Organizers:
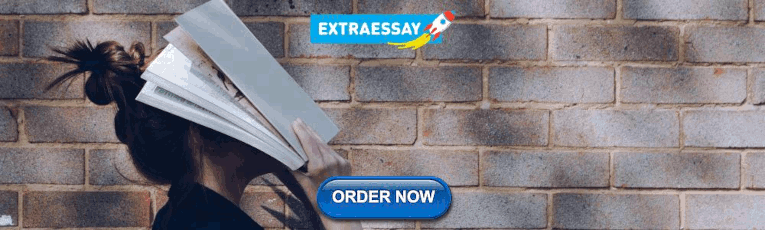
A Review on Deep Learning Approaches for 3D Data Representations in Retrieval and Classifications
Ieee account.
- Change Username/Password
- Update Address
Purchase Details
- Payment Options
- Order History
- View Purchased Documents
Profile Information
- Communications Preferences
- Profession and Education
- Technical Interests
- US & Canada: +1 800 678 4333
- Worldwide: +1 732 981 0060
- Contact & Support
- About IEEE Xplore
- Accessibility
- Terms of Use
- Nondiscrimination Policy
- Privacy & Opting Out of Cookies
A not-for-profit organization, IEEE is the world's largest technical professional organization dedicated to advancing technology for the benefit of humanity. © Copyright 2024 IEEE - All rights reserved. Use of this web site signifies your agreement to the terms and conditions.
Deep Learning Advances on Different 3D Data Representations: A Survey
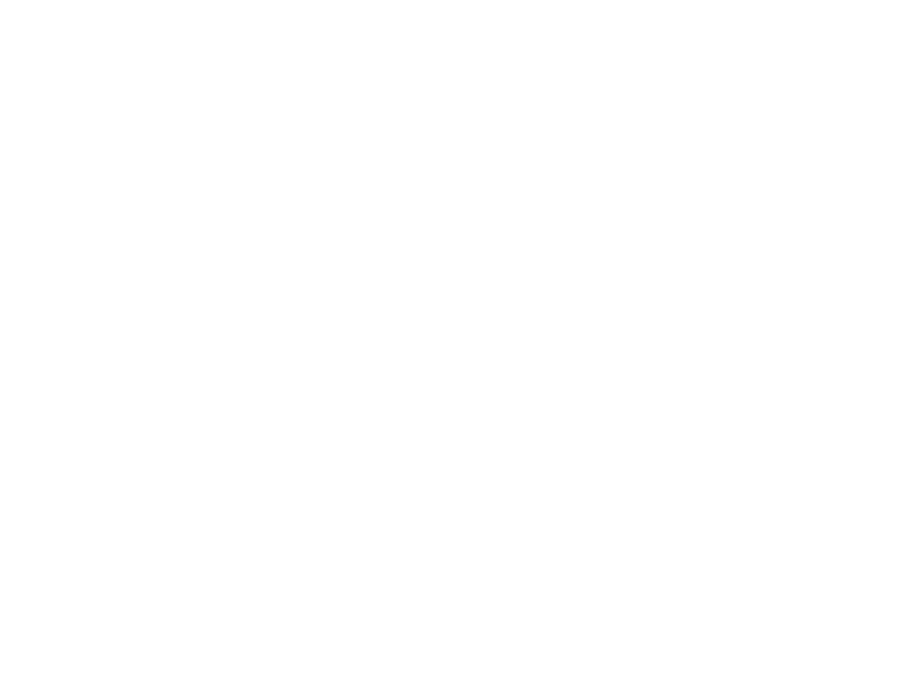
3D data is a valuable asset in the field of computer vision as it provides rich information about the full geometry of sensed objects and scenes. With the recent availability of large 3D datasets and the increase in computational power, it is today possible to consider applying deep learning to learn specific tasks on 3D data such as segmentation, recognition and correspondence. Depending on the considered 3D data representation, different challenges may be foreseen in using existent deep learning architectures. In this paper, we provide a comprehensive overview of various 3D data representations highlighting the difference between Euclidean and non-Euclidean ones. We also discuss how deep learning methods are applied on each representation, analyzing the challenges to overcome.

Alexandre Saint
Abd El Rahman Shabayek
Kseniya Cherenkova
Djamila Aouada
Björn Ottersten
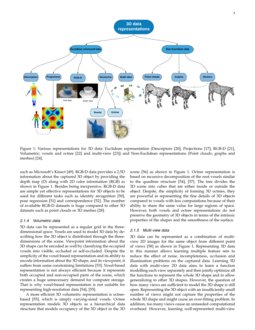
Related Research
Deep learning for scene classification: a survey, a survey on deep geometry learning: from a representation perspective, face recognition: from traditional to deep learning methods, hyperbolic deep learning in computer vision: a survey, deep neural networks and tabular data: a survey, recent advances on non-line-of-sight imaging: conventional physical models, deep learning, and new scenes, supporting future electrical utilities: using deep learning methods in ems and dms algorithms.
Please sign up or login with your details
Generation Overview
AI Generator calls
AI Video Generator calls
AI Chat messages
Genius Mode messages
Genius Mode images
AD-free experience
Private images
- Includes 500 AI Image generations, 1750 AI Chat Messages, 30 AI Video generations, 60 Genius Mode Messages and 60 Genius Mode Images per month. If you go over any of these limits, you will be charged an extra $5 for that group.
- For example: if you go over 500 AI images, but stay within the limits for AI Chat and Genius Mode, you'll be charged $5 per additional 500 AI Image generations.
- Includes 100 AI Image generations and 300 AI Chat Messages. If you go over any of these limits, you will have to pay as you go.
- For example: if you go over 100 AI images, but stay within the limits for AI Chat, you'll have to reload on credits to generate more images. Choose from $5 - $1000. You'll only pay for what you use.
Out of credits
Refill your membership to continue using DeepAI
Share your generations with friends
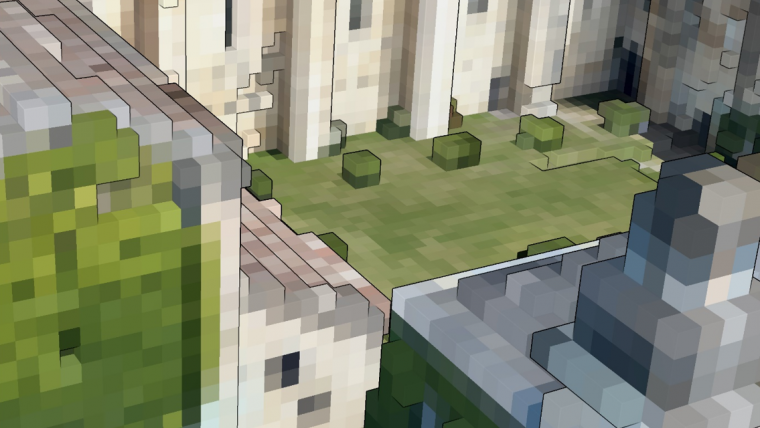
Representing 3D point cloud data
A visual guide
Figure 1: A 3D point cloud of an abbey acquired in 2014 using photogrammetry or Lidar.

Voxel-based models
Parametric model (cad).

Projections
Implicit representation.

- 3D point clouds are simple and efficient but lack connectivity
- 3D models such as 3D meshes, parametric models and voxel assemblies provide dedicated levels of additional information but approximate the base data
- Depth maps are well known and compact but essentially deal with 2.5D data
- Implicit representation encompasses all of the above and is beneficial for advanced processes that benefit from informative features that are difficult to represent visually
- Multi-view is complementary and leverages raster imagery but is prone to failure due to suboptimal viewpoint selection.
- Poux and R. Billen, Voxel-based 3D point cloud semantic segmentation: unsupervised geometric and relationship featuring vs deep learning methods, ISPRS International Journal of Geo-Information , vol. 8, no. 5, p. 213, May 2019.
- Poux, The Smart Point Cloud: Structuring 3D intelligent point data, Liège, 2019.
- Karara, R. Hajji, and F. Poux, 3D point cloud semantic augmentation: Instance segmentation of 360â—¦ panoramas by deep learning techniques, Remote Sensing , vol. 13, no. 18, p. 3647, Sep. 2021.

Value staying current with geomatics?
Stay on the map with our expertly curated newsletters.
We provide educational insights, industry updates, and inspiring stories to help you learn, grow, and reach your full potential in your field. Don't miss out - subscribe today and ensure you're always informed, educated, and inspired.
Latest Articles
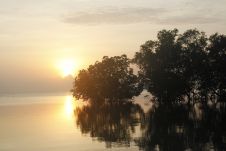
The case for natural capital accounting
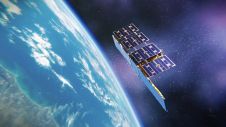
Why hasn’t the Earth observation industry taken off?
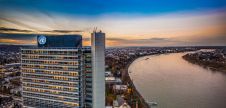
New UN organization to highlight the value of geodesy
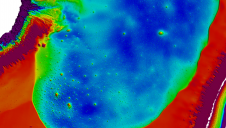
How can aerial surveying help to monitor climate change?
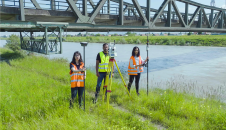
Rethinking service in the surveying industry
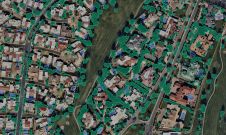
Mapping the mood of the geospatial community
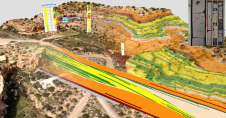
3D geospatial visualization
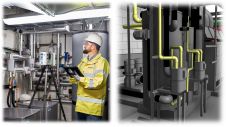
The era of smart manufacturing: why you need a good data strategy
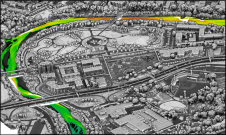
Harnessing topobathymetric Lidar for climate resilience
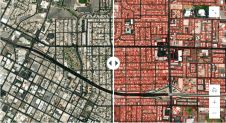
Today’s GIS technology puts multipurpose cadastre within reach
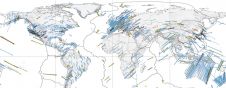
The importance of geodetic reference frames
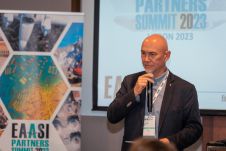
Review of the EAASI Partners Summit 2023: navigating the future of aerial surveying
Latest news.
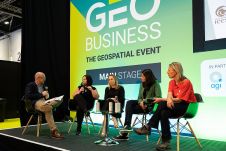
GEO Business 2024: unlocking geospatial innovation at ExCeL London
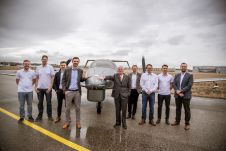
DA62 MPP SurveyStar delivered to RIEGL for calibration flights
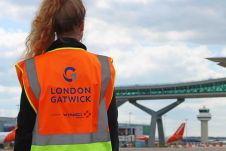
London Gatwick Airport builds geospatial platform with Esri GIS
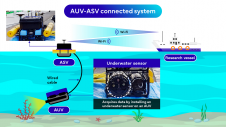
Fujitsu shaping tomorrow’s underwater world with pioneering technology
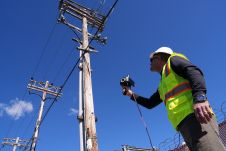
Looq AI launches AI-enabled digital twin platform
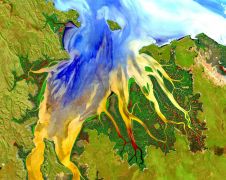
Australia joins US satellite initiative for Landsat 2030 international partnership
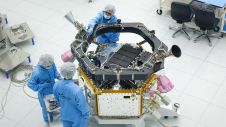
SIIS prepares for SpaceEye-T's high-resolution satellite launch
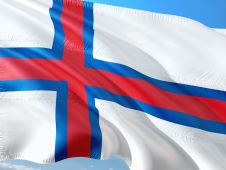
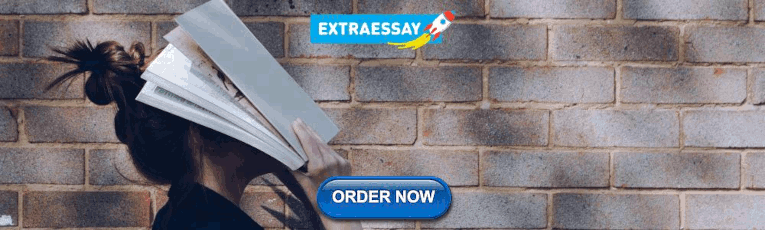
Faroese Environment Agency joins EuroGeographics
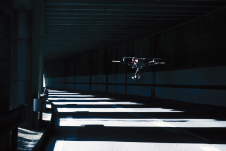
Inertial Labs partners with Sony for UAV-Lidar solution
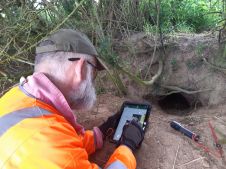
RSK Group supports company growth with enterprise GIS from Esri UK
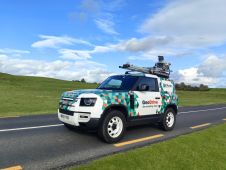
Woolpert acquires Murphy Geospatial
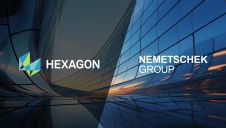
Nemetschek Group and Hexagon announce strategic collaboration
Download pdf or print.
Download this article as a print friendly PDF and receive our weekly overview of the most important geomatics news and insightful articles and case studies.
Already subscribed or no desire to subscribe to our newsletter? Skip this step and head to the download directly. By submitting this form, you agree to our Terms of Service and Privacy Policy .
Sharing this article
Ofcourse we encourage you to share this article with your peers if you enjoyed reading it. Copy the URL below or share it on your social media of choice.
This site uses cookies. By continuing to use this website, you agree to our Cookies Policy . Agree
MIT Libraries home DSpace@MIT
- DSpace@MIT Home
- MIT Libraries
- Doctoral Theses
Learning 3D Representations from Data

Terms of use
Date issued, collections.

1. Introduction
2. mathematical background.
- 3. Uniform and random samplings of SO(3)
4. Material textures and the square torus representation
5. discussion and conclusions.

research papers \(\def\hfill{\hskip 5em}\def\hfil{\hskip 3em}\def\eqno#1{\hfil {#1}}\)

Applications of the Clifford torus to material textures
a Department of Materials Science and Engineering, Carnegie Mellon University, Pittsburgh, PA 15213-3890, USA * Correspondence e-mail: [email protected]
This paper introduces a new 2D representation of the orientation distribution function for an arbitrary material texture. The approach is based on the isometric square torus mapping of the Clifford torus, which allows for points on the unit quaternion hypersphere (each corresponding to a 3D orientation) to be represented in a periodic 2D square map. The combination of three such orthogonal mappings into a single RGB (red–green–blue) image provides a compact periodic representation of any set of orientations. Square torus representations of five different orientation sampling methods are compared and analyzed in terms of the Riesz s energies that quantify the uniformity of the samplings. The effect of crystallographic symmetry on the square torus map is analyzed in terms of the Rodrigues fundamental zones for the rotational symmetry groups. The paper concludes with example representations of important texture components in cubic and hexagonal materials. The new RGB representation provides a convenient and compact way of generating training data for the automated analysis of material textures by means of neural networks.
Keywords: orientation distribution functions ; texture ; symmetry ; quaternions ; Clifford torus .
2.1. Definitions
Scaling the circle to a radius of ρ and taking the Cartesian product of two such circles results in the Clifford torus,
The Clifford torus has the special property that it is flat, i.e. there exists an isometry from the torus to a 2D square with periodic boundaries; the edges of the square have length 2 π and cover the interval [− π , π ]. The isometric mapping, which can be shown to have a unit Jacobian, consists of taking the ratios
and inverting the relations to the coordinates ( X , Y ) = ( θ , φ ) in the square,
2.2. Projection of unit quaternions onto the Clifford torus
The projected coordinates in the square torus are then readily shown to be given by
We can think of the three coordinate pairs as three different isometric projections of an orientation onto three orthogonal square tori. We will label the square tori by their coordinate symbols; when no coordinate label is present, the ( X , Z Y ) projection will be assumed. In terms of the Rodrigues–Frank vector components, the cyclic permutations correspond to 120° rotations about the principal diagonal axis of the Rodrigues reference frame.
2.3. Relation between the square torus map and the Euler angle representation
This means that the ( Z , Y X ) square torus map is identical to a projection of Euler space along the Φ axis followed by a 45° rotation, bringing the φ 1 = φ 2 diagonal parallel to the Y X axis of the square torus map. The two other maps, ( X , Z Y ) and ( Y , X Z ), do not appear to have simple interpretations in terms of linear projections through Euler space; they are more complicated nonlinear projections.
2.4. Zone-plate function representation
with 〈 p , q 〉 the standard dot product between two quaternions projected onto the Clifford torus. q f is an arbitrary point on the torus, so that the zone-plate function uses the geodesic distance between q and q f along the surface of the torus. In this paper, we select the reference point
3. Uniform and random samplings of SO (3)
In this section, we explore a number of different orientation sampling approaches and their representation on the square torus using a zone-plate function. The following sampling approaches are used to generate orientation sets:
4.1. Fundamental zone representations
4.1.1. cyclic point-group symmetry, 4.1.2. dihedral, tetrahedral and octahedral point-group symmetry.
A few general trends can be observed in the zone plates for the four dihedral groups:
4.2. Basic texture-type representations
4.3. experimental texture representations.
corresponding to the Rodrigues vector
4.4. Fiber textures
4.4.1. f.c.c. fibers.
Consider the α fiber in an f.c.c. material. Its orientations are located around the line ( φ 1 , π /4, π /2) in Euler space, with φ 1 ∈ [0, π /2]. The corresponding unit quaternions are obtained by setting
4.4.2. B.c.c. fibers
Consider the α , γ and ε fibers in a b.c.c. material. In Euler space, all orientations lie along the following lines:
After conversion to the square torus coordinates, we find that the α fiber is represented by the curve
between the points ( X , Y ) = (0, − π /2) for Φ = 0 and (− π /4, − π /4) for Φ = π /2.
For the ε fiber we find
The γ fiber sits in between the two curves and is represented by
The intersection points of the γ fiber with the α and ε fibers have coordinates
for the α fiber and
4.5. Experimental fiber texture example
Different from more conventional 3D representations of material textures, the RGB square torus map representation opens a unique path to the use of neural networks to automate the analysis of material textures, in particular to determine the mixture of texture components that are present in the orientation distribution. The use of ST maps in this context is the topic of ongoing investigations.
Acknowledgements
The author would like to acknowledge stimulating discussions with A. D. Rollet, M. P. Echlin, T. M. Pollock, S. Wright, W. Lenthe, D. Rowenhorst, B. Hutchinson, C. Lafond, G. Austin and S. Niezgoda.
Funding information
The author acknowledges financial support from the National Science Foundation, Directorate for Mathematical and Physical Sciences (grant No. DMR-2203378), and the use of the computational resources of the Materials Characterization Facility at Carnegie Mellon University (grant No. MCF-677785). The author also acknowledges support from the John and Claire Bertucci Distinguished Professorship in Engineering.
This is an open-access article distributed under the terms of the Creative Commons Attribution (CC-BY) Licence , which permits unrestricted use, distribution, and reproduction in any medium, provided the original authors and source are cited.
Help | Advanced Search
Computer Science > Computer Vision and Pattern Recognition
Title: compgs: efficient 3d scene representation via compressed gaussian splatting.
Abstract: Gaussian splatting, renowned for its exceptional rendering quality and efficiency, has emerged as a prominent technique in 3D scene representation. However, the substantial data volume of Gaussian splatting impedes its practical utility in real-world applications. Herein, we propose an efficient 3D scene representation, named Compressed Gaussian Splatting (CompGS), which harnesses compact Gaussian primitives for faithful 3D scene modeling with a remarkably reduced data size. To ensure the compactness of Gaussian primitives, we devise a hybrid primitive structure that captures predictive relationships between each other. Then, we exploit a small set of anchor primitives for prediction, allowing the majority of primitives to be encapsulated into highly compact residual forms. Moreover, we develop a rate-constrained optimization scheme to eliminate redundancies within such hybrid primitives, steering our CompGS towards an optimal trade-off between bitrate consumption and representation efficacy. Experimental results show that the proposed CompGS significantly outperforms existing methods, achieving superior compactness in 3D scene representation without compromising model accuracy and rendering quality. Our code will be released on GitHub for further research.
Submission history
Access paper:.
- HTML (experimental)
- Other Formats
References & Citations
- Google Scholar
- Semantic Scholar
BibTeX formatted citation

Bibliographic and Citation Tools
Code, data and media associated with this article, recommenders and search tools.
- Institution
arXivLabs: experimental projects with community collaborators
arXivLabs is a framework that allows collaborators to develop and share new arXiv features directly on our website.
Both individuals and organizations that work with arXivLabs have embraced and accepted our values of openness, community, excellence, and user data privacy. arXiv is committed to these values and only works with partners that adhere to them.
Have an idea for a project that will add value for arXiv's community? Learn more about arXivLabs .
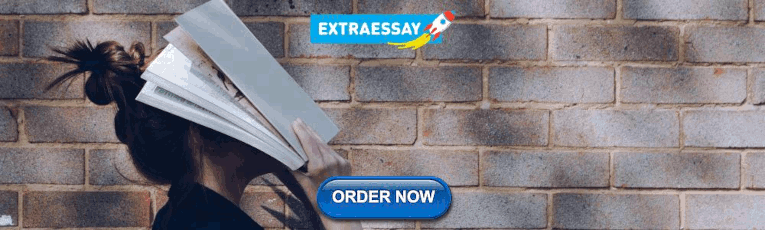
IMAGES
VIDEO
COMMENTS
One of the biggest differences between 2D and 3D deep learning is the data representation format. Regular images are typically represented in 1D or 2D arrays. 3D images, on the other hand, can have different representation formats and here are a few most popular ones: multi-view, volumetric, point cloud, mesh and volumetric display. ...
The second type of 3D data representation is non-Euclidean data. Unlike Euclidean data, it doesn't have a global parametrization or a common coordinate system.
The representation of 3D data is the foundation of a number of important applications such as computer-aided geometric design, visualisation and graphics. In this section, we summarise various 3D representations which we classify as raw data (i.e. that is delivered by a 3D sensing device), surfaces (i.e. 2D manifolds embedded in 3D space) and ...
This tutorial covers deep learning algorithms that analyze or synthesize 3D data. Different from 2D images that have a dominant representation as pixel arrays, 3D data possesses multiple popular representations, such as point cloud, mesh, volumetric field, multi-view images and parametric models, each fitting their own application scenarios.
The choice of a data structure to represent the 3D geometry is therefore crucial as it will determine the type of algorithms that can be employed in order to learn from 3D data. This is the reason why, before looking at machine learning model architectures for 3D data, it is important to understand the pros and cons of each 3D data representation.
Recently, researchers started to shift focus from 2D to 3D space, considering that 3D data is more closely aligned with our physical world and holds immense practical potential. However, unlike 2D images, which possess an inherent and efficient representation (\textit{i.e.}, a pixel grid), representing 3D data poses significantly greater ...
One of the major challenges to develop deep learning models for 3D data is its representation issue. Unlike images that have a dominant representation as 2D pixel arrays, 3D has many popular representations, as shown in Fig. 11.1. In choosing or designing a deep learning model we need to first determine which 3D representation to use.
on the considered 3D data representation, different challenges may be foreseen in using existent deep learning architectures. In this paper, we provide a comprehensive overview of various 3D data representations highlighting the difference between Euclidean and non-Euclidean ones. We also discuss how deep learning methods are applied on each ...
Based on the categorization of the different 3D data representations proposed in this paper, the importance of choosing a suitable 3D data representation which depends on simplicity, usability, and efficiency has been highlighted. Furthermore, the origin and contents of the major 3D datasets were discussed in detail.
1.1.2 3D data projections. Projecting 3D data into another 2D space is another representation for raw 3D data where the projection converts the 3D object into a 2D grid with specific features. The projected data encapsulates some of the key properties of the original 3D shape. The type of preserved features is dependent on the type of projection.
In computer vision, various 3D data representations are used to understand spatial environments and objects, combining mathematical principles, machine learning, and computer vision. Point cloud ...
With the recent availability of large 3D datasets and the increase in computational power, it is today possible to consider applying deep learning to learn specific tasks on 3D data such as segmentation, recognition and correspondence. Depending on the considered 3D data representation, different challenges may be foreseen in using existent ...
🥸3D data representation Selecting the right data representation is a critical decision when working with 3D deep learning systems. Different representations offer various advantages and challenges.
Various 3D data representation. With the latest advances in 3D sensing technologies and the increased availability of affordable 3D data acquisition devices such as structured-lighted 3D scanners ...
3D mesh. A mesh is a geometric data structure that allows the representation of surface subdivisions by a set of polygons. Meshes are mainly used in computer graphics to represent surfaces, or in modelling to discretize a continuous or implicit surface. A mesh is made up of vertices (or a vertex), connected by edges making faces (or facets) of ...
In research of 3D deep learning, 3D data possesses multiple popular representations, such as multi-view images, point cloud, and Voxel-based occupancy grid representation [30]. For the voxel-based ...
Finally, 3D annotations are scarce and hard to obtain. Annotating 3D data usually requires more human effort, which hinders supervised learning from 3D data. Therefore, learning 3D representations from data remains challenging and demands further study. This thesis investigates how to learn representations from 3D data efficiently and effectively.
A survey on Deep Learning Advances on Different 3D Data Representations. Eman Ahmed, Alexandre Saint, Abd El Rahman Shabayek, Kseniya Cherenkova, Rig Das, Gleb Gusev, Djamila Aouada, Bjorn Ottersten. 3D data is a valuable asset the computer vision filed as it provides rich information about the full geometry of sensed objects and scenes.
3D data visualization provides a more intuitive and comprehensive representation of complex data sets. By visualizing data in three dimensions, users can perceive spatial relationships, patterns, and correlations that might be challenging to identify in traditional 2D representations, enabling deeper insights and understanding of data ...
Abstract. Many people interact with scientific data by means of 2D or 3D representations such as scatterplots. In this thesis we study mechanisms for simplifying this interaction process. First, we propose a method that allows users to easily rotate, with a high degree of control, complex 3D shapes to inspect them from specific viewpoints.
II. 3D DATA REPRESENTATIONS. 3D data provides rich information about the geometry of the object, hence their adequate representation is of significant importance for computer vision tasks. Because ...
The 3D representation learning method uses cloud points for 3D understanding of the object, and this field has been explored by developers a lot in the recent past, and it has been observed that these cloud points can be pre-trained under self-supervision using specific 3D pretext tasks including mask point modeling, self-reconstruction, and ...
While recent advances in 3D-aware Generative Adversarial Networks (GANs) have aided the development of near-frontal view human face synthesis, the challenge of comprehensively synthesizing a full 3D head viewable from all angles still persists. Although PanoHead proves the possibilities of using a large-scale dataset with images of both frontal and back views for full-head synthesis, it often ...
The 3D organisation of the genome provides an intricate relationship between the chromatin architecture and its effects on the functional state of the cell. Recent advances in high-throughput sequencing and chromosome conformation capture technologies elucidated a comprehensive view of chromatin interactions on a genome-wide scale but provides only a 2D representation of how the chromatin is ...
This paper introduces a new 2D representation of the orientation distribution function for an arbitrary material texture. The approach is based on the isometric square torus mapping of the Clifford torus, which allows for points on the unit quaternion hypersphere (each corresponding to a 3D orientation) to be represented in a periodic 2D square map.
Gaussian splatting, renowned for its exceptional rendering quality and efficiency, has emerged as a prominent technique in 3D scene representation. However, the substantial data volume of Gaussian splatting impedes its practical utility in real-world applications. Herein, we propose an efficient 3D scene representation, named Compressed Gaussian Splatting (CompGS), which harnesses compact ...