that the laws of a theory are better thought of, not as encoding general truths about the world, but rather as open-ended statements that can be filled in various ways in the process of building more specific scientific models. Similar positions have also been defended by Teller (2001) and van Fraassen (1989).
The multiple-models problem mentioned in Section 5.1 also raises the question of how different models are related. Evidently, multiple models for the same target system do not generally stand in a deductive relationship, as they often contradict each other. Some (Cartwright 1999; Hacking 1983) have suggested a picture of science according to which there are no systematic relations that hold between different models. Some models are tied together because they represent the same target system, but this does not imply that they enter into any further relationships (deductive or otherwise). We are confronted with a patchwork of models, all of which hold ceteris paribus in their specific domains of applicability.
Some argue that this picture is at least partially incorrect because there are various interesting relations that hold between different models or theories. These relations range from thoroughgoing reductive relations (Scheibe 1997, 1999, 2001: esp. Chs. V.23 and V.24) and controlled approximations over singular limit relations (Batterman 2001 [2016]) to structural relations (Gähde 1997) and rather loose relations called “stories” (Hartmann 1999; see also Bokulich 2003; Teller 2002; and the essays collected in Part III of Hartmann et al. 2008). These suggestions have been made on the basis of case studies, and it remains to be seen whether a more general account of these relations can be given and whether a deeper justification for them can be provided, for instance, within a Bayesian framework (first steps towards a Bayesian understanding of reductive relations can be found in Dizadji-Bahmani et al. 2011; Liefke and Hartmann 2018; and Tešić 2019).
Models also figure in the debate about reduction and emergence in physics. Here, some authors argue that the modern approach to renormalization challenges Nagel’s (1961) model of reduction or the broader doctrine of reductions (for a critical discussion, see, for instance, Batterman 2002, 2010, 2011; Morrison 2012; and Saatsi and Reutlinger 2018). Dizadji-Bahmani et al. (2010) provide a defense of the Nagel–Schaffner model of reduction, and Butterfield (2011a,b, 2014) argues that renormalization is consistent with Nagelian reduction. Palacios (2019) shows that phase transitions are compatible with reductionism, and Hartmann (2001) argues that the effective-field-theories research program is consistent with reductionism (see also Bain 2013 and Franklin forthcoming). Rosaler (2015) argues for a “local” form of reduction which sees the fundamental relation of reduction holding between models, not theories, which is, however, compatible with the Nagel–Schaffner model of reduction. See also the entries on intertheory relations in physics and scientific reduction .
In the social sciences, agent-based models (ABMs) are increasingly used (Klein et al. 2018). These models show how surprisingly complex behavioral patterns at the macro-scale can emerge from a small number of simple behavioral rules for the individual agents and their interactions. This raises questions similar to the questions mentioned above about reduction and emergence in physics, but so far one only finds scattered remarks about reduction in the literature. See Weisberg and Muldoon (2009) and Zollman (2007) for the application of ABMs to the epistemology and the social structure of science, and Colyvan (2013) for a discussion of methodological questions raised by normative models in general.
- Achinstein, Peter, 1968, Concepts of Science: A Philosophical Analysis , Baltimore, MD: Johns Hopkins Press.
- Akerlof, George A., 1970, “The Market for ‘Lemons’: Quality Uncertainty and the Market Mechanism”, The Quarterly Journal of Economics , 84(3): 488–500. doi:10.2307/1879431
- Apostel, Leo, 1961, “Towards the Formal Study of Models in the Non-Formal Sciences”, in Freudenthal 1961: 1–37. doi:10.1007/978-94-010-3667-2_1
- Bailer-Jones, Daniela M., 1999, “Tracing the Development of Models in the Philosophy of Science”, in Magnani, Nersessian, and Thagard 1999: 23–40. doi:10.1007/978-1-4615-4813-3_2
- –––, 2002, “Scientists’ Thoughts on Scientific Models”, Perspectives on Science , 10(3): 275–301. doi:10.1162/106361402321899069
- –––, 2009, Scientific Models in Philosophy of Science , Pittsburgh, PA: University of Pittsburgh Press.
- Bailer-Jones, Daniela M. and Coryn A. L. Bailer-Jones, 2002, “Modeling Data: Analogies in Neural Networks, Simulated Annealing and Genetic Algorithms”, in Magnani and Nersessian 2002: 147–165. doi:10.1007/978-1-4615-0605-8_9
- Bain, Jonathan, 2013, “Emergence in Effective Field Theories”, European Journal for Philosophy of Science , 3(3): 257–273. doi:10.1007/s13194-013-0067-0
- Bandyopadhyay, Prasanta S. and Malcolm R. Forster (eds.), 2011, Philosophy of Statistics (Handbook of the Philosophy of Science 7), Amsterdam: Elsevier.
- Barberousse, Anouk and Pascal Ludwig, 2009, “Fictions and Models”, in Suárez 2009: 56–75.
- Bartha, Paul, 2010, By Parallel Reasoning: The Construction and Evaluation of Analogical Arguments , New York: Oxford University Press. doi:10.1093/acprof:oso/9780195325539.001.0001
- –––, 2013 [2019], “Analogy and Analogical Reasoning”, in Edward N. Zalta (ed.), The Stanford Encyclopedia of Philosophy (Spring 2019 Edition). URL = < https://plato.stanford.edu/archives/spr2019/entries/reasoning-analogy/ >
- Batterman, Robert W., 2002, The Devil in the Details: Asymptotic Reasoning in Explanation, Reduction, and Emergence , Oxford: Oxford University Press. doi:10.1093/0195146476.001.0001
- –––, 2001 [2016], “Intertheory Relations in Physics”, in Edward N. Zalta (ed.), The Stanford Encyclopedia of Philosophy (Fall 2016 Edition). URL = < https://plato.stanford.edu/archives/fall2016/entries/physics-interrelate >
- –––, 2010, “Reduction and Renormalization”, in Gerhard Ernst and Andreas Hüttemann (eds.), Time, Chance and Reduction: Philosophical Aspects of Statistical Mechanics , Cambridge: Cambridge University Press, pp. 159–179.
- –––, 2011, “Emergence, Singularities, and Symmetry Breaking”, Foundations of Physics , 41(6): 1031–1050. doi:10.1007/s10701-010-9493-4
- Batterman, Robert W. and Collin C. Rice, 2014, “Minimal Model Explanations”, Philosophy of Science , 81(3): 349–376. doi:10.1086/676677
- Baumberger, Christoph and Georg Brun, 2017, “Dimensions of Objectual Understanding”, in Stephen R. Grimm, Christoph Baumberger, and Sabine Ammon (eds.), Explaining Understanding: New Perspectives from Epistemology and Philosophy of Science , New York: Routledge, pp. 165–189.
- Bell, John and Moshé Machover, 1977, A Course in Mathematical Logic , Amsterdam: North-Holland.
- Berry, Michael, 2002, “Singular Limits”, Physics Today , 55(5): 10–11. doi:10.1063/1.1485555
- Black, Max, 1962, Models and Metaphors: Studies in Language and Philosophy , Ithaca, NY: Cornell University Press.
- Bogen, James and James Woodward, 1988, “Saving the Phenomena”, The Philosophical Review , 97(3): 303–352. doi:10.2307/2185445
- Bokulich, Alisa, 2003, “Horizontal Models: From Bakers to Cats”, Philosophy of Science , 70(3): 609–627. doi:10.1086/376927
- –––, 2008, Reexamining the Quantum–Classical Relation: Beyond Reductionism and Pluralism , Cambridge: Cambridge University Press. doi:10.1017/CBO9780511751813
- –––, 2009, “Explanatory Fictions”, in Suárez 2009: 91–109.
- –––, 2011, “How Scientific Models Can Explain”, Synthese , 180(1): 33–45. doi:10.1007/s11229-009-9565-1
- –––, 2012, “Distinguishing Explanatory from Nonexplanatory Fictions”, Philosophy of Science , 79(5): 725–737. doi:10.1086/667991
- Braithwaite, Richard, 1953, Scientific Explanation , Cambridge: Cambridge University Press.
- Braun, Norman and Nicole J. Saam (eds.), 2015, Handbuch Modellbildung und Simulation in den Sozialwissenschaften , Wiesbaden: Springer Fachmedien. doi:10.1007/978-3-658-01164-2
- Brewer, William F. and Clark A. Chinn, 1994, “Scientists’ Responses to Anomalous Data: Evidence from Psychology, History, and Philosophy of Science”, in PSA 1994: Proceedings of the 1994 Biennial Meeting of the Philosophy of Science Association , Vol. 1, pp. 304–313. doi:10.1086/psaprocbienmeetp.1994.1.193035
- Brown, James, 1991, The Laboratory of the Mind: Thought Experiments in the Natural Sciences , London: Routledge.
- Brzezinski, Jerzy and Leszek Nowak (eds.), 1992, Idealization III: Approximation and Truth , Amsterdam: Rodopi.
- Butterfield, Jeremy, 2011a, “Emergence, Reduction and Supervenience: A Varied Landscape”, Foundations of Physics , 41(6): 920–959. doi:10.1007/s10701-011-9549-0
- –––, 2011b, “Less Is Different: Emergence and Reduction Reconciled”, Foundations of Physics , 41(6): 1065–1135. doi:10.1007/s10701-010-9516-1
- –––, 2014, “Reduction, Emergence, and Renormalization”, Journal of Philosophy , 111(1): 5–49. doi:10.5840/jphil201411111
- Callender, Craig and Jonathan Cohen, 2006, “There Is No Special Problem about Scientific Representation”, Theoria , 55(1): 67–85.
- Campbell, Norman, 1920 [1957], Physics: The Elements , Cambridge: Cambridge University Press. Reprinted as Foundations of Science , New York: Dover, 1957.
- Carnap, Rudolf, 1938, “Foundations of Logic and Mathematics”, in Otto Neurath, Charles Morris, and Rudolf Carnap (eds.), International Encyclopaedia of Unified Science , Volume 1, Chicago, IL: University of Chicago Press, pp. 139–213.
- Cartwright, Nancy, 1983, How the Laws of Physics Lie , Oxford: Oxford University Press. doi:10.1093/0198247044.001.0001
- –––, 1989, Nature’s Capacities and Their Measurement , Oxford: Oxford University Press. doi:10.1093/0198235070.001.0001
- –––, 1999, The Dappled World: A Study of the Boundaries of Science , Cambridge: Cambridge University Press. doi:10.1017/CBO9781139167093
- Colombo, Matteo, Stephan Hartmann, and Robert van Iersel, 2015, “Models, Mechanisms, and Coherence”, The British Journal for the Philosophy of Science , 66(1): 181–212. doi:10.1093/bjps/axt043
- Colyvan, Mark, 2013, “Idealisations in Normative Models”, Synthese , 190(8): 1337–1350. doi:10.1007/s11229-012-0166-z
- Contessa, Gabriele, 2010, “Scientific Models and Fictional Objects”, Synthese , 172(2): 215–229. doi:10.1007/s11229-009-9503-2
- Crowther, Karen, Niels S. Linnemann, and Christian Wüthrich, forthcoming, “What We Cannot Learn from Analogue Experiments”, Synthese , first online: 4 May 2019. doi:10.1007/s11229-019-02190-0
- Da Costa, Newton and Steven French, 2000, “Models, Theories, and Structures: Thirty Years On”, Philosophy of Science , 67(supplement): S116–S127. doi:10.1086/392813
- Dardashti, Radin, Stephan Hartmann, Karim Thébault, and Eric Winsberg, 2019, “Hawking Radiation and Analogue Experiments: A Bayesian Analysis”, Studies in History and Philosophy of Science Part B: Studies in History and Philosophy of Modern Physics , 67: 1–11. doi:10.1016/j.shpsb.2019.04.004
- Dardashti, Radin, Karim P. Y. Thébault, and Eric Winsberg, 2017, “Confirmation via Analogue Simulation: What Dumb Holes Could Tell Us about Gravity”, The British Journal for the Philosophy of Science , 68(1): 55–89. doi:10.1093/bjps/axv010
- de Regt, Henk, 2009, “Understanding and Scientific Explanation”, in de Regt, Leonelli, and Eigner 2009: 21–42.
- –––, 2017, Understanding Scientific Understanding , Oxford: Oxford University Press. doi:10.1093/oso/9780190652913.001.0001
- de Regt, Henk, Sabina Leonelli, and Kai Eigner (eds.), 2009, Scientific Understanding: Philosophical Perspectives , Pittsburgh, PA: University of Pittsburgh Press.
- Dizadji-Bahmani, Foad, Roman Frigg, and Stephan Hartmann, 2010, “Who’s Afraid of Nagelian Reduction?”, Erkenntnis , 73(3): 393–412. doi:10.1007/s10670-010-9239-x
- –––, 2011, “Confirmation and Reduction: A Bayesian Account”, Synthese , 179(2): 321–338. doi:10.1007/s11229-010-9775-6
- Downes, Stephen M., 1992, “The Importance of Models in Theorizing: A Deflationary Semantic View”, PSA: Proceedings of the Biennial Meeting of the Philosophy of Science Association , 1992(1): 142–153. doi:10.1086/psaprocbienmeetp.1992.1.192750
- Elgin, Catherine Z., 2010, “Telling Instances”, in Roman Frigg and Matthew Hunter (eds.), Beyond Mimesis and Convention (Boston Studies in the Philosophy of Science 262), Dordrecht: Springer Netherlands, pp. 1–17. doi:10.1007/978-90-481-3851-7_1
- –––, 2017, True Enough . Cambridge, MA, and London: MIT Press.
- Elgin, Mehmet and Elliott Sober, 2002, “Cartwright on Explanation and Idealization”, Erkenntnis , 57(3): 441–450. doi:10.1023/A:1021502932490
- Epstein, Joshua M., 2008, “Why Model?”, Journal of Artificial Societies and Social Simulation , 11(4): 12. [ Epstein 2008 available online ]
- Fisher, Grant, 2006, “The Autonomy of Models and Explanation: Anomalous Molecular Rearrangements in Early Twentieth-Century Physical Organic Chemistry”, Studies in History and Philosophy of Science Part A , 37(4): 562–584. doi:10.1016/j.shpsa.2006.09.009
- Franklin, Alexander, forthcoming, “Whence the Effectiveness of Effective Field Theories?”, The British Journal for the Philosophy of Science , first online: 3 August 2018. doi:10.1093/bjps/axy050
- Freudenthal, Hans (ed.), 1961, The Concept and the Role of the Model in Mathematics and Natural and Social Sciences , Dordrecht: Reidel. doi:10.1007/978-94-010-3667-2
- Friedman, Michael, 1974, “Explanation and Scientific Understanding”, Journal of Philosophy , 71(1): 5–19. doi:10.2307/2024924
- Frigg, Roman, 2010a, “Fiction in Science”, in John Woods (ed.), Fictions and Models: New Essays , Munich: Philosophia Verlag, pp. 247–287.
- –––, 2010b, “Models and Fiction”, Synthese , 172(2): 251–268. doi:10.1007/s11229-009-9505-0
- Frigg, Roman, Seamus Bradley, Hailiang Du, and Leonard A. Smith, 2014, “Laplace’s Demon and the Adventures of His Apprentices”, Philosophy of Science , 81(1): 31–59. doi:10.1086/674416
- Frigg, Roman and James Nguyen, 2016, “The Fiction View of Models Reloaded”, The Monist , 99(3): 225–242. doi:10.1093/monist/onw002 [ Frigg and Nguyen 2016 available online ]
- –––, forthcoming, “Mirrors without Warnings”, Synthese , first online: 21 May 2019. doi:10.1007/s11229-019-02222-9
- Fumagalli, Roberto, 2016, “Why We Cannot Learn from Minimal Models”, Erkenntnis , 81(3): 433–455. doi:10.1007/s10670-015-9749-7
- Gähde, Ulrich, 1997, “Anomalies and the Revision of Theory-Elements: Notes on the Advance of Mercury’s Perihelion”, in Maria Luisa Dalla Chiara, Kees Doets, Daniele Mundici, and Johan van Benthem (eds.), Structures and Norms in Science (Synthese Library 260), Dordrecht: Springer Netherlands, pp. 89–104. doi:10.1007/978-94-017-0538-7_6
- Galison, Peter, 1997, Image and Logic: A Material Culture of Microphysics , Chicago, IL: University of Chicago Press.
- Gelfert, Axel, 2016, How to Do Science with Models: A Philosophical Primer (Springer Briefs in Philosophy), Cham: Springer International Publishing. doi:10.1007/978-3-319-27954-1
- Gendler, Tamar Szabó, 2000, Thought Experiment: On the Powers and Limits of Imaginary Cases , New York and London: Garland.
- Gibbard, Allan and Hal R. Varian, 1978, “Economic Models”, The Journal of Philosophy , 75(11): 664–677. doi:10.5840/jphil1978751111
- Giere, Ronald N., 1988, Explaining Science: A Cognitive Approach , Chicago, IL: University of Chicago Press.
- –––, 1999, Science Without Laws , Chicago, IL: University of Chicago Press.
- –––, 2006, Scientific Perspectivism , Chicago, IL: University of Chicago Press.
- –––, 2009, “Why Scientific Models Should Not be Regarded as Works of Fiction”, in Suárez 2009: 248–258.
- –––, 2010, “An Agent-Based Conception of Models and Scientific Representation”, Synthese , 172(2): 269–281. doi:10.1007/s11229-009-9506-z
- Godfrey-Smith, Peter, 2007, “The Strategy of Model-Based Science”, Biology & Philosophy , 21(5): 725–740. doi:10.1007/s10539-006-9054-6
- –––, 2009, “Abstractions, Idealizations, and Evolutionary Biology”, in Anouk Barberousse, Michel Morange, and Thomas Pradeu (eds.), Mapping the Future of Biology: Evolving Concepts and Theories (Boston Studies in the Philosophy of Science 266), Dordrecht: Springer Netherlands, pp. 47–56. doi:10.1007/978-1-4020-9636-5_4
- Groenewold, H. J., 1961, “The Model in Physics”, in Freudenthal 1961: 98–103. doi:10.1007/978-94-010-3667-2_9
- Grüne-Yanoff, Till, 2009, “Learning from Minimal Economic Models”, Erkenntnis , 70(1): 81–99. doi:10.1007/s10670-008-9138-6
- Hacking, Ian, 1983, Representing and Intervening: Introductory Topics in the Philosophy of Natural Science , Cambridge: Cambridge University Press. doi:10.1017/CBO9780511814563
- Hale, Susan C., 1988, “Spacetime and the Abstract/Concrete Distinction”, Philosophical Studies , 53(1): 85–102. doi:10.1007/BF00355677
- Harré, Rom, 2004, Modeling: Gateway to the Unknown (Studies in Multidisciplinarity 1), ed. by Daniel Rothbart, Amsterdam etc.: Elsevier.
- Harris, Todd, 2003, “Data Models and the Acquisition and Manipulation of Data”, Philosophy of Science , 70(5): 1508–1517. doi:10.1086/377426
- Hartmann, Stephan, 1995, “Models as a Tool for Theory Construction: Some Strategies of Preliminary Physics”, in Herfel et al. 1995: 49–67.
- –––, 1996, “The World as a Process: Simulations in the Natural and Social Sciences”, in Hegselmann, Mueller, and Troitzsch 1996: 77–100. doi:10.1007/978-94-015-8686-3_5
- –––, 1998, “Idealization in Quantum Field Theory”, in Shanks 1998: 99–122.
- –––, 1999, “Models and Stories in Hadron Physics”, in Morgan and Morrison 1999: 326–346. doi:10.1017/CBO9780511660108.012
- –––, 2001, “Effective Field Theories, Reductionism and Scientific Explanation”, Studies in History and Philosophy of Science Part B: Studies in History and Philosophy of Modern Physics , 32(2): 267–304. doi:10.1016/S1355-2198(01)00005-3
- Hartmann, Stephan, Carl Hoefer, and Luc Bovens (eds.), 2008, Nancy Cartwright’s Philosophy of Science (Routledge Studies in the Philosophy of Science), New York: Routledge.
- Hegselmann, Rainer, Ulrich Mueller, and Klaus G. Troitzsch (eds.), 1996, Modelling and Simulation in the Social Sciences from the Philosophy of Science Point of View (Theory and Decision Library 23), Dordrecht: Springer Netherlands. doi:10.1007/978-94-015-8686-3
- Helman, David H. (ed.), 1988, Analogical Reasoning: Perspectives of Artificial Intelligence, Cognitive Science, and Philosophy (Synthese Library 197), Dordrecht: Springer Netherlands. doi:10.1007/978-94-015-7811-0
- Hempel, Carl G., 1965, Aspects of Scientific Explanation and Other Essays in the Philosophy of Science , New York: Free Press.
- Herfel, William, Wladiysław Krajewski, Ilkka Niiniluoto, and Ryszard Wojcicki (eds.), 1995, Theories and Models in Scientific Process (Poznań Studies in the Philosophy of Science and the Humanities 44), Amsterdam: Rodopi.
- Hesse, Mary, 1963, Models and Analogies in Science , London: Sheed and Ward.
- –––, 1967, “Models and Analogy in Science”, in Paul Edwards (ed.), Encyclopedia of Philosophy , New York: Macmillan, pp. 354–359.
- –––, 1974, The Structure of Scientific Inference , London: Macmillan.
- Hodges, Wilfrid, 1997, A Shorter Model Theory , Cambridge: Cambridge University Press.
- Holyoak, Keith and Paul Thagard, 1995, Mental Leaps: Analogy in Creative Thought , Cambridge, MA: MIT Press.
- Horowitz, Tamara and Gerald J. Massey (eds.), 1991, Thought Experiments in Science and Philosophy , Lanham, MD: Rowman & Littlefield.
- Isaac, Alistair M. C., 2013, “Modeling without Representation”, Synthese , 190(16): 3611–3623. doi:10.1007/s11229-012-0213-9
- Jebeile, Julie and Ashley Graham Kennedy, 2015, “Explaining with Models: The Role of Idealizations”, International Studies in the Philosophy of Science , 29(4): 383–392. doi:10.1080/02698595.2015.1195143
- Jones, Martin R., 2005, “Idealization and Abstraction: A Framework”, in Jones and Cartwright 2005: 173–217. doi:10.1163/9789401202732_010
- Jones, Martin R. and Nancy Cartwright (eds.), 2005, Idealization XII: Correcting the Model (Poznań Studies in the Philosophy of the Sciences and the Humanities 86), Amsterdam and New York: Rodopi. doi:10.1163/9789401202732
- Kelvin, William Thomson, Baron, 1884 [1987], Notes of lectures on molecular dynamics and the wave theory of light. Delivered at the Johns Hopkins University, Baltimore (aka Lord Kelvin’s Baltimore Lectures), A. S. Hathaway (recorder). A revised version was published in 1904, London: C.J. Clay and Sons. Reprint of the 1884 version in Robert Kargon and Peter Achinstein (eds.), Kelvin’s Baltimore Lectures and Modern Theoretical Physics , Cambridge, MA: MIT Press, 1987.
- Khalifa, Kareem, 2017, Understanding, Explanation, and Scientific Knowledge , Cambridge: Cambridge University Press. doi:10.1017/9781108164276
- Klein, Dominik, Johannes Marx, and Kai Fischbach, 2018, “Agent-Based Modeling in Social Science History and Philosophy: An Introduction”, Historical Social Research , 43(1): 243–258.
- Knuuttila, Tarja, 2005, “Models, Representation, and Mediation”, Philosophy of Science , 72(5): 1260–1271. doi:10.1086/508124
- –––, 2011, “Modelling and Representing: An Artefactual Approach to Model-Based Representation”, Studies in History and Philosophy of Science Part A , 42(2): 262–271. doi:10.1016/j.shpsa.2010.11.034
- Kroes, Peter, 1989, “Structural Analogies Between Physical Systems”, The British Journal for the Philosophy of Science , 40(2): 145–154. doi:10.1093/bjps/40.2.145
- Lange, Marc, 2015, “On ‘Minimal Model Explanations’: A Reply to Batterman and Rice”, Philosophy of Science , 82(2): 292–305. doi:10.1086/680488
- Lavis, David A., 2008, “Boltzmann, Gibbs, and the Concept of Equilibrium”, Philosophy of Science , 75(5): 682–692. doi:10.1086/594514
- Laymon, Ronald, 1982, “Scientific Realism and the Hierarchical Counterfactual Path from Data to Theory”, PSA: Proceedings of the Biennial Meeting of the Philosophy of Science Association , 1982(1): 107–121. doi:10.1086/psaprocbienmeetp.1982.1.192660
- –––, 1985, “Idealizations and the Testing of Theories by Experimentation”, in Peter Achinstein and Owen Hannaway (eds.), Observation, Experiment, and Hypothesis in Modern Physical Science , Cambridge, MA: MIT Press, pp. 147–173.
- –––, 1991, “Thought Experiments by Stevin, Mach and Gouy: Thought Experiments as Ideal Limits and Semantic Domains”, in Horowitz and Massey 1991: 167–191.
- Leonelli, Sabina, 2010, “Packaging Small Facts for Re-Use: Databases in Model Organism Biology”, in Peter Howlett and Mary S. Morgan (eds.), How Well Do Facts Travel? The Dissemination of Reliable Knowledge , Cambridge: Cambridge University Press, pp. 325–348. doi:10.1017/CBO9780511762154.017
- –––, 2016, Data-Centric Biology: A Philosophical Study , Chicago, IL, and London: University of Chicago Press.
- –––, 2019, “What Distinguishes Data from Models?”, European Journal for Philosophy of Science , 9(2): article 22. doi:10.1007/s13194-018-0246-0
- Leonelli, Sabina and Rachel A. Ankeny, 2012, “Re-Thinking Organisms: The Impact of Databases on Model Organism Biology”, Studies in History and Philosophy of Science Part C: Studies in History and Philosophy of Biological and Biomedical Sciences , 43(1): 29–36. doi:10.1016/j.shpsc.2011.10.003
- Leplin, Jarrett, 1980, “The Role of Models in Theory Construction”, in Thomas Nickles (ed.), Scientific Discovery, Logic, and Rationality (Boston Studies in the Philosophy of Science 56), Dordrecht: Springer Netherlands, 267–283. doi:10.1007/978-94-009-8986-3_12
- Levy, Arnon, 2012, “Models, Fictions, and Realism: Two Packages”, Philosophy of Science , 79(5): 738–748. doi:10.1086/667992
- –––, 2015, “Modeling without Models”, Philosophical Studies , 172(3): 781–798. doi:10.1007/s11098-014-0333-9
- Levy, Arnon and Adrian Currie, 2015, “Model Organisms Are Not (Theoretical) Models”, The British Journal for the Philosophy of Science , 66(2): 327–348. doi:10.1093/bjps/axt055
- Levy, Arnon and Peter Godfrey-Smith (eds.), 2020, The Scientific Imagination: Philosophical and Psychological Perspectives , New York: Oxford University Press.
- Liefke, Kristina and Stephan Hartmann, 2018, “Intertheoretic Reduction, Confirmation, and Montague’s Syntax–Semantics Relation”, Journal of Logic, Language and Information , 27(4): 313–341. doi:10.1007/s10849-018-9272-8
- Lipton, Peter, 2009, “Understanding without Explanation”, in de Regt, Leonelli, and Eigner 2009: 43–63.
- Luczak, Joshua, 2017, “Talk about Toy Models”, Studies in History and Philosophy of Science Part B: Studies in History and Philosophy of Modern Physics , 57: 1–7. doi:10.1016/j.shpsb.2016.11.002
- Magnani, Lorenzo, 2012, “Scientific Models Are Not Fictions: Model-Based Science as Epistemic Warfare”, in Lorenzo Magnani and Ping Li (eds.), Philosophy and Cognitive Science: Western & Eastern Studies (Studies in Applied Philosophy, Epistemology and Rational Ethics 2), Berlin and Heidelberg: Springer, pp. 1–38. doi:10.1007/978-3-642-29928-5_1
- Magnani, Lorenzo and Claudia Casadio (eds.), 2016, Model-Based Reasoning in Science and Technology: Logical, Epistemological, and Cognitive Issues (Studies in Applied Philosophy, Epistemology and Rational Ethics 27), Cham: Springer International Publishing. doi:10.1007/978-3-319-38983-7
- Magnani, Lorenzo and Nancy J. Nersessian (eds.), 2002, Model-Based Reasoning: Science, Technology, Values , Boston, MA: Springer US. doi:10.1007/978-1-4615-0605-8
- Magnani, Lorenzo, Nancy J. Nersessian, and Paul Thagard (eds.), 1999, Model-Based Reasoning in Scientific Discovery , Boston, MA: Springer US. doi:10.1007/978-1-4615-4813-3
- Mäki, Uskali, 1994, “Isolation, Idealization and Truth in Economics”, in Bert Hamminga and Neil B. De Marchi (eds.), Idealization VI: Idealization in Economics (Poznań Studies in the Philosophy of the Sciences and the Humanities 38), Amsterdam: Rodopi, pp. 147–168.
- Massimi, Michela, 2017, “Perspectivism”, in Juha Saatsi (ed.), The Routledge Handbook of Scientific Realism , London: Routledge, pp. 164–175.
- –––, 2018a, “Four Kinds of Perspectival Truth”, Philosophy and Phenomenological Research , 96(2): 342–359. doi:10.1111/phpr.12300
- –––, 2018b, “Perspectival Modeling”, Philosophy of Science , 85(3): 335–359. doi:10.1086/697745
- –––, 2019, “Two Kinds of Exploratory Models”, Philosophy of Science , 86(5): 869–881. doi:10.1086/705494
- Massimi, Michela and Casey D. McCoy (eds.), 2019, Understanding Perspectivism: Scientific Challenges and Methodological Prospects , New York: Routledge. doi:10.4324/9781315145198
- Mayo, Deborah, 1996, Error and the Growth of Experimental Knowledge , Chicago, IL: University of Chicago Press.
- –––, 2018, Statistical Inference as Severe Testing: How to Get Beyond the Statistics Wars , Cambridge: Cambridge University Press. doi:10.1017/9781107286184
- McMullin, Ernan, 1968, “What Do Physical Models Tell Us?”, in B. Van Rootselaar and J. Frits Staal (eds.), Logic, Methodology and Philosophy of Science III (Studies in Logic and the Foundations of Mathematics 52), Amsterdam: North Holland, pp. 385–396. doi:10.1016/S0049-237X(08)71206-0
- –––, 1985, “Galilean Idealization”, Studies in History and Philosophy of Science Part A , 16(3): 247–273. doi:10.1016/0039-3681(85)90003-2
- Morgan, Mary S., 1999, “Learning from Models”, in Morgan and Morrison 1999: 347–388. doi:10.1017/CBO9780511660108.013
- Morgan, Mary S. and Marcel J. Boumans, 2004, “Secrets Hidden by Two-Dimensionality: The Economy as a Hydraulic Machine”, in Soraya de Chadarevian and Nick Hopwood (eds.), Model: The Third Dimension of Science , Stanford, CA: Stanford University Press, pp. 369–401.
- Morgan, Mary S. and Margaret Morrison (eds.), 1999, Models as Mediators: Perspectives on Natural and Social Science , Cambridge: Cambridge University Press. doi:10.1017/CBO9780511660108
- Morrison, Margaret, 1999, “Models as Autonomous Agents”, in Morgan and Morrison 1999: 38–65. doi:10.1017/CBO9780511660108.004
- –––, 2000, Unifying Scientific Theories: Physical Concepts and Mathematical Structures , Cambridge: Cambridge University Press. doi:10.1017/CBO9780511527333
- –––, 2005, “Approximating the Real: The Role of Idealizations in Physical Theory”, in Jones and Cartwright 2005: 145–172. doi:10.1163/9789401202732_009
- –––, 2009, “Understanding in Physics and Biology: From the Abstract to the Concrete”, in de Regt, Leonelli, and Eigner 2009: 123–145.
- –––, 2012, “Emergent Physics and Micro-Ontology”, Philosophy of Science , 79(1): 141–166. doi:10.1086/663240
- Musgrave, Alan, 1981, “‘Unreal Assumptions’ in Economic Theory: The F-Twist Untwisted”, Kyklos , 34(3): 377–387. doi:10.1111/j.1467-6435.1981.tb01195.x
- Nagel, Ernest, 1961, The Structure of Science: Problems in the Logic of Scientific Explanation , New York: Harcourt, Brace and World.
- Nersessian, Nancy J., 1999, “Model-Based Reasoning in Conceptual Change”, in Magnani, Nersessian, and Thagard 1999: 5–22. doi:10.1007/978-1-4615-4813-3_1
- –––, 2010, Creating Scientific Concepts , Cambridge, MA: MIT Press.
- Nguyen, James, forthcoming, “It’s Not a Game: Accurate Representation with Toy Models”, The British Journal for the Philosophy of Science , first online: 23 March 2019. doi:10.1093/bjps/axz010
- Nguyen, James and Roman Frigg, forthcoming, “Mathematics Is Not the Only Language in the Book of Nature”, Synthese , first online: 28 August 2017. doi:10.1007/s11229-017-1526-5
- Norton, John D., 1991, “Thought Experiments in Einstein’s Work”, in Horowitz and Massey 1991: 129–148.
- –––, 2003, “Causation as Folk Science”, Philosopher’s Imprint , 3: article 4. [ Norton 2003 available online ]
- –––, 2012, “Approximation and Idealization: Why the Difference Matters”, Philosophy of Science , 79(2): 207–232. doi:10.1086/664746
- Nowak, Leszek, 1979, The Structure of Idealization: Towards a Systematic Interpretation of the Marxian Idea of Science , Dordrecht: D. Reidel.
- Palacios, Patricia, 2019, “Phase Transitions: A Challenge for Intertheoretic Reduction?”, Philosophy of Science , 86(4): 612–640. doi:10.1086/704974
- Peschard, Isabelle, 2011, “Making Sense of Modeling: Beyond Representation”, European Journal for Philosophy of Science , 1(3): 335–352. doi:10.1007/s13194-011-0032-8
- Piccinini, Gualtiero and Carl Craver, 2011, “Integrating Psychology and Neuroscience: Functional Analyses as Mechanism Sketches”, Synthese , 183(3): 283–311. doi:10.1007/s11229-011-9898-4
- Pincock, Christopher, 2012, Mathematics and Scientific Representation , Oxford: Oxford University Press. doi:10.1093/acprof:oso/9780199757107.001.0001
- –––, forthcoming, “Concrete Scale Models, Essential Idealization and Causal Explanation”, British Journal for the Philosophy of Science .
- Portides, Demetris P., 2007, “The Relation between Idealisation and Approximation in Scientific Model Construction”, Science & Education , 16(7–8): 699–724. doi:10.1007/s11191-006-9001-6
- –––, 2014, “How Scientific Models Differ from Works of Fiction”, in Lorenzo Magnani (ed.), Model-Based Reasoning in Science and Technology (Studies in Applied Philosophy, Epistemology and Rational Ethics 8), Berlin and Heidelberg: Springer, pp. 75–87. doi:10.1007/978-3-642-37428-9_5
- Potochnik, Angela, 2007, “Optimality Modeling and Explanatory Generality”, Philosophy of Science , 74(5): 680–691.
- –––, 2017, Idealization and the Aims of Science , Chicago, IL: University of Chicago Press.
- Poznic, Michael, 2016, “Make-Believe and Model-Based Representation in Science: The Epistemology of Frigg’s and Toon’s Fictionalist Views of Modeling”, Teorema: Revista Internacional de Filosofía , 35(3): 201–218.
- Psillos, Stathis, 1995, “The Cognitive Interplay between Theories and Models: The Case of 19th Century Optics”, in Herfel et al. 1995: 105–133.
- Redhead, Michael, 1980, “Models in Physics”, The British Journal for the Philosophy of Science , 31(2): 145–163. doi:10.1093/bjps/31.2.145
- Reiss, Julian, 2003, “Causal Inference in the Abstract or Seven Myths about Thought Experiments”, in Causality: Metaphysics and Methods Research Project , Technical Report 03/02. London: London School of Economics.
- –––, 2006, “Social Capacities”, in Hartmann et al. 2006: 265–288.
- –––, 2012, “The Explanation Paradox”, Journal of Economic Methodology , 19(1): 43–62. doi:10.1080/1350178X.2012.661069
- Reutlinger, Alexander, 2017, “Do Renormalization Group Explanations Conform to the Commonality Strategy?”, Journal for General Philosophy of Science , 48(1): 143–150. doi:10.1007/s10838-016-9339-7
- Reutlinger, Alexander, Dominik Hangleiter, and Stephan Hartmann, 2018, “Understanding (with) Toy Models”, The British Journal for the Philosophy of Science , 69(4): 1069–1099. doi:10.1093/bjps/axx005
- Rice, Collin C., 2015, “Moving Beyond Causes: Optimality Models and Scientific Explanation”, Noûs , 49(3): 589–615. doi:10.1111/nous.12042
- –––, 2016, “Factive Scientific Understanding without Accurate Representation”, Biology & Philosophy , 31(1): 81–102. doi:10.1007/s10539-015-9510-2
- –––, 2018, “Idealized Models, Holistic Distortions, and Universality”, Synthese , 195(6): 2795–2819. doi:10.1007/s11229-017-1357-4
- –––, 2019, “Models Don’t Decompose That Way: A Holistic View of Idealized Models”, The British Journal for the Philosophy of Science , 70(1): 179–208. doi:10.1093/bjps/axx045
- Rosaler, Joshua, 2015, “Local Reduction in Physics”, Studies in History and Philosophy of Science Part B: Studies in History and Philosophy of Modern Physics , 50: 54–69. doi:10.1016/j.shpsb.2015.02.004
- Rueger, Alexander, 2005, “Perspectival Models and Theory Unification”, The British Journal for the Philosophy of Science , 56(3): 579–594. doi:10.1093/bjps/axi128
- Rueger, Alexander and David Sharp, 1998, “Idealization and Stability: A Perspective from Nonlinear Dynamics”, in Shanks 1998: 201–216.
- Saatsi, Juha, 2016, “Models, Idealisations, and Realism”, in Emiliano Ippoliti, Fabio Sterpetti, and Thomas Nickles (eds.), Models and Inferences in Science (Studies in Applied Philosophy, Epistemology and Rational Ethics 25), Cham: Springer International Publishing, pp. 173–189. doi:10.1007/978-3-319-28163-6_10
- Saatsi, Juha and Alexander Reutlinger, 2018, “Taking Reductionism to the Limit: How to Rebut the Antireductionist Argument from Infinite Limits”, Philosophy of Science , 85(3): 455–482. doi:10.1086/697735
- Salis, Fiora, forthcoming, “The New Fiction View of Models”, The British Journal for the Philosophy of Science , first online: 20 April 2019. doi:10.1093/bjps/axz015
- Salmon, Wesley C., 1984, Scientific Explanation and the Causal Structure of the World , Princeton, NJ: Princeton University Press.
- Schaffner, Kenneth F., 1969, “The Watson–Crick Model and Reductionism”, The British Journal for the Philosophy of Science , 20(4): 325–348. doi:10.1093/bjps/20.4.325
- Scheibe, Erhard, 1997, Die Reduktion physikalischer Theorien: Ein Beitrag zur Einheit der Physik, Teil I: Grundlagen und elementare Theorie , Berlin: Springer.
- –––, 1999, Die Reduktion physikalischer Theorien: Ein Beitrag zur Einheit der Physik, Teil II: Inkommensurabilität und Grenzfallreduktion , Berlin: Springer.
- –––, 2001, Between Rationalism and Empiricism: Selected Papers in the Philosophy of Physics , Brigitte Falkenburg (ed.), New York: Springer. doi:10.1007/978-1-4613-0183-7
- Shanks, Niall (ed.), 1998, Idealization in Contemporary Physics , Amsterdam: Rodopi.
- Shech, Elay, 2018, “Idealizations, Essential Self-Adjointness, and Minimal Model Explanation in the Aharonov–Bohm Effect”, Synthese , 195(11): 4839–4863. doi:10.1007/s11229-017-1428-6
- Sismondo, Sergio and Snait Gissis (eds.), 1999, Modeling and Simulation , Special Issue of Science in Context , 12(2).
- Sorensen, Roy A., 1992, Thought Experiments , New York: Oxford University Press. doi:10.1093/019512913X.001.0001
- Spector, Marshall, 1965, “Models and Theories”, The British Journal for the Philosophy of Science , 16(62): 121–142. doi:10.1093/bjps/XVI.62.121
- Staley, Kent W., 2004, The Evidence for the Top Quark: Objectivity and Bias in Collaborative Experimentation , Cambridge: Cambridge University Press.
- Sterrett, Susan G., 2006, “Models of Machines and Models of Phenomena”, International Studies in the Philosophy of Science , 20(1): 69–80. doi:10.1080/02698590600641024
- –––, forthcoming, “Scale Modeling”, in Diane Michelfelder and Neelke Doorn (eds.), Routledge Handbook of Philosophy of Engineering , Chapter 32. [ Sterrett forthcoming available online ]
- Strevens, Michael, 2004, “The Causal and Unification Approaches to Explanation Unified—Causally”, Noûs , 38(1): 154–176. doi:10.1111/j.1468-0068.2004.00466.x
- –––, 2008, Depth: An Account of Scientific Explanation , Cambridge, MA, and London: Harvard University Press.
- –––, 2013, Tychomancy: Inferring Probability from Causal Structure , Cambridge, MA, and London: Harvard University Press.
- Suárez, Mauricio, 2003, “Scientific Representation: Against Similarity and Isomorphism”, International Studies in the Philosophy of Science , 17(3): 225–244. doi:10.1080/0269859032000169442
- –––, 2004, “An Inferential Conception of Scientific Representation”, Philosophy of Science , 71(5): 767–779. doi:10.1086/421415
- ––– (ed.), 2009, Fictions in Science: Philosophical Essays on Modeling and Idealization , London: Routledge. doi:10.4324/9780203890103
- Sugden, Robert, 2000, “Credible Worlds: The Status of Theoretical Models in Economics”, Journal of Economic Methodology , 7(1): 1–31. doi:10.1080/135017800362220
- Sullivan, Emily and Kareem Khalifa, 2019, “Idealizations and Understanding: Much Ado About Nothing?”, Australasian Journal of Philosophy , 97(4): 673–689. doi:10.1080/00048402.2018.1564337
- Suppe, Frederick, 2000, “Theory Identity”, in William H. Newton-Smith (ed.), A Companion to the Philosophy of Science , Oxford: Wiley-Blackwell, pp. 525–527.
- Suppes, Patrick, 1960, “A Comparison of the Meaning and Uses of Models in Mathematics and the Empirical Sciences”, Synthese , 12(2–3): 287–301. Reprinted in Freudenthal 1961: 163–177, and in Suppes 1969: 10–23. doi:10.1007/BF00485107 doi:10.1007/978-94-010-3667-2_16
- –––, 1962, “Models of Data”, in Ernest Nagel, Patrick Suppes, and Alfred Tarski (eds.), Logic, Methodology and Philosophy of Science: Proceedings of the 1960 International Congress , Stanford, CA: Stanford University Press, pp. 252–261. Reprinted in Suppes 1969: 24–35.
- –––, 1969, Studies in the Methodology and Foundations of Science: Selected Papers from 1951 to 1969 , Dordrecht: Reidel.
- –––, 2007, “Statistical Concepts in Philosophy of Science”, Synthese , 154(3): 485–496. doi:10.1007/s11229-006-9122-0
- Swoyer, Chris, 1991, “Structural Representation and Surrogative Reasoning”, Synthese , 87(3): 449–508. doi:10.1007/BF00499820
- Tabor, Michael, 1989, Chaos and Integrability in Nonlinear Dynamics: An Introduction , New York: John Wiley.
- Teller, Paul, 2001, “Twilight of the Perfect Model”, Erkenntnis , 55(3): 393–415. doi:10.1023/A:1013349314515
- –––, 2002, “Critical Study: Nancy Cartwright’s The Dappled World: A Study of the Boundaries of Science ”, Noûs , 36(4): 699–725. doi:10.1111/1468-0068.t01-1-00408
- –––, 2009, “Fictions, Fictionalization, and Truth in Science”, in Suárez 2009: 235–247.
- –––, 2018, “Referential and Perspectival Realism”, Spontaneous Generations: A Journal for the History and Philosophy of Science , 9(1): 151–164. doi:10.4245/sponge.v9i1.26990
- Tešić, Marko, 2019, “Confirmation and the Generalized Nagel–Schaffner Model of Reduction: A Bayesian Analysis”, Synthese , 196(3): 1097–1129. doi:10.1007/s11229-017-1501-1
- Thomasson, Amie L., 1999, Fiction and Metaphysics , New York: Cambridge University Press. doi:10.1017/CBO9780511527463
- –––, 2020, “If Models Were Fictions, Then What Would They Be?”, in Levy and Godfrey-Smith 2020: 51–74.
- Thomson-Jones, Martin, 2006, “Models and the Semantic View”, Philosophy of Science , 73(5): 524–535. doi:10.1086/518322
- –––, 2020, “Realism about Missing Systems”, in Levy and Godfrey-Smith 2020: 75–101.
- Toon, Adam, 2012, Models as Make-Believe: Imagination, Fiction and Scientific Representation , Basingstoke: Palgrave Macmillan.
- Trout, J. D., 2002, “Scientific Explanation and the Sense of Understanding”, Philosophy of Science , 69(2): 212–233. doi:10.1086/341050
- van Fraassen, Bas C., 1989, Laws and Symmetry , Oxford: Oxford University Press. doi:10.1093/0198248601.001.0001
- Walton, Kendall L., 1990, Mimesis as Make-Believe: On the Foundations of the Representational Arts , Cambridge, MA: Harvard University Press.
- Weisberg, Michael, 2007, “Three Kinds of Idealization”, Journal of Philosophy , 104(12): 639–659. doi:10.5840/jphil20071041240
- –––, 2013, Simulation and Similarity: Using Models to Understand the World , Oxford: Oxford University Press. doi:10.1093/acprof:oso/9780199933662.001.0001
- Weisberg, Michael and Ryan Muldoon, 2009, “Epistemic Landscapes and the Division of Cognitive Labor”, Philosophy of Science , 76(2): 225–252. doi:10.1086/644786
- Wimsatt, William, 1987, “False Models as Means to Truer Theories”, in Matthew Nitecki and Antoni Hoffman (eds.), Neutral Models in Biology , Oxford: Oxford University Press, pp. 23–55.
- –––, 2007, Re-Engineering Philosophy for Limited Beings: Piecewise Approximations to Reality , Cambridge, MA: Harvard University Press.
- Woodward, James, 2003, Making Things Happen: A Theory of Causal Explanation , Oxford: Oxford University Press. doi:10.1093/0195155270.001.0001
- Woody, Andrea I., 2004, “More Telltale Signs: What Attention to Representation Reveals about Scientific Explanation”, Philosophy of Science , 71(5): 780–793. doi:10.1086/421416
- Zollman, Kevin J. S., 2007, “The Communication Structure of Epistemic Communities”, Philosophy of Science , 74(5): 574–587. doi:10.1086/525605
How to cite this entry . Preview the PDF version of this entry at the Friends of the SEP Society . Look up topics and thinkers related to this entry at the Internet Philosophy Ontology Project (InPhO). Enhanced bibliography for this entry at PhilPapers , with links to its database.
- Internet Encyclopedia of Philosophy article on models
- Bibliography (1450–2008), Mueller Science
- Interactive models from various sciences (Phet, University of Colorado, Boulder)
- Models of the global climate (Climate.gov)
- Double-helix model of DNA (Proteopedia)
- A Biologist’s Guide to Mathematical Modeling in Ecology and Evolution (Sarah Otto and Troy Day)
- Lotka–Volterra model (analyticphysics.com)
- Schelling’s Model of Segregation (Frank McCown)
- Modeling Commons (NetLogo)
- Social and Economic Networks: Models and Analysis (Stanford Online course)
- Neural Network Models (TensorFlow)
analogy and analogical reasoning | laws of nature | science: unity of | scientific explanation | scientific realism | scientific representation | scientific theories: structure of | simulations in science | thought experiments
Acknowledgments
We would like to thank Joe Dewhurst, James Nguyen, Alexander Reutlinger, Collin Rice, Dunja Šešelja, and Paul Teller for helpful comments on the drafts of the revised version in 2019. When writing the original version back in 2006 we benefitted from comments and suggestions by Nancy Cartwright, Paul Humphreys, Julian Reiss, Elliott Sober, Chris Swoyer, and Paul Teller.
Copyright © 2020 by Roman Frigg < r . p . frigg @ lse . ac . uk > Stephan Hartmann < stephan . hartmann @ lrz . uni-muenchen . de >
- Accessibility
Support SEP
Mirror sites.
View this site from another server:
- Info about mirror sites
The Stanford Encyclopedia of Philosophy is copyright © 2023 by The Metaphysics Research Lab , Department of Philosophy, Stanford University
Library of Congress Catalog Data: ISSN 1095-5054
NOTIFICATIONS
Scientific modelling.
- + Create new collection
In science, a model is a representation of an idea, an object or even a process or a system that is used to describe and explain phenomena that cannot be experienced directly. Models are central to what scientists do, both in their research as well as when communicating their explanations.
Models are a mentally visual way of linking theory with experiment, and they guide research by being simplified representations of an imagined reality that enable predictions to be developed and tested by experiment.
Why scientists use models
Models have a variety of uses – from providing a way of explaining complex data to presenting as a hypothesis. There may be more than one model proposed by scientists to explain or predict what might happen in particular circumstances. Often scientists will argue about the ‘rightness’ of their model, and in the process, the model will evolve or be rejected. Consequently, models are central to the process of knowledge-building in science and demonstrate how science knowledge is tentative.
Think about a model showing the Earth – a globe. Until 2005, globes were always an artist’s representation of what we thought the planet looked like. (In 2005, the first globe using satellite pictures from NASA was produced.) The first known globe to be made (in 150BC) was not very accurate. The globe was constructed in Greece so perhaps only showed a small amount of land in Europe, and it wouldn’t have had Australia, China or New Zealand on it! As the amount of knowledge has built up over hundreds of years, the model has improved until, by the time a globe made from real images was produced, there was no noticeable difference between the representation and the real thing.
Building a model
Scientists start with a small amount of data and build up a better and better representation of the phenomena they are explaining or using for prediction as time goes on. These days, many models are likely to be mathematical and are run on computers, rather than being a visual representation, but the principle is the same.
Using models for predicting
In some situations, models are developed by scientists to try and predict things. The best examples are climate models and climate change. Humans don’t know the full effect they are having on the planet, but we do know a lot about carbon cycles, water cycles and weather. Using this information and an understanding of how these cycles interact, scientists are trying to figure out what might happen. Models further rely on the work of scientists to collect quality data to feed into the models. To learn more about work to collate data for models, look at the Argo Project and the work being done to collect large-scale temperature and salinity data to understand what role the ocean plays in climate and climate change.
For example, they can use data to predict what the climate might be like in 20 years if we keep producing carbon dioxide at current rates – what might happen if we produce more carbon dioxide and what would happen if we produce less. The results are used to inform politicians about what could happen to the climate and what can be changed.
Another common use of models is in management of fisheries. Fishing and selling fish to export markets is an important industry for many countries including New Zealand (worth $1.4 billion dollars in 2009). However, overfishing is a real risk and can cause fishing grounds to collapse. Scientists use information about fish life cycles, breeding patterns, weather, coastal currents and habitats to predict how many fish can be taken from a particular area before the population is reduced below the point where it can’t recover.
Models can also be used when field experiments are too expensive or dangerous, such as models used to predict how fire spreads in road tunnels and how a fire might develop in a building .
How do we know if a model works?
Models are often used to make very important decisions, for example, reducing the amount of fish that can be taken from an area might send a company out of business or prevent a fisher from having a career that has been in their family for generations.
The costs associated with combating climate change are almost unimaginable, so it’s important that the models are right, but often it is a case of using the best information available to date. Models need to be continually tested to see if the data used provides useful information. A question scientists can ask of a model is: Does it fit the data that we know?
For climate change, this is a bit difficult. It might fit what we know now, but do we know enough? One way to test a climate change model is to run it backwards. Can it accurately predict what has already happened? Scientists can measure what has happened in the past, so if the model fits the data, it is thought to be a little more trustworthy. If it doesn’t fit, it’s time to do some more work.
This process of comparing model predictions with observable data is known as ‘ground-truthing’. For fisheries management, ground-truthing involves going out and taking samples of fish at different areas. If there are not as many fish in the region as the model predicts, it is time to do some more work.
Learn more about ground truthing in Satellites measure sea ice thickness . Here scientists are validating sateliite data on ice thickness in Antarctica so the data can be used to model how the Earth’s climate, sea temperature and sea levels may be changing.
Nature of science
Models have always been important in science and continue to be used to test hypotheses and predict information. Often they are not accurate because the scientists may not have all the data. It is important that scientists test their models and be willing to improve them as new data comes to light. Model-building can take time – an accurate globe took more than 2,000 years to create – hopefully, an accurate model for climate change will take significantly less time.
Useful links
An example of a scientific model on YouTube.
See our newsletters here .
Would you like to take a short survey?
This survey will open in a new tab and you can fill it out after your visit to the site.
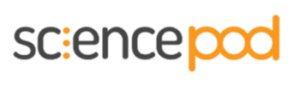
Understanding Scientific Models: A Comprehensive Guide
This article presents a comprehensive guide to understanding scientific models, indispensable tools in scientific research and technological innovation.
It explores their types, uses, limitations, and evolution, shedding light on their critical role in diverse fields.
By delving into the intricacies of these models, this guide aims to foster a deeper understanding of their contribution to scientific discovery and knowledge progression.
Key Takeaways
- Scientific models are representations of systems that help explain and visualise scientific processes and natural phenomena.
- Models can take different forms, such as representational, descriptive, spatial, mathematical, and computational models.
- Scientific models have limitations and may not capture all the details or accurately represent reality.
- Models can change over time due to new discoveries and the need for updated or revised information.
The Fundamentals of Scientific Models
The fundamentals of scientific models are rooted in their ability to provide a physical, mathematical, or conceptual representation of complex systems, thereby aiding in the explanation, visualization, and prediction of scientific processes and natural phenomena. These models form the cornerstone of scientific inquiry, enabling a more comprehensive understanding of the intricate workings of the natural world.
A scientific model serves as a simplified approximation of a system, offering valuable insights into its various components and their interactions. This representation helps scientists to test theories, formulate predictions, and facilitate communication within the scientific community. Despite their inherent limitations and assumptions, science models are paramount to the progression of scientific knowledge, providing a robust framework for investigation and discovery.
Diverse Types of Scientific Models
In the realm of scientific inquiry, diverse types of models have been developed over time to assist in the understanding and prediction of complex systems and phenomena. These models include representational, descriptive, spatial, mathematical, and computational models.
Representational models use shapes and analogies to describe a system. They provide a visual representation that helps researchers and scientists understand the structure and behavior of a system.
Descriptive models, on the other hand, leverage language to explain processes. These models use written or verbal descriptions to capture the essence of a system and its dynamics.
Spatial models focus on representing systems through three-dimensional relationships. They use spatial arrangements and configurations to depict how different components of a system interact with one another.
Mathematical models employ known mathematical relationships to predict outcomes of systems. These models use equations and formulas to describe the behavior of a system and make predictions based on mathematical principles.
Computational models require computer power to execute complex calculations for their predictive capabilities. These models use algorithms and simulations to simulate the behavior of a system and predict its future states.
Despite their limitations, such as oversimplification of systems or dependence on computational power, these models are invaluable tools in advancing scientific knowledge and technology. They allow scientists to test hypotheses, make predictions, and gain insights into complex systems that would otherwise be difficult or impossible to understand.
The Role of Limitations in Scientific Models
Despite their invaluable contributions to scientific understanding and prediction, models often have inherent limitations, such as simplification of complex systems and dependence on computational power, which must continuously be scrutinised and adapted over time to ensure their ongoing validity and utility.
Models are, by nature, a reduction of reality, making certain assumptions for the sake of computational and conceptual efficiency. Yet, this can pose issues in capturing the full complexity of the system being modeled.
Additionally, the accuracy of models is often tied to the computational power available, creating potential constraints. However, these limitations do not detract from the utility of models. Instead, they serve as a reminder of the need for continuous refinement, testing, and adaptation in response to new data and technological advancements.
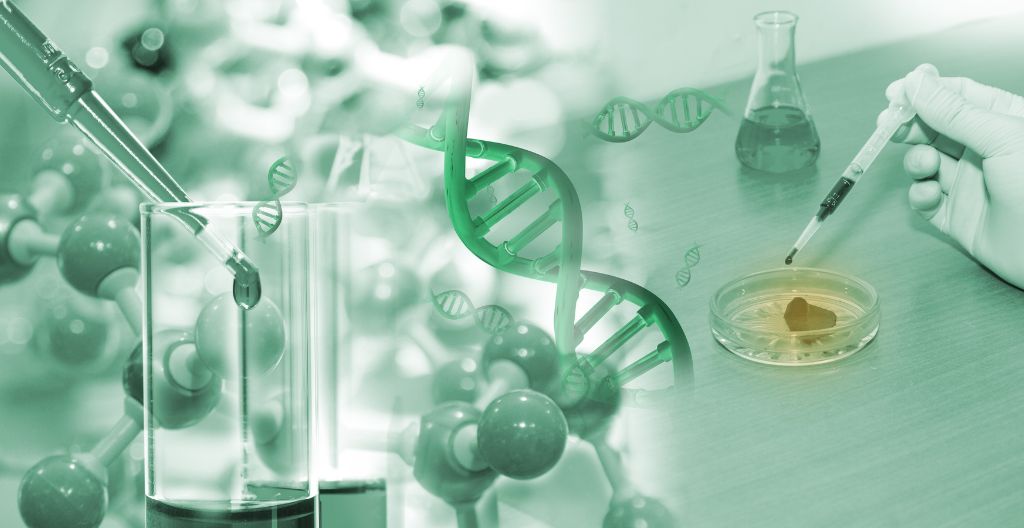
The Evolution and Adaptation of Scientific Models
Evolving and adapting over time, scientific models continue to serve as crucial tools for understanding complex systems, but they also highlight the need for ongoing scrutiny and modification in light of new discoveries and technological advancements. The evolution of scientific models is not a linear process, but rather, a dynamic interplay of hypothesis generation, testing, and refinement. This iterative process allows for the accommodation of new data, ensuring that models remain relevant and accurate. However, these adaptations are not without challenges, including the need for increased computational power and the complexity of integrating diverse datasets.
Practical Applications of Scientific Models in Various Fields
Scientific models, which are used extensively in numerous fields such as physics, biology, and climate science, serve not only as theoretical constructs but also as practical tools for prediction and experimentation, thus playing a critical role in advancing our understanding of the world.
In medicine, models can simulate human physiology and predict the effects of treatments. In environmental science, they are used to forecast climate trends and assess ecological impacts. Physics utilises models to explain fundamental properties of the universe. Similarly, in economics, models can illustrate complex market dynamics and guide policy decisions.
Despite inherent limitations and uncertainties, the practical applications of scientific models offer invaluable perspectives, enhance predictive capacities, and facilitate innovative solutions, thus driving progress across diverse fields.
Sign up for our Publishing Newsletter and start delivering creative, concise content
Frequently asked questions, how are scientific models used in the decision-making process in policy-making and environmental management.
Scientific models play a vital role in policy-making and environmental management by providing evidence-based insights. They predict outcomes, quantify risks, and help in scenario planning, thus informing decisions and promoting sustainable practices.
What Is the Role of Scientific Modeling in Artificial Intelligence and Machine Learning?
Scientific modeling plays a crucial role in artificial intelligence and machine learning by providing a structured method for algorithm development, data interpretation, prediction making, and decision-making processes, enhancing accuracy, efficiency, and predictive capabilities.
How Does the Development of Technology Impact the Creation and Use of Scientific Models?
The advent of technology significantly impacts the creation and use of scientific models by enabling more complex simulations, facilitating data-driven modeling, and allowing for collaborative efforts through open-source platforms for greater accuracy and understanding.
How Do Scientific Models Contribute to the Advancement of Medical and Health Research?
Scientific models significantly contribute to medical and health research by providing a simplified representation of complex biological processes. These models facilitate the prediction and understanding of disease progression and the efficacy of potential treatments.
Can Scientific Models Accurately Predict Natural Disasters and if So, How Is This Achieved?
Scientific models can predict natural disasters with varying degrees of accuracy by using data and mathematical algorithms. These models analyze patterns, assess risk factors, and simulate potential scenarios to forecast disaster occurrences and impacts.
In essence, scientific models offer invaluable tools for understanding complex phenomena, despite their inherent limitations. Their adaptability and evolution over time reflect the dynamic nature of scientific discovery.
Their applications span across multiple domains, catalyzing technological advancements and fostering innovation. Therefore, a comprehensive understanding of these models is integral to scientific processes and contributes significantly to the broader trajectory of scientific research and understanding.
Discover the ScioWire research newsfeed: summarised scientific knowledge ready to digest.
- Publications
- Conferences & Events
- Professional Learning
- Science Standards
- Awards & Competitions
- Instructional Materials
- Free Resources
- American Rescue Plan
- For Preservice Teachers
- NCCSTS Case Collection
- Partner Jobs in Education
- Interactive eBooks+
- Digital Catalog
- Regional Product Representatives
- e-Newsletters
- Bestselling Books
- Latest Books
- Popular Book Series
- Prospective Authors
- Web Seminars
- Exhibits & Sponsorship
- Conference Reviewers
- National Conference • Denver 24
- Leaders Institute 2024
- National Conference • New Orleans 24
- Submit a Proposal
- Latest Resources
- Professional Learning Units & Courses
- For Districts
- Online Course Providers
- Schools & Districts
- College Professors & Students
- The Standards
- Teachers and Admin
- eCYBERMISSION
- Toshiba/NSTA ExploraVision
- Junior Science & Humanities Symposium
- Teaching Awards
- Climate Change
- Earth & Space Science
- New Science Teachers
- Early Childhood
- Middle School
- High School
- Postsecondary
- Informal Education
- Journal Articles
- Lesson Plans
- e-newsletters
- Science & Children
- Science Scope
- The Science Teacher
- Journal of College Sci. Teaching
- Connected Science Learning
- NSTA Reports
- Next-Gen Navigator
- Science Update
- Teacher Tip Tuesday
- Trans. Sci. Learning
MyNSTA Community
- My Collections
Using Models to Teach Science
The Science Teacher—November/December 2019 (Volume 87, Issue 4)
By Byung-Yeol Park, Laura Rodriguez, and Todd Campbell
Share Start a Discussion
The Next Generation Science Standards ( NGSS ) focus on students’ application of sense-making and problem-solving skills to deepen their understanding about naturally occurring phenomena (NGSS Lead States 2013). The NGSS identifies three dimensions: disciplinary core ideas (DCIs), science and engineering practices (SEPs), and crosscutting concepts (CCCs) for teachers to consider as they design challenging and worthwhile instruction.
The role of SEPs as stated in the Framework for K–12 Science Education is to “help students understand how scientific knowledge develops, … and gives them an appreciation of the wide range of approaches that are used to investigate, model, and explain the world” (NRC 2012). Among the SEPs, Developing and Using Models offers students a sense-making tool to reason about how real-world events or system works. As students engage with peers in developing models, they can move from using models to unpack their initial ideas to explain phenomena, to using evidence from observations, readings, and experiments to revise or refine their models. Through these processes, students can visualize and understand complex systems as scientists do when they explore the natural world.
Cultivating students’ scientific knowledge and developing their capability in scientific inquiry depends on a teacher’s ability to use existing resources to design rich learning opportunities (Brown and Edelson 2003). Designing such experiences is not easy, and it is particularly challenging for new teachers who have little experience making decisions about the best way to develop specific knowledge and practices, especially in relation to choosing appropriate resources for engaging students in activity (Kaufmann et al. 2002). In addition, a limited amount of guidance to support teachers in the practical aspects of combining the three dimensions currently exists (i.e. DCIs, SEPs, and CCCs) in K–12 science instruction (McFadden and Roehrig 2017).
More resources and preparation are needed to support teachers in using the three dimensions effectively in teaching science (Krajcik 2015). A special issue of The Science Teacher in September 2013 focused on the theme “Developing and Using Models,” and other supportive guides have also been released. However, engaging students in developing and using models in science classrooms is still difficult given how modeling has only recently been emphasized in science classrooms as an important practice for knowledge development (Campbell and Oh 2015).
Given this, the following four questions can be used to guide our use of models in teaching science:
- What is the purpose of a model?
- How can we develop a model?
- How can we use models in teaching science?
- What should we consider when teaching with models?
Each of these is considered along with relevant examples from high school students’ modeling activities.
What is the purpose of a model?
The purpose of a scientific model is for “describing, explaining, and predicting natural phenomena and communicating scientific ideas to others” (Oh and Oh 2011). As an example, a model of how the formation of clouds may involve the condensation of water vapor with a connection to air movement and temperature is shown in Figure 1.
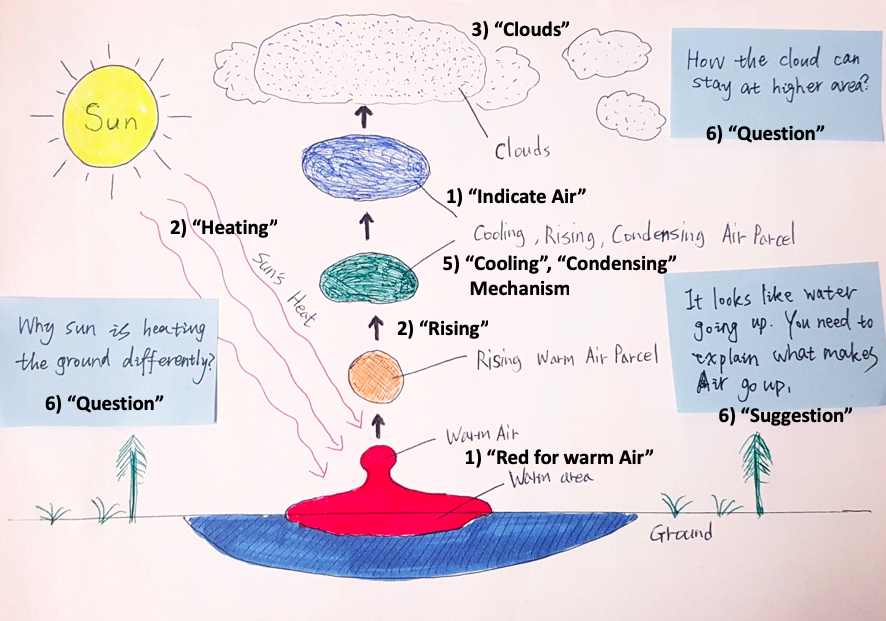
Example model for the formation of a cloud.
To explain how clouds are made in this model, students use different colors and circle sizes to show changes as clouds form. Arrows indicate the process of changing air conditions. Words such as “Sun’s Heat,” “Cooling,” “Rising,” and “Condensing” help the reader understand scientific mechanisms that can be difficult to explain with just figures or lines. Generally, models involving scientific processes and mechanisms tend to have many causal relations with directions labeled in between the objects.
We can use this kind of model to help students understand scientific processes and mechanisms in natural phenomena. Moreover, we can use a model to help students predict what kind of cloud would be around their location at any given time. For this, creating new models including more specific information about their environment and air conditions can be useful in making predictions.
How can we develop a model?
While many things can be described as a model, from representations to objects, developing and using models as an SEP in the NGSS focuses on how models serve as tools for reasoning. More specifically, developing and using models aligned to the NGSS is most concerned with “the process by which scientists [and students] represent ideas about the natural world to each other, and then collaboratively make changes to these representations over time in response to new evidence and understandings” (Ambitious Science Teaching 2015). Given this, to use models in learning science, teachers need to provide students with opportunities to understand what a model is, how to develop a model, and the role of symbols and other features of models can play in supporting their reasoning.
Scientists develop models based on information they collect both from their initial ideas or things they have learned previously, investigations, and in connection to ideas they gather from background research of the literature (Passmore and Svoboda 2012). In some cases, they revise models developed by others to adapt to new information or new problems. The explanatory power of a model depends on how the author decides to condense and represent information.
Consider a model that was created to explain the interaction between a watershed and its environment containing many symbols (e.g., tree, grass, water, fish, building, car, and load) with different colors, and lines, arrows, words, short sentences, and numbers showing the relationship between the components (see Figure 2). For example, the figure of smoke and short expression of “Smoke affects water life. ex. Fish” helps indicate the impact of human activity on aquatic animals. The expression of “Cement can’t absorb water. Pollutants from run-off goes into river” explains how pollutants impact water quality and why it happens. Numbers, like “Avg. Turbidity 67%,” and “pH 7” represent the important conditions of objects within the model (i.e., water). Lines and arrows explain the connections between the objects in the model. In the end, it can be seen that there are many ways in which students can draw on a range of tools in their attempts to explain what they observed with models.
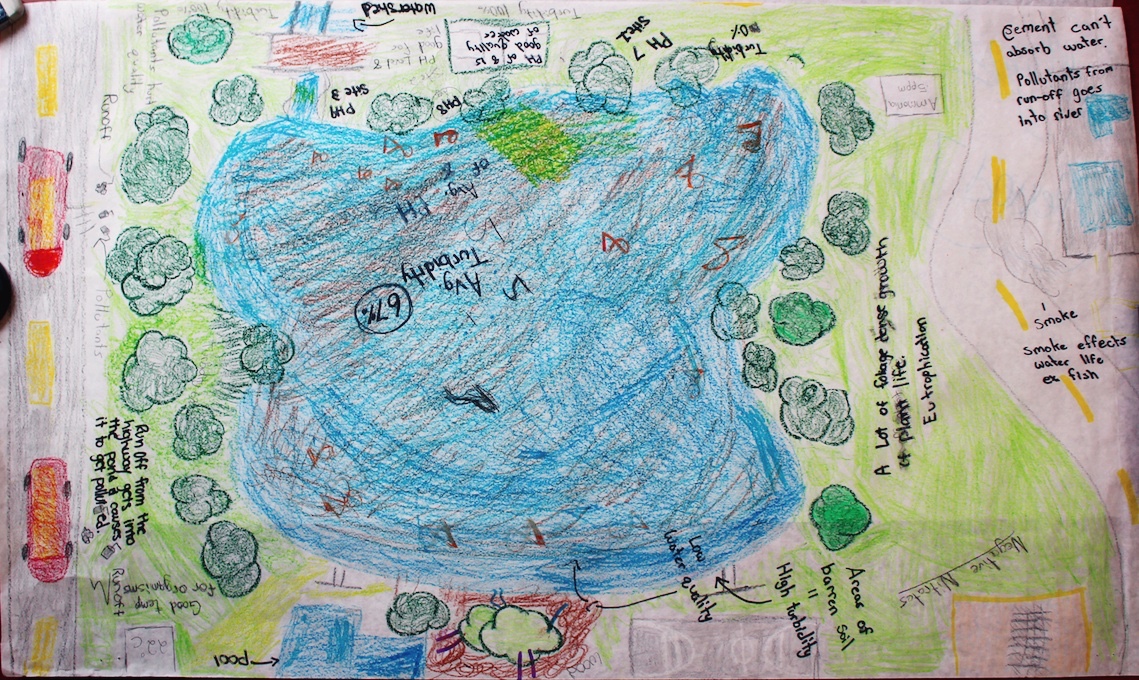
Example model watershed and environment.
Learning to make decisions about how to use these tools in one’s model is challenging for students, especially those who may never have made a model. First, a class or a small group of students need to agree on what arrows mean, and on their shape and directionality. Then one must consider the range of symbols one can use. For example, in Figure 1, students use different colors to represent air temperature. Consider some of the following representational options that can be used in models:
- Lines can be used to suggest connections. Different colors or thickness are helpful to show emphasis, and modified lines; such as, dotted or curved lines; can be useful to differentiate types of connections.
- Arrows can be used to indicate a causal relationship and give direction (one-way arrows are good to indicate cause and effect, and both ways or more than two-way arrows can illustrate more complex relationships).
- Symbols and figures can be used to show concepts (simple symbols or figures can afford more explanatory power).
- Numbers can be used to indicate quantity or level.
- Words and sentences can be used to describe mechanisms and explain processes. Clear sentences for the mechanisms or processes can help readers interpret the model.
- Sticky-notes can also be used to suggest and negotiate more scientific ideas. Notes from others are good for upgrading models.
How can we use models in teaching science?
Modeling does not end after developing the first model; constructed models should be tested, evaluated, and revised. Through this process, students will be able to learn how to use evidence in order to support their ideas with other sense-making practices (e.g., planning and carrying out investigations). Campbell, Gray, and Fazio (Forthcoming), in their work focused on Model-Based Inquiry (MBI), draw on principles from Ambitious Science Teaching (Windschitl, Thompson, and Braaten 2018), to suggest four stages that we can follow as we use modeling in teaching.
The first stage is planning for engagement with important science ideas . Teachers unpack standards to identify learning targets, an anchoring phenomenon, and a driving question that orients students to some interesting aspect of the anchoring phenomenon. We also construct final target models and explanations that teachers can use as guides for planning and supporting students across a unit. Target models and explanations are not shared with students; instead, they are behind-the-scenes planning tools and maps for helping the teacher think about productive ways students might engage in modeling an anchoring phenomenon.
The second stage is eliciting student ideas . Teachers introduce the anchoring phenomenon and driving question to the students at the beginning of this stage. Students develop initial hypotheses and initial models based on observations and shared ideas.
The third stage is supporting ongoing changes in thinking . Students, in this stage, will have opportunities to reconstruct, test, evaluate, and revise their initial models based on the results of scientific inquiry (e.g., observations, experiments, or discussions) and engagement with many other science practices (e.g., Engaging in Argument From Evidence). One method to keep track of changes in thinking as students engage in investigations is the use of a summary table , an ongoing record the whole class adds to after engaging in investigations. It can detail patterns recognized, explanations for these patterns, and how these explanations can help the class think about explaining the anchoring phenomenon.
Finally, the fourth stage is pressing for evidence-based explanations . In this last stage, students finalize their models by considering all they have learned across the unit through engaging in investigations, activities, opportunities to read relevant texts, and working collectively as a class to build general agreement about their models.
What should we consider when teaching with models?
Many teachers say that the most challenging part of teaching with models is assessment. There are numerous ways one might consider assessing instruction where using and revising models is central. One strategy is to ask students to draw from their groups’ final model, using the summary table, to develop individual evidence-based explanations. In this way, students can work with peers throughout the unit to engage in SEPs in developing and refining models, before they are asked to develop individual evidence-based explanations.
In the evidence-based explanation, students are asked to include the story of what happened when considering the driving question, important science ideas (i.e., DCIs & CCCs) necessary to explain mechanistically what happened, and evidence of how we know what we know about each important science idea that relates to the story. In the end, this evidence-based explanation can be assessed with a rubric similar to the one created and shared from the Model-Based Inquiry website (See “On the web”).
Some teachers report that it is difficult to align their instruction with the NGSS , even though they have extensive teaching experience in high school. As we begin to explore how to integrate developing and using models in our instruction, the four questions posed here might provide helpful stepping stones for building effective science lessons. Additionally, the resources listed under “On the web” will give more insight into important considerations related to using models in the science classroom.
Byung-Yeol Park ( [email protected] ) is a PhD candidate in the Department of Curriculum and Instruction at the University of Connecticut. Laura Rodriguez is an assistant professor of science education at Eastern Connecticut State University, Willimantic, Connecticut. Todd Campbell is a professor of science education at the University of Connecticut, Storrs, Connecticut.
Rubric for student’s evidence-based explanations :
“ Models and Modeling: An Introduction ” from Ambitious Science Teaching group
“ Tools for Ambitious Science Teaching ” from Ambitious Science Teaching group
Model Based Inquiry (MBI)
Ambitious Science Teaching. 2015. Models and Modeling: An Introduction . http://ambitiousscienceteaching.org/wp-content/uploads/2014/09/Models-and-Modeling-An-Introduction1.pdf
Brown, M., and D. Edelson. 2003. Teaching as design: Can we better understand the ways in which teachers use materials so we can better design materials to support their changes in practice? Evanston, IL: The Center for Learning Technologies in Urban Schools.
Campbell, T., and P.S. Oh. 2015. Engaging students in modeling as an epistemic practice of science: An introduction to the special issue. Journal of Science Education and Technology, 24 (2–3): 125–131.
Campbell, T., R. Gray, and X. Fazio. Forthcoming. Representing scientific activity: Affordances and constraints of central design and enactment features of a model-based inquiry unit. School Science and Mathematics.
Kaufmann, D., S.M. Johnson, S.M. Kardos, E. Liu, and H.G. Peske. 2002. “Lost at sea”: New teachers’ experiences with curriculum and assessment. Teachers College Record 104 (2): 273–300.
Krajcik, J. 2015. Three-dimensional instruction: Using a new type of teaching in the science classroom. Science and Children 53 (3): 6–8.
McFadden, J.R., and G.H. Roehrig. 2017. Exploring teacher design team endeavors while creating an elementary-focused STEM-integrated curriculum. International Journal of STEM Education 4 (1): 1–22.
National Research Council. 2012. A framework for K–12 science education: Practices, crosscutting concepts, and core ideas . Washington, DC: National Academies Press.
NGSS Lead States. 2013. Next generation science standards: For states, by states . Washington, DC: National Academies Press.
Oh, P.S., and S.J. Oh. 2011. What teachers of science need to know about models: An overview. International Journal of Science Education 33 (8): 1109–1130.
Passmore, C.M., and J. Svoboda. 2012. Exploring opportunities for argumentation in modelling classrooms. International Journal of Science Education 34 (10): 1535–1554.
Windschitl, M., J. Thompson, and M. Braaten. 2018. Ambitious science teaching. Cambridge, MA: Harvard University Press.
Environmental Science NGSS Teaching Strategies High School
You may also like
Reports Article
Web Seminar
Join us on Tuesday, June 4, 2024, from 7:00 PM to 8:30 PM ET, to learn about the free lesson plans and storyline units designed for high school studen...

O/L 2021 Science – New Model Paper Free Download
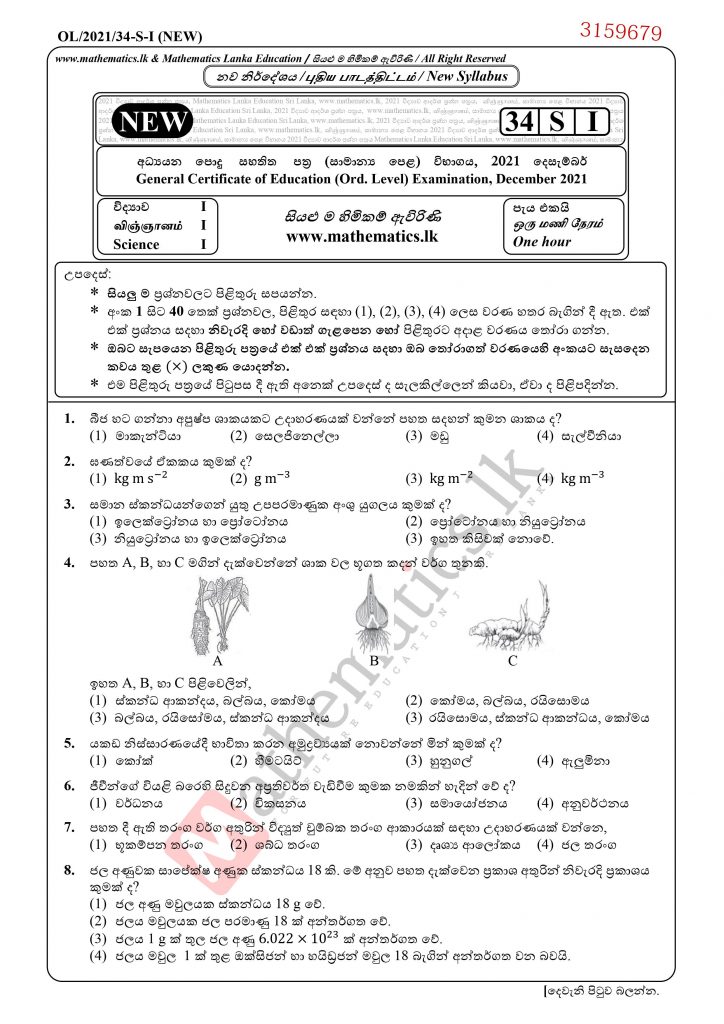
You can now download the O/L 2021 Science Question Paper here. For the first time, our website is releasing a Science Model Question Paper for the GCE Ordinary Level Examination this year.
We believe that we have been able to fill the huge gap that existed in our country so far. Because, until this year, students did not get a chance to get a quality built science model question paper. So we decided to give it to the students from this year.
We have prepared this 2021 O / L Science question paper according to the new syllabus. Also the previous exam question papers and prototype question papers were used to make this question paper.
How do we get the marking scheme for these 2021 O/L science model papers?
Follow the steps below to get the marking scheme of these question papers for you.
Enter your Name, School, and Address and type as “2021 Mathematics.lk Model Paper” and send an SMS or WhatsApp message to 071 723 73 93 .
Enter your Name, School and Address, and type as “2021 Mathematics.lk Model Paper” and send an email to [email protected]
Method 02 for getting 2021 O/L marking schemes
You can easily join our WhatsApp Groups & Telegram Groups . You can scan below QR Codes and join our educational social media groups. Therefore please follow these instructions.
To join our WhatsApp Group
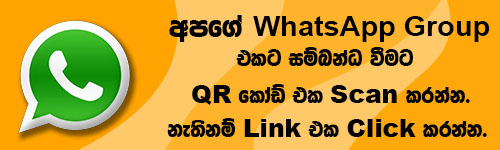
WhatsApp Group Link – https://chat.whatsapp.com/KJY7oc5y8KMBhl9wEGSrAv
To join our Telegram Group
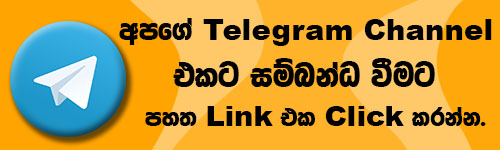
Telegram Channel Link – https://t.me/OLevelMathematicsOnlineClass
Introduction to new 2021/2022 science model question paper
This YouTube video gives you an idea of the 2021 and 2022 Mathematics and Science Model Question Papers. It also includes an explanation of the question pattern and the structure of the question paper.
What are the types of questions contained in this O/L 2021 Science paper?
This question paper contains 40 questions related to the science first paper. Also, all 40 of these questions in this questionnaire are newly created questions. Therefore, it will provide an opportunity for children studying science and technology to learn in a new dimension.
There is an acknowledgment that science is a somewhat difficult subject among students in GCE Ordinary Level subjects. We are preparing to dispel that misconception. You may be wondering how!
This question paper help to cover every unit in the school science textbook. Because we used the school textbook and O/L Science past papers to design this model paper. Then the students will have the opportunity to memorize the subject again and again.
After writing the answer to this question paper you can check if your answers are correct. Therefore You can buy the answer sheet from the above methods.
If you want to download science past paper, Please read below article.
https://www.mathematics.lk/download-science-past-question-papers/
Similar Posts
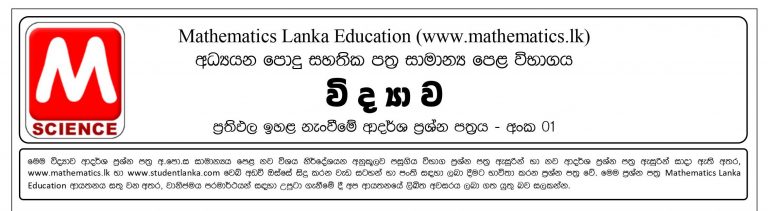
Science Model Paper 01 | Sinhala Medium O/L Exam
Click here to share on social media.The science curriculum has become a difficult subject for many children. Due to this,…
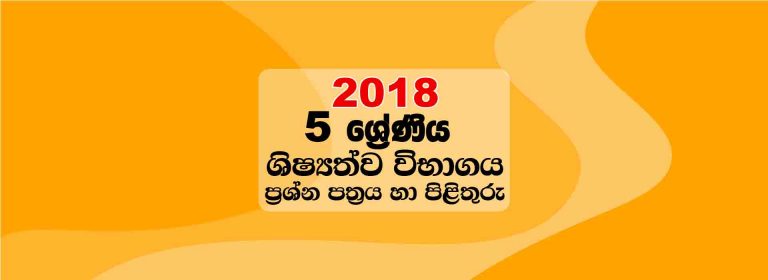
2018 Scholarship Paper | Grade 5 Exam Paper & Answer
Click here to share on social media.Here you can download the 2018 Scholarship Paper. The scholarship exam is a challenging…
Download Advanced Level A/L Physics Paper
Click here to share on social media.A/L Physics paper is a highly competitive subject in Advanced Level. Therefore, if students…
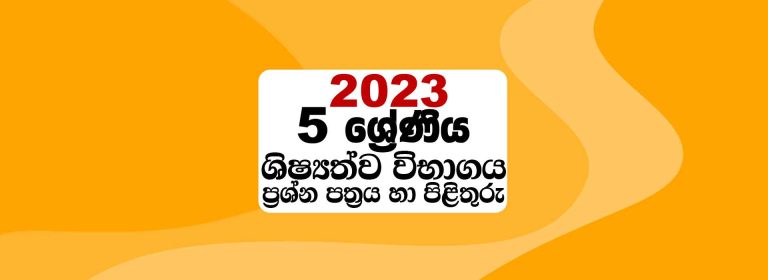
2023 Scholarship Paper | Grade 5 Exam Past Paper & Answer (Sinhala Medium)
Click here to share on social media.Here you can download the Sinhala Medium 2023 Scholarship Paper. The scholarship exam is…
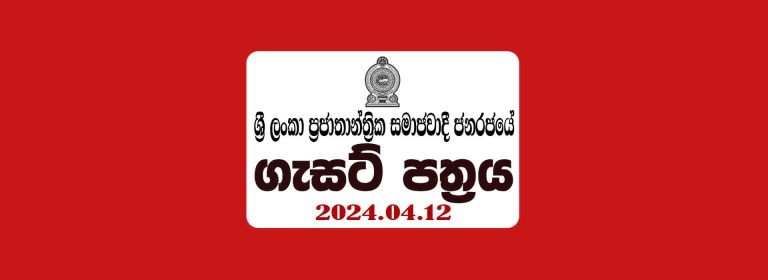
Government Gazette 2024 April 12 – Gazette Sri Lanka Download
Click here to share on social media.Here you can download the Government Gazette 2024 April 12. Generally, several Government Gazettes…
Practical Examination In Western Music – 2019
Click here to share on social media.Examination department called applications for practical Examination In Western Music in 2019. As a…
Leave a Reply Cancel reply
Your email address will not be published. Required fields are marked *
Advertisement
Models in science and in science education: an introduction
- Published: 18 May 2007
- Volume 16 , pages 647–652, ( 2007 )
Cite this article
- Michael R. Matthews 1
1527 Accesses
53 Citations
Explore all metrics
This collection of papers contributes to the tradition of model-related research in education, and it does so in both the fields of pedagogy and epistemology. Hopefully the papers will deepen understanding and move the research field forward. The ubiquity of models in the history and current practice of science is widely recognised. In the past half-century historians and philosophers of science have devoted considerable time to documenting and understanding the role of models in science and social science. A minority of studies have engaged with the central epistemic question of the truth-status of models, and of how models relate, or do not relate, to the real-world processes that they are putatively representing. In Education research there has been a rich vein of such research investigating the use of models as aids to memory, as ways of bridging between the experience and understanding of learners and the more abstract theories of science; and there has been a lot of research on the efficacy of children’s own experiences of model construction and testing. Attention to the epistemological side of model construction, functioning and testing is of special current importance because of the unprecedented importance being given to ‘Nature of Science’ objectives in science curricula around the world.
This is a preview of subscription content, log in via an institution to check access.
Access this article
Price includes VAT (Russian Federation)
Instant access to the full article PDF.
Rent this article via DeepDyve
Institutional subscriptions
Similar content being viewed by others
Semantic Views on Models: An Appraisal for Science Education
Models in Science and in Learning Science: Focusing Scientific Practice on Sense-making
Nature of science in the science curriculum: origin, development, implications and shifting emphases.
Bailer-Jones DM (2002) Models, metaphors and analogies. In: Machamer P, Silberstein M (eds) The Blackwell guide to the philosophy of science. Blackwell Publishers, Malden, MA, pp 108–127
Google Scholar
Barnes J (ed) (1984) The complete works of Aristotle, two volumes. Princeton University Press, Princeton, NJ
Black M (1954) Metaphor. Proc Aristotelian Soc 55:273–294
Black M (1962) Models and metaphors. Cornell University Press, Ithaca, NY
Bunge, M (1967/1998) Scientific research 1, the search for system, Transaction Publishers, New Brunswick
Bunge M (1973) Method, model and matter. Reidel, Dordrecht
Duit R (1991) The role of analogy and metaphor in learning science. Sci Educ 75:649–672
Article Google Scholar
Gentner D, Stevens A (eds) (1983) Mental models. Lawrence Earlbaum, Hillsdale, NJ
Gilbert JK, Boulter C (eds) (2001) Developing models in science education. Kluwer Academic Publishers, Dordrecht
Gilbert JK (2005) Conceptions of science and technology education. Routledge, London
Grandy RE (2003) What are models and why do we need them? Sci Educ 12:773–2003
Halloun IA, Hestenes D (1987) Modeling instruction in mechanics. Am J Phys 55(5):455–462
Halloun, IA (1984) The use of models in teaching Newtonian mechanics. Unpublished PhD dissertation, Arizona State University, Tempe, AZ
Halloun IA (2004) Modeling theory in science education. Kluwer Academic Publishers, Dordrecht
Harré R (1960) Metaphor, Model and Mechanism. Proc Aristotelian Soc 60:101–122
Hesse MB (1953) Models in physics. Br J Philos Sci 4:198–214
Hesse MB (1961) Forces and fields: the concept of action at a distance in the history of physics. Thomas Nelson & Sons, London
Hesse MB (1966) Models and analogies in science. University of Notre Dame Press, South Bend, IN
Hestenes D (1987) Toward a modeling theory of physics instruction. Am J Phys 60(8):732–748
Johnson-Laird PN (1983) Mental models. Harvard University Press, Cambridge, MA
Justi R, Gilbert J (2000) History and philosophy of science through models: some challenges in the case of the atom. Int J Sci Educ 22(9):993–1009
Justi R, Gilbert J (2003) Teachers views on the nature of models’. Int J Sci Educ 25(11):1369–1386
Leatherdale WH (1974) The role of analogy, model and metaphor in science. Oxford University Press, Oxford
Lind G (1980) Models in physics: some pedagogical reflections based on the history of science. Eur J Sci Educ 2:15–23
Machamer P (2000) The nature of metaphor and scientific descriptions. In: Hallyn F (ed) Metaphor and analogy in the sciences. Kluwer Academic Publishers, Dordrecht, pp 35–52
Matthews MR (2000) Time for science education: how teaching the history and philosophy of pendulum motion can contribute to science literacy. Kluwer Academic Publishers, New York
Matthews, MR (2005) Idealisation and Galileo’s pendulum discoveries: historical, philosophical and pedagogical considerations. In Matthews MR, Gauld CF, Stinner A (eds) The pendulum: scientific, historical, philosophical and educational perspectives. Springer, pp 209–236
Muscari PG (1988) The metaphor in science and in the science classroom. Sci Educ 72:423–431
Sutton C (1978) Metaphorically speaking: the role of metaphor in teaching and learning science. Science Education Series, University of Leicester
Wagenschein M (1962) Die Padagogische Dimension der Physik. Westermann, Braunschweig
Wartofsky, MW (1966/1979) The model muddle: proposals for an immodest realism. In his models: representation and scientific understanding. Reidel, Dordrecht, The Netherlands, pp 1–11
Weller CM (1970) The role of analogy in teaching science. J Res Teach Sci 7:113–119
Woodward J (1989) Data and phenomena. Synthese 79:393–472
Download references
Author information
Authors and affiliations.
School of Education, University of New South Wales, Sydney, 2052, Australia
Michael R. Matthews
You can also search for this author in PubMed Google Scholar
Corresponding author
Correspondence to Michael R. Matthews .
Rights and permissions
Reprints and permissions
About this article
Matthews, M.R. Models in science and in science education: an introduction. Sci & Educ 16 , 647–652 (2007). https://doi.org/10.1007/s11191-007-9089-3
Download citation
Published : 18 May 2007
Issue Date : August 2007
DOI : https://doi.org/10.1007/s11191-007-9089-3
Share this article
Anyone you share the following link with will be able to read this content:
Sorry, a shareable link is not currently available for this article.
Provided by the Springer Nature SharedIt content-sharing initiative
- Epistemology
- Mental models
- Find a journal
- Publish with us
- Track your research
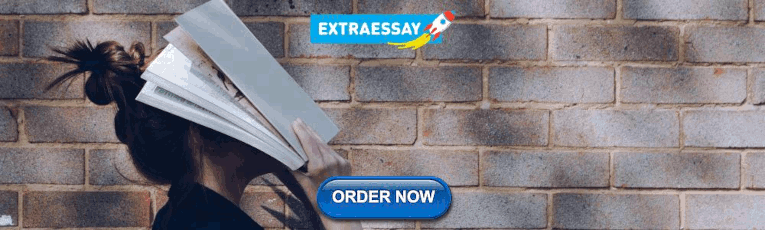
Help Us Stay Paywall-Free
Democracy depends on the free exchange of ideas. Help sustain it with a tax-deductible donation today.
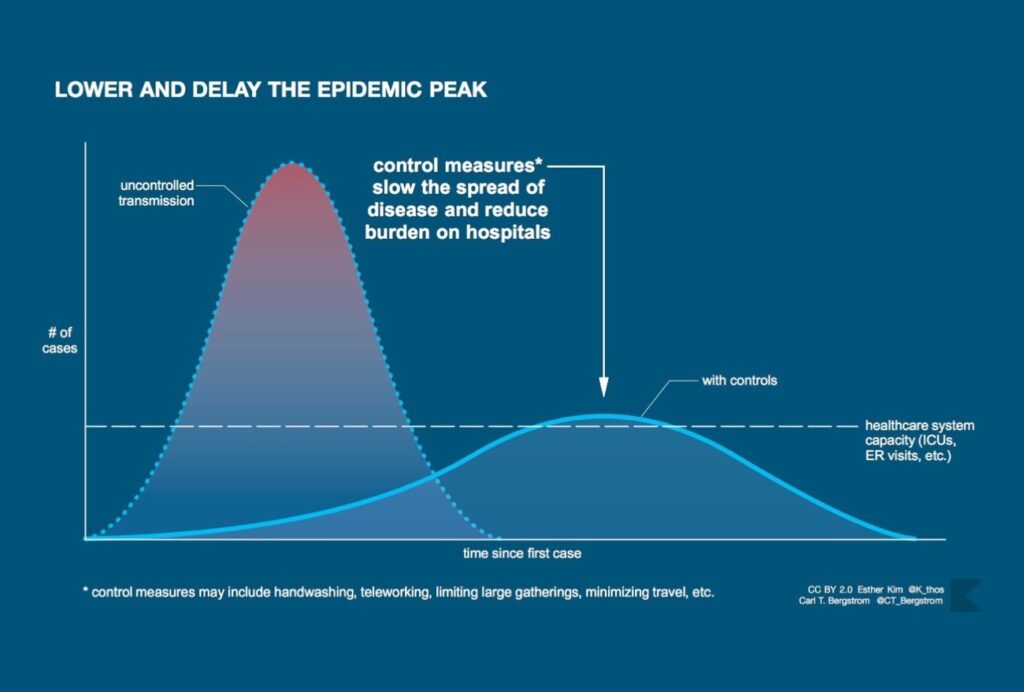
Image: Wikimedia Commons
Models v. Evidence
COVID-19 has revealed a contest between two competing philosophies of scientific knowledge. To manage the crisis, we must draw on both.
Jonathan Fuller
- May 5, 2020
Editors’ Note : Read responses to this essay by the epidemiologists Marc Lipsitch and John Ioannidis , as well as a final response by Jonathan Fuller . All these pieces appear in print in Thinking in a Pandemic .
The lasting icon of the COVID-19 pandemic will likely be the graphic associated with “flattening the curve.” The image is now familiar: a skewed bell curve measuring coronavirus cases that towers above a horizontal line—the health system’s capacity—only to be flattened by an invisible force representing “non-pharmaceutical interventions” such as school closures, social distancing, and full-on lockdowns.
How do the coronavirus models generating these hypothetical curves square with the evidence? What roles do models and evidence play in a pandemic? Answering these questions requires reconciling two competing philosophies in the science of COVID-19.
Public health epidemiology and clinical epidemiology are distinct traditions in health care.
In one camp are infectious disease epidemiologists, who work very closely with institutions of public health. They have used a multitude of models to create virtual worlds in which sim viruses wash over sim populations—sometimes unabated, sometimes held back by a virtual dam of social interventions. This deluge of simulated outcomes played a significant role in leading government actors to shut borders as well as doors to schools and businesses. But the hypothetical curves are smooth, while real-world data are rough. Some detractors have questioned whether we have good evidence for the assumptions the models rely on, and even the necessity of the dramatic steps taken to curb the pandemic. Among this camp are several clinical epidemiologists, who typically provide guidance for clinical practice—regarding, for example, the effectiveness of medical interventions—rather than public health.
The latter camp has won significant media attention in recent weeks. Bill Gates—whose foundation funds the research behind the most visible outbreak model in the United States, developed by the Institute for Health Metrics and Evaluation (IHME) at the University of Washington—worries that COVID-19 might be a “once-in-a-century pandemic.” A notable detractor from this view is Stanford’s John Ioannidis, a clinical epidemiologist, meta-researcher, and reliable skeptic who has openly wondered whether the coronavirus pandemic might rather be a “once-in-a-century evidence fiasco.” He argues that better data are needed to justify the drastic measures undertaken to contain the pandemic in the United States and elsewhere.
Ioannidis claims, in particular, that our data about the pandemic are unreliable, leading to exaggerated estimates of risk. He also points to a systematic review published in 2011 of the evidence regarding physical interventions that aim to reduce the spread of respiratory viruses, worrying that the available evidence is nonrandomized and prone to bias. (A systematic review specific to COVID-19 has now been published; it concurs that the quality of evidence is “low” to “very low” but nonetheless supports the use of quarantine and other public health measures.) According to Ioannidis, the current steps we are taking are “non-evidence-based.”
This talk of “biased evidence” and “evidence-based interventions” is characteristic of the evidence-based medicine (EBM) community, a close relative of clinical epidemiology. In a series of blog posts, for example, Tom Jefferson and Carl Heneghan of the Oxford Centre for Evidence-Based Medicine similarly lament the poor-quality data and evidence guiding action in the pandemic and even suggest that lockdown is the wrong call .
Models without evidence are blind, while evidence without models is inert.
In the other corner, Harvard’s Marc Lipsitch, an infectious disease epidemiologist, agrees that we lack good data in many respects. Countering Ioannidis’s hesitation, however, Lipsitch responds : “We know enough to act; indeed, there is an imperative to act strongly and swiftly.” According to this argument, we could not afford to wait for better data when the consequences of delaying action are disastrous, and did have reason enough to act decisively.
Public health epidemiologists and clinical epidemiologists have overlapping methods and expertise; they all seek to improve health by studying populations. Yet to some extent, public health epidemiology and clinical epidemiology are distinct traditions in health care, competing philosophies of scientific knowledge. Public health epidemiology, including infectious disease epidemiology, tends to embrace theory and diversity of data; it is methodologically liberal and pragmatic . Clinical epidemiology, by contrast, tends to champion evidence and quality of data; it is comparatively more methodologically conservative and skeptical. (There is currently a movement in public health epidemiology that is in some ways closer to the clinical epidemiology philosophy, but I won’t discuss it here.)
To be clear, these comparisons are fair only writ large; they describe disciplinary orthodoxy as a whole rather than the work of any given epidemiologist. Still, it is possible to discern two distinct philosophies in epidemiology, and both have something to offer in the coronavirus crisis over models and evidence. A deeper understanding of modeling and evidence is the key not only to reconciling these divergent scientific mindsets but also to resolving the crisis.
Public health epidemiology uses theory, especially theory from other health sciences like microbiology, to model infection and understand patterns and causes of disease. Many of the epidemic models that the public and public health researchers alike have been voraciously consuming—including models produced by Imperial College London that informed the U.K. and U.S. coronavirus response—are SIR-type models. The theory underlying these models is old, originating in the Kermack–McKendrick theory in the 1920s and ’30s, and even earlier in the germ theory in the second half of the nineteenth century. The SIR framework partitions a population into at least three groups: those who are susceptible to future infection (S), those who are currently infectious (I), and those who have been removed from the infectious group through recovery or death (R). An SIR model uses a system of differential equations to model the dynamics of the outbreak, the movement of individuals among the various groups over time.
The most important question we can ask of a model is not whether its assumptions are accurate but how well it predicts the future.
Other models in the SIR family add additional groups to these three basic ones, such as a group for those who are infected with the virus but not yet infectious to others. Agent-based models also represent infection dynamics (how the number of cases changes over time), but they do so by modeling behaviors for each member of the simulated population individually. Curve-fitting models like the one used by the IHME are less theoretical; they extrapolate from previous infection curves to make predictions about the future. All these different models have been used in the COVID-19 pandemic. The diversity of approaches, along with divergent estimates for model parameters, partly explains the range of predictions we have seen.
Public health epidemiology also relies on a diversity of data—from multiple regions, using a variety of methods—to answer any one scientific question. In the coronavirus pandemic, in particular, research groups have used estimates of multiple key parameters of the outbreak (infection rate, average duration of illness) derived from multiple settings (China, Italy) and produced by various kinds of studies (population-based, laboratory-based, clinically based) to make projections. Public health epidemiology is liberal in the sense of relying on multiple tools, including modeling techniques (the Imperial College team has used several models ), and also in the sense of simulating various possibilities by tweaking a model’s assumptions. Finally, its philosophy is pragmatic. It embraces theory, diversity of data, and modeling as a means to reaching a satisfactory decision, often in circumstances where the evidence is far from definitive but time or practical constraints get in the way of acquiring better evidence.
A formative scientific moment for the public health epidemiology tradition was the epidemiological research on smoking and lung cancer in the 1950s and ’60s. Although lung cancer is not an infectious disease and SIR modeling played no starring role in this research, it featured a similar scientific approach and philosophical outlook. The public health epidemiology philosophy is especially necessary early on in an outbreak of a novel pathogen, when untested assumptions greatly outnumber data, yet predictions and decisions must still be made.
Neil Ferguson, one of the leading epidemiologists behind the Imperial College models, describes epidemic modeling as “building simplified representations of reality.” The characterization is apt because SIR-type models have variables and equations meant to represent real features of the populations modeled. (Other types of scientific tools, such as black box neural nets used in machine learning, work differently: they do not attempt to mirror the world but simply to predict its behavior.) We could therefore ask how well an SIR-type model mirrors reality. However, the primary use of the models, especially early on in an epidemic, is to predict the future of the outbreak, rather than to help us explain or understand it. As a result, the most important question we can ask of an outbreak model during a crisis is not whether its assumptions are accurate but instead how well it predicts the future—a hard-nosed practical question rather than a theoretical one.
Public health epidemiology is pragmatic, embracing theory, diversity of data, and modeling.
Of course, predictive power is not totally unrelated to a model’s representational accuracy. One way to improve the predictive prowess of model is to go out and collect data that can confirm or deny the accuracy of its assumptions. But that’s not the only way. By running many simulations of the same model under different assumptions (so-called sensitivity analysis), a modeler can determine how sensitive the model’s predictions are to changes in its assumptions. By learning from multiple different models, a scientist can also triangulate, so to speak, on a more robust prediction that is less susceptible to the faults of any one model. Both strategies were used in determining U.K. coronavirus policy.
Finally, often a single, more accurate prediction based on high-quality evidence is less useful than a range of modeling predictions that capture best-case and worst-case scenarios (such as the range of death counts the White House coronavirus taskforce presented at the end of March). It might be prudent to plan for the worst case and not only the most likely possibility. A pragmatic philosophy generally serves public health decision makers well.
However, when certain predictions based on plausible model assumptions would lead decision makers to radically different policy recommendations, the assumptions should be investigated with further evidence. A team at Oxford University , for example, performed epidemic modeling specifically to illustrate that worrying coronavirus projections depend crucially on estimates of the number of individuals previously infected and now immune to the virus. It is this kind of uncertainty that serves as fodder for the evidence thumpers.
Clinical epidemiologists are playing their own part in the pandemic: they are designing clinical trials of COVID-19 treatments, such as the World Health Organization–organized multi-country Solidarity trial . In keeping with the high standards of evidence in the EBM movement, these trials are randomized: individuals are randomly allocated to receive one treatment or another (or a different combination of treatments). Although opinions on the exact virtues of randomization vary slightly, the most popular idea is that randomization reduces systematic bias. In a clinical trial, randomization eliminates selection bias, resulting in trial groups that are more representative or comparable in terms of causally relevant background features. Randomized studies are preferred because they can generate evidence that is less biased and more accurate.
The clinical epidemiology tradition cautions that theory can sometimes mislead us, smuggling in unproven assumptions.
The concept of evidence is central to clinical epidemiology and EBM alike. Clinical epidemiology research produces evidence, while EBM experts critically appraise it. Good evidence, this tradition says, consists mainly in the results of clinical epidemiology studies. The tradition is generally suspicious of theory, including reasoning based on pathophysiology and models of disease. It often cautions that theory can sometimes mislead us—for instance, by smuggling in unproven assumptions that have not been empirically established in human populations. In the coronavirus case, models assume—based on experience with other pathogens, but not concrete evidence with the new coronavirus—that individuals who recover from infection will develop immunity against reinfection, at least in the short term.
A central concern for this philosophy is not the diversity but the quality of data. A founding principle of EBM is that the best medical decisions are those that are based on the best available evidence, and evidence is better if it consists of higher-quality data. EBM provides guidance on which evidence is best, but clinical epidemiological methods such as meta-analysis do not allow one to amalgamate diverse kinds of evidence. The tradition is also conservative in basing conclusions only on well-established empirical results rather than speculative modeling, preferring “gold standard” randomized studies to hypothetical simulations. Finally, this tradition is skeptical, challenging assumptions, authority, and dogma, always in search of study design flaws and quick to point out the limitations of research.
A formative moment for the clinical epidemiology tradition was the British Medical Research Council’s 1948 trial of streptomycin for tuberculosis, widely considered to be one of the first modern randomized clinical trials. This philosophy can be especially helpful as an outbreak of a novel pathogen evolves, as better evidence becomes available to scrutinize previous assumptions and settle unanswered questions. Clinical epidemiology has the expertise to contribute much of this evidence.
In advocating for evidence-based public health measures , Ioannidis suggests subjecting interventions like social distancing measures to randomized trials. His suggestion may not be feasible in the United States given multiple levels of governance over social distancing policies, among other logistical difficulties. But the suggestion that we should be studying the effectiveness of our public health interventions is as important as it is obvious, and clinical epidemiology is well placed to contribute to this endeavor. While public health epidemiology is adept at studying the distributions and determinants of disease, clinical epidemiology is at home in studying the effectiveness of healthcare interventions. (I do not mean to suggest that public health epidemiology lacks the resources to study its own interventions. Consider, for example, this clever impact study by Imperial College London.)
Measuring the effects of public health measures is far from trivial. Social distancing is not an intervention: it is a mixed bag of individual behaviors, some voluntary and some involuntary. These behaviors are represented in outbreak models by simulating reduced social interactions. The models sometimes suppose that certain specific interventions, such as school or business closures, will produce particular patterns of social mixing. But the effects of specific interventions on patterns of social mixing is not the target of a classic SIR model. The modeler inputs patterns of social interaction; the model doesn’t spit them out. (However, disease-behavior models do model social dynamics together with viral dynamics.) Rigorous research is needed to separate out the effects of individual interventions that have often been implemented simultaneously and are difficult to disentangle from independent behavior changes. Moreover, our interventions might have independent effects (on health, on the economy), and an outbreak model isn’t broad enough in scope to predict these effects.
Too much skepticism is paralyzing, but it can provide a check on the pragmatic ethos of public health epidemiology.
Ioannidis also suggests a solution to the problem of inaccurate pandemic statistics: testing representative population samples, rather than relying on samples subject to sampling bias. In order to estimate the number of infected people and the growth of the pandemic over time, we can repeatedly sample from key demographics and perform diagnostic testing. Representative sampling and antibody assays can also help estimate the number of previously infected individuals who may be immune to reinfection. This information can help to rule out the Oxford scenario in which the susceptible population is much, much smaller than we think. It can also help in estimating the infection fatality ratio, the proportion of COVID-19 patients who die from their infection. Ioannidis argues that the infection fatality ratio has been greatly overestimated in certain contexts due to biased testing. Antibody testing has already begun in the United States and other countries, including a (not yet peer-reviewed) study by Ioannidis and colleagues estimating much higher prevalence of past COVID-19 infections in Santa Clara County than the official count. Ironically, the study was immediately criticized by scientists partly for its Facebook recruitment strategy on the grounds it may have resulted in a biased sample.
The key to proper representative sampling is clinical epidemiology’s favorite motto: randomize it! Random sampling can overcome the sampling bias that has plagued modeling projections alongside the coronavirus. The clinical epidemiology tradition, transfixed with unbiased evidence, provides a ready solution to an urgent problem facing public health epidemiology.
The final gift that clinical epidemiology offers is its skeptical disposition. Institutionalized skepticism is important in science and policymaking. Too much of it is paralyzing, especially in contexts of information poverty that call for pragmatism—like at the outset of a pandemic involving a novel pathogen when we don’t have gold-standard evidence to guide us, but inaction carries the risk of dire consequences. But clinical epidemiology’s skeptical orientation can provide a check on the pragmatic ethos of public health epidemiology, preventing action from outrunning evidence, or at least helping evidence to catch up.
At the same time, a myopic focus on evidence alone would do a disservice to epidemiology. Were we to conduct randomized trials of public health interventions, the evidence generated would be inherently local—specific to the context in which the trials are run—because the effects of public health interventions (really, all interventions) depend on what other causal factors are in play. We can’t simply extrapolate from one context to another. Similarly, we should not blindly extrapolate infection statistics from one location to another; all these parameters—the reproductive number, the attack rate, the infection fatality ratio—are context-sensitive . None of these statistics is an intrinsic property of the virus or our interventions; they emerge from the interaction among intervention, pathogen, population, and place.
It is theory, along with a reliance on a diverse range of data, that make coronavirus evidence collected in one place relevant to another. Evidence for the effects of interventions on social interactions must be combined with outbreak models representing those interactions. Evidence for age-stratified infection fatality ratios must be combined with local data about the age structure of a population to be of any use in predicting fatalities in that population. In an outbreak, models without evidence are blind, while evidence without models is inert.
Where does this clash of sensibilities leave us? In my own work, I have modeled prediction in evidence-based medicine as a chain of inferences. Each individual inference is a link forged from assumptions in need of evidence; the chain is broken if any assumption breaks down. In their book Evidence-Based Policy (2012), the philosopher of science Nancy Cartwright and the economist Jeremy Hardie represent predictions about the effectiveness of a policy using a pyramid. The top level, the hypothesis that the policy will work in some local context, rests on several assumptions, which rest on further assumptions, and so on. Without evidence for the assumptions, the entire structure falls.
Cooperation in society should be matched by cooperation across disciplinary divides.
Either picture is a good metaphor for the relationship between evidence and models. Evidence is needed to support modeling assumptions to generate predictions that are more precise and accurate. Evidence is also needed to rule out alternative assumptions, and thus alternative predictions. Models represent a multiverse of hypothetical futures. Evidence helps us predict which future will materialize directly by filling in its contours, and indirectly by scratching out other hypothetical worlds.
The need for evidence and modeling will not dissolve when the dust settles in our future world. In evaluating the choices we made and the effectiveness of our policies, we will need to predict what would have happened otherwise. Such a judgment involves comparing worlds: the actual world that materialized and some hypothetical world that did not. How many COVID-19 deaths did our social distancing measures prevent? We can estimate the number of COVID-19 deaths in our actual socially distanced world by counting, but to predict the number of COVID-19 deaths in an unchosen world without social distancing we will need to dust off our models and evidence.
Just as we should embrace both models and evidence, we should welcome both of epidemiology’s competing philosophies. This may sound like a boring conclusion, but in the coronavirus pandemic there is no glory, and there are no winners. Cooperation in society should be matched by cooperation across disciplinary divides. The normal process of scientific scrutiny and peer review has given way to a fast track from research offices to media headlines and policy panels. Yet the need for criticism from diverse minds remains.
I mentioned that the discovery that smoking causes lung cancer was a discipline-defining achievement for public health epidemiology, while the British Medical Research Council’s streptomycin trial was a formative episode in the history of clinical epidemiology. The epidemiologist Austin Bradford Hill played a role in both scientific achievements. He promoted the clinical trial in medicine and also provided nine criteria (“Hill’s Viewpoints”) still used in public health epidemiology for making causal inferences from a diversity of data.
Like Hill, epidemiology should be of two minds. It must combine theory with evidence and make use of diverse data while demanding data of increasingly higher quality. It must be liberal in its reasoning but conservative in its conclusions, pragmatic in its decision making while remaining skeptical of its own science. It must be split-brained, acting with one hand while collecting more information with the other. Only by borrowing from both ways of thinking will we have the right mind for a pandemic.
Boston Review is nonprofit and relies on reader funding. To support work like this, please donate here .
Jonathan Fuller, MD, PhD, is Assistant Professor of History and Philosophy of Science at the University of Pittsburgh.
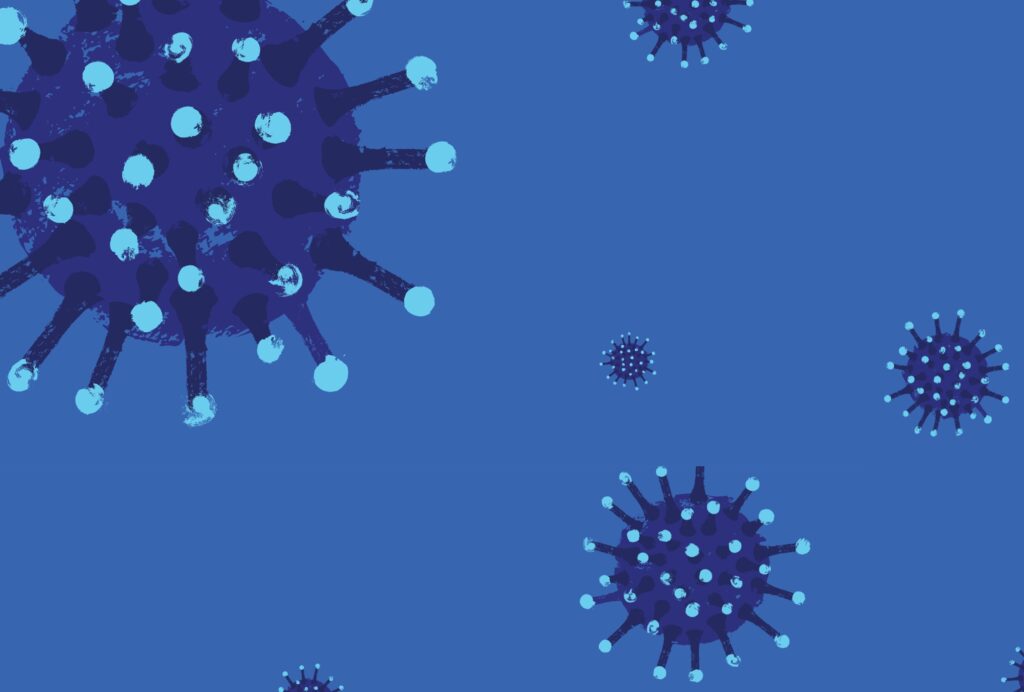
Thinking in a Pandemic
Boston review is nonprofit and reader funded..
We rely on readers to keep our pages free and open to all. Help sustain a public space for collective reasoning and imagination: become a supporting reader today .
Readers Also Liked
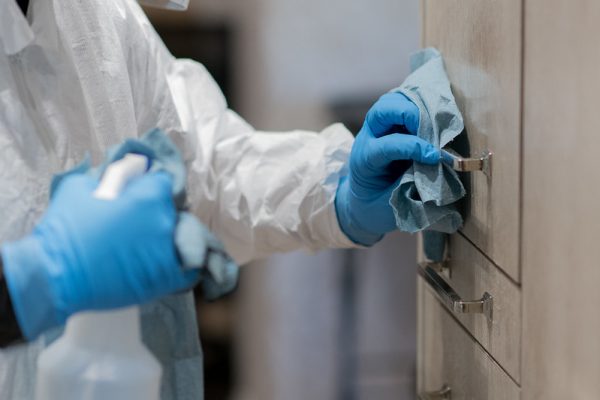
The Totality of the Evidence
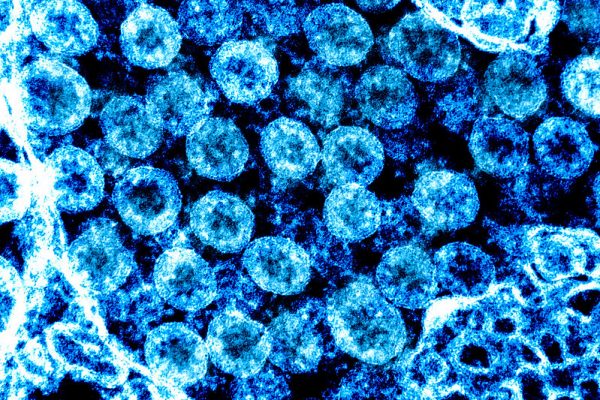
Good Science Is Good Science
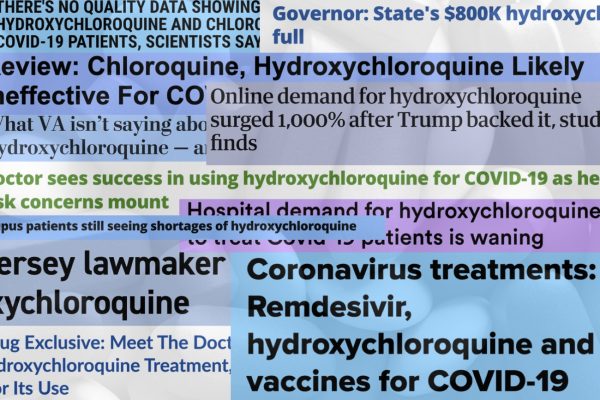
Hydroxychloroquine and the Political Polarization of Science
Donate to support work like this:, sign up for our newsletter.
The best writing on ideas, politics, and culture delivered straight to your inbox.
Most Recent
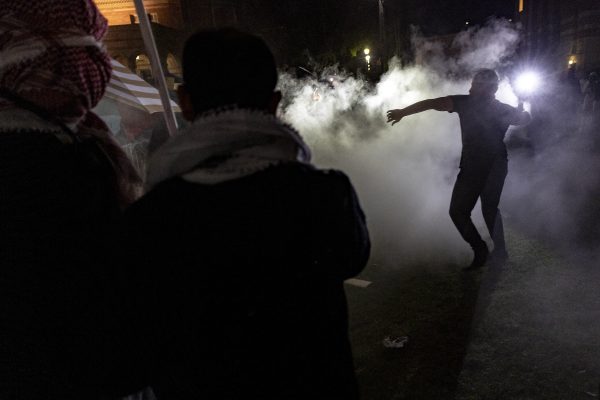
UCLA’s Unholy Alliance
House Republicans accuse student protesters of vicious anti-Semitism, but it is administrators who are courting violence.
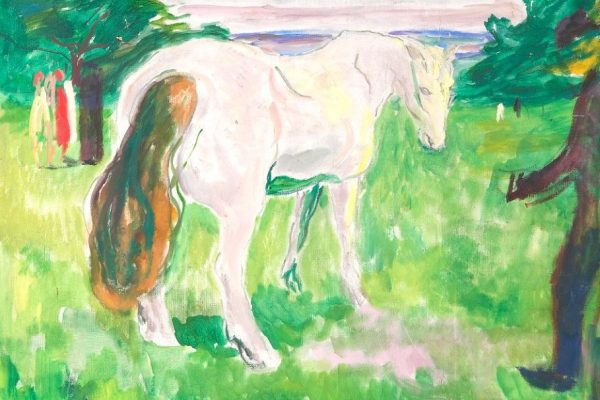
Three Poems
Before I left him / on his deathbed, my father used to say the ice is breathing : this quivering song of things once-broken, mending. / This song of them breaking again.
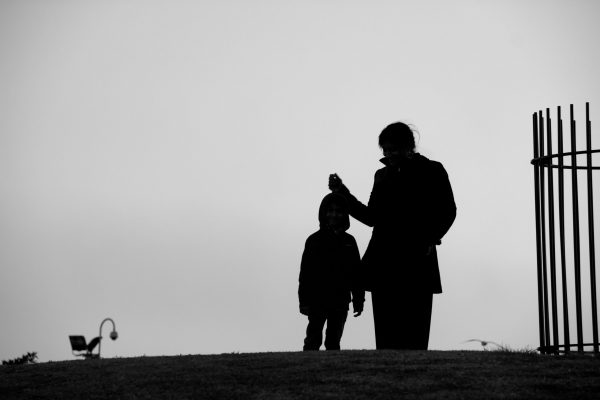
You can say my mother didn’t know jack about no line breaks, but she’ll tell you that one thing leads to another; and violence and love can happen all at once.
A political and literary forum, independent and nonprofit since 1975. Registered 501(c)(3) organization. Learn more about our mission
Make a tax-deductible donation today
Get Our Newsletter
Vital reading on politics, literature, and more in your inbox
"A tremendous resource in this time of chaos."
We publish leading scholars, activists, and writers on the most pressing political debates of our time.
But as a small nonprofit, we rely on reader support. Will you help support bold thinking about a more just world?
"An indispensable pillar of the public sphere."
That’s what sociologist Alondra Nelson says of Boston Review . Independent and nonprofit, we believe in the power of collective reasoning and imagination to create a more just world.
That’s why there are no paywalls on our website, but we can’t do it without the support of our readers. Will you make a tax-deductible contribution today?
e-Kalvi e-Kalvi is the e-learning portal for general education. This is specially designed according to syllabuses of the students from grade 1 to 13.
2023 o/l science model paper sinhala medium.
admin March 29, 2023 Science Comments Off on 2023 O/L Science Model Paper Sinhala Medium 6,852 Views
Download 2023 G.C.E O/L Science Model Paper Sinhala Medium, Model Paper available in PDF format. Prepared and Published by Ministry of Education, Sri Lanka .

Preview of Science Model Paper Sinhala Medium
Download PDF
- G.C.E O/L Science Marking Scheme 2021
- G.C.E O/L Science Past Paper 2021
- Stumbleupon
Related Articles

2024 G.C.E O/L Science Model Papers Sinhala Medium
April 9, 2024

2023 Grade 11 3rd Term Test Papers Sinhala Medium – NWP
April 2, 2024

2022 (2023) O/L Science Marking Scheme Sinhala Medium
November 30, 2023

2022 (2023) O/L Science Past Paper Sinhala Medium
November 29, 2023

2021 (2022) O/L Science Marking Scheme Sinhala Medium PDF
February 12, 2023
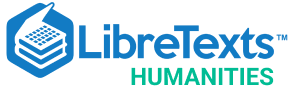
- school Campus Bookshelves
- menu_book Bookshelves
- perm_media Learning Objects
- login Login
- how_to_reg Request Instructor Account
- hub Instructor Commons
Margin Size
- Download Page (PDF)
- Download Full Book (PDF)
- Periodic Table
- Physics Constants
- Scientific Calculator
- Reference & Cite
- Tools expand_more
- Readability
selected template will load here
This action is not available.
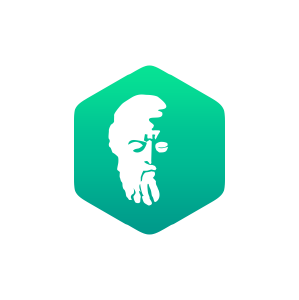
3.4: Cause and Effect Model Essay 3
- Last updated
- Save as PDF
- Page ID 174290
\( \newcommand{\vecs}[1]{\overset { \scriptstyle \rightharpoonup} {\mathbf{#1}} } \)
\( \newcommand{\vecd}[1]{\overset{-\!-\!\rightharpoonup}{\vphantom{a}\smash {#1}}} \)
\( \newcommand{\id}{\mathrm{id}}\) \( \newcommand{\Span}{\mathrm{span}}\)
( \newcommand{\kernel}{\mathrm{null}\,}\) \( \newcommand{\range}{\mathrm{range}\,}\)
\( \newcommand{\RealPart}{\mathrm{Re}}\) \( \newcommand{\ImaginaryPart}{\mathrm{Im}}\)
\( \newcommand{\Argument}{\mathrm{Arg}}\) \( \newcommand{\norm}[1]{\| #1 \|}\)
\( \newcommand{\inner}[2]{\langle #1, #2 \rangle}\)
\( \newcommand{\Span}{\mathrm{span}}\)
\( \newcommand{\id}{\mathrm{id}}\)
\( \newcommand{\kernel}{\mathrm{null}\,}\)
\( \newcommand{\range}{\mathrm{range}\,}\)
\( \newcommand{\RealPart}{\mathrm{Re}}\)
\( \newcommand{\ImaginaryPart}{\mathrm{Im}}\)
\( \newcommand{\Argument}{\mathrm{Arg}}\)
\( \newcommand{\norm}[1]{\| #1 \|}\)
\( \newcommand{\Span}{\mathrm{span}}\) \( \newcommand{\AA}{\unicode[.8,0]{x212B}}\)
\( \newcommand{\vectorA}[1]{\vec{#1}} % arrow\)
\( \newcommand{\vectorAt}[1]{\vec{\text{#1}}} % arrow\)
\( \newcommand{\vectorB}[1]{\overset { \scriptstyle \rightharpoonup} {\mathbf{#1}} } \)
\( \newcommand{\vectorC}[1]{\textbf{#1}} \)
\( \newcommand{\vectorD}[1]{\overrightarrow{#1}} \)
\( \newcommand{\vectorDt}[1]{\overrightarrow{\text{#1}}} \)
\( \newcommand{\vectE}[1]{\overset{-\!-\!\rightharpoonup}{\vphantom{a}\smash{\mathbf {#1}}}} \)
Read the following essay, fill in the blanks with suitable cause-effect signal words and phrases. Pay attention to the sentence structure to help you decide. There may be more than one correct sign word or phrase.
First Name Last Name
Professor Name
Course Name and Number
Month Day Year
The Effects of Dropping Out of High School
(1) Every year thousands of teenagers drop out of high school. Some of these teenagers are wage earners who need to contribute to the family income. For them, the immediate need of helping out financially overrides their need for a diploma. Some teenagers did not get a strong academic foundation in elementary and middle school, so the high school curriculum proves to be too challenging, which leads to frustration. They find it easier to leave school and start life as working adults. Still others become involved in illegal activities, which often includes drug use. Such a lifestyle is not compatible with regular attendance and study necessary for high school success. In spite of the efforts of parents, teachers, and counselors, these teens end up leaving school to face whatever “real life” brings them. In their situations, it is difficult for them to see that leaving school will have profound effects on their finances, their ability to function in society, and even their sense of self-worth.
(2) Clearly, leaving high school without a diploma will have a negative (a) ____________on a person’s finances. Though there are jobs that do not require a high school diploma, these are often not suitable for the long term. Most of these are minimum-wage paying jobs, paying hourly wages that barely cover basic living expenses. Such jobs are usually in the service industry and are often physically demanding. They typically do not offer a lot of job satisfaction. Though there are some better-paying jobs that do not require a high school diploma such as utility line installers, heavy equipment operators, and communications installers, these jobs rarely (b)______________ higher-level managerial positions without a high school diploma. (c) _____________, for the rest of his life, the high school dropout is locked in a job with little hope of significant pay increases to keep up with the cost of living or to provide for a family beyond life’s basics.
(3) Dropping out of high school can also negatively (d) ______________a person’s ability to function as a fully participating member of society. High school math skills, which go beyond the basics, are necessary to function as an informed consumer. These skills affect good decision making about everything from home mortgages to retirement plans. The advanced reading and critical thinking skills that are part of the high school curriculum are also necessary to make good choices, whether choosing a cell phone plan or voting in local and national elections. The ability to be a fully participating member of society is severely hindered if a person lacks the skills learned in high school.
(4) Finally, a less obvious (e) ________________ of dropping out of high school concerns the person’s sense of self-worth. A high school diploma is considered a measure of a basic level of learning for citizens in modern societies. The vast majority of people complete this educational milestone. A person who drops out, however, cannot claim this accomplishment. (f) _________________, he may feel that he is not as valuable to society as others or that other people are somehow better than he is. Though this is not necessarily always the case, the lack of a diploma can lead to a lack of confidence. This could affect his interaction with other people, whether during a casual conversation when meeting new people at a party or during a high stakes job interview. A person may even feel he does not belong to a group of people with more education than he has.
(5) In short, the knowledge and skills learned in high school form a foundation for full participation in our society. Without a diploma, a teen has limited opportunities financially and socially. For those who choose not to complete this degree, life will provide a series of unique challenges, so it is important that anyone thinking of dropping out really understands the consequences.
Questions about the Model 3
1. Highlight the thesis statement of this essay.
2. Does the writer focus on causes or effects?
3. Does the writer give any causes? If so, where? What are they?
4. Can you think of any additional effects?
- Grade 5 Scholarship |
- O/L Past papers |
- 2024 O/L Model Papers |
- Royal College |
- Western Province |
- Online Book Shop
- Combined Maths
- Agricultural Science
- Business Studies
- Business Statistics
- Christianity
- Buddhist Civilization
- Drama and Theatre
- Political Science
- General English
- Agriculture
- Home Economics
- Indian History
- Sri Lankan History
- Grade 11 Papers
- Grade 10 Papers
- Grade 09 Papers
- Grade 08 Papers
- Grade 07 Papers
- Civic Education
- English Language
- Mathematics
- Second Language
- Sinhala Language
- Tamil Language
- Western Music
- Scholarship Exam Past Papers
- Scholarship Model Papers
- Environment
- Catholicism
- Grade 11 English Medium
- Grade 10 English Medium
- Grade 09 English Medium
- Grade 08 English Medium
- Grade 07 English Medium
- Grade 06 English Medium
- Sinhala Medium
- Sinhala Medium Answers
- English Medium
- English Medium Answers
- Tamil Medium
- Sinhala Medim Papers
- English Medium Papers
- Tamil Medium Papers
- Sinhala Medium Marking
- English Medium Marking
- Tamil Medium Marking
- Western Province
- North Western Province
- Southern Province
- North Central Province
- Central Province
- Sabaragamuwa Province
- Royal College
- Ananda College
- D.S.Senanayake
- Devi Balika
- Nalanda College
- Rathnavali Balika
- Visakha College
- Grade 11 Textbooks
- Grade 10 Textbooks
- Grade 9 Textbooks
- Grade 8 Textbooks
- Grade 7 Textbooks
- Grade 6 Textbooks
- WIKI Forum! Join
2023(2024) O/L Model Papers
Download 2023(2024) o/l model papers with answers as a pdf file. we include the last three years ol model question papers developed by the ministry of education, sri lanka. these model papers were prepared by well-experienced teachers, nie, and provincial education departments in sri lanka., 2023(2024) o/l maths model paper (ministry of education) | tamil medium.
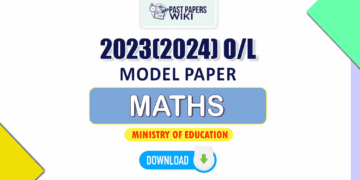
Download 2023(2024) O/L Maths Model Paper in Tamil medium which has been released by the Ministry of Education and published...
2023(2024) O/L Sinhala Literature Model Paper (Ministry of Education) | Sinhala Medium
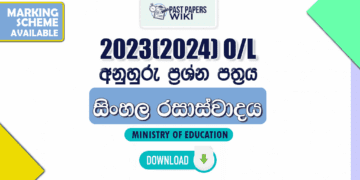
Download 2023(2024) O/L Sinhala Literature Model Paper in Sinhala medium which has been released by the Ministry of Education and...
2023(2024) O/L Sinhala Language Model Paper (Ministry of Education)
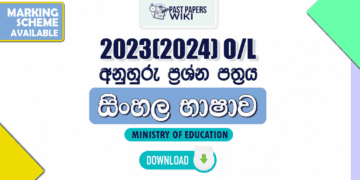
Download 2023(2024) O/L Sinhala Language Model Paper in Sinhala medium which has been released by the Ministry of Education and...
2023(2024) O/L Maths Model Paper (Ministry of Education) | Sinhala Medium
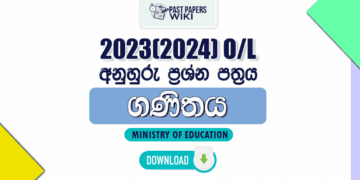
Download 2023(2024) O/L Maths Model Paper in Sinhala medium which has been released by the Ministry of Education and published...
2023(2024) O/L Maths Model Paper (Ministry of Education) | English Medium
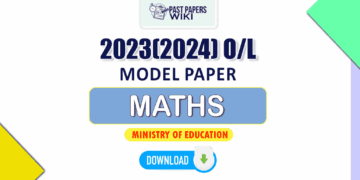
Download 2023(2024) O/L Maths Model Paper in English medium which has been released by the Ministry of Education and published...
2023(2024) O/L ICT Model Paper (English Medium) – North Western Province
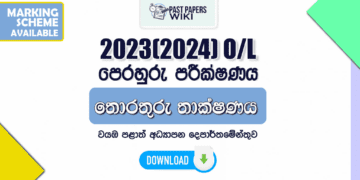
Download 2023(2024) O/L ICT Model Paper in English Medium which has been released by the Department of Education, North Western Province....
2023(2024) O/L Dancing Model Paper – North Western Province
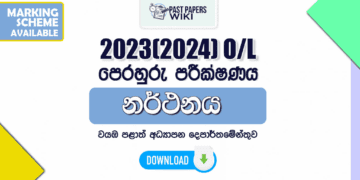
Download 2023(2024) O/L Dancing Model Paper in Sinhala medium which has been released by the Department of Education, North Western Province....
2023(2024) O/L Home Science Model Paper – North Western Province
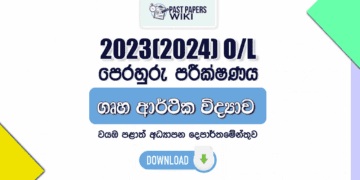
Download 2023(2024) O/L Home Science Model Paper in Sinhala medium which has been released by the Department of Education, North Western...
2023(2024) O/L Western Music Model Paper (English Medium) – North Western Province
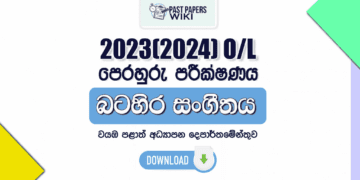
Download 2023(2024) O/L Civic Model Paper in English medium which has been released by the Department of Education, North Western Province....
2023(2024) O/L Oriental Music Model Paper – North Western Province
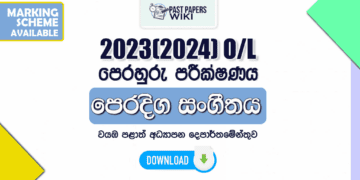
Download 2023(2024) O/L Oriental Music Model Paper in Sinhala medium which has been released by the Department of Education, North Western...
Buy Books Online
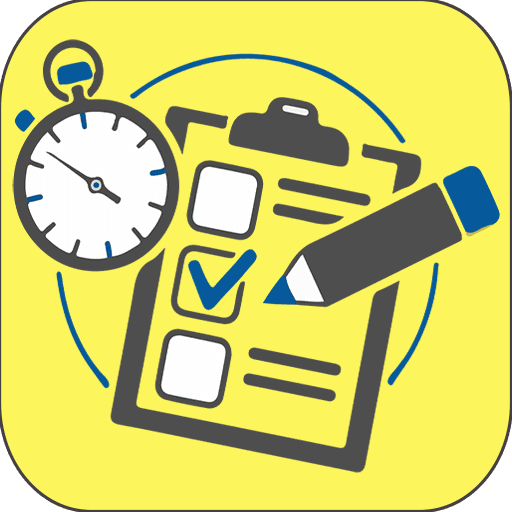
Pastpapers wiki is a free resource site for O/L and A/L Students In Sri Lanka. Past Papers WiKi was founded in October 2019 by Education Resources.lk. The main goal of this site is to provide Past Papers, Marking Schemes, Notes, and other resources that allow students to improve their knowledge.
https://forum.pastpapers.wiki/ Email: [email protected]
Become a Volunteer – Help Others 🙋♂️
We are currently looking for volunteers who are willing to share their expertise and contribute to our community by sharing educational materials in our forum. We’re looking for individuals who can help us create and share educational materials such as articles, videos, and social media posts that will inspire and educate others.
Share Past Papers 📝 | Help Others 🤝 Join With: https://forum.pastpapers.wiki/
Disclaimer of Past Papers WiKi
This website is continued for your personal appreciation or educational purposes only . All Content of this website is published by extracting the information from online sources such as official government websites, social media, other websites, etc. The copyrights of these contents belong to the responsible owners . If a modification will happen in this information, our website does not assume any responsibility. If you have any questions or suggestions, please contact us.
– Mobile No: 071-8540371 – Email: [email protected]
- Science stream
- Commerce stream
- Technology stream
- Arts Stream
- Common Subjects
- WIKI Forum!
Copyright 2019 -2021 © All rights reserved.
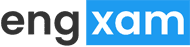
Essay – examples & model answers | B2 First (FCE)
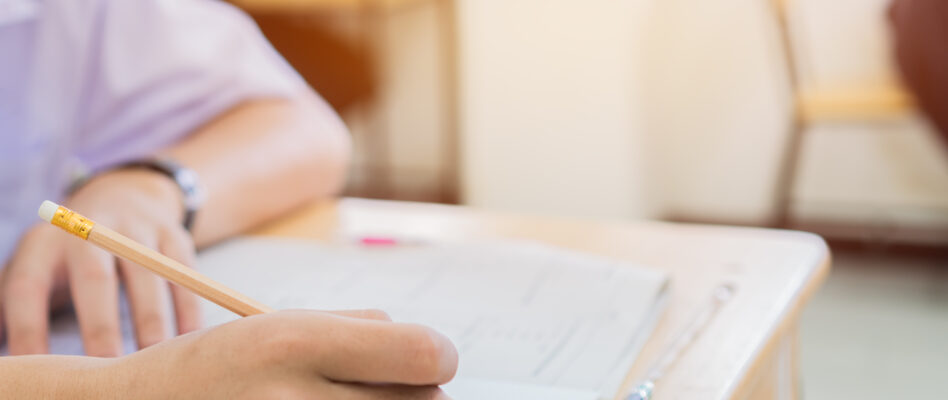
FCE Essay Examples: Topic (Environment)
Example exam task:, example answer (grade: 3), example answer:.
I think that my country has problems with pollution to the environment like all other countries. This problem is normal for Russia. We have big problems with transport because there are too much cars in our country. And because of that we have problems with atmospeer, air in my city and in all Russia is really dirty and sometimes I can’t make a sigh because it smells around me and of course around that cars on the road. I’ve heard about tradition of one country. They don’t go anywhere by car one day a month or a year, they just use bycicle or their feet. I think it could be very good if we had a tradition like that.
So, what about the rivers and the seas? Yeah, there are some really good and clean rivers and seas where you can go, but there are not many of them. Once I saw the river OB in my city, it was about two years ago but I stil remember that in some places it was not blue, it was green or purple I didn’t really understand because it had different colours.
I don’t know what should we do. Maybe we should just open our eyes and look what we did. But Russian people don’t care about the world around them many people care only about themselves an that’s all.
So, the best idea is look around and try to do something good for our planet and for us and our children.
FCE, CAE, CPE
Practice, write & improve, examiners comments & grade:, example answer (grade: 3-4).
To begin with pollution and damage to the environment is the most serious and difficult problem for countries of all over the world. Scientists of different countries predict a global ecocatastrophe if people won’t change their attitude to our planet.
First of all a huge damage to the environment brings a transport. People can’t imagine their living without cars, buses, trains, ships and planes. But it’s an open secret that one of disadvantage of these accustomed things is harmful exhaust. Needless to say that use of environment friendly engines helps us to save atmosphere from pollution.
In addition to this our rivers and seas are in not less danger situation. It’s a fact of common knowledge that numerous factories and plants pour off their waste to ponds. Obviously that cleaning manufacturing water helps to avoid extinction of ocean residents.
Apart from this I’m inclined to believe that every person can and must contribute to solving this important problem. Doing a little steps for protection our environment every day we will be able to save our Earth. And it’s a task of each of us.
Model Answer (Grade: 5)
DEVELOPMENT VS ENVIRONMENT
If we surf the web looking for pollution and environmental catastrophes, we will find out that every country in the world suffers them. This is a natural consequence of the struggle between development and environment.
If a country decided to live isolated from the rest of the world, living on what it can naturally grow and produce, it surely wouldn’t be highly polluted. But we all want exotic food and technological items from all over the world, so we have to pay the price.
Investing on electrical transport would benefit the environment a lot. Even more if this electricity came from a natural source of energy like wind, rivers and solar boards. It’s difficult to achieve this because petrol companies will fight against these actions.
We also have to take care of our rivers and seas. We all have heard about factories throwing highly toxic substances to rivers, without minimizing their poisoning effects. A really strict law should be applied to fine these factories and make them change their policy.
But what about ourselves? We also can do a lot! If, when possible, we bought larger packs of food, we would be producing less rubbish. And this is only an example!
FCE Essay Examples: Topic (Fashion)
In today’s world, the fashion industry has a strong importance in people’s lives. The fashion industry say to the society what to wear and creates new types of clothes all the time.
Some people claim that the fashion industry has a bad effect on people’s lives, they say that the fashion industry creates clothes that the society has to wear. Furthermore, the clothes’ price is extremely high and people, who can’t afford it, should not be in the society.
In the other hand, the fashion industry guide the people to be in a good appearance, because, nowadays, the appearance of the person is more important than the person itself.
In my opinion, the fashion industry doesn’t has a bad influence on people’s lives. It’s something which was created to help people what to wear.
Example Answer (Grade: 4)
Fashion industry is very a discussed subject nowadays: they create and design new clothes everyday in order to satisfy some people needs.
There are many people who claim that the fashion industry is important and good for society. According to them, this industry design beautiful clothes and thanks to that every person can wear shirts, trousers or any acessory which is on today’s fashion.
On the other hand, the fashion industry in some people opinion, controls the market of clothes and because of that they can’t wear what they want to. In addition, the industry can increase the price of clothes, forcing people who don’t want to be “oldfashioned” to buy and pay a large amount of money to keep “beautiful”.
In my opinion, we can’t let the fashion industry decide what we must or musn’t wear. We shouldn’t judge people for its appearance,because that is not important. We must wear whatever we like, want and feel confortable with.
The society we live today is characterised by technology in constant development, fast speed processes, information travelling and getting to people at a blink of an eye and a complex web of social networking. In this context, the fashion industry is becoming increasingly important and having a more and more paramount role in our lives.
On one hand, the fashion industry is undeniably a source of profit and income. It hires millions of people all over the world and generates millions of dollars every year. Furthermore, such profitable business is also believed to be able to spread and make known the culture of a people, encouraging and enhancing a better understanding of each other.
Nevertheless, for those who are neither impressed nor motivated by numbers and figures, the fashion industry is seen as one which segregates people, isolating those who not fit their laws and commands. It is stated that people place too much importance on appearance and the material, world, sadly true, and the fashion industry just spurs on such situation. Moreover, not only are the costs of fashion item unrealistically high, it is thought to be a money better spent on more pressing issues, such as poverty and hunger.
I do believe that the fashion industry, as it is today, has a harmful effect, because it values a minority of people in detriment to the majority. However, it has such a wide reach that, it put into a good use, it can save lives.
FCE Essay Examples: Topic (Languages)
“There are more reasons to learn a foreign language than to pass a test”
Everything around us revolves around language(s), it is the most important thing in our lives. Society would just not function without it. They are It is our future and I would personaly love to learn as many as I possibly can.
Not everything in life is done because it is necessary. Learning a new language can be a lot of fun. Many people only do it as a hoby, or their knowledge is something that brings them pride and pleasure.
Secondly, we have people who do it simply to challenge themselves. Truly I believe that having a great outcome that stems from your hard work and dedication to learn something new is a wonderful way to challenge prove your ability to yourself and others. Then there is travelling. It is very important to be able to understand and have a conversation with someone abroad, unless you would like to get lost or worse.
To conclude, I think that learning a new language is an amazing thing no matter why you do it. It is always better to do things out of enjoyment, but even if you do it for a test, that knowledge will always be useful.
Learning a a foreign languages is very important nowadays. English, in particular, is essential because it allows is spoken all over the world. That’s the reason why we start studying it from the age of six years old. Going abroad and being able to speak to native people is very satisfying and that’s why I want to improve my knowledge about foreign languages.
I decided to take this exam to know how high my level of English is, but also because I need this certification to go abroad next summer. I really want to come back to Cornwall, an amazing region in the South-West of England. I’ve been there twice with my family, but now I want to go alone. Only being there to England I can really improve my English comprehension and speaking skills.
Fortunately I can will have some English lessons which taught in English at university and I can’t wait for it because it will be an interesting challenge for me. Studying foreign languages is essential to live and to travel. It isn’t simple and I surely have to challenge myself everyday, but the result is so satisfying that we I can’t do without it.
FCE Essay Example: Topic (History)
A very common topic that is being discussed nowadays is wether schools should teach subjects that some may consider useless later in life. A clear example is history, since it is quite difficult to learn and does not help us in day-to-day activities.
However, many people do not realize the importance of it or that it affects our lives today. For example, our political system would not be this way if it weren’t for the Ancient Greeks, numerous politicians and wars who helped shape democracy and our constitution. Yet it is still thought that it’s useless.
In addition, it is very important that we never forget about our past since we must know where we were standing years ago. Moreover, there are some things, such as World War II, that we have to remember to prevent them from happening again. We should also know where we we were standing a century ago: our origins, our identity. The more you learn about your ethnicity, the better.
All in all, I think that it is extremely important to learn about one’s own country’s history. Anyone who gets the chance to do this should not waste it, since they are very fortunate to have this opportunity
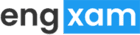
Would you pass B2 First (FCE)?
Help | Advanced Search
Computer Science > Computer Vision and Pattern Recognition
Title: mambaout: do we really need mamba for vision.
Abstract: Mamba, an architecture with RNN-like token mixer of state space model (SSM), was recently introduced to address the quadratic complexity of the attention mechanism and subsequently applied to vision tasks. Nevertheless, the performance of Mamba for vision is often underwhelming when compared with convolutional and attention-based models. In this paper, we delve into the essence of Mamba, and conceptually conclude that Mamba is ideally suited for tasks with long-sequence and autoregressive characteristics. For vision tasks, as image classification does not align with either characteristic, we hypothesize that Mamba is not necessary for this task; Detection and segmentation tasks are also not autoregressive, yet they adhere to the long-sequence characteristic, so we believe it is still worthwhile to explore Mamba's potential for these tasks. To empirically verify our hypotheses, we construct a series of models named MambaOut through stacking Mamba blocks while removing their core token mixer, SSM. Experimental results strongly support our hypotheses. Specifically, our MambaOut model surpasses all visual Mamba models on ImageNet image classification, indicating that Mamba is indeed unnecessary for this task. As for detection and segmentation, MambaOut cannot match the performance of state-of-the-art visual Mamba models, demonstrating the potential of Mamba for long-sequence visual tasks. The code is available at this https URL
Submission history
Access paper:.
- HTML (experimental)
- Other Formats
References & Citations
- Google Scholar
- Semantic Scholar
BibTeX formatted citation

Bibliographic and Citation Tools
Code, data and media associated with this article, recommenders and search tools.
- Institution
arXivLabs: experimental projects with community collaborators
arXivLabs is a framework that allows collaborators to develop and share new arXiv features directly on our website.
Both individuals and organizations that work with arXivLabs have embraced and accepted our values of openness, community, excellence, and user data privacy. arXiv is committed to these values and only works with partners that adhere to them.
Have an idea for a project that will add value for arXiv's community? Learn more about arXivLabs .
- Advanced search
- Peer review
To submit your Book proposal, please click here
- Record : found
- Abstract : found
- Book : found
Economics : Model Essays (Second Edition)
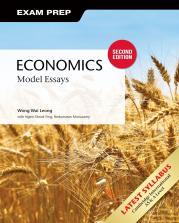
Read this book at
- Review book
- Invite someone to review
Economics: Model Essays is the 2nd edition of the best-selling revision guide that helps students gain a strong grade in the essay component of the 2023–2025 Cambridge International AS & A Level Economics (9708) examination paper. With over 50 new model essays, the book provides a clear essay structure for each essay, various tips, and conceptual diagrams on basic economics concepts to give an overall insight into each subject topic.
Related collections
Author and book information , comment on this book.

Century of statistical ecology reviewed
Crunching numbers isn't exactly how Neil Gilbert, a postdoctoral researcher at Michigan State University, envisioned a career in ecology.
"I think it's a little funny that I'm doing this statistical ecology work because I was always OK at math, but never particularly enjoyed it," he explained. "As an undergrad, I thought, I'll be an ecologist -- that means that I can be outside, looking at birds, that sort of thing."
As it turns out," he chuckled, "ecology is a very quantitative discipline."
Now, working in the Zipkin Quantitative Ecology lab, Gilbert is the lead author on a new article in a special collection of the journal Ecology that reviews the past century of statistical ecology .
Statistical ecology, or the study of ecological systems using mathematical equations, probability and empirical data, has grown over the last century. As increasingly large datasets and complex questions took center stage in ecological research, new tools and approaches were needed to properly address them.
To better understand how statistical ecology changed over the last century, Gilbert and his fellow authors examined a selection of 36 highly cited papers on statistical ecology -- all published in Ecology since its inception in 1920.
The team's paper examines work on statistical models across a range of ecological scales from individuals to populations, communities, ecosystems and beyond. The team also reviewed publications providing practical guidance on applying models. Gilbert noted that because, "many practicing ecologists lack extensive quantitative training," such publications are key to shaping studies.
Ecology is an advantageous place for such papers, because it is one of, "the first internationally important journals in the field. It has played an outsized role in publishing important work," said lab leader Elise Zipkin, a Red Cedar Distinguished Associate Professor in the Department of Integrative Biology.
"It has a reputation of publishing some of the most influential papers on the development and application of analytical techniques from the very beginning of modern ecological research."
The team found a persistent evolution of models and concepts in the field, especially over the past few decades, driven by refinements in techniques and exponential increases in computational power.
"Statistical ecology has exploded in the last 20 to 30 years because of advances in both data availability and the continued improvement of high-performance computing clusters," Gilbert explained.
Included among the 36 reviewed papers were a landmark 1945 study by Lee R. Dice on predicting the co-occurrence of species in space -- Ecology's most highly cited paper of all time -- and an influential 2002 paper led by Darryl MacKenzie on occupancy models. Ecologists use these models to identify the range and distribution of species in an environment.
Mackenzie's work on species detection and sampling, "played an outsized role in the study of species distributions," says Zipkin. MacKenzie's paper, which was cited more than 5,400 times, spawned various software packages that are now widely used by ecologists, she explained.
- Environmental Issues
- Computer Modeling
- Mathematical Modeling
- Origin of Life
- Early Climate
- Artificial intelligence
- Computational genomics
- Albert Einstein
- Bioinformatics
- Mathematical model
- Water turbine
- Numerical weather prediction
Story Source:
Materials provided by Michigan State University . Original written by Caleb Hess. Note: Content may be edited for style and length.
Journal Reference :
- Neil A. Gilbert, Bruna R. Amaral, Olivia M. Smith, Peter J. Williams, Sydney Ceyzyk, Samuel Ayebare, Kayla L. Davis, Wendy Leuenberger, Jeffrey W. Doser, Elise F. Zipkin. A century of statistical Ecology . Ecology , 2024; DOI: 10.1002/ecy.4283
Cite This Page :
Explore More
- High-Efficiency Photonic Integrated Circuit
- Life Expectancy May Increase by 5 Years by 2050
- Toward a Successful Vaccine for HIV
- Highly Efficient Thermoelectric Materials
- Toward Human Brain Gene Therapy
- Whale Families Learn Each Other's Vocal Style
- AI Can Answer Complex Physics Questions
- Otters Use Tools to Survive a Changing World
- Monogamy in Mice: Newly Evolved Type of Cell
- Sustainable Electronics, Doped With Air
Trending Topics
Strange & offbeat.
- Subscribe to BBC Science Focus Magazine
- Previous Issues
- Future tech
- Everyday science
- Planet Earth
- Newsletters
New issue: Something is wrong with our model of the Universe
Although our picture of the cosmos is incredibly detailed, as the tools we build to explore the Universe become more and more sensitive, our understanding starts to grow fuzzy. There are crucial pieces missing... and without them, our model of the Universe just doesn't make sense. We take a dive into some of the biggest head-scratchers that are baffling astronomers.
Holly Spanner

Covert narcissism
Not all narcissists are loudmouth egotists, according to a growing body of science. Narcissists are lurking everywhere and it’s not always easy to spot them. But there are telltale signs...
Experts are calling for legal protections to safeguard scientifically significant lunar sites from being irreparably damaged.
Seagrass rescue
Seagrass, corals and kelp are crucial to Australia's marine ecosystems, but they're under threat. With the help of communities and traditional knowledge, scientists are striving to restore these underwater forests to protect carbon stocks and marine wildlife.
Sober curious?
More and more of us are thinking 'I should probably drink less.' But what is booze really doing to our bodies? Is going completely tee-total as worthwhile as people say? And what's the best way to change, if not end, our relationship with alcohol?
- Forever chemicals: What risks do the pesticides and other ‘forever chemicals’ in our food pose to our health?
- Hidden gadgets: Let’s be honest, tech can ruin a home’s aesthetic. Blocky speakers, large projector units and tangles of cables are unsightly. Tech companies are finally starting to realise this and find ways to hide them. We've rounded up our favourite hidden tech.
- Delaying menopause: The project that aims to predict when women will start their menopause, and find ways to delay it.
Issue 405 on sale 14 May 2024
Subscribe to bbc science focus magazine.
Don’t forget that BBC Science Focus is also available on all major digital platforms. We have versions for Android , Kindle Fire and Kindle e-reader , as well as an iOS app for the iPad and iPhone.
Share this article
Staff Writer, BBC Science Focus

- Terms & Conditions
- Privacy policy
- Cookies policy
- Code of conduct
- Magazine subscriptions
- Manage preferences

- About the LSE Impact Blog
- Comments Policy
- Popular Posts
- Recent Posts
- Subscribe to the Impact Blog
- Write for us
- LSE comment
Abdelghani Maddi
May 16th, 2024, the best peer review reports are at least 947 words.
0 comments | 17 shares
Estimated reading time: 6 minutes
Based on an analysis of the relationship between peer review reports and subsequent citations, Abdelghani Maddi argues that longer and hence more constructive and engaged peer review reports are closely associated with papers that are more cited.
Peer review continues to be the focus of considerable debate across academic fields as diverse as the sociology of science, economics and biology. This focus reflects a divide within the scientific community regarding its efficacy and role in identifying errors, and instances of scientific misconduct. In recent years it has been fueled by a proliferation of notable cases of errors and misconduct detected in articles published in prestigious international journals, including those published by Elsevier and Springer Nature. The rise of generative AI has again only heightened concerns due to its overt and covert use in peer review .
Nonetheless, there exists a consensus within the scientific community regarding the pivotal role of peer review in knowledge generation and dissemination. Despite uncertainties surrounding its ability to detect fraud and questionable practices, reviewers’ comments typically aim to provide constructive feedback aimed at enhancing the quality of papers, encompassing both structural and substantive aspects, including methodological validation and alignment to recognised publication standards.
However, as we found in a recent paper , quantifying the added value and tangible impact conferred by peer review on scientific publications remains challenging. The approach we opted for was to focus on the length of peer review reports. Our theory being that there is a direct correlation between the length of reports and the extent of revisions and modifications requested from authors. Hence, longer reports are, generally, associated with a higher likelihood of authors making revisions and enhancements to their papers, thereby bolstering their quality, visibility, and citation metrics.
We used data from Publons to extract information regarding the length of reviewers’ reports for a corpus of 57,482 publications. To ensure generalisability, the structure of the Publons database was adjusted with that of the Web of Science database using the Raking Ratio method, employing a control group comprising 12.3 million articles. Consequently, the weighted sample faithfully mirrors the overall database structure across disciplinary distribution, collaborative patterns, open access practices, among other variables.
papers garnering the highest citation counts tended to be associated with longer reviewer reports, exceeding the average length.
Our analyses revealed a statistically significant impact of reviewers’ report length on citations received, with reports surpassing approximately one and a half pages (947 words) marking a critical threshold. Notably, papers garnering the highest citation counts tended to be associated with longer reviewer reports, exceeding the average length. Beyond this threshold, citation counts exhibited an increasing trend with longer report lengths, corroborating the initial hypothesis positing the synonymous relationship between the length of referees’ reports and the extent of revisions solicited, thereby enhancing manuscript “quality”.

This finding highlights the role of reviewers in enhancing the quality of scientific publications. Their contribution extends far beyond mere minor revisions including spelling or grammatical corrections. Reviewers, who can be aptly termed the “unsung heroes” of scientific research, significantly contribute to improving publication quality by providing detailed and constructive reports, even within shrinking deadlines. Yet, their contribution remains largely unrecognised and underestimated.
Another salient aspect raised by the study is the importance of time for conducting thorough peer review. Journal editors need to acknowledge that furnishing useful, comprehensive reports entails a considerable investment of time and effort from reviewers. Soliciting evaluations within extremely short time frames, such as a week for reading the publication and drafting the report, as practiced by certain “gray” publishers, can compromise the quality of assessments and consequently diminish the value added by peer review to manuscripts. This points to the need to reconsider the emphasis on speed in response times for peer review.
Journal editors need to acknowledge that furnishing useful, comprehensive reports entails a considerable investment of time and effort from reviewers.
A third implication is related to the current saturation of the scientific publishing system , where the increasing number of submitted articles strains journals, reviewers, and the scientific community as a whole. This workload overload risks compromising the focus of reviewers, who must assess a large number of articles within short deadlines. This could lead to a risk of disseminating “bad science” if certain important aspects of an articles are not properly evaluated due to time constraints. Especially so in a context where the speed of peer review has become a sort of “advertising” argument wielded by some publishers to attract authors. Even though post-publication peer review allows for partial “correction” of errors and questionable practices, the volume of annual publications far exceeds the capacities of the active community to scrutinise all publications. The scientific community requires a pre-publication evaluation system that functions properly.
Finally, open peer review offers promising prospects for enhancing transparency and vigilance. According to one recent study the peer review model adopted by a journal has a direct influence on the behaviour of both researchers and reviewers. The study highlights that journals implementing open peer review protocols incentivise heightened engagement from researchers and reviewers, as their actions directly impact their reputations. In addition, by allowing access to reviewer reports and promoting the reuse of evaluation data, this approach could facilitate deeper and more quantitative analyses of the impact of peer review on the quality of scientific publications and the advancement of science in general. Thus, open peer review represents an opportunity to rethink and improve peer review practices in a context where the increasing quantity of publications necessitates a more efficient and transparent approach.
This post draws on the authors article, On the peer review reports: does size matter? , published in Scientometrics.
The content generated on this blog is for information purposes only. This Article gives the views and opinions of the authors and does not reflect the views and opinions of the Impact of Social Science blog (the blog), nor of the London School of Economics and Political Science. Please review our comments policy if you have any concerns on posting a comment below.
Image Credit: nampix on Shutterstock .

About the author

Abdelghani Maddi is a research engineer at GEMASS (CNRS/Sorbonne University). Economist by training specializing in Scientometrics. He is passionate about understanding scientific knowledge production, promoting open science, and improving research evaluation.
Leave a Reply Cancel reply
Your email address will not be published. Required fields are marked *
Notify me of follow-up comments by email.
Related Posts
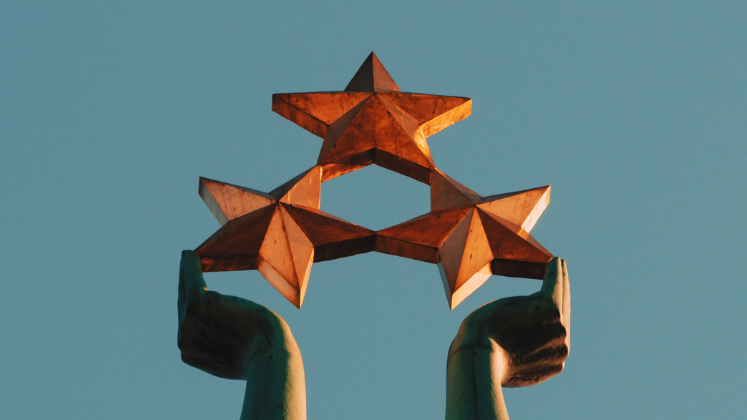
Can generative AI add anything to academic peer review?
September 26th, 2023.
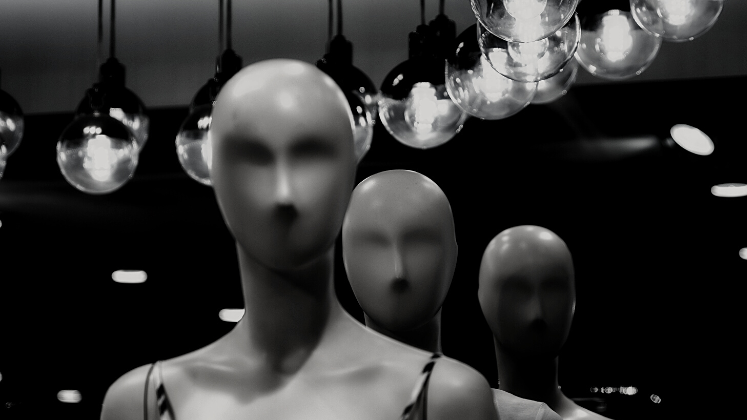
Double-anonymous review is an effective way of combating status bias in scholarly publishing
September 28th, 2023.
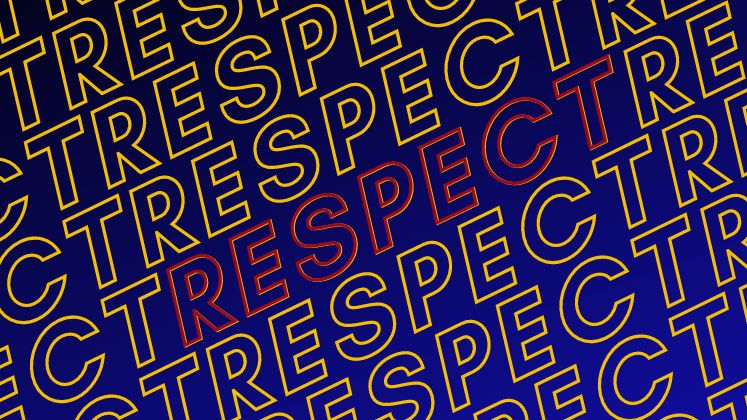
For Epistemic Respect – Against Reviewer 2
January 26th, 2023.

Reading Peer Review – What a dataset of peer review reports can teach us about changing research culture
March 31st, 2021.

Visit our sister blog LSE Review of Books
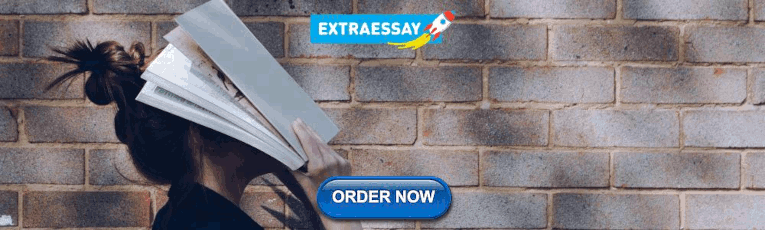
IMAGES
VIDEO
COMMENTS
This guide was inspired by Joshua Schimel's Writing Science: How to Write Papers that Get Cited and Proposals that Get Funded—an excellent book about scientific writing for graduate students and professional scientists—but designed to address undergraduate students. While the guide was written by a group of ecologists and evolutionary ...
Prototype Model Papers - O/L Exam: Prototype Model Papers Special thanks go to the : Colombo Zonal Education Office, State Ministry of Education Reforms, Promotion of Open Universities and Distance Learning , Nenasa TV , E thaksalawa, Sapelata Saviyak(WPEDU), & Department Of Education Central Province and Zonal Education Office - Matugama ...
Dr Peter Judge | Tutorial Essays for Science Subjects 1 Tutorial Essays for Science Subjects This guide is designed to provide help and advice on scientific writing. Although students studying Medical and Life Sciences are most likely to have to write essays for tutorials at Oxford, it is important all scientists
scholarship. There are models for different ways to organize an essay and tips to make sentences snap with style. Emphasis is placed on developing ideas in constant conversation with others and on strengthening papers through multiple rounds of revision. Jeffrey R. Wilson is a teacher-scholar at Harvard University. He is the
A science essay is an academic paper focusing on a scientific topic from physics, chemistry, biology, or any other scientific field. ... the traditional classroom model is undergoing a profound transformation. This essay explores the profound impact of technology on modern education, focusing on its ability to enhance learning experiences ...
Models are of central importance in many scientific contexts. The centrality of models such as inflationary models in cosmology, general-circulation models of the global climate, the double-helix model of DNA, evolutionary models in biology, agent-based models in the social sciences, and general-equilibrium models of markets in their respective domains is a case in point (the Other Internet ...
Scientific modelling. In science, a model is a representation of an idea, an object or even a process or a system that is used to describe and explain phenomena that cannot be experienced directly. Models are central to what scientists do, both in their research as well as when communicating their explanations.
Models of science aim to capture the structure and/or dynamics of science itself. Data on the science system - scholars, papers, patents, grants, jobs, etc., and their complex interdependencies and dynamics - are used to validate them. This chapter...
This article presents a comprehensive guide to understanding scientific models, indispensable tools in scientific research and technological innovation. It explores their types, uses, limitations, and evolution, shedding light on their critical role in diverse fields. By delving into the intricacies of these models, this guide aims to foster a ...
Figure 1. Example model for the formation of a cloud. To explain how clouds are made in this model, students use different colors and circle sizes to show changes as clouds form. Arrows indicate the process of changing air conditions. Words such as "Sun's Heat," "Cooling," "Rising," and "Condensing" help the reader understand ...
This question paper help to cover every unit in the school science textbook. Because we used the school textbook and O/L Science past papers to design this model paper. Then the students will have the opportunity to memorize the subject again and again. After writing the answer to this question paper you can check if your answers are correct.
This collection of papers contributes to the tradition of model-related research in education, and it does so in both the fields of pedagogy and epistemology. Hopefully the papers will deepen understanding and move the research field forward. The ubiquity of models in the history and current practice of science is widely recognised. In the past half-century historians and philosophers of ...
Example of a Great Essay | Explanations, Tips & Tricks. Published on February 9, 2015 by Shane Bryson . Revised on July 23, 2023 by Shona McCombes. This example guides you through the structure of an essay. It shows how to build an effective introduction, focused paragraphs, clear transitions between ideas, and a strong conclusion.
Philosophy, Science. Models v. Evidence. COVID-19 has revealed a contest between two competing philosophies of scientific knowledge. To manage the crisis, we must draw on both. Jonathan Fuller. COVID-19, Health, Science and Technology. May 5, 2020. Editors' Note: Read responses to this essay by the epidemiologists Marc Lipsitch and John ...
Google Scholar provides a simple way to broadly search for scholarly literature. Search across a wide variety of disciplines and sources: articles, theses, books, abstracts and court opinions.
This college essay tip is by Abigail McFee, Admissions Counselor for Tufts University and Tufts '17 graduate. 2. Write like a journalist. "Don't bury the lede!" The first few sentences must capture the reader's attention, provide a gist of the story, and give a sense of where the essay is heading.
Download 2023 G.C.E O/L Science Model Paper Sinhala Medium, available in PDF format. Prepared and Published by Ministry of Education, Sri Lanka. About US; ... 2024 G.C.E O/L Science Model Papers Sinhala Medium. April 9, 2024. 2023 Grade 11 3rd Term Test Papers Sinhala Medium - NWP. April 2, 2024.
Questions about the Model 3; Read the following essay, fill in the blanks with suitable cause-effect signal words and phrases. Pay attention to the sentence structure to help you decide. ... We also acknowledge previous National Science Foundation support under grant numbers 1246120, 1525057, and 1413739. Legal.
Download 2023 (2024) O/L Model Papers with answers as a PDF file. We Include the last three years OL model question papers developed by the Ministry of Education, Sri Lanka. These Model papers were prepared by well-experienced teachers, NIE, and Provincial Education Departments in Sri Lanka.
FCE Essays - Sample/model answers and examiner comments. An essay is always written for the teacher. It should answer the question given by addressing both content points and providinga new content point of the writer's own. The essay should be well organised, with an introduction and an appropriate conclusion,and should be written in an appropriate register and tone
Reach Us 12, Main AB Road, Bhawar Kuan, Indore, Madhya Pradesh, 452007 641, 1 st Floor, Mukherjee Nagar, Delhi-110009 ; 21, Pusa Rd, WEA, Karol Bagh, Delhi-110005
Mamba, an architecture with RNN-like token mixer of state space model (SSM), was recently introduced to address the quadratic complexity of the attention mechanism and subsequently applied to vision tasks. Nevertheless, the performance of Mamba for vision is often underwhelming when compared with convolutional and attention-based models. In this paper, we delve into the essence of Mamba, and ...
Economics: Model Essays is the 2nd edition of the best-selling revision guide that helps students gain a strong grade in the essay component of the 2023-2025 Cambridge International AS & A Level Economics (9708) examination paper. With over 50 new model essays, the book provides a clear essay structure for each essay, various tips, and conceptual diagrams on basic economics concepts to give ...
A special review examines highly-cited papers in statistical ecology. The review, which covers a century of research, details how models and concepts have evolved alongside increasing ...
Different models have its own advantages and limitations, such as the Priestley-Taylor model could be applied with less required inputs in potential ET estimation (Qiu et al., 2021). The Penman-Monteith (PM) model as a typical single-source model has been widely used (Li et al., 2016; Monteith, 1965; Xing et al., 2023, Zhou et al., 2019a ...
SEABIM® is a patented scan to BIM process that can generate a reliable and complete 3D model of a rubble-mound breakwater precast protective layer (Xbloc®, Accropode™, Core-loc™ etc.). Using computer vision techniques, the known 3D shape of the Concrete Armour Units (CAU) is detected in a high-resolution point cloud, which allows to obtain the position and orientation of each unit ...
Tutorial Essays for Science Subjects. It's important for scientists at all stages of their careers to be able to write clearly and concisely, to present their data and conclusions effectively. This guide is designed to provide you with some tips and advice as you write your first tutorial essays at Oxford.
As the tools we build to explore the Universe become more and more sensitive, our understanding starts to grow fuzzy. And although our picture of the cosmos is incredibly detailed, there are crucial pieces missing... and without them, our model of the Universe just doesn't make sense. We look at some of the biggest head-scratchers that are ...
papers garnering the highest citation counts tended to be associated with longer reviewer reports, exceeding the average length. Our analyses revealed a statistically significant impact of reviewers' report length on citations received, with reports surpassing approximately one and a half pages (947 words) marking a critical threshold ...