
An official website of the United States government
The .gov means it’s official. Federal government websites often end in .gov or .mil. Before sharing sensitive information, make sure you’re on a federal government site.
The site is secure. The https:// ensures that you are connecting to the official website and that any information you provide is encrypted and transmitted securely.
- Publications
- Account settings
Preview improvements coming to the PMC website in October 2024. Learn More or Try it out now .
- Advanced Search
- Journal List
- HHS Author Manuscripts

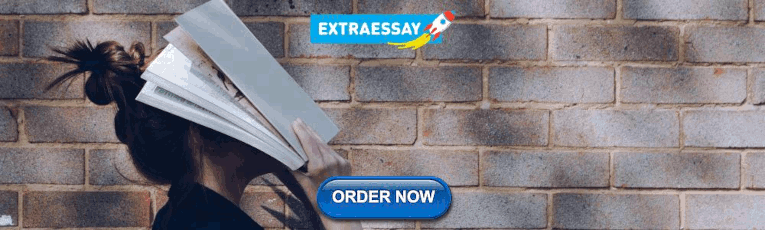
Know It When You See It: Identifying and Using Special Cause Variation for Quality Improvement
In this month’s Hospital Pediatrics , Liao et al 1 share their team’s journey to improve the accuracy of their institution’s electronic health record (EHR) problem list. They presented their results as statistical process control (SPC) charts, which are a mainstay for visualization and analysis for improvers to understand processes, test hypotheses, and quickly learn their interventions’ effectiveness. Although many readers might understand that 8 consecutive points above or below the mean signifies special cause variation resulting in a centerline “shift,” there are many more special cause variation rules revealed in these charts that likely provided valuable real-time information to the improvement team. These “signals” might not be apparent to casual readers when looking at the complete data set in article form.
Shewhart 2 first introduced SPC charts to the world with the publication of Economic Control of Quality of Manufactured Product in 1931. Although control charts were initially used more broadly in industrial settings, health care providers have also recently begun to understand that the use of SPC charts is vital in improvement work. 3 , 4 Deming, 5 often seen as the “grandfather” of quality improvement (QI), saw SPC charts as vital to understanding variation as part of his well-known Theory of Profound Knowledge, outlined in his book The New Economics for Industry Government, Education . Improvement science harnesses the scientific method in which improvers create and rapidly test hypotheses and learn from their data to determine if their hypotheses are correct. 6 This testing is central to the Model for Improvement’s plan-do-study-act cycle. 3 Liao et al 1 nicely laid out their hypotheses in a key driver diagram, and they tested these hypotheses with multiple interventions. In the following paragraphs, we will walk through some of their SPC charts to demonstrate how this improvement team was gaining valuable knowledge about their hypotheses through different types of special cause variation long before they had 8 points to reveal shifts. We recommend readers have the charts from the original article (OA) available for reference.
A fundamental concept in improvement science is understanding the difference between common cause and special cause variation. By understanding how to apply these concepts to your data, you will more quickly identify when a change has occurred and whether action should be taken. The authors’ SPC charts reveal examples of both common cause and special cause variation.
Common cause variations are those causes that are inherent in the system or process. 4 Evidence of common cause variation can be seen visually in the OA’s Fig 3, from January 2017 to October 2017, because the data points vary around the mean but remain between the upper and lower control limits (dotted lines). In contrast, special cause variations are causes of variations that are not inherent to the system. 4 Although there are different rules that signify special cause variation in SPC charts, some of the most common rules that we will focus on here include (1) a single data point outside of the control limits, (2) 8 consecutive points above or below the mean line, and (3) ≥6 consecutive points all moving in the same direction, termed a “trend.” 4 When any of these occur, it is paramount to identify when and why the special cause occurred, learn from the special cause, and then take appropriate action. By quickly detecting special cause variation, improvement teams can more readily assess the impact of interventions by validating whether their hypothesis for improvement is correct.
An example of special cause variation can be seen in the OA’s Fig 2, noted by the shift in the centerline in May 2018 from a baseline of 70% of problem lists revised during admission to a new centerline of 90% of problem lists reviewed during admission. Notice that this new, stable process represented by the new centerline starts after the team tested 3 separate interventions that were directly testing hypotheses related to their key drivers. Although the shift began in May 2018, the first special cause signal the improvement team would have seen is the first point outside of the upper control limit in January 2018, which comes immediately after their first 2 interventions. As more months go by, each month after continues to represent special cause variation because they are outside of the control limits. Finally, when the data point in May 2018 is plotted, it is apparent that an upward trend started in December 2017, with 6 consecutive data points increasing through May 2018. Therefore, the authors recognized special cause variation (a trend) by having 6 consecutive increasing points. Given their interventions were grounded in theory and the temporal relationship of the trend beginning in December 2017, with the preceding interventions in November and December 2017, there is a high degree of belief that the interventions are driving these results. In other words, their hypothesis that the EHR enhancements, the dissemination of a protocol, and the designation of a bonus would improve the percentage of times that the problem list is “reviewed” was confirmed as early as December 2017, long before the eventual centerline shift in May 2018.
Figure 3 in the OA is an SPC chart of one of the team’s process measures revealing the percentage of discharges with duplicate codes on the problem list. The authors demonstrate that the November 2017 EHR impacted the process, reducing the mean from 12% to 7%. The data contained in our Fig 1 are the same data as those shown in Fig 3 of the authors’ OA but without the first centerline shift, which reveals what the authors would have seen in real time during the course of their improvement efforts. With the November 2017 data point (labeled point 1 in Fig 1 ), the authors immediately have evidence of special cause variation, with a point outside of the lower control limit after their intervention. This continues with points 2 through 5, each below the lower control limit. Statistically speaking, any one of these is unlikely to happen by chance (which is why they are considered special cause), but the fact that the team is seeing this month after month reinforced their hypothesis. With the eighth consecutive point below the mean line occurring in June 2018 (circle), the team was able to finally shift the centerline. Looking at this from the perspective of the improvement team, the immediacy and consistency of feedback that they witnessed with points outside of the control limits from November 2017 through March 2018 were likely much more informative to their improvement efforts than the moment when they finally were able to shift the mean line. The authors highlight that the EHR enhancement was chosen for its higher reliability design concept, 7 making it easier for the providers to complete the intended behavior. The immediacy of special cause signal in November 2017 would indicate that their hypothesis was correct.

OA Fig 3 re-designed to represent data visualization prior to centerline shift.
Finally, viewing charts in combination provides further support of the team’s overall improvement theory. Notice that the special cause shift in Fig 3 of the OA (a process measure) occurs at the same time as the beginning of the special cause that is noted in Fig 2 of the OA, which is their outcome measure. In this case, a driving change in their process was temporally associated with recognizable change in their outcome. Similarly, the OA’ Fig 4, viewed in combination with its Figs 2 and 3, provide our final example of how revealing special cause variation across measures relates to the broader theory of the team’s improvement. Special cause variation is evident in Fig 4 of the OA, with points outside of the control limits associated with interventions in both November and December 2019. A similar pattern is seen in the authors’ other process measure chart, Fig 3 of the OA, during those same months associated with those interventions. Here, a couple of associations are addressed in the data. First, a high degree of belief that those two interventions affect those measures is provided in the data, as the authors hypothesized. Second, with such data, the authors also confirm the hypothesis that underlies the entire article: simply “reviewing” the problem list is also associated with active management of the problem list, and improvements to their process measures help drive their outcome. After >1.5 years of a fairly stable outcome measure (mainly common cause variation), the team’s use of these two interventions not only improved their process measures but also were associated with the December 2019 data point being outside of the control limits in the outcome measure in Fig 2 of the OA. In these situations, the team’s use of SPC charts provided the ability to understand relationships between process and outcome measures, in addition to rapidly testing hypotheses.
As revealed in the work by Liao et al, 1 we can improve the care we provide to patients every day with QI methodology. When researchers use SPC charts to report QI in scholarly venues such as this, readers often focus on centerline shifts. Although improvement teams take great joy in shifting a centerline, experienced teams much more commonly work to detect other types of special cause variation quickly to test their hypotheses and work through plan-do-study-act cycles. By understanding the rules of special cause variation and applying them to data in real time, teams will be provided with information that will inform hypotheses testing, bolster knowledge about a system, and ultimately accelerate improvement work.
Acknowledgments
FUNDING: Supported by the Agency for Healthcare Research and Quality (grant T32HS026122). The content is solely the responsibility of the authors and does not necessarily represent the official views of the Agency for Healthcare Research and Quality.
FINANCIAL DISCLOSURE: The authors have indicated they have no financial relationships relevant to this article to disclose.
POTENTIAL CONFLICT OF INTEREST: The authors have indicated they have no potential conflicts of interest to disclose.
Volume 8 Supplement 1
Proceedings of Advancing the Methods in Health Quality Improvement Research 2012 Conference
- Proceedings
- Open access
- Published: 19 April 2013
Understanding and managing variation: three different perspectives
- Michael E Bowen 1 , 2 , 3 &
- Duncan Neuhauser 4
Implementation Science volume 8 , Article number: S1 ( 2013 ) Cite this article
28k Accesses
4 Citations
13 Altmetric
Metrics details
Presentation
Managing variation is essential to quality improvement. Quality improvement is primarily concerned with two types of variation – common-cause variation and special-cause variation. Common-cause variation is random variation present in stable healthcare processes. Special-cause variation is an unpredictable deviation resulting from a cause that is not an intrinsic part of a process. By careful and systematic measurement, it is easier to detect changes that are not random variation.
The approach to managing variation depends on the priorities and perspectives of the improvement leader and the intended generalizability of the results of the improvement effort. Clinical researchers, healthcare managers, and individual patients each have different goals, time horizons, and methodological approaches to managing variation; however, in all cases, the research question should drive study design, data collection, and evaluation. To advance the field of quality improvement, greater understanding of these perspectives and methodologies is needed [ 1 ].
Clinical researcher perspective
The primary goal of traditional randomized controlled trials (RCTs) (ie a comparison of treatment A versus placebo) is to determine treatment or intervention efficacy in a specified population when all else is equal. In this approach, researchers seek to maximize internal validity. Through randomization, researchers seek to balance variation in baseline factors by randomizing patients, clinicians, or organizations to experimental and control groups. Researchers may also increase understanding of variation within a specific study using approaches such as stratification to examine for effect modification. Although the generalizability of outcomes in all research designs is limited by the study population and setting, this can be particularly challenging in traditional RCTs. When inclusion criteria are strict, study populations are not representative of “real world” patients, and the applicability of study findings to clinical practice may be unclear. Traditional RCTs are limited in their ability to evaluate complex processes that are purposefully and continually changing over time because they evaluate interventions in rigorously controlled conditions over fixed time frames [ 2 ]. However, using alternative designs such as hybrid, effectiveness studies discussed in these proceedings or pragmatic RCTs, researchers can rigorously answer a broader range of research questions [ 3 ].
Healthcare manager perspective
Healthcare managers seek to understand and reduce variation in patient populations by monitoring process and outcome measures. They utilize real-time data to learn from and manage variation over time. By comparing past, present, and desired performance, they seek to reduce undesired variation and reinforce desired variation. Additionally, managers often implement best practices and benchmark performance against them. In this process, efficient, time-sensitive evaluations are important. Run charts and Statistical Process Control (SPC) methods leverage the power of repeated measures over time to detect small changes in process stability and increase the statistical power and rapidity with which effects can be detected [ 1 ].
Patient perspective
While the clinical researcher and healthcare manager are interested in understanding and managing variation at a population level, the individual patient wants to know if a particular treatment will allow one to achieve health outcomes similar to those observed in study populations. Although the findings of RCTs help form the foundation of evidence-based practice and managers utilize these findings in population management, they provide less guidance about the likelihood of an individual patient achieving the average benefits observed across a population of patients. Even when RCT findings are statistically significant, many trial participants receive no benefit. In order to understand if group RCT results can be achieved with individual patients, a different methodological approach is needed. “N-of-1 trials” and the longitudinal factorial design of experiments allow patients and providers to systematically evaluate the independent and combined effects of multiple disease management variables on individual health outcomes [ 4 ]. This offers patients and providers the opportunity to collect, analyze, and understand data in real time to improve individual patient outcomes.
Advancing the field of improvement science and increasing our ability to understand and manage variation requires an appreciation of the complementary perspectives held and methodologies utilized by clinical researchers, healthcare managers, and patients. To accomplish this, clinical researchers, healthcare managers, and individual patients each face key challenges.
Recommendations
Clinical researchers are challenged to design studies that yield generalizable outcomes across studies and over time. One potential approach is to anchor research questions in theoretical frameworks to better understand the research problem and relationships among key variables. Additionally, researchers should expand methodological and analytical approaches to leverage the statistical power of multiple observations collected over time. SPC is one such approach. Incorporation of qualitative research and mixed methods can also increase our ability to understand context and the key determinants of variation.
Healthcare managers are challenged to identify best practices and benchmark their processes against them. However, the details of best practices and implementation strategies are rarely described in sufficient detail to allow identification of the key drivers of process improvement and adaption of best practices to local context. By advocating for transparency in process improvement and urging publication of improvement and implementation efforts, healthcare managers can enhance the spread of best practices, facilitate improved benchmarking, and drive continuous healthcare improvement.
Individual patients and providers are challenged to develop the skills needed to understand and manage individual processes and outcomes. As an example, patients with hypertension are often advised to take and titrate medications, modify dietary intake, and increase activity levels in a non-systematic manner. The longitudinal factorial design offers an opportunity to rigorously evaluate the impact of these recommendations, both in isolation and in combination, on disease outcomes [ 1 ]. Patients can utilize paper, smart phone applications, or even electronic health record portals to sequentially record their blood pressures. Patients and providers can then apply simple SPC rules to better understand variation in blood pressure readings and manage their disease [ 5 ].
As clinical researchers, healthcare managers, and individual patients strive to improve healthcare processes and outcomes, each stakeholder brings a different perspective and set of methodological tools to the improvement team. These perspectives and methods are often complementary such that it is not which methodological approach is “best” but rather which approach is best suited to answer the specific research question. By combining these perspectives and developing partnerships with organizational managers, improvement leaders can demonstrate process improvement to key decision makers in the healthcare organization. It is through such partnerships that the future of quality improvement research is likely to find financial support and ultimate sustainability.
Neuhauser D, Provost L, Bergman B: The meaning of variation to healthcare managers, clinical and health-services researchers, and individual patients. BMJ Qual Saf. 2011, 20 (Suppl 1): i36-40. 10.1136/bmjqs.2010.046334.
Article PubMed Central PubMed Google Scholar
Neuhauser D, Diaz M: Quality improvement research: are randomised trials necessary?. Qual Saf Health Care. 2007, 16: 77-80. 10.1136/qshc.2006.021584.
Article PubMed Central CAS PubMed Google Scholar
Eccles M, Grimshaw J, Campbell M, Ramsay C: Research designs for studies evaluating the effectiveness of change and improvement strategies. Quality and Safety in Health Care. 2003, 12: 47-52. 10.1136/qhc.12.1.47.
Olsson J, Terris D, Elg M, Lundberg J, Lindblad S: The one-person randomized controlled trial. Qual Manag Health Care. 2005, 14: 206-216.
Article PubMed Google Scholar
Hebert C, Neuhauser D: Improving hypertension care with patient-generated run charts: physician, patient, and management perspectives. Qual Manag Health Care. 2004, 13: 174-177.
Download references
Author information
Authors and affiliations.
VA National Quality Scholars Fellowship, Tennessee Valley Healthcare System, Nashville, Tennessee, 37212, USA
Michael E Bowen
Division of General Internal Medicine, Department of Medicine, University of Texas Southwestern Medical Center, Dallas, Texas, 75390, USA
Division of Outcomes and Health Services Research, Department of Clinical Sciences, University of Texas Southwestern Medical Center, Dallas, Texas, 75390, USA
Department of Epidemiology and Biostatistics, Case Western Reserve University, Cleveland, Ohio, 44106, USA
Duncan Neuhauser
You can also search for this author in PubMed Google Scholar
Corresponding author
Correspondence to Michael E Bowen .
Rights and permissions
This article is published under license to BioMed Central Ltd. This is an Open Access article distributed under the terms of the Creative Commons Attribution License ( http://creativecommons.org/licenses/by/2.0 ), which permits unrestricted use, distribution, and reproduction in any medium, provided the original work is properly cited.
Reprints and permissions
About this article
Cite this article.
Bowen, M.E., Neuhauser, D. Understanding and managing variation: three different perspectives. Implementation Sci 8 (Suppl 1), S1 (2013). https://doi.org/10.1186/1748-5908-8-S1-S1
Download citation
Published : 19 April 2013
DOI : https://doi.org/10.1186/1748-5908-8-S1-S1
Share this article
Anyone you share the following link with will be able to read this content:
Sorry, a shareable link is not currently available for this article.
Provided by the Springer Nature SharedIt content-sharing initiative
- Statistical Process Control
- Clinical Researcher
- Healthcare Manager
- Healthcare Process
- Quality Improvement Research
Implementation Science
ISSN: 1748-5908
- Submission enquiries: Access here and click Contact Us
- General enquiries: [email protected]
Table of Contents
Types of variance, common cause variation, common cause variation examples, special cause variation, special cause variation example, choose the right program, common cause variation vs. special cause variation.
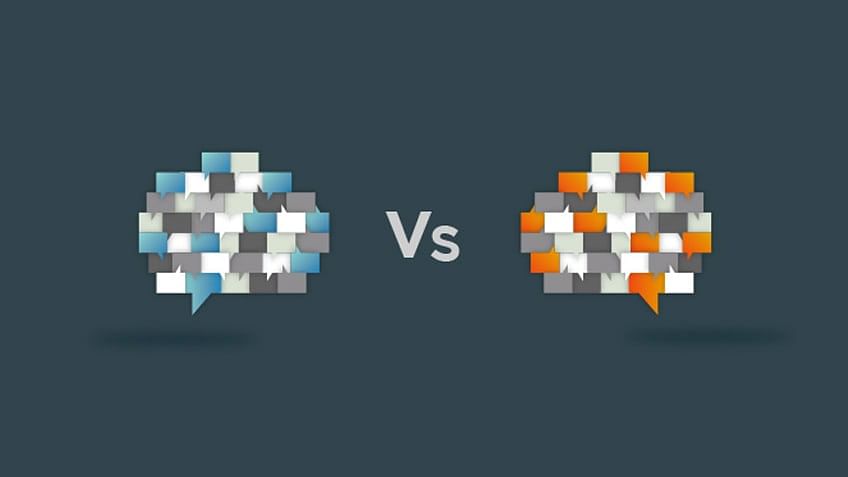
Every piece of data which is measured will show some degree of variation: no matter how much we try, we could never attain identical results for two different situations - each result will be different, even if the difference is slight. Variation may be defined as “the numerical value used to indicate how widely individuals in a group vary.”
In other words, variance gives us an idea of how data is distributed about an expected value or the mean. If you attain a variance of zero, it indicates that your results are identical - an uncommon condition. A high variance shows that the data points are spread out from each other—and the mean, while a smaller variation indicates that the data points are closer to the mean. Variance is always nonnegative.
Are you looking forward to making a mark in the Project Management field? If yes, enroll in the PMP Certification Program now and get a step closer to your career goal!
Change is inevitable, even in statistics. You’ll need to know what kind of variation affects your process because the course of action you take will depend on the type of variance. There are two types of Variance: Common Cause Variation and Special Cause Variation. You’ll need to know about Common Causes Variation vs Special Causes Variation because they are two subjects that are tested on the PMP Certification and CAPM Certification exams.
Common Cause Variation, also referred to as “Natural Problems, “Noise,” and “Random Cause” was a term coined by Harry Alpert in 1947. Common causes of variance are the usual quantifiable and historical variations in a system that are natural. Though variance is a problem, it is an inherent part of a process—variance will eventually creep in, and it is not much you can do about it. Specific actions cannot be taken to prevent this failure from occurring. It is ongoing, consistent, and predictable.
Characteristics of common causes variation are:
- Variation predictable probabilistically
- Phenomena that are active within the system
- Variation within a historical experience base which is not regular
- Lack of significance in individual high and low values
This variation usually lies within three standard deviations from the mean where 99.73% of values are expected to be found. On a control chart, they are indicated by a few random points that are within the control limit. These kinds of variations will require management action since there can be no immediate process to rectify it. You will have to make a fundamental change to reduce the number of common causes of variation. If there are only common causes of variation on your chart, your process is said to be “statistically stable.”
When this term is applied to your chart, the chart itself becomes fairly stable. Your project will have no major changes, and you will be able to continue process execution hassle-free.
Become a Data Science & Business Analytics Professional
- 28% Annual Job Growth By 2026
- 11.5 M Expected New Jobs For Data Science By 2026
Data Scientist
- Add the IBM Advantage to your Learning
- 25 Industry-relevant Projects and Integrated labs
Big Data Engineer
- Live interaction with IBM leadership
- 8X higher live interaction in live online classes by industry experts
Here's what learners are saying regarding our programs:
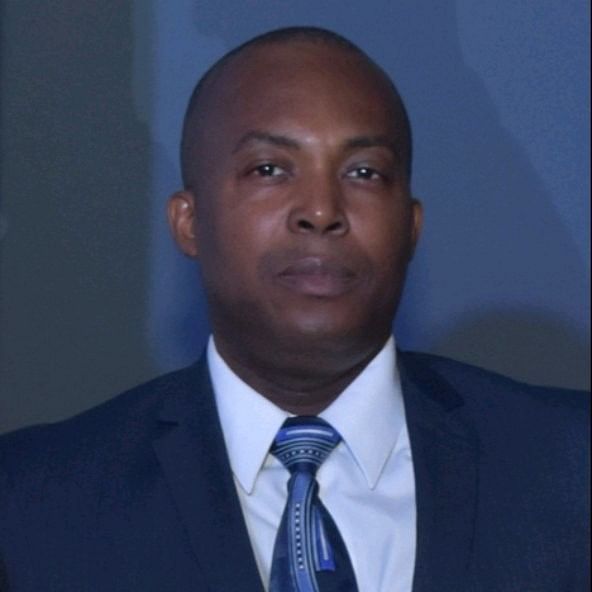
A.Anthony Davis
Simplilearn has one of the best programs available online to earn real-world skills that are in demand worldwide. I just completed the Machine Learning Advanced course, and the LMS was excellent.
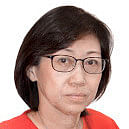
I encountered problems when trying to solve the projects in my Data Science class for Python. The Teaching Assistant was accommodating and provided step-by-step guidance through web chat sessions to resolve the issues.
Consider an employee who takes a little longer than usual to complete a specific task. He is given two days to do a task, and instead, he takes two and a half days; this is considered a common cause variation. His completion time would not have deviated very much from the mean since you would have had to consider the fact that he could submit it a little late.
Here’s another example: you estimate 20 minutes to get ready and ten minutes to get to work. Instead, you take five minutes extra to get ready because you had to pack lunch and 15 additional minutes to get to work because of traffic.
Other examples that relate to projects are inappropriate procedures, which can include the lack of clearly defined standard procedures, poor working conditions, measurement errors, normal wear and tear, computer response times, etc. These are all common cause variation.
Special Cause Variation, on the other hand, refers to unexpected glitches that affect a process. The term Special Cause Variation was coined by W. Edwards Deming and is also known as an “Assignable Cause.” These are variations that were not observed previously and are unusual, non-quantifiable variations.
These causes are sporadic, and they are a result of a specific change that is brought about in a process resulting in a chaotic problem. It is not usually part of your normal process and occurs out of the blue. Causes are usually related to some defect in the system or method. However, this failure can be corrected by making changes to affected methods, components, or processes.
Characteristics of special cause variation are:
- New and unanticipated or previously neglected episode within the system
- This kind of variation is usually unpredictable and even problematic
- The variation has never happened before and is thus outside the historical experience base
On a control chart, the points lie beyond the preferred control limit or even as random points within the control limit. Once identified on a chart, this type of problem needs to be found and addressed immediately you can help prevent it from recurring.
Let’s say you are driving to work, and you estimate arrival in 10 minutes every day. One day, it took you 20 minutes to arrive at work because you were caught in the traffic from an accident zone and were held up.
Examples relating to project management are if machine malfunctions, computer crashes, there is a power cut, etc. These kinds of random things that can happen during a project are examples of special cause variation.
One way to evaluate a project’s health is to track the difference between the original project plan and what is happening. The use of control charts helps to differentiate between the common cause variation and the special cause variation, making the process of making changes and amends easier.
Learn new trends, emerging practices, tailoring considerations, and core competencies required of a Project Management professional with the PMP Certification course .
Unlock your project management potential with Simplilearn's comprehensive training. Gain the skills and knowledge needed to lead successful projects, boost efficiency, and exceed goals. Choose the right project management course today and advance your career with confidence.
Program Name PMP® Certification Training Course PMP Plus Post Graduate Program In Project Management Geo All Geos All Geos All Geos University PMI Simplilearn University of Massachusetts Amherst Course Duration 90 Days of Flexible Access to Online Classes 36 Months 6 Months Coding experience reqd No No No Skills you wll learn 8+ PM skills including Work Breakdown Structure, Gantt Charts, Resource Allocation, Leadership and more. 6 courses including Project Management, Agile Scrum Master, Implementing a PMO, and More 9+ skills including Project Management, Quality Management, Agile Management, Design Thinking and More. Additional Benefits Experiential learning through case studies Global Teaching Assistance 35PDUs Learn by working on real-world problems 24x7 Learning support from mentors Earn 60+ PDU’s 3 year course access Cost $$ $$$$ $$$$ Explore Program Explore Program Explore Program
This article has explained special cause variation vs common cause variation which are the two important concepts in project management when it comes to data validation. Simplilearn offers multiple Project Management training courses like the Post Graduate Program in Project Management and learning paths that can help aspiring project managers get the education they need to pass not only exams like the PMP certification and CAPM® but also real-world knowledge useful for any project management career.
PMP is a registered mark of the Project Management Institute, Inc.
Our Project Management Courses Duration And Fees
Project Management Courses typically range from a few weeks to several months, with fees varying based on program and institution.
Get Free Certifications with free video courses
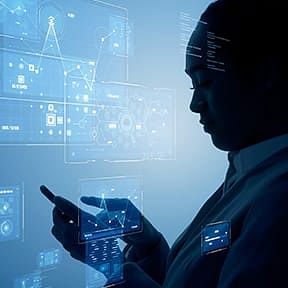
Data Science & Business Analytics
Introduction to Data Science
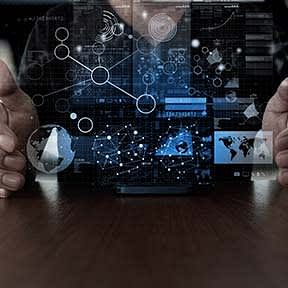
Introduction to Big Data Tools for Beginners
Learn from Industry Experts with free Masterclasses
Learner Spotlight: Watch How Prasann Upskilled in Data Science and Transformed His Career
Open Gates to a Successful Data Scientist Career in 2024 with Simplilearn Masters program
Career Fast-track
Redefining Future-Readiness for the Modern Graduate: Expert Tips for a Successful Career
Recommended Reads
Four Proven Reasons Why Gamification Improves Employee Training
10 Major Causes of Project Failure
Data Analyst vs. Data Scientist: The Ultimate Comparison
Free eBook: Top 25 Interview Questions and Answers: Big Data Analytics
Root Cause Analysis: All You Need to Know
A Comprehensive Comparison of NFT Vs. Crypto
Get Affiliated Certifications with Live Class programs
- PMP, PMI, PMBOK, CAPM, PgMP, PfMP, ACP, PBA, RMP, SP, and OPM3 are registered marks of the Project Management Institute, Inc.
- All Episodes
Weaponizing Special Causes: Deming in Education with David P. Langford (Part 10)
Aug 24, 2022
In this episode, David and Andrew talk about Common Cause Variation vs Special Cause Variation, and the problem of confusing the two. Using the example of transgender students, David describes how a system's capability should be expanded rather than using that special cause situation as a weapon to destroy the entire system.
Stotz: My name is Andrew Stotz, and I'll be your host as we continue our journey into the teachings of Dr. W Edwards Deming. Today, I am continuing my discussion with David P. Langford, who has devoted his life to applying Dr. Deming's philosophy to education, and he offers us his practical advice for implementation. Today's topic is weaponizing special causes. David take it away.
Langford: It sounds very dangerous, and it is. So I wanted to get into a little bit about special and common causes about what Deming talked about. So once again, we'll go back to Deming's system of profound knowledge. And as part of that he talked a lot about understanding variation in systems and without getting too statistical about things, he basically got people to identify two different types of variation. So there's common cause variation, which typically makes up anywhere from oh 94% to 98, 99% of what goes on in any system or any process. And then there's special cause variation, which is generally less than 2% or less than 1% of what goes on. So that sounds pretty innocuous until you actually start thinking about how that works out in society and systems and classrooms, especially in education in schools and Deming said over and over and over that there are basically two problems with special and common cause. So people treat special cause as if it's common, okay. Which is, and he called these deadly diseases, or they treat common cause variation as if it's special.
Langford: So sometimes it's difficult to sort of understand what is, what does that mean? So let's take an example like in a, in a school common cause variation would be say the, the performance of a whole school or a state or a nation or whatever it might be. You can chart that out over a long period of time and start to see a certain level of predictability of performance on anything you wanna look at, whether you're talking about behavior or you're talking about test scores or grades or kids showing up for school every day. Doesn't really matter what system you wanna look at. When you start looking at it from a systems perspective, you wanna look at as much data as you can.So at least six or seven data points, but preferably 12 and some, a lot of statisticians will say up to 20 data points.
Langford: So if you're just looking at, say test scores over a long period of time, well, you'd wanna actually look at average test scores over a 20 year period. So that, that could take a really long time to see data systems like that emerge, especially in education where we have what I call slow data that emerges. You’re familiar with like manufacturing environments and business, where you have a lot of fast data. So you may be making something and you're collecting a hundred thousand data points in a single day or a month or a week where in education, it, it really doesn't really work like that.
Stotz: I have an example that may be helpful for those people that aren't familiar with the topic. And that is in my coffee factory, we fill bags of coffee with, let's say a hundred grams of coffee. If we fill it with 101, well, we're giving away. If we fill it with 99, we're not delivering what we say. And what first lesson that we learned is that nobody's perfect. No, there is no way to consistently hit 100 is always gonna be some variation. Now that variation may be 100.0 1, 4 7, but ultimately variation around that is bad. And what you find is that maybe when the system is not that strong, you could be putting in 95 grams on sometimes and you could be putting in 105 on sometimes and something in between those. But those are all kind of common causes.
Stotz: There's just there's variation about that average, let's say. And then the only way to improve that would to be say, ‘oh, well, we need to have a more precise piece of equipment.’ It’s not that the workers weren't working hard enough or something, but we just didn't have, so we replace a piece of equipment that's measuring and all of a sudden we weigh more consistently the old clunky one didn't work that well, and now we're getting more and more narrow. And then one day the electricity goes off or we have a, a problem with the electricity and all of a sudden it's throwing the whole system off. Well, that would be some special cause as opposed to this common variation around the 100 that we're aiming for, would I be describing it? Right or how would you add to that?
Langford: Yeah, that, that that's exactly right. And so if we charted out filling your coffee bean bags over a long period of time, we'd probably find out that you'r, you probably have a really good system, right? So let's put it in terms of like grams or something. So you might find out that your variation is anywhere from 98 grams to 101 or a hundred, two grams. Right. And this also ties in with the last podcast we did about loss function. Because if you say, well, we're selling hundred gram bags, well, the further we move away from optimization or the optimum of a hundred grams, like what you were saying, if we're filling it too much, we're losing money. And if you're filling it too little, well, there might be a customer out there that feels cheated and might not ever buy your coffee ever again, because they, they weighed it and said, oh, this is only 99 grams.
Langford: Right? So there, and if you, the further that you would go away from that optimum 100 grams, and let's say that all of a sudden, you sent out a coffee bag that only had 90 grams in it. Now somebody could get really upset, right? Because that's a long way from the optimum of a, of a hundred grams or the opposite, right? If, if you're all of a sudden, randomly filling bags at 111 grams, well, now you take that 11 grams times the price of coffee beans. And like you said, you're losing a lot of money a lot of time.
Stotz: So it's one, one thing I would add to it is that now imagine that we’re measuring it very well, and we're getting a little variation. We're getting 101 sometimes. And 99, occasionally we get 102, occasionally we get 98, but it's in a relatively tight range. Now imagine that I start rewarding the employees when it goes, you know to a certain level and I start to identify if you hit this, that you're gonna get a bonus, I'm gonna dock your pay. Well, I would just be messing around with what are really just common causes of variation that have nothing to do with the employees. They have to do what that system can do.
Langford: That's what Deming called tampering. You're tampering with the system and you're, and you're making false assumptions, cuz you’re assuming that, oh, by holding somebody up as employee of the month that'll make everybody else work harder. So your assumption is that everybody else is not working harder. And the only way to get 'em to work harder is for me to manipulate 'em in some way. And Deming said that you you're now tampering with the system. You're making things worse, not better because pretty soon you have employees that say, well I'm not gonna do a really good job this month because you're offering that bonus you know, for most improved employee. Well, I can't be most improved if I'm always great, right? So one month I'm gonna look bad so I can look really good the next month.
Langford: So I can win that trip to Aruba, right? And now your coffee, the grams per sack are going down. And so everything's going haywire and everything else. And then, then you wanna blame the people in the system and not understanding that as management, you did this. You caused this to happen. And it's exactly the same way in a school classroom for education. If you start tampering with learning systems in the classroom. So most of the variation or performance of a system is built into the system. And that's what Deming talked about. Statistically like in 98, 98% of the result is coming from the system itself.
Stotz: Right. So don't like the results? Don’t blame the people, blame the system. So are, are there people problems and systems that are special causes? Absolutely. And what do you do when that happens?
Langford: So like in your example, with your coffee company once in a while, maybe you just, you hire someone that hates coffee, really shouldn't be there, they're there for the wrong reasons. They don't really love what's going on, you know? So what do you do? Well, instead of like changing the whole system based on that special cause person, right? Now, everybody has to do something different because we have this one person who's got defective behavior, so to speak, you just, you deal with the one person. Maybe they're just in the wrong job. I mean, they, they should be answering the phone in the company, not actually dealing with products or, or vice versa. Maybe they just can't deal with people. So maybe they should be in a position. So you're gonna make an adjustment. You're gonna shift that one person. And ultimately, maybe they're just in the wrong profession and maybe you need to help them find another job.
Stotz: And I just, I just wanna go back because it's such a common thing that people talk about about what Deming says about the output of the system is coming from a certain amount and the output the impact that a worker has for instance. And one of the sources of that is looking at the standard deviations. From the average, if you look at one standard deviation plus and minus you come up with 68% are within that range. If you go two standard deviations, now you're at 95%. And I believe it was that two standard deviation where he's saying, look, if something's happening within these two standard deviation, it's just common cause variation. No. He was actually talking about three standard deviations.
Stotz: Yeah. So three standard deviations would be what? 99.7%
Langford: Yeah. St yeah. Statistically it's like 99.9999998%. Right. Of something. And you know, why three standard deviations away from the, the mean, or the average? Well, because when you get to that point, if something is falling in that less than 1%, you could be pretty sure that's, that's, that's a special cause. Right? This doesn't normally happen in our, in our system. Well, the same thing in a classroom, if, if a teacher's teaching a lesson and every year when they, he or she teaches this lesson, everybody in the class scores, like, let's say 95 to a hundred. Right, right. And every year I get better and better. And my variations shrinks. And pretty soon I've got, now I'm getting an average about 98 percentile of people when they go through this lesson, right? Well, that's telling you that most of the variation is good within that system. So when people are randomly thrown into that classroom, that teacher is so good they can get that same result with almost anybody thrown it into that classroom.
Stotz: And then now.
Langford: What happens, that system is like that it becomes predictable. Now I can predict that next year. I'm probably gonna get an average somewhere between 95 and a hundred percent, if I keep doing what I've been doing.
Stotz: And what happens to that teacher when they see, oh, wait a minute, we've got this one kid who's getting a 62.
Langford: Then obviously, because you understand systems performance and you understand a little bit about special and common cause data, obviously this is a special cause there's okay. So it could be a learning difficulty. It, it could be home situation. It could be something psychological, it could be, could be a lot of things. Right. And so what do you do with a special cause? Well, typically like in the education system, we basically tried to get rid of 'em for years and we get, we would get rid of them in many different ways. Like, I'll, I'll just give them a failing grade and that that'll get rid of 'em eventually. I’ll send 'em out in the hallway, isolate them. I'll, we'll send them to special education classes. We don't we don't wanna have a mainstream in the class because it's a special cause.
Langford: And it's gonna take special effort to do, to work with that special cause and work through that. So veteran teachers that are really super good at dealing with students with special causes are amazing. Just amazing how no matter what the difficulty is or what the special cause that child starts to feel like they're part of the system, part of what's going on. Now, they may never get the same data as 98% of the rest of the students that come through that system, but they can also get better and better and better with, within that same system. And you that’s the exciting thing about education is to think about how special causes can be transformed.
Stotz: You remind me when I was in living in West Hartford, Connecticut, when I was just a little kid, I was all kinds of trouble in the classroom. And I was just, I just was all kinds of trouble. And they sent me to some special class to get some special help. And I ended up reentering the normal class and coming back kind of into a normal behavior, cuz they helped me kind of work on some of the things that were issues for me. And I ended up becoming a good student. But imagine...
Langford: You had, you had shock shock therapy or something.
Stotz: Yeah. They, they got me, they just a minor lobotomy this, but so now let's think about this cuz the, the title of today is weaponizing special causes. We've had a great discussion now about what are common, what are special? And I'm just imagining, like, let's just say that a teacher's doing really well, but they have this one student doing poorly and we know it's identified as a special cause. And then all of a sudden they decide, wow, I've gotta redo my whole way that I'm educating because of this particular unique situation. Would that be wise?
Langford: Well, it be insanity is what it would be. And we don't have to go very far, especially in the United States right now to see this happening. So there are transgendered children that that's, that's a fact, everybody knows this is happening, etcetera. But because I have one transgender child in my classroom or my school does not mean I changed the entire system based on a special cause. And now I'm disrupting 99.8% of all, all the students and parents and everybody else that comes through the system because I'm making this system twist to accommodate only a special cause. So and Deming said, this is one of the deadly diseases. So I'm gonna, I'm gonna change the whole bathroom structure into building. I'm gonna change the whole, how PE is run. I'm gonna, we're gonna change. We're gonna change everything because we have two children out of 1400 that may be transgender.
Langford: So I don't want to, I don't wanna offend anybody by this or anything else, but I just wanna point out that you can spend literally millions of not billions of dollars. go weaponizing special causes. And this is, this is just one example and PE uh districts are now building schools., where's, they're changing the bathrooms and getting rid of gender and all kinds of things based on only a few special causes. Whereas what should be happening is what do special causes needing these special, special help? So if they need a special bathroom, that's, that's fine. And there's a transition or gender bathroom for those two students out of 1400. Yeah. But just suddenly you start making rules, regulations policy I've seen district policy. That's just crazy based on maybe one instant instance every 10 years or so. And you're, you're changing an entire system based on that. is it that maybe gonna help that one individual? Yeah. But it's the same example as, as you gave, right? instead of changing the whole school because you were having difficulty in a classroom, what did we do? Well, we got you some help, right? Yeah. And basically you need discipline and people don't, people don't understand what discipline means. The first definition of discipline and the dictionary is training.
Langford: So you needed some training, right? This is what you do and this is what you can't do and in a classroom and everything else. And when you got that kind of training and it was better for you because you felt like you were belonging and you didn't have to be disruptive anymore. And it was better for everybody else because they could accept you. Right. They didn't, they weren't all of a sudden afraid that you were gonna fly out the handle or go crazy.
Stotz: Yeah. It's, it's interesting about the transgender stuff because in Thailand Thai people are just really accommodating to transgender. It's not a big deal. I mean, and in fact, the transgender people here are people who really speak up for kind of their rights. They're more outspoken. Whereas other people kind of go along more than let's say we're used to in the west. so they're there, it's just been fascinating to watch. And I think that the point is, and it's a little bit like handicap as an example we did decide to put in ramps to, to locations. And that was an adaptation to the system to accommodate the needs of that small group, but we didn't reshape a huge amount of things and other people in...
Langford: That. Well, what you're trying to do is well we see it now in medicating children with drugs and all kinds of stuff to help them learn better. And and in some cases, some schools and places you're, you're talking about 20, 25% of the population is now being medicated. Well, that's the, that's the opposite of what Deming talked about is treating special causes as if they're common that this is a common thing. So we're gonna do this with everybody. But these special causes are very, very rare, whether it's some kind of a mental thing or transgender thing or whatever it might be, you're talking about less than 1% of the population generally. And when you start to transfer a special cause to a whole population, that's what I'm talking about, about weapon weaponizing a special cause. And then ultimately you can do that with anything, but what you're really trying to do, and you comment about the, the handicap access, etcetera. What you're really trying to do is expand the capability of a system so that there are fewer and fewer and fewer special causes, not going the opposite direction, where the system becomes less and less and less and less capable. And so pretty soon everybody starts looking like a special cause.
Langford: And and that becomes hugely expensive and not very productive when you're treating everybody like their special cause.
Stotz: So let me try to summarize a little bit about what we've talked about. First of all, we talked about the importance of understanding variation. And we talked about the idea that 94 to 99% of variation is actually common cause, and only maybe one or 2% is special cause, and you, you mentioned about treating special causes as common was what Dr. Deming calls deadly diseases. You also talked about tampering, which is when you're chasing around common cause variation and either rewarding it or punishing it or highlighting it as success when in fact you really are having false assumptions. And the best way to think about that is that you walk into McDonald's and it's got a picture of the employee of the month and it's just a rotation. And then you talked about identifying the special cause, let's say it's a poor performing student.
Stotz: And then thinking about how do we how do we deal with this special cause? And it doesn't make sense necessarily to change the whole system because of what we're seeing with the special cause. And finally, I'm gonna add in my last little bit I wrote down when you were speaking is let variation run! Allow variation. It is the beauty of nature. It is the beauty of human. It is the beauty of system. Stop trying to attack every variation through medicine or through all these different things. Let variation free. Let variation run. Anything you'd add to that.
Langford: No, it's no, that's, that's good. It's a good summary. Yeah. It's in some ways it's very, very simple to think about. But in other ways, it's, it's very complex and in many ways, very contrary to the common society and businesses and schools and the way they’re run today.
Stotz: It's simple. But not always easy.
Langford: Yeah. And I think that's why Deming calls it profound knowledge. You have to have profound knowledge and profound means deep. You have to have a deep knowledge of something if you're gonna manage properly.
Stotz: Fantastic. Well, David, on behalf of everyone at Deming Institute, I want to thank you again for the discussion for listeners. Remember to go to deming.org to continue your journey. David, what's the best way that people can contact you if they wanna learn more?
Langford: You could go to our website, which is LangfordLearning.com, and there you can find out resources and support material etcetera.
Stotz: Fantastic. Well, this is your host, Andrew Stotz, and I'll leave you with one of my favorite quotes from Dr. Deming. People are entitled to joy in work.
- Deming in Education
About the Podcast
Our podcasts feature interviews with members of the Deming community, including industry leaders, practitioners, educators, Deming family members, and others who share their stories of transformation and success through innovative management and quality theories of Dr. W. Edwards Deming. Podcast episodes prior to 2022 were hosted, produced, and edited by Tripp Babbitt.
Share This Episode
Private premium login.
Having trouble logging in?

Common Cause & Special Cause Variation Explained with Examples
Editorial Team
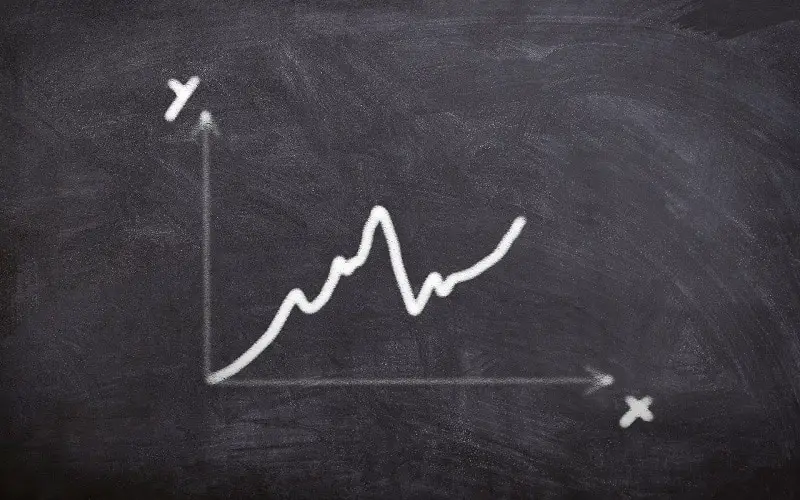
In any business operation, it is important to ensure consistency in products as well as repeatable results. Managers and workers alike have to be aware of the processes and methods on how to produce consistent outcomes at all costs. However, we cannot deny that producing exactly identical products or results is almost impossible as variance tends to exist. Variation is not necessarily a bad thing as long as it is within the standard of the critical to qualities (CTQs) specification limits.
Process variation is the occurrence when a system deviates from its fixed pattern and produces a result which differs from the usual ones. This is a major key as it concerns the consistencies of the transactional as well as the manufacturing of the business systems. Variation should be evaluated as it portrays the reliability of the business for the customers and stakeholders. Variation may also cost money hence it is crucial to keep variation at bay to prevent too much cost spent on variation. It is crucial to be able to distinguish the types of variance that occur in your business process since it will give the lead on what course of action to take. Mistakes in coming up with an effective reaction plan towards the variance may worsen the processes of the business.
There are two types of process variation which will be further elaborated in this article. The variations are known as common cause variation and special cause variation.
Common Cause Variation Definition
Common cause variation refers to the natural and measurable anomalies that occur in the system or business processes. It naturally exists within the system. While it is true that variance may bring a negative impact to business operations, we cannot escape from this aspect. It is inherent and will always be. In most cases, the common cause variant is constant, regular, and could be predicted within the business operations. The other term used to describe this variation is Natural Problems, Noise, or Random Cause. Common cause variance could be presented and analysed using histogram.
What is Common Cause Variation
There are several distinguishable characteristics of common cause variation. Firstly, the variation pattern is predictable. Common cause variation occurring is also an active event in the operations. it is controlled and is not significantly different from the usual phenomenon.
There are many factors and reasons for common cause variation and it is quite difficult to pinpoint and eliminate them. Some common cause variations are accepted within the business process and operations as long as they are within a tolerable level. Eradicating them is an arduous effort unless a drastic measure is implemented towards the operation.
Common Cause Variation Examples
There is a wide range of examples for common cause variation. Let’s take driving as an example. Usually, a driver is well aware of their destinations and the conditions of the path to reach the destination. Since they have been regularly using the same road, any defects or problems such as bumps, conditions of the road, and usual traffic are normal. They may not be able to precisely arrive at the destination at the same duration every time due to these common causes. However, the duration to arrive at the destination may not be largely differing day to day.
In terms of project-related variations, some of the examples include technical issues, human errors, downtime, high trafficking, poor computer response times, mistakes in standard procedures, and many more. Some other examples of common causes include poor design of products, outdated systems, and poor maintenance. Inconducive working conditions may also result in to common cause variants which could comprise of ventilation, temperature, humidity, noise, lighting, dirt, and so forth. Errors such as quality control and measurement could also be counted as common cause variation.
Special Cause Variation Definition
On the other hand, special cause variation refers to the unforeseen anomalies or variance that occurs within business operations. This variation, as the name suggests, is special in terms of being rare, having non-quantifiable patterns, and may not have been observed before. It is also known as Assignable Cause. Other opinions also mentioned that special cause variation is not only variance that happens for the first time, a previously overlooked or ignored problem could also be considered a special cause variation.
What is Special Cause Variation
Special cause variation is irregular occurrences and usually happens due to changes that were brought about in the business operations. It is not your mundane defects and may be very unpredictable. Most of the time, special cause variation happens following the flaws within the business processes or mechanism. While it may sound serious and taxing, there are ways to fix this which is by modifying the affected procedures or materials.
One of the characteristics of special cause variation is that it is uncontrolled and hardly predictable. The outcome of special causes variation is significantly different from the usual phenomenon. Since the issues are not predictable, it is usually problematic and may not even be recorded in the historical experience base.
Special Cause Variation Examples
As mentioned earlier, special cause variations are unexpected variants that occur due to factors that may affect the business system or operations. Let’s have an example of a special cause using the same scenario as previously elaborated for common cause variation example. The mentioned defects were common. Now, imagine if there is an unexpected accident that happens on the same road you usually take. Due to this accident, the time for the driver to arrive at the same destination may take longer than normal. Hence this accident is considered as a special cause variation. It is unexpected and results in a significantly different outcome, in this case, a longer time to arrive at the destination.
The example of special cause variation in the manufacturing sector includes environment, materials, manpower, technology, equipment, and many more. In terms of manpower, imagine a new employee is recruited into the team and still lacking in experience. The coaching and instructions should be adapted to consider that the person needs more training to be able to perform their tasks efficiently. Cases where a new supplier is needed in a short amount of time due to issues faced by the existing supplier are also unforeseen hence considered a special cause variation. Natural hazards that are beyond predictions may also be categorized into special cause variation. Some other examples include irregular traffic or fraud attack. An unexpected computer crash or malfunction in some of the components may also be considered as a special cause variation.
Common Cause and Special Cause Variation Detection
Control chart
One of the ways to keep track of common cause and special cause variation is by implementing control charts. When using control charts, the important aspect to be considered is firstly, establishing the average point of measurement. Next, establish the control limits. Usually, there are three standard deviations which are marked above and below the average point earlier. The last step is by determining which points exceed the upper and lower control limits established earlier. The points beyond the limits are special cause variation.
Before we get into the control chart of common cause and special cause variation, let’s have a look at the eight control chart rules first. If a process is stable, the points displayed in the chart will be near the average point and will not exceed the control limits.
However, it should be noted that not all rules are applicable to all types of control charts. That aside, it is quite tough to identify the causes of the patterns since special cause variation may be related to the specific type of processes. The table presented is the general rule that could be applied in most cases but is also subject to changes or differences. Studying the chart should be accompanied by knowledge and experiences in order to pinpoint the reasons for the patterns or variations.
A process is considered stable if special cause variation is not present, even if a common cause exists. A stable operation is important before it could be assessed or being improved. We could look at the stability or instability of the processes as displayed in control charts or run charts .
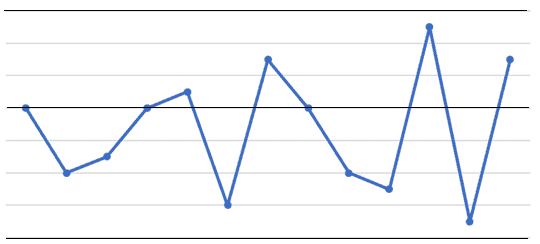
The points displayed in the chart above are randomly distributed and do not defy any of the eight rules listed earlier. This indicates that the process is stable.
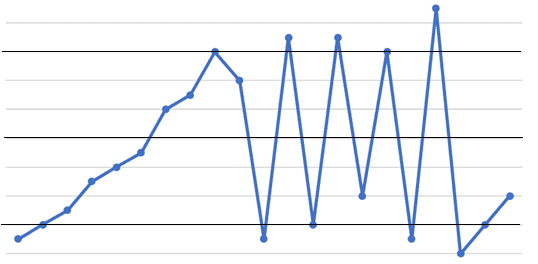
The chart presented above is an example of an unstable process. This is because some of the rules for control chart tests mentioned earlier are violated.
Simply, if the points are randomly distributed and are within the limit, they may be considered as the common cause variation. However, if there is a drastic irregularity or points exceeding the limit, you may want to analyse more into it to determine if it is a special cause variation.
Histogram is a type of bar graph that could be used to present the distribution of occurrences of data. It is easily understandable and analysed. A histogram provides information on the history of the processes done as well as forecasting the future performance of the operations. To ensure the reliability of the data presented in the histogram, it is essential for the process to be stable. As mentioned earlier, although affected by common cause variation, the processes are still considered stable, hence histogram may be used on this occasion, especially if the processes undergo regular measurement and assessment.
The data is considered to be normally distributed if it portrays a “bell” shape in the histogram. The data are grouped around the central value and this cluster is known as variation. There are several other examples of more complicated patterns, such as having several peaks in the histogram or a shortened histogram. Whenever these examples of complex structures appear in the histogram, it is fundamental to look into the data and operations more deeply.
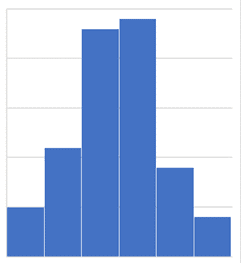
The above bar graph is an example of the histogram with a “bell” shape.
However, it should be noted that just because the histogram displays a “bell” shaped distribution, that does not mean the process is only experiencing common cause variation. A deeper analysis should be done to investigate if there were other underlying factors or causes that lead towards the pattern of the distribution displayed in the histogram.
Countering common cause and special cause variation
Once the causes of the variation have been pinpointed, here comes the attempt to combat and resolve it. Different measures are implemented to counter different types of variation, i.e. common cause variation and special cause variation. Common cause variation is quite tough to be completely eliminated. Drastic or long-term process modification could be used to counter common cause variation. A new method should be introduced and constantly conducted to achieve the long-term goal of eliminating the common cause variation. Some other effects may happen to the operations but as time passes, the cause may be gradually solved. As for special cause variation, it could be countered using contingency plans. Usually, additional processes are implemented into the usual operation in order to counter the special cause variation.
- What To Do If You Have “A Perfect Student” Syndrome?
- How to Effectively Find and Use the Best Possible Suppliers in 2022
- 5 Reasons to Screen Potential Employees
- Why It’s Important to Hire an InventHelp Patent Attorney?
most recent
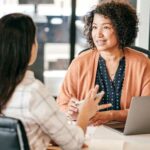
Top 33 SeaWorld Parks Entertainment Interview Questions and Answers 2024
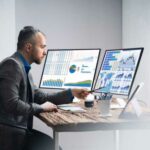
Top 33 Senior Financial Analyst Interview Questions and Answers 2024
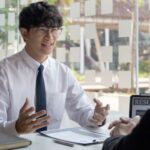
Top 33 Time Series Analysis Interview Questions and Answers 2024
© 2024 Copyright ProjectPractical.com
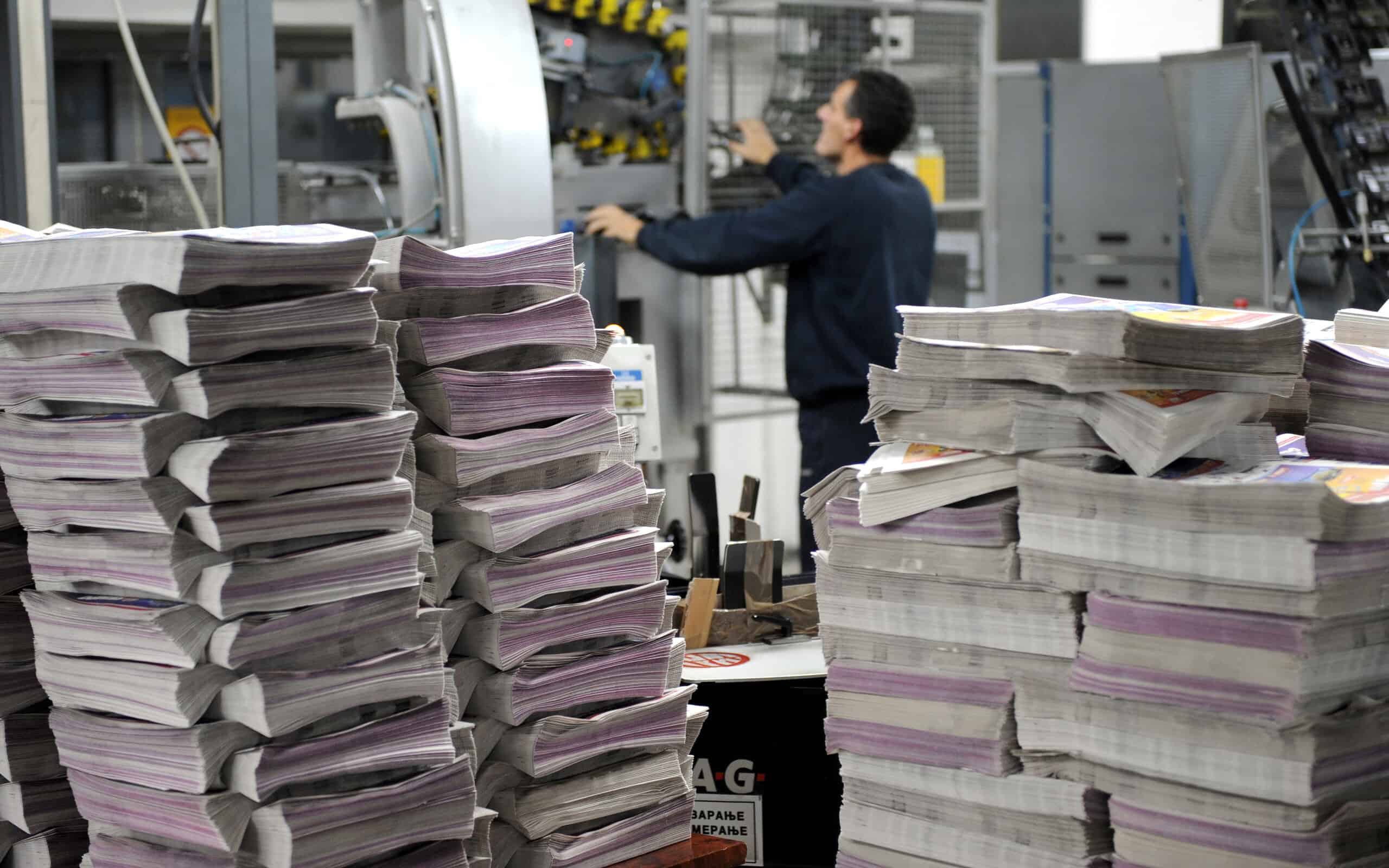
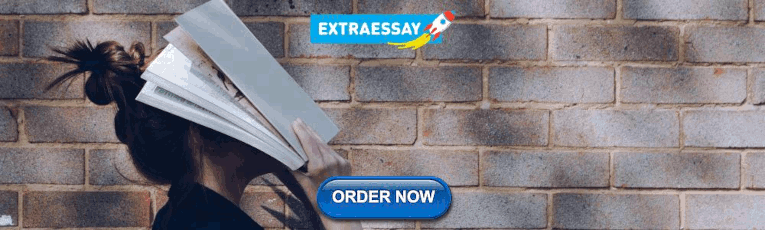
How to Determine and Address Common Cause Variation in Your Business
Updated: March 30, 2023 by Lori Kinney
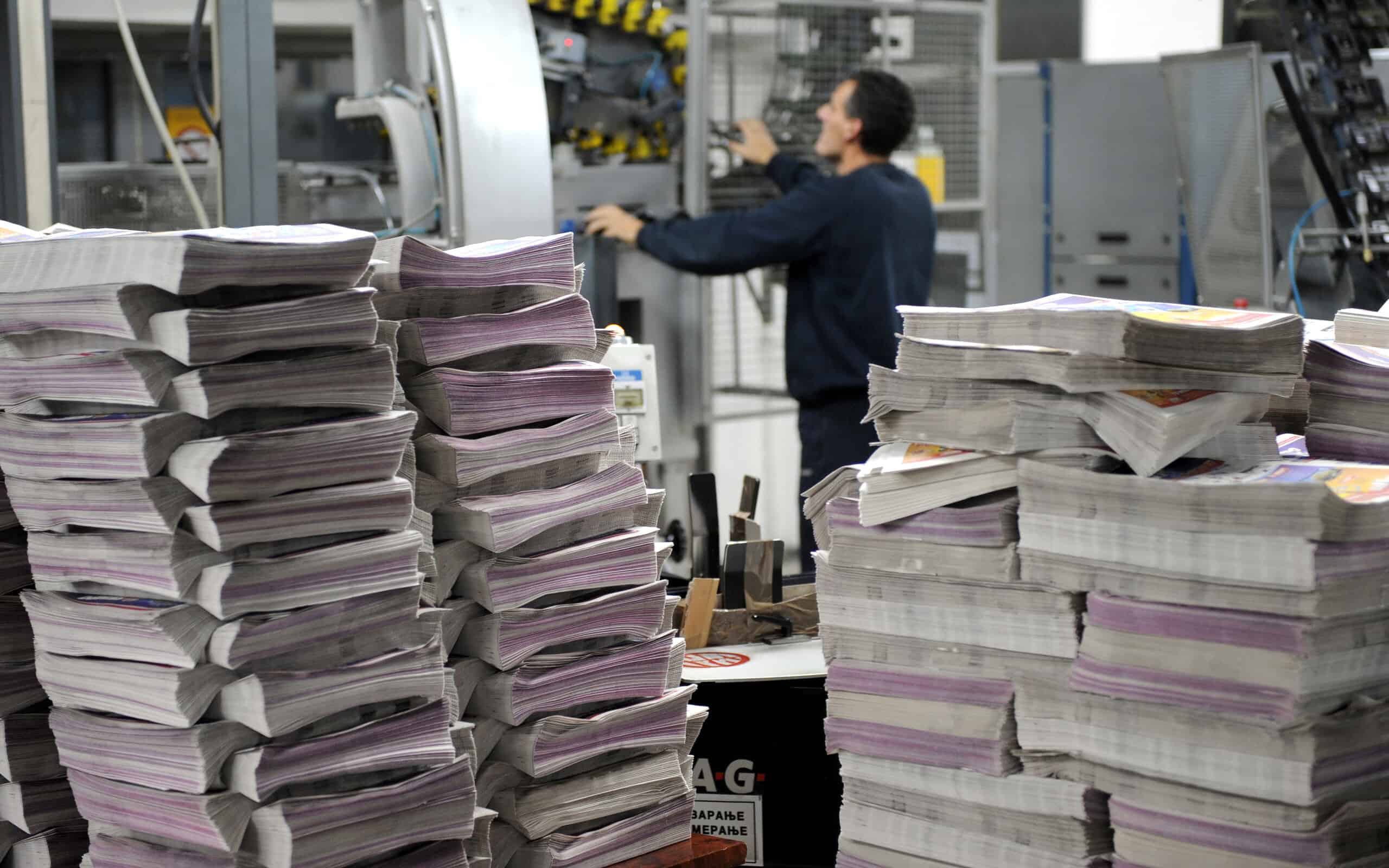
Special cause variations account for about 10% of the variations in measurements. There are specific causes for these variations, they are assignable, and they must be dealt with. The other 90% or so are common cause variations.
Overview: What is variation (common cause)?
Common cause variation can be defined as the natural variation in a process. There are many causes of this type of variation, and it is difficult to remove them without re-engineering the process.
3 benefits of variation (common cause)
It would be difficult to say there are many benefits to variation as they are a hindrance to quality, but there are significant advantages of common cause variation over special cause variation:
Common cause variation is ongoing and is more seen as variation that is accepted and lived with as opposed to special cause variation which can halt processes and requires action.
2. Consistency
Common cause variation is consistent and can therefore be planned around.
3. Predictability
There is a predictability to common cause variation, which means that it does not cause any negative surprises.
Why is common cause variation important to understand?
Common cause variation is important to understand for the following reasons:
It is an inherent part of the process
It is important to understand common cause variation as just a part of the process so that no unnecessary alarm bells go off when these sorts of variations come up and a working process ends up being needlessly overhauled.
Understanding common cause variation allows you the peace of mind of knowing your process is statistically stable if the predominant variations observed are common cause.
Long-term adjustment of processes
If you understand common cause variations, you understand what kinds of variations might require immediate adjustment of processes as opposed to adjustments that should be made incrementally over the long term.
An industry example of variation (common cause)
At a newspaper, suddenly the printing press runs out of paper. This is a regular occurrence and slows down production as long as it takes to reload paper. This is an example of the type of variation that would be considered common cause and is no real need for concern or overhauling processes.
3 best practices when thinking about variation (common cause)
Here are some practices to keep in mind about common cause variation:
1. Avoid overcorrection
Many try to control common cause variation by making drastic changes and then inadvertently causing even more variation. Do not let this happen in your business. Know how much variation is acceptable and how to react to it.
2. Look at charts to determine process stability
A process is stable if the data points on a chart appear to be random and do not violate any of the 8 control chart tests.
3. Keep an eye out for variations that deviate from the mean
These are more likely to be special causes.
Frequently Asked Questions (FAQ) about common cause variation
What are some examples of common cause variation as they relate to projects.
Some examples of common cause variation as they relate to projects would be normal wear and tear, computer response times, and poor working conditions.
Why is variation seen as the enemy of quality?
Variation prohibits businesses from being able to guarantee a 100% consistent and reliable product or service.
What amount of variation is likely common cause?
Typically, if variation hovers around 2 to 3%, it is likely common cause variation.
Should You Address Common Cause Variation?
In your business, having exemplary quality control is always desired. Unfortunately, there is just some amount of common cause variation that is part of doing business that is likely out of your control. As long as you plan accordingly for it, it should not pose too big of a risk.
About the Author
Lori Kinney

- Quality Improvement Zone
- Quality Improvement journey
- Measurement
Understanding variation
This page explores what variation is, and why we need to understand it. We'll look at types of variation, how to look at variation, Statistical Process Control (SPC) charts and reacting to variation.
Leaders in the science of improvement should speak the language of variation. Knowledge about separating variation of outcomes of a process or system in to common and special causes helps to decide appropriate actions for that process or system. Inappropriate action may make things worse.
The Improvement Guide (Langley et al, page 81)
Measurements of all outcomes and processes will vary over time. Some variation is intentional, for example varying services for people depending on their needs. In contrast, unintended variation is due to differences not connected with different needs. For example we would want everyone to have a good experience. Unintended variation results in poor quality, waste and harm. This often forms the focus for improvement.
Often we look at data aggregated over long time periods, such as by year or by quarter. This hides the variation in the data. Measuring more often (hourly, daily, weekly or monthly) can be more informative. A central aspect of improvement science is looking at charts of data over time. Run charts and SPC charts can help us understand variation and decide whether a change is an improvement.
To tell whether a process or its outcome is improving, it is important to understand changes in the data. We need to understand whether these changes are due to actions we've taken, or due to random chance. Making decisions without understanding the causes of variation can make things worse.
The two types of variation defined by Shewhart and Deming are:
Common cause - those causes that are inherent in the system over time. Causes that affect everyone working in the system, and affect all outcomes of the system. These include random variation.
Special cause - those non-random causes that are not part of the system all the time. Causes that do not affect everyone, but arise because of specific circumstances.
SPC charts are a good way to separate common cause and special cause variation.
Run charts can signal whether the variation present is exhibiting random or non-random patterns. It cannot signal whether these are special or common cause. If there are non-random signals in your run charts, it is likely that special cause variation is present.
SPC charts minimise the chance of making either of two possible mistakes that can make things worse rather than better:
1. Inappropriately reacting to common cause variation, as if it were due to a special cause. This "tampering" may exacerbate the variation. If the system is stable and only common cause is present, but not working at an acceptable level, then it requires fundamental change. Reacting to individual data points is not helpful.
2. Inappropriately ignoring special cause variation, treating it as if it were common cause. We need to remove special cause variation to get stability and predictability. This will then give us the ability to understand the effect of any deliberate changes.
Process capability is about predicting what the future values of a measure are likely to be. The predicted range can be compared with the desired range to see if the process is capable of meeting its specifications. SPC methods help us to work out process capability. For information about how to calculate capability consult the Healthcare Data Guide.
A process could be stable and only showing common cause variation, but not capable. We would need to make a fundamental change if we wish to see an improvement. If we keep on doing what we've always done, we'll keep on getting what we've always got.
If we also detect special causes we need to investigate what might be happening and learn from them. Not all special cause variation is bad. If we notice a special cause which shows good performance we could consider testing it as a change to the process.
If we want to make a process better by changing something, we are introducing a special cause. If we notice special cause variation causing problems in how things work, we'll want to get rid of them.
Measurement plan
A measurement plan sets out details for each measure proposed for an improvement project.
A run chart is a line graph of data plotted over time. By collecting and charting data over time, you can find trends or patterns in the process.
SPC charts
Statistical Process Control (SPC) Charts are simple graphical tools that enable process performance monitoring.
Funnel plot
A funnel plot is a chart that helps to understand variation within a system.
Scatter plot
A graph in which the values of two variables are plotted along two axes, the pattern of the resulting points revealing any correlation present.
Pareto chart
A Pareto Chart is a tool to help you understand your system.
Histogram
A histogram is a plot that lets you discover, and show, the underlying frequency distribution (shape) of a set of continuous data.
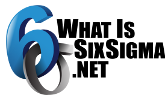
- Lean Six Sigma
Common Cause Variation
Thoughts on preparing for the turbulence that comes with business.
Any business making legitimate strides toward a positive goal is moving in some direction , and any business that is moving is naturally going to face obstacles and bumps in the road. These bumps in the road range from day-to-day variances to unique, major variances that sway a business away from its primary goal of producing a product or service in a consistent and timely manner. Identifying and defining both common and infrequent obstacles is a critical part of business success and survival.
In this article, we will focus primarily on day-to-day, expected variations in productivity. In the Six Sigma system of process improvement, these are called common cause variations. To help bring understanding to the differentiation, let’s look at a couple of important definitions.
Six Sigma: Primary Types of Variation
In the Six Sigma system of process improvement, two primary types of variations from ideal (or average) productivity are defined:
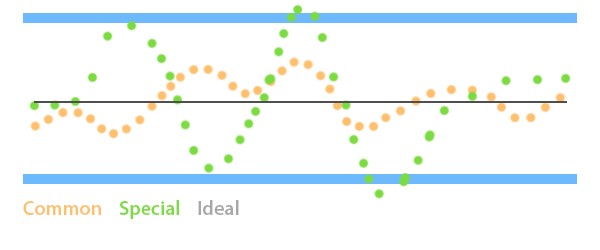
Day-to-day, hour-by-hour variations due to common, daily activities. These variations are unavoidable and built into the process.
- Special Cause Variations
One-time or infrequent variations caused by rare circumstances, such as disasters. These variations are typically not foreseeable and need corrective action.
The Gravel Road
To illustrate the overall picture, we’ll use the example of a car driving down a gravel road:
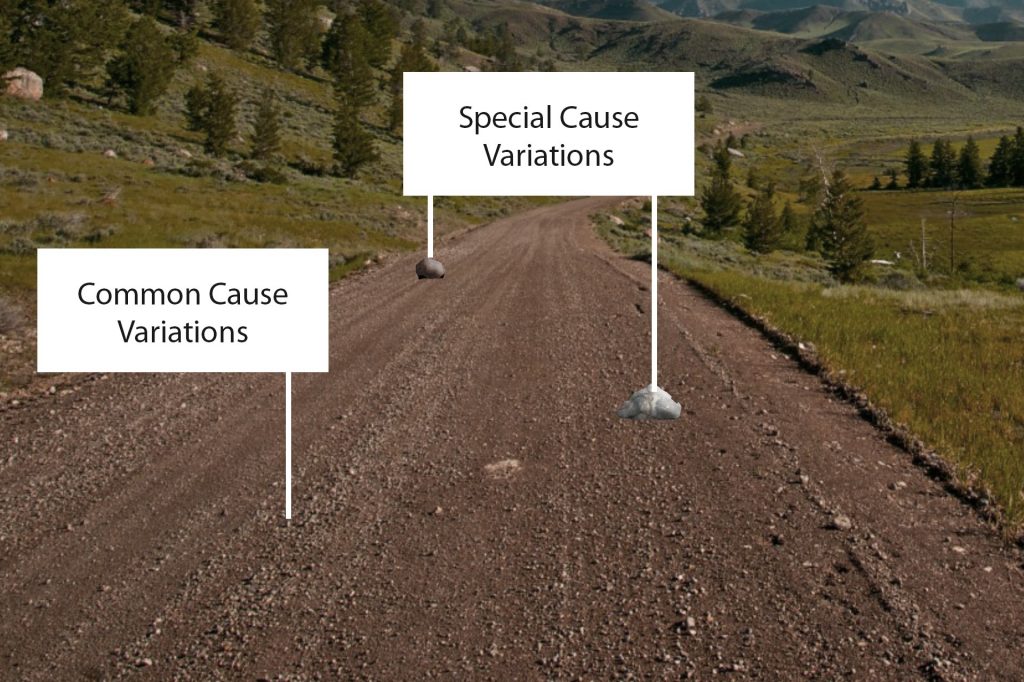
When you drive down a gravel road, you have a feeling of movement. You know the car is headed where you want to go, despite the somewhat rough ride. In the end, the car is moving in the right direction. Small bits of gravel that cover the road and over which the car rolls do create a constant bumpy ride, but it is bearable. There is no need for oversteering or constant braking to respond to each bit of gravel.
The gravel is your constant, expected turbulence–your common cause variation–that is minor enough to continue forward without disrupting the trip.
Special cause variation, on the other hand, would be like large rocks and potholes that you come across occasionally on the road. To avoid these, substantial steering, swerving, and/or braking is necessary to safely navigate. If one of these is struck, it’s possible that extra steering will be necessary to recover the vehicle’s normal trajectory. These larger obstacles do not pop up often, but it is good to be ready when they do.
What do these variances look like in the business world? It helps to first observe that no business is perfect. The result is that there must be some level of standard variation from ideal productivity that is deemed acceptable.
For example, take a ridesharing service like Uber or Lyft. Riders request many rides in concentrated cities where there are plenty of drivers present to make quick pickups the norm. Let’s say the organization aims for a standard wait time between a rider requesting a ride and a driver arriving for pickup of four minutes. In reality, drivers arrive in three to seven minutes on average. This is because there are stoplights, traffic, pedestrians, weather conditions, and other common obstacles that lie between the driver and the rider–and the amount of delay they cause varies constantly. There is no need to respond to these common delays because these delays are built into the process.
Special Cause Variation
Now consider that a sinkhole occurs in the middle of a main intersection and shuts it down. This causes major delays and backups for everyone, bringing the average wait time for riders to sixteen minutes. This is a major disruption and one that should be responded to with best-case alternative strategy.
How Should Organizations Respond to Variations?
In day-to-day business, there are some occasional issues that warrant a major corrective response and others that do not. We alluded to this in our prior example, pointing out that major response to normal traffic in a city is not needed; it is normal. A disruptive sinkhole does require alternative strategy.
Our focus here is the common. Other examples of common cause variation are a printer running out of paper, an assembly line arm needed to pause for regular maintenance, or a freight truck needing an oil change. These things can cause small variations in production time, but they are expected and planned for. They are not a surprise. An organization does not need to hold a conference call to decide how to respond to an empty printer. A worker pauses, grabs another ream, and pops it in. Back to business.
One other note is that variations can also be positive, warranting a good change in process. But that is a topic for the special variance section.
Prep, Not Avoidance
One might think that a major key to business success is avoiding trouble altogether. However, consider this simple law of physics: Every moving object faces a level of resistance. All the same, any time we are moving–whether it be toward our personal goals or business goals–there will be problems in our way that we must decide how to handle.
The issue at hand is not how to avoid all trouble, but how to respond to it and what to respond to. The key Six Sigma categories of common cause variation and special cause variation are helpful aids in planning how your organization will conserve time and material resources by responding
Previous post: Control Plan
Next post: Western Electric Rules
- 10 Things You Should Know About Six Sigma
- Famous Six Sigma People
- Six Sigma Software
Recent Posts
- Control System Expansion
- Energy Audit Management
- Industrial Project Management
- Network Diagram
- Supply Chain and Logistics
- Visual Management
- Utilizing Pareto Charts in Business Analysis
- Privacy Policy
Lean Six Sigma Training Certification

- Facebook Instagram Twitter LinkedIn YouTube
- (877) 497-4462
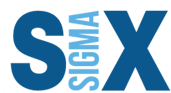
What is Special Cause Variation? How to Identify It?
March 8th, 2024
Variations are inherent in any process and the sources of these variations play a crucial role in determining process performance and stability. While common cause variations are predictable and result from the process design itself, special cause variation arise due to unexpected factors and lead to process instability.
Managing these special cause variations by identifying and eliminating their root causes is an integral part of quality management frameworks like Six Sigma and Lean. By doing so, processes can achieve stability and enhanced capability.
This enables improved competitiveness through cost reduction, lead time reduction, improved quality, and higher customer satisfaction.
Thus, organizations invest significant efforts in detecting and managing special causes of variation in their business processes.
Understanding Special Cause Variation
Special cause variation refers to fluctuations in a process that happen because of unique or unusual factors that are not normally present.
These unnatural variations are unpredictable and assignable to a specific cause. They indicate that the process has become unstable and is producing defects or undesirable outcomes.
Difference from Common Cause Variation
Common cause variation refers to natural or expected variability that exists in any process. This type of variation happens due to minor, ubiquitous causes that are inherent in the process design.
Common causes lead to random scattering of data points around the mean. On the other hand, special causes produce data points that stand out from the normal pattern of variation and signal that the process is out of control.
While common cause variation indicates a stable process, special cause variation implies instability requiring investigation and corrective action.
Importance in Quality Management
Effective management of special cause variation is crucial for organizations looking to enhance their quality management programs.
By properly identifying and addressing special causes, companies can boost competitiveness, better meet customer expectations, and positively impact financials.
Enhancing Competitiveness with Special Cause Variation
In today’s business landscape, quality has become a key competitive differentiator across industries. Companies that demonstrate consistent product/service quality and continuous improvement initiatives can gain an edge.
The ability to rapidly detect and mitigate special causes of variation enables organizations to enhance process stability .
This prevents the occurrence of unexpected defects and non-conformances which can erode quality perception. Maintaining robust quality standards is essential to stay ahead.
Connecting with Quality-Focused Customers
Customers are increasingly assessing providers based on their quality management record and commitment to zero defects.
By tackling special cause variations through root cause analysis , corrective actions, and control mechanisms. etc. companies can limit unexpected issues that lead to customer dissatisfaction.
This accountability and proactive quality management culture aligns with customer requirements and values.
Communicating such initiatives and results also reassures customers regarding consistency. This helps strengthen loyalty and presents partnership opportunities with quality-focused customers.
Identifying Special Cause Variation
Special cause variation is often difficult to detect without the right analysis tools. Identifying special causes requires going beyond typical process monitoring to specialized statistical techniques.
There are three main methods for recognizing when variation is due to special causes:
Control Charts
Control charts are the most common way to identify special cause variation. Control charts plot data over time with statistically derived upper and lower control limits.
When data points fall outside these control limits , it indicates something unexpected has happened to the process.
This unexpected variance points to special causes rather than expected normal variation.
Common control charts used to monitor special causes include Xbar-R charts , Xbar-S charts , Individual charts, moving range charts, and P charts.
Statistical process control software makes it easy to generate control charts and receive alerts when special causes occur.
Statistical Process Control
Statistical process control (SPC) is an analytical methodology that makes extensive use of control charts.
SPC carefully monitors processes to distinguish between expected and unexpected variations using statistical methods.
By visually separating normal and special cause variations on control charts, SPC provides objective evidence that can pinpoint the timing and magnitude of special causes.
SPC also calculates metrics like Cp and Cpk that quantify overall process variation and process capability.
Tracking these metrics provides further insight into process stability versus areas with heightened special cause variation.
Root Cause Analysis
When control charts or SPC identify special cause variation, the next step is to determine the root cause. Root cause analysis gets to the underlying reason why the unexpected process change happened.
Various tools like the 5 Whys , fishbone diagrams , and failure mode analysis help uncover root causes. Tracing special cause variation back to the root cause is crucial for addressing problems permanently.
Otherwise, the same special cause could keep affecting the process unpredictably.
Implications of Special Cause Variation
Special cause variation can have major implications if left unchecked.
Understanding and properly dealing with special causes is crucial for organizations that want to achieve process stability, improve customer satisfaction, and boost financial performance.
Process Stability with Special Cause Variation
The presence of special cause variation indicates an unstable process. The output and performance metrics of an unstable process fluctuate unpredictably.
This makes the process unreliable and difficult to control. Identifying and eliminating special causes is the first step toward stabilizing a process.
Stable processes have consistent output and are predictable, easier to monitor, and simpler to improve.
Customer Satisfaction
Customers expect consistent quality and on-time delivery of products and services. An unstable process leads to unpredictable product quality and delivery delays which frustrates customers. Fixing special causes improves process stability and capability.
This enables organizations to reliably meet customer expectations and increase satisfaction levels. Satisfied customers lead to repeat purchases and valuable word-of-mouth publicity.
Financial Performance
Unstable processes lead to increased waste, rework, and returns. The additional effort and resources needed to fix these issues drive up costs and hurt profitability.
Special cause variation can also result in production and shipment delays that mean missed revenue opportunities and penalties.
Eliminating special causes reduces costs and improves process efficiency . This directly translates into increased profit margins, higher ROI, and stronger financial performance.
Strategies for Management of Special Cause Variation
Effectively managing special cause variation is critical for organizations looking to improve quality, reduce costs, and boost customer satisfaction.
When a process experiences special cause variation, it indicates an unexpected change that needs to be addressed. Implementing targeted strategies can help get processes back into a state of statistical control.
Corrective Actions
- Once a special cause is identified through statistical process control methods, structured problem-solving methodologies like PDCA (Plan-Do-Check-Act) or DMAIC (Define, Measure, Analyze, Improve, Control) can be utilized to develop corrective actions.
- Brainstorming sessions to determine potential causes and solutions can provide useful insights from team members. Fishbone diagrams are an effective tool to visually map out causes.
- Pilot testing proposed improvements on a small scale first to evaluate potential impact.
- Verify the effectiveness of corrective actions by monitoring the process over time using control charts. Special causes should be removed and variation should return to baseline common cause levels.
Continual Improvement with Special Cause Variation
- Schedule periodic reviews of processes, even in the absence of special causes, to identify opportunities for incremental improvements.
- Utilize lean tools like 5S , poka-yoke , and process mapping to reduce waste and enhance process stability.
- Keep the workforce engaged by encouraging them to share improvement ideas and recognize implementation efforts.
- Consider automating certain process steps to reduce variability induced by human errors.
- Review process performance metrics regularly to ensure improvements are sustained over longer periods.
- Conduct refresher training for employees on methods like statistical process control, Six Sigma, and hypothesis testing.
By taking a structured approach combining short-term corrective actions and long-term continual improvement , organizations can effectively manage special cause variation events.
This drives greater consistency and stability in processes, directly enhancing product quality and customer satisfaction.
SixSigma.us offers both Live Virtual classes as well as Online Self-Paced training. Most option includes access to the same great Master Black Belt instructors that teach our World Class in-person sessions. Sign-up today!
Virtual Classroom Training Programs Self-Paced Online Training Programs
SixSigma.us Accreditation & Affiliations
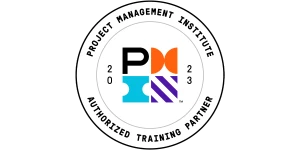
Monthly Management Tips
- Be the first one to receive the latest updates and information from 6Sigma
- Get curated resources from industry-experts
- Gain an edge with complete guides and other exclusive materials
- Become a part of one of the largest Six Sigma community
- Unlock your path to become a Six Sigma professional
" * " indicates required fields
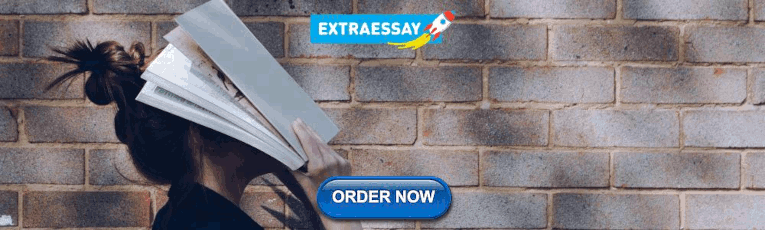
IMAGES
VIDEO
COMMENTS
Common cause variation indicates a process that's in statistical control, or stable. Use common cause variation to: 1. Predict the range of values you can expect from your process measure. For example, a control chart of yield is operating in control at an average of 90% with an upper control limit of 92% and a lower control limit of 88%. 2.
Common cause variations are the natural result of the system. In a stable system, common cause variation will be predictable within certain limits. Special cause variations represent a unique event that is outside the system: for example, a natural disaster. Distinguishing the difference between variation, as well as understanding its causes ...
The difference between these two types of variation lies in how common cause variations are normal and expected variations that do not deviate from the natural order of a process. With common cause variations, a process remains stable. With special cause variations, however, a process is dramatically affected and becomes unstable.
Common and special causes are the two distinct origins of variation in a process, as defined in the statistical thinking and methods of Walter A. Shewhart and W. Edwards Deming. Briefly, "common causes", also called natural patterns, are the usual, historical, quantifiable variation in a system, while "special causes" are unusual, not ...
This exploration of common cause variation aimed to progress readers along the journey of statistical thinking to drive data-based operational excellence. In a nutshell: Common cause variation is natural randomness indistinguishable from noise that demands analytical interpretation.
Quality Glossary Definition: Variation. The Law of Variation is defined as the difference between an ideal and an actual situation. Variation or variability is most often encountered as a change in data, expected outcomes, or slight changes in production quality. Variation usually occurs in four separate areas: Special causes. Common causes.
Abstract. Knowing the difference between common causes and special causes of variation is vital to improving a process or system. Common causes are inherent in the process, while special causes occur under certain circumstances. A process is stable or in statistical control if it has only common causes of variation.
Common cause variation and special cause variation are two types of variations common in any process. Common cause variation is a natural part of the process and is generally thought to be limited to three standard deviations of the mean. ... The New Economics for Industry, Government, Education. MIT Press, Cambridge, Mass (2000) Google Scholar ...
Clause 8.5.2 defines steps required for corrective action (CA), including determination of the nonconformity cause, along with determination and implementation of necessary action to prevent recurrence. Similar wording is also present in clause 8.5.3 regarding preventive action (PA). A cause of a nonconformance should coincide with a variation ...
Common cause variations are those causes that are inherent in the system or process. 4 Evidence of common cause variation can be seen visually in the OA's Fig 3, from January 2017 to October 2017, because the data points vary around the mean but remain between the upper and lower control limits (dotted lines). In contrast, special cause variations are causes of variations that are not ...
Common-cause variation is random variation present in stable healthcare processes. Special-cause variation is an unpredictable deviation resulting from a cause that is not an intrinsic part of a process. By careful and systematic measurement, it is easier to detect changes that are not random variation.
Common Cause Variation. Common Cause Variation, also referred to as "Natural Problems, "Noise," and "Random Cause" was a term coined by Harry Alpert in 1947. Common causes of variance are the usual quantifiable and historical variations in a system that are natural. Though variance is a problem, it is an inherent part of a process ...
In this episode, David and Andrew talk about Common Cause Variation vs Special Cause Variation, and the problem of confusing the two. Using the example of transgender students, David describes how a system's capability should be expanded rather than using that special cause situation as a weapon to destroy the entire system. TRANSCRIPT Stotz: My name is Andrew Stotz, and I'll be your host as ...
The variations are known as common cause variation and special cause variation. Common Cause Variation Definition. Common cause variation refers to the natural and measurable anomalies that occur in the system or business processes. It naturally exists within the system. While it is true that variance may bring a negative impact to business ...
1. Ongoing. Common cause variation is ongoing and is more seen as variation that is accepted and lived with as opposed to special cause variation which can halt processes and requires action. 2. Consistency. Common cause variation is consistent and can therefore be planned around. 3.
The two types of variation defined by Shewhart and Deming are: Common cause - those causes that are inherent in the system over time. Causes that affect everyone working in the system, and affect all outcomes of the system. These include random variation. Special cause - those non-random causes that are not part of the system all the time ...
Common cause variation includes causes that are inherent in a process over time and affect every outcome of the process and everyone working in the process. A second pattern of variation includes special causes (aka "uncontrolled variation" or "signal"). Special cause variation is a name for sources of variation that are not part of the ...
Common Cause Variations. Day-to-day, hour-by-hour variations due to common, daily activities. These variations are unavoidable and built into the process. Special Cause Variations. One-time or infrequent variations caused by rare circumstances, such as disasters. These variations are typically not foreseeable and need corrective action.
Common cause variation stems from the design of the system, and an external source or unique feature is usually the root cause of special cause variation. Since identifying the root cause of variation is often challenging, several methods have been proposed to identify root causes. Root cause diagrams (cause-and-effect) diagrams investigate
Common causes lead to random scattering of data points around the mean. On the other hand, special causes produce data points that stand out from the normal pattern of variation and signal that the process is out of control. While common cause variation indicates a stable process, special cause variation implies instability requiring ...