
- Advanced Search
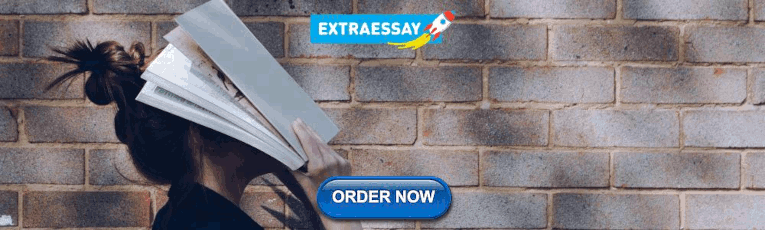
The Journal of Machine Learning Research
Volume 24, Issue 1
January 2023
- new algorithms with empirical, theoretical, psychological, or biological justification;
- experimental and/or theoretical studies yielding new insight into the design and behavior of learning in intelligent systems;
- accounts of applications of existing techniques that shed light on the strengths and weaknesses of the methods;
- formalization of new learning tasks (e.g., in the context of new applications) and of methods for assessing performance on those tasks;
- development of new analytical frameworks that advance theoretical studies of practical learning methods;
- computational models of data from natural learning systems at the behavioral or neural level; or
- extremely well-written surveys of existing work.
JMLR has a commitment to rigorous yet rapid reviewing. Final versions are published electronically (ISSN 1533-7928) immediately upon receipt. Printed volumes (ISSN: 1532-4435) are now published by Microtome Publishing and available for sale .
Subject Areas
Announcements.
ACM Updates Its Peer Review Policy
ACM is pleased to announce that its Publications Board has approved an updated Peer Review Policy . If you have any questions regarding the update, the associated FAQ addresses topics such as confidentiality, the use of large language models in the peer review process, conflicts of interest, and several other relevant concerns. If there are any issues that are not addressed in the FAQ, please contact ACM’s Director of Publications, Scott Delman .
New ACM Policy on Authorship ACM has a new Policy on Authorship , covering a range of key topics, including the use of generative AI tools. Please familiarize yourself with the new policy and the associated list of Frequently Asked Questions .
Most Frequent Affiliations
Most cited authors, latest issue.
- Volume 24, Issue 1 January 2023 ISSN: 1532-4435 EISSN: 1533-7928 View Table of Contents
The measure and mismeasure of fairness
Department of Statistics, Harvard University, Cambridge, MA
Department of Computer Science, Stanford University, Stanford, CA
Department of Applied Statistics, Social Science, and Humanities, New York University, New York, NY
Harvard Kennedy School, Harvard University, Cambridge, MA
Weisfeiler and Leman go machine learning: the story so far
Department of Computer Science, RWTH Aachen University, Aachen, Germany
Meta AI Research, Department of Computer Science and Applied Mathematics, Weizmann Institute of Science, Rehovot, Israel
NVIDIA Research, Tel Aviv, Israel

AIDOS Lab, Institute of AI for Health Helmholtz Zentrum München and Technical University of Munich Munich, Germany
Faculty of Computer Science and Research Network Data Science, University of Vienna, Vienna, Austria
Kumo.AI, Mountain View, CA
Machine Learning & Computational Biology Lab, Department of Biosystems Science and Engineering, ETH Zürich, Basel, Switzerland and Swiss Institute of Bioinformatics, Lausanne, Switzerland
Recent Award Winners
Most popular, other acm journals.

Volume 2, Issue 1

Volume 3, Issue 1
January-March 2024

Volume 56, Issue 9
October 2024

Volume 5, Issue 1

Volume 36, Issue 1
Export Citations
- Please download or close your previous search result export first before starting a new bulk export. Preview is not available. By clicking download, a status dialog will open to start the export process. The process may take a few minutes but once it finishes a file will be downloadable from your browser. You may continue to browse the DL while the export process is in progress. Download
- Download citation
- Copy citation
We are preparing your search results for download ...
We will inform you here when the file is ready.
Your file of search results citations is now ready.
Your search export query has expired. Please try again.
Journal of Machine Learning Research

The Journal of Machine Learning Research (JMLR) provides an international forum for the electronic and paper publication of high-quality scholarly articles in all areas of machine learning. All published papers are freely available online.
JMLR has a commitment to rigorous yet rapid reviewing. Final versions are published electronically (ISSN 1533-7928) immediately upon receipt. Until the end of 2004, paper volumes (ISSN 1532-4435) were published 8 times annually and sold to libraries and individuals by the MIT Press. Paper volumes (ISSN 1532-4435) are now published and sold by Microtome Publishing .
- This website is under active development

Submit a paper
- You need to be registered in order to submit a paper. If you are not already registered, register here
- The submission guidelines can be found here
Start a submission »
More information
- Editorial Board
- Frequently asked questions
- Survey Paper
- Open access
- Published: 31 March 2021
Review of deep learning: concepts, CNN architectures, challenges, applications, future directions
- Laith Alzubaidi ORCID: orcid.org/0000-0002-7296-5413 1 , 5 ,
- Jinglan Zhang 1 ,
- Amjad J. Humaidi 2 ,
- Ayad Al-Dujaili 3 ,
- Ye Duan 4 ,
- Omran Al-Shamma 5 ,
- J. Santamaría 6 ,
- Mohammed A. Fadhel 7 ,
- Muthana Al-Amidie 4 &
- Laith Farhan 8
Journal of Big Data volume 8 , Article number: 53 ( 2021 ) Cite this article
399k Accesses
2264 Citations
37 Altmetric
Metrics details
In the last few years, the deep learning (DL) computing paradigm has been deemed the Gold Standard in the machine learning (ML) community. Moreover, it has gradually become the most widely used computational approach in the field of ML, thus achieving outstanding results on several complex cognitive tasks, matching or even beating those provided by human performance. One of the benefits of DL is the ability to learn massive amounts of data. The DL field has grown fast in the last few years and it has been extensively used to successfully address a wide range of traditional applications. More importantly, DL has outperformed well-known ML techniques in many domains, e.g., cybersecurity, natural language processing, bioinformatics, robotics and control, and medical information processing, among many others. Despite it has been contributed several works reviewing the State-of-the-Art on DL, all of them only tackled one aspect of the DL, which leads to an overall lack of knowledge about it. Therefore, in this contribution, we propose using a more holistic approach in order to provide a more suitable starting point from which to develop a full understanding of DL. Specifically, this review attempts to provide a more comprehensive survey of the most important aspects of DL and including those enhancements recently added to the field. In particular, this paper outlines the importance of DL, presents the types of DL techniques and networks. It then presents convolutional neural networks (CNNs) which the most utilized DL network type and describes the development of CNNs architectures together with their main features, e.g., starting with the AlexNet network and closing with the High-Resolution network (HR.Net). Finally, we further present the challenges and suggested solutions to help researchers understand the existing research gaps. It is followed by a list of the major DL applications. Computational tools including FPGA, GPU, and CPU are summarized along with a description of their influence on DL. The paper ends with the evolution matrix, benchmark datasets, and summary and conclusion.
Introduction
Recently, machine learning (ML) has become very widespread in research and has been incorporated in a variety of applications, including text mining, spam detection, video recommendation, image classification, and multimedia concept retrieval [ 1 , 2 , 3 , 4 , 5 , 6 ]. Among the different ML algorithms, deep learning (DL) is very commonly employed in these applications [ 7 , 8 , 9 ]. Another name for DL is representation learning (RL). The continuing appearance of novel studies in the fields of deep and distributed learning is due to both the unpredictable growth in the ability to obtain data and the amazing progress made in the hardware technologies, e.g. High Performance Computing (HPC) [ 10 ].
DL is derived from the conventional neural network but considerably outperforms its predecessors. Moreover, DL employs transformations and graph technologies simultaneously in order to build up multi-layer learning models. The most recently developed DL techniques have obtained good outstanding performance across a variety of applications, including audio and speech processing, visual data processing, natural language processing (NLP), among others [ 11 , 12 , 13 , 14 ].
Usually, the effectiveness of an ML algorithm is highly dependent on the integrity of the input-data representation. It has been shown that a suitable data representation provides an improved performance when compared to a poor data representation. Thus, a significant research trend in ML for many years has been feature engineering, which has informed numerous research studies. This approach aims at constructing features from raw data. In addition, it is extremely field-specific and frequently requires sizable human effort. For instance, several types of features were introduced and compared in the computer vision context, such as, histogram of oriented gradients (HOG) [ 15 ], scale-invariant feature transform (SIFT) [ 16 ], and bag of words (BoW) [ 17 ]. As soon as a novel feature is introduced and is found to perform well, it becomes a new research direction that is pursued over multiple decades.
Relatively speaking, feature extraction is achieved in an automatic way throughout the DL algorithms. This encourages researchers to extract discriminative features using the smallest possible amount of human effort and field knowledge [ 18 ]. These algorithms have a multi-layer data representation architecture, in which the first layers extract the low-level features while the last layers extract the high-level features. Note that artificial intelligence (AI) originally inspired this type of architecture, which simulates the process that occurs in core sensorial regions within the human brain. Using different scenes, the human brain can automatically extract data representation. More specifically, the output of this process is the classified objects, while the received scene information represents the input. This process simulates the working methodology of the human brain. Thus, it emphasizes the main benefit of DL.
In the field of ML, DL, due to its considerable success, is currently one of the most prominent research trends. In this paper, an overview of DL is presented that adopts various perspectives such as the main concepts, architectures, challenges, applications, computational tools and evolution matrix. Convolutional neural network (CNN) is one of the most popular and used of DL networks [ 19 , 20 ]. Because of CNN, DL is very popular nowadays. The main advantage of CNN compared to its predecessors is that it automatically detects the significant features without any human supervision which made it the most used. Therefore, we have dug in deep with CNN by presenting the main components of it. Furthermore, we have elaborated in detail the most common CNN architectures, starting with the AlexNet network and ending with the High-Resolution network (HR.Net).
Several published DL review papers have been presented in the last few years. However, all of them have only been addressed one side focusing on one application or topic such as the review of CNN architectures [ 21 ], DL for classification of plant diseases [ 22 ], DL for object detection [ 23 ], DL applications in medical image analysis [ 24 ], and etc. Although these reviews present good topics, they do not provide a full understanding of DL topics such as concepts, detailed research gaps, computational tools, and DL applications. First, It is required to understand DL aspects including concepts, challenges, and applications then going deep in the applications. To achieve that, it requires extensive time and a large number of research papers to learn about DL including research gaps and applications. Therefore, we propose a deep review of DL to provide a more suitable starting point from which to develop a full understanding of DL from one review paper. The motivation behinds our review was to cover the most important aspect of DL including open challenges, applications, and computational tools perspective. Furthermore, our review can be the first step towards other DL topics.
The main aim of this review is to present the most important aspects of DL to make it easy for researchers and students to have a clear image of DL from single review paper. This review will further advance DL research by helping people discover more about recent developments in the field. Researchers would be allowed to decide the more suitable direction of work to be taken in order to provide more accurate alternatives to the field. Our contributions are outlined as follows:
This is the first review that almost provides a deep survey of the most important aspects of deep learning. This review helps researchers and students to have a good understanding from one paper.
We explain CNN in deep which the most popular deep learning algorithm by describing the concepts, theory, and state-of-the-art architectures.
We review current challenges (limitations) of Deep Learning including lack of training data, Imbalanced Data, Interpretability of data, Uncertainty scaling, Catastrophic forgetting, Model compression, Overfitting, Vanishing gradient problem, Exploding Gradient Problem, and Underspecification. We additionally discuss the proposed solutions tackling these issues.
We provide an exhaustive list of medical imaging applications with deep learning by categorizing them based on the tasks by starting with classification and ending with registration.
We discuss the computational approaches (CPU, GPU, FPGA) by comparing the influence of each tool on deep learning algorithms.
The rest of the paper is organized as follows: “ Survey methodology ” section describes The survey methodology. “ Background ” section presents the background. “ Classification of DL approaches ” section defines the classification of DL approaches. “ Types of DL networks ” section displays types of DL networks. “ CNN architectures ” section shows CNN Architectures. “ Challenges (limitations) of deep learning and alternate solutions ” section details the challenges of DL and alternate solutions. “ Applications of deep learning ” section outlines the applications of DL. “ Computational approaches ” section explains the influence of computational approaches (CPU, GPU, FPGA) on DL. “ Evaluation metrics ” section presents the evaluation metrics. “ Frameworks and datasets ” section lists frameworks and datasets. “ Summary and conclusion ” section presents the summary and conclusion.
Survey methodology
We have reviewed the significant research papers in the field published during 2010–2020, mainly from the years of 2020 and 2019 with some papers from 2021. The main focus was papers from the most reputed publishers such as IEEE, Elsevier, MDPI, Nature, ACM, and Springer. Some papers have been selected from ArXiv. We have reviewed more than 300 papers on various DL topics. There are 108 papers from the year 2020, 76 papers from the year 2019, and 48 papers from the year 2018. This indicates that this review focused on the latest publications in the field of DL. The selected papers were analyzed and reviewed to (1) list and define the DL approaches and network types, (2) list and explain CNN architectures, (3) present the challenges of DL and suggest the alternate solutions, (4) assess the applications of DL, (5) assess computational approaches. The most keywords used for search criteria for this review paper are (“Deep Learning”), (“Machine Learning”), (“Convolution Neural Network”), (“Deep Learning” AND “Architectures”), ((“Deep Learning”) AND (“Image”) AND (“detection” OR “classification” OR “segmentation” OR “Localization”)), (“Deep Learning” AND “detection” OR “classification” OR “segmentation” OR “Localization”), (“Deep Learning” AND “CPU” OR “GPU” OR “FPGA”), (“Deep Learning” AND “Transfer Learning”), (“Deep Learning” AND “Imbalanced Data”), (“Deep Learning” AND “Interpretability of data”), (“Deep Learning” AND “Overfitting”), (“Deep Learning” AND “Underspecification”). Figure 1 shows our search structure of the survey paper. Table 1 presents the details of some of the journals that have been cited in this review paper.
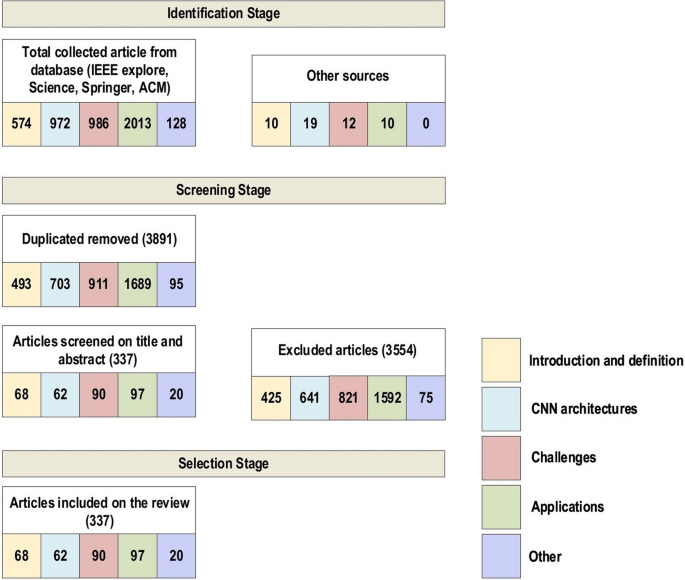
Search framework
This section will present a background of DL. We begin with a quick introduction to DL, followed by the difference between DL and ML. We then show the situations that require DL. Finally, we present the reasons for applying DL.
DL, a subset of ML (Fig. 2 ), is inspired by the information processing patterns found in the human brain. DL does not require any human-designed rules to operate; rather, it uses a large amount of data to map the given input to specific labels. DL is designed using numerous layers of algorithms (artificial neural networks, or ANNs), each of which provides a different interpretation of the data that has been fed to them [ 18 , 25 ].
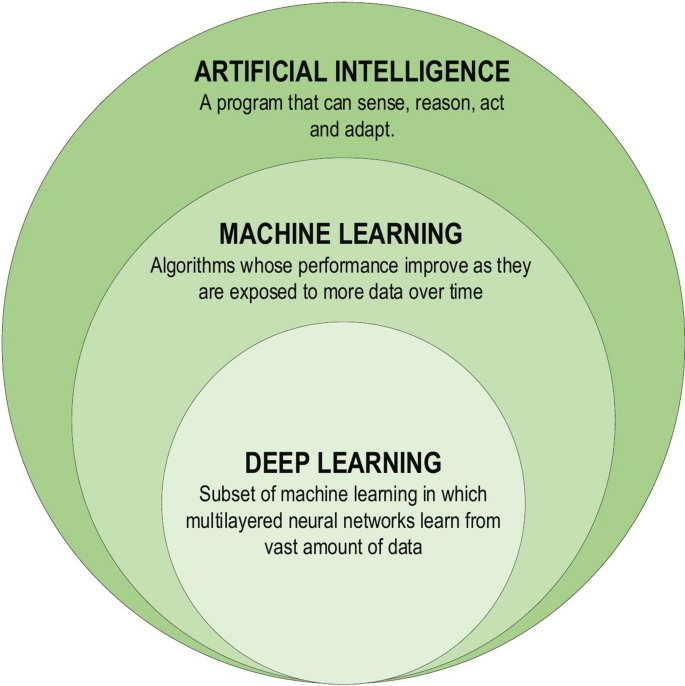
Deep learning family
Achieving the classification task using conventional ML techniques requires several sequential steps, specifically pre-processing, feature extraction, wise feature selection, learning, and classification. Furthermore, feature selection has a great impact on the performance of ML techniques. Biased feature selection may lead to incorrect discrimination between classes. Conversely, DL has the ability to automate the learning of feature sets for several tasks, unlike conventional ML methods [ 18 , 26 ]. DL enables learning and classification to be achieved in a single shot (Fig. 3 ). DL has become an incredibly popular type of ML algorithm in recent years due to the huge growth and evolution of the field of big data [ 27 , 28 ]. It is still in continuous development regarding novel performance for several ML tasks [ 22 , 29 , 30 , 31 ] and has simplified the improvement of many learning fields [ 32 , 33 ], such as image super-resolution [ 34 ], object detection [ 35 , 36 ], and image recognition [ 30 , 37 ]. Recently, DL performance has come to exceed human performance on tasks such as image classification (Fig. 4 ).
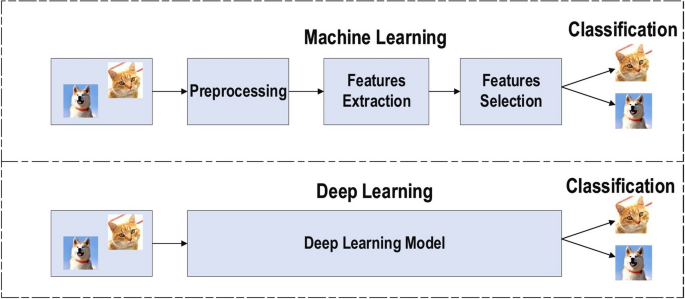
The difference between deep learning and traditional machine learning
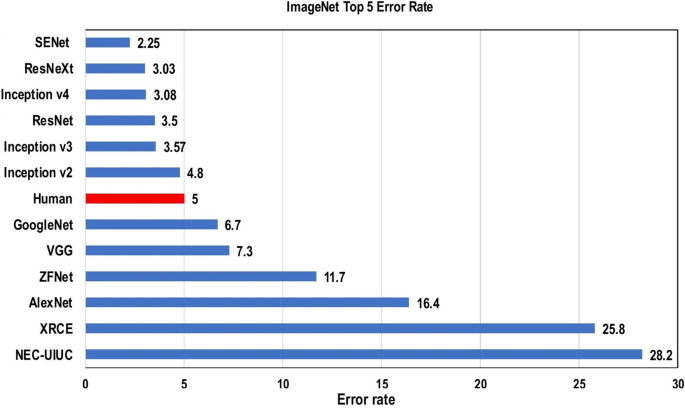
Deep learning performance compared to human
Nearly all scientific fields have felt the impact of this technology. Most industries and businesses have already been disrupted and transformed through the use of DL. The leading technology and economy-focused companies around the world are in a race to improve DL. Even now, human-level performance and capability cannot exceed that the performance of DL in many areas, such as predicting the time taken to make car deliveries, decisions to certify loan requests, and predicting movie ratings [ 38 ]. The winners of the 2019 “Nobel Prize” in computing, also known as the Turing Award, were three pioneers in the field of DL (Yann LeCun, Geoffrey Hinton, and Yoshua Bengio) [ 39 ]. Although a large number of goals have been achieved, there is further progress to be made in the DL context. In fact, DL has the ability to enhance human lives by providing additional accuracy in diagnosis, including estimating natural disasters [ 40 ], the discovery of new drugs [ 41 ], and cancer diagnosis [ 42 , 43 , 44 ]. Esteva et al. [ 45 ] found that a DL network has the same ability to diagnose the disease as twenty-one board-certified dermatologists using 129,450 images of 2032 diseases. Furthermore, in grading prostate cancer, US board-certified general pathologists achieved an average accuracy of 61%, while the Google AI [ 44 ] outperformed these specialists by achieving an average accuracy of 70%. In 2020, DL is playing an increasingly vital role in early diagnosis of the novel coronavirus (COVID-19) [ 29 , 46 , 47 , 48 ]. DL has become the main tool in many hospitals around the world for automatic COVID-19 classification and detection using chest X-ray images or other types of images. We end this section by the saying of AI pioneer Geoffrey Hinton “Deep learning is going to be able to do everything”.
When to apply deep learning
Machine intelligence is useful in many situations which is equal or better than human experts in some cases [ 49 , 50 , 51 , 52 ], meaning that DL can be a solution to the following problems:
Cases where human experts are not available.
Cases where humans are unable to explain decisions made using their expertise (language understanding, medical decisions, and speech recognition).
Cases where the problem solution updates over time (price prediction, stock preference, weather prediction, and tracking).
Cases where solutions require adaptation based on specific cases (personalization, biometrics).
Cases where size of the problem is extremely large and exceeds our inadequate reasoning abilities (sentiment analysis, matching ads to Facebook, calculation webpage ranks).
Why deep learning?
Several performance features may answer this question, e.g
Universal Learning Approach: Because DL has the ability to perform in approximately all application domains, it is sometimes referred to as universal learning.
Robustness: In general, precisely designed features are not required in DL techniques. Instead, the optimized features are learned in an automated fashion related to the task under consideration. Thus, robustness to the usual changes of the input data is attained.
Generalization: Different data types or different applications can use the same DL technique, an approach frequently referred to as transfer learning (TL) which explained in the latter section. Furthermore, it is a useful approach in problems where data is insufficient.
Scalability: DL is highly scalable. ResNet [ 37 ], which was invented by Microsoft, comprises 1202 layers and is frequently applied at a supercomputing scale. Lawrence Livermore National Laboratory (LLNL), a large enterprise working on evolving frameworks for networks, adopted a similar approach, where thousands of nodes can be implemented [ 53 ].
Classification of DL approaches
DL techniques are classified into three major categories: unsupervised, partially supervised (semi-supervised) and supervised. Furthermore, deep reinforcement learning (DRL), also known as RL, is another type of learning technique, which is mostly considered to fall into the category of partially supervised (and occasionally unsupervised) learning techniques.
Deep supervised learning
Deep semi-supervised learning.
In this technique, the learning process is based on semi-labeled datasets. Occasionally, generative adversarial networks (GANs) and DRL are employed in the same way as this technique. In addition, RNNs, which include GRUs and LSTMs, are also employed for partially supervised learning. One of the advantages of this technique is to minimize the amount of labeled data needed. On other the hand, One of the disadvantages of this technique is irrelevant input feature present training data could furnish incorrect decisions. Text document classifier is one of the most popular example of an application of semi-supervised learning. Due to difficulty of obtaining a large amount of labeled text documents, semi-supervised learning is ideal for text document classification task.
Deep unsupervised learning
This technique makes it possible to implement the learning process in the absence of available labeled data (i.e. no labels are required). Here, the agent learns the significant features or interior representation required to discover the unidentified structure or relationships in the input data. Techniques of generative networks, dimensionality reduction and clustering are frequently counted within the category of unsupervised learning. Several members of the DL family have performed well on non-linear dimensionality reduction and clustering tasks; these include restricted Boltzmann machines, auto-encoders and GANs as the most recently developed techniques. Moreover, RNNs, which include GRUs and LSTM approaches, have also been employed for unsupervised learning in a wide range of applications. The main disadvantages of unsupervised learning are unable to provide accurate information concerning data sorting and computationally complex. One of the most popular unsupervised learning approaches is clustering [ 54 ].
Deep reinforcement learning
For solving a task, the selection of the type of reinforcement learning that needs to be performed is based on the space or the scope of the problem. For example, DRL is the best way for problems involving many parameters to be optimized. By contrast, derivative-free reinforcement learning is a technique that performs well for problems with limited parameters. Some of the applications of reinforcement learning are business strategy planning and robotics for industrial automation. The main drawback of Reinforcement Learning is that parameters may influence the speed of learning. Here are the main motivations for utilizing Reinforcement Learning:
It assists you to identify which action produces the highest reward over a longer period.
It assists you to discover which situation requires action.
It also enables it to figure out the best approach for reaching large rewards.
Reinforcement Learning also gives the learning agent a reward function.
Reinforcement Learning can’t utilize in all the situation such as:
In case there is sufficient data to resolve the issue with supervised learning techniques.
Reinforcement Learning is computing-heavy and time-consuming. Specially when the workspace is large.
Types of DL networks
The most famous types of deep learning networks are discussed in this section: these include recursive neural networks (RvNNs), RNNs, and CNNs. RvNNs and RNNs were briefly explained in this section while CNNs were explained in deep due to the importance of this type. Furthermore, it is the most used in several applications among other networks.
Recursive neural networks
RvNN can achieve predictions in a hierarchical structure also classify the outputs utilizing compositional vectors [ 57 ]. Recursive auto-associative memory (RAAM) [ 58 ] is the primary inspiration for the RvNN development. The RvNN architecture is generated for processing objects, which have randomly shaped structures like graphs or trees. This approach generates a fixed-width distributed representation from a variable-size recursive-data structure. The network is trained using an introduced back-propagation through structure (BTS) learning system [ 58 ]. The BTS system tracks the same technique as the general-back propagation algorithm and has the ability to support a treelike structure. Auto-association trains the network to regenerate the input-layer pattern at the output layer. RvNN is highly effective in the NLP context. Socher et al. [ 59 ] introduced RvNN architecture designed to process inputs from a variety of modalities. These authors demonstrate two applications for classifying natural language sentences: cases where each sentence is split into words and nature images, and cases where each image is separated into various segments of interest. RvNN computes a likely pair of scores for merging and constructs a syntactic tree. Furthermore, RvNN calculates a score related to the merge plausibility for every pair of units. Next, the pair with the largest score is merged within a composition vector. Following every merge, RvNN generates (a) a larger area of numerous units, (b) a compositional vector of the area, and (c) a label for the class (for instance, a noun phrase will become the class label for the new area if two units are noun words). The compositional vector for the entire area is the root of the RvNN tree structure. An example RvNN tree is shown in Fig. 5 . RvNN has been employed in several applications [ 60 , 61 , 62 ].
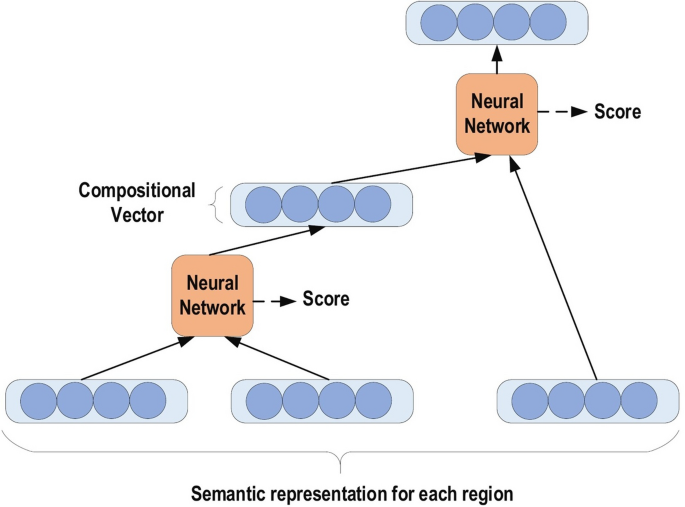
An example of RvNN tree
Recurrent neural networks
RNNs are a commonly employed and familiar algorithm in the discipline of DL [ 63 , 64 , 65 ]. RNN is mainly applied in the area of speech processing and NLP contexts [ 66 , 67 ]. Unlike conventional networks, RNN uses sequential data in the network. Since the embedded structure in the sequence of the data delivers valuable information, this feature is fundamental to a range of different applications. For instance, it is important to understand the context of the sentence in order to determine the meaning of a specific word in it. Thus, it is possible to consider the RNN as a unit of short-term memory, where x represents the input layer, y is the output layer, and s represents the state (hidden) layer. For a given input sequence, a typical unfolded RNN diagram is illustrated in Fig. 6 . Pascanu et al. [ 68 ] introduced three different types of deep RNN techniques, namely “Hidden-to-Hidden”, “Hidden-to-Output”, and “Input-to-Hidden”. A deep RNN is introduced that lessens the learning difficulty in the deep network and brings the benefits of a deeper RNN based on these three techniques.
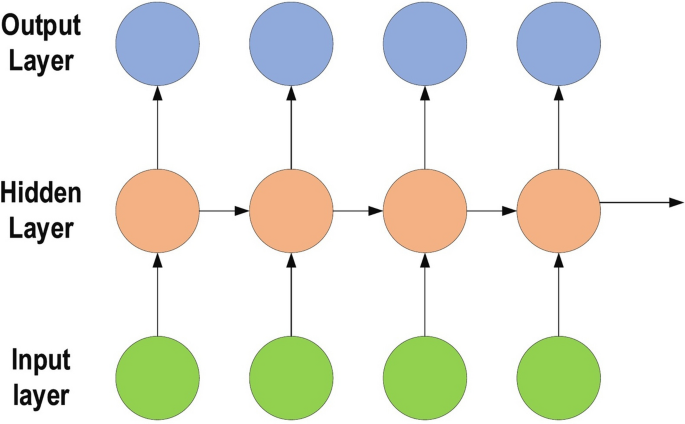
Typical unfolded RNN diagram
However, RNN’s sensitivity to the exploding gradient and vanishing problems represent one of the main issues with this approach [ 69 ]. More specifically, during the training process, the reduplications of several large or small derivatives may cause the gradients to exponentially explode or decay. With the entrance of new inputs, the network stops thinking about the initial ones; therefore, this sensitivity decays over time. Furthermore, this issue can be handled using LSTM [ 70 ]. This approach offers recurrent connections to memory blocks in the network. Every memory block contains a number of memory cells, which have the ability to store the temporal states of the network. In addition, it contains gated units for controlling the flow of information. In very deep networks [ 37 ], residual connections also have the ability to considerably reduce the impact of the vanishing gradient issue which explained in later sections. CNN is considered to be more powerful than RNN. RNN includes less feature compatibility when compared to CNN.
Convolutional neural networks
In the field of DL, the CNN is the most famous and commonly employed algorithm [ 30 , 71 , 72 , 73 , 74 , 75 ]. The main benefit of CNN compared to its predecessors is that it automatically identifies the relevant features without any human supervision [ 76 ]. CNNs have been extensively applied in a range of different fields, including computer vision [ 77 ], speech processing [ 78 ], Face Recognition [ 79 ], etc. The structure of CNNs was inspired by neurons in human and animal brains, similar to a conventional neural network. More specifically, in a cat’s brain, a complex sequence of cells forms the visual cortex; this sequence is simulated by the CNN [ 80 ]. Goodfellow et al. [ 28 ] identified three key benefits of the CNN: equivalent representations, sparse interactions, and parameter sharing. Unlike conventional fully connected (FC) networks, shared weights and local connections in the CNN are employed to make full use of 2D input-data structures like image signals. This operation utilizes an extremely small number of parameters, which both simplifies the training process and speeds up the network. This is the same as in the visual cortex cells. Notably, only small regions of a scene are sensed by these cells rather than the whole scene (i.e., these cells spatially extract the local correlation available in the input, like local filters over the input).
A commonly used type of CNN, which is similar to the multi-layer perceptron (MLP), consists of numerous convolution layers preceding sub-sampling (pooling) layers, while the ending layers are FC layers. An example of CNN architecture for image classification is illustrated in Fig. 7 .
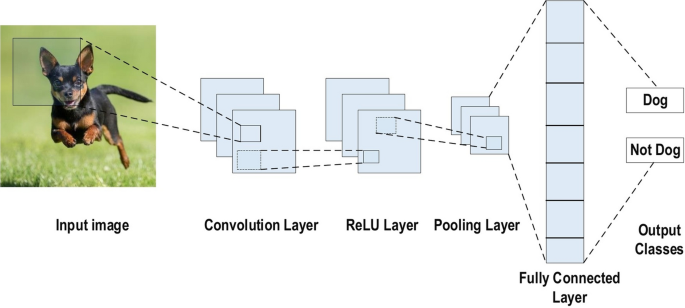
An example of CNN architecture for image classification
The input x of each layer in a CNN model is organized in three dimensions: height, width, and depth, or \(m \times m \times r\) , where the height (m) is equal to the width. The depth is also referred to as the channel number. For example, in an RGB image, the depth (r) is equal to three. Several kernels (filters) available in each convolutional layer are denoted by k and also have three dimensions ( \(n \times n \times q\) ), similar to the input image; here, however, n must be smaller than m , while q is either equal to or smaller than r . In addition, the kernels are the basis of the local connections, which share similar parameters (bias \(b^{k}\) and weight \(W^{k}\) ) for generating k feature maps \(h^{k}\) with a size of ( \(m-n-1\) ) each and are convolved with input, as mentioned above. The convolution layer calculates a dot product between its input and the weights as in Eq. 1 , similar to NLP, but the inputs are undersized areas of the initial image size. Next, by applying the nonlinearity or an activation function to the convolution-layer output, we obtain the following:
The next step is down-sampling every feature map in the sub-sampling layers. This leads to a reduction in the network parameters, which accelerates the training process and in turn enables handling of the overfitting issue. For all feature maps, the pooling function (e.g. max or average) is applied to an adjacent area of size \(p \times p\) , where p is the kernel size. Finally, the FC layers receive the mid- and low-level features and create the high-level abstraction, which represents the last-stage layers as in a typical neural network. The classification scores are generated using the ending layer [e.g. support vector machines (SVMs) or softmax]. For a given instance, every score represents the probability of a specific class.
Benefits of employing CNNs
The benefits of using CNNs over other traditional neural networks in the computer vision environment are listed as follows:
The main reason to consider CNN is the weight sharing feature, which reduces the number of trainable network parameters and in turn helps the network to enhance generalization and to avoid overfitting.
Concurrently learning the feature extraction layers and the classification layer causes the model output to be both highly organized and highly reliant on the extracted features.
Large-scale network implementation is much easier with CNN than with other neural networks.
The CNN architecture consists of a number of layers (or so-called multi-building blocks). Each layer in the CNN architecture, including its function, is described in detail below.
Convolutional Layer: In CNN architecture, the most significant component is the convolutional layer. It consists of a collection of convolutional filters (so-called kernels). The input image, expressed as N-dimensional metrics, is convolved with these filters to generate the output feature map.
Kernel definition: A grid of discrete numbers or values describes the kernel. Each value is called the kernel weight. Random numbers are assigned to act as the weights of the kernel at the beginning of the CNN training process. In addition, there are several different methods used to initialize the weights. Next, these weights are adjusted at each training era; thus, the kernel learns to extract significant features.
Convolutional Operation: Initially, the CNN input format is described. The vector format is the input of the traditional neural network, while the multi-channeled image is the input of the CNN. For instance, single-channel is the format of the gray-scale image, while the RGB image format is three-channeled. To understand the convolutional operation, let us take an example of a \(4 \times 4\) gray-scale image with a \(2 \times 2\) random weight-initialized kernel. First, the kernel slides over the whole image horizontally and vertically. In addition, the dot product between the input image and the kernel is determined, where their corresponding values are multiplied and then summed up to create a single scalar value, calculated concurrently. The whole process is then repeated until no further sliding is possible. Note that the calculated dot product values represent the feature map of the output. Figure 8 graphically illustrates the primary calculations executed at each step. In this figure, the light green color represents the \(2 \times 2\) kernel, while the light blue color represents the similar size area of the input image. Both are multiplied; the end result after summing up the resulting product values (marked in a light orange color) represents an entry value to the output feature map.
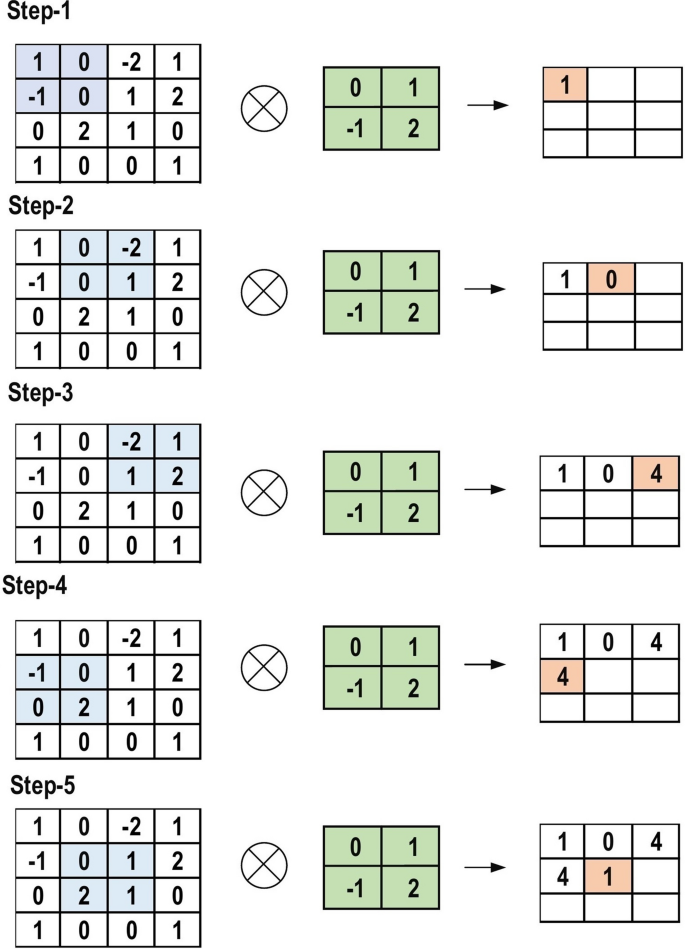
The primary calculations executed at each step of convolutional layer
However, padding to the input image is not applied in the previous example, while a stride of one (denoted for the selected step-size over all vertical or horizontal locations) is applied to the kernel. Note that it is also possible to use another stride value. In addition, a feature map of lower dimensions is obtained as a result of increasing the stride value.
On the other hand, padding is highly significant to determining border size information related to the input image. By contrast, the border side-features moves carried away very fast. By applying padding, the size of the input image will increase, and in turn, the size of the output feature map will also increase. Core Benefits of Convolutional Layers.
Sparse Connectivity: Each neuron of a layer in FC neural networks links with all neurons in the following layer. By contrast, in CNNs, only a few weights are available between two adjacent layers. Thus, the number of required weights or connections is small, while the memory required to store these weights is also small; hence, this approach is memory-effective. In addition, matrix operation is computationally much more costly than the dot (.) operation in CNN.
Weight Sharing: There are no allocated weights between any two neurons of neighboring layers in CNN, as the whole weights operate with one and all pixels of the input matrix. Learning a single group of weights for the whole input will significantly decrease the required training time and various costs, as it is not necessary to learn additional weights for each neuron.
Pooling Layer: The main task of the pooling layer is the sub-sampling of the feature maps. These maps are generated by following the convolutional operations. In other words, this approach shrinks large-size feature maps to create smaller feature maps. Concurrently, it maintains the majority of the dominant information (or features) in every step of the pooling stage. In a similar manner to the convolutional operation, both the stride and the kernel are initially size-assigned before the pooling operation is executed. Several types of pooling methods are available for utilization in various pooling layers. These methods include tree pooling, gated pooling, average pooling, min pooling, max pooling, global average pooling (GAP), and global max pooling. The most familiar and frequently utilized pooling methods are the max, min, and GAP pooling. Figure 9 illustrates these three pooling operations.
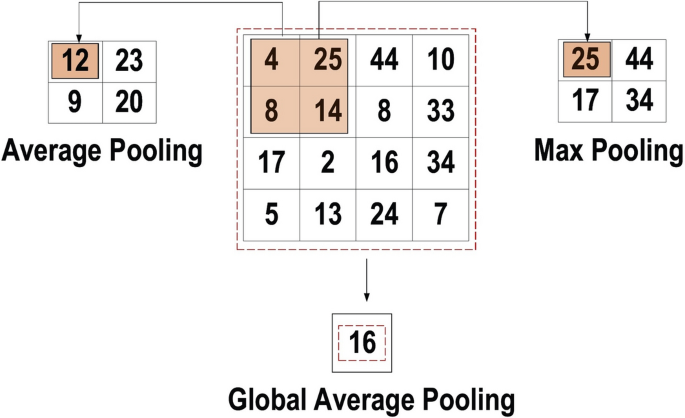
Three types of pooling operations
Sometimes, the overall CNN performance is decreased as a result; this represents the main shortfall of the pooling layer, as this layer helps the CNN to determine whether or not a certain feature is available in the particular input image, but focuses exclusively on ascertaining the correct location of that feature. Thus, the CNN model misses the relevant information.
Activation Function (non-linearity) Mapping the input to the output is the core function of all types of activation function in all types of neural network. The input value is determined by computing the weighted summation of the neuron input along with its bias (if present). This means that the activation function makes the decision as to whether or not to fire a neuron with reference to a particular input by creating the corresponding output.
Non-linear activation layers are employed after all layers with weights (so-called learnable layers, such as FC layers and convolutional layers) in CNN architecture. This non-linear performance of the activation layers means that the mapping of input to output will be non-linear; moreover, these layers give the CNN the ability to learn extra-complicated things. The activation function must also have the ability to differentiate, which is an extremely significant feature, as it allows error back-propagation to be used to train the network. The following types of activation functions are most commonly used in CNN and other deep neural networks.
Sigmoid: The input of this activation function is real numbers, while the output is restricted to between zero and one. The sigmoid function curve is S-shaped and can be represented mathematically by Eq. 2 .
Tanh: It is similar to the sigmoid function, as its input is real numbers, but the output is restricted to between − 1 and 1. Its mathematical representation is in Eq. 3 .
ReLU: The mostly commonly used function in the CNN context. It converts the whole values of the input to positive numbers. Lower computational load is the main benefit of ReLU over the others. Its mathematical representation is in Eq. 4 .
Occasionally, a few significant issues may occur during the use of ReLU. For instance, consider an error back-propagation algorithm with a larger gradient flowing through it. Passing this gradient within the ReLU function will update the weights in a way that makes the neuron certainly not activated once more. This issue is referred to as “Dying ReLU”. Some ReLU alternatives exist to solve such issues. The following discusses some of them.
Leaky ReLU: Instead of ReLU down-scaling the negative inputs, this activation function ensures these inputs are never ignored. It is employed to solve the Dying ReLU problem. Leaky ReLU can be represented mathematically as in Eq. 5 .
Note that the leak factor is denoted by m. It is commonly set to a very small value, such as 0.001.
Noisy ReLU: This function employs a Gaussian distribution to make ReLU noisy. It can be represented mathematically as in Eq. 6 .
Parametric Linear Units: This is mostly the same as Leaky ReLU. The main difference is that the leak factor in this function is updated through the model training process. The parametric linear unit can be represented mathematically as in Eq. 7 .
Note that the learnable weight is denoted as a.
Fully Connected Layer: Commonly, this layer is located at the end of each CNN architecture. Inside this layer, each neuron is connected to all neurons of the previous layer, the so-called Fully Connected (FC) approach. It is utilized as the CNN classifier. It follows the basic method of the conventional multiple-layer perceptron neural network, as it is a type of feed-forward ANN. The input of the FC layer comes from the last pooling or convolutional layer. This input is in the form of a vector, which is created from the feature maps after flattening. The output of the FC layer represents the final CNN output, as illustrated in Fig. 10 .
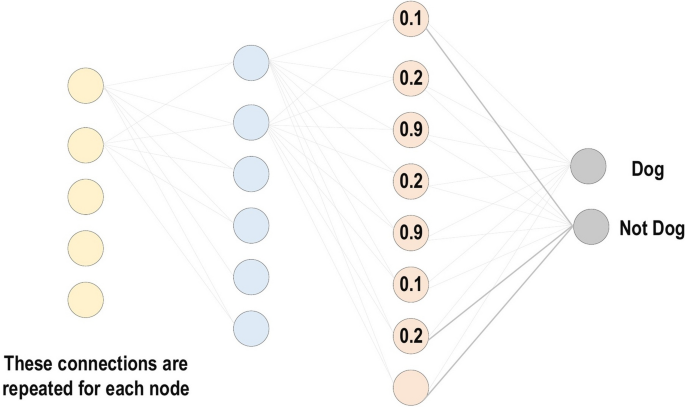
Fully connected layer
Loss Functions: The previous section has presented various layer-types of CNN architecture. In addition, the final classification is achieved from the output layer, which represents the last layer of the CNN architecture. Some loss functions are utilized in the output layer to calculate the predicted error created across the training samples in the CNN model. This error reveals the difference between the actual output and the predicted one. Next, it will be optimized through the CNN learning process.
However, two parameters are used by the loss function to calculate the error. The CNN estimated output (referred to as the prediction) is the first parameter. The actual output (referred to as the label) is the second parameter. Several types of loss function are employed in various problem types. The following concisely explains some of the loss function types.
Cross-Entropy or Softmax Loss Function: This function is commonly employed for measuring the CNN model performance. It is also referred to as the log loss function. Its output is the probability \(p \in \left\{ 0\left. , 1 \right\} \right. \) . In addition, it is usually employed as a substitution of the square error loss function in multi-class classification problems. In the output layer, it employs the softmax activations to generate the output within a probability distribution. The mathematical representation of the output class probability is Eq. 8 .
Here, \(e^{a_{i}}\) represents the non-normalized output from the preceding layer, while N represents the number of neurons in the output layer. Finally, the mathematical representation of cross-entropy loss function is Eq. 9 .
Euclidean Loss Function: This function is widely used in regression problems. In addition, it is also the so-called mean square error. The mathematical expression of the estimated Euclidean loss is Eq. 10 .
Hinge Loss Function: This function is commonly employed in problems related to binary classification. This problem relates to maximum-margin-based classification; this is mostly important for SVMs, which use the hinge loss function, wherein the optimizer attempts to maximize the margin around dual objective classes. Its mathematical formula is Eq. 11 .
The margin m is commonly set to 1. Moreover, the predicted output is denoted as \(p_{_{i}}\) , while the desired output is denoted as \(y_{_{i}}\) .
Regularization to CNN
For CNN models, over-fitting represents the central issue associated with obtaining well-behaved generalization. The model is entitled over-fitted in cases where the model executes especially well on training data and does not succeed on test data (unseen data) which is more explained in the latter section. An under-fitted model is the opposite; this case occurs when the model does not learn a sufficient amount from the training data. The model is referred to as “just-fitted” if it executes well on both training and testing data. These three types are illustrated in Fig. 11 . Various intuitive concepts are used to help the regularization to avoid over-fitting; more details about over-fitting and under-fitting are discussed in latter sections.
Dropout: This is a widely utilized technique for generalization. During each training epoch, neurons are randomly dropped. In doing this, the feature selection power is distributed equally across the whole group of neurons, as well as forcing the model to learn different independent features. During the training process, the dropped neuron will not be a part of back-propagation or forward-propagation. By contrast, the full-scale network is utilized to perform prediction during the testing process.
Drop-Weights: This method is highly similar to dropout. In each training epoch, the connections between neurons (weights) are dropped rather than dropping the neurons; this represents the only difference between drop-weights and dropout.
Data Augmentation: Training the model on a sizeable amount of data is the easiest way to avoid over-fitting. To achieve this, data augmentation is used. Several techniques are utilized to artificially expand the size of the training dataset. More details can be found in the latter section, which describes the data augmentation techniques.
Batch Normalization: This method ensures the performance of the output activations [ 81 ]. This performance follows a unit Gaussian distribution. Subtracting the mean and dividing by the standard deviation will normalize the output at each layer. While it is possible to consider this as a pre-processing task at each layer in the network, it is also possible to differentiate and to integrate it with other networks. In addition, it is employed to reduce the “internal covariance shift” of the activation layers. In each layer, the variation in the activation distribution defines the internal covariance shift. This shift becomes very high due to the continuous weight updating through training, which may occur if the samples of the training data are gathered from numerous dissimilar sources (for example, day and night images). Thus, the model will consume extra time for convergence, and in turn, the time required for training will also increase. To resolve this issue, a layer representing the operation of batch normalization is applied in the CNN architecture.
The advantages of utilizing batch normalization are as follows:
It prevents the problem of vanishing gradient from arising.
It can effectively control the poor weight initialization.
It significantly reduces the time required for network convergence (for large-scale datasets, this will be extremely useful).
It struggles to decrease training dependency across hyper-parameters.
Chances of over-fitting are reduced, since it has a minor influence on regularization.
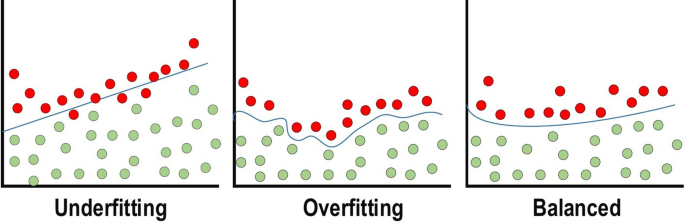
Over-fitting and under-fitting issues
Optimizer selection
This section discusses the CNN learning process. Two major issues are included in the learning process: the first issue is the learning algorithm selection (optimizer), while the second issue is the use of many enhancements (such as AdaDelta, Adagrad, and momentum) along with the learning algorithm to enhance the output.
Loss functions, which are founded on numerous learnable parameters (e.g. biases, weights, etc.) or minimizing the error (variation between actual and predicted output), are the core purpose of all supervised learning algorithms. The techniques of gradient-based learning for a CNN network appear as the usual selection. The network parameters should always update though all training epochs, while the network should also look for the locally optimized answer in all training epochs in order to minimize the error.
The learning rate is defined as the step size of the parameter updating. The training epoch represents a complete repetition of the parameter update that involves the complete training dataset at one time. Note that it needs to select the learning rate wisely so that it does not influence the learning process imperfectly, although it is a hyper-parameter.
Gradient Descent or Gradient-based learning algorithm: To minimize the training error, this algorithm repetitively updates the network parameters through every training epoch. More specifically, to update the parameters correctly, it needs to compute the objective function gradient (slope) by applying a first-order derivative with respect to the network parameters. Next, the parameter is updated in the reverse direction of the gradient to reduce the error. The parameter updating process is performed though network back-propagation, in which the gradient at every neuron is back-propagated to all neurons in the preceding layer. The mathematical representation of this operation is as Eq. 12 .
The final weight in the current training epoch is denoted by \(w_{i j^{t}}\) , while the weight in the preceding \((t-1)\) training epoch is denoted \(w_{i j^{t-1}}\) . The learning rate is \(\eta \) and the prediction error is E . Different alternatives of the gradient-based learning algorithm are available and commonly employed; these include the following:
Batch Gradient Descent: During the execution of this technique [ 82 ], the network parameters are updated merely one time behind considering all training datasets via the network. In more depth, it calculates the gradient of the whole training set and subsequently uses this gradient to update the parameters. For a small-sized dataset, the CNN model converges faster and creates an extra-stable gradient using BGD. Since the parameters are changed only once for every training epoch, it requires a substantial amount of resources. By contrast, for a large training dataset, additional time is required for converging, and it could converge to a local optimum (for non-convex instances).
Stochastic Gradient Descent: The parameters are updated at each training sample in this technique [ 83 ]. It is preferred to arbitrarily sample the training samples in every epoch in advance of training. For a large-sized training dataset, this technique is both more memory-effective and much faster than BGD. However, because it is frequently updated, it takes extremely noisy steps in the direction of the answer, which in turn causes the convergence behavior to become highly unstable.
Mini-batch Gradient Descent: In this approach, the training samples are partitioned into several mini-batches, in which every mini-batch can be considered an under-sized collection of samples with no overlap between them [ 84 ]. Next, parameter updating is performed following gradient computation on every mini-batch. The advantage of this method comes from combining the advantages of both BGD and SGD techniques. Thus, it has a steady convergence, more computational efficiency and extra memory effectiveness. The following describes several enhancement techniques in gradient-based learning algorithms (usually in SGD), which further powerfully enhance the CNN training process.
Momentum: For neural networks, this technique is employed in the objective function. It enhances both the accuracy and the training speed by summing the computed gradient at the preceding training step, which is weighted via a factor \(\lambda \) (known as the momentum factor). However, it therefore simply becomes stuck in a local minimum rather than a global minimum. This represents the main disadvantage of gradient-based learning algorithms. Issues of this kind frequently occur if the issue has no convex surface (or solution space).
Together with the learning algorithm, momentum is used to solve this issue, which can be expressed mathematically as in Eq. 13 .
The weight increment in the current \(t^{\prime} \text{th}\) training epoch is denoted as \( \Delta w_{i j^{t}}\) , while \(\eta \) is the learning rate, and the weight increment in the preceding \((t-1)^{\prime} \text{th}\) training epoch. The momentum factor value is maintained within the range 0 to 1; in turn, the step size of the weight updating increases in the direction of the bare minimum to minimize the error. As the value of the momentum factor becomes very low, the model loses its ability to avoid the local bare minimum. By contrast, as the momentum factor value becomes high, the model develops the ability to converge much more rapidly. If a high value of momentum factor is used together with LR, then the model could miss the global bare minimum by crossing over it.
However, when the gradient varies its direction continually throughout the training process, then the suitable value of the momentum factor (which is a hyper-parameter) causes a smoothening of the weight updating variations.
Adaptive Moment Estimation (Adam): It is another optimization technique or learning algorithm that is widely used. Adam [ 85 ] represents the latest trends in deep learning optimization. This is represented by the Hessian matrix, which employs a second-order derivative. Adam is a learning strategy that has been designed specifically for training deep neural networks. More memory efficient and less computational power are two advantages of Adam. The mechanism of Adam is to calculate adaptive LR for each parameter in the model. It integrates the pros of both Momentum and RMSprop. It utilizes the squared gradients to scale the learning rate as RMSprop and it is similar to the momentum by using the moving average of the gradient. The equation of Adam is represented in Eq. 14 .
Design of algorithms (backpropagation)
Let’s start with a notation that refers to weights in the network unambiguously. We denote \({\varvec{w}}_{i j}^{h}\) to be the weight for the connection from \(\text {ith}\) input or (neuron at \(\left. (\text {h}-1){\text{th}}\right) \) to the \(j{\text{t }}\) neuron in the \(\text {hth}\) layer. So, Fig. 12 shows the weight on a connection from the neuron in the first layer to another neuron in the next layer in the network.
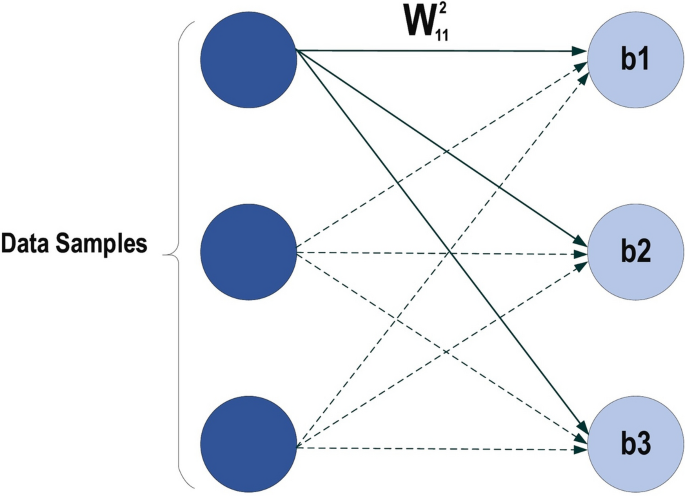
MLP structure
Where \(w_{11}^{2}\) has represented the weight from the first neuron in the first layer to the first neuron in the second layer, based on that the second weight for the same neuron will be \(w_{21}^{2}\) which means is the weight comes from the second neuron in the previous layer to the first layer in the next layer which is the second in this net. Regarding the bias, since the bias is not the connection between the neurons for the layers, so it is easily handled each neuron must have its own bias, some network each layer has a certain bias. It can be seen from the above net that each layer has its own bias. Each network has the parameters such as the no of the layer in the net, the number of the neurons in each layer, no of the weight (connection) between the layers, the no of connection can be easily determined based on the no of neurons in each layer, for example, if there are ten input fully connect with two neurons in the next layer then the number of connection between them is \((10 * 2=20\) connection, weights), how the error is defined, and the weight is updated, we will imagine there is there are two layers in our neural network,
where \(\text {d}\) is the label of induvial input \(\text {ith}\) and \(\text {y}\) is the output of the same individual input. Backpropagation is about understanding how to change the weights and biases in a network based on the changes of the cost function (Error). Ultimately, this means computing the partial derivatives \(\partial \text {E} / \partial \text {w}_{\text {ij}}^{h}\) and \(\partial \text {E} / \partial \text {b}_{\text {j}}^{h}.\) But to compute those, a local variable is introduced, \(\delta _{j}^{1}\) which is called the local error in the \(j{\text{th} }\) neuron in the \(h{\text{th} }\) layer. Based on that local error Backpropagation will give the procedure to compute \(\partial \text {E} / \partial \text {w}_{\text {ij}}^{h}\) and \(\partial \text {E} / \partial \text {b}_{\text {j}}^{h}\) how the error is defined, and the weight is updated, we will imagine there is there are two layers in our neural network that is shown in Fig. 13 .
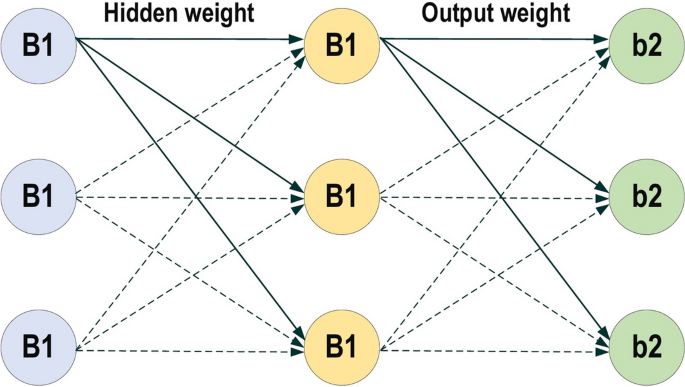
Neuron activation functions
Output error for \(\delta _{\text {j}}^{1}\) each \(1=1: \text {L}\) where \(\text {L}\) is no. of neuron in output
where \(\text {e}(\text {k})\) is the error of the epoch \(\text {k}\) as shown in Eq. ( 2 ) and \(\varvec{\vartheta }^{\prime }\left( {\varvec{v}}_{j}({\varvec{k}})\right) \) is the derivate of the activation function for \(v_{j}\) at the output.
Backpropagate the error at all the rest layer except the output
where \(\delta _{j}^{1}({\mathbf {k}})\) is the output error and \(w_{j l}^{h+1}(k)\) is represented the weight after the layer where the error need to obtain.
After finding the error at each neuron in each layer, now we can update the weight in each layer based on Eqs. ( 16 ) and ( 17 ).
Improving performance of CNN
Based on our experiments in different DL applications [ 86 , 87 , 88 ]. We can conclude the most active solutions that may improve the performance of CNN are:
Expand the dataset with data augmentation or use transfer learning (explained in latter sections).
Increase the training time.
Increase the depth (or width) of the model.
Add regularization.
Increase hyperparameters tuning.
CNN architectures
Over the last 10 years, several CNN architectures have been presented [ 21 , 26 ]. Model architecture is a critical factor in improving the performance of different applications. Various modifications have been achieved in CNN architecture from 1989 until today. Such modifications include structural reformulation, regularization, parameter optimizations, etc. Conversely, it should be noted that the key upgrade in CNN performance occurred largely due to the processing-unit reorganization, as well as the development of novel blocks. In particular, the most novel developments in CNN architectures were performed on the use of network depth. In this section, we review the most popular CNN architectures, beginning from the AlexNet model in 2012 and ending at the High-Resolution (HR) model in 2020. Studying these architectures features (such as input size, depth, and robustness) is the key to help researchers to choose the suitable architecture for the their target task. Table 2 presents the brief overview of CNN architectures.
The history of deep CNNs began with the appearance of LeNet [ 89 ] (Fig. 14 ). At that time, the CNNs were restricted to handwritten digit recognition tasks, which cannot be scaled to all image classes. In deep CNN architecture, AlexNet is highly respected [ 30 ], as it achieved innovative results in the fields of image recognition and classification. Krizhevesky et al. [ 30 ] first proposed AlexNet and consequently improved the CNN learning ability by increasing its depth and implementing several parameter optimization strategies. Figure 15 illustrates the basic design of the AlexNet architecture.
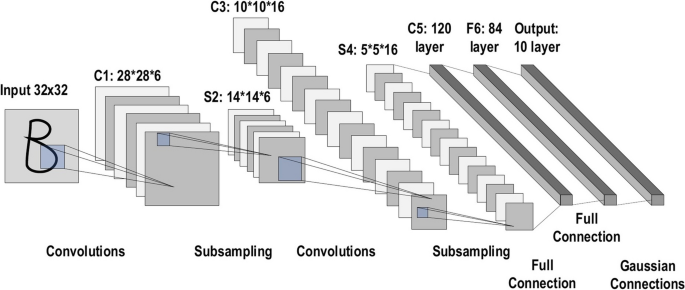
The architecture of LeNet
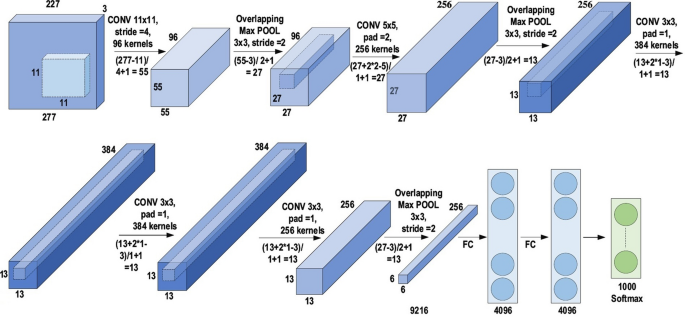
The architecture of AlexNet
The learning ability of the deep CNN was limited at this time due to hardware restrictions. To overcome these hardware limitations, two GPUs (NVIDIA GTX 580) were used in parallel to train AlexNet. Moreover, in order to enhance the applicability of the CNN to different image categories, the number of feature extraction stages was increased from five in LeNet to seven in AlexNet. Regardless of the fact that depth enhances generalization for several image resolutions, it was in fact overfitting that represented the main drawback related to the depth. Krizhevesky et al. used Hinton’s idea to address this problem [ 90 , 91 ]. To ensure that the features learned by the algorithm were extra robust, Krizhevesky et al.’s algorithm randomly passes over several transformational units throughout the training stage. Moreover, by reducing the vanishing gradient problem, ReLU [ 92 ] could be utilized as a non-saturating activation function to enhance the rate of convergence [ 93 ]. Local response normalization and overlapping subsampling were also performed to enhance the generalization by decreasing the overfitting. To improve on the performance of previous networks, other modifications were made by using large-size filters \((5\times 5 \; \text{and}\; 11 \times 11)\) in the earlier layers. AlexNet has considerable significance in the recent CNN generations, as well as beginning an innovative research era in CNN applications.
Network-in-network
This network model, which has some slight differences from the preceding models, introduced two innovative concepts [ 94 ]. The first was employing multiple layers of perception convolution. These convolutions are executed using a 1×1 filter, which supports the addition of extra nonlinearity in the networks. Moreover, this supports enlarging the network depth, which may later be regularized using dropout. For DL models, this idea is frequently employed in the bottleneck layer. As a substitution for a FC layer, the GAP is also employed, which represents the second novel concept and enables a significant reduction in the number of model parameters. In addition, GAP considerably updates the network architecture. Generating a final low-dimensional feature vector with no reduction in the feature maps dimension is possible when GAP is used on a large feature map [ 95 , 96 ]. Figure 16 shows the structure of the network.
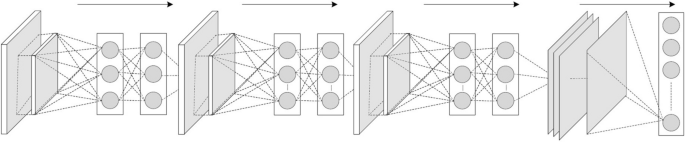
The architecture of network-in-network
Before 2013, the CNN learning mechanism was basically constructed on a trial-and-error basis, which precluded an understanding of the precise purpose following the enhancement. This issue restricted the deep CNN performance on convoluted images. In response, Zeiler and Fergus introduced DeconvNet (a multilayer de-convolutional neural network) in 2013 [ 97 ]. This method later became known as ZefNet, which was developed in order to quantitively visualize the network. Monitoring the CNN performance via understanding the neuron activation was the purpose of the network activity visualization. However, Erhan et al. utilized this exact concept to optimize deep belief network (DBN) performance by visualizing the features of the hidden layers [ 98 ]. Moreover, in addition to this issue, Le et al. assessed the deep unsupervised auto-encoder (AE) performance by visualizing the created classes of the image using the output neurons [ 99 ]. By reversing the operation order of the convolutional and pooling layers, DenconvNet operates like a forward-pass CNN. Reverse mapping of this kind launches the convolutional layer output backward to create visually observable image shapes that accordingly give the neural interpretation of the internal feature representation learned at each layer [ 100 ]. Monitoring the learning schematic through the training stage was the key concept underlying ZefNet. In addition, it utilized the outcomes to recognize an ability issue coupled with the model. This concept was experimentally proven on AlexNet by applying DeconvNet. This indicated that only certain neurons were working, while the others were out of action in the first two layers of the network. Furthermore, it indicated that the features extracted via the second layer contained aliasing objects. Thus, Zeiler and Fergus changed the CNN topology due to the existence of these outcomes. In addition, they executed parameter optimization, and also exploited the CNN learning by decreasing the stride and the filter sizes in order to retain all features of the initial two convolutional layers. An improvement in performance was accordingly achieved due to this rearrangement in CNN topology. This rearrangement proposed that the visualization of the features could be employed to identify design weaknesses and conduct appropriate parameter alteration. Figure 17 shows the structure of the network.
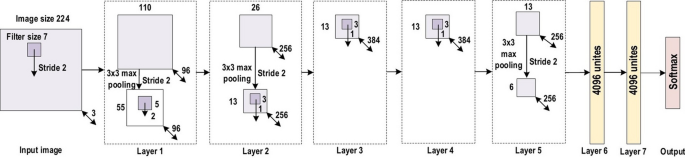
The architecture of ZefNet
Visual geometry group (VGG)
After CNN was determined to be effective in the field of image recognition, an easy and efficient design principle for CNN was proposed by Simonyan and Zisserman. This innovative design was called Visual Geometry Group (VGG). A multilayer model [ 101 ], it featured nineteen more layers than ZefNet [ 97 ] and AlexNet [ 30 ] to simulate the relations of the network representational capacity in depth. Conversely, in the 2013-ILSVRC competition, ZefNet was the frontier network, which proposed that filters with small sizes could enhance the CNN performance. With reference to these results, VGG inserted a layer of the heap of \(3\times 3\) filters rather than the \(5\times 5\) and 11 × 11 filters in ZefNet. This showed experimentally that the parallel assignment of these small-size filters could produce the same influence as the large-size filters. In other words, these small-size filters made the receptive field similarly efficient to the large-size filters \((7 \times 7 \; \text{and}\; 5 \times 5)\) . By decreasing the number of parameters, an extra advantage of reducing computational complication was achieved by using small-size filters. These outcomes established a novel research trend for working with small-size filters in CNN. In addition, by inserting \(1\times 1\) convolutions in the middle of the convolutional layers, VGG regulates the network complexity. It learns a linear grouping of the subsequent feature maps. With respect to network tuning, a max pooling layer [ 102 ] is inserted following the convolutional layer, while padding is implemented to maintain the spatial resolution. In general, VGG obtained significant results for localization problems and image classification. While it did not achieve first place in the 2014-ILSVRC competition, it acquired a reputation due to its enlarged depth, homogenous topology, and simplicity. However, VGG’s computational cost was excessive due to its utilization of around 140 million parameters, which represented its main shortcoming. Figure 18 shows the structure of the network.
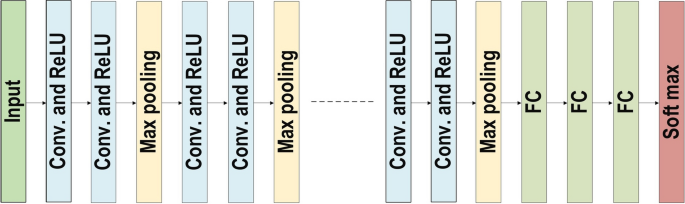
The architecture of VGG
In the 2014-ILSVRC competition, GoogleNet (also called Inception-V1) emerged as the winner [ 103 ]. Achieving high-level accuracy with decreased computational cost is the core aim of the GoogleNet architecture. It proposed a novel inception block (module) concept in the CNN context, since it combines multiple-scale convolutional transformations by employing merge, transform, and split functions for feature extraction. Figure 19 illustrates the inception block architecture. This architecture incorporates filters of different sizes ( \(5\times 5, 3\times 3, \; \text{and} \; 1\times 1\) ) to capture channel information together with spatial information at diverse ranges of spatial resolution. The common convolutional layer of GoogLeNet is substituted by small blocks using the same concept of network-in-network (NIN) architecture [ 94 ], which replaced each layer with a micro-neural network. The GoogLeNet concepts of merge, transform, and split were utilized, supported by attending to an issue correlated with different learning types of variants existing in a similar class of several images. The motivation of GoogLeNet was to improve the efficiency of CNN parameters, as well as to enhance the learning capacity. In addition, it regulates the computation by inserting a \(1\times 1\) convolutional filter, as a bottleneck layer, ahead of using large-size kernels. GoogleNet employed sparse connections to overcome the redundant information problem. It decreased cost by neglecting the irrelevant channels. It should be noted here that only some of the input channels are connected to some of the output channels. By employing a GAP layer as the end layer, rather than utilizing a FC layer, the density of connections was decreased. The number of parameters was also significantly decreased from 40 to 5 million parameters due to these parameter tunings. The additional regularity factors used included the employment of RmsProp as optimizer and batch normalization [ 104 ]. Furthermore, GoogleNet proposed the idea of auxiliary learners to speed up the rate of convergence. Conversely, the main shortcoming of GoogleNet was its heterogeneous topology; this shortcoming requires adaptation from one module to another. Other shortcomings of GoogleNet include the representation jam, which substantially decreased the feature space in the following layer, and in turn occasionally leads to valuable information loss.
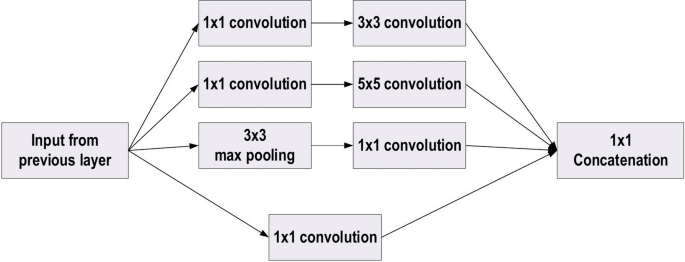
The basic structure of Google Block
Highway network
Increasing the network depth enhances its performance, mainly for complicated tasks. By contrast, the network training becomes difficult. The presence of several layers in deeper networks may result in small gradient values of the back-propagation of error at lower layers. In 2015, Srivastava et al. [ 105 ] suggested a novel CNN architecture, called Highway Network, to overcome this issue. This approach is based on the cross-connectivity concept. The unhindered information flow in Highway Network is empowered by instructing two gating units inside the layer. The gate mechanism concept was motivated by LSTM-based RNN [ 106 , 107 ]. The information aggregation was conducted by merging the information of the \(\i{\text{th}}-k\) layers with the next \(\i{\text{th}}\) layer to generate a regularization impact, which makes the gradient-based training of the deeper network very simple. This empowers the training of networks with more than 100 layers, such as a deeper network of 900 layers with the SGD algorithm. A Highway Network with a depth of fifty layers presented an improved rate of convergence, which is better than thin and deep architectures at the same time [ 108 ]. By contrast, [ 69 ] empirically demonstrated that plain Net performance declines when more than ten hidden layers are inserted. It should be noted that even a Highway Network 900 layers in depth converges much more rapidly than the plain network.
He et al. [ 37 ] developed ResNet (Residual Network), which was the winner of ILSVRC 2015. Their objective was to design an ultra-deep network free of the vanishing gradient issue, as compared to the previous networks. Several types of ResNet were developed based on the number of layers (starting with 34 layers and going up to 1202 layers). The most common type was ResNet50, which comprised 49 convolutional layers plus a single FC layer. The overall number of network weights was 25.5 M, while the overall number of MACs was 3.9 M. The novel idea of ResNet is its use of the bypass pathway concept, as shown in Fig. 20 , which was employed in Highway Nets to address the problem of training a deeper network in 2015. This is illustrated in Fig. 20 , which contains the fundamental ResNet block diagram. This is a conventional feedforward network plus a residual connection. The residual layer output can be identified as the \((l - 1){\text{th}}\) outputs, which are delivered from the preceding layer \((x_{l} - 1)\) . After executing different operations [such as convolution using variable-size filters, or batch normalization, before applying an activation function like ReLU on \((x_{l} - 1)\) ], the output is \(F(x_{l} - 1)\) . The ending residual output is \(x_{l}\) , which can be mathematically represented as in Eq. 18 .
There are numerous basic residual blocks included in the residual network. Based on the type of the residual network architecture, operations in the residual block are also changed [ 37 ].
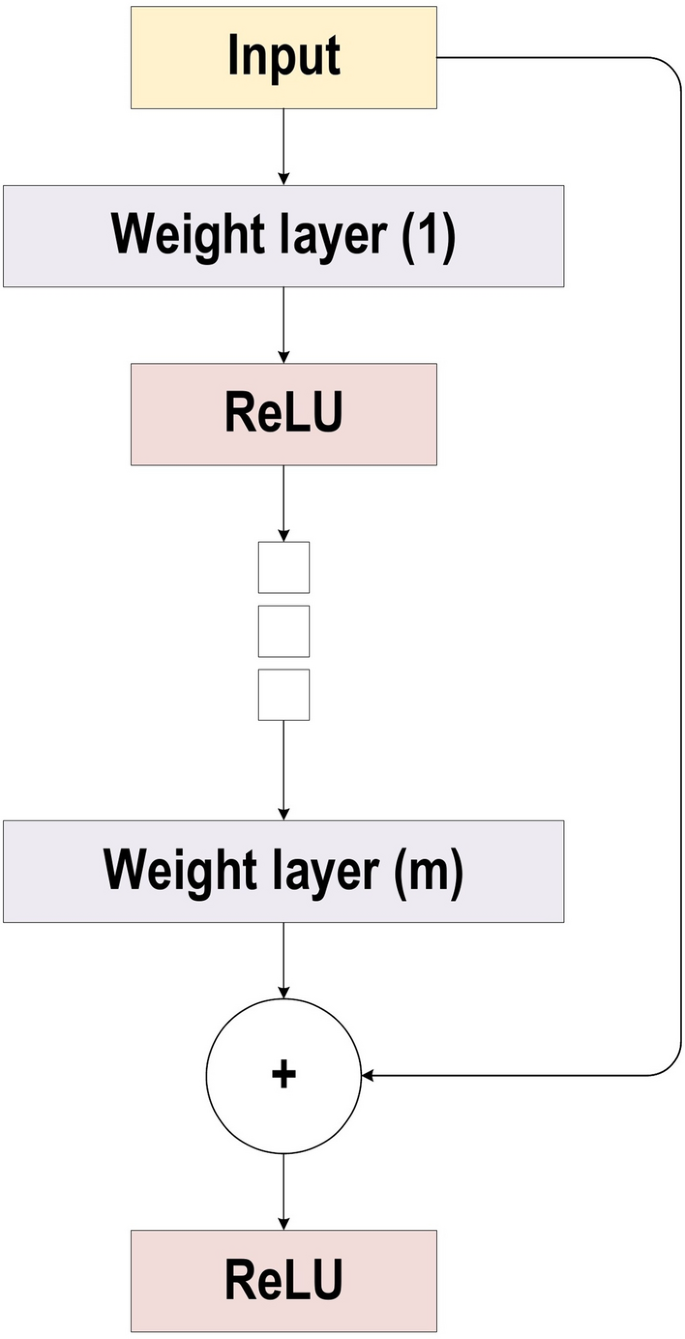
The block diagram for ResNet
In comparison to the highway network, ResNet presented shortcut connections inside layers to enable cross-layer connectivity, which are parameter-free and data-independent. Note that the layers characterize non-residual functions when a gated shortcut is closed in the highway network. By contrast, the individuality shortcuts are never closed, while the residual information is permanently passed in ResNet. Furthermore, ResNet has the potential to prevent the problems of gradient diminishing, as the shortcut connections (residual links) accelerate the deep network convergence. ResNet was the winner of the 2015-ILSVRC championship with 152 layers of depth; this represents 8 times the depth of VGG and 20 times the depth of AlexNet. In comparison with VGG, it has lower computational complexity, even with enlarged depth.
Inception: ResNet and Inception-V3/4
Szegedy et al. [ 103 , 109 , 110 ] proposed Inception-ResNet and Inception-V3/4 as upgraded types of Inception-V1/2. The concept behind Inception-V3 was to minimize the computational cost with no effect on the deeper network generalization. Thus, Szegedy et al. used asymmetric small-size filters ( \(1\times 5\) and \(1\times 7\) ) rather than large-size filters ( \( 7\times 7\) and \(5\times 5\) ); moreover, they utilized a bottleneck of \(1\times 1\) convolution prior to the large-size filters [ 110 ]. These changes make the operation of the traditional convolution very similar to cross-channel correlation. Previously, Lin et al. utilized the 1 × 1 filter potential in NIN architecture [ 94 ]. Subsequently, [ 110 ] utilized the same idea in an intelligent manner. By using \(1\times 1\) convolutional operation in Inception-V3, the input data are mapped into three or four isolated spaces, which are smaller than the initial input spaces. Next, all of these correlations are mapped in these smaller spaces through common \(5\times 5\) or \(3\times 3\) convolutions. By contrast, in Inception-ResNet, Szegedy et al. bring together the inception block and the residual learning power by replacing the filter concatenation with the residual connection [ 111 ]. Szegedy et al. empirically demonstrated that Inception-ResNet (Inception-4 with residual connections) can achieve a similar generalization power to Inception-V4 with enlarged width and depth and without residual connections. Thus, it is clearly illustrated that using residual connections in training will significantly accelerate the Inception network training. Figure 21 shows The basic block diagram for Inception Residual unit.
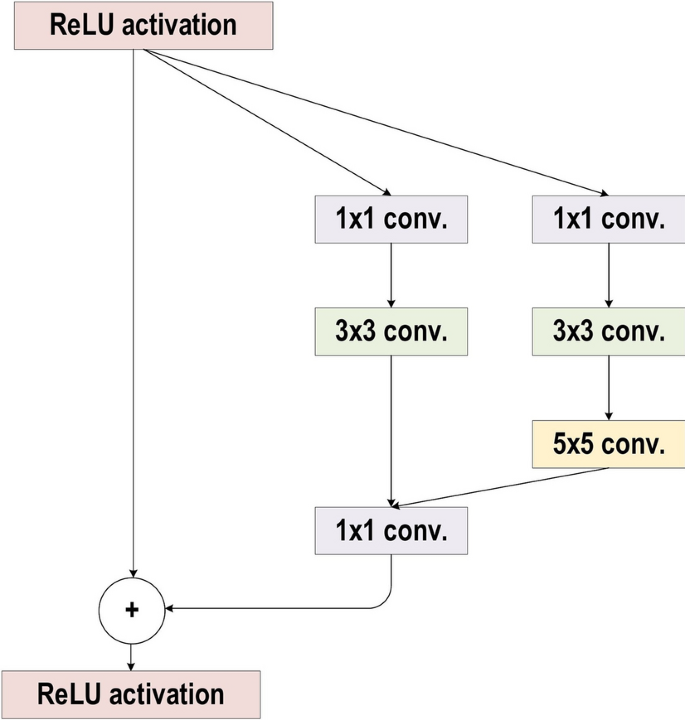
The basic block diagram for Inception Residual unit
To solve the problem of the vanishing gradient, DenseNet was presented, following the same direction as ResNet and the Highway network [ 105 , 111 , 112 ]. One of the drawbacks of ResNet is that it clearly conserves information by means of preservative individuality transformations, as several layers contribute extremely little or no information. In addition, ResNet has a large number of weights, since each layer has an isolated group of weights. DenseNet employed cross-layer connectivity in an improved approach to address this problem [ 112 , 113 , 114 ]. It connected each layer to all layers in the network using a feed-forward approach. Therefore, the feature maps of each previous layer were employed to input into all of the following layers. In traditional CNNs, there are l connections between the previous layer and the current layer, while in DenseNet, there are \(\frac{l(l+1)}{2}\) direct connections. DenseNet demonstrates the influence of cross-layer depth wise-convolutions. Thus, the network gains the ability to discriminate clearly between the added and the preserved information, since DenseNet concatenates the features of the preceding layers rather than adding them. However, due to its narrow layer structure, DenseNet becomes parametrically high-priced in addition to the increased number of feature maps. The direct admission of all layers to the gradients via the loss function enhances the information flow all across the network. In addition, this includes a regularizing impact, which minimizes overfitting on tasks alongside minor training sets. Figure 22 shows the architecture of DenseNet Network.
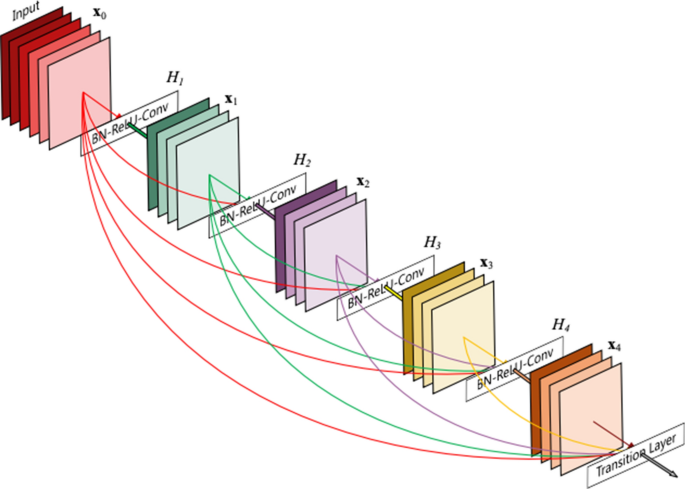
(adopted from [ 112 ])
The architecture of DenseNet Network
ResNext is an enhanced version of the Inception Network [ 115 ]. It is also known as the Aggregated Residual Transform Network. Cardinality, which is a new term presented by [ 115 ], utilized the split, transform, and merge topology in an easy and effective way. It denotes the size of the transformation set as an extra dimension [ 116 , 117 , 118 ]. However, the Inception network manages network resources more efficiently, as well as enhancing the learning ability of the conventional CNN. In the transformation branch, different spatial embeddings (employing e.g. \(5\times 5\) , \(3\times 3\) , and \(1\times 1\) ) are used. Thus, customizing each layer is required separately. By contrast, ResNext derives its characteristic features from ResNet, VGG, and Inception. It employed the VGG deep homogenous topology with the basic architecture of GoogleNet by setting \(3\times 3\) filters as spatial resolution inside the blocks of split, transform, and merge. Figure 23 shows the ResNext building blocks. ResNext utilized multi-transformations inside the blocks of split, transform, and merge, as well as outlining such transformations in cardinality terms. The performance is significantly improved by increasing the cardinality, as Xie et al. showed. The complexity of ResNext was regulated by employing \(1\times 1\) filters (low embeddings) ahead of a \(3\times 3\) convolution. By contrast, skipping connections are used for optimized training [ 115 ].
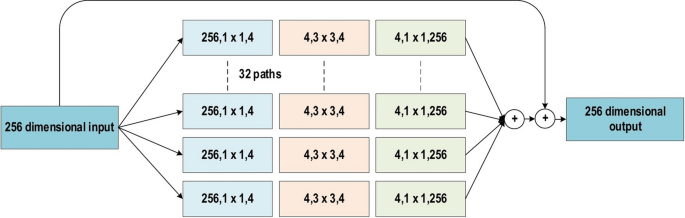
The basic block diagram for the ResNext building blocks
The feature reuse problem is the core shortcoming related to deep residual networks, since certain feature blocks or transformations contribute a very small amount to learning. Zagoruyko and Komodakis [ 119 ] accordingly proposed WideResNet to address this problem. These authors advised that the depth has a supplemental influence, while the residual units convey the core learning ability of deep residual networks. WideResNet utilized the residual block power via making the ResNet wider instead of deeper [ 37 ]. It enlarged the width by presenting an extra factor, k, which handles the network width. In other words, it indicated that layer widening is a highly successful method of performance enhancement compared to deepening the residual network. While enhanced representational capacity is achieved by deep residual networks, these networks also have certain drawbacks, such as the exploding and vanishing gradient problems, feature reuse problem (inactivation of several feature maps), and the time-intensive nature of the training. He et al. [ 37 ] tackled the feature reuse problem by including a dropout in each residual block to regularize the network in an efficient manner. In a similar manner, utilizing dropouts, Huang et al. [ 120 ] presented the stochastic depth concept to solve the slow learning and gradient vanishing problems. Earlier research was focused on increasing the depth; thus, any small enhancement in performance required the addition of several new layers. When comparing the number of parameters, WideResNet has twice that of ResNet, as an experimental study showed. By contrast, WideResNet presents an improved method for training relative to deep networks [ 119 ]. Note that most architectures prior to residual networks (including the highly effective VGG and Inception) were wider than ResNet. Thus, wider residual networks were established once this was determined. However, inserting a dropout between the convolutional layers (as opposed to within the residual block) made the learning more effective in WideResNet [ 121 , 122 ].
Pyramidal Net
The depth of the feature map increases in the succeeding layer due to the deep stacking of multi-convolutional layers, as shown in previous deep CNN architectures such as ResNet, VGG, and AlexNet. By contrast, the spatial dimension reduces, since a sub-sampling follows each convolutional layer. Thus, augmented feature representation is recompensed by decreasing the size of the feature map. The extreme expansion in the depth of the feature map, alongside the spatial information loss, interferes with the learning ability in the deep CNNs. ResNet obtained notable outcomes for the issue of image classification. Conversely, deleting a convolutional block—in which both the number of channel and spatial dimensions vary (channel depth enlarges, while spatial dimension reduces)—commonly results in decreased classifier performance. Accordingly, the stochastic ResNet enhanced the performance by decreasing the information loss accompanying the residual unit drop. Han et al. [ 123 ] proposed Pyramidal Net to address the ResNet learning interference problem. To address the depth enlargement and extreme reduction in spatial width via ResNet, Pyramidal Net slowly enlarges the residual unit width to cover the most feasible places rather than saving the same spatial dimension inside all residual blocks up to the appearance of the down-sampling. It was referred to as Pyramidal Net due to the slow enlargement in the feature map depth based on the up-down method. Factor l, which was determined by Eq. 19 , regulates the depth of the feature map.
Here, the dimension of the l th residual unit is indicated by \(d_{l}\) ; moreover, n indicates the overall number of residual units, the step factor is indicated by \(\lambda \) , and the depth increase is regulated by the factor \(\frac{\lambda }{n}\) , which uniformly distributes the weight increase across the dimension of the feature map. Zero-padded identity mapping is used to insert the residual connections among the layers. In comparison to the projection-based shortcut connections, zero-padded identity mapping requires fewer parameters, which in turn leads to enhanced generalization [ 124 ]. Multiplication- and addition-based widening are two different approaches used in Pyramidal Nets for network widening. More specifically, the first approach (multiplication) enlarges geometrically, while the second one (addition) enlarges linearly [ 92 ]. The main problem associated with the width enlargement is the growth in time and space required related to the quadratic time.
Extreme inception architecture is the main characteristic of Xception. The main idea behind Xception is its depthwise separable convolution [ 125 ]. The Xception model adjusted the original inception block by making it wider and exchanging a single dimension ( \(3 \times 3\) ) followed by a \(1 \times 1\) convolution to reduce computational complexity. Figure 24 shows the Xception block architecture. The Xception network becomes extra computationally effective through the use of the decoupling channel and spatial correspondence. Moreover, it first performs mapping of the convolved output to the embedding short dimension by applying \(1 \times 1\) convolutions. It then performs k spatial transformations. Note that k here represents the width-defining cardinality, which is obtained via the transformations number in Xception. However, the computations were made simpler in Xception by distinctly convolving each channel around the spatial axes. These axes are subsequently used as the \(1 \times 1\) convolutions (pointwise convolution) for performing cross-channel correspondence. The \(1 \times 1\) convolution is utilized in Xception to regularize the depth of the channel. The traditional convolutional operation in Xception utilizes a number of transformation segments equivalent to the number of channels; Inception, moreover, utilizes three transformation segments, while traditional CNN architecture utilizes only a single transformation segment. Conversely, the suggested Xception transformation approach achieves extra learning efficiency and better performance but does not minimize the number of parameters [ 126 , 127 ].
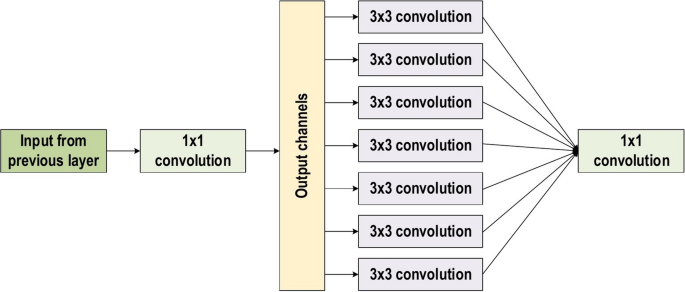
The basic block diagram for the Xception block architecture
Residual attention neural network
To improve the network feature representation, Wang et al. [ 128 ] proposed the Residual Attention Network (RAN). Enabling the network to learn aware features of the object is the main purpose of incorporating attention into the CNN. The RAN consists of stacked residual blocks in addition to the attention module; hence, it is a feed-forward CNN. However, the attention module is divided into two branches, namely the mask branch and trunk branch. These branches adopt a top-down and bottom-up learning strategy respectively. Encapsulating two different strategies in the attention model supports top-down attention feedback and fast feed-forward processing in only one particular feed-forward process. More specifically, the top-down architecture generates dense features to make inferences about every aspect. Moreover, the bottom-up feedforward architecture generates low-resolution feature maps in addition to robust semantic information. Restricted Boltzmann machines employed a top-down bottom-up strategy as in previously proposed studies [ 129 ]. During the training reconstruction phase, Goh et al. [ 130 ] used the mechanism of top-down attention in deep Boltzmann machines (DBMs) as a regularizing factor. Note that the network can be globally optimized using a top-down learning strategy in a similar manner, where the maps progressively output to the input throughout the learning process [ 129 , 130 , 131 , 132 ].
Incorporating the attention concept with convolutional blocks in an easy way was used by the transformation network, as obtained in a previous study [ 133 ]. Unfortunately, these are inflexible, which represents the main problem, along with their inability to be used for varying surroundings. By contrast, stacking multi-attention modules has made RAN very effective at recognizing noisy, complex, and cluttered images. RAN’s hierarchical organization gives it the capability to adaptively allocate a weight for every feature map depending on its importance within the layers. Furthermore, incorporating three distinct levels of attention (spatial, channel, and mixed) enables the model to use this ability to capture the object-aware features at these distinct levels.
Convolutional block attention module
The importance of the feature map utilization and the attention mechanism is certified via SE-Network and RAN [ 128 , 134 , 135 ]. The convolutional block attention (CBAM) module, which is a novel attention-based CNN, was first developed by Woo et al. [ 136 ]. This module is similar to SE-Network and simple in design. SE-Network disregards the object’s spatial locality in the image and considers only the channels’ contribution during the image classification. Regarding object detection, object spatial location plays a significant role. The convolutional block attention module sequentially infers the attention maps. More specifically, it applies channel attention preceding the spatial attention to obtain the refined feature maps. Spatial attention is performed using 1 × 1 convolution and pooling functions, as in the literature. Generating an effective feature descriptor can be achieved by using a spatial axis along with the pooling of features. In addition, generating a robust spatial attention map is possible, as CBAM concatenates the max pooling and average pooling operations. In a similar manner, a collection of GAP and max pooling operations is used to model the feature map statistics. Woo et al. [ 136 ] demonstrated that utilizing GAP will return a sub-optimized inference of channel attention, whereas max pooling provides an indication of the distinguishing object features. Thus, the utilization of max pooling and average pooling enhances the network’s representational power. The feature maps improve the representational power, as well as facilitating a focus on the significant portion of the chosen features. The expression of 3D attention maps through a serial learning procedure assists in decreasing the computational cost and the number of parameters, as Woo et al. [ 136 ] experimentally proved. Note that any CNN architecture can be simply integrated with CBAM.
Concurrent spatial and channel excitation mechanism
To make the work valid for segmentation tasks, Roy et al. [ 137 , 138 ] expanded Hu et al. [ 134 ] effort by adding the influence of spatial information to the channel information. Roy et al. [ 137 , 138 ] presented three types of modules: (1) channel squeeze and excitation with concurrent channels (scSE); (2) exciting spatially and squeezing channel-wise (sSE); (3) exciting channel-wise and squeezing spatially (cSE). For segmentation purposes, they employed auto-encoder-based CNNs. In addition, they suggested inserting modules following the encoder and decoder layers. To specifically highlight the object-specific feature maps, they further allocated attention to every channel by expressing a scaling factor from the channel and spatial information in the first module (scSE). In the second module (sSE), the feature map information has lower importance than the spatial locality, as the spatial information plays a significant role during the segmentation process. Therefore, several channel collections are spatially divided and developed so that they can be employed in segmentation. In the final module (cSE), a similar SE-block concept is used. Furthermore, the scaling factor is derived founded on the contribution of the feature maps within the object detection [ 137 , 138 ].
CNN is an efficient technique for detecting object features and achieving well-behaved recognition performance in comparison with innovative handcrafted feature detectors. A number of restrictions related to CNN are present, meaning that the CNN does not consider certain relations, orientation, size, and perspectives of features. For instance, when considering a face image, the CNN does not count the various face components (such as mouth, eyes, nose, etc.) positions, and will incorrectly activate the CNN neurons and recognize the face without taking specific relations (such as size, orientation etc.) into account. At this point, consider a neuron that has probability in addition to feature properties such as size, orientation, perspective, etc. A specific neuron/capsule of this type has the ability to effectively detect the face along with different types of information. Thus, many layers of capsule nodes are used to construct the capsule network. An encoding unit, which contains three layers of capsule nodes, forms the CapsuleNet or CapsNet (the initial version of the capsule networks).
For example, the MNIST architecture comprises \(28\times 28\) images, applying 256 filters of size \(9\times 9\) and with stride 1. The \(28-9+1=20\) is the output plus 256 feature maps. Next, these outputs are input to the first capsule layer, while producing an 8D vector rather than a scalar; in fact, this is a modified convolution layer. Note that a stride 2 with \(9\times 9\) filters is employed in the first convolution layer. Thus, the dimension of the output is \((20-9)/2+1=6\) . The initial capsules employ \(8\times 32\) filters, which generate 32 × 8 × 6 × 6 (32 for groups, 8 for neurons, while 6 × 6 is the neuron size).
Figure 25 represents the complete CapsNet encoding and decoding processes. In the CNN context, a max-pooling layer is frequently employed to handle the translation change. It can detect the feature moves in the event that the feature is still within the max-pooling window. This approach has the ability to detect the overlapped features; this is highly significant in detection and segmentation operations, since the capsule involves the weighted features sum from the preceding layer.
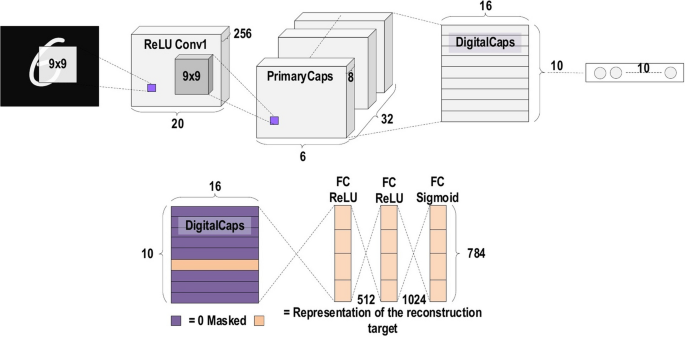
The complete CapsNet encoding and decoding processes
In conventional CNNs, a particular cost function is employed to evaluate the global error that grows toward the back throughout the training process. Conversely, in such cases, the activation of a neuron will not grow further once the weight between two neurons turns out to be zero. Instead of a single size being provided with the complete cost function in repetitive dynamic routing alongside the agreement, the signal is directed based on the feature parameters. Sabour et al. [ 139 ] provides more details about this architecture. When using MNIST to recognize handwritten digits, this innovative CNN architecture gives superior accuracy. From the application perspective, this architecture has extra suitability for segmentation and detection approaches when compared with classification approaches [ 140 , 141 , 142 ].
High-resolution network (HRNet)
High-resolution representations are necessary for position-sensitive vision tasks, such as semantic segmentation, object detection, and human pose estimation. In the present up-to-date frameworks, the input image is encoded as a low-resolution representation using a subnetwork that is constructed as a connected series of high-to-low resolution convolutions such as VGGNet and ResNet. The low-resolution representation is then recovered to become a high-resolution one. Alternatively, high-resolution representations are maintained during the entire process using a novel network, referred to as a High-Resolution Network (HRNet) [ 143 , 144 ]. This network has two principal features. First, the convolution series of high-to-low resolutions are connected in parallel. Second, the information across the resolutions are repeatedly exchanged. The advantage achieved includes getting a representation that is more accurate in the spatial domain and extra-rich in the semantic domain. Moreover, HRNet has several applications in the fields of object detection, semantic segmentation, and human pose prediction. For computer vision problems, the HRNet represents a more robust backbone. Figure 26 illustrates the general architecture of HRNet.
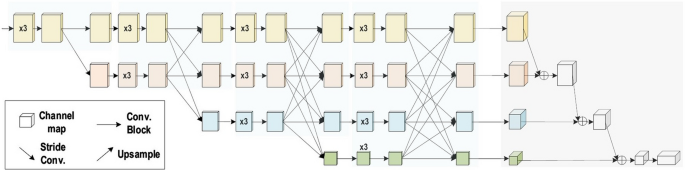
The general architecture of HRNet
Challenges (limitations) of deep learning and alternate solutions
When employing DL, several difficulties are often taken into consideration. Those more challenging are listed next and several possible alternatives are accordingly provided.
Training data
DL is extremely data-hungry considering it also involves representation learning [ 145 , 146 ]. DL demands an extensively large amount of data to achieve a well-behaved performance model, i.e. as the data increases, an extra well-behaved performance model can be achieved (Fig. 27 ). In most cases, the available data are sufficient to obtain a good performance model. However, sometimes there is a shortage of data for using DL directly [ 87 ]. To properly address this issue, three suggested methods are available. The first involves the employment of the transfer-learning concept after data is collected from similar tasks. Note that while the transferred data will not directly augment the actual data, it will help in terms of both enhancing the original input representation of data and its mapping function [ 147 ]. In this way, the model performance is boosted. Another technique involves employing a well-trained model from a similar task and fine-tuning the ending of two layers or even one layer based on the limited original data. Refer to [ 148 , 149 ] for a review of different transfer-learning techniques applied in the DL approach. In the second method, data augmentation is performed [ 150 ]. This task is very helpful for use in augmenting the image data, since the image translation, mirroring, and rotation commonly do not change the image label. Conversely, it is important to take care when applying this technique in some cases such as with bioinformatics data. For instance, when mirroring an enzyme sequence, the output data may not represent the actual enzyme sequence. In the third method, the simulated data can be considered for increasing the volume of the training set. It is occasionally possible to create simulators based on the physical process if the issue is well understood. Therefore, the result will involve the simulation of as much data as needed. Processing the data requirement for DL-based simulation is obtained as an example in Ref. [ 151 ].
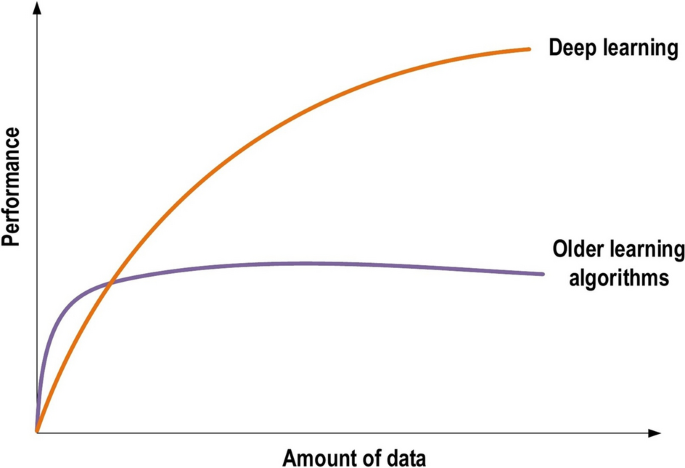
The performance of DL regarding the amount of data
- Transfer learning
Recent research has revealed a widespread use of deep CNNs, which offer ground-breaking support for answering many classification problems. Generally speaking, deep CNN models require a sizable volume of data to obtain good performance. The common challenge associated with using such models concerns the lack of training data. Indeed, gathering a large volume of data is an exhausting job, and no successful solution is available at this time. The undersized dataset problem is therefore currently solved using the TL technique [ 148 , 149 ], which is highly efficient in addressing the lack of training data issue. The mechanism of TL involves training the CNN model with large volumes of data. In the next step, the model is fine-tuned for training on a small request dataset.
The student-teacher relationship is a suitable approach to clarifying TL. Gathering detailed knowledge of the subject is the first step [ 152 ]. Next, the teacher provides a “course” by conveying the information within a “lecture series” over time. Put simply, the teacher transfers the information to the student. In more detail, the expert (teacher) transfers the knowledge (information) to the learner (student). Similarly, the DL network is trained using a vast volume of data, and also learns the bias and the weights during the training process. These weights are then transferred to different networks for retraining or testing a similar novel model. Thus, the novel model is enabled to pre-train weights rather than requiring training from scratch. Figure 28 illustrates the conceptual diagram of the TL technique.
Pre-trained models: Many CNN models, e.g. AlexNet [ 30 ], GoogleNet [ 103 ], and ResNet [ 37 ], have been trained on large datasets such as ImageNet for image recognition purposes. These models can then be employed to recognize a different task without the need to train from scratch. Furthermore, the weights remain the same apart from a few learned features. In cases where data samples are lacking, these models are very useful. There are many reasons for employing a pre-trained model. First, training large models on sizeable datasets requires high-priced computational power. Second, training large models can be time-consuming, taking up to multiple weeks. Finally, a pre-trained model can assist with network generalization and speed up the convergence.
A research problem using pre-trained models: Training a DL approach requires a massive number of images. Thus, obtaining good performance is a challenge under these circumstances. Achieving excellent outcomes in image classification or recognition applications, with performance occasionally superior to that of a human, becomes possible through the use of deep convolutional neural networks (DCNNs) including several layers if a huge amount of data is available [ 37 , 148 , 153 ]. However, avoiding overfitting problems in such applications requires sizable datasets and properly generalizing DCNN models. When training a DCNN model, the dataset size has no lower limit. However, the accuracy of the model becomes insufficient in the case of the utilized model has fewer layers, or if a small dataset is used for training due to over- or under-fitting problems. Due to they have no ability to utilize the hierarchical features of sizable datasets, models with fewer layers have poor accuracy. It is difficult to acquire sufficient training data for DL models. For example, in medical imaging and environmental science, gathering labelled datasets is very costly [ 148 ]. Moreover, the majority of the crowdsourcing workers are unable to make accurate notes on medical or biological images due to their lack of medical or biological knowledge. Thus, ML researchers often rely on field experts to label such images; however, this process is costly and time consuming. Therefore, producing the large volume of labels required to develop flourishing deep networks turns out to be unfeasible. Recently, TL has been widely employed to address the later issue. Nevertheless, although TL enhances the accuracy of several tasks in the fields of pattern recognition and computer vision [ 154 , 155 ], there is an essential issue related to the source data type used by the TL as compared to the target dataset. For instance, enhancing the medical image classification performance of CNN models is achieved by training the models using the ImageNet dataset, which contains natural images [ 153 ]. However, such natural images are completely dissimilar from the raw medical images, meaning that the model performance is not enhanced. It has further been proven that TL from different domains does not significantly affect performance on medical imaging tasks, as lightweight models trained from scratch perform nearly as well as standard ImageNet-transferred models [ 156 ]. Therefore, there exists scenarios in which using pre-trained models do not become an affordable solution. In 2020, some researchers have utilized same-domain TL and achieved excellent results [ 86 , 87 , 88 , 157 ]. Same-domain TL is an approach of using images that look similar to the target dataset for training. For example, using X-ray images of different chest diseases to train the model, then fine-tuning and training it on chest X-ray images for COVID-19 diagnosis. More details about same-domain TL and how to implement the fine-tuning process can be found in [ 87 ].
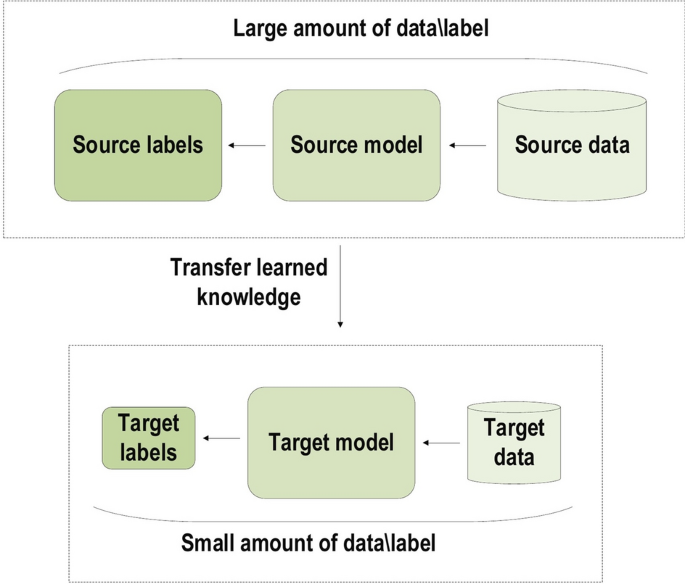
The conceptual diagram of the TL technique
Data augmentation techniques
If the goal is to increase the amount of available data and avoid the overfitting issue, data augmentation techniques are one possible solution [ 150 , 158 , 159 ]. These techniques are data-space solutions for any limited-data problem. Data augmentation incorporates a collection of methods that improve the attributes and size of training datasets. Thus, DL networks can perform better when these techniques are employed. Next, we list some data augmentation alternate solutions.
Flipping: Flipping the vertical axis is a less common practice than flipping the horizontal one. Flipping has been verified as valuable on datasets like ImageNet and CIFAR-10. Moreover, it is highly simple to implement. In addition, it is not a label-conserving transformation on datasets that involve text recognition (such as SVHN and MNIST).
Color space: Encoding digital image data is commonly used as a dimension tensor ( \(height \times width \times color channels\) ). Accomplishing augmentations in the color space of the channels is an alternative technique, which is extremely workable for implementation. A very easy color augmentation involves separating a channel of a particular color, such as Red, Green, or Blue. A simple way to rapidly convert an image using a single-color channel is achieved by separating that matrix and inserting additional double zeros from the remaining two color channels. Furthermore, increasing or decreasing the image brightness is achieved by using straightforward matrix operations to easily manipulate the RGB values. By deriving a color histogram that describes the image, additional improved color augmentations can be obtained. Lighting alterations are also made possible by adjusting the intensity values in histograms similar to those employed in photo-editing applications.
Cropping: Cropping a dominant patch of every single image is a technique employed with combined dimensions of height and width as a specific processing step for image data. Furthermore, random cropping may be employed to produce an impact similar to translations. The difference between translations and random cropping is that translations conserve the spatial dimensions of this image, while random cropping reduces the input size [for example from (256, 256) to (224, 224)]. According to the selected reduction threshold for cropping, the label-preserving transformation may not be addressed.
Rotation: When rotating an image left or right from within 0 to 360 degrees around the axis, rotation augmentations are obtained. The rotation degree parameter greatly determines the suitability of the rotation augmentations. In digit recognition tasks, small rotations (from 0 to 20 degrees) are very helpful. By contrast, the data label cannot be preserved post-transformation when the rotation degree increases.
Translation: To avoid positional bias within the image data, a very useful transformation is to shift the image up, down, left, or right. For instance, it is common that the whole dataset images are centered; moreover, the tested dataset should be entirely made up of centered images to test the model. Note that when translating the initial images in a particular direction, the residual space should be filled with Gaussian or random noise, or a constant value such as 255 s or 0 s. The spatial dimensions of the image post-augmentation are preserved using this padding.
Noise injection This approach involves injecting a matrix of arbitrary values. Such a matrix is commonly obtained from a Gaussian distribution. Moreno-Barea et al. [ 160 ] employed nine datasets to test the noise injection. These datasets were taken from the UCI repository [ 161 ]. Injecting noise within images enables the CNN to learn additional robust features.
However, highly well-behaved solutions for positional biases available within the training data are achieved by means of geometric transformations. To separate the distribution of the testing data from the training data, several prospective sources of bias exist. For instance, when all faces should be completely centered within the frames (as in facial recognition datasets), the problem of positional biases emerges. Thus, geometric translations are the best solution. Geometric translations are helpful due to their simplicity of implementation, as well as their effective capability to disable the positional biases. Several libraries of image processing are available, which enables beginning with simple operations such as rotation or horizontal flipping. Additional training time, higher computational costs, and additional memory are some shortcomings of geometric transformations. Furthermore, a number of geometric transformations (such as arbitrary cropping or translation) should be manually observed to ensure that they do not change the image label. Finally, the biases that separate the test data from the training data are more complicated than transitional and positional changes. Hence, it is not trivial answering to when and where geometric transformations are suitable to be applied.
Imbalanced data
Commonly, biological data tend to be imbalanced, as negative samples are much more numerous than positive ones [ 162 , 163 , 164 ]. For example, compared to COVID-19-positive X-ray images, the volume of normal X-ray images is very large. It should be noted that undesirable results may be produced when training a DL model using imbalanced data. The following techniques are used to solve this issue. First, it is necessary to employ the correct criteria for evaluating the loss, as well as the prediction result. In considering the imbalanced data, the model should perform well on small classes as well as larger ones. Thus, the model should employ area under curve (AUC) as the resultant loss as well as the criteria [ 165 ]. Second, it should employ the weighted cross-entropy loss, which ensures the model will perform well with small classes if it still prefers to employ the cross-entropy loss. Simultaneously, during model training, it is possible either to down-sample the large classes or up-sample the small classes. Finally, to make the data balanced as in Ref. [ 166 ], it is possible to construct models for every hierarchical level, as a biological system frequently has hierarchical label space. However, the effect of the imbalanced data on the performance of the DL model has been comprehensively investigated. In addition, to lessen the problem, the most frequently used techniques were also compared. Nevertheless, note that these techniques are not specified for biological problems.
Interpretability of data
Occasionally, DL techniques are analyzed to act as a black box. In fact, they are interpretable. The need for a method of interpreting DL, which is used to obtain the valuable motifs and patterns recognized by the network, is common in many fields, such as bioinformatics [ 167 ]. In the task of disease diagnosis, it is not only required to know the disease diagnosis or prediction results of a trained DL model, but also how to enhance the surety of the prediction outcomes, as the model makes its decisions based on these verifications [ 168 ]. To achieve this, it is possible to give a score of importance for every portion of the particular example. Within this solution, back-propagation-based techniques or perturbation-based approaches are used [ 169 ]. In the perturbation-based approaches, a portion of the input is changed and the effect of this change on the model output is observed [ 170 , 171 , 172 , 173 ]. This concept has high computational complexity, but it is simple to understand. On the other hand, to check the score of the importance of various input portions, the signal from the output propagates back to the input layer in the back-propagation-based techniques. These techniques have been proven valuable in [ 174 ]. In different scenarios, various meanings can represent the model interpretability.
Uncertainty scaling
Commonly, the final prediction label is not the only label required when employing DL techniques to achieve the prediction; the score of confidence for every inquiry from the model is also desired. The score of confidence is defined as how confident the model is in its prediction [ 175 ]. Since the score of confidence prevents belief in unreliable and misleading predictions, it is a significant attribute, regardless of the application scenario. In biology, the confidence score reduces the resources and time expended in proving the outcomes of the misleading prediction. Generally speaking, in healthcare or similar applications, the uncertainty scaling is frequently very significant; it helps in evaluating automated clinical decisions and the reliability of machine learning-based disease-diagnosis [ 176 , 177 ]. Because overconfident prediction can be the output of different DL models, the score of probability (achieved from the softmax output of the direct-DL) is often not in the correct scale [ 178 ]. Note that the softmax output requires post-scaling to achieve a reliable probability score. For outputting the probability score in the correct scale, several techniques have been introduced, including Bayesian Binning into Quantiles (BBQ) [ 179 ], isotonic regression [ 180 ], histogram binning [ 181 ], and the legendary Platt scaling [ 182 ]. More specifically, for DL techniques, temperature scaling was recently introduced, which achieves superior performance compared to the other techniques.
Catastrophic forgetting
This is defined as incorporating new information into a plain DL model, made possible by interfering with the learned information. For instance, consider a case where there are 1000 types of flowers and a model is trained to classify these flowers, after which a new type of flower is introduced; if the model is fine-tuned only with this new class, its performance will become unsuccessful with the older classes [ 183 , 184 ]. The logical data are continually collected and renewed, which is in fact a highly typical scenario in many fields, e.g. Biology. To address this issue, there is a direct solution that involves employing old and new data to train an entirely new model from scratch. This solution is time-consuming and computationally intensive; furthermore, it leads to an unstable state for the learned representation of the initial data. At this time, three different types of ML techniques, which have not catastrophic forgetting, are made available to solve the human brain problem founded on the neurophysiological theories [ 185 , 186 ]. Techniques of the first type are founded on regularizations such as EWC [ 183 ] Techniques of the second type employ rehearsal training techniques and dynamic neural network architecture like iCaRL [ 187 , 188 ]. Finally, techniques of the third type are founded on dual-memory learning systems [ 189 ]. Refer to [ 190 , 191 , 192 ] in order to gain more details.
Model compression
To obtain well-trained models that can still be employed productively, DL models have intensive memory and computational requirements due to their huge complexity and large numbers of parameters [ 193 , 194 ]. One of the fields that is characterized as data-intensive is the field of healthcare and environmental science. These needs reduce the deployment of DL in limited computational-power machines, mainly in the healthcare field. The numerous methods of assessing human health and the data heterogeneity have become far more complicated and vastly larger in size [ 195 ]; thus, the issue requires additional computation [ 196 ]. Furthermore, novel hardware-based parallel processing solutions such as FPGAs and GPUs [ 197 , 198 , 199 ] have been developed to solve the computation issues associated with DL. Recently, numerous techniques for compressing the DL models, designed to decrease the computational issues of the models from the starting point, have also been introduced. These techniques can be classified into four classes. In the first class, the redundant parameters (which have no significant impact on model performance) are reduced. This class, which includes the famous deep compression method, is called parameter pruning [ 200 ]. In the second class, the larger model uses its distilled knowledge to train a more compact model; thus, it is called knowledge distillation [ 201 , 202 ]. In the third class, compact convolution filters are used to reduce the number of parameters [ 203 ]. In the final class, the information parameters are estimated for preservation using low-rank factorization [ 204 ]. For model compression, these classes represent the most representative techniques. In [ 193 ], it has been provided a more comprehensive discussion about the topic.
Overfitting
DL models have excessively high possibilities of resulting in data overfitting at the training stage due to the vast number of parameters involved, which are correlated in a complex manner. Such situations reduce the model’s ability to achieve good performance on the tested data [ 90 , 205 ]. This problem is not only limited to a specific field, but involves different tasks. Therefore, when proposing DL techniques, this problem should be fully considered and accurately handled. In DL, the implied bias of the training process enables the model to overcome crucial overfitting problems, as recent studies suggest [ 205 , 206 , 207 , 208 ]. Even so, it is still necessary to develop techniques that handle the overfitting problem. An investigation of the available DL algorithms that ease the overfitting problem can categorize them into three classes. The first class acts on both the model architecture and model parameters and includes the most familiar approaches, such as weight decay [ 209 ], batch normalization [ 210 ], and dropout [ 90 ]. In DL, the default technique is weight decay [ 209 ], which is used extensively in almost all ML algorithms as a universal regularizer. The second class works on model inputs such as data corruption and data augmentation [ 150 , 211 ]. One reason for the overfitting problem is the lack of training data, which makes the learned distribution not mirror the real distribution. Data augmentation enlarges the training data. By contrast, marginalized data corruption improves the solution exclusive to augmenting the data. The final class works on the model output. A recently proposed technique penalizes the over-confident outputs for regularizing the model [ 178 ]. This technique has demonstrated the ability to regularize RNNs and CNNs.
Vanishing gradient problem
In general, when using backpropagation and gradient-based learning techniques along with ANNs, largely in the training stage, a problem called the vanishing gradient problem arises [ 212 , 213 , 214 ]. More specifically, in each training iteration, every weight of the neural network is updated based on the current weight and is proportionally relative to the partial derivative of the error function. However, this weight updating may not occur in some cases due to a vanishingly small gradient, which in the worst case means that no extra training is possible and the neural network will stop completely. Conversely, similarly to other activation functions, the sigmoid function shrinks a large input space to a tiny input space. Thus, the derivative of the sigmoid function will be small due to large variation at the input that produces a small variation at the output. In a shallow network, only some layers use these activations, which is not a significant issue. While using more layers will lead the gradient to become very small in the training stage, in this case, the network works efficiently. The back-propagation technique is used to determine the gradients of the neural networks. Initially, this technique determines the network derivatives of each layer in the reverse direction, starting from the last layer and progressing back to the first layer. The next step involves multiplying the derivatives of each layer down the network in a similar manner to the first step. For instance, multiplying N small derivatives together when there are N hidden layers employs an activation function such as the sigmoid function. Hence, the gradient declines exponentially while propagating back to the first layer. More specifically, the biases and weights of the first layers cannot be updated efficiently during the training stage because the gradient is small. Moreover, this condition decreases the overall network accuracy, as these first layers are frequently critical to recognizing the essential elements of the input data. However, such a problem can be avoided through employing activation functions. These functions lack the squishing property, i.e., the ability to squish the input space to within a small space. By mapping X to max, the ReLU [ 91 ] is the most popular selection, as it does not yield a small derivative that is employed in the field. Another solution involves employing the batch normalization layer [ 81 ]. As mentioned earlier, the problem occurs once a large input space is squashed into a small space, leading to vanishing the derivative. Employing batch normalization degrades this issue by simply normalizing the input, i.e., the expression | x | does not accomplish the exterior boundaries of the sigmoid function. The normalization process makes the largest part of it come down in the green area, which ensures that the derivative is large enough for further actions. Furthermore, faster hardware can tackle the previous issue, e.g. that provided by GPUs. This makes standard back-propagation possible for many deeper layers of the network compared to the time required to recognize the vanishing gradient problem [ 215 ].
Exploding gradient problem
Opposite to the vanishing problem is the one related to gradient. Specifically, large error gradients are accumulated during back-propagation [ 216 , 217 , 218 ]. The latter will lead to extremely significant updates to the weights of the network, meaning that the system becomes unsteady. Thus, the model will lose its ability to learn effectively. Grosso modo, moving backward in the network during back-propagation, the gradient grows exponentially by repetitively multiplying gradients. The weight values could thus become incredibly large and may overflow to become a not-a-number (NaN) value. Some potential solutions include:
Using different weight regularization techniques.
Redesigning the architecture of the network model.
Underspecification
In 2020, a team of computer scientists at Google has identified a new challenge called underspecification [ 219 ]. ML models including DL models often show surprisingly poor behavior when they are tested in real-world applications such as computer vision, medical imaging, natural language processing, and medical genomics. The reason behind the weak performance is due to underspecification. It has been shown that small modifications can force a model towards a completely different solution as well as lead to different predictions in deployment domains. There are different techniques of addressing underspecification issue. One of them is to design “stress tests” to examine how good a model works on real-world data and to find out the possible issues. Nevertheless, this demands a reliable understanding of the process the model can work inaccurately. The team stated that “Designing stress tests that are well-matched to applied requirements, and that provide good “coverage” of potential failure modes is a major challenge”. Underspecification puts major constraints on the credibility of ML predictions and may require some reconsidering over certain applications. Since ML is linked to human by serving several applications such as medical imaging and self-driving cars, it will require proper attention to this issue.
Applications of deep learning
Presently, various DL applications are widespread around the world. These applications include healthcare, social network analysis, audio and speech processing (like recognition and enhancement), visual data processing methods (such as multimedia data analysis and computer vision), and NLP (translation and sentence classification), among others (Fig. 29 ) [ 220 , 221 , 222 , 223 , 224 ]. These applications have been classified into five categories: classification, localization, detection, segmentation, and registration. Although each of these tasks has its own target, there is fundamental overlap in the pipeline implementation of these applications as shown in Fig. 30 . Classification is a concept that categorizes a set of data into classes. Detection is used to locate interesting objects in an image with consideration given to the background. In detection, multiple objects, which could be from dissimilar classes, are surrounded by bounding boxes. Localization is the concept used to locate the object, which is surrounded by a single bounding box. In segmentation (semantic segmentation), the target object edges are surrounded by outlines, which also label them; moreover, fitting a single image (which could be 2D or 3D) onto another refers to registration. One of the most important and wide-ranging DL applications are in healthcare [ 225 , 226 , 227 , 228 , 229 , 230 ]. This area of research is critical due to its relation to human lives. Moreover, DL has shown tremendous performance in healthcare. Therefore, we take DL applications in the medical image analysis field as an example to describe the DL applications.
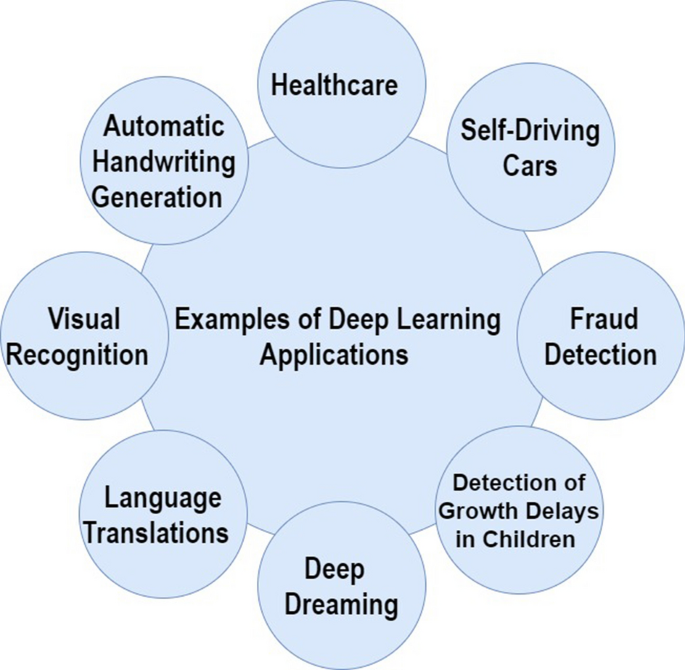
Examples of DL applications
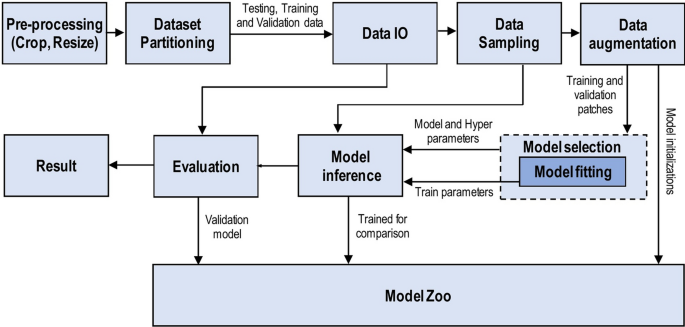
Workflow of deep learning tasks
Classification
Computer-Aided Diagnosis (CADx) is another title sometimes used for classification. Bharati et al. [ 231 ] used a chest X-ray dataset for detecting lung diseases based on a CNN. Another study attempted to read X-ray images by employing CNN [ 232 ]. In this modality, the comparative accessibility of these images has likely enhanced the progress of DL. [ 233 ] used an improved pre-trained GoogLeNet CNN containing more than 150,000 images for training and testing processes. This dataset was augmented from 1850 chest X-rays. The creators reorganized the image orientation into lateral and frontal views and achieved approximately 100% accuracy. This work of orientation classification has clinically limited use. As a part of an ultimately fully automated diagnosis workflow, it obtained the data augmentation and pre-trained efficiency in learning the metadata of relevant images. Chest infection, commonly referred to as pneumonia, is extremely treatable, as it is a commonly occurring health problem worldwide. Conversely, Rajpurkar et al. [ 234 ] utilized CheXNet, which is an improved version of DenseNet [ 112 ] with 121 convolution layers, for classifying fourteen types of disease. These authors used the CheXNet14 dataset [ 235 ], which comprises 112,000 images. This network achieved an excellent performance in recognizing fourteen different diseases. In particular, pneumonia classification accomplished a 0.7632 AUC score using receiver operating characteristics (ROC) analysis. In addition, the network obtained better than or equal to the performance of both a three-radiologist panel and four individual radiologists. Zuo et al. [ 236 ] have adopted CNN for candidate classification in lung nodule. Shen et al. [ 237 ] employed both Random Forest (RF) and SVM classifiers with CNNs to classify lung nodules. They employed two convolutional layers with each of the three parallel CNNs. The LIDC-IDRI (Lung Image Database Consortium) dataset, which contained 1010-labeled CT lung scans, was used to classify the two types of lung nodules (malignant and benign). Different scales of the image patches were used by every CNN to extract features, while the output feature vector was constructed using the learned features. Next, these vectors were classified into malignant or benign using either the RF classifier or SVM with radial basis function (RBF) filter. The model was robust to various noisy input levels and achieved an accuracy of 86% in nodule classification. Conversely, the model of [ 238 ] interpolates the image data missing between PET and MRI images using 3D CNNs. The Alzheimer Disease Neuroimaging Initiative (ADNI) database, containing 830 PET and MRI patient scans, was utilized in their work. The PET and MRI images are used to train the 3D CNNs, first as input and then as output. Furthermore, for patients who have no PET images, the 3D CNNs utilized the trained images to rebuild the PET images. These rebuilt images approximately fitted the actual disease recognition outcomes. However, this approach did not address the overfitting issues, which in turn restricted their technique in terms of its possible capacity for generalization. Diagnosing normal versus Alzheimer’s disease patients has been achieved by several CNN models [ 239 , 240 ]. Hosseini-Asl et al. [ 241 ] attained 99% accuracy for up-to-date outcomes in diagnosing normal versus Alzheimer’s disease patients. These authors applied an auto-encoder architecture using 3D CNNs. The generic brain features were pre-trained on the CADDementia dataset. Subsequently, the outcomes of these learned features became inputs to higher layers to differentiate between patient scans of Alzheimer’s disease, mild cognitive impairment, or normal brains based on the ADNI dataset and using fine-tuned deep supervision techniques. The architectures of VGGNet and RNNs, in that order, were the basis of both VOXCNN and ResNet models developed by Korolev et al. [ 242 ]. They also discriminated between Alzheimer’s disease and normal patients using the ADNI database. Accuracy was 79% for Voxnet and 80% for ResNet. Compared to Hosseini-Asl’s work, both models achieved lower accuracies. Conversely, the implementation of the algorithms was simpler and did not require feature hand-crafting, as Korolev declared. In 2020, Mehmood et al. [ 240 ] trained a developed CNN-based network called “SCNN” with MRI images for the tasks of classification of Alzheimer’s disease. They achieved state-of-the-art results by obtaining an accuracy of 99.05%.
Recently, CNN has taken some medical imaging classification tasks to different level from traditional diagnosis to automated diagnosis with tremendous performance. Examples of these tasks are diabetic foot ulcer (DFU) (as normal and abnormal (DFU) classes) [ 87 , 243 , 244 , 245 , 246 ], sickle cells anemia (SCA) (as normal, abnormal (SCA), and other blood components) [ 86 , 247 ], breast cancer by classify hematoxylin–eosin-stained breast biopsy images into four classes: invasive carcinoma, in-situ carcinoma, benign tumor and normal tissue [ 42 , 88 , 248 , 249 , 250 , 251 , 252 ], and multi-class skin cancer classification [ 253 , 254 , 255 ].
In 2020, CNNs are playing a vital role in early diagnosis of the novel coronavirus (COVID-2019). CNN has become the primary tool for automatic COVID-19 diagnosis in many hospitals around the world using chest X-ray images [ 256 , 257 , 258 , 259 , 260 ]. More details about the classification of medical imaging applications can be found in [ 226 , 261 , 262 , 263 , 264 , 265 ].
Localization
Although applications in anatomy education could increase, the practicing clinician is more likely to be interested in the localization of normal anatomy. Radiological images are independently examined and described outside of human intervention, while localization could be applied in completely automatic end-to-end applications [ 266 , 267 , 268 ]. Zhao et al. [ 269 ] introduced a new deep learning-based approach to localize pancreatic tumor in projection X-ray images for image-guided radiation therapy without the need for fiducials. Roth et al. [ 270 ] constructed and trained a CNN using five convolutional layers to classify around 4000 transverse-axial CT images. These authors used five categories for classification: legs, pelvis, liver, lung, and neck. After data augmentation techniques were applied, they achieved an AUC score of 0.998 and the classification error rate of the model was 5.9%. For detecting the positions of the spleen, kidney, heart, and liver, Shin et al. [ 271 ] employed stacked auto-encoders on 78 contrast-improved MRI scans of the stomach area containing the kidneys or liver. Temporal and spatial domains were used to learn the hierarchal features. Based on the organs, these approaches achieved detection accuracies of 62–79%. Sirazitdinov et al. [ 268 ] presented an aggregate of two convolutional neural networks, namely RetinaNet and Mask R-CNN for pneumonia detection and localization.
Computer-Aided Detection (CADe) is another method used for detection. For both the clinician and the patient, overlooking a lesion on a scan may have dire consequences. Thus, detection is a field of study requiring both accuracy and sensitivity [ 272 , 273 , 274 ]. Chouhan et al. [ 275 ] introduced an innovative deep learning framework for the detection of pneumonia by adopting the idea of transfer learning. Their approach obtained an accuracy of 96.4% with a recall of 99.62% on unseen data. In the area of COVID-19 and pulmonary disease, several convolutional neural network approaches have been proposed for automatic detection from X-ray images which showed an excellent performance [ 46 , 276 , 277 , 278 , 279 ].
In the area of skin cancer, there several applications were introduced for the detection task [ 280 , 281 , 282 ]. Thurnhofer-Hemsi et al. [ 283 ] introduced a deep learning approach for skin cancer detection by fine-tuning five state-of-art convolutional neural network models. They addressed the issue of a lack of training data by adopting the ideas of transfer learning and data augmentation techniques. DenseNet201 network has shown superior results compared to other models.
Another interesting area is that of histopathological images, which are progressively digitized. Several papers have been published in this field [ 284 , 285 , 286 , 287 , 288 , 289 , 290 ]. Human pathologists read these images laboriously; they search for malignancy markers, such as a high index of cell proliferation, using molecular markers (e.g. Ki-67), cellular necrosis signs, abnormal cellular architecture, enlarged numbers of mitotic figures denoting augmented cell replication, and enlarged nucleus-to-cytoplasm ratios. Note that the histopathological slide may contain a huge number of cells (up to the thousands). Thus, the risk of disregarding abnormal neoplastic regions is high when wading through these cells at excessive levels of magnification. Ciresan et al. [ 291 ] employed CNNs of 11–13 layers for identifying mitotic figures. Fifty breast histology images from the MITOS dataset were used. Their technique attained recall and precision scores of 0.7 and 0.88 respectively. Sirinukunwattana et al. [ 292 ] utilized 100 histology images of colorectal adenocarcinoma to detect cell nuclei using CNNs. Roughly 30,000 nuclei were hand-labeled for training purposes. The novelty of this approach was in the use of Spatially Constrained CNN. This CNN detects the center of nuclei using the surrounding spatial context and spatial regression. Instead of this CNN, Xu et al. [ 293 ] employed a stacked sparse auto-encoder (SSAE) to identify nuclei in histological slides of breast cancer, achieving 0.83 and 0.89 recall and precision scores respectively. In this field, they showed that unsupervised learning techniques are also effectively utilized. In medical images, Albarquoni et al. [ 294 ] investigated the problem of insufficient labeling. They crowd-sourced the actual mitoses labeling in the histology images of breast cancer (from amateurs online). Solving the recurrent issue of inadequate labeling during the analysis of medical images can be achieved by feeding the crowd-sourced input labels into the CNN. This method signifies a remarkable proof-of-concept effort. In 2020, Lei et al. [ 285 ] introduced the employment of deep convolutional neural networks for automatic identification of mitotic candidates from histological sections for mitosis screening. They obtained the state-of-the-art detection results on the dataset of the International Pattern Recognition Conference (ICPR) 2012 Mitosis Detection Competition.
Segmentation
Although MRI and CT image segmentation research includes different organs such as knee cartilage, prostate, and liver, most research work has concentrated on brain segmentation, particularly tumors [ 295 , 296 , 297 , 298 , 299 , 300 ]. This issue is highly significant in surgical preparation to obtain the precise tumor limits for the shortest surgical resection. During surgery, excessive sacrificing of key brain regions may lead to neurological shortfalls including cognitive damage, emotionlessness, and limb difficulty. Conventionally, medical anatomical segmentation was done by hand; more specifically, the clinician draws out lines within the complete stack of the CT or MRI volume slice by slice. Thus, it is perfect for implementing a solution that computerizes this painstaking work. Wadhwa et al. [ 301 ] presented a brief overview on brain tumor segmentation of MRI images. Akkus et al. [ 302 ] wrote a brilliant review of brain MRI segmentation that addressed the different metrics and CNN architectures employed. Moreover, they explain several competitions in detail, as well as their datasets, which included Ischemic Stroke Lesion Segmentation (ISLES), Mild Traumatic brain injury Outcome Prediction (MTOP), and Brain Tumor Segmentation (BRATS).
Chen et al. [ 299 ] proposed convolutional neural networks for precise brain tumor segmentation. The approach that they employed involves several approaches for better features learning including the DeepMedic model, a novel dual-force training scheme, a label distribution-based loss function, and Multi-Layer Perceptron-based post-processing. They conducted their method on the two most modern brain tumor segmentation datasets, i.e., BRATS 2017 and BRATS 2015 datasets. Hu et al. [ 300 ] introduced the brain tumor segmentation method by adopting a multi-cascaded convolutional neural network (MCCNN) and fully connected conditional random fields (CRFs). The achieved results were excellent compared with the state-of-the-art methods.
Moeskops et al. [ 303 ] employed three parallel-running CNNs, each of which had a 2D input patch of dissimilar size, for segmenting and classifying MRI brain images. These images, which include 35 adults and 22 pre-term infants, were classified into various tissue categories such as cerebrospinal fluid, grey matter, and white matter. Every patch concentrates on capturing various image aspects with the benefit of employing three dissimilar sizes of input patch; here, the bigger sizes incorporated the spatial features, while the lowest patch sizes concentrated on the local textures. In general, the algorithm has Dice coefficients in the range of 0.82–0.87 and achieved a satisfactory accuracy. Although 2D image slices are employed in the majority of segmentation research, Milletrate et al. [ 304 ] implemented 3D CNN for segmenting MRI prostate images. Furthermore, they used the PROMISE2012 challenge dataset, from which fifty MRI scans were used for training and thirty for testing. The U-Net architecture of Ronnerberger et al. [ 305 ] inspired their V-net. This model attained a 0.869 Dice coefficient score, the same as the winning teams in the competition. To reduce overfitting and create the model of a deeper 11-convolutional layer CNN, Pereira et al. [ 306 ] applied intentionally small-sized filters of 3x3. Their model used MRI scans of 274 gliomas (a type of brain tumor) for training. They achieved first place in the 2013 BRATS challenge, as well as second place in the BRATS challenge 2015. Havaei et al. [ 307 ] also considered gliomas using the 2013 BRATS dataset. They investigated different 2D CNN architectures. Compared to the winner of BRATS 2013, their algorithm worked better, as it required only 3 min to execute rather than 100 min. The concept of cascaded architecture formed the basis of their model. Thus, it is referred to as an InputCascadeCNN. Employing FC Conditional Random Fields (CRFs), atrous spatial pyramid pooling, and up-sampled filters were techniques introduced by Chen et al. [ 308 ]. These authors aimed to enhance the accuracy of localization and enlarge the field of view of every filter at a multi-scale. Their model, DeepLab, attained 79.7% mIOU (mean Intersection Over Union). In the PASCAL VOC-2012 image segmentation, their model obtained an excellent performance.
Recently, the Automatic segmentation of COVID-19 Lung Infection from CT Images helps to detect the development of COVID-19 infection by employing several deep learning techniques [ 309 , 310 , 311 , 312 ].
Registration
Usually, given two input images, the four main stages of the canonical procedure of the image registration task are [ 313 , 314 ]:
Target Selection: it illustrates the determined input image that the second counterpart input image needs to remain accurately superimposed to.
Feature Extraction: it computes the set of features extracted from each input image.
Feature Matching: it allows finding similarities between the previously obtained features.
Pose Optimization: it is aimed to minimize the distance between both input images.
Then, the result of the registration procedure is the suitable geometric transformation (e.g. translation, rotation, scaling, etc.) that provides both input images within the same coordinate system in a way the distance between them is minimal, i.e. their level of superimposition/overlapping is optimal. It is out of the scope of this work to provide an extensive review of this topic. Nevertheless, a short summary is accordingly introduced next.
Commonly, the input images for the DL-based registration approach could be in various forms, e.g. point clouds, voxel grids, and meshes. Additionally, some techniques allow as inputs the result of the Feature Extraction or Matching steps in the canonical scheme. Specifically, the outcome could be some data in a particular form as well as the result of the steps from the classical pipeline (feature vector, matching vector, and transformation). Nevertheless, with the newest DL-based methods, a novel conceptual type of ecosystem issues. It contains acquired characteristics about the target, materials, and their behavior that can be registered with the input data. Such a conceptual ecosystem is formed by a neural network and its training manner, and it could be counted as an input to the registration approach. Nevertheless, it is not an input that one might adopt in every registration situation since it corresponds to an interior data representation.
From a DL view-point, the interpretation of the conceptual design enables differentiating the input data of a registration approach into defined or non-defined models. In particular, the illustrated phases are models that depict particular spatial data (e.g. 2D or 3D) while a non-defined one is a generalization of a data set created by a learning system. Yumer et al. [ 315 ] developed a framework in which the model acquires characteristics of objects, meaning ready to identify what a more sporty car seems like or a more comfy chair is, also adjusting a 3D model to fit those characteristics while maintaining the main characteristics of the primary data. Likewise, a fundamental perspective of the unsupervised learning method introduced by Ding et al. [ 316 ] is that there is no target for the registration approach. In this instance, the network is able of placing each input point cloud in a global space, solving SLAM issues in which many point clouds have to be registered rigidly. On the other hand, Mahadevan [ 317 ] proposed the combination of two conceptual models utilizing the growth of Imagination Machines to give flexible artificial intelligence systems and relationships between the learned phases through training schemes that are not inspired on labels and classifications. Another practical application of DL, especially CNNs, to image registration is the 3D reconstruction of objects. Wang et al. [ 318 ] applied an adversarial way using CNNs to rebuild a 3D model of an object from its 2D image. The network learns many objects and orally accomplishes the registration between the image and the conceptual model. Similarly, Hermoza et al. [ 319 ] also utilize the GAN network for prognosticating the absent geometry of damaged archaeological objects, providing the reconstructed object based on a voxel grid format and a label selecting its class.
DL for medical image registration has numerous applications, which were listed by some review papers [ 320 , 321 , 322 ]. Yang et al. [ 323 ] implemented stacked convolutional layers as an encoder-decoder approach to predict the morphing of the input pixel into its last formation using MRI brain scans from the OASIS dataset. They employed a registration model known as Large Deformation Diffeomorphic Metric Mapping (LDDMM) and attained remarkable enhancements in computation time. Miao et al. [ 324 ] used synthetic X-ray images to train a five-layer CNN to register 3D models of a trans-esophageal probe, a hand implant, and a knee implant onto 2D X-ray images for pose estimation. They determined that their model achieved an execution time of 0.1 s, representing an important enhancement against the conventional registration techniques based on intensity; moreover, it achieved effective registrations 79–99% of the time. Li et al. [ 325 ] introduced a neural network-based approach for the non-rigid 2D–3D registration of the lateral cephalogram and the volumetric cone-beam CT (CBCT) images.
Computational approaches
For computationally exhaustive applications, complex ML and DL approaches have rapidly been identified as the most significant techniques and are widely used in different fields. The development and enhancement of algorithms aggregated with capabilities of well-behaved computational performance and large datasets make it possible to effectively execute several applications, as earlier applications were either not possible or difficult to take into consideration.
Currently, several standard DNN configurations are available. The interconnection patterns between layers and the total number of layers represent the main differences between these configurations. The Table 2 illustrates the growth rate of the overall number of layers over time, which seems to be far faster than the “Moore’s Law growth rate”. In normal DNN, the number of layers grew by around 2.3× each year in the period from 2012 to 2016. Recent investigations of future ResNet versions reveal that the number of layers can be extended up to 1000. However, an SGD technique is employed to fit the weights (or parameters), while different optimization techniques are employed to obtain parameter updating during the DNN training process. Repetitive updates are required to enhance network accuracy in addition to a minorly augmented rate of enhancement. For example, the training process using ImageNet as a large dataset, which contains more than 14 million images, along with ResNet as a network model, take around 30K to 40K repetitions to converge to a steady solution. In addition, the overall computational load, as an upper-level prediction, may exceed 1020 FLOPS when both the training set size and the DNN complexity increase.
Prior to 2008, boosting the training to a satisfactory extent was achieved by using GPUs. Usually, days or weeks are needed for a training session, even with GPU support. By contrast, several optimization strategies were developed to reduce the extensive learning time. The computational requirements are believed to increase as the DNNs continuously enlarge in both complexity and size.
In addition to the computational load cost, the memory bandwidth and capacity have a significant effect on the entire training performance, and to a lesser extent, deduction. More specifically, the parameters are distributed through every layer of the input data, there is a sizeable amount of reused data, and the computation of several network layers exhibits an excessive computation-to-bandwidth ratio. By contrast, there are no distributed parameters, the amount of reused data is extremely small, and the additional FC layers have an extremely small computation-to-bandwidth ratio. Table 3 presents a comparison between different aspects related to the devices. In addition, the table is established to facilitate familiarity with the tradeoffs by obtaining the optimal approach for configuring a system based on either FPGA, GPU, or CPU devices. It should be noted that each has corresponding weaknesses and strengths; accordingly, there are no clear one-size-fits-all solutions.
Although GPU processing has enhanced the ability to address the computational challenges related to such networks, the maximum GPU (or CPU) performance is not achieved, and several techniques or models have turned out to be strongly linked to bandwidth. In the worst cases, the GPU efficiency is between 15 and 20% of the maximum theoretical performance. This issue is required to enlarge the memory bandwidth using high-bandwidth stacked memory. Next, different approaches based on FPGA, GPU, and CPU are accordingly detailed.
CPU-based approach
The well-behaved performance of the CPU nodes usually assists robust network connectivity, storage abilities, and large memory. Although CPU nodes are more common-purpose than those of FPGA or GPU, they lack the ability to match them in unprocessed computation facilities, since this requires increased network ability and a larger memory capacity.
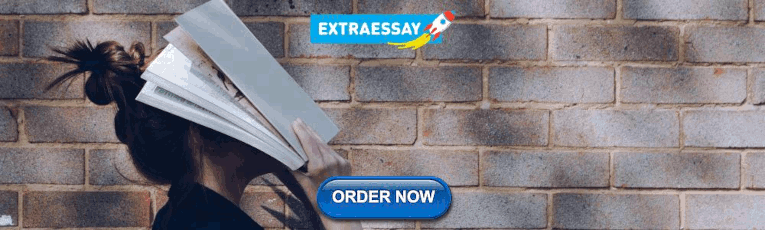
GPU-based approach
GPUs are extremely effective for several basic DL primitives, which include greatly parallel-computing operations such as activation functions, matrix multiplication, and convolutions [ 326 , 327 , 328 , 329 , 330 ]. Incorporating HBM-stacked memory into the up-to-date GPU models significantly enhances the bandwidth. This enhancement allows numerous primitives to efficiently utilize all computational resources of the available GPUs. The improvement in GPU performance over CPU performance is usually 10-20:1 related to dense linear algebra operations.
Maximizing parallel processing is the base of the initial GPU programming model. For example, a GPU model may involve up to sixty-four computational units. There are four SIMD engines per each computational layer, and each SIMD has sixteen floating-point computation lanes. The peak performance is 25 TFLOPS (fp16) and 10 TFLOPS (fp32) as the percentage of the employment approaches 100%. Additional GPU performance may be achieved if the addition and multiply functions for vectors combine the inner production instructions for matching primitives related to matrix operations.
For DNN training, the GPU is usually considered to be an optimized design, while for inference operations, it may also offer considerable performance improvements.
FPGA-based approach
FPGA is wildly utilized in various tasks including deep learning [ 199 , 247 , 331 , 332 , 333 , 334 ]. Inference accelerators are commonly implemented utilizing FPGA. The FPGA can be effectively configured to reduce the unnecessary or overhead functions involved in GPU systems. Compared to GPU, the FPGA is restricted to both weak-behaved floating-point performance and integer inference. The main FPGA aspect is the capability to dynamically reconfigure the array characteristics (at run-time), as well as the capability to configure the array by means of effective design with little or no overhead.
As mentioned earlier, the FPGA offers both performance and latency for every watt it gains over GPU and CPU in DL inference operations. Implementation of custom high-performance hardware, pruned networks, and reduced arithmetic precision are three factors that enable the FPGA to implement DL algorithms and to achieve FPGA with this level of efficiency. In addition, FPGA may be employed to implement CNN overlay engines with over 80% efficiency, eight-bit accuracy, and over 15 TOPs peak performance; this is used for a few conventional CNNs, as Xillinx and partners demonstrated recently. By contrast, pruning techniques are mostly employed in the LSTM context. The sizes of the models can be efficiently minimized by up to 20×, which provides an important benefit during the implementation of the optimal solution, as MLP neural processing demonstrated. A recent study in the field of implementing fixed-point precision and custom floating-point has revealed that lowering the 8-bit is extremely promising; moreover, it aids in supplying additional advancements to implementing peak performance FPGA related to the DNN models.
Evaluation metrics
Evaluation metrics adopted within DL tasks play a crucial role in achieving the optimized classifier [ 335 ]. They are utilized within a usual data classification procedure through two main stages: training and testing. It is utilized to optimize the classification algorithm during the training stage. This means that the evaluation metric is utilized to discriminate and select the optimized solution, e.g., as a discriminator, which can generate an extra-accurate forecast of upcoming evaluations related to a specific classifier. For the time being, the evaluation metric is utilized to measure the efficiency of the created classifier, e.g. as an evaluator, within the model testing stage using hidden data. As given in Eq. 20 , TN and TP are defined as the number of negative and positive instances, respectively, which are successfully classified. In addition, FN and FP are defined as the number of misclassified positive and negative instances respectively. Next, some of the most well-known evaluation metrics are listed below.
Accuracy: Calculates the ratio of correct predicted classes to the total number of samples evaluated (Eq. 20 ).
Sensitivity or Recall: Utilized to calculate the fraction of positive patterns that are correctly classified (Eq. 21 ).
Specificity: Utilized to calculate the fraction of negative patterns that are correctly classified (Eq. 22 ).
Precision: Utilized to calculate the positive patterns that are correctly predicted by all predicted patterns in a positive class (Eq. 23 ).
F1-Score: Calculates the harmonic average between recall and precision rates (Eq. 24 ).
J Score: This metric is also called Youdens J statistic. Eq. 25 represents the metric.
False Positive Rate (FPR): This metric refers to the possibility of a false alarm ratio as calculated in Eq. 26
Area Under the ROC Curve: AUC is a common ranking type metric. It is utilized to conduct comparisons between learning algorithms [ 336 , 337 , 338 ], as well as to construct an optimal learning model [ 339 , 340 ]. In contrast to probability and threshold metrics, the AUC value exposes the entire classifier ranking performance. The following formula is used to calculate the AUC value for two-class problem [ 341 ] (Eq. 27 )
Here, \(S_{p}\) represents the sum of all positive ranked samples. The number of negative and positive samples is denoted as \(n_{n}\) and \(n_{p}\) , respectively. Compared to the accuracy metrics, the AUC value was verified empirically and theoretically, making it very helpful for identifying an optimized solution and evaluating the classifier performance through classification training.
When considering the discrimination and evaluation processes, the AUC performance was brilliant. However, for multiclass issues, the AUC computation is primarily cost-effective when discriminating a large number of created solutions. In addition, the time complexity for computing the AUC is \(O \left( |C|^{2} \; n\log n\right) \) with respect to the Hand and Till AUC model [ 341 ] and \(O \left( |C| \; n\log n\right) \) according to Provost and Domingo’s AUC model [ 336 ].
Frameworks and datasets
Several DL frameworks and datasets have been developed in the last few years. various frameworks and libraries have also been used in order to expedite the work with good results. Through their use, the training process has become easier. Table 4 lists the most utilized frameworks and libraries.
Based on the star ratings on Github, as well as our own background in the field, TensorFlow is deemed the most effective and easy to use. It has the ability to work on several platforms. (Github is one of the biggest software hosting sites, while Github stars refer to how well-regarded a project is on the site). Moreover, there are several other benchmark datasets employed for different DL tasks. Some of these are listed in Table 5 .
Summary and conclusion
Finally, it is mandatory the inclusion of a brief discussion by gathering all the relevant data provided along this extensive research. Next, an itemized analysis is presented in order to conclude our review and exhibit the future directions.
DL already experiences difficulties in simultaneously modeling multi-complex modalities of data. In recent DL developments, another common approach is that of multimodal DL.
DL requires sizeable datasets (labeled data preferred) to predict unseen data and to train the models. This challenge turns out to be particularly difficult when real-time data processing is required or when the provided datasets are limited (such as in the case of healthcare data). To alleviate this issue, TL and data augmentation have been researched over the last few years.
Although ML slowly transitions to semi-supervised and unsupervised learning to manage practical data without the need for manual human labeling, many of the current deep-learning models utilize supervised learning.
The CNN performance is greatly influenced by hyper-parameter selection. Any small change in the hyper-parameter values will affect the general CNN performance. Therefore, careful parameter selection is an extremely significant issue that should be considered during optimization scheme development.
Impressive and robust hardware resources like GPUs are required for effective CNN training. Moreover, they are also required for exploring the efficiency of using CNN in smart and embedded systems.
In the CNN context, ensemble learning [ 342 , 343 ] represents a prospective research area. The collection of different and multiple architectures will support the model in improving its generalizability across different image categories through extracting several levels of semantic image representation. Similarly, ideas such as new activation functions, dropout, and batch normalization also merit further investigation.
The exploitation of depth and different structural adaptations is significantly improved in the CNN learning capacity. Substituting the traditional layer configuration with blocks results in significant advances in CNN performance, as has been shown in the recent literature. Currently, developing novel and efficient block architectures is the main trend in new research models of CNN architectures. HRNet is only one example that shows there are always ways to improve the architecture.
It is expected that cloud-based platforms will play an essential role in the future development of computational DL applications. Utilizing cloud computing offers a solution to handling the enormous amount of data. It also helps to increase efficiency and reduce costs. Furthermore, it offers the flexibility to train DL architectures.
With the recent development in computational tools including a chip for neural networks and a mobile GPU, we will see more DL applications on mobile devices. It will be easier for users to use DL.
Regarding the issue of lack of training data, It is expected that various techniques of transfer learning will be considered such as training the DL model on large unlabeled image datasets and next transferring the knowledge to train the DL model on a small number of labeled images for the same task.
Last, this overview provides a starting point for the community of DL being interested in the field of DL. Furthermore, researchers would be allowed to decide the more suitable direction of work to be taken in order to provide more accurate alternatives to the field.
Availability of data and materials
Not applicable.
Rozenwald MB, Galitsyna AA, Sapunov GV, Khrameeva EE, Gelfand MS. A machine learning framework for the prediction of chromatin folding in Drosophila using epigenetic features. PeerJ Comput Sci. 2020;6:307.
Article Google Scholar
Amrit C, Paauw T, Aly R, Lavric M. Identifying child abuse through text mining and machine learning. Expert Syst Appl. 2017;88:402–18.
Hossain E, Khan I, Un-Noor F, Sikander SS, Sunny MSH. Application of big data and machine learning in smart grid, and associated security concerns: a review. IEEE Access. 2019;7:13960–88.
Crawford M, Khoshgoftaar TM, Prusa JD, Richter AN, Al Najada H. Survey of review spam detection using machine learning techniques. J Big Data. 2015;2(1):23.
Deldjoo Y, Elahi M, Cremonesi P, Garzotto F, Piazzolla P, Quadrana M. Content-based video recommendation system based on stylistic visual features. J Data Semant. 2016;5(2):99–113.
Al-Dulaimi K, Chandran V, Nguyen K, Banks J, Tomeo-Reyes I. Benchmarking hep-2 specimen cells classification using linear discriminant analysis on higher order spectra features of cell shape. Pattern Recogn Lett. 2019;125:534–41.
Liu W, Wang Z, Liu X, Zeng N, Liu Y, Alsaadi FE. A survey of deep neural network architectures and their applications. Neurocomputing. 2017;234:11–26.
Pouyanfar S, Sadiq S, Yan Y, Tian H, Tao Y, Reyes MP, Shyu ML, Chen SC, Iyengar S. A survey on deep learning: algorithms, techniques, and applications. ACM Comput Surv (CSUR). 2018;51(5):1–36.
Alom MZ, Taha TM, Yakopcic C, Westberg S, Sidike P, Nasrin MS, Hasan M, Van Essen BC, Awwal AA, Asari VK. A state-of-the-art survey on deep learning theory and architectures. Electronics. 2019;8(3):292.
Potok TE, Schuman C, Young S, Patton R, Spedalieri F, Liu J, Yao KT, Rose G, Chakma G. A study of complex deep learning networks on high-performance, neuromorphic, and quantum computers. ACM J Emerg Technol Comput Syst (JETC). 2018;14(2):1–21.
Adeel A, Gogate M, Hussain A. Contextual deep learning-based audio-visual switching for speech enhancement in real-world environments. Inf Fusion. 2020;59:163–70.
Tian H, Chen SC, Shyu ML. Evolutionary programming based deep learning feature selection and network construction for visual data classification. Inf Syst Front. 2020;22(5):1053–66.
Young T, Hazarika D, Poria S, Cambria E. Recent trends in deep learning based natural language processing. IEEE Comput Intell Mag. 2018;13(3):55–75.
Koppe G, Meyer-Lindenberg A, Durstewitz D. Deep learning for small and big data in psychiatry. Neuropsychopharmacology. 2021;46(1):176–90.
Dalal N, Triggs B. Histograms of oriented gradients for human detection. In: 2005 IEEE computer society conference on computer vision and pattern recognition (CVPR’05), vol. 1. IEEE; 2005. p. 886–93.
Lowe DG. Object recognition from local scale-invariant features. In: Proceedings of the seventh IEEE international conference on computer vision, vol. 2. IEEE; 1999. p. 1150–7.
Wu L, Hoi SC, Yu N. Semantics-preserving bag-of-words models and applications. IEEE Trans Image Process. 2010;19(7):1908–20.
Article MathSciNet MATH Google Scholar
LeCun Y, Bengio Y, Hinton G. Deep learning. Nature. 2015;521(7553):436–44.
Yao G, Lei T, Zhong J. A review of convolutional-neural-network-based action recognition. Pattern Recogn Lett. 2019;118:14–22.
Dhillon A, Verma GK. Convolutional neural network: a review of models, methodologies and applications to object detection. Prog Artif Intell. 2020;9(2):85–112.
Khan A, Sohail A, Zahoora U, Qureshi AS. A survey of the recent architectures of deep convolutional neural networks. Artif Intell Rev. 2020;53(8):5455–516.
Hasan RI, Yusuf SM, Alzubaidi L. Review of the state of the art of deep learning for plant diseases: a broad analysis and discussion. Plants. 2020;9(10):1302.
Xiao Y, Tian Z, Yu J, Zhang Y, Liu S, Du S, Lan X. A review of object detection based on deep learning. Multimed Tools Appl. 2020;79(33):23729–91.
Ker J, Wang L, Rao J, Lim T. Deep learning applications in medical image analysis. IEEE Access. 2017;6:9375–89.
Zhang Z, Cui P, Zhu W. Deep learning on graphs: a survey. IEEE Trans Knowl Data Eng. 2020. https://doi.org/10.1109/TKDE.2020.2981333 .
Shrestha A, Mahmood A. Review of deep learning algorithms and architectures. IEEE Access. 2019;7:53040–65.
Najafabadi MM, Villanustre F, Khoshgoftaar TM, Seliya N, Wald R, Muharemagic E. Deep learning applications and challenges in big data analytics. J Big Data. 2015;2(1):1.
Goodfellow I, Bengio Y, Courville A, Bengio Y. Deep learning, vol. 1. Cambridge: MIT press; 2016.
MATH Google Scholar
Shorten C, Khoshgoftaar TM, Furht B. Deep learning applications for COVID-19. J Big Data. 2021;8(1):1–54.
Krizhevsky A, Sutskever I, Hinton GE. Imagenet classification with deep convolutional neural networks. Commun ACM. 2017;60(6):84–90.
Bhowmick S, Nagarajaiah S, Veeraraghavan A. Vision and deep learning-based algorithms to detect and quantify cracks on concrete surfaces from uav videos. Sensors. 2020;20(21):6299.
Goh GB, Hodas NO, Vishnu A. Deep learning for computational chemistry. J Comput Chem. 2017;38(16):1291–307.
Li Y, Zhang T, Sun S, Gao X. Accelerating flash calculation through deep learning methods. J Comput Phys. 2019;394:153–65.
Yang W, Zhang X, Tian Y, Wang W, Xue JH, Liao Q. Deep learning for single image super-resolution: a brief review. IEEE Trans Multimed. 2019;21(12):3106–21.
Tang J, Li S, Liu P. A review of lane detection methods based on deep learning. Pattern Recogn. 2020;111:107623.
Zhao ZQ, Zheng P, Xu ST, Wu X. Object detection with deep learning: a review. IEEE Trans Neural Netw Learn Syst. 2019;30(11):3212–32.
He K, Zhang X, Ren S, Sun J. Deep residual learning for image recognition. In: Proceedings of the IEEE conference on computer vision and pattern recognition; 2016. p. 770–8.
Ng A. Machine learning yearning: technical strategy for AI engineers in the era of deep learning. 2019. https://www.mlyearning.org .
Metz C. Turing award won by 3 pioneers in artificial intelligence. The New York Times. 2019;27.
Nevo S, Anisimov V, Elidan G, El-Yaniv R, Giencke P, Gigi Y, Hassidim A, Moshe Z, Schlesinger M, Shalev G, et al. Ml for flood forecasting at scale; 2019. arXiv preprint arXiv:1901.09583 .
Chen H, Engkvist O, Wang Y, Olivecrona M, Blaschke T. The rise of deep learning in drug discovery. Drug Discov Today. 2018;23(6):1241–50.
Benhammou Y, Achchab B, Herrera F, Tabik S. Breakhis based breast cancer automatic diagnosis using deep learning: taxonomy, survey and insights. Neurocomputing. 2020;375:9–24.
Wulczyn E, Steiner DF, Xu Z, Sadhwani A, Wang H, Flament-Auvigne I, Mermel CH, Chen PHC, Liu Y, Stumpe MC. Deep learning-based survival prediction for multiple cancer types using histopathology images. PLoS ONE. 2020;15(6):e0233678.
Nagpal K, Foote D, Liu Y, Chen PHC, Wulczyn E, Tan F, Olson N, Smith JL, Mohtashamian A, Wren JH, et al. Development and validation of a deep learning algorithm for improving Gleason scoring of prostate cancer. NPJ Digit Med. 2019;2(1):1–10.
Google Scholar
Esteva A, Kuprel B, Novoa RA, Ko J, Swetter SM, Blau HM, Thrun S. Dermatologist-level classification of skin cancer with deep neural networks. Nature. 2017;542(7639):115–8.
Brunese L, Mercaldo F, Reginelli A, Santone A. Explainable deep learning for pulmonary disease and coronavirus COVID-19 detection from X-rays. Comput Methods Programs Biomed. 2020;196(105):608.
Jamshidi M, Lalbakhsh A, Talla J, Peroutka Z, Hadjilooei F, Lalbakhsh P, Jamshidi M, La Spada L, Mirmozafari M, Dehghani M, et al. Artificial intelligence and COVID-19: deep learning approaches for diagnosis and treatment. IEEE Access. 2020;8:109581–95.
Shorfuzzaman M, Hossain MS. Metacovid: a siamese neural network framework with contrastive loss for n-shot diagnosis of COVID-19 patients. Pattern Recogn. 2020;113:107700.
Carvelli L, Olesen AN, Brink-Kjær A, Leary EB, Peppard PE, Mignot E, Sørensen HB, Jennum P. Design of a deep learning model for automatic scoring of periodic and non-periodic leg movements during sleep validated against multiple human experts. Sleep Med. 2020;69:109–19.
De Fauw J, Ledsam JR, Romera-Paredes B, Nikolov S, Tomasev N, Blackwell S, Askham H, Glorot X, O’Donoghue B, Visentin D, et al. Clinically applicable deep learning for diagnosis and referral in retinal disease. Nat Med. 2018;24(9):1342–50.
Topol EJ. High-performance medicine: the convergence of human and artificial intelligence. Nat Med. 2019;25(1):44–56.
Kermany DS, Goldbaum M, Cai W, Valentim CC, Liang H, Baxter SL, McKeown A, Yang G, Wu X, Yan F, et al. Identifying medical diagnoses and treatable diseases by image-based deep learning. Cell. 2018;172(5):1122–31.
Van Essen B, Kim H, Pearce R, Boakye K, Chen B. Lbann: livermore big artificial neural network HPC toolkit. In: Proceedings of the workshop on machine learning in high-performance computing environments; 2015. p. 1–6.
Saeed MM, Al Aghbari Z, Alsharidah M. Big data clustering techniques based on spark: a literature review. PeerJ Comput Sci. 2020;6:321.
Mnih V, Kavukcuoglu K, Silver D, Rusu AA, Veness J, Bellemare MG, Graves A, Riedmiller M, Fidjeland AK, Ostrovski G, et al. Human-level control through deep reinforcement learning. Nature. 2015;518(7540):529–33.
Arulkumaran K, Deisenroth MP, Brundage M, Bharath AA. Deep reinforcement learning: a brief survey. IEEE Signal Process Mag. 2017;34(6):26–38.
Socher R, Perelygin A, Wu J, Chuang J, Manning CD, Ng AY, Potts C. Recursive deep models for semantic compositionality over a sentiment treebank. In: Proceedings of the 2013 conference on empirical methods in natural language processing; 2013. p. 1631–42.
Goller C, Kuchler A. Learning task-dependent distributed representations by backpropagation through structure. In: Proceedings of international conference on neural networks (ICNN’96), vol 1. IEEE; 1996. p. 347–52.
Socher R, Lin CCY, Ng AY, Manning CD. Parsing natural scenes and natural language with recursive neural networks. In: ICML; 2011.
Louppe G, Cho K, Becot C, Cranmer K. QCD-aware recursive neural networks for jet physics. J High Energy Phys. 2019;2019(1):57.
Sadr H, Pedram MM, Teshnehlab M. A robust sentiment analysis method based on sequential combination of convolutional and recursive neural networks. Neural Process Lett. 2019;50(3):2745–61.
Urban G, Subrahmanya N, Baldi P. Inner and outer recursive neural networks for chemoinformatics applications. J Chem Inf Model. 2018;58(2):207–11.
Hewamalage H, Bergmeir C, Bandara K. Recurrent neural networks for time series forecasting: current status and future directions. Int J Forecast. 2020;37(1):388–427.
Jiang Y, Kim H, Asnani H, Kannan S, Oh S, Viswanath P. Learn codes: inventing low-latency codes via recurrent neural networks. IEEE J Sel Areas Inf Theory. 2020;1(1):207–16.
John RA, Acharya J, Zhu C, Surendran A, Bose SK, Chaturvedi A, Tiwari N, Gao Y, He Y, Zhang KK, et al. Optogenetics inspired transition metal dichalcogenide neuristors for in-memory deep recurrent neural networks. Nat Commun. 2020;11(1):1–9.
Batur Dinler Ö, Aydin N. An optimal feature parameter set based on gated recurrent unit recurrent neural networks for speech segment detection. Appl Sci. 2020;10(4):1273.
Jagannatha AN, Yu H. Structured prediction models for RNN based sequence labeling in clinical text. In: Proceedings of the conference on empirical methods in natural language processing. conference on empirical methods in natural language processing, vol. 2016, NIH Public Access; 2016. p. 856.
Pascanu R, Gulcehre C, Cho K, Bengio Y. How to construct deep recurrent neural networks. In: Proceedings of the second international conference on learning representations (ICLR 2014); 2014.
Glorot X, Bengio Y. Understanding the difficulty of training deep feedforward neural networks. In: Proceedings of the thirteenth international conference on artificial intelligence and statistics; 2010. p. 249–56.
Gao C, Yan J, Zhou S, Varshney PK, Liu H. Long short-term memory-based deep recurrent neural networks for target tracking. Inf Sci. 2019;502:279–96.
Zhou DX. Theory of deep convolutional neural networks: downsampling. Neural Netw. 2020;124:319–27.
Article MATH Google Scholar
Jhong SY, Tseng PY, Siriphockpirom N, Hsia CH, Huang MS, Hua KL, Chen YY. An automated biometric identification system using CNN-based palm vein recognition. In: 2020 international conference on advanced robotics and intelligent systems (ARIS). IEEE; 2020. p. 1–6.
Al-Azzawi A, Ouadou A, Max H, Duan Y, Tanner JJ, Cheng J. Deepcryopicker: fully automated deep neural network for single protein particle picking in cryo-EM. BMC Bioinform. 2020;21(1):1–38.
Wang T, Lu C, Yang M, Hong F, Liu C. A hybrid method for heartbeat classification via convolutional neural networks, multilayer perceptrons and focal loss. PeerJ Comput Sci. 2020;6:324.
Li G, Zhang M, Li J, Lv F, Tong G. Efficient densely connected convolutional neural networks. Pattern Recogn. 2021;109:107610.
Gu J, Wang Z, Kuen J, Ma L, Shahroudy A, Shuai B, Liu T, Wang X, Wang G, Cai J, et al. Recent advances in convolutional neural networks. Pattern Recogn. 2018;77:354–77.
Fang W, Love PE, Luo H, Ding L. Computer vision for behaviour-based safety in construction: a review and future directions. Adv Eng Inform. 2020;43:100980.
Palaz D, Magimai-Doss M, Collobert R. End-to-end acoustic modeling using convolutional neural networks for hmm-based automatic speech recognition. Speech Commun. 2019;108:15–32.
Li HC, Deng ZY, Chiang HH. Lightweight and resource-constrained learning network for face recognition with performance optimization. Sensors. 2020;20(21):6114.
Hubel DH, Wiesel TN. Receptive fields, binocular interaction and functional architecture in the cat’s visual cortex. J Physiol. 1962;160(1):106.
Ioffe S, Szegedy C. Batch normalization: accelerating deep network training by reducing internal covariate shift; 2015. arXiv preprint arXiv:1502.03167 .
Ruder S. An overview of gradient descent optimization algorithms; 2016. arXiv preprint arXiv:1609.04747 .
Bottou L. Large-scale machine learning with stochastic gradient descent. In: Proceedings of COMPSTAT’2010. Springer; 2010. p. 177–86.
Hinton G, Srivastava N, Swersky K. Neural networks for machine learning lecture 6a overview of mini-batch gradient descent. Cited on. 2012;14(8).
Zhang Z. Improved Adam optimizer for deep neural networks. In: 2018 IEEE/ACM 26th international symposium on quality of service (IWQoS). IEEE; 2018. p. 1–2.
Alzubaidi L, Fadhel MA, Al-Shamma O, Zhang J, Duan Y. Deep learning models for classification of red blood cells in microscopy images to aid in sickle cell anemia diagnosis. Electronics. 2020;9(3):427.
Alzubaidi L, Fadhel MA, Al-Shamma O, Zhang J, Santamaría J, Duan Y, Oleiwi SR. Towards a better understanding of transfer learning for medical imaging: a case study. Appl Sci. 2020;10(13):4523.
Alzubaidi L, Al-Shamma O, Fadhel MA, Farhan L, Zhang J, Duan Y. Optimizing the performance of breast cancer classification by employing the same domain transfer learning from hybrid deep convolutional neural network model. Electronics. 2020;9(3):445.
LeCun Y, Jackel LD, Bottou L, Cortes C, Denker JS, Drucker H, Guyon I, Muller UA, Sackinger E, Simard P, et al. Learning algorithms for classification: a comparison on handwritten digit recognition. Neural Netw Stat Mech Perspect. 1995;261:276.
Srivastava N, Hinton G, Krizhevsky A, Sutskever I, Salakhutdinov R. Dropout: a simple way to prevent neural networks from overfitting. J Mach Learn Res. 2014;15(1):1929–58.
MathSciNet MATH Google Scholar
Dahl GE, Sainath TN, Hinton GE. Improving deep neural networks for LVCSR using rectified linear units and dropout. In: 2013 IEEE international conference on acoustics, speech and signal processing. IEEE; 2013. p. 8609–13.
Xu B, Wang N, Chen T, Li M. Empirical evaluation of rectified activations in convolutional network; 2015. arXiv preprint arXiv:1505.00853 .
Hochreiter S. The vanishing gradient problem during learning recurrent neural nets and problem solutions. Int J Uncertain Fuzziness Knowl Based Syst. 1998;6(02):107–16.
Lin M, Chen Q, Yan S. Network in network; 2013. arXiv preprint arXiv:1312.4400 .
Hsiao TY, Chang YC, Chou HH, Chiu CT. Filter-based deep-compression with global average pooling for convolutional networks. J Syst Arch. 2019;95:9–18.
Li Z, Wang SH, Fan RR, Cao G, Zhang YD, Guo T. Teeth category classification via seven-layer deep convolutional neural network with max pooling and global average pooling. Int J Imaging Syst Technol. 2019;29(4):577–83.
Zeiler MD, Fergus R. Visualizing and understanding convolutional networks. In: European conference on computer vision. Springer; 2014. p. 818–33.
Erhan D, Bengio Y, Courville A, Vincent P. Visualizing higher-layer features of a deep network. Univ Montreal. 2009;1341(3):1.
Le QV. Building high-level features using large scale unsupervised learning. In: 2013 IEEE international conference on acoustics, speech and signal processing. IEEE; 2013. p. 8595–8.
Grün F, Rupprecht C, Navab N, Tombari F. A taxonomy and library for visualizing learned features in convolutional neural networks; 2016. arXiv preprint arXiv:1606.07757 .
Simonyan K, Zisserman A. Very deep convolutional networks for large-scale image recognition; 2014. arXiv preprint arXiv:1409.1556 .
Ranzato M, Huang FJ, Boureau YL, LeCun Y. Unsupervised learning of invariant feature hierarchies with applications to object recognition. In: 2007 IEEE conference on computer vision and pattern recognition. IEEE; 2007. p. 1–8.
Szegedy C, Liu W, Jia Y, Sermanet P, Reed S, Anguelov D, Erhan D, Vanhoucke V, Rabinovich A. Going deeper with convolutions. In: Proceedings of the IEEE conference on computer vision and pattern recognition; 2015. p. 1–9.
Bengio Y, et al. Rmsprop and equilibrated adaptive learning rates for nonconvex optimization; 2015. arXiv:1502.04390 corr abs/1502.04390
Srivastava RK, Greff K, Schmidhuber J. Highway networks; 2015. arXiv preprint arXiv:1505.00387 .
Kong W, Dong ZY, Jia Y, Hill DJ, Xu Y, Zhang Y. Short-term residential load forecasting based on LSTM recurrent neural network. IEEE Trans Smart Grid. 2017;10(1):841–51.
Ordóñez FJ, Roggen D. Deep convolutional and LSTM recurrent neural networks for multimodal wearable activity recognition. Sensors. 2016;16(1):115.
CireşAn D, Meier U, Masci J, Schmidhuber J. Multi-column deep neural network for traffic sign classification. Neural Netw. 2012;32:333–8.
Szegedy C, Ioffe S, Vanhoucke V, Alemi A. Inception-v4, inception-resnet and the impact of residual connections on learning; 2016. arXiv preprint arXiv:1602.07261 .
Szegedy C, Vanhoucke V, Ioffe S, Shlens J, Wojna Z. Rethinking the inception architecture for computer vision. In: Proceedings of the IEEE conference on computer vision and pattern recognition; 2016. p. 2818–26.
Wu S, Zhong S, Liu Y. Deep residual learning for image steganalysis. Multimed Tools Appl. 2018;77(9):10437–53.
Huang G, Liu Z, Van Der Maaten L, Weinberger KQ. Densely connected convolutional networks. In: Proceedings of the IEEE conference on computer vision and pattern recognition; 2017. p. 4700–08.
Rubin J, Parvaneh S, Rahman A, Conroy B, Babaeizadeh S. Densely connected convolutional networks for detection of atrial fibrillation from short single-lead ECG recordings. J Electrocardiol. 2018;51(6):S18-21.
Kuang P, Ma T, Chen Z, Li F. Image super-resolution with densely connected convolutional networks. Appl Intell. 2019;49(1):125–36.
Xie S, Girshick R, Dollár P, Tu Z, He K. Aggregated residual transformations for deep neural networks. In: Proceedings of the IEEE conference on computer vision and pattern recognition; 2017. p. 1492–500.
Su A, He X, Zhao X. Jpeg steganalysis based on ResNeXt with gauss partial derivative filters. Multimed Tools Appl. 2020;80(3):3349–66.
Yadav D, Jalal A, Garlapati D, Hossain K, Goyal A, Pant G. Deep learning-based ResNeXt model in phycological studies for future. Algal Res. 2020;50:102018.
Han W, Feng R, Wang L, Gao L. Adaptive spatial-scale-aware deep convolutional neural network for high-resolution remote sensing imagery scene classification. In: IGARSS 2018-2018 IEEE international geoscience and remote sensing symposium. IEEE; 2018. p. 4736–9.
Zagoruyko S, Komodakis N. Wide residual networks; 2016. arXiv preprint arXiv:1605.07146 .
Huang G, Sun Y, Liu Z, Sedra D, Weinberger KQ. Deep networks with stochastic depth. In: European conference on computer vision. Springer; 2016. p. 646–61.
Huynh HT, Nguyen H. Joint age estimation and gender classification of Asian faces using wide ResNet. SN Comput Sci. 2020;1(5):1–9.
Takahashi R, Matsubara T, Uehara K. Data augmentation using random image cropping and patching for deep cnns. IEEE Trans Circuits Syst Video Technol. 2019;30(9):2917–31.
Han D, Kim J, Kim J. Deep pyramidal residual networks. In: Proceedings of the IEEE conference on computer vision and pattern recognition; 2017. p. 5927–35.
Wang Y, Wang L, Wang H, Li P. End-to-end image super-resolution via deep and shallow convolutional networks. IEEE Access. 2019;7:31959–70.
Chollet F. Xception: Deep learning with depthwise separable convolutions. In: Proceedings of the IEEE conference on computer vision and pattern recognition; 2017. p. 1251–8.
Lo WW, Yang X, Wang Y. An xception convolutional neural network for malware classification with transfer learning. In: 2019 10th IFIP international conference on new technologies, mobility and security (NTMS). IEEE; 2019. p. 1–5.
Rahimzadeh M, Attar A. A modified deep convolutional neural network for detecting COVID-19 and pneumonia from chest X-ray images based on the concatenation of xception and resnet50v2. Inform Med Unlocked. 2020;19:100360.
Wang F, Jiang M, Qian C, Yang S, Li C, Zhang H, Wang X, Tang X. Residual attention network for image classification. In: Proceedings of the IEEE conference on computer vision and pattern recognition; 2017. p. 3156–64.
Salakhutdinov R, Larochelle H. Efficient learning of deep boltzmann machines. In: Proceedings of the thirteenth international conference on artificial intelligence and statistics; 2010. p. 693–700.
Goh H, Thome N, Cord M, Lim JH. Top-down regularization of deep belief networks. Adv Neural Inf Process Syst. 2013;26:1878–86.
Guan J, Lai R, Xiong A, Liu Z, Gu L. Fixed pattern noise reduction for infrared images based on cascade residual attention CNN. Neurocomputing. 2020;377:301–13.
Bi Q, Qin K, Zhang H, Li Z, Xu K. RADC-Net: a residual attention based convolution network for aerial scene classification. Neurocomputing. 2020;377:345–59.
Jaderberg M, Simonyan K, Zisserman A, et al. Spatial transformer networks. In: Advances in neural information processing systems. San Mateo: Morgan Kaufmann Publishers; 2015. p. 2017–25.
Hu J, Shen L, Sun G. Squeeze-and-excitation networks. In: Proceedings of the IEEE conference on computer vision and pattern recognition; 2018. p. 7132–41.
Mou L, Zhu XX. Learning to pay attention on spectral domain: a spectral attention module-based convolutional network for hyperspectral image classification. IEEE Trans Geosci Remote Sens. 2019;58(1):110–22.
Woo S, Park J, Lee JY, So Kweon I. CBAM: Convolutional block attention module. In: Proceedings of the European conference on computer vision (ECCV); 2018. p. 3–19.
Roy AG, Navab N, Wachinger C. Concurrent spatial and channel ‘squeeze & excitation’ in fully convolutional networks. In: International conference on medical image computing and computer-assisted intervention. Springer; 2018. p. 421–9.
Roy AG, Navab N, Wachinger C. Recalibrating fully convolutional networks with spatial and channel “squeeze and excitation’’ blocks. IEEE Trans Med Imaging. 2018;38(2):540–9.
Sabour S, Frosst N, Hinton GE. Dynamic routing between capsules. In: Advances in neural information processing systems. San Mateo: Morgan Kaufmann Publishers; 2017. p. 3856–66.
Arun P, Buddhiraju KM, Porwal A. Capsulenet-based spatial-spectral classifier for hyperspectral images. IEEE J Sel Topics Appl Earth Obs Remote Sens. 2019;12(6):1849–65.
Xinwei L, Lianghao X, Yi Y. Compact video fingerprinting via an improved capsule net. Syst Sci Control Eng. 2020;9:1–9.
Ma B, Li X, Xia Y, Zhang Y. Autonomous deep learning: a genetic DCNN designer for image classification. Neurocomputing. 2020;379:152–61.
Wang J, Sun K, Cheng T, Jiang B, Deng C, Zhao Y, Liu D, Mu Y, Tan M, Wang X, et al. Deep high-resolution representation learning for visual recognition. IEEE Trans Pattern Anal Mach Intell. 2020. https://doi.org/10.1109/TPAMI.2020.2983686 .
Cheng B, Xiao B, Wang J, Shi H, Huang TS, Zhang L. Higherhrnet: scale-aware representation learning for bottom-up human pose estimation. In: CVPR 2020; 2020. https://www.microsoft.com/en-us/research/publication/higherhrnet-scale-aware-representation-learning-for-bottom-up-human-pose-estimation/ .
Karimi H, Derr T, Tang J. Characterizing the decision boundary of deep neural networks; 2019. arXiv preprint arXiv:1912.11460 .
Li Y, Ding L, Gao X. On the decision boundary of deep neural networks; 2018. arXiv preprint arXiv:1808.05385 .
Yosinski J, Clune J, Bengio Y, Lipson H. How transferable are features in deep neural networks? In: Advances in neural information processing systems. San Mateo: Morgan Kaufmann Publishers; 2014. p. 3320–8.
Tan C, Sun F, Kong T, Zhang W, Yang C, Liu C. A survey on deep transfer learning. In: International conference on artificial neural networks. Springer; 2018. p. 270–9.
Weiss K, Khoshgoftaar TM, Wang D. A survey of transfer learning. J Big Data. 2016;3(1):9.
Shorten C, Khoshgoftaar TM. A survey on image data augmentation for deep learning. J Big Data. 2019;6(1):60.
Wang F, Wang H, Wang H, Li G, Situ G. Learning from simulation: an end-to-end deep-learning approach for computational ghost imaging. Opt Express. 2019;27(18):25560–72.
Pan W. A survey of transfer learning for collaborative recommendation with auxiliary data. Neurocomputing. 2016;177:447–53.
Deng J, Dong W, Socher R, Li LJ, Li K, Fei-Fei L. Imagenet: a large-scale hierarchical image database. In: 2009 IEEE conference on computer vision and pattern recognition. IEEE; 2009. p. 248–55.
Cook D, Feuz KD, Krishnan NC. Transfer learning for activity recognition: a survey. Knowl Inf Syst. 2013;36(3):537–56.
Cao X, Wang Z, Yan P, Li X. Transfer learning for pedestrian detection. Neurocomputing. 2013;100:51–7.
Raghu M, Zhang C, Kleinberg J, Bengio S. Transfusion: understanding transfer learning for medical imaging. In: Advances in neural information processing systems. San Mateo: Morgan Kaufmann Publishers; 2019. p. 3347–57.
Pham TN, Van Tran L, Dao SVT. Early disease classification of mango leaves using feed-forward neural network and hybrid metaheuristic feature selection. IEEE Access. 2020;8:189960–73.
Saleh AM, Hamoud T. Analysis and best parameters selection for person recognition based on gait model using CNN algorithm and image augmentation. J Big Data. 2021;8(1):1–20.
Hirahara D, Takaya E, Takahara T, Ueda T. Effects of data count and image scaling on deep learning training. PeerJ Comput Sci. 2020;6:312.
Moreno-Barea FJ, Strazzera F, Jerez JM, Urda D, Franco L. Forward noise adjustment scheme for data augmentation. In: 2018 IEEE symposium series on computational intelligence (SSCI). IEEE; 2018. p. 728–34.
Dua D, Karra Taniskidou E. Uci machine learning repository. Irvine: University of california. School of Information and Computer Science; 2017. http://archive.ics.uci.edu/ml
Johnson JM, Khoshgoftaar TM. Survey on deep learning with class imbalance. J Big Data. 2019;6(1):27.
Yang P, Zhang Z, Zhou BB, Zomaya AY. Sample subset optimization for classifying imbalanced biological data. In: Pacific-Asia conference on knowledge discovery and data mining. Springer; 2011. p. 333–44.
Yang P, Yoo PD, Fernando J, Zhou BB, Zhang Z, Zomaya AY. Sample subset optimization techniques for imbalanced and ensemble learning problems in bioinformatics applications. IEEE Trans Cybern. 2013;44(3):445–55.
Wang S, Sun S, Xu J. Auc-maximized deep convolutional neural fields for sequence labeling 2015. arXiv preprint arXiv:1511.05265 .
Li Y, Wang S, Umarov R, Xie B, Fan M, Li L, Gao X. Deepre: sequence-based enzyme EC number prediction by deep learning. Bioinformatics. 2018;34(5):760–9.
Li Y, Huang C, Ding L, Li Z, Pan Y, Gao X. Deep learning in bioinformatics: introduction, application, and perspective in the big data era. Methods. 2019;166:4–21.
Choi E, Bahadori MT, Sun J, Kulas J, Schuetz A, Stewart W. Retain: An interpretable predictive model for healthcare using reverse time attention mechanism. In: Advances in neural information processing systems. San Mateo: Morgan Kaufmann Publishers; 2016. p. 3504–12.
Ching T, Himmelstein DS, Beaulieu-Jones BK, Kalinin AA, Do BT, Way GP, Ferrero E, Agapow PM, Zietz M, Hoffman MM, et al. Opportunities and obstacles for deep learning in biology and medicine. J R Soc Interface. 2018;15(141):20170,387.
Zhou J, Troyanskaya OG. Predicting effects of noncoding variants with deep learning-based sequence model. Nat Methods. 2015;12(10):931–4.
Pokuri BSS, Ghosal S, Kokate A, Sarkar S, Ganapathysubramanian B. Interpretable deep learning for guided microstructure-property explorations in photovoltaics. NPJ Comput Mater. 2019;5(1):1–11.
Ribeiro MT, Singh S, Guestrin C. “Why should I trust you?” explaining the predictions of any classifier. In: Proceedings of the 22nd ACM SIGKDD international conference on knowledge discovery and data mining; 2016. p. 1135–44.
Wang L, Nie R, Yu Z, Xin R, Zheng C, Zhang Z, Zhang J, Cai J. An interpretable deep-learning architecture of capsule networks for identifying cell-type gene expression programs from single-cell RNA-sequencing data. Nat Mach Intell. 2020;2(11):1–11.
Sundararajan M, Taly A, Yan Q. Axiomatic attribution for deep networks; 2017. arXiv preprint arXiv:1703.01365 .
Platt J, et al. Probabilistic outputs for support vector machines and comparisons to regularized likelihood methods. Adv Large Margin Classif. 1999;10(3):61–74.
Nair T, Precup D, Arnold DL, Arbel T. Exploring uncertainty measures in deep networks for multiple sclerosis lesion detection and segmentation. Med Image Anal. 2020;59:101557.
Herzog L, Murina E, Dürr O, Wegener S, Sick B. Integrating uncertainty in deep neural networks for MRI based stroke analysis. Med Image Anal. 2020;65:101790.
Pereyra G, Tucker G, Chorowski J, Kaiser Ł, Hinton G. Regularizing neural networks by penalizing confident output distributions; 2017. arXiv preprint arXiv:1701.06548 .
Naeini MP, Cooper GF, Hauskrecht M. Obtaining well calibrated probabilities using bayesian binning. In: Proceedings of the... AAAI conference on artificial intelligence. AAAI conference on artificial intelligence, vol. 2015. NIH Public Access; 2015. p. 2901.
Li M, Sethi IK. Confidence-based classifier design. Pattern Recogn. 2006;39(7):1230–40.
Zadrozny B, Elkan C. Obtaining calibrated probability estimates from decision trees and Naive Bayesian classifiers. In: ICML, vol. 1, Citeseer; 2001. p. 609–16.
Steinwart I. Consistency of support vector machines and other regularized kernel classifiers. IEEE Trans Inf Theory. 2005;51(1):128–42.
Lee K, Lee K, Shin J, Lee H. Overcoming catastrophic forgetting with unlabeled data in the wild. In: Proceedings of the IEEE international conference on computer vision; 2019. p. 312–21.
Shmelkov K, Schmid C, Alahari K. Incremental learning of object detectors without catastrophic forgetting. In: Proceedings of the IEEE international conference on computer vision; 2017. p. 3400–09.
Zenke F, Gerstner W, Ganguli S. The temporal paradox of Hebbian learning and homeostatic plasticity. Curr Opin Neurobiol. 2017;43:166–76.
Andersen N, Krauth N, Nabavi S. Hebbian plasticity in vivo: relevance and induction. Curr Opin Neurobiol. 2017;45:188–92.
Zheng R, Chakraborti S. A phase ii nonparametric adaptive exponentially weighted moving average control chart. Qual Eng. 2016;28(4):476–90.
Rebuffi SA, Kolesnikov A, Sperl G, Lampert CH. ICARL: Incremental classifier and representation learning. In: Proceedings of the IEEE conference on computer vision and pattern recognition; 2017. p. 2001–10.
Hinton GE, Plaut DC. Using fast weights to deblur old memories. In: Proceedings of the ninth annual conference of the cognitive science society; 1987. p. 177–86.
Parisi GI, Kemker R, Part JL, Kanan C, Wermter S. Continual lifelong learning with neural networks: a review. Neural Netw. 2019;113:54–71.
Soltoggio A, Stanley KO, Risi S. Born to learn: the inspiration, progress, and future of evolved plastic artificial neural networks. Neural Netw. 2018;108:48–67.
Parisi GI, Tani J, Weber C, Wermter S. Lifelong learning of human actions with deep neural network self-organization. Neural Netw. 2017;96:137–49.
Cheng Y, Wang D, Zhou P, Zhang T. Model compression and acceleration for deep neural networks: the principles, progress, and challenges. IEEE Signal Process Mag. 2018;35(1):126–36.
Wiedemann S, Kirchhoffer H, Matlage S, Haase P, Marban A, Marinč T, Neumann D, Nguyen T, Schwarz H, Wiegand T, et al. Deepcabac: a universal compression algorithm for deep neural networks. IEEE J Sel Topics Signal Process. 2020;14(4):700–14.
Mehta N, Pandit A. Concurrence of big data analytics and healthcare: a systematic review. Int J Med Inform. 2018;114:57–65.
Esteva A, Robicquet A, Ramsundar B, Kuleshov V, DePristo M, Chou K, Cui C, Corrado G, Thrun S, Dean J. A guide to deep learning in healthcare. Nat Med. 2019;25(1):24–9.
Shawahna A, Sait SM, El-Maleh A. Fpga-based accelerators of deep learning networks for learning and classification: a review. IEEE Access. 2018;7:7823–59.
Min Z. Public welfare organization management system based on FPGA and deep learning. Microprocess Microsyst. 2020;80:103333.
Al-Shamma O, Fadhel MA, Hameed RA, Alzubaidi L, Zhang J. Boosting convolutional neural networks performance based on fpga accelerator. In: International conference on intelligent systems design and applications. Springer; 2018. p. 509–17.
Han S, Mao H, Dally WJ. Deep compression: compressing deep neural networks with pruning, trained quantization and huffman coding; 2015. arXiv preprint arXiv:1510.00149 .
Chen Z, Zhang L, Cao Z, Guo J. Distilling the knowledge from handcrafted features for human activity recognition. IEEE Trans Ind Inform. 2018;14(10):4334–42.
Hinton G, Vinyals O, Dean J. Distilling the knowledge in a neural network; 2015. arXiv preprint arXiv:1503.02531 .
Lenssen JE, Fey M, Libuschewski P. Group equivariant capsule networks. In: Advances in neural information processing systems. San Mateo: Morgan Kaufmann Publishers; 2018. p. 8844–53.
Denton EL, Zaremba W, Bruna J, LeCun Y, Fergus R. Exploiting linear structure within convolutional networks for efficient evaluation. In: Advances in neural information processing systems. San Mateo: Morgan Kaufmann Publishers; 2014. p. 1269–77.
Xu Q, Zhang M, Gu Z, Pan G. Overfitting remedy by sparsifying regularization on fully-connected layers of CNNs. Neurocomputing. 2019;328:69–74.
Zhang C, Bengio S, Hardt M, Recht B, Vinyals O. Understanding deep learning requires rethinking generalization. Commun ACM. 2018;64(3):107–15.
Xu X, Jiang X, Ma C, Du P, Li X, Lv S, Yu L, Ni Q, Chen Y, Su J, et al. A deep learning system to screen novel coronavirus disease 2019 pneumonia. Engineering. 2020;6(10):1122–9.
Sharma K, Alsadoon A, Prasad P, Al-Dala’in T, Nguyen TQV, Pham DTH. A novel solution of using deep learning for left ventricle detection: enhanced feature extraction. Comput Methods Programs Biomed. 2020;197:105751.
Zhang G, Wang C, Xu B, Grosse R. Three mechanisms of weight decay regularization; 2018. arXiv preprint arXiv:1810.12281 .
Laurent C, Pereyra G, Brakel P, Zhang Y, Bengio Y. Batch normalized recurrent neural networks. In: 2016 IEEE international conference on acoustics, speech and signal processing (ICASSP), IEEE; 2016. p. 2657–61.
Salamon J, Bello JP. Deep convolutional neural networks and data augmentation for environmental sound classification. IEEE Signal Process Lett. 2017;24(3):279–83.
Wang X, Qin Y, Wang Y, Xiang S, Chen H. ReLTanh: an activation function with vanishing gradient resistance for SAE-based DNNs and its application to rotating machinery fault diagnosis. Neurocomputing. 2019;363:88–98.
Tan HH, Lim KH. Vanishing gradient mitigation with deep learning neural network optimization. In: 2019 7th international conference on smart computing & communications (ICSCC). IEEE; 2019. p. 1–4.
MacDonald G, Godbout A, Gillcash B, Cairns S. Volume-preserving neural networks: a solution to the vanishing gradient problem; 2019. arXiv preprint arXiv:1911.09576 .
Mittal S, Vaishay S. A survey of techniques for optimizing deep learning on GPUs. J Syst Arch. 2019;99:101635.
Kanai S, Fujiwara Y, Iwamura S. Preventing gradient explosions in gated recurrent units. In: Advances in neural information processing systems. San Mateo: Morgan Kaufmann Publishers; 2017. p. 435–44.
Hanin B. Which neural net architectures give rise to exploding and vanishing gradients? In: Advances in neural information processing systems. San Mateo: Morgan Kaufmann Publishers; 2018. p. 582–91.
Ribeiro AH, Tiels K, Aguirre LA, Schön T. Beyond exploding and vanishing gradients: analysing RNN training using attractors and smoothness. In: International conference on artificial intelligence and statistics, PMLR; 2020. p. 2370–80.
D’Amour A, Heller K, Moldovan D, Adlam B, Alipanahi B, Beutel A, Chen C, Deaton J, Eisenstein J, Hoffman MD, et al. Underspecification presents challenges for credibility in modern machine learning; 2020. arXiv preprint arXiv:2011.03395 .
Chea P, Mandell JC. Current applications and future directions of deep learning in musculoskeletal radiology. Skelet Radiol. 2020;49(2):1–15.
Wu X, Sahoo D, Hoi SC. Recent advances in deep learning for object detection. Neurocomputing. 2020;396:39–64.
Kuutti S, Bowden R, Jin Y, Barber P, Fallah S. A survey of deep learning applications to autonomous vehicle control. IEEE Trans Intell Transp Syst. 2020;22:712–33.
Yolcu G, Oztel I, Kazan S, Oz C, Bunyak F. Deep learning-based face analysis system for monitoring customer interest. J Ambient Intell Humaniz Comput. 2020;11(1):237–48.
Jiao L, Zhang F, Liu F, Yang S, Li L, Feng Z, Qu R. A survey of deep learning-based object detection. IEEE Access. 2019;7:128837–68.
Muhammad K, Khan S, Del Ser J, de Albuquerque VHC. Deep learning for multigrade brain tumor classification in smart healthcare systems: a prospective survey. IEEE Trans Neural Netw Learn Syst. 2020;32:507–22.
Litjens G, Kooi T, Bejnordi BE, Setio AAA, Ciompi F, Ghafoorian M, Van Der Laak JA, Van Ginneken B, Sánchez CI. A survey on deep learning in medical image analysis. Med Image Anal. 2017;42:60–88.
Mukherjee D, Mondal R, Singh PK, Sarkar R, Bhattacharjee D. Ensemconvnet: a deep learning approach for human activity recognition using smartphone sensors for healthcare applications. Multimed Tools Appl. 2020;79(41):31663–90.
Zeleznik R, Foldyna B, Eslami P, Weiss J, Alexander I, Taron J, Parmar C, Alvi RM, Banerji D, Uno M, et al. Deep convolutional neural networks to predict cardiovascular risk from computed tomography. Nature Commun. 2021;12(1):1–9.
Wang J, Liu Q, Xie H, Yang Z, Zhou H. Boosted efficientnet: detection of lymph node metastases in breast cancer using convolutional neural networks. Cancers. 2021;13(4):661.
Yu H, Yang LT, Zhang Q, Armstrong D, Deen MJ. Convolutional neural networks for medical image analysis: state-of-the-art, comparisons, improvement and perspectives. Neurocomputing. 2021. https://doi.org/10.1016/j.neucom.2020.04.157 .
Bharati S, Podder P, Mondal MRH. Hybrid deep learning for detecting lung diseases from X-ray images. Inform Med Unlocked. 2020;20:100391.
Dong Y, Pan Y, Zhang J, Xu W. Learning to read chest X-ray images from 16000+ examples using CNN. In: 2017 IEEE/ACM international conference on connected health: applications, systems and engineering technologies (CHASE). IEEE; 2017. p. 51–7.
Rajkomar A, Lingam S, Taylor AG, Blum M, Mongan J. High-throughput classification of radiographs using deep convolutional neural networks. J Digit Imaging. 2017;30(1):95–101.
Rajpurkar P, Irvin J, Zhu K, Yang B, Mehta H, Duan T, Ding D, Bagul A, Langlotz C, Shpanskaya K, et al. Chexnet: radiologist-level pneumonia detection on chest X-rays with deep learning; 2017. arXiv preprint arXiv:1711.05225 .
Wang X, Peng Y, Lu L, Lu Z, Bagheri M, Summers RM. ChestX-ray8: Hospital-scale chest X-ray database and benchmarks on weakly-supervised classification and localization of common thorax diseases. In: Proceedings of the IEEE conference on computer vision and pattern recognition; 2017. p. 2097–106.
Zuo W, Zhou F, Li Z, Wang L. Multi-resolution CNN and knowledge transfer for candidate classification in lung nodule detection. IEEE Access. 2019;7:32510–21.
Shen W, Zhou M, Yang F, Yang C, Tian J. Multi-scale convolutional neural networks for lung nodule classification. In: International conference on information processing in medical imaging. Springer; 2015. p. 588–99.
Li R, Zhang W, Suk HI, Wang L, Li J, Shen D, Ji S. Deep learning based imaging data completion for improved brain disease diagnosis. In: International conference on medical image computing and computer-assisted intervention. Springer; 2014. p. 305–12.
Wen J, Thibeau-Sutre E, Diaz-Melo M, Samper-González J, Routier A, Bottani S, Dormont D, Durrleman S, Burgos N, Colliot O, et al. Convolutional neural networks for classification of Alzheimer’s disease: overview and reproducible evaluation. Med Image Anal. 2020;63:101694.
Mehmood A, Maqsood M, Bashir M, Shuyuan Y. A deep siamese convolution neural network for multi-class classification of Alzheimer disease. Brain Sci. 2020;10(2):84.
Hosseini-Asl E, Ghazal M, Mahmoud A, Aslantas A, Shalaby A, Casanova M, Barnes G, Gimel’farb G, Keynton R, El-Baz A. Alzheimer’s disease diagnostics by a 3d deeply supervised adaptable convolutional network. Front Biosci. 2018;23:584–96.
Korolev S, Safiullin A, Belyaev M, Dodonova Y. Residual and plain convolutional neural networks for 3D brain MRI classification. In: 2017 IEEE 14th international symposium on biomedical imaging (ISBI 2017). IEEE; 2017. p. 835–8.
Alzubaidi L, Fadhel MA, Oleiwi SR, Al-Shamma O, Zhang J. DFU_QUTNet: diabetic foot ulcer classification using novel deep convolutional neural network. Multimed Tools Appl. 2020;79(21):15655–77.
Goyal M, Reeves ND, Davison AK, Rajbhandari S, Spragg J, Yap MH. Dfunet: convolutional neural networks for diabetic foot ulcer classification. IEEE Trans Emerg Topics Comput Intell. 2018;4(5):728–39.
Yap MH., Hachiuma R, Alavi A, Brungel R, Goyal M, Zhu H, Cassidy B, Ruckert J, Olshansky M, Huang X, et al. Deep learning in diabetic foot ulcers detection: a comprehensive evaluation; 2020. arXiv preprint arXiv:2010.03341 .
Tulloch J, Zamani R, Akrami M. Machine learning in the prevention, diagnosis and management of diabetic foot ulcers: a systematic review. IEEE Access. 2020;8:198977–9000.
Fadhel MA, Al-Shamma O, Alzubaidi L, Oleiwi SR. Real-time sickle cell anemia diagnosis based hardware accelerator. In: International conference on new trends in information and communications technology applications, Springer; 2020. p. 189–99.
Debelee TG, Kebede SR, Schwenker F, Shewarega ZM. Deep learning in selected cancers’ image analysis—a survey. J Imaging. 2020;6(11):121.
Khan S, Islam N, Jan Z, Din IU, Rodrigues JJC. A novel deep learning based framework for the detection and classification of breast cancer using transfer learning. Pattern Recogn Lett. 2019;125:1–6.
Alzubaidi L, Hasan RI, Awad FH, Fadhel MA, Alshamma O, Zhang J. Multi-class breast cancer classification by a novel two-branch deep convolutional neural network architecture. In: 2019 12th international conference on developments in eSystems engineering (DeSE). IEEE; 2019. p. 268–73.
Roy K, Banik D, Bhattacharjee D, Nasipuri M. Patch-based system for classification of breast histology images using deep learning. Comput Med Imaging Gr. 2019;71:90–103.
Hameed Z, Zahia S, Garcia-Zapirain B, Javier Aguirre J, María Vanegas A. Breast cancer histopathology image classification using an ensemble of deep learning models. Sensors. 2020;20(16):4373.
Hosny KM, Kassem MA, Foaud MM. Skin cancer classification using deep learning and transfer learning. In: 2018 9th Cairo international biomedical engineering conference (CIBEC). IEEE; 2018. p. 90–3.
Dorj UO, Lee KK, Choi JY, Lee M. The skin cancer classification using deep convolutional neural network. Multimed Tools Appl. 2018;77(8):9909–24.
Kassem MA, Hosny KM, Fouad MM. Skin lesions classification into eight classes for ISIC 2019 using deep convolutional neural network and transfer learning. IEEE Access. 2020;8:114822–32.
Heidari M, Mirniaharikandehei S, Khuzani AZ, Danala G, Qiu Y, Zheng B. Improving the performance of CNN to predict the likelihood of COVID-19 using chest X-ray images with preprocessing algorithms. Int J Med Inform. 2020;144:104284.
Al-Timemy AH, Khushaba RN, Mosa ZM, Escudero J. An efficient mixture of deep and machine learning models for COVID-19 and tuberculosis detection using X-ray images in resource limited settings 2020. arXiv preprint arXiv:2007.08223 .
Abraham B, Nair MS. Computer-aided detection of COVID-19 from X-ray images using multi-CNN and Bayesnet classifier. Biocybern Biomed Eng. 2020;40(4):1436–45.
Nour M, Cömert Z, Polat K. A novel medical diagnosis model for COVID-19 infection detection based on deep features and Bayesian optimization. Appl Soft Comput. 2020;97:106580.
Mallio CA, Napolitano A, Castiello G, Giordano FM, D’Alessio P, Iozzino M, Sun Y, Angeletti S, Russano M, Santini D, et al. Deep learning algorithm trained with COVID-19 pneumonia also identifies immune checkpoint inhibitor therapy-related pneumonitis. Cancers. 2021;13(4):652.
Fourcade A, Khonsari R. Deep learning in medical image analysis: a third eye for doctors. J Stomatol Oral Maxillofac Surg. 2019;120(4):279–88.
Guo Z, Li X, Huang H, Guo N, Li Q. Deep learning-based image segmentation on multimodal medical imaging. IEEE Trans Radiat Plasma Med Sci. 2019;3(2):162–9.
Thakur N, Yoon H, Chong Y. Current trends of artificial intelligence for colorectal cancer pathology image analysis: a systematic review. Cancers. 2020;12(7):1884.
Lundervold AS, Lundervold A. An overview of deep learning in medical imaging focusing on MRI. Zeitschrift für Medizinische Physik. 2019;29(2):102–27.
Yadav SS, Jadhav SM. Deep convolutional neural network based medical image classification for disease diagnosis. J Big Data. 2019;6(1):113.
Nehme E, Freedman D, Gordon R, Ferdman B, Weiss LE, Alalouf O, Naor T, Orange R, Michaeli T, Shechtman Y. DeepSTORM3D: dense 3D localization microscopy and PSF design by deep learning. Nat Methods. 2020;17(7):734–40.
Zulkifley MA, Abdani SR, Zulkifley NH. Pterygium-Net: a deep learning approach to pterygium detection and localization. Multimed Tools Appl. 2019;78(24):34563–84.
Sirazitdinov I, Kholiavchenko M, Mustafaev T, Yixuan Y, Kuleev R, Ibragimov B. Deep neural network ensemble for pneumonia localization from a large-scale chest X-ray database. Comput Electr Eng. 2019;78:388–99.
Zhao W, Shen L, Han B, Yang Y, Cheng K, Toesca DA, Koong AC, Chang DT, Xing L. Markerless pancreatic tumor target localization enabled by deep learning. Int J Radiat Oncol Biol Phys. 2019;105(2):432–9.
Roth HR, Lee CT, Shin HC, Seff A, Kim L, Yao J, Lu L, Summers RM. Anatomy-specific classification of medical images using deep convolutional nets. In: 2015 IEEE 12th international symposium on biomedical imaging (ISBI). IEEE; 2015. p. 101–4.
Shin HC, Orton MR, Collins DJ, Doran SJ, Leach MO. Stacked autoencoders for unsupervised feature learning and multiple organ detection in a pilot study using 4D patient data. IEEE Trans Pattern Anal Mach Intell. 2012;35(8):1930–43.
Li Z, Dong M, Wen S, Hu X, Zhou P, Zeng Z. CLU-CNNs: object detection for medical images. Neurocomputing. 2019;350:53–9.
Gao J, Jiang Q, Zhou B, Chen D. Convolutional neural networks for computer-aided detection or diagnosis in medical image analysis: an overview. Math Biosci Eng. 2019;16(6):6536.
Article MathSciNet Google Scholar
Lumini A, Nanni L. Review fair comparison of skin detection approaches on publicly available datasets. Expert Syst Appl. 2020. https://doi.org/10.1016/j.eswa.2020.113677 .
Chouhan V, Singh SK, Khamparia A, Gupta D, Tiwari P, Moreira C, Damaševičius R, De Albuquerque VHC. A novel transfer learning based approach for pneumonia detection in chest X-ray images. Appl Sci. 2020;10(2):559.
Apostolopoulos ID, Mpesiana TA. COVID-19: automatic detection from X-ray images utilizing transfer learning with convolutional neural networks. Phys Eng Sci Med. 2020;43(2):635–40.
Mahmud T, Rahman MA, Fattah SA. CovXNet: a multi-dilation convolutional neural network for automatic COVID-19 and other pneumonia detection from chest X-ray images with transferable multi-receptive feature optimization. Comput Biol Med. 2020;122:103869.
Tayarani-N MH. Applications of artificial intelligence in battling against COVID-19: a literature review. Chaos Solitons Fractals. 2020;142:110338.
Toraman S, Alakus TB, Turkoglu I. Convolutional capsnet: a novel artificial neural network approach to detect COVID-19 disease from X-ray images using capsule networks. Chaos Solitons Fractals. 2020;140:110122.
Dascalu A, David E. Skin cancer detection by deep learning and sound analysis algorithms: a prospective clinical study of an elementary dermoscope. EBioMedicine. 2019;43:107–13.
Adegun A, Viriri S. Deep learning techniques for skin lesion analysis and melanoma cancer detection: a survey of state-of-the-art. Artif Intell Rev. 2020;54:1–31.
Zhang N, Cai YX, Wang YY, Tian YT, Wang XL, Badami B. Skin cancer diagnosis based on optimized convolutional neural network. Artif Intell Med. 2020;102:101756.
Thurnhofer-Hemsi K, Domínguez E. A convolutional neural network framework for accurate skin cancer detection. Neural Process Lett. 2020. https://doi.org/10.1007/s11063-020-10364-y .
Jain MS, Massoud TF. Predicting tumour mutational burden from histopathological images using multiscale deep learning. Nat Mach Intell. 2020;2(6):356–62.
Lei H, Liu S, Elazab A, Lei B. Attention-guided multi-branch convolutional neural network for mitosis detection from histopathological images. IEEE J Biomed Health Inform. 2020;25(2):358–70.
Celik Y, Talo M, Yildirim O, Karabatak M, Acharya UR. Automated invasive ductal carcinoma detection based using deep transfer learning with whole-slide images. Pattern Recogn Lett. 2020;133:232–9.
Sebai M, Wang X, Wang T. Maskmitosis: a deep learning framework for fully supervised, weakly supervised, and unsupervised mitosis detection in histopathology images. Med Biol Eng Comput. 2020;58:1603–23.
Sebai M, Wang T, Al-Fadhli SA. Partmitosis: a partially supervised deep learning framework for mitosis detection in breast cancer histopathology images. IEEE Access. 2020;8:45133–47.
Mahmood T, Arsalan M, Owais M, Lee MB, Park KR. Artificial intelligence-based mitosis detection in breast cancer histopathology images using faster R-CNN and deep CNNs. J Clin Med. 2020;9(3):749.
Srinidhi CL, Ciga O, Martel AL. Deep neural network models for computational histopathology: a survey. Med Image Anal. 2020;67:101813.
Cireşan DC, Giusti A, Gambardella LM, Schmidhuber J. Mitosis detection in breast cancer histology images with deep neural networks. In: International conference on medical image computing and computer-assisted intervention. Springer; 2013. p. 411–8.
Sirinukunwattana K, Raza SEA, Tsang YW, Snead DR, Cree IA, Rajpoot NM. Locality sensitive deep learning for detection and classification of nuclei in routine colon cancer histology images. IEEE Trans Med Imaging. 2016;35(5):1196–206.
Xu J, Xiang L, Liu Q, Gilmore H, Wu J, Tang J, Madabhushi A. Stacked sparse autoencoder (SSAE) for nuclei detection on breast cancer histopathology images. IEEE Trans Med Imaging. 2015;35(1):119–30.
Albarqouni S, Baur C, Achilles F, Belagiannis V, Demirci S, Navab N. Aggnet: deep learning from crowds for mitosis detection in breast cancer histology images. IEEE Trans Med Imaging. 2016;35(5):1313–21.
Abd-Ellah MK, Awad AI, Khalaf AA, Hamed HF. Two-phase multi-model automatic brain tumour diagnosis system from magnetic resonance images using convolutional neural networks. EURASIP J Image Video Process. 2018;2018(1):97.
Thaha MM, Kumar KPM, Murugan B, Dhanasekeran S, Vijayakarthick P, Selvi AS. Brain tumor segmentation using convolutional neural networks in MRI images. J Med Syst. 2019;43(9):294.
Talo M, Yildirim O, Baloglu UB, Aydin G, Acharya UR. Convolutional neural networks for multi-class brain disease detection using MRI images. Comput Med Imaging Gr. 2019;78:101673.
Gabr RE, Coronado I, Robinson M, Sujit SJ, Datta S, Sun X, Allen WJ, Lublin FD, Wolinsky JS, Narayana PA. Brain and lesion segmentation in multiple sclerosis using fully convolutional neural networks: a large-scale study. Mult Scler J. 2020;26(10):1217–26.
Chen S, Ding C, Liu M. Dual-force convolutional neural networks for accurate brain tumor segmentation. Pattern Recogn. 2019;88:90–100.
Hu K, Gan Q, Zhang Y, Deng S, Xiao F, Huang W, Cao C, Gao X. Brain tumor segmentation using multi-cascaded convolutional neural networks and conditional random field. IEEE Access. 2019;7:92615–29.
Wadhwa A, Bhardwaj A, Verma VS. A review on brain tumor segmentation of MRI images. Magn Reson Imaging. 2019;61:247–59.
Akkus Z, Galimzianova A, Hoogi A, Rubin DL, Erickson BJ. Deep learning for brain MRI segmentation: state of the art and future directions. J Digit Imaging. 2017;30(4):449–59.
Moeskops P, Viergever MA, Mendrik AM, De Vries LS, Benders MJ, Išgum I. Automatic segmentation of MR brain images with a convolutional neural network. IEEE Trans Med Imaging. 2016;35(5):1252–61.
Milletari F, Navab N, Ahmadi SA. V-net: Fully convolutional neural networks for volumetric medical image segmentation. In: 2016 fourth international conference on 3D vision (3DV). IEEE; 2016. p. 565–71.
Ronneberger O, Fischer P, Brox T. U-net: Convolutional networks for biomedical image segmentation. In: International conference on medical image computing and computer-assisted intervention. Springer; 2015. p. 234–41.
Pereira S, Pinto A, Alves V, Silva CA. Brain tumor segmentation using convolutional neural networks in MRI images. IEEE Trans Med Imaging. 2016;35(5):1240–51.
Havaei M, Davy A, Warde-Farley D, Biard A, Courville A, Bengio Y, Pal C, Jodoin PM, Larochelle H. Brain tumor segmentation with deep neural networks. Med Image Anal. 2017;35:18–31.
Chen LC, Papandreou G, Kokkinos I, Murphy K, Yuille AL. DeepLab: semantic image segmentation with deep convolutional nets, atrous convolution, and fully connected CRFs. IEEE Trans Pattern Anal Mach Intell. 2017;40(4):834–48.
Yan Q, Wang B, Gong D, Luo C, Zhao W, Shen J, Shi Q, Jin S, Zhang L, You Z. COVID-19 chest CT image segmentation—a deep convolutional neural network solution; 2020. arXiv preprint arXiv:2004.10987 .
Wang G, Liu X, Li C, Xu Z, Ruan J, Zhu H, Meng T, Li K, Huang N, Zhang S. A noise-robust framework for automatic segmentation of COVID-19 pneumonia lesions from CT images. IEEE Trans Med Imaging. 2020;39(8):2653–63.
Khan SH, Sohail A, Khan A, Lee YS. Classification and region analysis of COVID-19 infection using lung CT images and deep convolutional neural networks; 2020. arXiv preprint arXiv:2009.08864 .
Shi F, Wang J, Shi J, Wu Z, Wang Q, Tang Z, He K, Shi Y, Shen D. Review of artificial intelligence techniques in imaging data acquisition, segmentation and diagnosis for COVID-19. IEEE Rev Biomed Eng. 2020;14:4–5.
Santamaría J, Rivero-Cejudo M, Martos-Fernández M, Roca F. An overview on the latest nature-inspired and metaheuristics-based image registration algorithms. Appl Sci. 2020;10(6):1928.
Santamaría J, Cordón O, Damas S. A comparative study of state-of-the-art evolutionary image registration methods for 3D modeling. Comput Vision Image Underst. 2011;115(9):1340–54.
Yumer ME, Mitra NJ. Learning semantic deformation flows with 3D convolutional networks. In: European conference on computer vision. Springer; 2016. p. 294–311.
Ding L, Feng C. Deepmapping: unsupervised map estimation from multiple point clouds. In: Proceedings of the IEEE conference on computer vision and pattern recognition; 2019. p. 8650–9.
Mahadevan S. Imagination machines: a new challenge for artificial intelligence. AAAI. 2018;2018:7988–93.
Wang L, Fang Y. Unsupervised 3D reconstruction from a single image via adversarial learning; 2017. arXiv preprint arXiv:1711.09312 .
Hermoza R, Sipiran I. 3D reconstruction of incomplete archaeological objects using a generative adversarial network. In: Proceedings of computer graphics international 2018. Association for Computing Machinery; 2018. p. 5–11.
Fu Y, Lei Y, Wang T, Curran WJ, Liu T, Yang X. Deep learning in medical image registration: a review. Phys Med Biol. 2020;65(20):20TR01.
Haskins G, Kruger U, Yan P. Deep learning in medical image registration: a survey. Mach Vision Appl. 2020;31(1):8.
de Vos BD, Berendsen FF, Viergever MA, Sokooti H, Staring M, Išgum I. A deep learning framework for unsupervised affine and deformable image registration. Med Image Anal. 2019;52:128–43.
Yang X, Kwitt R, Styner M, Niethammer M. Quicksilver: fast predictive image registration—a deep learning approach. NeuroImage. 2017;158:378–96.
Miao S, Wang ZJ, Liao R. A CNN regression approach for real-time 2D/3D registration. IEEE Trans Med Imaging. 2016;35(5):1352–63.
Li P, Pei Y, Guo Y, Ma G, Xu T, Zha H. Non-rigid 2D–3D registration using convolutional autoencoders. In: 2020 IEEE 17th international symposium on biomedical imaging (ISBI). IEEE; 2020. p. 700–4.
Zhang J, Yeung SH, Shu Y, He B, Wang W. Efficient memory management for GPU-based deep learning systems; 2019. arXiv preprint arXiv:1903.06631 .
Zhao H, Han Z, Yang Z, Zhang Q, Yang F, Zhou L, Yang M, Lau FC, Wang Y, Xiong Y, et al. Hived: sharing a {GPU} cluster for deep learning with guarantees. In: 14th {USENIX} symposium on operating systems design and implementation ({OSDI} 20); 2020. p. 515–32.
Lin Y, Jiang Z, Gu J, Li W, Dhar S, Ren H, Khailany B, Pan DZ. DREAMPlace: deep learning toolkit-enabled GPU acceleration for modern VLSI placement. IEEE Trans Comput Aided Des Integr Circuits Syst. 2020;40:748–61.
Hossain S, Lee DJ. Deep learning-based real-time multiple-object detection and tracking from aerial imagery via a flying robot with GPU-based embedded devices. Sensors. 2019;19(15):3371.
Castro FM, Guil N, Marín-Jiménez MJ, Pérez-Serrano J, Ujaldón M. Energy-based tuning of convolutional neural networks on multi-GPUs. Concurr Comput Pract Exp. 2019;31(21):4786.
Gschwend D. Zynqnet: an fpga-accelerated embedded convolutional neural network; 2020. arXiv preprint arXiv:2005.06892 .
Zhang N, Wei X, Chen H, Liu W. FPGA implementation for CNN-based optical remote sensing object detection. Electronics. 2021;10(3):282.
Zhao M, Hu C, Wei F, Wang K, Wang C, Jiang Y. Real-time underwater image recognition with FPGA embedded system for convolutional neural network. Sensors. 2019;19(2):350.
Liu X, Yang J, Zou C, Chen Q, Yan X, Chen Y, Cai C. Collaborative edge computing with FPGA-based CNN accelerators for energy-efficient and time-aware face tracking system. IEEE Trans Comput Soc Syst. 2021. https://doi.org/10.1109/TCSS.2021.3059318 .
Hossin M, Sulaiman M. A review on evaluation metrics for data classification evaluations. Int J Data Min Knowl Manag Process. 2015;5(2):1.
Provost F, Domingos P. Tree induction for probability-based ranking. Mach Learn. 2003;52(3):199–215.
Rakotomamonyj A. Optimizing area under roc with SVMS. In: Proceedings of the European conference on artificial intelligence workshop on ROC curve and artificial intelligence (ROCAI 2004), 2004. p. 71–80.
Mingote V, Miguel A, Ortega A, Lleida E. Optimization of the area under the roc curve using neural network supervectors for text-dependent speaker verification. Comput Speech Lang. 2020;63:101078.
Fawcett T. An introduction to roc analysis. Pattern Recogn Lett. 2006;27(8):861–74.
Huang J, Ling CX. Using AUC and accuracy in evaluating learning algorithms. IEEE Trans Knowl Data Eng. 2005;17(3):299–310.
Hand DJ, Till RJ. A simple generalisation of the area under the ROC curve for multiple class classification problems. Mach Learn. 2001;45(2):171–86.
Masoudnia S, Mersa O, Araabi BN, Vahabie AH, Sadeghi MA, Ahmadabadi MN. Multi-representational learning for offline signature verification using multi-loss snapshot ensemble of CNNs. Expert Syst Appl. 2019;133:317–30.
Coupé P, Mansencal B, Clément M, Giraud R, de Senneville BD, Ta VT, Lepetit V, Manjon JV. Assemblynet: a large ensemble of CNNs for 3D whole brain MRI segmentation. NeuroImage. 2020;219:117026.
Download references
Acknowledgements
We would like to thank the professors from the Queensland University of Technology and the University of Information Technology and Communications who gave their feedback on the paper.
This research received no external funding.
Author information
Authors and affiliations.
School of Computer Science, Queensland University of Technology, Brisbane, QLD, 4000, Australia
Laith Alzubaidi & Jinglan Zhang
Control and Systems Engineering Department, University of Technology, Baghdad, 10001, Iraq
Amjad J. Humaidi
Electrical Engineering Technical College, Middle Technical University, Baghdad, 10001, Iraq
Ayad Al-Dujaili
Faculty of Electrical Engineering & Computer Science, University of Missouri, Columbia, MO, 65211, USA
Ye Duan & Muthana Al-Amidie
AlNidhal Campus, University of Information Technology & Communications, Baghdad, 10001, Iraq
Laith Alzubaidi & Omran Al-Shamma
Department of Computer Science, University of Jaén, 23071, Jaén, Spain
J. Santamaría
College of Computer Science and Information Technology, University of Sumer, Thi Qar, 64005, Iraq
Mohammed A. Fadhel
School of Engineering, Manchester Metropolitan University, Manchester, M1 5GD, UK
Laith Farhan
You can also search for this author in PubMed Google Scholar
Contributions
Conceptualization: LA, and JZ; methodology: LA, JZ, and JS; software: LA, and MAF; validation: LA, JZ, MA, and LF; formal analysis: LA, JZ, YD, and JS; investigation: LA, and JZ; resources: LA, JZ, and MAF; data curation: LA, and OA.; writing–original draft preparation: LA, and OA; writing—review and editing: LA, JZ, AJH, AA, YD, OA, JS, MAF, MA, and LF; visualization: LA, and MAF; supervision: JZ, and YD; project administration: JZ, YD, and JS; funding acquisition: LA, AJH, AA, and YD. All authors read and approved the final manuscript.
Corresponding author
Correspondence to Laith Alzubaidi .
Ethics declarations
Ethics approval and consent to participate, consent for publication, competing interests.
The authors declare that they have no competing interests.
Additional information
Publisher's note.
Springer Nature remains neutral with regard to jurisdictional claims in published maps and institutional affiliations.
Rights and permissions
Open Access This article is licensed under a Creative Commons Attribution 4.0 International License, which permits use, sharing, adaptation, distribution and reproduction in any medium or format, as long as you give appropriate credit to the original author(s) and the source, provide a link to the Creative Commons licence, and indicate if changes were made. The images or other third party material in this article are included in the article's Creative Commons licence, unless indicated otherwise in a credit line to the material. If material is not included in the article's Creative Commons licence and your intended use is not permitted by statutory regulation or exceeds the permitted use, you will need to obtain permission directly from the copyright holder. To view a copy of this licence, visit http://creativecommons.org/licenses/by/4.0/ .
Reprints and permissions
About this article
Cite this article.
Alzubaidi, L., Zhang, J., Humaidi, A.J. et al. Review of deep learning: concepts, CNN architectures, challenges, applications, future directions. J Big Data 8 , 53 (2021). https://doi.org/10.1186/s40537-021-00444-8
Download citation
Received : 21 January 2021
Accepted : 22 March 2021
Published : 31 March 2021
DOI : https://doi.org/10.1186/s40537-021-00444-8
Share this article
Anyone you share the following link with will be able to read this content:
Sorry, a shareable link is not currently available for this article.
Provided by the Springer Nature SharedIt content-sharing initiative
- Deep learning
- Machine learning
- Convolution neural network (CNN)
- Deep neural network architectures
- Deep learning applications
- Image classification
- Medical image analysis
- Supervised learning
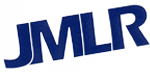
Frequently Asked Questions
Retrospectives from 20 Years of JMLR
Fabian Pedregosa , Tegan Maharaj , Alp Kucukelbir , Rajarshi Das , Valentina Borghesani , Francis Bach , David Blei , Bernhard Schölkopf 21 February 2022
In 2000, led by editor-in-chief Leslie Kaelbling, JMLR was founded as a fully free and open-access platform for publishing high-quality machine learning research. Twenty-one years later, JMLR publishes more than 250 papers per year and is one of the premiere publishing venues in the field of artificial intelligence. How did a community-driven journal, without any financial or managerial support from traditional publishing companies, become a leading journal in the field? Celebrating more than 20 years of history, we take a look back at the story of JMLR and the lessons that can be learnt from it.
Outline: 1. How JMLR works 2. Papers, decisions and publication time 3. The Human Cost of Sustaining a Growing Field 4. Mirroring trends and biases of the field 5. Outlook 6. Credits
1. How JMLR works
In summary . JMLR runs almost entirely on volunteer labor. [1] The JMLR team consists of three Editors-in-Chief (EiCs), two Managing Editors, an Editorial Assistant, a Production Editor, and a Webmaster, along with the Advisory Board and a large group of currently 133 of Action Editors (AEs). The AEs are senior researchers in the field (typically tenured or equivalent), recruited by invitation, and it's by relying on their expertise that JMLR can have such a small and agile management team. Expert AEs decentralize much of the editorial work that is typically centralized in EiCs in other journals – EiCs assign each paper to an AE, and from there on the AE takes responsibility for finding reviewers and making the final decision.
In detail . Authors upload submission to JMLR's own submission system , hosted at MIT (see section 3 for a precise cost estimate). This system was initially written by Christian Shelton in 2003. It has served us remarkably well, as the system continues to be in use today with minor improvements by subsequent managing editors. It is written in Perl and Python, and uses a PostgreSQL database.
Each manuscript is scanned by an Editor-in-Chief. If the Editor-in-Chief finds the paper out of scope, they will desk-reject the paper, otherwise they will assign the paper to an action editor (AE) who has expertise in the area of the paper. The AE decides whether to send the paper for a full review.
If the paper goes out for review, the AE solicits 2-3 expert reviewers to write detailed technical reviews of the paper. After review, the AE can then accept the paper as is, reject the paper, ask for minor revisions, or propose "reject with encouragement to resubmit", i.e. ask for major revisions.
If the paper is accepted, the production editor prepares the camera-ready version that is then uploaded to the website . Papers are accepted on a rolling basis and uploaded as they are processed, grouped into one Volume per year. The managing editors support all users of the submission system (authors, reviewers, and the editorial board) throughout the reviewing process; the webmaster takes care of uploading the final documents and is responsible for the maintenance of the public webpage.
Besides the main publication track, JMLR also has a track for open source software contributions called Machine Learning Open Source Software (MLOSS) . These papers have dedicated action editors and a page limit of 4 pages, while main track JMLR papers don't have page limit. Reviewers of MLOSS papers are also asked to follow a different set of reviewing criteria. JMLR has also hosted special issues for topics of timely relevance. In recent years, JMLR has also become an umbrella for different publications that we won't cover in this blog post, such as the Proceedings of Machine Learning Research (PMLR) and the recently-announced Transactions on Machine Learning Research (TMLR), which will start accepting submissions in March 2022.
2. Papers, decisions and publication time
The number of papers submitted and accepted has been steadily increasing throughout the years: from 10 papers published in 2002 to 290 papers published in 2021
Below we show the number of unique submissions (that is, excluding resubmissions) since 2003. [2] Color indicates the final decision of the paper:
- desk reject , for papers rejected by the action editor or editor-in-chief without sending the paper for review.
- reject , for papers whose final decision after review is either reject or reject with encouragement to resubmit (but whose authors decided to not resubmit), and
- accept , for papers that will be published on the website.
This plot shows the dramatic growth experienced in recent years. While it took 8 years for the number of submissions to double in size from 2004 to 2012, it only took 2 years from 2018 to 2020 for these to double again.
Acceptance rate . JMLR's only criteria for acceptance is quality, i.e., it does not enforce an annual acceptance rate. The action editor is responsible for deciding whether the paper is up to the standard of the journal. The following figure shows the yearly evolution of the different editorial decisions (desk reject, reject, accept).
Figure 2: For each year, we show the percentage of submissions that received an editorial decision of desk reject vs. reject vs. accept.
The percentage of accepted papers has been steadily declining since the 33% acceptance rate of 2007 to the current 17% acceptance. The trend can be explained by a constant strive for quality in face of an increasing number of submissions.
Time to receive the first round of reviews . Papers that are sent to reviewers can take a variable amount of time to come back to authors. Below we plot the median, 25-75 and 10-90 percentile for the number of days it took for authors to receive the first round of reviews (hence desk rejected papers are excluded).
Figure 3 . Days from submission to decision for papers that are sent to reviewers. The dark line shows median, while lighter intervals represent 25-75 and 10-10 percentile respectively.
This median delay has unfortunately been steadily increasing throughout the years, reaching 187 days in 2021. It's a priority for us to reduce the delay times without sacrificing the quality of the review process. The section below explores the cost of such an endeavor.
3. The Human Cost of Sustaining a Growing Field
The storage and bandwidth needs of the journal, although increasing throughout the years, remain negligible. The full jmlr.org website, including non-public under-review papers, backups, and a PostgreSQL database, occupies 49GB. Currently, MIT provides this storage for free, but it would have a cost of around $100 per month using a standard Cloud Platform.
The most precious resource is the human workforce. To ensure that published papers are technically sound and of the highest quality, JMLR relies on a group of experts all volunteers. In 2021, JMLR counted 1938 active reviewers (93% of the workforce), [3] 133 action editors (6%), and 8 members of the editorial board (0.3%), which includes the Editors-in-Chief, and technical time-consuming roles such as webmaster, production editor and managing editor.
To handle the increasing workload, the number of action editors and reviewers has been steadily increasing through the years. The figure below shows the number of Submissions, Action editors and Reviewers.
These figures highlight the increased labor that the action editors have taken in recent years: in 2010 the average number of submissions per action editor was 4.5, while in 2020 it was more than double, 11.7.
4. Mirroring trends and biases of the field
According to recent estimates, [4] only 12% of leading machine learning researchers are female and only 22% of jobs in artificial intelligence are held by them, with even fewer holding senior roles.
We sought to understand the representation of female scientists in the journal. Unfortunately, JMLR does not track gender information, so we appealed to the indirect means of inferring gender from first names, which only provides a rough estimate. We inferred gender for both action editors and corresponding authors. Below we plot the gender proportion of female AEs and authors. We only plot this data since 2012, which is when the number of AEs surpassed 100 members:
Figure 5: Percentage of female action editors and corresponding authors.
The years 2013-2016 are characterized by an exceptionally low number of female AEs, below 10%. This number has been increasingly steady throughout the last few years, reaching 17% in 2021. Although this is an improvement on previous years, we're far from the gender balance that we strive to achieve. This is an aspect we're committed to improving.
When it was founded in 2002, the first editors of JMLR sought to create an independent and open-access journal, with the most minimal operating costs. This was a radical and visionary experiment, and it was a success. Today, JMLR is a top journal in AI and ML, while remaining free, open, and community-driven.
Since 2002, the fields of AI and ML have grown and thrived, and JMLR has grown along with them. Of course, the increase in submissions means increased demands on all the volunteers’ time and energy. JMLR is indebted to the immense efforts of the leadership team, the action editors, and the many reviewers. Thank you for making JMLR such a great journal and keeping JMLR free.
Citing. Please consider citing this article as
Fabian Pedregosa, Tegan Maharaj, Alp Kucukelbir, Rajarshi Das, Valentina Borghesani, Francis Bach, David Blei, Bernhard Schölkopf, "Retrospectives from 20 Years of JMLR", https://www.jmlr.org/news/20_years.html
BibTex entry: @misc{pedregosa2022retrospectives, title={Retrospectives from 20 Years of JMLR}, author={Pedregosa, Fabian and Maharaj, Tegan and Kucukelbir, Alp and Das, Rajarshi and Borghesani, Valentina and Bach, Francis and Blei,David and Sch{\"o}lkopf, Bernhard}, url={https://jmlr.org/news/2022/retrospectives.html}, year={2022} }
Acknowledgements . We would like to thank Leslie Pack Kaelbling, Lawrence Saul and Barbara Engelhardt for providing feedback on this blog post.
[1] The only paid job at JMLR is that of the part-time editorial assistant, who provides email support to users of the journal and ensures the manuscripts flow smoothly through the submission and review system.
[2] Although the journal was established in the year 2000, the current submission system was created in 2003 thus prior statistics are not available.
[3] We count active reviewers as those that have performed at least one review during the year 2021.
[4] Bridging The Gender Gap In AI , Forbes magazine.
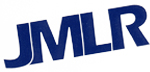
Frequently Asked Questions
JMLR Volume 23
Joint Estimation and Inference for Data Integration Problems based on Multiple Multi-layered Gaussian Graphical Models Subhabrata Majumdar, George Michailidis ; (1):1−53, 2022. [ abs ][ pdf ][ bib ] [ code ]
Debiased Distributed Learning for Sparse Partial Linear Models in High Dimensions Shaogao Lv, Heng Lian ; (2):1−32, 2022. [ abs ][ pdf ][ bib ]
Recovering shared structure from multiple networks with unknown edge distributions Keith Levin, Asad Lodhia, Elizaveta Levina ; (3):1−48, 2022. [ abs ][ pdf ][ bib ]
Exploiting locality in high-dimensional Factorial hidden Markov models Lorenzo Rimella, Nick Whiteley ; (4):1−34, 2022. [ abs ][ pdf ][ bib ] [ code ]
Empirical Risk Minimization under Random Censorship Guillaume Ausset, Stephan Clémençon, François Portier ; (5):1−59, 2022. [ abs ][ pdf ][ bib ]
XAI Beyond Classification: Interpretable Neural Clustering Xi Peng, Yunfan Li, Ivor W. Tsang, Hongyuan Zhu, Jiancheng Lv, Joey Tianyi Zhou ; (6):1−28, 2022. [ abs ][ pdf ][ bib ] [ code ]
Bayesian Multinomial Logistic Normal Models through Marginally Latent Matrix-T Processes Justin D. Silverman, Kimberly Roche, Zachary C. Holmes, Lawrence A. David, Sayan Mukherjee ; (7):1−42, 2022. [ abs ][ pdf ][ bib ] [ code ]
Deep Learning in Target Space Michael Fairbank, Spyridon Samothrakis, Luca Citi ; (8):1−46, 2022. [ abs ][ pdf ][ bib ] [ code ]
Scaling Laws from the Data Manifold Dimension Utkarsh Sharma, Jared Kaplan ; (9):1−34, 2022. [ abs ][ pdf ][ bib ] [ code ]
Interpolating Predictors in High-Dimensional Factor Regression Florentina Bunea, Seth Strimas-Mackey, Marten Wegkamp ; (10):1−60, 2022. [ abs ][ pdf ][ bib ]
Near Optimality of Finite Memory Feedback Policies in Partially Observed Markov Decision Processes Ali Kara, Serdar Yuksel ; (11):1−46, 2022. [ abs ][ pdf ][ bib ]
Approximate Information State for Approximate Planning and Reinforcement Learning in Partially Observed Systems Jayakumar Subramanian, Amit Sinha, Raihan Seraj, Aditya Mahajan ; (12):1−83, 2022. [ abs ][ pdf ][ bib ] [ code ]
Solving Large-Scale Sparse PCA to Certifiable (Near) Optimality Dimitris Bertsimas, Ryan Cory-Wright, Jean Pauphilet ; (13):1−35, 2022. [ abs ][ pdf ][ bib ] [ code ]
On Generalizations of Some Distance Based Classifiers for HDLSS Data Sarbojit Roy, Soham Sarkar, Subhajit Dutta, Anil K. Ghosh ; (14):1−41, 2022. [ abs ][ pdf ][ bib ]
A Stochastic Bundle Method for Interpolation Alasdair Paren, Leonard Berrada, Rudra P. K. Poudel, M. Pawan Kumar ; (15):1−57, 2022. [ abs ][ pdf ][ bib ] [ code ]
TFPnP: Tuning-free Plug-and-Play Proximal Algorithms with Applications to Inverse Imaging Problems Kaixuan Wei, Angelica Aviles-Rivero, Jingwei Liang, Ying Fu, Hua Huang, Carola-Bibiane Schönlieb ; (16):1−48, 2022. [ abs ][ pdf ][ bib ] [ code ]
Spatial Multivariate Trees for Big Data Bayesian Regression Michele Peruzzi, David B. Dunson ; (17):1−40, 2022. [ abs ][ pdf ][ bib ] [ code ]
Decimated Framelet System on Graphs and Fast G-Framelet Transforms Xuebin Zheng, Bingxin Zhou, Yu Guang Wang, Xiaosheng Zhuang ; (18):1−68, 2022. [ abs ][ pdf ][ bib ] [ code ]
Universal Approximation in Dropout Neural Networks Oxana A. Manita, Mark A. Peletier, Jacobus W. Portegies, Jaron Sanders, Albert Senen-Cerda ; (19):1−46, 2022. [ abs ][ pdf ][ bib ]
Supervised Dimensionality Reduction and Visualization using Centroid-Encoder Tomojit Ghosh, Michael Kirby ; (20):1−34, 2022. [ abs ][ pdf ][ bib ] [ code ]
Evolutionary Variational Optimization of Generative Models Jakob Drefs, Enrico Guiraud, Jörg Lücke ; (21):1−51, 2022. [ abs ][ pdf ][ bib ] [ code ]
LSAR: Efficient Leverage Score Sampling Algorithm for the Analysis of Big Time Series Data Ali Eshragh, Fred Roosta, Asef Nazari, Michael W. Mahoney ; (22):1−36, 2022. [ abs ][ pdf ][ bib ]
Fast and Robust Rank Aggregation against Model Misspecification Yuangang Pan, Ivor W. Tsang, Weijie Chen, Gang Niu, Masashi Sugiyama ; (23):1−35, 2022. [ abs ][ pdf ][ bib ]
On Biased Stochastic Gradient Estimation Derek Driggs, Jingwei Liang, Carola-Bibiane Schönlieb ; (24):1−43, 2022. [ abs ][ pdf ][ bib ]
Efficient MCMC Sampling with Dimension-Free Convergence Rate using ADMM-type Splitting Maxime Vono, Daniel Paulin, Arnaud Doucet ; (25):1−69, 2022. [ abs ][ pdf ][ bib ]
MurTree: Optimal Decision Trees via Dynamic Programming and Search Emir Demirović, Anna Lukina, Emmanuel Hebrard, Jeffrey Chan, James Bailey, Christopher Leckie, Kotagiri Ramamohanarao, Peter J. Stuckey ; (26):1−47, 2022. [ abs ][ pdf ][ bib ] [ code ]
Data-Derived Weak Universal Consistency Narayana Santhanam, Venkatachalam Anantharam, Wojciech Szpankowski ; (27):1−55, 2022. [ abs ][ pdf ][ bib ]
Novel Min-Max Reformulations of Linear Inverse Problems Mohammed Rayyan Sheriff, Debasish Chatterjee ; (28):1−46, 2022. [ abs ][ pdf ][ bib ]
Theoretical Convergence of Multi-Step Model-Agnostic Meta-Learning Kaiyi Ji, Junjie Yang, Yingbin Liang ; (29):1−41, 2022. [ abs ][ pdf ][ bib ]
A Class of Conjugate Priors for Multinomial Probit Models which Includes the Multivariate Normal One Augusto Fasano, Daniele Durante ; (30):1−26, 2022. [ abs ][ pdf ][ bib ]
An Improper Estimator with Optimal Excess Risk in Misspecified Density Estimation and Logistic Regression Jaouad Mourtada, Stéphane Gaïffas ; (31):1−49, 2022. [ abs ][ pdf ][ bib ]
Active Learning for Nonlinear System Identification with Guarantees Horia Mania, Michael I. Jordan, Benjamin Recht ; (32):1−30, 2022. [ abs ][ pdf ][ bib ]
Model Averaging Is Asymptotically Better Than Model Selection For Prediction Tri M. Le, Bertrand S. Clarke ; (33):1−53, 2022. [ abs ][ pdf ][ bib ]
SODEN: A Scalable Continuous-Time Survival Model through Ordinary Differential Equation Networks Weijing Tang, Jiaqi Ma, Qiaozhu Mei, Ji Zhu ; (34):1−29, 2022. [ abs ][ pdf ][ bib ] [ code ]
Optimality and Stability in Non-Convex Smooth Games Guojun Zhang, Pascal Poupart, Yaoliang Yu ; (35):1−71, 2022. [ abs ][ pdf ][ bib ]
Accelerated Zeroth-Order and First-Order Momentum Methods from Mini to Minimax Optimization Feihu Huang, Shangqian Gao, Jian Pei, Heng Huang ; (36):1−70, 2022. [ abs ][ pdf ][ bib ]
Projected Statistical Methods for Distributional Data on the Real Line with the Wasserstein Metric Matteo Pegoraro, Mario Beraha ; (37):1−59, 2022. [ abs ][ pdf ][ bib ] [ code ]
Score Matched Neural Exponential Families for Likelihood-Free Inference Lorenzo Pacchiardi, Ritabrata Dutta ; (38):1−71, 2022. [ abs ][ pdf ][ bib ] [ code ]
(f,Gamma)-Divergences: Interpolating between f-Divergences and Integral Probability Metrics Jeremiah Birrell, Paul Dupuis, Markos A. Katsoulakis, Yannis Pantazis, Luc Rey-Bellet ; (39):1−70, 2022. [ abs ][ pdf ][ bib ]
Structure-adaptive Manifold Estimation Nikita Puchkin, Vladimir Spokoiny ; (40):1−62, 2022. [ abs ][ pdf ][ bib ]
The Correlation-assisted Missing Data Estimator Timothy I. Cannings, Yingying Fan ; (41):1−49, 2022. [ abs ][ pdf ][ bib ]
Approximation and Optimization Theory for Linear Continuous-Time Recurrent Neural Networks Zhong Li, Jiequn Han, Weinan E, Qianxiao Li ; (42):1−85, 2022. [ abs ][ pdf ][ bib ]
Sampling Permutations for Shapley Value Estimation Rory Mitchell, Joshua Cooper, Eibe Frank, Geoffrey Holmes ; (43):1−46, 2022. [ abs ][ pdf ][ bib ]
PAC Guarantees and Effective Algorithms for Detecting Novel Categories Si Liu, Risheek Garrepalli, Dan Hendrycks, Alan Fern, Debashis Mondal, Thomas G. Dietterich ; (44):1−47, 2022. [ abs ][ pdf ][ bib ] [ code ]
Optimal Transport for Stationary Markov Chains via Policy Iteration Kevin O'Connor, Kevin McGoff, Andrew B. Nobel ; (45):1−52, 2022. [ abs ][ pdf ][ bib ] [ code ]
Beyond Sub-Gaussian Noises: Sharp Concentration Analysis for Stochastic Gradient Descent Wanrong Zhu, Zhipeng Lou, Wei Biao Wu ; (46):1−22, 2022. [ abs ][ pdf ][ bib ]
Cascaded Diffusion Models for High Fidelity Image Generation Jonathan Ho, Chitwan Saharia, William Chan, David J. Fleet, Mohammad Norouzi, Tim Salimans ; (47):1−33, 2022. [ abs ][ pdf ][ bib ] [ code ]
Overparameterization of Deep ResNet: Zero Loss and Mean-field Analysis Zhiyan Ding, Shi Chen, Qin Li, Stephen J. Wright ; (48):1−65, 2022. [ abs ][ pdf ][ bib ]
Innovations Autoencoder and its Application in One-class Anomalous Sequence Detection Xinyi Wang, Lang Tong ; (49):1−27, 2022. [ abs ][ pdf ][ bib ]
Analytically Tractable Hidden-States Inference in Bayesian Neural Networks Luong-Ha Nguyen, James-A. Goulet ; (50):1−33, 2022. [ abs ][ pdf ][ bib ]
Toolbox for Multimodal Learn (scikit-multimodallearn) Dominique Benielli, Baptiste Bauvin, Sokol Koço, Riikka Huusari, Cécile Capponi, Hachem Kadri, François Laviolette ; (51):1−7, 2022. (Machine Learning Open Source Software Paper) [ abs ][ pdf ][ bib ] [ code ]
LinCDE: Conditional Density Estimation via Lindsey's Method Zijun Gao, Trevor Hastie ; (52):1−55, 2022. [ abs ][ pdf ][ bib ]
DoubleML - An Object-Oriented Implementation of Double Machine Learning in Python Philipp Bach, Victor Chernozhukov, Malte S. Kurz, Martin Spindler ; (53):1−6, 2022. (Machine Learning Open Source Software Paper) [ abs ][ pdf ][ bib ] [ code ]
SMAC3: A Versatile Bayesian Optimization Package for Hyperparameter Optimization Marius Lindauer, Katharina Eggensperger, Matthias Feurer, André Biedenkapp, Difan Deng, Carolin Benjamins, Tim Ruhkopf, René Sass, Frank Hutter ; (54):1−9, 2022. (Machine Learning Open Source Software Paper) [ abs ][ pdf ][ bib ] [ code ]
Bayesian Pseudo Posterior Mechanism under Asymptotic Differential Privacy Terrance D. Savitsky, Matthew R.Williams, Jingchen Hu ; (55):1−37, 2022. [ abs ][ pdf ][ bib ]
solo-learn: A Library of Self-supervised Methods for Visual Representation Learning Victor Guilherme Turrisi da Costa, Enrico Fini, Moin Nabi, Nicu Sebe, Elisa Ricci ; (56):1−6, 2022. (Machine Learning Open Source Software Paper) [ abs ][ pdf ][ bib ] [ code ]
Inherent Tradeoffs in Learning Fair Representations Han Zhao, Geoffrey J. Gordon ; (57):1−26, 2022. [ abs ][ pdf ][ bib ]
A Statistical Approach for Optimal Topic Model Identification Craig M. Lewis, Francesco Grossetti ; (58):1−20, 2022. [ abs ][ pdf ][ bib ]
Causal Classification: Treatment Effect Estimation vs. Outcome Prediction Carlos Fernández-Loría, Foster Provost ; (59):1−35, 2022. [ abs ][ pdf ][ bib ]
A Unifying Framework for Variance-Reduced Algorithms for Findings Zeroes of Monotone operators Xun Zhang, William B. Haskell, Zhisheng Ye ; (60):1−44, 2022. [ abs ][ pdf ][ bib ]
Sparse Additive Gaussian Process Regression Hengrui Luo, Giovanni Nattino, Matthew T. Pratola ; (61):1−34, 2022. [ abs ][ pdf ][ bib ]
The AIM and EM Algorithms for Learning from Coarse Data Manfred Jaeger ; (62):1−55, 2022. [ abs ][ pdf ][ bib ] [ code ]
Additive Nonlinear Quantile Regression in Ultra-high Dimension Ben Sherwood, Adam Maidman ; (63):1−47, 2022. [ abs ][ pdf ][ bib ]
Stochastic Zeroth-Order Optimization under Nonstationarity and Nonconvexity Abhishek Roy, Krishnakumar Balasubramanian, Saeed Ghadimi, Prasant Mohapatra ; (64):1−47, 2022. [ abs ][ pdf ][ bib ]
On the Complexity of Approximating Multimarginal Optimal Transport Tianyi Lin, Nhat Ho, Marco Cuturi, Michael I. Jordan ; (65):1−43, 2022. [ abs ][ pdf ][ bib ]
New Insights for the Multivariate Square-Root Lasso Aaron J. Molstad ; (66):1−52, 2022. [ abs ][ pdf ][ bib ] [ code ]
Are All Layers Created Equal? Chiyuan Zhang, Samy Bengio, Yoram Singer ; (67):1−28, 2022. [ abs ][ pdf ][ bib ]
Scaling-Translation-Equivariant Networks with Decomposed Convolutional Filters Wei Zhu, Qiang Qiu, Robert Calderbank, Guillermo Sapiro, Xiuyuan Cheng ; (68):1−45, 2022. [ abs ][ pdf ][ bib ]
Asymptotic Network Independence and Step-Size for a Distributed Subgradient Method Alex Olshevsky ; (69):1−32, 2022. [ abs ][ pdf ][ bib ] [ code ]
Generalized Sparse Additive Models Asad Haris, Noah Simon, Ali Shojaie ; (70):1−56, 2022. [ abs ][ pdf ][ bib ] [ code ]
Multiple-Splitting Projection Test for High-Dimensional Mean Vectors Wanjun Liu, Xiufan Yu, Runze Li ; (71):1−27, 2022. [ abs ][ pdf ][ bib ]
Batch Normalization Preconditioning for Neural Network Training Susanna Lange, Kyle Helfrich, Qiang Ye ; (72):1−41, 2022. [ abs ][ pdf ][ bib ]
A Kernel Two-Sample Test for Functional Data George Wynne, Andrew B. Duncan ; (73):1−51, 2022. [ abs ][ pdf ][ bib ]
All You Need is a Good Functional Prior for Bayesian Deep Learning Ba-Hien Tran, Simone Rossi, Dimitrios Milios, Maurizio Filippone ; (74):1−56, 2022. [ abs ][ pdf ][ bib ] [ code ]
Mutual Information Constraints for Monte-Carlo Objectives to Prevent Posterior Collapse Especially in Language Modelling Gábor Melis, András György, Phil Blunsom ; (75):1−36, 2022. [ abs ][ pdf ][ bib ]
Joint Inference of Multiple Graphs from Matrix Polynomials Madeline Navarro, Yuhao Wang, Antonio G. Marques, Caroline Uhler, Santiago Segarra ; (76):1−35, 2022. [ abs ][ pdf ][ bib ]
Efficient Change-Point Detection for Tackling Piecewise-Stationary Bandits Lilian Besson, Emilie Kaufmann, Odalric-Ambrym Maillard, Julien Seznec ; (77):1−40, 2022. [ abs ][ pdf ][ bib ] [ code ]
Multi-Agent Online Optimization with Delays: Asynchronicity, Adaptivity, and Optimism Yu-Guan Hsieh, Franck Iutzeler, Jérôme Malick, Panayotis Mertikopoulos ; (78):1−49, 2022. [ abs ][ pdf ][ bib ]
Stacking for Non-mixing Bayesian Computations: The Curse and Blessing of Multimodal Posteriors Yuling Yao, Aki Vehtari, Andrew Gelman ; (79):1−45, 2022. [ abs ][ pdf ][ bib ]
Posterior Asymptotics for Boosted Hierarchical Dirichlet Process Mixtures Marta Catalano, Pierpaolo De Blasi, Antonio Lijoi, Igor Pruenster ; (80):1−23, 2022. [ abs ][ pdf ][ bib ]
Dependent randomized rounding for clustering and partition systems with knapsack constraints David G. Harris, Thomas Pensyl, Aravind Srinivasan, Khoa Trinh ; (81):1−41, 2022. [ abs ][ pdf ][ bib ]
FuDGE: A Method to Estimate a Functional Differential Graph in a High-Dimensional Setting Boxin Zhao, Y. Samuel Wang, Mladen Kolar ; (82):1−82, 2022. [ abs ][ pdf ][ bib ] [ code ]
Prior Adaptive Semi-supervised Learning with Application to EHR Phenotyping Yichi Zhang, Molei Liu, Matey Neykov, Tianxi Cai ; (83):1−25, 2022. [ abs ][ pdf ][ bib ] [ code ]
Distributed Bayesian Varying Coefficient Modeling Using a Gaussian Process Prior Rajarshi Guhaniyogi, Cheng Li, Terrance D. Savitsky, Sanvesh Srivastava ; (84):1−59, 2022. [ abs ][ pdf ][ bib ]
A Distribution Free Conditional Independence Test with Applications to Causal Discovery Zhanrui Cai, Runze Li, Yaowu Zhang ; (85):1−41, 2022. [ abs ][ pdf ][ bib ]
Robust and scalable manifold learning via landmark diffusion for long-term medical signal processing Chao Shen, Yu-Ting Lin, Hau-Tieng Wu ; (86):1−30, 2022. [ abs ][ pdf ][ bib ]
CD-split and HPD-split: Efficient Conformal Regions in High Dimensions Rafael Izbicki, Gilson Shimizu, Rafael B. Stern ; (87):1−32, 2022. [ abs ][ pdf ][ bib ] [ code ]
Generalized Ambiguity Decomposition for Ranking Ensemble Learning Hongzhi Liu, Yingpeng Du, Zhonghai Wu ; (88):1−36, 2022. [ abs ][ pdf ][ bib ]
Machine Learning on Graphs: A Model and Comprehensive Taxonomy Ines Chami, Sami Abu-El-Haija, Bryan Perozzi, Christopher Ré, Kevin Murphy ; (89):1−64, 2022. [ abs ][ pdf ][ bib ]
Accelerating Adaptive Cubic Regularization of Newton's Method via Random Sampling Xi Chen, Bo Jiang, Tianyi Lin, Shuzhong Zhang ; (90):1−38, 2022. [ abs ][ pdf ][ bib ]
When Hardness of Approximation Meets Hardness of Learning Eran Malach, Shai Shalev-Shwartz ; (91):1−24, 2022. [ abs ][ pdf ][ bib ]
Gauss-Legendre Features for Gaussian Process Regression Paz Fink Shustin, Haim Avron ; (92):1−47, 2022. [ abs ][ pdf ][ bib ]
Regularized K-means Through Hard-Thresholding Jakob Raymaekers, Ruben H. Zamar ; (93):1−48, 2022. [ abs ][ pdf ][ bib ] [ code ]
Multiple Testing in Nonparametric Hidden Markov Models: An Empirical Bayes Approach Kweku Abraham, Ismaël Castillo, Elisabeth Gassiat ; (94):1−57, 2022. [ abs ][ pdf ][ bib ]
Attraction-Repulsion Spectrum in Neighbor Embeddings Jan Niklas Böhm, Philipp Berens, Dmitry Kobak ; (95):1−32, 2022. [ abs ][ pdf ][ bib ] [ code ]
Rethinking Nonlinear Instrumental Variable Models through Prediction Validity Chunxiao Li, Cynthia Rudin, Tyler H. McCormick ; (96):1−55, 2022. [ abs ][ pdf ][ bib ]
Unlabeled Data Help in Graph-Based Semi-Supervised Learning: A Bayesian Nonparametrics Perspective Daniel Sanz-Alonso, Ruiyi Yang ; (97):1−28, 2022. [ abs ][ pdf ][ bib ]
PECOS: Prediction for Enormous and Correlated Output Spaces Hsiang-Fu Yu, Kai Zhong, Jiong Zhang, Wei-Cheng Chang, Inderjit S. Dhillon ; (98):1−32, 2022. [ abs ][ pdf ][ bib ] [ code ]
Distributed Learning of Finite Gaussian Mixtures Qiong Zhang, Jiahua Chen ; (99):1−40, 2022. [ abs ][ pdf ][ bib ] [ code ]
Total Stability of SVMs and Localized SVMs Hannes Köhler, Andreas Christmann ; (100):1−41, 2022. [ abs ][ pdf ][ bib ]
Towards An Efficient Approach for the Nonconvex lp Ball Projection: Algorithm and Analysis Xiangyu Yang, Jiashan Wang, Hao Wang ; (101):1−31, 2022. [ abs ][ pdf ][ bib ]
Sufficient reductions in regression with mixed predictors Efstathia Bura, Liliana Forzani, Rodrigo Garcia Arancibia, Pamela Llop, Diego Tomassi ; (102):1−47, 2022. [ abs ][ pdf ][ bib ] [ code ]
The EM Algorithm is Adaptively-Optimal for Unbalanced Symmetric Gaussian Mixtures Nir Weinberger, Guy Bresler ; (103):1−79, 2022. [ abs ][ pdf ][ bib ]
Efficient Least Squares for Estimating Total Effects under Linearity and Causal Sufficiency F. Richard Guo, Emilija Perković ; (104):1−41, 2022. [ abs ][ pdf ][ bib ] [ code ]
Globally Injective ReLU Networks Michael Puthawala, Konik Kothari, Matti Lassas, Ivan Dokmanić, Maarten de Hoop ; (105):1−55, 2022. [ abs ][ pdf ][ bib ]
Riemannian Stochastic Proximal Gradient Methods for Nonsmooth Optimization over the Stiefel Manifold Bokun Wang, Shiqian Ma, Lingzhou Xue ; (106):1−33, 2022. [ abs ][ pdf ][ bib ]
IALE: Imitating Active Learner Ensembles Christoffer Löffler, Christopher Mutschler ; (107):1−29, 2022. [ abs ][ pdf ][ bib ] [ code ]
Bayesian subset selection and variable importance for interpretable prediction and classification Daniel R. Kowal ; (108):1−38, 2022. [ abs ][ pdf ][ bib ] [ code ]
Conditions and Assumptions for Constraint-based Causal Structure Learning Kayvan Sadeghi, Terry Soo ; (109):1−34, 2022. [ abs ][ pdf ][ bib ]
EiGLasso for Scalable Sparse Kronecker-Sum Inverse Covariance Estimation Jun Ho Yoon, Seyoung Kim ; (110):1−39, 2022. [ abs ][ pdf ][ bib ] [ code ]
Advantage of Deep Neural Networks for Estimating Functions with Singularity on Hypersurfaces Masaaki Imaizumi, Kenji Fukumizu ; (111):1−54, 2022. [ abs ][ pdf ][ bib ]
Sum of Ranked Range Loss for Supervised Learning Shu Hu, Yiming Ying, Xin Wang, Siwei Lyu ; (112):1−44, 2022. [ abs ][ pdf ][ bib ] [ code ]
The Two-Sided Game of Googol José Correa, Andrés Cristi, Boris Epstein, José Soto ; (113):1−37, 2022. [ abs ][ pdf ][ bib ]
ReduNet: A White-box Deep Network from the Principle of Maximizing Rate Reduction Kwan Ho Ryan Chan, Yaodong Yu, Chong You, Haozhi Qi, John Wright, Yi Ma ; (114):1−103, 2022. [ abs ][ pdf ][ bib ] [ code ]
Cauchy–Schwarz Regularized Autoencoder Linh Tran, Maja Pantic, Marc Peter Deisenroth ; (115):1−37, 2022. [ abs ][ pdf ][ bib ]
An Error Analysis of Generative Adversarial Networks for Learning Distributions Jian Huang, Yuling Jiao, Zhen Li, Shiao Liu, Yang Wang, Yunfei Yang ; (116):1−43, 2022. [ abs ][ pdf ][ bib ]
OVERT: An Algorithm for Safety Verification of Neural Network Control Policies for Nonlinear Systems Chelsea Sidrane, Amir Maleki, Ahmed Irfan, Mykel J. Kochenderfer ; (117):1−45, 2022. [ abs ][ pdf ][ bib ] [ code ]
Under-bagging Nearest Neighbors for Imbalanced Classification Hanyuan Hang, Yuchao Cai, Hanfang Yang, Zhouchen Lin ; (118):1−63, 2022. [ abs ][ pdf ][ bib ]
A spectral-based analysis of the separation between two-layer neural networks and linear methods Lei Wu, Jihao Long ; (119):1−34, 2022. [ abs ][ pdf ][ bib ]
Switch Transformers: Scaling to Trillion Parameter Models with Simple and Efficient Sparsity William Fedus, Barret Zoph, Noam Shazeer ; (120):1−39, 2022. [ abs ][ pdf ][ bib ] [ code ]
Online Mirror Descent and Dual Averaging: Keeping Pace in the Dynamic Case Huang Fang, Nicholas J. A. Harvey, Victor S. Portella, Michael P. Friedlander ; (121):1−38, 2022. [ abs ][ pdf ][ bib ]
Depth separation beyond radial functions Luca Venturi, Samy Jelassi, Tristan Ozuch, Joan Bruna ; (122):1−56, 2022. [ abs ][ pdf ][ bib ]
Provable Tensor-Train Format Tensor Completion by Riemannian Optimization Jian-Feng Cai, Jingyang Li, Dong Xia ; (123):1−77, 2022. [ abs ][ pdf ][ bib ]
Darts: User-Friendly Modern Machine Learning for Time Series Julien Herzen, Francesco Lässig, Samuele Giuliano Piazzetta, Thomas Neuer, Léo Tafti, Guillaume Raille, Tomas Van Pottelbergh, Marek Pasieka, Andrzej Skrodzki, Nicolas Huguenin, Maxime Dumonal, Jan Kościsz, Dennis Bader, Frédérick Gusset, Mounir Benheddi, Camila Williamson, Michal Kosinski, Matej Petrik, Gaël Grosch ; (124):1−6, 2022. (Machine Learning Open Source Software Paper) [ abs ][ pdf ][ bib ] [ code ]
Foolish Crowds Support Benign Overfitting Niladri S. Chatterji, Philip M. Long ; (125):1−12, 2022. [ abs ][ pdf ][ bib ]
Neural Estimation of Statistical Divergences Sreejith Sreekumar, Ziv Goldfeld ; (126):1−75, 2022. [ abs ][ pdf ][ bib ]
Kernel Packet: An Exact and Scalable Algorithm for Gaussian Process Regression with Matérn Correlations Haoyuan Chen, Liang Ding, Rui Tuo ; (127):1−32, 2022. [ abs ][ pdf ][ bib ]
Power Iteration for Tensor PCA Jiaoyang Huang, Daniel Z. Huang, Qing Yang, Guang Cheng ; (128):1−47, 2022. [ abs ][ pdf ][ bib ]
On the Approximation of Cooperative Heterogeneous Multi-Agent Reinforcement Learning (MARL) using Mean Field Control (MFC) Washim Uddin Mondal, Mridul Agarwal, Vaneet Aggarwal, Satish V. Ukkusuri ; (129):1−46, 2022. [ abs ][ pdf ][ bib ]
Mean-field Analysis of Piecewise Linear Solutions for Wide ReLU Networks Alexander Shevchenko, Vyacheslav Kungurtsev, Marco Mondelli ; (130):1−55, 2022. [ abs ][ pdf ][ bib ]
Let's Make Block Coordinate Descent Converge Faster: Faster Greedy Rules, Message-Passing, Active-Set Complexity, and Superlinear Convergence Julie Nutini, Issam Laradji, Mark Schmidt ; (131):1−74, 2022. [ abs ][ pdf ][ bib ] [ code ]
An Optimization-centric View on Bayes' Rule: Reviewing and Generalizing Variational Inference Jeremias Knoblauch, Jack Jewson, Theodoros Damoulas ; (132):1−109, 2022. [ abs ][ pdf ][ bib ] [ code ]
Manifold Coordinates with Physical Meaning Samson J. Koelle, Hanyu Zhang, Marina Meila, Yu-Chia Chen ; (133):1−57, 2022. [ abs ][ pdf ][ bib ] [ code ]
Transfer Learning in Information Criteria-based Feature Selection Shaohan Chen, Nikolaos V. Sahinidis, Chuanhou Gao ; (134):1−105, 2022. [ abs ][ pdf ][ bib ] [ code ]
Recovery and Generalization in Over-Realized Dictionary Learning Jeremias Sulam, Chong You, Zhihui Zhu ; (135):1−23, 2022. [ abs ][ pdf ][ bib ]
Low-rank Tensor Learning with Nonconvex Overlapped Nuclear Norm Regularization Quanming Yao, Yaqing Wang, Bo Han, James T. Kwok ; (136):1−60, 2022. [ abs ][ pdf ][ bib ] [ code ]
On the Efficiency of Entropic Regularized Algorithms for Optimal Transport Tianyi Lin, Nhat Ho, Michael I. Jordan ; (137):1−42, 2022. [ abs ][ pdf ][ bib ]
Exact simulation of diffusion first exit times: algorithm acceleration Samuel Herrmann, Cristina Zucca ; (138):1−20, 2022. [ abs ][ pdf ][ bib ] [ code ]
No Weighted-Regret Learning in Adversarial Bandits with Delays Ilai Bistritz, Zhengyuan Zhou, Xi Chen, Nicholas Bambos, Jose Blanchet ; (139):1−43, 2022. [ abs ][ pdf ][ bib ]
Non-asymptotic and Accurate Learning of Nonlinear Dynamical Systems Yahya Sattar, Samet Oymak ; (140):1−49, 2022. [ abs ][ pdf ][ bib ]
The Importance of Being Correlated: Implications of Dependence in Joint Spectral Inference across Multiple Networks Konstantinos Pantazis, Avanti Athreya, Jesus Arroyo, William N Frost, Evan S Hill, Vince Lyzinski ; (141):1−77, 2022. [ abs ][ pdf ][ bib ]
A Perturbation-Based Kernel Approximation Framework Roy Mitz, Yoel Shkolnisky ; (142):1−26, 2022. [ abs ][ pdf ][ bib ] [ code ]
Reverse-mode differentiation in arbitrary tensor network format: with application to supervised learning Alex A. Gorodetsky, Cosmin Safta, John D. Jakeman ; (143):1−29, 2022. [ abs ][ pdf ][ bib ]
A Momentumized, Adaptive, Dual Averaged Gradient Method Aaron Defazio, Samy Jelassi ; (144):1−34, 2022. [ abs ][ pdf ][ bib ] [ code ]
A Generalized Projected Bellman Error for Off-policy Value Estimation in Reinforcement Learning Andrew Patterson, Adam White, Martha White ; (145):1−61, 2022. [ abs ][ pdf ][ bib ] [ code ]
Adversarial Robustness Guarantees for Gaussian Processes Andrea Patane, Arno Blaas, Luca Laurenti, Luca Cardelli, Stephen Roberts, Marta Kwiatkowska ; (146):1−55, 2022. [ abs ][ pdf ][ bib ] [ code ]
On the Robustness to Misspecification of α-posteriors and Their Variational Approximations Marco Avella Medina, José Luis Montiel Olea, Cynthia Rush, Amilcar Velez ; (147):1−51, 2022. [ abs ][ pdf ][ bib ]
Online Nonnegative CP-dictionary Learning for Markovian Data Hanbaek Lyu, Christopher Strohmeier, Deanna Needell ; (148):1−50, 2022. [ abs ][ pdf ][ bib ] [ code ]
Implicit Differentiation for Fast Hyperparameter Selection in Non-Smooth Convex Learning Quentin Bertrand, Quentin Klopfenstein, Mathurin Massias, Mathieu Blondel, Samuel Vaiter, Alexandre Gramfort, Joseph Salmon ; (149):1−43, 2022. [ abs ][ pdf ][ bib ] [ code ]
EV-GAN: Simulation of extreme events with ReLU neural networks Michaël Allouche, Stéphane Girard, Emmanuel Gobet ; (150):1−39, 2022. [ abs ][ pdf ][ bib ]
Universal Approximation of Functions on Sets Edward Wagstaff, Fabian B. Fuchs, Martin Engelcke, Michael A. Osborne, Ingmar Posner ; (151):1−56, 2022. [ abs ][ pdf ][ bib ]
Intrinsically Motivated Goal Exploration Processes with Automatic Curriculum Learning Sébastien Forestier, Rémy Portelas, Yoan Mollard, Pierre-Yves Oudeyer ; (152):1−41, 2022. [ abs ][ pdf ][ bib ]
Truncated Emphatic Temporal Difference Methods for Prediction and Control Shangtong Zhang, Shimon Whiteson ; (153):1−59, 2022. [ abs ][ pdf ][ bib ] [ code ]
Policy Evaluation and Temporal-Difference Learning in Continuous Time and Space: A Martingale Approach Yanwei Jia, Xun Yu Zhou ; (154):1−55, 2022. [ abs ][ pdf ][ bib ] [ code ]
Principal Components Bias in Over-parameterized Linear Models, and its Manifestation in Deep Neural Networks Guy Hacohen, Daphna Weinshall ; (155):1−46, 2022. [ abs ][ pdf ][ bib ]
Statistical Rates of Convergence for Functional Partially Linear Support Vector Machines for Classification Yingying Zhang, Yan-Yong Zhao, Heng Lian ; (156):1−24, 2022. [ abs ][ pdf ][ bib ]
A universally consistent learning rule with a universally monotone error Vladimir Pestov ; (157):1−27, 2022. [ abs ][ pdf ][ bib ]
ktrain: A Low-Code Library for Augmented Machine Learning Arun S. Maiya ; (158):1−6, 2022. (Machine Learning Open Source Software Paper) [ abs ][ pdf ][ bib ] [ code ]
Structure Learning for Directed Trees Martin E. Jakobsen, Rajen D. Shah, Peter Bühlmann, Jonas Peters ; (159):1−97, 2022. [ abs ][ pdf ][ bib ] [ code ]
Fairness-Aware PAC Learning from Corrupted Data Nikola Konstantinov, Christoph H. Lampert ; (160):1−60, 2022. [ abs ][ pdf ][ bib ]
Topologically penalized regression on manifolds Olympio Hacquard, Krishnakumar Balasubramanian, Gilles Blanchard, Clément Levrard, Wolfgang Polonik ; (161):1−39, 2022. [ abs ][ pdf ][ bib ] [ code ]
Explicit Convergence Rates of Greedy and Random Quasi-Newton Methods Dachao Lin, Haishan Ye, Zhihua Zhang ; (162):1−40, 2022. [ abs ][ pdf ][ bib ]
Scaling and Scalability: Provable Nonconvex Low-Rank Tensor Estimation from Incomplete Measurements Tian Tong, Cong Ma, Ashley Prater-Bennette, Erin Tripp, Yuejie Chi ; (163):1−77, 2022. [ abs ][ pdf ][ bib ] [ code ]
Solving L1-regularized SVMs and Related Linear Programs: Revisiting the Effectiveness of Column and Constraint Generation Antoine Dedieu, Rahul Mazumder, Haoyue Wang ; (164):1−41, 2022. [ abs ][ pdf ][ bib ]
Improved Classification Rates for Localized SVMs Ingrid Blaschzyk, Ingo Steinwart ; (165):1−59, 2022. [ abs ][ pdf ][ bib ]
Generalization Bounds and Representation Learning for Estimation of Potential Outcomes and Causal Effects Fredrik D. Johansson, Uri Shalit, Nathan Kallus, David Sontag ; (166):1−50, 2022. [ abs ][ pdf ][ bib ]
Unbiased estimators for random design regression Michał Dereziński, Manfred K. Warmuth, Daniel Hsu ; (167):1−46, 2022. [ abs ][ pdf ][ bib ]
A Worst Case Analysis of Calibrated Label Ranking Multi-label Classification Method Lucas Henrique Sousa Mello, Flávio Miguel Varejão, Alexandre Loureiros Rodrigues ; (168):1−30, 2022. [ abs ][ pdf ][ bib ]
D-GCCA: Decomposition-based Generalized Canonical Correlation Analysis for Multi-view High-dimensional Data Hai Shu, Zhe Qu, Hongtu Zhu ; (169):1−64, 2022. [ abs ][ pdf ][ bib ] [ code ]
Scalable and Efficient Hypothesis Testing with Random Forests Tim Coleman, Wei Peng, Lucas Mentch ; (170):1−35, 2022. [ abs ][ pdf ][ bib ]
Interlocking Backpropagation: Improving depthwise model-parallelism Aidan N. Gomez, Oscar Key, Kuba Perlin, Stephen Gou, Nick Frosst, Jeff Dean, Yarin Gal ; (171):1−28, 2022. [ abs ][ pdf ][ bib ]
Projection-free Distributed Online Learning with Sublinear Communication Complexity Yuanyu Wan, Guanghui Wang, Wei-Wei Tu, Lijun Zhang ; (172):1−53, 2022. [ abs ][ pdf ][ bib ]
Learning Rates as a Function of Batch Size: A Random Matrix Theory Approach to Neural Network Training Diego Granziol, Stefan Zohren, Stephen Roberts ; (173):1−65, 2022. [ abs ][ pdf ][ bib ]
Training and Evaluation of Deep Policies Using Reinforcement Learning and Generative Models Ali Ghadirzadeh, Petra Poklukar, Karol Arndt, Chelsea Finn, Ville Kyrki, Danica Kragic, Mårten Björkman ; (174):1−37, 2022. [ abs ][ pdf ][ bib ]
Improved Generalization Bounds for Adversarially Robust Learning Idan Attias, Aryeh Kontorovich, Yishay Mansour ; (175):1−31, 2022. [ abs ][ pdf ][ bib ]
Signature Moments to Characterize Laws of Stochastic Processes Ilya Chevyrev, Harald Oberhauser ; (176):1−42, 2022. [ abs ][ pdf ][ bib ]
Asymptotic Analysis of Sampling Estimators for Randomized Numerical Linear Algebra Algorithms Ping Ma, Yongkai Chen, Xinlian Zhang, Xin Xing, Jingyi Ma, Michael W. Mahoney ; (177):1−45, 2022. [ abs ][ pdf ][ bib ]
Logarithmic Regret for Episodic Continuous-Time Linear-Quadratic Reinforcement Learning over a Finite-Time Horizon Matteo Basei, Xin Guo, Anran Hu, Yufei Zhang ; (178):1−34, 2022. [ abs ][ pdf ][ bib ]
KL-UCB-Switch: Optimal Regret Bounds for Stochastic Bandits from Both a Distribution-Dependent and a Distribution-Free Viewpoints Aurélien Garivier, Hédi Hadiji, Pierre Ménard, Gilles Stoltz ; (179):1−66, 2022. [ abs ][ pdf ][ bib ]
Matrix Completion with Covariate Information and Informative Missingness Huaqing Jin, Yanyuan Ma, Fei Jiang ; (180):1−62, 2022. [ abs ][ pdf ][ bib ] [ code ]
Training Two-Layer ReLU Networks with Gradient Descent is Inconsistent David Holzmüller, Ingo Steinwart ; (181):1−82, 2022. [ abs ][ pdf ][ bib ] [ code ]
Extensions to the Proximal Distance Method of Constrained Optimization Alfonso Landeros, Oscar Hernan Madrid Padilla, Hua Zhou, Kenneth Lange ; (182):1−45, 2022. [ abs ][ pdf ][ bib ] [ code ]
Boulevard: Regularized Stochastic Gradient Boosted Trees and Their Limiting Distribution Yichen Zhou, Giles Hooker ; (183):1−44, 2022. [ abs ][ pdf ][ bib ] [ code ]
Statistical Optimality and Stability of Tangent Transform Algorithms in Logit Models Indrajit Ghosh, Anirban Bhattacharya, Debdeep Pati ; (184):1−42, 2022. [ abs ][ pdf ][ bib ]
A Primer for Neural Arithmetic Logic Modules Bhumika Mistry, Katayoun Farrahi, Jonathon Hare ; (185):1−58, 2022. [ abs ][ pdf ][ bib ] [ code ]
Estimating Density Models with Truncation Boundaries using Score Matching Song Liu, Takafumi Kanamori, Daniel J. Williams ; (186):1−38, 2022. [ abs ][ pdf ][ bib ] [ code ]
Adversarial Classification: Necessary Conditions and Geometric Flows Nicolás García Trillos, Ryan Murray ; (187):1−38, 2022. [ abs ][ pdf ][ bib ]
Active Structure Learning of Bayesian Networks in an Observational Setting Noa Ben-David, Sivan Sabato ; (188):1−38, 2022. [ abs ][ pdf ][ bib ] [ code ]
Learning to Optimize: A Primer and A Benchmark Tianlong Chen, Xiaohan Chen, Wuyang Chen, Howard Heaton, Jialin Liu, Zhangyang Wang, Wotao Yin ; (189):1−59, 2022. [ abs ][ pdf ][ bib ] [ code ]
Clustering with Semidefinite Programming and Fixed Point Iteration Pedro Felzenszwalb, Caroline Klivans, Alice Paul ; (190):1−23, 2022. [ abs ][ pdf ][ bib ]
Deep Limits and a Cut-Off Phenomenon for Neural Networks Benny Avelin, Anders Karlsson ; (191):1−29, 2022. [ abs ][ pdf ][ bib ]
A Bregman Learning Framework for Sparse Neural Networks Leon Bungert, Tim Roith, Daniel Tenbrinck, Martin Burger ; (192):1−43, 2022. [ abs ][ pdf ][ bib ] [ code ]
Gaussian process regression: Optimality, robustness, and relationship with kernel ridge regression Wenjia Wang, Bing-Yi Jing ; (193):1−67, 2022. [ abs ][ pdf ][ bib ]
Uniform deconvolution for Poisson Point Processes Anna Bonnet, Claire Lacour, Franck Picard, Vincent Rivoirard ; (194):1−36, 2022. [ abs ][ pdf ][ bib ]
Distributed Bootstrap for Simultaneous Inference Under High Dimensionality Yang Yu, Shih-Kang Chao, Guang Cheng ; (195):1−77, 2022. [ abs ][ pdf ][ bib ] [ code ]
Universal Approximation Theorems for Differentiable Geometric Deep Learning Anastasis Kratsios, Léonie Papon ; (196):1−73, 2022. [ abs ][ pdf ][ bib ]
InterpretDL: Explaining Deep Models in PaddlePaddle Xuhong Li, Haoyi Xiong, Xingjian Li, Xuanyu Wu, Zeyu Chen, Dejing Dou ; (197):1−6, 2022. (Machine Learning Open Source Software Paper) [ abs ][ pdf ][ bib ] [ code ]
Meta-analysis of heterogeneous data: integrative sparse regression in high-dimensions Subha Maity, Yuekai Sun, Moulinath Banerjee ; (198):1−50, 2022. [ abs ][ pdf ][ bib ] [ code ]
A Forward Approach for Sufficient Dimension Reduction in Binary Classification Jongkyeong Kang, Seung Jun Shin ; (199):1−31, 2022. [ abs ][ pdf ][ bib ]
A Nonconvex Framework for Structured Dynamic Covariance Recovery Katherine Tsai, Mladen Kolar, Oluwasanmi Koyejo ; (200):1−91, 2022. [ abs ][ pdf ][ bib ] [ code ]
Three rates of convergence or separation via U-statistics in a dependent framework Quentin Duchemin, Yohann De Castro, Claire Lacour ; (201):1−59, 2022. [ abs ][ pdf ][ bib ] [ code ]
abess: A Fast Best-Subset Selection Library in Python and R Jin Zhu, Xueqin Wang, Liyuan Hu, Junhao Huang, Kangkang Jiang, Yanhang Zhang, Shiyun Lin, Junxian Zhu ; (202):1−7, 2022. (Machine Learning Open Source Software Paper) [ abs ][ pdf ][ bib ] [ code ]
Testing Whether a Learning Procedure is Calibrated Jon Cockayne, Matthew M. Graham, Chris J. Oates, T. J. Sullivan, Onur Teymur ; (203):1−36, 2022. [ abs ][ pdf ][ bib ]
Selective Machine Learning of the Average Treatment Effect with an Invalid Instrumental Variable Baoluo Sun, Yifan Cui, Eric Tchetgen Tchetgen ; (204):1−40, 2022. [ abs ][ pdf ][ bib ]
Contraction rates for sparse variational approximations in Gaussian process regression Dennis Nieman, Botond Szabo, Harry van Zanten ; (205):1−26, 2022. [ abs ][ pdf ][ bib ]
Stochastic DCA with Variance Reduction and Applications in Machine Learning Hoai An Le Thi, Hoang Phuc Hau Luu, Hoai Minh Le, Tao Pham Dinh ; (206):1−44, 2022. [ abs ][ pdf ][ bib ]
Nonconvex Matrix Completion with Linearly Parameterized Factors Ji Chen, Xiaodong Li, Zongming Ma ; (207):1−35, 2022. [ abs ][ pdf ][ bib ]
tntorch: Tensor Network Learning with PyTorch Mikhail Usvyatsov, Rafael Ballester-Ripoll, Konrad Schindler ; (208):1−6, 2022. [ abs ][ pdf ][ bib ] [ code ]
Ranking and Tuning Pre-trained Models: A New Paradigm for Exploiting Model Hubs Kaichao You, Yong Liu, Ziyang Zhang, Jianmin Wang, Michael I. Jordan, Mingsheng Long ; (209):1−47, 2022. [ abs ][ pdf ][ bib ] [ code ]
A Unified Statistical Learning Model for Rankings and Scores with Application to Grant Panel Review Michael Pearce, Elena A. Erosheva ; (210):1−33, 2022. [ abs ][ pdf ][ bib ]
Efficient Inference for Dynamic Flexible Interactions of Neural Populations Feng Zhou, Quyu Kong, Zhijie Deng, Jichao Kan, Yixuan Zhang, Cheng Feng, Jun Zhu ; (211):1−49, 2022. [ abs ][ pdf ][ bib ]
Multi-Agent Multi-Armed Bandits with Limited Communication Mridul Agarwal, Vaneet Aggarwal, Kamyar Azizzadenesheli ; (212):1−24, 2022. [ abs ][ pdf ][ bib ]
Using Shapley Values and Variational Autoencoders to Explain Predictive Models with Dependent Mixed Features Lars H. B. Olsen, Ingrid K. Glad, Martin Jullum, Kjersti Aas ; (213):1−51, 2022. [ abs ][ pdf ][ bib ] [ code ]
When is the Convergence Time of Langevin Algorithms Dimension Independent? A Composite Optimization Viewpoint Yoav Freund, Yi-An Ma, Tong Zhang ; (214):1−32, 2022. [ abs ][ pdf ][ bib ]
Learning Operators with Coupled Attention Georgios Kissas, Jacob H. Seidman, Leonardo Ferreira Guilhoto, Victor M. Preciado, George J. Pappas, Paris Perdikaris ; (215):1−63, 2022. [ abs ][ pdf ][ bib ]
Kernel Partial Correlation Coefficient --- a Measure of Conditional Dependence Zhen Huang, Nabarun Deb, Bodhisattva Sen ; (216):1−58, 2022. [ abs ][ pdf ][ bib ]
Smooth Robust Tensor Completion for Background/Foreground Separation with Missing Pixels: Novel Algorithm with Convergence Guarantee Bo Shen, Weijun Xie, Zhenyu (James) Kong ; (217):1−40, 2022. [ abs ][ pdf ][ bib ] [ code ]
Learning Green's functions associated with time-dependent partial differential equations Nicolas Boullé, Seick Kim, Tianyi Shi, Alex Townsend ; (218):1−34, 2022. [ abs ][ pdf ][ bib ]
Structural Agnostic Modeling: Adversarial Learning of Causal Graphs Diviyan Kalainathan, Olivier Goudet, Isabelle Guyon, David Lopez-Paz, Michèle Sebag ; (219):1−62, 2022. [ abs ][ pdf ][ bib ] [ code ]
Robust Distributed Accelerated Stochastic Gradient Methods for Multi-Agent Networks Alireza Fallah, Mert Gürbüzbalaban, Asuman Ozdaglar, Umut Şimşekli, Lingjiong Zhu ; (220):1−96, 2022. [ abs ][ pdf ][ bib ]
Behavior Priors for Efficient Reinforcement Learning Dhruva Tirumala, Alexandre Galashov, Hyeonwoo Noh, Leonard Hasenclever, Razvan Pascanu, Jonathan Schwarz, Guillaume Desjardins, Wojciech Marian Czarnecki, Arun Ahuja, Yee Whye Teh, Nicolas Heess ; (221):1−68, 2022. [ abs ][ pdf ][ bib ]
Variance Reduced EXTRA and DIGing and Their Optimal Acceleration for Strongly Convex Decentralized Optimization Huan Li, Zhouchen Lin, Yongchun Fang ; (222):1−41, 2022. [ abs ][ pdf ][ bib ]
On Acceleration for Convex Composite Minimization with Noise-Corrupted Gradients and Approximate Proximal Mapping Qiang Zhou, Sinno Jialin Pan ; (223):1−59, 2022. [ abs ][ pdf ][ bib ]
Getting Better from Worse: Augmented Bagging and A Cautionary Tale of Variable Importance Lucas Mentch, Siyu Zhou ; (224):1−32, 2022. [ abs ][ pdf ][ bib ]
Two-Sample Testing on Ranked Preference Data and the Role of Modeling Assumptions Charvi Rastogi, Sivaraman Balakrishnan, Nihar B. Shah, Aarti Singh ; (225):1−48, 2022. [ abs ][ pdf ][ bib ]
Underspecification Presents Challenges for Credibility in Modern Machine Learning Alexander D'Amour, Katherine Heller, Dan Moldovan, Ben Adlam, Babak Alipanahi, Alex Beutel, Christina Chen, Jonathan Deaton, Jacob Eisenstein, Matthew D. Hoffman, Farhad Hormozdiari, Neil Houlsby, Shaobo Hou, Ghassen Jerfel, Alan Karthikesalingam, Mario Lucic, Yian Ma, Cory McLean, Diana Mincu, Akinori Mitani, Andrea Montanari, Zachary Nado, Vivek Natarajan, Christopher Nielson, Thomas F. Osborne, Rajiv Raman, Kim Ramasamy, Rory Sayres, Jessica Schrouff, Martin Seneviratne, Shannon Sequeira, Harini Suresh, Victor Veitch, Max Vladymyrov, Xuezhi Wang, Kellie Webster, Steve Yadlowsky, Taedong Yun, Xiaohua Zhai, D. Sculley ; (226):1−61, 2022. [ abs ][ pdf ][ bib ]
Gaussian Process Parameter Estimation Using Mini-batch Stochastic Gradient Descent: Convergence Guarantees and Empirical Benefits Hao Chen, Lili Zheng, Raed Al Kontar, Garvesh Raskutti ; (227):1−59, 2022. [ abs ][ pdf ][ bib ]
Asymptotic Study of Stochastic Adaptive Algorithms in Non-convex Landscape Sébastien Gadat, Ioana Gavra ; (228):1−54, 2022. [ abs ][ pdf ][ bib ]
Towards Practical Adam: Non-Convexity, Convergence Theory, and Mini-Batch Acceleration Congliang Chen, Li Shen, Fangyu Zou, Wei Liu ; (229):1−47, 2022. [ abs ][ pdf ][ bib ]
Multi-Task Dynamical Systems Alex Bird, Christopher K. I. Williams, Christopher Hawthorne ; (230):1−52, 2022. [ abs ][ pdf ][ bib ]
Representation Learning for Maximization of MI, Nonlinear ICA and Nonlinear Subspaces with Robust Density Ratio Estimation Hiroaki Sasaki, Takashi Takenouchi ; (231):1−55, 2022. [ abs ][ pdf ][ bib ]
Gaussian Process Boosting Fabio Sigrist ; (232):1−46, 2022. [ abs ][ pdf ][ bib ] [ code ]
An Efficient Sampling Algorithm for Non-smooth Composite Potentials Wenlong Mou, Nicolas Flammarion, Martin J. Wainwright, Peter L. Bartlett ; (233):1−50, 2022. [ abs ][ pdf ][ bib ]
Change point localization in dependent dynamic nonparametric random dot product graphs Oscar Hernan Madrid Padilla, Yi Yu, Carey E. Priebe ; (234):1−59, 2022. [ abs ][ pdf ][ bib ]
Bounding the Error of Discretized Langevin Algorithms for Non-Strongly Log-Concave Targets Arnak S. Dalalyan, Avetik Karagulyan, Lionel Riou-Durand ; (235):1−38, 2022. [ abs ][ pdf ][ bib ]
KoPA: Automated Kronecker Product Approximation Chencheng Cai, Rong Chen, Han Xiao ; (236):1−44, 2022. [ abs ][ pdf ][ bib ]
Nonparametric Principal Subspace Regression Yang Zhou, Mark Koudstaal, Dengdeng Yu, Dehan Kong, Fang Yao ; (237):1−28, 2022. [ abs ][ pdf ][ bib ]
A Wasserstein Distance Approach for Concentration of Empirical Risk Estimates Prashanth L.A., Sanjay P. Bhat ; (238):1−61, 2022. [ abs ][ pdf ][ bib ]
Simple and Optimal Stochastic Gradient Methods for Nonsmooth Nonconvex Optimization Zhize Li, Jian Li ; (239):1−61, 2022. [ abs ][ pdf ][ bib ]
MALTS: Matching After Learning to Stretch Harsh Parikh, Cynthia Rudin, Alexander Volfovsky ; (240):1−42, 2022. [ abs ][ pdf ][ bib ] [ code ]
Weakly Supervised Disentangled Generative Causal Representation Learning Xinwei Shen, Furui Liu, Hanze Dong, Qing Lian, Zhitang Chen, Tong Zhang ; (241):1−55, 2022. [ abs ][ pdf ][ bib ] [ code ]
Bayesian Covariate-Dependent Gaussian Graphical Models with Varying Structure Yang Ni, Francesco C. Stingo, Veerabhadran Baladandayuthapani ; (242):1−29, 2022. [ abs ][ pdf ][ bib ]
Tree-based Node Aggregation in Sparse Graphical Models Ines Wilms, Jacob Bien ; (243):1−36, 2022. [ abs ][ pdf ][ bib ] [ code ]
Mitigating the Effects of Non-Identifiability on Inference for Bayesian Neural Networks with Latent Variables Yaniv Yacoby, Weiwei Pan, Finale Doshi-Velez ; (244):1−54, 2022. [ abs ][ pdf ][ bib ]
Mappings for Marginal Probabilities with Applications to Models in Statistical Physics Mehdi Molkaraie ; (245):1−36, 2022. [ abs ][ pdf ][ bib ]
Multivariate Boosted Trees and Applications to Forecasting and Control Lorenzo Nespoli, Vasco Medici ; (246):1−47, 2022. [ abs ][ pdf ][ bib ] [ code ]
Quantile regression with ReLU Networks: Estimators and minimax rates Oscar Hernan Madrid Padilla, Wesley Tansey, Yanzhen Chen ; (247):1−42, 2022. [ abs ][ pdf ][ bib ] [ code ]
Double Spike Dirichlet Priors for Structured Weighting Huiming Lin, Meng Li ; (248):1−28, 2022. [ abs ][ pdf ][ bib ] [ code ]
Projected Robust PCA with Application to Smooth Image Recovery Long Feng, Junhui Wang ; (249):1−41, 2022. [ abs ][ pdf ][ bib ]
Non-asymptotic Properties of Individualized Treatment Rules from Sequentially Rule-Adaptive Trials Daiqi Gao, Yufeng Liu, Donglin Zeng ; (250):1−42, 2022. [ abs ][ pdf ][ bib ]
Using Active Queries to Infer Symmetric Node Functions of Graph Dynamical Systems Abhijin Adiga, Chris J. Kuhlman, Madhav V. Marathe, S. S. Ravi, Daniel J. Rosenkrantz, Richard E. Stearns ; (251):1−43, 2022. [ abs ][ pdf ][ bib ]
A Closer Look at Embedding Propagation for Manifold Smoothing Diego Velazquez, Pau Rodriguez, Josep M. Gonfaus, F. Xavier Roca, Jordi Gonzalez ; (252):1−27, 2022. [ abs ][ pdf ][ bib ]
Greedification Operators for Policy Optimization: Investigating Forward and Reverse KL Divergences Alan Chan, Hugo Silva, Sungsu Lim, Tadashi Kozuno, A. Rupam Mahmood, Martha White ; (253):1−79, 2022. [ abs ][ pdf ][ bib ]
Adaptive Greedy Algorithm for Moderately Large Dimensions in Kernel Conditional Density Estimation Minh-Lien Jeanne Nguyen, Claire Lacour, Vincent Rivoirard ; (254):1−74, 2022. [ abs ][ pdf ][ bib ]
Simple Agent, Complex Environment: Efficient Reinforcement Learning with Agent States Shi Dong, Benjamin Van Roy, Zhengyuan Zhou ; (255):1−54, 2022. [ abs ][ pdf ][ bib ]
On Constraints in First-Order Optimization: A View from Non-Smooth Dynamical Systems Michael Muehlebach, Michael I. Jordan ; (256):1−47, 2022. [ abs ][ pdf ][ bib ] [ code ]
Sparse Continuous Distributions and Fenchel-Young Losses André F. T. Martins, Marcos Treviso, António Farinhas, Pedro M. Q. Aguiar, Mário A. T. Figueiredo, Mathieu Blondel, Vlad Niculae ; (257):1−74, 2022. [ abs ][ pdf ][ bib ] [ code ]
Tree-Based Models for Correlated Data Assaf Rabinowicz, Saharon Rosset ; (258):1−31, 2022. [ abs ][ pdf ][ bib ]
Learning Temporal Evolution of Spatial Dependence with Generalized Spatiotemporal Gaussian Process Models Shiwei Lan ; (259):1−53, 2022. [ abs ][ pdf ][ bib ] [ code ]
A proof of convergence for the gradient descent optimization method with random initializations in the training of neural networks with ReLU activation for piecewise linear target functions Arnulf Jentzen, Adrian Riekert ; (260):1−50, 2022. [ abs ][ pdf ][ bib ]
Auto-Sklearn 2.0: Hands-free AutoML via Meta-Learning Matthias Feurer, Katharina Eggensperger, Stefan Falkner, Marius Lindauer, Frank Hutter ; (261):1−61, 2022. [ abs ][ pdf ][ bib ] [ code ]
Estimation and inference on high-dimensional individualized treatment rule in observational data using split-and-pooled de-correlated score Muxuan Liang, Young-Geun Choi, Yang Ning, Maureen A Smith, Ying-Qi Zhao ; (262):1−65, 2022. [ abs ][ pdf ][ bib ] [ code ]
The Interplay Between Implicit Bias and Benign Overfitting in Two-Layer Linear Networks Niladri S. Chatterji, Philip M. Long, Peter L. Bartlett ; (263):1−48, 2022. [ abs ][ pdf ][ bib ]
A Random Matrix Perspective on Random Tensors José Henrique de M. Goulart, Romain Couillet, Pierre Comon ; (264):1−36, 2022. [ abs ][ pdf ][ bib ]
Stochastic subgradient for composite convex optimization with functional constraints Ion Necoara, Nitesh Kumar Singh ; (265):1−35, 2022. [ abs ][ pdf ][ bib ]
Functional Linear Regression with Mixed Predictors Daren Wang, Zifeng Zhao, Yi Yu, Rebecca Willett ; (266):1−94, 2022. [ abs ][ pdf ][ bib ] [ code ]
Tianshou: A Highly Modularized Deep Reinforcement Learning Library Jiayi Weng, Huayu Chen, Dong Yan, Kaichao You, Alexis Duburcq, Minghao Zhang, Yi Su, Hang Su, Jun Zhu ; (267):1−6, 2022. (Machine Learning Open Source Software Paper) [ abs ][ pdf ][ bib ] [ code ]
A Computationally Efficient Framework for Vector Representation of Persistence Diagrams Kit C Chan, Umar Islambekov, Alexey Luchinsky, Rebecca Sanders ; (268):1−33, 2022. [ abs ][ pdf ][ bib ]
Learning linear non-Gaussian directed acyclic graph with diverging number of nodes Ruixuan Zhao, Xin He, Junhui Wang ; (269):1−34, 2022. [ abs ][ pdf ][ bib ]
Minimax Mixing Time of the Metropolis-Adjusted Langevin Algorithm for Log-Concave Sampling Keru Wu, Scott Schmidler, Yuansi Chen ; (270):1−63, 2022. [ abs ][ pdf ][ bib ]
Fast Stagewise Sparse Factor Regression Kun Chen, Ruipeng Dong, Wanwan Xu, Zemin Zheng ; (271):1−45, 2022. [ abs ][ pdf ][ bib ]
Communication-Constrained Distributed Quantile Regression with Optimal Statistical Guarantees Kean Ming Tan, Heather Battey, Wen-Xin Zhou ; (272):1−61, 2022. [ abs ][ pdf ][ bib ]
The Weighted Generalised Covariance Measure Cyrill Scheidegger, Julia Hörrmann, Peter Bühlmann ; (273):1−68, 2022. [ abs ][ pdf ][ bib ]
CleanRL: High-quality Single-file Implementations of Deep Reinforcement Learning Algorithms Shengyi Huang, Rousslan Fernand Julien Dossa, Chang Ye, Jeff Braga, Dipam Chakraborty, Kinal Mehta, João G.M. Araújo ; (274):1−18, 2022. (Machine Learning Open Source Software Paper) [ abs ][ pdf ][ bib ] [ code ]
Policy Gradient and Actor-Critic Learning in Continuous Time and Space: Theory and Algorithms Yanwei Jia, Xun Yu Zhou ; (275):1−50, 2022. [ abs ][ pdf ][ bib ] [ code ]
Deep Network Approximation: Achieving Arbitrary Accuracy with Fixed Number of Neurons Shijun Zhang, Zuowei Shen, Haizhao Yang ; (276):1−60, 2022. [ abs ][ pdf ][ bib ]
Nonstochastic Bandits with Composite Anonymous Feedback Nicolò Cesa-Bianchi, Tommaso Cesari, Roberto Colomboni, Claudio Gentile, Yishay Mansour ; (277):1−24, 2022. [ abs ][ pdf ][ bib ]
Jump Gaussian Process Model for Estimating Piecewise Continuous Regression Functions Chiwoo Park ; (278):1−37, 2022. [ abs ][ pdf ][ bib ] [ code ]
Convergence Guarantees for the Good-Turing Estimator Amichai Painsky ; (279):1−37, 2022. [ abs ][ pdf ][ bib ]
Generalized Resubstitution for Classification Error Estimation Parisa Ghane, Ulisses Braga-Neto ; (280):1−30, 2022. [ abs ][ pdf ][ bib ]
Nonparametric adaptive control and prediction: theory and randomized algorithms Nicholas M. Boffi, Stephen Tu, Jean-Jacques E. Slotine ; (281):1−46, 2022. [ abs ][ pdf ][ bib ]
On the Convergence Rates of Policy Gradient Methods Lin Xiao ; (282):1−36, 2022. [ abs ][ pdf ][ bib ]
De-Sequentialized Monte Carlo: a parallel-in-time particle smoother Adrien Corenflos, Nicolas Chopin, Simo Särkkä ; (283):1−39, 2022. [ abs ][ pdf ][ bib ] [ code ]
Exact Partitioning of High-order Models with a Novel Convex Tensor Cone Relaxation Chuyang Ke, Jean Honorio ; (284):1−28, 2022. [ abs ][ pdf ][ bib ]
Deepchecks: A Library for Testing and Validating Machine Learning Models and Data Shir Chorev, Philip Tannor, Dan Ben Israel, Noam Bressler, Itay Gabbay, Nir Hutnik, Jonatan Liberman, Matan Perlmutter, Yurii Romanyshyn, Lior Rokach ; (285):1−6, 2022. (Machine Learning Open Source Software Paper) [ abs ][ pdf ][ bib ] [ code ]
Integral Autoencoder Network for Discretization-Invariant Learning Yong Zheng Ong, Zuowei Shen, Haizhao Yang ; (286):1−45, 2022. [ abs ][ pdf ][ bib ] [ code ]
Information-Theoretic Characterization of the Generalization Error for Iterative Semi-Supervised Learning Haiyun He, Hanshu Yan, Vincent Y. F. Tan ; (287):1−52, 2022. [ abs ][ pdf ][ bib ] [ code ]
ReservoirComputing.jl: An Efficient and Modular Library for Reservoir Computing Models Francesco Martinuzzi, Chris Rackauckas, Anas Abdelrehim, Miguel D. Mahecha, Karin Mora ; (288):1−8, 2022. (Machine Learning Open Source Software Paper) [ abs ][ pdf ][ bib ] [ code ]
Estimating Causal Effects under Network Interference with Bayesian Generalized Propensity Scores Laura Forastiere, Fabrizia Mealli, Albert Wu, Edoardo M. Airoldi ; (289):1−61, 2022. [ abs ][ pdf ][ bib ]
Regularized and Smooth Double Core Tensor Factorization for Heterogeneous Data Davoud Ataee Tarzanagh, George Michailidis ; (290):1−49, 2022. [ abs ][ pdf ][ bib ] [ code ]
Generalized Matrix Factorization: efficient algorithms for fitting generalized linear latent variable models to large data arrays Lukasz Kidzinski, Francis K.C. Hui, David I. Warton, Trevor J. Hastie ; (291):1−29, 2022. [ abs ][ pdf ][ bib ] [ code ]
Two-mode Networks: Inference with as Many Parameters as Actors and Differential Privacy Qiuping Wang, Ting Yan, Binyan Jiang, Chenlei Leng ; (292):1−38, 2022. [ abs ][ pdf ][ bib ]
Expected Regret and Pseudo-Regret are Equivalent When the Optimal Arm is Unique Daron Anderson, Douglas J. Leith ; (293):1−12, 2022. [ abs ][ pdf ][ bib ]
Linearization and Identification of Multiple-Attractor Dynamical Systems through Laplacian Eigenmaps Bernardo Fichera, Aude Billard ; (294):1−35, 2022. [ abs ][ pdf ][ bib ]
Semiparametric Inference For Causal Effects In Graphical Models With Hidden Variables Rohit Bhattacharya, Razieh Nabi, Ilya Shpitser ; (295):1−76, 2022. [ abs ][ pdf ][ bib ] [ code ]
Stable Classification Dimitris Bertsimas, Jack Dunn, Ivan Paskov ; (296):1−53, 2022. [ abs ][ pdf ][ bib ]
Handling Hard Affine SDP Shape Constraints in RKHSs Pierre-Cyril Aubin-Frankowski, Zoltan Szabo ; (297):1−54, 2022. [ abs ][ pdf ][ bib ] [ code ]
JsonGrinder.jl: automated differentiable neural architecture for embedding arbitrary JSON data Šimon Mandlík, Matěj Račinský, Viliam Lisý, Tomáš Pevný ; (298):1−5, 2022. (Machine Learning Open Source Software Paper) [ abs ][ pdf ][ bib ] [ code ]
Interpretable Classification of Categorical Time Series Using the Spectral Envelope and Optimal Scalings Zeda Li, Scott A. Bruce, Tian Cai ; (299):1−31, 2022. [ abs ][ pdf ][ bib ] [ code ]
More Powerful Conditional Selective Inference for Generalized Lasso by Parametric Programming Vo Nguyen Le Duy, Ichiro Takeuchi ; (300):1−37, 2022. [ abs ][ pdf ][ bib ] [ code ]
Theoretical Foundations of t-SNE for Visualizing High-Dimensional Clustered Data T. Tony Cai, Rong Ma ; (301):1−54, 2022. [ abs ][ pdf ][ bib ]
On Instrumental Variable Regression for Deep Offline Policy Evaluation Yutian Chen, Liyuan Xu, Caglar Gulcehre, Tom Le Paine, Arthur Gretton, Nando de Freitas, Arnaud Doucet ; (302):1−40, 2022. [ abs ][ pdf ][ bib ] [ code ]
Graph Partitioning and Sparse Matrix Ordering using Reinforcement Learning and Graph Neural Networks Alice Gatti, Zhixiong Hu, Tess Smidt, Esmond G. Ng, Pieter Ghysels ; (303):1−28, 2022. [ abs ][ pdf ][ bib ] [ code ]
Variational Inference in high-dimensional linear regression Sumit Mukherjee, Subhabrata Sen ; (304):1−56, 2022. [ abs ][ pdf ][ bib ]
Tree-Values: Selective Inference for Regression Trees Anna C. Neufeld, Lucy L. Gao, Daniela M. Witten ; (305):1−43, 2022. [ abs ][ pdf ][ bib ] [ code ]
Pathfinder: Parallel quasi-Newton variational inference Lu Zhang, Bob Carpenter, Andrew Gelman, Aki Vehtari ; (306):1−49, 2022. [ abs ][ pdf ][ bib ] [ code ]
Learning from Noisy Pairwise Similarity and Unlabeled Data Songhua Wu, Tongliang Liu, Bo Han, Jun Yu, Gang Niu, Masashi Sugiyama ; (307):1−34, 2022. [ abs ][ pdf ][ bib ] [ code ]
On Regularized Square-root Regression Problems: Distributionally Robust Interpretation and Fast Computations Hong T.M. Chu, Kim-Chuan Toh, Yangjing Zhang ; (308):1−39, 2022. [ abs ][ pdf ][ bib ]
The Separation Capacity of Random Neural Networks Sjoerd Dirksen, Martin Genzel, Laurent Jacques, Alexander Stollenwerk ; (309):1−47, 2022. [ abs ][ pdf ][ bib ]
Detecting Latent Communities in Network Formation Models Shujie Ma, Liangjun Su, Yichong Zhang ; (310):1−61, 2022. [ abs ][ pdf ][ bib ]
Toward Understanding Convolutional Neural Networks from Volterra Convolution Perspective Tenghui Li, Guoxu Zhou, Yuning Qiu, Qibin Zhao ; (311):1−50, 2022. [ abs ][ pdf ][ bib ] [ code ]
Nystrom Regularization for Time Series Forecasting Zirui Sun, Mingwei Dai, Yao Wang, Shao-Bo Lin ; (312):1−42, 2022. [ abs ][ pdf ][ bib ]
Intrinsic Dimension Estimation Using Wasserstein Distance Adam Block, Zeyu Jia, Yury Polyanskiy, Alexander Rakhlin ; (313):1−37, 2022. [ abs ][ pdf ][ bib ]
Oracle Complexity in Nonsmooth Nonconvex Optimization Guy Kornowski, Ohad Shamir ; (314):1−44, 2022. [ abs ][ pdf ][ bib ]
d3rlpy: An Offline Deep Reinforcement Learning Library Takuma Seno, Michita Imai ; (315):1−20, 2022. (Machine Learning Open Source Software Paper) [ abs ][ pdf ][ bib ] [ code ]
WarpDrive: Fast End-to-End Deep Multi-Agent Reinforcement Learning on a GPU Tian Lan, Sunil Srinivasa, Huan Wang, Stephan Zheng ; (316):1−6, 2022. (Machine Learning Open Source Software Paper) [ abs ][ pdf ][ bib ] [ code ]
Nonparametric Neighborhood Selection in Graphical Models Hao Dong, Yuedong Wang ; (317):1−36, 2022. [ abs ][ pdf ][ bib ]
Hamilton-Jacobi equations on graphs with applications to semi-supervised learning and data depth Jeff Calder, Mahmood Ettehad ; (318):1−62, 2022. [ abs ][ pdf ][ bib ] [ code ]
Self-Healing Robust Neural Networks via Closed-Loop Control Zhuotong Chen, Qianxiao Li, Zheng Zhang ; (319):1−54, 2022. [ abs ][ pdf ][ bib ] [ code ]
Network Regression with Graph Laplacians Yidong Zhou, Hans-Georg Müller ; (320):1−41, 2022. [ abs ][ pdf ][ bib ] [ code ]
On Low-rank Trace Regression under General Sampling Distribution Nima Hamidi, Mohsen Bayati ; (321):1−49, 2022. [ abs ][ pdf ][ bib ] [ code ]
Community detection in sparse latent space models Fengnan Gao, Zongming Ma, Hongsong Yuan ; (322):1−50, 2022. [ abs ][ pdf ][ bib ]
Convergence Rates for Gaussian Mixtures of Experts Nhat Ho, Chiao-Yu Yang, Michael I. Jordan ; (323):1−81, 2022. [ abs ][ pdf ][ bib ]
Improving Bayesian Network Structure Learning in the Presence of Measurement Error Yang Liu, Anthony C. Constantinou, Zhigao Guo ; (324):1−28, 2022. [ abs ][ pdf ][ bib ] [ code ]
On Mixup Regularization Luigi Carratino, Moustapha Cissé, Rodolphe Jenatton, Jean-Philippe Vert ; (325):1−31, 2022. [ abs ][ pdf ][ bib ]
Project and Forget: Solving Large-Scale Metric Constrained Problems Rishi Sonthalia, Anna C. Gilbert ; (326):1−54, 2022. [ abs ][ pdf ][ bib ] [ code ]
Kernel Autocovariance Operators of Stationary Processes: Estimation and Convergence Mattes Mollenhauer, Stefan Klus, Christof Schütte, Péter Koltai ; (327):1−34, 2022. [ abs ][ pdf ][ bib ]
Distributed Stochastic Gradient Descent: Nonconvexity, Nonsmoothness, and Convergence to Local Minima Brian Swenson, Ryan Murray, H. Vincent Poor, Soummya Kar ; (328):1−62, 2022. [ abs ][ pdf ][ bib ]
Joint Continuous and Discrete Model Selection via Submodularity Jonathan Bunton, Paulo Tabuada ; (329):1−42, 2022. [ abs ][ pdf ][ bib ]
ALMA: Alternating Minimization Algorithm for Clustering Mixture Multilayer Network Xing Fan, Marianna Pensky, Feng Yu, Teng Zhang ; (330):1−46, 2022. [ abs ][ pdf ][ bib ]
The Geometry of Uniqueness, Sparsity and Clustering in Penalized Estimation Ulrike Schneider, Patrick Tardivel ; (331):1−36, 2022. [ abs ][ pdf ][ bib ]
Maximum sampled conditional likelihood for informative subsampling HaiYing Wang, Jae Kwang Kim ; (332):1−50, 2022. [ abs ][ pdf ][ bib ]
Distributional Random Forests: Heterogeneity Adjustment and Multivariate Distributional Regression Domagoj Cevid, Loris Michel, Jeffrey Näf, Peter Bühlmann, Nicolai Meinshausen ; (333):1−79, 2022. [ abs ][ pdf ][ bib ]
Fully General Online Imitation Learning Michael K. Cohen, Marcus Hutter, Neel Nanda ; (334):1−30, 2022. [ abs ][ pdf ][ bib ]
Causal Aggregation: Estimation and Inference of Causal Effects by Constraint-Based Data Fusion Jaime Roquero Gimenez, Dominik Rothenhäusler ; (335):1−60, 2022. [ abs ][ pdf ][ bib ]
Faster Randomized Interior Point Methods for Tall/Wide Linear Programs Agniva Chowdhury, Gregory Dexter, Palma London, Haim Avron, Petros Drineas ; (336):1−48, 2022. [ abs ][ pdf ][ bib ]
Statistical Optimality and Computational Efficiency of Nystrom Kernel PCA Nicholas Sterge, Bharath K. Sriperumbudur ; (337):1−32, 2022. [ abs ][ pdf ][ bib ]
Interval-censored Hawkes processes Marian-Andrei Rizoiu, Alexander Soen, Shidi Li, Pio Calderon, Leanne J. Dong, Aditya Krishna Menon, Lexing Xie ; (338):1−84, 2022. [ abs ][ pdf ][ bib ]
Early Stopping for Iterative Regularization with General Loss Functions Ting Hu, Yunwen Lei ; (339):1−36, 2022. [ abs ][ pdf ][ bib ]
Fundamental Limits and Tradeoffs in Invariant Representation Learning Han Zhao, Chen Dan, Bryon Aragam, Tommi S. Jaakkola, Geoffrey J. Gordon, Pradeep Ravikumar ; (340):1−49, 2022. [ abs ][ pdf ][ bib ]
Information-theoretic Classification Accuracy: A Criterion that Guides Data-driven Combination of Ambiguous Outcome Labels in Multi-class Classification Chihao Zhang, Yiling Elaine Chen, Shihua Zhang, Jingyi Jessica Li ; (341):1−65, 2022. [ abs ][ pdf ][ bib ] [ code ]
SGD with Coordinate Sampling: Theory and Practice Rémi Leluc, François Portier ; (342):1−47, 2022. [ abs ][ pdf ][ bib ] [ code ]
Global Optimality and Finite Sample Analysis of Softmax Off-Policy Actor Critic under State Distribution Mismatch Shangtong Zhang, Remi Tachet des Combes, Romain Laroche ; (343):1−91, 2022. [ abs ][ pdf ][ bib ] [ code ]
Vector-Valued Least-Squares Regression under Output Regularity Assumptions Luc Brogat-Motte, Alessandro Rudi, Céline Brouard, Juho Rousu, Florence d'Alché-Buc ; (344):1−50, 2022. [ abs ][ pdf ][ bib ]
Constraint Reasoning Embedded Structured Prediction Nan Jiang, Maosen Zhang, Willem-Jan van Hoeve, Yexiang Xue ; (345):1−40, 2022. [ abs ][ pdf ][ bib ] [ code ]
Minimax optimal approaches to the label shift problem in non-parametric settings Subha Maity, Yuekai Sun, Moulinath Banerjee ; (346):1−45, 2022. [ abs ][ pdf ][ bib ]
Existence, Stability and Scalability of Orthogonal Convolutional Neural Networks El Mehdi Achour, François Malgouyres, Franck Mamalet ; (347):1−56, 2022. [ abs ][ pdf ][ bib ] [ code ]
Scalable Gaussian-process regression and variable selection using Vecchia approximations Jian Cao, Joseph Guinness, Marc G. Genton, Matthias Katzfuss ; (348):1−30, 2022. [ abs ][ pdf ][ bib ] [ code ]
OMLT: Optimization & Machine Learning Toolkit Francesco Ceccon, Jordan Jalving, Joshua Haddad, Alexander Thebelt, Calvin Tsay, Carl D Laird, Ruth Misener ; (349):1−8, 2022. (Machine Learning Open Source Software Paper) [ abs ][ pdf ][ bib ] [ code ]
Approximate Bayesian Computation via Classification Yuexi Wang, Tetsuya Kaji, Veronika Rockova ; (350):1−49, 2022. [ abs ][ pdf ][ bib ]
Metrics of Calibration for Probabilistic Predictions Imanol Arrieta-Ibarra, Paman Gujral, Jonathan Tannen, Mark Tygert, Cherie Xu ; (351):1−54, 2022. [ abs ][ pdf ][ bib ] [ code ]
A generalisable tool path planning strategy for free-form sheet metal stamping through deep reinforcement and supervised learning
- Open access
- Published: 22 April 2024
Cite this article
You have full access to this open access article
- Shiming Liu 1 ,
- Zhusheng Shi ORCID: orcid.org/0000-0002-3640-3958 1 ,
- Jianguo Lin 1 &
69 Accesses
Explore all metrics
Due to the high cost of specially customised presses and dies and the advance of machine learning technology, there is some emerging research attempting free-form sheet metal stamping processes which use several common tools to produce products of various shapes. However, tool path planning strategies for the free forming process, such as reinforcement learning technique, derived from previous path planning experience are not generalisable for an arbitrary new sheet metal workpiece. Thus, in this paper, a generalisable tool path planning strategy is proposed for the first time to realise the tool path prediction for an arbitrary sheet metal part in 2-D space with no metal forming knowledge in prior, through deep reinforcement (implemented with 2 heuristics) and supervised learning technologies. Conferred by deep learning, the tool path planning process is corroborated to have self-learning characteristics. This method has been instantiated and verified by a successful application to a case study, of which the workpiece shape deformed by the predicted tool path has been compared with its target shape. The proposed method significantly improves the generalisation of tool path planning of free-form sheet metal stamping process, compared to strategies using pure reinforcement learning technologies. The successful instantiation of this method also implies the potential of the development of intelligent free-form sheet metal stamping process.
Similar content being viewed by others
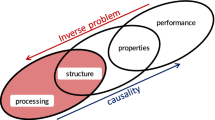
Deep reinforcement learning methods for structure-guided processing path optimization
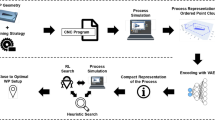
Deep representation learning and reinforcement learning for workpiece setup optimization in CNC milling
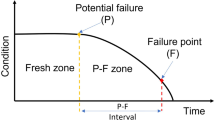
Deep Reinforcement Learning for autonomous pre-failure tool life improvement
Avoid common mistakes on your manuscript.
Introduction
Sheet metal components are nowadays ubiquitous in various industrial products, such as automobile, aircrafts and high-speed trains. Benefit from the short forming cycle of contemporary advanced sheet metal stamping techniques, which make it feasible for the mass production of lightweight sheet metals, the manufacturing budgets are constantly reduced, and a burgeoning era of industrialisation arises. However, the formed products from sheet metal stamping technology are subject to the unalterable shapes of punch and dies, for which the limited forming flexibility impedes the applicability of the off-the-shelf stamping equipment to new sheet metal components. In addition, the extraordinarily high capital cost for specialised punches and dies, especially for large-scale stamping, leads to expensive prototyping and arduous research and development of novel sheet metal designs. Thus, to extricate sheet metal manufacture from these constraints and to fulfill the requirement of high-volume personalised production in sheet metal forming industry nowadays (Bowen et al., 2022 ), flexible forming processes, which can change workpiece geometry without requiring different tool sets, were developed (Allwood & Utsunomiya, 2006 ). An emerging free-form sheet metal stamping technique was brought up (Liu et al., 2022 ), which consecutively deforms a sheet metal to its target shape from blank using several small-scale punch and dies of different shapes. In this regard, of particular concern is the generation and optimisation of the forming tool path which could yield the forming result comparable to the forming target.
Due to the forming characteristics of the traditional sheet metal stamping process, the sheet metal part is usually formed within a few or just one forming step, for which no research on tool path for stamping can be found. In sheet metal forming industry, most studies involving tool path generation and optimisation were performed for incremental sheet metal forming (ISF) process, which deforms sheet metal to its target shape with a sequence of incremental deformations. Attanasio et al. ( 2006 ) manually designed several tool paths for a two point ISF to manufacture an automotive part, by varying the step depth and scallop height. They found that setting low values of both these parameters can improve the final dimensional accuracy and surface quality. Similarly, Tanaka et al. ( 2005 ) manually generated tool paths for an incremental sheet punching (ISP) process based on the target workpiece CAD, tool shape, crossfeed, depth and tool path mode, of which the deformed workpiece had a maximum length of 76 mm. Azaouzi and Lebaal ( 2012 ) proposed a tool path optimisation strategy for single point ISF using the response surface method and sequential quadratic programming algorithm, which was tested for a spiral tool path and realised through finite element analysis (FEA). This method was reported to reduce the manufacturing time and improve the homogeneity of thickness distribution of asymmetric parts. Malhotra et al. ( 2011 ) proposed a tool path generation strategy to alleviate the unintentionally formed stepped features on the component base occurring in a multi-pass single point ISF process, by combining in-to-out and out-to-in tool paths for each intermediate shape. It was found that this strategy effectively reduced the occurrence of stepped features compared to pure out-to-in tool paths.
Over the past decade, machine learning technology has seen its unprecedented development in image recognition and natural language processing thanks to the remarkably increased computation power of central processing units (CPUs). Impressed by its extraordinary learning capability, researchers started to harness machine learning or deep learning technologies in sheet metal forming industry, such as ISF (Nagargoje et al., 2021 ). Most of them focused on process monitoring (Kubik et al., 2022 ), surrogate model for forming results prediction (Low et al., 2022 ) and process parameters prediction (Liu et al., 2021 ). Machine learning is well known through three categories of techniques (Monostori et al., 1996 ): supervised learning (SL), unsupervised learning, and reinforcement learning (RL). With regard to forming tool path planning, most applications exploited supervised and reinforcement learning techniques. Opritescu and Volk ( 2015 ) and Hartmann et al. ( 2019 ) utilised supervised learning neural networks for optimal tool path prediction for 2-D and 3-D automated driving processes (Kraftforming), respectively. Curvature distribution on target workpiece surface was computed as inputs, and they reported that the careful workpiece digitisation was of great importance to achieve good learning efficiency. The tool path for automated wheeling process was predicted by Rossi and Nicholas ( 2018 ) using fully convolutional network (FCN), with 75% prediction accuracy. Störkle et al. ( 2019 ) used linear regressor, decision tree, random decision forest, support vector machine and Gaussian process regressor to predict the optimal local support force and support angle distribution along a tool path in an ISF process. Liu et al. ( 2022 ) developed a recursive tool path prediction framework for a rubber-tool forming process, which embedded a deep supervised learning model for tool path planning. They compared the performance of three series of state-of-the-art models, including single CNNs, cascaded networks and convolutional long short-term memory (LSTM) models in tool path learning, from which the convolutional LSTM was reported to be the most superior. Compared to supervised learning, reinforcement learning applications to tool path planning of sheet metal forming process have been significantly ignored. This could be due to the expensive acquisition of computational or experimental data for RL algorithms training. Störkle et al. ( 2016 ) proposed a RL-based approach for the tool path planning and adjustment of an ISF process, which increased the geometric accuracy of the formed part. Liu et al. ( 2020 ) used a reinforcement learning algorithm, namely deep Q-learning, for the tool path learning of a simple free-form sheet metal stamping process. The FE computation was interfaced to the Q-learning algorithm as the RL environment, which provided real-time forming data for algorithm training.
Although there have been numerous studies of tool path planning for various sheet metal forming processes, they all have a common issue in generalising the methods to completely different target workpiece shapes, which hinders the widespread applications of machine learning based tool path planning strategies. In other words, new data have to be acquired to train the machine learning models or algorithms again to have a good prediction accuracy for different target, especially for approaches exploiting reinforcement learning. Generalisation gap is a common issue in RL applications (Kirk et al., 2021 ), which is a challenge under constant research. An evident reason leading to its inferior generalisation is that the data collected during RL training are mostly lying on the path towards a certain optimisation target. With a completely different target, the model would fail in generalisation since it was trained without useful data towards the new target.
Table 1 briefly compares the methods introduced above in tool path planning and summarises their deficiency in terms of real-world application. “Curse of dimensionality” indicates that the method can be error-prone once the target workpiece shape becomes complex, since the available data would become sparse and exponentially increased training data is required to obtain a reliable prediction result.
The aim of this research is to explore the generalisation of deep learning technologies in forming tool path planning for a 2-D free-form sheet metal stamping process. A generalisable tool path planning strategy, through the design of deep reinforcement and deep supervised learning technologies at different stages, was proposed in this paper. In this strategy, RL was used to explore the optimal tool paths for the target workpiece, with which the efficient tool path for a certain group of workpieces was learned using SL. With no metal forming knowledge in prior, the path planning process was corroborated to possess self-learning characteristics, from which the path planning results can be self-improved over time. The generalisation of this strategy was realised by factorising the entire target workpiece into several segments, which were classified into three groups. The optimal tool paths for several typical workpiece segments from each group were learned from scratch through deep reinforcement learning, and deep supervised learning models were used to generalise the intrinsic forming pattern of each group of segments. Six deep RL algorithms, from two different categories, were compared regarding their tool path learning performance for the free-form stamping process. The RL process was enhanced with the introduction of two forming heuristics. Three deep SL models were trained with two tool path datasets consist of different data amount and their performance were evaluated in terms of forming goal achievement and the dimension error of the deformed workpiece, and the forming results from a pure reinforcement learning method were also presented as comparisons. At last, a case study was performed to verify the generalisable tool path planning strategy with a completely new target workpiece.
The main contributions of this work are as following: 1) developing a generalisable tool path planning strategy for arbitrary 2-D free-formed sheet metal components for the first time, which successfully integrated deep RL and SL algorithms to learn and generalise efficient forming paths, and validating through a case study; 2) analysing a free-form rubber-tool forming process and discovering 2 close punch effects; 3) quantitatively analysing the performance of 6 deep RL algorithms and 3 deep SL models on tool path learning and generalisation, respectively. In addition, two heuristics were derived from real-world empirical experience and have been demonstrated to significantly facilitate the tool path learning process.
Methodology
In this section, the application of the proposed tool path planning strategy was first introduced in " Free-form stamping test and digitisation of forming process " section, followed by the detailed illustration of the generalisable tool path planning strategy in " Generalisable tool path planning strategy " section. " Forming goal and forming parameters design " section presents the forming goal that the strategy needs to achieve and the forming parameters to be selected. " Deep reinforcement learning algorithms and learning parameters " section and 2.5 illustrate the designation details of the RL and SL algorithms, respectively.
Free-form stamping test and digitisation of forming process
A rubber-tool forming process proposed in the Authors’ previous research (Liu et al., 2022 ) was adopted to consecutively deform a sheet metal while retaining a sound surface condition during the forming process. From the test setup and FE model shown in Fig. 1 , the workpiece was deformed by a rubber-wrapped punch on a workbench rubber. The specification of the setup is summarised in Table 2 . The deformation was accomplished by translating the punch towards the workpiece along Y-axis and lifting it up, considering springback. The workpiece was consecutively deformed at different locations towards its target shape. At each step of the free forming process, the workpiece was repositioned through rotation and translation to relocate the punch location, the details of which can be found in (Liu et al., 2022 ). The deformation process was set up, for simplicity, in 2-D space and computationally performed with Abaqus 2019. The FE plain strain model was configured with the material of AA6082 for the workpiece and natural rubber for the punch rubber and workbench rubber, with details in (Liu et al., 2022 ). Mesh for the workpiece was of size 0.1 mm, with 17,164 elements in total.
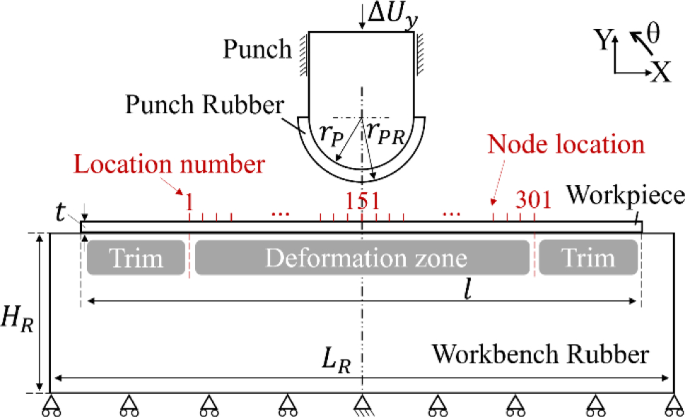
Test setup and FE model for the rubber-tool forming. The lengths of the deformation and trim zones are 30 and 10 mm, respectively
To realise the free forming process in FE simulations, the forming process was digitised and standardised for precise process control. As shown in Fig. 1 , the workpiece was divided into two zones, namely deformation zone and trim zone, with lengths of 30 and 10 mm, respectively. The punch could only work on the deformation zone, and the trim zone would be trimmed after the deformation had completed. The trim zone was reserved without deformation due to that the significant shear force from the edge of the workpiece could easily penetrate the workbench rubber, which would cause non-convergence issue in FE computation. The deformation zone was marked by 301 node locations, numbered from left to right, which are consistent with the mesh node locations.
To quantitatively observe and analyse the workpiece shape, a curvature distribution ( \(\varvec{K}\) ) graph was generated to represent the shape of the workpiece deformation zone, as shown in Fig. 2 . The local curvature \(K\) of a point on the workpiece was calculated by Menger curvature, which is the reciprocal of the radius of the circle passing through this point and its two adjacent points. Thus, a total of 303 mesh nodes on the top surface of the workpiece were used to generate the \(\varvec{K}\) -graph, including 301 nodes in the deformation zone and one additional node next to each end of the deformation zone. Using 0.1 mm of interval distance between each two contiguous node locations, the workpiece shape can be regenerated from its \(\varvec{K}\) -graph.
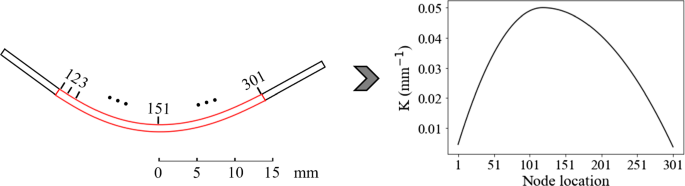
Example of workpiece shape (left) and its curvature \(\varvec{K}\) distribution along node locations (right). The region highlighted in red in the drawing denotes the deformation zone, and the \(\Delta \varvec{K}\) -graph is generated from the workpiece top surface of this zone
Generalisable tool path planning strategy
The proposed generalisable tool path planning strategy works by segmenting the target workpiece, based on the shape of three groups of segments classified in prior, into a few segments whose subpaths are generated through deep learning approach. The entire tool path for the target workpiece would be acquired by aggregating the subpaths for all workpiece segments. By classifying common groups of segments with the same shape features, any arbitrary workpiece can be regarded as assembled by segments from these groups. From the theoretical perspective, through dynamic programming, the tool path learning complexity for a complete workpiece was reduced to simpler subproblems of path learning for each group of workpiece segments. As the segments in each group are highly correlated in shape, the tool path learning for each segment group is significantly more generalisable than that for arbitrary workpieces. From the empirical perspective, representative groups of workpiece segments are finite, while there are infinite number of possible target workpiece shapes. After studying the efficient forming path for each segment group, the tool path for any arbitrary workpiece can be obtained by aggregating the tool path for all its segments, which yields the superior generalisability of this strategy.
To quantitatively measure the shape difference between the target and current workpiece, a curvature difference distribution graph ( \(\Delta \varvec{K}\) -graph) was generated by subtracting the current \({\varvec{K}}_{C}\) -graph from the target \({\varvec{K}}_{T}\) -graph to represent the workpiece state, as shown in Fig. 3 . The current workpiece was considered to be close to its target shape if the value of \(\Delta \varvec{K}\) approaches zero at any point along the longitudinal length. From the example in Fig. 3 , the \(\Delta \varvec{K}\) -graph was split into 6 segments, A-F. Through the segmental analysis of the \(\Delta \varvec{K}\) -graphs of real-world components (e.g. aerofoil), three groups of segments were classified, of which any arbitrary \(\Delta \varvec{K}\) -graph can be composed. Groups 1 and 2 consist of half-wave shaped and quarter-wave shaped segments, and Group 3 includes constant-value segments representing circular arcs or flat sheet.
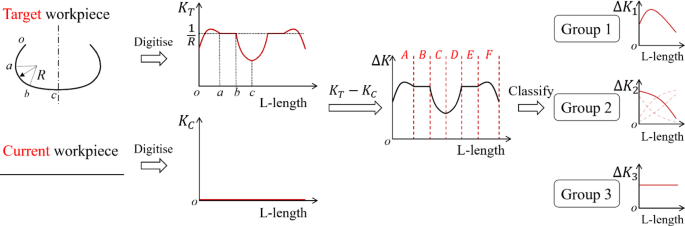
Schematic digitisation procedure for workpiece state representation \(\Delta \varvec{K}\) -graph and the classification of three groups of segments. The drawings for target and current workpieces depict their top surfaces. The dashed lines in Group 2 signify other segments having the same shape features as the solid line, which are also counted in this group. L-length denotes longitudinal length
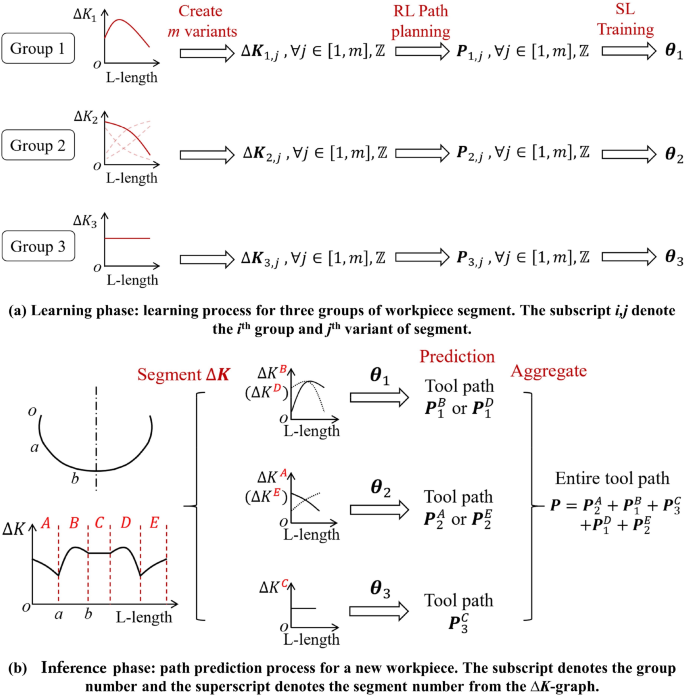
The generalisable tool path planning strategy through deep reinforcement and supervised learning
There are two phases in the generalisable tool path planning strategy, learning phase and inference phase, as shown in Fig. 4 . At the learning phase, for each group of segments, m variants of \(\Delta \varvec{K}\) -graphs, \(\Delta {\varvec{K}}_{i,j}\) , were created as shown in Fig. 4 a, where i is the group number and j is the variant number. The tool path, \({\varvec{P}}_{i,j}\) , for each of the variant of segment in each group was then learned and planned through deep reinforcement learning, without any path planning experience in prior. After the tool paths for all segments were obtained, a deep supervised learning model was trained with the tool path data, \({\varvec{P}}_{i,j}\) , for each group to generalise the efficient tool path patterns for segments from each group.
At the inference phase, as shown in Fig. 4 b, a new workpiece was firstly digitised to the \(\Delta \varvec{K}\) -graph and segmented in accordance with the three groups. Five segments, A-E, were obtained in this example, and their tool paths were predicted using the deep supervised learning model trained for their particular groups at the learning phase, respectively. At last, the entire tool path for the workpiece was obtained by aggregating all the tool path for each segment. To sum up, the RL and SL algorithms were utilised for different purposes in this strategy. The RL model explored the optimal tool path for each single target workpiece, which was used as the training data of the SL models to learn the efficient forming pattern for a group of workpieces with common features. In application, only SL models were used to infer the tool path of a new workpiece.
In the segmental analysis of the \(\Delta \varvec{K}\) -graphs, taking the workpiece in Fig. 4 b as an example, one can easily find that most segments are from Group 1. The segments from Group 2 can only be seen at the two ends of the components, and the segments from Group 3 only exist in workpiece with circular arc. Thus, Group 1 was used for instantiation of the generalisable tool path planning strategy, and a total of 25 variants of segments in this group were arbitrarily created through the method in Appendix A.
Forming goal and forming parameters design
In the context of the free-form sheet metal stamping test setup presented in Fig. 1 , at each step of the forming process, the stamping outcome is determined by the punch location and punch stroke. However, the large amount of punch location options, 301 in total, would incur considerably vast search space for the tool path planning problem. Thus, to simplify the problem, a forming heuristic (Heuristic 1) was applied to this forming process, which is in conformity with practical forming scenario, to allow the node location that had the most salient shape difference from the target workpiece to be selected at each forming step. In a word, the node location where the value of \(\Delta \varvec{K}\) is highest in the \(\Delta \varvec{K}\) -graph was selected at each step.
As the workpiece shape is close to its target when the \(\Delta \varvec{K}\) approaches zero at any point along the longitudinal length, the goal of the free forming in this research was considered to be achieved if \(\text{max}\left(\left|\Delta \varvec{K}\right|\right)\le 0.01\) mm −1 . Thus, in order to determine an appropriate range of punch stroke values to select during deformation, by which the forming goal is possible to achieve within a relatively small search space, a preliminary study was performed to investigate the free forming characteristics. Two phenomena, namely close punch effect 1 and 2 (CPE1 and CPE2), were discovered in this study, which are shown in Fig. 5 and Fig. 6 .
From Fig. 5 , the \(\Delta \varvec{K}\) -graphs of three workpieces before and after the same punch with stroke of 3.0 mm at the location of 151 are shown. The three workpieces had been consecutively deformed by 2, 3 and 4 punches in the vicinity of this node location, as shown respectively in Fig. 5 a, b and c. It can be seen that more prior deformation underwent near the node location of interest, less deformation was resulted in, i.e., larger punch stroke was required to accomplish a certain change of shape at this location. This phenomenon was named CPE1, which barely escalated with more than 4 punches in prior.
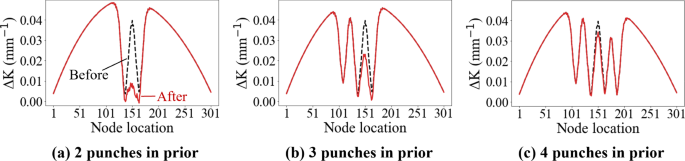
Close punch effect 1 on the punch with stroke of 3.0 mm at the location of 151. a , b and c present the \(\Delta \varvec{K}\) -graphs before and after this punch on the workpiece which has been consecutively deformed, in prior, by 2, 3 and 4 punches, respectively
Figure 6 shows the \(\Delta \varvec{K}\) -graphs of two workpieces before and after 1 punch and 50 punches, respectively. From Fig. 6 a, it can be seen that the \(\Delta \varvec{K}\) values around node location of 118 was decreased by about 0.002 mm −1 after deformation applied to location of 132, although the punch at the latter location had less effect on the \(\Delta \varvec{K}\) value than that at the former location did. From Fig. 6 b, it can be seen that the workpiece had been deformed at location of 118 since the 2nd step, and the \(\Delta \varvec{K}\) value at this location was affected by the punches nearby in the following 50 steps and decreased by about 0.008 mm −1 . This phenomenon was named CPE2, whose area of influence covers approximately 5 mm (about 50 node locations) around the node location.
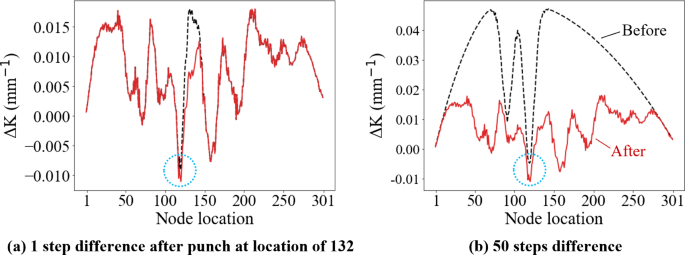
Close punch effect 2 from the punches near location of 118. a and b present the \(\Delta \varvec{K}\) -graphs before and after 1 punch and 50 punches, respectively. The area highlighted by dashed circle is where CPE2 was found
From the analysis above, it was found that a stroke of 2.1 mm can reach the forming goal at the 1st punch (with no CPE), and that of at least 3.6 mm was needed to overcome CPE2 and reach the forming goal. Thus, 19 options of punch strokes, ranging from 2.1 to 3.9 mm in 0.1 mm increments, were determined.
Deep reinforcement learning algorithms and learning parameters
Reinforcement learning is a technology which learns the optimal control strategy through active trial-and-error interaction with the problem environment. A reward is delivered by the environment as feedback for each interaction, and the goal of reinforcement learning is to maximise the total rewards. Almost all RL problems can be framed as a Markov Decision Process (MDP), which is defined as (Sutton & Barto, 2017 ):
where \(S\) is a set of possible states, \(\mathcal{A}\) is a set of possible actions, \(R\) is the reward function, \(P\) is the transition probability function and \(\gamma\) is the discounting ratio ( \(\gamma \in \left[\text{0,1}\right]\) ). In this research, \(S\) includes the workpiece state representation \(\Delta \varvec{K}\) and \(\mathcal{A}\) includes the options of punch stroke. \(P\) is unknown in this research problem, for which model-free RL algorithms are to be applied. The bold capital characters here are used to distinguish them from scalar values in the subsequent equations, such as state or action at a single step.
With the terms introduced above, the RL process can be briefly illustrated with a loop: from the state \({s}_{t}\) at time t , an action \({a}_{t}\) is based on the current policy, which leads to the next state \({s}_{t+1}\) and a reward \({r}_{t}\) for this step. To measure the goodness of a state, state-value and action-value (also called Q-value) are commonly used, which are respectively defined as follows (Sutton & Barto, 2017 ):
where \(\mathbb{E}\) denotes expectation and \(\pi\) denotes policy. The term \(\sum _{t=0}^{\infty }{\gamma }^{t}{r}_{t}|{s}_{t},\pi\) is the cumulative future rewards under policy \(\pi\) from t , known as return, of which the superscript and subscript denote exponent and time step, respectively. Thus, the optimal policy \({\pi }^{*}\) is achieved when the value functions produce the maximum return, \({V}^{*}\left({s}_{t}\right)\) and \({Q}^{*}\left({s}_{t},{a}_{t}\right)\) .
Using Bellman’s Equation (Sutton & Barto, 2017 ), which decompose the value functions to immediate reward plus the discounted future rewards, the optimal value functions can be iteratively computed for every state to obtain the optimal policy:
Two categories of RL algorithms were investigated in this research, namely value-based and policy-based approaches. When value functions, Eqs. ( 2 ) and ( 3 ), are approximated with neural network, traditional RL becomes deep reinforcement learning (DRL). For the value-based approaches, three Q-learning algorithms, namely deep Q-learning, double deep Q-learning and dueling deep Q-learning, were implemented. For the policy-based approaches, three policy gradient algorithms, namely Advantage Actor-Critic (A2C), Deep Deterministic Policy Gradient (DDPG) and Proximal Policy Optimisation (PPO), were implemented.
As shown in Eq. ( 5 ), the optimal policy is obtained by iteratively updating the Q-value function for each state-action pair. However, it is computationally infeasible to compute them all when the entire state and action space becomes enormous. Thus, Q-learning algorithm (Mnih et al., 2015 ) was brought up to estimate the Q-value function using a function approximator. Three function approximators were investigated in this study: Deep Q-Network (DQN), Double Deep Q-Network (Double-DQN) and Dueling Deep Q-Network (Dueling-DQN), whose objective functions can be found in existing works (Mnih et al., 2015 ; van Hasselt et al., 2016 ; Wang et al., 2015 ). It is noted that Double-DQN alleviates the Q-value overestimation problem for DQN by decomposing the max operation in the target Q-value into two operations of action selection and action evaluation. The Dueling-DQN specifically models the advantage-value, which measures the goodness of an action at a certain state and is arithmetically related to state-value and action-value by \(Q\left(s,a\right)=V\left(s\right)+A\left(s,a\right)\) .
Unlike Q-learning which achieves optimal policy by learning the optimal value functions, policy gradient algorithms parameterise the policy with a model and directly learn the policy. The objective function of policy gradient algorithms is configured to be the expected total return as shown by Eqs. ( 2 ) and ( 3 ), and the goal of the optimisation is to maximise the objective function. Through gradient ascent , the policy model which produces the highest return yields the optimal policy. Most of the policy gradient algorithms have the same theoretical foundation, Policy Gradient Theorem , which is defined in (Sutton & Barto, 2017 ).
The three policy gradient algorithms investigated in this research, A2C, DDPG and PPO, all use an Actor-Critic method (Sutton & Barto, 2017 ) for policy update, of which the critic model is used for value functions evaluation to assist the policy update and the actor model is used for policy evaluation which is updated in the direction suggested by the critic. The objective functions can be found in existing works (Mnih et al., 2016 ; Lillicrap et al., 2015 ; Schulman et al., 2017 ), of which DDPG was specially developed for problems with continuous action space. The A2C algorithm used an advantage term to assist the policy update, while DDPG using the gradient of Q-value with respect to the action and PPO uses Generalised Advantage Estimate (GAE) (Schulman, Moritz, et al., 2015 ). For A2C, the temporal difference was selected for the advantage estimate through a preliminary study compared with the Monte Carlo (MC) method. The PPO algorithm is a simplified version of Trust Region Policy Optimisation (TRPO) (Schulman et al., 2015a , 2015b ) by using a clipped objective function to prevent from extremely large online policy updates and learning instability. The hyperparameters in the PPO algorithm, future advantage discounting ratio and clip ratio, were set as 0.95 and 0.2 in this study following the original work (Schulman et al., 2017 ).
Learning setup and hyperparameters
For RL environment, Abaqus 2019 was interfaced with the RL algorithms to supply computation results during learning. The transient \(({s}_{t},{a}_{t},{r}_{t},{s}_{t+1})\) was formulated as follow:
The state/next state was in the form of workpiece state representation \(\Delta \varvec{K}\) -graph and a one-hot vector of size 1 × 301 indicating the punch location. Thus, it represents the shape difference between the current workpiece shape and its target shape, which can be easily used to construct the reward function. The one-hot vector was generated following Heuristic 1.
The action was the punch stroke, ranging from 2.1 to 3.9 mm (19 in total for discrete action space).
The reward was defined to measure the goodness of the selected action at given state, whose evaluation is shown in Fig. 7 . After each action at a given state, the reward was determined by the punch effectiveness ratio, which was defined as the ratio of the punch effect on \(\Delta \varvec{K}\) at given location at time step t ( \({p}_{t}\) ) to the expected effect at this location ( \({p}_{o}\) ), with the function \(r_{t} = 2\left( {p_{t} /p_{o} } \right)^{2} - 3\) (except for PPO: \({r}_{t}=2{\left({p}_{t}/{p}_{o}\right)}^{2}\) ). An exponential function was used to discourage non-effective punch, since the reward hardly changed at a low punch effectiveness ratio. If the workpiece was overpunched, the \(\Delta \varvec{K}\) at the punch location below the lower threshold \(-0.01 {mm}^{-1}\) , \({r}_{t}=-100\) (PPO: \({r}_{t}=-1\) and DDPG: \({r}_{t}=-\) 3); if the forming goal was achieved, i.e. \(\text{max}\left(\left|\Delta \varvec{K}\right|\right)\le 0.01 {mm}^{-1}\) , \({r}_{t}=0\) (PPO: \({r}_{t}=500-2.5\,\times\) episode step). Negative rewards were used for each step to penalise unnecessary steps, except for PPO where unnecessary steps were penalised by rewarding early termination. A reward of -3 was assigned for overpunch in DDPG learning rather than -100 since it was found that sparse rewards can cause failures in DDPG training (Matheron et al., 2019 ).
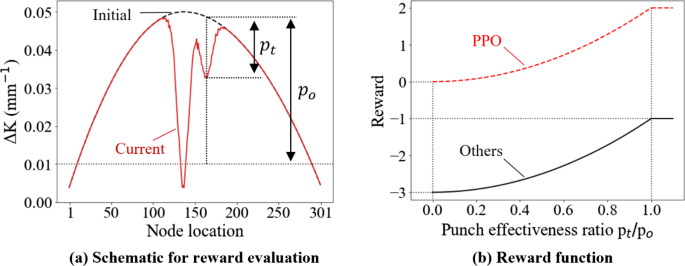
Reward function and its evaluation for each action. a shows the reward evaluation method for punch stroke of 2.2 mm at location of 162, and the two lines denote the initial and current \(\Delta \varvec{K}\) -graph; b shows the reward function for evaluation
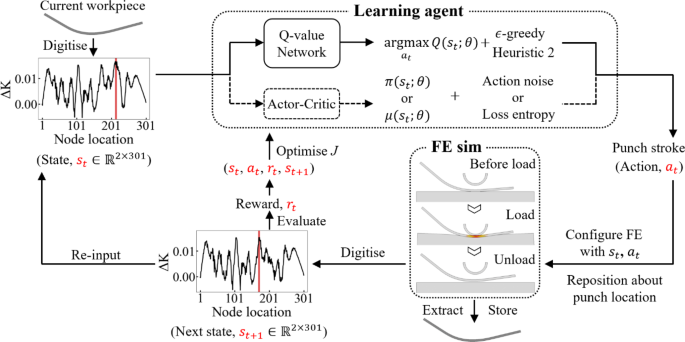
The reinforcement learning process for the tool path learning of the rubber-tool forming process, of which the FE simulation (FE sim) was used as the RL environment to provide real-time deformation results. The vertical line in the \(\Delta \varvec{K}\) -graph denotes the punch location
Figure 8 presents the reinforcement learning process configured for the tool path learning purpose, using FE simulations as the RL environment. The RL process collected data in a loop, starting from digitising the workpiece geometry to the state \({s}_{t}\) and feeding it to the learning agent. The learning agent predicted the stroke \({a}_{t}\) based on the current policy and exploration scheme. The FE simulation was configured by repositioning the current workpiece about the punch location and setting up the selected punch stroke, and the deformed workpiece geometry was extracted and stored. The deformed geometry was also digitised to obtain the next state \({s}_{t+1}\) , with which the reward \({r}_{t}\) was evaluated through the reward function. The collected transient \(({s}_{t},{a}_{t},{r}_{t},{s}_{t+1})\) at this time step was then used to optimise the objective function J and update the agent policy. The RL loop ended by re-inputting the next state to the agent as the state in the next loop.
The learning methods for the six RL algorithms, which are all model-free algorithms, are shown in Table 3 . The off-policy algorithms were trained with experience replay, of which all learning histories were stored to be uniformly sampled in minibatch for training, while the on-policy algorithms were trained with the immediate experience. Target network was used for action evaluation, which was updated by the online network periodically for stable learning progress. For exploration and exploitation, the Q-learning algorithms adopted \(\varepsilon\) -greedy policy, while A2C used an additional entropy term from (Williams & Peng, 1991 ) in the loss function and DDPG used a Gaussian distributed action noise. In addition to above, a forming heuristic (Heuristic 2) was developed to facilitate the learning process, which was defined as follows: the choice of the stroke at the current node location, if applicable, cannot be less than previous choices at the same location in one run, otherwise a larger value of stroke was randomly selected for this location. This heuristic was only applied in addition to \(\varepsilon\) -greedy policy as they have the same exploration mode, which would not disturb the training data structure.
The learning hyperparameters for RL are summarised in Table 4 . The maximum step per episode signifies the maximum forming step allowed for each run of the free forming trial. The episode would end if any of the following conditions was met: 1) forming goal achieved, 2) overpunch and 3) maximum step per episode (step/ep) attained. It is noted that the target network for Q-learning was updated every 20 learning steps, while that for DDPG is softly updated with \(\tau =0.01\) and that for PPO is updated every rollout (512 steps in this research) of the online policy. For \(\varepsilon\) -greedy policy, the value of \(\varepsilon\) decays from 1.0 to 0.1.
With regard to the models used for value function and policy function approximations in all six algorithms, the learning performance of a shallow multilayer perceptron (MLP) and a convolutional neural network (CNN) were compared as in (Lillicrap et al., 2015 ). Rectified linear unit ( ReLU ) was used for all hidden layers. There was no activation function for the output layer of the value network, while softmax and tanh were used for that of the policy network, respectively. The MLP had 2 hidden layers with 400 and 200 units, respectively (164,819 parameters). The network had 2 inputs, the \(\Delta \varvec{K}\) -graph and the one-hot vector for punch location, each followed by a half layer of neurons in the 1st layer before they were added together and fed into the 2nd layer. The CNN had the same architecture with the one used in (Mnih et al., 2015 ), with an additional hidden layer with 512 units, for the 2nd input, parallel to the last layer of the convolutional layers (1,299,891 parameters).
Virtual environment for RL algorithms comparison
Subject to the FE computational speed, it is considerably time-consumptive to test the feasibility of tool path learning for the free forming process using RL. Thus, a virtual environment was developed to imitate the rubber-tool forming behaviour by having similar punch effects on the \(\Delta \varvec{K}\) -graph to those computed by FE simulations, with which the performances of the six RL algorithms in tool path learning were compared. The virtual environment was composed to also manifest CPE1 and CPE2 as presented in " Forming goal and forming parameters design " section, and the effect of stroke value on the \(\Delta \varvec{K}\) -graph was also imitated by the virtual environment through a parametric study. The detailed setting of the virtual environment is presented in Appendix B.
Deep supervised learning models and training methods
After the optimal tool paths for the 25 variants of workpiece segments in Group 1 were acquired from deep reinforcement learning, they were used to train deep supervised learning models to learn the efficient tool path patterns for this group.
Deep neural networks
Three deep neural networks (DNNs), namely single CNN, cascaded networks and CNN LSTM, had been compared in predicting the tool path through a recursive prediction framework in the Authors’ previous research (Liu et al., 2022 ). Since the results revealed that the performance of CNN LSTM preceded that of the other two models, CNN LSTM was adopted in this research, with VGG16 (Simonyan & Zisserman, 2015 ), ResNet34 and ResNet50 (He et al., 2016 ) as the feature extractor, respectively. The model architectures for these models were the same as those used in (Liu et al., 2022 ), with a simple substitution of feature extractor with ResNet34 and ResNet50. The input to the LSTM was the partial forming sequence made up of the concatenation of the \(\Delta \varvec{K}\) -graph and the punch location vector for each time step, and the output from the model was the punch stroke prediction for the coming step. As the target workpiece information is already contained in the \(\Delta \varvec{K}\) -graph, it was not fed into the model as a 2nd input, different from (Liu et al., 2022 ).
Training method and hyperparameters
The DNNs were compiled in Python and trained using Keras with TensorFlow v2.2.0 as backend, and the computing facility had a NVIDIA Quadro RTX 6000 GPU with 24 GB of RAM memory. The training data for DNNs were all the tool paths learned from the RL algorithm, which were pre-processed to conform to the LSTM models and the labels (output features) were standardised to comparable scales. The tool path prediction with DNNs was configured to be a regression problem, for which the Mean Square Error (MSE) (Goodfellow et al., 2016 ) was the objective function for DNN training. Adam algorithm (Kingma & Ba, 2015 ), with default values of hyperparameters ( \({\beta }_{1},{\beta }_{2},\varepsilon\) ) in Keras, was used for optimisation. In addition, the learning rate \(\eta\) was set to exponentially decaying, from the initial learning rate \({\eta }_{0}\) , along with training process, with the same decaying rate and decaying steps as in (Liu et al., 2022 ). The key training parameters are shown in Table 5 , in which two amounts of training data are presented.
Learning results and discussions
Selection of reinforcement learning algorithm.
Two categories of reinforcement learning algorithm, namely Q-learning and policy gradient algorithms, were compared in terms of their performances in tool path learning for the rubber-tool forming process. Subjected to the prohibitively expensive FE computation, a virtual environment was developed to imitate the rubber-tool forming behaviour as introduced in " Virtual environment for RL algorithms comparison " section. A total of six RL algorithms were investigated with the data generated by the virtual environment, half of which belong to Q-learning and the other half belong to policy gradient method. The most superior one determined in this study is to be implemented with FE environment and learn the optimal tool path using FE computational data. The same target workpiece, as shown in Fig. 2 , was used for tool path learning in this study.
The learning setups for all algorithms, including the transient \(({s}_{t},{a}_{t},{r}_{t},{s}_{t+1})\) , learning method and hyperparameters, are summarised in Section 2.4.2. An additional exploration rule, namely Heuristic 2, was implemented along with the \(\varepsilon\) -greedy policy for Q-learning algorithms. Figure 9 shows the performances of DQN, Double-DQN and Dueling-DQN trained under the exploration scheme with and without Heuristic 2, of which the termination step signifies the total punch steps spent to achieve the forming goal. The same learning rate (1 × 10 −2 ) and value function approximator (CNN) were applied to each training. It can be seen that the average termination step was reduced by approximately 40%, from 62 to 37, after introducing Heuristic 2 for exploration in the training of each algorithm. In addition, with Heuristic 2, the first applicable tool path was found more quickly in each case than those without Heuristic 2 by 9K, 2K and 3K training steps, respectively. Thus, because of the consistent improvement of learning efficiency from Heuristic 2 in each case, it was implemented for the training of all the Q-learning algorithms for the following results.
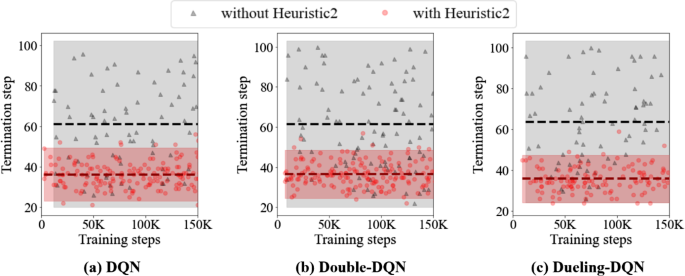
Comparison of the performances of three Q-learning algorithms trained at 10 −2 learning rate, with and without heuristic, for tool path learning in terms of termination step. The upper and lower dashed lines denote the average termination step (total punch steps spent to achieve the forming goal), estimated from Gaussian process regression, through the training process of the algorithms implemented with and without Heuristic 2, respectively. The shaded regions denote 95% confidence interval. The unit for training steps, K, denotes 10 3
To comprehensively evaluate and compare the performance of the six RL algorithms in tool path learning, four performance factors were raised, namely first termination step (1st Term. step), converge speed (Cvg. speed), average converge termination step (Avg. Cvg. Term. step) and average termination frequency (Avg. Term. Freq.). The first factor was quantified by the punch steps spent at the first time achieving the forming goal, which was used to evaluate the learning efficiency of each algorithm under the circumstance that no prior complete tool path planning experience was available and the agent learned the tool path from scratch. The 2nd and 3rd factors evaluated the learning progress and the learning results, and they were quantified by the first converged training step and the average termination step after convergence. The last factor described the learning steadiness in finding the tool path, which was computed as follow:
where \({T}_{total}\) denotes the total times of termination during training, and \({S}_{first}\) and \({S}_{final}\) denote the training step where the first and final terminations occur, respectively. For this research, the first termination needs to be reached as soon as possible due to the high computational expense. Thus, the importance of 1st Term. Step, Cvg. speed and Avg. Cvg. Term. step is regarded as the same and is greater than that of Avg. Term. Freq.
The six algorithms were trained at four different learning rates using two action/policy function approximators, respectively, as described in Section 2.4.2 on the RL learning setup. The learning performance of each algorithm quantified by the four performance factors is summarised in Tables 6 and 7 . The learning results where no termination was found were omitted from the tables, except for Dueling-DQN which was designed to be a CNN with shared convolutional layers and separate fully connected layers. For example, DDPG only managed to learn the tool path at the learning rate of 10 −2 with MLP function approximator. The best tweaking results for the learning rate and function approximator for each algorithm are highlighted in bold.
For Q-learning algorithms, the CNN function approximator was found to outperform the MLP one. Although they had close values of the first three performance factors, the average termination frequencies from the training of DQN and Double-DQN with CNN approximator were, in general, notably higher than those from algorithm trainings with MLP. It was seen that, with MLP, both DQN and Double-DQN cannot attain mere 0.3 terminations per thousand steps at 10 −4 learning rate, and the latter terminated only once through the whole learning process at the learning rate of 10 −1 . However, with CNN approximator, these two algorithms can both terminate steadily over one time per thousand steps at all learning rates. In regard to learning rate, 10 −2 , 10 −1 and 10 −3 were respectively selected for the three Q-learning algorithms because of the evidently better results for the first three performance factors than the other choices of learning rate.
For policy gradient algorithms, MLP was selected as the function approximator for A2C and DDPG while CNN was selected for PPO, and the best learning results were found at learning rate of 10 −2 for all three of them. It can be found that, compared to the Q-learning algorithms, the policy gradient algorithms tended to have a remarkably higher 1st termination step, of which those from A2C were approaching the maximum steps per episode (100). Although they converged to a comparable amount of average termination step to the Q-learning, they spent considerably more time in convergence, especially PPO which used over 200 thousand training steps (over 20 times longer than the Q-learning). In addition, the learning steadiness of the three policy gradient algorithms was poor, especially DDPG and PPO, although the best average termination frequency from them was over 9 times the best from the Q-learning. Trained with two different approximators and at four learning rates, DDPG only managed to learn the tool path once, which could be due to the reason that DDPG was developed for continuous action space problems.
Figure 10 shows the training process of the six RL algorithms, which were trained with the best hyperparameters from above. It can be seen that, unlike the Q-learning algorithms which almost instantly converged after a few terminations, the policy gradient ones had more discernible converging process. Although the tool paths learned from the policy gradient algorithms were about 1–3 times longer than those from the Q-learning at the start of training, they eventually converged to a comparable level of length. DDPG converged to the minimum average termination step of 29, however, its learning steadiness was the worst among all from Table 7 . The Q-learning algorithms outperformed the policy gradient ones in general in terms of the first termination step and convergence speed. This could be due to that A2C and PPO are on-policy learning which is less data-efficient than off-policy, and DDPG is created for learning problems with continuous action space which needs careful tuning for problems with discrete space. For Q-learning algorithms, the Double-DQN preceded DQN and Dueling-DQN for its lower average converge termination step and marginally faster first termination. The reason could be that Double-DQN alleviates the Q-value over-estimation problem in DQN learning, and Dueling-DQN is only particularly useful when the relevance of actions to the goal can be differentiated by separately learning state-value and advantage-value. However, each action in free-form deformation is highly relevant to the goal, for which the structure of Dueling-DQN, in turn, increases learning complexity and slows down learning speed. Thus, for the following results, Double-DQN was used to learn the optimal tool path.
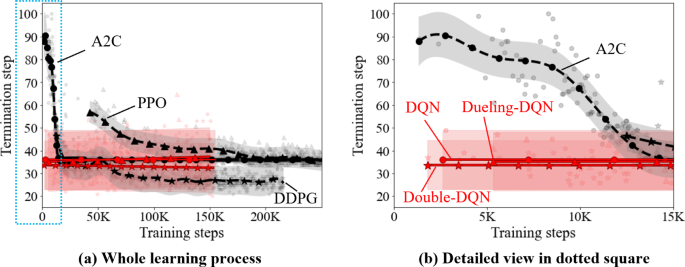
Comparison of the performances of 6 reinforcement learning algorithms studied in this research in terms of termination step. The solid and dashed lines denote the average termination step, estimated from Gaussian process regression, through the training process of the algorithms. The shaded regions denote 95% confidence interval
To assess the credibility of the algorithm selection study, the tool path learning processes and results of the Double-DQN, implemented with virtual environment (VE) and FE simulations, for the same target workpiece were compared in Fig. 11 . From Fig. 11 a and b, the history of forming step and total rewards per episode from the learning using VE have the pattern which highly resembled those from the learning using FE simulations. The average episode step over the training process from VE was marginally higher than that from FE simulations by about 6 steps, while the average episode total rewards from the former was less than the latter by about 15. This phenomenon indicates that, with FE, the agent is more predisposed to overpunch (episode ends) with less efficient tool path at each episode than with VE. In addition, the first termination was about 1200 episodes slower and the total reward was about 30 less than those with VE. The practical rubber-tool forming behaviour is more complex and nonlinear than the VE imitates. From Fig. 11 c, both tool paths had similar forming pattern of alternatingly selecting small and large stroke values which have, on average, slight increased throughout the tool path. Thus, in general, the virtual environment managed to imitate most of the forming behaviours in FE simulations, and the results from the pre-study on algorithm selection performed with the virtual environment are convincing regarding their learning efficiency in tool path learning.
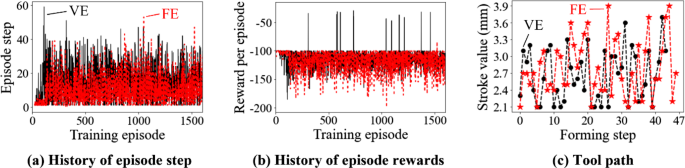
Comparison between RL using virtual environment (VE) and FE simulation environment (FE) in terms of a the history of steps in each episode, b the history of total rewards in each episode and c the tool path predictions
Tool path learning results for 25 workpieces using double-DQN
From Section 3.1, Double-DQN was selected to learn the optimal tool paths for 25 variants of workpiece segments in Group 1, whose \(\varvec{K}\) -graphs and real-scale shapes are shown in Fig. 12 . The workpieces were deformed through the rubber-tool forming process, which was simulated through FE computations. The \(\varvec{K}\) -graphs were arbitrarily created with the method shown in Appendix A, and the real-scale shapes in Fig. 12 b were reconstructed from the \(\varvec{K}\) -graphs using constant initial interval between two contiguous node locations (0.1 mm).
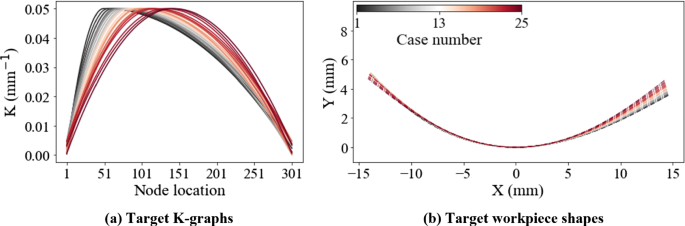
The \(\varvec{K}\) -graphs and workpiece shapes for all the generated target workpieces
An exemplary Double-DQN learning process is shown in Fig. 11 a and b, where the termination occurs at around episode 1500. It can be seen that the first 150 episodes ended with remarkably fewer forming steps than those thereafter, which is due to the effect of \(\varepsilon\) -greedy policy. Under this policy, the agent was more likely to randomly explore the search space than following the online policy learned from existing forming experiences before the \(\varepsilon\) value decayed to 0.5, which led to quicker overpunch thus less steps per episode. To analyse the learning process in the light of effective forming progress, an exemplary learning process concerning the maximum \(\Delta \varvec{K}\) value at the end of each episode is shown in Fig. 13 . As the forming goal is to achieve a workpiece state where its \(\text{max}\left(\left|\Delta \varvec{K}\right|\right)\le 0.01\) mm −1 , the learning history of episode end maximum \(\Delta \varvec{K}\) can reflect the learning progress of effective tool path. From Fig. 13 , there is a clear trend that the maximum value of \(\Delta \varvec{K}\) at the end of each episode gradually decreased from 0.05 mm −1 at the start of learning to below 0.01 mm −1 at about episode 1350, where the termination occurred. This learning curve demonstrates both the effectiveness of the Double-DQN algorithm and the reward function in searching the tool path. With progressing, the deformed sheet metal was more and more approaching its target shape, which was demonstrated by the troughs of the max ( \(\Delta \varvec{K}\) ) graph along the arrow marker.
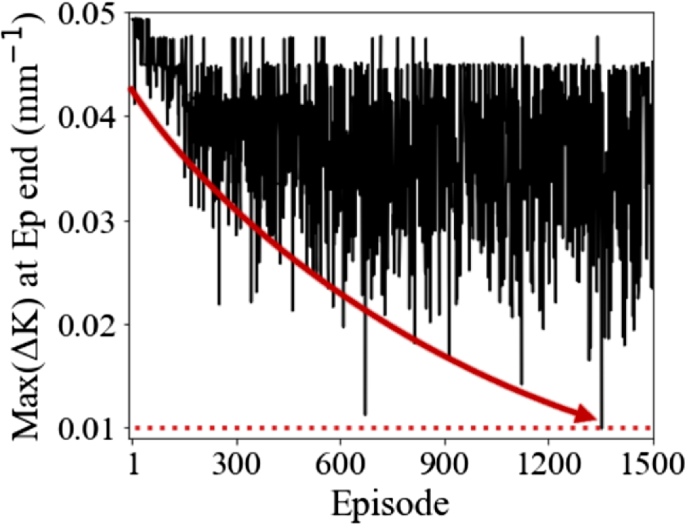
The history of the maximum \(\Delta \varvec{K}\) value at the end of each episode (Ep) throughout the learning process. The arrow shows the learning progress of effective tool path
In addition to the learning progress captured from maximum \(\Delta \varvec{K}\) curves, extra two self-learning characteristics of the tool path learning, which were measured from a more micro perspective than the former, were observed. Figure 14 shows two examples where the self-learning characteristics of tool path efficiency improvement and overpunch circumvention were captured, respectively. The three \(\Delta \varvec{K}\) -graphs were collected from the workpiece deformed by the same number of punches at different episodes during a learning process. In Fig. 14 a, the shaded hatch denotes the total advantage from the workpiece state at episode 527 over that at episode 245 in terms of the shape difference from the target shape, which is measured by the area of hatch. In turn, the unshaded hatch represents the opposite. Thus, it is clear that the tool path planned at the recent episode was more efficient than the one at previous time by about 1.21 with reference to the net area of hatch (shaded area minus unshaded area), accounting for 11.8% of the initial \(\Delta \varvec{K}\) -graph area. In Fig. 14 b, the shaded regions indicate two overpunch-prone locations at episode 527, where the \(\Delta \varvec{K}\) values were only within about 0.002 mm −1 away from the lower threshold (− 0.01 mm −1 ). Due to CPE2, the workpiece can be easily overpunched by deformation near the two locations. It was found that the agent selected smaller punch strokes at these two locations at episode 687, which circumvented the overpunch occurred in previous episodes.
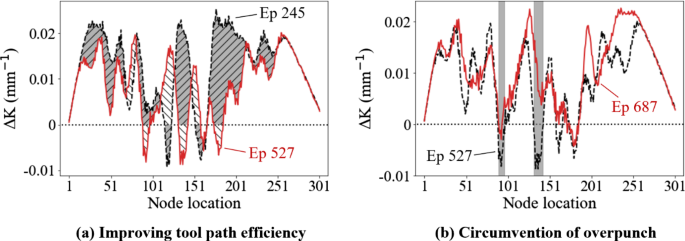
Examples showing self-learning characteristics of a improving tool path efficiency and b circumvention of overpunch. The results were from step 31 at three different episodes of the tool path learning process for a workpiece
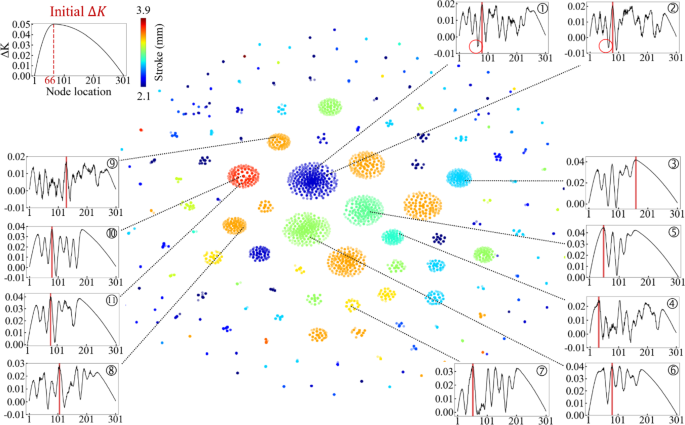
The two-dimensional embedding, generated through t-SNE, of the representations in the last hidden layer of the Double-DQN to workpiece states ( \(\Delta \varvec{K}\) -graphs) experienced during tool path learning. The points are coloured according to stroke values selected by the agent. The graph at the top left corner shows the initial \(\Delta \varvec{K}\) -graph, and the axis labels of the other \(\Delta \varvec{K}\) -graphs (numbered from ① to ⑪) are omitted for brevity. The vertical lines in the \(\Delta \varvec{K}\) -graphs denote the punch locations
To evaluate the performance of the Double-DQN algorithm in extracting and learning abstract information during tool path learning, the representations in the last hidden layer of the Double-DQN model to the workpiece states, which the agent experienced throughout the learning process, were retrieved and reduced to two-dimensional embeddings through t-SNE technique (Maaten & Hinton, 2008 ). The visualisation of these embeddings is shown in Fig. 15 , in which the embeddings are coloured according to selected stroke values by the agent. It can be seen that the CPE1, namely more prior deformation undergoes near the node location of interest results in larger punch stroke required to accomplish a certain change of shape at this location, was learned by the agent.
From Fig. 15 , the \(\Delta \varvec{K}\) -graphs ③, ④ and ⑤ were assigned relatively low value of stroke as there was no prior deformation to at least one side of the punch locations. In addition, larger stroke was assigned if the punch location was closer to the initial punch location 66, which is due to the higher amount of local shape difference. As the CPE1 escalated, higher stroke values were selected by the agent as shown by the rest of the \(\Delta \varvec{K}\) -graphs except for ① and ②. It was also captured that, from ⑥ and ⑦, the CPE1 became more severe with larger nearby prior punches. Apart from CPE1, CPE2 was also captured by the \(\Delta \varvec{K}\) -graphs ① and ②, where the effect was significantly more obvious than the one shown in Fig. 6 a. As indicated by the regions highlighted with circles in ① and ②, the \(\Delta \varvec{K}\) values in these regions were very close to the lower forming threshold, for which small strokes were assigned for them to prevent from overpunch. The punch effect at this region was reproduced as shown in Appendix C, where a mere increase of 0.1 mm of stroke can deteriorate the \(\Delta \varvec{K}\) values to the left of the punch location by about 0.007 mm −1 and caused overpunch in this context. Overall, the similar workpiece states were clustered together and assigned by reasonable stroke values. The agent had a good understanding in tool path planning through learning the abstract representations.
Figure 16 shows an example of the tool path learned by the Double-DQN. In Fig. 16 a, the initial \(\Delta \varvec{K}\) -graph between the blank sheet and the target workpiece was transformed to the final one (enclosed by a rectangle), where the \(\Delta \varvec{K}\) values at all node locations were within the forming thresholds, by 47 forming steps. Due to the Heuristic 1 that the location with the highest \(\Delta \varvec{K}\) value was selected as the punch location, the punch started from the location where the initial \(\Delta \varvec{K}\) value was the highest (about 65) and diverged to both ends of the workpiece, as shown by the top view of Fig. 16 b. Lower values of stroke were assigned to diverging punches than those inside the divergence area due to the CPE1, which led to the repeatedly alternating selection of small and large strokes along the forming progress in Fig. 16 a. As the forming progressed, the CPE1 escalated thus the larger stroke values were selected at later steps (after step 15) of the tool path than those at start. It is also worth noting that, from the side view in Fig. 16 b, large strokes were concentratedly assigned to punch locations with high initial \(\Delta \varvec{K}\) values and descended to those with low ones.
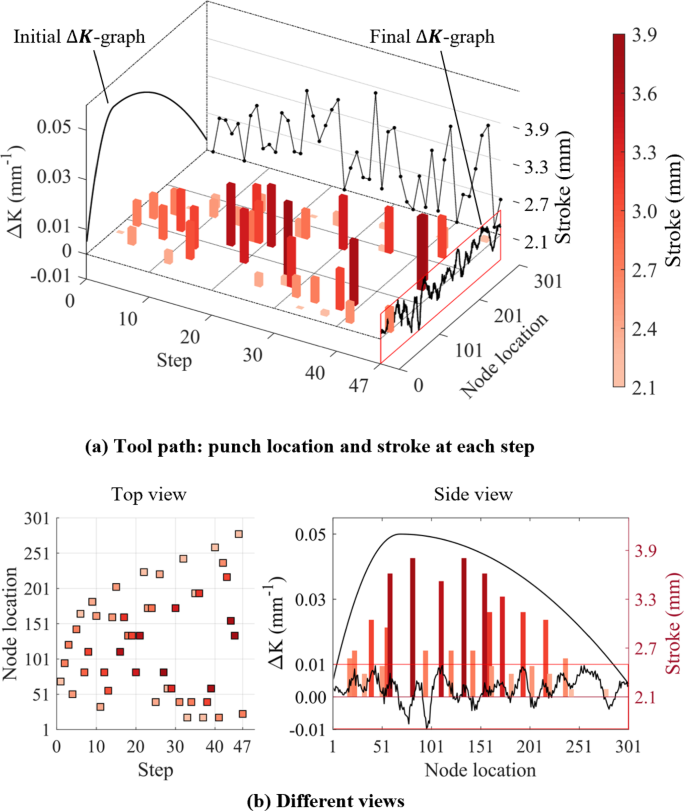
An example of a tool path learned by RL and b its top view and side view. The height of the bars in ( a ) were proportionally decreased for better visualisation
Figure 17 presents the deformation process of a workpiece from blank sheet to its target shape following the tool path learned from the Double-DQN and the dimension error (the geometry difference in Y-direction) between the target workpiece and the one after all punches. The target shape in the real-scale graph was reconstructed from the target \(\varvec{K}\) -graph, of which the same interval of 0.1 mm between two contiguous node locations along the deformed workpiece was used for reconstruction. It can be seen that the final shape of this deformed workpiece was in a good agreement with its target shape, with a maximum dimension error of just above 0.2 mm. The dimension error was at its minimum in the middle of the workpiece, from which the error increased to both ends due to the accumulation of shape difference. The average maximum dimension error for the 25 variants using the tool paths learned by the Double-DQN algorithm was 0.26 mm.
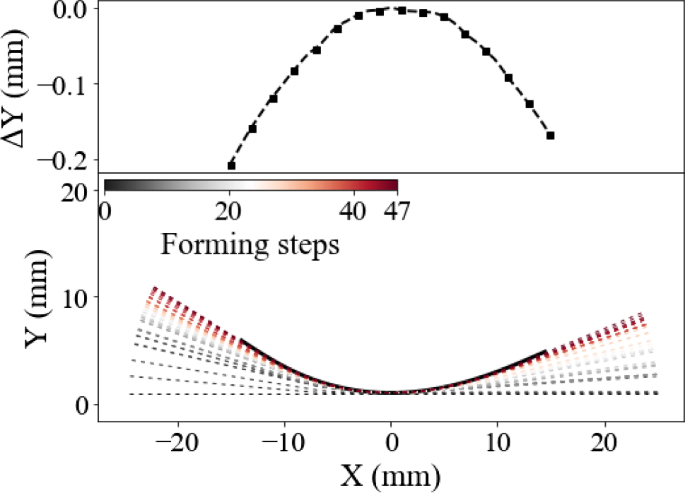
An example of workpiece deformed by the tool path predicted by the Double-DQN. Top: the dimension error between the target workpiece and the final workpiece deformed along the predicted tool path. Bottom: the workpiece shape at each forming step (dotted line) compared to its target shape (solid line). Forming step of 0 denotes blank sheet
Tool path learning generalisation using supervised learning
Through Double-DQN algorithm, the tool paths for the 25 variants of workpiece (shown in Fig. 12 ) segment were learned, whose length (total punch steps) for each variant is shown in Fig. 18 . The tool path lengths varied from 44 to 63, with most lying around 52.
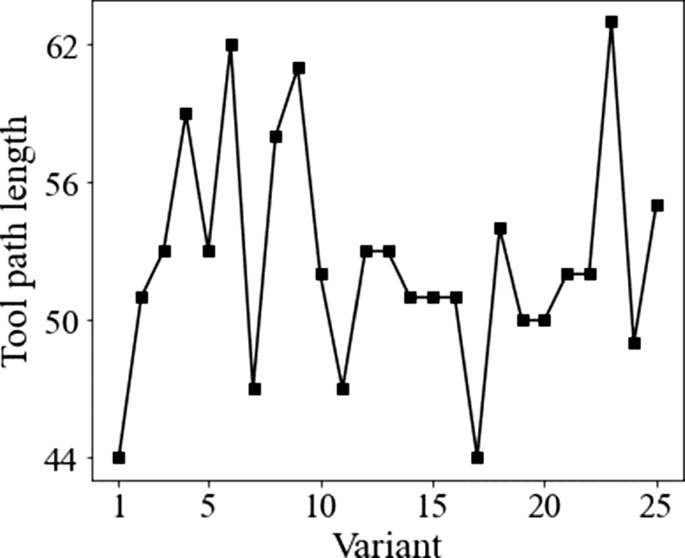
The length of the tool path learned through Double-DQN for each variant of workpiece segment
To learn the intrinsic efficient forming pattern for these workpiece variants (Group 1), the supervised learning model was used for training with the tool path data for the 25 variants. As introduced in " Deep neural networks " section, three LSTMs, which respectively used VGG16, ResNet34 and ResNet50 as the feature extractor, were investigated. The training data were the 25 tool paths pre-processed to the data format consistent with the input and output of the CNN LSTMs, with a total of 1315 data. These data were split into 90% for training and 10% for testing, and the other key training parameters are presented in Table 5 . The training processes of the three models are shown in Fig. 19 by generalisation loss (test loss) history, which would end early if the generalisation loss tended to increase (Goodfellow et al., 2016 ). In addition to training models using the total amount of 25 tool paths, the VGG16 LSTM was also trained with only 20 tool paths to study the effect of training data on the learning performance. The 20 tool paths were evenly sampled from the original 25 paths to avoid massive data missing, and the maximum tool path length among the 20 paths was 55. The generalisation loss has been de-standardised to stroke unit (mm), and the losses from the three models all converged to a comparable level of 0.25 mm, except for the VGG16 LSTM trained with 20 tool paths whose loss converged to about 0.33 mm. Thus, more training data was seen to improve the generalisation, which could be due to that more exhaustive data help to generalise the forming pattern during training. It is also noted that the loss from LSTMs with both ResNets sharply decreased before convergence, which could be due to the decaying learning rate during training. Before learning rate decreased to a certain level, the parameter update at each learning step could be so large that parameter value was jiggling around its suboptimum. Once the learning rate became smaller than this level, the model parameters could be closer to their optimal values and the loss would encounter a sharp drop.
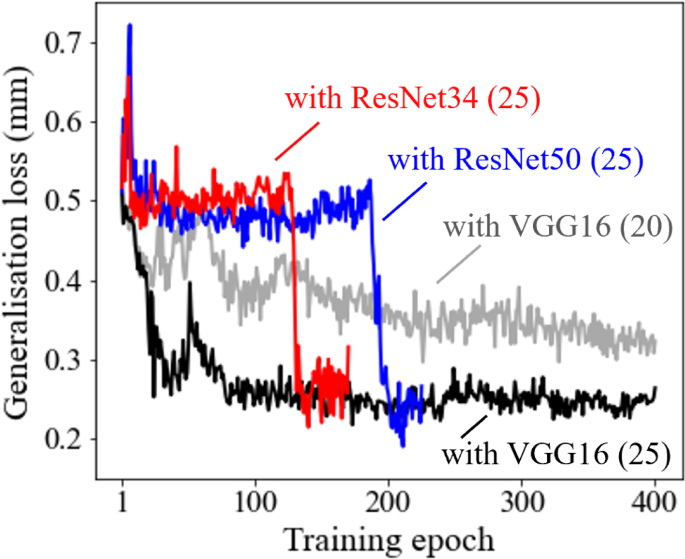
The generalisation loss curves along the training processes of the LSTM models with VGG16, ResNet34 and ResNet50 as the feature extractor, respectively. The number 25 and 20 in the parenthesis denote the amount of tool paths used for training
Figure 20 shows the prediction results for the same test workpiece from the three models trained with 25 tool paths and the VGG16 LSTM trained with 20 tool paths. The total time steps of the LSTMs were the maximum forming steps in the training data, namely 63 and 55 steps for models trained with 25 and 20 tool paths, respectively. It can be seen that the tool path predictions for the workpiece from the three supervised learning models trained with different amount of data all agreed well with the tool path learned through reinforcement learning. The punch started from small values of stroke and alternatingly selected small and large strokes along the forming progress, and the values of large strokes gradually increased as the CPE1 escalated in the forming process. However, it is worth noting that the ResNet50 LSTM tended to predict successive lower values of strokes, near the end of forming (from step 46 to 57), than those predicted by the other models.
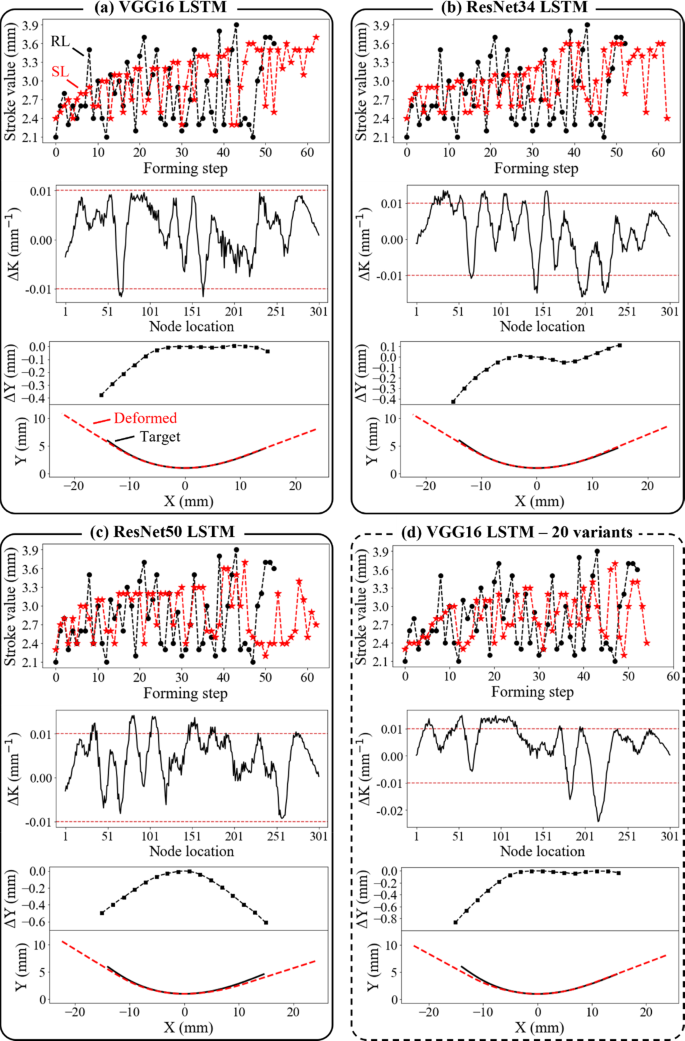
The learning performance of LSTMs trained with 25 tool paths data (solid squares) and 20 tool paths data (dashed squares) on a test workpiece. For the former, the prediction results from LSTMs with a VGG16, b ResNet34 and c ResNet50 are presented, while for the latter, that from d VGG16 LSTM are presented. The prediction results include three parts as followed. Top: the tool path prediction from LSTMs (SL) and its comparison to the tool path from reinforcement learning (RL); Mid: the final \(\Delta \varvec{K}\) -graph after deformation; Bottom: the dimension error and the comparison between the deformed workpiece shape and its target
With regard to the final \(\Delta \varvec{K}\) -graph of the test workpiece deformed through the tool path predicted by the LSTMs, the VGG16 LSTM trained with 25 tool paths was the most superior among all models, whose level of forming goal achievement ( \(G=1-{\Delta \varvec{K}}_{final}^{out\, THLD}/{\Delta \varvec{K}}_{initial}^{out\, THLD}\) , THLD denotes threshold) was up to 99.9%. However, the level of goal achievement of the other two models trained with 25 tool paths just reached 97%, and the LSTM trained with 20 tool paths only achieved 95%. From the final \(\Delta \varvec{K}\) -graphs from models trained with 25 tool paths in Fig. 20 , the one from the VGG16 LSTM was seen to have only two negligible overpunch at location 66 and 161, of which location 66 was the first punch location in the tool path and the overpunch was due to the accumulation of CPE2 near this location through the rest of the tool path. However, multiple evident overpunches and short (insufficient) punches were found in the \(\Delta \varvec{K}\) -graph from the ResNet34 LSTM and short punches in that from the ResNet50 LSTM. This is due to the over- and under-estimation of stroke values in the tool path, from the models, at the locations where overpunches and short punches occurred, and the short punches from ResNet50 LSTM could be caused by the massive punch steps of low stroke values near the end of forming. As for the \(\Delta \varvec{K}\) -graph from the VGG16 LSTM trained with 20 tool paths, the result was even worse than those trained with 25 tool paths. It was seen to have the worst overpunch at node location 213 among the four cases, and there was a continuous short punch region from location 72 to 122, indicating a consistent underestimation of stroke values for punches in this region. The consistent underestimation could be caused by the less training data, which led to the lack of useful tool path data for this region.
In terms of the final geometry difference between the deformed workpiece and its target shape, the tool path predicted by the VGG16 LSTM preceded those from the other models, through which the maximum dimension error was about 0.37 mm. However, the dimension errors resulted from other models were much higher, especially for ResNet50 LSTM and the one trained with less data whose predictions led to over 0.6 mm and 0.8 mm of dimension error, respectively. In addition, the final workpiece shapes from these two models had much more visible deviation from their targets than those from VGG16- and ResNet34- LSTMs trained with 25 tool paths.
It is worth noting that, although the \(\Delta \varvec{K}\) -graph from the ResNet34 LSTM was not as good as the one from the VGG16 LSTM according to the level of forming goal achievement G , the tool paths from both models resulted in comparable results of the final dimension error. On the other hand, good goal achievement does not entail good dimensional accuracy. For example, the right half of workpiece shape from ResNet50 LSTM remarkably differed from its target although its corresponding \(\Delta \varvec{K}\) -graph had a good goal achievement, which is due to the excessively more area of \(\Delta \varvec{K}\) -graph above the X-axis than that below it. The four models, the VGG16-, ResNet34- and ResNet50 LSTM trained with 25 tool paths and the VGG16 LSTM trained with 20 tool paths, were re-evaluated on 10 arbitrary variants, and the average level of forming goal achievement \(\stackrel{-}{G}\) from them was 99.54%, 96.86%, 97.15% and 97.19%, respectively. With regard to the maximum dimension error, the average value from the four models was 0.45, 0.40, 0.63 and 0.58 mm, respectively. It was seen that, although VGG16 LSTM had remarkably better goal achievement than the ResNet34 LSTM, it yielded slightly larger dimension error. This indicates that the overpunch and short punch in terms of the \(\Delta \varvec{K}\) thresholds can, to some extent, contribute to the final forming results. The results entail that moderate compromise of workpiece curvature smoothness could bring more effective tool path planning behaviour in terms of dimensional accuracy. Multi-objective optimisation could be considered in the future for learning the optimal trade-off between the final curvature smoothness and the dimensional accuracy. Thus, attaining the best level of goal achievement and leading to a high dimensional accuracy, the VGG16 LSTM trained with larger amount of data had the most superior performance in tool path learning generalisation.
To compare the tool path planning performance of the proposed generalisable strategy and the method exploiting pure reinforcement learning technology, a well-trained Double-DQN model was used for stroke prediction of the first punch for variants of workpiece with different initial \(\Delta \varvec{K}\) -graphs (i.e., different target shapes), including the target shape it was trained for.
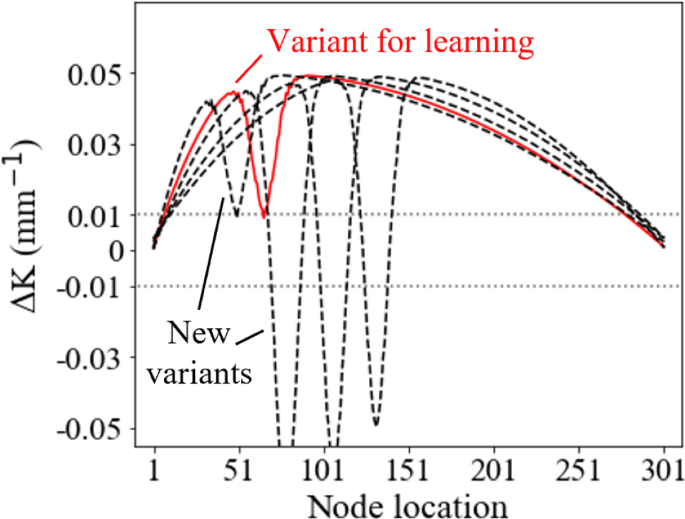
Evaluation of the pure RL strategy by assessing the tool path prediction results from the trained Double-DQN model for new variants of workpiece. The \(\Delta \varvec{K}\) -graphs were acquired after the first punch, predicted by the RL model, for the variant used for tool path learning through RL (solid line) and new variants that were never seen in the learning process (dashed line)
Figure 21 shows the \(\Delta \varvec{K}\) -graphs after the first punch of these workpieces with the stroke prediction from the Double-DQN, of which the node locations where the troughs reside indicate the punch locations. It can be seen that most of the stroke predictions for new variants were uncharacteristically large, which caused significant overpunch at the very first forming step. This indicates that the Double-DQN trained for the tool path learning of a certain target shape cannot be used to predict the tool path for different target workpieces, and the reinforcement learning process has to be gone through again for new applications.
Case study verifying the generalisable tool path planning strategy
To evaluate the generalisable tool path planning strategy presented in Fig. 4 , a new target workpiece of length 90.2 mm was arbitrarily generated as shown in Fig. 22 . The target workpiece was first digitised to its initial \(\Delta \varvec{K}\) -graph using 0.1 mm interval between two contiguous node locations, with 903 node locations in total. The \(\Delta \varvec{K}\) -graph can be segmented to 3 Group 1 segments, A, B and C, which were never seen in the training process of the proposed strategy. With the trained supervised learning model (VGG16 LSTM), the forming tool path for each of the segment was predicted and aggregated to the entire tool path for the target workpiece. It can be seen that the deformation took place segment-wise, and the final \(\Delta \varvec{K}\) -graph resided well in the threshold region with the level of forming goal achievement of 99.87%. Thus, the case study verifies the generalisation of the proposed strategy that an arbitrarily selected workpiece can be formed by solving its tool path in a dynamic programming way. By factorising the forming process of an entire workpiece into that of typical types of segments, the entire workpiece can be formed by consecutively forming each segment.
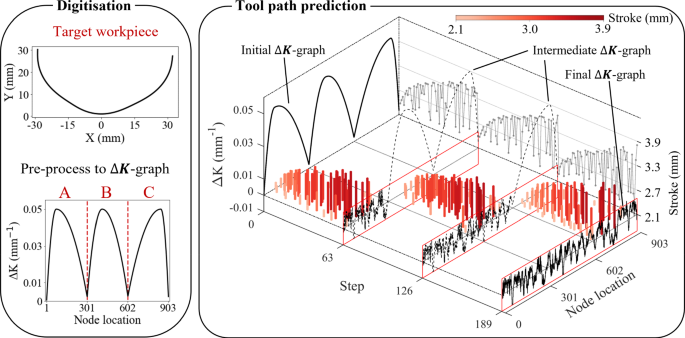
The entire tool path for a new workpiece, which is aggregated by the subpaths predicted by the VGG16 LSTM for each segment of the workpiece. The three segments were never seen in the LSTM training
Figure 23 presents the workpiece shape after deformation, computed by FE, through the generalisable tool path planning strategy and its target shape. From Fig. 23 a, due to the accumulation of \(\Delta \varvec{K}\) -graph area above the X-axis near the junction location (location 301 and 602) between two segments, there was a visible deviation between the deformed workpiece shape and its target, with a maximum dimension error of about 1.8 mm. With two supplementary punches at the two junction locations, the deformed workpiece shape had a clear approaching to the target shape, with a maximum dimension error of about 1 mm. Since the punch location is at the end of each segment where CPE1 was not escalated, small stroke values were selected for the two supplementary punches, which did not cause overpunch. Thus, the generalisable tool path planning strategy successfully yielded the final workpiece shape within a dimension error of 2%. Due to the error accumulation brought in by the junction area, the deformed workpiece shape can be further improved by a few supplementary punches in this area.
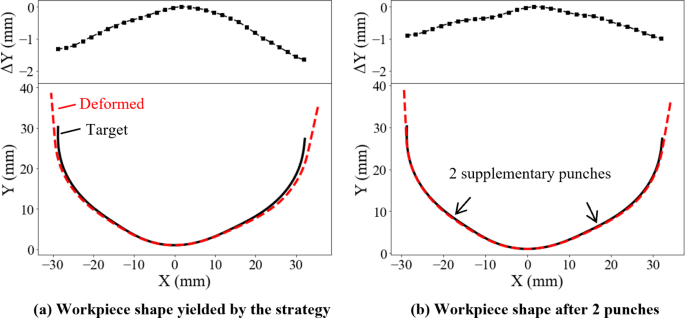
The comparison between the workpiece shape deformed through the generalisable tool path planning strategy and its target shape. a deformed workpiece shape yielded by the strategy and b workpiece shape after two supplementary punches near the junctions of the three workpiece segments
Conclusions
In this research, a generalisable tool path planning strategy for free-form sheet metal stamping was proposed through deep reinforcement and supervised learning technologies. By factorising the forming process of an entire workpiece into that of typical types of segments, the tool path planning problem was solved in a dynamic programming way, which yielded a generalisable tool path planning strategy for a curved component for the first time. RL algorithms and SL models were exploited in tool path learning and generalisation, and six deep RL algorithms and three deep SL models were investigated for performance comparison. The proposed strategy was verified through an application to a case study where the forming tool path for a completely different target workpiece from training data was predicted. From this study, it can be concluded that:
Q-learning algorithms are superior to policy gradient algorithms in tool path planning of free-form sheet metal stamping process, in which Double-DQN precedes DQN and Dueling-DQN. The forming heuristic is also corroborated to further improve the Q-learning performance.
Conferred by deep reinforcement learning, the generalisable tool path planning strategy manifests self-learning characteristics. Over the learning process, the tool path plan becomes more efficient and learns to circumvent overpunch-prone behaviours. With Double-DQN, the tool path for a free-form sheet metal stamping process can be successfully acquired, with the dimension error of the deformed workpiece below 0.26 mm (0.87%).
The efficient forming pattern for a group of workpiece segments have been successfully generalised using deep supervised learning models. The VGG16 LSTM precedes ResNet34- and ResNet50 LSTMs in the tool path learning generalisation, although they have comparable average generalisation loss. The VGG16 LSTM manages to predict the tool path for 10 test variants, with an average level of forming goal achievement of 99.54% and a dimension error of the deformed workpiece below 0.45 mm (1.5%). However, the pure reinforcement learning method cannot generalise plausible tool paths for completely new workpieces.
The generalisable tool path planning strategy successfully predicts the tool path for a completely new workpiece, which has never been seen in its previous learning experience. The level of goal achievement reached 99.87% and the dimension error of the deformed workpiece was 2%. The dimension error could be reduced to about 1.1% with two small supplementary punches near the junctions of the workpiece segments.
Through the proposed method, the tool path planning for an arbitrary sheet metal component is attempted with a generalisable strategy for the first time, and the poor generalisation issue of pure reinforcement learning approach for tool path planning is addressed. However, the efficiency of this strategy is subject to the design of forming the pattern and reward function. In future work, a multi-objective forming goal for tool path planning could be used for a trade-off between the final curvature smoothness and the dimensional accuracy. With moderate compromise of curvature smoothness, more efficient tool path in terms of dimensional accuracy might be yielded. CPE1 and CPE2 can also be embedded into the reward function design to facilitate the tool path learning process.
Appendix A: arbitrary generation of workpiece segments
The \(\Delta \varvec{K}\) -graph of each variant in Group 1 shown in Fig. 24 , which is composed of two parabolas \({\Delta \varvec{K}}_{a-b}\) and \({\Delta \varvec{K}}_{b-c}\) , is determined by five variables ( \({h}_{a}\) , \({h}_{c}\) , \({l}_{b}\) , \({w}_{ab}\) and \({w}_{bc}\) ) and two constants ( \({h}_{b}\) and \({l}_{c}\) ). The workpiece segments were arbitrarily generated by randomly sampling the values of these variables.
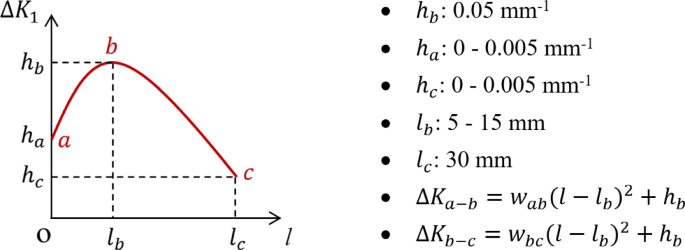
The variables and functions for creating the variants of segments in Group 1. \({\varvec{w}}_{\varvec{a}\varvec{b}}\) and \({\varvec{w}}_{\varvec{b}\varvec{c}}\) can be derived once \({\varvec{h}}_{\varvec{a}}\) , \({\varvec{h}}_{\varvec{b}}\) , \({\varvec{h}}_{\varvec{c}}\) and \({\varvec{l}}_{\varvec{b}}\) are generated
Appendix B. virtual environment configuration
The virtual environment (VE) is developed to imitate the rubber-tool forming behaviour in FE simulations, in which the DRL algorithms are trained to reduce computational expense. Since the forming process is extremely nonlinear, VE is only tweaked to qualitatively resemble FE simulation results, which, however, is sufficient for RL algorithms comparison. The VE is configured following the rules below, whose formulation and parameter selection were based on FE simulation results.
A single punch operation only affects the \(\Delta \varvec{K}\) values at 50 node locations (5 mm) around the punch location and the punch location itself in the \(\Delta \varvec{K}\) -graph.
If a node location has been punched with a stroke and this location is selected again for punching, the \(\Delta \varvec{K}\) -graph will only change if the new stroke is greater than the previous one.
The change of \(\varvec{K}\) value ( \({c}_{K}\) ) at the punch location by stroke ( \({d}_{s}\) ) without CPE1 and CPE2 is defined as: \({c}_{K0}=\left({d}_{s}-2.1\right)\times 0.05+0.045\) .
only one side of the punch location is pre-deformed: \({c}_{K1}={c}_{K0}/2\) ;
both sides of the punch location are pre-deformed by 1 punch: \({c}_{K2}=\left({c}_{K0}+0.035\right)/2\) ;
one side of the punch location is pre-deformed by 1 punch and the other side is pre-deformed by 2 punches: \({c}_{K3}=\left({c}_{K0}+0.005\right)/2\) ;
two sides of the punch locations are pre-deformed by 4 and over 4 punches in total: \({c}_{K4}=\left({c}_{K0}-0.01\right)/2\) .
The change of \(\varvec{K}\) value gradually decreases from \({c}_{Ki}\) ( \(i\in \left[\text{0,4}\right],\mathbb{ }\mathbb{Z}\) ) at the punch location to 0 at two ends of the 51 node locations in rule 1.
With CPE2: if the \(\Delta \varvec{K}\) -graph is changed by the punch, \(\Delta \varvec{K}\) values at the 51 node locations in rule 1 are reduced by 0.0005 mm −1 .
Appendix C: extraordinarily large CPE2
A phenomenon of extraordinarily large CPE2 is shown in Fig. 25 . It can be seen that, after a punch with stroke of 3.6 mm was applied to location 76, there is an evident effect of curvature at about location 70. When the applied stroke was increased by 0.1 mm, the CPE2 at location 70 increased by about 0.007 mm −1 .
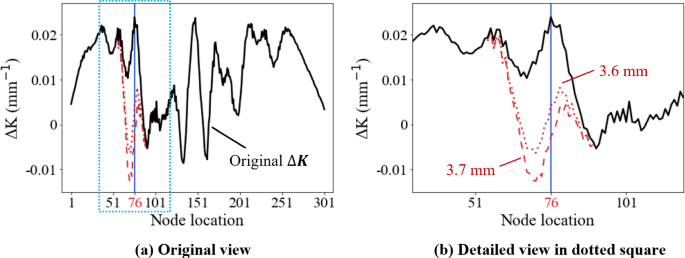
Extraordinarily large CPE2 occurring near punch location of 80. The vertical line denotes the punch location at the current workpiece state (original \(\Delta \varvec{K}\) -graph). The dotted line and dashed line denote the \(\Delta \varvec{K}\) -graphs after punches with stroke of 3.6 mm and 3.7 mm, respectively
Data availability
The data that support the findings of this study are available from the corresponding author upon request.
Allwood, J. M., & Utsunomiya, H. (2006). A survey of flexible forming processes in Japan. International Journal of Machine Tools and Manufacture , 46 (15), 1939–1960. https://doi.org/10.1016/j.ijmachtools.2006.01.034 .
Article Google Scholar
Attanasio, A., Ceretti, E., & Giardini, C. (2006). Optimization of tool path in two points incremental forming. Journal of Materials Processing Technology , 177 (1–3), 409–412. https://doi.org/10.1016/j.jmatprotec.2006.04.047 .
Azaouzi, M., & Lebaal, N. (2012). Tool path optimization for single point incremental sheet forming using response surface method. Simulation Modelling Practice and Theory , 24 , 49–58. https://doi.org/10.1016/j.simpat.2012.01.008 .
Bowen, D. T., Russo, I. M., Cleaver, C. J., Allwood, J. M., & Loukaides, E. G. (2022). From art to part: Learning from the traditional smith in developing flexible sheet metal forming processes. Journal of Materials Processing Technology , 299 , 117337. https://doi.org/10.1016/j.jmatprotec.2021.117337 .
Goodfellow, I., Bengio, Y., & Courville, A. (2016). Deep learning . The MIT.
Google Scholar
Hartmann, C., Opritescu, D., & Volk, W. (2019). An artificial neural network approach for tool path generation in incremental sheet metal free-forming. Journal of Intelligent Manufacturing , 30 (2), 757–770. https://doi.org/10.1007/s10845-016-1279-x .
He, K., Zhang, X., Ren, S., & Sun, J. (2016). Deep residual learning for image recognition. 2016 IEEE Conference on Computer Vision and Pattern Recognition (CVPR), (pp. 770–778). Las Vegas, NV, USA. https://doi.org/10.1109/CVPR.2016.90
Kingma, D. P., & Ba, J. L. (2015). Adam: a method for stochastic optimization. arXiv.1412.6980
Kirk, R., Zhang, A., Grefenstette, E. and Rocktäschel, T. (2021). A survey of zero-shot generalisation in deep reinforcement learning. arXiv.2111.09794
Kubik, C., Knauer, S. M., & Groche, P. (2022). Smart sheet metal forming: Importance of data acquisition, preprocessing and transformation on the performance of a multiclass support vector machine for predicting wear states during blanking. Journal of Intelligent Manufacturing , 33 (1), 259–282. https://doi.org/10.1007/s10845-021-01789-w .
Lillicrap, T. P., Hunt, J. J., Pritzel, A., Heess, N., Erez, T., Tassa, Y., Silver, D., & Wierstra, D. (2015). Continuous control with deep reinforcement learning. https://arxiv.org/abs/1509.02971
Liu, S., Shi, Z., Lin, J., & Li, Z. (2020). Reinforcement learning in free-form stamping of sheet-metals. Procedia Manufacturing , 50 , 444–449. https://doi.org/10.1016/j.promfg.2020.08.081 .
Liu, S., Xia, Y., Liu, Y., Shi, Z., Yu, H., Li, Z., & Lin, J. (2022). Tool path planning of consecutive free-form sheet metal stamping with deep learning. Journal of Materials Processing Technology , 303 , 117530. https://doi.org/10.1016/j.jmatprotec.2022.117530 .
Liu, S., Xia, Y., Shi, Z., Yu, H., Li, Z., & Lin, J. (2021). Deep learning in sheet metal bending with a novel theory-guided deep neural network. IEEE/CAA Journal of Automatica Sinica , 8 (3), 565–581. https://doi.org/10.1109/JAS.2021.1003871 .
Low, D. W. W., Chaudhari, A., Kumar, D., & Kumar, A. S. (2022). Convolutional neural networks for prediction of geometrical errors in incremental sheet metal forming. Journal of Intelligent Manufacturing . https://doi.org/10.1007/s10845-022-01932-1 .
Malhotra, R., Bhattacharya, A., Kumar, A., Reddy, N. V., & Cao, J. (2011). A new methodology for multi-pass single point incremental forming with mixed toolpaths. CIRP Annals , 60 (1), 323–326. https://doi.org/10.1016/j.cirp.2011.03.145 .
Matheron, G., Perrin, N., & Sigaud, O. (2019). The problem with DDPG: understanding failures in deterministic environments with sparse rewards. arXiv:1911.11679
Mnih, V., Badia, A. P., Mirza, M., Graves, A., Lillicrap, T. P., Harley, T., Silver, D., & Kavukcuoglu, K. (2016). Asynchronous methods for deep reinforcement learning. Proceedings of the 33rd International Conference on Machine Learning, PMLR 48 , 1928–1937. https://proceedings.mlr.press/v48/mniha16.html
Mnih, V., Kavukcuoglu, K., Silver, D., Rusu, A. A., Veness, J., Bellemare, M. G., Graves, A., Riedmiller, M., Fidjeland, A. K., Ostrovski, G., Petersen, S., Beattie, C., Sadik, A., Antonoglou, I., King, H., Kumaran, D., Wierstra, D., Legg, S., & Hassabis, D. (2015). Human-level control through deep reinforcement learning. Nature , 518 (7540), 529–533. https://doi.org/10.1038/nature14236 .
Monostori, L., Markus, A., Van Brussel, H., & Westkämpfer, E. (1996). Machine learning approaches to manufacturing. CIRP Annals , 45 (2), 675–712. https://doi.org/10.1016/s0007-8506(18)30216-6 .
Nagargoje, A., Kankar, P. K., Jain, P. K., & Tandon, P. (2021). Application of artificial intelligence techniques in incremental forming: A state-of-the-art review. Journal of Intelligent Manufacturing . https://doi.org/10.1007/s10845-021-01868-y .
Opritescu, D., & Volk, W. (2015). Automated driving for individualized sheet metal part production - A neural network approach. Robotics and Computer-Integrated Manufacturing , 35 , 144–150. https://doi.org/10.1016/j.rcim.2015.03.006 .
Rossi, G., & Nicholas (2018). Re/Learning the wheel: Methods to utilize neural networks as design tools for doubly curved metal surfaces. Proc 38th Annu Conf Assoc Comput Aided Des Archit , 146-155 , https://doi.org/10.52842/conf.acadia.2018.146 .
Schulman, J., Levine, S., Moritz, P., Jordan, M. I., & Abbeel, P. (2015a). Trust region policy optimization. Proceedings of the 32nd International Conference on Machine Learning, PMLR 37:1889–1897. https://proceedings.mlr.press/v37/schulman15.html
Schulman, J., Moritz, P., Levine, S., Jordan, M., & Abbeel, P. (2015b). High-dimensional continuous control using generalized advantage estimation. arXiv:1707.06347
Schulman, J., Wolski, F., Dhariwal, P., Radford, A., & Klimov, O. (2017). Proximal policy optimization algorithms. arXiv:1506.02438
Simonyan, K., & Zisserman, A. (2015). Very deep convolutional networks for large-scale image recognition. 3rd International Conference on Learning Representations, ICLR 2015 - Conference Track Proceedings , 1–14.
Störkle, D., Altmann, P., Möllensiep, D., Thyssen, L., & Kuhlenkötter, B. (2019). Automated parameterization of local support at every toolpath point in robot-based incremental sheet forming. Procedia Manufacturing , 29 , 67–73. https://doi.org/10.1016/j.promfg.2019.02.107 .
Störkle, D. D., Seim, P., Thyssen, L., & Kuhlenkötter, B. (2016). Machine learning in incremental sheet forming. 47st International Symposium on Robotics, 2016, 1–7.
Sutton, R. S., & Barto, A. G. (2017). Reinforcement Learning: An Introduction (Second edi) . The MIT Press Cambridge.
Tanaka, H., Asakawa, N., & Hirao, M. (2005). Development of a forging type rapid prototyping system; Automation of a free forging and metal hammering working. Journal of Robotics and Mechatronics , 17 (5), 523–528. https://doi.org/10.20965/jrm.2005.p0523 .
van der Maaten, L., & Hinton, G. (2008). Visualizing high-dimensional data using t-SNE. Journal of Machine Learning Research , 9 , 2579–2605.
van Hasselt, H., Guez, A., & Silver, D. (2016). Deep reinforcement learning with double Q-learning. Proceedings of the Thirtieth AAAI Conference on Artificial Intelligence (AAAI'16), (pp. 2094–2100. https://doi.org/10.1609/aaai.v30i1.10295
Wang, Z., Schaul, T., Hessel, M., van Hasselt, H., Lanctot, M., & de Freitas, N. (2015). Dueling network architectures for deep reinforcement learning. arXiv.1511.06581
Williams, R. J., & Peng, J. (1991). Function optimization using connectionist reinforcement learning algorithms. Connection Science , 3 (3), 241–268. https://doi.org/10.1080/09540099108946587 .
Download references
Acknowledgments
S. Liu is grateful for the support from China Scholarship Council (CSC) (Grant no. 201908060236).
S. Liu received subsistence allowance from China Scholarship Council (CSC) under Grant no. 201908060236.
Author information
Authors and affiliations.
Department of Mechanical Engineering, Imperial College London, London, SW7 2AZ, UK
Shiming Liu, Zhusheng Shi & Jianguo Lin
School of Creative Technologies, University of Portsmouth, Portsmouth, PO1 2DJ, UK
You can also search for this author in PubMed Google Scholar
Corresponding author
Correspondence to Zhusheng Shi .
Ethics declarations
Competing interest.
The authors have no competing interests to declare that are relevant to the content of this article.
Additional information
Publisher’s note.
Springer Nature remains neutral with regard to jurisdictional claims in published maps and institutional affiliations.
Rights and permissions
Open Access This article is licensed under a Creative Commons Attribution 4.0 International License, which permits use, sharing, adaptation, distribution and reproduction in any medium or format, as long as you give appropriate credit to the original author(s) and the source, provide a link to the Creative Commons licence, and indicate if changes were made. The images or other third party material in this article are included in the article's Creative Commons licence, unless indicated otherwise in a credit line to the material. If material is not included in the article's Creative Commons licence and your intended use is not permitted by statutory regulation or exceeds the permitted use, you will need to obtain permission directly from the copyright holder. To view a copy of this licence, visit http://creativecommons.org/licenses/by/4.0/ .
Reprints and permissions
About this article
Liu, S., Shi, Z., Lin, J. et al. A generalisable tool path planning strategy for free-form sheet metal stamping through deep reinforcement and supervised learning. J Intell Manuf (2024). https://doi.org/10.1007/s10845-024-02371-w
Download citation
Received : 08 November 2022
Accepted : 13 March 2024
Published : 22 April 2024
DOI : https://doi.org/10.1007/s10845-024-02371-w
Share this article
Anyone you share the following link with will be able to read this content:
Sorry, a shareable link is not currently available for this article.
Provided by the Springer Nature SharedIt content-sharing initiative
- Deep learning
- Deep reinforcement learning
- Deep supervised learning
- Sheet metal forming
- Intelligent manufacturing
- Tool path planning
- Find a journal
- Publish with us
- Track your research
This paper is in the following e-collection/theme issue:
Published on 17.4.2024 in Vol 26 (2024)
This is a member publication of National University of Singapore
Comparing Open-Access Database and Traditional Intensive Care Studies Using Machine Learning: Bibliometric Analysis Study
Authors of this article:

There are no citations yet available for this article according to Crossref .
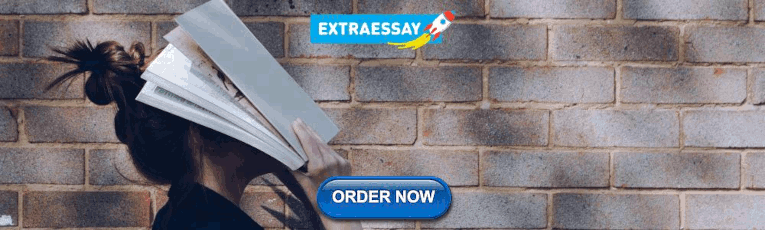
IMAGES
VIDEO
COMMENTS
We also strongly encourage authors to submit their data sets to the UCI Machine Learning Repository. Papers longer than 35 pages will take a longer time to review and may be rejected if an AE and reviewers cannot be found. Further, papers above 50 pages require a note of justification in the cover letter, and may be desk rejected.
The Journal of Machine Learning Research (JMLR), , provides an international forum for the electronic and paper publication of high-quality scholarly articles in all areas of machine learning. All published papers are freely available online. JMLR has a commitment to rigorous yet rapid reviewing. Final versions are (ISSN 1533-7928) immediately ...
A: The time needed to review a paper varies from paper to paper and JMLR does not guarantee that it will make a decision in a given period of time. The current average time to decision is around 3 months, see the statistics section of the webpage for the most recent statistics.
The Journal of Machine Learning Research (JMLR) provides an international forum for the electronic and paper publication of high-quality scholarly articles in all areas of machine learning.JMLR seeks previously unpublished papers that contain:new algorithms with empirical, theoretical, psychological, or biological justification; experimental and/or theoretical studies yielding new insight into ...
The Journal of Machine Learning Research is a peer-reviewed open access scientific journal covering machine learning. It was established in 2000 and the first editor-in-chief was Leslie Kaelbling. The current editors-in-chief are Francis Bach (Inria), David Blei (Columbia University) and Bernhard Schölkopf (Max Planck Institute for ...
The Journal of Machine Learning Research. Search within JMLR. Search Search. Home; Collections; Hosted Content; The Journal of Machine Learning Research; Archive; ... The count-min sketch (CMS) is a time and memory efficient randomized data structure that provides estimates of tokens' frequencies in a data stream of tokens, i.e. point queries ...
The Journal of Machine Learning Research (JMLR) provides an international forum for the electronic and paper publication of high-quality scholarly articles in all areas of machine learning. All published papers are freely available online. JMLR has a commitment to rigorous yet rapid reviewing. Final versions are published electronically (ISSN ...
JMLR Papers. Select a volume number to see its table of contents with links to the papers. Volume 23 (January 2022 - Present) . Volume 22 (January 2021 - December 2021) . Volume 21 (January 2020 - December 2020) . Volume 20 (January 2019 - December 2019) . Volume 19 (August 2018 - December 2018) . Volume 18 (February 2017 - August 2018) . Volume 17 (January 2016 - January 2017)
Journal of Machine Learning Research. The Journal of Machine Learning Research (JMLR), established in 2000, provides an international forum for the electronic and paper publication of high-quality scholarly articles in all areas of machine learning.All published papers are freely available online. News. 2022.02.18: New blog post: Retrospectives from 20 Years of JMLR .
Guidelines for JMLR reviewers. Please touch upon as many of the following points as practical: Goals: What are research goals and learning task? Description: Is the description adequately detailed for others to replicate the work? Is it clearly written in good style and does it include examples? Papers describing systems should clearly describe ...
Keep lettering consistently sized throughout your final-sized artwork, usually about 2-3 mm (8-12 pt). Variance of type size within an illustration should be minimal, e.g., do not use 8-pt type on an axis and 20-pt type for the axis label. Avoid effects such as shading, outline letters, etc.
Supervised: Supervised learning is typically the task of machine learning to learn a function that maps an input to an output based on sample input-output pairs [].It uses labeled training data and a collection of training examples to infer a function. Supervised learning is carried out when certain goals are identified to be accomplished from a certain set of inputs [], i.e., a task-driven ...
portant: (1) Machine learning is important in adjusting its struc-. ture to produce desired outputs due to the heavy amount. of data input into the system [57]. (2) Machine learning is also ...
Journal of Machine Learning Research, 23(198): 1-55, 2022a. Google Scholar; Y. Jia and X. Y. Zhou. Policy gradient and actor-critic learning in continuous time and space: Theory and algorithms. Journal of Machine Learning Research, 23(275):1-50, 2022b. Google Scholar; I. Karatzas and S. Shreve. Brownian Motion and Stochastic Calculus, volume ...
Journal of Machine Learning Research Special Issues. Machine learning is expanding in many subfields, interdisciplinary connections, and application areas. ... after which time it will be reviewed, and a different procedure may be implemented. Special issues (SIs) are overseen similar to a workshop, but with the review process being JMLR's ...
A Review of Robot Learning for Manipulation: Challenges, Representations, and Algorithms ... Improving Reproducibility in Machine Learning Research(A Report from the NeurIPS 2019 Reproducibility Program) ... Hamilton-Jacobi Deep Q-Learning for Deterministic Continuous-Time Systems with Lipschitz Continuous Controls Jeongho Kim, Jaeuk Shin, ...
Recently, machine learning (ML) has become very widespread in research and has been incorporated in a variety of applications, including text mining, spam detection, video recommendation, image classification, and multimedia concept retrieval [1,2,3,4,5,6].Among the different ML algorithms, deep learning (DL) is very commonly employed in these applications [7,8,9].
Journal of Machine Learning Research: Help / FAQ: Welcome Welcome to the JMLR paper submission and review software. If you already have an account, please continue to the Main Center. Otherwise, please ...
The aim is to provide a snapshot of some of the most exciting work published in the various research areas of the journal. Original Submission ... railways pose challenges due to the associated costs and time requirements. ... Askarzadeh. 2024. "Remote Sensing and Machine Learning for Safer Railways: A Review" Applied Sciences 14, no. 9: 3573 ...
Today, JMLR is a top journal in AI and ML, while remaining free, open, and community-driven. Since 2002, the fields of AI and ML have grown and thrived, and JMLR has grown along with them. Of course, the increase in submissions means increased demands on all the volunteers' time and energy.
Abstract. Machine learning (ML) is the scientific study of algorithms and statistical models that computer systems use to perform a specific task without being explicitly programmed. Learning ...
The journal disseminates impactful and re-usable scientific software through Original Software Publications which describe the application of the software to research and the published outputs. Machine Learning with Applications (MLWA) is a peer reviewed, open access journal focused on research related to machine learning.
International Journal of Machine Learning and Cybernetics is a dedicated platform for the confluence of machine learning and cybernetics research. Focused on the hybrid development of machine learning and cybernetics. Encourages the submission of new ideas, design alternatives, and case studies. Covers key research areas such as pattern ...
Journal of Machine Learning Research. JMLR Volume 23. Joint Estimation and Inference for Data Integration Problems based on Multiple Multi-layered Gaussian Graphical Models. Subhabrata Majumdar, George Michailidis; (1):1−53, 2022. [ abs ] [ pdf ] [ bib ] [ code ] Debiased Distributed Learning for Sparse Partial Linear Models in High Dimensions.
Due to the high cost of specially customised presses and dies and the advance of machine learning technology, there is some emerging research attempting free-form sheet metal stamping processes which use several common tools to produce products of various shapes. However, tool path planning strategies for the free forming process, such as reinforcement learning technique, derived from previous ...
Background: Intensive care research has predominantly relied on conventional methods like randomized controlled trials. However, the increasing popularity of open-access, free databases in the past decade has opened new avenues for research, offering fresh insights. Leveraging machine learning (ML) techniques enables the analysis of trends in a vast number of studies.