
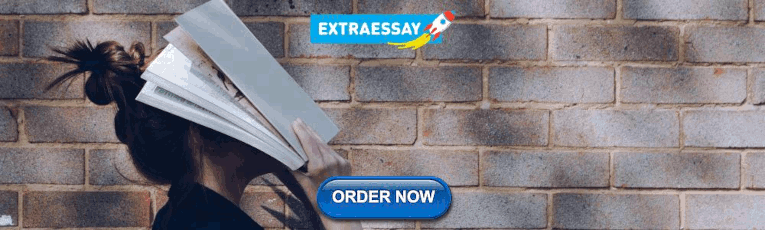
A Poultry Farm Management System
Content maybe subject to copyright Report
Citation Count
Study of Smart Management System in Poultry Farming
Related papers (5), smart poultry farm monitoring using iot and wireless sensor networks, e-poultry: an iot poultry management system for small farms, wireless sensor network: a complete solution for poultry farming, research on application technology of 5g internet of things and big data in dairy farm, an iot platform towards the enhancement of poultry production chains..

An official website of the United States government
The .gov means it’s official. Federal government websites often end in .gov or .mil. Before sharing sensitive information, make sure you’re on a federal government site.
The site is secure. The https:// ensures that you are connecting to the official website and that any information you provide is encrypted and transmitted securely.
- Publications
- Account settings
Preview improvements coming to the PMC website in October 2024. Learn More or Try it out now .
- Advanced Search
- Journal List
- Animals (Basel)

A Systematic Review of Precision Livestock Farming in the Poultry Sector: Is Technology Focussed on Improving Bird Welfare?
Elizabeth rowe.
1 Department of Zoology, University of Oxford, Mansfield Road, Oxford OX1 3SZ, UK
Marian Stamp Dawkins
Sabine g. gebhardt-henrich.
2 Center for Proper Housing: Poultry and Rabbits, Division of Animal Welfare, University of Bern, Burgerweg 22, CH-3052 Zollikofen, Switzerland
Simple Summary
Precision livestock farming (PLF) is the use of technology to help farmers monitor and manage their animals and their farm. This technology can help to improve animal welfare by enabling farmers to act as soon as any problem arises. However, the technology can also be used to increase production efficiency on the farm, which could be prioritised over the animals’ welfare. The aim of this study was to give an overview of PLF technology development in poultry farming, and to investigate whether improving welfare has been the main goal of PLF development. The results suggest that PLF development in poultry farming so far has focussed on improving animal health and welfare, more so than increasing production. However, despite the interest in PLF research for poultry farming across the world (especially in the USA, China and Belgium), most of the technology is still being developed (prototypes); only a few are available for farmers to buy and use. This means that future work should focus on making these technologies commercially available to farmers, so that systems developed to improve welfare can be used to improve the welfare of farmed birds in the real world.
Precision livestock farming (PLF) systems have the potential to improve animal welfare through providing a continuous picture of welfare states in real time and enabling fast interventions that benefit the current flock. However, it remains unclear whether the goal of PLF development has been to improve welfare or increase production efficiency. The aims of this systematic literature review are to provide an overview of the current state of PLF in poultry farming and investigate whether the focus of PLF research has been to improve bird welfare. The study characteristics extracted from 264 peer-reviewed publications and conference proceedings suggest that poultry PLF has received increasing attention on a global scale, but is yet to become a widespread commercial reality. PLF development has most commonly focussed on broiler farming, followed by laying hens, and mainly involves the use of sensors (environmental and wearable) and cameras. More publications had animal health and welfare than production as either one of or the only goal, suggesting that PLF development so far has focussed on improving animal health and welfare. Future work should prioritise improving the rate of commercialisation of PLF systems, so that their potential to improve bird welfare might be realised.
1. Introduction
The term ’Precision Livestock Farming’ (PLF) was coined at the start of the 21st century, with the first conference on PLF held in 2003 [ 1 ]. Since its inception, many different definitions of the term have been generated and used in the literature. Based on the main shared aspects of the various definitions, the authors generated the following definition: PLF is the use of technology to automatically monitor livestock, their products and the farming environment in real time, in order to aid farm management, through supplying the farmer with relevant information on which to base management decisions, or by activating automated control systems.
The ’precision’ in PLF can be considered to refer to control of processes. Through more precise control over production processes, PLF can help farmers to improve their productivity and profitability. Banhazi and Black [ 2 ] argue that a major benefit of adopting a PLF system is to ensure that “every process within a livestock enterprise, which can have a large positive or large negative effect on productivity and profitability, is always controlled and optimised within narrow limits.”
As well as the potential to increase production, PLF technologies have the potential to improve animal welfare. PLF allows for non-intrusive assessment of welfare, where information can be collected without the stress of disturbing or handling animals [ 3 ]. Continuous monitoring can also provide a more complete picture of the overall welfare state of animals, rather than a snapshot in time as offered by traditional human assessment [ 4 ]. Alerting farmers to problems as they arise in real time allows for fast and targeted interventions which will benefit the current flock, compared to traditional welfare assessments that occur at the end of the production cycle [ 4 ]. PLF technology may offer more objective measures of welfare than traditional assessment methods carried out by human observers. Banhazi and colleagues [ 5 ] argue that “PLF can greatly contribute to an objective discussion on animal welfare by providing real data to the otherwise very subjective (and sometimes emotional) discussion process.” Finally, Werkheiser [ 6 ] puts forward that PLF allows “modern, large-scale farms to replicate and even to improve on the benefits of caring farmers who know their animals, transferred to a much larger scale. This could be done via closer monitoring than farmers can provide to even a few animals, as well as integration of the data via decision algorithms that improve on the guesswork of traditional stockpersons.” There is, however, a concern amongst animal welfare advocates that PLF systems, in aiding the management of intensive farming systems, may entrench the use of such systems that have limited potential for achieving good welfare outcomes, and that PLF will be used as a substitute for addressing the root causes of welfare issues [ 7 ]. On the other hand, PLF technologies can serve to highlight the welfare issues of poor systems and inform evidence-based strategies for their improvement. PLF can only be part of a solution to improve welfare, alongside other interventions to address the root causes of issues, for example in broiler farming using slower growing strains, reducing stocking density and increasing the dark period length, which have been identified as factors contributing most to broiler welfare [ 8 ].
While the potential of PLF to improve animal welfare has been discussed, what remains unclear is whether improving welfare has been the goal of PLF development in poultry, or if the focus has been on increasing production efficiency. These two factors are not mutually exclusive; improvements in welfare can be linked to improvements in production, for example by reducing mortality [ 9 ]; therefore, PLF systems can aim to improve both animal welfare and production. What is important from an animal welfare perspective is that PLF is not intended to increase production at the expense of animal health and well-being.
Poultry farming, and in particular broiler farming, is an important area in which to focus efforts on improving welfare, because of the sheer number of animals involved and the potential for improvements in their welfare. Modern broilers suffer from problems such as sudden death syndrome, ascites, lameness and contact dermatitis as a result of their fast growth rate [ 10 , 11 ], which has increased through breeding programmes by 400% since the 1960s [ 12 ]. Broilers are the world’s most numerous bird, with a standing population of 22.7 billion, an order of magnitude greater than the standing stocks of any other farmed species [ 13 ]. The highest farm animal numbers are found in poultry operations, with up to tens of thousands of individuals in one barn. Each individual animal is worth relatively little and the turnover of flocks is very fast, with modern broiler strains in conventional intensive production systems reaching their target weight in just 5–6 weeks or less [ 14 ]. This means that concern for the welfare of an individual bird may be low. The profit margin for poultry farmers is small, creating further conflict between production and bird welfare.
While the number of chickens farmed for meat is already huge, meat consumption is currently predicted to increase, not only because of a growing human population, but also because of increasing incomes and urbanisation [ 15 ]. This means that poultry farming is set to increase in low income countries where animal welfare may not yet be seen as a priority. Poultry meat consumption specifically has grown in comparison to other meat types. In the OECD (Organisation for Economic Co-operation and Development, Paris, France), an organisation of 36 countries, beef pork and sheep meat consumption levels have varied very little between 1990 and 2017. Poultry meat consumption on the other hand has increased by 70.5% [ 16 ].
Therefore, poultry welfare is an especially important area to focus efforts on improving welfare, and PLF is one potential tool to achieve this (in addition to improving farming practices in other ways), through enabling continuous monitoring and fast interventions benefiting individuals in their lifetime. The question remains, is PLF currently fulfilling this potential to improve bird welfare, or has increasing production efficiency been the driver behind PLF development in the poultry sector?
The aim of this systematic review is two-fold. Firstly, we aim to present an updated overview of the research conducted on PLF technologies in the poultry sector, to assess how much PLF is currently being used in poultry farming. Secondly, we ask whether the development of PLF for poultry has so far focussed on improving bird welfare, or if increasing production efficiency been the main driving factor.
2. Materials and Methods
2.1. definition of precision livestock farming.
From the different definitions of PLF that have been used in the literature, the working definition of PLF that was developed and used in this review is: the use of technology to automatically monitor livestock, their products and the farming environment in real time, in order to aid farm management, through supplying the farmer with relevant information on which to base management decisions, or by activating automated control systems.
Therefore, to be considered as a PLF system according to the authors’ working definition, the system had to be automated at least in part, if not fully. For the purpose of this study, livestock and their products refer to living birds and eggs only; monitoring of carcasses, meat or manure were not included. Technology used to monitor birds prior to hatching, e.g., sexing of embryos was also excluded. Publications concerning the development of models or algorithms for use in PLF systems, such as computational fluid dynamics (CFD), machine learning, etc., were not included; although these are aspects of PLF systems, they do not fall under the authors’ working definition of PLF. In addition, publications concerning traceability, for example vehicle identification during the transport of poultry or poultry products, or data management systems for the traceability of information between each link of a poultry production chain, were not included. Although these can be considered as applications of PLF technology, they do not fall under the working definition of PLF used in this review.
2.2. Literature Search
A systematic search, following PRISMA guidelines [ 17 ], for published peer-reviewed literature and conference proceedings on the use of PLF technologies in poultry farming was carried out between 1 February and 3 April 2019. Searches were performed in the following databases: CAB Direct, PubMed, Scopus and Web of Science.
2.3. Selection of Search Terms
We selected 18 terms relating to PLF, and 11 poultry-related terms. Quotation marks were used to narrow the search to the exact phrase when the term contained common words.
Although review papers were excluded from the results (see eligibility screening below), reviews on the use of technology in poultry farming [ 18 , 19 , 20 , 21 ] were screened for relevant references. If there were references that had not been returned by the search terms used up to this point, search terms were generated from these papers. This process led to 14 additional terms related to PLF, and searches were conducted using these terms ( Table 1 ).
Search terms used in a systematic review of the use of precision livestock farming technologies in poultry farming.
1 PLF = precision livestock farming. 2 RFID = radio frequency identification. 3 UWB = ultra-wideband. 4 A “?” was used to replace a single character to account for UK and US spelling variations, except in PubMed where such spelling variations are automatically included.
2.4. Search Strategy
The search fields were “topic” (covering title, abstract, author keywords and keywords plus) in Web of Science, “article title, abstract, keywords” in Scopus, and “all fields” in PubMed and CAB Direct.
The poultry terms were combined with parentheses and the Boolean operator OR, and each precision farming term was combined in turn with the poultry terms using AND; for example: precision livestock farming AND (poultry OR chicken OR chick OR laying hen OR broiler OR pullet OR duck OR goose OR turkey OR hatchery OR slaughter).
For several of the precision farming terms, PubMed returned over 100 results; in these instances, the filter “other animal” was used to filter out irrelevant human-related studies. For several search terms, Web of Science also returned more than 100 results; in these instances, relevant categories were chosen from the list of Web of Science to filter for relevant results.
2.5. Eligibility Screening
Three inclusion/exclusion criteria were used to screen each search result. The publication had to describe novel research (no reviews) on PLF technologies used in poultry according to the above definition, in English, German, or French.
One author (ER) carried out the literature search and screening process. After the screening process, 20 papers were randomly selected using a random number generator [ 22 ], and agreement was checked between all authors on whether these met the inclusion criteria. If there were any papers the first author was unsure about during the screening process, they were discussed with the other authors until a decision was reached.
2.6. Study Characteristics
Results were categorised according to the headings in Table 2 , using information from the abstracts, and from the full text where available if the abstract was insufficient. Apart from the categories ’prototype or commercially available system’ and ’year’, papers could be classed in more than one category, meaning that percentages did not sum to 100%. Income groupings of countries (a development indicator) were based on the latest World Bank data [ 23 ]. Because of the large number of countries of author affiliation, any countries with under five publications were categorised together under ’other’; a full list of countries is given in Appendix A . PLF systems were classed as commercially available if they were available for purchase as a complete system or used commercially available sensors. The heading ’goal’ describes the study’s goal(s): whether this was to improve animal health and welfare, human health, production or sustainability. These categories are not mutually exclusive and studies could have more than one goal; therefore, publications could be classed in more than one category. Where the goal of the study was not stated explicitly, it was inferred from the keywords and the information in the abstract or full text. Where there was insufficient information to make this inference possible, the category was left blank, leading to a small amount of missing data. The category sensor included both sensors for environmental monitoring (e.g., temperature, humidity), as well as wearable sensors [ 24 ]. Wearable sensors included Radio-Frequency Identification (RFID) systems, which can be used as movement sensors [ 20 ].
Categories for extracting study characteristics from results of a systematic review of the use of precision livestock farming technologies in poultry farming.
a The category chicken was only used when the paper did not specify broiler or laying hen; b The category poultry was only used when the available text did not specify the species further.
For publications which had animal health and welfare as the only goal of the study, the type of parameters measured by the PLF system (i.e., used as welfare measures) were extracted.
3.1. Study Selection
A total of 6265 results were returned by the search strategy and screened for eligibility. This resulted in 264 papers that were included in the review, 203 of which the authors had access to the full text (see Figure A1 for PRISMA flow diagram).
3.2. Study Characteristics
Figure 1 and Table 3 , Table 4 , Table 5 , Table 6 , Table 7 and Table 8 describe the study characteristics extracted from the search results. The first papers on technology which can be classed as PLF in poultry farming, according to the authors’ definition, were published in 1992 ( Figure 1 ). The number of publications remained low until a marked increase in 2008, reaching a peak in 2017. Authors of the publications were affiliated with institutions from a total of 40 different countries ( Table A1 ). The biggest proportion of studies was contributed to by at least one author from the USA, followed by Belgium and China ( Table 3 ). The majority of publications were authored by at least one author from a high-income country; there were no publications with authors from a low-income country ( Table 4 ). Half of the studies described sensor technology, and over a third described the use of cameras; microphones represented a smaller proportion ( Table 5 ). The vast majority of studies described prototype systems (96.21%, n = 254); only 10 papers described commercially available systems (3.79%). The largest proportion of papers described PLF technology in broiler farming, followed by laying hens ( Table 6 ). The largest proportion of publications had animal health and welfare as one of the goals of the study, followed by production, which was one of the goals of over half the publications ( Table 7 ). A total of 105 papers (39.77% of all publications) had animal health and welfare as the only goal of the study, compared to 72 papers (27.27%) with production as the only goal. For the PLF systems with animal health and welfare as the only primary goal, most of the measurements used to assess animal health and welfare were behaviour-based; the largest proportion of publications used locomotory behaviour as a measure of welfare, followed by vocalisations or bird sounds ( Table 8 ). The category ’acceptability’ in Table 8 does not describe parameters measured directly by a PLF system, but instead concerns the acceptability to farmers of the PLF technology for studies where the goal was to improve animal health and welfare. These studies included: the acceptance of PLF technology by farmers in the EU-PLF project [ 25 ], the development of power-saving sensors [ 26 ], investigating birds’ reactions to the use of robots [ 27 ], the effect of wearable sensors on bird behaviour [ 28 , 29 , 30 ] physiology [ 29 , 30 ] and health [ 30 ], the effect of precision feeding systems on bird behaviour [ 31 , 32 ], and methods to extract chicken images from background noise in image analysis [ 33 ].

Number (data label) and percentage of publications by year. Publications in 2019 are up until 3 April.
Number and percentage of publications by country of author affiliation. Publications could have authors from more than one country.
Number and percentage of publications by country income grouping. Publications could have authors from more than one income grouping.
Number and percentage of publications by technology used. Publications could use more than one type of technology.
Number and percentage of publications by bird type. Publications could have more than one bird type.
Number and percentage of publications according to the goal(s) of the study. Publications could have more than one goal.
Number and percentage of publications with animal health and welfare as the only goal (n = 105) according to the parameter(s) that were measured by the precision livestock farming system.
4. Discussion
The first aim of this review was to provide an overview of the current state of PLF research in poultry farming, to assess the progress of this field since its inception. Previous reviews on PLF technologies in poultry farming [ 18 , 19 , 20 , 21 ] have given illustrative examples of technological developments in this field, but have not provided a comprehensive overview of all research in this area. By conducting a systematic literature search, the current review provides a more complete picture of the state of PLF development in the poultry sector thus far. Technology is developing rapidly in this field; this review provides an update on new PLF systems published subsequent to the previous reviews. The second aim was to investigate whether the focus of PLF research has been to improve bird welfare, because whilst the potential for PLF to increase welfare through improved monitoring has been discussed, there remains a risk that PLF will be utilised to prioritise production efficiency, which could come at the expense bird welfare.
Based on the results of the systematic literature search, research into PLF technology for poultry farming did not take off until the late 2000s. Since then, research output in this field has tended to increase up until 2017, waning slightly in 2018. This suggests a growing interest and investment in poultry PLF research. Evidencing the continued interest and investment in PLF technologies in the poultry sector, the Foundation for Food and Agriculture Research (FFAR) and McDonald’s Corporation have recently launched ’SMART Broiler’, a research grant of $4 million to drive the development and commercialisation of automated monitoring tools to assess broiler welfare [ 34 ].
Poultry PLF research has not been confined to a small number of countries; contributors to the publications were affiliated with institutions from 40 different countries ( Table A1 ). There were no authors affiliated with low-income countries, and the majority of publications were authored by at least one author from a high-income country. However, over a third of publications (37.88%) had at least one author affiliated with an upper-middle-income country, and 4.92% a lower-middle-income country. This suggests that it is not only the wealthiest countries that are interested and investing in PLF for poultry farming.
The main countries producing poultry PLF research were the USA (18.94% of publications had at least one author from the USA), followed by Belgium (18.56%), and China (17.05%). The USA is the world’s largest producer of poultry according to the most recent available data (20 million tonnes produced in 2014), followed by China (18 million tonnes produced in 2014) [ 35 ]. Therefore, it is logical that these countries are interested in the development of technology that could improve poultry farming. Belgium is the country where early pioneers of PLF were based [ 5 ] and continue to be active, potentially explaining why Belgium is one of the major contributors to poultry PLF research. It should be noted that only papers published in English, French or German were included in the review, as these were the languages that could be understood by at least one of the authors. Excluded papers in different languages, such as Chinese, could have altered the results of this review.
More PLF technology has been developed for broilers (43.18% of publications) than any other bird type, a finding that is in line with the fact that broilers are the most commonly farmed type of poultry [ 13 ]. Broilers may also be the bird type of interest in PLF development as the scope for broiler welfare improvement is great (as discussed earlier in this review). As laying hens are also a commercially important type of poultry, it follows that laying hens were the second most common type of bird for which PLF technology has been developed (25.38% of publications). Egg consumption is high in many countries around the world: in 2013, egg consumption per capita was 18.65 kg in China (one of the highest levels in the world), 14.58 kg in the USA, and 12.59 kg in Belgium [ 35 ].
The majority of PLF in poultry involved the use of sensors (51.89%), although cameras were used in a large proportion of the studies (42.42%). The use of microphones appears to be less popular in poultry PLF (14.02%). That over half the publications involved the use of sensors may be explained, at least in part, because this was the broadest category of technology. The category sensors included not only sensors to monitor environmental parameters such as temperature and humidity, but also ’wearable sensors’ [ 24 ]. This included RFID; although RFID is used for individual identification of animals, this technology can also be used as movement sensors [ 20 ], and used track behaviour, including locomotory behaviour. For example, the time difference between an RFID-tagged bird passing two RFID readers and the distance between these readers enables movement speed to be calculated, and behaviours such as time spent feeding and resting can also be monitored (e.g., [ 36 ]). As another example, RFID has been used to sense when a hen enters or exits a nest box which, along with a pressure sensor to detect the presence of an egg, has led to the design of a smart nest box to monitor the laying performance and behaviour of hens [ 37 ]. Previous reviews have noted a growing interest in wearable sensors for animal health management [ 24 ]. Environmental sensors are easier to interpret than cameras and microphones: the output of an environmental sensor such as a thermometer delivers the parameter of interest directly (a temperature reading), whereas the output of a camera or microphone must first be analysed and interpreted before the parameter of interest (for example locomotory behaviour) is produced. This may explain the apparent popularity of sensors (at least environmental sensors) in PLF systems.
Almost all papers (96.21%) described prototype systems, suggesting that there are very few PLF systems for poultry farms that are currently commercially available. The commercially available technologies were: the eYeNamic™ camera system [ 38 , 39 ], and environmental sensors to measure temperature [ 40 , 41 , 42 , 43 ], ambient dust [ 44 ], relative humidity [ 41 , 42 , 43 ], vibration [ 45 ], ammonia concentration [ 46 ], carbon dioxide concentrations [ 41 , 46 ], and a thickness and crack sensor for eggs [ 47 ]. The eYeNamic™ camera system is produced commercially by Fancom BV and collects and processes images in order to monitor chickens’ distribution and activity, which “can be conceived as valuable indicators of animal welfare” [ 38 ]. It should be noted that some of the prototype systems in this review used the commercially available eYeNamic™ cameras, but as the systems themselves were prototypes the publications were categorised as such (e.g., [ 48 ]). Conversely, publications that involved the use of commercial sensors described investigations of where best to place these sensors, and so could be categorised as commercially available systems (e.g., [ 41 ]). It should also be noted that some of the prototype systems could have become commercially available since the time the study was published. Nonetheless, this result suggests that the application of PLF technology in poultry farming is still a future prospect rather than a current reality.
The evident interest in the use of PLF for poultry farming raises the question: why are there not more commercial PLF systems in place on poultry farms? Wathes [ 49 ] suggests that PLF technologies remain uncommon because research does not involve manufacturing companies from the start. Such companies could help to develop specifications for commercial success. In addition, few systems undergo trials under commercial conditions, and these are vital in order to show technical success to farmers and other stakeholders [ 49 ]. Incomplete development of technology, especially when equipment shows poor robustness and reliability, will lead to rejection by early adopters [ 3 ]. It is also not clear whether there is a demand for new monitoring technologies from farmers [ 49 ], and farmers may lack confidence in technology-based production systems [ 3 ]. Furthermore, the payback period for farmers investing capital in PLF systems is uncertain [ 3 ].
The obstacles discussed above apply to PLF technologies in general, for all farmed species. However, it appears that PLF in the poultry sector lags behind that of other species, for example dairy cattle. Commercially available PLF technology in the dairy sector includes devices to identify, track and milk individual animals, feed animals automatically, and obtain diagnostic data about a range of health and performance related criteria [ 50 ]. The dairy sector has had a longer history of PLF development than the poultry sector: the first widespread application of PLF was the individual electronic milk meter for cows, which became commercially available in the 1970s [ 51 ], followed by automated oestrus detection devices in the 1980s [ 52 ], both decades before the term PLF was coined.
Caja and colleagues [ 50 ] suggest that “dairy farmers will pay for and use technologies that provide what is, to them, a straightforward answer to a straightforward question (should I inseminate cow x?) when they believe it will have positive economic impact.” This may highlight another reason why PLF technologies are more commercially established in the dairy sector: the benefits of using PLF are much clearer, whereas the advantages of using PLF in poultry farming have not yet been sufficiently demonstrated. Although PLF has the potential to improve bird health and welfare, its actual benefits, over and above those that could be obtained by simpler methods such as water use or greater attention to temperature and humidity, have not yet been demonstrated in practice.
More publications had animal health and welfare as one of the goals (63.64% of publications) than production (51.14%) (publications could have more than one goal). Likewise, for the publications with only one goal, more publications had animal health and welfare as the only goal (39.77%), compared to production (27.27%). This suggests that the majority of PLF development in poultry farming thus far has focussed on improving welfare. However, a substantial amount of research (almost a third) is focussing on production alone; in these cases, there could be a danger that production is prioritised over health and welfare. It should be noted that, where the goal of the study was not explicitly stated, it was inferred by the author (ER), which introduces a degree of subjectivity into the categorisation of study goal; this should be taken into account when interpreting these results.
In the absence (to the authors’ knowledge) of equivalent reviews, it is hard to compare the goals of PLF development in other livestock sectors. Taking the dairy sector again as an example, there are at least 11 commercially available accelerometers for oestrous detection, but only two commercially available sensors for lameness detection [ 50 ]; this could suggest that improving production processes has received more emphasis than welfare monitoring. However, there has been work focused on improving dairy cattle welfare through PLF technologies; for example, DairyCare was a 4-year (2014 to 2018) EU project with an objective of improving dairy animal well-being through technological advancement, including the development of biomarker-based, activity-based and systems-level welfare monitoring technologies [ 50 ].
Of the papers with animal health and welfare as the sole primary goal, most of the measurements used to monitor the birds were locomotory behaviour-based (43.81%). Locomotory behaviour included activity, distribution and occupation patterns (e.g., [ 48 , 53 ]), movement (e.g., [ 54 ]) and movement-related variables such as speed, step frequency, step length and the lateral body oscillation [ 55 ], location within the environment (e.g., [ 30 ]), optical flow (e.g., [ 56 ]), ranging behaviour (e.g., [ 57 ]), and clustering behaviour ([ 58 ]). The second largest proportion of publications (20.95%) used vocalisations [ 59 ] or bird sounds. Bird sounds were pecking sounds (e.g., [ 60 ]), or in one publication, rale sounds [ 61 ].
Other behaviour measures used were perching behaviour (e.g., [ 62 ]) and resting behaviour which included lying events [ 63 , 64 ] and latency to lie down [ 63 ]. Body posture was used to develop automated techniques of recognising and quantifying bird behaviours [ 65 ] such as wing spreading, scratching and preening [ 66 ]. The presence of birds was used by Zaninelli and colleagues [ 67 , 68 ] to detect whether hens in a free-range system were present in the housing area, with the aim of removing all hens to the outside area so the housing can be treated to reduce atmospheric ammonia and bacterial load. Monitoring of the presence of hens was also used to detect multiple occupations of a nest area to improve monitoring of laying behaviour [ 69 ]. Li and colleagues [ 70 , 71 ] used presence of hens at specific areas (feeding trough and nest boxes) for automated monitoring and quantification of feeding, drinking and nesting behaviour. Automated monitoring of hen presence was also used in an environmental preference test [ 72 ].
Welfare measures that focus on assessing the behaviour of animals are known as animal-based measures, or ’outcome’ measures [ 73 ]. These measure the animals directly and inform us of the effect (outcome) of an animal’s environment on its welfare state [ 74 ]. Outcome measures are considered to provide a more objective, accurate and direct picture of animal welfare than ’input’ measures, which describe what must be provided to animals in terms of housing, space, feed and water, veterinary care and management practices [ 74 ]. The use of outcome measures is considered best practice in welfare assessment schemes [ 73 ]. Furthermore, a ’continuous improvement’ approach is also considered best practice in welfare assessment schemes; this requires “regular monitoring of pre-defined criteria” ensuring that “preventive and corrective action is taken to maximise levels of these criteria” [ 73 ]. Therefore, most of the PLF systems proposed with the sole goal of improving welfare could help achieve best practice welfare assessment by continuously monitoring outcome measures in real-time, in order for preventive and corrective action to be taken.
However, to use behaviour as a welfare measure, the behaviours must be validated to show whether and how they are linked to an animal’s welfare status. For example, Fernandez and colleagues [ 48 ] compared data on locomotory behaviour to measures collected via a validated assessment protocol (Welfare Quality ® ). They found statistically significant correlations between locomotory behaviour (activity and occupation patterns) and welfare scores (for footpad lesions and hock burn), indicating that activity and occupation patterns are valid indicators of broiler welfare status.
This highlights a further potential impediment to PLF development. PLF technology can be used to monitor many parameters, such as behaviour, but whether and how the measurements taken by a PLF system are linked to a parameter, i.e., the internal validity of the measure (for example, how optical flow patterns are linked to broiler behaviour [ 56 ]), is not always clear. Furthermore, whether and how the monitored parameters are linked to welfare, i.e., the external validity of the measure (for example, what different patterns of optical flow tell us about the birds’ welfare status) must be established. Finally, the levels of this parameter at which interventions are necessary to improve welfare, and what interventions are appropriate, must also be determined. Therefore, while there is the potential for PLF to lead to improved standards of bird welfare through continuous, real-time monitoring, important steps before such systems can become successfully commercialised are internal and external validation by controlled trials to show that the system does in practice reliably monitor the parameter of interest, and that monitoring this parameter and appropriate intervening does lead to better welfare.
Most of the physiological measures made by PLF systems were body temperature of birds measured directly (e.g., [ 75 ]) as well as via thermal imaging (e.g., [ 76 ]). Environmental measures included temperature and humidity (e.g., [ 77 ]), CO 2 concentration [ 78 ], moisture content of litter [ 79 ] and citric acid concentration in drinking solutions [ 80 ]; these can be considered as input measures of welfare.
The results of this review suggest that future work on PLF technologies for poultry farming should focus on overcoming barriers to commercialisation and on expanding the range of welfare measures, particularly those involving behaviour, that can be used as part of PLF. There is a need for more large-scale commercial trials that involve manufacturing companies, farmers and other stakeholders from the outset, in order to demonstrate the value of PLF systems in raising standards of welfare in practice. Ensuring that PLF technologies are transferred from the lab to the farm was the overall objective of the EU-PLF project, which ran from 2012 to 2016 [ 81 ]. The main output of the project was the EU-PLF Blueprint: a manual with pragmatic guidance on how to implement PLF systems at the farm level [ 82 ]. The publication of the EU-PLF Blueprint may increase the success of commercialising poultry PLF technologies. Future work could incorporate the use of the Blueprint; instructions on how to access and use the manual are provided in Guarino et al. [ 82 ].
In addition, as well as using PLF technology to assess animal welfare in order to prevent and alleviate poor welfare states, future research should focus on using PLF to promote positive welfare and provide food animals with “a good life”, or at least “a life worth living” [ 83 ]. For example, Daniel Berckmans and Thomas Norton, two pioneers of PLF, explain that “the possibilities to use PLF technology to create an interesting, adventurous environment for curious animals is not so much used yet, so there is another opportunity here to realise playful events and environments for our animals to give them a life that is worth living” [ 84 ].
5. Conclusions
The development of PLF systems for poultry farming, especially broilers and laying hens, has received increasing attention on a global scale, notably in the USA, China and Belgium, and the largest proportion of studies have focussed on improving animal health and welfare. Despite the increasing research output, PLF is yet to become a widespread commercial reality in the poultry sector. Future work should focus on the commercialisation of PLF systems in the poultry sector, as well as their potential for promoting positive welfare.

Modified PRISMA flow diagram illustrating the systematic review search strategy and study selection.
A full list of all countries of author affiliation, and number and percentage of publications with authors affiliated with these countries. Publications could have authors from more than one country.
Author Contributions
Conceptualisation: M.S.D. and S.G.G.-H.; methodology: E.R., M.S.D. and S.G.G.-H.; literature search: E.R.; data extraction and analysis: E.R.; writing—original draft preparation: E.R.; writing—review and editing: E.R., M.S.D. and S.G.G.-H.; writing—final draft preparation: E.R.; funding acquisition: M.S.D.
This research was funded by ANIHWA ERA-Net ID114 BBSRC grant no. BB/N023803/1.
Conflicts of Interest
The authors declare no conflict of interest. The funder had no role in the design of the study; in the collection, analyses, or interpretation of data; in the writing of the manuscript, or in the decision to publish the results.
Design of an Efficient IoT System for Poultry Farm Management
- Conference paper
- First Online: 12 September 2022
- Cite this conference paper
- G. Rajakumar 40 ,
- K. Lakshmi Narayanan 40 ,
- R. Santhana Krishnan 41 ,
- Y. Harold Robinson 42 ,
- M. Subramanian 41 &
- M. Asirvatham 41
Part of the book series: Lecture Notes in Electrical Engineering ((LNEE,volume 903))
498 Accesses
1 Citations
In the past, chicken meat was very negatively viewed by people. Now, however, very high nutritious chicken meat is consumed by people from all over the world. Environmental parameters such as temperature, humidity, and nutrition are very important in chicken growth. So, society should grow and monitor this grass or this grass at good conditions. The automatic monitoring system is created to overcome several issues that may arise on the chicken farm. Changes in the environment affect the egg production and feed consumption of the bird. Thus, the PEST system's major function is to supervise and manage the environmental conditions such as heat, humidity, oxygen, methane, and water that exist on the poultry farm. If any changes have occurred in the poultry farms’ environmental condition, the system sends the relevant information to a mobile device like phones through telephone lines which reduces the human effort and time required. The interactive device has been created using the Arduino Uno microcontroller and various sensors, such as temperature, humidity, gas and water level sensors. As this system, the design takes on a very efficient way to farm poultry that will result in an increase in production and profit. It is high time to start thinking about solar technologies that can generate electricity. Although this technology is made for household, this has been successfully used in commercial farms for the energy saving of batteries and the opportunity to sell the power back to the grid.
This is a preview of subscription content, log in via an institution to check access.
Access this chapter
- Available as PDF
- Read on any device
- Instant download
- Own it forever
- Available as EPUB and PDF
- Compact, lightweight edition
- Dispatched in 3 to 5 business days
- Free shipping worldwide - see info
- Durable hardcover edition
Tax calculation will be finalised at checkout
Purchases are for personal use only
Institutional subscriptions
Asfaw YT, Ameni G, Medhin G, Gumi B, Wieland B (2021) Poultry health services in Ethiopia: availability of diagnostic, clinical and vaccination Services. Poultry Sci 100:10102
Article Google Scholar
Ananth Kumar T, John A, Ramesh Kumar C (2020) IoT technology and applications in Internet of Things, pp 43–62
Google Scholar
Rajakumar G, Kumar TA, Samuel TA, Kumaran EM (2018) IoT based milk monitoring system for detection of milk adulteration. Int J Pure Appl Math 118(9):21–32
Zeng Z, Zeng F, Han X, Elkhouchlaa H, Yu Q, Lü E (2021) Real-time monitoring of environmental parameters in a commercial gestating sow house using a ZigBee-based wireless sensor network. Appl Sci 11(3):972
Imtem N, Sirisamphanwong C, Ketjoy N (2021) Development and performance testing of the automated building energy management system with IoT (ABEMS-IoT) case study: big-scale automobile factory. In: IT convergence and security. Springer, Singapore, pp 97–107
Tadesse T, Nichols MA, Hewett EW, Fisher KJ (2001) Relative humidity around the fruit influences the mineral composition and incidence of blossom-end rot in weet pepper fruit. J Hortic Sci Biotechnol 76(1):9–16
Lu J, Okada H, Itoh T, Maeda R, Harada T (2013) Towards the world smallest wireless sensor nodes with low power consumption for ‘Green’sensor networks. In: Sensors. IEEE, pp 1–4
Dong F, Zhang N (2009) Wireless sensor networks applied on environmental monitoring in fowl farm. In: International conference on computer and computing technologies in agriculture. Springer, Heidelberg, pp 479–486
Ayaz M, Ammad-Uddin M, Sharif Z, Mansour A, Aggoune EHM (2019) Internet-of-Things (IoT)-based smart agriculture: toward making the fields talk. IEEE Access 7:129551–129583
Jindarat S, Wuttidittachotti P (2013) Smart farm monitoring using raspberry pi and Arduino. In: International conference on computer, communication, and control technology. https://doi.org/10.1109/I4CT.2015.7219582
Wathes CM, Kristensen HH, Aerts JM, Berckmans D (2008) Is precision livestock farming an engineer’s daydream or nightmare, an animal’s friend or foe, and a farmer’s panacea or pitfall? Comput Electron Agric 64(1):2–10
Liu W, Wang X, Song Y, Cao R, Wang L, Yan Z, Shan G (2020) Self-powered forest fire alarm system based on impedance matching effect between triboelectric nanogenerator and thermosensitive sensor. Nano Energy 73:104843
Behr CJ, Kumar A, Hancke GP (2016) A smart helmet for air quality and hazardous event detection for the mining industry. In: 2016 IEEE International Conference on Industrial Technology (ICIT). IEEE, pp 2026–2031
Jiang JA, Tseng CL, Lu FM, Yang EC, Wu ZS, Chen CP, Liao CS (2008) A GSM-based remote wireless automatic monitoring system for field information: a case study for ecological monitoring of the oriental fruit fly, Bactrocera dorsalis (Hendel). Comput Electron Agric 62(2):243–259
Varghese VT, Sasidhar K, Rekha P (2015) A status quo of WSN systems for agriculture. In: 2015 international conference on advances in computing, communications and informatics (ICACCI). IEEE, pp 1775–1781
Download references
Author information
Authors and affiliations.
Department of ECE, Francis Xavier Engineering College, Tirunelveli, India
G. Rajakumar & K. Lakshmi Narayanan
Department of ECE, SCAD College of Engineering and Technology, Tirunelveli, India
R. Santhana Krishnan, M. Subramanian & M. Asirvatham
School of Information Technology and Engineering, Vellore Institute of Technology, Vellore, India
Y. Harold Robinson
You can also search for this author in PubMed Google Scholar
Corresponding author
Correspondence to G. Rajakumar .
Editor information
Editors and affiliations.
Electrical Engineering, Indian Institute of Technology Patna, Patna, Bihar, India
Sudhan Majhi
Telecommunication Engineering, University of Jaén, Jaén, Spain
Rocío Pérez de Prado
Electronics and Communication, SJB Institute of Technology, Bengaluru, Karnataka, India
Chandrappa Dasanapura Nanjundaiah
Rights and permissions
Reprints and permissions
Copyright information
© 2022 The Author(s), under exclusive license to Springer Nature Singapore Pte Ltd.
About this paper
Cite this paper.
Rajakumar, G., Narayanan, K.L., Krishnan, R.S., Robinson, Y.H., Subramanian, M., Asirvatham, M. (2022). Design of an Efficient IoT System for Poultry Farm Management. In: Majhi, S., Prado, R.P.d., Dasanapura Nanjundaiah, C. (eds) Distributed Computing and Optimization Techniques. Lecture Notes in Electrical Engineering, vol 903. Springer, Singapore. https://doi.org/10.1007/978-981-19-2281-7_31
Download citation
DOI : https://doi.org/10.1007/978-981-19-2281-7_31
Published : 12 September 2022
Publisher Name : Springer, Singapore
Print ISBN : 978-981-19-2280-0
Online ISBN : 978-981-19-2281-7
eBook Packages : Computer Science Computer Science (R0)
Share this paper
Anyone you share the following link with will be able to read this content:
Sorry, a shareable link is not currently available for this article.
Provided by the Springer Nature SharedIt content-sharing initiative
- Publish with us
Policies and ethics
- Find a journal
- Track your research

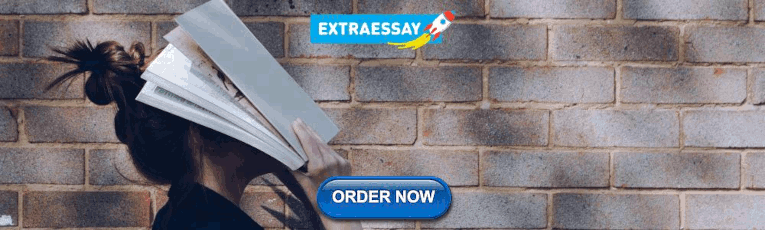
Poultry Farm Management Information System (A Case Study of Biyinzika Poultry International Limited)
Publication Date: 23/10/2021
DOI: 10.52589/BJCNIT-I1QIMWRR
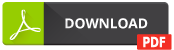
Author(s): Edison Kagona, Fahadi Bakaki, Makanto Mary Ngubteino, Djenny Bakwanamaha, Mayaza Issa Sarah.
Volume/issue: volume 4 , issue 2 (2021).
This report discusses a Poultry Farm Management Information System of Biyinzika Poultry Farm International Limited. The software is sectioned into the sales management section, Purchase management section, Product management section. This system was designed to overcome the problems identified with the current Poultry Farm Management information system of Biyinzika Poultry Farm International Limited. The Problems with the current system include: lack of information sharing in real-time, manual report generation on sales, purchases and the products, slow in information delivery especially in the determination of stock levels after transaction processes, a lot of time consumption, increasing the company costs, and lack of effective data recovery in case of any disaster such as fire outbreak, flood, damages among others. Literature relating to the Poultry Information Management Systems were reviewed. The legacy system used in the company was also studied in more details. With this, more requirements for the Poultry Farm Management Information System were obtained and the system was designed and implemented. The interfaces for the new system were implemented using HTML, Bootstrap and Java Script. MYSQL was also used for implementing the system database while PHP was used to create interactivity with the database. After the implementation, the new system was then tested and validated. When developing the system, the focus was on making the whole process of Information management in the poultry farm faster, more convenient and efficient for the company. This system automates the current poultry farm management information system in the organization.
Poultry Farm Management, Information System, Sales Management, Purchase Management, Product Management
No. of Downloads: 5248

Poultry Farm Control and Management System Using Wireless Sensor Networks
Ieee account.
- Change Username/Password
- Update Address
Purchase Details
- Payment Options
- Order History
- View Purchased Documents
Profile Information
- Communications Preferences
- Profession and Education
- Technical Interests
- US & Canada: +1 800 678 4333
- Worldwide: +1 732 981 0060
- Contact & Support
- About IEEE Xplore
- Accessibility
- Terms of Use
- Nondiscrimination Policy
- Privacy & Opting Out of Cookies
A not-for-profit organization, IEEE is the world's largest technical professional organization dedicated to advancing technology for the benefit of humanity. © Copyright 2024 IEEE - All rights reserved. Use of this web site signifies your agreement to the terms and conditions.
Management information system adoption at the farm level: evidence from the literature
British Food Journal
ISSN : 0007-070X
Article publication date: 2 November 2020
Issue publication date: 5 February 2021
This paper reviews the academic contributions that have emerged to date on the broad definition of farm-level management information systems (MISs). The purpose is twofold: (1) to identify the theories used in the literature to study the adoption of digital technologies and (2) to identify the drivers of and barriers to the adoption of such technologies.
Design/methodology/approach
The literature review was based on a comprehensive review of contributions published in the 1998–2019 period. The search was both automated and manual, browsing through references of works previously found via high-quality digital libraries.
Diffusion of innovations (DOIs) is the most frequently used theoretical framework in the literature reviewed, though it is often combined with other innovation adoption theories. In addition, farms’ and farmers’ traits, together with technological features, play a key role in explaining the adoption of these technologies.
Research limitations/implications
So far, research has positioned the determinants of digital technology adoption mainly within the boundaries of the farm.
Practical implications
On the practical level, the extensive determinants’ review has potential to serve the aim of policymakers and technology industries, to clearly and thoroughly understand adoption dynamics and elaborate specific strategies to deal with them.
Originality/value
This study’s contribution to the existing body of knowledge on the farm-level adoption of digital technologies is twofold: (1) it combines smart farming and existing technologies within the same category of farm-level MIS and (2) it extends the analysis to studies which not only focus directly on adoption but also on software architecture design and development.
- Smart farming
- Farm management information systems
- Enterprise resource planning
- Diffusion of innovation
- Digital divide
Giua, C. , Materia, V.C. and Camanzi, L. (2021), "Management information system adoption at the farm level: evidence from the literature", British Food Journal , Vol. 123 No. 3, pp. 884-909. https://doi.org/10.1108/BFJ-05-2020-0420
Emerald Publishing Limited
Copyright © 2020, Carlo Giua, Valentina Cristiana Materia and Luca Camanzi
Published by Emerald Publishing Limited. This article is published under the Creative Commons Attribution (CC BY 4.0) licence. Anyone may reproduce, distribute, translate and create derivative works of this article (for both commercial and non-commercial purposes), subject to full attribution to the original publication and authors. The full terms of this licence may be seen at: http://creativecommons.org/licences/by/4.0/legalcode
1. Introduction
Agri-food systems are on the verge of a new revolution based on the use of digital innovations throughout the supply chain ( Lehmann et al. , 2012 ; Trendov et al. , 2019 ; Wolfert et al. , 2017 ). Scholars have applied different names to define this digitalisation, including “digital agriculture”, “agriculture 4.0” and “smart farming”. That notwithstanding, there is common agreement on the central role that data play in the agri-food supply chain’s virtualisation ( Verdouw et al. , 2016 ).
Despite the general agreement on the beneficial effects that this revolution will have for the agri-food system in terms of greater efficiency, effectiveness and sustainability ( Fabregas et al. , 2019 ; Lehmann et al. , 2012 ), the process of adopting innovative digital technologies requires resources and competences which not all the actors possess ( Poppe and Renwick, 2015 ). For instance, the literature shows that amongst internal resources, farm size and financial availability can be important barriers to overcome, especially for small and medium enterprises (SMEs) ( Bucci et al. , 2018 ; Lawson et al. , 2011 ). Amongst external resources, poor Internet connectivity, data transfer and privacy concerns are just some of the factors reported as obstacles to the adoption process ( Kernecker et al. , 2019 ; Pivoto et al. , 2019 ). In addition, not only resources but also specific competences need to be developed by firms – the so-called dynamic capabilities ( Teece et al. , 2008 ) – in order to achieve a suitable digital transformation of their business ( Bouwman et al. , 2018 ; Warner and Wäger, 2019 ; Zahra and George, 2002 ).
Disparities in the process of adopting digital technologies might further aggravate the unequal distribution of value that already exists in certain cases between small–medium (especially in upstream production stages) and large players (downstream production stages, especially distribution and retailers). This is particularly true for the European agri-food productive structure which predominantly comprises SMEs ( Capitanio et al. , 2009 ; FoodDrinkEurope, 2016 ; Materia et al. , 2017 ). Furthermore, the digitalisation process can be quite different depending on the nature of the digital technologies and on the challenges that arise in different supply chain stages ( Pivoto et al. , 2019 ; Poppe and Renwick, 2015 ).
In light of the above considerations, this study aims to provide a more specific systematisation of the factors that enable and hinder the adoption of different technologies.
To this end, this research focusses specifically on the farm-level adoption of smart-farming solutions since the digitalisation of the supply chain’s upstream stage is an essential condition to successfully exploit this revolution and ensure that all stakeholders benefit. Indeed, farmers (particularly in small and medium agri-food enterprises) have traditionally been reluctant to innovate ( Long et al. , 2016 ) and they often lack the required resources and competences, especially for human capital-intensive innovations ( Dicecca et al. , 2016 ; Materia et al. , 2017 ; Warren, 2002 ).
The study is based on a comprehensive review of contributions from the scientific literature dealing with the adoption of farm-level digital innovations, with a specific focus on management information system (MIS) technologies, defined as a set of software systems used to support human decision-making within farm management activities ( Fountas et al. , 2015a ; Verdouw et al. , 2015 ). This study reviews the theoretical frameworks used as well as the drivers and barriers, both within and beyond farm boundaries.
The paper is structured as follows: section 2 sets out the scope and the objective of the study by means of providing a definition of the technologies considered and presenting the research questions. Section 3 outlines the methodology and discusses the sources of literature considered. Section 4 describes the main results, including descriptive characteristics and evidence gathered concerning the theoretical frameworks adopted and main evidence found in the literature reviewed. Finally, section 5 includes a discussion of these findings together with some recommendations for future research.
2. The scope and objectives
2.1 management information systems at the farm level.
In recent years, a large number of digital technologies have become available for actors in upstream stages within agri-food chains. Amongst these, advanced decision support systems (DSSs) have garnered increasing attention, enabling farmers to make informed decisions not only related to farming practices (precision agriculture technologies) but also financial and managerial operations ( Fountas et al. , 2015a ).
Amongst such software solutions, scholars have placed particular focus on the farm management and information systems (FMISs) category. Sørensen et al. (2010 , p. 38) defined FMISs as “a planned system for the collecting, processing, storing and disseminating of data in the form of information needed to carry out the functions of the farm”. In addition, they point out that FMISs can be considered “an integral part of the overall management system of a firm […] and part of tools such as Enterprise Resource Planning (ERP) and overall Information Systems (IS)” ( Sørensen et al. , 2010 , p. 38). By contrast, ERP technologies are defined as standardised software packages which incorporate information systems for multiple business functions to create a single integrated system ( Verdouw et al. , 2015 ).
In fact, even if FMIS and ERP applications have followed different evolutionary paths, today they communicate and collaborate within agri-food enterprises’ information technology (IT) systems. On the one hand, modern FMISs were developed to organise the increasing amount of data generated by precision agriculture technologies and combined them with an economic and holistic management perspective ( Fountas et al. , 2015a ; Verdouw et al. , 2015 ). On the other hand, ERPs stem from manufacturing resource planning systems and the necessity to integrate both across and within the various functional silos found in modern manufacturing ( Jacobs and Weston, 2007 ). Initially, the rigid standards of early ERP solutions were not well suited to deal with the complexity of agricultural biological processes. Nonetheless, new web-based and customisable ERP systems are much more flexible ( Møller, 2005 ) and capable of ensuring the interoperability required to integrate “many FMISs, DSSs and many applications in between, all covering different aspects of farm management” ( Verdouw et al. , 2015 , p. 127).
This is why various authors argue that integrating FMIS and ERP research can result in numerous and promising research opportunities that are worth investigating ( Haberli et al. , 2019 ; Verdouw et al. , 2015 ).
Hence, for the purposes of the present study, the literature analysed includes research conducted on the farm-level adoption of both FMIS and ERP as well as the intersections between these two technologies. Given the fast-developing nature of digital technologies, by including contributions dealing with different versions of FMIS (both computer- and mobile-based systems, applications, software as a service [SaaS], etc.), this review provides the opportunity to consider as many different contributions to the topic as possible. In the rest of the paper, the term “farm-level MIS” refers to this combined group of management information technologies.
2.2 Research questions
The literature on the farm-level adoption of MIS has mostly focussed on FMIS technologies. To the best of the authors’ knowledge, the first specific contribution dates back to the end of last century ( Lewis, 1998 ). At that time, the technical characteristics and functionalities of software and devices used were more akin to computers assisting agricultural production as compared to how FMISs are used today ( Tummers et al. , 2019 ). Amongst most recent contributions, Tummers et al. (2019) reviewed the obstacles to FMIS development and adoption found in the literature, besides providing a more updated state of the art on device functionalities. Amongst these barriers, some are more related to technical traits such as data standards and system integration, while others relate more specifically to factors linked to lower adoption, such as the comprehensibility of software, insufficient skills amongst farmers and regional/language barriers.
Although Tummers et al. (2019) referred to specific obstacles, FMIS adoption has been analysed only partially to determine the explanatory variables behind their diffusion, not as the actual object of study. In fact, to the best of the authors’ knowledge, the available literature still lacks a review which focusses specifically on farm-level MIS adoption processes.
Which theories have scholars used to study the adoption of farm-level MISs?
What are the main determinants affecting the adoption of farm-level MISs?
What are the main drivers?
What are the main barriers?
3. Materials and methods
The process of searching, collecting, selecting and synthesising literature occurred between September 2019 and January 2020, following the literature review’s approach proposed by Hart, who defined it as “the selection of available documents (both published and unpublished) on the topic, (…) to fulfil certain aims or express certain views on the nature of the topic and how it is to be investigated, and the effective evaluation of these documents in relation to the research being proposed” ( Hart, 1998 , p. 13).
This approach has been integrated with both specific guidelines on how to structure the review ( Torraco, 2005 ; Vom Brocke et al. , 2009 ) and existing examples of structured literature reviews ( Boehm and Thomas, 2013 ; Cronin et al. , 2008 ; Della Corte et al. , 2018 ; Giacomarra et al. , 2020 ; Svejvig and Andersen, 2015 ; Tell et al. , 2016 ), with the aim to clearly present the analysis and results. Therefore, the review is organised in the following three phases: (1) search strategy, (2) screening and selection and (3) data extraction and analysis.
3.1 The search strategy
The automated research component implied using two of the key high-quality digital libraries available: the Institute for Scientific Information (ISI) Web of Science and Scopus. As recommended by Hart (1998) , after different attempts to identify the most inclusive string, the final research query combined various sets of keywords such as farm MISs, FMISs, ERP and adoption. Search results included journal articles, conference papers and proceedings, white papers, reports and book sections, all published in English as found in the most recent FMIS review carried out by Tummers et al. (2019) . In order to take the technological evolution of these management systems into account while also being aware of the quickly developing literature on IT in agriculture, texts were limited to the 1998–2019 period, starting from the first review on FMIS available in these sources ( Lewis, 1998 ). This search identified additional studies that were then added to the screening process through snowball techniques. The authors identified these additional texts by manually browsing through references of works previously found via the automated search. A preliminary refinement of articles was based on selecting Web of Science categories pertaining to agricultural economics and policy, agriculture multidisciplinary, computer science interdisciplinary applications and management. A total of 849 studies were identified in these digital libraries (576 from ISI WOS and 273 from Scopus). An initial selection was carried out before downloading the studies, based on their titles and reading their abstracts. In this stage, the authors considered each contribution related to FMIS design, use and adoption and ERP adoption at the farm level for further refinement. A final screened sample of 70 studies was downloaded from said digital libraries (35 from ISI WOS and 35 from Scopus).
3.2 Screening and selection
All the studies identified were imported into a single library and processed using Mendeley citation manager software. Using the “Check for duplicates” feature, 12 studies were deleted, reducing the number of papers retained for analysis to 58. The screening selection process was based on two steps as found in Giacomarra et al. (2020) . First , the authors established the following inclusion criteria: (1) all studies which focussed on FMISs (computer- or mobile-based) and ERP adoption used in the agri-food sector and (2) all studies which focussed on FMIS design, development and technological evolutions (traceability, big data, cross compliance and cloud-based platforms). A total of nine additional studies were eliminated as a result of the previous step. Second, studies were further screened based on the exclusion criteria listed in Table 1 below.
The final subset included 35 studies for analysis and study (see Figure 1 below). The authors could not access the full text of one study due to licence restrictions and had to eliminate it from the sample. Moreover, 22 other studies did not satisfy the exclusion criteria indicated above, while additional nine works were eventually added to the final selection by browsing references of selected studies.
At this step, the authors did not only consider academic contributions which focussed solely on adoption. They expanded the scope to focus on other types of studies dedicated to FMIS software design and development. This choice was made for several reasons. First, one deals with the user-centric approach found in several texts ( Kaloxylos et al. , 2012 ; Kruize et al. , 2016 ; Sørensen et al. , 2011 ), an approach which “assumes that the users’ ideas and requirements reactions concerning the speciffc characteristics of the designed technology are integrated in the subsequent design” ( Sorensen et al. , 2010 , p. 45). As several scholars acknowledge, end-user involvement during the early stage of the devices’ design and development makes it easier to satisfy user requirements and, as a result, make user acceptance and adoption more likely. An example of this approach is found in Nurkka et al. (2007) , where data from interviews, questionnaires and farms visits served to identify users’ needs, demands and capabilities before designing a specific MIS, recognising, at the same time, problems and limitations that might occur in the adoption and use of such devices.
In addition, considering actual technology adoption and design simultaneously allows for a more complete picture on adoption determinants, including factors which might not be attributable solely to the adopter. This way, a more thorough understanding is possible, though not only of technology adoption, per se , but also some of its disparate consequences, one of which is the digital divide in the agri-food sector ( Bronson and Knezevic, 2016 ; Trendov et al. , 2019 ). This refers to the situation in which a substantial proportion of the population, identified by one or more shared characteristics, lags significantly behind others in the adoption of a new technology ( Warren, 2002 ). Indeed, as Bronson (2019 , p. 3) argues that “The bifurcation in the market for smart farming technologies may not simply be an adoption issue beginning on the farm (and with farmers); rather, it at least partly results from partial and normatively motivated design decisions which are helping to produce digital farming ‘haves’ and ‘have-nots’”. For these reasons, this paper includes various types of studies (both qualitative–descriptive and quantitative–deterministic), with the aim being to capture all the different determinants of farm-level MIS adoption identified until now.
3.3 Data extraction and the analysis
The authors uploaded all 35 papers to the ATLAS.ti 8 platform, a widely used piece of software to undertake qualitative data analyses and literature reviews ( Haan et al. , 2018 ; Hossain, 2016 ; Hossain et al. , 2019 ). The authors read the selected studies thoroughly and coded them with references to an extraction form organised in a matrix structure as suggested by Finfgeld-Connett (2014) and Leonidou et al. (2018) , with the aim to “minimize human error and document this procedure for replicability purposes” ( Leonidou et al. , 2018 , p. 3) . The matrix’s structure is based on (1) research questions for the literature review in keeping with Hart's recommendations (1998), (2) the data extraction method used by previous literature reviews to classify FMISs ( Tummers et al. , 2019 ) and (3) the reading of a sample of randomly selected studies to iteratively adjust the structure ( Hossain et al. , 2019 ; Tummers et al. , 2019 ).
The initial matrix structure comprised several features with the following classifications: general information (author, title, publication year and type of document), study description (sector, main theme, methods used, stakeholders involved, etc.) and adoption (theoretical framework – if any and including drivers and barriers). During the coding process, the authors created new themes when needed to categorise new codes. Most of the description elements were used to build statistic elaborations on the studies collected and together with the section focussed on adoption, they served to answer the research questions posited.
This section summarises the data obtained distributed into two sections, in keeping with the review structures used by Radu (2016) and Tummers et al. (2019) . The first focusses on descriptive statistics on the composition and main characteristics of the final sample of texts; the second provides findings to answer the research questions outlined above.
Trends regarding the number of publications and the composition of the sample of contributions studied provide interesting insights. The overall number of contributions is rather stable, apart from two peaks registered in 2010–2011 and in 2017 (see Figure 2 ). Both peaks denote the academic attention to evolved versions of FMISs with respect to more basic information systems available until that moment. In several cases, these studies may have been encouraged by international research projects (such as the EU-funded FUTUREFARM project or the SmartAgrifood and FiSpace projects, part of the European Future Internet Public–Private Partnership programme (FI-PPP)) [1] .
As this paper includes studies with research focusses other than just technology adoption, the authors classified the contributions by the main themes addressed and synthesised the findings into the following key categories: adoption, software design and other types of studies.
The “User adoption studies” category primarily comprises papers focussed specifically on MIS adoption by agri-food users; the “Software design studies” group incorporates contributions that do not focus on adoption, per se , but on the development of a software architecture model. As explained above, due to the user-centric approach applied in these papers, the authors of this study were able to identify user requirements and barriers to FMIS adoption and use. Last, the “Other studies” category includes contributions with a broader focus. Some of the texts included in the latter group report on the state of the art of FMIS development and use, along with future perspectives ( Allen and Wolfert, 2011 ; Fountas et al. , 2015a ; Kuhlmann and Brodersen, 2001 ; Tsiropoulos et al. , 2017 ). Other contributions focus on the development of integrated platforms (software ecosystems) to make smart-farming technologies (such as FMIS) interoperable ( Barmpounakis et al. , 2015 ; Kruize et al. , 2016 ).
In this study, the authors classify the literature according to the type of research conducted (qualitative vs quantitative): 20 studies were classified as qualitative and the remaining 15 as quantitative [2] . Table 2 below shows how all these studies are distributed.
While almost all the qualitative texts are included in the “Software architectural models” and “Other studies” categories, only two of the 15 studies on MIS adoption are qualitative. In the next sections, the authors discuss additional details on adoption studies.
4.1 The oretical and methodological approaches in adoption studies
Studies which focussed specially on farm-level MIS adoption are listed in Table 3 below. A technological evolution occurred in the devices objects of adoption studies, from computer-based management information systems ( Alvarez and Nuthall, 2006 ; Batte, 2005 ; Lewis, 1998 ; Tiffin and Balcombe, 2011 ) to more sophisticated, application-based and Internet-connected versions ( Carrer et al. , 2017 ; Fox et al. , 2018 ; Pivoto et al. , 2019 ). A total of three papers focussed on ERP adoption at the farm level ( Haberli et al. , 2017 , 2019 ; Verdouw et al. , 2016 ). The authors checked the technological coherence of this software with FMISs (discussed above) during the first selection step since only contributions that studied ERP as aligned with FMIS were considered in adoption studies.
In terms of theoretical approaches, 11 of the 15 studies in this group used at least one conceptual framework to study technology adoption. The most used theory was the diffusion of innovation (DOI), devised originally by Rogers (2003 , 1995) . DOI theory studies the spread of innovations and how they proliferate through different channels over time and within a particular social environment. According to Rogers, the innovation–decision process involves five steps: (1) knowledge, (2) persuasion, (3) decision, (4) implementation and (5) confirmation. These stages typically follow each other in a time-ordered manner. Adoption then depends on how individuals might perceive what Rogers (1982) defined as five innovation attributes, i.e. relative advantage, compatibility, complexity, trialability and observability.
Verdouw et al. (2015) are the only scholars in this review who used DOI as a unique framework. Also, two studies ( Haberli et al. , 2017 , 2019 ) integrated DOI with technology–organisation–environment (TOE), another widely used theory in adoption studies ( Molinillo and Japutra, 2017 ; Oliveira and Martins, 2011 ). The TOE framework identifies the process used by a company to adopt and implement innovations, taking into account the technological, organisational and environmental context ( De Pietro et al. , 1990 ). Fox et al. (2018) and Ibrahim et al. (2018) applied the unified theory of adoption and use of technology (UTAUT). UTAUT integrates eight theories on technology adoption: the technology acceptance model (TAM), the theory of reasoned action (TRA), DOI, the motivational model, the theory of planned behaviour, a combined theory of planned behaviour/ TAM, a model of personal computer use and social cognitive theory.
4.2 Adoption drivers reported in the literature
This section presents the major drivers behind the adoption of farm-level MIS found through the review. To ensure an efficient synthesis, only drivers coded at least four times are reported in this paper ( Tables A1 and A2 in the appendix) in keeping with Tummers et al. (2019) .
Results indicate that the most recurrent drivers are technology usability, farm size and farmer education level. Pignatti et al. (2015) categorised FMIS adoption drivers into three different groups: the innovations’ technological features, farm and farmer traits and external environment features. This study applies this same classification.
The International Organization for Standardization (ISO) defines usability/ease of use as follows: “the extent to which a product can be used by specified users to achieve specified goals with effectiveness, efficiency and satisfaction in a specified context of use” ( Bevan and Carter, 2016 , p. 269). This driver can thus be considered part of the ‘technological features’ category. As found in ( Carli and Canavari, 2013 ; Husemann and Novkovic, 2014 ; Nikkilä et al. , 2010 ; Rosskopf and Wagner, 2003 ; Sopegno et al. , 2016 ) and Alvarez and Nuthall (2006) , important factors when deciding to adopt a given MIS include user-friendly interfaces and easy-to-use software to input data and retrieve data output from the system. Furthermore, the possibility of customising a farm-level MIS or its flexibility is another driver included in this category. Indeed, when customised technologies meet farms’ specific needs while providing standard functionalities, users are more likely to adopt them ( Mackrell et al. , 2009 ; Rosskopf and Wagner, 2003 ).
Another driver category is related to farm characteristics. Farm size – generally measured not only in terms of land extension but also in terms of gross sales in some cases ( Batte, 2005 ) or the number of paid workers ( Tiffin and Balcombe, 2011 ) – is the most recurrent driver. Larger farms have to manage more complex production processes and they need to gather and process more information (e.g. precision agriculture data); therefore, farm-level MIS have to organise management data and control complexity ( Carrer et al. , 2017 ; Pivoto et al. , 2019 ). Another driver included in this category is the farm’s initial technology, a factor which includes all the different technologies already in use on the farm (current IT systems, other smart-farming technologies, etc.) and which might favour the adoption of a complementary farm-level MIS ( Alvarez and Nuthall, 2006 ; Lewis, 1998 ).
The third most recurrent driver is farmers’ education, included in the user characteristics category. Several studies found that more educated farmers were more likely to adopt MIS ( Alvarez and Nuthall, 2006 ; Carrer et al. , 2017 ; Engler and Toledo, 2010 ; Kaloxylos et al. , 2014 ; Lewis, 1998 ; Tiffin and Balcombe, 2011 ). As explained by Carrer et al. : “Farmers with higher education manifest greater demand for information and stronger ability to evaluate the beneffts of using computers as a tool to support management decision-making” ( Carrer et al. , 2017 , p. 16). Another user characteristic which might have a positive influence on adoption is the existing level of IT skills needed to use management systems, including familiarity with computers or information systems ( Allen and Wolfert, 2011 ; Kuhlmann and Brodersen, 2001 ; Nurkka et al. , 2007 ) and with Internet usage ( Kaloxylos et al. , 2014 ). In addition, there are several important factors within this group related to users’ beliefs, perceptions and needs which drive farm-level MIS adoption. Amongst the individual perception factors, several studies point to the perceived relative advantage. Rogers defined this as “the degree to which an innovation is perceived as better than the idea it supersedes” ( Rogers, 1982 , p. 213). In this sense, there are different interconnected subdimensions regarding relative advantages related to farm management: the degree of economic profitability, costs, benefits in terms of time and effort, etc. Haberli et al. (2019) , Rosskopf and Wagner (2003) , Tsiropoulos et al. (2017) and Verdouw et al. (2015) determined that perceived benefits include greater transparency, greater integration and improved efficiency, as well as expected profitability and other aspects related to the economic dimension of relative advantage.
When only considering studies that specifically focus on adoption, the most recurrent driving factors are limited to farms’ and farmers’ characteristics, such as size, education and relative advantage. No recurrent drivers were identified in the external environment group of features.
4.3 Adoption barriers reported in the literature
This study applied the same analysis to adoption barriers (see Table A1 in the appendix). When applying the same categorisation to factors which directly or indirectly impede adoption, it seems that technological features are the most relevant barriers.
As Tummers et al. (2019) described, problems related to interoperability or system integration between FMISs and their components hinder “interchangeability” between applications and platforms, reducing their applicability and, thus, the future adoption of such technology ( Kruize et al. , 2016 ). For Rosskopf and Wagner (2003) , an important requirement expressed by some farmers is the possibility of adapting/integrating new and old software. Interoperability issues are strictly connected to data standards since a lack of industry-wide data exchange protocols causes difficulties in data exchange, thus limiting farm-level MIS applicability ( Allen and Wolfert, 2011 ; Fountas et al. , 2015a ; Kruize et al. , 2016 ). Another aspect related to technological features refers to concerns regarding data ownership, privacy and security, representing issues for users and possible adopters ( Allen and Wolfert, 2011 ; Fountas et al. , 2009 ; Kaloxylos et al. , 2014 ; Zheleva et al. , 2017 ).
Included amongst technological factors which hinder adoption more directly is the complexity of these technologies; this not only includes unintuitive or excessively complicated interfaces but also too many features when compared to users’ actual needs, making farm-level MIS technologies difficult to implement, understand and use ( Haberli et al. , 2017 ; Nikkilä et al. , 2010 ; Verdouw et al. , 2015 ). This perceived complexity is one of the causes that makes these technologies time-consuming for possible adopters, especially in terms of learning how to use them ( Alvarez and Nuthall, 2006 ; Pivoto et al. , 2019 ), for example, when manually inputting data ( Mackrell et al. , 2009 ; Steffe, 2000 ). These aspects are inversely connected to usability as a driver as discussed in the previous section, underscoring how much software design influences its adoption.
Between the technological feature and farm resource categories, the cost of these technologies certainly plays a role in adoption decisions. For Allen and Wolfert (2011) , farmers are reluctant to invest too much in this software due to lower farm profitability and high market volatility. Kaloxylos et al. (2014) reported some concerns about the cost of other smart-farming technologies related to their implementation; these can be even more prohibitive in the case of smallholders or SMEs ( Zheleva et al. , 2017 ). Finally, when it comes to user characteristics, several studies cite age as a common adoption barrier ( Allen and Wolfert, 2011 ; Alvarez and Nuthall, 2006 ; Batte, 2005 ; Fountas et al. , 2015b ; Lewis, 1998 ; Tsiropoulos et al. , 2017 ). The common argument is that new generations of farmers are usually more educated and computer skilled, thus more willing to use new technologies. Again, when only considering studies specifically focussed on adoption, age is the most important adoption barrier ( Alvarez and Nuthall, 2006 ; Batte, 2005 ; Engler and Toledo, 2010 ; Lewis, 1998 ; Tiffin and Balcombe, 2011 ). Interestingly, a limited perception of the benefits of these tools is another recurrent barrier. Indeed, as Rosskopf and Wagner (2003 , p. 653) explained that “[...] While the scientists saw the cost of technology and the lack of user friendliness as the main problems, the participants of this study thought that lack of training and failure to understand the possible benefits were the greatest impediments”. In fact, cost is not found to be a recurrent barrier in these studies as opposed to their complexity in terms of the time required to assimilate these technologies ( Alvarez and Nuthall, 2006 ; Mackrell et al. , 2009 ; Pivoto et al. , 2019 ).
No features from the external environment were included amongst the most recurrent barriers. The only barrier which might be considered to belong to this group is infrastructural deficiency, specially the lack of network connectivity.
5. Discussion and conclusions
The aim of this paper was to review the literature on farm-level MIS adoption. Given the fast development of these technologies and our attempt to consider all the possible contributions to the research topic, the study considered different types of ITs used for farm management (both computer- and mobile-based FMIS and integrated farm-level ERP systems) and extended the analysis to studies which do not only focus directly on adoption but also on software architecture design and development.
When it comes to adoption determinants, the results obtained confirm other scholars’ previous findings ( Pierpaoli et al. , 2013 ; Tsiropoulos et al. , 2017 ; Tummers et al. , 2019 ), specifically that technologies’ technical features in fact seem to play an important role in shaping the diffusion of MIS at the farm level. On the one hand, “systemic” technical problems, such as a lack of interoperability amongst devices, a lack of data standards and elevated costs, can limit a given technology’s full potential; on the other hand, another key issue that seems to determine farm-level MIS adoption and the intention to use the latter is users’ individual perceptions (farmers and farm employees) of innovation attributes such as the technology’s relative advantage, usability, complexity and possible customisation. Furthermore, users’ characteristics together with farm features are also important adoption determinants. Age, education level and existing computer skills (users’ characteristics) as well as farm size and initial technology in use (farm features) are the most recurrent factors. When limiting the focus to studies which solely address adoption, the most recurrent determinant factors seem limited to farm size, user age and education and the technology’s perceived relative advantage.
5.1 Research implications
The main practical implications of this study stem from the provision of a comprehensive systematisation of factors which might determine MIS technologies adoption at the farm level. In facts, the inclusion of both conceptual and empirical types of contributions can reasonably show that adoption drivers and barriers might be due not only to users’ characteristics and resources but also to technology features ( Bronson, 2019 ). This is particularly relevant if seen as a cause behind today’s unequal access to technology, i.e. the digital divide ( Bronson, 2019 ; Carolan, 2016 ; van der Burg et al. , 2019 ). In this perspective, our results might be of particular interest and utility for policymakers who might find strategic to acknowledge the relevance at the same time of different types of determinants of adoption, when defining proper policies and when aiming at guaranteeing a fair and inclusive digitalisation of the agri-food sector. Moreover, technology providers and the related smart-farming technologies’ industry are another type of actors who might benefit from a deeper understanding of adoption determinants. It might be easier for them to understand and successfully satisfy the requirements their customers demand.
For what concerns the theoretical implications, this study deals not only with adoption determinants but it also provides a classification of studies in terms of research focus, methods and theoretical frameworks used. Studies which focus specifically on adoption are mainly quantitative in nature (see Table 3 ). Looking at the evolution of these studies over time, it seems that, until 2019, scholars focussed their attention predominantly on software design and development rather than on their adoption, though adoption analyses have grown in number due to the increasing attention paid to the whole group of smart-farming technologies ( Kernecker et al. , 2019 ; Knierim et al. , 2019 ; Pivoto et al. , 2019 ). When the theories used to investigate the adoption are analysed, results indicate that some scholars have used different conceptual frameworks such as DOI and TOE together to capture all the relevant factors (individual and organisational) which might drive MIS adoption and implementation ( Alvarez and Nuthall, 2006 ; Haberli et al. , 2017 , 2019 ; Lewis, 1998 ; Mackrell et al. , 2009 ). However, the majority of relevant factors identified in the literature so far seem to be limited to the individual action sphere. Accordingly, in the studies considered in the literature review, farmers (and, at times, both farm managers and farm employees) are generally considered the key decision-makers.
Nonetheless, in Tummers et al. 's (2019) recent review of FMIS literature, a wider scope of stakeholders is considered in the implementation and use of modern FMISs in the agri-food sector, as occurred in prior studies ( Barmpounakis et al. , 2015 ; Kaloxylos et al. , 2012 ). Moreover, in their review on big data and smart-farming technologies, Wolfert et al. referred to a new possible network of stakeholders built around farms which might produce a major shift in roles and power relations amongst different players in existing agri-food chains: “We observed the changing roles of old and new software suppliers in relation to Big Data and farming and emerging landscape of data-driven initiatives with prominent role of big tech and data companies like Google and IBM’’ ( Wolfert et al. , 2017 , p. 75). This suggests that not only actors but also factors beyond farms’ or farmers’ individual circumstances might play an even more relevant role in determining MIS diffusion and adoption.
In fact, main theoretical implications deriving from the recent literature on farm-level MIS adoption indicate that the main adoption determinants identified so far in the literature reside mainly within the boundaries of the farm, in adopter units' characteristics (farmers and farms) and the technology features themselves. This study confirms that this last factor is relevant when the analysis is extended to contributions which do not focus solely on adoption.
5.2 Limitations and implications for future research
The main limitations of the study relate to the literature review approach adopted. Although the authors followed a clear structure as found in many other contributions, the review remains non-systematic; thus, it poses some limits to the replicability of the results. To reduce potential biases, the authors made any effort to clearly explicit each stage of the review process as well as any reference to how the review has been structured. Furthermore, although the authors clearly defined the technologies to be considered within the review, technological development in digital agriculture is so quick that MISs might considerably change their characteristics and as a consequence, some of their adoption determinants.
Nonetheless, the study achieved manifold and insightful results in respect to methods and theories used in recent literature. Moreover, the determinants of adoption identified so far have been classified and broadly discussed. In this respect, especially when MIS technologies are considered part of smart-farming technologies with their related ecosystems and new stakeholder networks, several interesting avenue for future research can be identified. On the one hand, an interesting research direction in studying adoption determinants would be to extend the focus to the role of additional factors beyond individual dimension ( Klerkx et al. , 2019 ). In this regard, actors and organisational factors relative to the supply chain where farmers and farms carry out their activities might play an important explanatory role in unravelling the adoption and diffusion in the entire agri-food sector’s digitalisation.
Another aspect which might deserve attention, as some scholars have recently pointed out, is the role played by dynamic capabilities in the digital transformation of mature industries ( Warner and Wäger, 2019 ). Dynamic capabilities are defined as “the firm’s ability to integrate, build, and reconfigure internal and external competences to address rapidly changing environments” ( Teece et al. , 2008 , p. 516). Some authors explored how these might impact business model innovation (e.g. in scouting new digital technologies or developing collaborative approaches within the innovation ecosystem) and thus enable digital transformation of the industry. Although the agricultural industry has shown traditionally poor propensity to innovate, especially in the case of small and medium farms, how the development of dynamic capabilities together with digital innovation adoption might lead to business model innovations in the agri-food sector is a topic that deserves to be further explored.
Flow diagram of the review process
Time distribution of studies
Second-step selection criteria
Thetype of research (qualitative or quantitative) by research focus
Studies focussed on adoption
https://cordis.europa.eu/project/rcn/88262/reporting/en , https://www.fispace.eu/ , http://smartagrifood.eu/ .
It should be noted that, in some cases, quantitative methods (such as multivariate data analyses or econometric models) were preceded by qualitative methods such as interviews or focus groups ( Haberli et al. , 2019 ).
Allen , J. and Wolfert , S. ( 2011 ), Farming for the Future towards Better Information-Based Decision-Making and Communication Phase I: Australasian Stocktake of Farm Management Tools Used by Farmers and Rural Professionals , New Zealand Centre of Excellence in Farm Business Management , Wageningen , available at: https://edepot.wur.nl/194811 .
Alvarez , J. and Nuthall , P. ( 2006 ), “ Adoption of computer based information systems: the case of dairy farmers in Canterbury, NZ, and Florida, Uruguay ”, Computers and Electronics in Agriculture , Vol. 50 , pp. 48 - 60 , doi: 10.1016/j.compag.2005.08.013 .
Barmpounakis , S. , Kaloxylos , A. , Groumas , A. , Katsikas , L. , Sarris , V. , Dimtsa , K. , Fournier , F. , Antoniou , E. , Alonistioti , N. and Wolfert , S. ( 2015 ), “ Management and control applications in agriculture domain via a future internet business-to-business platform ”, Information Processing in Agriculture , Vol. 2 , pp. 51 - 63 , doi: 10.1016/j.inpa.2015.04.002 .
Batte , M.T. ( 2005 ), “ Changing computer use in agriculture: evidence from Ohio ”, Computers and Electronics in Agriculture , Vol. 47 No. 1 , pp. 1 - 13 , doi: 10.1016/j.compag.2004.08.002 .
Bevan , N. and Carter , J. ( 2016 ), “ Human-computer interaction–theory, design, development and practice ”, International Conference on Human-Computer Interaction , Springer , Cham , Vol. 9731 , pp. 268 - 278 , doi: 10.1007/978-3-319-39510-4 .
Boehm , M. and Thomas , O. ( 2013 ), “ Looking beyond the rim of one’s teacup: a multidisciplinary literature review of product-service systems in information systems, business management, and engineering and design ”, Journal of Cleaner Production , Vol. 51 , pp. 245 - 260 , doi: 10.1016/j.jclepro.2013.01.019 .
Bouwman , H. , Nikou , S. , Molina-Castillo , F.J. and de Reuver , M. ( 2018 ), “ The impact of digitalization on business models ”, Digital Policy, Regulator Government , Vol. 20 No. 2 , pp. 105 - 124 , doi: 10.1108/DPRG-07-2017-0039 .
Bronson , K. ( 2019 ), “ Looking through a responsible innovation lens at uneven engagements with digital farming ”, NJAS - Wageningen Journal of Life Sciences , Vols 90–91 , 100294 , doi: 10.1016/j.njas.2019.03.001 .
Bronson , K. and Knezevic , I. ( 2016 ), “ Big data in food and agriculture ”, Big Data Society , Vol. 3 , pp. 1 - 5 , doi: 10.1177/2053951716648174 .
Bucci , G. , Bentivoglio , D. and Finco , A. ( 2018 ), “ Precision agriculture as a driver for sustainable farming systems: state of art in litterature and research ”, Quality - Access to Success , Vol. 19 , pp. 114 - 121 .
Capitanio , F. , Coppola , A. and Pascucci , S. ( 2009 ), “ Indications for drivers of innovation in the food sector ”, British Food Journal , Vol. 111 , pp. 820 - 838 , doi: 10.1108/00070700910980946 .
Carli , G. and Canavari , M. ( 2013 ), “ Introducing direct costing and activity based costing in a farm management system: a conceptual model ”, Procedia Technology , Elsevier BV , Vol. 8 , pp. 397 - 405 .
Carolan , M. ( 2016 ), “ The politics of big data food governance meets ‘weak’ resistance ”, Agri-Environmental Governance as an Assemblage: Multiplicity, Power, and Transformation , pp. 195 - 212 .
Carrer , M.J. , de Souza Filho , H.M. and Batalha , M.O. ( 2017 ), “ Factors influencing the adoption of farm management information systems (FMIS) by Brazilian citrus farmers ”, Computers and Electronics in Agriculture , Vol. 138 , pp. 11 - 19 , doi: 10.1016/j.compag.2017.04.004 .
Cronin , P. , Ryan , F. and Coughlan , M. ( 2008 ), “ Undertaking a literature review: a step-by-step approach ”, British Journal of Nursing , Vol. 17 , pp. 38 - 43 , doi: 10.12968/bjon.2008.17.1.28059 .
Della Corte , V. , Del Gaudio , G. and Sepe , F. ( 2018 ), “ Ethical food and the kosher certification: a literature review ”, British Food Journal , Vol. 120 , pp. 2270 - 2288 , doi: 10.1108/BFJ-09-2017-0538 .
De Pietro , R. , Wiarda , E. and Fleischer , M. ( 1990 ), “ The context for change: organization, technology and environment ”, in Tornatzky , L.G. and Fleischer , M. (Eds), The Processes of Technological Innovation , Lexington , pp. 151 - 175 .
Dicecca , R. , Pascucci , S. and Contò , F. ( 2016 ), “ Understanding reconfiguration pathways of agri-food value chains for smallholder farmers ”, British Food Journal , Vol. 118 , pp. 1857 - 1882 , doi: 10.1108/BFJ-05-2016-0194 .
Engler , A. and Toledo , R. ( 2010 ), “ An analysis of factors affecting the adoption of economic and productive data recording methods of Chilean farmers ”, Ciencia e Investigacian Agraria , Vol. 37 , pp. 101 - 109 .
Fabregas , R. , Kremer , M. and Schilbach , F. ( 2019 ), “ Realizing the potential of digital development: the case of agricultural advice ”, Science , Vol. 366 , doi: 10.1126/science.aay3038 .
Finfgeld-Connett , D. ( 2014 ), “ Use of content analysis to conduct knowledge-building and theory-generating qualitative systematic reviews ”, Qualitative Research , Vol. 14 , pp. 341 - 352 , doi: 10.1177/1468794113481790 .
FoodDrinkEurope ( 2016 ), A Competitive EU Food and Drink Industry for Growth and Jobs , Bruxelles , available at: http://www.fooddrinkeurope.eu/uploads/publications_documents/Competitive_food_industry_growth_jobs_report.pdf .
Fountas , S. , Kyhn , M. , Jakobsen , H.L. , Wulfsohn , D. , Blackmore , S. and Griepentrog , H.W. ( 2009 ), “ A systems analysis of information system requirements for an experimental farm ”, Precision Agriculture , Vol. 10 , pp. 247 - 261 , doi: 10.1007/s11119-008-9098-5 .
Fountas , S. , Carli , G. , Sørensen , C.G. , Tsiropoulos , Z. , Cavalaris , C. , Vatsanidou , A. , Liakos , B. , Canavari , M. , Wiebensohn , J. and Tisserye , B. ( 2015a ), “ Farm management information systems: current situation and future perspectives ”, Computers and Electronics in Agriculture , Vol. 115 , pp. 40 - 50 , doi: 10.1016/j.compag.2015.05.011 .
Fountas , S. , Sorensen , C.G. , Tsiropoulos , Z. , Cavalaris , C. , Liakos , V. and Gemtos , T. ( 2015b ), “ Farm machinery management information system ”, Computers and Electronics in Agriculture , Vol. 110 , pp. 131 - 138 , doi: 10.1016/j.compag.2014.11.011 .
Fox , G. , Mooney , J. , Rosati , P. , Paulsson , V. and Lynn , T. ( 2018 ), “ Towards an understanding of farmers’ mobile technology adoption: a comparison of adoption and continuance intentions ”, Americas Conference on Information Systems 2018: Digital Disruption, AMCIS 2018 , New Orleans, LA , available at: https://www.scopus.com/inward/record.uri?eid=2-s2.0-85054202435&partnerID=40&md5=d8d90a9ef7aa68f4be54bd5107c33db5 .
Giacomarra , M. , Galati , A. , Crescimanno , M. and Vrontis , D. ( 2020 ), “ Geographical cues: evidences from new and old world countries’ wine consumers ”, British Food Journal , Vol. 122 No. 4 , pp. 1252 - 1267 , doi: 10.1108/BFJ-08-2019-0580 .
Haan , M.M. , Van Gurp , J.L.P. , Naber , S.M. and Groenewoud , A.S. ( 2018 ), “ Impact of moral case deliberation in healthcare settings: a literature review ”, BMC Medical Ethics , Vol. 19 , pp. 1 - 15 , doi: 10.1186/s12910-018-0325-y .
Haberli , C. , Oliveira , T. and Yanaze , M. ( 2017 ), “ Understanding the determinants of adoption of enterprise resource planning (ERP) technology within the agrifood context: the case of the Midwest of Brazil ”, The International Food and Agribusiness Management Review , Vol. 20 , pp. 729 - 746 , doi: 10.22434/IFAMR2016.0093 .
Haberli , C.J. , Oliveira , T. and Yanaze , M. ( 2019 ), “ The adoption stages (evaluation, adoption, and routinisation) of ERP systems with business analytics functionality in the context of farms ”, Computers and Electronics in Agriculture , Vol. 156 , pp. 334 - 348 , doi: 10.1016/j.compag.2018.11.028 .
Hart , C. ( 1998 ), Doing a Literature Review: Realeasing the Social Science Research Immagination , SAGE Publications , London .
Hossain , M. ( 2016 ), “ Grassroots innovation: a systematic review of two decades of research ”, Journal of Cleaner Production , Vol. 137 , pp. 973 - 981 , doi: 10.1016/j.jclepro.2016.07.140 .
Hossain , M. , Leminen , S. and Westerlund , M. ( 2019 ), “ A systematic review of living lab literature ”, Journal of Cleaner Production , Vol. 213 , pp. 976 - 988 , doi: 10.1016/j.jclepro.2018.12.257 .
Husemann , C. and Novkovic , N. ( 2014 ), “ Farm management information systems: a case study on a German multifunctional farm ”, Ekonomika Poljoprivrede , Centre for Evaluation in Education and Science (CEON/CEES) , Vol. 61 No. 2 , pp. 441 - 453 .
Ibrahim , A.M. , Hassan , M.S. and Gusau , A.L. ( 2018 ), “ Factors influencing acceptance and use of ICT innovations by agribusinesses ”, Journal of Global Information Management , Vol. 26 , pp. 113 - 134 , doi: 10.4018/jgim.2018100107 .
Jacobs , R.F. and Weston , T.F.C. ( 2007 ), “ Enterprise resource planning (ERP)-A brief history ”, Journal of Operations Management , Vol. 25 , pp. 357 - 363 , doi: 10.1016/j.jom.2006.11.005 .
Kaloxylos , A. , Eigenmann , R. , Teye , F. , Politopoulou , Z. , Wolfert , S. , Shrank , C. , Dillinger , M. , Lampropoulou , I. , Antoniou , E. , Pesonen , L. , Nicole , H. , Thomas , F. , Alonistioti , N. and Kormentzas , G. ( 2012 ), “ Farm management systems and the future internet era ”, Computers and Electronics in Agriculture , Vol. 89 , pp. 130 - 144 , doi: 10.1016/j.compag.2012.09.002 .
Kaloxylos , A. , Groumas , A. , Sarris , V. , Katsikas , L. , Magdalinos , P. , Antoniou , E. , Politopoulou , Z. , Wolfert , S. , Brewster , C. , Eigenmann , R. and Maestre Terol , C. ( 2014 ), “ A cloud-based farm management system: architecture and implementation ”, Computers and Electronics in Agriculture , Vol. 100 , pp. 168 - 179 , doi: 10.1016/j.compag.2013.11.014 .
Kernecker , M. , Knierim , A. , Wurbs , A. , Kraus , T. and Borges , F. ( 2019 ), “ Experience versus expectation: farmers’ perceptions of smart farming technologies for cropping systems across Europe ”, Precision Agriculture . doi: 10.1007/s11119-019-09651-z .
Klerkx , L. , Jakku , E. and Labarthe , P. ( 2019 ), “ A review of social science on digital agriculture, smart farming and agriculture 4.0: new contributions and a future research agenda ”, NJAS - Wageningen Journal of Life Sciences , Vols 90-91 , 100315 , doi: 10.1016/j.njas.2019.100315 .
Knierim , A. , Kernecker , M. , Erdle , K. , Kraus , T. , Borges , F. and Wurbs , A. ( 2019 ), “ Smart farming technology innovations – insights and reflections from the German smart-AKIS hub ”, NJAS - Wageningen Journal of Life Sciences , Vols 90-91 , 100314 , doi: 10.1016/j.njas.2019.100314 .
Kruize , J.W. , Wolfert , J. , Scholten , H. , Verdouw , C.N. , Kassahun , A. and Beulens , A.J.M. ( 2016 ), “ A reference architecture for farm software ecosystems ”, Computers and Electronics in Agriculture , Vol. 125 , pp. 12 - 28 , doi: 10.1016/j.compag.2016.04.011 .
Kuhlmann , F. and Brodersen , C. ( 2001 ), “ Information technology and farm management: developments and perspectives ”, Computers and Electronics in Agriculture , Vol. 30 , pp. 71 - 83 , doi: 10.1016/S0168-1699(00)00157-5 .
Lawson , L.G. , Pedersen , S.M. , Sørensen , C.G. , Pesonen , L. , Fountas , S. , Werner , A. , Oudshoorn , F.W. , Herold , L. , Chatzinikos , T. , Kirketerp , I.M. and Blackmore , S. ( 2011 ), “ A four nation survey of farm information management and advanced farming systems: a descriptive analysis of survey responses ”, Computers and Electronics in Agriculture , Vol. 77 , pp. 7 - 20 , doi: 10.1016/j.compag.2011.03.002 .
Lehmann , R.J. , Reiche , R. and Schiefer , G. ( 2012 ), “ Future internet and the agri-food sector: state-of-the-art in literature and research ”, Computers and Electronics in Agriculture , Vol. 89 , pp. 158 - 174 , doi: 10.1016/j.compag.2012.09.005 .
Leonidou , E. , Christofi , M. , Vrontis , D. and Thrassou , A. ( 2018 ), “ An integrative framework of stakeholder engagement for innovation management and entrepreneurship development ”, Journal of Business Research , Elsevier , pp. 1 - 14 , doi: 10.1016/j.jbusres.2018.11.054 , in press .
Lewis , T. ( 1998 ), “ Evolution of farm management information systems ”, Computers and Electronics in Agriculture , Vol. 19 , pp. 233 - 248 , doi: 10.1016/S0168-1699(97)00040-9 .
Long , T.B. , Blok , V. and Coninx , I. ( 2016 ), “ Barriers to the adoption and diffusion of technological innovations for climate-smart agriculture in Europe: evidence from The Netherlands, France, Switzerland and Italy ”, Journal of Cleaner Production , Vol. 112 , pp. 9 - 21 , doi: 10.1016/j.jclepro.2015.06.044 .
Mackrell , D. , Kerr , D. and von Hellens , L. ( 2009 ), “ A qualitative case study of the adoption and use of an agricultural decision support system in the Australian cotton industry: the socio-technical view ”, Decision Support Systems , Vol. 47 , pp. 143 - 153 , doi: 10.1016/j.dss.2009.02.004 .
Materia , V.C. , Pascucci , S. and Dries , L. ( 2017 ), “ Are in-house and outsourcing innovation strategies correlated? Evidence from the European agri-food sector ”, Journal of Agricultural Economics , Vol. 68 No. 1 , pp. 249 - 268 , doi: 10.1111/1477-9552.12206 .
Molinillo , S. and Japutra , A. ( 2017 ), “ Organizational adoption of digital information and technology: a theoretical review ”, Bottom Line , Vol. 30 , pp. 33 - 46 , doi: 10.1108/BL-01-2017-0002 .
Møller , C. ( 2005 ), “ ERP II: a conceptual framework for next-generation enterprise systems? ”, Journal of Enterprise Information Management , Vol. 18 , pp. 483 - 497 , doi: 10.1108/17410390510609626 .
Nikkilä , R. , Seilonen , I. and Koskinen , K. ( 2010 ), “ Software architecture for farm management information systems in precision agriculture ”, Computers and Electronics in Agriculture , Vol. 70 , pp. 328 - 336 , doi: 10.1016/j.compag.2009.08.013 .
Nurkka , P. , Norros , L. and Pesonen , L. ( 2007 ), Improving Usability and User Acceptance of Information Systems in Farming , EFITA 2007 Proceeding , Glas , p. 6 .
Oliveira , T. and Martins , M.F. ( 2011 ), “ Literature review of information technology adoption models at firm level ”, Electronic Journal of Information Systems Evaluation , Vol. 14 , pp. 110 - 121 .
Pierpaoli , E. , Carli , G. , Pignatti , E. and Canavari , M. ( 2013 ), “ Drivers of precision agriculture technologies adoption: a literature review ”, Procedia Technol , Vol. 8 , pp. 61 - 69 , doi: 10.1016/j.protcy.2013.11.010 .
Pignatti , E. , Carli , G. and Canavari , M. ( 2015 ), “ What really matters? A qualitative analysis on the adoption of innovations in agriculture ”, Journal of Agricultural Informatics , Vol. 6 , doi: 10.17700/jai.2015.6.4.212 .
Pivoto , D. , Barham , B. , Waquil , P.D. , Foguesatto , C.R. , Corte , V.F.D. , Zhang , D. and Talamini , E. ( 2019 ), “ Factors influencing the adoption of smart farming by Brazilian grain farmers ”, The International Food and Agribusiness Management Review , Vol. 22 , pp. 571 - 588 , doi: 10.22434/ifamr2018.0086 .
Poppe , K. and Renwick , A. ( 2015 ), “ A European perspective on the economics of big data dairy farm systems for the future view project ”, Farm Policy Journal , Vol. 12 No. 1 , pp. 11 - 19 .
Radu , L.D. ( 2016 ), “ Determinants of green ICT adoption in organizations: a theoretical perspective ”, Sustainable Times , Vol. 8 , doi: 10.3390/su8080731 .
Rogers , E.M. ( 1982 ), Diffusion of Innovations , The Free Press , New York, NY , doi: 10.4324/9780203710753-35 .
Rogers , E.M. ( 1995 ), “ Lessons for guidelines from the diffusion of innovations ”, Joint Commission Journal on Quality Improvement , Vol. 21 , pp. 324 - 328 , doi: 10.1016/S1070-3241(16)30155-9 .
Rogers , E.M. ( 2003 ), “ Diffusion of innovations ”, Diffusion of Innovations , Free Press , New York, NY , pp. 118 - 125 .
Rosskopf , K. and Wagner , P. ( 2003 ), “ Requirement for agricultural software - results of empirical studies ”, European Federation for Information Technologies in Agriculture (EFITA) , Debrecen , pp. 651 - 657 .
Sopegno , A. , Calvo , A. , Berruto , R. , Busato , P. and Bocthis , D. ( 2016 ), “ A web mobile application for agricultural machinery cost analysis ”, Computers and Electronics in Agriculture , Vol. 130 , pp. 158 - 168 .
Sørensen , C.G. , Fountas , S. , Nash , E. , Pesonen , L. , Bochtis , D. , Pedersen , S.M. , Basso , B. , Blackmore , S.B. , Sorensen , C.G. , Fountas , S. , Nash , E. , Pesonen , L. , Bochtis , D. , Pedersen , S.M. , Basso , B. and Blackmore , S.B. ( 2010 ), “ Conceptual model of a future farm management information system ”, Computers and Electronics in Agriculture , Vol. 72 , pp. 37 - 47 , doi: 10.1016/j.compag.2010.02.003 .
Sorensen , C.G. , Pesonen , L. , Fountas , S. , Suomi , P. , Bochtis , D. , Bildsøe , P. and Pedersen , S.M. ( 2010 ), “ A user-centric approach for information modelling in arable farming ”, Computers and Electronics in Agriculture , Vol. 73 , pp. 44 - 55 , doi: 10.1016/j.compag.2010.04.003 .
Sørensen , C.G. , Pesonen , L. , Bochtis , D.D. , Vougioukas , S.G. and Suomi , P. ( 2011 ), “ Functional requirements for a future farm management information system ”, Computers and Electronics in Agriculture , Vol. 76 , pp. 266 - 276 , doi: 10.1016/j.compag.2011.02.005 .
Steffe , J. ( 2000 ), “ Evolution of the farm environment: the need to produce a general information system ”, PACIOLI 7 Agenda 2000 and the FADN Agenda Workshop Report .
Svejvig , P. and Andersen , P. ( 2015 ), “ Rethinking project management: a structured literature review with a critical look at the brave new world ”, International Journal of Project Management , Vol. 33 , pp. 278 - 290 , doi: 10.1016/j.ijproman.2014.06.004 .
Teece , D.J. , Pisano , G. and Shuen , A. ( 2008 ), “ Dynamic capabilities and strategic management ”, Technological Know-How, Organ Capabilities Strategic Management Business Strategy Enterprise Development Competitive Environment , Vol. 18 , pp. 27 - 52 , doi: 10.1142/9789812834478_0002 .
Tell , J. , Hoveskog , M. , Ulvenblad , P. , Ulvenblad , P.O. , Barth , H. and Ståhl , J. ( 2016 ), “ Business model innovation in the agri-food sector: a literature review ”, British Food Journal , Vol. 118 , pp. 1462 - 1476 , doi: 10.1108/BFJ-08-2015-0293 .
Tiffin , R. and Balcombe , K. ( 2011 ), “ The determinants of technology adoption by UK farmers using Bayesian model averaging: the cases of organic production and computer usage ”, The Australian Journal of Agricultural and Resource Economics , Vol. 55 , pp. 579 - 598 , doi: 10.1111/j.1467-8489.2011.00549.x .
Torraco , R.J. ( 2005 ), “ Writing integrative literature reviews: guidelines and examples ”, Human Resource Development Review , Vol. 4 , pp. 356 - 367 , doi: 10.1177/1534484305278283 .
Trendov , M.N. , Varas , S. and Zeng , M. ( 2019 ), Digital Technologies in Agriculture and Rural Areas – Status Report , FAO , Rome .
Tsiropoulos , Z. , Carli , G. , Pignatti , E. and Fountas , S. ( 2017 ), “ Future perspectives of farm management information systems ”, in Pedersen , S.M. and Lind , K.M. (Eds), Precision Agriculture: Technology and Economic Perspectives , Springer International Publishing , Cham , pp. 181 - 200 , doi: 10.1007/978-3-319-68715-5_9 .
Tummers , J. , Kassahun , A. and Tekinerdogan , B. ( 2019 ), “ Obstacles and features of farm management information systems: a systematic literature review ”, Computers and Electronics in Agriculture , Vol. 157 , pp. 189 - 204 , doi: 10.1016/j.compag.2018.12.044 .
van der Burg , S. , Bogaardt , M.J. and Wolfert , S. ( 2019 ), “ Ethics of smart farming: current questions and directions for responsible innovation towards the future ”, NJAS - Wageningen Journal of Life Sciense , Vols 90-91 , 100289 , doi: 10.1016/j.njas.2019.01.001 .
Verdouw , C.N. , Robbemond , R.M. and Wolfert , J. ( 2015 ), “ ERP in agriculture: lessons learned from the Dutch horticulture ”, Computers and Electronics in Agriculture , Vol. 114 , pp. 125 - 133 , doi: 10.1016/j.compag.2015.04.002 .
Verdouw , C.N. , Wolfert , J. , Beulens , A.J.M. and Rialland , A. ( 2016 ), “ Virtualization of food supply chains with the internet of things ”, Journal of Food Engineering , Vol. 176 , pp. 128 - 136 , doi: 10.1016/j.jfoodeng.2015.11.009 .
Vom Brocke , J. , Simons , A. , Niehaves , B. , Riemer , K. , Plattfaut , R. and Cleven , A. ( 2009 ), “ Reconstructing the giant: on the importance of rigour in documenting the literature search process ”, 17th European Conference on Information Systems, ECIS 2009 , Verona, Italy .
Warner , K.S.R. and Wäger , M. ( 2019 ), “ Building dynamic capabilities for digital transformation: an ongoing process of strategic renewal ”, Long Range Planning , Vol. 52 , pp. 326 - 349 , doi: 10.1016/j.lrp.2018.12.001 .
Warren , M. ( 2002 ), “ Digital divide and the adoption of information and communication technology in UK ”, International Journal of Information Technology and Management , Vol. 1 , pp. 385 - 405 .
Wolfert , S. , Ge , L. , Verdouw , C. and Bogaardt , M.J. ( 2017 ), “ Big data in smart farming – a review ”, Agricultural Systems , Vol. 153 , pp. 69 - 80 , doi: 10.1016/j.agsy.2017.01.023 .
Zahra and George ( 2002 ), “ Absorptive capacity: a review, reconceptualization, and extension ”, Academy of Management , Vol. 27 , pp. 185 - 203 .
Zheleva , M. , Bogdanov , P. , Zois , D.S. , Xiong , W. , Chandra , R. and Kimball , M. ( 2017 ), “ Smallholder agriculture in the information age: limits and opportunities ”, LIMITS 2017 - Proceeding 2017 Work Computing Within Limits , pp. 59 - 70 , doi: 10.1145/3080556.3080563 .
Acknowledgements
Corrigendum: It has come to our attention that “Management information system adoption at the farm level: evidence from the literature” by Carlo Giua, Valentina Cristiana Materia and Luca Camanzi, published in the British Food Journal , [Vol. ahead-of-print No. ahead-of-print, 10.1108/BFJ-05-2020-0420], contained several mistakes within the author affiliations. This has now been corrected in the online article. The authors sincerely apologise for this.
Corresponding author
Related articles, we’re listening — tell us what you think, something didn’t work….
Report bugs here
All feedback is valuable
Please share your general feedback
Join us on our journey
Platform update page.
Visit emeraldpublishing.com/platformupdate to discover the latest news and updates
Questions & More Information
Answers to the most commonly asked questions here
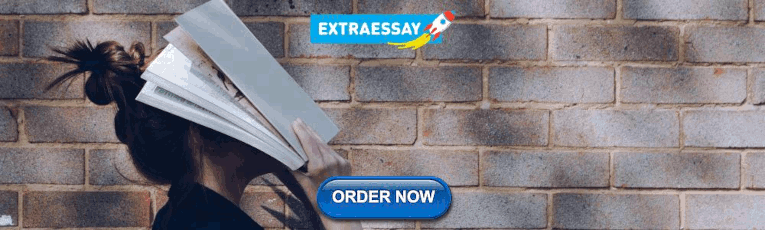
IMAGES
VIDEO
COMMENTS
Simple Summary. Informatization can effectively improve the production and management efficiency in the poultry farming process. In this study, a management system was designed to realize the acquisition, transmission, storage, and management of information, and upload the data to the cloud database to increase the flexibility and scalability of the system.
Poultry Farm Management Information System (A Case Study of Biyinzika Poultry International Limited) October 2021 British Journal of Computer Networking and Information Technology Volume 4(Issue 2 ...
The smart poultry management system is a crucial component of a modern poultry farm, but the majority manage the data using outdated technologies and platforms instead of modern IT solutions. Small enterprises still rely on manual processes and only the most advanced and large enterprises use smart IT solutions that allow practicing advanced ...
Literature review. An "IoT Smart ... regarding remote poultry farm management, Bluetooth is not a suitable candidate due to its short communication range limitations. Method and materials. The proposed remote poultry management system is made up of various blocks/units which are the input sensors, Wi-Fi enabled microcontroller unit, ...
The temperature r ange of the smart system is set between 32 and 3 5 degrees Celsius in week one, with the. button labelled week 1. When the temperature rises above 35 degrees Celsius, the fan ...
Aiming at breaking down the bottleneck problems of different scale of poultry farms, the low profitability of poultry farming, and backward information management in China, a safe and efficient information management system for poultry farming was designed. This system consists of (1) a management system application layer, (2) a data service layer, and (3) an information sensing layer. The ...
1. Introduction. By the year 2050, global demand for poultry meat will be over double what it was in 2005, and the demand for chicken eggs will be almost 40% greater (Smith et al., 2015).This increase in demand, combined with a global increase in competitiveness for agriculture related resources (Godfray et al., 2010) has potential to affect the profitability of poultry production systems.
Definition. As opposed to conventional poultry management that mainly relies on basic environment control systems, smart poultry management utilizes multiple sensors, analysis, visualization, and communication technologies to assist decision making and optimize farm stewardship. With the aid of these advanced technologies, poultry farmers will ...
A smart poultry management system is a crucial component of modern poultry farm. Such systems can help decision-making processes to reduce costs and use of resources in production, addressing multiple other aspects, animal well-being, reduction of gas emissions, smart management of energy consumption and others.
Growth, structure and strength of Indian poultry industry: A review. Indian Journal Poultry Science, 56(1): 1-10. ... IoT Based Smart Automated Poultry Farm Management System. April 2022. Bharati ...
A Poultry Farm Management System. Bhushan Balasaheb Phalke, Vaibhav Londhe, Archana Arudkar. 12 Jul 2020 - Vol. 3, Iss: 7, pp 41-43. TL;DR: In this paper, the authors highlighted the solution technology-based solution for low cost, asset-saving, quality-oriented and productive management of chicken farming, which used an embedded framework and ...
1. Introduction. The demand for poultry meat is progressively increasing because of its high protein, low energy, and low cholesterol (Lashari et al., 2018).However, poultry high production depends on the environmental condition, disease outbreaks, breeding process, and active management operations (Lashari et al., 2018).Therefore, efficient poultry health and welfare management is essential ...
The aims of this systematic literature review are to provide an overview of the current state of PLF in poultry farming and investigate whether the focus of PLF research has been to improve bird welfare. The study characteristics extracted from 264 peer-reviewed publications and conference proceedings suggest that poultry PLF has received ...
The poultry farm temperature should be monitored so that the growth of the chicken will not be affected. Temperature sensor used in the proposed system is LM35. LM 35 measures the temperature more accurately compared to other sensor modules. The measuring temperature range is about "−55 to 150 ℃''and consumes low power.
A management system was designed to realize the acquisition, transmission, storage, and management of information, and upload the data to the cloud database to increase the flexibility and scalability of the system. Simple Summary Informatization can effectively improve the production and management efficiency in the poultry farming process. In this study, a management system was designed to ...
Keywords Smart farming, Farm management information systems, Enterprise resource planning, Adoption, Diffusion of innovation, Digital divide Paper type Literature review. BritishFoodJournal Vol.123No.3,2021 pp.884-909 EmeraldPublishingLimited. 0007-070X DOI10.1108/BFJ-05-2020-0420.
Explore the latest full-text research PDFs, articles, conference papers, preprints and more on POULTRY MANAGEMENT. Find methods information, sources, references or conduct a literature review on ...
After the implementation, the new system was then tested and validated. When developing the system, the focus was on making the whole process of Information management in the poultry farm faster, more convenient and efficient for the company. This system automates the current poultry farm management information system in the organization. Keywords:
A Farm Management Information System (FMIS) or, in short, a Farm Management System (FMS) is developed to support the management of diverse farm enterprises. ... scheduling taking into consideration the characteristics of the crop field for intelligent and efficient agricultural management. This literature review article aims to explore the new ...
Nowadays, Automation plays an important role in everyday life. IoT based poultry farming is useful in situations where remote monitoring and maintenance is needed and this in turn modifies the conventional farm into a modern farm with various automated features. To improve chicken health and growth, various factors that affect the chicken health are regularly monitored. This gives an idea on ...
The study is based on a comprehensive review of contributions from the scientific literature dealing with the adoption of farm-level digital innovations, with a specific focus on management information system (MIS) technologies, defined as a set of software systems used to support human decision-making within farm management activities (Fountas ...
LITERATURE REVIEW [1] This focuses on gathering, storing, and managing the information of the chicken farm in order to deliver meals that are both ... Prof. N.A. Dawande, Smart poultry farm automation and monitoring system, IEEE, June 2017. [3] Raghudathesh G P1, Deepak D J2"IOT based intelligent poultry management system using Linux embedded ...
A Farm Management Information System (FMIS) or, in short, a Farm Management System (FMS) is developed to support the management of diverse farm enterprises. An FMIS is essentially a Management Information System (MIS) for the agriculture sector. ... To our best effort we were unable to find a systematic literature review on FMISs, and in this ...