Masks Strongly Recommended but Not Required in Maryland, Starting Immediately
Due to the downward trend in respiratory viruses in Maryland, masking is no longer required but remains strongly recommended in Johns Hopkins Medicine clinical locations in Maryland. Read more .
- Vaccines
- Masking Guidelines
- Visitor Guidelines
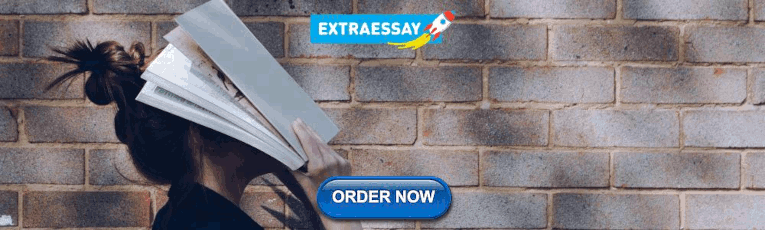
Understanding Clinical Trials
Clinical research: what is it.
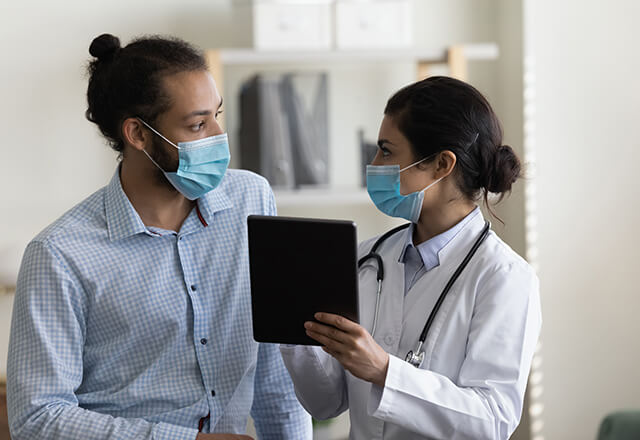
Your doctor may have said that you are eligible for a clinical trial, or you may have seen an ad for a clinical research study. What is clinical research, and is it right for you?
Clinical research is the comprehensive study of the safety and effectiveness of the most promising advances in patient care. Clinical research is different than laboratory research. It involves people who volunteer to help us better understand medicine and health. Lab research generally does not involve people — although it helps us learn which new ideas may help people.
Every drug, device, tool, diagnostic test, technique and technology used in medicine today was once tested in volunteers who took part in clinical research studies.
At Johns Hopkins Medicine, we believe that clinical research is key to improve care for people in our community and around the world. Once you understand more about clinical research, you may appreciate why it’s important to participate — for yourself and the community.
What Are the Types of Clinical Research?
There are two main kinds of clinical research:
Observational Studies
Observational studies are studies that aim to identify and analyze patterns in medical data or in biological samples, such as tissue or blood provided by study participants.
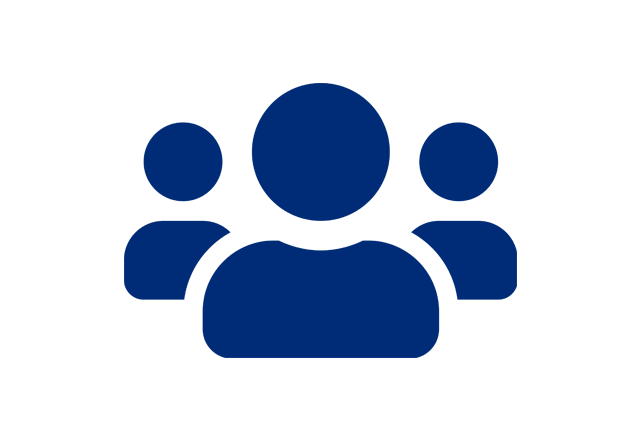
Clinical Trials
Clinical trials, which are also called interventional studies, test the safety and effectiveness of medical interventions — such as medications, procedures and tools — in living people.
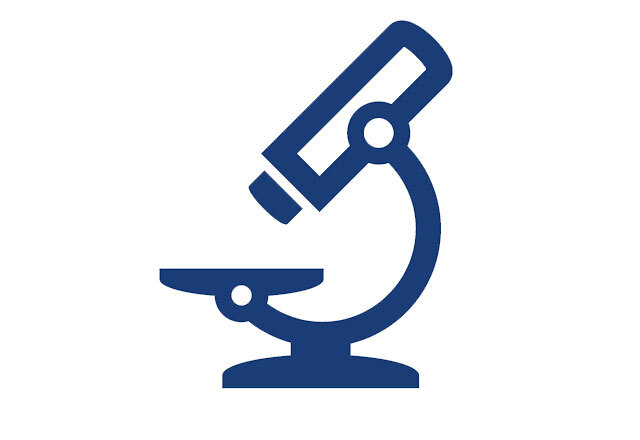
Clinical research studies need people of every age, health status, race, gender, ethnicity and cultural background to participate. This will increase the chances that scientists and clinicians will develop treatments and procedures that are likely to be safe and work well in all people. Potential volunteers are carefully screened to ensure that they meet all of the requirements for any study before they begin. Most of the reasons people are not included in studies is because of concerns about safety.
Both healthy people and those with diagnosed medical conditions can take part in clinical research. Participation is always completely voluntary, and participants can leave a study at any time for any reason.
“The only way medical advancements can be made is if people volunteer to participate in clinical research. The research participant is just as necessary as the researcher in this partnership to advance health care.” Liz Martinez, Johns Hopkins Medicine Research Participant Advocate
Types of Research Studies
Within the two main kinds of clinical research, there are many types of studies. They vary based on the study goals, participants and other factors.
Biospecimen studies
Healthy volunteer studies.
Clinical trials study the safety and effectiveness of interventions and procedures on people’s health. Interventions may include medications, radiation, foods or behaviors, such as exercise. Usually, the treatments in clinical trials are studied in a laboratory and sometimes in animals before they are studied in humans. The goal of clinical trials is to find new and better ways of preventing, diagnosing and treating disease. They are used to test:
Drugs or medicines
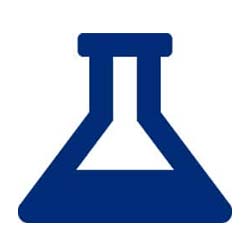
New types of surgery
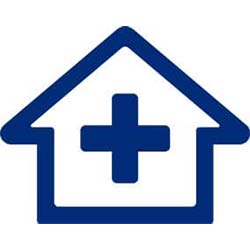
Medical devices
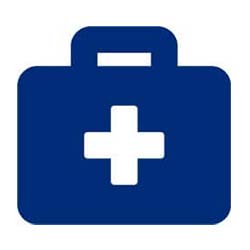
New ways of using current treatments
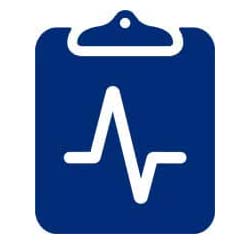
New ways of changing health behaviors
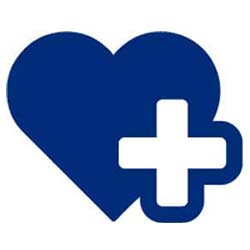
New ways to improve quality of life for sick patients
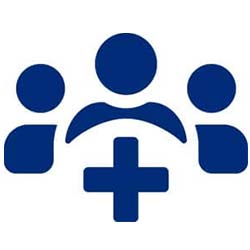
Goals of Clinical Trials
Because every clinical trial is designed to answer one or more medical questions, different trials have different goals. Those goals include:
Treatment trials
Prevention trials, screening trials, phases of a clinical trial.
In general, a new drug needs to go through a series of four types of clinical trials. This helps researchers show that the medication is safe and effective. As a study moves through each phase, researchers learn more about a medication, including its risks and benefits.
Is the medication safe and what is the right dose? Phase one trials involve small numbers of participants, often normal volunteers.
Does the new medication work and what are the side effects? Phase two trials test the treatment or procedure on a larger number of participants. These participants usually have the condition or disease that the treatment is intended to remedy.
Is the new medication more effective than existing treatments? Phase three trials have even more people enrolled. Some may get a placebo (a substance that has no medical effect) or an already approved treatment, so that the new medication can be compared to that treatment.
Is the new medication effective and safe over the long term? Phase four happens after the treatment or procedure has been approved. Information about patients who are receiving the treatment is gathered and studied to see if any new information is seen when given to a large number of patients.
“Johns Hopkins has a comprehensive system overseeing research that is audited by the FDA and the Association for Accreditation of Human Research Protection Programs to make certain all research participants voluntarily agreed to join a study and their safety was maximized.” Gail Daumit, M.D., M.H.S., Vice Dean for Clinical Investigation, Johns Hopkins University School of Medicine
Is It Safe to Participate in Clinical Research?
There are several steps in place to protect volunteers who take part in clinical research studies. Clinical Research is regulated by the federal government. In addition, the institutional review board (IRB) and Human Subjects Research Protection Program at each study location have many safeguards built in to each study to protect the safety and privacy of participants.
Clinical researchers are required by law to follow the safety rules outlined by each study's protocol. A protocol is a detailed plan of what researchers will do in during the study.
In the U.S., every study site's IRB — which is made up of both medical experts and members of the general public — must approve all clinical research. IRB members also review plans for all clinical studies. And, they make sure that research participants are protected from as much risk as possible.
Earning Your Trust
This was not always the case. Many people of color are wary of joining clinical research because of previous poor treatment of underrepresented minorities throughout the U.S. This includes medical research performed on enslaved people without their consent, or not giving treatment to Black men who participated in the Tuskegee Study of Untreated Syphilis in the Negro Male. Since the 1970s, numerous regulations have been in place to protect the rights of study participants.
Many clinical research studies are also supervised by a data and safety monitoring committee. This is a group made up of experts in the area being studied. These biomedical professionals regularly monitor clinical studies as they progress. If they discover or suspect any problems with a study, they immediately stop the trial. In addition, Johns Hopkins Medicine’s Research Participant Advocacy Group focuses on improving the experience of people who participate in clinical research.
Clinical research participants with concerns about anything related to the study they are taking part in should contact Johns Hopkins Medicine’s IRB or our Research Participant Advocacy Group .
Learn More About Clinical Research at Johns Hopkins Medicine
For information about clinical trial opportunities at Johns Hopkins Medicine, visit our trials site.
Video Clinical Research for a Healthier Tomorrow: A Family Shares Their Story
Clinical Research for a Healthier Tomorrow: A Family Shares Their Story
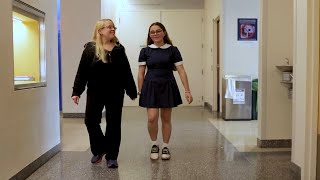
Principles of Research Methodology
A Guide for Clinical Investigators
- © 2012
- Phyllis G. Supino 0 ,
- Jeffrey S. Borer 1
, Cardiovascular Medicine, SUNY Downstate Medical Center, Brooklyn, USA
You can also search for this editor in PubMed Google Scholar
, Cardiovascualr Medicine, SUNY Downstate Medical Center, Brooklyn, USA
- Based on a highly regarded and popular lecture series on research methodology
- Comprehensive guide written by experts in the field
- Emphasizes the essentials and fundamentals of research methodologies
23 Citations
7 Altmetric
This is a preview of subscription content, log in via an institution to check access.
Access this book
- Available as EPUB and PDF
- Read on any device
- Instant download
- Own it forever
- Compact, lightweight edition
- Dispatched in 3 to 5 business days
- Free shipping worldwide - see info
- Durable hardcover edition
Tax calculation will be finalised at checkout
Other ways to access
Licence this eBook for your library
Institutional subscriptions
About this book
Principles of Research Methodology: A Guide for Clinical Investigators is the definitive, comprehensive guide to understanding and performing clinical research. Designed for medical students, physicians, basic scientists involved in translational research, and other health professionals, this indispensable reference also addresses the unique challenges and demands of clinical research and offers clear guidance in becoming a more successful member of a medical research team and critical reader of the medical research literature. The book covers the entire research process, beginning with the conception of the research problem to publication of findings. Principles of Research Methodology: A Guide for Clinical Investigators comprehensively and concisely presents concepts in a manner that is relevant and engaging to read. The text combines theory and practical application to familiarize the reader with the logic of research design and hypothesis construction, the importance of research planning, the ethical basis of human subjects research, the basics of writing a clinical research protocol and scientific paper, the logic and techniques of data generation and management, and the fundamentals and implications of various sampling techniques and alternative statistical methodologies. Organized in thirteen easy to read chapters, the text emphasizes the importance of clearly-defined research questions and well-constructed hypothesis (reinforced throughout the various chapters) for informing methods and in guiding data interpretation. Written by prominent medical scientists and methodologists who have extensive personal experience in biomedical investigation and in teaching key aspects of research methodology to medical students, physicians and other health professionals, the authors expertly integrate theory with examples and employ language that is clear and useful for a general medical audience. A major contribution to the methodology literature, Principles of Research Methodology: A Guide for Clinical Investigators is an authoritative resource for all individuals who perform research, plan to perform it, or wish to understand it better.
Similar content being viewed by others
History of Research
Research (See Clinical Research; Research Ethics)
General Study Objectives
Table of contents (13 chapters), front matter, overview of the research process.
Phyllis G. Supino
Developing a Research Problem
- Phyllis G. Supino, Helen Ann Brown Epstein
The Research Hypothesis: Role and Construction
Design and interpretation of observational studies: cohort, case–control, and cross-sectional designs.
- Martin L. Lesser
Fundamental Issues in Evaluating the Impact of Interventions: Sources and Control of Bias
Protocol development and preparation for a clinical trial.
- Joseph A. Franciosa
Data Collection and Management in Clinical Research
- Mario Guralnik
Constructing and Evaluating Self-Report Measures
- Peter L. Flom, Phyllis G. Supino, N. Philip Ross
Selecting and Evaluating Secondary Data: The Role of Systematic Reviews and Meta-analysis
- Lorenzo Paladino, Richard H. Sinert
Sampling Methodology: Implications for Drawing Conclusions from Clinical Research Findings
- Richard C. Zink
Introductory Statistics in Medical Research
- Todd A. Durham, Gary G. Koch, Lisa M. LaVange
Ethical Issues in Clinical Research
- Eli A. Friedman
How to Prepare a Scientific Paper
Jeffrey S. Borer
Back Matter
From the reviews:
Editors and Affiliations
Bibliographic information.
Book Title : Principles of Research Methodology
Book Subtitle : A Guide for Clinical Investigators
Editors : Phyllis G. Supino, Jeffrey S. Borer
DOI : https://doi.org/10.1007/978-1-4614-3360-6
Publisher : Springer New York, NY
eBook Packages : Medicine , Medicine (R0)
Copyright Information : Springer Science+Business Media, LLC 2012
Hardcover ISBN : 978-1-4614-3359-0 Published: 22 June 2012
Softcover ISBN : 978-1-4939-4292-3 Published: 23 August 2016
eBook ISBN : 978-1-4614-3360-6 Published: 22 June 2012
Edition Number : 1
Number of Pages : XVI, 276
Topics : Oncology , Cardiology , Internal Medicine , Endocrinology , Neurology
- Publish with us
Policies and ethics
- Find a journal
- Track your research
A Nature Research Service
Clinical Research Methodology
Key features.
Supports clinicians with strategies to improve the impact and influence of their clinical studies
Includes a workbook with keypoints, activities to reinforce the content, and additional online resources
Interactive workshop where the trainer stimulates engagement and discussion with the attendees
1-day live workshop delivered by an expert trainer
Auditorium or classroom style
Available in-person or virtually
Up to 250 early-career clinical researchers
Masterclass in Clinical Research Methodology
Conducting clinical research has a tremendous impact on patient care and management as well as improving public health. Therefore, it is essential that the study is designed and conducted properly to maximize impact. This one-day workshop aims to give researchers the necessary skills to develop a robust clinical research study that will advance evidence-based medicine. Nature Masterclasses are available in-person and virtually.
Clinical Research Methodology workshop - led by experts
Planning a clinical study
- Discusses how to properly choose a research question that is necessary for the field
- Reviews efficient literature searching strategies and how to stay up-to-date
- Discusses focusing the research problem in a manageable and realistic manner
- Reviews different primary and secondary outcomes related to the research question that should be the focus of the study
Choosing the right study design
- Covers how to identify which study design is appropriate for the research problem and outcome
- Briefly introduces systematic reviews, randomised controlled trials (RCTs), cohort studies, case-controlled studies, cross-sectional studies, and case reports/series
- Highlights the advantages and limitations of the above that should be considered
- Discusses RCTs, given their important impact in clinical research
Research Methods Resources
Methods at a glance.
This section provides information and examples of methodological issues to be aware of when working with different study designs. Virtually all studies face methodological issues regarding the selection of the primary outcome(s), sample size estimation, missing outcomes, and multiple comparisons. Randomized studies face additional challenges related to the method for randomization. Other studies face specific challenges associated with their study design such as those that arise in effectiveness-implementation research; multiphase optimization strategy (MOST) studies; sequential, multiple assignment, randomized trials (SMART); crossover designs; non-inferiority trials; regression discontinuity designs; and paired availability designs. Some face issues involving exact tests, adherence to behavioral interventions, noncompliance in encouragement designs, evaluation of risk prediction models, or evaluation of surrogate endpoints.
Learn more about broadly applicable methods
Experiments, including clinical trials, differ considerably in the methods used to assign participants to study conditions (or study arms) and to deliver interventions to those participants.
This section provides information related to the design and analysis of experiments in which (1) participants are assigned in groups (or clusters) and individual observations are analyzed to evaluate the effect of the intervention, (2) participants are assigned individually but receive at least some of their intervention with other participants or through an intervention agent shared with other participants, and (3) participants are assigned in groups (or clusters) but groups cross-over to the intervention condition at pre-determined time points in sequential, staggered fashion until all groups receive the intervention.
This material is relevant for both human and animal studies as well as basic and applied research. And while it is important for investigators to become familiar with the issues presented on this website, it is even more important that they collaborate with a methodologist who is familiar with these issues.
In a parallel group-randomized trial, also called a parallel cluster-randomized trial, groups or clusters are randomized to study conditions, and observations are taken on the members of those groups with no crossover of groups or clusters to a different condition or study arm during the trial.
Learn more about GRTs
In an individually randomized group-treatment trial, also called a partially clustered design, individuals are randomized to study conditions but receive at least some of their intervention with other participants or through an intervention agent shared with other participants.
Learn more about IRGTs
In a stepped wedge group-randomized trial, also called a stepped wedge cluster-randomized trial, groups or clusters are randomized to sequences which cross-over to the intervention condition at predetermined time points in a sequential, staggered fashion until all groups receive the intervention.
Learn more about SWGRTs
NIH Clinical Trial Requirements
The NIH launched a series of initiatives to enhance the accountability and transparency of clinical research. These initiatives target key points along the entire clinical trial lifecycle, from concept to reporting the results.
Check out the Frequently Asked Questions section or send us a message .
Disclaimer: Substantial effort has been made to provide accurate and complete information on this website. However, we cannot guarantee that there will be no errors. Neither the U.S. Government nor the National Institutes of Health (NIH) assumes any legal liability for the accuracy, completeness, or usefulness of any information, products, or processes disclosed herein, or represents that use of such information, products, or processes would not infringe on privately owned rights. The NIH does not endorse or recommend any commercial products, processes, or services. The views and opinions of authors expressed on NIH websites do not necessarily state or reflect those of the U.S. Government, and they may not be used for advertising or product endorsement purposes.
Foundations of Clinical Research
This Harvard Medical School six-month, application-based certificate program provides the essential skill sets and fundamental knowledge required to begin or expand your clinical research career.

Associated Schools
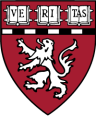
Harvard Medical School
What you'll learn.
Understand and apply the foundational concepts of biostatistics and epidemiology
Develop a research question and formulate a testable hypothesis
Design and begin to implement a clinical research study
Cultivate the skills required to present a clinical research study
Critically evaluate the research findings in medical literature
Synthesize crucial statistical analyses using Stata software
Course description
The Foundations of Clinical Research program is rooted in the belief that clinical research training is critical to professional development in health care. Clinical research training not only creates potential independent investigators, but also enables clinicians to advance their careers through a greater understanding of research evidence. Designed to provide learners with the foundational knowledge and skill sets required to produce high-quality clinical research, our program will lay the fundamental groundwork in epidemiology and biostatistics required for a multifaceted career in clinical research.
The overarching goal of the Foundations of Clinical Research program is to equip the next generation of researchers with the skill sets essential to evaluating evidence, understanding biostatistics, and beginning their clinical research careers. Our aim is to ensure that learners develop a strong foundation in the design, implementation, analysis and interpretation of clinical research studies.
During the program, our innovative active learning approach emphasizes the traditional tutorial system with weekly live video tutorials, seminars and symposia anchored by 3 live intense weekend online workshops. The Foundations of Clinical Research program’s six-month online curriculum emphasizes real-time skill-based learning.
Participants will be eligible for Associate Alumni status upon successful completion of the program. Early tuition and need-based tuition reductions may be available.
Course Outline
Live Workshops
The interactive workshop curriculum will focus on hands-on skill development through active learning. To that end, the intensive schedule is designed to accelerate the growth of high-yield clinical research skills via individual and team-based workshop exercises. Students will be immersed in a dynamic learning environment that encourages collaboration and collegial networking with faculty and peers.
Essential elements of the workshop include instruction and practical exercises in the core concepts of biostatistics, epidemiology and research question development, as well as critical assessment of the medical literature and practical training in statistical software using real-life datasets. In addition to providing training in mentorship, academic career development and leadership, we create a supportive and active learning environment where opportunities for knowledge retention and networking abound.
Live Symposia, Tutorials and Seminars
Symposia, tutorials and seminars are mandatory and will be delivered live online and organized according to eight specific clinical research topics.
Eight 3-Hour Symposia
- Instruction on a specific clinical research topic (e.g., cohort study design and interpretation)
- In-depth discussion on a related epidemiology concept (e.g., odds ratio)
- Hands-on guidance for implementing the related analysis with statistical programming in Stata
Eight 1-Hour Tutorials
- Interpret and report on papers related to the specific clinical research topic
Eight 1-Hour Special-Topic Seminars
- The biostatistical and epidemiological concepts to specific clinical research topics with concrete examples
Assignments
All students will be expected to complete all assignments by the due dates. Assignments will be graded as either “pass” or “fail.”
Individual Assignment 1
Individual Research Question and Study Design
- Generate a novel research question in the evidence-based PICO format
- Receive expert faculty review
Individual Assignment 2
Design, Implement and Present an Original Abstract
- Design and implement a clinical research study based on a publicly available dataset
- Analyze and create data visualizations via a user-friendly R Shiny web app
- Write a formal 350-word abstract suitable for submission to an international conference
- Present a digital poster to faculty at Workshop 3
Online Lectures
Research Study Introduction
- Designing a Clinical Research Study I–III
- Introduction to Evidence-Based Medicine, Systematic Review and Meta-Analysis
- Study Design 1 – Observational
- Study Design 2 – Randomized Controlled Trials
- Study Design 3 – Quasi-Experimental Studies
- Introduction to Biostatistics
- An Investigator’s Responsibility for Protection of Research Subjects
- How to Search PubMed
- Overview of Evidence-Based Medicine
Statistical Programming in Stata
- Loading Data
- Basic Programming Commands
- Data Cleansing
- Data Analytics I – Central Tendency
- Data Analytics II – Statistical Testing
- Data Analytics III – Regression Testing
Instructors
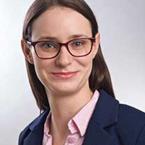
Jamie Robertson
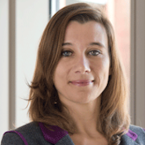
Djøra Soeteman
You may also like.
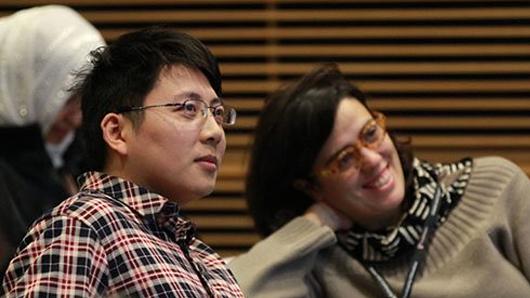
Global Clinical Scholars Research Training
This Harvard Medical School one-year, application-based certificate program provides advanced training in health care research and methods.
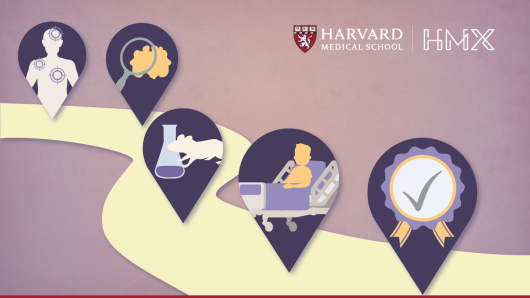
Clinical Drug Development
Learning about the process of clinical drug development has important implications for anyone working in health care and related sectors.
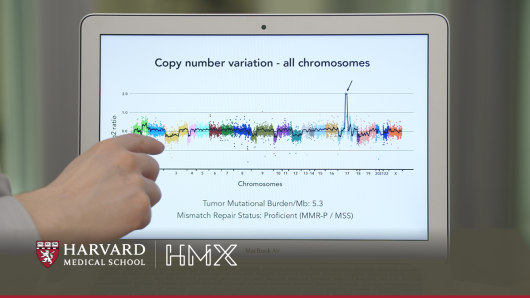
Cancer Genomics and Precision Oncology
Learn how cancer treatment is evolving due to advances in genetics., join our list to learn more.
Skip to content
Read the latest news stories about Mailman faculty, research, and events.
Departments
We integrate an innovative skills-based curriculum, research collaborations, and hands-on field experience to prepare students.
Learn more about our research centers, which focus on critical issues in public health.
Our Faculty
Meet the faculty of the Mailman School of Public Health.
Become a Student
Life and community, how to apply.
Learn how to apply to the Mailman School of Public Health.
Clinical Research Methods
Director: Todd Ogden, PhD
The Mailman School offers the degree of Master of Science in Biostatistics, with an emphasis on issues in the statistical analysis and design of clinical studies. The Clinical Research Methods track was conceived and designed for clinicians who are pursuing research careers in academic medicine. Candidacy in the CRM program is open to anyone who holds a medical/doctoral degree and/or has several years of clinical research experience.
Competencies
In addition to achieving the MS in Biostatistics core competencies, graduates of the 30 credit MS Clinical Research Methods Track develop specific competencies in data analysis and computing, public health and collaborative research, and data management. MS/CRM graduates will be able to:
Data Analysis and Computing
- Apply the basic tenets of research design and analysis for the purpose of critically reviewing research and programs in disciplines outside of biostatistics;
- Differentiate between quantitative problems that can be addressed with standard methods and those requiring input from a professional biostatistician.
Public Health and Collaborative Research
- Formulate and prepare a written statistical plan for analysis of public health research data that clearly reflects the research hypotheses of the proposal in a manner that resonates with both co-investigators and peer reviewers;
- Prepare written summaries of quantitative analyses for journal publication, presentations at scientific meetings, grant applications, and review by regulatory agencies;
Data Management
- Identify the uses to which data management can be put in practical statistical analysis, including the establishment of standards for documentation, archiving, auditing, and confidentiality; guidelines for accessibility; security; structural issues; and data cleaning;
- Differentiate between analytical and data management functions through knowledge of the role and functions of databases, different types of data storage, and the advantages and limitations of rigorous database systems in conjunction with statistical tools;
- Describe the different types of database management systems, the ways these systems can provide data for analysis and interact with statistical software, and methods for evaluating technologies pertinent to both; and
- Assess database tools and the database functions of statistical software, with a view to explaining the impact of data management processes and procedures on their own research.
Required Courses
The required courses enable degree candidates to gain proficiency in study design, application of commonly-used statistical procedures, use of statistical software packages, and successful interpretation and communication of analysis results. A required course may be waived for students with demonstrated expertise in that field of study. If a student places out of one or more required courses, that student must substitute other courses, perhaps a more advanced course in the same area or another elective course in biostatistics or another discipline, with the approval of the student’s faculty advisor.
The program, which consists of 30 credits of coursework and research, may be completed in one year, provided the candidate begins study during the summer semester of his or her first year. If preferred, candidates may pursue the MS/CRM on a part-time basis. The degree program must be completed within five years of the start date.
The curriculum, described below, is comprised of 24 credits of required courses, including a 3-credit research project (the “Master’s essay”) to be completed during the final year of study, and two electives of 6 credits. Note that even if a course is waived, students must still complete a minimum of 30 credits to be awarded the MS degree.
Commonly chosen elective courses include:
Master's Essay
As part of MS/CRM training, each student is required to register for the 3-credit Master's essay course (P9160). This course provides direct support and supervision for the completion of the required research project, or Master's essay, consisting of a research paper of publishable quality. CRM candidates should register for the Master's essay during the spring semester of their final year of study. Students are required to come to the Master's essay course with research data in hand for analysis and interpretation.
CRM graduates have written excellent Master's essays over the years, many of which were ultimately published in the scientific literature. Some titles include:
- A Comprehensive Analysis of the Natural History and the Effect of Treatment on Patients with Malignant Pleural Mesothelioma
- Prevalence and Modification of Cardiovascular Risk Factors in Early Chronic Kidney Disease: Data from the Third National Health and Nutrition Examination Survey
- Perspectives on Pediatric Outcomes: A Comparison of Parents' and Children's Ratings of Health-Related Quality of Life
- Clinical and Demographic Profiles of Cancer Discharges throughout New York State Compared to Corresponding Incidence Rates, 1990-1994
Sample Timeline
Candidates may choose to complete the CRM program track on a part-time basis, or complete all requirements within one year (July through May). To complete the degree in one year, coursework must commence during the summer term.
Note that course schedules change from year to year, so that class days/times in future years will differ from the sample schedule below; you must check the current course schedule for each year on the course directory page .
Paul McCullough Director of Academic Programs Department of Biostatistics Columbia University [email protected] 212-342-3417
More information on Admission Requirements and Deadlines.

- Weill Cornell Medicine
Wayfinder menu
- National CTSA
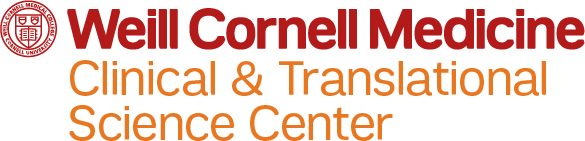
Clinical Research Methodology Curriculum
Application instructions.

The Clinical Research Methodology Curriculum (CRMC) is a one-year clinical research methodology for investigators with clinical research experience seeking to obtain up-to-date knowledge in the field of clinical research. It is conducted at Memorial Sloan Kettering Cancer Center to promote greater flexibility for trainees from across the CTSC partner institutes. The CRMC curriculum allow participants to either enroll in the entire program or audit specific components that address self-identified educational needs.
The Clinical Research Methodology Curriculum is currently accepting applications for the 2023-2024 academic year. The application deadline to submit is Friday, August 18, 2023 at 5:00PM .

Clinical & Translational Science Center 1300 York Ave., Box 149 New York, NY 10065
- Open access
- Published: 16 May 2024
Integrating qualitative research within a clinical trials unit: developing strategies and understanding their implementation in contexts
- Jeremy Segrott ORCID: orcid.org/0000-0001-6215-0870 1 ,
- Sue Channon 2 ,
- Amy Lloyd 4 ,
- Eleni Glarou 2 , 3 ,
- Josie Henley 5 ,
- Jacqueline Hughes 2 ,
- Nina Jacob 2 ,
- Sarah Milosevic 2 ,
- Yvonne Moriarty 2 ,
- Bethan Pell 6 ,
- Mike Robling 2 ,
- Heather Strange 2 ,
- Julia Townson 2 ,
- Qualitative Research Group &
- Lucy Brookes-Howell 2
Trials volume 25 , Article number: 323 ( 2024 ) Cite this article
6 Altmetric
Metrics details
Background/aims
The value of using qualitative methods within clinical trials is widely recognised. How qualitative research is integrated within trials units to achieve this is less clear. This paper describes the process through which qualitative research has been integrated within Cardiff University’s Centre for Trials Research (CTR) in Wales, UK. We highlight facilitators of, and challenges to, integration.
We held group discussions on the work of the Qualitative Research Group (QRG) within CTR. The content of these discussions, materials for a presentation in CTR, and documents relating to the development of the QRG were interpreted at a workshop attended by group members. Normalisation Process Theory (NPT) was used to structure analysis. A writing group prepared a document for input from members of CTR, forming the basis of this paper.
Actions to integrate qualitative research comprised: its inclusion in Centre strategies; formation of a QRG with dedicated funding/roles; embedding of qualitative research within operating systems; capacity building/training; monitoring opportunities to include qualitative methods in studies; maximising the quality of qualitative research and developing methodological innovation. Facilitators of these actions included: the influence of the broader methodological landscape within trial/study design and its promotion of the value of qualitative research; and close physical proximity of CTR qualitative staff/students allowing sharing of methodological approaches. Introduction of innovative qualitative methods generated interest among other staff groups. Challenges included: pressure to under-resource qualitative components of research, preference for a statistical stance historically in some research areas and funding structures, and difficulties faced by qualitative researchers carving out individual academic profiles when working across trials/studies.
Conclusions
Given that CTUs are pivotal to the design and conduct of RCTs and related study types across multiple disciplines, integrating qualitative research into trials units is crucial if its contribution is to be fully realised. We have made explicit one trials unit’s experience of embedding qualitative research and present this to open dialogue on ways to operationalise and optimise qualitative research in trials. NPT provides a valuable framework with which to theorise these processes, including the importance of sense-making and legitimisation when introducing new practices within organisations.
Peer Review reports
The value of using qualitative methods within randomised control trials (RCTs) is widely recognised [ 1 , 2 , 3 ]. Qualitative research generates important evidence on factors affecting trial recruitment/retention [ 4 ] and implementation, aiding interpretation of quantitative data [ 5 ]. Though RCTs have traditionally been viewed as sitting within a positivist paradigm, recent methodological innovations have developed new trial designs that draw explicitly on both quantitative and qualitative methods. For instance, in the field of complex public health interventions, realist RCTs seek to understand the mechanisms through which interventions generate hypothesised impacts, and how interactions across different implementation contexts form part of these mechanisms. Proponents of realist RCTs—which integrate experimental and realist paradigms—highlight the importance of using quantitative and qualitative methods to fully realise these aims and to generate an understanding of intervention mechanisms and how context shapes them [ 6 ].
A need for guidance on how to conduct good quality qualitative research is being addressed, particularly in relation to feasibility studies for RCTs [ 7 ] and process evaluations embedded within trials of complex interventions [ 5 ]. There is also guidance on the conduct of qualitative research within trials at different points in the research cycle, including development, conduct and reporting [ 8 , 9 ].
A high proportion of trials are based within or involve clinical trials units (CTUs). In the UK the UKCRC Registered CTU Network describes them as:
… specialist units which have been set up with a specific remit to design, conduct, analyse and publish clinical trials and other well-designed studies. They have the capability to provide specialist expert statistical, epidemiological, and other methodological advice and coordination to undertake successful clinical trials. In addition, most CTUs will have expertise in the coordination of trials involving investigational medicinal products which must be conducted in compliance with the UK Regulations governing the conduct of clinical trials resulting from the EU Directive for Clinical Trials.
Thus, CTUs provide the specialist methodological expertise needed for the conduct of trials, and in the case of trials of investigational medicinal products, their involvement may be mandated to ensure compliance with relevant regulations. As the definition above suggests, CTUs also conduct and support other types of study apart from RCTs, providing a range of methodological and subject-based expertise.
However, despite their central role in the conduct and design of trials, (and other evaluation designs) little has been written about how CTUs have integrated qualitative work within their organisation at a time when such methods are, as stated above, now recognised as an important aspect of RCTs and evaluation studies more generally. This is a significant gap, since integration at the organisational level arguably shapes how qualitative research is integrated within individual studies, and thus it is valuable to understand how CTUs have approached the task. There are different ways of involving qualitative work in trials units, such as partnering with other departments (e.g. social science) or employing qualitative researchers directly. Qualitative research can be imagined and configured in different ways—as a method that generates data to inform future trial and intervention design, as an embedded component within an RCT or other evaluation type, or as a parallel strand of research focusing on lived experiences of illness, for instance. Understanding how trials units have integrated qualitative research is valuable, as it can shed light on which strategies show promise, and in which contexts, and how qualitative research is positioned within the field of trials research, foregrounding the value of qualitative research. However, although much has been written about its use within trials, few accounts exist of how trials units have integrated qualitative research within their systems and structures.
This paper discusses the process of embedding qualitative research within the work of one CTU—Cardiff University’s Centre for Trials Research (CTR). It highlights facilitators of this process and identifies challenges to integration. We use the Normalisation Process Theory (NPT) as a framework to structure our experience and approach. The key gap addressed by this paper is the implementation of strategies to integrate qualitative research (a relatively newly adopted set of practices and processes) within CTU systems and structures. We acknowledge from the outset that there are multiple ways of approaching this task. What follows therefore is not a set of recommendations for a preferred or best way to integrate qualitative research, as this will comprise diverse actions according to specific contexts. Rather, we examine the processes through which integration occurred in our own setting and highlight the potential value of these insights for others engaged in the work of promoting qualitative research within trials units.
Background to the integration of qualitative research within CTR
The CTR was formed in 2015 [ 10 ]. It brought together three existing trials units at Cardiff University: the South East Wales Trials Unit, the Wales Cancer Trials Unit, and the Haematology Clinical Trials Unit. From its inception, the CTR had a stated aim of developing a programme of qualitative research and integrating it within trials and other studies. In the sections below, we map these approaches onto the framework offered by Normalisation Process Theory to understand the processes through which they helped achieve embedding and integration of qualitative research.
CTR’s aims (including those relating to the development of qualitative research) were included within its strategy documents and communicated to others through infrastructure funding applications, annual reports and its website. A Qualitative Research Group (QRG), which had previously existed within the South East Wales Trials Unit, with dedicated funding for methodological specialists and group lead academics, was a key mechanism through which the development of a qualitative portfolio was put into action. Integration of qualitative research within Centre systems and processes occurred through the inclusion of qualitative research in study adoption processes and representation on committees. The CTR’s study portfolio provided a basis to track qualitative methods in new and existing studies, identify opportunities to embed qualitative methods within recently adopted studies (at the funding application stage) and to manage staff resources. Capacity building and training were an important focus of the QRG’s work, including training courses, mentoring, creation of an academic network open to university staff and practitioners working in the field of healthcare, presentations at CTR staff meetings and securing of PhD studentships. Standard operating procedures and methodological guidance on the design and conduct of qualitative research (e.g. templates for developing analysis plans) aimed to create a shared understanding of how to undertake high-quality research, and a means to monitor the implementation of rigorous approaches. As the QRG expanded its expertise it sought to develop innovative approaches, including the use of visual [ 11 ] and ethnographic methods [ 12 ].
Understanding implementation—Normalisation Process Theory (NPT)
Normalisation Process Theory (NPT) provides a model with which to understand the implementation of new sets of practices and their normalisation within organisational settings. The term ‘normalisation’ refers to how new practices become routinised (part of the everyday work of an organisation) through embedding and integration [ 13 , 14 ]. NPT defines implementation as ‘the social organisation of work’ and is concerned with the social processes that take place as new practices are introduced. Embedding involves ‘making practices routine elements of everyday life’ within an organisation. Integration takes the form of ‘sustaining embedded practices in social contexts’, and how these processes lead to the practices becoming (or not becoming) ‘normal and routine’ [ 14 ]. NPT is concerned with the factors which promote or ‘inhibit’ attempts to embed and integrate the operationalisation of new practices [ 13 , 14 , 15 ].
Embedding new practices is therefore achieved through implementation—which takes the form of interactions in specific contexts. Implementation is operationalised through four ‘generative mechanisms’— coherence , cognitive participation , collective action and reflexive monitoring [ 14 ]. Each mechanism is characterised by components comprising immediate and organisational work, with actions of individuals and organisations (or groups of individuals) interdependent. The mechanisms operate partly through forms of investment (i.e. meaning, commitment, effort, and comprehension) [ 14 ].
Coherence refers to how individuals/groups make sense of, and give meaning to, new practices. Sense-making concerns the coherence of a practice—whether it ‘holds together’, and its differentiation from existing activities [ 15 ]. Communal and individual specification involve understanding new practices and their potential benefits for oneself or an organisation. Individuals consider what new practices mean for them in terms of tasks and responsibilities ( internalisation ) [ 14 ].
NPT frames the second mechanism, cognitive participation , as the building of a ‘community of practice’. For a new practice to be initiated, individuals and groups within an organisation must commit to it [ 14 , 15 ]. Cognitive participation occurs through enrolment —how people relate to the new practice; legitimation —the belief that it is right for them to be involved; and activation —defining which actions are necessary to sustain the practice and their involvement [ 14 ]. Making the new practices work may require changes to roles (new responsibilities, altered procedures) and reconfiguring how colleagues work together (changed relationships).
Third, Collective Action refers to ‘the operational work that people do to enact a set of practices’ [ 14 ]. Individuals engage with the new practices ( interactional workability ) reshaping how members of an organisation interact with each other, through creation of new roles and expectations ( relational interaction ) [ 15 ]. Skill set workability concerns how the work of implementing a new set of practices is distributed and the necessary roles and skillsets defined [ 14 ]. Contextual integration draws attention to the incorporation of a practice within social contexts, and the potential for aspects of these contexts, such as systems and procedures, to be modified as a result [ 15 ].
Reflexive monitoring is the final implementation mechanism. Collective and individual appraisal evaluate the value of a set of practices, which depends on the collection of information—formally and informally ( systematisation ). Appraisal may lead to reconfiguration in which procedures of the practice are redefined or reshaped [ 14 , 15 ].
We sought to map the following: (1) the strategies used to embed qualitative research within the Centre, (2) key facilitators, and (3) barriers to their implementation. Through focused group discussions during the monthly meetings of the CTR QRG and in discussion with the CTR senior management team throughout 2019–2020 we identified nine types of documents (22 individual documents in total) produced within the CTR which had relevant information about the integration of qualitative research within its work (Table 1 ). The QRG had an ‘open door’ policy to membership and welcomed all staff/students with an interest in qualitative research. It included researchers who were employed specifically to undertake qualitative research and other staff with a range of study roles, including trial managers, statisticians, and data managers. There was also diversity in terms of career stage, including PhD students, mid-career researchers and members of the Centre’s Executive team. Membership was therefore largely self-selected, and comprised of individuals with a role related to, or an interest in, embedding qualitative research within trials. However, the group brought together diverse methodological perspectives and was not solely comprised of methodological ‘champions’ whose job it was to promote the development of qualitative research within the centre. Thus whilst the group (and by extension, the authors of this paper) had a shared appreciation of the value of qualitative research within a trials centre, they also brought varied methodological perspectives and ways of engaging with it.
All members of the QRG ( n = 26) were invited to take part in a face-to-face, day-long workshop in February 2019 on ‘How to optimise and operationalise qualitative research in trials: reflections on CTR structure’. The workshop was attended by 12 members of staff and PhD students, including members of the QRG and the CTR’s senior management team. Recruitment to the workshop was therefore inclusive, and to some extent opportunistic, but all members of the QRG were able to contribute to discussions during regular monthly group meetings and the drafting of the current paper.
The aim of the workshop was to bring together information from the documents in Table 1 to generate discussion around the key strategies (and their component activities) that had been adopted to integrate qualitative research into CTR, as well as barriers to, and facilitators of, their implementation. The agenda for the workshop involved four key areas: development and history of the CTR model; mapping the current model within CTR; discussing the structure of other CTUs; and exploring the advantages and disadvantages of the CTR model.
During the workshop, we discussed the use of NPT to conceptualise how qualitative research had been embedded within CTR’s systems and practices. The group produced spider diagrams to map strategies and actions on to the four key domains (or ‘generative mechanisms’ of NPT) summarised above, to aid the understanding of how they had functioned, and the utility of NPT as a framework. This is summarised in Table 2 .
Detailed notes were made during the workshop. A core writing group then used these notes and the documents in Table 1 to develop a draft of the current paper. This was circulated to all members of the CTR QRG ( n = 26) and stored within a central repository accessible to them to allow involvement and incorporate the views of those who were not able to attend the workshop. This draft was again presented for comments in the monthly CTR QRG meeting in February 2021 attended by n = 10. The Standards for QUality Improvement Reporting Excellence 2.0 (SQUIRE) guidelines were used to inform the structure and content of the paper (see supplementary material) [ 16 ].
In the following sections, we describe the strategies CTR adopted to integrate qualitative research. These are mapped against NPT’s four generative mechanisms to explore the processes through which the strategies promoted integration, and facilitators of and barriers to their implementation. A summary of the strategies and their functioning in terms of the generative mechanisms is provided in Table 2 .
Coherence—making sense of qualitative research
In CTR, many of the actions taken to build a portfolio of qualitative research were aimed at enabling colleagues, and external actors, to make sense of this set of methodologies. Centre-level strategies and grant applications for infrastructure funding highlighted the value of qualitative research, the added benefits it would bring, and positioned it as a legitimate set of practices alongside existing methods. For example, a 2014 application for renewal of trials unit infrastructure funding stated:
We are currently in the process of undertaking […] restructuring for our qualitative research team and are planning similar for trial management next year. The aim of this restructuring is to establish greater hierarchical management and opportunities for staff development and also provide a structure that can accommodate continuing growth.
Within the CTR, various forms of communication on the development of qualitative research were designed to enable staff and students to make sense of it, and to think through its potential value for them, and ways in which they might engage with it. These included presentations at staff meetings, informal meetings between project teams and the qualitative group lead, and the visibility of qualitative research on the public-facing Centre website and Centre committees and systems. For instance, qualitative methods were included (and framed as a distinct set of practices) within study adoption forms and committee agendas. Information for colleagues described how qualitative methods could be incorporated within funding applications for RCTs and other evaluation studies to generate new insights into questions research teams were already keen to answer, such as influences on intervention implementation fidelity. Where externally based chief investigators approached the Centre to be involved in new grant applications, the existence of the qualitative team and group lead enabled the inclusion of qualitative research to be actively promoted at an early stage, and such opportunities were highlighted in the Centre’s brochure for new collaborators. Monthly qualitative research network meetings—advertised across CTR and to external research collaborators, were also designed to create a shared understanding of qualitative research methods and their utility within trials and other study types (e.g. intervention development, feasibility studies, and observational studies). Training events (discussed in more detail below) also aided sense-making.
Several factors facilitated the promotion of qualitative research as a distinctive and valuable entity. Among these was the influence of the broader methodological landscape within trial design which was promoting the value of qualitative research, such as guidance on the evaluation of complex interventions by the Medical Research Council [ 17 ], and the growing emphasis placed on process evaluations within trials (with qualitative methods important in understanding participant experience and influences on implementation) [ 5 ]. The attention given to lived experience (both through process evaluations and the move to embed public involvement in trials) helped to frame qualitative research within the Centre as something that was appropriate, legitimate, and of value. Recognition by research funders of the value of qualitative research within studies was also helpful in normalising and legitimising its adoption within grant applications.
The inclusion of qualitative methods within influential methodological guidance helped CTR researchers to develop a ‘shared language’ around these methods, and a way that a common understanding of the role of qualitative research could be generated. One barrier to such sense-making work was the varying extent to which staff and teams had existing knowledge or experience of qualitative research. This varied across methodological and subject groups within the Centre and reflected the history of the individual trials units which had merged to form the Centre.
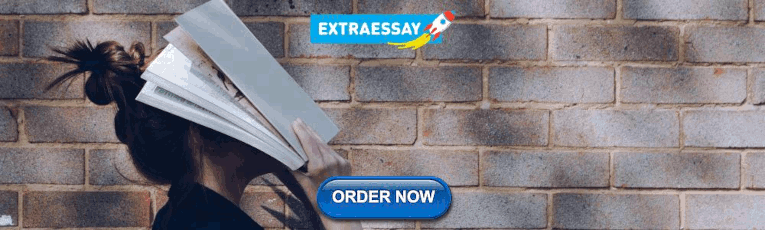
Cognitive participation—legitimising qualitative research
Senior CTR leaders promoted the value and legitimacy of qualitative research. Its inclusion in centre strategies, infrastructure funding applications, and in public-facing materials (e.g. website, investigator brochures), signalled that it was appropriate for individuals to conduct qualitative research within their roles, or to support others in doing so. Legitimisation also took place through informal channels, such as senior leadership support for qualitative research methods in staff meetings and participation in QRG seminars. Continued development of the QRG (with dedicated infrastructure funding) provided a visible identity and equivalence with other methodological groups (e.g. trial managers, statisticians).
Staff were asked to engage with qualitative research in two main ways. First, there was an expansion in the number of staff for whom qualitative research formed part of their formal role and responsibilities. One of the three trials units that merged to form CTR brought with it a qualitative team comprising methodological specialists and a group lead. CTR continued the expansion of this group with the creation of new roles and an enlarged nucleus of researchers for whom qualitative research was the sole focus of their work. In part, this was linked to the successful award of projects that included a large qualitative component, and that were coordinated by CTR (see Table 3 which describes the PUMA study).
Members of the QRG were encouraged to develop their own research ideas and to gain experience as principal investigators, and group seminars were used to explore new ideas and provide peer support. This was communicated through line management, appraisal, and informal peer interaction. Boundaries were not strictly demarcated (i.e. staff located outside the qualitative team were already using qualitative methods), but the new team became a central focus for developing a growing programme of work.
Second, individuals and studies were called upon to engage in new ways with qualitative research, and with the qualitative team. A key goal for the Centre was that groups developing new research ideas should give more consideration in general to the potential value and inclusion of qualitative research within their funding applications. Specifically, they were asked to do this by thinking about qualitative research at an early point in their application’s development (rather than ‘bolting it on’ after other elements had been designed) and to draw upon the expertise and input of the qualitative team. An example was the inclusion of questions on qualitative methods within the Centre’s study adoption form and representation from the qualitative team at the committee which reviewed new adoption requests. Where adoption requests indicated the inclusion of qualitative methods, colleagues were encouraged to liaise with the qualitative team, facilitating the integration of its expertise from an early stage. Qualitative seminars offered an informal and supportive space in which researchers could share initial ideas and refine their methodological approach. The benefits of this included the provision of sufficient time for methodological specialists to be involved in the design of the proposed qualitative component and ensuring adequate costings had been drawn up. At study adoption group meetings, scrutiny of new proposals included consideration of whether new research proposals might be strengthened through the use of qualitative methods where these had not initially been included. Meetings of the QRG—which reviewed the Centre’s portfolio of new studies and gathered intelligence on new ideas—also helped to identify, early on, opportunities to integrate qualitative methods. Communication across teams was useful in identifying new research ideas and embedding qualitative researchers within emerging study development groups.
Actions to promote greater use of qualitative methods in funding applications fed through into a growing number of studies with a qualitative component. This helped to increase the visibility and legitimacy of qualitative methods within the Centre. For example, the PUMA study [ 12 ], which brought together a large multidisciplinary team to develop and evaluate a Paediatric early warning system, drew heavily on qualitative methods, with the qualitative research located within the QRG. The project introduced an extensive network of collaborators and clinical colleagues to qualitative methods and how they could be used during intervention development and the generation of case studies. Further information about the PUMA study is provided in Table 3 .
Increasing the legitimacy of qualitative work across an extensive network of staff, students and collaborators was a complex process. Set within the continuing dominance of quantitative methods with clinical trials, there were variations in the extent to which clinicians and other collaborators embraced the value of qualitative methods. Research funding schemes, which often continued to emphasise the quantitative element of randomised controlled trials, inevitably fed through into the focus of new research proposals. Staff and external collaborators were sometimes uncertain about the added value that qualitative methods would bring to their trials. Across the CTR there were variations in the speed at which qualitative research methods gained legitimacy, partly based on disciplinary traditions and their influences. For instance, population health trials, often located within non-health settings such as schools or community settings, frequently involved collaboration with social scientists who brought with them experience in qualitative methods. Methodological guidance in this field, such as MRC guidance on process evaluations, highlighted the value of qualitative methods and alternatives to the positivist paradigm, such as the value of realist RCTs. In other, more clinical areas, positivist paradigms had greater dominance. Established practices and methodological traditions across different funders also influenced the ease of obtaining funding to include qualitative research within studies. For drugs trials (CTIMPs), the influence of regulatory frameworks on study design, data collection and the allocation of staff resources may have played a role. Over time, teams gained repeated experience of embedding qualitative research (and researchers) within their work and took this learning with them to subsequent studies. For example, the senior clinician quoted within the PUMA case study (Table 3 below) described how they had gained an appreciation of the rigour of qualitative research and an understanding of its language. Through these repeated interactions, embedding of qualitative research within studies started to become the norm rather than the exception.
Collective action—operationalising qualitative research
Collective action concerns the operationalisation of new practices within organisations—the allocation and management of the work, how individuals interact with each other, and the work itself. In CTR the formation of a Qualitative Research Group helped to allocate and organise the work of building a portfolio of studies. Researchers across the Centre were called upon to interact with qualitative research in new ways. Presentations at staff meetings and the inclusion of qualitative research methods in portfolio study adoption forms were examples of this ( interactive workability ). It was operationalised by encouraging study teams to liaise with the qualitative research lead. Development of standard operating procedures, templates for costing qualitative research and methodological guidance (e.g. on analysis plans) also helped encourage researchers to interact with these methods in new ways. For some qualitative researchers who had been trained in the social sciences, working within a trials unit meant that they needed to interact in new and sometimes unfamiliar ways with standard operating procedures, risk assessments, and other trial-based systems. Thus, training needs and capacity-building efforts were multidirectional.
Whereas there had been a tendency for qualitative research to be ‘bolted on’ to proposals for RCTs, the systems described above were designed to embed thinking about the value and design of the qualitative component from the outset. They were also intended to integrate members of the qualitative team with trial teams from an early stage to promote effective integration of qualitative methods within larger trials and build relationships over time.
Standard Operating Procedures (SOPs), formal and informal training, and interaction between the qualitative team and other researchers increased the relational workability of qualitative methods within the Centre—the confidence individuals felt in including these methods within their studies, and their accountability for doing so. For instance, study adoption forms prompted researchers to interact routinely with the qualitative team at an early stage, whilst guidance on costing grants provided clear expectations about the resources needed to deliver a proposed set of qualitative data collection.
Formation of the Qualitative Research Group—comprised of methodological specialists, created new roles and skillsets ( skill set workability ). Research teams were encouraged to draw on these when writing funding applications for projects that included a qualitative component. Capacity-building initiatives were used to increase the number of researchers with the skills needed to undertake qualitative research, and for these individuals to develop their expertise over time. This was achieved through formal training courses, academic seminars, mentoring from experienced colleagues, and informal knowledge exchange. Links with external collaborators and centres engaged in building qualitative research supported these efforts. Within the Centre, the co-location of qualitative researchers with other methodological and trial teams facilitated knowledge exchange and building of collaborative relationships, whilst grouping of the qualitative team within a dedicated office space supported a collective identity and opportunities for informal peer support.
Some aspects of the context in which qualitative research was being developed created challenges to operationalisation. Dependence on project grants to fund qualitative methodologists meant that there was a continuing need to write further grant applications whilst limiting the amount of time available to do so. Similarly, researchers within the team whose role was funded largely by specific research projects could sometimes find it hard to create sufficient time to develop their personal methodological interests. However, the cultivation of a methodologically varied portfolio of work enabled members of the team to build significant expertise in different approaches (e.g. ethnography, discourse analysis) that connected individual studies.
Reflexive monitoring—evaluating the impact of qualitative research
Inclusion of questions/fields relating to qualitative research within the Centre’s study portfolio database was a key way in which information was collected ( systematisation ). It captured numbers of funding applications and funded studies, research design, and income generation. Alongside this database, a qualitative resource planner spreadsheet was used to link individual members of the qualitative team with projects and facilitate resource planning, further reinforcing the core responsibilities and roles of qualitative researchers within CTR. As with all staff in the Centre, members of the qualitative team were placed on ongoing rather than fixed-term contracts, reflecting their core role within CTR. Planning and strategy meetings used the database and resource planner to assess the integration of qualitative research within Centre research, identify opportunities for increasing involvement, and manage staff recruitment and sustainability of researcher posts. Academic meetings and day-to-day interaction fulfilled informal appraisal of the development of the group, and its position within the Centre. Individual appraisal was also important, with members of the qualitative team given opportunities to shape their role, reflect on progress, identify training needs, and further develop their skillset, particularly through line management systems.
These forms of systematisation and appraisal were used to reconfigure the development of qualitative research and its integration within the Centre. For example, group strategies considered how to achieve long-term integration of qualitative research from its initial embedding through further promoting the belief that it formed a core part of the Centre’s business. The visibility and legitimacy of qualitative research were promoted through initiatives such as greater prominence on the Centre’s website. Ongoing review of the qualitative portfolio and discussion at academic meetings enabled the identification of areas where increased capacity would be helpful, both for qualitative staff, and more broadly within the Centre. This prompted the qualitative group to develop an introductory course to qualitative methods open to all Centre staff and PhD students, aimed at increasing understanding and awareness. As the qualitative team built its expertise and experience it also sought to develop new and innovative approaches to conducting qualitative research. This included the use of visual and diary-based methods [ 11 ] and the adoption of ethnography to evaluate system-level clinical interventions [ 12 ]. Restrictions on conventional face-to-face qualitative data collection due to the COVID-19 pandemic prompted rapid adoption of virtual/online methods for interviews, observation, and use of new internet platforms such as Padlet—a form of digital note board.
In this paper, we have described the work undertaken by one CTU to integrate qualitative research within its studies and organisational culture. The parallel efforts of many trials units to achieve these goals arguably come at an opportune time. The traditional designs of RCTs have been challenged and re-imagined by the increasing influence of realist evaluation [ 6 , 18 ] and the widespread acceptance that trials need to understand implementation and intervention theory as well as assess outcomes [ 17 ]. Hence the widespread adoption of embedded mixed methods process evaluations within RCTs. These broad shifts in methodological orthodoxies, the production of high-profile methodological guidance, and the expectations of research funders all create fertile ground for the continued expansion of qualitative methods within trials units. However, whilst much has been written about the importance of developing qualitative research and the possible approaches to integrating qualitative and quantitative methods within studies, much less has been published on how to operationalise this within trials units. Filling this lacuna is important. Our paper highlights how the integration of a new set of practices within an organisation can become embedded as part of its ‘normal’ everyday work whilst also shaping the practices being integrated. In the case of CTR, it could be argued that the integration of qualitative research helped shape how this work was done (e.g. systems to assess progress and innovation).
In our trials unit, the presence of a dedicated research group of methodological specialists was a key action that helped realise the development of a portfolio of qualitative research and was perhaps the most visible evidence of a commitment to do so. However, our experience demonstrates that to fully realise the goal of developing qualitative research, much work focuses on the interaction between this ‘new’ set of methods and the organisation into which it is introduced. Whilst the team of methodological specialists was tasked with, and ‘able’ to do the work, the ‘work’ itself needed to be integrated and embedded within the existing system. Thus, alongside the creation of a team and methodological capacity, promoting the legitimacy of qualitative research was important to communicate to others that it was both a distinctive and different entity, yet similar and equivalent to more established groups and practices (e.g. trial management, statistics, data management). The framing of qualitative research within strategies, the messages given out by senior leaders (formally and informally) and the general visibility of qualitative research within the system all helped to achieve this.
Normalisation Process Theory draws our attention to the concepts of embedding (making a new practice routine, normal within an organisation) and integration —the long-term sustaining of these processes. An important process through which embedding took place in our centre concerned the creation of messages and systems that called upon individuals and research teams to interact with qualitative research. Research teams were encouraged to think about qualitative research and consider its potential value for their studies. Critically, they were asked to do so at specific points, and in particular ways. Early consideration of qualitative methods to maximise and optimise their inclusion within studies was emphasised, with timely input from the qualitative team. Study adoption systems, centre-level processes for managing financial and human resources, creation of a qualitative resource planner, and awareness raising among staff, helped to reinforce this. These processes of embedding and integration were complex and they varied in intensity and speed across different areas of the Centre’s work. In part this depended on existing research traditions, the extent of prior experience of working with qualitative researchers and methods, and the priorities of subject areas and funders. Centre-wide systems, sometimes linked to CTR’s operation as a CTU, also helped to legitimise and embed qualitative research, lending it equivalence with other research activity. For example, like all CTUs, CTR was required to conform with the principles of Good Clinical Practice, necessitating the creation of a quality management system, operationalised through standard operating procedures for all areas of its work. Qualitative research was included, and became embedded, within these systems, with SOPs produced to guide activities such as qualitative analysis.
NPT provides a helpful way of understanding how trials units might integrate qualitative research within their work. It highlights how new practices interact with existing organisational systems and the work needed to promote effective interaction. That is, alongside the creation of a team or programme of qualitative research, much of the work concerns how members of an organisation understand it, engage with it, and create systems to sustain it. Embedding a new set of practices may be just as important as the quality or characteristics of the practices themselves. High-quality qualitative research is of little value if it is not recognised and drawn upon within new studies for instance. NPT also offers a helpful lens with which to understand how integration and embedding occur, and the mechanisms through which they operate. For example, promoting the legitimacy of a new set of practices, or creating systems that embed it, can help sustain these practices by creating an organisational ambition and encouraging (or requiring) individuals to interact with them in certain ways, redefining their roles accordingly. NPT highlights the ways in which integration of new practices involves bi-directional exchanges with the organisation’s existing practices, with each having the potential to re-shape the other as interaction takes place. For instance, in CTR, qualitative researchers needed to integrate and apply their methods within the quality management and other systems of a CTU, such as the formalisation of key processes within standard operating procedures, something less likely to occur outside trials units. Equally, project teams (including those led by externally based chief investigators) increased the integration of qualitative methods within their overall study design, providing opportunities for new insights on intervention theory, implementation and the experiences of practitioners and participants.
We note two aspects of the normalisation processes within CTR that are slightly less well conceptualised by NPT. The first concerns the emphasis within coherence on identifying the distinctiveness of new practices, and how they differ from existing activities. Whilst differentiation was an important aspect of the integration of qualitative research in CTR, such integration could be seen as operating partly through processes of de-differentiation, or at least equivalence. That is, part of the integration of qualitative research was to see it as similar in terms of rigour, coherence, and importance to other forms of research within the Centre. To be viewed as similar, or at least comparable to existing practices, was to be legitimised.
Second, whilst NPT focuses mainly on the interaction between a new set of practices and the organisational context into which it is introduced, our own experience of introducing qualitative research into a trials unit was shaped by broader organisational and methodological contexts. For example, the increasing emphasis placed upon understanding implementation processes and the experiences of research participants in the field of clinical trials (e.g. by funders), created an environment conducive to the development of qualitative research methods within our Centre. Attempts to integrate qualitative research within studies were also cross-organisational, given that many of the studies managed within the CTR drew together multi-institutional teams. This provided important opportunities to integrate qualitative research within a portfolio of studies that extended beyond CTR and build a network of collaborators who increasingly included qualitative methods within their funding proposals. The work of growing and integrating qualitative research within a trials unit is an ongoing one in which ever-shifting macro-level influences can help or hinder, and where the organisations within which we work are never static in terms of barriers and facilitators.
The importance of utilising qualitative methods within RCTs is now widely recognised. Increased emphasis on the evaluation of complex interventions, the influence of realist methods directing greater attention to complexity and the widespread adoption of mixed methods process evaluations are key drivers of this shift. The inclusion of qualitative methods within individual trials is important and previous research has explored approaches to their incorporation and some of the challenges encountered. Our paper highlights that the integration of qualitative methods at the organisational level of the CTU can shape how they are taken up by individual trials. Within CTR, it can be argued that qualitative research achieved high levels of integration, as conceptualised by Normalisation Process Theory. Thus, qualitative research became recognised as a coherent and valuable set of practices, secured legitimisation as an appropriate focus of individual and organisational activity and benefitted from forms of collective action which operationalised these organisational processes. Crucially, the routinisation of qualitative research appeared to be sustained, something which NPT suggests helps define integration (as opposed to initial embedding). However, our analysis suggested that the degree of integration varied by trial area. This variation reflected a complex mix of factors including disciplinary traditions, methodological guidance, existing (un)familiarity with qualitative research, and the influence of regulatory frameworks for certain clinical trials.
NPT provides a valuable framework with which to understand how these processes of embedding and integration occur. Our use of NPT draws attention to the importance of sense-making and legitimisation as important steps in introducing a new set of practices within the work of an organisation. Integration also depends, across each mechanism of NPT, on the building of effective relationships, which allow individuals and teams to work together in new ways. By reflecting on our experiences and the decisions taken within CTR we have made explicit one such process for embedding qualitative research within a trials unit, whilst acknowledging that approaches may differ across trials units. Mindful of this fact, and the focus of the current paper on one trials unit’s experience, we do not propose a set of recommendations for others who are working to achieve similar goals. Rather, we offer three overarching reflections (framed by NPT) which may act as a useful starting point for trials units (and other infrastructures) seeking to promote the adoption of qualitative research.
First, whilst research organisations such as trials units are highly heterogenous, processes of embedding and integration, which we have foregrounded in this paper, are likely to be important across different contexts in sustaining the use of qualitative research. Second, developing a plan for the integration of qualitative research will benefit from mapping out the characteristics of the extant system. For example, it is valuable to know how familiar staff are with qualitative research and any variations across teams within an organisation. Thirdly, NPT frames integration as a process of implementation which operates through key generative mechanisms— coherence , cognitive participation , collective action and reflexive monitoring . These mechanisms can help guide understanding of which actions help achieve embedding and integration. Importantly, they span multiple aspects of how organisations, and the individuals within them, work. The ways in which people make sense of a new set of practices ( coherence ), their commitment towards it ( cognitive participation ), how it is operationalised ( collective action ) and the evaluation of its introduction ( reflexive monitoring ) are all important. Thus, for example, qualitative research, even when well organised and operationalised within an organisation, is unlikely to be sustained if appreciation of its value is limited, or people are not committed to it.
We present our experience of engaging with the processes described above to open dialogue with other trials units on ways to operationalise and optimise qualitative research in trials. Understanding how best to integrate qualitative research within these settings may help to fully realise the significant contribution which it makes the design and conduct of trials.
Availability of data and materials
Some documents cited in this paper are either freely available from the Centre for Trials Research website or can be requested from the author for correspondence.
O’Cathain A, Thomas KJ, Drabble SJ, Rudolph A, Hewison J. What can qualitative research do for randomised controlled trials? A systematic mapping review. BMJ Open. 2013;3(6):e002889.
Article PubMed PubMed Central Google Scholar
O’Cathain A, Thomas KJ, Drabble SJ, Rudolph A, Goode J, Hewison J. Maximising the value of combining qualitative research and randomised controlled trials in health research: the QUAlitative Research in Trials (QUART) study – a mixed methods study. Health Technol Assess. 2014;18(38):1–197.
Clement C, Edwards SL, Rapport F, Russell IT, Hutchings HA. Exploring qualitative methods reported in registered trials and their yields (EQUITY): systematic review. Trials. 2018;19(1):589.
Hennessy M, Hunter A, Healy P, Galvin S, Houghton C. Improving trial recruitment processes: how qualitative methodologies can be used to address the top 10 research priorities identified within the PRioRiTy study. Trials. 2018;19:584.
Moore GF, Audrey S, Barker M, Bond L, Bonell C, Hardeman W, et al. Process evaluation of complex interventions: Medical Research Council guidance. BMJ. 2015;350(mar19 6):h1258.
Bonell C, Fletcher A, Morton M, Lorenc T, Moore L. Realist randomised controlled trials: a new approach to evaluating complex public health interventions. Soc Sci Med. 2012;75(12):2299–306.
Article PubMed Google Scholar
O’Cathain A, Hoddinott P, Lewin S, Thomas KJ, Young B, Adamson J, et al. Maximising the impact of qualitative research in feasibility studies for randomised controlled trials: guidance for researchers. Pilot Feasibility Stud. 2015;1:32.
Cooper C, O’Cathain A, Hind D, Adamson J, Lawton J, Baird W. Conducting qualitative research within Clinical Trials Units: avoiding potential pitfalls. Contemp Clin Trials. 2014;38(2):338–43.
Rapport F, Storey M, Porter A, Snooks H, Jones K, Peconi J, et al. Qualitative research within trials: developing a standard operating procedure for a clinical trials unit. Trials. 2013;14:54.
Cardiff University. Centre for Trials Research. Available from: https://www.cardiff.ac.uk/centre-for-trials-research . Accessed 10 May 2024.
Pell B, Williams D, Phillips R, Sanders J, Edwards A, Choy E, et al. Using visual timelines in telephone interviews: reflections and lessons learned from the star family study. Int J Qual Methods. 2020;19:160940692091367.
Thomas-Jones E, Lloyd A, Roland D, Sefton G, Tume L, Hood K, et al. A prospective, mixed-methods, before and after study to identify the evidence base for the core components of an effective Paediatric Early Warning System and the development of an implementation package containing those core recommendations for use in th. BMC Pediatr. 2018;18:244.
May C, Finch T, Mair F, Ballini L, Dowrick C, Eccles M, et al. Understanding the implementation of complex interventions in health care: the normalization process model. BMC Health Serv Res. 2007;7:148.
May C, Finch T. Implementing, embedding, and integrating practices: an outline of normalization process theory. Sociology. 2009;43(3):535–54.
Article Google Scholar
May CR, Mair F, Finch T, Macfarlane A, Dowrick C, Treweek S, et al. Development of a theory of implementation and integration: normalization process theory. Implement Sci. 2009;4:29.
Ogrinc G, Davies L, Goodman D, Batalden PB, Davidoff F, Stevens D. SQUIRE 2.0 (Standards for QUality Improvement Reporting Excellence): Revised publication guidelines from a detailed consensus process. BMJ Quality and Safety. 2016;25:986-92.
Craig P, Dieppe P, Macintyre S, Michie S, Nazareth I, Petticrew M. Developing and evaluating complex interventions: the new Medical Research Council guidance. BMJ. 2008;337:a1655.
Jamal F, Fletcher A, Shackleton N, Elbourne D, Viner R, Bonell C. The three stages of building and testing mid-level theories in a realist RCT: a theoretical and methodological case-example. Trials. 2015;16(1):466.
Download references
Acknowledgements
Members of the Centre for Trials Research (CTR) Qualitative Research Group were collaborating authors: C Drew (Senior Research Fellow—Senior Trial Manager, Brain Health and Mental Wellbeing Division), D Gillespie (Director, Infection, Inflammation and Immunity Trials, Principal Research Fellow), R Hale (now Research Associate, School of Social Sciences, Cardiff University), J Latchem-Hastings (now Lecturer and Postdoctoral Fellow, School of Healthcare Sciences, Cardiff University), R Milton (Research Associate—Trial Manager), B Pell (now PhD student, DECIPHer Centre, Cardiff University), H Prout (Research Associate—Qualitative), V Shepherd (Senior Research Fellow), K Smallman (Research Associate), H Stanton (Research Associate—Senior Data Manager). Thanks are due to Kerry Hood and Aimee Grant for their involvement in developing processes and systems for qualitative research within CTR.
No specific grant was received to support the writing of this paper.
Author information
Authors and affiliations.
Centre for Trials Research, DECIPHer Centre, Cardiff University, Neuadd Meirionnydd, Heath Park, Cardiff, CF14 4YS, UK
Jeremy Segrott
Centre for Trials Research, Cardiff University, Neuadd Meirionnydd, Heath Park, Cardiff, CF14 4YS, UK
Sue Channon, Eleni Glarou, Jacqueline Hughes, Nina Jacob, Sarah Milosevic, Yvonne Moriarty, Mike Robling, Heather Strange, Julia Townson & Lucy Brookes-Howell
Division of Population Medicine, School of Medicine, Cardiff University, Neuadd Meirionnydd, Heath Park, Cardiff, CF14 4YS, UK
Eleni Glarou
Wales Centre for Public Policy, Cardiff University, Sbarc I Spark, Maindy Road, Cardiff, CF24 4HQ, UK
School of Social Sciences, Cardiff University, King Edward VII Avenue, Cardiff, CF10 3WA, UK
Josie Henley
DECIPHer Centre, School of Social Sciences, Cardiff University, Sbarc I Spark, Maindy Road, Cardiff, CF24 4HQ, UK
Bethan Pell
You can also search for this author in PubMed Google Scholar
Qualitative Research Group
- , D. Gillespie
- , J. Latchem-Hastings
- , R. Milton
- , V. Shepherd
- , K. Smallman
- & H. Stanton
Contributions
JS contributed to the design of the work and interpretation of data and was responsible for leading the drafting and revision of the paper. SC contributed to the design of the work, the acquisition of data and the drafting and revision of the paper. AL contributed to the design of the work, the acquisition of data and the drafting and revision of the paper. EG contributed to a critical review of the manuscript and provided additional relevant references. JH provided feedback on initial drafts of the paper and contributed to subsequent revisions. JHu provided feedback on initial drafts of the paper and contributed to subsequent revisions. NG provided feedback on initial drafts of the paper and contributed to subsequent revisions. SM was involved in the acquisition and analysis of data and provided a critical review of the manuscript. YM was involved in the acquisition and analysis of data and provided a critical review of the manuscript. MR was involved in the interpretation of data and critical review and revision of the paper. HS contributed to the conception and design of the work, the acquisition and analysis of data, and the revision of the manuscript. JT provided feedback on initial drafts of the paper and contributed to subsequent revisions. LB-H made a substantial contribution to the design and conception of the work, led the acquisition and analysis of data, and contributed to the drafting and revision of the paper.
Corresponding author
Correspondence to Jeremy Segrott .
Ethics declarations
Ethics approval and consent to participate.
Ethical approval was not sought as no personal or identifiable data was collected.
Consent for publication
Competing interests.
All authors are or were members of staff or students in the Centre for Trials Research. JS is an associate editor of Trials .
Additional information
Publisher’s note.
Springer Nature remains neutral with regard to jurisdictional claims in published maps and institutional affiliations.
Supplementary Information
Supplementary material 1., rights and permissions.
Open Access This article is licensed under a Creative Commons Attribution 4.0 International License, which permits use, sharing, adaptation, distribution and reproduction in any medium or format, as long as you give appropriate credit to the original author(s) and the source, provide a link to the Creative Commons licence, and indicate if changes were made. The images or other third party material in this article are included in the article's Creative Commons licence, unless indicated otherwise in a credit line to the material. If material is not included in the article's Creative Commons licence and your intended use is not permitted by statutory regulation or exceeds the permitted use, you will need to obtain permission directly from the copyright holder. To view a copy of this licence, visit http://creativecommons.org/licenses/by/4.0/ . The Creative Commons Public Domain Dedication waiver ( http://creativecommons.org/publicdomain/zero/1.0/ ) applies to the data made available in this article, unless otherwise stated in a credit line to the data.
Reprints and permissions
About this article
Cite this article.
Segrott, J., Channon, S., Lloyd, A. et al. Integrating qualitative research within a clinical trials unit: developing strategies and understanding their implementation in contexts. Trials 25 , 323 (2024). https://doi.org/10.1186/s13063-024-08124-7
Download citation
Received : 20 October 2023
Accepted : 17 April 2024
Published : 16 May 2024
DOI : https://doi.org/10.1186/s13063-024-08124-7
Share this article
Anyone you share the following link with will be able to read this content:
Sorry, a shareable link is not currently available for this article.
Provided by the Springer Nature SharedIt content-sharing initiative
- Qualitative research
- Qualitative methods
- Trials units
- Normalisation Process Theory
- Randomised controlled trials
ISSN: 1745-6215
- Submission enquiries: Access here and click Contact Us
- General enquiries: [email protected]
- Open access
- Published: 24 May 2024
VER-Net: a hybrid transfer learning model for lung cancer detection using CT scan images
- Anindita Saha ORCID: orcid.org/0000-0002-5780-9252 1 ,
- Shahid Mohammad Ganie ORCID: orcid.org/0000-0001-9925-0402 2 ,
- Pijush Kanti Dutta Pramanik ORCID: orcid.org/0000-0001-9438-9309 3 ,
- Rakesh Kumar Yadav ORCID: orcid.org/0000-0002-0151-4981 4 ,
- Saurav Mallik ORCID: orcid.org/0000-0003-4107-6784 5 &
- Zhongming Zhao ORCID: orcid.org/0000-0002-3477-0914 6
BMC Medical Imaging volume 24 , Article number: 120 ( 2024 ) Cite this article
1 Altmetric
Metrics details
Lung cancer is the second most common cancer worldwide, with over two million new cases per year. Early identification would allow healthcare practitioners to handle it more effectively. The advancement of computer-aided detection systems significantly impacted clinical analysis and decision-making on human disease. Towards this, machine learning and deep learning techniques are successfully being applied. Due to several advantages, transfer learning has become popular for disease detection based on image data.
In this work, we build a novel transfer learning model (VER-Net) by stacking three different transfer learning models to detect lung cancer using lung CT scan images. The model is trained to map the CT scan images with four lung cancer classes. Various measures, such as image preprocessing, data augmentation, and hyperparameter tuning, are taken to improve the efficacy of VER-Net. All the models are trained and evaluated using multiclass classifications chest CT images.
The experimental results confirm that VER-Net outperformed the other eight transfer learning models compared with. VER-Net scored 91%, 92%, 91%, and 91.3% when tested for accuracy, precision, recall, and F1-score, respectively. Compared to the state-of-the-art, VER-Net has better accuracy.
VER-Net is not only effectively used for lung cancer detection but may also be useful for other diseases for which CT scan images are available.
Peer Review reports
Introduction
Lung cancer is one of the leading causes of cancer-related deaths globally. It is broadly classified as small and non-small-cell lung cancer [ 1 ]. Lung cancer is a significant contributor to cancer-related deaths worldwide, with the highest mortality rate among all types of cancer. According to the World Health Organization Footnote 1 , cancer is a significant contributor to global mortality, resulting in approximately 10 million fatalities in 2020, which accounts for roughly one out of every six deaths. WHO estimated that one in 16 people would be diagnosed with lung cancer worldwide by 2022. Figure 1 represents the incidence cases and deaths of cancers for both sexes and all age groups worldwide Footnote 2 . The x-axis represents the number of people, while the y-axis denotes the types of cancers. Amongst all cancers, lung cancer has a significantly higher mortality rate. Additionally, when considering the number of incident cases, lung cancer ranks second among all types of cancer.
Roughly one-third of cancer-related deaths can be attributed to tobacco usage, a high body mass index, alcohol consumption, inadequate consumption of fruits and vegetables, and a lack of physical activity [ 2 ]. In addition, international agencies for cancer research have identified several risk factors that contribute to the development of various cancers, including alcohol, dietary exposures, infections, obesity, radiation, and many more that contribute towards cancer diseases. Lung cancer is caused by the abnormal growth of cells that form a tumour and can have serious consequences if left untreated. Early detection and effective treatment can lead to successful cures for many forms of cancer. Also, it is crucial for improving the survival rate and reducing mortality [ 3 ].
Lung cancer is a respiratory illness that affects people of all ages. Symptoms of lung cancer include changes in voice, coughing, chest pain, shortness of breath, weight loss, wheezing, and other painful symptoms [ 4 ]. Non-small-cell lung cancer has various subtypes, including Adenocarcinoma, squamous cell cancer, and large cell carcinoma, and is frequently observed [ 5 ]. However, small-cell lung cancer spreads faster and is often fatal.
Over the decades, clinical pathways and pathological treatments for lung cancer have included chemotherapy, targeted drugs, and immunotherapy [ 6 ]. In hospitals, doctors use different imaging techniques; while chest X-rays are the most cost-effective method of diagnosis, they require skilled radiologists to interpret the images accurately, as these can be complex and may overlap with other lung conditions [ 7 ]. Various lung diagnosis methods exist in the medical industry that use CT (computed tomography), isotopes, X-rays, MRI (magnetic resonance imaging), etc. [ 8 , 9 ].
Manual identification of lung cancer can be a time-consuming process subject to interpretation, causing delays in diagnosis and treatment. Additionally, the severity of the disease infection may not be apparent on X-ray images.
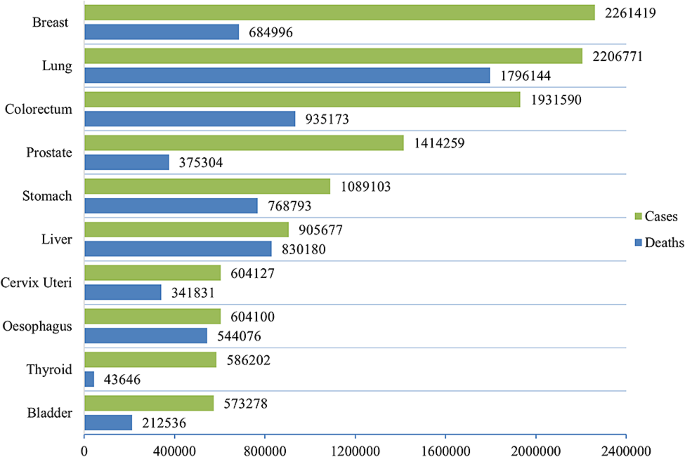
Incident cases and mortality rate of different cancers
As artificial intelligence (AI) has advanced, deep learning has become increasingly popular in analyzing medical images. Deep learning is a technique that can automatically discover high dimensionality, as compared to the more intuitive visual assessment of images that is often performed by skilled clinicians [ 10 , 11 , 12 ]. Convolutional neural networks (CNNs) are promising for extracting more powerful and deeper features from these images [ 13 ]. Significant improvements have been achieved in the potential to identify images and extract features inside images due to the development of CNN [ 14 , 15 ]. Advanced CNNs have been shown to improve the accuracy of predictions significantly. In recent years, the development of computer-aided detection (CAD) has shown promising results in medical image analysis [ 16 , 17 ]. Deep learning techniques, particularly transfer learning, have emerged as a powerful technique for leveraging pre-trained models and improving the performance of deep learning models [ 18 ].
Transfer learning has gained significant attention and success in various fields of AI, including medical image diagnosis [ 19 ], computer vision [ 20 ], natural language processing [ 21 ], speech recognition [ 22 ], and many more. Transfer learning involves using pre-trained neural networks to take the knowledge gained from one task (source task) and apply it to a different but related task (target task) [ 23 ]. In transfer learning, a model pre-trained on a large dataset for a specific task can be fine-tuned on similar datasets for different tasks.
Transfer learning has recently shown much promise in making it easier to detect lung cancer from medical imaging data. Integrating transfer learning methodologies into pipelines for lung cancer detection has demonstrated enhanced accuracy and efficiency across multiple research investigations. It offers a practical and effective way to leverage existing knowledge and resources to develop accurate and efficient models for lung cancer detection. It starts with a pre-trained CNN model and fine-tunes its layers on a dataset of lung images. This allows the model to quickly learn to identify relevant features associated with lung cancer without requiring extensive labelled lung cancer images. The advantages of transfer learning for lung cancer detection are listed in Fig. 2 .
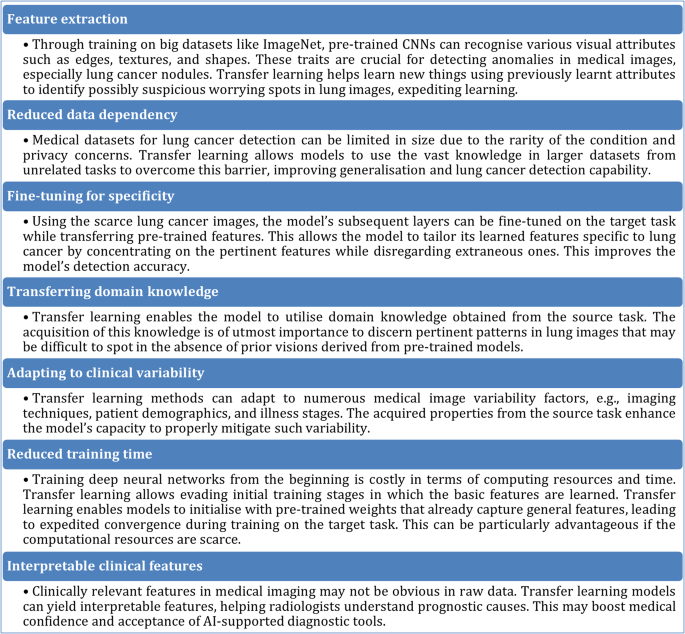
Advantages of transfer learning for lung cancer detection
In this paper, we employed different transfer learning models for lung cancer detection using CT images. We proposed a hybrid model to enhance the prediction capability of the pre-trained models. The key contributions of this paper are:
The original image dataset is resized into 460 × 460 × 3.
Random oversampling is applied to fuse synthetic images in the minority class.
Data augmentation is applied by applying shear_range, zoom_range, rotation_range, horizontal_flip, and vertical_flip.
Eight transfer learning models, viz. NASNetLarge, Xception, DenseNet201, MobileNet, ResNet101, EfficientNetB0, EfficientNetB4, and VGG19 are tried with the processed dataset.
A novel transfer learning model (VER-Net) is built by stacking VGG19, EfficientNetB0, and ResNet101. The outputs of all three models are individually flattened and concatenated afterwards.
Seven deep dense layers are added to optimize the performance of VER-Net.
The performance of VER-Net is validated on eight different matrices (accuracy, loss, precision, recall, F1-score, macro average, weighted average, and standard deviation) and compared with the other eight considered models.
The accuracy of VER-Net is compared with the state-of-the-art.
The rest of the paper is organized as follows. Similar recent research addressing identifying lung cancer through transfer learning is discussed in Sect. 2. The working principle, details of the dataset preparation, and considered transfer learning models are discussed in Sect. 3. Section 4 presents the details of the proposed stacking model, including architecture and parameters. Section 5 presents the proposed model’s experimental details, results, and performance analysis. Section 6 concludes the paper, mentioning the limitations of this study and future scopes.
Related work
Deep learning techniques provide reliable, consistent, and accurate results. Due to this, they are widely applied across multiple domains to solve real-world problems [ 24 , 25 , 26 , 27 ]. Researchers have carried out diverse literature that includes datasets, algorithms, and methodology to facilitate future research in the classification and detection of lung cancer. Some of the prominent attempts to detect lung cancer using transfer learning are discussed in this section.
Wang et al. [ 28 ] experimented with a novel residual neural network with a transfer learning technique to identify pathology in lung cancer subtypes from medical images for an accurate and reliable diagnosis. The suggested model was pre-trained on the public medical image dataset luna16 and fine-tuned using their intellectual property lung cancer dataset from Shandong Provincial Hospital. Their approach accurately identifies pathological lung cancer from CT scans at 85.71%. Han et al. [ 29 ] developed a framework to assess the potential of PET/CT images in distinguishing between different histologic subtypes of non-small cell lung cancer (NSCLC). They evaluated ten feature selection techniques, ten machine learning models, and the VGG16 deep learning algorithm to construct an optimal classification model. The VGG16 achieved the highest accuracy rate of 84.1% among all the models. Vijayan et al. [ 30 ] employed three optimizers with six deep learning models. These models included AlexNet, GoogleNet, ResNet, Inception V3, EfficientNet b0, and SqueezeNet. While evaluating the various models, their effectiveness is measured by comparing their results with a stochastic gradient, momentum, Adam, and RMSProp optimization strategies. According to the findings of their study, GoogleNet using Adam as the optimizer achieves an accuracy of 92.08%. Nóbrega et al. [ 31 ] developed the classification model using deep transfer learning based on CT scan lung images. Several feature extraction models, including VGG16, VGG19, MobileNet, Xception, InceptionV3, ResNet50, Inception-ResNet-V2, DenseNet169, DenseNet201, NASNetMobile and NASNetLarge, were utilized to analyze the Lung Image Database Consortium and Image Database Resource Initiative (LIDC/IDRI). Among all the algorithms, the CNN-ResNet50 and SVM-RBF (support vector machine– radial basis function) combination was found to be the most effective deep extractor and classifier for identifying lung nodule malignancy in chest CT images, achieving an accuracy of 88.41% and an AUC of 93.19%. The authors have calculated the other performance evaluation matrices to validate the proposed model. Dadgar & Neshat [ 32 ] proposed a novel hybrid convolutional deep transfer learning model to detect three common types of lung cancer - Squamous Cell Carcinoma (SCC), Large Cell Carcinoma (LCC), and Adenocarcinoma. The model included several pre-trained deep learning architectures, such as VGG16, ResNet152V2, MobileNetV3 (small and large), InceptionResNetV2, and EfficientNetV2, which were compared and evaluated in combination with fully connected, dropout, and batch-normalization layers, with adjustments made to the hyper-parameters. After preprocessing 1000 CT scans from a public dataset, the best-performing model was identified as InceptionResNetV2 with transfer learning, achieving an accuracy of 91.1%, precision of 84.9%, AUC of 95.8%, and F1-score of 81.5% in classifying three types of lung cancer from normal samples. Worku et al. [ 33 ] proposed a denoising first two-path CNN (DFD-Net) for lung cancer detection. During preprocessing, a residual learning denoising model (DR-Net) is used to remove the noise. Then, a two-path convolutional neural network was used to identify lung cancer, with the denoised image from DR-Net as an input. The combined integration of local and global aspects is the main emphasis of the two pathways. Further, the performance of the model was enhanced, and a method other than the traditional feature concatenation techniques was employed, which directly integrated two sets of features from several CNN layers. Also, the authors overcame image label imbalance difficulties and achieved an accuracy of 87.8% for predicting lung cancer. Sari et al. [ 34 ] implemented CAD system using deep learning on CT images to classify lung cancer. They used transfer learning and a modified ResNet50 architecture to classify lung cancer images into four categories. The results obtained from this modified architecture show an accuracy of 93.33%, sensitivity of 92.75%, precision of 93.75%, F1-score of 93.25%, and AUC of 0.559. The study found that the modified ResNet50 outperforms the other two architectures, EfficientNetB1 and AlexNet, in accurately classifying lung cancer images into Adenocarcinoma, large carcinoma, normal, and squamous carcinoma categories.
Overall, these studies show that transfer learning has the potential to improve how well medical imaging data can be used to find lung cancer. Using pre-trained deep neural networks can significantly reduce the need for large datasets and reduce training time, making them more accessible for clinical applications. However, more research is needed to find the best architecture for transfer learning and the best fine-tuning strategy for spotting lung cancer. Further studies can focus on improving the interpretability and generalization of transfer learning models for real-world applications.
Research methodology
The details of the requirements and experimental steps carried out in this paper are discussed in this section.
The proposed model follows seven phases of structure, as shown in Fig. 3 . After acquiring the chest CT scan images, they were preprocessed and augmented to make the experiment suitable. The processed dataset is divided into training, validation, and testing sets. Eight popular transfer learning models were executed based on this data. Among them, the top three were selected and stacked to build a new prediction model. The model was fine-tuned repeatedly to improve the classification accuracy while reducing the required training time. The model was trained and validated to classify three cancer classes and a normal class. Finally, the model was tested.
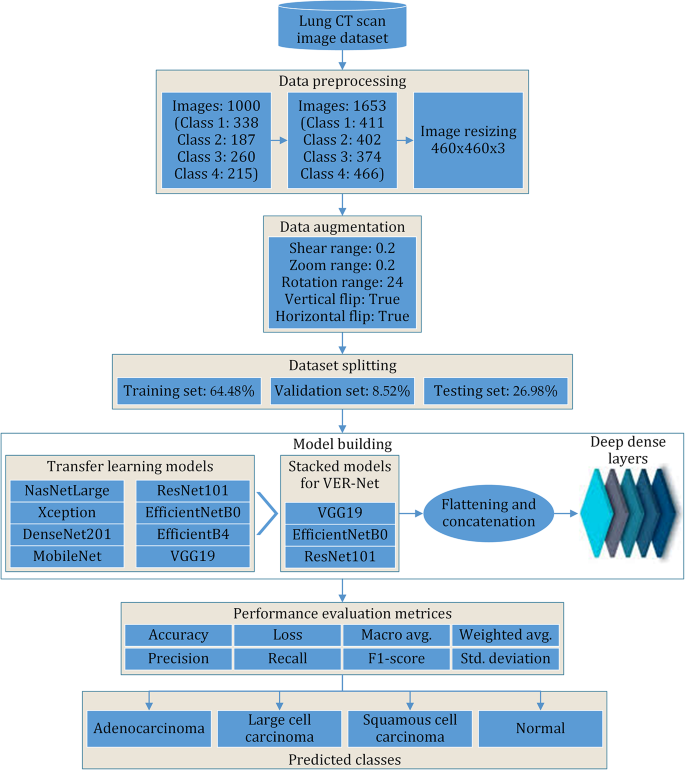
Framework of the proposed methodology
Dataset description
The chest CT images utilized in this study were obtained from Kaggle Footnote 3 . The dataset contains CT scan images of three types of lung cancers: Adenocarcinoma, Large cell carcinoma, and Squamous cell carcinoma. During the cancer prediction process, the lung cancer image dataset taken from Kaggle consists of 1653 CT images, of which 1066 images are used for training, 446 images for testing and the remaining 141 for validation purposes to determine the efficiency of the cancer prediction system. Class-wise samples of lung cancer CT images are depicted in Fig. 4 . The detailed distribution of the dataset in terms of the total images, number of images in each class, number of classes, and labelling in each category is elucidated in Table 1 .
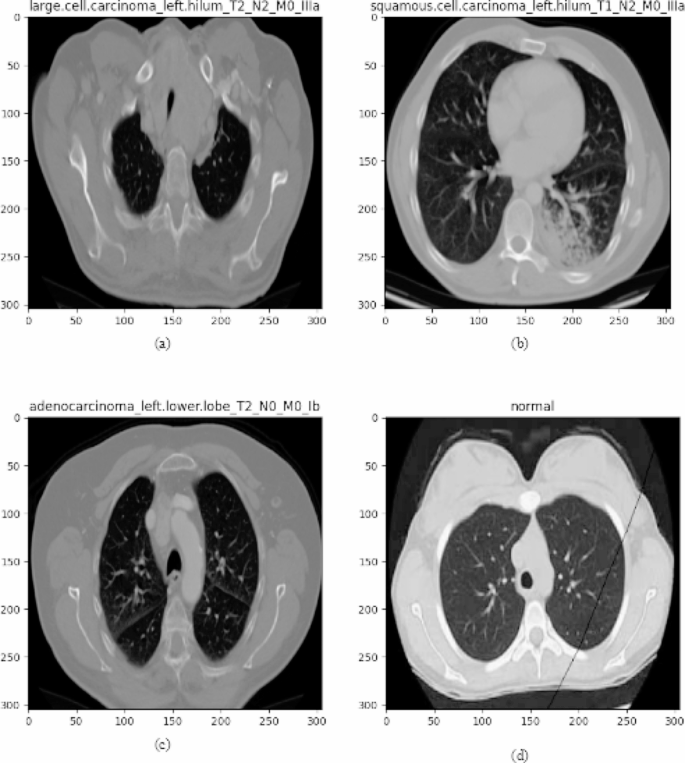
Sample images from chest CT imaging dataset (a) large cell, (b) squamous cell, (c) adenocarcinoma, and (d) normal
Adenocarcinoma
Lung adenocarcinoma Footnote 4 is the most common form of lung cancer, accounting for 30% of all cases and about 40% of all non-small cell lung cancer occurrences. Adenocarcinomas are found in several common cancers, including breast, prostate and colorectal. Adenocarcinomas of the lung are found in the outer region of the lung in glands that secrete mucus and help us breathe. Symptoms include coughing, hoarseness, weight loss and weakness.
Large cell carcinoma
Large-cell undifferentiated carcinoma Footnote 5 lung cancer grows and spreads quickly and can be found anywhere in the lung. This type of lung cancer usually accounts for 10 to 15% of all cases. Large-cell undifferentiated carcinoma tends to grow and spread quickly.
Squamous cell carcinoma
Squamous cell carcinoma Footnote 6 is found centrally in the lung, where the larger bronchi join the trachea to the lung or in one of the main airway branches. Squamous cell lung cancer is responsible for about 30% of all non-small cell lung cancers and is generally linked to smoking.
The last category is the normal CT scan images.
Data preprocessing
To develop a robust and reliable automated system, data preprocessing plays a crucial role in the model-building process [ 35 , 36 , 37 ]. Preprocessing is an essential step to eliminate the distortions from the images. In this study, data preprocessing, image resizing, and data augmentation were used for better classification and detection of lung cancer, as discussed in the subsections below.
Image resizing
The loaded images are standardized and normalized using a standard scaler and min-max scaler as the normalization functions. The files are resized from 224 × 224 to 460 × 460 using a resize function. The classes undergo label encoding, i.e., 0 for class Adenocarcinoma, 1 for class Large cell carcinoma, 2 for class Normal and 3 for class Squamous cell carcinoma.
Data augmentation
Random oversampling was applied afterwards to add randomly duplicate examples in the minority class by adding additional images to the classes containing fewer samples in the dataset. Initially, the dataset comprised 1000 images, with each class containing 338, 187, 260 and 215 images. The final dataset after oversampling contains 1653 images, with each class containing 411, 402, 374 and 466 images, as shown in Table 2 .
After that, data augmentation was applied by applying shear_range = 0.2, zoom_range = 0.2, rotation_range = 24, horizontal_flip = True, and vertical_flip = True. Finally, the dataset is split into training, testing and validation in 64.48%, 26.98% and 8.52%, respectively. After the preprocessing followed by the Train-test split, the data is fed to models for training.
Transfer learning models
Transfer learning models play a significant role in healthcare for medical image processing [ 23 , 31 ]. Medical imaging technologies, such as X-rays, CT scans, MRI scans, and histopathology slides, generate vast amounts of visual data that require accurate and efficient analysis. Transfer learning enables the utilization of pre-trained models trained on large datasets from various domains, such as natural images, to tackle medical image processing tasks [ 28 ]. The transfer learning models that are considered in this experiment are described in this section.
NasNetLarge
Google created the NasNetLarge [ 38 ], a neural architecture search network designed for powerful computational resources. This model addresses the issue of crafting an ideal CNN architecture by formulating it as a reinforcement learning challenge. NasNetLarge introduces an approach where a machine assists in designing neural network architecture and constructing a deep neural network without relying on traditional underlying models that concentrate on tensor decomposition or quantization techniques. Notably, NasNetLarge demonstrated exceptional performance in the ImageNet competition, showcasing its state-of-the-art capabilities. The model is tailored to a specific image input size of 331 × 331, which remains fixed and unmodifiable.
The unique advantages of NasNetLarge are:
Efficient architecture design using neural architecture search.
Achieves state-of-the-art performance on various image classification tasks.
Good balance between accuracy and computational efficiency.
The Xception architecture is a popular and strong convolutional neural network through various significant principles, including the convolutional layer, depth-wise separable convolution layer, residual connections, and the inception module [ 39 ]. Additionally, the activation function in the CNN architecture plays a crucial role, where the Swish activation function has been introduced to enhance the conventional activation function. The foundation of Xception is rooted in the Inception module, which effectively separates cross-channel correlations and spatial relationships within CNN feature maps, resulting in a fully independent arrangement.
The unique advantages of Xception are:
Deep and efficient convolutional neural network architecture.
Achieves high accuracy on image classification tasks.
Separable convolutions reduce the number of parameters and operations.
DenseNet201
DenseNet201 [ 40 ] is a CNN with 201 layers. It is based on the DenseNet concept of densely connecting every layer to every other layer in a feedforward manner, which helps improve the flow of information and gradient propagation through the network. It is a part of the DenseNet family of models, designed to address the problem of vanishing gradients in very deep neural networks. The output of densely connected and transition layers can be calculated using Eq. 1 and Eq. 2 .
where H i is the output of the current layer, f is the activation function, and [ H 0 , H 1 , H 2 , …, H i−1 ] are the outputs of all previous layers concatenated together. Also, W i+1 is the set of weights for the convolutional layer, BN is the batch normalization operation, f is the activation function, and W i+1 is the output of the transition layer.
The unique advantages of DenseNet201 are:
Dense connectivity pattern between layers, allowing for feature reuse.
Reduces the vanishing gradient problem and encourages feature propagation.
Achieves high accuracy while using fewer parameters compared to other models.
MobileNet [ 38 ] is a popular deep neural network architecture designed for mobile and embedded devices with limited computational resources. The architecture is based on a lightweight building block called a MobileNet unit, which consists of a depth-wise separable convolution layer followed by a pointwise convolution layer. The depth-wise separable convolution is a factorized convolution that decomposes a standard convolution into a depth-wise convolution and a pointwise convolution, which reduces the number of parameters and computations. The output of a MobileNet unit and inverted residual block can be calculated using Eq. 3 to Eq. 7 .
where X is the input tensor, DW is the depth-wise convolution operation, Conv 1 × 1 is the pointwise convolution operation, σ is the activation function, BN is the batch normalization operation, and Y is the output tensor. Also, X in is the input tensor, X is the output tensor of the bottleneck layer, Conv 1 × 1 and DW are the pointwise and depthwise convolution operations.
The unique advantages of MobileNet are:
Specifically designed for mobile and embedded vision applications.
Lightweight architecture with depth-wise separable convolutions.
Achieves a good balance of accuracy and model size, making it ideal for resource-constrained environments.
Residual Neural Networks (ResNets) are a type of deep learning model that has become increasingly popular in recent years, particularly for computer vision applications. The ResNet101 [ 41 ] model allows us to train extremely deep neural networks with 101 layers successfully. It addresses the vanishing gradient problem by using skip connections, which allow the output of one layer to be added to the previous layer’s output. This creates a shortcut that bypasses the intermediate layers, which helps to preserve the gradient and makes it easier to train very deep networks. This model architecture results in a more efficient network for training and provides good performance in terms of accuracy. Mathematically, the residual block can be expressed as given by Eq. 8
where x is the input to the block, F is a set of convolutional layers with weights W i , and y is the block output. The skip connection adds the input x to the output y to produce the final output of the block.
The unique advantages of ResNet101 are:
Residual connections that mitigate the vanishing gradient problem.
Permits deeper network architecture without compromising performance.
It is easy to train and achieves excellent accuracy.
- EfficientNetB0
EfficientNetB0 [ 42 ] is a CNN architecture belonging to the EfficientNet model family. These models are specifically crafted to achieve top-tier performance while maintaining computational efficiency, rendering them suitable for various computer vision tasks. The central concept behind EfficientNet revolves around harmonizing model depth, width, and resolution to attain optimal performance. This is achieved through a compound scaling technique that uniformly adjusts these three dimensions to generate a range of models, with EfficientNetB0 as the baseline. The network comprises 16 blocks, each characterized by its width, determined by the number of channels (filters) in every convolutional layer. The number of channels is adjusted using a scaling coefficient. Additionally, the input image resolution for EfficientNetB0 typically remains fixed at 224 × 224 pixels.
The unique advantages of EfficientNetB0 are:
Achieve state-of-the-art accuracy on image classification tasks.
Use a compound scaling method to balance model depth, width, and resolution.
A more accurate and computationally efficient architecture design.
EfficientNetB4
The EfficientB4 [ 43 ] neural network, consisting of blocks and segments, has residual units and parallel GPU utilization points. It is a part of the EfficientNet family of models, designed to be more computationally efficient than previous models while achieving state-of-the-art accuracy on various computer vision tasks, including image classification and object detection. The CNN backbone in EfficientNetB4 consists of a series of convolutional blocks, each with a set of operations, including convolution, batch normalization, and activation. The output of each block is fed into the next block as input. The final convolutional block is followed by a set of fully connected layers responsible for classifying the input image. The output of a convolutional block can be calculated using Eq. 9 .
where x i−1 is the input to the current block, W i is the set of weights for the convolutional layer, BN is the batch normalization operation, f is the activation function, and y i is the block output.
Being in the same family, EfficientB4 shares the advantages of EfficientNetB0.
Visual Geometry Group (VGG) is a traditional CNN architecture. The VGG19 [ 44 ] model consists of 19 layers with 16 convolutional layers and three fully connected layers. The max-pooling layers are applied after every two or three convolutional layers. It has achieved high accuracy on various computer vision tasks, including image classification, object detection, and semantic segmentation. One of the main contributions of the VGG19 network is the use of very small convolution filters (3 × 3) in each layer, which allows for deeper architectures to be built with fewer parameters. The output of the convolutional layers can be calculated using Eq. 10 .
where x is the input image, W is the weight matrix of the convolutional layer, b is the bias term, and f is the activation function, which is usually a rectified linear unit (ReLU) in VGG19. The output y is a feature map that captures the important information from the input image.
The unique advantages of VGG19 are:
Simple and straightforward architecture.
Achieves good performance on various computer vision tasks.
Its simplicity and ease of use make it a favourite among educators.
Proposed VER-Net model
To find out the best-performing models among the ones discussed in the previous section, we ran them and assessed their performance individually. Among them, VGG19 and EfficientNetB0 were the best performers in all metrics. However, EfficientNetB4 and ResNet101 competed with each other to take the third spot. In some metrics, EfficientNetB4 did better, while in some, ResNet101 was better. Nevertheless, we picked ResNet101 over EfficientNetB4 because it has better testing accuracy and precision, which is crucial for detecting life-threatening diseases like cancer. Therefore, we stacked VGG19, EfficientNetB0, and ResNet101 in our proposed VER-Net model. The complete algorithm for this procedure is shown in Algorithm 1.
Model Architecture
The architecture of the proposed VER-Net model is shown in Fig. 5 . The input shape is 460 × 460 × 3, which is mapped to four classes as output. We used three different dense layers for three stacked transfer learning models in the model. Thereafter, the same convolution layers of 7 × 7 × 1024 for all three and three different max-pooling layers are used. The outputs are flattened before sending to three 3 fully connected layers (1024 × 512 × 256). The three outputs of these connected layers are then concatenated using majority voting, and accordingly, the classified outputs are generated. The architectural description of VER-Net is shown in Table 3 .
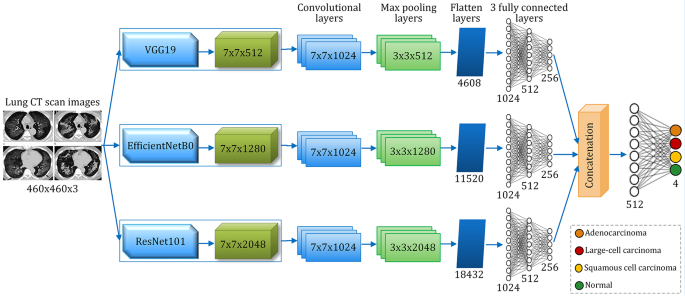
VER-Net’s architecture
Model parameters
The details of hyperparameters settings for VER-Net are listed in Table 4 . In Table 5 , the details of data augmentation are listed. Here, we used the RandomFlip and RandomRotation functions available in TensorFlow.Keras for data augmentation.
Experiment, results and performance analysis
In this section, the experimental details, including system setup and evaluation metrics, are covered. Also, the results are elaborately presented, and the performance of the proposed model is extensively assessed.
Experimental system setup
The experiment was conducted on a Dell workstation with a Microsoft Windows environment. Python was used to program on the Anaconda framework. The details of the system are given in Table 6 .
Evaluation Metrics
Evaluation metrics are used to assess the performance of a model on a problem statement. Different evaluation metrics are used depending on the problem type and the data’s nature. In this study, the experimental findings for the presented models are evaluated using various performance metrics, summarised in Table 7 .
VER-Net model implementation
After background and designing the VER-Net model, we implemented it. The results are discussed in the following.
Confusion matrix
The classification performance of VER-Net is evaluated using a confusion matrix, as shown in Fig. 6 . Since there are four output classes, the confusion matrix is a 4 × 4 matrix. Every column in the matrix represents a predicted class, whereas every row represents an actual class. The principal diagonal cells denote the respective classes’ correct predictions (TP). Besides the TP cell, all other cells in the same row denote TN. For example, in the first row, except the first column, five of the Adenocarcinoma were falsely classified as large cell carcinoma, and four were categorized as Squamous cell carcinoma. So, 9 (5 + 0 + 4) are TN classifications for the Adenocarcinoma class. Similarly, all other cells in the same column denote FP besides the TP cell. For example, in the first column, except the first row, four Large cell carcinoma, four normal cells, and 21 Squamous cell carcinoma are falsely classified as Adenocarcinoma. So, 29 (4 + 4 + 21) FN classifications exist for the Adenocarcinoma class. The rest of the cells denote FN predictions.
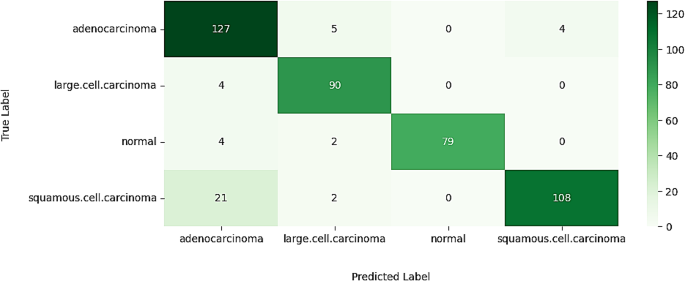
Confusion matrix of VER-Net
Accuracy and loss of VER-Net
The accuracy and loss of our VER-Net model are plotted in Figs. 7 and 8 , respectively. The x-axis denotes the number of epochs (100), while the y-axis reflects accuracy in Fig. 7 and loss in Fig. 8 . The training curve suggests how well VER-Net is trained. It can be observed that both accuracy and loss for validation/testing converge approximately after 20 epochs. It is further noticed that the model did not exhibit significant underfitting and overfitting upon hyperparameter tuning. In our experiment, we tried with different epoch numbers (40, 60, 100, and 200). We got the best results with 100 epochs.
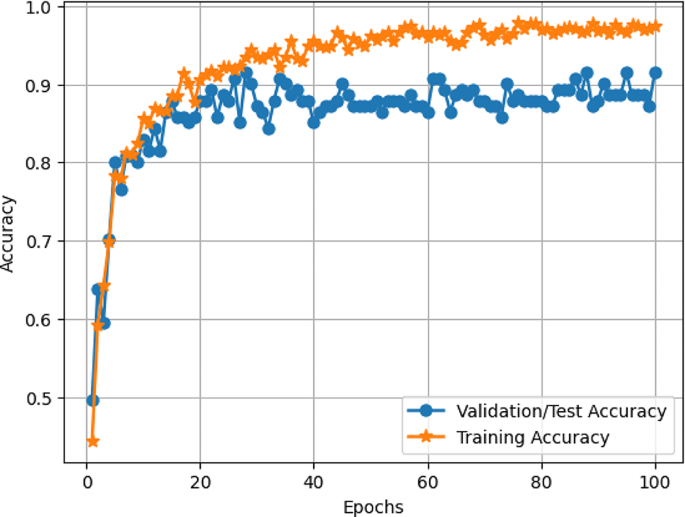
Training and validation/test accuracy VER-Net model
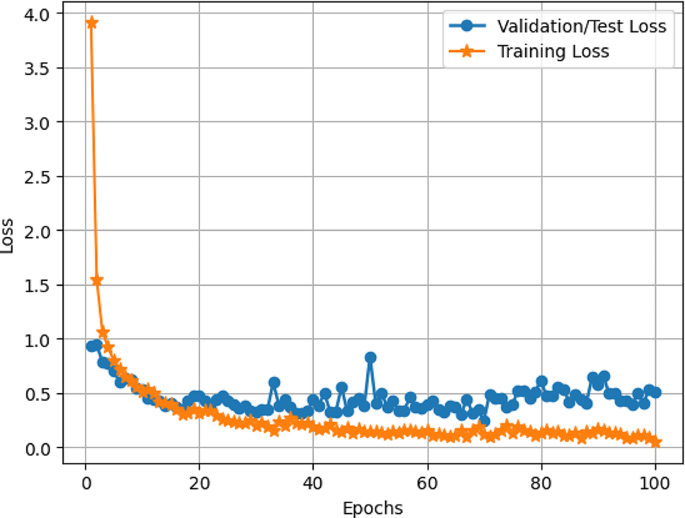
Training and validation/test loss VER-Net model
Performance analysis of VER-Net
In this section, we exhaustively analyze the performance of VER-Net model. For this, we adopted a comparative analysis approach. We compared VER-Net with other transfer learning models and the results of similar research works.
Comparing VER-Net with other transfer learning models
First, we compare the performance of VER-Net with the individual transfer learning models, mentioned in Sect. 3.4. All the models were trained and tested on the same dataset and validated with the same parameters.
Figures 9 and 10 present the accuracy and loss comparisons. VER-Net and VGG19 both achieved the highest accuracy of 97.47% for training, but for testing, VER-Net emerged as the sole highest accuracy achiever with 91%. NASNetLarge got the lowest accuracy on both occasions, with 69.51% and 64% training and testing accuracy, respectively. Similar to accuracy, VER-Net and VGG19 both managed the lowest loss of 0.07% for training, and VER-Net was the sole lowest loss achiever with 0.34%. Here also, NASNetLarge performed worst on both occasions with 0.66% and 0.80% training and testing loss, respectively.
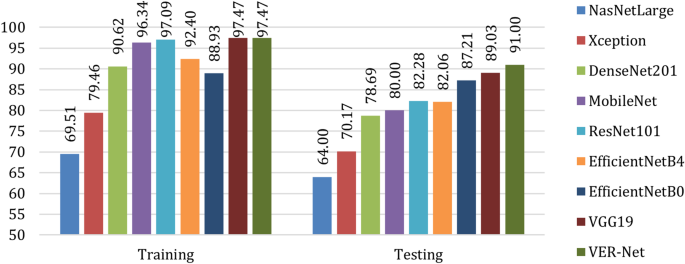
Accuracy comparison of the proposed ensemble method (VER-Net) with other transfer learning models
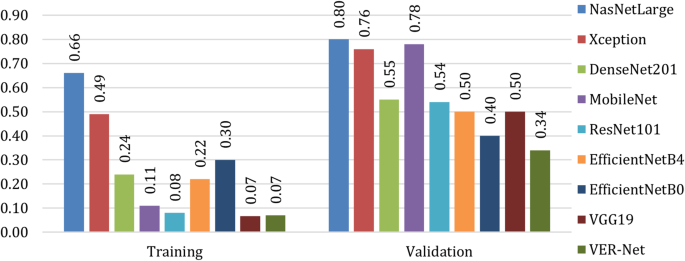
Loss comparison of the proposed ensemble method (VER-Net) with other transfer learning models
Table 8 notes all classes’ precision, recall and F1-score values to compare VER-Net with other models. The macro average of these metrics for all four classes is shown in Fig. 11 . For all three instances, i.e., precision, recall and F1-score, VER-Net outperformed with 0.920, 0.910, and 0.913, respectively. VGG19 and EficientNetB0 emerged as the second and third-best performers, whereas NASNetLarge was the worst performer with 0.693, 0.645, and 0.645 for precision, recall and F1-score, respectively.
In Fig. 12 , VER-Net is compared with others in terms of weighted average for precision, recall and F1-score. Here, we used a uniform weight of 1.5 for all classes. Like the macro average, VER-Net was the top performer for all three metrics, followed by VGG19 and EficientNetB0, and NasNetLarge was the worst performer. As shown in Table 8 , NasNetLarge classifies the non-cancerous cells with 100% accuracy; in fact, it performs the best among all models but performs very poorly for the cancerous cells.
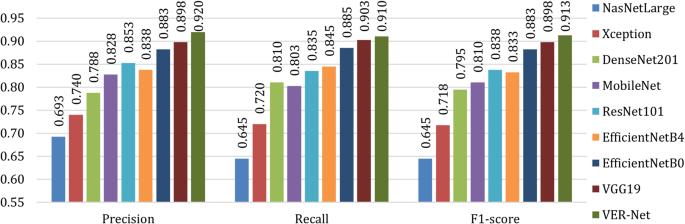
Macro average comparison of VER-Net and other models
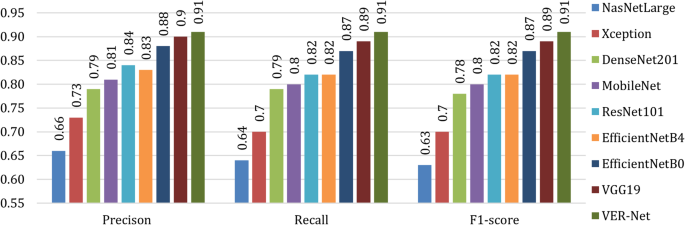
Weighted average comparison of VER-Net and other models
To assess the performance variations of VER-Net, we calculated the standard deviation to calculate the mean-variance across the classes for precision, recall and F1-score. A lower value suggests that the model is effective for all classes equally. In contrast, a higher variation suggests bias to a certain class. From Fig. 13 , it can be observed that VER-Net has the lowest variations for recall and F1-score of 0.062 and 0.04, respectively. However, as an exception in the case of precision, VER-Net is bettered by DenseNet201 with a margin of 0.042 variations. This can be reasoned as VER-Net attained 100% precision for the Normal class. Nevertheless, VER-Net has significantly lower variance across three metrics than DenseNet201.
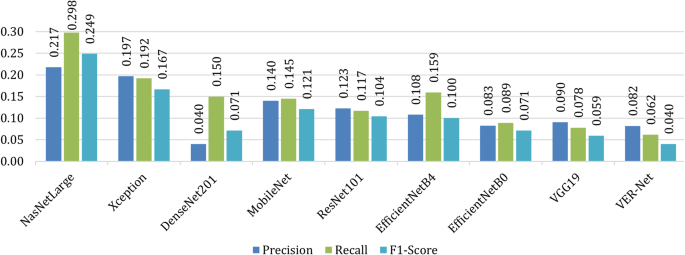
Standard deviation for precision, recall and F1-score of all classes
Comparing VER-Net with literature
In the previous section, we established the superiority of VER-Net over other established transfer learning models. To prove the ascendency of VER-Net further, we compared it with the results of some similar recent experiments, available in the literature pertaining to detecting lung cancer based on CT scan images using transfer learning methods. A comparative summary is given in Table 9 .
The above experiments and results clearly show that the proposed VER-NET performed well in detecting lung cancer in most of the performance testing. It is the overall best performer among the nine transfer learning models. One of the reasons for this is that we incorporated the best three models (considered in this experiment) into the VER-NET. Besides, we optimally designed the VER-NET architecture for its best performance. Furthermore, to make the model more generalized, we generated additional synthetic lung cancer images in addition to the original image dataset.
To balance the dataset, we performed image augmentation, which might make slight changes in the real images. So, the performance of VER-Net might vary little on a balanced real dataset where there is no need for synthetic augmentation. The images were generated with 64 × 64 pixels, which is insufficient for the analysis of medical images. For cancer cell detection based on cell images, high-resolution images are crucial.
Since VER-Net is an ensembled model comprising three transfer learning, it is obvious that it should increase the computational complexity, requiring longer for training. However, this should not be a discouraging factor in a lifesaving application like cancer detection, where accuracy and precision matter most.
Conclusions and future scope
Incorporating transfer learning into lung cancer detection models has shown improved performance and robustness in various studies. In this paper, we concatenated three transfer learning models, namely, VGG19 + EfficientNetB0 + ResNet101, to build an ensembled VER-Net model to detect lung cancer. We used CT scan images as input to the model. To make VER-Net effective, we conducted data preprocessing and data augmentation. We compared the performance of VER-Net with eight other transfer learning models. The comparative results were assessed through various performance evaluation metrics. It was observed that VER-Net performed best in all metrics. VER-Net also exhibited better accuracy than similar empirical studies from the recent literature.
Here, we incorporated the three top-performing transfer models in the hybrid VER-Net architecture. Further experimentation can be done on this ensembling approach. For example, other models can be tried in different combinations. Also, transfer learning models of different families can be tried.
We plan to extend the use of the VER-Net model for identifying lung cancer where only chest X-ray images are available. Furthermore, this model can also be applied to assess the severity of lung cancer if the patient is already infested. Considering the success of VER-Net in detecting lung cancer, it can be used for other diseases where CT scan images are useful to identify the disease.
Data availability
No datasets were generated or analysed during the current study.
https://www.who.int/news-room/fact-sheets/detail/cancer .
https://www.iarc.who.int/ .
https://www.kaggle.com/datasets/mohamedhanyyy/chest-ctscan-images .
https://www.cancercenter.com/cancer-types/lung-cancer/types/adenocarcinoma-of-the-lung .
https://www.verywellhealth.com/large-cell-carcinoma-of-the-lungs-2249356 .
https://www.mayoclinic.org/diseases-conditions/squamous-cell-carcinoma/ .
Siegel RL, Miller KD, Fuchs HE, Jemal A. Cancer Stat 2021 CA Cancer J Clin. Jan. 2021;71(1):7–33. https://doi.org/10.3322/caac.21654 .
Dela Cruz CS, Tanoue LT, Matthay RA. Lung Cancer: Epidemiology, etiology, and Prevention. Clin Chest Med. Dec. 2011;32:605–44. https://doi.org/10.1016/j.ccm.2011.09.001 . no. 4.
Wankhade S. A novel hybrid deep learning method for early detection of lung cancer using neural networks. Healthc Analytics. 2023;3:100195. https://doi.org/10.1016/j.health.2023.100195 .
Article Google Scholar
Ruano-Raviña A et al. Lung cancer symptoms at diagnosis: results of a nationwide registry study, ESMO Open , vol. 5, no. 6, p. e001021, 2020, https://doi.org/10.1136/esmoopen-2020-001021 .
Zappa C, Mousa SA. Non-small cell lung cancer: current treatment and future advances. Transl Lung Cancer Res. Jun. 2016;5(3):288–300. https://doi.org/10.21037/tlcr.2016.06.07 .
Otty Z, Brown A, Sabesan S, Evans R, Larkins S. Optimal care pathways for people with lung cancer-a scoping review of the literature. Int J Integr Care. 2020;20(3):1–9. https://doi.org/10.5334/ijic.5438 .
Xiang D, Zhang B, Doll D, Shen K, Kloecker G, Freter C. Lung cancer screening: from imaging to biomarker. Biomark Res. 2013;1(1). https://doi.org/10.1186/2050-7771-1-4 .
Woznitza N, Piper K, Rowe S, Bhowmik A. Immediate reporting of chest X-rays referred from general practice by reporting radiographers: a single centre feasibility study, Clin Radiol , vol. 73, no. 5, pp. 507.e1-507.e8, 2018, https://doi.org/10.1016/j.crad.2017.11.016 .
McAuliffe MJ, Lalonde FM, McGarry D, Gandler W, Csaky K, Trus BL. Medical Image Processing, Analysis and Visualization in clinical research, in Proceedings 14th IEEE Symposium on Computer-Based Medical Systems. CBMS 2001, 2001, pp. 381–386. https://doi.org/10.1109/CBMS.2001.941749 .
Puttagunta M, Ravi S. Medical image analysis based on deep learning approach. Multimed Tools Appl. 2021;80(16):24365–98. https://doi.org/10.1007/s11042-021-10707-4 .
Article PubMed PubMed Central Google Scholar
Shen D, Wu G, Il Suk H. Deep learning in Medical Image Analysis. Annu Rev Biomed Eng. Jun. 2017;19:221–48. https://doi.org/10.1146/annurev-bioeng-071516-044442 .
Tsuneki M. Deep learning models in medical image analysis. J Oral Biosci. 2022;64(3):312–20. https://doi.org/10.1016/j.job.2022.03.003 .
Article PubMed Google Scholar
Dara S, Tumma P, Eluri NR, Rao Kancharla G. Feature Extraction In Medical Images by Using Deep Learning Approach. [Online]. Available: http://www.acadpubl.eu/hub/ .
Kuwil FH. A new feature extraction approach of medical image based on data distribution skew. Neurosci Inf. 2022;2(3):100097. https://doi.org/10.1016/j.neuri.2022.100097 .
Bar Y, Diamant I, Wolf L, Lieberman S, Konen E, Greenspan H. Chest pathology identification using deep feature selection with non-medical training. Comput Methods Biomech Biomed Eng Imaging Vis. May 2018;6(3):259–63. https://doi.org/10.1080/21681163.2016.1138324 .
Pandiyarajan M, Thimmiaraja J, Ramasamy J, Tiwari M, Shinde S, Chakravarthi MK. Medical Image Classification for Disease Prediction with the Aid of Deep Learning Approaches, in 2022 2nd International Conference on Advance Computing and Innovative Technologies in Engineering (ICACITE) , 2022, pp. 724–727. https://doi.org/10.1109/ICACITE53722.2022.9823417 .
Hemachandran K et al. Feb., Performance Analysis of Deep Learning Algorithms in Diagnosis of Malaria Disease, Diagnostics , vol. 13, no. 3, 2023, https://doi.org/10.3390/diagnostics13030534 .
Kumar Mallick P, Ryu SH, Satapathy SK, Mishra S, Nguyen GN, Tiwari P. Brain MRI image classification for Cancer Detection using deep Wavelet Autoencoder-based deep neural network. IEEE Access. 2019;7:46278–87. https://doi.org/10.1109/ACCESS.2019.2902252 .
Yu X, Wang J, Hong Q-Q, Teku R, Wang S-H, Zhang Y-D. Transfer learning for medical images analyses: a survey. Neurocomputing. 2022;489:230–54. https://doi.org/10.1016/j.neucom.2021.08.159 .
Li X, et al. Transfer learning in computer vision tasks: remember where you come from. Image Vis Comput. 2020;93:103853. https://doi.org/10.1016/j.imavis.2019.103853 .
Alyafeai Z, AlShaibani MS, Ahmad I. A Survey on Transfer Learning in Natural Language Processing, May 2020, [Online]. Available: http://arxiv.org/abs/2007.04239 .
Wang D, Zheng TF. Transfer learning for speech and language processing, in 2015 Asia-Pacific Signal and Information Processing Association Annual Summit and Conference (APSIPA) , 2015, pp. 1225–1237. https://doi.org/10.1109/APSIPA.2015.7415532 .
Kim HE, Cosa-Linan A, Santhanam N, Jannesari M, Maros ME, Ganslandt T. Transfer learning for medical image classification: a literature review. BMC Med Imaging. 2022;22(1):69. https://doi.org/10.1186/s12880-022-00793-7 .
Sarker IH. Deep Learning: A Comprehensive Overview on Techniques, Taxonomy, Applications and Research Directions, SN Computer Science , vol. 2, no. 6. Springer, Nov. 01, 2021. https://doi.org/10.1007/s42979-021-00815-1 .
Egger J, et al. Medical deep learning—A systematic meta-review. Comput Methods Programs Biomed. 2022;221:106874. https://doi.org/10.1016/j.cmpb.2022.106874 .
Huang J, Chai J, Cho S. Deep learning in finance and banking: A literature review and classification, Frontiers of Business Research in China , vol. 14, no. 1. Springer, Dec. 01, 2020. https://doi.org/10.1186/s11782-020-00082-6 .
Haleem A, Javaid M, Asim Qadri M, Pratap R, Singh, Suman R. Artificial intelligence (AI) applications for marketing: a literature-based study. Int J Intell Networks. 2022;3:119–32. https://doi.org/10.1016/j.ijin.2022.08.005 .
Wang S, Dong L, Wang X, Wang X. Classification of pathological types of lung cancer from CT images by deep residual neural networks with transfer learning strategy, Open Medicine (Poland) , vol. 15, no. 1, pp. 190–197, Jan. 2020, https://doi.org/10.1515/med-2020-0028 .
Han Y, et al. Histologic subtype classification of non-small cell lung cancer using PET/CT images. Eur J Nucl Med Mol Imaging. 2021;48(2):350–60. https://doi.org/10.1007/s00259-020-04771-5 .
Vijayan N, Kuruvilla J. The impact of transfer learning on lung cancer detection using various deep neural network architectures, in 2022 IEEE 19th India Council International Conference (INDICON) , 2022, pp. 1–5. https://doi.org/10.1109/INDICON56171.2022.10040188 .
da Nóbrega RVM, Peixoto SA, da Silva SPP, Filho PPR. Lung Nodule Classification via Deep Transfer Learning in CT Lung Images, in 2018 IEEE 31st International Symposium on Computer-Based Medical Systems (CBMS) , 2018, pp. 244–249. https://doi.org/10.1109/CBMS.2018.00050 .
Dadgar S, Neshat M. Comparative Hybrid Deep Convolutional Learning Framework with Transfer Learning for Diagnosis of Lung Cancer, in Proceedings of the 14th International Conference on Soft Computing and Pattern Recognition (SoCPaR 2022) , A. Abraham, T. Hanne, N. Gandhi, P. Manghirmalani Mishra, A. Bajaj, and P. Siarry, Eds., Cham: Springer Nature Switzerland, 2023, pp. 296–305.
Sori WJ, Feng J, Godana AW, Liu S, Gelmecha DJ. DFD-Net: lung cancer detection from denoised CT scan image using deep learning. Front Comput Sci. 2020;15(2):152701. https://doi.org/10.1007/s11704-020-9050-z .
Sari S, Soesanti I, Setiawan NA. Best Performance Comparative Analysis of Architecture Deep Learning on CT Images for Lung Nodules Classification, in 2021 IEEE 5th International Conference on Information Technology, Information Systems and Electrical Engineering (ICITISEE) , 2021, pp. 138–143. https://doi.org/10.1109/ICITISEE53823.2021.9655872 .
Gonzalez Zelaya CV. Towards Explaining the Effects of Data Preprocessing on Machine Learning, in 2019 IEEE 35th International Conference on Data Engineering (ICDE) , 2019, pp. 2086–2090. https://doi.org/10.1109/ICDE.2019.00245 .
Hassler AP, Menasalvas E, García-García FJ, Rodríguez-Mañas L, Holzinger A. Importance of medical data preprocessing in predictive modeling and risk factor discovery for the frailty syndrome. BMC Med Inf Decis Mak. 2019;19(1):33. https://doi.org/10.1186/s12911-019-0747-6 .
Komorowski M, Marshall DC, Salciccioli JD, Crutain Y. Exploratory Data Analysis. In: Data MITC, editor. Secondary Analysis of Electronic Health Records. Cham: Springer International Publishing; 2016. pp. 185–203. https://doi.org/10.1007/978-3-319-43742-2_15 .
Chapter Google Scholar
Meem RF, Hasan KT. Osteosarcoma Tumor Detection using Transfer Learning Models, May 2023, [Online]. Available: http://arxiv.org/abs/2305.09660 .
Kusniadi I, Setyanto A. Fake Video Detection using Modified XceptionNet, in 2021 4th International Conference on Information and Communications Technology (ICOIACT) , 2021, pp. 104–107. https://doi.org/10.1109/ICOIACT53268.2021.9563923 .
Wang S-H, Zhang Y-D. DenseNet-201-Based Deep Neural Network with Composite Learning Factor and Precomputation for Multiple Sclerosis Classification, ACM Trans. Multimedia Comput. Commun. Appl , vol. 16, no. 2s, Jun. 2020, https://doi.org/10.1145/3341095 .
Zhang Q. A novel ResNet101 model based on dense dilated convolution for image classification. SN Appl Sci. Jan. 2022;4(1). https://doi.org/10.1007/s42452-021-04897-7 .
Abdulhussein WR, El NK, Abbadi, Gaber AM. Hybrid Deep Neural Network for Facial Expressions Recognition, Indonesian Journal of Electrical Engineering and Informatics , vol. 9, no. 4, pp. 993–1007, Dec. 2021, https://doi.org/10.52549/ijeei.v9i4.3425 .
Kurt Z, Işık Ş, Kaya Z, Anagün Y, Koca N, Çiçek S. Evaluation of EfficientNet models for COVID-19 detection using lung parenchyma. Neural Comput Appl. 2023;35(16):12121–32. https://doi.org/10.1007/s00521-023-08344-z .
Mateen M, Wen J, Nasrullah S, Song, Huang Z. Fundus image classification using VGG-19 architecture with PCA and SVD, Symmetry (Basel) , vol. 11, no. 1, Jan. 2019, https://doi.org/10.3390/sym11010001 .
Chon A, Balachandar N, Lu P. Deep Convolutional Neural Networks for Lung Cancer Detection.
Download references
ZZ is partially funded by his startup fund at The University of Texas Health Science Center at Houston, Houston, Texas, USA.
Author information
Authors and affiliations.
Department of Computing Science and Engineering, IFTM University, Moradabad, Uttar Pradesh, India
Anindita Saha
AI Research Centre, Department of Analytics, School of Business, Woxsen University, Hyderabad, Telangana, 502345, India
Shahid Mohammad Ganie
School of Computer Applications and Technology, Galgotias University, Greater Noida, Uttar Pradesh, 203201, India
Pijush Kanti Dutta Pramanik
Department of Computer Science & Engineering, MSOET, Maharishi University of Information Technology, Lucknow, Uttar Pradesh, India
Rakesh Kumar Yadav
Department of Environmental Health, Harvard T. H. Chan School of Public Health, Boston, MA, USA
Saurav Mallik
Center for Precision Health, McWilliams School of Biomedical Informatics, The University of Texas Health Science Center at Houston, Houston, TX, 77030, USA
Zhongming Zhao
You can also search for this author in PubMed Google Scholar
Contributions
AS: Conceptualization, Data curation, Methodology; SMG: Formal analysis, Methodology, Validation, Visualization, Prepared figures, Writing - original draft, Writing - review & editing; PKDP: Investigation, Formal analysis, Validation, Prepared figures, Writing - original draft, Writing - review & editing; RKY: Supervision, Writing - review & editing; SM: Validation, Writing - review & editing; ZZ: Supervision, Funding, Writing - review & editing.
Corresponding authors
Correspondence to Pijush Kanti Dutta Pramanik or Zhongming Zhao .
Ethics declarations
Ethics approval and consent to participate.
Not applicable.
Consent for publication
Competing interests.
The authors declare no competing interests.
Additional information
Publisher’s note.
Springer Nature remains neutral with regard to jurisdictional claims in published maps and institutional affiliations.
Rights and permissions
Open Access This article is licensed under a Creative Commons Attribution 4.0 International License, which permits use, sharing, adaptation, distribution and reproduction in any medium or format, as long as you give appropriate credit to the original author(s) and the source, provide a link to the Creative Commons licence, and indicate if changes were made. The images or other third party material in this article are included in the article’s Creative Commons licence, unless indicated otherwise in a credit line to the material. If material is not included in the article’s Creative Commons licence and your intended use is not permitted by statutory regulation or exceeds the permitted use, you will need to obtain permission directly from the copyright holder. To view a copy of this licence, visit http://creativecommons.org/licenses/by/4.0/ . The Creative Commons Public Domain Dedication waiver ( http://creativecommons.org/publicdomain/zero/1.0/ ) applies to the data made available in this article, unless otherwise stated in a credit line to the data.
Reprints and permissions
About this article
Cite this article.
Saha, A., Ganie, S.M., Pramanik, P.K.D. et al. VER-Net: a hybrid transfer learning model for lung cancer detection using CT scan images. BMC Med Imaging 24 , 120 (2024). https://doi.org/10.1186/s12880-024-01238-z
Download citation
Received : 25 November 2023
Accepted : 05 March 2024
Published : 24 May 2024
DOI : https://doi.org/10.1186/s12880-024-01238-z
Share this article
Anyone you share the following link with will be able to read this content:
Sorry, a shareable link is not currently available for this article.
Provided by the Springer Nature SharedIt content-sharing initiative
- Lung cancer detection
- Transfer learning
- Image processing
BMC Medical Imaging
ISSN: 1471-2342
- General enquiries: [email protected]
Case Study Research Method in Psychology
Saul Mcleod, PhD
Editor-in-Chief for Simply Psychology
BSc (Hons) Psychology, MRes, PhD, University of Manchester
Saul Mcleod, PhD., is a qualified psychology teacher with over 18 years of experience in further and higher education. He has been published in peer-reviewed journals, including the Journal of Clinical Psychology.
Learn about our Editorial Process
Olivia Guy-Evans, MSc
Associate Editor for Simply Psychology
BSc (Hons) Psychology, MSc Psychology of Education
Olivia Guy-Evans is a writer and associate editor for Simply Psychology. She has previously worked in healthcare and educational sectors.
On This Page:
Case studies are in-depth investigations of a person, group, event, or community. Typically, data is gathered from various sources using several methods (e.g., observations & interviews).
The case study research method originated in clinical medicine (the case history, i.e., the patient’s personal history). In psychology, case studies are often confined to the study of a particular individual.
The information is mainly biographical and relates to events in the individual’s past (i.e., retrospective), as well as to significant events that are currently occurring in his or her everyday life.
The case study is not a research method, but researchers select methods of data collection and analysis that will generate material suitable for case studies.
Freud (1909a, 1909b) conducted very detailed investigations into the private lives of his patients in an attempt to both understand and help them overcome their illnesses.
This makes it clear that the case study is a method that should only be used by a psychologist, therapist, or psychiatrist, i.e., someone with a professional qualification.
There is an ethical issue of competence. Only someone qualified to diagnose and treat a person can conduct a formal case study relating to atypical (i.e., abnormal) behavior or atypical development.
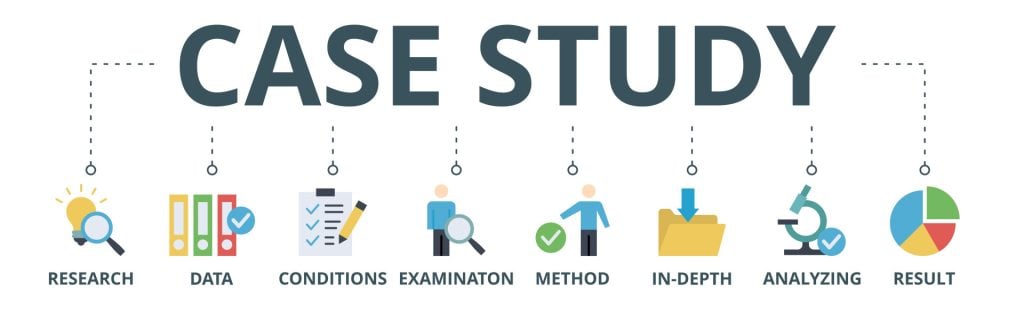
Famous Case Studies
- Anna O – One of the most famous case studies, documenting psychoanalyst Josef Breuer’s treatment of “Anna O” (real name Bertha Pappenheim) for hysteria in the late 1800s using early psychoanalytic theory.
- Little Hans – A child psychoanalysis case study published by Sigmund Freud in 1909 analyzing his five-year-old patient Herbert Graf’s house phobia as related to the Oedipus complex.
- Bruce/Brenda – Gender identity case of the boy (Bruce) whose botched circumcision led psychologist John Money to advise gender reassignment and raise him as a girl (Brenda) in the 1960s.
- Genie Wiley – Linguistics/psychological development case of the victim of extreme isolation abuse who was studied in 1970s California for effects of early language deprivation on acquiring speech later in life.
- Phineas Gage – One of the most famous neuropsychology case studies analyzes personality changes in railroad worker Phineas Gage after an 1848 brain injury involving a tamping iron piercing his skull.
Clinical Case Studies
- Studying the effectiveness of psychotherapy approaches with an individual patient
- Assessing and treating mental illnesses like depression, anxiety disorders, PTSD
- Neuropsychological cases investigating brain injuries or disorders
Child Psychology Case Studies
- Studying psychological development from birth through adolescence
- Cases of learning disabilities, autism spectrum disorders, ADHD
- Effects of trauma, abuse, deprivation on development
Types of Case Studies
- Explanatory case studies : Used to explore causation in order to find underlying principles. Helpful for doing qualitative analysis to explain presumed causal links.
- Exploratory case studies : Used to explore situations where an intervention being evaluated has no clear set of outcomes. It helps define questions and hypotheses for future research.
- Descriptive case studies : Describe an intervention or phenomenon and the real-life context in which it occurred. It is helpful for illustrating certain topics within an evaluation.
- Multiple-case studies : Used to explore differences between cases and replicate findings across cases. Helpful for comparing and contrasting specific cases.
- Intrinsic : Used to gain a better understanding of a particular case. Helpful for capturing the complexity of a single case.
- Collective : Used to explore a general phenomenon using multiple case studies. Helpful for jointly studying a group of cases in order to inquire into the phenomenon.
Where Do You Find Data for a Case Study?
There are several places to find data for a case study. The key is to gather data from multiple sources to get a complete picture of the case and corroborate facts or findings through triangulation of evidence. Most of this information is likely qualitative (i.e., verbal description rather than measurement), but the psychologist might also collect numerical data.
1. Primary sources
- Interviews – Interviewing key people related to the case to get their perspectives and insights. The interview is an extremely effective procedure for obtaining information about an individual, and it may be used to collect comments from the person’s friends, parents, employer, workmates, and others who have a good knowledge of the person, as well as to obtain facts from the person him or herself.
- Observations – Observing behaviors, interactions, processes, etc., related to the case as they unfold in real-time.
- Documents & Records – Reviewing private documents, diaries, public records, correspondence, meeting minutes, etc., relevant to the case.
2. Secondary sources
- News/Media – News coverage of events related to the case study.
- Academic articles – Journal articles, dissertations etc. that discuss the case.
- Government reports – Official data and records related to the case context.
- Books/films – Books, documentaries or films discussing the case.
3. Archival records
Searching historical archives, museum collections and databases to find relevant documents, visual/audio records related to the case history and context.
Public archives like newspapers, organizational records, photographic collections could all include potentially relevant pieces of information to shed light on attitudes, cultural perspectives, common practices and historical contexts related to psychology.
4. Organizational records
Organizational records offer the advantage of often having large datasets collected over time that can reveal or confirm psychological insights.
Of course, privacy and ethical concerns regarding confidential data must be navigated carefully.
However, with proper protocols, organizational records can provide invaluable context and empirical depth to qualitative case studies exploring the intersection of psychology and organizations.
- Organizational/industrial psychology research : Organizational records like employee surveys, turnover/retention data, policies, incident reports etc. may provide insight into topics like job satisfaction, workplace culture and dynamics, leadership issues, employee behaviors etc.
- Clinical psychology : Therapists/hospitals may grant access to anonymized medical records to study aspects like assessments, diagnoses, treatment plans etc. This could shed light on clinical practices.
- School psychology : Studies could utilize anonymized student records like test scores, grades, disciplinary issues, and counseling referrals to study child development, learning barriers, effectiveness of support programs, and more.
How do I Write a Case Study in Psychology?
Follow specified case study guidelines provided by a journal or your psychology tutor. General components of clinical case studies include: background, symptoms, assessments, diagnosis, treatment, and outcomes. Interpreting the information means the researcher decides what to include or leave out. A good case study should always clarify which information is the factual description and which is an inference or the researcher’s opinion.
1. Introduction
- Provide background on the case context and why it is of interest, presenting background information like demographics, relevant history, and presenting problem.
- Compare briefly to similar published cases if applicable. Clearly state the focus/importance of the case.
2. Case Presentation
- Describe the presenting problem in detail, including symptoms, duration,and impact on daily life.
- Include client demographics like age and gender, information about social relationships, and mental health history.
- Describe all physical, emotional, and/or sensory symptoms reported by the client.
- Use patient quotes to describe the initial complaint verbatim. Follow with full-sentence summaries of relevant history details gathered, including key components that led to a working diagnosis.
- Summarize clinical exam results, namely orthopedic/neurological tests, imaging, lab tests, etc. Note actual results rather than subjective conclusions. Provide images if clearly reproducible/anonymized.
- Clearly state the working diagnosis or clinical impression before transitioning to management.
3. Management and Outcome
- Indicate the total duration of care and number of treatments given over what timeframe. Use specific names/descriptions for any therapies/interventions applied.
- Present the results of the intervention,including any quantitative or qualitative data collected.
- For outcomes, utilize visual analog scales for pain, medication usage logs, etc., if possible. Include patient self-reports of improvement/worsening of symptoms. Note the reason for discharge/end of care.
4. Discussion
- Analyze the case, exploring contributing factors, limitations of the study, and connections to existing research.
- Analyze the effectiveness of the intervention,considering factors like participant adherence, limitations of the study, and potential alternative explanations for the results.
- Identify any questions raised in the case analysis and relate insights to established theories and current research if applicable. Avoid definitive claims about physiological explanations.
- Offer clinical implications, and suggest future research directions.
5. Additional Items
- Thank specific assistants for writing support only. No patient acknowledgments.
- References should directly support any key claims or quotes included.
- Use tables/figures/images only if substantially informative. Include permissions and legends/explanatory notes.
- Provides detailed (rich qualitative) information.
- Provides insight for further research.
- Permitting investigation of otherwise impractical (or unethical) situations.
Case studies allow a researcher to investigate a topic in far more detail than might be possible if they were trying to deal with a large number of research participants (nomothetic approach) with the aim of ‘averaging’.
Because of their in-depth, multi-sided approach, case studies often shed light on aspects of human thinking and behavior that would be unethical or impractical to study in other ways.
Research that only looks into the measurable aspects of human behavior is not likely to give us insights into the subjective dimension of experience, which is important to psychoanalytic and humanistic psychologists.
Case studies are often used in exploratory research. They can help us generate new ideas (that might be tested by other methods). They are an important way of illustrating theories and can help show how different aspects of a person’s life are related to each other.
The method is, therefore, important for psychologists who adopt a holistic point of view (i.e., humanistic psychologists ).
Limitations
- Lacking scientific rigor and providing little basis for generalization of results to the wider population.
- Researchers’ own subjective feelings may influence the case study (researcher bias).
- Difficult to replicate.
- Time-consuming and expensive.
- The volume of data, together with the time restrictions in place, impacted the depth of analysis that was possible within the available resources.
Because a case study deals with only one person/event/group, we can never be sure if the case study investigated is representative of the wider body of “similar” instances. This means the conclusions drawn from a particular case may not be transferable to other settings.
Because case studies are based on the analysis of qualitative (i.e., descriptive) data , a lot depends on the psychologist’s interpretation of the information she has acquired.
This means that there is a lot of scope for Anna O , and it could be that the subjective opinions of the psychologist intrude in the assessment of what the data means.
For example, Freud has been criticized for producing case studies in which the information was sometimes distorted to fit particular behavioral theories (e.g., Little Hans ).
This is also true of Money’s interpretation of the Bruce/Brenda case study (Diamond, 1997) when he ignored evidence that went against his theory.
Breuer, J., & Freud, S. (1895). Studies on hysteria . Standard Edition 2: London.
Curtiss, S. (1981). Genie: The case of a modern wild child .
Diamond, M., & Sigmundson, K. (1997). Sex Reassignment at Birth: Long-term Review and Clinical Implications. Archives of Pediatrics & Adolescent Medicine , 151(3), 298-304
Freud, S. (1909a). Analysis of a phobia of a five year old boy. In The Pelican Freud Library (1977), Vol 8, Case Histories 1, pages 169-306
Freud, S. (1909b). Bemerkungen über einen Fall von Zwangsneurose (Der “Rattenmann”). Jb. psychoanal. psychopathol. Forsch ., I, p. 357-421; GW, VII, p. 379-463; Notes upon a case of obsessional neurosis, SE , 10: 151-318.
Harlow J. M. (1848). Passage of an iron rod through the head. Boston Medical and Surgical Journal, 39 , 389–393.
Harlow, J. M. (1868). Recovery from the Passage of an Iron Bar through the Head . Publications of the Massachusetts Medical Society. 2 (3), 327-347.
Money, J., & Ehrhardt, A. A. (1972). Man & Woman, Boy & Girl : The Differentiation and Dimorphism of Gender Identity from Conception to Maturity. Baltimore, Maryland: Johns Hopkins University Press.
Money, J., & Tucker, P. (1975). Sexual signatures: On being a man or a woman.
Further Information
- Case Study Approach
- Case Study Method
- Enhancing the Quality of Case Studies in Health Services Research
- “We do things together” A case study of “couplehood” in dementia
- Using mixed methods for evaluating an integrative approach to cancer care: a case study

Related Articles
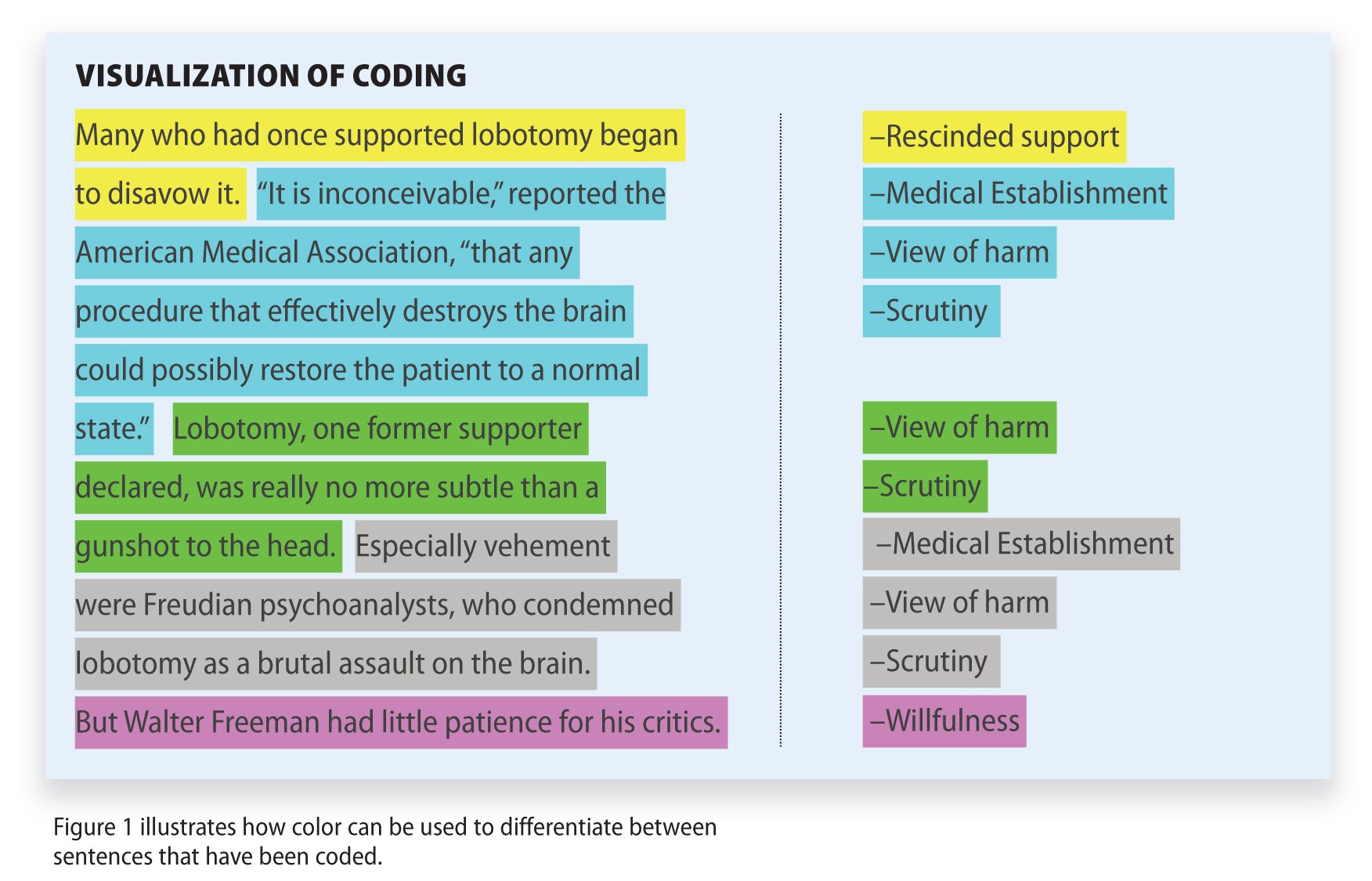
Research Methodology
Qualitative Data Coding
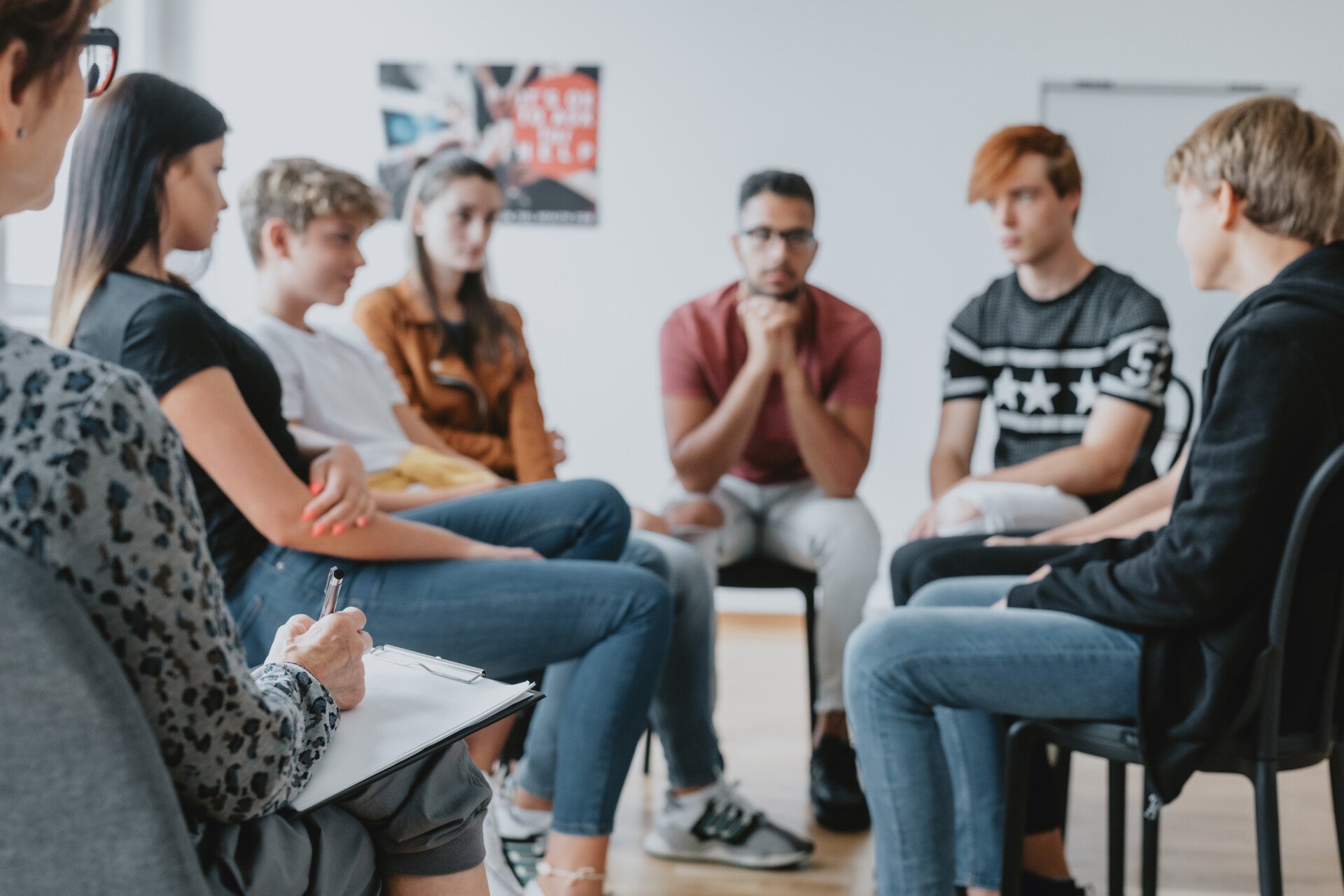
What Is a Focus Group?
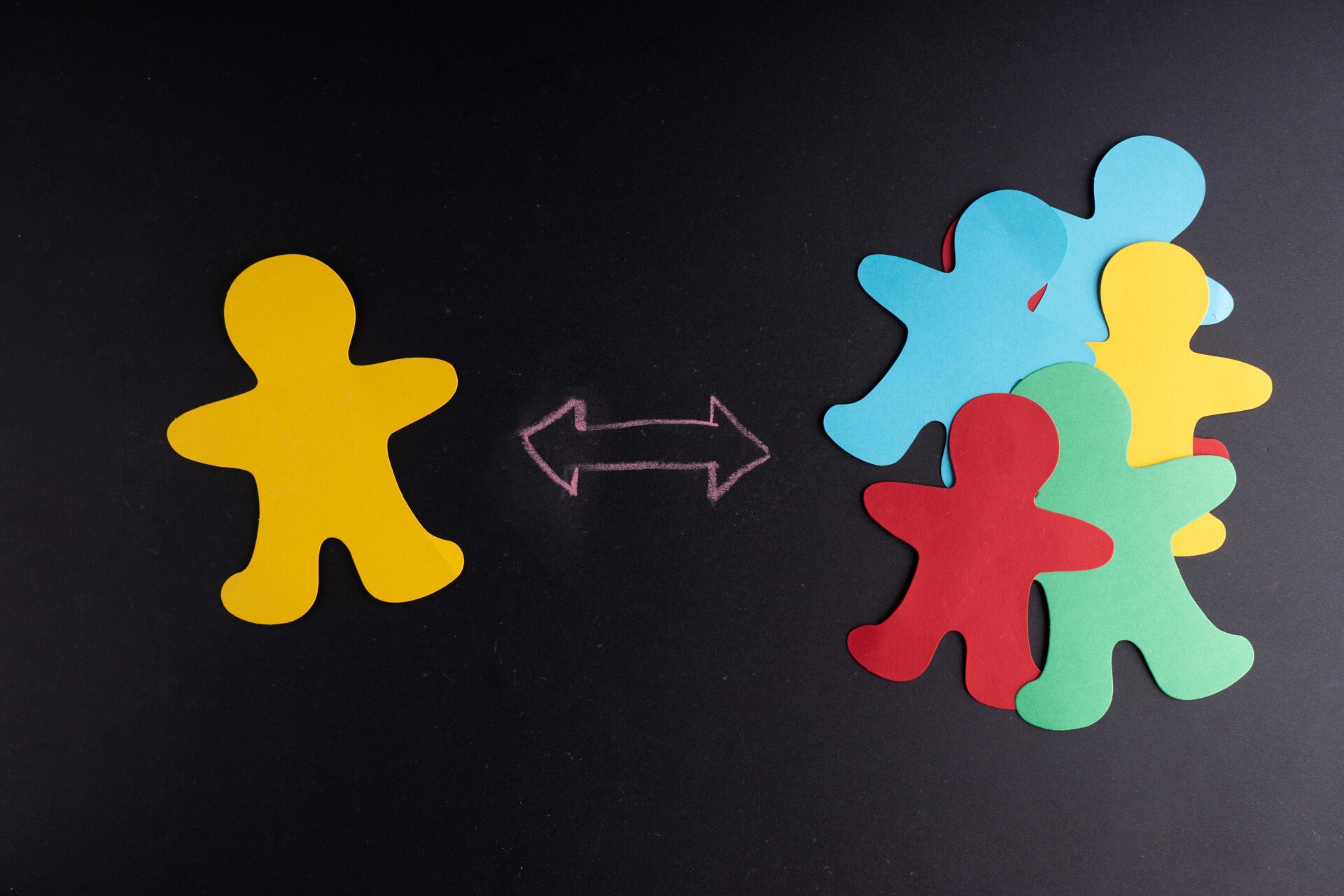
Cross-Cultural Research Methodology In Psychology
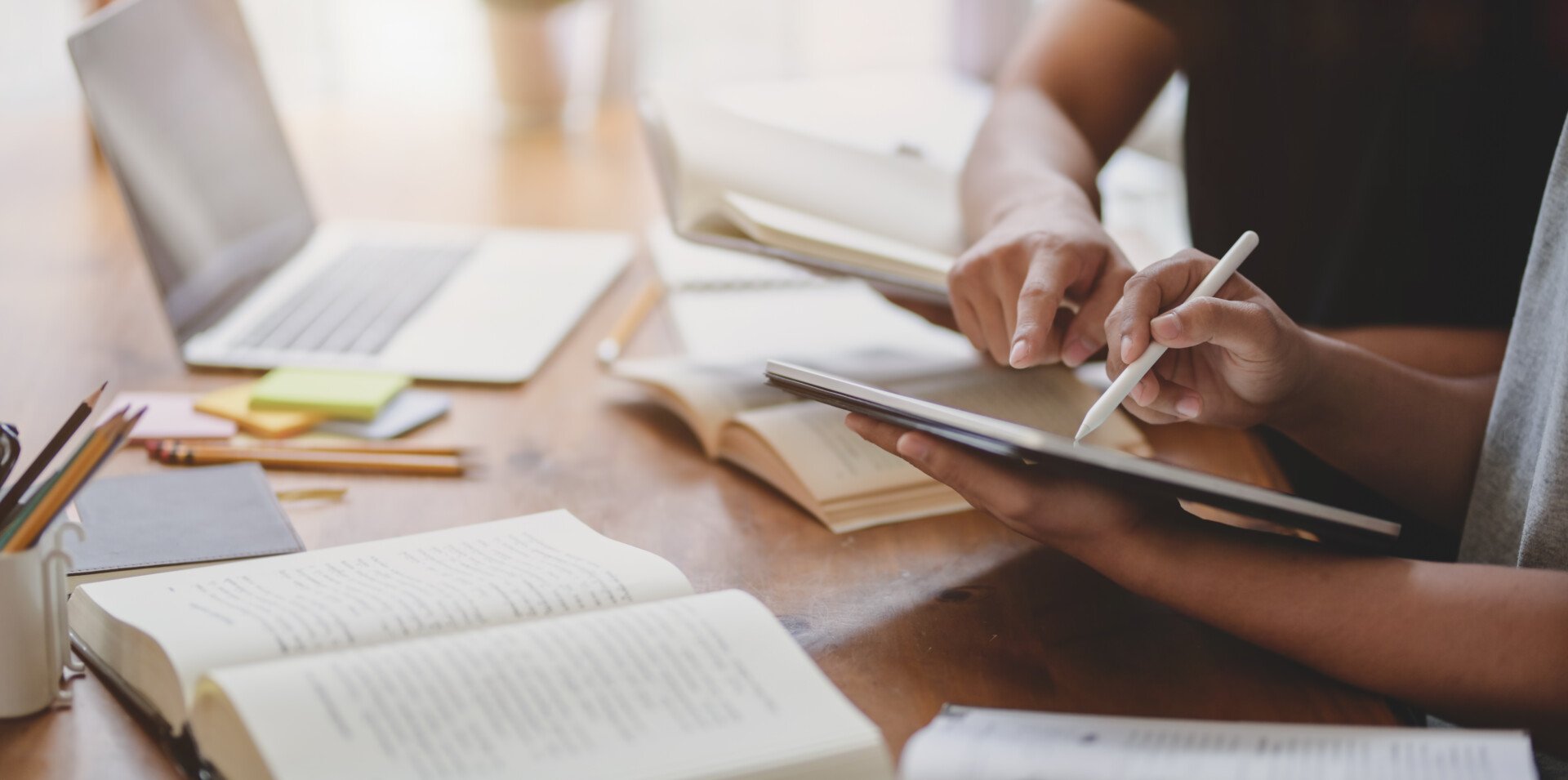
What Is Internal Validity In Research?
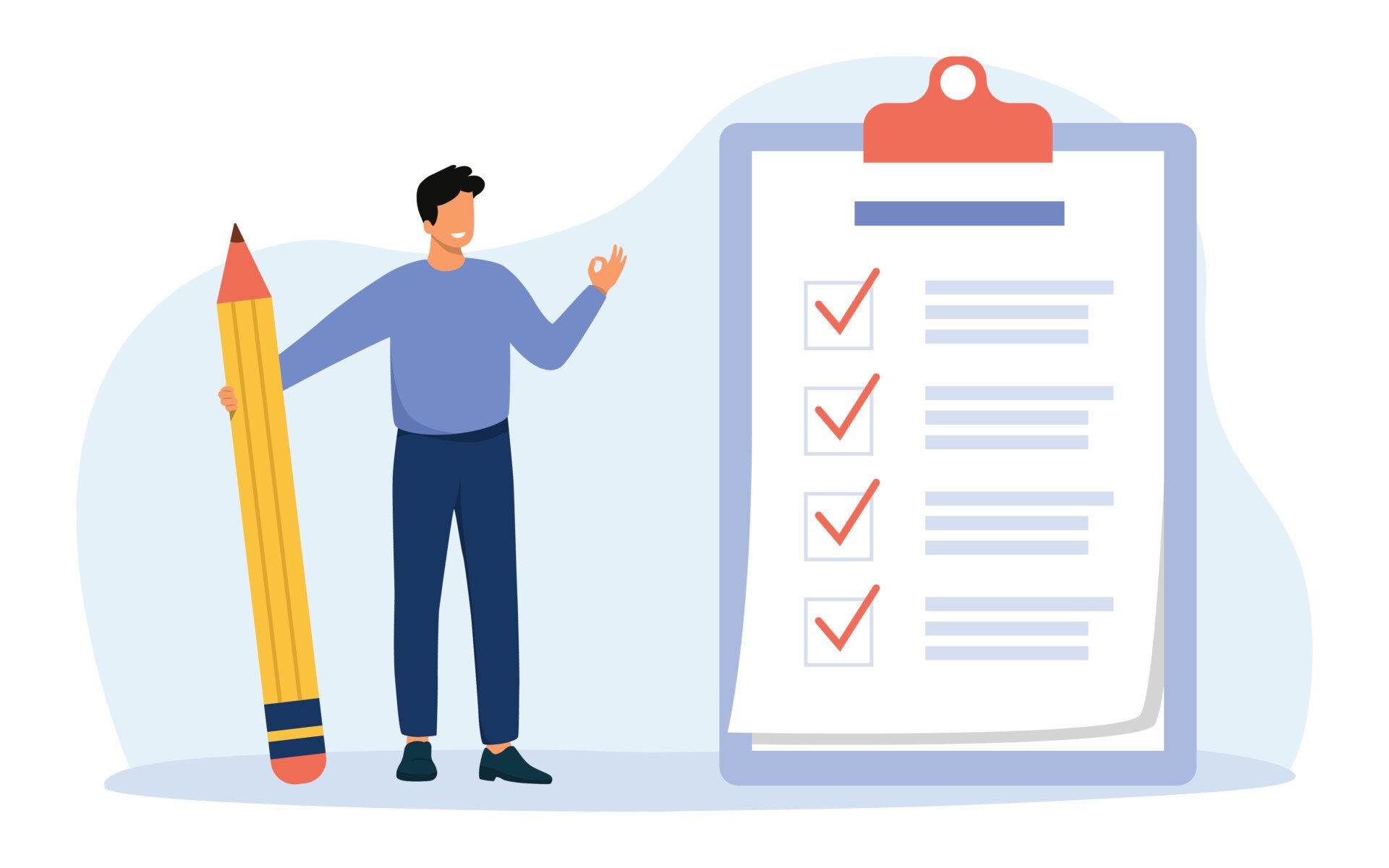
Research Methodology , Statistics
What Is Face Validity In Research? Importance & How To Measure
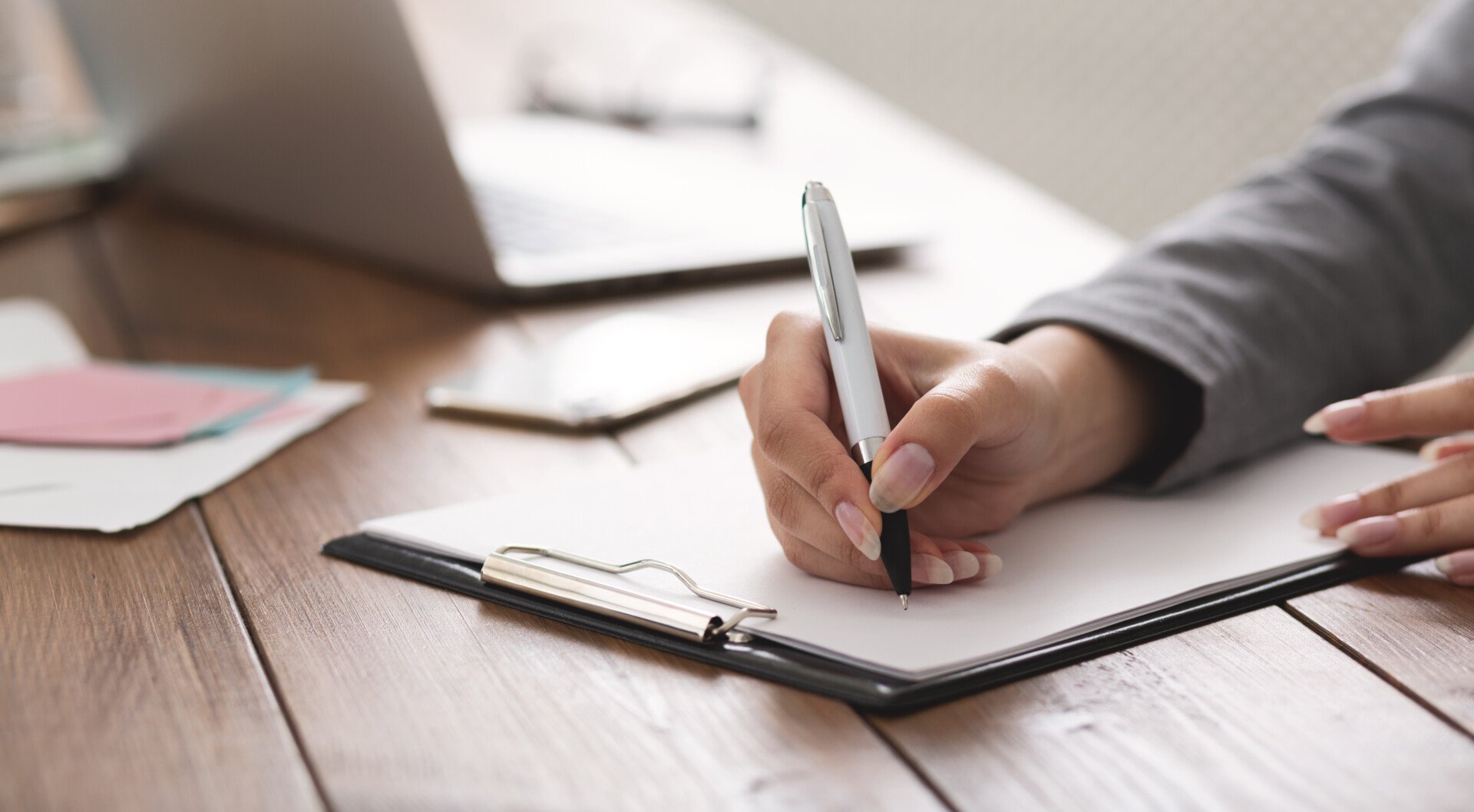
Criterion Validity: Definition & Examples

An official website of the United States government
The .gov means it’s official. Federal government websites often end in .gov or .mil. Before sharing sensitive information, make sure you’re on a federal government site.
The site is secure. The https:// ensures that you are connecting to the official website and that any information you provide is encrypted and transmitted securely.
- Publications
- Account settings
Preview improvements coming to the PMC website in October 2024. Learn More or Try it out now .
- Advanced Search
- Journal List
- HHS Author Manuscripts

Clinical Research Methodology I: Introduction to Randomized Trials
With increasing initiatives to improve the effectiveness and safety of patient care, there is a growing emphasis on evidence-based medicine and incorporation of high-quality evidence into clinical practice. The cornerstone of evidence-based medicine is the randomized controlled trial (RCT). The World Health Organization defines a clinical trial as “any research study that prospectively assigns human participants or groups of humans to one or more health-related interventions to evaluate the effects on health outcomes.” 1 Randomization refers to the method of assignment of the intervention or comparison(s). Fewer than 10% of clinical studies reported in surgical journals are RCTs, 2 – 4 and treatments in surgery are only half as likely to be based on RCTs as treatments in internal medicine. 5
Multiple factors impede surgeons performing definitive RCTs, including the inability to blind health care providers and patients, small sample sizes, variations in procedural competence, and strong surgeon or patient preferences. 5 – 8 Not all questions can be addressed in an RCT; Solomon and colleagues 8 estimated that only 40% of treatment questions involving surgical procedures are amenable to evaluation by an RCT, even in an ideal clinical setting. In surgical oncology, trials evaluating survival after operations for a rare malignancy can require an unreasonably large sample size. Pawlik and colleagues 9 estimated that only 0.3% of patients with pancreatic adenocarcinoma could benefit from pancreaticoduodenectomy with extended lymphadenectomy; a randomized trial of 202,000 patients per arm would be necessary to detect a difference in survival.
These reasons should not dissuade surgeons from performing RCTs. Even for rare diseases, randomized trials remain the best method to obtain unbiased estimates of treatment effect. 10 , 11 Rigorously conducted RCTs minimize bias by controlling for known and unknown factors (confounders) that affect outcomes and distort the apparent treatment effect. Observational studies, including those with the most sophisticated design and analysis, 12 , 13 can control only for known confounders and might not adequately control for those. Many surgical and medical interventions recommended based on observational studies have later been demonstrated to be ineffective or even harmful. These have included blood transfusions to maintain a hemoglobin >10 mg/dL in critically ill patients, 14 , 15 bone marrow transplantation for breast cancer, 16 – 19 and extracranial-intracranial bypass for carotid artery stenosis. 20 , 21
Another major reason for RCTs to be of interest to surgeons is that patients enrolled in trials can have improved short-term outcomes, even if the intervention is ineffective. 22 – 25 Potential sources of this benefit include enrollment of lower-risk patients, use of standardized protocols and improved supportive care, and greater effort to prevent and address treatment hazards. Different outcomes can also be observed in trial participants because of either the Hawthorne or placebo effect, both of which can distort the apparent treatment effect and threaten the validity of the trial. The Hawthorne effect occurs when changes in clinicians’ or patients’ behavior, because of being observed, results in improved outcomes. For example, a prospective observational study evaluating operating room efficiency after an intervention can demonstrate improvement over historic performance, in part because the staff is aware of being observed rather than as a result of the intervention. The placebo effect occurs when the patient derives benefit not from the treatment itself, but from the patient’s expectations for benefit. In a randomized trial of arthroscopic surgery versus sham surgery for osteoarthritis of the knee, the placebo procedure had equivalent results to debridement and lavage, despite lack of any therapeutic intervention. 26
Despite the advantages of well-conducted RCTs, poorly conducted trials or inadequately reported results can yield misleading information. 27 , 28 Recently, Chang and colleagues 29 demonstrated the continued paucity of high-level evidence in surgical journals and called for articles on clinical research methodology to educate surgeons. The purpose of this article is to serve as an introduction to RCTs, focusing on procedures for assigning treatment groups that serve to minimize bias and error in estimating treatment effects. Common threats to validity and potential solutions to difficulties in randomizing patients in surgical trials will also be discussed.
OBSERVATIONAL COHORT STUDIES
RCTs are the gold standard for evaluating the effectiveness of an intervention. Many therapies have historically been evaluated in surgery using observational cohort studies where groups of patients are followed for a period of time, either consecutively or concurrently. These studies can be conducted retrospectively or prospectively. The fundamental criticism of observational cohort studies is that confounding can result in biased estimates of treatment effect. 30 , 31
A confounder is a known or unknown factor that is related to the variable of interest (eg, an intervention) and is a cause of the outcomes. For example, suppose a study finds that patients undergoing a procedure by surgeon A have increased mortality when compared with surgeon B. The outcomes difference might not be a result of inferior operative technique of surgeon A, but rather confounders, such as patients’ comorbidities or severity of disease (eg, if surgeon A is referred the more complicated patients).
Observational studies cannot account for unknown confounders. Novel statistical methods can improve estimates of treatment effect because of known and unknown confounders in nonrandomized trials, but are still subject to limitations. 32 , 33 Traditionally, nonrandomized or observational studies adjust for known confounders in the statistical analysis. Adjustment refers to the mathematic modeling of the relationship between one or more predictor variables and the outcomes to estimate the isolated effect of each variable. Even with advanced statistical analyses, such as propensity scoring, these models cannot completely adjust for all of the confounders. 12 Although observational cohort studies have a role in clinical research, such as in answering questions about harm, well-designed RCTs are the gold standard for evaluating an intervention because they minimize bias from known and unknown confounders.
OVERVIEW OF RANDOMIZATION AND ALLOCATION CONCEALMENT
Properly designed RCTs minimize imbalances in baseline characteristics between groups and could distort the apparent effect of the difference in treatment on patient outcomes. The randomization procedure used to assign treatment and prevent prediction of treatment assignment resulting in allocation of intervention bias is especially important. With random assignment, each patient has the same chance of being assigned to a specific treatment group. Equal (1:1) allocation results in the same likelihood of assignment to either group (50:50) and the greatest power to detect a difference in outcomes between the groups. Unequal or weighted randomization allows the investigators to maintain a balance between groups in their baseline characteristics, but allocate more patients to one group (eg, with a 2:1 allocation, two-thirds of patients will be assigned the first treatment and one-third to the second). Unequal randomization can be used to decrease costs when one treatment is considerably more expensive to provide than the other. 34 , 35
Valid methods of randomization include flipping a coin, rolling a die, using a table of random numbers, or running a computerized random allocation generator (eg, http://www.random.org ). Randomization should be performed in such a way that the investigator should not be able to anticipate treatment group. 36 – 38 Not all published trials reported as “randomized” are truly randomized. In fact, only 33% to 58% of published surgical trials describe a valid randomization process where the treatment assignment cannot be predicted. 39 , 40 For example, in a randomized trial evaluating screening mammography, participants were assigned based on which day of the month they were born (patients born between the 1st and 10th of the month or 21st and 31st were assigned mammography and patients born between the 11th and the 20th were assigned the control). 41 Results were questioned because anticipation of treatment group can inadvertently influence whether a patient is considered to meet eligibility criteria or how much effort is devoted to securing informed consent 42 and so can cause selection biases with baseline differences between groups that can influence the results. Other “pseudorandom” or “quasirandom” schemes include use of medical record number or date of enrollment.
Allocation concealment prevents the investigator or trial participant from consciously or subconsciously influencing the treatment assignment and causing selection bias. Allocation concealment, which occurs before randomization, should not be confused with blinding (also known as masking), which occurs after randomization. Where a valid randomization scheme has been used, allocation can still be inadequately concealed (eg, use of translucent envelopes containing treatment assignments). Methods of allocation concealment include use of sequentially numbered, sealed, opaque envelopes or allocation by a central office. Allocation concealment is always possible, although blinding is not. 38 Yet, between 1999 and 2003, only 29% of published surgical trials reported allocation concealment. 40 Although allocation concealment can be used but not noted in published reports, inadequate allocation concealment appears to be a large and generally unrecognized source of bias. Schulz and colleagues 43 found that treatment effect was overestimated by 41% when allocation concealment was inadequate or unclear.
VARIATIONS IN RANDOMIZATION SCHEMES
Simple randomization.
The most straightforward scheme for allocating patients is simple randomization ( Fig. 1A ), with treatment assigned using one of the methods mentioned previously (eg, computer-generated random sequence). Simple randomization can result, by chance alone, in unequal numbers in each group—the smaller the sample size, the larger the likelihood of a major imbalance in the number of patients or the baseline characteristics in each group. 44 An example of simple randomization would be the sequence of 20 random numbers generated using a computer program ( Table 1 ).

Randomization procedures. In this example, balls represent patients and the color represents a prognostic factor (eg, ethnicity). (A) In simple randomization, group assignment can be determined by a flip of a coin, roll of a die, random number table, or computer program. With small sample sizes, there can be an unequal number in each treatment arm or an unequal distribution of prognostic factors, or both. Note that the numbers in the treatment arms are unequal and the prognostic factor is unevenly distributed between the two. (B) In blocked randomization with uniform or equal blocks, randomization occurs in groups (blocks), and the total sample size is a multiple of the block size. In the figure, balls were randomized in blocks of six. Note that the number in each treatment arm is equal, but the prognostic factor is not equally balanced between the two. (C) In blocked randomization with varied blocks, the size of the blocks changes either systematically or randomly to avoid predictability of treatment assignment. In the figure, the first block has eight balls and the second block has four balls. Again, the number in each treatment arm is equal, but the prognostic factor is not balanced between them. (D) In stratified blocked randomization, the total sample is divided into one or more subgroups (strata) and then randomized within each stratum. In this example, the sample population is divided into black and white balls and then randomized in blocks of six. There are an equal number of balls in both arms, and the distribution of the black and white balls is balanced as well.
Examples of Randomization
Treatment could be allocated as evens receiving treatment A and odds receiving treatment B. In this case, 9 patients would receive treatment A and 11 patients would receive treatment B ( Table 1 ).
Alternatively, patients assigned to a number between 1 and 50 could receive treatment A and patients assigned to a number between 51 and 100 could receive treatment B. In this case, 6 patients would receive treatment A and 14 would receive treatment B. In small trials or in larger trials with planned interim analyses, simple randomization can result in imbalanced group numbers. Even if the groups have equal numbers, there can be important differences in baseline characteristics that would distort the apparent treatment effect.
Blocked (restricted randomization)
Simple randomization can result not only in imbalanced groups, but also chronological bias in which one treatment is predominantly assigned earlier and the other later in the trial. Chronological bias is important if outcomes changes with time, as when surgeons become more adept at the procedure under investigation or increasing referrals for the procedures changes the patient population. 45 Chronological bias results in an inability to differentiate between the effects of temporally related factors, such as surgeon experience and treatment. For these reasons, blocked (restricted) randomization schemes can be used ( Figs. 1B, 1C ).
For example, a uniform block size of 4 can be used with 1:1 allocation and two treatment arms. The two arms will never differ at any time by more than two patients, or half of the block length. There are six possible assignment orders for each block (called a permuted block) of four patients: AABB, ABAB, ABBA, BAAB, BABA, and BBAA. Although blocked randomization will maintain equal or nearly equal group sizes across time, selection bias can occur if the investigators are not blinded to block size and treatment assignment. If the first three patients in the trial received treatments A, A, and B, then the unblinded investigator might anticipate that the fourth patient will receive treatment B. The decision whether to enroll the next study candidate could be inadvertently affected, as a result, by the investigator’s treatment preference. 42 This problem can generally be avoided by randomly or systematically varying the block sizes ( Fig. 1C ).
Stratified randomization
Imbalances in prognostic factors between treatment arms can occur because of chance alone, even if randomization and allocation concealment are properly performed. Important imbalances are most likely to occur by chance in small trials or during interim analyses of large RCTs. 46 Prognostic stratification can be used to avoid such imbalances. Patients can be first categorized based on several prognostic factors into strata and then randomized within each stratum, guaranteeing no major imbalance between groups in these factors ( Fig. 1D ). For example, Fitzgibbons and colleagues 47 performed an RCT to evaluate whether watchful waiting is an acceptable alternative to tension-free repair for inguinal hernias in minimally symptomatic or asymptomatic patients. Eligible patients were stratified by center (six total), whether the hernia was primary or recurrent, and whether the hernia was unilateral or bilateral. The total number of strata for this study was 24, or the product of the number of levels of each factor (6 × 2 × 2). Once assigned to a stratum, patients were then randomized to either watchful waiting or hernia repair.
Because important prognostic factors will be balanced, stratified randomization can decrease the chance of a type I error (finding a difference between treatment arms because of chance alone) and can increase the power (the chance of finding a difference if one exists) of small studies, where the stratified factors have a large effect on outcomes. 46 Additionally, stratification can increase the validity of subgroup or interim analyses. 46 If too many strata are used, some strata might not be filled with equal patients in both groups, leading to imbalances in other prognostic factors. 46 Excessive stratification also unduly increases the complexity of trial administration, randomization, and analysis. Stratification is usually performed using only a small number of carefully selected variables likely to have a large impact on outcomes.
Adaptive randomization
Another strategy to minimize imbalances in prognostic factors is to use an adaptive randomization scheme when randomization is influenced by analysis of either the baseline characteristics or outcomes of previous patients. When treatment assignment is based on patient characteristics, the adaptive randomization procedure known as minimization assigns the next treatment to minimize any imbalance in prognostic factors among previously enrolled patients. For the computer algorithm to run, minimization should be limited to larger trials. 48
One response-adaptive randomization procedure used in trials examining a dichotomous outcomes (eg, yes/no or survival/death) involves the “play-the-winner” strategy to allocate treatment based on outcomes of the last patient enrolled ( Fig. 2 ). The more successful a treatment, the more likely that the next patient will be randomized to that treatment. 49 For short-term trials where the treatments have been well-evaluated for safety, play-the-winner trials can reduce the likelihood that a patient is assigned to an ineffective or harmful treatment. 49 Adaptive trials are being increasingly used in phase 1 or 2 cancer trials. 50 – 52 The downside is that these trials are complex to plan and analyze, quite susceptible to chronological bias, and might not be persuasive.

Adaptive randomization: play the winner randomization rule. In this example, the color of the ball represents the treatment assignment (white = treatment A, black = treatment B). A ball is selected and then replaced. Based on the outcomes of the treatment selected, a ball representing the same or opposite treatment is added to the sample. The rule repeats itself. If the two treatments have similar outcomes, then there will be an equal distribution of balls at the end of the trial. If one treatment has substantially better outcomes, then there will be more balls representing that treatment. Patients entering the trial have a better chance of having an effective treatment and less of a chance of having an ineffective or harmful treatment.
The most well-known and controversial play-the-winner randomized trial was the Michigan Extracorporeal Membrane Oxygenation (ECMO) trial for neonatal respiratory failure by Bartlett and colleagues. 53 Neonates with respiratory failure and a predicted ≥ 80% chance of mortality were randomized to either ECMO or conventional treatment. The investigators’ intent was to perform a methodologically sound, randomized trial that minimized the number of critically ill infants given the inferior treatment. The study was designed to end after 10 patients had received ECMO or 10 patients had received the control. The first patient enrolled received ECMO and survived; the second patient received the control and died. The trial was terminated after 11 patients, only 1 of whom received the control. The investigators concluded that ECMO improved survival when compared with conventional treatment in neonates with respiratory failure. The main criticism of the trial was that the control group included only one patient. 54 Widespread acceptance of ECMO for neonates with respiratory failure did not occur until after larger and more conventional trials were performed. 55
RANDOMIZED DESIGNS INCORPORATING PATIENT AND SURGEON PREFERENCES
Patient preference trials.
Strong patient preferences can result in failure to enroll patients into surgical RCTs or serve as a theoretical threat to validity. 6 , 35 , 56 Patients with strong preferences for one treatment can differ from those without, resulting in selection bias or a systematic difference in patients enrolled in trials from those not enrolled. 57 Patient preference can also be an independent prognostic factor or can interact with the treatment to affect outcomes, particularly in unblended RCTs. 58 For example, patients randomized to their preferred treatment can perform better because of increased compliance or a placebo effect, and patients randomized to their nonpreferred treatment can perform worse. 57 One potential solution is to measure baseline patient preferences and to mathematically adjust for the interaction between preference and treatment, but this approach increases sample size. 58
Another solution is to modify the trial design to incorporate patient or physician preferences using a comprehensive cohort design, Zelen’s design, or Wennberg’s design. 35 , 56 , 59 – 61 These trial designs have not been commonly used. In the comprehensive cohort design, patients without strong preferences are randomized, and patients with strong preferences are assigned to their treatment of choice. 35 It is often used in trials where participation in an RCT might be low because of strong patient preferences. Even if the proportion of patients randomized is low, this design should be less susceptible to bias than a nonrandomized cohort study, which is often encouraged by statisticians when problems in accrual can limit the power of a conventional randomized trial. Because of the lower randomization rates, these trials can be more expensive, require more total patients, and be more difficult to interpret than conventional trials. 57
The National Institute of Child Health and Human Development Neonatal Research Network is currently planning a comprehensive cohort trial comparing laparotomy and peritoneal drainage for extremely low birth weight infants with severe necrotizing enterocolitis or isolated intestinal perforation (personal communication, Blakely). These conditions in this patient population are associated with a 50% mortality rate and a 72% rate of death or neurodevelopmental impairment. Surgical practice varies widely; caregivers often have strong treatment preferences, 62 , 63 and the consent rate can be no higher than 50% because of problems obtaining consent for emergency therapies. In this trial, the same risk and outcomes data will be collected for nonrandomized patients (observational or preference cohort) and for randomized patients. In the primary analysis, treatment effect will be assessed as the relative risk of death or neurodevelopmental impairment with laparotomy (relative to drainage) among randomized patients. The relative risk for death or impairment with laparotomy will also be assessed in the observational cohort after adjusting for important known risk factors. If the relative risk for observational cohort is similar to that for randomized patients, all patients can be combined in a supplemental analysis to increase the power, precision, and generalizability of the study in assessing treatment effect. An analysis with all patients combined would not be performed if the relative risk is not comparable for the randomized patients and the observational cohort. In this circumstance, the difference might well be because of an inability to adjust for unknown or unmeasured confounders among patients treated according to physician or parent preference
Zelen’s design, also known as the postrandomization consent design, has two variants. In the single-consent design, patients randomized to the standard therapy are not informed of the trial or offered alternative therapy. Consent is sought only for patients randomized to the intervention. If consent is refused, they are administered standard therapy but analyzed with the intervention group. The single-consent design raises ethical concerns because patients are randomized before consent and because patients receiving standard therapy are included without informed consent of their participation in the trial. In the double-consent design, consent is sought for patients randomized to a standard therapy and those randomized to the intervention, and both groups are informed of the trial and both groups are allowed to receive the opposite treatment if consent is refused for the treatment to which they were randomized. Zelen’s design has been used to evaluate screening tools such as fecal occult blood testing for colorectal cancer. 64
With Wennberg’s design, patients are randomized to either a preference group or a randomization group. Patients in the preference group are offered their treatment of choice, and patients in the other group are assigned treatment based on randomization. All groups are analyzed to assess the impact of patient preference on outcomes. 35 , 59 Although patient preference trials are an alternative to RCTs, downsides include potential for additional differences between treatment groups other than preference and increased sample size requirements or cost to complete a trial. 34 , 57
EXPERTISE-BASED TRIALS
A proposed solution to the problem of variation between surgeons in skill and preference is the expertise-based RCT. In a conventional RCT evaluating two surgical procedures (eg, open versus laparoscopic hernia repair), a surgeon can be asked to perform both procedures, even though he or she might be adept with only one. Differential expertise bias can result from favoring the less technically challenging or more familiar procedure if a higher percentage of experienced surgeons performed that procedure. 45 Additionally, differential expertise can result in increased cross-over from one procedure to another (eg, conversion from laparoscopic to open hernia repair), or bias resulting from use of different co-interventions. 45
An expertise-based trial differs from a conventional RCT because surgeons perform only the procedure at which they believe they are most skilled. Proponents argue that expertise-based trials minimize bias resulting from differences in technical competency and surgeon preference, decrease crossover from one intervention to the other, and can be more ethical than conventional RCTs. 45 On the other hand, expertise-based RCTs present challenges in coordinating trials in which there are few experts for one or both procedures; changing surgeons after the initial patient contact, or generalizing the results to surgeons with less expertise. 45
For example, in a trial comparing open with endovascular aortic aneurysm repair, the investigators required each participating surgeon to have performed 20 endovascular aortic aneurysm repair procedures to control for expertise bias. 65 The trial demonstrated no difference in all-cause mortality between the groups. 66 Performance of 60 endovascular repairs, or 40 more than the minimum requirement for surgeon participation in this study, appears to be necessary to achieve an acceptable failure rate of < 10%. The minimum number of procedures required to participate in a trial is often less than the number needed to reach the plateau of the learning curve, biasing the results. 45 An expertise-based RCT, NExT ERA: National Expertise Based Trial of Elective Repair of Abdominal Aortic Aneurysms: A Pilot Study, is planned to prevent problems in interpreting the trial because of differential surgical expertise from affecting outcomes after aneurysm repair ( www.clinicaltrials.gov ; {"type":"clinical-trial","attrs":{"text":"NCT00358085","term_id":"NCT00358085"}} NCT00358085 ).
INTERNAL AND EXTERNAL VALIDITY IN RANDOMIZED TRIALS
Before applying the results of RCTs to individual patients, the internal and external validity of the trial must be examined. Internal validity refers to the adequacy of the trial design to provide a true estimate of association between an exposure and outcomes in patients studied, and external validity assesses the generalizability of the results to other patients. Threats to internal validity can result from either random or systematic error. Random errors result in errors in either direction, but systematic errors are a result of bias, resulting in consistent variation in the same direction. An example of random error is the up and down variability in blood pressure measurements based on the precision of an automatic cuff. A systematic error occurs when all of the blood pressure measurements are high because the cuff is too small. Bias can occur at any point in a trial, including during design, selection of the participants, execution of the intervention, outcomes measurement, data analysis, results interpretation, or publication. Specific types of bias include selection bias that results from systematic differences between treatment groups, confounding, ascertainment bias that results from lack of blinding of outcomes assessors, compliance bias because of differential adherence to the study protocols, and bias because of losses or withdrawals to followup. 67
External validity is dependent on multiple factors, including the characteristics of the participants, the intervention, and the setting of the trial. 68 Enrolled patients can differ substantially from eligible patients and ineligible patients with the condition of interest, or both, representing only a select population. An analysis of RCTs in high-impact medical journals found that only 47% of exclusion criteria were well-justified, and large subpopulations, such as women, children, the elderly, and patients with common medical conditions, were often excluded from RCTs. 69 In evaluating external validity, the difference between efficacy (explanatory) and effectiveness (management or pragmatic) trials must also be considered. Efficacy trials test whether therapies work under ideal conditions (eg, highly protocolized interventions and small number of homogeneous patients), and effectiveness trials test whether therapies work under routine or “real-world” circumstances (eg, large number of diverse patients and broad range of clinically acceptable co-interventions). Efficacy trials maximize internal validity and effectiveness trials emphasize external validity. 70
Well-designed RCTs reduce systematic errors from selection bias, biased treatment assignment, ascertainment bias, and confounding. Even with adequate randomization and allocation concealment, as described here, both random and systematic errors can still occur. Larger sample sizes can decrease the risk of imbalances because of chance and can increase the external validity of trials as well by including more diverse patients (eg, pragmatic trials). A description of all potential threats to validity is beyond the scope of this article.
Despite the perceived barriers to performing randomized clinical trials in surgery, they remain the gold standard for evaluating an intervention. Surgeons must be aware of the potential methodologic flaws that can invalidate results, both in interpreting and applying the literature and in designing future trials. To promote rigorous, high-quality studies, surgeons should be aware of variations in trial design, and increase use of alternative designs when conventional trials would not be feasible or suitable.
Acknowledgments
Dr Kao is supported by the Robert Wood Johnson Foundation Physician Faculty Scholars Award and the National Institutes of Health (K23RR020020-01). Dr Lally was supported by the National Institutes of Health (K24RR17050-05).
Competing Interests Declared: None .
Author Contributions Study conception and design: Kao, Lally
Acquisition of data: Kao
Analysis and interpretation of data: Kao, Lally
Drafting of manuscript: Kao, Tyson, Blakely, Lally
Critical revision: Kao, Tyson, Blakely, Lally
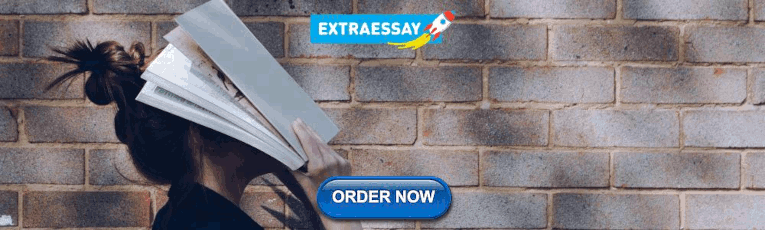
IMAGES
VIDEO
COMMENTS
Background Methodological studies - studies that evaluate the design, analysis or reporting of other research-related reports - play an important role in health research. They help to highlight issues in the conduct of research with the aim of improving health research methodology, and ultimately reducing research waste. Main body We provide an overview of some of the key aspects of ...
Similar in essence, clinical research methods differ somewhat, depending on the type of study. Type is an integral element of study design and depends on the research question to answer. It should be specified before the start of any study . Selecting an inappropriate study type results in flawed methodology, and if it occurs after commencement ...
In clinical research, our aim is to design a study which would be able to derive a valid and meaningful scientific conclusion using appropriate statistical methods. The conclusions derived from a research study can either improve health care or result in inadvertent harm to patients. Hence, this requires a well‐designed clinical research ...
The goal of this review was to present the essential steps in the entire process of clinical research. Research should begin with an educated idea arising from a clinical practice issue. ... Springer's Journal Author Academy, and SAGE's Research methods [34-37]. Standardized research reporting guidelines often come in the form of checklists ...
What is clinical research, and is it right for you? Clinical research is the comprehensive study of the safety and effectiveness of the most promising advances in patient care. Clinical research is different than laboratory research. It involves people who volunteer to help us better understand medicine and health.
Abstract. Clinical research can be categorized by the timing of data collection: retrospective or prospective. Clinical research also can be categorized by study design. In case-control studies, investigators compare previous exposures (including genetic and other personal factors, environmental influences, and medical treatments) among groups ...
Clinical research can be categorized by the timing of data collection: retrospective or prospective. Clinical research also can be categorized by study design. In cross-sectional studies, exposure and outcome are evaluated simultaneously. In case-control studies, investigators compare previous exposures (including genetic and other personal ...
Principles of Research Methodology: A Guide for Clinical Investigators is the definitive, comprehensive guide to understanding and performing clinical research. Designed for medical students, physicians, basic scientists involved in translational research, and other health professionals, this indispensable reference also addresses the unique challenges and demands of clinical research and ...
Publish your healthcare research with BMC Medical Research Methodology, with 4.0 Impact Factor and 40 days to first decision. Focusing on manuscripts ...
Masterclass in Clinical Research Methodology. Conducting clinical research has a tremendous impact on patient care and management as well as improving public health. Therefore, it is essential that the study is designed and conducted properly to maximize impact. This one-day workshop aims to give researchers the necessary skills to develop a ...
Clinical trials - methods. 2. Biomedical research - methods. 3. Ethics, Research. 4. Manuals. I. World Health Organization. ISBN 92 4 159392 X (NLM classifi cation: W 20.5) Contents Preamble 1 Introduction 3 ... Clinical research is necessary to establish the safety and effective-ness of specifi c health and medical products and practices ...
Finally, also pre-clinical studies are of outmost importance, representing the steppingstone of clinical trials. It is therefore important to understand the types of method for clinical research. Thus, this review focused on various aspects of the methodology and describes the crucial steps of the conceptual and executive stages.
The Research Methods Resources website provides investigators with important research methods resources to help them design their studies using the best available methods. The material is relevant to both randomized and non-randomized trials, human and animal studies, and basic and applied research. The information provided here represents ...
Foundations of Clinical Research. This Harvard Medical School six-month, application-based certificate program provides the essential skill sets and fundamental knowledge required to begin or expand your clinical research career. Learn More. September 28, 2024 - April 6, 2025. $6,900 - $7,900.
The Clinical Research Methods (CRM) track in Biostatistics responds to a pressing need for advanced training in clinical research design and analysis. As medical school curricula become increasingly full and apprenticeship prospects wane, pathways to becoming a clinical researcher have narrowed. This program offers talented-but-novice ...
They help to highlight issues in the conduct of research with the aim of improving health research methodology, and ultimately reducing research waste. ... Geerlings MI, Vergouwe Y, Steyerberg EW, Altman DG, Moons KGM. Reporting and methods in clinical prediction research: a systematic review. PLoS Med. 2012; 9 (5):1-12. [PMC free article ...
The book on "Basic Principles of Clinical Research and Methodology" contributed by the eminent professionals from Academia and Industry will fulfill the long felt need for such a publication in the country. I congratulate Institute of Clinical Research (India) and Professor S.K. Gupta for bringing out this excellent publication.
Health research methodology: A guide for training in research methods INTRODUCTION This is a revised version of an earlier manual on Health Research Methodology and deals with the basic concepts and principles of scientific research methods with particular attention to research in the health field. The research process is the cornerstone for ...
The best free clinical research courses available are Clinical Data Management, Clinical Research, Research Methods, ... Clinical Research is a healthcare science that determines the safety and effectiveness of interventions designed to prevent or treat disease in humans. These interventions can be drugs, devices, surgeries, behavioral ...
Clinical Research Methods. Director: Todd Ogden, PhD. The Mailman School offers the degree of Master of Science in Biostatistics, with an emphasis on issues in the statistical analysis and design of clinical studies. The Clinical Research Methods track was conceived and designed for clinicians who are pursuing research careers in academic medicine.
The Clinical Research Methodology Curriculum (CRMC) is a one-year clinical research methodology for investigators with clinical research experience seeking to obtain up-to-date knowledge in the field of clinical research. It is conducted at Memorial Sloan Kettering Cancer Center to promote greater flexibility for trainees from across the CTSC partner institutes.
The Relationship between Clinical Questions and Research Methods. Clinical questions often emerge from direct patient care or epidemiological observations and guide the selection of appropriate research methods by exposing key elements of the research focus, enabling the selection of methodologies to provide accurate and reliable results.
The value of using qualitative methods within clinical trials is widely recognised. How qualitative research is integrated within trials units to achieve this is less clear. This paper describes the process through which qualitative research has been integrated within Cardiff University's Centre for Trials Research (CTR) in Wales, UK. We highlight facilitators of, and challenges to, integration.
Clinical research is an alternative terminology used to describe medical research. Clinical research involves people, and it is generally carried out to evaluate the efficacy of a therapeutic drug, a medical/surgical procedure, or a device as a part of treatment and patient management. ... Clinical trial methods and terminologies. This table ...
ocrtraining.cit.nih.gov
Deep learning techniques provide reliable, consistent, and accurate results. Due to this, they are widely applied across multiple domains to solve real-world problems [24,25,26,27].Researchers have carried out diverse literature that includes datasets, algorithms, and methodology to facilitate future research in the classification and detection of lung cancer.
Some clinical trials of masks and respirators did not adequately define or optimize the intervention or maintain its fidelity, used heterogenous interventions and outcomes, or failed to measure whether masks were actually worn (see Clinical Trials of Masks and Respirators). ... with varying research questions, methods, and interpretations ...
Case studies are in-depth investigations of a person, group, event, or community. Typically, data is gathered from various sources using several methods (e.g., observations & interviews). The case study research method originated in clinical medicine (the case history, i.e., the patient's personal history). In psychology, case studies are ...
The World Health Organization defines a clinical trial as "any research study that prospectively assigns human participants or groups of humans to one or more health-related interventions to evaluate the effects on health outcomes." 1 Randomization refers to the method of assignment of the intervention or comparison (s).
Clinical Trials Database and Methodology ... ResearchAndMarkets.com is the world's leading source for international market research reports and market data. We provide you with the latest data on ...