Edinburgh Research Archive

- ERA Home
- Geosciences, School of
- GeoSciences PhD thesis and dissertation collection

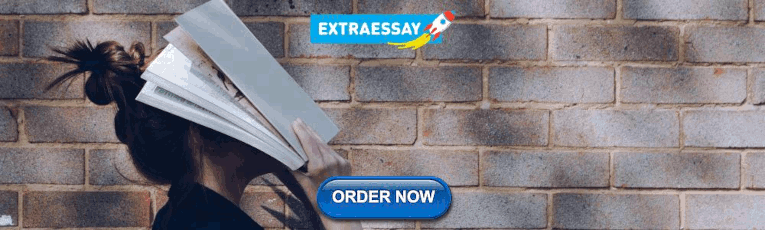
Climate-smart agriculture and rural livelihoods: the case of the dairy sector in Malawi
Collections.

Andrew E. Harding
Phd thesis, december 2006, "changes in mediterranean climate extremes: patterns, causes, and impacts of change".
- Graduate Program
Interdepartmental PhD Emphasis in Climate Sciences and Climate Change
Rationale for this phd emphasis.
Climate Sciences is the study of the physical processes that control climate on Earth including variations and interactions among the atmosphere, oceans, land and hydrosphere. Climate variations and changes are known to occur on broad ranges of spatial and temporal scales, ranging from decades, centuries, millennia and millions of years. Climate science can also inform the study of climate change, which is broadly defined as changes to the baseline of mean conditions and variability over long periods. Climate change since the beginning of the industrial revolution is one of the major issues affecting the environment and the future of humanity.
Anthropogenic influences on climate are already detectable and expected to continue into the future; examples of the impacts of climate change include extreme precipitation, droughts, heat waves, sea level rise, loss of habitats, food and water insecurity, economic and political stability to name just a few. Mitigation and Adaptation might involve economic regulations such as cap-and-trade or carbon tax, which put a price on carbon emissions.
Research in Climate Sciences and Climate Change requires specialized training in specific disciplines such as Atmospheric Sciences, Oceanography, Geology, Geography, Ecology, Economics, as well as interdisciplinary education across different areas. UCSB has a long tradition for carrying out research in Climate Sciences and Climate Change impacts. This research includes the study of the fundamental physical processes controlling climate on Earth and its response to human activities as well as the impacts of climate on humans and the environment. Research and teaching at UCSB is highly specialized as well as interdisciplinary.
This Interdepartmental PhD Emphasis in Climate Sciences and Climate Change provides doctoral students a broader understanding of the physical principles governing climate on Earth, climate changes associated with natural variability and anthropogenic forcings, and the impacts of climate change on the environment and society. The PhD emphasis provides graduate students with both core-training opportunities to gain access to methodological expertise across UCSB as well as to interact with Faculty, Researchers and graduate students in disciplines other than their own. Furthermore, the PhD Emphasis provides graduate students opportunities to learn how to effectively teach Climate Sciences and Climate Change. The Emphasis is administered in the Department of Geography. The PhD Emphasis formally acknowledges and builds upon existing collaborations among the departments and the Bren School listed herein.
Program of Study
Participation in this emphasis is optional and independent of the doctoral curriculum and degree requirements established by the student’s home department.
Admission to the Emphasis
Applications to the PhD Emphasis are accepted at any time during a graduate student’s academic tenure at UCSB. It is expected that most students will apply for admission between their first and third year of graduate study. Application materials consist of:
- Application form
- Student’s letter including research interests in climate sciences and climate change, expectations related to the emphasis and career goals
- Letter of support from PhD Advisor
The Director of the PhD Emphasis (see Faculty roster) reviews applications on a routine basis and informs applicants the outcome of their applications. Criteria for admission will include:
- Admission into a PhD program at UCSB
- Good academic standing
- Recommendation and strong support from the student’s PhD Advisor
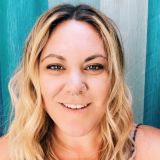
Kathryn Ficke
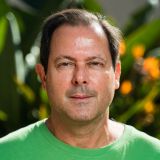
Charles Jones
Departments & programs.
- Bren School of Environmental Science and Management
Earth Science
- Interdepartmental Graduate Program in Marine Science
Related Faculty
- Elizabeth Ackert, Geography
- Diana Arya, Associate Professor, Education
- Kathy Baylis, Geography
- Leila Carvalho, Geography
- Kelly Caylor, Geography / Bren School
- Olivier Deschenes, Economics
- Timothy DeVries, Geography / IGPMS
- Qinghua Ding, Geography / IGPMS
- Steve Gaines, Bren School
- Vamsi Ganti, Geography
- Kostas Goulias, Geography
- Danielle Harlow, Professor and Associate Dean, Education
- Charles Jones, Geography - Director of the Emphasis
- David Lea, Earth Science / IGPMS
- Lorraine Lisiecki, Earth Science / IGPMS
- Hugo Loaciga, Geography
- Karin Lohwasser, Assistant Teaching Professor, Education
- David Lopez-Carr, Geography
- Joe McFadden, Geography
- Sally MacIntyre, IGPMS / Bren School
- Kyle Meng, Bren School / Economics
- Andrew Plantinga, Bren School
- Samantha Stevenson, Bren School
- Stuart Sweeney, Geography, Chair
- Naomi Tague, Bren School
- Anna Trugman, Geography
- Syee Weldeab, Earth Science / IGPMS
- Dave Siegel, Geography / IGPMS
- Ian Walker, Geography
Required Coursework
All students enrolled in this PhD Emphasis need to fulfill the following requirements:
Students are required to enroll and successfully pass a one-quarter, 4 Unit seminar course: GEOG 287 Seminar in Climate Sciences and Climate Change. The instructor for this course will be one of the Faculty participating in the Emphasis. This course covers key concepts and research methods related to climate, climate variability and change and impacts. Lectures consist of guest seminars primarily from Faculty participating in the Emphasis; the course serves as a venue to foster interaction among graduate students participating in the Emphasis, Researchers and Faculty.
Students are required to take two courses from the following list:
- GEOG 266 Introduction to Atmospheric Sciences Units: 4 – Prerequisite: graduate standing
- GEOG 263 Introduction to Physical Oceanography Units: 4 – Prerequisite: graduate standing
- GEOG 276 Geographical Time Series Analysis Units: 3 – Prerequisite: GEOG 172
- GEOG 213 Polar Environments Units:4 – Prerequisite: GEOG 3 or Geog4, ES 1 or 2, or EARTH1
- GEOG 243 Vegetation-Atmosphere Interactions Units: 4 – Prerequisite: graduate standing
- GEOG 246 Advanced Hydrologic Modeling Units: 4 – Prerequisite: GEOG 112 and 116
- GEOG 267 Chemical Oceanography Units: 4 (cross-listed with EARTH 276) – Prerequisite: CHEM 1C and graduate standing
- EARTH 205 Earth’s Climate: Past and Present Units: 3 – Prerequisite: graduate standing
- EARTH 206 Introduction to Climate Modeling Units: 4 – Prerequisite: graduate standing
- EARTH 266 Chemical Oceanography Units:4 (cross-listed with GEOG 267) – Prerequisite: CHEM 1C and graduate standing
- EARTH 276 Geological Oceanography Units: 4 – Prerequisite: graduate standing
Bren School
- ESM 203 Earth System Science Units: 4 – Prerequisite: GEOG 3 or equivalent IGPMS
- EARTH 266/GEOG 267 Chemical Oceanography Units: 4 – Prerequisite: CHEM 1C and graduate standing
- GEOG 263 Introduction to Physical Oceanography Units: 4– Prerequisite: graduate standing
The total number of units will vary depending on which courses are selected from this list:
- Geog 244 Society and Hazards Units: 4 – Prerequisite: graduate standing
- Geog 254 Demography Units: 4 – Prerequisite: graduate standing
- ESM 229 Economics and Policy of Climate Change Units: 4 – Prerequisite: ESM 204
- ESM 237 Climate Change Impacts and Adaptation Units: 4 – Prerequisite: graduate standing
- ECON 260D: Natural Resource Economics: Dynamic Programming Methods Units: 2 – Prerequisite: graduate standing
- ECON 260E Natural Resource Economics: Continuous-Time Methods Units: 2 Prerequisite: graduate standing
- ECON 260F Demand for Environmental Goods Units: 2 – Prerequisite: graduate standing
- ECON 260G Environmental Externalities and Regulation Units: 2 – Prerequisite: graduate standing
- ECON 260H Climate Change, Adaptation, and Policy Units: 2 – Prerequisite: graduate standing
- ECON 260I Time, Uncertainty, and Environmental Policy Units: 2 – Prerequisite: graduate standing
- ECON 260J Environmental Macroeconomics Units: 2 – Prerequisite: graduate standing
- ED 256 Technology and Education Contexts
- ED 287 Informal STEM Education
- ED 221H Design-based Research and Research-based design
Students are required to enroll and present their research in the GEOG 280 Geography Climate Research Meetings, which are a forum for researchers and students to discuss research topics in Climate Sciences and Climate Change. The meeting is held in the Earth Research Institute (ERI). Students are required to enroll in the Climate Research Meetings for a minimum of three quarters as a way to foment their participation in climate research topics.
The PhD dissertation of students participating in this Emphasis needs to have a strong focus in Climate Sciences and/or Climate Change. Furthermore, a member of the student’s PhD committee needs to be a member of the core Faculty participating in the Emphasis in Climate Sciences and Climate Change. No other limitations are set for the other members of the PhD committee.
Alumnus Testimonial
Emily Williams, PhD: “Participating in the Interdepartmental PhD Emphasis in Climate Sciences and Climate Change helped me build a robust interdisciplinary lens and toolbox through which to engage with climate science and policy. Through the emphasis, I was able to take courses across departments on climate sciences, policy, and impacts, providing me with foundational knowledge of the socio-political and physical dimensions of climate change. The emphasis also offered opportunities for professional development, such as presenting my graduate research to students and faculty in the climate seminar, thereby receiving invaluable feedback from distinguished scholars in the field. The rich training I gained has set me up to do both postgraduate research and advocacy, as I engage with academia and non-profits on issues of climate change and historical justice”.
Support Services
- Business Operations
- Technical Support
- Research Facilities
- Information
- Terms of Use
Department of Geography • 1832 Ellison Hall • UC Santa Barbara , CA 93106-4060 2024 © Regents of the University of California
Thank you for visiting nature.com. You are using a browser version with limited support for CSS. To obtain the best experience, we recommend you use a more up to date browser (or turn off compatibility mode in Internet Explorer). In the meantime, to ensure continued support, we are displaying the site without styles and JavaScript.
- View all journals
- My Account Login
- Explore content
- About the journal
- Publish with us
- Sign up for alerts
- Open access
- Published: 19 April 2019
Analysis of climate variability, trends, and prediction in the most active parts of the Lake Chad basin, Africa
- Rashid Mahmood ORCID: orcid.org/0000-0003-3364-5297 1 ,
- Shaofeng Jia 1 , 2 , 3 &
- Wenbin Zhu 1
Scientific Reports volume 9 , Article number: 6317 ( 2019 ) Cite this article
26k Accesses
78 Citations
1 Altmetric
Metrics details
- Climate change
- Projection and prediction
An understanding of climate variability, trends, and prediction for better water resource management and planning in a basin is very important. Since the water resources of the Lake Chad basin (LCB) are highly vulnerable to changing climate, in the present study, a combination of trend analysis methods was used to examine the climate variability and trends for the period of 1951–2015 using observed and Climate Research Unit (CRU) data, and a combination of spectral analysis techniques was used for the prediction of temperature and precipitation using CRU data. Eighty-four percent of the temperature time series indicated extremely strong signals of increasing trends (α = 0.001) and 25–38% of the precipitation time series indicated strong decreasing trends (α = 0.05). Temperature is expected to increase and precipitation is expected to decrease in the future. However, surprisingly, in some regions located in the South, the temperature was predicted to decrease slightly in 2021–2030 relative to 2006–2015. This decrease might occur because these regions are highly protected natural resource areas and forests are frequently present. On the whole, the temperature was predicted to increase by 0.65–1.6 °C and precipitation was predicted to decrease by 13–11% in the next two decades (i.e., 2016–2025 and 2026–2035) relative to 1961–1990. Periodic analysis showed a 20- to 25-year cycle in precipitation in all basins and a 40- to 45-year cycle in temperature but only in the Chari-Logone basin.
Similar content being viewed by others
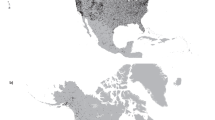
North American historical monthly spatial climate dataset, 1901–2016
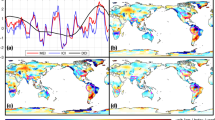
Identifying ENSO-related interannual and decadal variability on terrestrial water storage
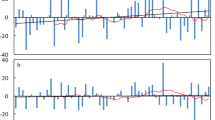
A Comparison Study of Precipitation in the Poyang and the Dongting Lake Basins from 1960–2015
Introduction.
The changing climate, global warming, and global energy imbalance are considered the main consequences of the dramatic increase in the concentration of greenhouse gases due to human activities, especially industrialization, the burning of fossil fuel, and land use/land cover changes 1 , 2 , 3 . One noticeable example of changing climate is an increase in the global average temperature by 0.74 °C ± 0.18 °C in only the last 100 years (1906–2005) 4 . This global warming has been accelerating and intensifying the water cycle of the world, which can result in more violent storms, floods, and droughts 5 . The intensified water cycle can influence every aspect of human activities, such as human health, water energy exploitation, ecosystems, food security, and industrial and municipal water supplies 3 . However, changes in the water cycle vary from region to region throughout the world 6 . Although climate change is one of the largest threats to the world, the poor regions, especially in Africa, have been suffering the most because these areas are least equipped to cope with climate change impacts 7 , 8 , 9 . According to Serdeczny et al . 10 , Africa has been identified as the most vulnerable continent to climate change impacts, and specifically, the water resources of Africa are highly vulnerable to climate change. One noticeable example is the water resources of the Lake Chad basin (LCB), where inflow to Lake Chad (LC) has diminished by 70–80% 11 , and the surface area of the lake has been reduced to approximately 2,000 km 2 from 25,000 km 2 (approximately 90%) over the last 40 years 12 , and even in the 1980s, it shrank to 300 km 2 12 . In addition, the lake was divided into the southern and northern pools in 1975 because of a devastating drought over the Sahel belt during the 1970s. Since then, the northern pool has hardly been flooded 13 . Furthermore, due to the shallow water level, LC is highly sensitive to changing climate, especially changes in precipitation and temperature 13 .
This occurrence shows that water resources of the LCB are highly vulnerable to rapidly changing global and regional climate, especially changes in temperature and precipitation, which are considered the most important elements in climate description 14 . The changes in these two variable can easily alter the hydrological cycle and environmental processes 15 . A good description of temperature and precipitation trends and variability is essential for many studies related to hydrology, climatology, and agriculture. Long-term trend analysis in temperature and precipitation is highly important for rainfed agricultural regions, where farmland is mainly reliant on precipitation, and for irrigated regions, where both temperature and precipitation can affect irrigation scheduling 15 . Trend analysis of climate variables is the central process in assessing the state of the climate of a region and provides an overall estimate about the variations in the climate variables 16 . Therefore, a good knowledge of trends in temperature and precipitation is very important for better water resource management in a basin, water demand and supply, agricultural water use and regulation, and even for better planning in a region.
Because knowledge of climate variability and trends is important for many aspects, the accurate forecast/prediction of climate variables is also equally important for the policymakers, planners, and other people working on water resource management and on mitigation and adaptation measures to cope with climate change. In addition, such predictions are also important for managing water supply and demand, mitigating flood and drought, maintaining reservoir water levels, planning and preparing for disasters, reducing the uncertainty by providing information on the future availability of water, improving water resources allocation, etc. 15 , 17 , 18 , 19 .
For the assessment of climate variability and trends, different parametric (e.g., T-test, F-test, and linear regression) and nonparametric (e.g., Mann–Kendall test, Kruskal–Wallis test, and Sen’s slope estimator) methods have been reported in the literature and are reviewed in detail in these studies, such as Esterby 3.0.CO;2-8 (1996)." href="/articles/s41598-019-42811-9#ref-CR20" id="ref-link-section-d10826476e448">20 , Zang et al . 21 , and Sonali and Nagesh 22 . Although parametric methods are more powerful, their applications are restricted to normally distributed time series. Since most of the climatic time series, specifically precipitation, do not fulfill normality requirement, nonparametric methods are most frequently applied in trend analysis, as in Burn et al . 23 , Fu et al . 24 , Oyerinde et al . 25 , Tekleab et al . 26 and Wang et al . 27 . In addition, the nonparametric methods are considered more robust than parametric methods against the outliers in a time series 21 , 22 .
On the other hand, for climate prediction, time series modeling is one of the major tools used when predicting short- and long-term changes in a climatic time series 17 , 28 . Time series analyses are usually used for monitoring, forecasting, and feedback by fitting a suitable model to a long time series. These time series analyses are widely used scientific methods in different field of studies, such as regional development and planning, business management, weather forecasting, market potential prediction, hydrological forecasting, environmental pollution control, astronomy, and oceanography 29 , 30 . Different kinds of techniques, such as moving average (MA), autoregressive (AR), autoregressive and moving average (ARMA), integrated ARMA (ARIMA), exponential smoothing (ES), seasonal decomposition, neural network, and spectral analysis, have been developed to analyze patterns in time series and for forecasting 31 . However, time series, specifically precipitation, are mostly considered difficult to predict because these consist of different components, such as trends, periodic patters, cyclic behavior, and white noise (random component), which limit the application of simple and widely used forecasting methods as mentioned above. Thus, the time series having complex cyclic behavior of different frequencies are better addressed with spectral analysis 32 . The spectral analysis is mostly used to study the physical processes in geophysics 33 , to study the stars in astronomy 34 and to predict the weather in meteorology 35 .
Different studies have been reported in the literature to explore the climate variability and general trends in or around the LCB, such as Adeyeri et al . 36 , Funk et al . 37 , Nkiaka et al . 38 and Okonkwo et al . 39 . Adeyeri et al . 36 conducted trend analysis in the Komadugu-Yobe basin located in the northwest of the LCB using observed and gridded reanalysis precipitation for the period of 1979–2015, and Nkiaka et al . 38 used observed precipitation for 1950–2000 in the Logone River basin located in the south of the LCB. Okonkwo et al . 39 applied linear trends in CRU precipitation for rainy season (June–September) for the period of 1970–2010. However, they used only mean values from the southern LCB and northern LCB. Funk et al . 37 explored trends in observed temperature and precipitation over Chad for the period 1900–2009 but only for the rainy season (June–September). However, these studies covered the LCB only partially, and none of the studies have been reported to cover the whole conventional basin, which is the most active part in the LCB and contributes entirely to LC; in addition, they did not even cover the Chari-Logone River basin, the river that provides more than 90% water to the lake. Moreover, most studies focused on precipitation and only the rainy season.
Many studies such as Buontempo 40 , Francois et al . 41 , Sarr 42 , Sultan et al . 43 , Sylla et al . 44 , Sylla et al . 45 , and Vizy et al . 46 reported projections for the temperature and precipitation in the 21 st century around the LCB using RCMs and/or GCMs under RCPs. However, with the best of our knowledge, no studies have been reported in the LCB for predicting temperature and precipitation for the near future using time series analysis, which is also a reasonably good, fast technique for use in predicting meteorological variables for the near future. It also requires less computational requirements and is simple to use.
Thus, the study had the following as the main objectives: (1) to assess the climate variability and climatic trends in the LCB by a combination of linear regression, polynomial model, Sen’s slope, and Mann-Kendal test of the annual and seasonal temperature and precipitation, which is essential for water resource management, and (2) to apply a combination of spectral analysis and harmonic regression to predict both variables and changes were assessed in these variables relative to the present condition, which is of great importance for water resource planning under anticipated climate change and for the mitigation of flood and droughts in the basin. In addition, periodic analysis was also performed to explore the cyclic behavior of temperature and precipitation. These analyses were performed in the most active parts of the LCB, encompassing the Chari-Logone, Komadugu-Yobe, Yedseram, El-Beid, Ngadda, Gubio, and Batha River basins (Fig. 1 ) for the period of 1951–2015. Temperature and precipitation were predicted for the next two decades (i.e., 2016–2025 and 2026–2035), and decadal changes were examined relative to the baseline period (1961–1990).
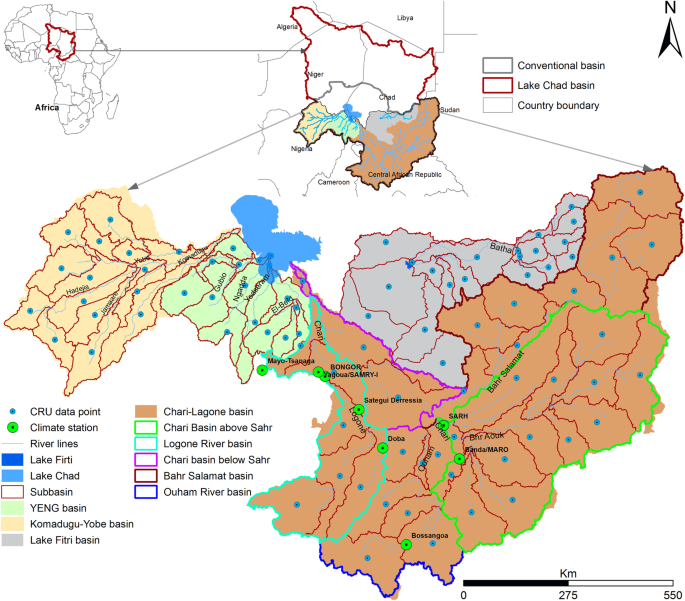
Location map showing the extracted CRU climate data points, observed meteorological stations, and subbasins in the study area, the Lake Chad basin. CRU data points are obtained by taking the mean of all grids inside a subbasin. YENG refers to Yedseram, El-Beid, Nagada and Gubio River .
This study is a part of an ongoing project “Feasibility of Inter Basin Water Transfer Project” of Power-China International Limited, aiming to restore the rapidly shrinking LC to its required ecological level by transferring water from the Congo River. The present study will help in water resources management and planning as well as in mitigating floods and droughts in the basin.
Study Area, Data and Methods
The Lake Chad basin (LCB) is the world’s biggest endorheic and transboundary drainage basin, covering an area of 2.5 × 10 6 km², which is approximately 8% of Africa 11 , 12 . It is situated between 5.2 and 25.3°N latitude and between 6.9 and 24.5°E longitude and straddles the borders of seven countries: Algeria, Cameroon, Central African Republic, Chad, Libya, Niger, Nigeria, and Sudan, as shown in Fig. 1 . This basin is a part of the Sahel belt and has a semiarid climate 39 . The whole basin mainly contributes water to Lake Chad (LC), which is one of the biggest lakes in the world. It is a vital source of fresh water for pastoral land, agricultural land, and fishing 47 . Among these uses, agriculture is the major user of its water resources, which provides livelihood to approximately 60% of the population in the LCB 48 . The LCB receives an average annual rainfall of 415 mm, ranging from 20–150 mm in the northern parts (e.g., Algeria) to 1200–1600 mm in the southwestern parts of the basin (e.g., Central African Republic) 49 . The average annual temperature varies between 35 °C and 40 °C. The basin is hot and dry during March to June, wet and hot during June to October, and dry and relatively cool from November to February 50 .
The present study was conducted in the most active parts of the LCB, which include the Chari-Logone, Komadugu-Yobe, Yedseram, El-Beid, Ngadda, Gubio, and Batha River basins (Fig. 1 ) and cover approximately 36% (911202 km 2 ) of the LCB 8 , 50 . All these rivers contribute directly to LC except the Batha River, which provides water only to Lake Fitri (Fig. 1 ). Among them, the Chari-Logone River is the main river system, which enters to the southern pool of the lake and contributes more than 90% to the total water of the lake. The Komadugu-Yobe River (KYR) is the second main river system, which enters to the northern pool of the lake and contributes 2–5% water to the lake 12 , 51 . In addition, the YENG (Yedseram, El-Beid, Ngadda, and Gubio Rivers) flows in the southwest of LC and contributes even less than 2% 50 .
Data description
Monthly precipitation data from 8 meteorological stations were obtained from the Lake Chad Basin Commission (LCBC) for the period of 1950–2013, as shown in Fig. 1 ; these stations did not adequately cover the large study area.
Recently, leading scientific research centers from around the world have created globally interpolated gridded datasets for understanding and predicting weather, water, and climate of the world, such as Climate Research Unit (CRU) dataset, Global Precipitation Climatology Center (GPCC), CICS High-resolution Optimally interpolated Microwave Precipitation from Satellite (CHOMPS), National Center for Environmental Protection (NCEP), and the Tropical Rainfall Measurement Mission (TRMM). These datasets have been created by observed, satellite, reanalysis, and combination of satellite and observed data.
In the present study, due to a scarcity of observed data, monthly mean temperature and precipitation were obtained from the latest version CRU-TS4.00 for the period of 1951–2015 because this dataset has been used widely in different studies in Africa 52 , 53 , 54 and in different studies for the evaluation of global climate models, regional climate modes, and hydrological models, as in Chiyuan et al . 55 , McMahon et al . 56 , and Smiatek et al . 57 . In addition, CRU data were evaluated with in situ observed data using different statistical indicators in Mahmood and Jia (2018), which showed that CRU data can be used with high confidence. This is a high-resolution (0.5° × 0.5°) monthly climatic dataset, including precipitation, max temperature, min temperature, mean temperature, wet days, potential evapotranspiration, diurnal temperature range, vapor pressure, cloud cover, and frost-day frequency for the period of 1901 to 2016 58 .
In the present study, the whole study area was divided into 84 subbasins using digital elevation model (DEM) to take care of spatial variability, as shown in Fig. 1 , and the CRU data was extracted for each subbasin by taking the average of CRU-grids covering that subbasin. Therefore, the CRU extracted data for each basin and observed data were used in trend analysis, and only the CRU data was used in the time series analysis for predicting temperature and precipitation.
Trend analysis
In the present study, Mann-Kendal (MK) 59 , 60 , a widely used nonparametric method, was applied to identify statistically significant trends in temperature and precipitation for the period of 1951–2015, using both observed and CRU data. For a time series x 1, x 2, x 3, …, x n , with n > 10, the MK test statistic (S), the variance of MK test statistic V(S), and the associated standard normal test statistic (Z) are calculated as below 15 , 16 , 61 :
where q represents total number of tied groups. A set of the same values in a dataset is referred to as a tied group. Each tied group is denoted by t p . The positive values of Z indicate upward (increasing) trends in time series, and the negative values show downward (decreasing) trends. Trends are then tested against some critical values ( Z 1− α ) to show that either they are statistically significant or not. For example, if | Z| > Z 1 − α , (e.g., Z 1 − α at α = 0.05); the null hypothesis of no-trend is rejected, and alternative hypothesis of significant trend is accepted.
To quantify the magnitude of detected trends, a frequently used nonparametric method, the Sen’s slope method 62 , was applied in the present study, as in Khattak et al . 6 , Burn et al . 47 , Kumar et al . 63 , and Mahmood and JIA 16 . This method is robust against outliers in a time series. Sen’s slope ( SS ) is calculated as below:
where xi is the value of data at time step i and x j at time step j . Before application of the MK test, the time series must be free of serial correlation, which can mislead the actual result of the trends. To remove serial correlation from a time series, Trend-Free Pre-Whitening (TFPW) 64 was used in the present study. This method has been used for different studies, as in Burn et al . 23 , Khattak et al . 6 , and Kumar et al . 63 , to remove serial correlation.
In the present study, the MK and SS methods were applied on 84 time series for each variable (temperature and precipitation) to explore trends and to calculate the magnitude of trends, after application of the TFPW method. The trends were detected for annual CRU temperature as well as for annual and seasonal (dry and wet) CRU and observed precipitation in the study area for the period of 1951–2015 at four significance levels (i.e., α = 0.10, 0.05, 0.01, and 0.001). Two seasons, the wet season and the dry season, are present in the all basins but have different time intervals, as described in Komble et al . 50 . According to Komble et al . 50 , precipitation for the wet season was calculated from May to October in the CLRB, from June to September in the KYRB and YENG, and from July to September in the LFB, and precipitation for the dry season was obtained from the rest of the months.
Time series analysis
In the present study, a combination of harmonic regression and spectral analysis was used to better deal with time series, such as precipitation, which has complex periodic and cyclic behavior. Therefore, the periodic component (sinusoidal component) in the time series ( Y t ) can be attained by the following model, as in Grzesica and Więcek 32 and Hintze 65 :
where µ is the mean of the time series, R is the amplitude (height or magnitude) of the wave, f shows the frequency of periodic variation, the wavelength of each wave can be obtained by dividing 2π by f , and d is the horizontal offset or phase. Changes in d shifts the beginning of the cycle. ∈ t is a white noise or random error in the time series, which causes difficulty to determine the periodic pattern in the series. t is the time period of observation ( t = 1, 2, 3…, N), where N is the total number of observations.
In practice, capturing the variation of a time series requires the sum of several different sinusoidal waves of different wavelengths (or frequencies). Further, since temperature and precipitation time series have strong trends, especially in temperature, eventually the time series are either removed by differencing (lag 1) to make it stationary, which is the requirement of this method, or the trend term ( mt ) can be added to the harmonic regression model to forecast the time series properly. By adding the trend term, as in Hintze 65 , in the harmonic regression, the final model can be written in the form of sum of k frequencies required to fit the model into a time series.
where a j (j = 1, 2, 3…k) and b j are regression coefficients to be estimated in this harmonic regression model. In this method, the central process is to determine an appropriate set of frequencies to regenerate the time series, which can explain the cyclic behavior of the time series. This is mostly done by inspecting the periodogram, which is obtained by spectral analysis (Fourier analysis). A periodogram is a plot to identify a set of frequencies (wavelengths) in the time series. In the present study, we used NCSS statistical software for spectral analysis and harmonic regression for forecasting of temperature and precipitation. From these periodogram plots, peak values are selected and used in the harmonic regression model. However, these values are just estimates; the most suitable frequencies are selected by trial and error method. More detail about spectral analysis and harmonic regression is given in 29 , 32 , 65 , 66 , 67 .
In this study, time series analysis was performed on 84 time series (37 for the CLRB, 15 for the KYRB, 17 for the YENG, and 15 for the LFB) of each climatic variable (i.e., temperature and precipitation) extracted from the CRU datasets. For detailed analysis, the CLRB was divided into 5 subbasins (Fig. 1 ): that is, the Chari River basin above Sarh (CRBAS), the Chari River basin below Sarh (CRBBS), the Bahr Salamat basin (BSB), the Ouham River basin (ORB), and the Logone River basin (LRB). The other three basins (i.e., KYRB, YENG, and LFB) were divided into the northern, southern, eastern, and western regions.
Figure 2 shows the temperature and precipitation trends calculated by MK test for the period of 1951–2015 in the study area (i.e., the CLRB, KYRB, YENG, and LFB), and the magnitude of trend lines calculated by Sen’s slope method are displayed in Figs 3 and 4 . The strength of the trend signals was divided into four categories on the basis of significance level: (1) trends at α = 0.1 (weak signal of trend), (2) trends at α = 0.05 (strong), (3) trends at α = 0.01 (very strong), and (4) trends at α = 0.001 (extremely strong), as shown in Fig. 2 .
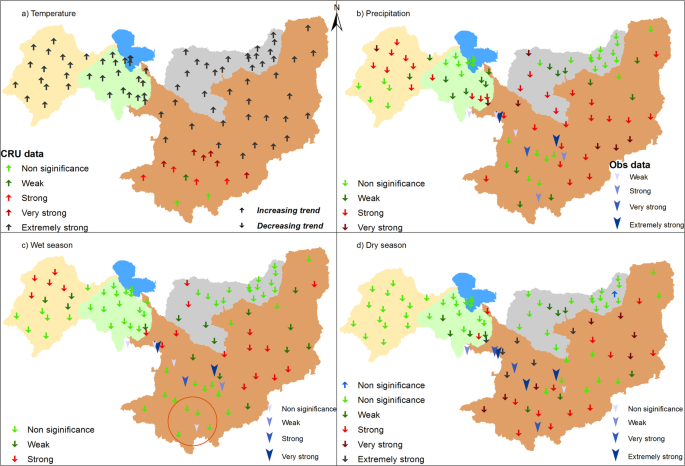
Spatial distribution of trends ( a ) annual temperature, ( b ) annual precipitation, ( c ) wet season precipitation, and ( d ) dry season precipitation in the study area, calculated from CRU and observed data for the period of 1951–2015. Trend strength is categorized as (1) trends at α = 0.1 (weak signal of trend), (2) trends at α = 0.05 (strong), (3) trends at α = 0.01 (very strong), and (4) trends at α = 0.001 (extremely strong).
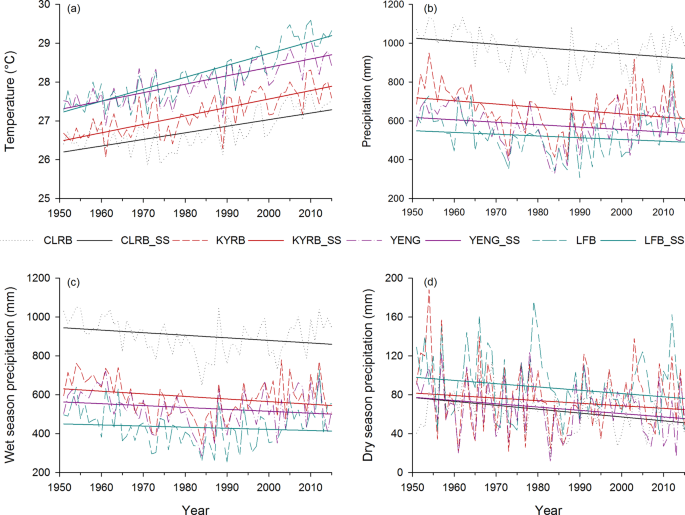
Temporal trends of ( a ) annual temperature, ( b ) annual precipitation, ( c ) wet season precipitation, and ( d ) dry season precipitation in the study area. Here, CLRB (Chari-Logone River basin), KYRB (Komadugu-Yobe River basin), YENG (Yedseram, El-Beid, Nagada, and Gubio River basins), LFB (Lake Fitri basin), and SS (Sen’s slope estimator).
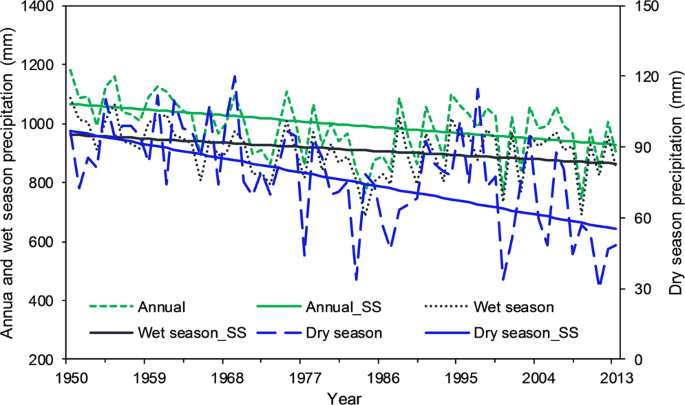
Trend lines for observed mean annual and seasonal precipitation for the period of 1950–2013 in the Chari-Logone River basin (SS Sen’s slope estimator).
Temperature trends
Figure 2a shows spatially distributed annual temperature trends obtained in the study area and displays upward trends in all time series. Among them, 84% explored extremely strong increasing trends. However, all time series in the KYB, YENG, and LFB had extremely significant rising trends. In the CLRB, approximately 96% of the time series showed increasing trends. Among them, most of the time series (approximately 64%) displayed extremely strong increasing trends, and only 2 time series, situated in the south of the CLRB, displayed nonsignificant trends. Figure 2a also shows stronger effects of global warming in the northern areas relative to the southern regions. Figure 3a shows temperature trend lines obtained with Sen’s slope method in the CLRB, KYRB, YENG and LFB, representing significant upward trends. The trend line of the LFB before 1960s was lower than YENG’s trend line, but then it increased at a faster rate than YENG’s rate of increase. This suggests that increasing rate in temperature is higher in the northeastern parts of the basin than southwestern regions. In the whole study area, an average rate of increasing temperature was estimated to be 0.022 °C yr −1 (1.43 °C per 65 years), with 0.017 °C yr −1 , 0.021 °C yr −1 , 0.022 °C yr −1 , and 0.029 °C yr −1 in the CLRB, KYRB, YENG, and LFB, respectively.
Precipitation trends
Annual trends using cru data.
Figure 2b displays spatially distributed annual precipitation trends in the study area for the period of 1951–2015, showing downward trends on all time series. Among them, 38% of total time series disclosed strong and 9% very strong evidence of decreasing precipitation over the whole study area. Nonetheless, 53% of the time series showed no or weak decreasing signals, and none of the time series showed extremely strong deceasing trends. Fifty-seven percent (21 time series out of 37) of the time series in the CLRB and 53% (8 out of 15) in the KYB were explored to have strong to very strong decrease trends. In the YENG and LFB, most of the time series (70–75%) displayed no or weak decreasing signals. Figure 3b shows precipitation trends lines calculated by Sen’s slope (SS) method in the CLRB, KYRB, YENG. All trend lines showed downward trends in all four basins, although the rate of decrease was not as strong as the temperature’s rate of increase. A decreasing rate of 1.7 mm yr −1 , 1.5 mm yr −1 , 1.22 mm yr −1 , and 1.20 mm yr −1 were explored in the CLRB, KYB, YENG, and LFB, respectively, with an average decrease of 1.4 (14 mm decade −1 ) in the whole study area. This means that, in the next 100 years, the basin will receive approximately 20–25% less precipitation relative to the current conditions if the same rate of decrease will continue in the basin. These results show that the study area is not much affected in the case of decreasing precipitation in comparison to the increasing temperature because, after the 1980s, a gradual increase in precipitation was observed (Fig. 3b ). However, a little change in precipitation may have greater impacts on water resources.
Seasonal trends using CRU data
Figure 2c,d show the trends for wet and dry seasons distributed spatially in the study area, for the period of 1951–2015. In the wet season, only 25% of the time series were observed to have strong decreasing trends, and 75% disclosed nonsignificant decreasing trends in the whole study area. Thirty-two percent of the time series in the CLRB, 33% in the KYRB, and 17% in the LFB had strong decreasing trends, whereas none of the time series in the YENG showed this trend. In the dry season, extremely strong decreasing trends were observed on 8% of the time series, very strong on 9%, and strong on 17% in the whole study area. However, 66% showed none significant decreasing signals. Most of the strong to extremely strong decreasing signals (approximately 68%) were explored in the CLRB, and almost none of the time series showed significant decreasing in other three basins, except 1 time series in the YENG basin with strong signal. In the whole CB, only 1 time series located in the LFB was found to have an increasing, though nonsignificant, signal.
The trend lines in all 4 basins for the wet and dry seasons are shown in Fig. 3c,d , indicating downward trend lines in all basins, though not highly significant. An average decreasing rate of precipitation in the wet season was estimated as 1.07 mm yr −1 (11 mm decade −1 ) in the whole basin, with the highest decline in the CLRB (1.4 mm yr −1 ) and the lowest in the LFB (0.8 mm yr −1 ), which are, nonetheless, less than the annual precipitation rates. In the case of dry season, an average decreasing rate was 0.3 mm yr −1 (3 mm decade −1 ), with the maximum (0.4 mm yr −1 ) in the CLRB (like in wet season) and minimum (0.2 mm yr −1 ) in the KYRB. In the next 100 years, if these decreasing rates prevail in the basin, then 17% of the precipitation is expected to decrease in the wet season and 36% in the dry season relative to the current conditions. Although the decreasing rate in the wet season was higher than in the dry season, the percentage decrease was higher in the dry season because the dry season receives much lower annual precipitation (70–100 mm yr −1 ) than the wet season (500–1100 mm yr −1 ). Therefore, a little change in dry season will have a greater effect.
Annual and seasonal trends using observed data
Figure 2b–d show annual and seasonal precipitation trends spatially distributed in the basin, for the period of 1951–2013, while covering only a very small part of the study area and mostly located in the CLRB. In annual precipitation, 5 of the time series (62%) displayed strong decreasing signals; among them 2 showed extremely strong, 1 very strong, and 2 strong, and the rest (3 time series) showed no significant trends. In the wet season, 37% (3 gauges) of the time series showed strong decreasing signals. However, in the case of the dry season, 75% of the gauges (6 gauges) had extremely strong signals of decreasing. The results obtained from observed stations were stronger but closely matched with the results obtained from CRU measuring points. For example, a nonsignificant trend was shown by observed stations in the wet season (Fig. 2c , circle) at the bottom of the CLRB, and the same trends were detected on the CRU measuring point near the observed precipitation station.
Figure 4 shows the trend lines of annual as well as wet and dry seasonal precipitation for 1950–2013, indicating a clear picture of decreasing precipitation in both annual and seasonal cases. This trend also showed that the dry season’s precipitation decreased at a faster rate than in the annual and wet season, which is the same as for the CRU. The rates of decrease in precipitation in the annual, wet season, and dry season were estimated to be 2.4 mm yr −1 , 1.7 mm yr −1 , and 0.62 mm yr −1 , respectively. Fourteen percent, 11%, and 43% precipitation decreased in the annual, wet and dry cases, respectively, since 1951. According to these rates, in the next 100 years, the basin will receive 22% less annual precipitation relative to the current condition, 17% less precipitation in the wet season, and 66% less precipitation in the dry season.
Predictions of temperature and precipitation
Evaluation of the fitted model.
For the evaluation of the fitted model, coefficient of determination (R 2 ), root mean square error (RMSE), error in CRU and simulated means (E m ), and error in CRU and simulated standard deviations (E std ) were calculated from CRU and simulated data (i.e., temperature and precipitation) for the period of 1951–2015, for each time series in the study area. The average values of these indicators for the whole study area are described in Table 1 . R 2 values ranged from 0.83 to 0.89 for temperature and from 0.76 to 0.89 for precipitation, and the RMSE values were 0.15–0.23 °C and 29–55 mm yr −1 , respectively, for temperature and precipitation. E m values were extremely small, 0.0001–0.0013 °C (temperature) and 0.003–0.032% (precipitation). Although the model mostly underestimated in the case of E std values, these were reasonably well inside the acceptable range, with 0.003–0.032% for temperature and 10–13% for precipitation. For more illustration, the simulated temperature and precipitation of each subbasin of the CLRB, KYRB, YENG, and LFB were plotted against the CRU to check how accurately the model follows the variations of CRU, as shown in Figs 5 – 9 . These plots showed that the fitted model captured the variations of the CRU temperature and precipitation well in each subbasin. These indicators and plots showed that the model performed extremely well to regenerate a time series during the evaluation period 1951–2015 in all basins, and thus, has capability to predict temperature and precipitation.
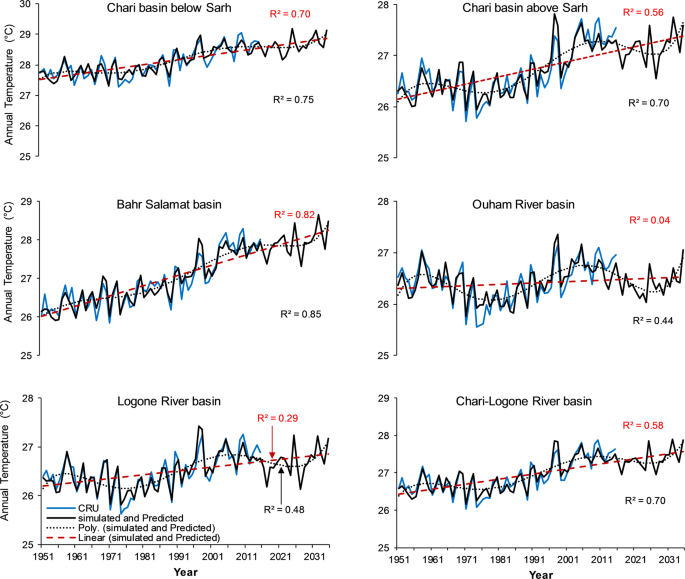
Simulated (1951–2015) and predicted (2016–2035) temperature time series against the CRU temperature in the subbasins of Chari-Logone River basin. R 2 (red) regression coefficient and R 2 (black) polynomial regression coefficient.
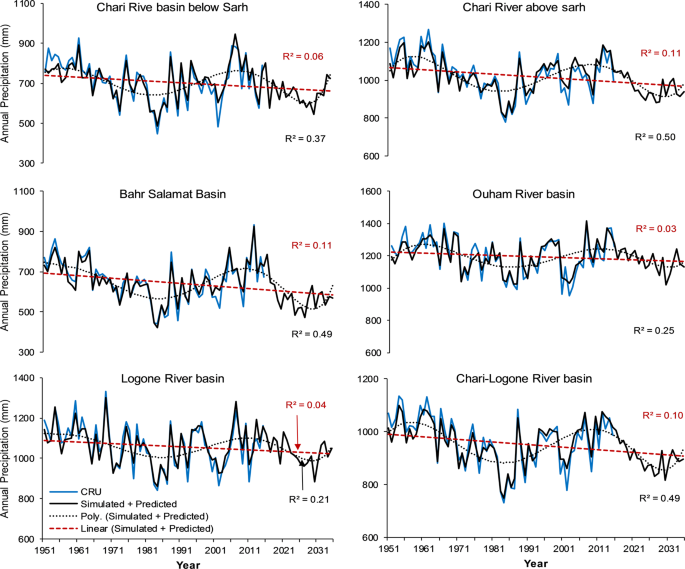
Simulated (1951–2015) and predicted (2016–2035) precipitation time series against the CRU in the subbasins of Chari-Logone River basin. R 2 (red) linear regression coefficient and R 2 (black) polynomial regression coefficient.
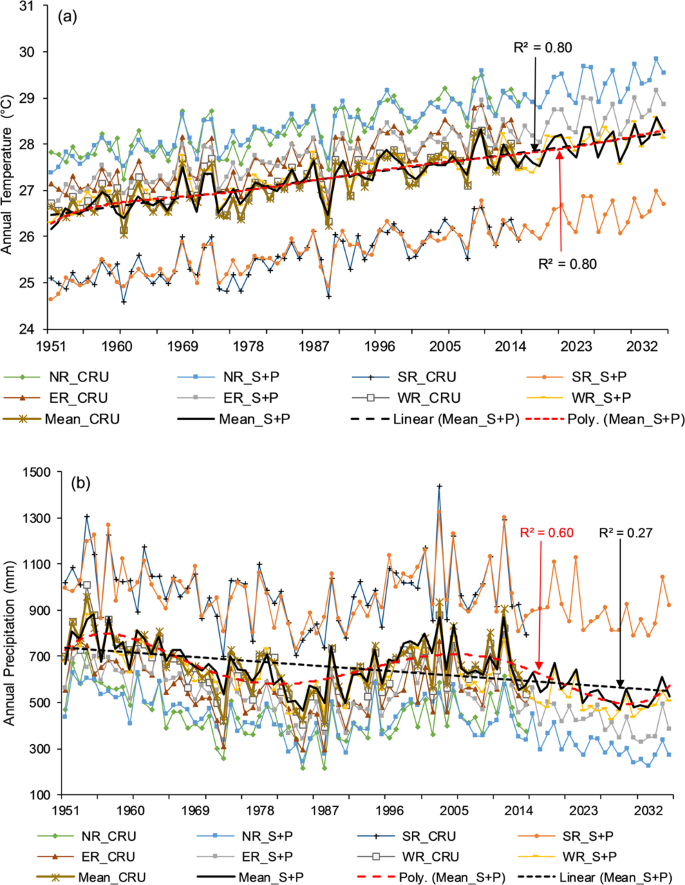
Simulated (1951–2015) and predicted (2016–2035) ( a ) temperature and ( b ) precipitation against the CRU in the Komadugu-Yobe basin. Herein, NR (Northern region), SR (Southern region), ER (Eastern region), WR (Western region), S + P (Simulated + Predicted), R 2 (red) linear regression coefficient, and R 2 (black) polynomial regression coefficient.
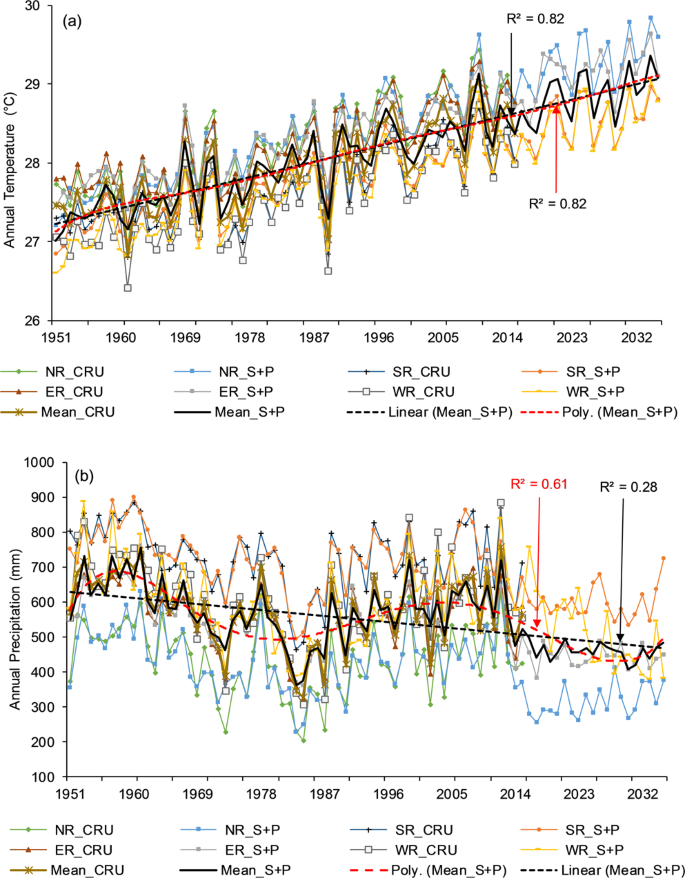
Caption of Fig. 8 for the YENG basin.
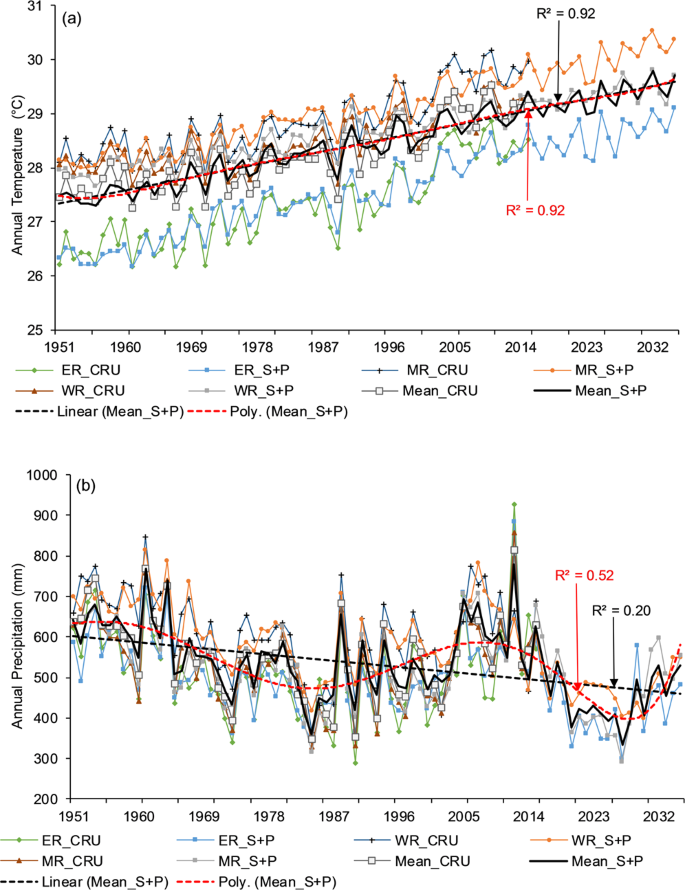
Simulated (1951–2015) and predicted (2016–2035) ( a ) temperature and ( b ) precipitation against the CRU in the Komadugu-Yobe basin. Herein, ER (Eastern region), MR (Middle region), WR (Western region), S + P (Simulated + Predicted), linear regression coefficient R2 (red), and polynomial regression coefficient R2 (black).
After a successful evaluation, the fitted model was used to generate temperature and precipitation for the next 20 (values) years (2016–2035), as shown in Figs 5 – 9 . The generated data were divided into 2016–2025 (First Decade—FD) and 2026–2035 (Second Decade—SD), and decadal changes were assessed with respect to the baseline period (1961–1990). Linear regression and polynomial (Hexic degree) trend lines were also fitted to simulate (1951–2015) and predict (2016–2035) data (Figs 5 – 9 ) to explore the linear trends and periodic cycles in the time series. We also estimated changes in 2021–2030 (2020 s) relative to 2006–2015 (the present decade—PD) because the troughs of the cycles occurred during the 2020 s (Figs 5 – 9 ), and the cyclic behavior showed that there could be more interesting results in the 2020 s relative to the present decade, especially in the CLRB.
Chari-Logone River basin
Table 2 describes the changes in temperature and precipitation in the FD and SD with respect to the baseline period and changes in the 2020 s relative to the present decade, in the CLRB. In the FD and SD, an increase in temperature of 0.12–1.28 °C and 0.34–1.63 °C, respectively, was predicted in the basin. The lowest increase was noticed in the ORB located in the South and the highest in the BSB located in the northeast in both decades. In the whole CLRB, the temperature was predicted to increase by 0.65 °C and 0.93 °C in the FD and SD, respectively, indicating clear signals of warming in the basin in the near future. Nonetheless, in the 2020 s, the LRB, CRBAS, and ORB displayed decreases in temperature relative to the PD, with −0.13, −0.16, and −0.33 °C, respectively, showing a cooler climate in the 2020 s relative to the present decade.
In the case of precipitation, all the subbasins showed negative (decreasing) changes in the next two decades relative to the baseline, with 3.9% decrease in the FD and 4.9% in the SD in the whole CLRB. The highest decrease of 12.6% (FD) and 7.8% (SD) were estimated in the BSB, and the lowest decrease of 0.1% (FD) and 3.1% (SD) were observed in the ORB and CRBBS, respectively. Some regions (i.e., BSB and CRBBS, CRBAS) showed a greater increase in precipitation in the SD than FD. However, the decrease in precipitation in the 2020 s was estimated to be more severe relative to the PD, with an average decrease of 14.4% (10–25%) in the whole CLRB.
Figures 5 and 6 shows linear regression and polynomial trend lines (Hexic degree) fitted to simulated (1951–2015) and predicted (2016–2035) temperature and precipitation in the subbasins of the CLRB. All linear regression trend lines showed increasing temperature, with strong regression coefficient ( R 2 ) except in the ORB and LRB. These lines also showed that increasing rate was faster in the northern and eastern regions (e.g., BSB, and CRBAS) than the southwestern regions such as LRB and ORB. It was also observed that R 2 were higher with lines having faster rates of increase. By polynomial lines and spectral analysis, cyclic behavior was explored in temperature, repeating every 45–49 years (Fig. 5 ) in the different subbasins; however, these showed an upward trend. R 2 for polynomial lines were even stronger than the linear regression lines (Fig. 5 ). As mentioned above, these polynomial lines also showed that 2020 s will be cooler than the PD, especially in the CRBAS, ORB, and LRB.
In the case of precipitation (Fig. 6 ), linear regression lines illustrated decreasing trends; however, R 2 were not as strong as temperature. These lines also showed that rates of decrease in precipitation were faster in the regions located in the northeast of the basin (e.g., BSB, CRBBS) than the regions in the south (e.g., ORB). By polynomial lines and spectral analysis, we explored a cyclic behavior of wet and dry periods in precipitation repeating every 20–25 years (Fig. 6 ), with a downward direction. The repeating cycle showed that next decades will be drier than the present decade.
Komadugu-Yobe River basin
The changes in temperature and precipitation for the FD and SD relative to baseline and for the 2020 s relative to the PD are described in Table 3 . Temperature was predicted to rise by 0.9–1.1 °C and 1.2–1.3 °C in the FD and SD, respectively, in different regions of the basin. Unlike the CLRB, temperature was estimated to increase in the 2020 s, with an overall increase of 0.31 °C. In contrast, precipitation was predicted to reduce by 1–21% and 5–29% in the FD and SD, respectively, in different parts of the basin. The highest increase of 1.1 °C (1.3 °C) in temperature and the highest decline of 21% (29%) in precipitation were explored in the northern region in the FD (SD). In the 2020 s, decreasing precipitation ranged between 10% and 25% relative to the PD, slightly higher than the SD. In the whole KYRB, the temperature will increase by 1.0 °C in the FD and 1.2 °C, in the SD, and precipitation will decrease by 11% and 15% in the FD and SD, respectively.
The annual simulated and predicted temperature and precipitation are plotted in Fig. 7a,b , and regression and polynomial lines were fitted to the mean data of the KYRB. Regression lines showed clear increasing trend in temperature and decreasing in precipitation, and polynomial lines fitted to temperature displayed same trend such as regression (Fig. 7a ). However, from the polynomial line and spectral analysis, we explored a repeating cycle (20–25 years) of wet and dry period in precipitation (Fig. 7b ), similar to the LCRB. Linear and polynomial models were better (with high values of R 2 ) fitted to temperature and precipitation in the KYRB than the CLRB. Unlike the CLRB, the polynomial model (Hexic order) did not showed any clear repeating cycle of hot and cold periods in the KYRB.
Table 4 describes the temperature and precipitation changes in the FD and SD with respect to the baseline and in the 2020 s relative to the PD in different regions of the YENG basin. Temperature was predicted to increase by 1.0–1.2 °C and 1.2–1.4 °C in the FD and SD, respectively. In the 2020 s, temperature will be 0.33 °C higher than in the present decade. On the other hand, precipitation was predicted to decrease by 4–24% in the FD and 13–23% in the SD, and in the 2020 s, the reduction in precipitation was even higher, ranging between 19% and 31% relative to the PD. The most affected region by increase in temperature and decrease in precipitation was the northern region in the basin, same as the CLRB and KYRB. In the whole basin, the temperature will increase by 1.1 °C in the FD and 1.3 °C in the SD, and precipitation will decrease by 14% in the FD and 16% in the SD. The simulated/predicted annual temperature and precipitation are shown in Fig. 8(a,b) , along with the linear and polynomial lines. Strong linear and polynomial trends (R 2 = 0.81) in temperature displayed a definite increase but no periodic behavior such as that observed in the CLRB (Fig. 8a ). Both linear and polynomial models showed downward lines in the case of precipitation, and a similar cyclic behavior of the wet and dry period moving downward (Fig. 8b ) was observed in the basin by spectral and polynomial analysis.
Lake Fitri basin
Table 5 describes the temperature and precipitation changes in the FD (SD) and 2020 s relative to the baseline period and the PD, respectively, in different regions of the LFB. In the whole basin, temperature was predicted to increase by 1.24 °C in the FD and 1.6 °C in the SD. Unlike the CLRB, the basin will be warmer in the 2020 s, with 0.34 °C higher temperature than the present decade. On the other hand, precipitation was expected to decrease by 21% and 8% in the FD and SD, respectively, in the whole basin. The highest decrease of 21% and 16% was estimated in the middle and western parts of the basin in the FD and SD, respectively. Unlike the other three basins, the reduction of precipitation was lower in the SD than in the FD. This means precipitation will start increasing after the FD of drought. In the 2020 s, the decline in precipitation ranged between 29% and 37% relative to the PD, which was much higher than other basins. The eastern parts of the basin will be warmer than the other parts. Figure 9 shows an annual time series of simulated/predicted temperature and precipitation in the LFB along with the regression and polynomial lines. The linear and polynomial lines, with strong R 2 , showed a definite increase in temperature (Fig. 9a ), and linear and polynomial lines fitted to precipitation indicated a decline, with cyclic behavior, showing wet and dry spells in the basin (Fig. 9b ). Figure 9a also shows that the temperature range in the basin has reduced over time. This means that relatively cold regions (eastern region) are warming more rapidly than the warmer regions in the basin. In comparison with the other three basins (i.e., CLRB, KYRB, and YENG), the LFB will be warmer than other basins in both FD and SD, and a reduction in precipitation will also be higher than other basin in the FD. However, in the SD, the highest reduction in precipitation was predicted in the Komadugu-Yobe and YENG basins.
Temperature and precipitation trends
In the whole study area, temperature showed an extremely strong increasing trend, with an average rate of 0.022 °C yr −1 (1.43 °C 65 yr −1 ), which is even much higher than the global average temperature rate of 0.0074 °C yr −1 (0.74 °C 100 yr −1 ) 37 . Sarr 42 and Funk et al . 37 also examined the faster rate of increasing temperature (approximately 0.023 °C yr −1 ) than the global warming in the West Africa and Chad, respectively, and Collins 68 , over Africa, with an increasing rate of 0.016 °C yr −1 C. This mean that if the temperature follows the same rate in the basin, then in the next 100 years, this region may face approximately 2–3 °C hotter climate relative to the current condition. A similar increase in temperature (2.6–4.8 °C) has been reported by IPCC 4 for the globe, 3–4 °C rise by Collins 68 over Africa, and 1.6–6 °C increase by Sylla et al . 44 over West Africa at the end of the 21 st century. Extremely strong signals of increasing temperature found in this study suggests that the LCB is highly vulnerable to global warming. According to the Fifth Assessment Report of the IPCC, the increasing concentrations of greenhouse gases in the atmosphere has been the dominant cause of observed warming since 1950, with 95% confidence 4 . Africa is the most affected continent due to the global warming, although it contributes least to the global warming, with an average emission of greenhouse gases of 1 metric ton 69 . This increasing temperature in the basin can cause stress on water resources, a reduction in crop productivity, reduction in fishery, more floods and droughts, and increase in vector- and waterborne diseases. However, the LCB already faced a devastating droughts during the 1970s and 1980s, when the lake not only faced high shrinkage but also split into two parts 13 .
This study showed that annual and seasonal precipitations have reduced in the whole study area for the period of the 1951–2015, with moderate downward trends obtained using CRU precipitation and relatively strong downward trends calculated by observed precipitation. Similar results have been explored by Niel et al . 70 in the LCB, Nkiaka et al . 38 in the Logone River basin of the LCB, Funk et al . 37 in Chad, Ifabiyi and Ojoye 71 over the Sudano-Sahelian Ecological Zone of Nigeria, Sarr 42 over West Africa, Conway et al . 53 over sub-Saharan Africa regions, and Ali and Lebel 72 over the Sahel region, with study periods starting before or from 1960s. The study by Niel et al . 70 in the LCB used those stations which are located around LC and not covering the whole study area spatially. These decreasing trends in the LCB could be, to the great extent, due to the Sahel droughts in the 1970s and 1980s, which resulted in devastating impacts on the local population, biodiversity, agriculture, and pastoral activities 38 , 54 . Sarr 42 attributed the decreasing trends to a significant drop in rainy days and a slight increase in dry spells during 1960–1990, as well as to the decline in precipitation intensity in the region. Epule et al . 73 reviewed comprehensively the causes and effects of the Sahel droughts and explored the four major possible causes of decreased precipitation in the region: human-induced climate change, dust feedbacks, changes in sea surface temperature, and land and vegetation degradation. Among them, anthropogenic climate change is considered the most influencing factor because it also controls the other three factors. They also revealed some serious implications of the decrease in precipitation on forest and food in the region, especially in the case of agriculture, which provides livelihood to approximately 60% of the population in the LCB 48 and 80% in most African countries 74 . Dilley et al . 75 revealed that severe food shortages were recorded during the 1982 drought in 27 Sahelian countries with famines in Chad, Sudan, and Ethiopia. On the other hand, Dickson and Steve 76 reported a decrease in per capita food and drop in food self-sufficiency ratio from 98% in the 1960s to 86% in the mid-1980s due to decreasing precipitation in the region.
However, some studies such as Okonkwo et al . 39 , Adeyeri et al . 36 , Ndehedehe et al . 77 and Zhu et al . 78 showed an increase in the precipitation in the LCB, and other studies such as Sylla et al . 44 , Nicholson 79 , Ibrahim et al . 80 , and Mahé and Paturel 81 also reported, to some extent, similar kind of findings around the LCB, especially over the Sahel belt, but these studies used a study period later than 1980s. It can also be observed in Figs 3 and 4 that precipitation started increasing after the 1980s, especially in the case of annual amounts and the wet season. Another study by Lebel and Ali 82 indicated that precipitation over the central Sahel increased by approximately 10% for the period of 1990–2007 relative to 1970–1989. Ibrahim et al . 80 have explored not only the increase in the total precipitation but also the increase in the number of rainy days in the last two decades, resulting in the partial recovery of precipitation. This increase could be due to the partial recovery of precipitation during the 1990s in the region, which can be the result of warming over the northern Atlantic Ocean, which draws rains during the wet season in the region 36 . In addition, this increase can be attributed to the increased anthropogenic greenhouse gases and aerosol emission in the atmosphere 54 . Although an increase in precipitation, in general, could have beneficial impacts on agriculture, biodiversity, and pastoral activities, it could also create negative socioeconomic problems, in the form of floods. Floods could also accelerate the risk of water-related diseases where better flood management systems are not present, especially in poor countries. Therefore, the studies with the study period starting before 1980s to the present showed a decrease in precipitation, and the studies with the study period starting after 1980s displayed an increase in precipitation. Spatial analysis of temperature and precipitation is very important to identify the regions at high risk and vulnerable to floods and droughts and to enable local authorities to pay attention on these regions by developing more suitable water management practices and measures.
Precipitation trends obtained by observed data showed a stronger decrease than obtained by the CRU data. This could be because of very limited collected station data (only 8 stations) and cover a very small part of the LCB. The main dataset (CRU) used in the present study also has some uncertainties because this dataset is prepared by interpolating available observed data in the region, which is so scarce. Bastola and François 83 , who evaluated different satellite and the CRU precipitation with gauge data in the Lake LCB, concluded that these datasets overestimate rainfall in the northern semiarid regions of the basin and underestimate it in the humid regions located in the south. They also reported that the number of rain gauges used in the derivation of the CRU dataset has declined markedly from 150 to 75 since 1990 due to financial problem. For further studies, it is suggested to include all stations available in the basin and other global datasets such as GPCC and NCEP to use different trend analysis techniques and to use different time periods to take care of all possible uncertainties. The satellite datasets can also be of good option for the recent trends since the 1990s.
Temperature and precipitations predictions
This present study suggests that temperature in the basin will increase in the next two decades with respect to the baseline period (1961–1990). The results are well supported by Sylla et al . 44 , who used the RCM multimodel approach to project temperature over the Sahel region, under RCP4.5 and RCP8.5. They predicted an average increase of 1.8 °C until 2035 relative to 1976–2005. According to IPCC 84 , global warming would be more intense in Africa. Different studies such as Buontempo 40 , Francois et al . 41 , Niang et al . 85 , Sarr 42 , and Serdeczny et al . 10 have projected an increase in temperature in different parts of Africa, especially over the Sahel.
In some parts of the CLRB, temperature was predicted to decrease in the 2020 s with respect to the present decade (2006–2015). This might be due to the frequent occurrence of forests in these regions 8 . These regions are also included in highly protected natural resource areas in the basin 50 . This result also may be due to assessing changes against a very short period and very recent period (2006–2015). However, we did not find any study showing a decrease in temperature in the future in the region because most studies show temperature anomalies relative to the long-term baseline period (e.g., 1961–1990 or 1971–2000) and not with the very short and recent period that we used. Therefore, further study is needed in the region using the most advanced techniques and tools, such as GCMs and RCMs, with multimodel approaches to confirm these results.
In the case of precipitation, according to the results, the LCB will receive less precipitation in the next two decades relative to the baseline period (1961–1990). This means that in the next 20 years, the basin is going to face more droughts. According to the results, these droughts could be stronger than in the 1970s and 1980s because our baseline period also includes the driest period (1970–1990). Sultan et al . 43 projected a decrease in annual precipitation in the Western Sahel but an increase in the Central Sahel during 2031–2060 under RCP8.5. Vizy et al . 46 showed an increase in summer precipitation during 1941–1960 over the Sahel region, using RCM under RCP8.5, and using RCMs, Sylla et al . 45 projected, for the last two decades of the 21 st century, drier conditions over the Sahel region and wetter conditions over the orographic regions According to Niang et al . 85 , annual precipitation is likely to increase in Central and eastern Africa in the beginning of the mid-21 st century, while it is very likely to decrease over the Mediterranean areas of northern Africa in the mid and late 21 st century with respect to 1986–2005 under RCP8.5. If we examine Figs 6 , 7b , 8b and 9b , all figures show an increase in precipitation at the end of 2035 and display a wet and dry cycle, repeating in 20–25 years. Therefore, if we follow this cycle, then after 2035, a wet period may occur for 2035–2060. The above-mentioned studies also showed an increase in precipitation between 2031 and 2060 in the Sahel region, covering the LCB. However, more uncertainties are associated with precipitation projections than temperature projections 86 , and precipitation projections exhibit higher seasonal and spatial dependence than projections of temperature 87 . Future projection of precipitation are quite uncertain because some models predict a significance decrease, some a significant increase, and others no significance change 42 . Although more uncertainty lies in predicting precipitation with time series analysis because precipitation is a heterogeneous variable, the uncertainty cannot be removed completely even by using the most advanced tools such as RCMs and GCMs. Nonetheless, time series analyses are very fast, require less computation, and perform reasonably well.
Conclusions
In the present study, first, a combination of linear regression, polynomial model, Sens’ slope estimator, and Mann-Kendal test was used to explore temporospatial trends and climate variability in the most active part of the LCB (i.e., the Chari-Logone, Komadugu-Yobe, YENG, and Lake Fitri basins), using observed and CRU annual temperature and precipitation for the period of 1951–2015. Second, temperature and precipitation were predicted by a combination of spectral analysis and harmonic regression for the next 20 years (2016–2035), and decadal changes (2016–2025 and 2026–2035) were obtained with respect to the baseline period (1960–1990) and changes in the 2020 s (2021–2030) with respect to the present decade (PD) (2006–2015). The following are the key findings obtained from the present study:
Temperature has increased in the study area, with an increasing rate of 0.22 °C decade −1 . Most increase in temperature was observed in the LFB.
Annual precipitation has decreased in the study area, with a decreasing rate of 14 mm decade −1 . Nonetheless, most time series showed weak and nonsignificant decreasing trends. In the CLRB, which contributes 90% to Lake Chad, decreasing trends were even stronger than for the other basins.
Temperature was predicted to increase in the Lake Chad basin by 0.65 °C and 1.6 °C in the first decade—FD (2016–2025) and second decade—SD (2026–2035), respectively, with respect to 1960–1990. However, unexpectedly, in the CLRB in the 2020 s, temperature was predicted to decrease relative to the PD in some parts of the CLRB, showing cooler climate in the 2020 s. This result might be because these regions are located in highly protected natural resources areas and have dense forests.
Precipitation was predicted to decrease in all basin, with 4–21% decrease in the FD and 5–16% in the SD relative to 1961–1990. In the 2020 s, precipitation will be decreased by 14–33% relative to the PD in the study area.
Cyclic behavior was explored in temperature, repeating every 45–49 years, but only in the CLRB, and a cycle of wet and dry periods, repeating 20–25 years, was explored in precipitation in all basins.
These findings clearly indicate that the Lake Chad basin is highly sensitive to perturbed natural climate, and thus, the water resources of the basin could be highly vulnerable to climate change.
The key findings of the present study may be helpful to water resource managers for planning and managing water resources on a seasonal and annual basis and to policymakers for advising some suitable adaptation and mitigation policies to cope with anticipated climate variability and climate change. However, further studies should include all available observed station data, different trend analysis techniques, different time steps (e.g., monthly and decadal), and should consider a study period before and after the major droughts (e.g., the 1970s) in the Lake Chad basin to cover possible uncertainties. On the other hand, the most advanced tools, such as RCMs/GCMs and multimodel and multi scenarios approaches, could be useful to take care of uncertainties related to prediction of temperature and precipitation. In addition, further study can be done using different time series techniques to predict monthly and seasonal temperature and precipitation, which would be useful for farmers. Furthermore, a standardized precipitation index (SPI) and other relevant indices can be used to monitor flood and droughts in the Lake Chad basin, especially in the Chari-Logone River basin.
Mahmood, R. & Babel, M. S. Evaluation of SDSM developed by annual and monthly sub-models for downscaling temperature and precipitation in the Jhelum basin, Pakistan and India. Theor Appl Climatol 113 , 27–44, https://doi.org/10.1007/s00704-012-0765-0 (2013).
Article ADS Google Scholar
Huang, J. et al . Estimation of future precipitation change in the Yangtze River basin by using statistical downscaling method. Stoch. Environ. Res. Risk Assess. 25 , 781–792, https://doi.org/10.1007/s00477-010-0441-9 (2010).
Article Google Scholar
Chu, J., Xia, J., Xu, C. Y. & Singh, V. Statistical downscaling of daily mean temperature, pan evaporation and precipitation for climate change scenarios in Haihe River, China. Theor Appl Climatol 99 , 149–161, https://doi.org/10.1007/s00704-009-0129-6 (2010).
IPCC. Climate Change 2013: The Physical Science Basis. Contribution of Working Group I to the Fifth Assessment Report of the Intergovern-mental Panel on Climate Change 1535 (Cambridge University Press, Cambridge, United Kingdom and New York, NY, USA, 2013).
Syed, T. H., Famiglietti, J. S., Chambers, D. P., Willis, J. K. & Hilburn, K. Satellite-based global-ocean mass balance estimates of interannual variability and emerging trends in continental freshwater discharge. Proceedings of the National Academy of Sciences 107 , 17916–17921, https://doi.org/10.1073/pnas.1003292107 (2010).
Khattak, M. S., Babel, M. S. & Sharif, M. Hydro-meteorological trends in the upper Indus River basin in Pakistan. Clim Res. 46 , 103–119, https://doi.org/10.3354/cr00957 (2011).
IPCC. Summary for policymakers. In: Climate change 2014: Impacts, adaptation, and vulnerability. Part A: Global and sectoral aspects. Contribution of Working Group II to the Fifth Assessment Report of the Intergovermental Panel on Climate Change 1–32 (Cambridge, UK and New York, 2014).
Mahmood, R. & Jia, S. Analysis of causes of decreasing inflow to the Lake Chad due to climate variability and human activities. Hydrol. Earth Syst. Sci. Discuss. 2018 , 1–42, https://doi.org/10.5194/hess-2018-139 (2018).
UNFCCC. Climate change: impacts, vulnerabilities and adaptation in developing countries. 68 (Bonn, Germany, 2010).
Serdeczny, O. et al . Climate change impacts in Sub-Saharan Africa: from physical changes to their social repercussions. Regional Environmental Change 17 , 1585–1600, https://doi.org/10.1007/s10113-015-0910-2 (2017).
Coe, M. T. & Foley, J. A. Human and natural impacts on the water resources of the Lake Chad basin. J. Geophys. Res-Atmos. 106 , 3349–3356, https://doi.org/10.1029/2000JD900587 (2001).
Gao, H., Bohn, T. J., Podest, E., McDonald, K. C. & Lettenmaier, D. P. On the causes of the shrinking of Lake Chad. Environ. Res. Lett. 6 , 7, https://doi.org/10.1088/1748-9326/6/3/034021 (2011).
Lemoalle, J., Bader, J.-C., Leblanc, M. & Sedick, A. Recent changes in Lake Chad: Observations, simulations and management options (1973–2011). Global Planet. Change 80-81 , 247–254, https://doi.org/10.1016/j.gloplacha.2011.07.004 (2012).
Tarbuck, E. J. & Lutgens, F. K. In Earth Science (Pearson, 2016).
Feng, G. et al . Trend analysis and forecast of precipitation, reference evapotranspiration, and rainfall deficit in the Blackland Prairie of Eastern Mississippi. J. Appl. Meteorol. Clim. 55 , 1425–1439, https://doi.org/10.1175/JAMC-D-15-0265.1 (2016).
Mahmood, R. & Jia, S. Spatial and temporal hydro-climatic trends in the transboundary Jhelum River basin. Journal of Water and Climate Change 8 , 423–440, https://doi.org/10.2166/wcc.2017.005 (2017).
Nury, A. H., Koch, M. & Alam, M. J. B. In 4th International Conference on Environmental Aspects of Bangladesh. 4 (BENJapan).
Sunday, R. K. M., Masih, I., Werner, M. & van der Zaag, P. Streamflow forecasting for operational water management in the Incomati River Basin, Southern Africa. Physics and Chemistry of the Earth, Parts A/B/C 72–75 , 1–12, https://doi.org/10.1016/j.pce.2014.09.002 (2014).
Teresa, R. et al . Using Time Series Analysis to support the Water Resources Management in the Upper Basin of the Suquía River. Pinnacle Environmental & Earth Science 2 , 1–10 (2015).
Esterby, S. R. Review of methods for the detection and estimation of trends with emphasis on water quality applications. Hydrological Processes 10 , 127–149, 10.1002/(SICI)1099-1085(199602)10:2<127::AID-HYP354>3.0.CO;2-8 (1996).
Zhang, Q., Liu, C., Xu, C.-Y., Xu, Y. & Jiang, T. Observed trends of annual maximum water level and streamflow during past 130 years in the Yangtze River basin, China. J. Hydrol. 324 , 255–265, https://doi.org/10.1016/j.jhydrol.2005.09.023 (2006).
Sonali, P. & Nagesh, K. D. Review of trend detection methods and their application to detect temperature changes in India. J. Hydrol. 476 , 212–227, https://doi.org/10.1016/j.jhydrol.2012.10.034 (2013).
Burn, D. H., Cunderlik, J. M. & Pietroniro, A. Hydrological trends and variability in the Liard River basin / Tendances hydrologiques et variabilité dans le basin de la rivière Liard. Hydrological Sciences Journal 49 , 53–67, https://doi.org/10.1623/hysj.49.1.53.53994 (2004).
Fu, G., Barber, M. E. & Chen, S. Hydro-climatic variability and trends in Washington State for the last 50 years. Hydrological Processes 24 , 866–878, https://doi.org/10.1002/hyp.7527 (2010).
Oyerinde, G. et al . Hydro-climatic changes in the Niger basin and consistency of local perceptions. Regional Environmental Change, 1–11, https://doi.org/10.1007/s10113-014-0716-7 (2014).
Tekleab, S., Mohamed, Y. & Uhlenbrook, S. Hydro-climatic trends in the Abay/Upper Blue Nile basin, Ethiopia. Physics and Chemistry of the Earth, Parts A/B/C 61–62 , 32–42, https://doi.org/10.1016/j.pce.2013.04.017 (2013).
Wang, H. et al . Hydro-climatic trends in the last 50 years in the lower reach of the Shiyang River Basin, NW China. CATENA 95 , 33–41, https://doi.org/10.1016/j.catena.2012.03.003 (2012).
Soltani, S., Modarres, R. & Eslamian, S. S. The use of time series modeling for the determination of rainfall climates of Iran. International Journal of Climatology 27 , 819–829, https://doi.org/10.1002/joc.1427 (2006).
Yang, L. & Lu, W. In International Symposium on Water Resource and Environmental Protection. 3063–3065 (2011).
Prins, J. In Engineering Statitistics Handbook (NIST/SEMATECH e-Handbook of Statistical Methods) Ch. 6 (2012).
Hill, T., Lewicki, P. & Lewicki, P. Statistics: Methods and Applications: a Comprehensive Reference for Science, Industry, and Data Mining (StatSoft, 2006).
Grzesica, D. & Więcek, P. Advanced Forecasting Methods Based on Spectral Analysis. Procedia. Engineer. 161 , 253–258, https://doi.org/10.1016/j.proeng.2016.08.546 (2016).
Buttkus, B. Spectral Analysis and Filter Theory in Applied Geophysics. Vol. 1 (Springer, 2000).
Chattopadhyay, A. K. & Chattopadhyay, T. Statistical Methods for Astronomical Data Analysis. Vol. 3 (Springer-Verlag New York, 2014).
Ehrendorfer, M. Spectral Numerical Weather Prediction Models. (Society for Industrial and Applied Mathematics, 2011).
Adeyeri, O. E., Lamptey, B. L., Lawin, A. E. & Sanda, I. S. Spatio-temporal precipitation trend and homogeneity analysis in Komadugu-Yobe basin, Lake Chad region. J. Climatol. Weather Forecasting 5 , 12, https://doi.org/10.4172/2332-2594.1000214 (2017).
Funk, C. C., Rowland, J., Adoum, A., Eilerts, G. & White, L. A climate trend analysis of Chad. Report No. 2012–3070, (Reston, VA, 2012).
Nkiaka, E., Nawaz, N. R. & Lovett, J. C. Analysis of rainfall variability in the Logone catchment, Lake Chad basin. Int. J. Climatol. 37 , 3553–3564, https://doi.org/10.1002/joc.4936 (2017).
Okonkwo, C., Demoz, B. & Gebremariam, S. Characteristics of Lake Chad level variability and links to ENSO, precipitation, and River discharge. Sci. World J. 2014 , 13, https://doi.org/10.1155/2014/145893 (2014).
Buontempo, C. Sahelian climate: part, current, projections. 20 (Met Office Hadley Centre, Devon, United Kingdom, 2010).
Francois, E. et al . Projections of rapidly rising surface temperatures over Africa under low mitigation. Environmental Research Letters 10 , 085004, https://doi.org/10.1088/1748-9326/10/8/085004 (2015).
Article CAS Google Scholar
Sarr, B. Present and future climate change in the semi-arid region of West Africa: a crucial input for practical adaptation in agriculture. Atmospheric Science Letters 13 , 108–112, https://doi.org/10.1002/asl.368 (2012).
Sultan, B. et al . Robust features of future climate change impacts on sorghum yields in West Africa. Environmental Research Letters 9 , 104006, https://doi.org/10.1088/1748-9326/9/10/104006 (2014).
Article ADS CAS Google Scholar
Sylla, M., Nikiema, M., Gibba, P., Kebe, I. & Klutse, N. A. B. Climate Change over West Africa: Recent Trends and Future Projections (2016).
Sylla, M. B., Gaye, A. T., Jenkins, G. S., Pal, J. S. & Giorgi, F. Consistency of projected drought over the Sahel with changes in the monsoon circulation and extremes in a regional climate model projections. Journal of Geophysical Research: Atmospheres 115 , https://doi.org/10.1029/2009JD012983 (2010).
Vizy, E. K., Cook, K. H., Crétat, J. & Neupane, N. Projections of a Wetter Sahel in the Twenty-First Century from Global and Regional Models. Journal of Climate 26 , 4664–4687, https://doi.org/10.1175/JCLI-D-12-00533.1 (2013).
Buma, W., Lee, S.-I. & Seo, J. Hydrological evaluation of Lake Chad Basin using space borne and hydrological model observations. Water 8 , 205, https://doi.org/10.3390/w8050205 (2016).
UNEP. Africa’s Lakes: Atlas of our changing environment 89 (Nairobi, Kenya, 2006).
Frenken, K. Irrigation potential in Africa: A basin approach. 177 (1997).
Komble, M. D., Kostoingue, B. & Hamit, A. Report on the state of the Lake Chad Basin ecosystem. 236 (Bonn, Germany, 2016).
Lemoalle, J. & Magrin, G. Le développement du lac Tchad/Development of Lake Chad: Situation actuelle et futurs possibles/Current Situation and Possible Outcomes. 216 (IRD Editions, 2014).
Okonkwo, C., Demoz, B. & Onyeukwu, K. Characteristics of drought indices and rainfall in Lake Chad Basin. International Journal of Remote Sensing 34 , 7945–7961, https://doi.org/10.1080/01431161.2013.827813 (2013).
Conway, D. et al . Rainfall and Water Resources Variability in Sub-Saharan Africa during the Twentieth Century. Journal of Hydrometeorology 10 , 41–59, https://doi.org/10.1175/2008JHM1004.1 (2009).
Dong, B. & Sutton, R. Dominant role of greenhouse-gas forcing in the recovery of Sahel rainfall. Nature Climate Change 5 , 757, https://doi.org/10.1038/nclimate2664 (2015).
Chiyuan, M. et al . Assessment of CMIP5 climate models and projected temperature changes over Northern Eurasia. Environ. Res. Lett. 9 , 055007, https://doi.org/10.1088/1748-9326/9/5/055007 (2014).
McMahon, T. A., Peel, M. C. & Karoly, D. J. Assessment of precipitation and temperature data from CMIP3 global climate models for hydrologic simulation. Hydrol. Earth Syst. Sci . 19 , https://doi.org/10.5194/hess-19-361-2015 (2015).
Smiatek, G., Kunstmann, H., Knoche, R. & Marx, A. Precipitation and temperature statistics in high-resolution regional climate models: Evaluation for the European Alps. J. Geophys. Res-Atmos. 114 , n/a-n/a, https://doi.org/10.1029/2008JD011353 (2009).
Harris, I., Jones, P. D., Osborn, T. J. & Lister, D. H. Updated high-resolution grids of monthly climatic observations – the CRU TS3.10 Dataset. Int. J. Climatol. 34 , 623–642, https://doi.org/10.1002/joc.3711 (2014).
Mann, H. B. Nonparametric tests against trend. Econometrica 13 , 245–259, https://doi.org/10.2307/1907187 (1945).
Article MathSciNet MATH Google Scholar
Kendall, M. G. Rank correlation methods (Charles Griffin). 272 (Oxford University Press, 1975).
Hu, Z., Wang, L., Wang, Z., Hong, Y. & Zheng, H. Quantitative assessment of climate and human impacts on surface water resources in a typical semi-arid watershed in the middle reaches of the Yellow River from 1985 to 2006. Int. J. Climatol. 35 , 97–113, https://doi.org/10.1002/joc.3965 (2015).
Sen, P. K. Estimates of the regression coefficient based on Kendall’s Tau. J. Am. Stat. Assoc. 63 , 1379–1389, https://doi.org/10.2307/2285891 (1968).
Kumar, S., Merwade, V., Kam, J. & Thurner, K. Streamflow trends in Indiana: Effects of long term persistence, precipitation and subsurface drains. J. Hydrol. 374 , 171–183, https://doi.org/10.1016/j.jhydrol.2009.06.012 (2009).
Yue, S., Pilon, P., Phinney, B. & Cavadias, G. The influence of autocorrelation on the ability to detect trend in hydrological series. Hydrological Processes 16 , 1807–1829, https://doi.org/10.1002/hyp.1095 (2002).
Hintze, J. L. User’s guid I: NCSS Statistical Sysstem. 629 (NCSS, Kaysville, Utah, USA, 2007).
Balıbey, M. & Türkyılmaz, S. A time series approach for precipitation in Turkey. Gazi University Journalal of Sciences 28 , 549–559 (2015).
Google Scholar
Kozłowski, E., Kowalska, B., Kowalski, D. & Mazurkiewicz, D. Water demand forecasting by trend and harmonic analysis. Arch. Civ. Mech. Eng. 18 , 140–148, https://doi.org/10.1016/j.acme.2017.05.006 (2018).
Collins, J. M. Temperature Variability over Africa. Journal of Climate 24 , 3649–3666, https://doi.org/10.1175/2011JCLI3753.1 (2011).
Fields, S. Continental divide: why Africa’s climate change burden is greater. Environ Health Perspect 113 , A534–A537, https://doi.org/10.1289/ehp.113-a534 (2005).
Article PubMed PubMed Central Google Scholar
Niel, H., Leduc, C. & Dieulin, C. Caractérisation de la Variabilité Spatiale et Temporelle des Précipitations Annuelles sur le Bassin du Lac Tchad au Cours du 20ème Siècle/Spatial and Temporal Variability of Annual Rainfall in the Lake Chad Basin During the 20th Century. Hydrological Sciences Journal 50 , null–243, https://doi.org/10.1623/hysj.50.2.223.61800 (2005).
Ifabiyi, I. P. & Ojoye, S. Rainfall Trends in the Sudano-Sahelian Ecological Zone of Nigeria. 2 , 194–202, https://doi.org/10.5539/esr.v2n2p194 (2013).
Ali, A. & Lebel, T. The Sahelian standardized rainfall index revisited. International Journal of Climatology 29 , 1705–1714, https://doi.org/10.1002/joc.1832 (2008).
Epule, T. E., Peng, C., Lepage, L. & Chen, Z. The causes, effects and challenges of Sahelian droughts: a critical review. Regional Environmental Change 14 , 145–156, https://doi.org/10.1007/s10113-013-0473-z (2014).
Epule, T. E., Peng, C., Lepage, L. & Chen, Z. Rainfall and Deforestation Dilemma for Cereal Production in the Sudano-Sahel of Cameroon. Journal of Agricultural Science 4 , https://doi.org/10.5539/jas.v4n2p1 (2012).
Dilley, M. et al . Natural disaster hotspots: A global risk analysis (English). 148 (World Bank, Washington, DC, 2005).
Book Google Scholar
Dickson, M. N. & Steve, W. Household food insecurity in sub‐Saharan Africa: lessons from Kenya. British Food Journal 99 , 249–262, https://doi.org/10.1108/00070709710179363 (1997).
Ndehedehe, C. E., Agutu, N. O., Okwuashi, O. & Ferreira, V. G. Spatio-temporal variability of droughts and terrestrial water storage over Lake Chad Basin using independent component analysis. J. Hydrol. 540 , 106–128, https://doi.org/10.1016/j.jhydrol.2016.05.068 (2016).
Zhu, W., Yan, J. & Jia, S. Monitoring recent fluctuations of the Southern Pool of Lake Chad using multiple remote sensing data: Implications for water bnalance analysis. Remote Sensing 9 , https://doi.org/10.3390/rs9101032 (2017).
Nicholson, S. On the question of the “recovery” of the rains in the West African Sahel. Journal of Arid Environments 63 , 615–641, https://doi.org/10.1016/j.jaridenv.2005.03.004 (2005).
Ibrahim, B., Karambiri, H., Polcher, J., Yacouba, H. & Ribstein, P. Changes in rainfall regime over Burkina Faso under the climate change conditions simulated by 5 regional climate models. Climate Dynamics 42 , 1363–1381, https://doi.org/10.1007/s00382-013-1837-2 (2014).
Mahé, G. & Paturel, J.-E. 1896–2006 Sahelian annual rainfall variability and runoff increase of Sahelian Rivers. Comptes Rendus Geoscience 341 , 538–546, https://doi.org/10.1016/j.crte.2009.05.002 (2009).
Lebel, T. & Ali, A. Recent trends in the Central and Western Sahel rainfall regime (1990–2007). Journal of Hydrology 375 , 52–64, https://doi.org/10.1016/j.jhydrol.2008.11.030 (2009).
Bastola, S. & François, D. Temporal extension of meteorological records for hydrological modelling of Lake Chad Basin (Africa) using satellite rainfall data and reanalysis datasets. Meteorological Applications 19 , 54–70, https://doi.org/10.1002/met.257 (2012).
IPCC. Climate Change 2007: Impacts, Adaptation and Vulnerability. Contribution of Working Group II to the Fourth Assessment Report of the Intergovernmental Panel on Climate Change. 976 (Cambridge, UK, 2007).
Niang, I. et al . In Climate Change 2014: Impacts, Adaptation, and Vulnerability. Part B: Regional Aspects. Contribution of Working Group II to the Fifth Assessment Report of the Intergovernmental Panel of Climate Change (eds Barros, V. R. et al .) Ch. 22, 1199-1265 (Cambridge University Press, 2014).
Rowell, D. P. Sources of uncertainty in future changes in local precipitation. Climate Dynamics 39 , 1929–1950, https://doi.org/10.1007/s00382-011-1210-2 (2012).
Orlowsky, B. & Seneviratne, S. I. Global changes in extreme events: regional and seasonal dimension. Climatic Change 110 , 669–696, https://doi.org/10.1007/s10584-011-0122-9 (2012).
Download references
Acknowledgements
This study is the part of feasibility study of water transfer project in the LCB. This research was supported by the Strategic Priority Research Program of the Chinese Academy of Sciences (XDA20010201) and Power China International Limited. We thank the National Aeronautics and Space Administration (NASA), the Climatic Research Unit (CRU), and the Lake Chad Basin Commission (LCBC) for providing their valuable datasets.
Author information
Authors and affiliations.
Key Laboratory of Water Cycle & Related Land Surface Processes/Institute of Geographic Science and Natural Resources Research, Chinese Academy of Sciences, Beijing, 100101, China
Rashid Mahmood, Shaofeng Jia & Wenbin Zhu
Qinghai Key Laboratory of Basin Water Cycle and Ecology, Qinghai Institute of Water Resources and Hydropower, Xining, China
Shaofeng Jia
School of Geographical Sciences, Qinghai Normal University, Xining, China
You can also search for this author in PubMed Google Scholar
Contributions
The first author, Dr Rashid Mahmood, designed and conducted this study under the supervision of the second author, Professor JIA Shaofeng, and Dr. Zhu helped prepare the graph and figures and reviewed the manuscript.
Corresponding author
Correspondence to Rashid Mahmood .
Ethics declarations
Competing interests.
The authors declare no competing interests.
Additional information
Publisher’s note: Springer Nature remains neutral with regard to jurisdictional claims in published maps and institutional affiliations.
Rights and permissions
Open Access This article is licensed under a Creative Commons Attribution 4.0 International License, which permits use, sharing, adaptation, distribution and reproduction in any medium or format, as long as you give appropriate credit to the original author(s) and the source, provide a link to the Creative Commons license, and indicate if changes were made. The images or other third party material in this article are included in the article’s Creative Commons license, unless indicated otherwise in a credit line to the material. If material is not included in the article’s Creative Commons license and your intended use is not permitted by statutory regulation or exceeds the permitted use, you will need to obtain permission directly from the copyright holder. To view a copy of this license, visit http://creativecommons.org/licenses/by/4.0/ .
Reprints and permissions
About this article
Cite this article.
Mahmood, R., Jia, S. & Zhu, W. Analysis of climate variability, trends, and prediction in the most active parts of the Lake Chad basin, Africa. Sci Rep 9 , 6317 (2019). https://doi.org/10.1038/s41598-019-42811-9
Download citation
Received : 26 June 2018
Accepted : 04 April 2019
Published : 19 April 2019
DOI : https://doi.org/10.1038/s41598-019-42811-9
Share this article
Anyone you share the following link with will be able to read this content:
Sorry, a shareable link is not currently available for this article.
Provided by the Springer Nature SharedIt content-sharing initiative
This article is cited by
Trend analysis and forecasting of meteorological variables in the lower thoubal river watershed, india using non-parametrical approach and machine learning models.
- Md Hibjur Rahaman
- Tamal Kanti Saha
- Haroon Sajjad
Modeling Earth Systems and Environment (2024)
Effects of Climate change on temperature and precipitation in the Lake Toba region, Indonesia, based on ERA5-land data with quantile mapping bias correction
- Hendri Irwandi
- Mohammad Syamsu Rosid
Scientific Reports (2023)
Application of Boruta algorithms as a robust methodology for performance evaluation of CMIP6 general circulation models for hydro-climatic studies
- I. M. Lawal
- A. H. Jagaba
Theoretical and Applied Climatology (2023)
Gendered Vulnerability, Perception and Adaptation Options of Smallholder Farmers to Climate Change in Eastern Ethiopia
- Mengistu M. Maja
- Abdurahim A. Idiris
- Muluneh M. Fashe
Earth Systems and Environment (2023)
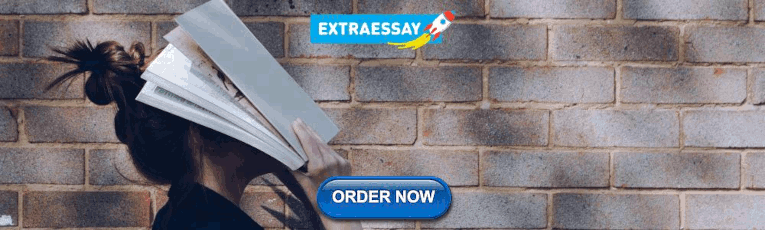
Time series analysis of climatic variability and trends in Shiwalik to Pir Panjal mountain range in the Indian western Himalaya
- Zishan Ahmad Wani
- Susheel Verma
Environment, Development and Sustainability (2023)
By submitting a comment you agree to abide by our Terms and Community Guidelines . If you find something abusive or that does not comply with our terms or guidelines please flag it as inappropriate.
Quick links
- Explore articles by subject
- Guide to authors
- Editorial policies
Sign up for the Nature Briefing newsletter — what matters in science, free to your inbox daily.

Loading metrics
Open Access
Peer-reviewed
Research Article
Adoption of climate-smart agricultural practices by smallholder farmers in rural Ghana: An application of the theory of planned behavior
Roles Data curation, Formal analysis, Methodology, Software, Writing – original draft
Affiliation Department of Environmental Science, Kwame Nkrumah University of Science and Technology, Kumasi, Ghana

Roles Conceptualization, Formal analysis, Funding acquisition, Investigation, Methodology, Writing – original draft, Writing – review & editing
* E-mail: [email protected] , [email protected]
Roles Formal analysis, Writing – original draft, Writing – review & editing
Affiliation Department of Environment and Geography, University of York, York, United Kingdom
Affiliation Department of Agribusiness, Joseph Sarwuan Tarkwa University, Markurdi, Benue State, Nigeria
Roles Writing – original draft, Writing – review & editing
Affiliation Ghana Agricultural Sector Investment Programme, Ministry of Food and Agriculture, Accra, Ghana
Affiliation School of Life and Environmental Sciences, University of Lincoln, Bradford Way, Lincoln, United Kingdom
- Jonathan Atta-Aidoo,
- Philip Antwi-Agyei,
- Andrew John Dougill,
- Christopher Elaigwu Ogbanje,
- Edmund Kyei Akoto-Danso,
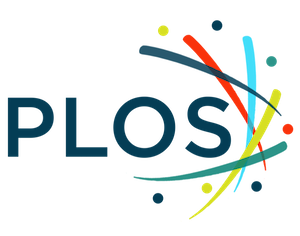
- Published: October 10, 2022
- https://doi.org/10.1371/journal.pclm.0000082
- Reader Comments
Climate-Smart Agricultural (CSA) practices are crucial in managing climatic shocks faced by smallholder farmers in sub-Saharan Africa. However, evidence on the socio-psychological drivers of farmers’ adoption of CSA practices remains limited. This study employed the Theory of Planned Behavior framework to analyze smallholder farmers’ intention and adoption behavior toward CSA practices in rural Ghana. The study sampled 350 smallholder farmers from the Upper East and North-East Regions of Ghana and employed the Structural Equation Model to understand smallholder farmers’ intention and adoption behavior toward CSA practices. Results showed that farmers’ attitudes (notably their beneficial evaluation of CSA practices) had a significant impact (0.25) on their intention to adopt CSA practices. Social pressure exerted on farmers to use CSA practices (Subjective norm) also had a significant impact (0.52) on farmers’ adoption behavior. Perceived behavior control which measures the controllability and use of CSA practices also had a significant impact on both the intention (0.43) and adoption behavior (0.20) of smallholder farmers. Findings highlight the role socio-psychological factors play in explaining the adoption of CSA practices in rural Ghana. We recommend the need to create awareness of CSA practices by sharing relevant information more widely on CSA practices through community leaders, chief farmers, assembly members, and clan heads in order to exert influence on farmer’s adoption of CSA practices.
Citation: Atta-Aidoo J, Antwi-Agyei P, Dougill AJ, Ogbanje CE, Akoto-Danso EK, Eze S (2022) Adoption of climate-smart agricultural practices by smallholder farmers in rural Ghana: An application of the theory of planned behavior. PLOS Clim 1(10): e0000082. https://doi.org/10.1371/journal.pclm.0000082
Editor: Ferdous Ahmed, IUBAT: International University of Business Agriculture and Technology, MALAYSIA
Received: July 14, 2022; Accepted: September 3, 2022; Published: October 10, 2022
Copyright: © 2022 Atta-Aidoo et al. This is an open access article distributed under the terms of the Creative Commons Attribution License , which permits unrestricted use, distribution, and reproduction in any medium, provided the original author and source are credited.
Data Availability: All data are in the supporting information file.
Funding: The work was supported by the Royal Society, London, through study received funding from the FLAIR Collaboration Grant by the Royal Society, London [FCG\R1\211025 to AJD and PA-A]. This work was also supported by the Future Leaders-African Independent Research (FLAIR) Fellowships funded by the Royal Society, London [FLR\R1\201640 to PA-A]. The funders had no role in study design, data collection and analysis, decision to publish, or preparation of the manuscript.
Competing interests: The authors have declared that no competing interests exist.
1. Introduction
Increased rainfall variability and drought associated with climate change poses the greatest challenge to the food systems and sustainable agricultural development of sub-Saharan Africa (SSA) [ 1 , 2 ] and to the region’s food and nutrition security [ 3 ]. SSA is regarded as the most vulnerable region to the negative impacts of climate change, because of structural and institutional weakness, high poverty levels and the low adoption of modern techniques that limits farmers’ capacity to adapt [ 4 – 6 ].
Agriculture in Ghana, like most SSA countries is largely rainfall-dependent and employs about 75% of the rural population [ 7 ], but extreme weather events arising from climate change pose a serious threat to the agricultural sector and agri-based livelihoods. Projections from climate models point to a worsening situation in Ghana. For example [ 8 ], reported that the annual mean temperature is projected to increase by 2.0°C and 3.9°C while rainfall is also projected to decrease by 10.9% and 18.6% by the years 2050 and 2080, respectively. Historical data indicate a worrying trend of shifting climatic conditions that encompass erratic and declining rainfall patterns and a warming trend across all the agro-ecological zones of Ghana [ 9 ]. These climatic changes are estimated to reduce cassava and rice yields by 13.5% and 8% by the year 2050 [ 10 ]. As such, crop yields will continue to decline unless farmers adopt and utilize Climate-Smart Agricultural (CSA) practices [ 11 ].
Climate-Smart Agriculture aims to achieve three pillars: (1) sustainably increase agricultural productivity and incomes; (2) enhance farmers’ adaptive capacity and build resilience; and (3) reduce the emission of greenhouse gases (GHGs) [ 12 , 13 ]. It has become imperative for farmers in developing countries to adopt and use CSA practices since they include numerous inexpensive farm-based sustainable agricultural land management techniques such as water management, zero/minimum tillage, residue management, and agroforestry among others. Additionally, CSA practices mostly include traditional practices and indigenous knowledge that are widely known to, and used by, farmers in addressing climatic risks [ 3 , 14 ].
Ghana, like many SSA countries, has sought to promote CSA through its sustainable agricultural development policy [ 15 ]. A National Climate-Smart Agriculture and Food Security Action Plan was developed with the aim of facilitating and operationalizing the National Climate Change Policy for effective incorporation of climate change into food and agriculture sector development policies and programs [ 16 ]. The action plan sought to provide a multi-sectoral institutional mechanism for climate-smart agriculture [ 16 ]. Over the years, numerous efforts have been made by the Government of Ghana and international organizations to promote the adoption of CSA practices to help mitigate the impact of climate change [ 17 , 18 ]. Despite these efforts CSA adoption remains low among smallholder farmers in many parts of Ghana [ 19 ]. However, the few farmers that have adopted practices attest to their effectiveness in increasing farm productivity and incomes, enhancing food security, and conserving the natural resources in Ghana [ 20 – 22 ].
Several studies have examined the determinants of the adoption and impact of CSA practices in SSA countries [ 23 – 25 ] and in Ghana specifically [ 20 , 22 , 26 ]. The determinants identified by these studies were mostly socio-demographic factors. Other determinants identified were access to extension services, awareness of climate change/variability, agricultural insurance, membership of farmer-based organization, and location of the farmer. Some adoption-related studies identified economic incentives as the major determining factor of the adoption of climate smart agricultural practices [ 27 , 28 ]. However, the factors affecting adoption of agricultural practices goes beyond just socio-demographic factors and economic incentives and are largely influenced by individual and intrinsic motivations [ 29 , 30 ] and other perceptions which can best be explained by psychological theories [ 31 , 32 ]. As yet, there is a dearth of empirical studies on the influence of individual and intrinsic motivation on the adoption of CSA practices in SSA and Ghana in particular. This study addresses this gap by using the Theory of Planned Behavior developed by [ 33 ] to examine the behavioral intention and actual adoption behavior of smallholder farmers toward CSA practices in Ghana. The Theory of Planned Behavior was chosen for this work because it provides socio-psychological basis for understanding human behavior [ 34 , 35 ] in diverse fields to encourage behavior change [ 36 ]. The main aim of the study was to identify the socio-psychological factors that influence farmers’ behavioral intention and actual behavior towards the adoption of CSA practices in rural Ghana using the Theory of Planned Behavior. The specific objectives of this study were to:
- Determine which psychological factors exert greater influence on farmers’ behavioral intention and behavior towards the adoption of CSA practices in rural Ghana.
- Examine whether farmers’ behavioral intention towards CSA practices translate into actual adoption behavior of CSA practices in rural Ghana.
We contribute to the literature on the adoption of CSA practices by identifying the relative significance of the Theory of Planned Behavior constructs on farmers’ behavioral intention and adoption behavior towards CSA practices in dryland farming systems. Insights can inform policymakers the areas of possible interventions that can be impactful at the household level to positively alter farmers’ behavior and enhance their adoption of CSA practices.
2. Theoretical framework and hypotheses development
2.1. the theory of planned behavior.
This study aimed to explain the adoption of CSA practices using the Theory of Planned Behavior (TPB) as developed by [ 33 ] ( Fig 1 ). Although a plethora of studies indicate the importance of economic incentives in driving the adoption behavior of farmers [ 31 , 37 – 39 ], the TPB has proven valuable in explaining the decision-making process of farmers [ 40 , 41 ]. This is because farmers are not only profit-maximizing entities [ 42 ], but can be influenced by other individual and intrinsic motivations especially when the decision may have both social and environmental consequences [ 29 , 30 ]. The TPB predicts people’s intention to follow a particular behavior based on the assumption that human behavior is regulated by behavioral intentions which are determined by the attitude, subjective norm, and perceived behavior control of individuals [33, 43; Fig 1 ].
- PPT PowerPoint slide
- PNG larger image
- TIFF original image
Source: [ 33 ].
https://doi.org/10.1371/journal.pclm.0000082.g001
The behavior intention of a farmer can be defined as that farmer’s motivation regarding their plan or conscious decision to apply effort to carry out a particular behavior [ 44 , 45 ]. Behavior intention represents the immediate antecedent and best predictor of performing an actual behavior [ 33 ]. By implication, stronger behavior intention towards a behavior indicates a stronger likelihood of performance of that behavior [ 46 ]. Such behavioral intention can accurately be estimated from the farmer’s attitude towards that particular behavior, subjective norm, and perceived behavior control [ 33 , 43 ]. However, limited studies [ 47 , 48 ] have examined the relationship between behavior intention and actual behavior due to the difficulty in measuring actual behavior. In addressing this, our study used past adoption behavior as a proxy for future adoption behavior particularly because farmers’ adoption of CSA practices shows a high degree of temporal stability [ 47 , 49 ].
Attitude refers to the favorable or unfavorable assessment of behavior. The overall assessment of behavior and belief in its desired results determine the attitude towards a behavior [ 50 ]. By implication, a more positive attitude towards a behavior leads to a better intention of carrying out that behavior [ 51 ]. Several studies [ 47 , 52 , 53 ] have indicated the role of attitude in predicting farmers’ intention to adopt farm practices. Attitude can be regarded as a significant determinant of an individual’s intention and behavior [ 54 , 55 ].
Subjective norm includes perceived social influence from internal and/or external sources to carry out or not to carry out a particular behavior. Such pressure may arise from internal sources such as family members and relatives or external sources such as friends and personnel from a government agency or an NGO [ 56 ]. The perceived approval of behavior by important people within a community also serves as a source of pressure that induces individuals’ intention of performing that particular behavior [ 57 ]. Subjective norm, therefore, measures the influence of the society on the decision-making process of a farmer [ 58 ]. Subjective norm has been estimated to be the most important determining factor of farmers’ intention to adopt new practices [ 59 – 61 ].
Perceived behavior control relates to the perceived ease or difficulty in performing a particular behavior. Perceived behavior control concerns itself with the existence of control factors that may hamper or enable the performance of a particular behavior [ 43 ]. These control factors may be in the form of money, skills, time as well as cooperation with others [ 62 ] and these may determine the farmers’ ability to carry out a particular behavior. A farmer’s engagement in a given behavior is subject to the farmer’s belief in the likelihood of having access to the required resources and opportunities [ 44 ]. Perceived behavior control is an essential predictor of farmers’ intention to adopt farm practices [ 60 , 63 , 64 ]. By extension, Perceived Behavior Control has a direct influence on intention and behavior [ 54 , 65 ].
Hypotheses . Based on the TPB model, seven hypotheses were developed for the study as follows:
H 1 : Attitude has a positive influence on farmers’ intention to adopt CSA practices.
H 2 : Subjective norm has a positive influence on farmers’ intention to adopt CSA practices.
H 3 : Perceived behavior control has a positive influence on farmers’ intention to adopt CSA practices.
H 4 : Behavioral intention mediates the positive effects of attitude, subjective norm, and perceived behavior control on farmers’ adoption of CSA practices.
H 5 : Attitude has a positive influence on farmers’ adoption of CSA practices.
H 6 : Subjective norm has a positive influence on farmers’ adoption of CSA practices.
H 7 : Perceived behavior control has a positive influence on farmers’ adoption of CSA practices.
The TPB was extended with additional two hypotheses (H 5 and H 6 ) which showed a direct relationship between attitude and behavior, and subjective norm and behavior. Sapp et al. [ 66 ] argue that, behavior intentions may be ill-informed at certain times leading to inconsistency between intention and actual behavior. It is therefore critical to examine the attitude–behavior and subjective norm–behavior relation to provide a better understanding of their impact on actual behavior because such relation has been largely ignored in the literature. Studies such as [ 67 , 68 ] have asserted that psychological factors such as attitudes and subjective norms are not always mediated by intention but can have a direct influence on actual behavior.
3. Methodology
3.1. study area.
The study was carried out in the West Mamprusi Municipality in the North East Region, and the Bongo District and Bolgatanga Municipality in the Upper East Region of Ghana ( Fig 2 ). These districts lie within the Sudan savannah agro-ecological zone and have a single rainfall pattern that lasts from May/June to September/October.
https://doi.org/10.1371/journal.pclm.0000082.g002
The West Mamprusi Municipality lies between longitudes 0°35’ W and 1°45’ W and latitudes 9°55’ N and 10°35’ N. The municipality has a total population of 175,755, comprising 85,712 males and 90,043 females [ 69 ]. West Mamprusi municipality is rural with agriculture being the mainstay of the local economy [ 70 ]. The main agricultural activities in the municipality include the rearing of livestock and the production of maize, millet, sorghum, and groundnuts.
The Bongo District is located between longitudes 0°W and 1°30’W and latitudes 10°30’N and 11°N. The Bongo district has a total population of 120,254, comprising 56,920 males and 63,334 females [ 69 ]. Subsistence agriculture involving the production of sorghum, millet, rice, groundnuts, and maize is the main economic activity in the district [ 71 ].
The Bolgatanga Municipality is located between longitudes 0°30’W and 1°00’W and latitudes 10°30N and 10°50’N. The Bolgatanga Municipality has a total population of 139,864, comprising 66,607 males and 73,257 females. Despite being a relatively urbanized municipality, livestock farming and crop production continue to be the main economic activity employing over 60% of the labor force within the municipality [ 72 ].
These districts were selected because they are among the most vulnerable to drought in Ghana and the majority of the populace are dependent on rain-fed agriculture for their livelihood [ 73 , 74 ]. Consequently, several projects and interventions such as the knowledge systems and advisory services supporting CSA aimed at enhancing farmers’ adoption of CSA practices have been instituted in these areas.
3.2. Sampling procedure
Three districts, namely West Mamprusi Municipality in the North East Region and Bongo District and Bolgatanga Municipality in the Upper East Region of Ghana, which have significant rural populations with agriculture as the main source of livelihoods were purposively selected. These districts were selected because they host several CSA demonstration fields of the Ghana Agricultural Sector Investment Program (GASIP). Subsequently, with the assistance of district agricultural officers, Sagadugu and Minima in the West Mamprusi Municipality, Yikene and Zaare in the Bolgatanga Municipality, and Ayelbia, Sinabisi, and Feo-Asabere in the Bongo District were selected.
Three hundred and fifty (350) household surveys were conducted in the seven study communities. A total of 87 households (38 in Sagadugu and 49 in Mimima) were interviewed in the West Mamprusi Municipality. Eighty-eight (88) households (46 in Ayelbia, 20 in Sinabisi and 22 in Feo-Asabere) were interviewed in the Bongo District while 175 households (87 in Yikene and 88 in Zaare) were interviewed in the Bolgatanga Municipality.
3.3. Ethics statement
Ethical approval for this study was provided by the Humanities and Social Sciences Research Committee (HuSSRECC) of the Kwame Nkrumah University of Science and Technology, Ghana. HuSSRECC subjected the protocol to a thorough review and, among other things, observed that the necessary precautions have been taken to ensure that the participants in study will be well protected from risks and other distasteful occurrences they may face in the administration of questionnaire in particular. Formal consent for participation was obtained verbally from each study participant after the study objectives have been interpreted to them in their local dialect. Study participants were assured of anonymity and confidentiality.
3.4. Questionnaire design and measurement scale
Smallholder farmers were randomly selected using the Census and Survey Processing System (CSPro) software in the seven farming communities. The survey was conducted between August 2021 and September 2021 using locally trained enumerators. Interviews were conducted at the convenience of the farmers at their homes and lasted between 45 to 60 minutes. The survey instrument consisted of a questionnaire that solicited information on the socio-demographic characteristics of the respondents, and questions framed base on the theory of planned behavior about CSA practices ( S1 File ). Four of the latent constructs (i.e. behavioral intention, attitude, subjective norm, and perceived behavioral control) were measured using twenty-two items adopted and modified from [ 75 , 76 ]. A five-point Likert scale was used for all the items (Part 1 in S1 File ).
Following the TPB guidelines, constructs for behavioral intention, attitude, subjective norm, and perceived behavioral control followed the principle of compatibility to avoid the occurrence of weaker and less-robust correlations among the constructs [ 77 ]. These constructs were defined in terms of the same element (i.e., CSA practices) to ensure construct compatibility and we also ensured that measurement scales were compatible across study sites to achieve scale compatibility [ 34 , 43 ]. Behavioral intention to adopt CSA practices was measured by four items, which enquired about farmers’ willingness to utilize CSA practices (with or without support) and their willingness to overcome barriers in terms of finance and information. Attitude towards CSA was measured using six items, three of which were concerned with the importance, convenience, and practicability of CSA practices. The other three considered the possible contributions of CSA practices in terms of increases in yield, on-farm income, and reputation. Subjective norm toward CSA was measured using six items, three of these items were about the motivation to use CSA practices while the other three covered the perception of others concerning adopting CSA practices.
Perceived behavior control was measured with six items. These items covered the control a farmer had over actions needed to adopt CSA practices. The fifth latent construct (CSA adoption) consisted of eight items covering CSA practices such as; the use of drought-tolerant varieties, cover cropping, zero tillage, no burning of crop residues, mixed cropping, planting early maturing varieties, water management/irrigation, and intercropping with legumes. A four-point Likert scale was used for these items.
3.5. Data analysis
The study used Structural Equation Modeling (SEM) with latent constructs to analyze the collected data following [ 59 , 78 , 79 ]. The first step involved Confirmatory Factor Analysis (CFA) to acquire a suitable measurement model. Step two covered the development and testing of the structural model. CFA was carried out to assess the validity of constructs as well as to evaluate the fitness of the model. [ 80 , 81 ] indicate the need for conducting CFA because construct validity reveals the extent to which the measured items reflect the hypothetical construct they are intended to measure. The validity of the measurement model was assessed using the overall goodness-of-fit statistics. Overall goodness-of-fit was assessed by checking the chi-squared value, the root mean square error of approximation (RMSEA), the comparative fit index (CFI), and the standardized root mean square residual (SRMR) [ 59 , 78 ]. Cronbach alpha and factor loadings were used to establish the reliability of the constructs and various items.
The structural modeling involved the estimation of a set of multiple regressions with particular emphasis on the nature and magnitude of the relation between the latent constructs [ 78 , 81 ] in this case attitude (ATT), subjective norms (SN), perceived behavior control (PBC), behavioral intention (BI) and actual behavior (CSA adoption). The predictive power and the ability of the SEM to estimate multiple regressions simultaneously made it the appropriate tool to examine the causal relations that exist among the TPB constructs and to test the underlying hypotheses. The SEM was estimated using the maximum likelihood procedure because maximum likelihood estimation procedure has proven to produce reliable and robust results under different circumstances compared to other estimation procedures [ 82 ].
3.6. Limitations of the study methods
Disagreement from respondents on what constituted climate smart practices is a limitation of the current study. The researchers resolved this limitation by providing further explanations as to what CSA practices were and the goals they seek to achieve. Another limitation of the study was focusing solely on the original factors of the Theory of Planned Behavior in explaining adoption of CSA practices. However, the authors saw this as necessary due to the extensive literature available on other factors affecting adoption decision of farmers. In spite of the limitations, the current study has strengths in terms of measuring CSA practices by not limiting it to a simple yes/no response but by measuring the frequency of use of these practices. The use of a Likert scale in measuring the adoption helps to ensure that a farmer who uses any CSA practice on yearly basis has a greater adoption score than a farmer who rarely uses or had used the given practice only once. Future study can build on this study by recategorizing the CSA practices under similar themes so as to measure the impact of the psychological factors on these sub-themes.
4.1. Descriptive statistics
The demographic and socioeconomic information of farmers are presented in Table 1 . The majority of respondents are smallholder farmers with 68.9% estimated farm sizes to be 2 hectares or below.
https://doi.org/10.1371/journal.pclm.0000082.t001
4.2. Item measurement in the TPB model
Table 2 presents descriptive statistics, factor loadings, and Cronbach alpha for the various constructs of the TPB framework. All the 350 respondents reported that they used at least one of the eight CSA practices most prevalent in their localities. A comparison of the eight items that make up the CSA adoption construct shows that mixed cropping (94.9%) was the most used practice followed by intercropping with legumes (82.9%), planting early maturing varieties (73.1%), no burning of crop residues (67.4%), cover cropping (62.6%), use of drought-tolerant varieties (60.9%), zero tillage (57.4%) and water management/irrigation (17.7%).
https://doi.org/10.1371/journal.pclm.0000082.t002
In terms of behavioral intention to adopt CSA practices, a cumulative 5% of the sample expressed disagreeable intention to adopt CSA practices while 3% showed neither agreeable nor disagreeable intention to adopt CSA practices. Item b1n6 (“I am willing to learn about CSA practices”) shows the highest mean score while item b1n1 (“I am willing to adopt CSA practices by myself; with or without financial support”) shows the least mean score.
Farmers expressed a positive attitude (mean of 4.44) towards the adoption of CSA practices. The majority of farmers interviewed expressed an agreeable attitude towards the adoption of CSA practices. About 2% of the sample expressed a disagreeable attitude towards the adoption of CSA practices, while 6% seem indifferent about the adoption of CSA practices.
About 25% of the sample expressed disagreeable subjective norms towards the adoption of CSA practices while about 10% of the sample expressed neither disagreeableness nor agreeableness towards the adoption of CSA practices. Sn6 (“CSA practices are something I speak about with important referents”) showed the highest mean score compared to sn8 (“I feel under pressure from extension agents to integrate CSA practices in my farming”) which received the lowest mean score. In terms of perceived behavioral control, 15% of the sample indicated disagreeableness while about 9% indicated that they were neither agreed nor disagreed with the items under this construct. Pbc4 (“I have the resources to implement the CSA practices”) showed the least mean score while pbc5 (“I can easily command to use CSA practices on my farm”) showed the highest mean score.
Factor loadings from the confirmatory factor analysis ( Table 2 ) show that the observed variables were significant at the p < 0.01 level and can be considered adequate, ranging from 0.18 to 0.89. Although, six items recorded factor loadings less than 0.30 as recommended for a sample size of at least 350 [ 82 ], they were maintained because they were greater than 0.10 and proved to establish a simple structure [ 42 , 82 ] and suggested at least good contributions of these items to their respective constructs [ 83 ]. The factor loadings ( Table 2 ) indicate that all the five latent variables satisfied the convergent validity test. The Cronbach alpha which was used to test for the reliability of the constructs indicated that all five constructs–attitude, subjective norm, perceived behavior control, behavioral intention and CSA adoption–recorded Cronbach alpha of above 0.60, implying that measurement scales for all the variables were internally consistent and reliable [ 82 , 84 ].
4.3. Goodness-of-fit statistics
Based on the “cut-off” points developed by [ 82 ] and presented in Table 3 , we chose four measures namely: chi-squared/degrees-of-freedom (χ 2 / df), comparative fit index (CFI), root mean square error of approximation (RMSEA), and standardized root mean squared residual (SRMR) to determine the overall model fit. Although a significant χ 2 indicates an unfit model, this was expected due to the large sample size and a high number of observed variables hence the χ 2 is not sufficient to measure the overall fit of the model [ 82 , 85 , 86 ]. The CFI which is less sensitive to model complexity shows that the model is fit given the “cut off” point of 0.92 for large sample sizes [ 82 ]. The observed value for RMSEA which attempts to rectify the tendency of using χ 2 to reject models with large sample sizes [ 82 , 87 ] indicates a good fit given an observed value of 0.069. The observed value of 0.08 for SRMR suggests no problem with the model fit indicating that the estimated model is significant and inferences made can be reliable [ 82 , 88 ].
https://doi.org/10.1371/journal.pclm.0000082.t003
4.4. Hypotheses testing
Table 4 shows that the attitude, subjective norm, and perceived behavior control of smallholder farmers jointly explained 25% of the variations in farmers’ intention to adopt CSA practices. Subsequently, behavioral intention, attitude, subjective norm, and perceived behavior control collectively explained a 30% variance in the adoption of CSA practices by smallholder farmers.
https://doi.org/10.1371/journal.pclm.0000082.t004
Standardized parameter estimates from the model are presented in Table 5 to show the different pathways. Subjective norm was revealed to have no significant effect on farmers’ intention to adopt CSA practices hence, there was no evidence to support H 2 . Perceived behavior control was estimated to have a greater influence (β = 0.43) than either attitude (0.25) or subjective norm (β = 0.02) on farmers’ intention to adopt CSA practices. Farmers’ attitude was estimated to have a positive and significant effect (β = 0.25, p < 0.000) on farmers’ intention to adopt CSA practices, providing evidence to support H 1 . The perceived behavior control of farmers was estimated to have a positive and significant (β = 0.43, p < 0.000) on farmers’ intention to adopt CSA practices, thus supporting H 3 .
https://doi.org/10.1371/journal.pclm.0000082.t005
Table 5 shows that farmers’ behavioral intention to adopt CSA practices played no mediating role on farmers’ actual adoption of CSA practices. However, the subjective norm was estimated to have a positive and significant effect (β = 0.52, p < 0.000) on farmers’ adoption of CSA practices, supporting H 6 . Perceived behavior control was estimated to have a direct positive and significant effect (β = 0.20, p < 0.010) on farmers’ adoption of CSA practices, thus, supporting H 7 . Our results show that attitude and perceived behavior control positively affect farmers’ intention to adopt CSA practices while subjective norm and perceived behavior control affect farmers’ adoption of CSA practices.
4.5. Discussion
Results from the SEM indicated that farmers’ attitude has a positive and significant impact on farmers’ intention to adopt CSA practices. A positive significant impact of attitude on farmers’ intention implies that favorable opinions about CSA practices increases a farmer’s chances of forming intentions to adopt such CSA practices. Forming such positive attitudes towards CSA practices depend on farmers’ witnessing the positive impacts CSA practices have on farm output. Our results are consistent with the findings of previous studies [ 44 , 67 , 78 , 79 , 89 ] suggesting that attitude is the best starting point for behavioral change. Attitude is regarded as an important component in shaping farmers’ intentions as it is the response to behavioral beliefs [ 42 , 78 , 90 ]. Farmers’ attitude has been documented to significantly impact behavioral intention towards Conservation Agriculture [ 91 ]. Studies including [ 47 , 92 , 93 ] have indicated that attitude has the largest impact on farmers’ behavior intention, however, our findings indicate the contrary and it is in line with the findings of [ 52 ] with attitude estimated to have a slightly negative impact on the actual adoption of CSA practices albeit insignificant. A possible explanation for such negative impact of attitude on the adoption behavior of farmers is due to the minimal exposure of farmers to the actual results of CSA practices on farms that use an appropriate mix of CSA practices. [ 26 ] indicate that being close to a climate-smart village increases the likelihood of adopting climate-smart practices because farmers that have witnessed firsthand the results of CSA practices tend to develop a positive attitude toward such practices. The lack of a visible “success story” about the use of CSA practices casts doubts about the expected results and hence farmers are likely to develop a neutral or negative attitude towards CSA practices. This has implications for the adoption of CSA practices in farming communities in the study area where slight changes in rainfall can cause significant crop yield losses.
Perceived behavior control had a positive and significant effect on farmers’ intention to adopt CSA practices. By implication, the perception of farmers about their own capabilities to apply CSA practices significantly influences their behavioral intention and their subsequent adoption of such practices. Our results are consistent with the literature [ 44 , 56 , 91 , 94 ], suggesting that higher perceived capability to apply CSA practices invariably leads to greater intention towards the application of CSA practices. The PBC component of the TPB suggests that, farmers who can overcome the different limitations in adopting CSA practices such as lack of information and resources will gain the motivation and develop the intention to adopt CSA practices [ 44 , 95 ]. PBC was found to have a significant and positive effect on the actual usage of CSA practices. PBC had a significant and positive impact on CSA usage because farmers mostly want to feel in charge of their adoption behavior [ 67 , 96 ]. This suggests that the perception of farmers about their capacity and degree of control over adoption significantly influences their intention and actual behavior [ 53 ]. However, studies such as [ 52 ] contend that PBC is not an important predictor of smallholder farmers’ intention to adopt production practices.
Subjective norm was found to have a significant and positive direct effect on CSA adoption. This suggests that farmers’ adoption behavior is influenced by perceived social pressure [ 56 ]. Social relations such as family members, neighbors, and opinion leaders play an active role in farmers’ adoption behavior. Subjective norm had the greatest effect on farmers’ adoption of CSA practices because farmers’ adoption decision is largely influenced by other’s opinion [ 92 ]. Social norm was found to have no significant effect on farmers’ intention to adopt CSA practices, this result is consistent with that of other studies such as [ 89 , 97 ]. Although studies including [ 92 , 93 ] have found subjective norm to significantly influence farmers’ intentions, their results revealed that social pressure/influence had extremely low impact on intentions relative to the attitude and perceived behavior control.
Contrary to the findings of [ 47 , 96 ], our model indicated that behavioral intention plays no mediating role between attitude, subjective norm, perceived behavior control, and CSA adoption. The difference in the mediating role of behavioral intention may stem from constraints. This result implies that unanticipated events, insufficient time and resources, lack of requisite skills and several other factors may prevent farmers from acting on their intentions [ 98 ]. It is therefore important these constraints are addressed to enable farming communities to successfully implement appropriate CSA interventions aimed at moderating the adverse effects of climate change and variability on agro-based livelihoods.
It is important to stress that self-reported measures of behavior and intentions may differ from actual behavior and as such lead to no correlation between the measures [ 99 ]. The difficulty in accurately measuring the actual behavior of farmers has prevented researchers from going beyond just intentions. Our study contributes theoretically to this sparsely researched area by estimating actual behavior from past behavior. This is critical considering the projected increases in temperature and erratic rainfall partners across Ghana and West Africa more widely. Findings from this study provides important information for policy makers to design climate change adaptation policy that take cognizance of the different psychological and behavioral factors that have the potential to influence the adoption of CSA practices.
5. Conclusion and policy implications
The study examined the different factors affecting smallholder farmers’ intention to adopt and their adoption behavior towards CSA practices using a Structural Equation Model (SEM) based on the Theory of Planned Behavior (TPB). We have provided evidence of the extent to which the attitude, subjective norm, and perceived behavior control of smallholder farmers drive their intentions and subsequently their actual adoption of CSA practices in rural Ghana.
Findings showed that farmers’ attitudes had a positive impact on farmers’ intention to adopt CSA practices but had no direct impact on farmers’ actual adoption behavior. This suggests that, the more positive attitudes farmers develop, the better the chances of increasing their intention to adopt CSA practices. Subjective norm had no impact on farmers’ intention to adopt CSA practices but significantly impacted the actual adoption of CSA practices by farmers. The perceived influence from both internal and external sources had the largest impact on farmers’ adoption behavior. Perceived behavior control had a significant impact on both farmers’ intention to adopt and the actual adoption of CSA practices. That is, farmers’ perception about their control over factors that could facilitate or hamper their adoption of CSA practices was the most significant driver of farmers’ adoption of CSA practices in Ghana.
We recommend that more efforts should go into creating awareness among smallholder farmers to develop a more positive attitude towards CSA practices. Such positive attitudes by farmers towards CSA practices can be harnessed if demonstration fields of CSA practices are made available to demonstrate the positive effects CSA practices have on crop yields. The study recommends the need to create awareness of CSA practices by sharing that relevant information on CSA practices through community leaders including chief farmers, assembly members, clan heads, etc. so that such information can be easily passed on to farmers. Policy makers should encourage the establishment of demonstrating farms for farmers to appreciate the benefits associated with such practices.
Supporting information
S1 file. sample questionnaire for data collection..
https://doi.org/10.1371/journal.pclm.0000082.s001
S1 Appendix.
https://doi.org/10.1371/journal.pclm.0000082.s002
Acknowledgments
The authors would like to thank the districts and communities that participated in this research.
- View Article
- Google Scholar
- 6. Boko M., Niang I., Nyong A., Vogel C., Githeko A., Medany M., et al. (2007): Africa. In: Climate Change 2007: Impacts, Adaptation and Vulnerability. Contribution of Working Group II to the Fourth Assessment Report of the Intergovernmental Panel on Climate Change [Parry M.L., Canziani O.F., Palutikof J.P, van der Linden P.J., & Hanson C.E. (eds.)]. Cambridge University Press, Cambridge, UK and New York, NY, USA, pp. 433–467.
- 12. Lipper L., McCarthy N., Zilberman D., Asfaw S., & Branca G. (2014). Climate smart agriculture : building resilience to climate change (p. 630). Springer Nature.
- 13. Food and Agriculture Organisation. (2010). The state of food insecurity in the world 2010.
- 15. Lipper L., & Zilberman D. (2018). A short history of the evolution of the climate smart agriculture approach and its links to climate change and sustainable agriculture debates. In: Climate smart agriculture (pp. 13–30). Springer, Cham.
- 17. MoFA (2015). Medium Term Agricultural Sector Investment Plan (METASIP II). Ministry of Food and Agriculture, Accra.
- PubMed/NCBI
- 62. Ajzen I. (1985). From intentions to actions: A theory of planned behavior. In Action control (pp. 11–39). Springer, Berlin, Heidelberg.
- 77. Fishbein M., & Ajzen I. (2011). Predicting and changing behavior : The reasoned action approach . Psychology press.
- 80. Kline R. B. (2011). Principles and practice of structural equation modeling (3 ed.). New York: The Guilford Press.
- 81. Hair J. F. Jr, Black W. C., Babin B. J., & Anderson R. E, (2010). Multivariate Data Analysis: A Global Perspective, 7th Edition. Pearson Education International.

An official website of the United States government
The .gov means it’s official. Federal government websites often end in .gov or .mil. Before sharing sensitive information, make sure you’re on a federal government site.
The site is secure. The https:// ensures that you are connecting to the official website and that any information you provide is encrypted and transmitted securely.
- Publications
- Account settings
Preview improvements coming to the PMC website in October 2024. Learn More or Try it out now .
- Advanced Search
- Journal List
- Vet Anim Sci
- v.16; 2022 Jun
Effects of climate variability on livestock productivity and pastoralists perception: The case of drought resilience in Southeastern Ethiopia
Matiwos habte.
a Africa Center of Excellence for Climate Smart Agriculture and Biodiversity Conservation, Haramaya University, P.O.Box 138 Dire Dawa, Ethiopia
b Department of Animal and Range Sciences, Dilla University, P.O.Box 419 Dilla, Ethiopia
Mitiku Eshetu
c School of Animal and Range Sciences, Haramaya University, P.O.Box 138 Dire Dawa, Ethiopia
Melesse Maryo
d Ethiopian Biodiversity Institute, Addis Ababa, Ethiopia
Dereje Andualem
Abiyot legesse.
e Department of Geography and Environmental studies, Dilla University, P.O.Box 419 Dilla, Ethiopia
This study examines the perception of the pastoral community on climate change and performance, resilience and adaptive capacity of livestock under climatic stress in southeastern Ethiopia. The study used a mixed research approach whereby quantitative and qualitative data were gathered from multiple sources to address the impacts of climate variability on livestock production and livelihood of pastoral-agro-pastoral communities of Guji zone. Data about pastoralist perception on climate change were collected from 198 randomly selected households using a semi-structured questionnaire. Furthermore, climate data were obtained from the national meteorological agency, and climatic water balance was assessed. The household survey result indicated increasing patterns of temperature (82.8%)and drought intensity (84.8%). Majority of respondents perceived decreasing trends of rainfall and feed availability. Similarly, the trend analysis of rainfall showed declining trends of annual (-4.7 mm/year), autumn (-4.5 mm) and winter (-0.54 mm). Rainfall Anomaly Index identifies 13 drought years over the past 32 years, of which 53.85% occurred between 2007- 2017. Significantly higher (p<0.01) cattle and small ruminants than camel per household died during the disastrous drought occurred in 2008/9 and 2015/16. Nonetheless, the result indicated significantly higher (p<0.01) amounts of milk yield (3.32 litre/day) of dairying camel during dry periods than cattle and small ruminants. Camel and goats are perceived as drought-resistant livestock species and cattle keepers shifting to have more camel and goat in response to prevailing drought in the study area. Poor attention is given to identify climate-smart/resilient livestock species and strains. Therefore, extensive investigations are required to select and identify purpose-specific camel and goat strains for drought-prone areas.
Abbreviations
1. introduction.
Most Africans depend on agriculture for their livelihoods, which is the backbone of national economies for almost all countries in Africa. The sector employs 70-90% of the total labour forces, supports about 50% of feed demands and 50% of the income of the households. Among the agricultural sub-sectors, livestock rearing supports the income and livelihood for about one-third of African populations and provides 30-50% of agricultural GDP ( AU-IBAR, 2016 ). Livestock is the principal asset of the poor in most pastoral and agro-pastoral communities, though the sector is highly susceptible to extreme climatic events ( Fereja, 2016 ). Climate extremes are having a significant impact on livestock productivity in Eastern and Western Africa. Increasing frequency and intensity of droughts; changes in water availability; increasing patterns of temperature and rainfall variability, all are profoundly threatening livelihoods of drought-prone areas, and the existence of arid and semi-arid remote regions ( Palombi & Sessa, 2013 ; Ulrichs, Slater, & Costella, 2019 ). Climate change is threatening the productivity of agricultural land, by shortening growing periods and decreasing crop/pasture yields ( UNFCCC, 2007 ).
In Ethiopia, climate variability and change has triggered frequent droughts, floods, heat waves, heavy rains, and strong winds ( FDRE, 2007 ). The country is suffering from the impacts of climate change such as an increase in average surface temperature, changes in rainfall patterns, recurrent drought, El Niño southern oscillation (ENSO), floods and La Nina ( Melees & Samuel, 2017 ; Melkamu, 2017 ). The country necessarily needs to switch to a new sustainable development strategy to cope with and adapt to the changing climatic condition ( Anita, Dominic, & Neil, 2010 ; FDRE, 2011).
The vulnerability of livestock to climate variability varies across species based on their adaptive mechanism ( Fereja, 2016 ). According to Wako, Tadesse, and Angassa (2017) , a significantly higher number of cattle and sheep death were recorded during drought condition, while the death of goat and camel were eventually low. Loss of animals is associated with drought and the cumulative impacts of rainfall and surface temperature variability. The climate variability alters plants' growth potential, deterioration of livestock feed resources 56 and livestock's physiological response. Increasing patterns of temperature and decreasing rainfall trends is a global phenomenon, pastoral and agro-pastoral communities who rely on natural resources for livestock production rigorously feel its adverse effects. Climate variability alters the niche of forage species and may modify animal feed resources. The rising surface temperature may increase fodder and pasture browse species' productivity while the productivity of grassland is severely declined ( Thornton, Herrero, & Ericksen, 2011 ). Changes in grassland composition lead to inadequate grassland serving capacity and the areas left with browse feed resources ( Fereja, 2016 ; Yilma, Haile, GuerneBleich, & Ababa, 2009 ).
Climate change is affecting livestock productivity by altering ecosystem services like water availability, forage quality and quantity ( Gashaw, Asresie, & Haylom, 2014 ; Hidosa & Guyo, 2017 ; Kefyalew & Tegegn, 2012 ), diseases outbreak, and animals stress due to heat shock ( Bagath et al., 2019 ; Morand, 2015 ), and eroding livestock species diversities ( Yilma et al., 2009 ). Ecosystem health and animal health are directly linked to the impacts of climate change ( Al-Amin & Alam, 2011 ). The incidence and distributions of livestock diseases are currently increasing, which is assumed that these might have emerged due to climate change. The distribution of vectors and pathogens are positively correlated to wind and its blowing direction ( Lubroth, 2012 ; Van den Bossche & Coetzer, 2008 ; Yatoo, Kumar, Dimri, & Sharma, 2012 ). Climate change diminishes available feed resources and creates conducive environment to survive, complete cycle and transfer of disease which directly leads to increase the susceptibility of livestock species and distributions to vectors and pathogens ( Desalegn, 2016 ; Lubroth, 2012 ; Morand, 2015 ; Yilma et al., 2009 ).
Although, the interest of camel research increased from time to time in the current era, according to Tefera and Abebe (2012) most of the past research findings concentrated on assessing morphology and anatomy (34%), veterinary aspect (16%), general physiology (12%) and reproduction (10%). Those of which recently conducted were based on either short period observation or one-time survey and restricted to Somali and Afar Regional States in Ethiopia and almost all studies failed to assess impacts of climate change on livestock production potential ( Mebrahtu, Asgedom, & Hadush, 2017 ; Simenew et al., 2013 ; Yosef et al., 2014 ).
According to IPCC (2014) , global surface temperature increases, rainfall patterns become uneven, and heat waves events are the potential consequences of the climate variability and changes. Effects of climate change will directly impact the livestock sector and rangeland resources, directly linked to pastoralists livelihoods and food security. The loss (death) of livestock has been observed by climate-driven impacts such as recurrent drought, which negatively impacts pastoralists' livelihood security. The pastoral and agro-pastoral communities are particularly vulnerable to climate variability and changes due to their livestock dependence for food and livelihood. For preparing people to face these challenges, decision-makers and policy planners need information on climate change. Pastoral and agro-pastoral communities in Ethiopia have become vulnerable to the effects of recurrent drought. However, pastoralists have gradually developed mechanisms to survive in a risky environment. The communities lived in drought-prone regions, adapted to fragile environments, and sustainably conserved the natural resources. A comprehensive assessment of the pastoralists perceptions on climate change and vulnerability, i.e., the degree to which livestock species is susceptible to climate variability and extremes, is needed to reduce its impacts and respond effectively. Pastoralists in the study area are rainfall dependent, and any variation in its pattern affects livestock productivity and survivability. Some livestock species are more vulnerable than others, depending on their resilience and adaptive capacity. Assessing community perception of climate change and livestock production potential under climatic stress is valuable in addressing livestock herder vulnerability to climate extremes. Therefore, this study examines the perception of the pastoral community on climate change and performance, resilience and adaptive capacity of livestock under climatic stress in southeastern Ethiopia.
2. Material and Methods
2.1. study area description.
East Guji Zone is located in southeastern parts of Ethiopia ( Fig. 1 ), enclosing 13 districts, of which five of them host pastoral and agro-pastoral communities. Three neighbouring pastoral and agro-pastoral districts, namely Liben, Gumi Eldallo and Goro Dola have been selected for the study. The chosen areas are located 5°02′ to 44’N and 39°28′ to 42′E and cover about 742,644.14 ha. The altitude of the study districts ranges between 1370 and 1560 m.a.s.l . The agro-climatic condition of pastoral and agro-pastoral areas of the East Guji zone is mostly arid and semi-arid with an average annual rainfall of 526.75 mm. The pattern of the rain is bimodal with the main rainy season (Ganna) contributing about 60% of yearly rainfall which extend from March to May while minor season (Hagayya) providing approximately 40% of annual rainfall ranges from September and November. Meteorologically, there are four-seasons in east Guji zone; autumn (Ganna), summer (Adolessa), spring (Hagayya) and winter. Autumn (March-May) is the long rainy season while spring (September – November) is short and erratic rainfall period in the study area. Winter (December – February) is the driest season of the year and summer (June – August) is moderately dried period between the long rainy season (autumn/Ganna) and the short rainy season (spring/Hagayya) in east Guji zone ( Abate, 2016 ; Alhamshry, Fenta, Yasuda, Kimura, & Shimizu, 2020 ; NMA, 2015 ). The annual mean temperature of the pastoral and agro-pastoral districts of the Zone ranges from 24 to 30°C ( Adi & Swoboda-Reinhold, 2003 ). The area is prone to drought every five to ten years ( Abate, 2016 ).

Location map of the study area
The pastoral and agro-pastoral districts of east Guji zone are homes to 192,121 populations, of whom 97,062 are males, and 95,059 are females ( CSA, 2007 ). The pastoral districts of the Zone have a total population of 1,285,392 cattle, 282,302 camels, 1,247,484 goats, 231,523 sheep, 163,015 donkeys and 777,402 chickens. Rain-fed based livestock rearing is the main livelihood supporting the economy of pastoral community of the study area under the mercy of nature. Pastoralism in the study area is constrained by drought, erratic rainfall, poor livestock productivity, grassland degradation, eroding forage species, and increasing livestock disease occurrences and livestock death. As a result, most people of the study area chronically face food insecurity ( Abebe et al., 2012 ; Kebebew, Synnevaag, & Tsegaye, 2001 ; Mirkena et al., 2018 ).
2.2. Data types and source
This study primarily focused on collecting information related to camel resilience ability to climate variability influence on pastoral communities livelihood options such as livestock production and productivity. Qualitative and quantitative data were gathered using semi-structured questionnaires from the selected districts. The survey encompasses the economic benefit of camel including socio-economic characteristics , intensities of drought and disease prevalence, effects of drought on livestock, mainly camel production in the face of climate change. Furthermore, data on camel keepers' indigenous knowledge and practices, potential milk production under hostile environmental condition, benefits, challenges and opportunities of camel production over cattle, goat, and sheep were collected.
Thirty-two years (1986-2017) of time series daily precipitation and temperature data were obtained from National meteorological agency collected at “Negelle” station to analyze rainfall and temperature trends. The daily record of climate data was missed with about 5.8% (673 values) of rainfall and 4.6% (534 values) of temperature. However, the missed values were assumed based on the estimation method of climatological data where the missing values equal to the mean of the same period of non-missing years.
2.3. Sampling procedures and design
Three study districts namely Liben, Gumi Eldallo and Goro Dola were randomly selected from the five pastoral and agro-pastoral districts of East Guji Zone by using drawing lots procedure indicated in ( Gomez & Gomez, 1984 ). The study considered two traditionally classified geographical locations namely Golba (covers the altitude below 1450 m.a.s.l) and Dida (the altitude up-to 1650 m.a.s.l). Two kebeles (the smallest administrative area of Ethiopian Government) were randomly selected from each districts; Hadhessa and Qoratti from Gumi Eldallo, Siminto and Kalada from Liben and Gofi Ambo and Nura Umba from Goro Dola district. Accordingly, Hadhessa, Qoratti and Siminto kebeles from Dida and Kalada, Gofi Ambo and Nura Umba from Golba study location. The wealth status of participating respondents was classified based on livestock number owned following Gemedo-Dalle, Isselstein, and Maass (2006) . Up-to-date list of camel owners was obtained from the zonal and Districts pastoralism offices, from which 198 sample respondents were chosen using systematic random sampling methods. The required sample size was determined based on an adopted formula following Yamane (1967) and Israel (1992) at a 95% confidence interval and 7% precision level (sampling error).
where n is sample size, N = total household of selected kebels, e = precesion level, z= 1.96 (at 95%).
Sample Size = n = 2441 1 − 2441 ( 0.07 ) 1.96 = 198
The sample size for each kebeles was allocated according to Bowley (1925) formula as follows.
where ni = assigned sample size of kebeles or wealth rank, n = Total sample size, Ni = Household size of single kebeles or single wealth group, N = Total household size
2.4. Method of Data Collection
2.4.1. household survey.
The survey was conducted through exploring the discussion points listed in the questionnaires by moving on the respondent's perception, opinions and experience on climate change, rainfall and temperature trends, livestock trends, livestock resilience abilities and camel production. The discussion was primarily focused on the perception of pastoralists/agro-pastoralists on climate change and its effects on livestock productivity and livelihood strategies by asking the questions and recording the response. The assessment was conducted by explaining the significance of the study and maintaining the comfortable atmosphere through appealing the instincts of pride of respondents. Furthermore, prepared questionnaires were translated into the local language (Afan Oromo) since the study locations are solely Oromo's ethnic group. The enumerators assured not to reveal the respondent's identity and only used the data in aggregate form.
Enumerators were recruited and trained to have better knowledge on the questionnaires. The questionnaires were pre-tested before the actual data collection, and appropriate components were modified and corrected as per the feedback obtained. Respondents were interviewed following face-to-face survey method using the door-to-door approach.
2.4.2. Key informant Interviews
Key-informant interview was conducted to obtain a general overview of the climate change situation and its impacts on livestock production as well as socio-economics of the community following ( Geilfus, 2008 ). Accordingly, eight key-interview questions were developed using semi-structured dialogue methods. Eight key informant elders (4 male and two female pastoralists, and two male government experts) were selected and interviewed.
2.4.3. Focus group discussion
Thirty six participants from both sexes representing all wealth groups, locations and education level were purposively selected to obtain relevant information on climate variability and camel resilience ability following ( Geilfus, 2008 ). Six FGDs, one in each kebeles consisted of eight participants were employed.
2.5. Data analysis
All collected data were subjected to Kruskal-Wallis test using R-software (version 3.6.0) to test against normality to manage the outliers. Mean differences among variable were determined using Duncan's multiple range test ( Duncan, 1955 ). A General Linear Model (GLM) and multivariate linear model procedure were used for analysis of quantitative variables.
Analysis of Variance was used to examine the variance of the quantitative variables with Standard deviation (SD). Descriptive statistical tests were also used to analyze qualitative variables. Furthermore, the non-numeric data such as key informant interviews, notes, focus group discussions, images and audio recordings were analyzed using narrative and discourse methods of qualitative data analysis following the procedure indicated in O'Connor and Gibson (2003) and Bernard and Bernard (2013) .
Linear trend analysis was used to compute trends of temperature and rainfall. The intra- seasonal, annual variabilities and drought conditions were characterized using standard precipitation evapotranspiration index (SPEI) using SPEI package in R-software ( Vicente-Serrano, Beguería, & López-Moreno, 2010 ), rainfall anomaly index and coefficient of variation were also analyzed. The significance of trend analysis was tested using Mann-Kendall's test of time series significance. Hydrological and agricultural drought was quantified using SPEI for 1986-2017 period ( Table 1 ). Moreover, Sen's slope of estimator applied to quantify gradient of temperature and rainfall over the years and across seasons. Pettitts test was also computed to show the period at which significant change observed ( Mann, 1945 ). The frequency and intensity of drought was quantified using Rainfall anomaly index taking the record of the dry and wet years of historical precipitation data following Van Rooy (1965) and the modified equation by Hänsel, Schucknecht, and Matschullat (2016) .
SPEI and CV value to characterize conditions drought and variability.
Source: McKee, Doesken, and Kleist (1993) and Hare (1983)
R A I = − 3 * ( N − N ¯ X ¯ − N ¯ ¯ ) ,For negative anomalies
R A I = 3 * ( N − N ¯ M ¯ − N ¯ ¯ ) , For Positive anomalies
N = current monthly/seasonally/yearly rainfall when RAI generated (mm)
N ¯ = Yearly average rainfall of historical (32 years) series (mm)
M ¯ = average of the ten highest yearly precipitation of historical series (mm)
X ¯ = average of the lowest ten yearly/seasonal precipitation of historical series (mm)
Historical series = 1986-2017
== Positive anomalies have the value above average and negative anomalies have their values below average.
3. Result and Discussion
3.1. perception of climate variability among pastoralists.
East Guji pastoralists perceived the changing climatic condition in terms of temperature, rainfall and intensity of drought ( Table 2 ). There is no statistically differ between the perception of pastoral and agro-pastoral communities at both study location regarding onset of rainy season, temperature pattern, drought intensity, duration and duration of rainfall. Significant (p< 0.05) proportion of the pastoralists in the study area perceived the late onset of the rainy season (p = 0.0005, 72.7%) and increasing patterns of temperature (p <.0001, 82.8%) as compared with constant rainfall and temperature over years. In agreement to this finding, revealed significant increasing trends of mean maximum and minimum temperature as well as increasing frequency of drought in recent years in Ethiopia. Similarly, Nicholson, Funk, and Fink (2018) revealed the decreasing patterns of annual and seasonal rainfall in Ethiopia. According to ATPS (2013) , pastoral and agro-pastoral communities are perceived decreasing patterns of rainfall, late onset of rainy season and increasing trends of temperature and heat stresses.
Pastoralist/agro-pastoralist Perception of temperature, rainfall and drought change (Frequency (%))
The result of this finding extrapolates the increasing trends of temperature patterns of the 2010s as compared to 1980s. As indicated in Table 2 , significant number of pastoralist and agro-pastoralist from Golba (p <.0001, 80.2%) and Dida (p <.0001, 85.3%) locations thought an increasing rate of temperature patterns. In some occasions, the temperature trend may decrease when precipitation and amount of rainfall increase as well as when la Nina climatic condition appears. In line with this finding, Ayal and Leal-Filho (2017) reported an increasing trend of temperature farmers perception in Ethiopia. Similarly, Afar and Somali pastoralists are well aware of increasing trends of temperature from time to time as reported by ATPS (2013) .
Drought intensity increased in the 2010s compared with 1980s ( Table 2 ). The result of the current finding revealed that significantly higher (p < 0.05) proportions of pastoral and agro-pastoral communities perceived increasing trends of drought intensity (p <.0001, 84.8%) over the years. According to Getachew (2018) , drought affected geographic coverage were being increasing in Ethiopia. Furthermore, Ververs (2012) and Osborn, Barichivich, Harris, Van Der Schrier, and Jones (2018) reported the declining trends of rainfall and increasing rate of drought affected communities in eastern African and all over the globe since 1950/51.
3.2. Historical Climate Data Analysis
3.2.1. rainfall trends.
The analysis of precipitation data over the past 32 years (1986-2017) showed a decreasing trend of annual rainfall in the study area ( Fig. 2 ). Similarly, the majority of respondents perceived that the amount of rainfall has being decreasing from year to year and becoming too little in its amounts. Meteorological record of precipitation data of the study area corroborates the perception of most respondents. In contrary to this finding Tsige (2018) revealed a lack of congruence of meteorological data with farmers perception of rainfall trends. The analysis of linear trend during the historical period of 1986-2017 showed that decreasing (4.68mm) pattern of the annual rainfall. In agreement to this study, Nicholson et al. (2018) and Asfaw, Simane, Hassen, and Bantider (2018) reported decreasing patterns of annual rainfall in African continent and in Ethiopia, respectively. Similarly, Pachauri and Reisinger (2008) reported the declining trends of rainfall patterns over the dry region and southeastern parts of Ethiopia Chris et al. (2012) . also discussed 15-20% declining rate of summer rainfall in some parts of Ethiopia since the mid-1970s.

Total annual trends of rainfall in East Guji zone
Data source: NMA, 2018
The result of rainfall anomaly index of the current study revealed that there are an increasing intensity and frequency of drought years during the 1986-2017 historical periods. The rainfall anomaly index resulted in 13 driest years (1991, 2016, 2017, 1992, 1999, 2015, 1998, 2010, 2000, 1988, 2009, 2007 and 2012) within 32 years (1986-2017). Of which, 46.2% (6 driest years) were recorded during 1986-2006 (within 21 years) while about 53.8% (seven driest years) noted during 2007 – 2017 (within 11 years), indicating increasing trends of drought years in the last 11 years. Similarly, the majority of pastoralist perceived an increasing trend of drought intensity (84.8%) in the recent decade ( Table 2 ).
The rainfall anomaly index of east Guji zone during the historical period of 1986-2017 ranges from -6.34 in 1991 (the driest years) to 4.31 in 2005 (the wettest year) ( Fig. 3 ). The mean annual rainfall of the historical period (1986-2017) is about 637.6 with standard deviation of 109.3 and coefficient of variation of 17.2% CV value ( Table 3 ), indicating less inter-annual variability over the past 32 years. Changes in distribution, regularity and seasonality of rainfall in the study area was a major concern than amounts of rainfall overall historical period (1986-2017). The long rainy season progressively becomes shortening, which is in line with the perceptions of large proportion of respondents who have perceived amounts of rainfall receive over the years to be too little. In agreement with this finding, Tsige (2018) reported higher seasonal variability of rainfall in Sidama Zone of the southern Ethiopia. Similarly, Herrero et al. (2010) revealed a remarkable inter-annual variability of precipitation across Ethiopia, Kenya, Tanzania and Somalia.

Annual rainfall anomaly Index in East Guji zone
Mean Seasonal and annual rainfall amount along with the coefficient of Variation
3.2.2. Seasonal rainfall patterns and variability
The maximum and minimum rainfall of the long rainy season (autumn /Ganna) over the last 32 years (1986 – 2017) was 633.5 mm and 172.4 mm, respectively. The mean autumn season rainfall was 368.3 mm with about standard deviation of 109.1 and CV of 29.6%, indicating moderate inter-seasonal variability of rainfall over the last 32 years. The patterns of autumn rainfall have shown a declining trend from 1986-2017 ( Fig. 4 ). The trend result of autumn season rainfall showed a declining pattern by 5.4 mm per year during the historical period. The current finding revealed that higher seasonal variability in summer (CV = 78.2%), spring (CV = 39.0%) and winter (CV = 94.0%) with a non-significant decreasing trend of winter and slightly increasing trends of spring rainfall by 1.16 mm ( Fig. 4 ; Table 3 ). The CV values of rainfall of summer, winter and spring season lie within the highly variable category while the autumn season lie within the moderate variable category. Thus, the trend analysis of the autumn season rainfall indicates declining patterns from year to year over the past 32 years. Therefore, the decreasing trends of the long rainy season (autumn/Ganna) could be the primary reason for the declining annual rainfall patterns. However, minor rainy season (spring) and summer meteorological season trend line of rainfall showed an increasing amount of rainfall over the historical time series of the past three decades.

Autumn season (March – May) rainfall (mm) trends of East Guji Zone
Note: The Autumn season showed in Fig. 3 includes March, April and May months (mm). The data panel shows the raw rainfall data in the autumn season. The seasonal panel shows the seasonality of rainfall data. The trend panel shows rainfall trends of the autumn season from 1988 to 2018. The remainder panel shows the residual of the rainfall data.
Similarly, NMA (2016), Bekele (2017) , Asfaw et al. (2018) & Teshome and Zhang (2019) revealed decreasing trends of autumn, summer and spring (short rainy season) amounts of rainfall in east and southeast parts of Ethiopia. The analysis of rainfall data collected from meteorological stations located in Rift Valley areas indicates high variability and erratic nature of rainfall in amount and distribution in the last four decades ( Regassa, Givey, & Castillo, 2010 ). In contrary to the current finding, Tsige (2018) revealed increasing trends in rainfall during the long rainy season (June to September) and decreasing pattern during the short rainy season (February to May). According to IPCC (2014) , climate change might alter the distribution, frequency and duration of precipitation in general . The observed historical data in this study depicted significant variability of rainfall across the study area. This variability became a bottleneck of livestock productivity and household's livelihood in the study area.
3.2.3. Standardized Precipitation Evapotranspiration Index (SPEI)
East Guji Zone experiences hydrological drought during the years of 1991, 1992, 1999, 2015, 2016 and 2017 ( Fig. 5 ). However, the rainfall anomaly index showed 13 years (1988, 1991, 1992, 1998, 1999, 2000, 2007, 2009, 2010, 2012, 2015, 2016 and 2017) of the below-average annual rainfall of historical period (1986 – 2017). Of which 1991, 1992, 2015, 2016 and 2017 years were characterized as severely dry years while the SPEI value of 1991 lies within the moderately dry category. The SPEI results of the long rainy season (autumn) indicated the agricultural drought during 1990, 1991, 2003, 2015, 2016 and 2017. The SPEI result of the spring season in 1990 (-1.04), 1992 (-1.37), 2003 (-1.05) and 2017 (-1.42) lies within a moderately dry category while 2015 (-1.93) and 2016 (-1.73) lies within the severely dry category of agricultural drought ( Fig. 5 (a)).

Rainy a) season and b) annual standardized precipitation Evapotranspiration Index (SPEI) of East Guji Zone
Fig. 5 a indicated severe agricultural drought in 1991 (SPEI = -1.53), 1992 (SPEI = -1.70) and 2016 (SPEI = -1.84) in autumn rainy season. Thus, the study area has been suffering from agricultural and hydrological drought over the last 32 years (1986-2017). According to Teshome and Zhang (2019) , the chronology of extreme events compiled from international disaster database showed, increasing frequency of drought in the last 32 years in Ethiopia and 11 drought years (1984, 1985, 1987, 1988, 1990, 1991, 1992, 1997, 2002, 2009, 2015, 2016) has recorded during the recent four decades of the historical period (1980 - 2016). Similarly, Temam, Uddameri, Mohammadi, Hernandez, and Ekwaro-Osire (2019) reported an increasing intensity and frequency of agricultural and meteorological drought over the last century in Ethiopia. According to Kebebew et al. (2001) , acute drought (a long period of abnormally low rainfall) covered the whole Borana during the years of 1984, 1990, 1991, 1992, 1993, 1994, 1999 and 2000, which has resulted scarcity of grazing feed resources, drinking water and human food. During these periods, animals were unable to provide milk and produce adequate meat for household consumption, and a large number of animal died.
3.2.4. Trends of temperature
Mean maximum and minimum temperature record of the last 32 years were 26.7 °C and 15.8 °C, respectively Fig. 6 . shows an increasing trend of annual maximum and minimum temperature by 0.08 °C and 0.084 °C per year, respectively. Over the past three decades, indicating a slightly faster increasing rate of minimum temperature than maximum. The mean annual maximum temperature steadily increasing from 25.53 °C (1986) to 28.88 °C (2016) while minimum temperature increased from 12.71 °C (1991) to 16.93 °C (2012). In agreement to this finding, Tsige (2018) reported steadily increasing minimum and maximum temperature during the historical period of 1987-2017 in Sidama Zone of Southern Ethiopia. Similarly, Asfaw et al. (2018) discussed increasing trends of mean maximum and minimum annual temperature by 0.067 °C and 0.026°C per decades, respectively, during the historical period of 1901-2014. A report from NMA (2016) depicted increasing trends of mean minimum temperature by 0.36°C per decades in Ethiopia Teshome and Zhang (2019) . Also showed increasing trends of mean maximum and minimum temperature in Ethiopia with 0.04 and 0.05°C, respectively during 1980-2010. Moreover, Hussen-Ahimed, Biru, and Yadessa (2011) reported that the mean annual maximum and minimum temperature has been increasing with one and 0.25°C per decades throughout the country. At global level, Bindoff et al. (2013) and Huang et al. (2017) indicated significant increasing patterns of mean annual temperature and surface warming by 0.6-1.3 °C at almost all location during the historical period of 1951 – 2010.

Trends of annual maximum and minimum temperature
Increasing trends of mean annual temperature may cause heat stress in livestock species, and loss of animal may be the consequence. Increasing trends of mean annual temperature are consistent with the perception of the majority of the pastoralist who indicated increasing patterns of temperature and warming situation ( Table 2 ). According to Sejian, Gaughan, Bhatta, and Naqvi (2016) , a substantial decline in domestic animals performance inflicting heavy economic losses when animals are subjected to heat stress. Climate change especially increases in temperature, has a direct impact on increasing heat stress in animals ( Coffey, 2008 ; Valtorta, 2002 ). Similarly, Robinson, Strzepek, and Cervigni (2013) reported that surface Warming is expected to alter the feed intake, mortality, growth, reproduction, maintenance, and production of animals. Heat stress due to high temperature accompanied by excess humidity cause infertility in most of the farm species and adversely affecting the reproductive performance of farm animals ( Desalegn, 2016 ). Furthermore, comfortable environmental temperature ranges 20-25°C for sheep ( Khalek, 2007 ), 25-30°C for goat ( Lu, 1989 ), 5-20°C for cattle and 15 to 42°C for camel ( Barnes et al., 2004 ; DeShazer, 2009 ; Kerr, 2015 ; Khan, Arshad, & Riaz, 2003 ). According to ( Peters & Peters, 1986 ), small animals cool and loss heat faster than large animals, and they are sensitive to climate change. In this scenario, weather plays a significant role in determining the thermoneutral zone (TNZ) of livestock, which determines livestock productivity since adverse weather condition can yield production loss ( Kerr, 2015 ; Valtorta, 2002 ; Vining, 1990 ).
3.2.5. Trend analysis using Mann-Kendall's/ MK test
The level of significance, the magnitude of trend and change point of time series data detected using Mann-Kendall's, Sen's slope estimator and Pettitt's test, respectively ( Table 4 ). The Mann-Kendall's trend analysis applied to all seasons of the year (summer, autumn, winter and spring) and the entire years of the historical period. The MK test result for spring (the minor rainy season of the study area) and summer season rainfall data indicated non-significant increasing trend (p< 0.05). The result of Pettitt's test suggested that higher rainfall value recorded after October 2014 for spring and June 1994 for the summer season than before. The current finding revealed statistically non-significant decreasing trends of winter, autumn (the long rainy season of the study area) and annual rainfall (p< 0.05). According to the test made with Pettitt's change value, insignificant downward rainfall change value was recorded after February 2010, March 2010 and September 1993 for winter, summer and total annual rainfall, respectively. This finding is in line with a report from Asfaw et al. (2018) who discussed significant decreasing trends of annual long rainy season at 5 and 10% significance levels. In contrary to this finding Tsige (2018) reported significantly increasing annual main rainy season patterns of the rainfall.
Significance test using Mann-Kendall's
The MK test result for minimum and maximum temperature in this study showed significantly increasing (p< 0.05. The result of Pettitt's test indicated that significant change of maximum and minimum temperature after November 2006 and January 2000, respectively. Similarly, studies by Asfaw et al. (2018) and Tsige (2018) revealed significant increasing patterns of maximum and minimum temperature during the last three to four decades. According to IPCC (2019) , the changes of global surface air temperature over the land has considerably risen above 1.5 °C. According to FAO (2007) , nearly 20 to 30% of animal and plant species are at risk of extinction when the surface temperature increases by 1.5 to 2.5 °C.
3.3. Camel raising and its history among pastoral and agro-pastoral communities of East Guji Zone
Table 5 depicts the period at which they start to raise a camel as a farm animal. The result showed that most pastoralists (P<.0001, 33.93%) included the camel in their farm animal stock during the Boru Goyo Gada period (1984 – 1992) followed by Boru Madha (1992 - 2000) Gada Period (24.37%). Declining trend of rainfall, increasing patterns of temperature and intensified drought condition may be forced to shifting to camel rearing. Drought is one of the climate driven impacts and it is the primary determinant factor of livestock feed quality and availability. Climate change is the reason for increasing level of the invasive bush, poisonous (Xanthium, Parthenium hysterophorus L and Prosopis hysterophorus) and thorny plant species (Acacia mellifera and Acacia Senegal). Decreasing trends of palatable grass and browse species such as elephant grass and Acacia brevispica was also the primary factor of climatic change. Therefore, the factors that motivated pastoral communities to rear or owning camel during 1984-1992 was the high death rate of cattle, sheep and goat species during severe Ethiopian famine condition of 1983 – 84. Worst drought that occurred in 1983-85 significantly reduced livestock population and the death rate was as high as 90% ( Kebebew et al., 2001 ). According to Abebe et al. (2012) , increasing rate of poisonous, thorny and invasive bush and declining trends of grass plant species due to drought and erratic nature of rainfall in Borana rangeland forced pastoral and agro-pastoral communities to have more drought resistant browse livestock species like camel and goat.
Time period at which pastoralist starts to raise camel in the study area(Frequency (%)) as per wealth
Similarly, Sandford and Habtu (2000) reported significant decreasing rate (78%) of cattle population during 1985-87 in the Borana and Ethiopian Somali alongside Ethio-Kenyan border. For this reason, Guji and Borana pastoralists started to raise camels to cope with recurring drought condition. The Borana and Guji pastoralists still prefer raising cattle than camel. However, they also want to keep more camels and goat as they are safely supported by the changing ecological condition and have drought-resistant capacity ( Abebe et al., 2012 ). Similarly, Boru, Schwartz, Kam, and Degen (2014) showed significantly decreasing trends of cattle population due to drought and land desertification. As a result, Borana pastoralists are shifting to drought-resistant livestock species such as camel and goat.
Moreover, the key informant interviewee was confirmed that the result revealed through quantitative development. One of the interviewees expressed his emotion and experience in the face of drought challenge as follow
“I am Doyo Guyo, 61 years old, living in Liban woreda. We are Borana; Boranas are mainly cattle keeper. Before 1984, we had cattle, 325 goats, 34 sheep and no camel in our herd. We lost more than 200 cattle, 150 goat and 28 sheep during the severe drought in 1984. Our neighbour Ethio-Somali pastoralists are mainly camel herder who was rescued when we lost most of our assets during the drought period. At that time, we decide to exchange two cattle with one camel and owned at least two camels in 1984. The livestock death rate is now increasing than before because of drought caused by a decline in rainfall (amount, intensity and duration), limited grazing feed resources caused by bush encroachments, land degradation (loss of land productivity), new emerging livestock diseases and heat stresses. Our cattle number has been decreasing from 306 to 16 over the last six years. However, our camel population increased to 53; the camel is considered as a drought-resistant animal, can browse on thorny plant species, give us milk at least for household consumption, and our livelihood is now shifting from cattle to the camel raising.”
The relationship between climate variability and camel population in the study area over the years have showed an increasing pattern as per indicated in Fig. 7 . Average annual temperature and camel population are strongly correlated as annual temperature increases; camel population also tends to increase positively with correlation coefficient of 0.85 and p-value <0.001. Whereas, non-significant (p < 0.05) weak negative relationship between annual rainfall and camel population with correlation coefficient of -0.16 and p-value of 0.36 was observed. The camel population is being increasing with raising annual average temperature. The increasing pattern of camel population is attributed to decreasing patterns of rainfall and increasing trends of temperature, which in turn depresses grazing lands, increasing bush encroachments and browse feed resources. Therefore, herders decided to have more camels than cattle and small stocks to adapt to changing ecological condition. Current finding revealed that camel population is consistently increasing from 1984 to 2017 (from Jilo Aga to Guyo Goba Gada Periods).

The relationship between climate variability and camel population
“I am Naji Abdullah, 49 years old, living in Goro Dola Woreda. We inherited raising camel from our parent. Before 1984 we had more than 200 cattle, 184 goats, 20 sheep and three camels. It is heartbreaking, most of our cattle, sheep and goat lost because of drought and livestock diseases occurred in 1984. We have recovered, and our livestock population turn back just before 15 years, but in 2011 afterwards, we lost most of our cattle and goat once again. In 2008 we had 27 cattle, but now it declined to 13 head, and our goat population was decreased from 117 to 10 head within the last ten years. However, our camel population is being increased and now supporting us as food and income source. Nevertheless, our camel population and its productivity declined in the last four years due to disease and extreme desertification. We had 47 head of camel before six years, but now we have 16. Before 20 years, our family has one of the rich men but now am one of the low-income family and regimen for safety net program.”
Respondents declared that the main drivers for livestock decline in the study area is drought and drought-driven impacts ( Table 6 ). In general, the ranking of livestock decline drivers in Dida and Golba study locations showed that drought received high ranking. Respondents of the study area believed that diseases and shortage of grazing feed resources associated with drought incidence. Drought condition attributed to the shortage of available feed resources, leading to poor in body condition, which in turn diminish body immune system and make the animal easily exposed to the disease. The primary climate stresses in dryland areas are drought, inadequate water, heat and inadequate feed resources, which lowers immunity system of livestock and exposed them to new pathogens and vectors. The result of the current study agreed with the finding of ( Boru et al., 2014 ) who reported drought and land fragmentation drove livestock death and declining cattle population in eastern Guji zone.
Drivers for livestock decline in the study area
3.4. Effects of Drought on livestock production
3.4.1. livestock mortality.
People, plants and animals depend on the ecosystem services. However, climate change disrupting the provisioning and regulation services such as shrinking of food and water supply, and unable to control the diseases. Drought and drought-driven factors are the most common climate change impacts and chief the reasons for livestock death of the study areas Table 8 . indicates significantly higher (p < 0.01) number of camel (11 head/HH) death due to the emerging disease prevalence while drought was the major death factor of cattle. However, it was not statistically significant (p < 0.05) ( Table 7 ). Total number of 8627 cattle, 5648 goat and 9 camel were died during drought event at different periods and significantly differ across the livestock species (p < 0.001). Similarly, a significantly higher number of goats (40 head/HH) and cattle (34 head/ HH) died due to several disease occurrences than camels (11 head/HH) and statistically significant among the species (p ≤ 0.001).
Reason for Livestock death summary (mean ± Std.D)
*** indicates statistical significance at 1% level, letter superscript in parenthesis indicates statistical significance across the row and out of parenthesis shows column comparison.
H 0 : drought is the major determinant factors for death of all livestock species
Drought resilience ability of camel (mean ± Std.D)
* indicates statistical significance at 5% level; *** indicates significance level at 1%; Means within the same row (abc) and column abc bearing different superscripts are significantly different at p<0.01
The result indicates that significantly resilience ability of camel towards drought and emerging disease conditions. Nearly 11 camel/HH and 40 goat/HH died in average due to the disease while 1 camel/HH and 28 goat/HH died because of drought within the last ten years and there significantly differ among the death factors (p ≤ 0.001). However, the cattle death rate is not significant for both death factors ( Table 7 ). Cattle is the only livestock species that tends to have weaker resilience ability (high death rate) for both death factors. The result shows that camel is relatively the most resilient farm animals for both drought and disease among the livestock species. The mean drought livestock loss observed in this study is in line with the report of Dirriba Mengistu (2016) who showed a significant number of cattle death (68%) as compared to goat (5%) and camel (2%) during disastrous drought condition. This result is agreed with the report of Coppock (1994) ; Sandford and Habtu (2000) ; Kebebew et al. (2001) who reported decreasing cattle population by 90% in 1984-85, 60% in 1983-85 and 78% in 1995-97 drought events, respectively.
3.4.2. Milk production
The camel milk production potential was significantly influenced by the season of the year in the study area ( Table 8 ). Camel provides significantly higher amounts of daily milk yield during the wet season (≈ 7.2 litres/day) as compared to the dry season (3.32 litre/day) and significantly differ across the production seasons (p<0.01). Higher daily milk yield record during the wet season is associated with availability of better drinking water and feed. The result of the current study showed that significantly higher (p<0.01) milk yield of dairying camel at both dry periods. Daily milk yield of Camel > cattle > goats at both dry and wet season. Similarly, Kedija-Hussen, Tegegne, Kurtu, and Gebremedhin (2008) reported significantly higher mean daily milk yield of a camel across production seasons and dairying species (camel, cattle and goat).
This study found that lower daily milk yield of camel, cattle and goat during the dry period as compared to the wet season and significantly vary across species and season (p<0.01). Only camels continue to survive and produce milk in drought-stricken areas where drought decimate goat, cattle and sheep populations and hinder milk yielding capacities ( Alhadrami & Faye, 2016 ). Similarly, CSA (2018) reported 3.91 and 1.4 litres of mean daily milk yield of camel and cattle, respectively. The significant variation of mean daily milk yield across dairying livestock species in this study is convenient with the finding of Kedija-Hussen et al. (2008) who revealed that better milk yield potential of the camel at both dry and wet season. However, cattle's mean daily milk yield recorded in this study is lower than the report of Kedija-Hussen et al. (2008) who reported about 3.26 and 1.63 litres per day during the wet and dry condition, respectively. Location effect, climatic stress, genotypic variations, feeding and physiological status of the cattle might be the reason for the variation. The variation of mean daily milk yield of the camel as per the production season recorded in this study is in agreement with the finding of Zeleke (2007) .
The current finding revealed that the milk yield potential of dairy livestock in the study area shows the significant decreasing trends ( Fig. 8 ). The camel milk yield before 2008 is more than ten lit/day/head, showing decreased to about four lit/day/head in 2019. Similarly, cattle milk yield declined from about three lit/day/head to one lit/day/head. The reason for decreasing in milk yield may be related to intensive climatic stresses such as water scarcity, expanding desertification, recurring drought and heat stress. The focal group discussants underline that camel is better a milking animals and good milk yield at both dry and rainy season than cattle and goat. It is the camel, which can mainly support the family nutrition and household's livelihood during drought condition.

milk yield trends of camel, cattle and goat before ten years and currently in East Guji Zone (0.5L)
“I am Tari Dita, 33 years old, living in Gumi Eldallo Woreda near to Somali regional state border. We start raising camel about 17 years ago. In the beginning, we bought three camels from Somali pastoralists when the drought takes off most of our cattle. Just before 2005, we had more than 137 cattle, 42 goats, 28 sheep and three camels. However, our livestock population is dramatically decreasing to 31 cattle, 27 goats and 16 sheep, but our camel population is increasing, and now we have 47 head. We believe that the main driving factor of decreasing cattle, sheep and goat population is drought and drought-driven impacts such as conflict lack of water, heat stress and feed resources. Our cattle milk yield is not worthwhile; however, the camel milked up to two litres/day/head during surplus feeding resources and under suitable environmental conditions, which could be zero during the drought conditions. However, the camel milk yield is estimated to be 4-5 litre/day/head under the good environmental condition and pleasant feed availability. However, the milk yield potential declined during the drought condition. Nevertheless, the dairying camel can still give milk up to 2-3 litre/day under the harsh environmental condition and poor quality feed availability.”
3.5. Climate change coping strategy
The Fig. 9 displays farmers coping strategic measures taken to reduce the impacts of recurring drought. As a result, conservation of feed resources through circumscribing with a fence during the rainy season, and ample feed availability preferred as a primary coping mechanism as it indicated by 33% followed by herd mobility (25%) and shifting to browse livestock species (19%) in Golba study location. Whereas, herd mobility and migration during the disastrous drought period to where feed and water available area is the most preferred drought coping strategy as it indicated by 49% (p<0.01) followed by reducing livestock number through selling (20%) and shifting to camel raising (16%) in Dida study location.

Drought coping strategies used by pastoralist in East Guji Zone
The primary coping strategy observed in this study is in line with the result of Dirriba Mengistu and Haji (2016) and Opiyo, Wasonga, Nyangito, Schilling, and Munang (2015) who reported herd mobility and migration as a mutual drought coping strategy of Ethiopian Borana and Northern Kenyan pastoralists. In contrary, Tsige (2018) reported that reducing livestock number through selling is the leading climate change coping measures taken by the farmers of Hawassa Zuria woreda. Although Kebebew et al. (2001) in his report indicated that selling of cattle and other animals does not encourage by Gadaa system, this study shows that east Guji pastoralist attempt to sell some animals in good condition when they observe early stages of drought. In agreement to this study, Sandford and Habtu (2000) reported none of the pastoral groups in Ethiopia diversifies their livelihood option as a measure of drought coping strategy. However, Kebebew et al. (2001) revealed the attempt of income diversification in Borana pastoralists in order to avert the severe consequences of drought.
4. Conclusion
Pastoral/agro-pastoral communities in the study area had a perception of decreasing patterns of rainfall, increasing trends of temperature and drought intensities between 1986 and 2017 likewise there is strong meteorological evidence of the same climate trend direction during that period. This study revealed that increasing trends of intensities and frequency of drought occurrence in the study area. Accordingly, RAI identifies 13 drought years within the historical periods of 1986-2017, of which 54% (seven drought years) occurred within the last ten years (2007-2017).
Pastoralists/agro-pastoralists in the study area have encountered climate-related problems like rainfall/water accessibility, feed quality and availability, risk of animal disease, heat stress and hence, reduced livestock performance. Temporary migration/nomadism to areas with better pasture is the most commonly practicing coping strategy so far to address feed and water shortage. Moreover, reducing livestock number, reserve grazing/standing hay and shifting to browse livestock species (camel and goat) are also preferred coping mechanism to tackle climate-related problems. Camel and goats are perceived as drought-resistant livestock species and Boranas cattle keepers shifting to have more camel and goat in response to prevailing drought. Pastoral and agro-pastoral communities in the study area are well aware of climate change, variability, its trend direction, and its potential pessimistic impacts on their livelihood in the future. Cooperating adaptation programs of intended beneficiaries is very critical to develop the traditional coping mechanism of pastoralists/agro-pastoralists in response to pessimistic impacts of climate change. Moreover, extensive investigation is required to select and identify purpose-specific camel and goat strains for drought-prone areas.
This research was supported by Africa center of excellence for Climate Smart Agriculture and Biodiversity Conservation, Haramaya University in collaboration with World Bank Group.
Etical Statement
No potential Ethical Statement for this piece of work
Conflict of Interest Declaration
No potential conflicts of interest to declare
Acknowledgment
We thank Nigussie Zeray (PhD) for assistance with statistical analysis of some parameters of this research. We would also like to show our gratitude to Mr. Liban Boru (Dilla University), Mr. Mieso Kaweti (Haramya University, Climate Smart Agriculture PhD candidate), Mr. Guye Aga (East Guji Zone livestock and fisheries office), Dr. Wako Bonaya (Gumi Eldallo District Livestock health office) and Mr. Birhanu Admassu (Dilla University) for sharing their pearls of wisdom with us during the course of fieldwork.
- Abate T. Indigenous ecological knowledge and pastoralist perception on rangeland management and degradation in Guji Zone of south Ethiopia. Sustainable Development. 2016;(15):192–218. [ Google Scholar ]
- Abebe A., Eik L.O., Holand Ø., Ådnøy T., Tolera A. Pastoralists’ perceptions of feed scarcity and livestock poisoning in southern rangelands. Ethiopia. Journal Tropical animal health. 2012; 44 (1):149–157. [ PubMed ] [ Google Scholar ]
- Adi, S., & Swoboda-Reinhold, M. (2003). The Borana Pastoral production and Livelihood system (BPPLS), BLPDP/GTZ, Addis Ababa and Negelle/Borana. Retrieved from Addis Ababa:.
- Al-Amin A.Q., Alam G.M. The impacts of climate change on animal health and economy: A way forward for policy option. Asian J. Anim. Vet. Adv. 2011; 6 :1061–1068. [ Google Scholar ]
- Alhadrami, G., & Faye, B. (2016). Animals that produce dairy foods: Camel. In Reference Module in Food Science (pp. 1-12): Academic Press Amsterdam.
- Alhamshry A., Fenta A.A., Yasuda H., Kimura R., Shimizu K. Seasonal Rainfall Variability in Ethiopia and Its Long-Term Link to Global Sea Surface Temperatures. J Water. 2020; 12 (1):55. [ Google Scholar ]
- Anita, W., Dominic, M., & Neil, A. (2010). Climate change and agriculture impacts, adaptation and Mitigation: Impacts, adaptation and Mitigation: OECD publishing.
- Asfaw A., Simane B., Hassen A., Bantider A. Variability and time series trend analysis of rainfall and temperature in northcentral Ethiopia: A case study in Woleka sub-basin. Weather. 2018; 19 :29–41. [ Google Scholar ]
- ATPS Rationale and Capacity of Pastoral Community Innovative Adaptation to Climate Change in Ethiopia. African Technology Policy Studies Network. ATPS Research Paper. 2013; 25 (25) Nairobi, Kenya. [ Google Scholar ]
- AU-IBAR. (2016). Livestock Policy Landscape in Africa: A Review. Reinforcing Veterinary Governance in Africa. Nairobi, Kenya.
- Ayal D.Y., Leal-Filho W. Farmers' perceptions of climate variability and its adverse impacts on crop and livestock production in Ethiopia. Journal of Arid Environments. 2017; 140 :20–28. [ Google Scholar ]
- Bagath, M., Krishnan, G., Devaraj, C., Rashamol, V., Pragna, P., Lees, A., & Sejian, V. (2019). The impact of heat stress on the immune system in dairy cattle: A review. Research in veterinary science, 126, 9. doi:10.1016/j.rvsc.2019.08.011. [ PubMed ]
- Barnes A., Beatty D., Taylor E., Stockman C., Maloney S., McCarthy M. Physiology of heat stress in cattle and sheep. Meat Livestock Australia. 2004; 209 :1–36. [ Google Scholar ]
- Bekele F. Analysis of current rainfall variability and trends over Bale-Zone, South Eastern highland of Ethiopia. SF J Global Warming. 2017; 1 (2) [ Google Scholar ]
- Bernard, H. R., & Bernard, H. R. (2013). Social research methods: Qualitative and quantitative approaches: Sage.
- Bindoff N.L., Stott P.A., AchutaRao K.M., Allen M.R., Gillett N., Gutzler D., Jain S. Detection and attribution of climate change: from global to regional. The Physical Science Basis, TF IPCC, 2013 report. 2013 2013. [ Google Scholar ]
- Boru D., Schwartz M., Kam M., Degen A.A. Cattle reduction and livestock diversification among Borana pastoralists in southern Ethiopia. Nomadic Peoples. 2014; 18 (1):115–145. [ Google Scholar ]
- Bowley, A. L. (1925). Measurement of the precision attained in sampling: Cambridge University Press.
- Chris F., Jim R., Gary E., Emebet K., Nigist B., Libby W., Gideon G. Climate Trend Analysis of Ethiopia: Famine Early Warning Systems Network-Informing Climate Change Adaptation Series. Fact Sheet. 2012:3053. [ Google Scholar ]
- Coffey, S. G. (2008). A Systems Approach to Climate Change Impacts on Livestock Production. Retrieved from.
- Coppock, D. L. (1994). The Borana plateau of southern Ethiopia: Synthesis of pastoral research, development, and change, 1980-91 (Vol. 5): ILRI (aka ILCA and ILRAD).
- CSA Central Statistical Authority population estimates in Ethiopia, Addis Ababa. 2010. Agriculture Sample Survey 2009/2010 (2002E. C.) Volume I Report on Area and Production of Crops (Private Peasant holdings Meher Season) Statistical Bulletin. 2007:446. Addis Ababa. [ Google Scholar ]
- CSA. (2018). Agricultural Sample Survey 2017/18 [2010 E.C.]. Retrieved from ADDIS ABABA:.
- Desalegn K. The climate change impacts on livestock production: A Review. Global Veterinaria. 2016; 16 (2):206–212. [ Google Scholar ]
- DeShazer, J. A. (2009). Livestock energetics and thermal environmental management: American Society of Agricultural and Biological Engineers.
- Duncan D.B. Multiple range and multiple F tests. Biometrics. 1955; 11 (1):1–42. [ Google Scholar ]
- FAO. (2007). Adaptation to Climate Change in Agriculture, Forestry and Fisheries: Perspective, Framework and Priorities. Food Agriculture Organization. In. FAO Rome: Food Agriculture Organization.
- FDRE. (2007). Climate change adaptation programme of action (NAPA) of Ethiopia.
- Fereja G.B. The impacts of climate change on livestock production and productivities in developing countries: a review. International Journal of Research-Granthaalayah. 2016; 4 (8):181–187. [ Google Scholar ]
- Gashaw T., Asresie A., Haylom M. Climate change and livestock production in Ethiopia. Adv Life Sci Technol. 2014; 22 :39–42. [ Google Scholar ]
- Geilfus, F. (2008). 80 tools for participatory development: appraisal, planning, follow-up and evaluation (9290399104). Retrieved from.
- Gemedo-Dalle Isselstein, J., Maass B.L. Indigenous ecological knowledge of Borana pastoralists in southern Ethiopia and current challenges. The International Journal of Sustainable Development World Ecology. 2006; 13 (2):113–130. [ Google Scholar ]
- Getachew A.M. Drought and its impacts in Ethiopia. Weather and Climate Extremes. 2018; 22 :12. doi:doi.org/10.1016/j.wace.2018.10.002. [ Google Scholar ]
- Gomez, K. A., & Gomez, A. A. (1984). Statistical procedures for agricultural research: John Wiley & Sons.
- Hänsel S., Schucknecht A., Matschullat J. The Modified Rainfall Anomaly Index (mRAI)—is this an alternative to the Standardised Precipitation Index (SPI) in evaluating future extreme precipitation characteristics? Theoretical applied climatology. 2016; 123 (3-4):827–844. [ Google Scholar ]
- Hare, F. K. (1983). Climate and desertification: a revised analysis (Vol. 44): World Meteorological Organization.
- Herrero M., Thornton P.K., Notenbaert A.M., Wood S., Msangi S., Freeman H., van de Steeg J. Smart investments in sustainable food production: revisiting mixed crop-livestock systems. Science. 2010; 327 (5967):822–825. [ PubMed ] [ Google Scholar ]
- Hidosa D., Guyo M. Climate Change Effects on Livestock Feed Resources: A. Review. Fish. Livest Prod. 2017; 5 :259. [ Google Scholar ]
- Huang J., Zhang X., Zhang Q., Lin Y., Hao M., Luo Y., Wang L. Recently amplified arctic warming has contributed to a continual global warming trend. Nature climate change. 2017; 7 (12):875–879. [ Google Scholar ]
- Hussen-Ahimed, Biru, D., & Yadessa, B. (2011). Oromia National Regional State Program of plan on Adaptation to climate change Finfinne, Ethiopia: Oromia National Regional State Program.
- IPCC. (2014). Climate Change 2013: The physical science basis: Working group I contribution to the fifth assessment report of the Intergovernmental Panel on Climate Change: Cambridge University Press.
- IPCC Summary for Policymakers. In: Climate Change and Land: an IPCC special report on climate change, desertification, land degradation, sustainable land management, food security, and greenhouse gas fluxes in terrestrial ecosystems. Summary for Policymakers, In press. 2019; 36 https://www.ipcc.ch/srccl/ [ Google Scholar ]
- Israel, G. D. (1992). Determining sample size.
- Kebebew, F., Synnevaag, G., & Tsegaye, D. (2001). Traditional coping strategies of the Afar and Borana pastoralists in response to drought: Drylands Coordination Group.
- Kedija-Hussen, Tegegne, A., Kurtu, M. Y., & Gebremedhin, B. (2008). Traditional Cow an Camel Milk Production and Marketing in Agro-Pastoral and Mixed Crop-Livestock Systems: the Case of Mieso District, Oromia Regional State, Ethiopia: ILRI (aka ILCA and ILRAD).
- Kefyalew, A., & Tegegn, F. (2012). The effect of climate change on ruminant livestock population dynamics in Ethiopia. 24(10), 185.
- Kerr, S. R. (2015). Livestock heat stress: recognition, response, and prevention.
- Khalek T. Thermoregulatory responses of sheep to starvation and heat stress conditions. Egyptian Journal of Animal Production. 2007; 44 (2):137–150. [ Google Scholar ]
- Khan, B. B., Arshad, I., & Riaz, M. (2003). Production and management of camels: University of Agriculture, Faisalabad, Department of Livestock Management.
- Lu C. Effects of heat stress on goat production. Small Ruminant Research. 1989; 2 (2):151–162. doi: 10.1016/0921-4488(89)90040-0. [ CrossRef ] [ Google Scholar ]
- Lubroth J.J.B. Climate change and animal health. 2012; 23 :63. [ Google Scholar ]
- Mann H.B. Nonparametric tests against trend. Econometrica: Journal of the Econometric Society. 1945:245–259. [ Google Scholar ]
- McKee T.B., Doesken N.J., Kleist J. The relationship of drought frequency and duration to time scales. Paper presented at the Proceedings of the 8th Conference on Applied Climatology. 1993 [ Google Scholar ]
- Mebrahtu, S., Asgedom, K., & Hadush, A. (2017). Camel Milk Production and Marketing: Challenges and Opportunities in Afar Regional State, Ethiopia.
- Melees, W., & Samuel, S. (2017). Review on the Impact of El Niño-Southern Oscillation (ENSO) Climate Changes in Ethiopia.
- Melkamu B.N. The Effects of El Nino on Agricultural GDP of Ethiopia. American Journal of Water Science and Engineering. 2017; 3 (4):45–49. [ Google Scholar ]
- Mengistu D. Impacts of Drought and Conventional Coping Strategies of Borana Community, Southern Ethiopia. Research Journal on Humanities Social Sciences. 2016; 6 (23):37. [ Google Scholar ]
- Mengistu D., Haji J. Traditional Coping Strategies of Borana Pastoralists for Climate Extremes: A case of Yabello District, Borana Zone, Ethiopia. International Journal of Engineering Innovation & Research. 2016; 5 (3):7. [ Google Scholar ]
- Mirkena T., Walelign E., Tewolde N., Gari G., Abebe G., Newman S. Camel production systems in Ethiopia: a review of literature with notes on MERS-CoV risk factors. Pastoralism. 2018; 8 (1):30. [ PMC free article ] [ PubMed ] [ Google Scholar ]
- Morand, S. (2015). Impact of climate change on livestock disease occurrences. In Climate Change Impact on Livestock: Adaptation and Mitigation (pp. 113-122): Springer.
- Nicholson S.E., Funk C., Fink A.H. Rainfall over the African continent from the 19th through the 21st century. Global planetary change. 2018; 165 :114–127. [ Google Scholar ]
- NMA. (2015). Seasonal agro meteorological bulletin. Kiremt Seasonal Outlook for Ethiopia.
- O'Connor H., Gibson N. A step-by-step guide to qualitative data analysis. A Journal of Indigenous Aboriginal Community Health. 2003; 1 (1):63–90. [ Google Scholar ]
- Opiyo F., Wasonga O., Nyangito M., Schilling J., Munang R. Drought adaptation and coping strategies among the Turkana pastoralists of northern Kenya. nternational Journal of Disaster Risk Science. 2015; 6 (3):295–309. [ Google Scholar ]
- Osborn, T., Barichivich, J., Harris, I., Van Der Schrier, G., & Jones, P. (2018). Drought: Monitoring global drought using the self-calibrating Palmer Drought Severity Index [in" State of the Climate 2017"]. In B. o. t. A. M. Society (Ed.), Bulletin (Vol. 99, pp. S36-S37). USA: American Meteorological Society.
- Pachauri, R., & Reisinger, A. (2008). Climate change 2007. Synthesis report. Contribution of Working Groups I, II and III to the fourth assessment report. Retrieved from Cambridge University Press, Cambridge:.
- Palombi, L., & Sessa, R. (2013). Climate-smart agriculture: sourcebook. Rome, Italy: Food and Agriculture Organization of the United Nations.
- Peters, R. H., & Peters, R. H. (1986). The ecological implications of body size (Vol. 2): Cambridge University Press.
- Regassa, S., Givey, C., & Castillo, G. (2010). The rain doesn't come on time anymore: Poverty, vulnerability, and climate variability in Ethiopia. Oxfam Policy Practice: Climate Change Resilience, (6, 1).
- Robinson, S., Strzepek, K., & Cervigni, R. (2013). The cost of adapting to climate change in Ethiopia: Sector-wise and macro-economic estimates. Retrieved from.
- Sandford, S., & Habtu, Y. (2000). Emergency response interventions in pastoral areas of Ethiopia.
- Sejian V., Gaughan J., Bhatta R., Naqvi S. Impact of climate change on livestock productivity. Feedipedia-Animal Feed Resources Information System-INRA CIRAD AFZ, FAO. 2016:1–4. [ Google Scholar ]
- Simenew K., Dejen T., Tesfaye S., Fekadu R., Tesfu K., Fufa D. Characterization of camel production system in Afar pastoralists. North East Ethiopia. Asian Journal of Agricultural Sciences. 2013; 5 (2):16–24. [ Google Scholar ]
- Tefera M, Abebe G. The Camel in Ethiopia. Ethiopian Veterinary Association. 2012 M. Tefera & G. Abebe Eds. [ Google Scholar ]
- Temam D., Uddameri V., Mohammadi G., Hernandez E.A., Ekwaro-Osire S. Long-Term Drought Trends in Ethiopia with Implications for Dryland Agriculture. Water. 2019; 11 (12):2571. [ Google Scholar ]
- Teshome A., Zhang J. Increase of Extreme Drought over Ethiopia under Climate Warming. Advances in Meteorology. 2019 2019. [ Google Scholar ]
- Thornton P.K., Herrero M.T., Ericksen P.J. Livestock and climate change. Livestock Thematic Papers Tools for project design (pp. 20): International Fund for Agricultural Development (IFAD) 2011 [ Google Scholar ]
- Tsige, F. (2018). Impact of Climate Change on Livestock production and its Adaptation Strategies in Hawassa Zuria and Hula Districts of Sidama Zonen, Southern Ethiopia. (MSc Thesis submitted to the Program of climate change and sustainable agriculture), Hawassa University Unpublished.
- Ulrichs M., Slater R., Costella C. Building resilience to climate risks through social protection: from individualised models to systemic transformation. Disasters. 2019; 43 S368-S387. [ PMC free article ] [ PubMed ] [ Google Scholar ]
- UNFCCC. (2007). Climate change: impacts, vulnerabilities andadaptation in developing countries.
- Valtorta S.E. Animal production in a changing climate: impacts and mitigation. 2002:1–12. [ Google Scholar ]
- Van den Bossche P., Coetzer J. Climate change and animal health in. Africa. Rev Sci Tech. 2008; 27 (2):551–562. [ PubMed ] [ Google Scholar ]
- Van Rooy, M. (1965). A Rainfall Anomally Index Independent of Time and Space, Notos.
- Ververs M.-T. The East African food crisis: did regional early warning systems function? The Journal of nutrition. 2012; 142 (1):131–133. [ PubMed ] [ Google Scholar ]
- Vicente-Serrano S.M., Beguería S., López-Moreno J. A multiscalar drought index sensitive to global warming: the standardized precipitation evapotranspiration index. Journal of climate. 2010; 23 (7):1696–1718. [ Google Scholar ]
- Vining K.C. Effects of weather on agricultural crops and livestock: an overview. International journal of environmental studies. 1990; 36 (1-2):27–39. [ Google Scholar ]
- Wako G., Tadesse M., Angassa A. Camel management as an adaptive strategy to climate change by pastoralists in southern Ethiopia. Ecological Processes. 2017; 6 (1):1–12. [ Google Scholar ]
- Yamane, T. (1967). Statistics: An introductory analysis. Retrieved from.
- Yatoo M., Kumar P., Dimri U., Sharma M. Effects of climate change on animal health and diseases. International Journal of Livestock Research. 2012; 2 (3):15–24. [ Google Scholar ]
- Yilma, Z., Haile, A., GuerneBleich, E., & Ababa, A. (2009). Effect of climate change on livestock production and livelihood of pastoralists in selected pastoral areas of Borana, Ethiopia. ESAP Proceedings.
- Yosef T., Kefelegn K., Mohammed Y., Mengistu U., Solomon A., Tadelle D., Han J. Morphological diversities and eco-geographical structuring of Ethiopian camel (Camelus dromedarius) populations. Emirates Journal of Food Agriculture. 2014:371–389. [ Google Scholar ]
- Zeleke Z. Non-genetic factors affecting milk yield and milk composition of traditionally managed camels (Camelus dromedarius) in Eastern Ethiopia. Livestock Research for Rural Development. 2007; 19 (85):97. [ Google Scholar ]
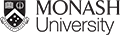
An assessment of climate variability and climate change on heat wave characteristics in India
Campus location, principal supervisor, additional supervisor 1, year of award, department, school or centre, additional institution or organisation, degree type, usage metrics.
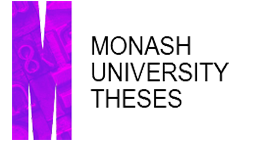
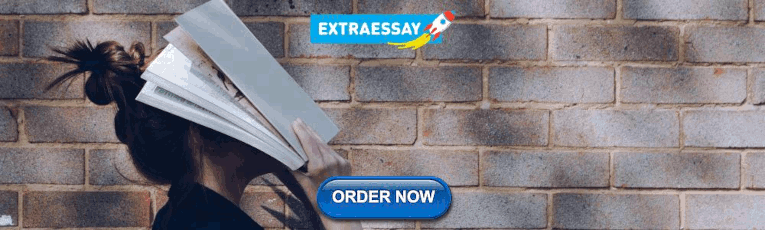
IMAGES
VIDEO
COMMENTS
Effects of climate variability and functional changes on carbon cycling in a temperate deciduous forest Wu, Jian Publication date: 2013 Document Version Publisher's PDF, also known as Version of record Link back to DTU Orbit Citation (APA): Wu, J. (2013). Effects of climate variability and functional changes on carbon cycling in a temperate ...
Secondly, the thesis adopted a participatory survey approach to quantify farmers' response to the factors influencing maize yield and adaptation to climate variability in the zone. A five-point Likert scale questionnaire of 35 items was designed, and 68 maize farmers were systematically sampled from communities in each zone.
iii Publications arising from this Thesis Peer review journal articles Guodaar, L., Bardsley, D.K. and Suh, J. (2021). Integrating local perceptions with scientific evidence to understand climate change variability in northern Ghana: A mixed-methods approach.
This thesis aims to explore the consequences of climate change impacts for development in the context of the increasing attention and recognition being given to the former. In particular, the thesis focuses on the local variability of climate impacts on rural households, on how the resilience of households to climate change impacts
IMPACTS OF CLIMATIC VARIABILITY, WATER SCARCITY AND SOCIO-ECONOMIC DEMOGRAPHICS ON FARMERS' MENTAL HEALTH IN AUSTRALIA Sahar Daghagh Yazd A thesis submitted for the degree of Doctor of Philosophy
Co-ADISOR: MULUKEN MEKURIYA (PhD) A THESIS RESULT SUBMITTED TO THE DEPARTMENT OF AGRO FORESTRY, WONDOGENET COLLEGE OF FORESTRY AND NATURAL RESOURCES, ... Co-advisor: Muluken Mekuriya (PhD) ABSTRACT Climate change and variability are worldwide phenomena which could exert adverse impact on agriculture mainly on crop production. The impacts create ...
Climate Variability and Farm-households in the Sudan Savannah Zone of Ghana Gerald Atampugre B.A. (Honours), University of Cape Coast, Ghana MPhil, University of Cape Coast, Ghana MSc. (Distinction), Wageningen University and Research, The Netherlands Thesis submitted for the Degree of Doctor of Philosophy
This dissertation looks at the adoption levels of various, potentially climate-smart agricultural practices by smallholder dairy farmers in Malawi, with the view of establishing the current level of engagement in these practices, and identifying the factors that influence adoption. Results show the importance of the socio-economic and ...
the adaptation of fishing communities to climate variability and change. The findings of this thesis form the basis for further detailed research into the vulnerability and adaptation of small-scale fishing communities to climate variability and change. Based on the above findings, this thesis also provides some suggestions for reducing
PhD thesis, December 2006 "Changes in Mediterranean Climate Extremes: Patterns, Causes, and Impacts of Change" ... is an inversion of a dendroclimatology technique that relies on spatial variability, with Principal Components Analysis (PCA) at its core. Radial Basis Function Artificial Neural Networking (RBF ANN) is a machine-learning pattern ...
Climate variability and poor distribution of rainfall often causes serious agricultural production losses and worsens food insecurity. Given that the direct effects of climate change and variability are transmitted through the agricultural sector, improving farm households' capacities to adapt to the adverse effects of climate-related shocks is an
V) Dissertation Requirements. The PhD dissertation of students participating in this Emphasis needs to have a strong focus in Climate Sciences and/or Climate Change. Furthermore, a member of the student's PhD committee needs to be a member of the core Faculty participating in the Emphasis in Climate Sciences and Climate Change.
climate variability and a warming trend in Sub-Saharan Africa, and particularly in East Africa, there have been changes towards extreme rainfall events, and an increase in seasonal mean temperature [3]. Such trends can bring about an impact on vegetation change and agricultural production, especially in
An understanding of climate variability, trends, and prediction for better water resource management and planning in a basin is very important. Since the water resources of the Lake Chad basin ...
This thesis seek to advance our knowledge in decadal precipitation variability of climate as well as its relationship to extremes. We do this by examining the cross timescale relationships between decadal mean precipitation and monthly-scale extremes; and how oceanic variability influences this relationship. Our key findings are as follows: 1. Decadal variability is most often regulated by ...
The determinants influencing farmers' perception of climate variability and change were estimated by the logit regression model, and results are presented in Table 2. Household demographic, socio-economic, institutional and biophysical characteristics were evaluated to predict how they influence the perception of climate variability and change.
1. Introduction. Increased rainfall variability and drought associated with climate change poses the greatest challenge to the food systems and sustainable agricultural development of sub-Saharan Africa (SSA) [1, 2] and to the region's food and nutrition security [].SSA is regarded as the most vulnerable region to the negative impacts of climate change, because of structural and ...
Using three climate scenarios, we project how climate change will cause AEZs to shift, causing changes in acreage and net revenue per hectare of cropland. Our results predict that Africa will suffer heavy annual welfare losses by 2070-2100, ranging between US$14 billion and US$70 billion, depending on the climate scenario and cropland measure ...
IPCC (2007) definition of vulnerability: - "the degree to which a system is susceptible to, and unable to cope with, adverse effects of climate change, including climate variability and extremes. Vulnerability is a function of the character, magnitude, and rate of climate change and variation to which a system is exposed, its.
2.3. Trends in Climate Variability 8 2.4. Impacts of Climate Variability in Ethiopia 9 2.5. Impact of Climate Variability on Crop Production 10 2.5.1. Impact of Climate Variability on Maize Productivity 10 2.5.1. Temperature variability and crop production 12 2.5.2. Rainfall variability and crop production 13 2.6. Smallholder Farmers ...
The climate variability alters plants' growth potential, deterioration of livestock feed resources 56 and livestock's physiological response. Increasing patterns of temperature and decreasing rainfall trends is a global phenomenon, pastoral and agro-pastoral communities who rely on natural resources for livestock production rigorously feel its ...
An understanding of heat wave characteristics is a pre-requisite to assess vulnerabilities due to heat waves. The primary objective of this thesis was to understand and analyze the climatic mechanisms that are driving changes in heat waves in India. Daily observed maximum temperature data and a combination of re-analysis data for upper air ...
The scientists study both the role of the ocean in the climate system and the impact of climate change on the ocean. Depending on the area of interest, climate research can be undertaken within existing JP focal areas or as interdisciplinary studies across multiple areas, including the atmosphere and the cryosphere.