
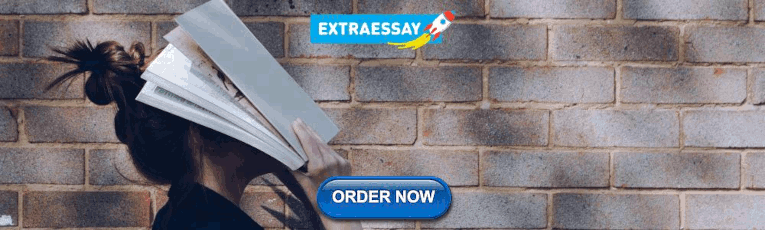
How To Write The Results/Findings Chapter
For quantitative studies (dissertations & theses).
By: Derek Jansen (MBA) | Expert Reviewed By: Kerryn Warren (PhD) | July 2021
So, you’ve completed your quantitative data analysis and it’s time to report on your findings. But where do you start? In this post, we’ll walk you through the results chapter (also called the findings or analysis chapter), step by step, so that you can craft this section of your dissertation or thesis with confidence. If you’re looking for information regarding the results chapter for qualitative studies, you can find that here .
Overview: Quantitative Results Chapter
- What exactly the results chapter is
- What you need to include in your chapter
- How to structure the chapter
- Tips and tricks for writing a top-notch chapter
- Free results chapter template
What exactly is the results chapter?
The results chapter (also referred to as the findings or analysis chapter) is one of the most important chapters of your dissertation or thesis because it shows the reader what you’ve found in terms of the quantitative data you’ve collected. It presents the data using a clear text narrative, supported by tables, graphs and charts. In doing so, it also highlights any potential issues (such as outliers or unusual findings) you’ve come across.
But how’s that different from the discussion chapter?
Well, in the results chapter, you only present your statistical findings. Only the numbers, so to speak – no more, no less. Contrasted to this, in the discussion chapter , you interpret your findings and link them to prior research (i.e. your literature review), as well as your research objectives and research questions . In other words, the results chapter presents and describes the data, while the discussion chapter interprets the data.
Let’s look at an example.
In your results chapter, you may have a plot that shows how respondents to a survey responded: the numbers of respondents per category, for instance. You may also state whether this supports a hypothesis by using a p-value from a statistical test. But it is only in the discussion chapter where you will say why this is relevant or how it compares with the literature or the broader picture. So, in your results chapter, make sure that you don’t present anything other than the hard facts – this is not the place for subjectivity.
It’s worth mentioning that some universities prefer you to combine the results and discussion chapters. Even so, it is good practice to separate the results and discussion elements within the chapter, as this ensures your findings are fully described. Typically, though, the results and discussion chapters are split up in quantitative studies. If you’re unsure, chat with your research supervisor or chair to find out what their preference is.
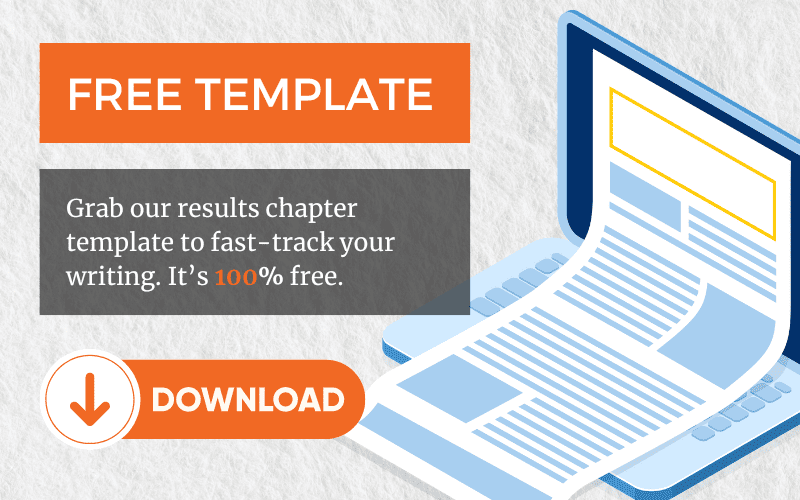
What should you include in the results chapter?
Following your analysis, it’s likely you’ll have far more data than are necessary to include in your chapter. In all likelihood, you’ll have a mountain of SPSS or R output data, and it’s your job to decide what’s most relevant. You’ll need to cut through the noise and focus on the data that matters.
This doesn’t mean that those analyses were a waste of time – on the contrary, those analyses ensure that you have a good understanding of your dataset and how to interpret it. However, that doesn’t mean your reader or examiner needs to see the 165 histograms you created! Relevance is key.
How do I decide what’s relevant?
At this point, it can be difficult to strike a balance between what is and isn’t important. But the most important thing is to ensure your results reflect and align with the purpose of your study . So, you need to revisit your research aims, objectives and research questions and use these as a litmus test for relevance. Make sure that you refer back to these constantly when writing up your chapter so that you stay on track.
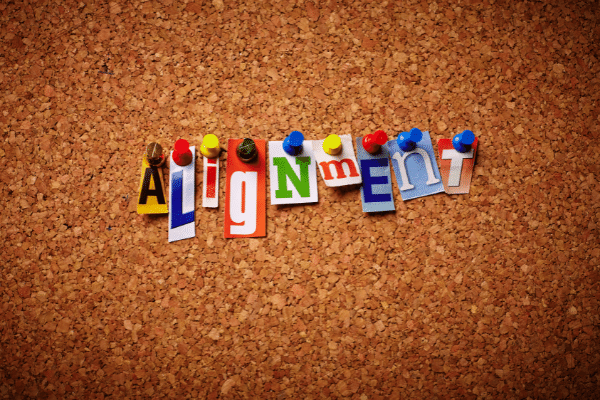
As a general guide, your results chapter will typically include the following:
- Some demographic data about your sample
- Reliability tests (if you used measurement scales)
- Descriptive statistics
- Inferential statistics (if your research objectives and questions require these)
- Hypothesis tests (again, if your research objectives and questions require these)
We’ll discuss each of these points in more detail in the next section.
Importantly, your results chapter needs to lay the foundation for your discussion chapter . This means that, in your results chapter, you need to include all the data that you will use as the basis for your interpretation in the discussion chapter.
For example, if you plan to highlight the strong relationship between Variable X and Variable Y in your discussion chapter, you need to present the respective analysis in your results chapter – perhaps a correlation or regression analysis.
Need a helping hand?
How do I write the results chapter?
There are multiple steps involved in writing up the results chapter for your quantitative research. The exact number of steps applicable to you will vary from study to study and will depend on the nature of the research aims, objectives and research questions . However, we’ll outline the generic steps below.
Step 1 – Revisit your research questions
The first step in writing your results chapter is to revisit your research objectives and research questions . These will be (or at least, should be!) the driving force behind your results and discussion chapters, so you need to review them and then ask yourself which statistical analyses and tests (from your mountain of data) would specifically help you address these . For each research objective and research question, list the specific piece (or pieces) of analysis that address it.
At this stage, it’s also useful to think about the key points that you want to raise in your discussion chapter and note these down so that you have a clear reminder of which data points and analyses you want to highlight in the results chapter. Again, list your points and then list the specific piece of analysis that addresses each point.
Next, you should draw up a rough outline of how you plan to structure your chapter . Which analyses and statistical tests will you present and in what order? We’ll discuss the “standard structure” in more detail later, but it’s worth mentioning now that it’s always useful to draw up a rough outline before you start writing (this advice applies to any chapter).
Step 2 – Craft an overview introduction
As with all chapters in your dissertation or thesis, you should start your quantitative results chapter by providing a brief overview of what you’ll do in the chapter and why . For example, you’d explain that you will start by presenting demographic data to understand the representativeness of the sample, before moving onto X, Y and Z.
This section shouldn’t be lengthy – a paragraph or two maximum. Also, it’s a good idea to weave the research questions into this section so that there’s a golden thread that runs through the document.
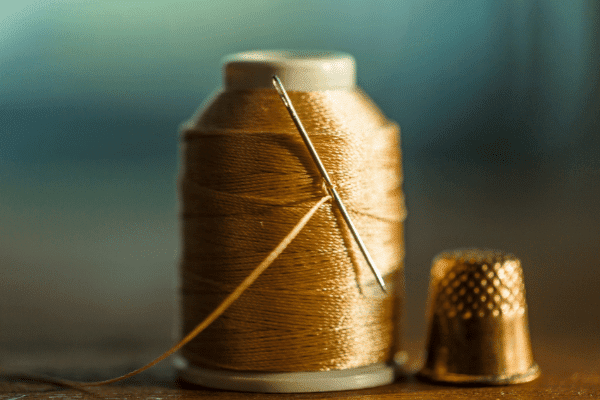
Step 3 – Present the sample demographic data
The first set of data that you’ll present is an overview of the sample demographics – in other words, the demographics of your respondents.
For example:
- What age range are they?
- How is gender distributed?
- How is ethnicity distributed?
- What areas do the participants live in?
The purpose of this is to assess how representative the sample is of the broader population. This is important for the sake of the generalisability of the results. If your sample is not representative of the population, you will not be able to generalise your findings. This is not necessarily the end of the world, but it is a limitation you’ll need to acknowledge.
Of course, to make this representativeness assessment, you’ll need to have a clear view of the demographics of the population. So, make sure that you design your survey to capture the correct demographic information that you will compare your sample to.
But what if I’m not interested in generalisability?
Well, even if your purpose is not necessarily to extrapolate your findings to the broader population, understanding your sample will allow you to interpret your findings appropriately, considering who responded. In other words, it will help you contextualise your findings . For example, if 80% of your sample was aged over 65, this may be a significant contextual factor to consider when interpreting the data. Therefore, it’s important to understand and present the demographic data.
Step 4 – Review composite measures and the data “shape”.
Before you undertake any statistical analysis, you’ll need to do some checks to ensure that your data are suitable for the analysis methods and techniques you plan to use. If you try to analyse data that doesn’t meet the assumptions of a specific statistical technique, your results will be largely meaningless. Therefore, you may need to show that the methods and techniques you’ll use are “allowed”.
Most commonly, there are two areas you need to pay attention to:
#1: Composite measures
The first is when you have multiple scale-based measures that combine to capture one construct – this is called a composite measure . For example, you may have four Likert scale-based measures that (should) all measure the same thing, but in different ways. In other words, in a survey, these four scales should all receive similar ratings. This is called “ internal consistency ”.
Internal consistency is not guaranteed though (especially if you developed the measures yourself), so you need to assess the reliability of each composite measure using a test. Typically, Cronbach’s Alpha is a common test used to assess internal consistency – i.e., to show that the items you’re combining are more or less saying the same thing. A high alpha score means that your measure is internally consistent. A low alpha score means you may need to consider scrapping one or more of the measures.
#2: Data shape
The second matter that you should address early on in your results chapter is data shape. In other words, you need to assess whether the data in your set are symmetrical (i.e. normally distributed) or not, as this will directly impact what type of analyses you can use. For many common inferential tests such as T-tests or ANOVAs (we’ll discuss these a bit later), your data needs to be normally distributed. If it’s not, you’ll need to adjust your strategy and use alternative tests.
To assess the shape of the data, you’ll usually assess a variety of descriptive statistics (such as the mean, median and skewness), which is what we’ll look at next.
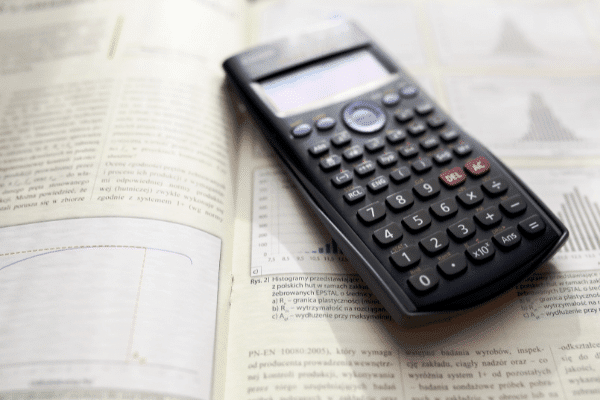
Step 5 – Present the descriptive statistics
Now that you’ve laid the foundation by discussing the representativeness of your sample, as well as the reliability of your measures and the shape of your data, you can get started with the actual statistical analysis. The first step is to present the descriptive statistics for your variables.
For scaled data, this usually includes statistics such as:
- The mean – this is simply the mathematical average of a range of numbers.
- The median – this is the midpoint in a range of numbers when the numbers are arranged in order.
- The mode – this is the most commonly repeated number in the data set.
- Standard deviation – this metric indicates how dispersed a range of numbers is. In other words, how close all the numbers are to the mean (the average).
- Skewness – this indicates how symmetrical a range of numbers is. In other words, do they tend to cluster into a smooth bell curve shape in the middle of the graph (this is called a normal or parametric distribution), or do they lean to the left or right (this is called a non-normal or non-parametric distribution).
- Kurtosis – this metric indicates whether the data are heavily or lightly-tailed, relative to the normal distribution. In other words, how peaked or flat the distribution is.
A large table that indicates all the above for multiple variables can be a very effective way to present your data economically. You can also use colour coding to help make the data more easily digestible.
For categorical data, where you show the percentage of people who chose or fit into a category, for instance, you can either just plain describe the percentages or numbers of people who responded to something or use graphs and charts (such as bar graphs and pie charts) to present your data in this section of the chapter.
When using figures, make sure that you label them simply and clearly , so that your reader can easily understand them. There’s nothing more frustrating than a graph that’s missing axis labels! Keep in mind that although you’ll be presenting charts and graphs, your text content needs to present a clear narrative that can stand on its own. In other words, don’t rely purely on your figures and tables to convey your key points: highlight the crucial trends and values in the text. Figures and tables should complement the writing, not carry it .
Depending on your research aims, objectives and research questions, you may stop your analysis at this point (i.e. descriptive statistics). However, if your study requires inferential statistics, then it’s time to deep dive into those .
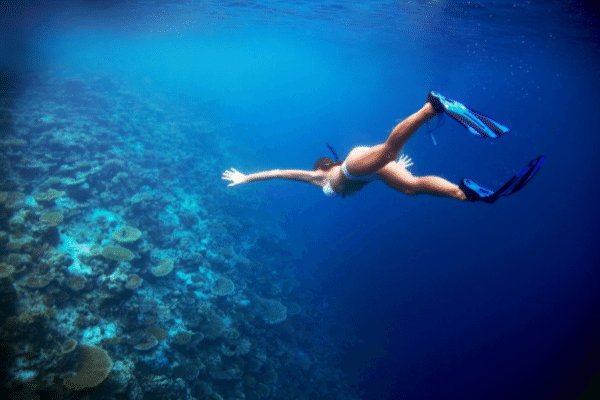
Step 6 – Present the inferential statistics
Inferential statistics are used to make generalisations about a population , whereas descriptive statistics focus purely on the sample . Inferential statistical techniques, broadly speaking, can be broken down into two groups .
First, there are those that compare measurements between groups , such as t-tests (which measure differences between two groups) and ANOVAs (which measure differences between multiple groups). Second, there are techniques that assess the relationships between variables , such as correlation analysis and regression analysis. Within each of these, some tests can be used for normally distributed (parametric) data and some tests are designed specifically for use on non-parametric data.
There are a seemingly endless number of tests that you can use to crunch your data, so it’s easy to run down a rabbit hole and end up with piles of test data. Ultimately, the most important thing is to make sure that you adopt the tests and techniques that allow you to achieve your research objectives and answer your research questions .
In this section of the results chapter, you should try to make use of figures and visual components as effectively as possible. For example, if you present a correlation table, use colour coding to highlight the significance of the correlation values, or scatterplots to visually demonstrate what the trend is. The easier you make it for your reader to digest your findings, the more effectively you’ll be able to make your arguments in the next chapter.
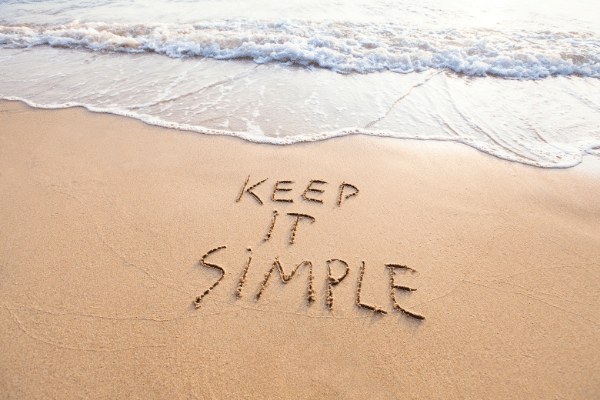
Step 7 – Test your hypotheses
If your study requires it, the next stage is hypothesis testing. A hypothesis is a statement , often indicating a difference between groups or relationship between variables, that can be supported or rejected by a statistical test. However, not all studies will involve hypotheses (again, it depends on the research objectives), so don’t feel like you “must” present and test hypotheses just because you’re undertaking quantitative research.
The basic process for hypothesis testing is as follows:
- Specify your null hypothesis (for example, “The chemical psilocybin has no effect on time perception).
- Specify your alternative hypothesis (e.g., “The chemical psilocybin has an effect on time perception)
- Set your significance level (this is usually 0.05)
- Calculate your statistics and find your p-value (e.g., p=0.01)
- Draw your conclusions (e.g., “The chemical psilocybin does have an effect on time perception”)
Finally, if the aim of your study is to develop and test a conceptual framework , this is the time to present it, following the testing of your hypotheses. While you don’t need to develop or discuss these findings further in the results chapter, indicating whether the tests (and their p-values) support or reject the hypotheses is crucial.
Step 8 – Provide a chapter summary
To wrap up your results chapter and transition to the discussion chapter, you should provide a brief summary of the key findings . “Brief” is the keyword here – much like the chapter introduction, this shouldn’t be lengthy – a paragraph or two maximum. Highlight the findings most relevant to your research objectives and research questions, and wrap it up.
Some final thoughts, tips and tricks
Now that you’ve got the essentials down, here are a few tips and tricks to make your quantitative results chapter shine:
- When writing your results chapter, report your findings in the past tense . You’re talking about what you’ve found in your data, not what you are currently looking for or trying to find.
- Structure your results chapter systematically and sequentially . If you had two experiments where findings from the one generated inputs into the other, report on them in order.
- Make your own tables and graphs rather than copying and pasting them from statistical analysis programmes like SPSS. Check out the DataIsBeautiful reddit for some inspiration.
- Once you’re done writing, review your work to make sure that you have provided enough information to answer your research questions , but also that you didn’t include superfluous information.
If you’ve got any questions about writing up the quantitative results chapter, please leave a comment below. If you’d like 1-on-1 assistance with your quantitative analysis and discussion, check out our hands-on coaching service , or book a free consultation with a friendly coach.
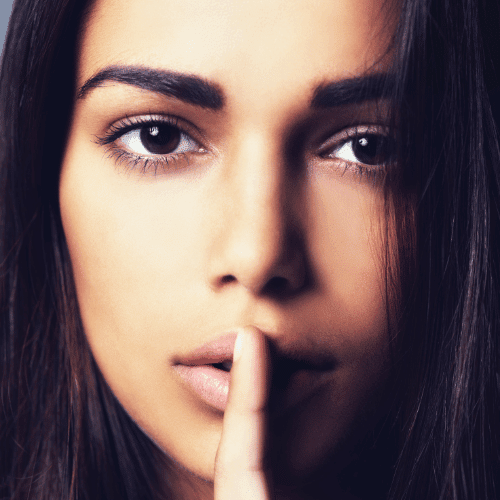
Psst... there’s more!
This post was based on one of our popular Research Bootcamps . If you're working on a research project, you'll definitely want to check this out ...
You Might Also Like:
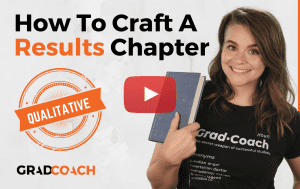
Thank you. I will try my best to write my results.
Awesome content 👏🏾
this was great explaination
Submit a Comment Cancel reply
Your email address will not be published. Required fields are marked *
Save my name, email, and website in this browser for the next time I comment.
- Print Friendly
- How it works
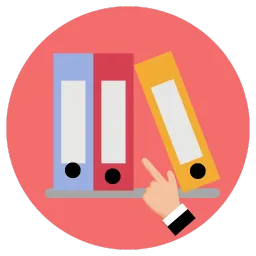
Chapter 4 – Data Analysis and Discussion (example)
Disclaimer: This is not a sample of our professional work. The paper has been produced by a student. You can view samples of our work here . Opinions, suggestions, recommendations and results in this piece are those of the author and should not be taken as our company views.
Type of Academic Paper – Dissertation Chapter
Academic Subject – Marketing
Word Count – 2964 words
Reliability Analysis
Before conducting any analysis on the data, all the data’s reliability was analyzed based on Cronbach’s Alpha value. The reliability analysis was performed on the complete data of the questionnaire. The reliability of the data was found to be (0.922), as shown in the results of the reliability analysis provided below in table 4.1. However, the complete results output of the reliability analysis is given in the appendix.
Reliability Analysis (N=200)
The Cronbach’s Alpha value between (0.7-1.0) is considered to have excellent reliability. The Cronbach’s Alpha value of the data was found to be (0.922); therefore, this indicated that the questionnaire data had excellent reliability. All of the 29 items of the questionnaire had excellent reliability, and if they are taken for further analysis, they can generate results with 92.2% reliability.
Frequency Distribution Analysis
First of all, the frequency distribution analysis was performed on the demographic variables using SPSS to identify the respondents’ demographic composition. Section 1 of the questionnaire had 5 demographic questions to identify; gender, age group, annual income, marital status, and education level of the research sample. The frequency distribution results shown in table 4.2 below indicated that there were 200 respondents in total, out of which 50% were male, and 50% were female. This shows that the research sample was free from gender-based biases as males and females had equal representation in the sample.
Moreover, the frequency distribution analysis suggested three age groups; ‘20-35’, ‘36-60’ and ‘Above 60’. 39% of the respondents belonged to the ‘20-35’ age group, while 56.5% of the respondents belonged to the ‘36-60’ age group and the remaining 4.5% belonged to the age group of ‘Above 60’.
Furthermore, the annual income level was divided into four categories. The income values were in GBP. It was found that 13% of the respondents had income ‘up to 30000’, 27% had income between ‘31000 to 50000’, 52.5% had income between ‘51000 to 100000’, and 7.5% had income ‘Above 100000’. This suggests that most of the respondents had an annual income between ‘31000 to 50000’ GBP.
The frequency distribution analysis indicated that 61% of respondents were single, while 39% were married, as indicated in table 4.2. This means that most of the respondents were single. Based on frequency distribution, it was also found that the education level of the respondents was analyzed using four categories of education level, namely; diploma, graduate, master, and doctorate. The results depicted that 37% of the respondents were diploma holders, 46% were graduates, 16% had master-level education, while only 2% had a doctorate. This suggests that most of the respondents were either graduate or diploma holders.
Frequency Distribution of the Demographic Characteristics of the respondents (N=200)
Multiple Regression Analysis
The hypotheses were tested using linear multiple regression analysis to determine which of the dependent variables had a significant positive effect on the customer loyalty of the five-star hotel brands. The results of the regression analysis are summarized in the following table 4.3. However, the complete SPSS output of the regression analysis is given in the appendix. Table 4.3
Multiple regression analysis showing the predictive values of dependent variables (Brand image, corporate identity, public relation, perceived quality, and trustworthiness) on customer loyalty (N=200)
Predictors: (Constant), Trustworthiness, Public Relation, Brand Image, Corporate Identity, Perceived Quality Dependent Variable: Customer Loyalty
The significance value (p-value) of ANOVA was found to be (0.000) as shown in the above
table, which was less than 0.05. This suggested that the model equation was significantly fitted
on the data. Moreover, the adjusted R-Square value was (0.897), which indicated that the model’s predictors explained 89.7% variation in customer loyalty.
Furthermore, the presence of the significant effect of the 5 predicting variables on customer loyalty was identified based on their sig. Values. The effect of a predicting variable is significant if its sig. Value is less than 0.05 or if its t-Statistics value is greater than 2. It was found that the variable ‘brand image’ had sig. Value (0.046), the variable ‘corporate identity had sig. Value (0.482), the variable ‘public relation’ had sig. Value (0.400), while the variable ‘perceived quality’ had sig. value (0.000), and the variable ‘trustworthiness’ had sig. value (0.652).
Hire an Expert Dissertation Chapter Writer
Orders completed by our expert writers are
- Formally drafted in an academic style
- Free Amendments and 100% Plagiarism Free – or your money back!
- 100% Confidential and Timely Delivery!
- Free anti-plagiarism report
- Appreciated by thousands of clients. Check client reviews
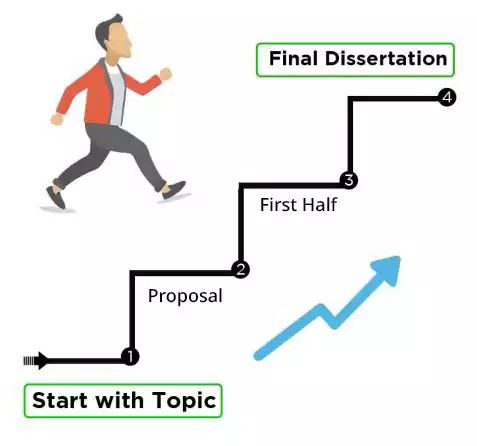
Hypotheses Assessment
Based on the regression analysis, it was found that brand image and perceived quality have a significant positive effect on customer loyalty. In contrast, corporate identity, public relations, and trustworthiness have an insignificant effect on customer loyalty. Therefore the two hypotheses; H1 and H4 were accepted, however the three hypotheses; H2, H3, and H5 were rejected as indicated in table 4.4.
Hypothesis Assessment Summary Table (N=200)
The insignificant variables (corporate identity, public relation and trustworthiness) were excluded from equation 1. After excluding the insignificant variables from the model equation 1, the final equation becomes as follows;
Customer loyalty = α + 0.074 (Brand image) + 0.991 (Perceived quality) + €
The above equation suggests that a 1 unit increase in brand image is likely to result in 0.074 units increase customer loyalty. In comparison, 1 unit increase in perceived quality can result in 0.991 units increase in customer loyalty.
Cross Tabulation Analysis
To further explore the results, the demographic variables’ data were cross-tabulated against the respondents’ responses regarding customer loyalty using SPSS. In this regards the five demographic variables; gender, age group, annual income, marital status and education level were cross-tabulated against the five questions regarding customer loyalty to know the difference between the customer loyalty of five-star hotels of UK based on demographic differences. The results of the cross-tabulation analysis are given in the appendix. The results are graphically presented in bar charts too, which are also given in the appendix.
Cross Tabulation of Gender against Customer Loyalty
The gender was cross-tabulated against question 1 to 5 of the questionnaire to identify the gender differences between male and female respondents’ responses regarding customer loyalty of five-star hotels of the UK. The results indicated that out of 100 males, 57% were extremely agreed that they stay at one hotel, while out of 100 females, 80% were extremely agreed they stay at one hotel. This shows that in comparison with a male, females were more agreed that they stayed at one hotel and were found to be more loyal towards their respective hotel brands.
The cross-tabulation results further indicated that out of 100 males, 53% agreed that they always say positive things about their respective hotel brand to other people. In contrast, out of 100 females, 77% were extremely agreed. Based on the results, the females were found to be in more agreement than males that they always say positive things about their respective hotel brand to other people.
It was further found that out of 100 males, 53% were extremely agreed that they recommend their hotel brand to others, however, out of 100 females, 74% were extremely agreed to this statement. This result also suggested that females were more in agreement than males to recommend their hotel brand to others.
Moreover, it was found that out of 100 males, 54% were extremely agreed that they don’t seek alternative hotel brands, while out of 100 females, 79% were extremely agreed to this statement. This result also suggested that females were more agreed than males that they don’t seek alternative hotel brands, and so were found to be more loyal than males.
Furthermore, it was identified that out of 100 male respondents 56% were extremely agreed that they would continue to go to the same hotel irrespective of the prices, however out of 100 females 79% were extremely agreed. Based on this result, it was clear that females were more agreed than males that they would continue to go to the same hotel irrespective of the prices, so females were found to be more loyal than males.
After cross tabulating ‘gender’ against the response of the 5 questions regarding customer loyalty the females were found to be more loyal customers of the five-star hotel brands than males as they were found to be more in agreement than the man that they stay at one hotel, always say positive things about their hotel brand to other people, recommend their hotel brand to others, don’t seek alternative hotel brands and would continue to go to the same hotel irrespective of the prices.
Cross Tabulation of Age Group against Customer Loyalty
Afterward, the second demographic variable, ‘age groups’ was cross-tabulated against questions 1 to 5 of the questionnaire to identify the difference between the customer loyalty of customers of different age groups. The results indicated that out of 78 respondents between 20 to 35 years of age, 61.5% were extremely agreed that they stayed at one hotel. While out of 113 respondents who were between 36 to 60 years of age, 72.6% were extremely agreed that they always stay at one hotel. However, out of 9 respondents who were above 60 years of age, 77.8% agreed that they always stay at one hotel. This indicated that customers of 36-60 and above 60 age groups were more loyal to their hotel brands as they were keener to stay at a respective hotel brand.
Content removed…
Cross Tabulation of Annual Income against Customer Loyalty
The third demographic variable, ‘annual income’ was cross-tabulated against questions 1 to 5 of the questionnaire to identify which of the customers were most loyal based on their respective annual income levels. The results indicated that out of 26 respondents who had annual income up to 30000 GBP, 84.6% were extremely agreed that they always stay at one hotel. However, out of 54 respondents who had annual income from 31000 to 50000 GBP, 98.1% agreed that they always stay at one hotel. Although out of 105 respondents had annual income from 50000 to 100000 GBP, 49.5% were extremely agreed that they always stay at one hotel. While out of 10 respondents who had annual income from 50000 to 1000000 GBP, 66.7% agreed that they always stay at one hotel. This indicated that customers of annual income levels from 31000 to 50000 GBP were more loyal to their hotel brands than the customers having other annual income levels.
Cross Tabulation of Marital Status against Customer Loyalty
Furthermore, the fourth demographic variable the ‘marital status’ was cross-tabulated against questions 1 to 5 of the questionnaire to understand the difference between married and unmarried respondents regarding customer loyalty of five-star hotels of the UK. The cross-tabulation analysis results indicated that out of 122 single respondents, 59.8% were extremely agreed that they stay at one hotel. However, out of 78 married respondents, around 82% of respondents agreed that they stay at one hotel. Thus, the married customers were more loyal to their hotel brands than unmarried customers because, in comparison, married customers prefer to stay at one hotel brand.
To proceed with the cross-tabulation results, out of 122 single respondents, 55.7% were extremely agreed upon always saying positive things about their hotel brands to other people. On the other hand, out of 78 married respondents, 79.5% were extremely agreed. Hence, upon evaluating the results, it can be said that married customers have more customer loyalty as they are in more agreement than singles. They always give positive feedback regarding their respective hotel brand to other people.
Cross Tabulation of Education Level against Customer Loyalty
Subsequently, the fifth demographic variable, ‘education level’ was cross-tabulated against questions 1 to 5 of the questionnaire to identify which of the customers were most loyal based on their respective education levels. The results indicated that out of 50 respondents who were diploma holders, 67.6% were extremely agreed that they always stay at one hotel. While out of 64 respondents who were graduates, 69.6% were extremely agreed that they always stay at one hotel. Although out of 22 respondents who were masters, 68.8% were extremely agreed that they always stay at one hotel. However, out of 2 respondents with doctorates, 50% were extremely agreed to always stay at one hotel. This indicated that customers who were graduates were more loyal than the customers with diplomas, masters, or doctorates.
Moreover, 66.2% of the diploma holders were extremely agreed that they always say positive things about their hotel brand to other people. In comparison, 64.1% of the respondents who were graduates were extremely agreed. However, 65.5% of the respondents who had masters were extremely agreed, and 50% of the respondents who had doctorates agreed with the statement. Based on this result customers having masters were the most loyal customers of their respective five-star hotel brands.
Need a Dissertation Chapter On a Similar Topic?
In this subsection, the findings of this study are compared and contrasted with the literature to identify which of the past research supports the present research findings. This present study based on regression analysis suggested that brand image can have a significant positive effect on the customer loyalty of five-star hotels in the UK. This finding was supported by the research of Heung et al. (1996), who also suggested that the hotel’s brand image can play a vital role in preserving a high ratio of customer loyalty.
Moreover, this present study also suggested that perceived quality was the second factor that was found to have a significant positive effect on customer loyalty. The perceived quality was evaluated based on; service quality, comfort, staff courtesy, customer satisfaction, and service quality expectations. In this regard, Tat and Raymond (2000) research supports the findings of this study. The staff service quality was found to affect customer loyalty and the level of satisfaction. Teas (1994) had also found service quality to affect customer loyalty. However, Teas also found that staff empathy (staff courtesy) towards customers can also affect customer loyalty. The research of Rowley and Dawes (1999) also supports the finding of this present study. The users’ expectations about the quality and nature of the services affect customer loyalty. A study by Oberoi and Hales (1990) was found to agree with the present study’s findings, as they had found the quality of staff service to affect customer loyalty.
Summary of the Findings
- The brand image was found to have a significant positive effect on customer loyalty. Therefore customer loyalty is likely to increase with the increase in brand image.
- The corporate identity was found to have an insignificant effect on customer loyalty. Therefore customer loyalty is not likely to increase with the increase in corporate identity.
- Public relations was found to have an insignificant effect on customer loyalty. Therefore customer loyalty is not likely to increase with the increase in public relations.
- Perceived quality was found to have a significant positive effect on customer loyalty. Therefore customer loyalty is likely to increase with the increase in perceived quality.
- Trustworthiness was found to have an insignificant effect on customer loyalty. Therefore customer loyalty is not likely to increase with the increase in trustworthiness.
- The female customers were found to be more loyal customers of the five-star hotel brands than male customers.
- The customers of age from 36 to 60 years were more loyal to their hotel brands than the customers of age from 20 to 35 and above 60.
- The customers who had annual income from 31000 to 50000 were more loyal customers of their respective hotel brands than those who had an annual income level of less than 31000 or more than 50000.
- The married respondents had more customer loyalty than unmarried customers, towards five-star hotel brands of the UK.
The customers who had bachelor degrees and the customers who had master degrees were more loyal to the customers who had a diploma or doctorate.
Bryman, A., Bell, E., 2015. Business Research Methods. Oxford University Press.
Daum, P., 2013. International Synergy Management: A Strategic Approach for Raising Efficiencies in the Cross-border Interaction Process. Anchor Academic Publishing (aap_verlag).
Dümke, R., 2002. Corporate Reputation and its Importance for Business Success: A European
Perspective and its Implication for Public Relations Consultancies. diplom.de.
Guetterman, T.C., 2015. Descriptions of Sampling Practices Within Five Approaches to Qualitative Research in Education and the Health Sciences. Forum Qualitative Sozialforschung /
Forum: Qualitative Social Research 16.
Haq, M., 2014. A Comparative Analysis of Qualitative and Quantitative Research Methods and a Justification for Adopting Mixed Methods in Social Research (PDF Download Available).
ResearchGate 1–22. doi:http://dx.doi.org/10.13140/RG.2.1.1945.8640
Kelley, ., Clark, B., Brown, V., Sitzia, J., 2003. Good practice in the conduct and reporting of survey research. Int J Qual Health Care 15, 261–266. doi:10.1093/intqhc/mzg031
Lewis, S., 2015. Qualitative Inquiry and Research Design: Choosing Among Five Approaches.
Health Promotion Practice 16, 473–475. doi:10.1177/1524839915580941
Saunders, M., 2003. Research Methods for Business Students. Pearson Education India.
Saunders, M.N.K., Tosey, P., 2015. Handbook of Research Methods on Human Resource
Development. Edward Elgar Publishing.
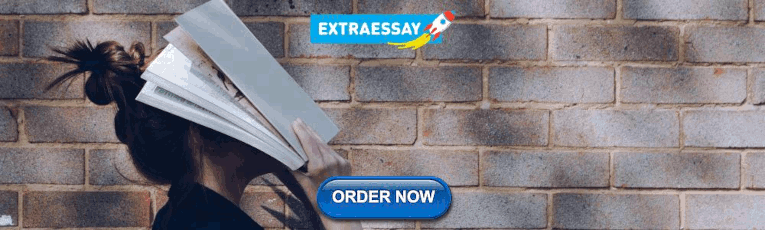
DMCA / Removal Request
If you are the original writer of this Dissertation Chapter and no longer wish to have it published on the www.ResearchProspect.com then please:
Request The Removal Of This Dissertation Chapter
Frequently Asked Questions
How to write the results chapter of a dissertation.
To write the Results chapter of a dissertation:
- Present findings objectively.
- Use tables, graphs, or charts for clarity.
- Refer to research questions/hypotheses.
- Provide sufficient details.
- Avoid interpretation; save that for the Discussion chapter.
USEFUL LINKS
LEARNING RESOURCES
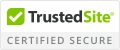
COMPANY DETAILS
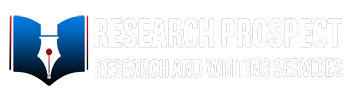
- How It Works
Best Practice: How to Write a Dissertation or Thesis Quantitative Chapter 4
Statistics Blog
In the first paragraph of your quantitative chapter 4, the results chapter, restate the research questions that will be examined. This reminds the reader of what you’re going to investigate after having been trough the details of your methodology. It’s helpful too that the reader knows what the variables are that are going to be analyzed.
Spend a paragraph telling the reader how you’re going to clean the data. Did you remove univariate or multivariate outlier? How are you going to treat missing data? What is your final sample size?
The next paragraph should describe the sample using demographics and research variables. Provide frequencies and percentages for nominal and ordinal level variables and means and standard deviations for the scale level variables. You can provide this information in figures and tables.
Here’s a sample:
Frequencies and Percentages. The most frequently observed category of Cardio was Yes ( n = 41, 72%). The most frequently observed category of Shock was No ( n = 34, 60%). Frequencies and percentages are presented.
Summary Statistics. The observations for MiniCog had an average of 25.49 ( SD = 14.01, SE M = 1.87, Min = 2.00, Max = 55.00). The observations for Digital had an average of 29.12 ( SD = 10.03, SE M = 1.33, Min = 15.50, Max = 48.50). Skewness and kurtosis were also calculated. When the skewness is greater than 2 in absolute value, the variable is considered to be asymmetrical about its mean. When the kurtosis is greater than or equal to 3, then the variable’s distribution is markedly different than a normal distribution in its tendency to produce outliers (Westfall & Henning, 2013).
Now that the data is clean and descriptives have been conducted, turn to conducting the statistics and assumptions of those statistics for research question 1. Provide the assumptions first, then the results of the statistics. Have a clear accept or reject of the hypothesis statement if you have one. Here’s an independent samples t-test example:
Introduction. An two-tailed independent samples t -test was conducted to examine whether the mean of MiniCog was significantly different between the No and Yes categories of Cardio.
Assumptions. The assumptions of normality and homogeneity of variance were assessed.
Normality. A Shapiro-Wilk test was conducted to determine whether MiniCog could have been produced by a normal distribution (Razali & Wah, 2011). The results of the Shapiro-Wilk test were significant, W = 0.94, p = .007. These results suggest that MiniCog is unlikely to have been produced by a normal distribution; thus normality cannot be assumed. However, the mean of any random variable will be approximately normally distributed as sample size increases according to the Central Limit Theorem (CLT). Therefore, with a sufficiently large sample size ( n > 50), deviations from normality will have little effect on the results (Stevens, 2009). An alternative way to test the assumption of normality was utilized by plotting the quantiles of the model residuals against the quantiles of a Chi-square distribution, also called a Q-Q scatterplot (DeCarlo, 1997). For the assumption of normality to be met, the quantiles of the residuals must not strongly deviate from the theoretical quantiles. Strong deviations could indicate that the parameter estimates are unreliable. Figure 1 presents a Q-Q scatterplot of MiniCog.
Homogeneity of variance. Levene’s test for equality of variance was used to assess whether the homogeneity of variance assumption was met (Levene, 1960). The homogeneity of variance assumption requires the variance of the dependent variable be approximately equal in each group. The result of Levene’s test was significant, F (1, 54) = 18.30, p < .001, indicating that the assumption of homogeneity of variance was violated. Consequently, the results may not be reliable or generalizable. Since equal variances cannot be assumed, Welch’s t-test was used instead of the Student’s t-test, which is more reliable when the two samples have unequal variances and unequal sample sizes (Ruxton, 2006).
Results. The result of the two-tailed independent samples t -test was significant, t (46.88) = -4.81, p < .001, indicating the null hypothesis can be rejected. This finding suggests the mean of MiniCog was significantly different between the No and Yes categories of Cardio. The mean of MiniCog in the No category of Cardio was significantly lower than the mean of MiniCog in the Yes category. Present the results of the two-tailed independent samples t -test, and present the means of MiniCog(No) and MiniCog(Yes).
In the next paragraphs, conduct stats and assumptions for your other research questions. Again, assumptions first, then the results of the statistics with appropriate tables and figures.
Be sure to add all of the in-text citations to your reference section. Here is a sample of references.
Conover, W. J., & Iman, R. L. (1981). Rank transformations as a bridge between parametric and nonparametric statistics. The American Statistician, 35 (3), 124-129.
DeCarlo, L. T. (1997). On the meaning and use of kurtosis. Psychological Methods, 2(3), 292-307.
Levene, H. (1960). Contributions to Probability and Statistics. Essays in honor of Harold Hotelling, I. Olkin et al. eds., Stanford University Press, 278-292.
Razali, N. M., & Wah, Y. B. (2011). Power comparisons of Shapiro-Wilk, Kolmogorov-Smirnov, Lilliefors and Anderson-Darling tests. Journal of Statistical Modeling and Analytics, 2 (1), 21-33.
Ruxton, G. D. (2006). The unequal variance t-test is an underused alternative to Student’s t-test and the Mann-Whitney U test. Behavioral Ecology, 17 (4), 688-690.
Intellectus Statistics [Online computer software]. (2019). Retrieved from https://analyze.intellectusstatistics.com/
Stevens, J. P. (2009). Applied multivariate statistics for the social sciences (5th ed.). Mahwah, NJ: Routledge Academic.
Westfall, P. H., & Henning, K. S. S. (2013). Texts in statistical science: Understanding advanced statistical methods. Boca Raton, FL: Taylor & Francis.
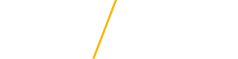
- Chapter Four: Quantitative Methods (Part 3 - Making Sense of Your Study)
After you have designed your study, collected your data, and analyzed it, you have to figure out what it means and communicate that to potential interested audiences. This section of the chapter is about how to make sense of your study, in terms of data interpretation, data write-up, and data presentation, as seen in the above diagram.
- Chapter One: Introduction
- Chapter Two: Understanding the distinctions among research methods
- Chapter Three: Ethical research, writing, and creative work
- Chapter Four: Quantitative Methods (Part 1)
- Chapter Four: Quantitative Methods (Part 2 - Doing Your Study)
- Chapter Five: Qualitative Methods (Part 1)
- Chapter Five: Qualitative Data (Part 2)
- Chapter Six: Critical / Rhetorical Methods (Part 1)
- Chapter Six: Critical / Rhetorical Methods (Part 2)
- Chapter Seven: Presenting Your Results
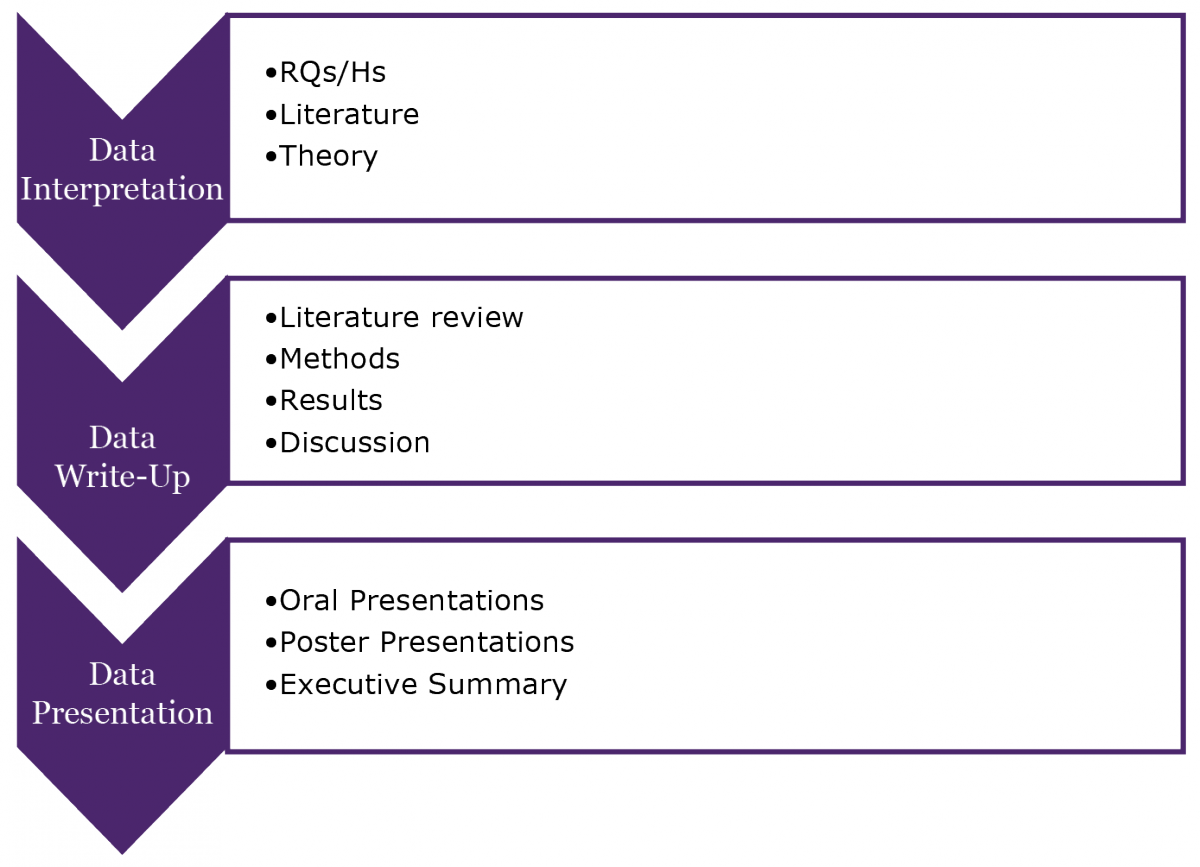
Data Interpretation
Once you have run your statistics, you have to figure out what your findings mean or interpret your data. To do this, you need to tie back your findings to your research questions and/or hypotheses, think about how your findings relate to what you discovered beforehand about the already existing literature, and determine how your findings take the literature or current theory in the field further. Your interpretation of the data you collected will be found in the last section of your paper, what is commonly called the "discussion" section.
Remember Your RQs/Hs
Your research questions and hypotheses, once developed, should guide your study throughout the research process. As you are choosing your research design, choosing how to operationalize your variables, and choosing/conducting your statistical tests, you should always keep your RQs and Hs in mind.
What were you wanting to discover by your study? What were you wanting to test? Make sure you answer these questions clearly for the reader of your study in both the results and discussion section of the paper. (Specific guidelines for these sections will be covered later in this chapter, including the common practice of placing the data as you present it with each research question in the results section.)
Tie Findings to Your Literature Review
As you have seen in chapter 3 and the Appendix, and will see in chapter 7, the literature review is what you use to set up your quantitative study and to show why there is a need for your study. It should start out broad, with the context for your study, and lead into showing what still needs to be known and studied about your topic area, justifying your focus in the study. It will be brought in again in the last section of the paper you write, i.e., the discussion section.
Your paper is like an hourglass – starting out broad and narrowing down in the middle with your actual study and findings, and then moving to broad implications for the larger context of your study near the end.
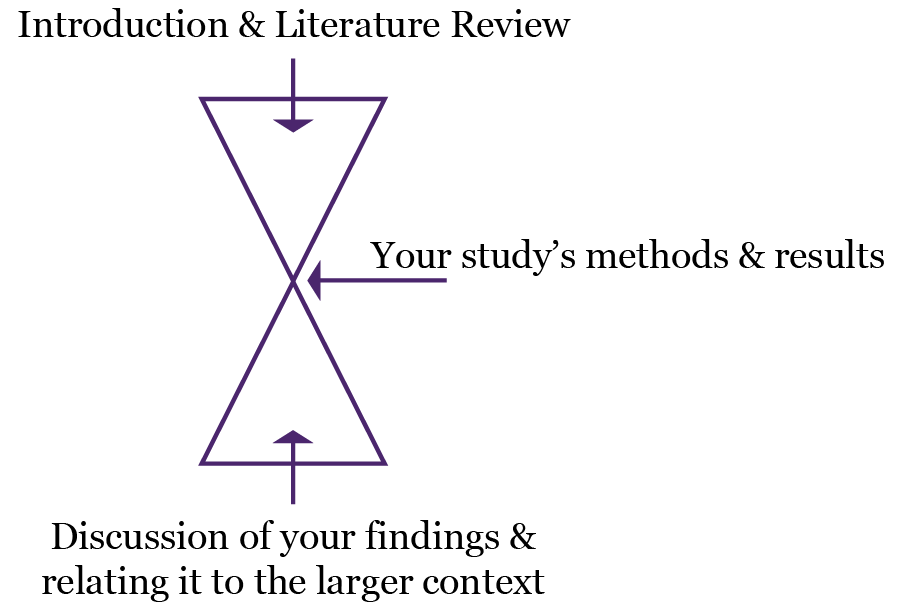
Think about Relationship of Findings to Theory
One of the things you will write about in your discussion or last section of your paper is the implications of what you found. These implications are both practical and theoretical. Practical implications are how the research can provide practical applications to real-world people and issues. Theoretical implications are how the research takes the current academic literature further, specifically, in relationship to theory-building.
Did any of the research you reviewed for your literature review mention a theory your findings could expand upon? If so, you should think about how your findings related to this theory. If not, then think about the theories you have already studied in your communication classes. Would any of them provide a possible explanation of what you found? Would your findings help expand that theory to a different context, the context you studied? Does a theory need to be developed in the area of your research? If so, then what aspects of that theory could your findings help explain?
Data Write-Up
All quantitative studies, when written, have four parts. The first part is the introduction and literature review, the second part is the methods section, the third section is the results or findings, and the fourth section is the discussion section. This portion of this chapter will explain what elements you will need to include in each of these sections.
Literature Review
The beginning of your paper and first few pages sets the tone for your study. It tells the reader what the context of your study is and what other people who are also interested in your topic have studied about your topic.
There are many ways to organize a literature review, as can be seen in the following website. Literature Reviews — The Writing Center at UNC-Chapel Hill
After you have done a thorough literature search on your topic, then you have to organize your literature into topics of some kind. Your main goal is to show what has been done and what still needs to be done, to show the need for your study, so at the end of each section of your literature review, you should identify what still needs to be known about that particular area.
For quantitative research, you should do your literature review before coming up with your research questions/hypotheses. Your questions and hypotheses should flow from the literature. This is different from the other two research methods discussed in this book, which do not rely so heavily on a literature review to situation the study before conducting it.
In the methods section, you should tell your reader how you conducted your study, from start to finish, explaining why you made the choices you did along the way. A reader should be able to replicate your study from the descriptions you provide in this section of your write-up. Common headings in the methods section include a description of the participants, procedures, and analysis.
Participants
For the participants' subheading of the methods section, you should minimally report the demographics of your sample in terms of biological sex (frequencies/percentages), age (range of ages and mean), and ethnicity (frequencies/percentages). If you collected data on other demographics, such as socioeconomic status, religious affiliation, type of occupation, etc., then you can report data for that also in the participants' sub-section.
For the procedures sub-section, you report everything you did to collect your data: how you recruited your participants, including what type of sampling you used (probability or non-probability) and informed consent procedures; how you operationalized your variables (including your survey questions, which often are explained in the methods section briefly while the whole survey can be found in an appendix of your paper); the validity and reliability of your survey instrument or methods you used; and what type of study design you had (experimental, quasi-experimental, or non-experimental). For each one of these design issues, in this sub-section of the methods part, you need to explain why you made the decisions you did in order to answer your research questions or test your hypotheses.
In this section, you explain how you converted your data for analysis and how you analyzed your data. You need to explain what statistics you chose to run for each of your research questions/hypotheses and why.
In this section of your paper, you organize the results by your research questions/hypotheses. For each research question/hypothesis, you should present any descriptive statistic results first and then your inferential statistics results. You do not make any interpretation of what your results mean or why you think you got the results you did. You merely report your results.
Reporting Significant Results
For each of the inferential statistics, there is a typical template you can follow when reporting significant results: reporting the test statistic value, the degrees of freedom 3 , and the probability level. Examples follow for each of the statistics we have talked about in this text.
T-test results
"T-tests results show there was a significant difference found between men and women on their levels of self-esteem, t (df) = t value, p < .05, with men's self-esteem being higher (or lower) (men's mean & standard deviation) than women's self-esteem (women's mean & standard deviation)."
ANOVA results
"ANOVA results indicate there was a significant difference found between [levels of independent variable] on [dependent variable], F (df) = F value, p < .05."
If doing a factorial ANOVA, you would report the above sentence for all of your independent variables (main effects), as well as for the interaction (interaction effect), with language something like: "ANOVA results indicate a significant main effect for [independent variable] on [dependent variable], F (df) = F value, p < .05. .... ANOVA results indicate a significant interaction effect between [independent variables] on [dependent variable], F (df) = F value, p < .05."
See example YouTube tutorial for writing up a two-way ANOVA at the following website.
Factorial Design (Part C): Writing Up Results
Chi-square results
For goodness of fit results, your write-up would look something like: "Using a chi-square goodness of fit test, there was a significant difference found between observed and expected values of [variable], χ2 (df) = chi-square value, p < .05." For test of independence results, it would like like: "Using a chi-square test of independence, there was a significant interaction between [your two variables], χ2 (df) = chi-square value, p < .05."
Correlation results
"Using Pearson's [or Spearman's] correlation coefficient, there was a significant relationship found between [two variables], r (df) = r value, p < .05." If there are a lot of significant correlation results, these results are often presented in a table form.
For more information on these types of tables, see the following website: Correlation Tables .
Regression results
Reporting regression results is more complicated, but generally, you want to inform the reader about how much variance is accounted by the regression model, the significance level of the model, and the significance of the predictor variable. For example:
A regression analysis, predicting GPA scores from GRE scores, was statistically significant, F (1,8) = 10.34, p < .05.
Coefficientsa
The regression equation is: Ŷ = .411 * .005X. For every one unit increase in GRE score, there is a corresponding increase in GPA of .005 (Walen-Frederick, n.d., p. 4).
For more write-up help on regression and other statistics, see the following website location:
Multiple Regression (pp. 217-220)
Reporting Non-Significant Results
You can follow a similar template when reporting non-significant results for all of the above inferential statistics. It is the same as provided in the above examples, except the word "non-significant" replaces the word "significant," and the p values are adjusted to indicate p > .05.
Many times readers of articles do not read the whole article, especially if they are afraid of the statistical sections. When this happens, they often read the discussion section, which makes this a very important section in your writing. You should include the following elements in your discussion section: (a) a summary of your findings, (b) implications, (c) limitations, and (d) future research ideas.
Summary of Findings
You should summarize the answers to your research questions or what you found when testing your hypotheses in this sub-section of the discussion section. You should not report any statistical data here, but just put your results into narrative form. What did you find out that you did not know before doing your study? Answer that question in this sub- section.
Implications
You need to indicate why your study was important, both theoretically and practically. For the theoretical implications, you should relate what you found to the already existing literature, as discussed earlier when the "hourglass" format was mentioned as a way of conceptualizing your whole paper. If your study added anything to the existing theory on a particular topic, you talk about this here as well.
For practical implications, you need to identify for the reader how this study can help people in their real-world experiences related to your topic. You do not want your study to just be important to academic researchers, but also to other professionals and persons interested in your topic.
Limitations
As you get through conducting your study, you are going to realize there are things you wish you had done differently. Rather than hide these things from the reader, it is better to forthrightly state these for the reader. Explain why your study is limited and what you wish you had done in this sub-section.
Future Research
The limitations sub-section usually is tied directly to the future research sub-section, as your limitations mean that future research should be done to deal with these limitations. There may also be other things that could be studied, however, as a result of what you have found. What would other people say are the "gaps" your study left unstudied on your topic? These should be identified, with some suggestions on how they might be studied.
Other Aspects of the Paper
There are other parts of the academic paper you should include in your final write-up. We have provided useful resources for you to consider when including these aspects as part of your paper. For an example paper that uses the required APA format for a research paper write-up, see the following source: Varying Definitions of Online Communication .
Abstract & Titles.
Research Abstracts General Format
Tables, References, & Other Materials.
APA Tables and Figures 1 Reference List: Basic Rules
Data Presentation
You will probably be called upon to present your data in other venues besides in writing. Two of the most common venues are oral presentations such as in class or at conferences, and poster presentations, such as what you might find at conferences. You might also be called upon to not write an academic write-up of your study, but rather to provide an executive summary of the results of your study to the "powers that be," who do not have time to read more than 5 pages or so of a summary. There are good resources for doing all of these online, so we have provided these here.
Oral Presentations
Oral Presentations Delivering Presentations
Poster Presentations
Executive Summary
Executive Summaries Complete the Report Good & Poor Examples of Executive Summaries with the following link: http://unilearning.uow.edu.au/report/4bi1.html
Congratulations! You have learned a great deal about how to go about using quantitative methods for your future research projects. You have learned how to design a quantitative study, conduct a quantitative study, and write about a quantitative study. You have some good resources you can take with you when you leave this class. Now, you just have to apply what you have learned to projects that will come your way in the future.
Remember, just because you may not like one method the best does not mean you should not use it. Your research questions/hypotheses should ALWAYS drive your choice of which method you use. And remember also that you can do quantitative methods!
[NOTE: References are not provided for the websites cited in the text, even though if this was an actual research article, they would need to be cited.]
Baker, E., Baker, W., & Tedesco, J. C. (2007). Organizations respond to phishing: Exploring the public relations tackle box. Communication Research Reports, 24 (4), 327-339.
Benoit, W. L., & Hansen, G. J. (2004). Presidential debate watching, issue knowledge, character evaluation, and vote choice. Human Communication Research, 30 (1), 121-144.
Chatham, A. (1991). Home vs. public schooling: What about relationships in adolescence? Doctoral dissertation, University of Oklahoma.
Cousineau, T. M., Rancourt, D., and Green, T. C. (2006). Web chatter before and after the women's health initiative results: A content analysis of on-line menopause message boards. Journal of Health Communication, 11 (2), 133-147.
Derlega, V., Winstead, B. A., Mathews, A., and Braitman, A. L. (2008). Why does someone reveal highly personal information?: Attributions for and against self-disclosure in close relationships. Communication Research Reports, 25 , 115-130.
Fischer, J., & Corcoran, K. (2007). Measures for clinical practice and research: A sourcebook (volumes 1 & 2) . New York: Oxford University Press.
Guay, S., Boisvert, J.-M., & Freeston, M. H. (2003). Validity of three measures of communication for predicting relationship adjustment and stability among a sample of young couples. Psychological Assessment , 15(3), 392-398.
Holbert, R. L., Tschida, D. A., Dixon, M., Cherry, K., Steuber, K., & Airne, D. (2005). The West Wing and depictions of the American Presidency: Expanding the domains of framing in political communication. Communication Quarterly, 53 (4), 505-522.
Jensen, J. D. (2008). Scientific uncertainty in news coverage of cancer research: Effects of hedging on scientists' and journalists' credibility. Human Communication Research, 34 , 347- 369.
Keyton, J. (2011). Communicating research: Asking questions, finding answers . New York: McGraw Hill.
Lenhart, A., Ling, R., Campbell, S., & Purcell, K. (2010, Apr. 10). Teens and mobile phones . Report from the Pew Internet and American Life Project, retrieved from http://www.pewinternet.org/Reports/2010/Teens-and-Mobile-Phones.aspx .
Maddy, T. (2008). Tests: A comprehensive reference for assessments in psychology, education, and business . Austin, TX: Pro-Ed.
McCollum Jr., J. F., & Bryant, J. (2003). Pacing in children's television programming. Mass Communication and Society, 6 (2), 115-136.
Medved, C. E., Brogan, S. M., McClanahan, A. M., Morris, J. F., & Shepherd, G. J. (2006). Family and work socializing communication: Messages, gender, and ideological implications. Journal of Family Communication, 6 (3), 161-180.
Moyer-Gusé, E., & Nabi, R. L. (2010). Explaining the effects of narrative in an entertainment television program: Overcoming resistance to persuasion. Human Communication Research, 36 , 26-52.
Nabi, R. L. (2009). Cosmetic surgery makeover programs and intentions to undergo cosmetic enhancements: A consideration of three models of media effects. Human Communication Research, 35 , 1-27.
Pearson, J. C., DeWitt, L., Child, J. T., Kahl Jr., D. H., and Dandamudi, V. (2007). Facing the fear: An analysis of speech-anxiety content in public-speaking textbooks. Communication Research Reports, 24 (2), 159-168.
Rubin. R. B., Rubin, A. M., Graham, E., Perse, E. M., & Seibold, D. (2009). Communication research measures II: A sourcebook . New York: Routledge.
Serota, K. B., Levine, T. R., and Boster, F. J. (2010). The prevalence of lying in America: Three studies of reported deception. Human Communication Research, 36 , 1-24.
Sheldon, P. (2008). The relationship between unwillingness-to-communicate and students' facebook use. Journal of Media Psychology, 20 (2), 67–75.
Trochim, W. M. K. (2006). Reliability and validity. Research methods data base , retrieved from http://www.socialresearchmethods.net/kb/relandval.php .
Walen-Frederick, H. (n.d.). Help sheet for reading SPSS printouts . Retrieved from http://www.scribd.com/doc/51982223/help-sheet-for-reading-spss-printouts .
Weaver, A. J., & Wilson, B. J. (2009). The role of graphic and sanitized violence in the enjoyment of television dramas. Human Communication Research, 35 (3), 442-463.
Weber, K., Corrigan, M., Fornash, B., & Neupauer, N. C. (2003). The effect of interest on recall: An experiment. Communication Research Reports, 20 (2), 116-123.
Witt, P. L., & Schrodt, P. (2006). The influence of instructional technology use and teacher immediacy on student affect for teacher and course. Communication Reports, 19 (1), 1-15.
3 Degrees of freedom (df) relate to your sample size and to the number of groups being compared. SPSS always computes the df for your statistics. For more information on degrees of freedom, see the following web-based resources: http://www.youtube.com/watch?v=wsvfasNpU2s and http://www.creative-wisdom.com/pub/df/index.htm .
Back to Previous Spot
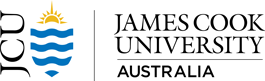
Want to create or adapt books like this? Learn more about how Pressbooks supports open publishing practices.
3.4 Sampling Techniques in Quantitative Research
Target population.
The target population includes the people the researcher is interested in conducting the research and generalizing the findings on. 40 For example, if certain researchers are interested in vaccine-preventable diseases in children five years and younger in Australia. The target population will be all children aged 0–5 years residing in Australia. The actual population is a subset of the target population from which the sample is drawn, e.g. children aged 0–5 years living in the capital cities in Australia. The sample is the people chosen for the study from the actual population (Figure 3.9). The sampling process involves choosing people, and it is distinct from the sample. 40 In quantitative research, the sample must accurately reflect the target population, be free from bias in terms of selection, and be large enough to validate or reject the study hypothesis with statistical confidence and minimise random error. 2
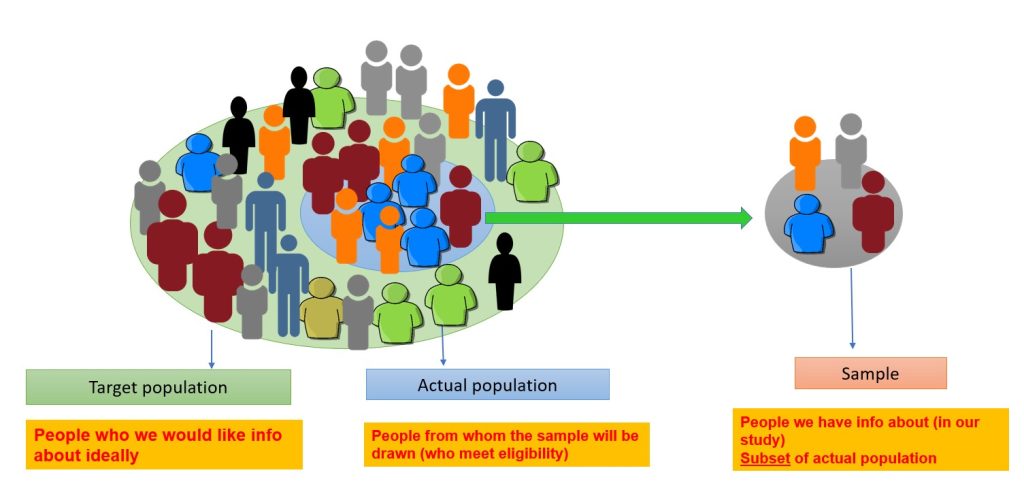
Sampling techniques
Sampling in quantitative research is a critical component that involves selecting a representative subset of individuals or cases from a larger population and often employs sampling techniques based on probability theory. 41 The goal of sampling is to obtain a sample that is large enough and representative of the target population. Examples of probability sampling techniques include simple random sampling, stratified random sampling, systematic random sampling and cluster sampling ( shown below ). 2 The key feature of probability techniques is that they involve randomization. There are two main characteristics of probability sampling. All individuals of a population are accessible to the researcher (theoretically), and there is an equal chance that each person in the population will be chosen to be part of the study sample. 41 While quantitative research often uses sampling techniques based on probability theory, some non-probability techniques may occasionally be utilised in healthcare research. 42 Non-probability sampling methods are commonly used in qualitative research. These include purposive, convenience, theoretical and snowballing and have been discussed in detail in chapter 4.
Sample size calculation
In order to enable comparisons with some level of established statistical confidence, quantitative research needs an acceptable sample size. 2 The sample size is the most crucial factor for reliability (reproducibility) in quantitative research. It is important for a study to be powered – the likelihood of identifying a difference if it exists in reality. 2 Small sample-sized studies are more likely to be underpowered, and results from small samples are more likely to be prone to random error. 2 The formula for sample size calculation varies with the study design and the research hypothesis. 2 There are numerous formulae for sample size calculations, but such details are beyond the scope of this book. For further readings, please consult the biostatistics textbook by Hirsch RP, 2021. 43 However, we will introduce a simple formula for calculating sample size for cross-sectional studies with prevalence as the outcome. 2
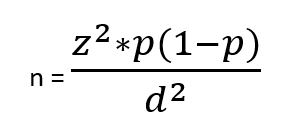
z is the statistical confidence; therefore, z = 1.96 translates to 95% confidence; z = 1.68 translates to 90% confidence
p = Expected prevalence (of health condition of interest)
d = Describes intended precision; d = 0.1 means that the estimate falls +/-10 percentage points of true prevalence with the considered confidence. (e.g. for a prevalence of 40% (0.4), if d=.1, then the estimate will fall between 30% and 50% (0.3 to 0.5).
Example: A district medical officer seeks to estimate the proportion of children in the district receiving appropriate childhood vaccinations. Assuming a simple random sample of a community is to be selected, how many children must be studied if the resulting estimate is to fall within 10% of the true proportion with 95% confidence? It is expected that approximately 50% of the children receive vaccinations
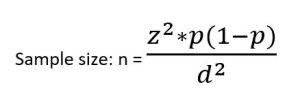
z = 1.96 (95% confidence)
d = 10% = 10/ 100 = 0.1 (estimate to fall within 10%)
p = 50% = 50/ 100 = 0.5
Now we can enter the values into the formula

Given that people cannot be reported in decimal points, it is important to round up to the nearest whole number.
An Introduction to Research Methods for Undergraduate Health Profession Students Copyright © 2023 by Faith Alele and Bunmi Malau-Aduli is licensed under a Creative Commons Attribution-NonCommercial 4.0 International License , except where otherwise noted.
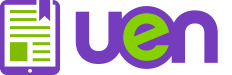
Part II: Data Analysis Methods in Quantitative Research
Data analysis methods in quantitative research.
We started this module with levels of measurement as a way to categorize our data. Data analysis is directed toward answering the original research question and achieving the study purpose (or aim). Now, we are going to delve into two main statistical analyses to describe our data and make inferences about our data:
Descriptive Statistics and Inferential Statistics.
Descriptive Statistics:
Before you panic, we will not be going into statistical analyses very deeply. We want to simply get a good overview of some of the types of general statistical analyses so that it makes some sense to us when we read results in published research articles.
Descriptive statistics summarize or describe the characteristics of a data set. This is a method of simply organizing and describing our data. Why? Because data that are not organized in some fashion are super difficult to interpret.
Let’s say our sample is golden retrievers (population “canines”). Our descriptive statistics tell us more about the same.
- 37% of our sample is male, 43% female
- The mean age is 4 years
- Mode is 6 years
- Median age is 5.5 years
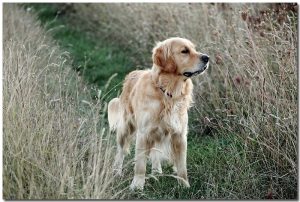
Let’s explore some of the types of descriptive statistics.
Frequency Distributions : A frequency distribution describes the number of observations for each possible value of a measured variable. The numbers are arranged from lowest to highest and features a count of how many times each value occurred.
For example, if 18 students have pet dogs, dog ownership has a frequency of 18.
We might see what other types of pets that students have. Maybe cats, fish, and hamsters. We find that 2 students have hamsters, 9 have fish, 1 has a cat.
You can see that it is very difficult to interpret the various pets into any meaningful interpretation, yes?
Now, let’s take those same pets and place them in a frequency distribution table.
As we can now see, this is much easier to interpret.
Let’s say that we want to know how many books our sample population of students have read in the last year. We collect our data and find this:
We can then take that table and plot it out on a frequency distribution graph. This makes it much easier to see how the numbers are disbursed. Easier on the eyes, yes?
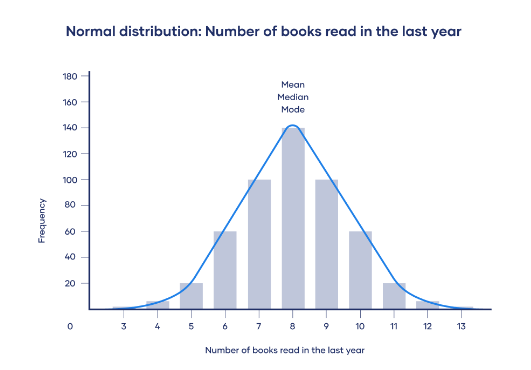
Here’s another example of symmetrical, positive skew, and negative skew:
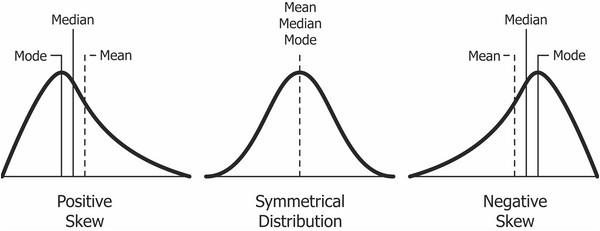
Correlation : Relationships between two research variables are called correlations . Remember, correlation is not cause-and-effect. Correlations simply measure the extent of relationship between two variables. To measure correlation in descriptive statistics, the statistical analysis called Pearson’s correlation coefficient I is often used. You do not need to know how to calculate this for this course. But, do remember that analysis test because you will often see this in published research articles. There really are no set guidelines on what measurement constitutes a “strong” or “weak” correlation, as it really depends on the variables being measured.
However, possible values for correlation coefficients range from -1.00 through .00 to +1.00. A value of +1 means that the two variables are positively correlated, as one variable goes up, the other goes up. A value of r = 0 means that the two variables are not linearly related.
Often, the data will be presented on a scatter plot. Here, we can view the data and there appears to be a straight line (linear) trend between height and weight. The association (or correlation) is positive. That means, that there is a weight increase with height. The Pearson correlation coefficient in this case was r = 0.56.
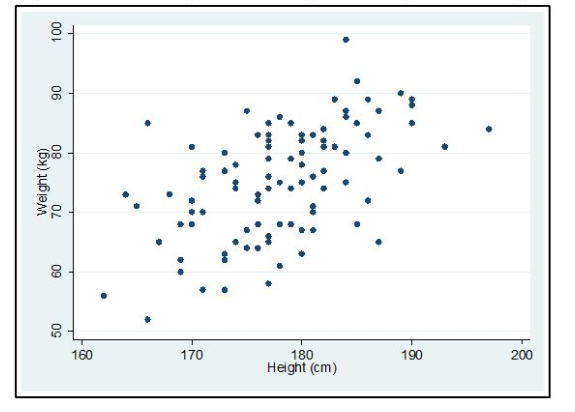
A type I error is made by rejecting a null hypothesis that is true. This means that there was no difference but the researcher concluded that the hypothesis was true.
A type II error is made by accepting that the null hypothesis is true when, in fact, it was false. Meaning there was actually a difference but the researcher did not think their hypothesis was supported.
Hypothesis Testing Procedures : In a general sense, the overall testing of a hypothesis has a systematic methodology. Remember, a hypothesis is an educated guess about the outcome. If we guess wrong, we might set up the tests incorrectly and might get results that are invalid. Sometimes, this is super difficult to get right. The main purpose of statistics is to test a hypothesis.
- Selecting a statistical test. Lots of factors go into this, including levels of measurement of the variables.
- Specifying the level of significance. Usually 0.05 is chosen.
- Computing a test statistic. Lots of software programs to help with this.
- Determining degrees of freedom ( df ). This refers to the number of observations free to vary about a parameter. Computing this is easy (but you don’t need to know how for this course).
- Comparing the test statistic to a theoretical value. Theoretical values exist for all test statistics, which is compared to the study statistics to help establish significance.
Some of the common inferential statistics you will see include:
Comparison tests: Comparison tests look for differences among group means. They can be used to test the effect of a categorical variable on the mean value of some other characteristic.
T-tests are used when comparing the means of precisely two groups (e.g., the average heights of men and women). ANOVA and MANOVA tests are used when comparing the means of more than two groups (e.g., the average heights of children, teenagers, and adults).
- t -tests (compares differences in two groups) – either paired t-test (example: What is the effect of two different test prep programs on the average exam scores for students from the same class?) or independent t-test (example: What is the difference in average exam scores for students from two different schools?)
- analysis of variance (ANOVA, which compares differences in three or more groups) (example: What is the difference in average pain levels among post-surgical patients given three different painkillers?) or MANOVA (compares differences in three or more groups, and 2 or more outcomes) (example: What is the effect of flower species on petal length, petal width, and stem length?)
Correlation tests: Correlation tests check whether variables are related without hypothesizing a cause-and-effect relationship.
- Pearson r (measures the strength and direction of the relationship between two variables) (example: How are latitude and temperature related?)
Nonparametric tests: Non-parametric tests don’t make as many assumptions about the data, and are useful when one or more of the common statistical assumptions are violated. However, the inferences they make aren’t as strong as with parametric tests.
- chi-squared ( X 2 ) test (measures differences in proportions). Chi-square tests are often used to test hypotheses. The chi-square statistic compares the size of any discrepancies between the expected results and the actual results, given the size of the sample and the number of variables in the relationship. For example, the results of tossing a fair coin meet these criteria. We can apply a chi-square test to determine which type of candy is most popular and make sure that our shelves are well stocked. Or maybe you’re a scientist studying the offspring of cats to determine the likelihood of certain genetic traits being passed to a litter of kittens.
Inferential Versus Descriptive Statistics Summary Table
Statistical Significance Versus Clinical Significance
Finally, when it comes to statistical significance in hypothesis testing, the normal probability value in nursing is <0.05. A p=value (probability) is a statistical measurement used to validate a hypothesis against measured data in the study. Meaning, it measures the likelihood that the results were actually observed due to the intervention, or if the results were just due by chance. The p-value, in measuring the probability of obtaining the observed results, assumes the null hypothesis is true.
The lower the p-value, the greater the statistical significance of the observed difference.
In the example earlier about our diabetic patients receiving online diet education, let’s say we had p = 0.05. Would that be a statistically significant result?
If you answered yes, you are correct!
What if our result was p = 0.8?
Not significant. Good job!
That’s pretty straightforward, right? Below 0.05, significant. Over 0.05 not significant.
Could we have significance clinically even if we do not have statistically significant results? Yes. Let’s explore this a bit.
Statistical hypothesis testing provides little information for interpretation purposes. It’s pretty mathematical and we can still get it wrong. Additionally, attaining statistical significance does not really state whether a finding is clinically meaningful. With a large enough sample, even a small very tiny relationship may be statistically significant. But, clinical significance is the practical importance of research. Meaning, we need to ask what the palpable effects may be on the lives of patients or healthcare decisions.
Remember, hypothesis testing cannot prove. It also cannot tell us much other than “yeah, it’s probably likely that there would be some change with this intervention”. Hypothesis testing tells us the likelihood that the outcome was due to an intervention or influence and not just by chance. Also, as nurses and clinicians, we are not concerned with a group of people – we are concerned at the individual, holistic level. The goal of evidence-based practice is to use best evidence for decisions about specific individual needs.
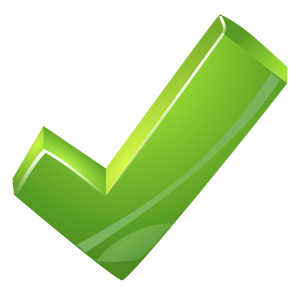
Additionally, begin your Discussion section. What are the implications to practice? Is there little evidence or a lot? Would you recommend additional studies? If so, what type of study would you recommend, and why?
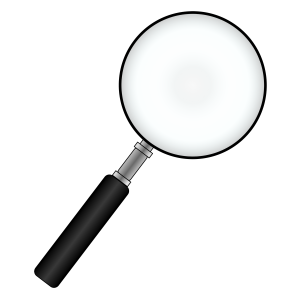
- Were all the important results discussed?
- Did the researchers discuss any study limitations and their possible effects on the credibility of the findings? In discussing limitations, were key threats to the study’s validity and possible biases reviewed? Did the interpretations take limitations into account?
- What types of evidence were offered in support of the interpretation, and was that evidence persuasive? Were results interpreted in light of findings from other studies?
- Did the researchers make any unjustifiable causal inferences? Were alternative explanations for the findings considered? Were the rationales for rejecting these alternatives convincing?
- Did the interpretation consider the precision of the results and/or the magnitude of effects?
- Did the researchers draw any unwarranted conclusions about the generalizability of the results?
- Did the researchers discuss the study’s implications for clinical practice or future nursing research? Did they make specific recommendations?
- If yes, are the stated implications appropriate, given the study’s limitations and the magnitude of the effects as well as evidence from other studies? Are there important implications that the report neglected to include?
- Did the researchers mention or assess clinical significance? Did they make a distinction between statistical and clinical significance?
- If clinical significance was examined, was it assessed in terms of group-level information (e.g., effect sizes) or individual-level results? How was clinical significance operationalized?
References & Attribution
“ Green check mark ” by rawpixel licensed CC0 .
“ Magnifying glass ” by rawpixel licensed CC0
“ Orange flame ” by rawpixel licensed CC0 .
Polit, D. & Beck, C. (2021). Lippincott CoursePoint Enhanced for Polit’s Essentials of Nursing Research (10th ed.). Wolters Kluwer Health
Vaid, N. K. (2019) Statistical performance measures. Medium. https://neeraj-kumar-vaid.medium.com/statistical-performance-measures-12bad66694b7
Evidence-Based Practice & Research Methodologies Copyright © by Tracy Fawns is licensed under a Creative Commons Attribution-NonCommercial-ShareAlike 4.0 International License , except where otherwise noted.
Share This Book
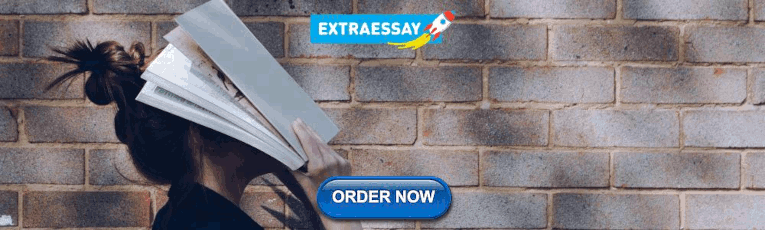
IMAGES
VIDEO
COMMENTS
4.1 INTRODUCTION To complete this study properly, it is necessary to analyse the data collected in order to test the hypothesis and answer the research questions. As already indicated in the preceding chapter, data is interpreted in a descriptive form. This chapter comprises the analysis, presentation and interpretation of the findings resulting
DATA PRESENTATION, ANALYSIS AND INTERPRETATION. 4.0 Introduction. This chapter is concerned with data pres entation, of the findings obtained through the study. The. findings are presented in ...
Quantitative Research Dissertation Chapters 4 and 5 (Suggested Content) Information below is suggested content; seek guidance from committee chair about content of all chapters in the dissertation. Brief Review - Chapter 3: Method (not Methodology) There is a tendency to report results of sample and measurement information in Chapter 4.
CHAPTER IV PRESENTATION, ANALYSIS AND INTERPRETATION OF DATA. This chapter presents the results, the analysis and interpretation of data gathered. from the answers to the questionnaires distributed to the field. The said data were. presented in tabular form in accordance with the specific questions posited on the. statement of the problem.
As a general guide, your results chapter will typically include the following: Some demographic data about your sample; Reliability tests (if you used measurement scales); Descriptive statistics; Inferential statistics (if your research objectives and questions require these); Hypothesis tests (again, if your research objectives and questions require these); We'll discuss each of these ...
older represented 10% of the sample, 35% were between 51 and 60, 20% were between the. ages of 41-50. The 31-40 age group was also 20% of the sample and 15% of the participants. declined to answer. Graphic displays of demographics on company size, work status, age, and industry sector are provided in Appendix F.
How to write Chapter 4 Discussion Quantitative Study | Simple Correlation (Part 1)With review on how to write Chapter 3 Results
The income values were in GBP. It was found that 13% of the respondents had income 'up to 30000', 27% had income between '31000 to 50000', 52.5% had income between '51000 to 100000', and 7.5% had income 'Above 100000'. This suggests that most of the respondents had an annual income between '31000 to 50000' GBP.
In the first paragraph of your quantitative chapter 4, the results chapter, restate the research questions that will be examined. This reminds the reader of what you're going to investigate after having been trough the details of your methodology. ... The next paragraph should describe the sample using demographics and research variables ...
Chapter 4 Considerations. Topic 1: Chapter 4. How do you organize your chapter? Your chapter needs to be organized in a way that answers your research questions. The information must be organized in a way that is logical and easy to follow for your reader. You may describe your sample here if this is something that emerged from your data ...
4.1 General Approach The approach applied in this chapter yields an overall estimate of expected out-comes at a given school size. As such the approach can be considered a type of meta-analysis. However, common meta-analysis methods cannot be applied when dealing with research on the effects of school size. The main reason for this is that
The researcher narrates a summary of the demographics of the sample, and if the table is concise, presents demographics in a table format after the narration. ... two paragraphs of the results of the research study findings (Creswell, 2004). Add a ... Writing a Dissertation's Chapter 4 and 5 7 Chapter Four - Quantitative Version
DATA ANALYSIS AND PRESENTATION OF RES EARCH FINDINGS 4.1 Introduction. The chapter contains presentation, analysis and dis cussion of the data collected by the researcher. during the data ...
These parts can also be used as a checklist when working through the steps of your study. Specifically, part 1 focuses on planning a quantitative study (collecting data), part two explains the steps involved in doing a quantitative study, and part three discusses how to make sense of your results (organizing and analyzing data). Research Methods.
Present Demographics. Present the descriptive data: explaining the age, gender, or relevant related information on the population (describe the sample). Summarize the demographics of the sample, and present in a table format after the narration (Simon, 2006). Otherwise, the table is included as an Appendix and referred to in the narrative of ...
Chapter PDF Available. Chapter 4. Quantitative Analysis. January 2022. DOI: 10.1039/9781839162695-00081. In book: Advances in Portable X-ray Fluorescence Spectrometry (pp.81-107) Authors: Brandon ...
4.1 INTRODUCTION. This chapter presents the data and a discussion of the findings. A quantitative, descriptive survey design was used to collect data from subjects. Two questionnaires, one for diabetic patients and the other for family members of diabetic patients, were administered to subjects by the researcher personally.
Chapter Two: Understanding the distinctions among research methods. Chapter Three: Ethical research, writing, and creative work. Chapter Four: Quantitative Methods. Chapter Four: Quantitative Methods (Part 1) Chapter Five: Qualitative Methods. Chapter Six: Critical / Rhetorical Methods. Chapter Seven: Presenting Your Results.
Chapter 4. What needs to be included in the chapter? The topics below are typically included in this chapter, and often in this order (check with your Chair): Introduction. Remind the reader what your research questions were. In a qualitative study you will restate the research questions. In a quantitative study you will present the hypotheses.
4.1 INTRODUCTION. This chapter describes the analysis of data followed by a discussion of the research findings. The findings relate to the research questions that guided the study. Data were analyzed to identify, describe and explore the relationship between death anxiety and death attitudes of nurses in a private acute care hospital and to ...
Sample size calculation. In order to enable comparisons with some level of established statistical confidence, quantitative research needs an acceptable sample size. 2 The sample size is the most crucial factor for reliability (reproducibility) in quantitative research. It is important for a study to be powered - the likelihood of identifying a difference if it exists in reality. 2 Small ...
CHAPTER IV Findings The purpose of this chapter is to present the analysis of the data. This study assesses the initial and exiting attitudes toward mathematics and academic outcomes of students placed in DVM001 Basic Arithmetic at a community college. The study included 329 freshman and sophomore students who were tested and placed in DVM001 ...
Let's say our sample is golden retrievers (population "canines"). Our descriptive statistics tell us more about the same. 37% of our sample is male, 43% female; The mean age is 4 years; Mode is 6 years; Median age is 5.5 years; Find Kevin by Franco Vannini. CC BY 2.0. Let's explore some of the types of descriptive statistics.