- Skip to main content
- Accessibility information
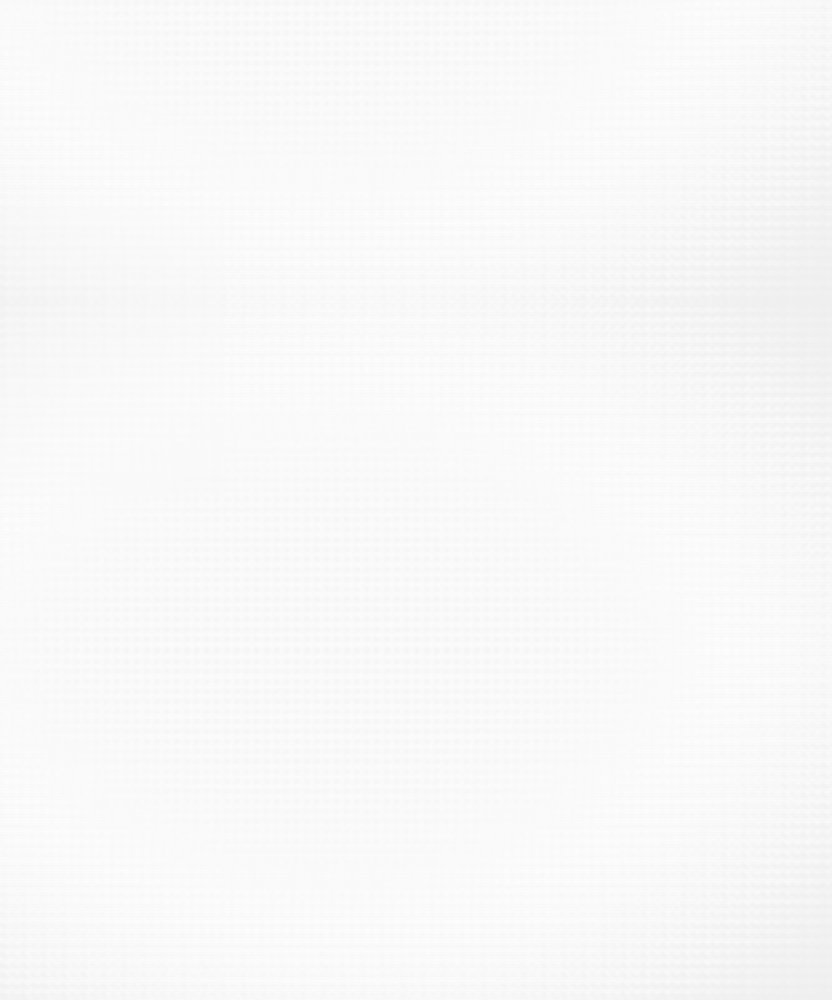
- Enlighten Enlighten
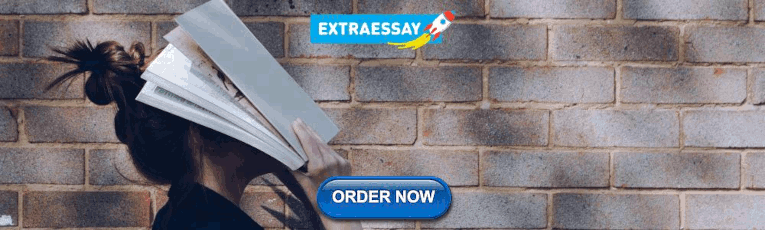
Enlighten Theses
- Latest Additions
- Browse by Year
- Browse by Subject
- Browse by College/School
- Browse by Author
- Browse by Funder
- Login (Library staff only)
In this section
Text-mining in macroeconomics: the wealth of words
Azqueta Gavaldon, Andres (2020) Text-mining in macroeconomics: the wealth of words. PhD thesis, University of Glasgow.
The coming to life of the Royal Society in 1660 surely represented an important milestone in the history of science, not least in Economics. Yet, its founding motto, ``Nullius in verba'', could be somewhat misleading. Words in fact may play an important role in Economics. In order to extract relevant information that words provide, this thesis relies on state-of-the-art methods from the information retrieval and computer science communities.
Chapter 1 shows how policy uncertainty indices can be constructed via unsupervised machine learning models. Using unsupervised algorithms proves useful in terms of the time and resources needed to compute these indices. The unsupervised machine learning algorithm, called Latent Dirichlet Allocation (LDA), allows obtaining the different themes in documents without any prior information about their context. Given that this algorithm is widely used throughout this thesis, this chapter offers a detailed while intuitive description of its underlying mechanics.
Chapter 2 uses the LDA algorithm to categorize the political uncertainty embedded in the Scottish media. In particular, it models the uncertainty regarding Brexit and the Scottish referendum for independence. These referendum-related indices are compared with the Google search queries ``Scottish independence'' and ``Brexit'', showing strong similarities. The second part of the chapter examines the relationship of these indices on investment in a longitudinal panel dataset of 2,589 Scottish firms over the period 2008-2017. It presents evidence of greater sensitivity for firms that are financially constrained or whose investment is to a greater degree irreversible. Additionally, it is found that Scottish companies located on the border with England have a stronger negative correlation with Scottish political uncertainty than those operating in the rest of the country. Contrary to expectations, we notice that investment coming from manufacturing companies appears less sensitive to political uncertainty.
Chapter 3 builds eight different policy-related uncertainty indicators for the four largest euro area countries using press-media in German, French, Italian and Spanish from January 2000 until May 2019. This is done in two steps. Firstly, a continuous bag of word model is used to obtain semantically similar words to ``economy'' and ``uncertainty'' across the four languages and contexts. This allows for the retrieval of all news-articles relevant to economic uncertainty. Secondly, LDA is again employed to model the different sources of uncertainty for each country, highlighting how easily LDA can adapt to different languages and contexts. Using a Bayesian Structural Vector Autoregressive set up (BSVAR) a strong heterogeneity in the relationship between uncertainty and investment in machinery and equipment is then documented. For example, while investment in France, Italy and Spain reacts heavily to political uncertainty shocks, in Germany it is more sensitive to trade uncertainty shocks.
Finally, Chapter 4 analyses English language media from Europe, India and the United States, augmented by a sentiment analysis to study how different narratives concerning cryptocurrencies influence their prices. The time span ranges from April 2013 to December 2018 a period where cryptocurrency prices experienced a parabolic behaviour. In addition, this case study is motivated by Shiller's belief that narratives around cryptocurrencies might have led to this price behaviour. Nonetheless, the relationship between narratives and prices ought to be driven by complex interactions. For example, articles written in the media about a specific phenomenon will attract or detract new investors depending on their content and tone (sentiment). Moreover, the press might also react to price changes by increasing the coverage of a given topic. For this reason, a recent causal model, Convergent Cross Mapping (CCM), suited to discovering causal relationships in complex dynamical ecosystems is used. I find bidirectional causal relationships between narratives concerning investment and regulation while a mild unidirectional causal association exists in narratives that relate technology and security to prices.
Actions (login required)
Downloads per month over past year
View more statistics

The University of Glasgow is a registered Scottish charity: Registration Number SC004401
Thank you for visiting nature.com. You are using a browser version with limited support for CSS. To obtain the best experience, we recommend you use a more up to date browser (or turn off compatibility mode in Internet Explorer). In the meantime, to ensure continued support, we are displaying the site without styles and JavaScript.
- View all journals
- Explore content
- About the journal
- Publish with us
- Sign up for alerts
- NEWS AND VIEWS
- 03 July 2019
Text mining facilitates materials discovery
- Olexandr Isayev 0
Olexandr Isayev is in the Eshelman School of Pharmacy, University of North Carolina at Chapel Hill, Chapel Hill, North Carolina 27599, USA.
You can also search for this author in PubMed Google Scholar
The total number of materials that can potentially be made — sometimes referred to as materials space — is vast, because there are countless combinations of components and structures from which materials can be fabricated. The accumulation of experimental data that represent pockets of this space has created a foundation for the emerging field of materials informatics, which integrates high-throughput experiments, computations and data-driven methods into a tight feedback loop that enables rational materials design. Writing in Nature , Tshitoyan et al . 1 report that knowledge of materials science ‘hidden’ in the text of published papers can be mined effectively by computers without any guidance from humans.
Access options
Access Nature and 54 other Nature Portfolio journals
Get Nature+, our best-value online-access subscription
24,99 € / 30 days
cancel any time
Subscribe to this journal
Receive 51 print issues and online access
185,98 € per year
only 3,65 € per issue
Rent or buy this article
Prices vary by article type
Prices may be subject to local taxes which are calculated during checkout
Nature 571 , 42-43 (2019)
doi: https://doi.org/10.1038/d41586-019-01978-x
Tshitoyan, V. at al. Nature 571 , 95–98 (2019).
Article Google Scholar
Mikolov, T., Sutskever, I., Chen, K., Corrado, G. S. & Dean, J. Proc. 26th Int. Conf. Neural Information Processing Syst. go.nature.com/2wvucor (2013).
Spangler, S. et al. Proc. 20th ACM SIGKDD Int. Conf. Knowledge Discovery Data Mining 1877–1886 (ACM, 2014); https://doi.org/10.1145/2623330.2623667
Google Scholar
Choi, B.-K. et al. Proc. Natl Acad. Sci. USA 115 , 10666–10671 (2018).
Article PubMed Google Scholar
Brown, F. K. Annu. Rep. Med. Chem. 33 , 375–384 (1998).
Butler, K. T., Davies, D. W., Cartwright, H., Isayev, O. & Walsh, A. Nature 559 , 547–555 (2018)
Download references
Reprints and permissions
Related Articles
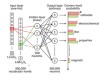
See all News & Views
- Materials science
- Computer science
Elastic films of single-crystal two-dimensional covalent organic frameworks
Article 08 MAY 24
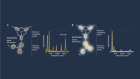
Vibration isolation could boost performance of near-infrared organic LEDs
News & Views 08 MAY 24
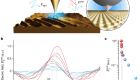
All-optical subcycle microscopy on atomic length scales
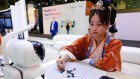
AI now beats humans at basic tasks — new benchmarks are needed, says major report
News 15 APR 24
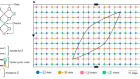
High-threshold and low-overhead fault-tolerant quantum memory
Article 27 MAR 24
Three reasons why AI doesn’t model human language
Correspondence 19 MAR 24
Faculty Positions at the Center for Machine Learning Research (CMLR), Peking University
CMLR's goal is to advance machine learning-related research across a wide range of disciplines.
Beijing, China
Center for Machine Learning Research (CMLR), Peking University
Faculty Positions at SUSTech Department of Biomedical Engineering
We seek outstanding applicants for full-time tenure-track/tenured faculty positions. Positions are available for both junior and senior-level.
Shenzhen, Guangdong, China
Southern University of Science and Technology (Biomedical Engineering)
Southeast University Future Technology Institute Recruitment Notice
Professor openings in mechanical engineering, control science and engineering, and integrating emerging interdisciplinary majors
Nanjing, Jiangsu (CN)
Southeast University
Staff Scientist
A Staff Scientist position is available in the laboratory of Drs. Elliot and Glassberg to study translational aspects of lung injury, repair and fibro
Maywood, Illinois
Loyola University Chicago - Department of Medicine
W3-Professorship (with tenure) in Inorganic Chemistry
The Institute of Inorganic Chemistry in the Faculty of Mathematics and Natural Sciences at the University of Bonn invites applications for a W3-Pro...
53113, Zentrum (DE)
Rheinische Friedrich-Wilhelms-Universität
Sign up for the Nature Briefing newsletter — what matters in science, free to your inbox daily.
Quick links
- Explore articles by subject
- Guide to authors
- Editorial policies
PhDs with Industry Partners – Assessing Collaboration and Topic Distribution Using a Text Mining Methodology
- First Online: 22 February 2022
Cite this chapter
- Kilian Buehling 6 &
- Matthias Geissler 7
Part of the book series: International Studies in Entrepreneurship ((ISEN,volume 52))
403 Accesses
2 Citations
Collaboration between universities and industry partners is thought to facilitate knowledge diffusion and provide resources and new ideas for academic researchers. However, recent evidence also suggests a possible trade-off or cost with regard to individual productivity. Given its focus on quantitative output, the literature is rather silent on possible qualitative shifts in researchers’ agendas when engaging with industry partners. We contribute to a discussion on potential negative effects of university-industry engagement by comparing the topic distributions of PhD theses based on collaborative and noncollaborative research. The results indicate little difference between the two kinds of dissertation projects. We conclude that fears of agenda setting in collaborative research are unwarranted.
This is a preview of subscription content, log in via an institution to check access.
Access this chapter
- Available as EPUB and PDF
- Read on any device
- Instant download
- Own it forever
- Compact, lightweight edition
- Dispatched in 3 to 5 business days
- Free shipping worldwide - see info
- Durable hardcover edition
Tax calculation will be finalised at checkout
Purchases are for personal use only
Institutional subscriptions
We are aware that our second restriction leaves room for discussion and leads to a somewhat blurred understanding of “collaboration”. However, constructing the training sample for the classification algorithm and subsequently doing a fair amount of manual classification convinced us that donating sample materials or allowing the use of equipment did not necessarily involve an element of knowledge exchange. Firms also engage in these “giveaways” for strategic reasons, for example to expand the diffusion of specific materials/equipment among the scientific community. Notwithstanding the fact that firms may benefit from this kind of activities to some extent, we believe it is not justified to label these actions as “collaboration”.
For topic distributions following “Cao” the results did not significantly differ from 0. As the three procedures were based on different methods to determine the optimal topic number, the “Cao” procedure seems to systematically estimate a lower number. The mean number of topics according to the “Cao” procedure was 5.5 in our setting, whereas the “Griffith” and “Arun” procedures presented an average of 14.3 and 14.1 topics, respectively.
In an unreported variant, we employed a university fixed-effect model with no significant effect on the estimated coefficients.
Initial fears of “collaborative” dissertations being treated differently may be soothed by talks at a practitioner conference that assure researchers that the German bureaucracy treats all dissertations equally.
Arun, R., Suresh, V., Veni Madhavan, C. E., & Narasimha Murthy, M. N. (2010). On finding the natural number of topics with latent Dirichlet allocation: Some observations. In M. J. Zaki, J. X. Yu, B. Ravindran, & V. Pudi (Eds.), Advances in knowledge discovery and data mining (pp. 391–402). Lecture Notes in Computer Science. Springer.
Chapter Google Scholar
Azagra-Caro, J. M., & Consoli, D. (2016). Knowledge flows, the influence of national R&D structure and the moderating role of public–private cooperation. The Journal of Technology Transfer, 41 (1), 152–172.
Article Google Scholar
Banal-Estañol, A., Jofre-Bonet, M., & Lawson, C. (2015). The double-edged Sword of industry collaboration: Evidence from engineering academics in the UK. Research Policy, 44 (6), 1160–1175.
Bikard, M., Vakili, K., & Teodoridis, F. (2018). When collaboration bridges institutions: The impact of university–industry collaboration on academic productivity. Organization Science., 30 , 426.
Blei, D. M., Ng, A. Y., & Jordan, M. I. (2003). Latent Dirichlet allocation. Journal of Machine Learning Research, 3 (Jan), 993–1022.
Google Scholar
Bruneel, J., D’Este, P., & Salter, A. (2010). Investigating the factors that diminish the barriers to university–industry collaboration. Research Policy, 39 (7), 858–868.
Cao, J., Xia, T., Li, J., Zhang, Y., & Tang, S. (2009). A density-based method for adaptive LDA model selection. Neurocomputing, 72 (7–9), 1775–1781.
Choi, H., Oh, S., Choi, S., & Yoon, J. (2018). Innovation topic analysis of technology: The case of augmented reality patents. IEEE Access, 6 , 16119–16137.
Cohen, W. M., Nelson, R. R., & Walsh, J. P. (2002). Links and impacts: The influence of public research on industrial R&D. Management Science, 48 (1), 1–23.
Espeland, W. N., & Sauder, M. (2007). Rankings and reactivity: How public measures recreate social worlds. American Journal of Sociology, 113 (1), 1–40.
Etzkowitz, H., & Leydesdorff, L. (2000). The dynamics of innovation: From National Systems and ‘Mode 2′ to a triple Helix of university–industry–government relations. Research Policy, 29 (2), 109–123.
Fudickar, R., Hottenrott, H., & Lawson, C. (2018). What’s the price of academic consulting? Effects of public and private sector consulting on academic research. Industrial and Corporate Change, 27 (4), 699–722.
Giles, C. L., & Councill, I. G. (2004). Who gets acknowledged: Measuring scientific contributions through automatic acknowledgment indexing. Proceedings of the National Academy of Sciences, 101 (51), 17599–17604.
Griffiths, T. L., & Steyvers, M. (2004). Finding scientific topics. Proceedings of the National Academy of Sciences, 101 (suppl 1), 5228–5235.
Hottenrott, H., & Lawson, C. (2014). Research grants, sources of ideas and the effects on academic research. Economics of Innovation and New Technology, 23 (2), 109–133.
Hottenrott, H., & Thorwarth, S. (2011). Industry funding of university research and scientific productivity. Kyklos, 64 (4), 534–555.
Jensen, R., & Thursby, M. (2001). Proofs and prototypes for sale: The licensing of university inventions. American Economic Review, 91 (1), 240–259.
Joachims, T. (2001). A statistical learning learning model of text classification for support vector machines. In Proceedings of the 24th annual international ACM SIGIR conference on research and development in information retrieval (pp. 128–136. SIGIR ‘01). ACM.
Korobkin, D., Fomenkov, S., Kravets, A., & Kolesnikov, S. (2017). Methods of statistical and semantic patent analysis. In A. Kravets, M. Shcherbakov, M. Kultsova, & P. Groumpos (Hrsg.), Creativity in intelligent technologies and data science (pp. 48–61). Springer International Publishing.
Laredo, P. (2007). Revisiting the third mission of universities: Toward a renewed categorization of university activities? Higher Education Policy, 20 (4), 441–456.
Lee, S., & Bozeman, B. (2005). The impact of research collaboration on scientific productivity. Social Studies of Science, 35 (5), 673–702.
Lee, Y. S. (2000). The sustainability of university-industry research collaboration: An empirical assessment. The Journal of Technology Transfer, 25 (2), 111–133.
Lodhi, H., Saunders, C., Shawe-Taylor, J., Cristianini, N., & Watkins, C. (2002). Text classification using string kernels. Journal of Machine Learning Research, 2 (Feb), 419–444.
Manjarrés-Henríquez, L., Gutiérrez-Gracia, A., Carrión-García, A., & Vega-Jurado, J. (2009). The effects of university–industry relationships and academic research on scientific performance: Synergy or substitution? Research in Higher Education, 50 (8), 795.
Manjarrés-Henríquez, L., Gutiérrez-Gracia, A., & Vega-Jurado, J. (2008). Coexistence of university-industry relations and academic research: Barrier to or incentive for scientific productivity. Scientometrics, 76 (3), 561–576.
Merton, R. K. (1968). The Matthew Effect in science: The reward and communication systems of science are considered. Science, 159 (3810), 56–63.
Morichika, N., & Shibayama, S. (2016). Use of dissertation data in science policy research. Scientometrics, 108 (1), 221–241.
Murray, F., & Stern, S. (2007). Do formal intellectual property rights hinder the free flow of scientific knowledge?: An empirical test of the anti-commons hypothesis. Journal of Economic Behavior & Organization , Academic Science and Entrepreneurship: Dual engines of growth , 63 (4), 648–687.
Ooms, W., Werker, C., & Hopp, C. (2018). Moving up the ladder: Heterogeneity influencing academic careers through research orientation, gender, and mentors. Studies in Higher Education, 44 , 1–22.
Paul-Hus, A., Mongeon, P., Sainte-Marie, M., & Larivière, V. (2017). The sum of it all: Revealing collaboration patterns by combining authorship and acknowledgements. Journal of Informetrics, 11 (1), 80–87.
Perkmann, M., Tartari, V., McKelvey, M., Autio, E., Broström, A., D’Este, P., Fini, R., et al. (2013). Academic engagement and commercialisation: A review of the literature on university–industry relations. Research Policy, 42 (2), 423–442.
Perkmann, M., & Walsh, K. (2009). The two faces of collaboration: Impacts of university-industry relations on public research. Industrial and Corporate Change, 18 (6), 1033–1065.
Salimi, N., Bekkers, R., & Frenken, K. (2015). Does working with industry come at a price? A study of doctoral candidates’ performance in collaborative vs. non-collaborative Ph.D. projects. Technovation, 41–42 (July), 51–61.
Shane, S. A. (2004). Academic entrepreneurship: University spinoffs and wealth creation . Edward Elgar Publishing.
Book Google Scholar
Sun, A., Lim, E. P., & Liu, Y. (2009). On strategies for imbalanced text classification using SVM: A comparative study. Decision Support Systems, 48 (1), 191–201.
Suominen, A., Toivanen, H., & Seppänen, M. (2017). Firms’ knowledge profiles: Mapping patent data with unsupervised learning. Technological Forecasting and Social Change, 115, 131–142.
Tartari, V., & Breschi, S. (2012). Set them free: Scientists’ evaluations of the benefits and costs of university–industry research collaboration. Industrial and Corporate Change, 21 (5), 1117–1147.
Van Looy, Bart, M. R., Callaert, J., Debackere, K., & Zimmermann, E. (2004). Combining entrepreneurial and scientific performance in academia: Towards a compounded and reciprocal Matthew-effect? Research Policy, 33 (3), 425–441.
Von Hayek, & August, F. (1989). The pretence of knowledge. The American Economic Review, 79 (6), 3–7.
Wei, X., & Croft, W. B. (2006). LDA-based document models for Ad-Hoc retrieval. In Proceedings of the 29th annual international ACM SIGIR conference on research and development in information retrieval (pp. 178–185). ACM.
Woltmann, S., Clemmensen, L. H., & Alkærsig, L. (2016). From university research to innovation detecting knowledge transfer via text mining. In 21st international conference on science and technology indicators (STI 2016) science and technology indicators conference .
Yan, E., Ding, Y., Milojević, S., & Sugimoto, C. R. (2012). Topics in dynamic research communities: An exploratory study for the field of information retrieval. Journal of Informetrics, 6 (1), 140–153.
Yau, C.-K., Porter, A., Newman, N., & Suominen, A. (2014). Clustering scientific documents with topic modeling. Scientometrics, 100 (3), 767–786.
Zucker, L. G., & Darby, M. R. (1996). Star scientists and institutional transformation: Patterns of invention and innovation in the formation of the biotechnology industry. Proceedings of the National Academy of Sciences, 93 (23), 12709–12716.
Download references
Acknowledgments
This article benefitted from the comments and suggestions made by participants of the Technology Transfer Society Conference 2018 in Valencia, Spain, the 12th Workshop on Organisation, Economics and Policy of Scientific Research 2018 in Bath, UK and several appreciated colleagues throughout the research process. The Stifterverband fuer die Deutsche Wissenschaft provided research funding for Kilian Buehling via its INNcentive grant, which we gratefully acknowledge. The authors would also like to thank the reviewers for very helpful comments.
Author information
Authors and affiliations.
Technische Universität Dresden, Research Group Knowledge and Technology Transfer, Dresden, Germany
Kilian Buehling
Rationalisierungs- und Innovationszentrum der Deutschen Wirtschaft e. V., RKW Kompetenzzentrum, Digitalization and Innovation Section, Eschborn, Germany
Matthias Geissler
You can also search for this author in PubMed Google Scholar
Corresponding author
Correspondence to Kilian Buehling .
Editor information
Editors and affiliations.
INGENIO [CSIC-UPV], Universitat Politecnica de Valencia, Valencia, Spain
Joaquín M. Azagra-Caro
Universitat Politecnica de Valencia, Valencia, Valencia, Spain
Pablo D'Este
David Barberá-Tomás
Rights and permissions
Reprints and permissions
Copyright information
© 2022 Springer Nature Switzerland AG
About this chapter
Buehling, K., Geissler, M. (2022). PhDs with Industry Partners – Assessing Collaboration and Topic Distribution Using a Text Mining Methodology. In: Azagra-Caro, J.M., D'Este, P., Barberá-Tomás, D. (eds) University-Industry Knowledge Interactions. International Studies in Entrepreneurship, vol 52. Springer, Cham. https://doi.org/10.1007/978-3-030-84669-5_2
Download citation
DOI : https://doi.org/10.1007/978-3-030-84669-5_2
Published : 22 February 2022
Publisher Name : Springer, Cham
Print ISBN : 978-3-030-84668-8
Online ISBN : 978-3-030-84669-5
eBook Packages : Business and Management Business and Management (R0)
Share this chapter
Anyone you share the following link with will be able to read this content:
Sorry, a shareable link is not currently available for this article.
Provided by the Springer Nature SharedIt content-sharing initiative
- Publish with us
Policies and ethics
- Find a journal
- Track your research
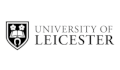
Text mining analysis on Pubmed data base
Due to the increasing amount of unstructured text data, information retrieval from large volumes of data has become highly important. Applying most of the algorithms, such as classification and clustering, is challenging because of the high dimensionality of the text data.
This study investigates a novel, co-occurrence model of text data to help reduce the dimension of the data set. We present a graph-based text mining approach for discovering similar documents in a scientific corpus and use it in a search engine that is built into the R Shiny web application. The Biological Scientific Corpus (BSC) is a collection of 764,213 PubMed-indexed English abstracts of research papers and proceedings papers, chosen to reflect the widest range of abstracts of scientific works published in 2012. Analysis of the co-occurrence matrix helps to understand the feature of interconnection between the words. Applying the community detection method, we discovered hubs and strong communities in the co-occurrence network and use them to reduce the dimension of the network.
After dimension reduction, we produced meaningful clusters of the data set. To see whether or not the clustering is correct we investigated the distribution of the authors of the papers over the clusters and the results were satisfactory. Finally, we used a hierarchal approach to develop a search engine on the data set that accepts a query from a user and responds with a set of retrieved documents. The main advantage of this search engine is the ability to take long text, and abstracts, as a query.
Another part of this work is to reproduce the well-known Elastic Map algorithm in R as an open resource for data visualization. We used the R Elastic Map package we developed to present a zoomable and rotatable visualization of a map fitted to clustered data in a two and three-dimensional space.
Supervisor(s)
Date of award, author affiliation, awarding institution, qualification level, qualification name, usage metrics.
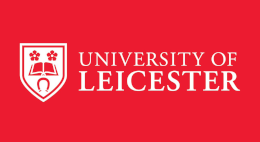
- Uncategorized
Academia.edu no longer supports Internet Explorer.
To browse Academia.edu and the wider internet faster and more securely, please take a few seconds to upgrade your browser .
Enter the email address you signed up with and we'll email you a reset link.
- We're Hiring!
- Help Center
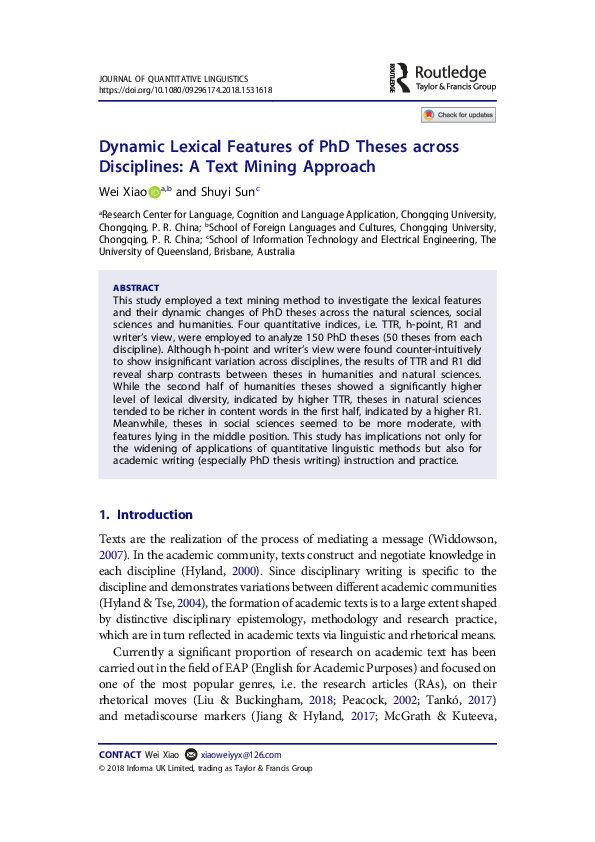
Dynamic Lexical Features of PhD Theses across Disciplines: A Text Mining Approach

2020, Journal of Quantitative Linguistics
This study employed a text mining method to investigate the lexical features and their dynamic changes of PhD theses across the natural sciences, social sciences and humanities. Four quantitative indices, i.e. TTR, h-point, R1 and writer's view, were employed to analyze 150 PhD theses (50 theses from each discipline). Although h-point and writer's view were found counter-intuitively to show insignificant variation across disciplines, the results of TTR and R1 did reveal sharp contrasts between theses in humanities and natural sciences. While the second half of humanities theses showed a significantly higher level of lexical diversity, indicated by higher TTR, theses in natural sciences tended to be richer in content words in the first half, indicated by a higher R1. Meanwhile, theses in social sciences seemed to be more moderate, with features lying in the middle position. This study has implications not only for the widening of applications of quantitative linguistic methods but also for academic writing (especially PhD thesis writing) instruction and practice.
Related Papers
International Journal of English Linguistics
Dr. Rafique Ahmed Memon
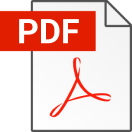
Glottometrics
Shuyi Amelia Sun
As an innovative and systematic genre in the academic community, Ph.D. theses have been heatedly researched in the field of English for Academic Purposes. Although research on the functional and formal features of Ph.D. theses has been abundant, their stylometric traits regarding textual activity have not been explored. Accordingly, this study explored the textual activity of Ph.D. theses and its dynamic changes across natural sciences, social sciences and humanities. A total of 150 Ph.D. theses (50 from each discipline) were analyzed, and the and χ 2 values were calculated to determine the textual activity of theses as well as its dynamic changes with the progression of texts. The results showed that, although the theses were found to be active in general, significant differences across disciplines do exist, in that the theses in natural sciences and humanities were more active while those in social sciences were more likely to lean towards the descriptive mode. This study has implications for widening the scope of cross-disciplinary academic genre analyses from an innovative quantitative linguistic perspective.
Luiz Mesquita
Open Linguistics
Shuyi Amelia Sun , Peter Crosthwaite
The past few decades have witnessed an upsurge of scholarly interest in the generic descriptions of PhD theses following Swales' seminal Genre Analysis. Fitted within the Create A Research Space (CARS) model, the thesis introduction plays a key role in justifying research originality/significance, where novice writers engage with academic communities through "establishing a research territory" (Move 1), "establishing a niche" (Move 2), and "occupying the niche" (Move 3). As the hinge of the CARS model, Move 2 (hereinafter EN) is of strategic importance as it enables writers to "sell" their ideas by pointing to the gap/ niche in the "marketplace" of previous research, which is typically realized through the co-occurrences of negation alongside other interpersonal language resources. Negation, as a disclaim marker within Martin and White's appraisal framework, is a prominent linguistic indicator of EN. Nevertheless, little research has systematically examined the use of negation in ENs of PhD thesis introductions. Accordingly, the study investigated negation via the appraisal framework addressing subtypes of negation (disalignment, cautious detachment, unfulfilled expectation) within ENs in the introduction sections of 120 PhD theses. The results showed that disalignment is the most frequent subtype of negation, while "not" and "no" are commonly used as indicators of negation. Our findings also revealed intriguing co-occurrences of negation sub-categories alongside other relevant appraisal resources. The corpus-informed results are expected to shed light on the nature and practice of PhD theses that educators may take into account during thesis writing instruction.
Akshey Sharma
LSP International Journal
Hadina Habil
In measuring the quality of written text, especially academic writing, lexical features are as important as grammatical features and should not be ignored. The highly computable nature of lexicons can make them a good criterion for determining and measuring the quality of text. In this article three lexical features: lexical density, complexity, and formality are reviewed and justified as measurement tools of academic texts. Furthermore, a measurement method is offered to evaluate lexical complexity level of an academic text.
Dr. Musarrat Azher (Fulbrighter)
With the concept of language variation, it has become utmost important to analyze linguistic patterns across register. Pakistani academic writing like other registers in Pakistan is an area that still seeks the attention of the researchers and linguists. This target register needs to be fully described in terms of linguistic characteristics to strengthen the distinct identity of Pakistani academic writing as a register. The present research strives to explore linguistic variation across research sections of Pakistani academic writing as a register along with five new textual dimensions explored through the technique of Multidimensional analysis (Azher & Mehmood, 2016). The research is based on the corpus of 235 M. Phil and PhD research dissertations taken from different universities all over Pakistan. The corpus was further divided into five research sections and was tagged for 189 linguistic features. The ANOVA results on variation among research sections indicate that there lie statistically significant differences among research sections of Pakistani Academic Writing on all the new textual dimensions.
chan swee heng
The present paper reviews the use of lexical bundles in academic writing from two different viewpoints namely linguistic and discipline, directed at how academic writers belonging to different disciplines or linguistic backgrounds construct their discourses through lexical bundles. As cohesive devices, lexical bundles are an indispensible part of the text and play a crucial role in shaping propositions, evolving the text, guiding readers through the flow of information and gaining the writer's proffered meaning. By using lexical bundles, academic writers are able to attain naturalness in their writings and create a more reader-friendly approach to the unfolding text. Bearing the significance of lexical bundles in mind, this review paper aims to examine the effect of disciplinary variation and linguistic differences on the use of lexical bundles in academic writing. Most researchers believe that the frequency as well as the use of lexical bundles is different across disciplines a...
Teaching and Learning to Co-create
Merve Gecikli
This paper presents a contrastive analysis of the introduction sections of 20 PhD theses in Turkish and in English in the field of English Language Teaching (ELT). The main aim of the study is to explore whether the authors from different academic institutions within the same discourse community performed the same rhetorical strategies in the introduction parts of doctorate dissertations. The study proceeded on a qualitative research design, through a content analysis including both genre and discourse analysis, which was carried on the basis of CARS model 2004 version under the scope of Swalesian approach. In general, the findings on the employment frequencies of each rhetorical strategy show that, although the dissertations have been written by the authors within the same discourse community (the realm of ELT), and context-Turkish context-, English thesis introductions have a more complex rhetorical organization than the introductory parts of Turkish theses.
RELATED PAPERS
Larasyah Ayu Lestari Fiero
Magnetic Resonance Imaging
soheil hanna
sitiayu magita.hsc5
Latin American Journal of Pharmacy
Silvia Inés Cazorla
Forray R. Katalin
dewi nurjanah
Research Papers in Economics
Arina Motova
Journal of Pediatric Nursing
Manal Kassab
Nordic Pulp & Paper Research Journal
Torbjörn Wahlström
World Development
Laurie DeRose
Pain Medicine
David Fishbain
Proceedings of the 1st Economics and Business International Conference 2017 (EBIC 2017)
faisal matriadi
Marc Mangel
Guillermo F . Carrión Páez
Takashi SHIGA
DOAJ (DOAJ: Directory of Open Access Journals)
José Borello
Reports of Practical Oncology & Radiotherapy
Edyta Wolny-Rokicka
Pharmaceuticals
Madalena Pinto
Kaygı. Uludağ Üniversitesi Fen-Edebiyat Fakültesi Felsefe Dergisi
Zeynep Berke
CHEST Journal
Hossein Almassi
Clinical Immunology
Mario Clerici
Tasya Aspiranti
Coastal Management
Elizabeth Turner
Science and Spiritual Healing: A Critical Review of …
RELATED TOPICS
- We're Hiring!
- Help Center
- Find new research papers in:
- Health Sciences
- Earth Sciences
- Cognitive Science
- Mathematics
- Computer Science
- Academia ©2024
- Our Promise
- Our Achievements
- Our Mission
- Proposal Writing
- System Development
- Paper Writing
- Paper Publish
- Synopsis Writing
- Thesis Writing
- Assignments
- Survey Paper
- Conference Paper
- Journal Paper
- Empirical Paper
- Journal Support
- PhD Projects in Text Mining
“Text mining is a scholarly process of regaining fine info from large datasets.”
PhD projects in text mining are the premier research service for PhD/MS scholars.
At present, it applies to data retrieval, named entity recognition, pattern recognition, and more.
LATEST TEXT MINING RESEARCH NOTIONS
- Collection and management of documents from the web
- Deduplication and cleansing techniques
- Digital libraries and archives preservation systems
- Massive data collection and synchronization
- document and index representation
- Text analytics techniques for fast data retrieval
- Entity/noun phrase extraction for web mining
- User customized web search techniques
- Auto-categorization and auto-metadata generation
- Relevant feedback based searching systems
- Natural language processing approaches
Apart from this, we assist you in other data mining fields too . Once you get a tie-up with us, then PhD projects in text mining give “A to Z” service in your project life cycle. Not only this but we also ready to provide you other services.
Your time is good, so only you reach us today…If you want your time to be good throughout your trip, then join with us right now…
PhD Projects in text mining
On the one hand, we are effective in designing apt problem-solving solutions . On the other hand, we assure you that we will take full responsibility to enhance your research quality . To be sure, we will prove these words in real at the end.
An effective function for Neural Named based on Entity Recognition and Multi-Type Normalization Tool aimed at Biomedical Text Mining
An innovative scheme for Pattern Based on Comprehensive Urdu Stemmer and Short Text Classification
The novel mechanism for Unstructured Text Resource Access Control Attribute Mining Technology Based on Convolutional Neural Network
A new mechanism for Distributed Framework used for Automating Opinion Discretization From Text Corpora on Facebook scheme
On the use of Data Mining Analysis based on Digital Watermarking Method used for Text Document Protection
An inventive performance for Ontology Driven Feature Engineering aimed at Opinion Mining
A new-fangled method for Ensemble Data Reduction Techniques and Multi-RSMOTE via Fuzzy Integral for Bug Report Classification
The novel methodology function for Roles of Review Numerical and Textual Characteristics based on Review Helpfulness Across 3 Different Types of Evaluations
An inventive thing for Generating Multimedia Storyline aimed at Effective Disaster Information Awareness scheme
An efficient mechanism for Person Entity Attribute Extraction Based on Siamese Network
A novel methodology function for Single Attention-Based on Combination of CNN and RNN for Relation Classification practice
The novel performance for Gesture Recognition Based on CNN and DCGAN for Calculation and Text Output scheme
An effectual function for Overview of Co-Clustering by Matrix Factorization system
An innovative mechanism for DEEP-HEAR function based on Multimodal Subtitle Positioning System Dedicated into Deaf and Hearing-Impaired People
The novel method for Semantic-Emotion Neural Network aimed at Emotion Recognition from Text scheme
An effectual process for Entity Linking based on Chinese Microblogs via Deep Neural Network
The novel mechanism for Multi-class sentiment analysis based on twitter
An innovative performance for Matching Descriptions into Spatial Entities By a Siamese Hierarchical Attention Network scheme
An inventive system for Web Services Classification Based on Wide & Bi-LSTM Model
The new method for Big Data Software Engineering Using LDA-Based on Topic Modeling practice
MILESTONE 1: Research Proposal
Finalize journal (indexing).
Before sit down to research proposal writing, we need to decide exact journals. For e.g. SCI, SCI-E, ISI, SCOPUS.
Research Subject Selection
As a doctoral student, subject selection is a big problem. Phdservices.org has the team of world class experts who experience in assisting all subjects. When you decide to work in networking, we assign our experts in your specific area for assistance.
Research Topic Selection
We helping you with right and perfect topic selection, which sound interesting to the other fellows of your committee. For e.g. if your interest in networking, the research topic is VANET / MANET / any other
Literature Survey Writing
To ensure the novelty of research, we find research gaps in 50+ latest benchmark papers (IEEE, Springer, Elsevier, MDPI, Hindawi, etc.)
Case Study Writing
After literature survey, we get the main issue/problem that your research topic will aim to resolve and elegant writing support to identify relevance of the issue.
Problem Statement
Based on the research gaps finding and importance of your research, we conclude the appropriate and specific problem statement.
Writing Research Proposal
Writing a good research proposal has need of lot of time. We only span a few to cover all major aspects (reference papers collection, deficiency finding, drawing system architecture, highlights novelty)
MILESTONE 2: System Development
Fix implementation plan.
We prepare a clear project implementation plan that narrates your proposal in step-by step and it contains Software and OS specification. We recommend you very suitable tools/software that fit for your concept.
Tools/Plan Approval
We get the approval for implementation tool, software, programing language and finally implementation plan to start development process.
Pseudocode Description
Our source code is original since we write the code after pseudocodes, algorithm writing and mathematical equation derivations.
Develop Proposal Idea
We implement our novel idea in step-by-step process that given in implementation plan. We can help scholars in implementation.
Comparison/Experiments
We perform the comparison between proposed and existing schemes in both quantitative and qualitative manner since it is most crucial part of any journal paper.
Graphs, Results, Analysis Table
We evaluate and analyze the project results by plotting graphs, numerical results computation, and broader discussion of quantitative results in table.
Project Deliverables
For every project order, we deliver the following: reference papers, source codes screenshots, project video, installation and running procedures.
MILESTONE 3: Paper Writing
Choosing right format.
We intend to write a paper in customized layout. If you are interesting in any specific journal, we ready to support you. Otherwise we prepare in IEEE transaction level.
Collecting Reliable Resources
Before paper writing, we collect reliable resources such as 50+ journal papers, magazines, news, encyclopedia (books), benchmark datasets, and online resources.
Writing Rough Draft
We create an outline of a paper at first and then writing under each heading and sub-headings. It consists of novel idea and resources
Proofreading & Formatting
We must proofread and formatting a paper to fix typesetting errors, and avoiding misspelled words, misplaced punctuation marks, and so on
Native English Writing
We check the communication of a paper by rewriting with native English writers who accomplish their English literature in University of Oxford.
Scrutinizing Paper Quality
We examine the paper quality by top-experts who can easily fix the issues in journal paper writing and also confirm the level of journal paper (SCI, Scopus or Normal).
Plagiarism Checking
We at phdservices.org is 100% guarantee for original journal paper writing. We never use previously published works.
MILESTONE 4: Paper Publication
Finding apt journal.
We play crucial role in this step since this is very important for scholar’s future. Our experts will help you in choosing high Impact Factor (SJR) journals for publishing.
Lay Paper to Submit
We organize your paper for journal submission, which covers the preparation of Authors Biography, Cover Letter, Highlights of Novelty, and Suggested Reviewers.
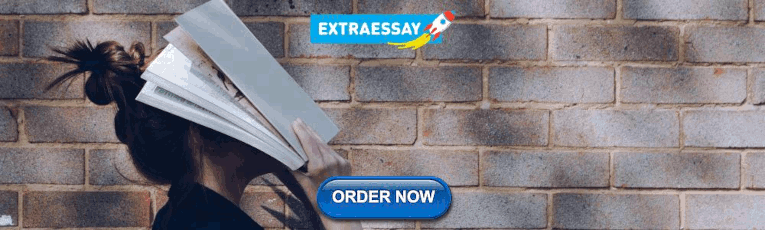
Paper Submission
We upload paper with submit all prerequisites that are required in journal. We completely remove frustration in paper publishing.
Paper Status Tracking
We track your paper status and answering the questions raise before review process and also we giving you frequent updates for your paper received from journal.
Revising Paper Precisely
When we receive decision for revising paper, we get ready to prepare the point-point response to address all reviewers query and resubmit it to catch final acceptance.
Get Accept & e-Proofing
We receive final mail for acceptance confirmation letter and editors send e-proofing and licensing to ensure the originality.
Publishing Paper
Paper published in online and we inform you with paper title, authors information, journal name volume, issue number, page number, and DOI link
MILESTONE 5: Thesis Writing
Identifying university format.
We pay special attention for your thesis writing and our 100+ thesis writers are proficient and clear in writing thesis for all university formats.
Gathering Adequate Resources
We collect primary and adequate resources for writing well-structured thesis using published research articles, 150+ reputed reference papers, writing plan, and so on.
Writing Thesis (Preliminary)
We write thesis in chapter-by-chapter without any empirical mistakes and we completely provide plagiarism-free thesis.
Skimming & Reading
Skimming involve reading the thesis and looking abstract, conclusions, sections, & sub-sections, paragraphs, sentences & words and writing thesis chorological order of papers.
Fixing Crosscutting Issues
This step is tricky when write thesis by amateurs. Proofreading and formatting is made by our world class thesis writers who avoid verbose, and brainstorming for significant writing.
Organize Thesis Chapters
We organize thesis chapters by completing the following: elaborate chapter, structuring chapters, flow of writing, citations correction, etc.
Writing Thesis (Final Version)
We attention to details of importance of thesis contribution, well-illustrated literature review, sharp and broad results and discussion and relevant applications study.
How PhDservices.org deal with significant issues ?
1. novel ideas.
Novelty is essential for a PhD degree. Our experts are bringing quality of being novel ideas in the particular research area. It can be only determined by after thorough literature search (state-of-the-art works published in IEEE, Springer, Elsevier, ACM, ScienceDirect, Inderscience, and so on). SCI and SCOPUS journals reviewers and editors will always demand “Novelty” for each publishing work. Our experts have in-depth knowledge in all major and sub-research fields to introduce New Methods and Ideas. MAKING NOVEL IDEAS IS THE ONLY WAY OF WINNING PHD.
2. Plagiarism-Free
To improve the quality and originality of works, we are strictly avoiding plagiarism since plagiarism is not allowed and acceptable for any type journals (SCI, SCI-E, or Scopus) in editorial and reviewer point of view. We have software named as “Anti-Plagiarism Software” that examines the similarity score for documents with good accuracy. We consist of various plagiarism tools like Viper, Turnitin, Students and scholars can get your work in Zero Tolerance to Plagiarism. DONT WORRY ABOUT PHD, WE WILL TAKE CARE OF EVERYTHING.
3. Confidential Info
We intended to keep your personal and technical information in secret and it is a basic worry for all scholars.
- Technical Info: We never share your technical details to any other scholar since we know the importance of time and resources that are giving us by scholars.
- Personal Info: We restricted to access scholars personal details by our experts. Our organization leading team will have your basic and necessary info for scholars.
CONFIDENTIALITY AND PRIVACY OF INFORMATION HELD IS OF VITAL IMPORTANCE AT PHDSERVICES.ORG. WE HONEST FOR ALL CUSTOMERS.
4. Publication
Most of the PhD consultancy services will end their services in Paper Writing, but our PhDservices.org is different from others by giving guarantee for both paper writing and publication in reputed journals. With our 18+ year of experience in delivering PhD services, we meet all requirements of journals (reviewers, editors, and editor-in-chief) for rapid publications. From the beginning of paper writing, we lay our smart works. PUBLICATION IS A ROOT FOR PHD DEGREE. WE LIKE A FRUIT FOR GIVING SWEET FEELING FOR ALL SCHOLARS.
5. No Duplication
After completion of your work, it does not available in our library i.e. we erased after completion of your PhD work so we avoid of giving duplicate contents for scholars. This step makes our experts to bringing new ideas, applications, methodologies and algorithms. Our work is more standard, quality and universal. Everything we make it as a new for all scholars. INNOVATION IS THE ABILITY TO SEE THE ORIGINALITY. EXPLORATION IS OUR ENGINE THAT DRIVES INNOVATION SO LET’S ALL GO EXPLORING.
Client Reviews
I ordered a research proposal in the research area of Wireless Communications and it was as very good as I can catch it.
I had wishes to complete implementation using latest software/tools and I had no idea of where to order it. My friend suggested this place and it delivers what I expect.
It really good platform to get all PhD services and I have used it many times because of reasonable price, best customer services, and high quality.
My colleague recommended this service to me and I’m delighted their services. They guide me a lot and given worthy contents for my research paper.
I’m never disappointed at any kind of service. Till I’m work with professional writers and getting lot of opportunities.
- Christopher
Once I am entered this organization I was just felt relax because lots of my colleagues and family relations were suggested to use this service and I received best thesis writing.
I recommend phdservices.org. They have professional writers for all type of writing (proposal, paper, thesis, assignment) support at affordable price.
You guys did a great job saved more money and time. I will keep working with you and I recommend to others also.
These experts are fast, knowledgeable, and dedicated to work under a short deadline. I had get good conference paper in short span.
Guys! You are the great and real experts for paper writing since it exactly matches with my demand. I will approach again.
I am fully satisfied with thesis writing. Thank you for your faultless service and soon I come back again.
Trusted customer service that you offer for me. I don’t have any cons to say.
I was at the edge of my doctorate graduation since my thesis is totally unconnected chapters. You people did a magic and I get my complete thesis!!!
- Abdul Mohammed
Good family environment with collaboration, and lot of hardworking team who actually share their knowledge by offering PhD Services.
I enjoyed huge when working with PhD services. I was asked several questions about my system development and I had wondered of smooth, dedication and caring.
I had not provided any specific requirements for my proposal work, but you guys are very awesome because I’m received proper proposal. Thank you!
- Bhanuprasad
I was read my entire research proposal and I liked concept suits for my research issues. Thank you so much for your efforts.
- Ghulam Nabi
I am extremely happy with your project development support and source codes are easily understanding and executed.
Hi!!! You guys supported me a lot. Thank you and I am 100% satisfied with publication service.
- Abhimanyu
I had found this as a wonderful platform for scholars so I highly recommend this service to all. I ordered thesis proposal and they covered everything. Thank you so much!!!
Related Pages
Phd Projects In Wordnet
Phd Projects In Rtool
Phd Projects In Web Mining
Phd Projects In It
Phd Projects In Web Technology
Phd Projects In Java
Phd Projects In Multimedia
Phd Projects In Python
Phd Projects In Webservice
Phd Projects In Weka
Phd Projects In Image Mining
Phd Projects In Cse
Phd Research Topics In Data Mining
Phd Projects In Computer Graphics
Phd Projects In Computer Science Engineering
Dynamic Lexical Features of PhD Theses across Disciplines: A Text Mining Approach
Author: xiao, wei, author: sun, shuyi.
This study employed a text mining method to investigate the lexical features and their dynamic changes of PhD theses across the natural sciences, social sciences and humanities. Four quantitative indices, i.e. TTR, h-point, R1 and writer’s view, were employed to analyze 150 PhD theses (50 theses from each discipline). Although h-point and writer’s view were found counter-intuitively to show insignificant variation across disciplines, the results of TTR and R1 did reveal sharp contrasts between theses in humanities and natural sciences. While the second half of humanities theses showed a significantly higher level of lexical diversity, indicated by higher TTR, theses in natural sciences tended to be richer in content words in the first half, indicated by a higher R1. Meanwhile, theses in social sciences seemed to be more moderate, with features lying in the middle position. This study has implications not only for the widening of applications of quantitative linguistic methods but also for academic writing (especially PhD thesis writing) instruction and practice.
PhD Research Topics in Text Mining
Text mining is a crucial extraction of hidden and useful information from large datasets. It opens up the research on automatic and semantic knowledge discovery .
PhD research topics in text mining are a hub of a terrific amount of creative ideas for PhD/MS scholars. We help you to work on each corner of the research. It ends up in promising findings and execution.
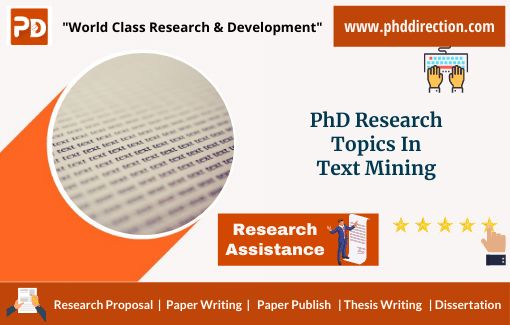
Recent PhD/MS Concepts in Text Mining
Text preprocessing.
- Perceptual grouping
- PoS tagging and stemming
- Full and shallow parsing
- Entity and relation extraction
- Conference resolution
- Syntactic/semantic analysis
Feature extraction and selection
- Fuzzy theory
- Entropy method
- Mutual Information theory
- BFO, ACO, BFO
Classification
- Supervised ( like ANN, SVM, Multi-kernel regression and also CNN)
- Unsupervised (like K-means, K-means++, KNN and also APN)
- Reinforcement learning
“ Web 3.0 ” is a new wave of the internet that uses semantic text mining. To address this growth, our PhD research topics in text mining cover all aspects of this area. And, we assure you that we direct you on the right path to complete your research.
Some Emerging Research Topics in Text Mining
- Social sentiment analysis
- Opinion and also in frequent item mining
- Mining from complex lexical structures
- Tools for profiling digital games
- Ontology and also in corpus based mining
- Secure and also in privacy preservation
- Also in Information retrieval from cloud/fog
We know that as a novice, shaping your research scope will be a big deal for you. To draw your research scope, join with us at any point. Once you get to connect with us, then we will predict your scope of the research. Since we also have been working with thousands of researchers.
Last, of all, we are here to assist you in the phases of your research. So, please make use of us at any point in your research.
Take a glance over following new ideas of PhD research topics in text mining,
An effectual function for Drug-Drug Interaction Extraction Based on Transfer Weight Matrix and Memory Network system
The novel mechanism for Category Theory-Based Mobile User Interface Pattern Recommendation Method
An efficient mechanism for Business Process Analytics and Big Data Systems
The new-fangled mechanism for Fast genre classification of web images using global and local features
An innovative performance for Integrating Deep Learning Approaches for Identifying News Reprint Relation scheme
An inventive scheme for Sensitive Information Topics-Based on Sentiment Analysis Method for Big Data
An effectual process for Semantic-aware Visual Abstraction of Large-scale Social Media Data with Geo-Tags
An innovative performance for Social Media Based on Topic Modeling Correlation Analysis Method
An effective performance for Survey of Sentiment Analysis Based on Transfer Learning scheme
An effectual function for Detecting Regions of Maximal Divergence intended for Spatio-Temporal Anomaly Detection method
The novel approach for Multi-Scale Attentive Interaction Networks system
An inventive process for Multiple-Perspective Semantics-Crossover Model for Matching Sentences system
An innovative performance for Bootstrapping Approach With CRF and Deep Learning Models for Improving the Biomedical Named Entity Recognition in Multi-Domains
An Unsupervised Approach of Truth Discovery from Multi-Sourced Text Data scheme
An innovative mechanism for Artificial Intelligence Driven Multi-Feature Extraction Scheme aimed at Big Data Detection
An effectual source for Gastroenterology Ontology Construction By Synonym Identification and Relation Extraction
A new mechanism for Trajectory big data processing based on frequent activity
An inventive process for Active Learning for Uneven Noisy Labeled Data in Mention-Level Relation Mining
An effectual function for Semantic Clustering-Based on Deep Hypergraph Model aimed at Online Reviews Semantic Classification in Cyber-Physical-Social Systems
The novel mechanism for Automatic Knowledge Discovery in Lecturing Videos via Deep Representation scheme
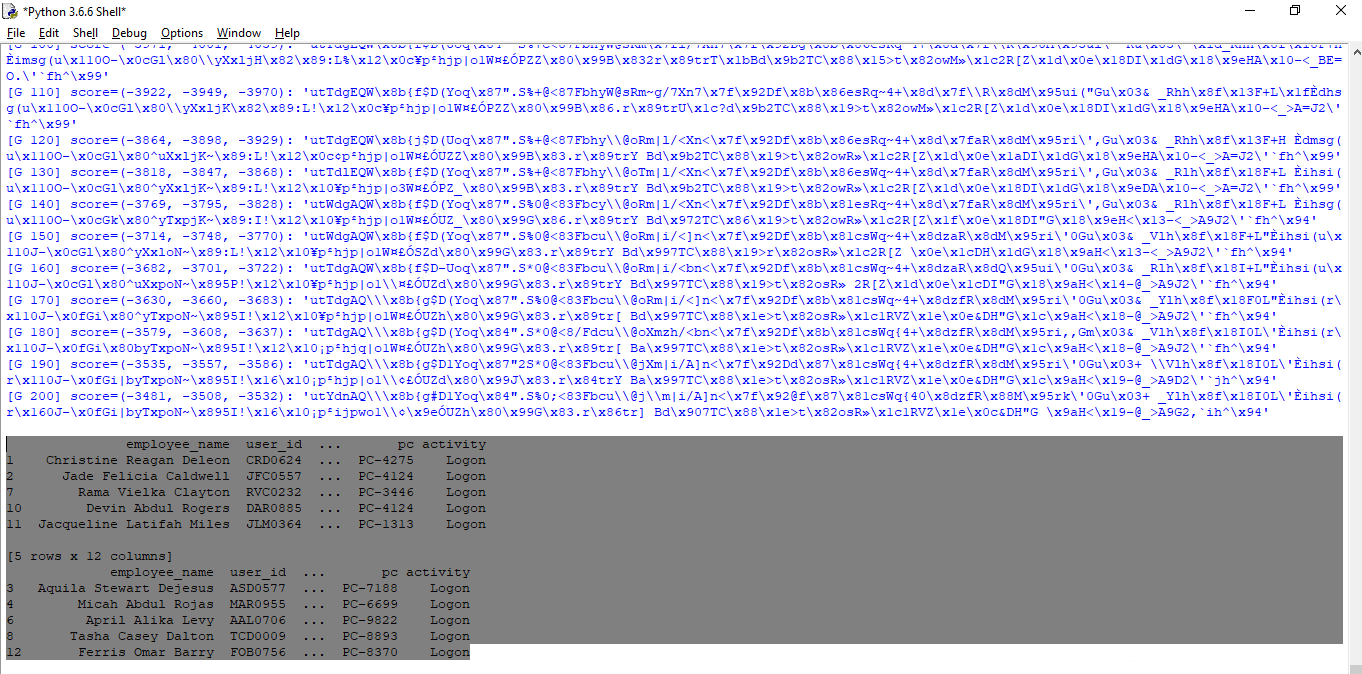
Why Work With Us ?
Senior research member, research experience, journal member, book publisher, research ethics, business ethics, valid references, explanations, paper publication, 9 big reasons to select us.
Our Editor-in-Chief has Website Ownership who control and deliver all aspects of PhD Direction to scholars and students and also keep the look to fully manage all our clients.
Our world-class certified experts have 18+years of experience in Research & Development programs (Industrial Research) who absolutely immersed as many scholars as possible in developing strong PhD research projects.
We associated with 200+reputed SCI and SCOPUS indexed journals (SJR ranking) for getting research work to be published in standard journals (Your first-choice journal).
PhDdirection.com is world’s largest book publishing platform that predominantly work subject-wise categories for scholars/students to assist their books writing and takes out into the University Library.
Our researchers provide required research ethics such as Confidentiality & Privacy, Novelty (valuable research), Plagiarism-Free, and Timely Delivery. Our customers have freedom to examine their current specific research activities.
Our organization take into consideration of customer satisfaction, online, offline support and professional works deliver since these are the actual inspiring business factors.
Solid works delivering by young qualified global research team. "References" is the key to evaluating works easier because we carefully assess scholars findings.
Detailed Videos, Readme files, Screenshots are provided for all research projects. We provide Teamviewer support and other online channels for project explanation.
Worthy journal publication is our main thing like IEEE, ACM, Springer, IET, Elsevier, etc. We substantially reduces scholars burden in publication side. We carry scholars from initial submission to final acceptance.
Related Pages
Phd Research Topics In Image Mining
Phd Research Topics In Weka
Phd Research Topics In Rtool
Phd Research Topics In Webservice
Phd Research Topics In Scilab
Phd Research Topics In Information Security
Phd Research Topics In Video Streaming
Phd Research Topics In Nlp
Phd Research Topics In Video Processing
Phd Research Topics In Web Technology
Phd Research Topics In Visual Cryptography
Phd Research Topics In Web Mining
Phd Research Topics In Information Technology
Phd Research Topics In Information Forensics Security
Phd Research Topics In Natural Language Processing
Our Benefits
Throughout reference, confidential agreement, research no way resale, plagiarism-free, publication guarantee, customize support, fair revisions, business professionalism, domains & tools, we generally use, wireless communication (4g lte, and 5g), ad hoc networks (vanet, manet, etc.), wireless sensor networks, software defined networks, network security, internet of things (mqtt, coap), internet of vehicles, cloud computing, fog computing, edge computing, mobile computing, mobile cloud computing, ubiquitous computing, digital image processing, medical image processing, pattern analysis and machine intelligence, geoscience and remote sensing, big data analytics, data mining, power electronics, web of things, digital forensics, natural language processing, automation systems, artificial intelligence, mininet 2.1.0, matlab (r2018b/r2019a), matlab and simulink, apache hadoop, apache spark mlib, apache mahout, apache flink, apache storm, apache cassandra, pig and hive, rapid miner, support 24/7, call us @ any time, +91 9444829042, [email protected].
Questions ?
Click here to chat with us
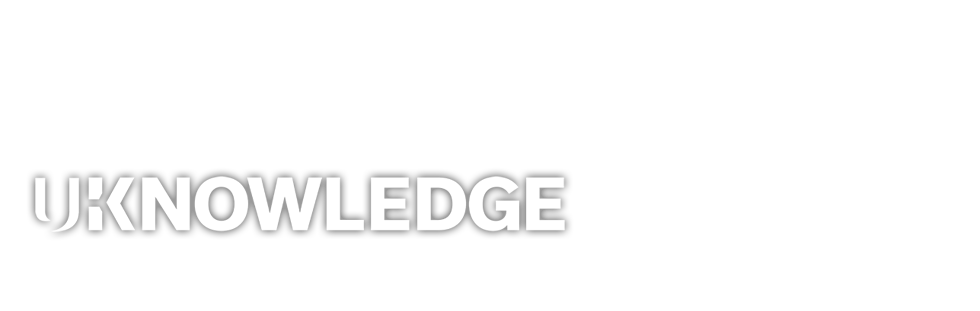
UKnowledge > College of Engineering > Mining Engineering > Theses & Dissertations
Theses and Dissertations--Mining Engineering
Theses/dissertations from 2024 2024.
THE METHODOLOGY FOR INTEGRATING ROBOTIC SYSTEMS IN UNDEGROUND MINING MACHINES , Peter Kolapo
DISCRETE ELEMENT MODELING TO PREDICT MUCKPILE PROFILES FROM CAST BLASTING , Russell Lamont
AUTONOMOUS SHUTTLE CAR DOCKING TO A CONTINUOUS MINER USING RGB-DEPTH IMAGERY , Sky Rose
Theses/Dissertations from 2023 2023
ASSESSMENT OF AIR OVERPRESSURE FROM BLASTING USING COMPUTATIONAL FLUID DYNAMICS , Cecilia Estefania Aramayo
RECOVERY OF VALUABLE METALS FROM ELECTRONIC WASTE USING A NOVEL AMMONIA-BASED HYDROMETALLURGICAL PROCESS , Peijia Lin
AN ACID BAKING APPROACH TO ENHANCE RARE EARTH ELEMENT RECOVERY FROM BITUMINOUS COAL SOURCES , Ahmad Nawab
PREDICTION OF DYNAMIC SUBSIDENCE IN THE PROXIMITY OF LONGWALL PANEL BOUNDARIES , JESUS DAVID ROMERO BENITEZ
Prediction of Blast-Induced Ground Vibrations: A Comparison Between Empirical and Artificial-Neural-Network Approaches , Luis F. Velasquez
A LABORATORY AND NUMERICAL INVESTIGATION OF THE STRENGTH OF IRREGULARLY SHAPED PILLARS , Zachary Wedding
Theses/Dissertations from 2022 2022
DEVELOPMENT OF UNIVARIATE AND MULTIVARIATE FORECASTING MODELS FOR METHANE GAS EMISSIONS IN UNDERGROUND COAL MINES , Juan Diaz
PARAMETRIC NUMERICAL ANALYSIS OF INCLINED COAL PILLARS , Robin Flattery
Strain Energy Analysis Related To Strata Failure During Caving Operations , Caroline Gerwig
LAPTOP RECYCLING CASE STUDY: ESTIMATING THE CONTAINED VALUE AND VALUE RECOVERY PROCESS FEASIBILITY OF END-OF-LIFE CONSUMER ELECTRONICS , Zebulon Hart
INVESTIGATION INTO, & ANALYSIS OF TEMPERATURE & STRAIN DATA FOR COAL MINE SEAL MATERIAL DURING CURING , Stephanus Jaco van den Berg
Theses/Dissertations from 2021 2021
DEVELOPMENT OF AN AUTONOMOUS NAVIGATION SYSTEM FOR THE SHUTTLE CAR IN UNDERGROUND ROOM & PILLAR COAL MINES , Vasileios Androulakis
Investigation of Coal Burst Potential Using Numerical Modeling and Rock Burst Indices , Cristian David Cardenas Triana
Capture of Respirable Dust using Maintenance Free Impingement Screen , Neeraj Kumar Gupta
OXIDATION PRETREATMENT FOR ENHANCED LEACHABILITY OF RARE EARTH ELEMENTS FROM BITUMINOUS COAL SOURCES , Tushar Gupta
AN APPROACH FOR PREDICTING FLOW CHARACTERISTICS AT THE CONTINUOUS MINER FACE , Kayla Henderson
CONCEPTS FOR DEVELOPMENT OF SHUTTLE CAR AUTONOMOUS DOCKING WITH CONTINUOUS MINER USING 3-D DEPTH CAMERA , Sibley Miller
MODELING OF RARE EARTH SOLVENT EXTRACTION PROCESS FOR FLOWSHEET DESIGN AND OPTIMIZATION , Vaibhav Kumar Srivastava
Application of a Novel Ventilation Simplification Algorithm , Caitlin V. Strong
A METHODOLOGY FOR AUTONOMOUS ROOF BOLT INSTALLATION USING INDUSTRIAL ROBOTICS , Anastasia Xenaki
Theses/Dissertations from 2020 2020
NUMERICAL APPROXIMATION OF THE GROUND REACTION AND SUPPORT REACTION CURVES FOR UNDERGROUND LIMESTONE MINES , Jesus Castillo Gomez
Advanced Search
- Notify me via email or RSS
Browse by Author
- Collections
- Disciplines
Author Corner
- Submit Research
New Title Here
Below. --> connect.
- Law Library
- Special Collections
- Copyright Resource Center
- Graduate School
- Scholars@UK
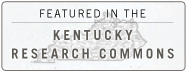
- We’d like your feedback
Home | About | FAQ | My Account | Accessibility Statement
Privacy Copyright
University of Kentucky ®
An Equal Opportunity University Accreditation Directory Email Privacy Policy Accessibility Disclosures
- Open access
- Published: 08 May 2024
Measurement and analysis of change in research scholars’ knowledge and attitudes toward statistics after PhD coursework
- Mariyamma Philip 1
BMC Medical Education volume 24 , Article number: 512 ( 2024 ) Cite this article
102 Accesses
Metrics details
Knowledge of statistics is highly important for research scholars, as they are expected to submit a thesis based on original research as part of a PhD program. As statistics play a major role in the analysis and interpretation of scientific data, intensive training at the beginning of a PhD programme is essential. PhD coursework is mandatory in universities and higher education institutes in India. This study aimed to compare the scores of knowledge in statistics and attitudes towards statistics among the research scholars of an institute of medical higher education in South India at different time points of their PhD (i.e., before, soon after and 2–3 years after the coursework) to determine whether intensive training programs such as PhD coursework can change their knowledge or attitudes toward statistics.
One hundred and thirty research scholars who had completed PhD coursework in the last three years were invited by e-mail to be part of the study. Knowledge and attitudes toward statistics before and soon after the coursework were already assessed as part of the coursework module. Knowledge and attitudes towards statistics 2–3 years after the coursework were assessed using Google forms. Participation was voluntary, and informed consent was also sought.
Knowledge and attitude scores improved significantly subsequent to the coursework (i.e., soon after, percentage of change: 77%, 43% respectively). However, there was significant reduction in knowledge and attitude scores 2–3 years after coursework compared to the scores soon after coursework; knowledge and attitude scores have decreased by 10%, 37% respectively.
The study concluded that the coursework program was beneficial for improving research scholars’ knowledge and attitudes toward statistics. A refresher program 2–3 years after the coursework would greatly benefit the research scholars. Statistics educators must be empathetic to understanding scholars’ anxiety and attitudes toward statistics and its influence on learning outcomes.
Peer Review reports
A PhD degree is a research degree, and research scholars submit a thesis based on original research in their chosen field. Doctor of Philosophy (PhD) degrees are awarded in a wide range of academic disciplines, and the PhD students are usually referred as research scholars. A comprehensive understanding of statistics allows research scholars to add rigour to their research. This approach helps them evaluate the current practices and draw informed conclusions from studies that were undertaken to generate their own hypotheses and to design, analyse and interpret complex clinical decisions. Therefore, intensive training at the beginning of the PhD journey is essential, as intensive training in research methodology and statistics in the early stages of research helps scholars design and plan their studies efficiently.
The University Grants Commission of India has taken various initiatives to introduce academic reforms to higher education institutions in India and mandated in 2009 that coursework be treated as a prerequisite for PhD preparation and that a minimum of four credits be assigned to one or more courses on research methodology, which could cover areas such as quantitative methods, computer applications, and research ethics. UGC also clearly states that all candidates admitted to PhD programmes shall be required to complete the prescribed coursework during the initial two semesters [ 1 ]. National Institute of Mental Health and Neurosciences (NIMHANS) at Bangalore, a tertiary care hospital and medical higher education institute in South India, that trains students in higher education in clinical fields, also introduced coursework in the PhD program for research scholars from various backgrounds, such as basic, behavioral and neurosciences, as per the UGC mandate. Research scholars undertake coursework programs soon after admission, which consist of several modules that include research methodology and statistical software training, among others.
Most scholars approach a course in statistics with the prejudice that statistics is uninteresting, demanding, complex or involve much mathematics and, most importantly, it is not relevant to their career goals. They approach statistics with considerable apprehension and negative attitudes, probably because of their inability to grasp the relevance of the application of the methods in their fields of study. This could be resolved by providing sufficient and relevant examples of the application of statistical techniques from various fields of medical research and by providing hands-on experience to learn how these techniques are applied and interpreted on real data. Hence, research methodology and statistical methods and the application of statistical methods using software have been given much importance and are taught as two modules, named Research Methodology and Statistics and Statistical Software Training, at this institute of medical higher education that trains research scholars in fields as diverse as basic, behavioural and neurosciences. Approximately 50% of the coursework curriculum focused on these two modules. Research scholars were thus given an opportunity to understand the theoretical aspects of the research methodology and statistical methods. They were also given hands-on training on statistical software to analyse the data using these methods and to interpret the findings. The coursework program was designed in this specific manner, as this intensive training would enable the research scholars to design their research studies more effectively and analyse their data in a better manner.
It is important to study attitudes toward statistics because attitudes are known to impact the learning process. Also, most importantly, these scholars are expected to utilize the skills in statistics and research methods to design research projects or guide postgraduate students and research scholars in the near future. Several authors have assessed attitudes toward statistics among various students and examined how attitudes affect academic achievement, how attitudes are correlated with knowledge in statistics and how attitudes change after a training program. There are studies on attitudes toward statistics among graduate [ 2 , 3 , 4 ] and postgraduate [ 5 ] medical students, politics, sociology, ( 6 – 7 ) psychology [ 8 , 9 , 10 ], social work [ 11 ], and management students [ 12 ]. However, there is a dearth of related literature on research scholars, and there are only two studies on the attitudes of research scholars. In their study of doctoral students in education-related fields, Cook & Catanzaro (2022) investigated the factors that contribute to statistics anxiety and attitudes toward statistics and how anxiety, attitudes and plans for future research use are connected among doctoral students [ 13 ]. Another study by Sohrabi et al. (2018) on research scholars assessed the change in knowledge and attitude towards teaching and educational design of basic science PhD students at a Medical University after a two-day workshop on empowerment and familiarity with the teaching and learning principles [ 14 ]. There were no studies that assessed changes in the attitudes or knowledge of research scholars across the PhD training period or after intensive training programmes such as PhD coursework. Even though PhD coursework has been established in institutes of higher education in India for more than a decade, there are no published research on the effectiveness of coursework from Indian universities or institutes of higher education.
This study aimed to determine the effectiveness of PhD coursework and whether intensive training programs such as PhD coursework can influence the knowledge and attitudes toward statistics of research scholars. Additionally, it would be interesting to know if the acquired knowledge could be retained longer, especially 2–3 years after the coursework, the crucial time of PhD data analysis. Hence, this study compares the scores of knowledge in statistics and attitude toward statistics of the research scholars at different time points of their PhD training, i.e., before, soon after and 2–3 years after the coursework.
Participants
This is an observational study of single group with repeated assessments. The institute offers a three-month coursework program consisting of seven modules, the first module is ethics; the fifth is research methodology and statistics; and the last is neurosciences. The study was conducted in January 2020. All research scholars of the institute who had completed PhD coursework in the last three years were considered for this study ( n = 130). Knowledge and attitudes toward statistics before and soon after the coursework module were assessed as part of the coursework program. They were collected on the first and last day of the program respectively. The author who was also the coordinator of the research methodology and statistics module of the coursework have obtained the necessary permission to use the data for this study. The scholars invited to be part of the study by e-mail. Knowledge and attitude towards statistics 2–3 years after the coursework were assessed online using Google forms. They were also administered a semi structured questionnaire to elicit details about the usefulness of coursework. Participation was voluntary, and consent was also sought online. The confidentiality of the data was assured. Data were not collected from research scholars of Biostatistics or from research scholars who had more than a decade of experience or who had been working in the institute as faculty, assuming that their scores could be higher and could bias the findings. This non funded study was reviewed and approved by the Institute Ethics Committee.
Instruments
Knowledge in Statistics was assessed by a questionnaire prepared by the author and was used as part of the coursework evaluation. The survey included 25 questions that assessed the knowledge of statistics on areas such as descriptive statistics, sampling methods, study design, parametric and nonparametric tests and multivariate analyses. Right answers were assigned a score of 1, and wrong answers were assigned a score of 0. Total scores ranged from 0 to 25. Statistics attitudes were assessed by the Survey of Attitudes toward Statistics (SATS) scale. The SATS is a 36-item scale that measures 6 domains of attitudes towards statistics. The possible range of scores for each item is between 1 and 7. The total score was calculated by dividing the summed score by the number of items. Higher scores indicate more positive attitudes. The SAT-36 is a copyrighted scale, and researchers are allowed to use it only with prior permission. ( 15 – 16 ) The author obtained permission for use in the coursework evaluation and this study. A semi structured questionnaire was also used to elicit details about the usefulness of coursework.
Statistical analysis
Descriptive statistics such as mean, standard deviation, number and percentages were used to describe the socio-demographic data. General Linear Model Repeated Measures of Analysis of variance was used to compare knowledge and attitude scores across assessments. Categorical data from the semi structured questionnaire are presented as percentages. All the statistical tests were two-tailed, and a p value < 0.05 was set a priori as the threshold for statistical significance. IBM SPSS (28.0) was used to analyse the data.
One hundred and thirty research scholars who had completed coursework (CW) in the last 2–3 years were considered for the study. These scholars were sent Google forms to assess their knowledge and attitudes 2–3 years after coursework. 81 scholars responded (62%), and 4 scholars did not consent to participate in the study. The data of 77 scholars were merged with the data obtained during the coursework program (before and soon after CW). Socio-demographic characteristics of the scholars are presented in Table 1 .
The age of the respondents ranged from 23 to 36 years, with an average of 28.7 years (3.01), and the majority of the respondents were females (65%). Years of experience (i.e., after masters) before joining a PhD programme ranged from 0.5 to 9 years, and half of them had less than three years of experience before joining the PhD programme (median-3). More than half of those who responded were research scholars from the behavioural sciences (55%), while approximately 30% were from the basic sciences (29%).
General Linear Model Repeated Measures of Analysis of variance was used to compare the knowledge and attitude scores of scholars before, soon after and 2–3 after the coursework (will now be referred as “later the CW”), and the results are presented below (Table 2 ; Fig. 1 ).
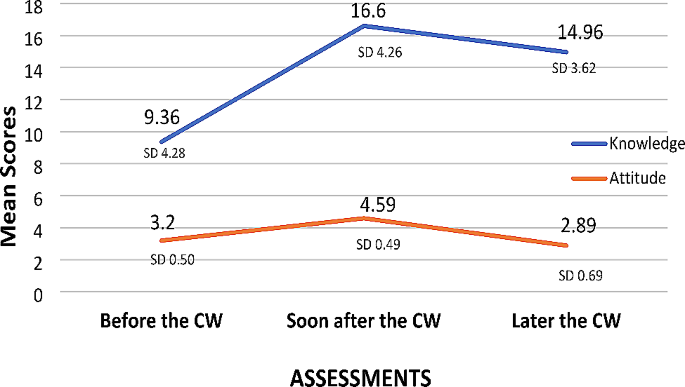
Comparison of knowledge and attitude scores across the assessments. Later the CW – 2–3 years after the coursework
The scores for knowledge and attitude differed significantly across time. Scores of knowledge and attitude increased soon after the coursework; the percentage of change was 77% and 43% respectively. However, significant reductions in knowledge and attitude scores were observed 2–3 years after the coursework compared to scores soon after the coursework. The reduction was higher for attitude scores; knowledge and attitude scores have decreased by 10% and 37% respectively. The change in scores across assessments is evident from the graph, and clearly the effect size is higher for attitude than knowledge.
The scores of knowledge or attitude before the coursework did not significantly differ with respect to gender or age or were not correlated with years of experience. Hence, they were not considered as covariates in the above analysis.
A semi structured questionnaire with open ended questions was also administered to elicit in-depth information about the usefulness of the coursework programme, in which they were also asked to self- rate their knowledge. The data were mostly categorical or narratives. Research scholars’ self-rated knowledge scores (on a scale of 0–10) also showed similar changes; knowledge improved significantly and was retained even after the training (Fig. 2 ).
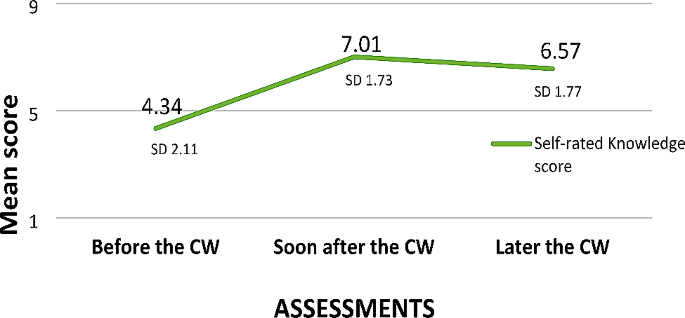
Self-rated knowledge scores of research scholars over time. Later the CW – 2–3 years after the coursework
The response to the question “ How has coursework changed your attitude toward statistics?”, is presented in Fig. 3 . The responses were Yes, positively, Yes - Negatively, No change – still apprehensive, No change – still appreciate, No change – still hate statistics. The majority of the scholars (70%) reported a positive change in their attitude toward statistics. Moreover, none of the scholars reported negative changes. Approximately 9% of the scholars reported that they were still apprehensive about statistics or hate statistics after the coursework.
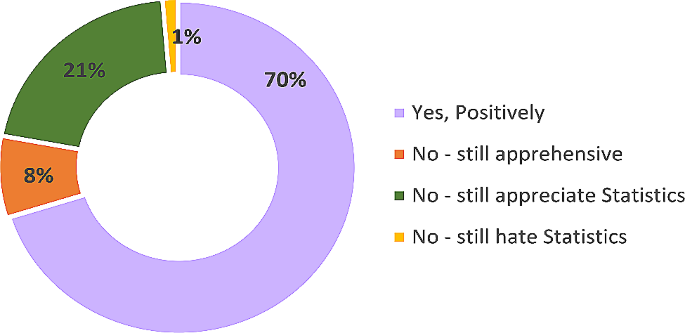
How has coursework changed your attitude toward statistics?
Those scholars who reported that they were apprehensive about statistics or hate statistics noted the complexity of the subject, lack of clarity, improper instructions and fear of mathematics as major reasons for their attitude. Some responses are listed below.
“The statistical concepts were not taught in an understandable manner from the UG level” , “I am weak in mathematical concepts. The equations and formulae in statistics scare me”. “Lack of knowledge about the importance of statistics and fear of mathematical equations”. “The preconceived notion that Statistics is difficult to learn” . “In most of the places, it is not taught properly and conceptual clarity is not focused on, and because of this an avoidance builds up, which might be a reason for the negative attitude”.
Majority of the scholars (92%) felt that coursework has helped them in their PhD, and they were happy to recommend it for other research scholars (97%). The responses of the scholars to the question “ How was coursework helpful in your PhD journey ?”, are listed below.
“Course work gave a fair idea on various things related to research as well as statistics” . “Creating the best design while planning methodology, which is learnt form course work, will increase efficiency in completing the thesis, thereby making it faster”. “Course work give better idea of how to proceed in many areas like literature search, referencing, choosing statistical methods, and learning about research procedures”. “Course work gave a good idea of research methodology, biostatistics and ethics. This would help in writing a better protocol and a better thesis”. “It helps us to plan our research well and to formulate, collect and plan for analysis”. “It makes people to plan their statistical analysis well in advance” .
This study evaluated the effectiveness of the existing coursework programme in an institution of higher medical education, and investigated whether the coursework programme benefits research scholars by improving their knowledge of statistics and attitudes towards statistics. The study concluded that the coursework program was beneficial for improving scholars’ knowledge about statistics and attitudes toward statistics.
Unlike other studies that have assessed attitudes toward statistics, the study participants in this study were research scholars. Research scholars need extensive training in statistics, as they need to apply statistical tests and use statistical reasoning in their research thesis, and in their profession to design research projects or their future student dissertations. Notably, no studies have assessed the attitudes or knowledge of research scholars in statistics either across the PhD training period or after intensive statistics training programs. However, the findings of this study are consistent with the findings of a study that compared the knowledge and attitudes toward teaching and education design of PhD students after a two-day educational course and instructional design workshop [ 14 ].
Statistics educators need not only impart knowledge but they should also motivate the learners to appreciate the role of statistics and to continue to learn the quantitative skills that is needed in their professional lives. Therefore, the role of learners’ attitudes toward statistics requires special attention. Since PhD coursework is possibly a major contributor to creating a statistically literate research community, scholars’ attitudes toward statistics need to be considered important and given special attention. Passionate and engaging statistics educators who have adequate experience in illustrating relatable examples could help scholars feel less anxious and build competence and better attitudes toward statistics. Statistics educators should be aware of scholars’ anxiety, fears and attitudes toward statistics and about its influence on learning outcomes and further interest in the subject.
Strengths and limitations
Analysis of changes in knowledge and attitudes scores across various time points of PhD training is the major strength of the study. Additionally, this study evaluates the effectiveness of intensive statistical courses for research scholars in terms of changes in knowledge and attitudes. This study has its own limitations: the data were collected through online platforms, and the nonresponse rate was about 38%. Ability in mathematics or prior learning experience in statistics, interest in the subject, statistics anxiety or performance in coursework were not assessed; hence, their influence could not be studied. The reliability and validity of the knowledge questionnaire have not been established at the time of this study. However, author who had prepared the questionnaire had ensured questions from different areas of statistics that were covered during the coursework, it has also been used as part of the coursework evaluation. Despite these limitations, this study highlights the changes in attitudes and knowledge following an intensive training program. Future research could investigate the roles of age, sex, mathematical ability, achievement or performance outcomes and statistics anxiety.
The study concluded that a rigorous and intensive training program such as PhD coursework was beneficial for improving knowledge about statistics and attitudes toward statistics. However, the significant reduction in attitude and knowledge scores after 2–3 years of coursework indicates that a refresher program might be helpful for research scholars as they approach the analysis stage of their thesis. Statistics educators must develop innovative methods to teach research scholars from nonstatistical backgrounds. They also must be empathetic to understanding scholars’ anxiety, fears and attitudes toward statistics and to understand its influence on learning outcomes and further interest in the subject.
Data availability
The data that support the findings of this study are available from the corresponding author upon request.
UGC Regulations on Minimum Standards and Procedure for the award of, M.Phil/Ph D, Degree R. 2009. Ugc.ac.in. [cited 2023 Oct 26]. https://www.ugc.ac.in/oldpdf/regulations/mphilphdclarification.pdf .
Althubaiti A. Attitudes of medical students toward statistics in medical research: Evidence from Saudi Arabia. J Stat Data Sci Educ [Internet]. 2021;29(1):115–21. https://doi.org/10.1080/10691898.2020.1850220 .
Hannigan A, Hegarty AC, McGrath D. Attitudes towards statistics of graduate entry medical students: the role of prior learning experiences. BMC Med Educ [Internet]. 2014;14(1):70. https://doi.org/10.1186/1472-6920-14-70 .
Hasabo EA, Ahmed GEM, Alkhalifa RM, Mahmoud MD, Emad S, Albashir RB et al. Statistics for undergraduate medical students in Sudan: associated factors for using statistical analysis software and attitude toward statistics among undergraduate medical students in Sudan. BMC Med Educ [Internet]. 2022;22(1):889. https://doi.org/10.1186/s12909-022-03960-0 .
Zhang Y, Shang L, Wang R, Zhao Q, Li C, Xu Y et al. Attitudes toward statistics in medical postgraduates: measuring, evaluating and monitoring. BMC Med Educ [Internet]. 2012;12(1):117. https://doi.org/10.1186/1472-6920-12-117 .
Bechrakis T, Gialamas V, Barkatsas A. Survey of attitudes towards statistics (SATS): an investigation of its construct validity and its factor structure invariance by gender. Int J Theoretical Educational Pract. 2011;1(1):1–15.
Google Scholar
Khavenson T, Orel E, Tryakshina M. Adaptation of survey of attitudes towards statistics (SATS 36) for Russian sample. Procedia Soc Behav Sci [Internet]. 2012; 46:2126–9. https://doi.org/10.1016/j.sbspro.2012.05.440 .
Coetzee S, Van Der Merwe P. Industrial psychology students’ attitudes towards statistics. J Industrial Psychol. 2010;36(1):843–51.
Francesca C, Primi C. Assessing statistics attitudes among College Students: Psychometric properties of the Italian version of the Survey of attitudes toward statistics (SATS). Learn Individual Differences. 2009;2:309–13.
Counsell A, Cribbie RA. Students’ attitudes toward learning statistics with R. Psychol Teach Rev [Internet]. 2020;26(2):36–56. https://doi.org/10.53841/bpsptr.2020.26.2.36 .
Yoon E, Lee J. Attitudes toward learning statistics among social work students: Predictors for future professional use of statistics. J Teach Soc Work [Internet]. 2022;42(1):65–81. https://doi.org/10.1080/08841233.2021.2014018 .
Melad AF. Students’ attitude and academic achievement in statistics: a Correlational Study. J Posit School Psychol. 2022;6(2):4640–6.
Cook KD, Catanzaro BA. Constantly Working on My Attitude Towards Statistics! Education Doctoral Students’ Experiences with and Motivations for Learning Statistics. Innov High Educ. 2023; 48:257–84. https://doi.org/10.1007/s10755-022-09621-w .
Sohrabi Z, Koohestani HR, Nahardani SZ, Keshavarzi MH. Data on the knowledge, attitude, and performance of Ph.D. students attending an educational course (Tehran, Iran). Data Brief [Internet]. 2018; 21:1325–8. https://doi.org/10.1016/j.dib.2018.08.081 .
Chau C, Stevens J, Dauphine T. Del V. A: The development and validation of the survey of attitudes toward statistics. Educ Psychol Meas. 1995;(5):868–75.
Student attitude surveys. and online educational consulting [Internet]. Evaluationandstatistics.com. [cited 2023 Oct 26]. https://www.evaluationandstatistics.com/ .
Download references
Acknowledgements
The author would like to thank the participants of the study and peers and experts who examined the content of the questionnaire for their time and effort.
This research did not receive any grants from funding agencies in the public, commercial, or not-for-profit sectors.
Author information
Authors and affiliations.
Department of Biostatistics, Dr. M.V. Govindaswamy Centre, National Institute of Mental Health and Neurosciences (NIMHANS), Bangalore, 560 029, India
Mariyamma Philip
You can also search for this author in PubMed Google Scholar
Contributions
Mariyamma Philip: Conceptualization, Methodology, Validation, Investigation, Writing- Original draft, Reviewing and Editing.
Corresponding author
Correspondence to Mariyamma Philip .
Ethics declarations
Ethics approval and consent to participate.
This study used data already collected data (before and soon after coursework). The data pertaining to knowledge and attitude towards statistics 2–3 years after coursework were collected from research scholars through the online survey platform Google forms. The participants were invited to participate in the survey through e-mail. The study was explained in detail, and participation in the study was completely voluntary. Informed consent was obtained online in the form of a statement of consent. The confidentiality of the data was assured, even though identifiable personal information was not collected. This non-funded study was reviewed and approved by NIMHANS Institute Ethics Committee (No. NIMHANS/21st IEC (BS&NS Div.)
Consent for publication
Not applicable because there is no personal information or images that could lead to the identification of a study participant.
Competing interests
The authors declare no competing interests.
Additional information
Publisher’s note.
Springer Nature remains neutral with regard to jurisdictional claims in published maps and institutional affiliations.
Rights and permissions
Open Access This article is licensed under a Creative Commons Attribution 4.0 International License, which permits use, sharing, adaptation, distribution and reproduction in any medium or format, as long as you give appropriate credit to the original author(s) and the source, provide a link to the Creative Commons licence, and indicate if changes were made. The images or other third party material in this article are included in the article’s Creative Commons licence, unless indicated otherwise in a credit line to the material. If material is not included in the article’s Creative Commons licence and your intended use is not permitted by statutory regulation or exceeds the permitted use, you will need to obtain permission directly from the copyright holder. To view a copy of this licence, visit http://creativecommons.org/licenses/by/4.0/ . The Creative Commons Public Domain Dedication waiver ( http://creativecommons.org/publicdomain/zero/1.0/ ) applies to the data made available in this article, unless otherwise stated in a credit line to the data.
Reprints and permissions
About this article
Cite this article.
Philip, M. Measurement and analysis of change in research scholars’ knowledge and attitudes toward statistics after PhD coursework. BMC Med Educ 24 , 512 (2024). https://doi.org/10.1186/s12909-024-05487-y
Download citation
Received : 27 October 2023
Accepted : 29 April 2024
Published : 08 May 2024
DOI : https://doi.org/10.1186/s12909-024-05487-y
Share this article
Anyone you share the following link with will be able to read this content:
Sorry, a shareable link is not currently available for this article.
Provided by the Springer Nature SharedIt content-sharing initiative
- Knowledge of statistics
- Attitude towards statistics
- PhD coursework
- Research scholars
BMC Medical Education
ISSN: 1472-6920
- Submission enquiries: [email protected]
- General enquiries: [email protected]
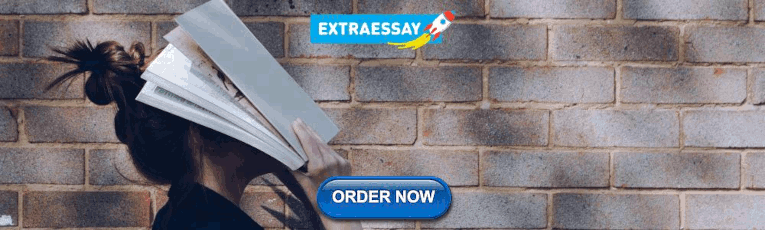
IMAGES
VIDEO
COMMENTS
Text mining has developed over decades and across scientific disciplines to form a now-substantial and diverse body of literature on the computer-aided analysis of textual data. This includes, among others, fields such as statistics, computer science, (computational) linguistics, library science, and computer science (Miner et al., 2012 ...
Words in fact may play an important role in Economics. In order to extract relevant information that words provide, this thesis relies on state-of-the-art methods from the information retrieval and computer science communities. Chapter 1 shows how policy uncertainty indices can be constructed via unsupervised machine learning models.
This study employed a text mining method to investigate the lexical features and their dynamic changes of PhD theses across the natural sciences, social sciences and humanities. Four quantitative ...
To do so, we employ a corpus of PhD thesis ab-stracts collected from the Digital Library of the University of Bologna,2 and we compare the per-formance of LDA to the results obtained by using other text mining methods. 2 Corpus Recently, Italian universities started to offer online institutional repositories of the doctoral theses de-
Text mining is involved in a broad scope of applications in diverse domains that main-ly, but not exclusively, serve political, commercial, medical and academic needs. ... Ye Wang, declare that the PhD thesis entitled Robust Text Mining in Online Social Net-work Content is no more than 100,000 words in length including quotes and exclusive of ...
This study employed a text mining method to investigate the lexical features and their dynamic changes of PhD theses across the natural sciences, social sciences and humanities. Four quantitative indices, i.e. TTR, h-point, R1 and writer's view, were employed to analyze 150 PhD theses (50 theses from each discipline).
in the text of published papers can be mined ... PhD thesis, Univ. Cambridge (1987). ... COMPUTATIONAL MATERIALS SCIENCE Text mining facilitates materials discovery
Text mining and visualization using VOSviewer Nees Jan van Eck and Ludo Waltman Centre for Science and Technology Studies, Leiden University, The Netherlands ... PhD thesis on bibliometric mapping of science (Van Eck, 2011). Each paragraph of the full text was treated as a separate document. The 218 most relevant noun phrases were included
The research reported in this thesis proposal is connected to a two year project "PROKEX". This PROKEX project (EUREKA, 2013), aims to develop a new knowledge management ... Enhancement in existing knowledge by using text mining techniques DOI: 10.14267/phd.2015065. 3 3. Develop a new semantic similarity measure to filter irrelevant ...
PhD Thesis Review: Knowledge Acquisition through Text Mining . by Dragoş Marcel VESPAN. The evolution of internet as a mean for sending information led to the growth of online - knowledge resources to the diversification of forms and formats used for their storage and transmission: text, data, video and audio.
The paper proceeds with a short literature summary on the effects of university-industry collaboration and "industrial" dissertation projects and text-mining techniques in Sect. 2.2. Section 2.3 introduces the sample and the semisupervised machine-learning approach used to classify projects as collaborative or noncollaborative.
A text mining method was employed to investigate the lexical features and their dynamic changes of PhD theses across the natural sciences, social sciences and humanities, and revealed sharp contrasts between theses in humanities and natural sciences. ABSTRACT This study employed a text mining method to investigate the lexical features and their dynamic changes of PhD theses across the natural ...
Text mining analysis on Pubmed data base. Download (50.62 MB) thesis. posted on 2023-02-22, 04:20 authored by Seyedeh Z. Rezaei Lalami. Due to the increasing amount of unstructured text data, information retrieval from large volumes of data has become highly important. Applying most of the algorithms, such as classification and clustering, is ...
PHD RESEARCH TOPIC IN TEXT MINING is on the bloom due to the excessive use of computer storage in the form of documents. Internet usage is growing exponentially which have large amount of information which leads to overhead problem. Generally, information is stored in various formats like structured, unstructured and semi-structured.
Abstract: In this study, we examined 6,834 master's and PhD theses conducted on computer science. and engineering between 199 4 and 2013 in Turkey. Thesis data were compiled from the YÖK ...
text mining, with news and social media. The combination of news features and market data may improve prediction accuracy. Despite of this, existing systems do not appear to have efficiently or effectively integrated news features and market data. In this dissertation, various of data and text mining techniques are used to iden-
This study employed a text mining method to investigate the lexical features and their dynamic changes of PhD theses across the natural sciences, social sciences and humanities. Four quantitative indices, i.e. TTR, h-point, R1 and writer's view,
Home. PhD Projects in Text Mining. "Text mining is a scholarly process of regaining fine info from large datasets.". PhD projects in text mining are the premier research service for PhD/MS scholars. At present, it applies to data retrieval, named entity recognition, pattern recognition, and more.
This study employed a text mining method to investigate the lexical features and their dynamic changes of PhD theses across the natural sciences, social sciences and humanities. Four quantitative indices, i.e. TTR, h-point, R1 and writer's view, were employed to analyze 150 PhD theses (50 theses from each discipline). Although h-point and writer's view were found counter-intuitively to ...
Social sentiment analysis. Opinion and also in frequent item mining. Mining from complex lexical structures. Tools for profiling digital games. Ontology and also in corpus based mining. Secure and also in privacy preservation. Also in Information retrieval from cloud/fog. We know that as a novice, shaping your research scope will be a big deal ...
I am a PhD candidate at Brasilia University (Brazil). I am currently looking for a Text Mining tool for analysing PhD Thesis (in portuguese language).
THE METHODOLOGY FOR INTEGRATING ROBOTIC SYSTEMS IN UNDEGROUND MINING MACHINES, Peter Kolapo. PDF. DISCRETE ELEMENT MODELING TO PREDICT MUCKPILE PROFILES FROM CAST BLASTING, Russell Lamont. Theses/Dissertations from 2023 PDF. ASSESSMENT OF AIR OVERPRESSURE FROM BLASTING USING COMPUTATIONAL FLUID DYNAMICS, Cecilia Estefania Aramayo. PDF
Knowledge of statistics is highly important for research scholars, as they are expected to submit a thesis based on original research as part of a PhD program. As statistics play a major role in the analysis and interpretation of scientific data, intensive training at the beginning of a PhD programme is essential. PhD coursework is mandatory in universities and higher education institutes in ...