- Accountancy
- Business Studies
- Organisational Behaviour
- Human Resource Management
- Entrepreneurship
- CBSE Class 11 Statistics for Economics Notes
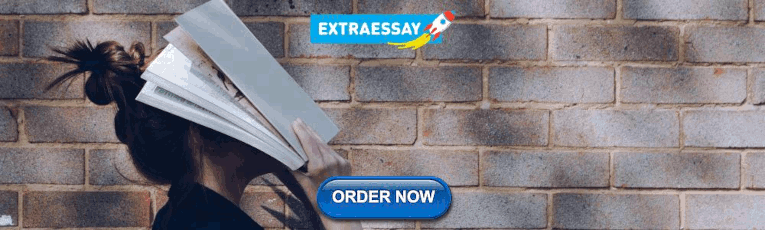
Chapter 1: Concept of Economics and Significance of Statistics in Economics
- Statistics for Economics | Functions, Importance, and Limitations
Chapter 2: Collection of Data
- Data Collection & Its Methods
- Sources of Data Collection | Primary and Secondary Sources
- Direct Personal Investigation: Meaning, Suitability, Merits, Demerits and Precautions
- Indirect Oral Investigation : Suitability, Merits, Demerits and Precautions
- Difference between Direct Personal Investigation and Indirect Oral Investigation
- Information from Local Source or Correspondents: Meaning, Suitability, Merits, and Demerits
- Questionnaires and Schedules Method of Data Collection
- Difference between Questionnaire and Schedule
- Qualities of a Good Questionnaire and types of Questions
- What are the Published Sources of Collecting Secondary Data?
- What Precautions should be taken before using Secondary Data?
- Two Important Sources of Secondary Data: Census of India and Reports & Publications of NSSO
- What is National Sample Survey Organisation (NSSO)?
- What is Census Method of Collecting Data?
- Sample Method of Collection of Data
- Methods of Sampling
- Father of Indian Census
- What makes a Sampling Data Reliable?
- Difference between Census Method and Sampling Method of Collecting Data
- What are Statistical Errors?
Chapter 3: Organisation of Data
- Organization of Data
- Objectives and Characteristics of Classification of Data
- Classification of Data in Statistics | Meaning and Basis of Classification of Data
- Concept of Variable and Raw Data
- Types of Statistical Series
- Difference between Frequency Array and Frequency Distribution
- Types of Frequency Distribution
Chapter 4: Presentation of Data: Textual and Tabular
- Textual Presentation of Data: Meaning, Suitability, and Drawbacks
Tabular Presentation of Data: Meaning, Objectives, Features and Merits
- Different Types of Tables
- Classification and Tabulation of Data
Chapter 5: Diagrammatic Presentation of Data
- Diagrammatic Presentation of Data: Meaning , Features, Guidelines, Advantages and Disadvantages
- Types of Diagrams
- Bar Graph | Meaning, Types, and Examples
- Pie Diagrams | Meaning, Example and Steps to Construct
- Histogram | Meaning, Example, Types and Steps to Draw
- Frequency Polygon | Meaning, Steps to Draw and Examples
- Ogive (Cumulative Frequency Curve) and its Types
- What is Arithmetic Line-Graph or Time-Series Graph?
- Diagrammatic and Graphic Presentation of Data
Chapter 6: Measures of Central Tendency: Arithmetic Mean
- Measures of Central Tendency in Statistics
- Arithmetic Mean: Meaning, Example, Types, Merits, and Demerits
- What is Simple Arithmetic Mean?
- Calculation of Mean in Individual Series | Formula of Mean
- Calculation of Mean in Discrete Series | Formula of Mean
- Calculation of Mean in Continuous Series | Formula of Mean
- Calculation of Arithmetic Mean in Special Cases
- Weighted Arithmetic Mean
Chapter 7: Measures of Central Tendency: Median and Mode
- Median(Measures of Central Tendency): Meaning, Formula, Merits, Demerits, and Examples
- Calculation of Median for Different Types of Statistical Series
- Calculation of Median in Individual Series | Formula of Median
- Calculation of Median in Discrete Series | Formula of Median
- Calculation of Median in Continuous Series | Formula of Median
- Graphical determination of Median
- Mode: Meaning, Formula, Merits, Demerits, and Examples
- Calculation of Mode in Individual Series | Formula of Mode
- Calculation of Mode in Discrete Series | Formula of Mode
- Grouping Method of Calculating Mode in Discrete Series | Formula of Mode
- Calculation of Mode in Continuous Series | Formula of Mode
- Calculation of Mode in Special Cases
- Calculation of Mode by Graphical Method
- Mean, Median and Mode| Comparison, Relationship and Calculation
Chapter 8: Measures of Dispersion
- Measures of Dispersion | Meaning, Absolute and Relative Measures of Dispersion
- Range | Meaning, Coefficient of Range, Merits and Demerits, Calculation of Range
- Calculation of Range and Coefficient of Range
- Interquartile Range and Quartile Deviation
- Partition Value | Quartiles, Deciles and Percentiles
- Quartile Deviation and Coefficient of Quartile Deviation: Meaning, Formula, Calculation, and Examples
- Quartile Deviation in Discrete Series | Formula, Calculation and Examples
- Quartile Deviation in Continuous Series | Formula, Calculation and Examples
- Mean Deviation: Coefficient of Mean Deviation, Merits, and Demerits
- Calculation of Mean Deviation for different types of Statistical Series
- Mean Deviation from Mean | Individual, Discrete, and Continuous Series
- Mean Deviation from Median | Individual, Discrete, and Continuous Series
- Standard Deviation: Meaning, Coefficient of Standard Deviation, Merits, and Demerits
- Standard Deviation in Individual Series
- Methods of Calculating Standard Deviation in Discrete Series
- Methods of calculation of Standard Deviation in frequency distribution series
- Combined Standard Deviation: Meaning, Formula, and Example
- How to calculate Variance?
- Coefficient of Variation: Meaning, Formula and Examples
- Lorenz Curveb : Meaning, Construction, and Application
Chapter 9: Correlation
- Correlation: Meaning, Significance, Types and Degree of Correlation
- Methods of measurements of Correlation
- Calculation of Correlation with Scattered Diagram
- Spearman's Rank Correlation Coefficient
- Karl Pearson's Coefficient of Correlation
- Karl Pearson's Coefficient of Correlation | Methods and Examples
Chapter 10: Index Number
- Index Number | Meaning, Characteristics, Uses and Limitations
- Methods of Construction of Index Number
- Unweighted or Simple Index Numbers: Meaning and Methods
- Methods of calculating Weighted Index Numbers
- Fisher's Index Number as an Ideal Method
- Fisher's Method of calculating Weighted Index Number
- Paasche's Method of calculating Weighted Index Number
- Laspeyre's Method of calculating Weighted Index Number
- Laspeyre's, Paasche's, and Fisher's Methods of Calculating Index Number
- Consumer Price Index (CPI) or Cost of Living Index Number: Construction of Consumer Price Index|Difficulties and Uses of Consumer Price Index
- Methods of Constructing Consumer Price Index (CPI)
- Wholesale Price Index (WPI) | Meaning, Uses, Merits, and Demerits
- Index Number of Industrial Production : Characteristics, Construction & Example
- Inflation and Index Number
Important Formulas in Statistics for Economics
- Important Formulas in Statistics for Economics | Class 11
What is Tabulation?
The systematic presentation of numerical data in rows and columns is known as Tabulation . It is designed to make presentation simpler and analysis easier. This type of presentation facilitates comparison by putting relevant information close to one another, and it helps in further statistical analysis and interpretation. One of the most important devices for presenting the data in a condensed and readily comprehensible form is tabulation. It aims to provide as much information as possible in the minimum possible space while maintaining the quality and usefulness of the data.
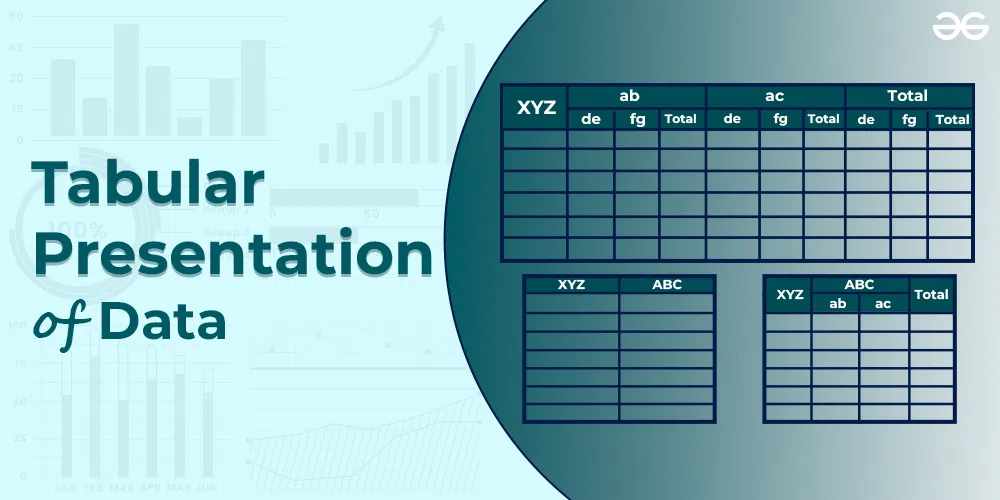
“Tabulation involves the orderly and systematic presentation of numerical data in a form designed to elucidate the problem under consideration.” – L.R. Connor
Objectives of Tabulation
The aim of tabulation is to summarise a large amount of numerical information into the simplest form. The following are the main objectives of tabulation:
- To make complex data simpler: The main aim of tabulation is to present the classified data in a systematic way. The purpose is to condense the bulk of information (data) under investigation into a simple and meaningful form.
- To save space: Tabulation tries to save space by condensing data in a meaningful form while maintaining the quality and quantity of the data.
- To facilitate comparison: It also aims to facilitate quick comparison of various observations by providing the data in a tabular form.
- To facilitate statistical analysis: Tabulation aims to facilitate statistical analysis because it is the stage between data classification and data presentation. Various statistical measures, including averages, dispersion, correlation, and others, are easily calculated from data that has been systematically tabulated.
- To provide a reference: Since data may be easily identifiable and used when organised in tables with titles and table numbers, tabulation aims to provide a reference for future studies.
Features of a Good Table
Tabulation is a very specialised job. It requires a thorough knowledge of statistical methods, as well as abilities, experience, and common sense. A good table must have the following characteristics:
- Title: The top of the table must have a title and it needs to be very appealing and attractive.
- Manageable Size: The table shouldn’t be too big or too small. The size of the table should be in accordance with its objectives and the characteristics of the data. It should completely cover all significant characteristics of data.
- Attractive: A table should have an appealing appearance that appeals to both the sight and the mind so that the reader can grasp it easily without any strain.
- Special Emphasis: The data to be compared should be placed in the left-hand corner of columns, with their titles in bold letters.
- Fit with the Objective: The table should reflect the objective of the statistical investigation.
- Simplicity: To make the table easily understandable, it should be simple and compact.
- Data Comparison: The data to be compared must be placed closely in the columns.
- Numbered Columns and Rows: When there are several rows and columns in a table, they must be numbered for reference.
- Clarity: A table should be prepared so that even a layman may make conclusions from it. The table should contain all necessary information and it must be self-explanatory.
- Units: The unit designations should be written on the top of the table, below the title. For example, Height in cm, Weight in kg, Price in ₹, etc. However, if different items have different units, then they should be mentioned in the respective rows and columns.
- Suitably Approximated: If the figures are large, then they should be rounded or approximated.
- Scientifically Prepared: The preparation of the table should be done in a systematic and logical manner and should be free from any kind of ambiguity and overlapping.
Components of a Table
A table’s preparation is an art that requires skilled data handling. It’s crucial to understand the components of a good statistical table before constructing one. A table is created when all of these components are put together in a systematic order. In simple terms, a good table should include the following components:
1. Table Number:
Each table needs to have a number so it may be quickly identified and used as a reference.
- If there are many tables, they should be numbered in a logical order.
- The table number can be given at the top of the table or the beginning of the table title.
- The table is also identified by its location using subscripted numbers like 1.2, 2.1, etc. For instance, Table Number 3.1 should be seen as the first table of the third chapter.
Each table should have a suitable title. A table’s contents are briefly described in the title.
- The title should be simple, self-explanatory, and free from ambiguity.
- A title should be brief and presented clearly, usually below the table number.
- In certain cases, a long title is preferable for clarification. In these cases, a ‘Catch Title’ may be placed above the ‘Main Title’. For instance , the table’s contents might come after the firm’s name, which appears as a catch title.
- Contents of Title: The title should include the following information: (i) Nature of data, or classification criteria (ii) Subject-matter (iii) Place to which the data relates (iv) Time to which the data relates (v) Source to which the data belongs (vi) Reference to the data, if available.
3. Captions or Column Headings:
A column designation is given to explain the figures in the column at the top of each column in a table. This is referred to as a “Column heading” or “Caption”.
- Captions are used to describe the names or heads of vertical columns.
- To save space, captions are generally placed in small letters in the middle of the columns.
4. Stubs or Row Headings:
Each row of the table needs to have a heading, similar to a caption or column heading. The headers of horizontal rows are referred to as stubs. A brief description of the row headers may also be provided at the table’s left-hand top.
5. Body of Table:
The table’s most crucial component is its body, which contains data (numerical information).
- The location of any one figure or data in the table is fixed and determined by the row and column of the table.
- The columns and rows in the main body’s arrangement of numerical data are arranged from top to bottom.
- The size and shape of the main body should be planned in accordance with the nature of the figures and the purpose of the study.
- As the body of the table summarises the facts and conclusions of the statistical investigation, it must be ensured that the table does not have irrelevant information.
6. Unit of Measurement:
If the unit of measurement of the figures in the table (real data) does not change throughout the table, it should always be provided along with the title.
- However, these units must be mentioned together with stubs or captions if rows or columns have different units.
- If there are large figures, they should be rounded up and the rounding method should be stated.
7. Head Notes:
If the main title does not convey enough information, a head note is included in small brackets in prominent words right below the main title.
- A head-note is included to convey any relevant information.
- For instance, the table frequently uses the units of measurement “in million rupees,” “in tonnes,” “in kilometres,” etc. Head notes are also known as Prefatory Notes .
8. Source Note:
A source note refers to the place where information was obtained.
- In the case of secondary data, a source note is provided.
- Name of the book, page number, table number, etc., from which the data were collected should all be included in the source. If there are multiple sources, each one must be listed in the source note.
- If a reader wants to refer to the original data, the source note enables him to locate the data. Usually, the source note appears at the bottom of the table. For example, the source note may be: ‘Census of India, 2011’.
- Importance: A source note is useful for three reasons: -> It provides credit to the source (person or group), who collected the data; -> It provides a reference to source material that may be more complete; -> It offers some insight into the reliability of the information and its source.
9. Footnotes:
The footnote is the last part of the table. The unique characteristic of the data content of the table that is not self-explanatory and has not previously been explained is mentioned in the footnote.
- Footnotes are used to provide additional information that is not provided by the heading, title, stubs, caption, etc.
- When there are many footnotes, they are numbered in order.
- Footnotes are identified by the symbols *, @, £, etc.
- In general, footnotes are used for the following reasons: (i) To highlight any exceptions to the data (ii)Any special circumstances affecting the data; and (iii)To clarify any information in the data.
.webp)
Merits of Tabular Presentation of Data
The following are the merits of tabular presentation of data:
- Brief and Simple Presentation: Tabular presentation is possibly the simplest method of data presentation. As a result, information is simple to understand. A significant amount of statistical data is also presented in a very brief manner.
- Facilitates Comparison: By grouping the data into different classes, tabulation facilitates data comparison.
- Simple Analysis: Analysing data from tables is quite simple. One can determine the data’s central tendency, dispersion, and correlation by organising the data as a table.
- Highlights Characteristics of the Data: Tabulation highlights characteristics of the data. As a result of this, it is simple to remember the statistical facts.
- Cost-effective: Tabular presentation is a very cost-effective way to convey data. It saves time and space.
- Provides Reference: As the data provided in a tabular presentation can be used for other studies and research, it acts as a source of reference.
Please Login to comment...
Similar reads.
- Statistics for Economics
Improve your Coding Skills with Practice
What kind of Experience do you want to share?
Presentation of Data
Statistics deals with the collection, presentation and analysis of the data, as well as drawing meaningful conclusions from the given data. Generally, the data can be classified into two different types, namely primary data and secondary data. If the information is collected by the investigator with a definite objective in their mind, then the data obtained is called the primary data. If the information is gathered from a source, which already had the information stored, then the data obtained is called secondary data. Once the data is collected, the presentation of data plays a major role in concluding the result. Here, we will discuss how to present the data with many solved examples.
What is Meant by Presentation of Data?
As soon as the data collection is over, the investigator needs to find a way of presenting the data in a meaningful, efficient and easily understood way to identify the main features of the data at a glance using a suitable presentation method. Generally, the data in the statistics can be presented in three different forms, such as textual method, tabular method and graphical method.
Presentation of Data Examples
Now, let us discuss how to present the data in a meaningful way with the help of examples.
Consider the marks given below, which are obtained by 10 students in Mathematics:
36, 55, 73, 95, 42, 60, 78, 25, 62, 75.
Find the range for the given data.
Given Data: 36, 55, 73, 95, 42, 60, 78, 25, 62, 75.
The data given is called the raw data.
First, arrange the data in the ascending order : 25, 36, 42, 55, 60, 62, 73, 75, 78, 95.
Therefore, the lowest mark is 25 and the highest mark is 95.
We know that the range of the data is the difference between the highest and the lowest value in the dataset.
Therefore, Range = 95-25 = 70.
Note: Presentation of data in ascending or descending order can be time-consuming if we have a larger number of observations in an experiment.
Now, let us discuss how to present the data if we have a comparatively more number of observations in an experiment.
Consider the marks obtained by 30 students in Mathematics subject (out of 100 marks)
10, 20, 36, 92, 95, 40, 50, 56, 60, 70, 92, 88, 80, 70, 72, 70, 36, 40, 36, 40, 92, 40, 50, 50, 56, 60, 70, 60, 60, 88.
In this example, the number of observations is larger compared to example 1. So, the presentation of data in ascending or descending order is a bit time-consuming. Hence, we can go for the method called ungrouped frequency distribution table or simply frequency distribution table . In this method, we can arrange the data in tabular form in terms of frequency.
For example, 3 students scored 50 marks. Hence, the frequency of 50 marks is 3. Now, let us construct the frequency distribution table for the given data.
Therefore, the presentation of data is given as below:
The following example shows the presentation of data for the larger number of observations in an experiment.
Consider the marks obtained by 100 students in a Mathematics subject (out of 100 marks)
95, 67, 28, 32, 65, 65, 69, 33, 98, 96,76, 42, 32, 38, 42, 40, 40, 69, 95, 92, 75, 83, 76, 83, 85, 62, 37, 65, 63, 42, 89, 65, 73, 81, 49, 52, 64, 76, 83, 92, 93, 68, 52, 79, 81, 83, 59, 82, 75, 82, 86, 90, 44, 62, 31, 36, 38, 42, 39, 83, 87, 56, 58, 23, 35, 76, 83, 85, 30, 68, 69, 83, 86, 43, 45, 39, 83, 75, 66, 83, 92, 75, 89, 66, 91, 27, 88, 89, 93, 42, 53, 69, 90, 55, 66, 49, 52, 83, 34, 36.
Now, we have 100 observations to present the data. In this case, we have more data when compared to example 1 and example 2. So, these data can be arranged in the tabular form called the grouped frequency table. Hence, we group the given data like 20-29, 30-39, 40-49, ….,90-99 (As our data is from 23 to 98). The grouping of data is called the “class interval” or “classes”, and the size of the class is called “class-size” or “class-width”.
In this case, the class size is 10. In each class, we have a lower-class limit and an upper-class limit. For example, if the class interval is 30-39, the lower-class limit is 30, and the upper-class limit is 39. Therefore, the least number in the class interval is called the lower-class limit and the greatest limit in the class interval is called upper-class limit.
Hence, the presentation of data in the grouped frequency table is given below:
Hence, the presentation of data in this form simplifies the data and it helps to enable the observer to understand the main feature of data at a glance.
Practice Problems
- The heights of 50 students (in cms) are given below. Present the data using the grouped frequency table by taking the class intervals as 160 -165, 165 -170, and so on. Data: 161, 150, 154, 165, 168, 161, 154, 162, 150, 151, 162, 164, 171, 165, 158, 154, 156, 172, 160, 170, 153, 159, 161, 170, 162, 165, 166, 168, 165, 164, 154, 152, 153, 156, 158, 162, 160, 161, 173, 166, 161, 159, 162, 167, 168, 159, 158, 153, 154, 159.
- Three coins are tossed simultaneously and each time the number of heads occurring is noted and it is given below. Present the data using the frequency distribution table. Data: 0, 1, 2, 2, 1, 2, 3, 1, 3, 0, 1, 3, 1, 1, 2, 2, 0, 1, 2, 1, 3, 0, 0, 1, 1, 2, 3, 2, 2, 0.
To learn more Maths-related concepts, stay tuned with BYJU’S – The Learning App and download the app today!
Leave a Comment Cancel reply
Your Mobile number and Email id will not be published. Required fields are marked *
Request OTP on Voice Call
Post My Comment

- Share Share
Register with BYJU'S & Download Free PDFs
Register with byju's & watch live videos.

Presentation of Statistical Data
- First Online: 24 February 2016
Cite this chapter
- Abdul Quader Miah 2
2729 Accesses
Data are collected often in raw form. These are then not useable unless summarized. The techniques of presentation in tabular and graphical forms are introduced. Some illustrations provided are real-world examples. Graphical presentations cover bar chart, pie chart, histogram, frequency polygon, pareto chart, frequency curve and line diagram.
This is a preview of subscription content, log in via an institution to check access.
Access this chapter
- Available as EPUB and PDF
- Read on any device
- Instant download
- Own it forever
- Compact, lightweight edition
- Dispatched in 3 to 5 business days
- Free shipping worldwide - see info
- Durable hardcover edition
Tax calculation will be finalised at checkout
Purchases are for personal use only
Institutional subscriptions
Asian Institute of Technology, AIT Alumni 1961–1987 Tracer Study , Bangkok: AIT, 1990, p.9
Google Scholar
International Monetary Fund
Ministry of Finance, Royal Thai Government, Thailand
NESDB, National Economic and Social Development Board, Thailand
National Statistical Office, Thailand
Pacific Asia Travel Association
Toyota Motors, Thailand
U.S. Bureau of the Census. The Census Bureau as cities and other incorporated places, which have 2500, or more inhabitants define “Urban areas”
Download references
Author information
Authors and affiliations.
Asian Institute of Technology, Bangkok, Thailand
Abdul Quader Miah
You can also search for this author in PubMed Google Scholar
Corresponding author
Correspondence to Abdul Quader Miah .
Rights and permissions
Reprints and permissions
Copyright information
© 2016 Springer Science+Business Media Singapore
About this chapter
Miah, A.Q. (2016). Presentation of Statistical Data. In: Applied Statistics for Social and Management Sciences. Springer, Singapore. https://doi.org/10.1007/978-981-10-0401-8_2
Download citation
DOI : https://doi.org/10.1007/978-981-10-0401-8_2
Published : 24 February 2016
Publisher Name : Springer, Singapore
Print ISBN : 978-981-10-0399-8
Online ISBN : 978-981-10-0401-8
eBook Packages : Mathematics and Statistics Mathematics and Statistics (R0)
Share this chapter
Anyone you share the following link with will be able to read this content:
Sorry, a shareable link is not currently available for this article.
Provided by the Springer Nature SharedIt content-sharing initiative
- Publish with us
Policies and ethics
- Find a journal
- Track your research

Statistics Made Easy
What is Tabular Data? (Definition & Example)
In statistics, tabular data refers to data that is organized in a table with rows and columns.

Within the table, the rows represent observations and the columns represent attributes for those observations.
For example, the following table represents tabular data:

This dataset has 9 rows and 5 columns.
Each row represents one basketball player and the five columns describe different attributes about the player including:
- Player name
- Minutes played
The opposite of tabular data would be visual data , which would be some type of plot or chart that helps us visualize the values in a dataset.
For example, we might have the following bar chart that helps us visualize the total minutes played by each player in the dataset:

This would be an example of visual data .
It contains the exact same information about player names and minutes played for the players in the dataset, but it’s simply displayed in a visual form instead of a tabular form.
Or we might have the following scatterplot that helps us visualize the relationship between minutes played and points scored for each player:

This is another example of visual data .
When is Tabular Data Used in Practice?
In practice, tabular data is the most common type of data that you’ll run across in the real world.
In the real world, most data that is saved in an Excel spreadsheet is considered tabular data because the rows represent observations and the columns represent attributes for those observations.
For example, here’s what our basketball dataset from earlier might look like in an Excel spreadsheet:

This format is one of the most natural ways to collect and store values in a dataset, which is why it’s used so often.
Additional Resources
The following tutorials explain other common terms in statistics:
Why is Statistics Important? Why is Sample Size Important in Statistics? What is an Observation in Statistics? What is Considered Raw Data in Statistics?
Featured Posts

Hey there. My name is Zach Bobbitt. I have a Masters of Science degree in Applied Statistics and I’ve worked on machine learning algorithms for professional businesses in both healthcare and retail. I’m passionate about statistics, machine learning, and data visualization and I created Statology to be a resource for both students and teachers alike. My goal with this site is to help you learn statistics through using simple terms, plenty of real-world examples, and helpful illustrations.
Leave a Reply Cancel reply
Your email address will not be published. Required fields are marked *
Join the Statology Community
Sign up to receive Statology's exclusive study resource: 100 practice problems with step-by-step solutions. Plus, get our latest insights, tutorials, and data analysis tips straight to your inbox!
By subscribing you accept Statology's Privacy Policy.
- Textual And Tabular Presentation Of Data
Think about a scenario where your report cards are printed in a textual format. Your grades and remarks about you are presented in a paragraph format instead of data tables. Would be very confusing right? This is why data must be presented correctly and clearly. Let us take a look.
Suggested Videos
Presentation of data.
Presentation of data is of utter importance nowadays. Afterall everything that’s pleasing to our eyes never fails to grab our attention. Presentation of data refers to an exhibition or putting up data in an attractive and useful manner such that it can be easily interpreted. The three main forms of presentation of data are:
- Textual presentation
- Data tables
- Diagrammatic presentation
Here we will be studying only the textual and tabular presentation, i.e. data tables in some detail.
Textual Presentation
The discussion about the presentation of data starts off with it’s most raw and vague form which is the textual presentation. In such form of presentation, data is simply mentioned as mere text, that is generally in a paragraph. This is commonly used when the data is not very large.
This kind of representation is useful when we are looking to supplement qualitative statements with some data. For this purpose, the data should not be voluminously represented in tables or diagrams. It just has to be a statement that serves as a fitting evidence to our qualitative evidence and helps the reader to get an idea of the scale of a phenomenon .
For example, “the 2002 earthquake proved to be a mass murderer of humans . As many as 10,000 citizens have been reported dead”. The textual representation of data simply requires some intensive reading. This is because the quantitative statement just serves as an evidence of the qualitative statements and one has to go through the entire text before concluding anything.
Further, if the data under consideration is large then the text matter increases substantially. As a result, the reading process becomes more intensive, time-consuming and cumbersome.
Data Tables or Tabular Presentation
A table facilitates representation of even large amounts of data in an attractive, easy to read and organized manner. The data is organized in rows and columns. This is one of the most widely used forms of presentation of data since data tables are easy to construct and read.
Components of Data Tables
- Table Number : Each table should have a specific table number for ease of access and locating. This number can be readily mentioned anywhere which serves as a reference and leads us directly to the data mentioned in that particular table.
- Title: A table must contain a title that clearly tells the readers about the data it contains, time period of study, place of study and the nature of classification of data .
- Headnotes: A headnote further aids in the purpose of a title and displays more information about the table. Generally, headnotes present the units of data in brackets at the end of a table title.
- Stubs: These are titles of the rows in a table. Thus a stub display information about the data contained in a particular row.
- Caption: A caption is the title of a column in the data table. In fact, it is a counterpart if a stub and indicates the information contained in a column.
- Body or field: The body of a table is the content of a table in its entirety. Each item in a body is known as a ‘cell’.
- Footnotes: Footnotes are rarely used. In effect, they supplement the title of a table if required.
- Source: When using data obtained from a secondary source, this source has to be mentioned below the footnote.
Construction of Data Tables
There are many ways for construction of a good table. However, some basic ideas are:
- The title should be in accordance with the objective of study: The title of a table should provide a quick insight into the table.
- Comparison: If there might arise a need to compare any two rows or columns then these might be kept close to each other.
- Alternative location of stubs: If the rows in a data table are lengthy, then the stubs can be placed on the right-hand side of the table.
- Headings: Headings should be written in a singular form. For example, ‘good’ must be used instead of ‘goods’.
- Footnote: A footnote should be given only if needed.
- Size of columns: Size of columns must be uniform and symmetrical.
- Use of abbreviations: Headings and sub-headings should be free of abbreviations.
- Units: There should be a clear specification of units above the columns.
The Advantages of Tabular Presentation
- Ease of representation: A large amount of data can be easily confined in a data table. Evidently, it is the simplest form of data presentation.
- Ease of analysis: Data tables are frequently used for statistical analysis like calculation of central tendency, dispersion etc.
- Helps in comparison: In a data table, the rows and columns which are required to be compared can be placed next to each other. To point out, this facilitates comparison as it becomes easy to compare each value.
- Economical: Construction of a data table is fairly easy and presents the data in a manner which is really easy on the eyes of a reader. Moreover, it saves time as well as space.
Classification of Data and Tabular Presentation
Qualitative classification.
In this classification, data in a table is classified on the basis of qualitative attributes. In other words, if the data contained attributes that cannot be quantified like rural-urban, boys-girls etc. it can be identified as a qualitative classification of data.
Quantitative Classification
In quantitative classification, data is classified on basis of quantitative attributes.
Temporal Classification
Here data is classified according to time. Thus when data is mentioned with respect to different time frames, we term such a classification as temporal.
Spatial Classification
When data is classified according to a location, it becomes a spatial classification.
A Solved Example for You
Q: The classification in which data in a table is classified according to time is known as:
- Qualitative
- Quantitative
Ans: The form of classification in which data is classified based on time frames is known as the temporal classification of data and tabular presentation.
Customize your course in 30 seconds
Which class are you in.

- Diagrammatic Presentation of Data
Leave a Reply Cancel reply
Your email address will not be published. Required fields are marked *
Download the App


An official website of the United States government
The .gov means it’s official. Federal government websites often end in .gov or .mil. Before sharing sensitive information, make sure you’re on a federal government site.
The site is secure. The https:// ensures that you are connecting to the official website and that any information you provide is encrypted and transmitted securely.
- Publications
- Account settings
Preview improvements coming to the PMC website in October 2024. Learn More or Try it out now .
- Advanced Search
- Journal List
- An Bras Dermatol
- v.89(2); Mar-Apr 2014
Presenting data in tables and charts *
Rodrigo pereira duquia.
1 Universidade Federal de Ciências da Saúde de Porto Alegre (UFCSPA) - Porto Alegre (RS), Brazil.
João Luiz Bastos
2 Universidade Federal de Santa Catarina (UFSC) - Florianópolis (SC) Brazil.
Renan Rangel Bonamigo
David alejandro gonzález-chica, jeovany martínez-mesa.
3 Latin American Cooperative Oncology Group (LACOG) - Porto Alegre (RS) Brazil.
The present paper aims to provide basic guidelines to present epidemiological data using tables and graphs in Dermatology. Although simple, the preparation of tables and graphs should follow basic recommendations, which make it much easier to understand the data under analysis and to promote accurate communication in science. Additionally, this paper deals with other basic concepts in epidemiology, such as variable, observation, and data, which are useful both in the exchange of information between researchers and in the planning and conception of a research project.
INTRODUCTION
Among the essential stages of epidemiological research, one of the most important is the identification of data with which the researcher is working, as well as a clear and synthetic description of these data using graphs and tables. The identification of the type of data has an impact on the different stages of the research process, encompassing the research planning and the production/publication of its results. For example, the use of a certain type of data impacts the amount of time it will take to collect the desired information (throughout the field work) and the selection of the most appropriate statistical tests for data analysis.
On the other hand, the preparation of tables and graphs is a crucial tool in the analysis and production/publication of results, given that it organizes the collected information in a clear and summarized fashion. The correct preparation of tables allows researchers to present information about tens or hundreds of individuals efficiently and with significant visual appeal, making the results more easily understandable and thus more attractive to the users of the produced information. Therefore, it is very important for the authors of scientific articles to master the preparation of tables and graphs, which requires previous knowledge of data characteristics and the ability of identifying which type of table or graph is the most appropriate for the situation of interest.
BASIC CONCEPTS
Before evaluating the different types of data that permeate an epidemiological study, it is worth discussing about some key concepts (herein named data, variables and observations):
Data - during field work, researchers collect information by means of questions, systematic observations, and imaging or laboratory tests. All this gathered information represents the data of the research. For example, it is possible to determine the color of an individual's skin according to Fitzpatrick classification or quantify the number of times a person uses sunscreen during summer. 1 , 2 All the information collected during research is generically named "data." A set of individual data makes it possible to perform statistical analysis. If the quality of data is good, i.e., if the way information was gathered was appropriate, the next stages of database preparation, which will set the ground for analysis and presentation of results, will be properly conducted.
Observations - are measurements carried out in one or more individuals, based on one or more variables. For instance, if one is working with the variable "sex" in a sample of 20 individuals and knows the exact amount of men and women in this sample (10 for each group), it can be said that this variable has 20 observations.
Variables - are constituted by data. For instance, an individual may be male or female. In this case, there are 10 observations for each sex, but "sex" is the variable that is referred to as a whole. Another example of variable is "age" in complete years, in which observations are the values 1 year, 2 years, 3 years, and so forth. In other words, variables are characteristics or attributes that can be measured, assuming different values, such as sex, skin type, eye color, age of the individuals under study, laboratory results, or the presence of a given lesion/disease. Variables are specifically divided into two large groups: (a) the group of categorical or qualitative variables, which is subdivided into dichotomous, nominal and ordinal variables; and (b) the group of numerical or quantitative variables, which is subdivided into continuous and discrete variables.
Categorical variables
- Dichotomous variables, also known as binary variables: are those that have only two categories, i.e., only two response options. Typical examples of this type of variable are sex (male and female) and presence of skin cancer (yes or no).
- Ordinal variables: are those that have three or more categories with an obvious ordering of the categories (whether in an ascending or descending order). For example, Fitzpatrick skin classification into types I, II, III, IV and V. 1
- Nominal variables: are those that have three or more categories with no apparent ordering of the categories. Example: blood types A, B, AB, and O, or brown, blue or green eye colors.
Numerical variables
- Discrete variables: are observations that can only take certain numerical values. An example of this type of variable is subjects' age, when assessed in complete years of life (1 year, 2 years, 3 years, 4 years, etc.) and the number of times a set of patients visited the dermatologist in a year.
- Continuous variables: are those measured on a continuous scale, i.e., which have as many decimal places as the measuring instrument can record. For instance: blood pressure, birth weight, height, or even age, when measured on a continuous scale.
It is important to point out that, depending on the objectives of the study, data may be collected as discrete or continuous variables and be subsequently transformed into categorical variables to suit the purpose of the research and/or make interpretation easier. However, it is important to emphasize that variables measured on a numerical scale (whether discrete or continuous) are richer in information and should be preferred for statistical analyses. Figure 1 shows a diagram that makes it easier to understand, identify and classify the abovementioned variables.

Types of variables
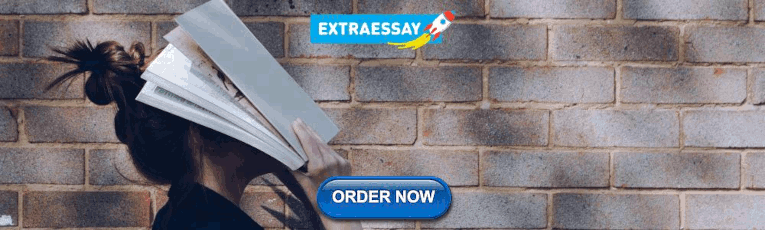
DATA PRESENTATION IN TABLES AND GRAPHS
Firstly, it is worth emphasizing that every table or graph should be self-explanatory, i.e., should be understandable without the need to read the text that refers to it refers.
Presentation of categorical variables
In order to analyze the distribution of a variable, data should be organized according to the occurrence of different results in each category. As for categorical variables, frequency distributions may be presented in a table or a graph, including bar charts and pie or sector charts. The term frequency distribution has a specific meaning, referring to the the way observations of a given variable behave in terms of its absolute, relative or cumulative frequencies.
In order to synthesize information contained in a categorical variable using a table, it is important to count the number of observations in each category of the variable, thus obtaining its absolute frequencies. However, in addition to absolute frequencies, it is worth presenting its percentage values, also known as relative frequencies. For example, table 1 expresses, in absolute and relative terms, the frequency of acne scars in 18-year-old youngsters from a population-based study conducted in the city of Pelotas, Southern Brazil, in 2010. 3
Absolute and relative frequencies of acne scar in 18- year-old adolescents (n = 2.414). Pelotas, Brazil, 2010
The same information from table 1 may be presented as a bar or a pie chart, which can be prepared considering the absolute or relative frequency of the categories. Figures 2 and and3 3 illustrate the same information shown in table 1 , but present it as a bar chart and a pie chart, respectively. It can be observed that, regardless of the form of presentation, the total number of observations must be mentioned, whether in the title or as part of the table or figure. Additionally, appropriate legends should always be included, allowing for the proper identification of each of the categories of the variable and including the type of information provided (absolute and/or relative frequency).

Absolute frequencies of acne scar in 18-year-old adolescents (n = 2.414). Pelotas, Brazil, 2010

Relative frequencies of acne scar in 18-year-old adolescents (n = 2.414). Pelotas, Brazil, 2010
Presentation of numerical variables
Frequency distributions of numerical variables can be displayed in a table, a histogram chart, or a frequency polygon chart. With regard to discrete variables, it is possible to present the number of observations according to the different values found in the study, as illustrated in table 2 . This type of table may provide a wide range of information on the collected data.
Educational level of 18-year-old adolescents (n = 2,199). Pelotas, Brazil, 2010
Table 2 shows the distribution of educational levels among 18-year-old youngsters from Pelotas, Southern Brazil, with absolute, relative, and cumulative relative frequencies. In this case, absolute and relative frequencies correspond to the absolute number and the percentage of individuals according to their distribution for this variable, respectively, based on complete years of education. It should be noticed that there are 450 adolescents with 8 years of education, which corresponds to 20.5% of the subjects. Tables may also present the cumulative relative frequency of the variable. In this case, it was found that 50.6% of study subjects have up to 8 years of education. It is important to point that, although the same data were used, each form of presentation (absolute, relative or cumulative frequency) provides different information and may be used to understand frequency distribution from different perspectives.
When one wants to evaluate the frequency distribution of continuous variables using tables or graphs, it is necessary to transform the variable into categories, preferably creating categories with the same size (or the same amplitude). However, in addition to this general recommendation, other basic guidelines should be followed, such as: (1) subtracting the highest from the lowest value for the variable of interest; (2) dividing the result of this subtraction by the number of categories to be created (usually from three to ten); and (3) defining category intervals based on this last result.
For example, in order to categorize height (in meters) of a set of individuals, the first step is to identify the tallest and the shortest individual of the sample. Let us assume that the tallest individual is 1.85m tall and the shortest, 1.55m tall, with a difference of 0.3m between these values. The next step is to divide this difference by the number of categories to be created, e.g., five. Thus, 0.3m divided by five equals 0.06m, which means that categories will have exactly this range and will be numerically represented by the following range of values: 1st category - 1.55m to 1.60m; 2nd category - 1.61m to 1.66m; 3rd category - 1.67m to 1.72m; 4th category - 1.73m to 1.78m; 5th category - 1.79m to 1.85m.
Table 3 illustrates weight values at 18 years of age in kg (continuous numerical variable) obtained in a study with youngsters from Pelotas, Southern Brazil. 4 , 5 Figure 4 shows a histogram with the variable weight categorized into 20-kg intervals. Therefore, it is possible to observe that data from continuous numerical variables may be presented in tables or graphs.
Weight distribution among 18-year-old young male sex (n = 2.194). Pelotas, Brazil, 2010

Weight distribution at 18 years of age among youngsters from the city of Pelotas. Pelotas (n = 2.194), Brazil, 2010
Assessing the relationship between two variables
The forms of data presentation that have been described up to this point illustrated the distribution of a given variable, whether categorical or numerical. In addition, it is possible to present the relationship between two variables of interest, either categorical or numerical.
The relationship between categorical variables may be investigated using a contingency table, which has the purpose of analyzing the association between two or more variables. The lines of this type of table usually display the exposure variable (independent variable), and the columns, the outcome variable (dependent variable). For example, in order to study the effect of sun exposure (exposure variable) on the development of skin cancer (outcome variable), it is possible to place the variable sun exposure on the lines and the variable skin cancer on the columns of a contingency table. Tables may be easier to understand by including total values in lines and columns. These values should agree with the sum of the lines and/or columns, as appropriate, whereas relative values should be in accordance with the exposure variable, i.e., the sum of the values mentioned in the lines should total 100%.
It is such a display of percentage values that will make it possible for risk or exposure groups to be compared with each other, in order to investigate whether individuals exposed to a given risk factor show higher frequency of the disease of interest. Thus, table 4 shows that 75.0%, 9.0%, and 0.3% of individuals in the study sample who had been working exposed to the sun for 20 years or more, for less than 20 years, and had never been working exposed to the sun, respectively, developed non-melanoma skin cancer. Another way of interpreting this table is observing that 25.0%, 91%,.0%, and 99.7% of individuals who had been working exposed to the sun for 20 years of more, for less than 20 years, and had never been working exposed to the sun did not develop non-melanoma skin cancer. This form of presentation is one of the most used in the literature and makes the table easier to read.
Sun exposure during work and non-melanoma skin cancer (hypothetical data).
The relationship between two numerical variables or between one numerical variable and one categorical variable may be assessed using a scatter diagram, also known as dispersion diagram. In this diagram, each pair of values is represented by a symbol or a dot, whose horizontal and vertical positions are determined by the value of the first and second variables, respectively. By convention, vertical and horizontal axes should correspond to outcome and exposure variables, respectively. Figure 5 shows the relationship between weight and height among 18-year-old youngsters from Pelotas, Southern Brazil, in 2010. 3 , 4 The diagram presented in figure 5 should be interpreted as follows: the increase in subjects' height is accompanied by an increase in their weight.

Point diagram for the relationship between weight (kg) and height (cm) among 18-year-old youngsters from the city of Pelotas (n = 2.194). Pelotas, Brazil, 2010.
BASIC RULES FOR THE PREPARATION OF TABLES AND GRAPHS
Ideally, every table should:
- Be self-explanatory;
- Present values with the same number of decimal places in all its cells (standardization);
- Include a title informing what is being described and where, as well as the number of observations (N) and when data were collected;
- Have a structure formed by three horizontal lines, defining table heading and the end of the table at its lower border;
- Not have vertical lines at its lateral borders;
- Provide additional information in table footer, when needed;
- Be inserted into a document only after being mentioned in the text; and
- Be numbered by Arabic numerals.
Similarly to tables, graphs should:
- Include, below the figure, a title providing all relevant information;
- Be referred to as figures in the text;
- Identify figure axes by the variables under analysis;
- Quote the source which provided the data, if required;
- Demonstrate the scale being used; and
- Be self-explanatory.
The graph's vertical axis should always start with zero. A usual type of distortion is starting this axis with values higher than zero. Whenever it happens, differences between variables are overestimated, as can been seen in figure 6 .

Figure showing how graphs in which the Y-axis does not start with zero tend to overestimate the differences under analysis. On the left there is a graph whose Y axis does not start with zero and on the right a graph reproducing the same data but with the Y axis starting with zero.
Understanding how to classify the different types of variables and how to present them in tables or graphs is an essential stage for epidemiological research in all areas of knowledge, including Dermatology. Mastering this topic collaborates to synthesize research results and prevents the misuse or overuse of tables and figures in scientific papers.
Conflict of Interest: None
Financial Support: None
How to cite this article: Duquia RP, Bastos JL, Bonamigo RR, González-Chica DA, Martínez-Mesa J. Presenting data in tables and charts. An Bras Dermatol. 2014;89(2):280-5.
* Work performed at the Dermatology service, Universidade Federal de Ciências da Saúde de Porto Alegre (UFCSPA), Departamento de Saúde Pública e Departamento de Nutrição da UFSC.
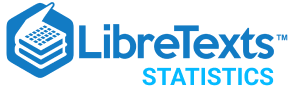
- school Campus Bookshelves
- menu_book Bookshelves
- perm_media Learning Objects
- login Login
- how_to_reg Request Instructor Account
- hub Instructor Commons
Margin Size
- Download Page (PDF)
- Download Full Book (PDF)
- Periodic Table
- Physics Constants
- Scientific Calculator
- Reference & Cite
- Tools expand_more
- Readability
selected template will load here
This action is not available.
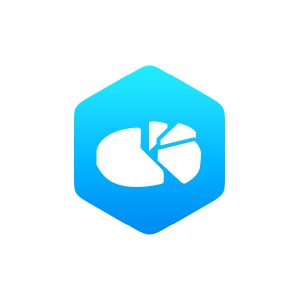
2.2: Tabular Displays
- Last updated
- Save as PDF
- Page ID 24024
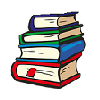
- Rachel Webb
- Portland State University
\( \newcommand{\vecs}[1]{\overset { \scriptstyle \rightharpoonup} {\mathbf{#1}} } \)
\( \newcommand{\vecd}[1]{\overset{-\!-\!\rightharpoonup}{\vphantom{a}\smash {#1}}} \)
\( \newcommand{\id}{\mathrm{id}}\) \( \newcommand{\Span}{\mathrm{span}}\)
( \newcommand{\kernel}{\mathrm{null}\,}\) \( \newcommand{\range}{\mathrm{range}\,}\)
\( \newcommand{\RealPart}{\mathrm{Re}}\) \( \newcommand{\ImaginaryPart}{\mathrm{Im}}\)
\( \newcommand{\Argument}{\mathrm{Arg}}\) \( \newcommand{\norm}[1]{\| #1 \|}\)
\( \newcommand{\inner}[2]{\langle #1, #2 \rangle}\)
\( \newcommand{\Span}{\mathrm{span}}\)
\( \newcommand{\id}{\mathrm{id}}\)
\( \newcommand{\kernel}{\mathrm{null}\,}\)
\( \newcommand{\range}{\mathrm{range}\,}\)
\( \newcommand{\RealPart}{\mathrm{Re}}\)
\( \newcommand{\ImaginaryPart}{\mathrm{Im}}\)
\( \newcommand{\Argument}{\mathrm{Arg}}\)
\( \newcommand{\norm}[1]{\| #1 \|}\)
\( \newcommand{\Span}{\mathrm{span}}\) \( \newcommand{\AA}{\unicode[.8,0]{x212B}}\)
\( \newcommand{\vectorA}[1]{\vec{#1}} % arrow\)
\( \newcommand{\vectorAt}[1]{\vec{\text{#1}}} % arrow\)
\( \newcommand{\vectorB}[1]{\overset { \scriptstyle \rightharpoonup} {\mathbf{#1}} } \)
\( \newcommand{\vectorC}[1]{\textbf{#1}} \)
\( \newcommand{\vectorD}[1]{\overrightarrow{#1}} \)
\( \newcommand{\vectorDt}[1]{\overrightarrow{\text{#1}}} \)
\( \newcommand{\vectE}[1]{\overset{-\!-\!\rightharpoonup}{\vphantom{a}\smash{\mathbf {#1}}}} \)
Frequency Tables for Quantitative Data
To create many of these graphs, you must first create the frequency distribution. The idea of a frequency distribution is to take the interval that the data spans and divide it into equal sized subintervals called classes. The grouped frequency distribution gives either the frequency (count) or the relative frequency (usually expressed as a percent) of individuals who fall into each class. When creating frequency distributions, it is important to note that the number of classes that are used and the value of the first class boundary will change the shape of, and hence the impression given by, the distribution. There is no one correct width to the class boundaries. It usually takes several tries to create a frequency distribution that looks just the way you want. As a reader and interpreter of such tables, you should be aware that such features are selected so that the table looks the way it does to show a particular point of view. For small samples, we usually have between four and seven classes, and as you get more data you will need more classes.
We will start with an example of a random sample of 35 ages from credit card applications given below in no particular order. Organize the data in a frequency distribution table.
Note that the class limits should never overlap; we call these mutually exclusive classes. For example, if your class went from 20-25, 25-30 etc., the 25-year-olds would fall within both classes. Also, make sure each of the classes have the same width. A more formal way to pick your classes uses the following process.
Steps involved in making a frequency distribution table:
- Find the range = largest value – smallest value.
- Pick the number of classes to use. Usually the number of classes is between five and twenty. Five classes are used if there are a small number of data points and twenty classes if there are a large number of data points (over 1,000 data points).
- Class width = \(\frac{\text { range }}{\text { # of classes }}\). Always round up to the next integer (if the answer is already a whole number, go to the next integer). If you do not round up, your last class will not contain your largest data value, and you would have to add another class just for it. If you round up, then your largest data value will fall in the last class, and there are no issues.
- Create the classes. Each class has limits that determine which values fall in each class. To find the class limits , set the smallest value in the data set as the lower class limit for the first class. Then add the class width to the lower class limit to get the next lower class limit. Repeat until you get all the classes. The upper class limit for a class is one less than the lower limit for the next class.
- If your data value has decimal places, then round up the class width to the nearest value with the same number of decimal places as the original data. As an example, if your data was out to two decimal places, and you divided your range by the number of classes to get 4.8333, then the class width would round the second decimal place up and end on 4.84.
- The frequency for a class is the number of data values that fall in the class.
For the age data let us use 6 classes. Find the range by taking 49 – 20 = 29 and divide this by the number of classes 29/6 = 4.8333. Round this number up to 5 and use 5 for the class width. Once you determine your class width and class limits, place each of these classes in a table and then tally up the ages that fall within each class. Count the tally marks and record the number in the frequency table.
The total of the frequency column should be the number of observations in the data. You may want to total the frequencies to make sure you did not leave any of the numbers out of the table.
Using the frequency table, we can now see that there are more people in the 25- 29 year-old class, followed by the 45-49 year-old class.
We call this most frequent category the modal class . There may be no mode at all or more than one mode.
Frequency Tables for Qualitative Data
A frequency distribution can also be made for qualitative data.
Suppose you have the following data for which type of car students at a college drive. Make a frequency table to summarize the data.
For nominal data, either alphabetize the classes or arrange the classes from most frequent to least frequent, with the “other” category always at the end. For ordinal data put the classes in their order with the “other” category at the end.
Relative Frequency Tables
Frequencies by themselves are not as useful to tell other people what is going on in the data. If you want to know what percentage the category is of the total sample then we can use the relative frequency of each category. The relative frequency is just the frequency divided by the total. The relative frequency is the proportion in each category and may be given as a decimal, percentage, or fraction.
Using the car data’s frequency distribution, we will create a third column labeled relative frequency. Take each frequency and divide by the sample size, see Figure 2-2. The relative frequencies should add up to one (ignoring rounding error).
Many people understand percentages better than proportions so we may want to multiply each of these decimals by 100% to get the following relative frequency percent table.
We can summarize the car data and see that for college students Chevy and Toyota make up 48% of the car models.
Recall the frequency table for the credit card applicants. The relative frequency table for the random sample of 35 ages from credit card applications follows.
The relative frequency table lets us quickly see that a little more than half 34% + 20% = 54% of the ages of the credit card holders are between the ages of 25 – 29 and 45 – 49 years-old.
Cumulative & Cumulative Relative Frequency Tables
Another useful piece of information is how many data points fall below a particular class. As an example, a teacher may want to know how many students received below a 70%, a doctor may want to know how many adults have cholesterol above 160, or a manager may want to know how many stores gross less than $2,000 per day. This calculation is known as a cumulative frequency and is used for ordinal or quantitative data. If you want to know what percent of the data falls below a certain class, then this fact would be a cumulative relative frequency .
To create a cumulative frequency distribution , count the number of data points that are below the upper class limit, starting with the first class and working up to the top class. The last upper class should have all of the data points below it.
Recall the credit card applicants. Make a cumulative frequency table.
Use Excel to add up the values for you.
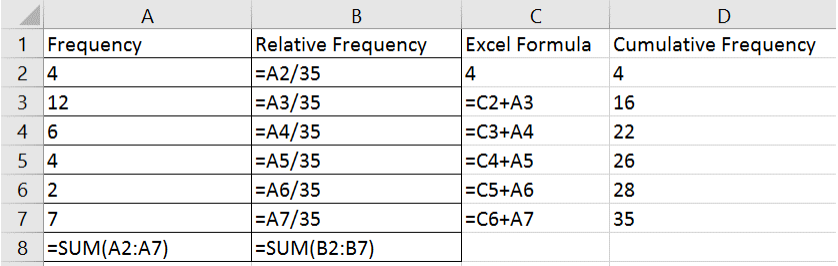
You can also express the cumulative relative frequencies as a percentage instead of a proportion.
If a manager wanted to know how many applicants were under the age of 40 we could look across the 35-39 year-old class to see that there were 26 applicants that were under 40 (39 years old or younger), or if we used the cumulative relative frequency, 74% of the applicants were under 40 years old. If the manager wants to know how many applicants were over 44, we could subtract 100% – 80% = 20%.
Contingency Tables
A contingency table provides a way of portraying data that can facilitate calculating probabilities. A contingency table summarizes the frequency of two qualitative variables. The table displays sample values in relation to two different variables that may be dependent or contingent on one another. There are other names for contingency tables. Excel calls the contingency table a pivot table. Other common names are two-way table, cross-tabulation, or cross-tab for short.
A fitness center coach kept track of members over the last year. They recorded if the person stretched before they exercised, and whether they sustained an injury. The following contingency table shows their results. Find the relative frequency for each value in the table.
We can quickly summarize the number of athletes for each category. The bottom right-hand number in Figure 2-4 is the grand total and represents the total number of 400 people. There were 322 people that stretched before exercising. There were 73 people that sustained an injury while exercising, etc.
If we find the relative frequency for each value in the table, we can find the proportion of the 400 people for each category. To find a relative frequency we divide each value in the table by the grand total. See Figure 2-5.
When data is collected, it is usually presented in a spreadsheet where each row represents the responses from an individual or case.
“Of course, one never has the slightest notion what size or shape different species are going to turn out to be, but if you were to take the findings of the latest Mid‐Galactic Census report as any kind of accurate Guide to statistical averages you would probably guess that the craft would hold about six people, and you would be right. You'd probably guessed that anyway. The Census report, like most such surveys, had cost an awful lot of money and didn't tell anybody anything they didn't already know - except that every single person in the Galaxy had 2.4 legs and owned a hyena. Since this was clearly not true the whole thing had eventually to be scrapped.” (Adams, 2002)
Make a pivot table using Excel. A random sample of 500 records from the 2010 United States Census were downloaded to Excel. Below is an image of just the first 20 people.
There are seven variables:
- Total family income (in United States dollars)
- Biological Sex (with reported categories female and male)
- Race (with reported categories American Indian or Alaska Native, Black, Chinese, Japanese, Other Asian or Pacific Islander, Two major races, White, Other)
- Marital status (with reported categories Divorced, Married/spouse absent, Married/spouse present, Never married/single, Separated, Widowed)
- Total personal income (in United States dollars).

In Excel, select the Insert tab, then select Pivot Table.
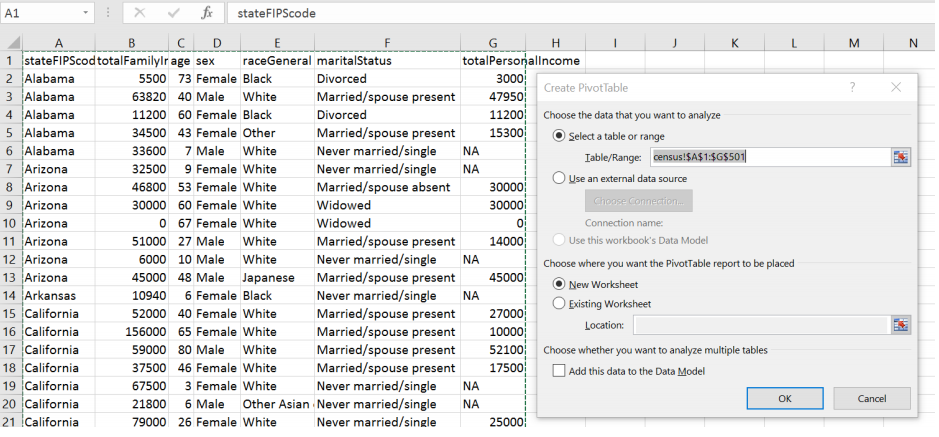
Excel should automatically select all 500 rows in the Table/Range cell, if not then use your mouse and highlight all the data including the labels. Then select OK.
Each version of Excel may look different at this point. One common area, though, is the bottom right-hand drag and drop area of the Pivot Table dialogue box.
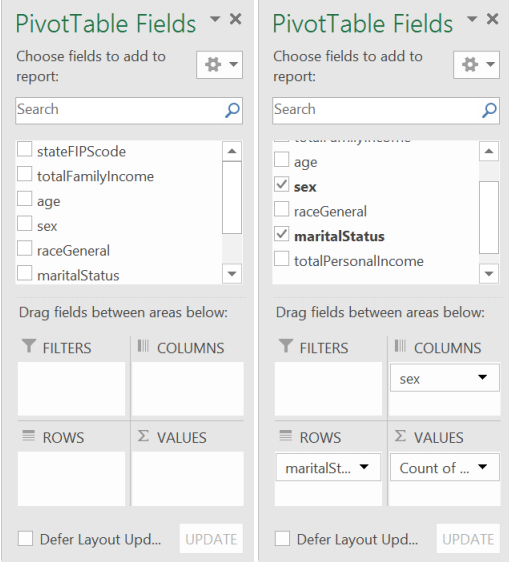
Drag the sex variable to the COLUMNS box, and marital status variable to the ROWS box.
You will see the contingency table column and row headers appear as you drop the variables in the boxes.
To get the counts to appear, drag and drop marital status into the Values box and the default will usually say, “Count of maritalStatus,” if not then change it to count in the drop-down menu. A contingency table should appear on your spreadsheet as you fill in the pivot table dialogue box. Contingency tables go by many names including pivot tables, two-way tables, cross tabulations, or cross-tabs.
Talk to our experts
1800-120-456-456
- Tabular Presentation of Data
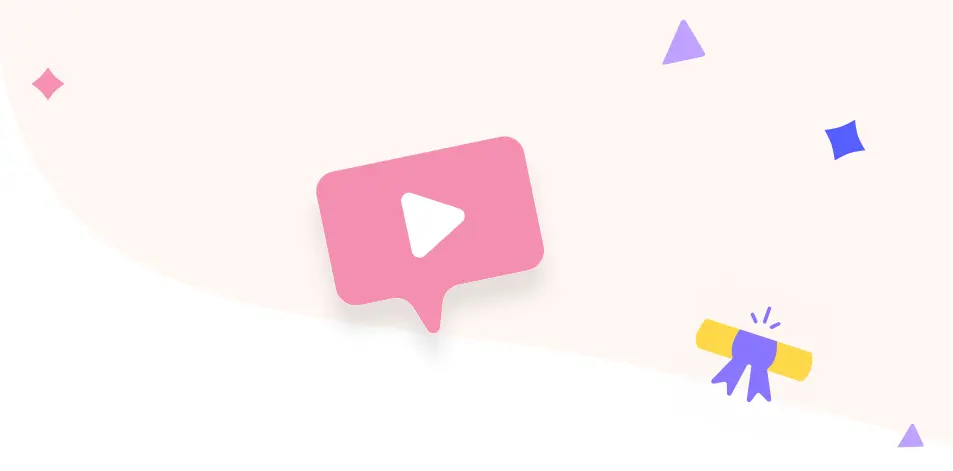
What is Tabular Presentation of Data in Detail
The presentation of data is essential. A tabular presentation of data helps the viewer to understand and to interpret the information better. Take, for example, your annual report card that is presented in a tabular format. You have your subjects written in one column of the table and your grades on the other. The third column mentions any teachers’ remarks. A single glance at your report card lets you read through the grades and subjects as well as the remarks with ease.
Now think, what would have happened if the same information was presented to you in the form of a paragraph. You would have to go through each line to know the grade that you got and the teachers’ remarks on a particular subject. This would make it tedious and also confusing to understand the report card.
Presentation of Data
Data must be presented properly. If the information is pleasing to the eyes, then it immediately gets attention. Data presentation is about using the same information to exhibit it in an attractive and useful way that can be read and interpreted easily. Data presentation is of three broad kinds. These are:
Textual presentation.
Data tables.
Diagrammatic presentation.
On this presentation of data Class 11 page, you will get to understand the textual and tabular data presentation or the data tables.
Textual Presentation
Data is first obtained in a textual format. It is a vague and raw format of the data. The data is mentioned in the text form, which is usually written in a paragraph. The textual presentation of data is used when the data is not large and can be easily comprehended by the reader just when he reads the paragraph.
This data format is useful when some qualitative statement is to be supplemented with data. The reader does not want to read volumes of data to be represented in the tabular format. Does he want to understand the data in a diagrammatic form? All that the reader wants to know is the data that provides evidence to the statement written. This is enough to let the reader gauge the intensity of the statement.
The textual data is evidence of the qualitative statement, and one needs to go through the complete text before he concludes anything.
For example, the coronavirus death toll in India today is 447. The reader does not need a lot of data here. The entire text of the state-wise breakup is accumulated to arrive at the national death figure. This is enough information for the reader.
Data Tables or Tabular Presentation
Data Tables or Tabular presentation of data is known to be the arrangement of certain values recorded in tables such that they are easy to manage and read. It is mostly done for a reader to gain the idea about the data without making it too complicated. The data presentation can be used for proper matter which is informative and creative at the same time.
What is Data Presentation?
If the reader has to interpret a lot of data, then this has to be organized in an easy to read format. The data should be laid out in rows and columns so that the reader can get what he wants at a single glance. Data tables are easy to construct and also easy to read, which makes them popular.
Components of Data Tables
Below are the key components of the data table.
Table Number - Each table has a table number that makes it easy to locate it. This number serves as a reference and leads one to a particular table.
Title - The table should also have a title that lets the reader understand what information the table provides. The place of study, the period, and the nature of data classification are also mentioned in the title.
Headnotes - The headnotes give further information. It provides the unit of data in brackets which is mentioned at the end of the title. The headnote aids the title to offer more information that the reader would need to interpret the data.
Stubs - These are the titles that tell you what the row represents. In other words, the stubs give information about what data is contained in each row.
Caption - The caption is the column title in the data table. It gives information about what is contained in each column.
Body or Field - The body or the field is the entire content in the table. Each item that is present in the body is the cell.
Footnotes - Footnotes are not commonly used, but these are used to supplement the table title if needed.
Source - If the data used in the table is taken from a secondary source, then that has to be mentioned in the footnote.
Construction of Data Tables
Tabular presentation can be constructed in many ways. Here are some ways that are commonly followed.
The title of the table should be able to reflect on the table content.
If two rows or columns have to be compared, then these should be placed adjacent to each other.
If the rows in the table are lengthy, then the stub can be placed on the right-hand part of the table.
Headings should always be in the singular.
Footnotes are not compulsory and should be provided only if required.
The column size should be symmetrical and uniform.
There should be no abbreviations in the headings and the subheadings.
The units should be specified above the column.
The Advantages of Tabular Presentation
Makes representation of data easy.
Makes it easy to analyze the data.
Makes it easy to compare data.
The data is represented in a readable manner which saves space and the reader’s time.
Classification of Data and Tabular Presentation
Classification of data and Tabular presentation is needed to arrange complex, heterogeneous data into a more simple and sophisticated manner. This is done for the convenience of the audience studying the data so the values are easy to distinguish. There are four ways in which one can classify the data and Tabular presentation. These are as follows.
Qualitative Classification
In qualitative classification, the data is classified based on its qualitative attributes. This is when the data has attributes that cannot be quantified. These could be boys-girls, rural-urban, etc.
Quantitative Classification
In quantitative classification, the data is classified based on the quantitative attributes. These could be marks where the data is categorized into 0-50, 51-100, etc.
Temporal Classification
In this tabular presentation, the data is classified according to the time. Here the data is represented in varied time frames like in the year 2016, 2018, etc.
Spatial Classification
In this method of classification, the data is classified according to location, like India, Pakistan, Russia, etc.
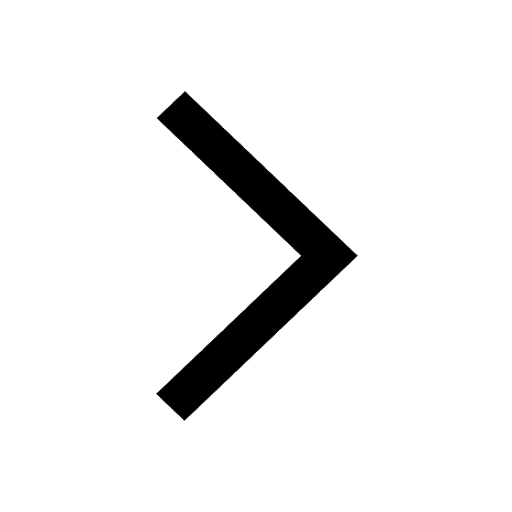
FAQs on Tabular Presentation of Data
1. What do you Mean by the Tabular Presentation of Data?
When data is presented in a tabular form, it makes the information easy to read and to engage. The data is arranged in rows and columns. The tabular method of presenting data is the most widely used. The tabular representation of data coordinates the information for decision making, and any presentation of data in statistics use. Data in the tabular format is divided into 4 kinds. These are the Qualitative (based on traits), Quantitative (based on quantitative features), Temporal (based on time), and spatial (based on location) presentation of data.
2. Explain the Difference Between the Tabular and Textual Presentation of Data ?
In the tabular representation of data, the data is presented in the form of tables and diagrams. The textual presentation uses words to present the data.Tabular data is self-explanatory as there are segments that depict what the data wants to convey. The textual data need to be explained with words.The key difference thus is that the textual representation of data is subjective. In a tabular format, the data is mentioned in the form of tables. This makes tabular data perfect for the vast amount of data which makes it easy for the reader to read and interpret the information.
3. Where can I get the most appropriate Textual and Tabular Presentation of Data - Advantages, Classification and FAQs?
At Vedantu, the students can find different types of study material which help them ace their exams. Whether it is sample tests, mock tests, important questions, notes you want, Vedantu has it all. All of these are curated by our master teachers who make sure that you score the highest of marks. For finding the Textual and Tabular Presentation of data - Advantages, Classification and FAQs, all students have to do is sign in Vedantu.com using the Vedantu app or website.
4. What is meant by textual and Tabular Presentation?
Data around us is represented in different ways to us on an everyday basis. Two of these methods are either presenting it via texts which are known as textual presentation and the other one is known as Tabular Presentation by which the data is presented using tables. The tabular presentation is attractive and helps one to visualize the given data, although some may consider textual presentation for a detailed and proper explanation. It depends entirely on the individual how they want their data to be produced, however, most people consider the tabular presentation.
5. Why should I know about textual and Tabular Presentation?
We need data to share information with others, for this, it is important for the students to know how to use the different ways of data presentation. Knowing about Textual and Tabular presentation of data helps an individual to choose how they need their information to be conveyed. Textual data representation is basic and it is important that a student already knows about it completely when they move on to studying the tabular presentation of data. This makes sure that you have your concepts clear and for your progress to attain great heights.
Refer to Vedantu for free solutions chapter wise and get free access to other online resources to improve your learning in several folds.

A .gov website belongs to an official government organization in the United States.
A lock ( ) or https:// means you've safely connected to the .gov website. Share sensitive information only on official, secure websites.
- Causes and Spread
- Data and Maps for West Nile
- Illness Info
- Clinical Signs and Symptoms
- Clinical Testing and Diagnosis
- Treatment and Prevention
- West Nile Virus Surveillance and Control
- Transmission
- West Nile Virus Resources
- Vector-Borne Diseases
West Nile Virus
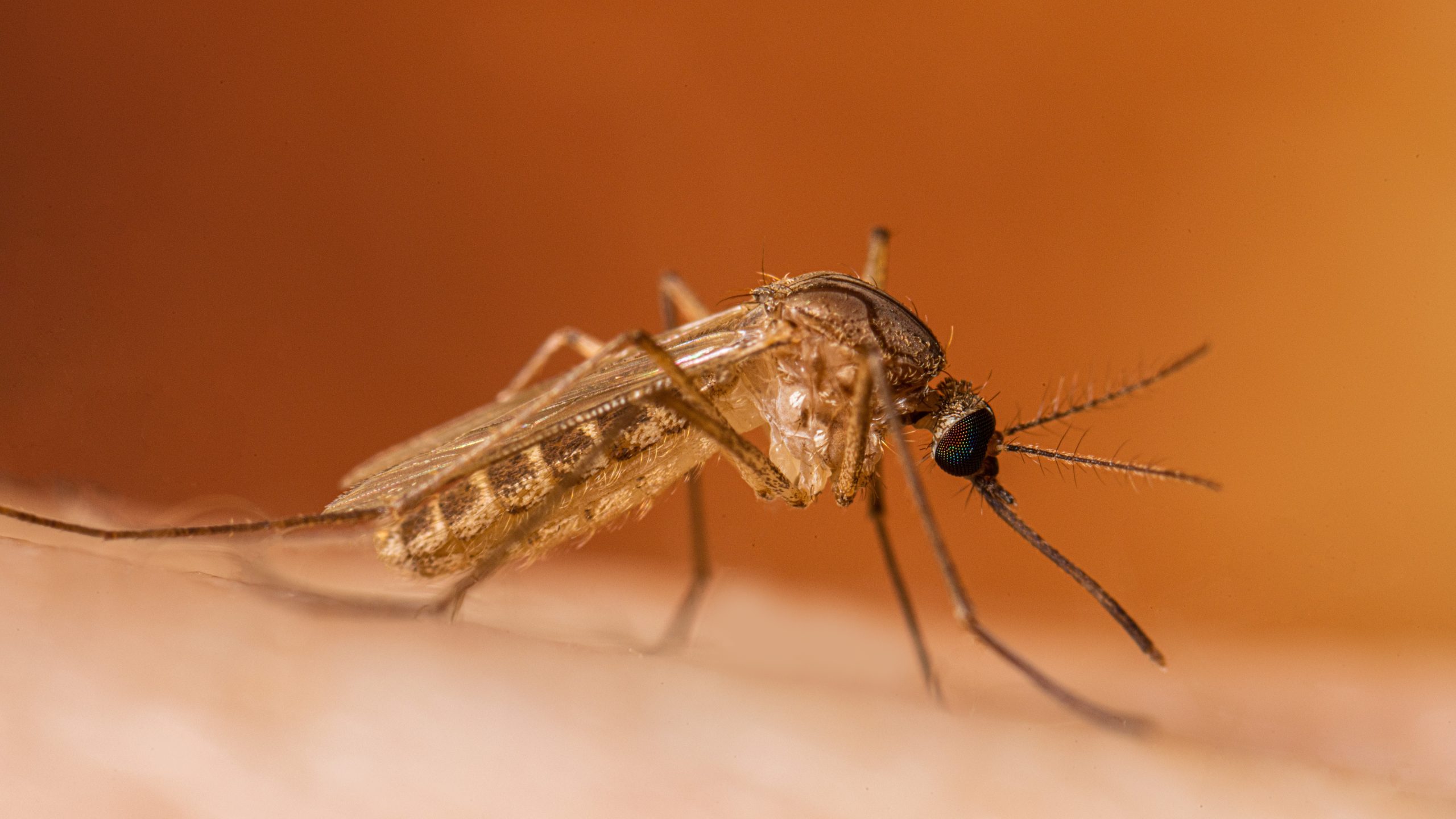
About West Nile
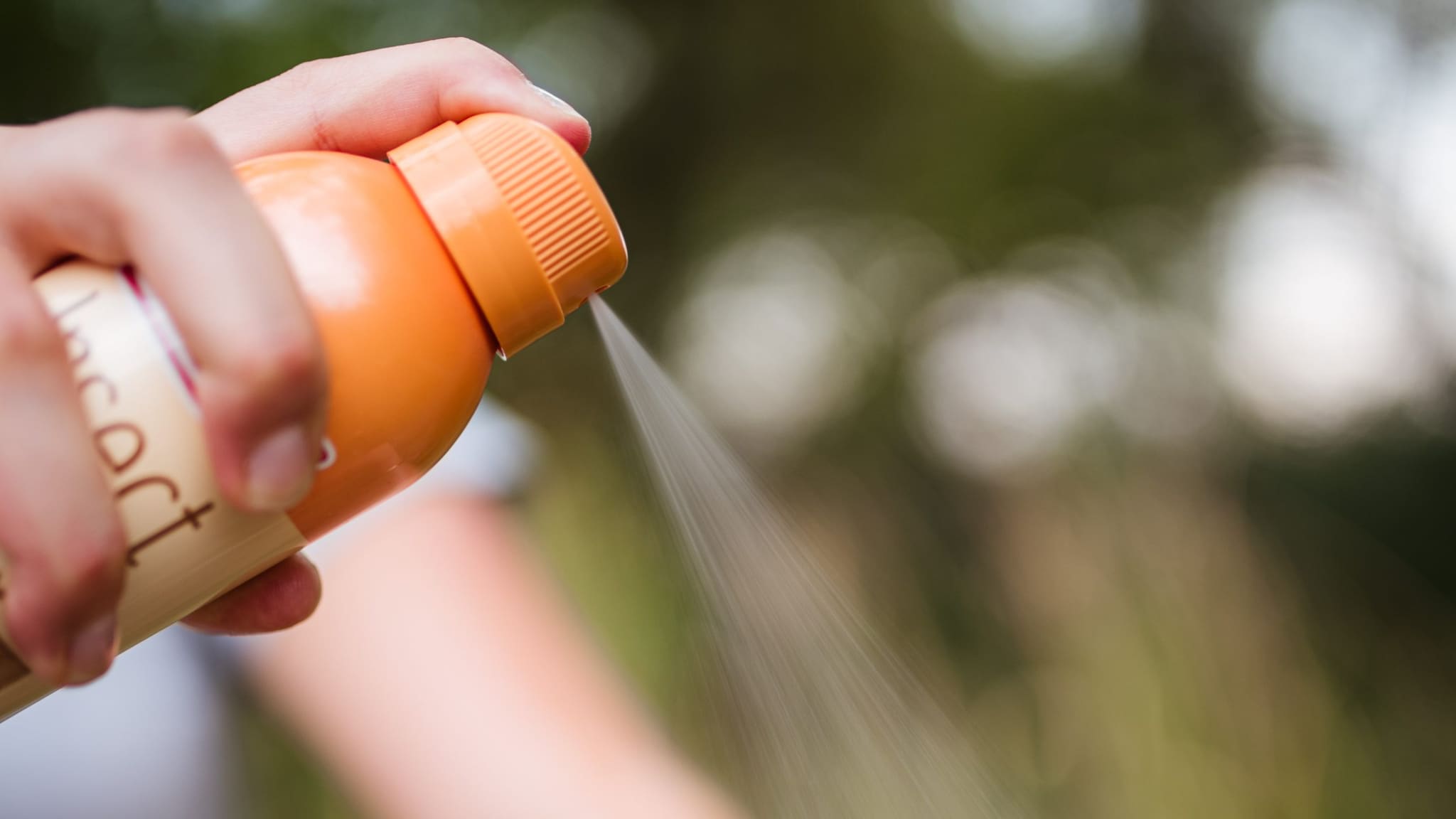
Preventing West Nile
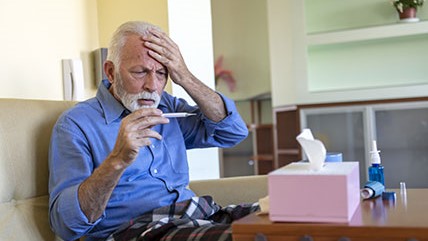
West Nile: Symptoms, Diagnosis, & Treatment
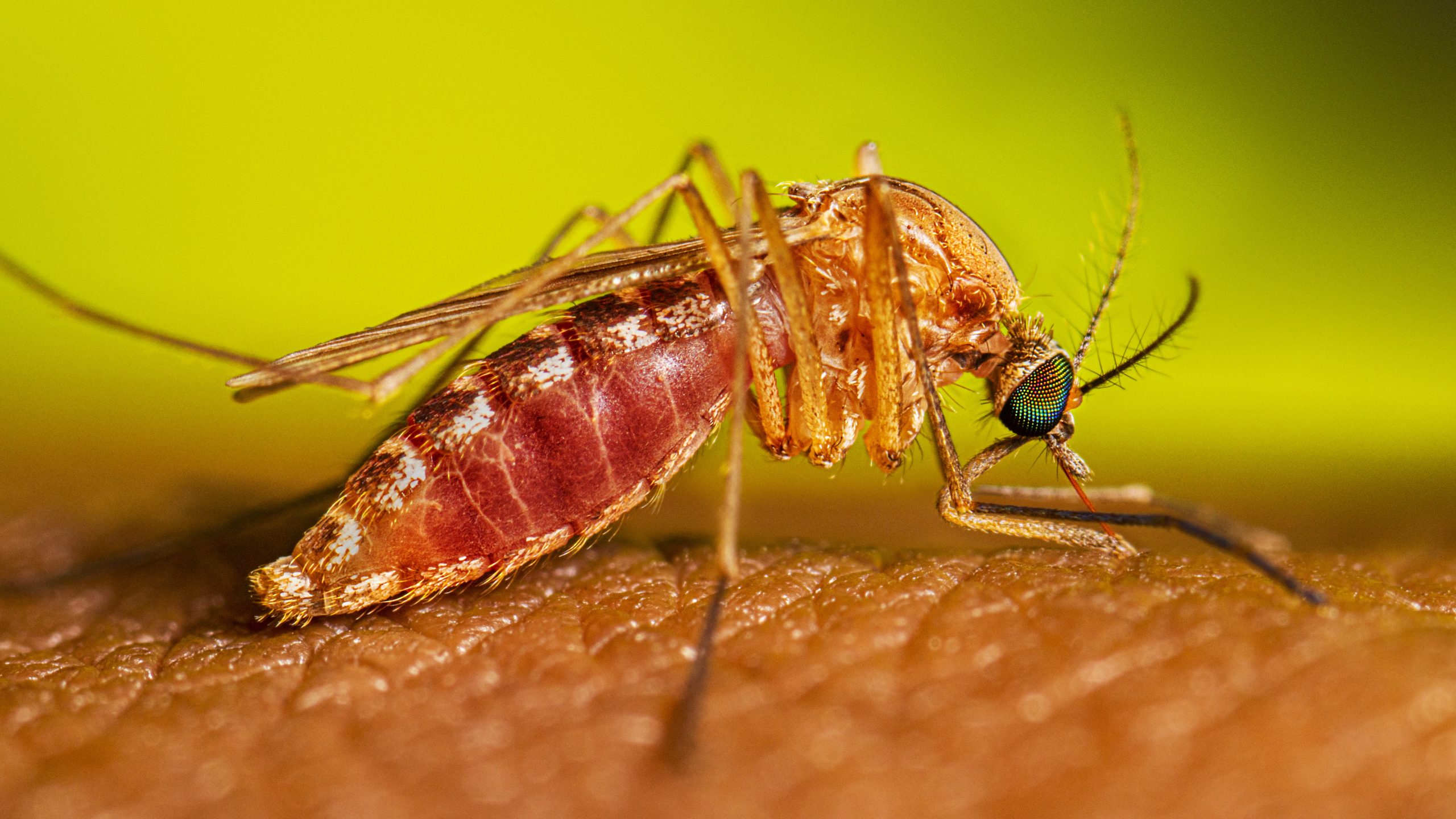
West Nile: Causes and How It Spreads
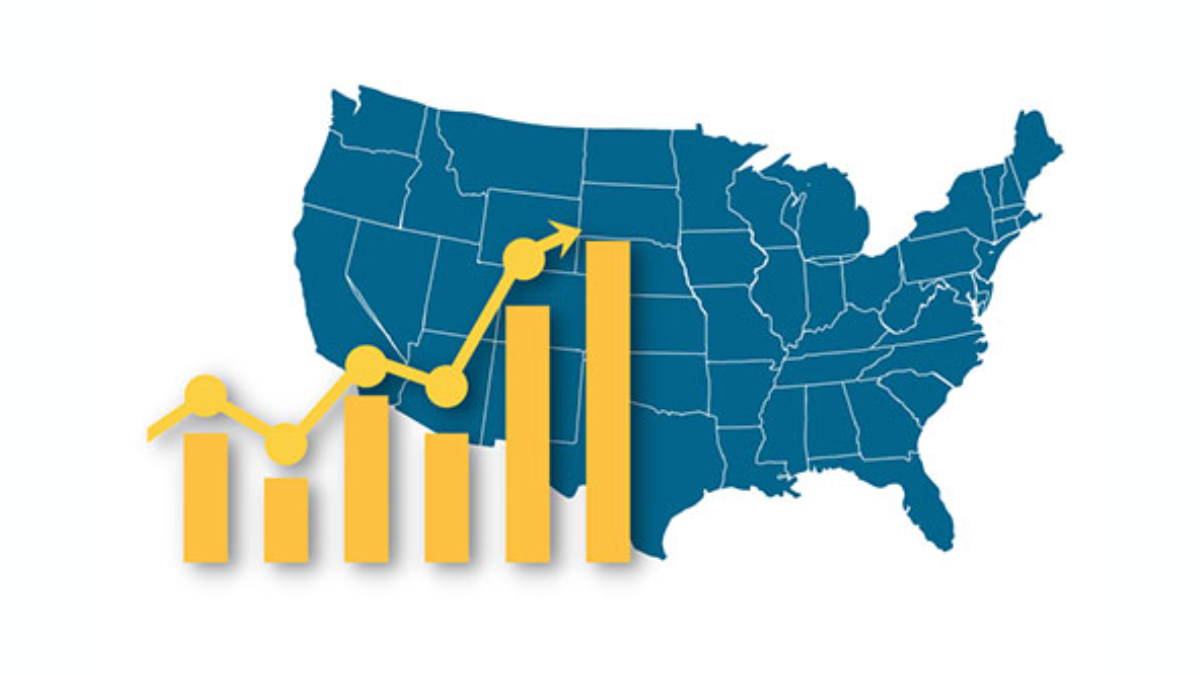
For Professionals
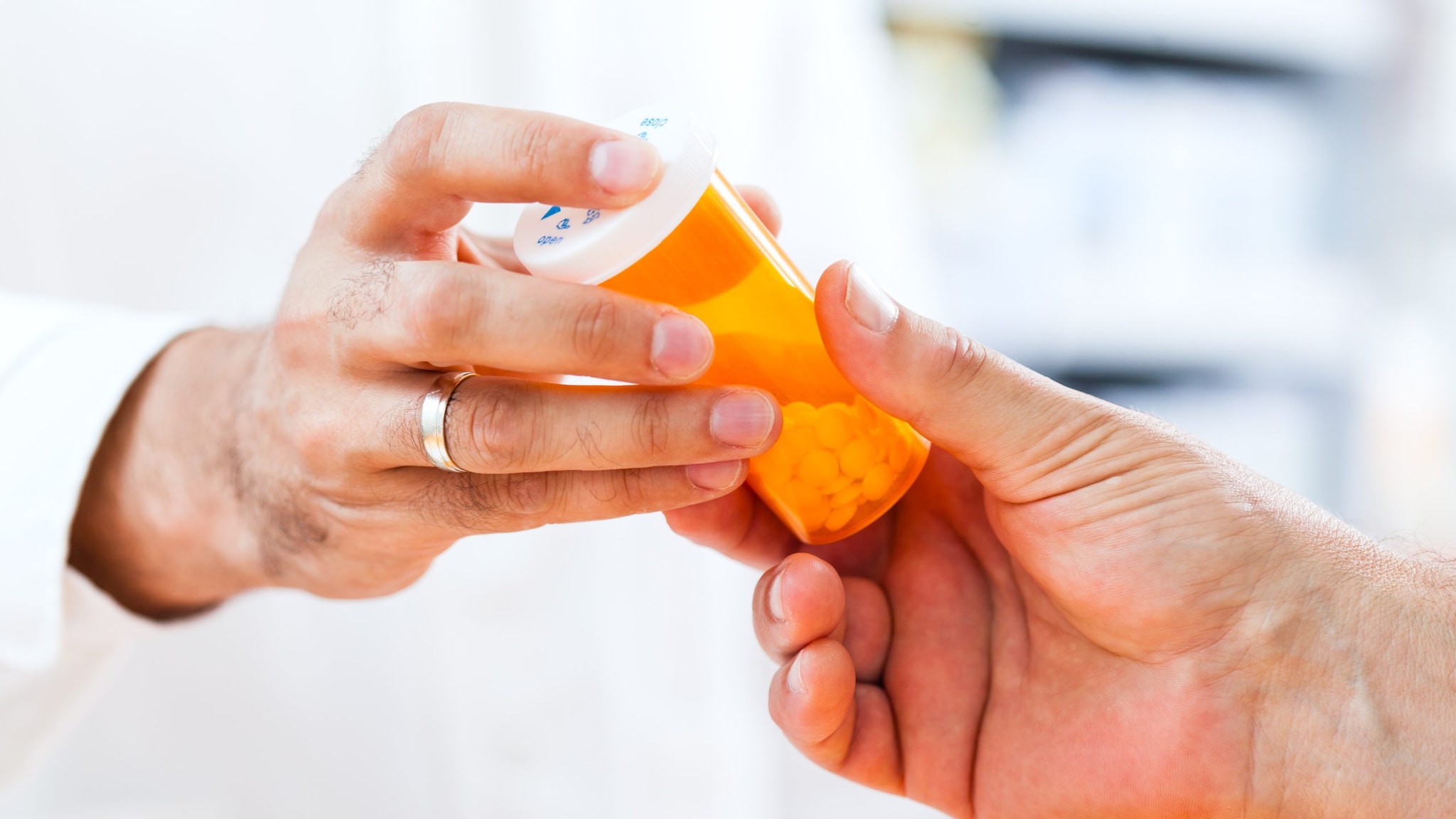
Treatment and Prevention of West Nile Virus Disease
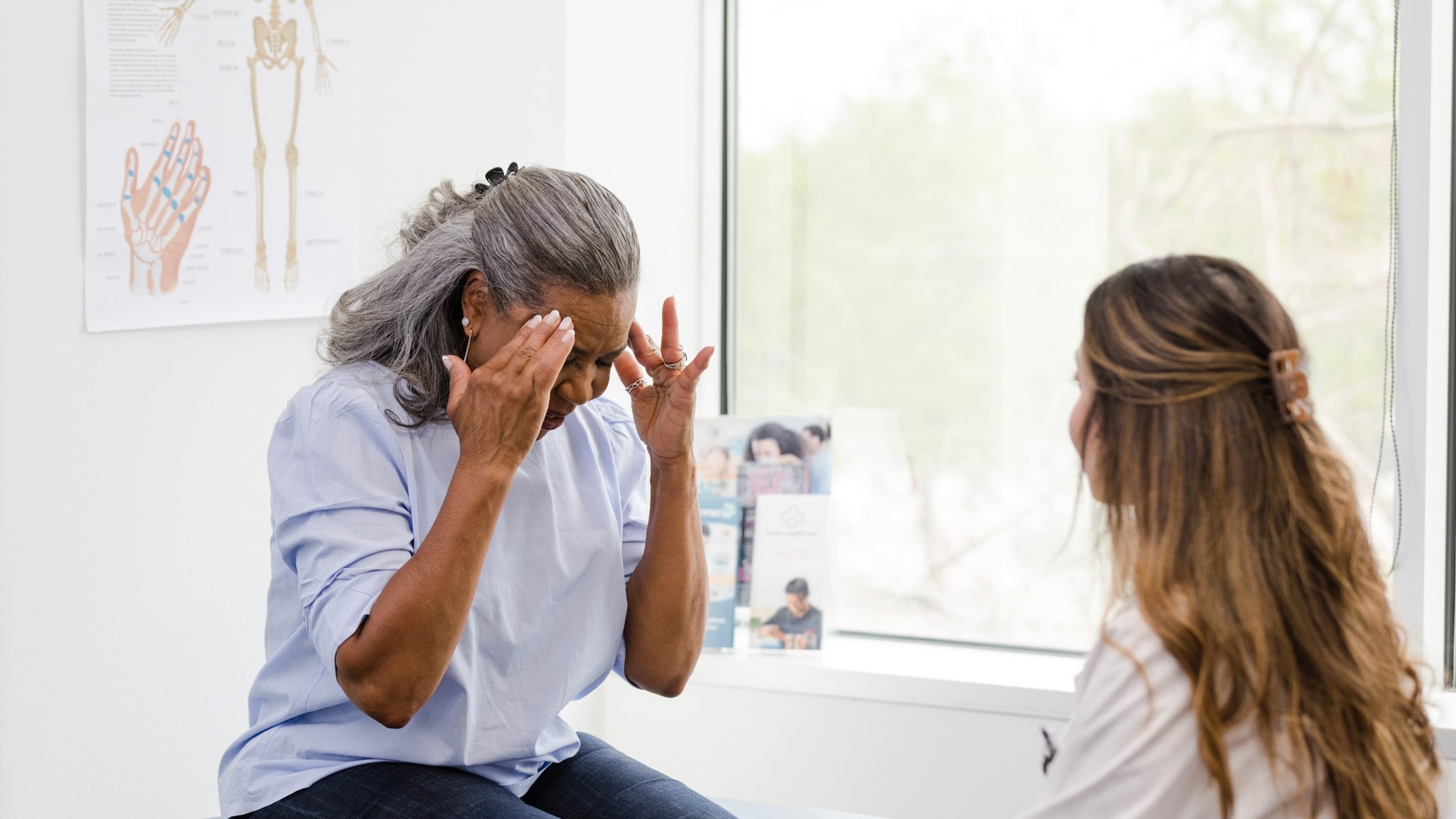
Clinical Signs and Symptoms of West Nile Virus Disease
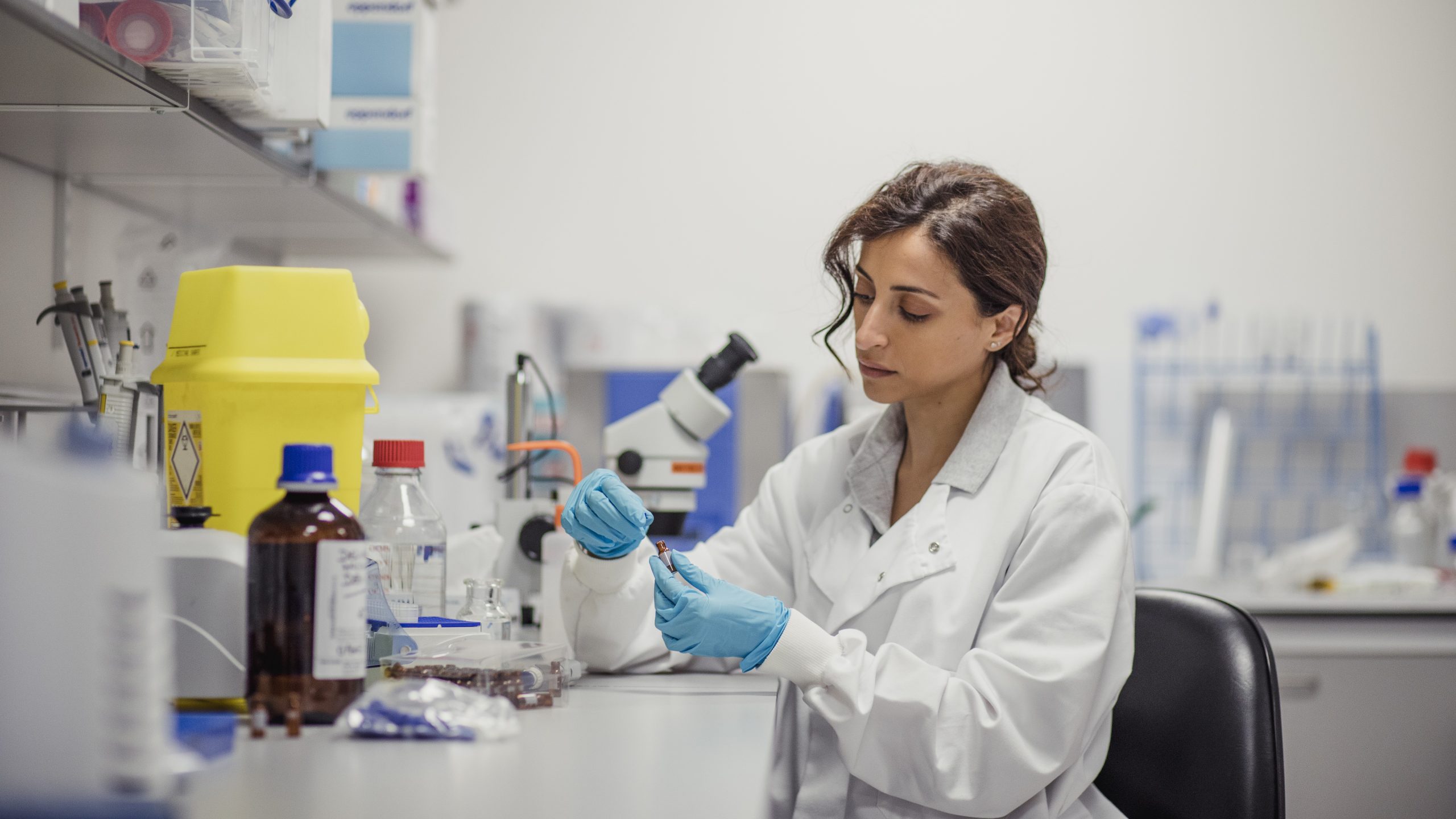
Clinical Testing and Diagnosis for West Nile Virus Disease
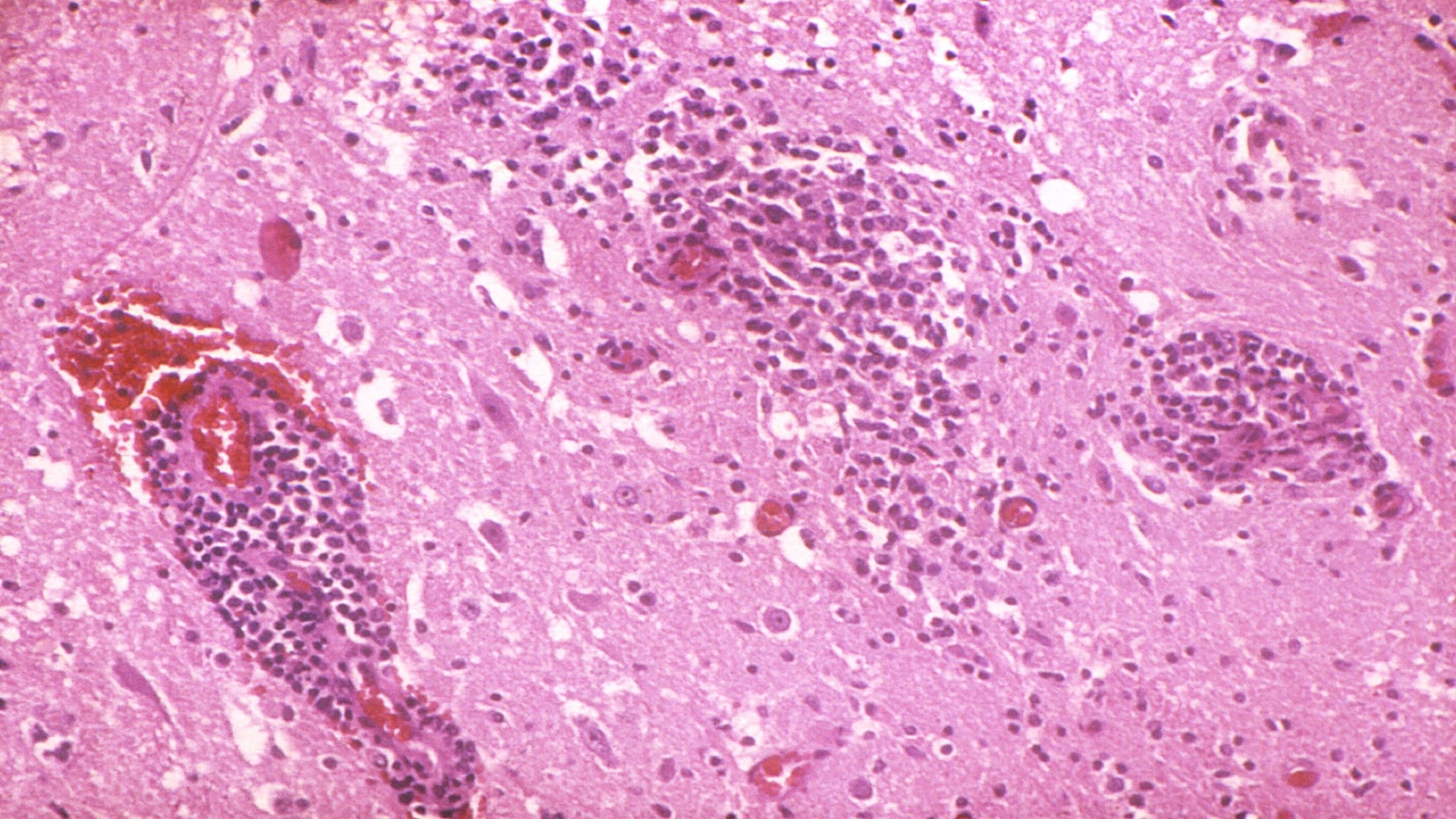
Transmission of West Nile Virus
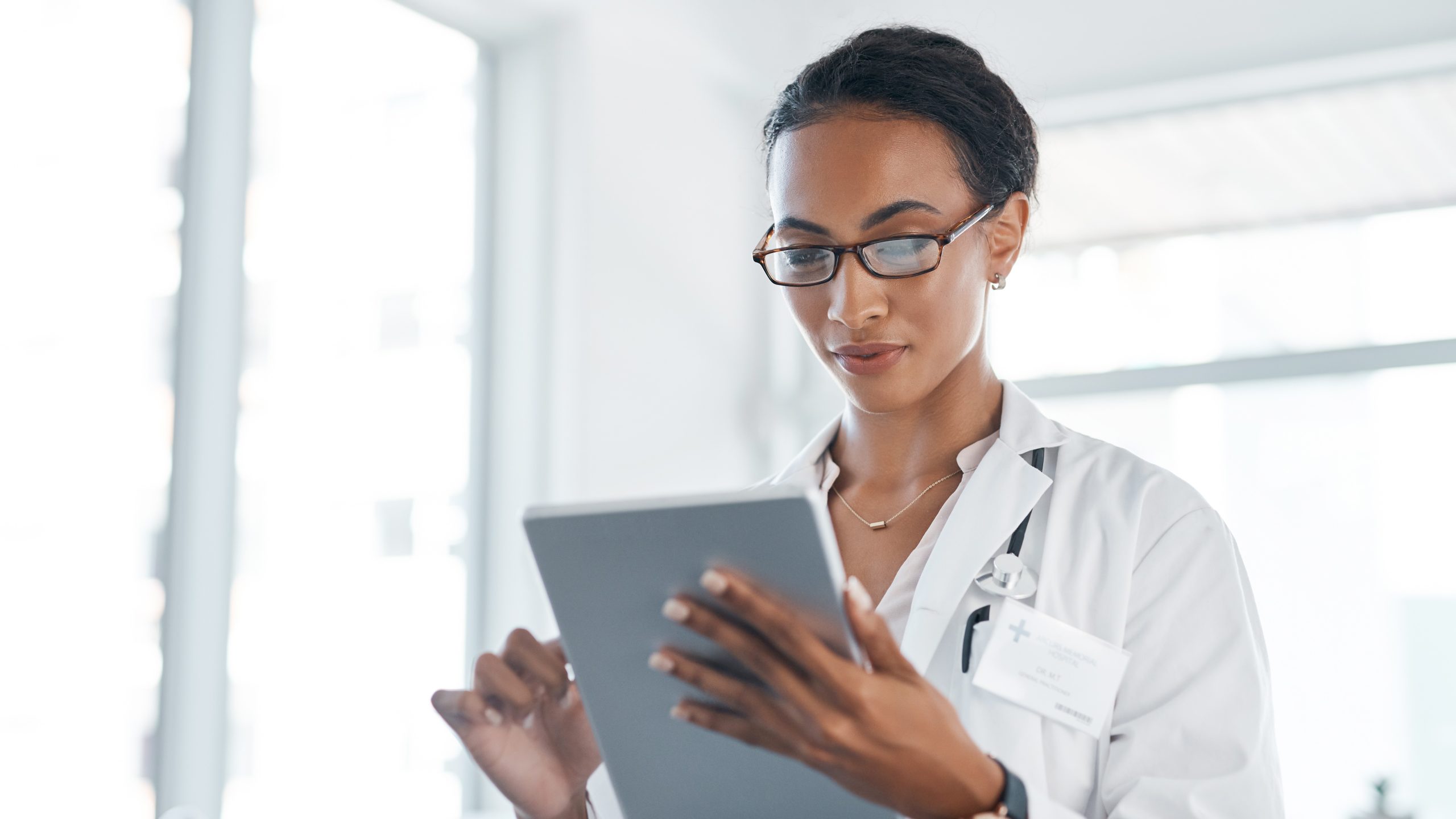
West Nile Virus Clinician Training – Free Continuing Medical Education (CME) Credit
West Nile virus is primarily spread by mosquitoes. Learn about areas at risk, the illness it causes, and ways to prevent becoming infected.
For Everyone
Health care providers, public health.
Please log in to save materials. Log in
- Resource Library
- Data Organization
- Data Presentation
- Frankie Fran
Activity Sheet-Organization and Presentation of Data in Textual, Tabular and Graphical Form
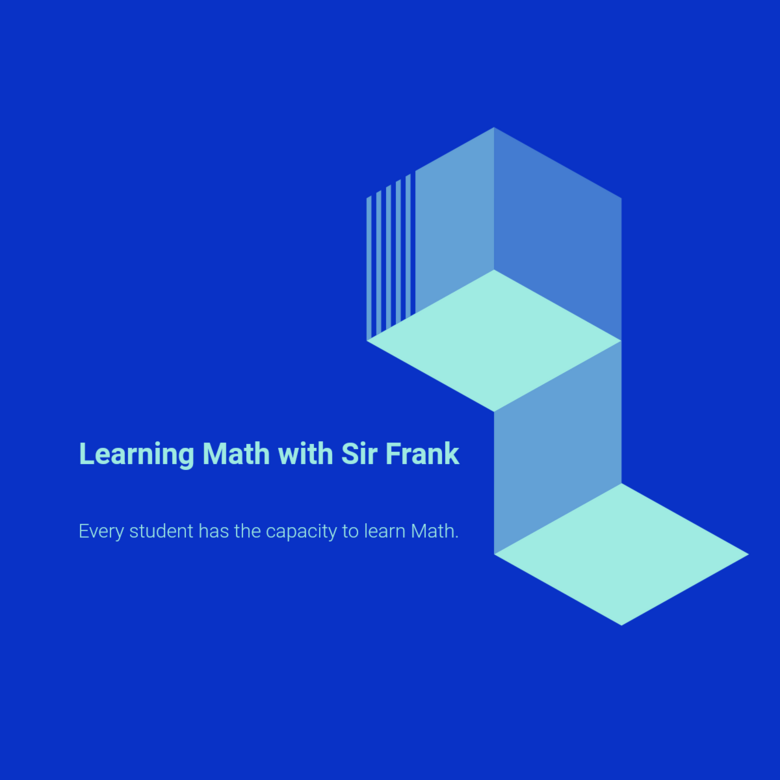
This resource can be used in providing real-life activity for students by conducting survey. Results of their survey will be organized and presented through text, graphs and tables with research ethics observed.
This activity sheet about the Organization and Presentation of Data in Textual, Tabular and Graphical Form for Basic Statistics Class.
Microsoft Power BI Blog
Power bi may 2024 feature summary.
Welcome to the May 2024 update! Here are a few, select highlights of the many we have for Power BI. There are new On-object Interaction updates, DAX query view is now generally available, find out how to view reports in OnDrive and SharePoint with live connected semantic models.
There is much more to explore, please continue to read on!
Microsoft Build Announcements
At Microsoft Build 2024, we are thrilled to announce a huge array of innovations coming to the Microsoft Fabric platform that will make Microsoft Fabric’s capabilities even more robust and even customizable to meet the unique needs of each organization. To learn more about these changes, read the “ Unlock real-time insights with AI-powered analytics in Microsoft Fabric ” announcement blog by Arun Ulag.
Earn a discount on your Microsoft Fabric certification exam!
We’d like to thank the thousands of you who completed the Fabric AI Skills Challenge and earned a free voucher for Exam DP-600 which leads to the Fabric Analytics Engineer Associate certification.
If you earned a free voucher, you can find redemption instructions in your email. We recommend that you schedule your exam now, before your discount voucher expires on June 24 th . All exams must be scheduled and completed by this date.
If you need a little more help with exam prep, visit the Fabric Career Hub which has expert-led training, exam crams, practice tests and more.
Missed the Fabric AI Skills Challenge? We have you covered. For a limited time , you could earn a 50% exam discount by taking the Fabric 30 Days to Learn It Challenge .

- Version number: v: 2.129.905.0
- Date published: 5/21/24
Modern Tooltip now on by Default
Matrix layouts, line updates, on-object interaction updates.

- Announcing general availability of DAX query view
New Manage relationships dialog
Refreshing calculated columns and calculated tables referencing directquery sources with single sign-on, announcing general availability of model explorer and authoring calculation groups in power bi desktop, microsoft entra id sso support for oracle database, certified connector updates, view reports in onedrive and sharepoint with live connected semantic models, storytelling in powerpoint – image mode in the power bi add-in for powerpoint, storytelling in powerpoint – data updated notification, git integration support for direct lake semantic models, editor’s pick of the quarter, new visuals in appsource, financial reporting matrix by profitbase, horizon chart by powerviz, sunburst chart by powerviz, stacked bar chart with line by jta.
Power BI tooltips are embarking on an evolution to enhance their functionality. To lay the groundwork, we are introducing the modern tooltip as the new default , a feature that many users may already recognize from its previous preview status. This change is more than just an upgrade; it’s the first step in a series of remarkable improvements. These future developments promise to revolutionize tooltip management and customization, offering possibilities that were previously only imaginable. As we prepare for the general availability of the modern tooltip, this is an excellent opportunity for users to become familiar with its features and capabilities.

Discover the full potential of the new tooltip feature by visiting our dedicated blog . Dive into the details and explore the comprehensive vision we’ve crafted for tooltips, designed to enhance your Power BI experience.
We’ve listened to our community’s feedback on improving our tabular visuals (Table and Matrix), and we’re excited to initiate their transformation. Drawing inspiration from the familiar PivotTable in Excel , we aim to build new features and capabilities upon a stronger foundation. In our May update, we’re introducing ‘ Layouts for Matrix .’ Now, you can select from compact , outline , or tabular layouts to alter the arrangement of components in a manner akin to Excel.

As an extension of the new layout options, report creators can now craft custom layout patterns by repeating row headers. This powerful control, inspired by Excel’s PivotTable layout, enables the creation of a matrix that closely resembles the look and feel of a table. This enhancement not only provides greater flexibility but also brings a touch of Excel’s intuitive design to Power BI’s matrix visuals. Only available for Outline and Tabular layouts.

To further align with Excel’s functionality, report creators now have the option to insert blank rows within the matrix. This feature allows for the separation of higher-level row header categories, significantly enhancing the readability of the report. It’s a thoughtful addition that brings a new level of clarity and organization to Power BI’s matrix visuals and opens a path for future enhancements for totals/subtotals and rows/column headers.

We understand your eagerness to delve deeper into the matrix layouts and grasp how these enhancements fulfill the highly requested features by our community. Find out more and join the conversation in our dedicated blog , where we unravel the details and share the community-driven vision behind these improvements.
Following last month’s introduction of the initial line enhancements, May brings a groundbreaking set of line capabilities that are set to transform your Power BI experience:
- Hide/Show lines : Gain control over the visibility of your lines for a cleaner, more focused report.
- Customized line pattern : Tailor the pattern of your lines to match the style and context of your data.
- Auto-scaled line pattern : Ensure your line patterns scale perfectly with your data, maintaining consistency and clarity.
- Line dash cap : Customize the end caps of your customized dashed lines for a polished, professional look.
- Line upgrades across other line types : Experience improvements in reference lines, forecast lines, leader lines, small multiple gridlines, and the new card’s divider line.
These enhancements are not to be missed. We recommend visiting our dedicated blog for an in-depth exploration of all the new capabilities added to lines, keeping you informed and up to date.
This May release, we’re excited to introduce on-object formatting support for Small multiples , Waterfall , and Matrix visuals. This new feature allows users to interact directly with these visuals for a more intuitive and efficient formatting experience. By double-clicking on any of these visuals, users can now right-click on the specific visual component they wish to format, bringing up a convenient mini-toolbar. This streamlined approach not only saves time but also enhances the user’s ability to customize and refine their reports with ease.

We’re also thrilled to announce a significant enhancement to the mobile reporting experience with the introduction of the pane manager for the mobile layout view. This innovative feature empowers users to effortlessly open and close panels via a dedicated menu, streamlining the design process of mobile reports.

Publish to folders
We recently announced a public preview for folders in workspaces, allowing you to create a hierarchical structure for organizing and managing your items. In the latest Desktop release, you can now publish your reports to specific folders in your workspace.
When you publish a report, you can choose the specific workspace and folder for your report. The interface is simplistic and easy to understand, making organizing your Power BI content from Desktop better than ever.

To publish reports to specific folders in the service, make sure the “Publish dialogs support folder selection” setting is enabled in the Preview features tab in the Options menu.

Learn more about folders in workspaces.
You can now ask Copilot questions about data in your model
We’re excited to preview a new capability for Power BI Copilot allowing you to ask questions about the data in your model! You could already ask questions about the data present in the visuals on your report pages – and now you can go deeper by getting answers directly from the underlying model. Just ask questions about your data, and if the answer isn’t already on your report, Copilot will then query your model for the data instead and return the answer to your question in the form of a visual!

We’re starting this capability off in both Edit and View modes in Power BI Service. Because this is a preview feature, you’ll need to enable it via the preview toggle in the Copilot pane. You can learn more about all the details of the feature in our announcement post here! (will link to announcement post)
Announcing general availability of DAX query view
We are excited to announce the general availability of DAX query view. DAX query view is the fourth view in Power BI Desktop to run DAX queries on your semantic model.
DAX query view comes with several ways to help you be as productive as possible with DAX queries.
- Quick queries. Have the DAX query written for you from the context menu of tables, columns, or measures in the Data pane of DAX query view. Get the top 100 rows of a table, statistics of a column, or DAX formula of a measure to edit and validate in just a couple clicks!
- DirectQuery model authors can also use DAX query view. View the data in your tables whenever you want!
- Create and edit measures. Edit one or multiple measures at once. Make changes and see the change in action in a DA query. Then update the model when you are ready. All in DAX query view!
- See the DAX query of visuals. Investigate the visuals DAX query in DAX query view. Go to the Performance Analyzer pane and choose “Run in DAX query view”.
- Write DAX queries. You can create DAX queries with Intellisense, formatting, commenting/uncommenting, and syntax highlighting. And additional professional code editing experiences such as “Change all occurrences” and block folding to expand and collapse sections. Even expanded find and replace options with regex.
Learn more about DAX query view with these resources:
- Deep dive blog: https://powerbi.microsoft.com/blog/deep-dive-into-dax-query-view-and-writing-dax-queries/
- Learn more: https://learn.microsoft.com/power-bi/transform-model/dax-query-view
- Video: https://youtu.be/oPGGYLKhTOA?si=YKUp1j8GoHHsqdZo
Copilot to write and explain DAX queries in DAX query view updates
DAX query view includes an inline Fabric Copilot to write and explain DAX queries, which remains in public preview. This month we have made the following updates.
- Run the DAX query before you keep it . Previously the Run button was disabled until the generated DAX query was accepted or Copilot was closed. Now you can Run the DAX query then decide to Keep or Discard the DAX query.

3. Syntax checks on the generated DAX query. Previously there was no syntax check before the generated DAX query was returned. Now the syntax is checked, and the prompt automatically retried once. If the retry is also invalid, the generated DAX query is returned with a note that there is an issue, giving you the option to rephrase your request or fix the generated DAX query.

Learn more about DAX queries with Copilot with these resources:
- Deep dive blog: https://powerbi.microsoft.com/en-us/blog/deep-dive-into-dax-query-view-with-copilot/
- Learn more: https://learn.microsoft.com/en-us/dax/dax-copilot
- Video: https://www.youtube.com/watch?v=0kE3TE34oLM
We are excited to introduce you to the redesigned ‘Manage relationships’ dialog in Power BI Desktop! To open this dialog simply select the ‘Manage relationships’ button in the modeling ribbon.

Once opened, you’ll find a comprehensive view of all your relationships, along with their key properties, all in one convenient location. From here you can create new relationships or edit an existing one.

Additionally, you have the option to filter and focus on specific relationships in your model based on cardinality and cross filter direction.

Learn more about creating and managing relationships in Power BI Desktop in our documentation .
Ever since we released composite models on Power BI semantic models and Analysis Services , you have been asking us to support the refresh of calculated columns and tables in the Service. This month, we have enabled the refresh of calculated columns and tables in Service for any DirectQuery source that uses single sign-on authentication. This includes the sources you use when working with composite models on Power BI semantic models and Analysis Services.
Previously, the refresh of a semantic model that uses a DirectQuery source with single-sign-on authentication failed with one of the following error messages: “Refresh is not supported for datasets with a calculated table or calculated column that depends on a table which references Analysis Services using DirectQuery.” or “Refresh over a dataset with a calculated table or a calculated column which references a Direct Query data source is not supported.”
Starting today, you can successfully refresh the calculated table and calculated columns in a semantic model in the Service using specific credentials as long as:
- You used a shareable cloud connection and assigned it and/or
- Enabled granular access control for all data connection types
Here’s how to do this:
- Create and publish your semantic model that uses a single sign-on DirectQuery source. This can be a composite model but doesn’t have to be.
- In the semantic model settings, under Gateway and cloud connections , map each single sign-on DirectQuery connection to a specific connection. If you don’t have a specific connection yet, select ‘Create a connection’ to create it:

- If you are creating a new connection, fill out the connection details and click Create , making sure to select ‘Use SSO via Azure AD for DirectQuery queries:

2. Finally, select the connection for each single sign-on DirectQuery source and select Apply :

We are excited to announce the general availability of Model Explorer in the Model view of Power BI, including the authoring of calculation groups. Semantic modeling is even easier with an at-a-glance tree view with item counts, search, and in context paths to edit the semantic model items with Model Explorer. Top level semantic model properties are also available as well as the option to quickly create relationships in the properties pane. Additionally, the styling for the Data pane is updated to Fluent UI also used in Office and Teams.
A popular community request from the Ideas forum, authoring calculation groups is also included in Model Explorer. Calculation groups significantly reduce the number of redundant measures by allowing you to define DAX formulas as calculation items that can be applied to existing measures. For example, define a year over year, prior month, conversion, or whatever your report needs in DAX formula once as a calculation item and reuse it with existing measures. This can reduce the number of measures you need to create and make the maintenance of the business logic simpler.
Available in both Power BI Desktop and when editing a semantic model in the workspace, take your semantic model authoring to the next level today!

Learn more about Model Explorer and authoring calculation groups with these resources:
- Use Model explorer in Power BI (preview) – Power BI | Microsoft Learn
- Create calculation groups in Power BI (preview) – Power BI | Microsoft Learn
Data connectivity
We’re happy to announce that the Oracle database connector has been enhanced this month with the addition of Single Sign-On support in the Power BI service with Microsoft Entra ID authentication.
Microsoft Entra ID SSO enables single sign-on to access data sources that rely on Microsoft Entra ID based authentication. When you configure Microsoft Entra SSO for an applicable data source, queries run under the Microsoft Entra identity of the user that interacts with the Power BI report.

We’re pleased to announce the new and updated connectors in this release:
- [New] OneStream : The OneStream Power BI Connector enables you to seamlessly connect Power BI to your OneStream applications by simply logging in with your OneStream credentials. The connector uses your OneStream security, allowing you to access only the data you have based on your permissions within the OneStream application. Use the connector to pull cube and relational data along with metadata members, including all their properties. Visit OneStream Power BI Connector to learn more. Find this connector in the other category.
- [New] Zendesk Data : A new connector developed by the Zendesk team that aims to go beyond the functionality of the existing Zendesk legacy connector created by Microsoft. Learn more about what this new connector brings.
- [New] CCH Tagetik
- [Update] Azure Databricks
Are you interested in creating your own connector and publishing it for your customers? Learn more about the Power Query SDK and the Connector Certification program .
Last May, we announced the integration between Power BI and OneDrive and SharePoint. Previously, this capability was limited to only reports with data in import mode. We’re excited to announce that you can now seamlessly view Power BI reports with live connected data directly in OneDrive and SharePoint!
When working on Power BI Desktop with a report live connected to a semantic model in the service, you can easily share a link to collaborate with others on your team and allow them to quickly view the report in their browser. We’ve made it easier than ever to access the latest data updates without ever leaving your familiar OneDrive and SharePoint environments. This integration streamlines your workflows and allows you to access reports within the platforms you already use. With collaboration at the heart of this improvement, teams can work together more effectively to make informed decisions by leveraging live connected semantic models without being limited to data only in import mode.
Utilizing OneDrive and SharePoint allows you to take advantage of built-in version control, always have your files available in the cloud, and utilize familiar and simplistic sharing.

While you told us that you appreciate the ability to limit the image view to only those who have permission to view the report, you asked for changes for the “Public snapshot” mode.
To address some of the feedback we got from you, we have made a few more changes in this area.
- Add-ins that were saved as “Public snapshot” can be printed and will not require that you go over all the slides and load the add-ins for permission check before the public image is made visible.
- You can use the “Show as saved image” on add-ins that were saved as “Public snapshot”. This will replace the entire add-in with an image representation of it, so the load time might be faster when you are presenting your presentation.
Many of us keep presentations open for a long time, which might cause the data in the presentation to become outdated.
To make sure you have in your slides the data you need, we added a new notification that tells you if more up to date data exists in Power BI and offers you the option to refresh and get the latest data from Power BI.
Direct Lake semantic models are now supported in Fabric Git Integration , enabling streamlined version control, enhanced collaboration among developers, and the establishment of CI/CD pipelines for your semantic models using Direct Lake.

Learn more about version control, testing, and deployment of Power BI content in our Power BI implementation planning documentation: https://learn.microsoft.com/power-bi/guidance/powerbi-implementation-planning-content-lifecycle-management-overview
Visualizations
– Animator for Power BI Innofalls Charts SuperTables Sankey Diagram for Power BI by ChartExpo Dynamic KPI Card by Sereviso Shielded HTML Viewer Text search slicer
Mapa Polski – Województwa, Powiaty, Gminy Workstream Income Statement Table
Gas Detection Chart
Seasonality Chart PlanIn BI – Data Refresh Service
Chart Flare
PictoBar ProgBar
Counter Calendar Donut Chart image
Making financial statements with a proper layout has just become easier with the latest version of the Financial Reporting Matrix.
Users are now able to specify which rows should be classified as cost-rows, which will make it easier to get the conditional formatting of variances correctly:

Selecting a row, and ticking “is cost” will tag the row as cost. This can be used in conditional formatting to make sure that positive variances on expenses are a bad for the result, while a positive variance on an income row is good for the result.
The new version also includes more flexibility in measuring placement and column subtotals.
Measures can be placed either:
- Default (below column headers)
- Above column headers

- Conditionally hide columns
- + much more
Highlighted new features:
- Measure placement – In rows
- Select Column Subtotals
- New Format Pane design
- Row Options
Get the visual from AppSource and find more videos here !
A Horizon Chart is an advanced visual, for time-series data, revealing trends and anomalies. It displays stacked data layers, allowing users to compare multiple categories while maintaining data clarity. Horizon Charts are particularly useful to monitor and analyze complex data over time, making this a valuable visual for data analysis and decision-making.
Key Features:
- Horizon Styles: Choose Natural, Linear, or Step with adjustable scaling.
- Layer: Layer data by range or custom criteria. Display positive and negative values together or separately on top.
- Reference Line : Highlight patterns with X-axis lines and labels.
- Colors: Apply 30+ color palettes and use FX rules for dynamic coloring.
- Ranking: Filter Top/Bottom N values, with “Others”.
- Gridline: Add gridlines to the X and Y axis.
- Custom Tooltip: Add highest, lowest, mean, and median points without additional DAX.
- Themes: Save designs and share seamlessly with JSON files.
Other features included are ranking, annotation, grid view, show condition, and accessibility support.
Business Use Cases: Time-Series Data Comparison, Environmental Monitoring, Anomaly Detection
🔗 Try Horizon Chart for FREE from AppSource
📊 Check out all features of the visual: Demo file
📃 Step-by-step instructions: Documentation
💡 YouTube Video: Video Link
📍 Learn more about visuals: https://powerviz.ai/
✅ Follow Powerviz : https://lnkd.in/gN_9Sa6U
Milestone Trend Analysis Chart by Nova Silva
Exciting news! Thanks to your valuable feedback, we’ve enhanced our Milestone Trend Analysis Chart even further. We’re thrilled to announce that you can now switch between horizontal and vertical orientations, catering to your preferred visualization style.
The Milestone Trend Analysis (MTA) Chart remains your go-to tool for swiftly identifying deadline trends, empowering you to take timely corrective actions. With this update, we aim to enhance deadline awareness among project participants and stakeholders alike.

In our latest version, we seamlessly navigate between horizontal and vertical views within the familiar Power BI interface. No need to adapt to a new user interface – enjoy the same ease of use with added flexibility. Plus, it benefits from supported features like themes, interactive selection, and tooltips.
What’s more, ours is the only Microsoft Certified Milestone Trend Analysis Chart for Power BI, ensuring reliability and compatibility with the platform.
Ready to experience the enhanced Milestone Trend Analysis Chart? Download it from AppSource today and explore its capabilities with your own data – try for free!
We welcome any questions or feedback at our website: https://visuals.novasilva.com/ . Try it out and elevate your project management insights now!
Powerviz’s Sunburst Chart is an interactive tool for hierarchical data visualization. With this chart, you can easily visualize multiple columns in a hierarchy and uncover valuable insights. The concentric circle design helps in displaying part-to-whole relationships.
- Arc Customization: Customize shapes and patterns.
- Color Scheme: Accessible palettes with 30+ options.
- Centre Circle: Design an inner circle with layers. Add text, measure, icons, and images.
- Conditional Formatting: Easily identify outliers based on measure or category rules.
- Labels: Smart data labels for readability.
- Image Labels: Add an image as an outer label.
- Interactivity: Zoom, drill down, cross-filtering, and tooltip features.
Other features included are annotation, grid view, show condition, and accessibility support.
Business Use Cases:
- Sales and Marketing: Market share analysis and customer segmentation.
- Finance : Department budgets and expenditures distribution.
- Operations : Supply chain management.
- Education : Course structure, curriculum creation.
- Human Resources : Organization structure, employee demographics.
🔗 Try Sunburst Chart for FREE from AppSource

Clustered bar chart with the possibility to stack one of the bars
Stacked Bar Chart with Line by JTA seamlessly merges the simplicity of a traditional bar chart with the versatility of a stacked bar, revolutionizing the way you showcase multiple datasets in a single, cohesive display.
Unlocking a new dimension of insight, our visual features a dynamic line that provides a snapshot of data trends at a glance. Navigate through your data effortlessly with multiple configurations, gaining a swift and comprehensive understanding of your information.
Tailor your visual experience with an array of functionalities and customization options, enabling you to effortlessly compare a primary metric with the performance of an entire set. The flexibility to customize the visual according to your unique preferences empowers you to harness the full potential of your data.
Features of Stacked Bar Chart with Line:
- Stack the second bar
- Format the Axis and Gridlines
- Add a legend
- Format the colors and text
- Add a line chart
- Format the line
- Add marks to the line
- Format the labels for bars and line
If you liked what you saw, you can try it for yourself and find more information here . Also, if you want to download it, you can find the visual package on the AppSource .

We have added an exciting new feature to our Combo PRO, Combo Bar PRO, and Timeline PRO visuals – Legend field support . The Legend field makes it easy to visually split series values into smaller segments, without the need to use measures or create separate series. Simply add a column with category names that are adjacent to the series values, and the visual will do the following:
- Display separate segments as a stack or cluster, showing how each segment contributed to the total Series value.
- Create legend items for each segment to quickly show/hide them without filtering.
- Apply custom fill colors to each segment.
- Show each segment value in the tooltip
Read more about the Legend field on our blog article
Drill Down Combo PRO is made for creators who want to build visually stunning and user-friendly reports. Cross-chart filtering and intuitive drill down interactions make data exploration easy and fun for any user. Furthermore, you can choose between three chart types – columns, lines, or areas; and feature up to 25 different series in the same visual and configure each series independently.
📊 Get Drill Down Combo PRO on AppSource
🌐 Visit Drill Down Combo PRO product page
Documentation | ZoomCharts Website | Follow ZoomCharts on LinkedIn
That is all for this month! Please continue sending us your feedback and do not forget to vote for other features that you would like to see in Power BI! We hope that you enjoy the update! If you installed Power BI Desktop from the Microsoft Store, please leave us a review .
Also, don’t forget to vote on your favorite feature this month on our community website.
As always, keep voting on Ideas to help us determine what to build next. We are looking forward to hearing from you!
- Microsoft Fabric
Please enter your information to subscribe to the Microsoft Fabric Blog.
Microsoft fabric updates blog.
Microsoft Fabric May 2024 Update
- Monthly Update
Welcome to the May 2024 update.
Here are a few, select highlights of the many we have for Fabric. You can now ask Copilot questions about data in your model, Model Explorer and authoring calculation groups in Power BI desktop is now generally available, and Real-Time Intelligence provides a complete end-to-end solution for ingesting, processing, analyzing, visualizing, monitoring, and acting on events.
There is much more to explore, please continue to read on.
Microsoft Build Announcements
At Microsoft Build 2024, we are thrilled to announce a huge array of innovations coming to the Microsoft Fabric platform that will make Microsoft Fabric’s capabilities even more robust and even customizable to meet the unique needs of each organization. To learn more about these changes, read the “ Unlock real-time insights with AI-powered analytics in Microsoft Fabric ” announcement blog by Arun Ulag.
Fabric Roadmap Update
Last October at the Microsoft Power Platform Community Conference we announced the release of the Microsoft Fabric Roadmap . Today we have updated that roadmap to include the next semester of Fabric innovations. As promised, we have merged Power BI into this roadmap to give you a single, unified road map for all of Microsoft Fabric. You can find the Fabric Roadmap at https://aka.ms/FabricRoadmap .
We will be innovating our Roadmap over the coming year and would love to hear your recommendation ways that we can make this experience better for you. Please submit suggestions at https://aka.ms/FabricIdeas .
Earn a discount on your Microsoft Fabric certification exam!
We’d like to thank the thousands of you who completed the Fabric AI Skills Challenge and earned a free voucher for Exam DP-600 which leads to the Fabric Analytics Engineer Associate certification.
If you earned a free voucher, you can find redemption instructions in your email. We recommend that you schedule your exam now, before your discount voucher expires on June 24 th . All exams must be scheduled and completed by this date.
If you need a little more help with exam prep, visit the Fabric Career Hub which has expert-led training, exam crams, practice tests and more.
Missed the Fabric AI Skills Challenge? We have you covered. For a limited time , you could earn a 50% exam discount by taking the Fabric 30 Days to Learn It Challenge .
Modern Tooltip now on by Default
Matrix layouts, line updates, on-object interaction updates, publish to folders in public preview, you can now ask copilot questions about data in your model (preview), announcing general availability of dax query view, copilot to write and explain dax queries in dax query view public preview updates, new manage relationships dialog, refreshing calculated columns and calculated tables referencing directquery sources with single sign-on, announcing general availability of model explorer and authoring calculation groups in power bi desktop, microsoft entra id sso support for oracle database, certified connector updates, view reports in onedrive and sharepoint with live connected semantic models, storytelling in powerpoint – image mode in the power bi add-in for powerpoint, storytelling in powerpoint – data updated notification, git integration support for direct lake semantic models.
- Editor’s pick of the quarter
- New visuals in AppSource
- Financial Reporting Matrix by Profitbase
- Horizon Chart by Powerviz
Milestone Trend Analysis Chart by Nova Silva
- Sunburst Chart by Powerviz
- Stacked Bar Chart with Line by JTA
Fabric Automation
Streamlining fabric admin apis, microsoft fabric workload development kit, external data sharing, apis for onelake data access roles, shortcuts to on-premises and network-restricted data, copilot for data warehouse, unlocking insights through time: time travel in data warehouse, copy into enhancements, faster workspace resource assignment powered by just in time database attachment, runtime 1.3 (apache spark 3.5, delta lake 3.1, r 4.3.3, python 3.11) – public preview.
- Native Execution Engine for Fabric Runtime 1.2 (Apache Spark 3.4) – Public Preview
Spark Run Series Analysis
Comment @tagging in notebook, notebook ribbon upgrade, notebook metadata update notification, environment is ga now, rest api support for workspace data engineering/science settings, fabric user data functions (private preview), introducing api for graphql in microsoft fabric (preview), copilot will be enabled by default, the ai and copilot setting will be automatically delegated to capacity admins, abuse monitoring no longer stores your data, real-time hub, source from real-time hub in enhanced eventstream, use real-time hub to get data in kql database in eventhouse, get data from real-time hub within reflexes, eventstream edit and live modes, default and derived streams, route streams based on content in enhanced eventstream, eventhouse is now generally available, eventhouse onelake availability is now generally available, create a database shortcut to another kql database, support for ai anomaly detector, copilot for real-time intelligence, eventhouse tenant level private endpoint support, visualize data with real-time dashboards, new experience for data exploration, create triggers from real-time hub, set alert on real-time dashboards, taking action through fabric items, general availability of the power query sdk for vs code, refresh the refresh history dialog, introducing data workflows in data factory, introducing trusted workspace access in fabric data pipelines.
- Introducing Blob Storage Event Triggers for Data Pipelines
- Parent/child pipeline pattern monitoring improvements
Fabric Spark job definition activity now available
Hd insight activity now available, modern get data experience in data pipeline.
Power BI tooltips are embarking on an evolution to enhance their functionality. To lay the groundwork, we are introducing the modern tooltip as the new default , a feature that many users may already recognize from its previous preview status. This change is more than just an upgrade; it’s the first step in a series of remarkable improvements. These future developments promise to revolutionize tooltip management and customization, offering possibilities that were previously only imaginable. As we prepare for the general availability of the modern tooltip, this is an excellent opportunity for users to become familiar with its features and capabilities.
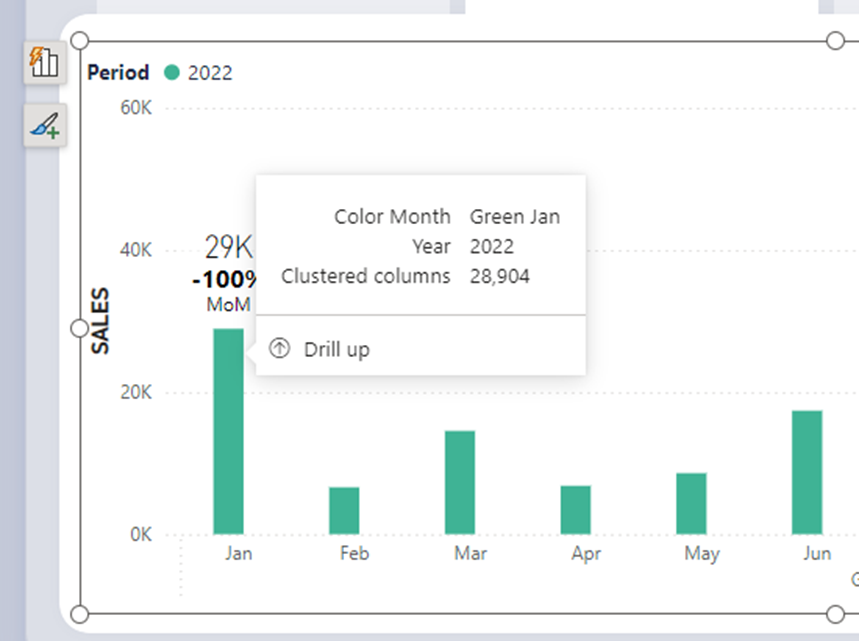
Discover the full potential of the new tooltip feature by visiting our dedicated blog . Dive into the details and explore the comprehensive vision we’ve crafted for tooltips, designed to enhance your Power BI experience.
We’ve listened to our community’s feedback on improving our tabular visuals (Table and Matrix), and we’re excited to initiate their transformation. Drawing inspiration from the familiar PivotTable in Excel , we aim to build new features and capabilities upon a stronger foundation. In our May update, we’re introducing ‘ Layouts for Matrix .’ Now, you can select from compact , outline , or tabular layouts to alter the arrangement of components in a manner akin to Excel.
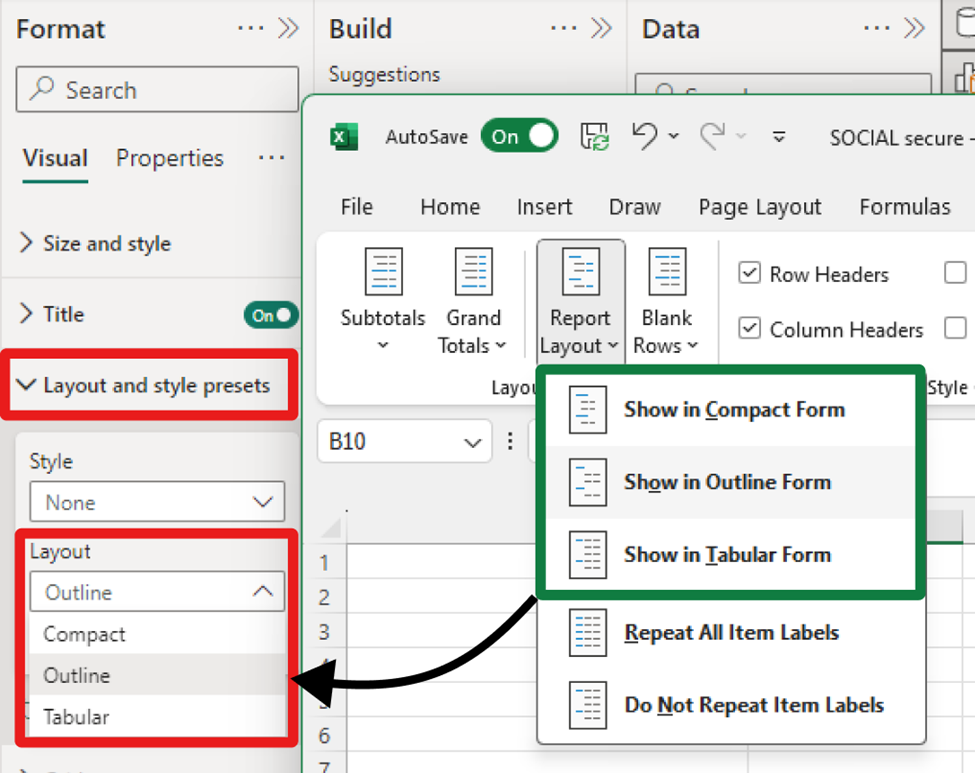
As an extension of the new layout options, report creators can now craft custom layout patterns by repeating row headers. This powerful control, inspired by Excel’s PivotTable layout, enables the creation of a matrix that closely resembles the look and feel of a table. This enhancement not only provides greater flexibility but also brings a touch of Excel’s intuitive design to Power BI’s matrix visuals. Only available for Outline and Tabular layouts.
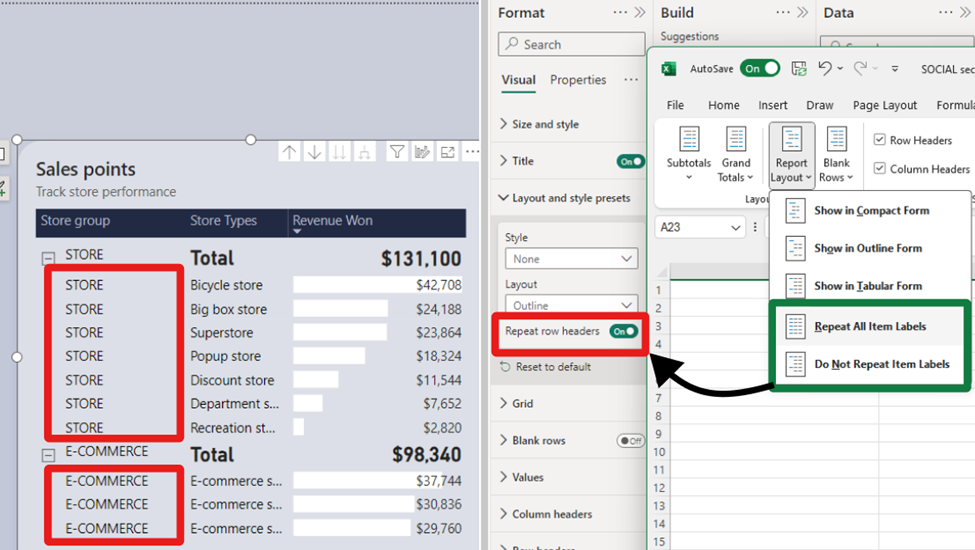
To further align with Excel’s functionality, report creators now have the option to insert blank rows within the matrix. This feature allows for the separation of higher-level row header categories, significantly enhancing the readability of the report. It’s a thoughtful addition that brings a new level of clarity and organization to Power BI’s matrix visuals and opens a path for future enhancements for totals/subtotals and rows/column headers.
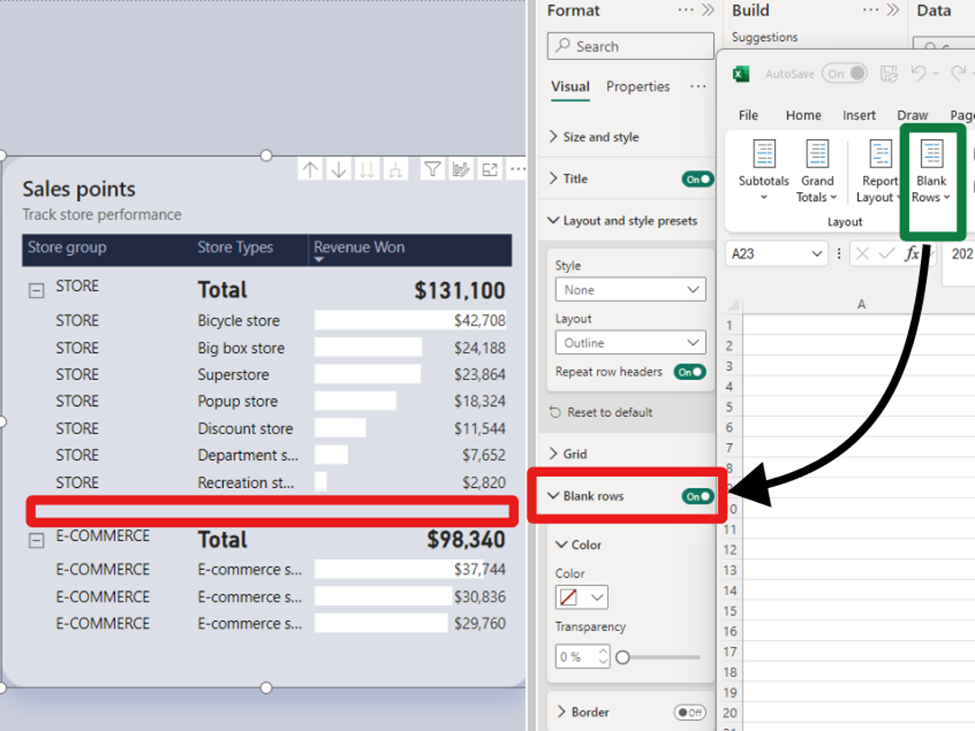
We understand your eagerness to delve deeper into the matrix layouts and grasp how these enhancements fulfill the highly requested features by our community. Find out more and join the conversation in our dedicated blog , where we unravel the details and share the community-driven vision behind these improvements.
Following last month’s introduction of the initial line enhancements, May brings a groundbreaking set of line capabilities that are set to transform your Power BI experience:
- Hide/Show lines : Gain control over the visibility of your lines for a cleaner, more focused report.
- Customized line pattern : Tailor the pattern of your lines to match the style and context of your data.
- Auto-scaled line pattern : Ensure your line patterns scale perfectly with your data, maintaining consistency and clarity.
- Line dash cap : Customize the end caps of your customized dashed lines for a polished, professional look.
- Line upgrades across other line types : Experience improvements in reference lines, forecast lines, leader lines, small multiple gridlines, and the new card’s divider line.
These enhancements are not to be missed. We recommend visiting our dedicated blog for an in-depth exploration of all the new capabilities added to lines, keeping you informed and up to date.
This May release, we’re excited to introduce on-object formatting support for Small multiples , Waterfall , and Matrix visuals. This new feature allows users to interact directly with these visuals for a more intuitive and efficient formatting experience. By double-clicking on any of these visuals, users can now right-click on the specific visual component they wish to format, bringing up a convenient mini-toolbar. This streamlined approach not only saves time but also enhances the user’s ability to customize and refine their reports with ease.
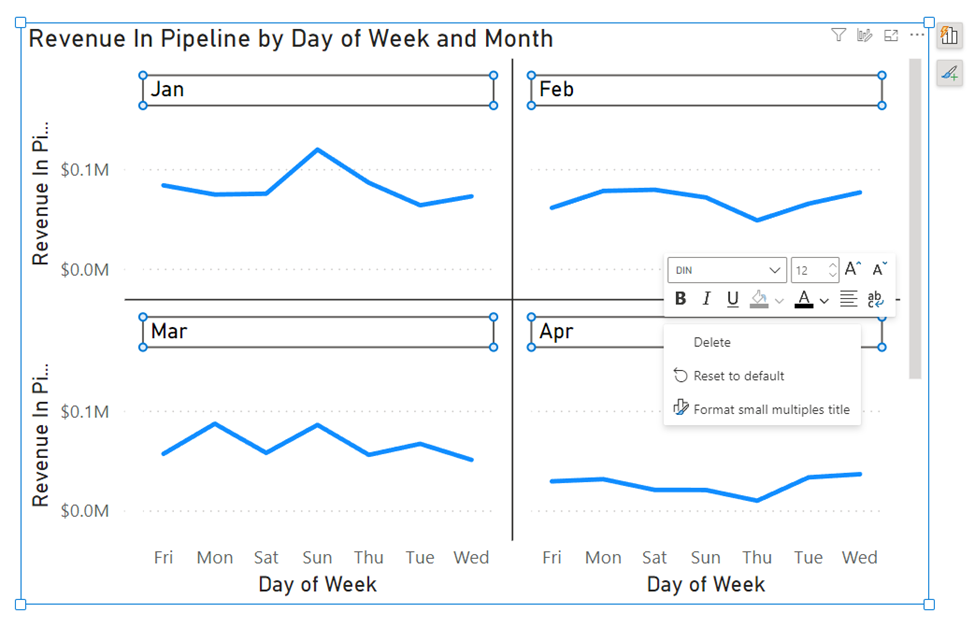
We’re also thrilled to announce a significant enhancement to the mobile reporting experience with the introduction of the pane manager for the mobile layout view. This innovative feature empowers users to effortlessly open and close panels via a dedicated menu, streamlining the design process of mobile reports.
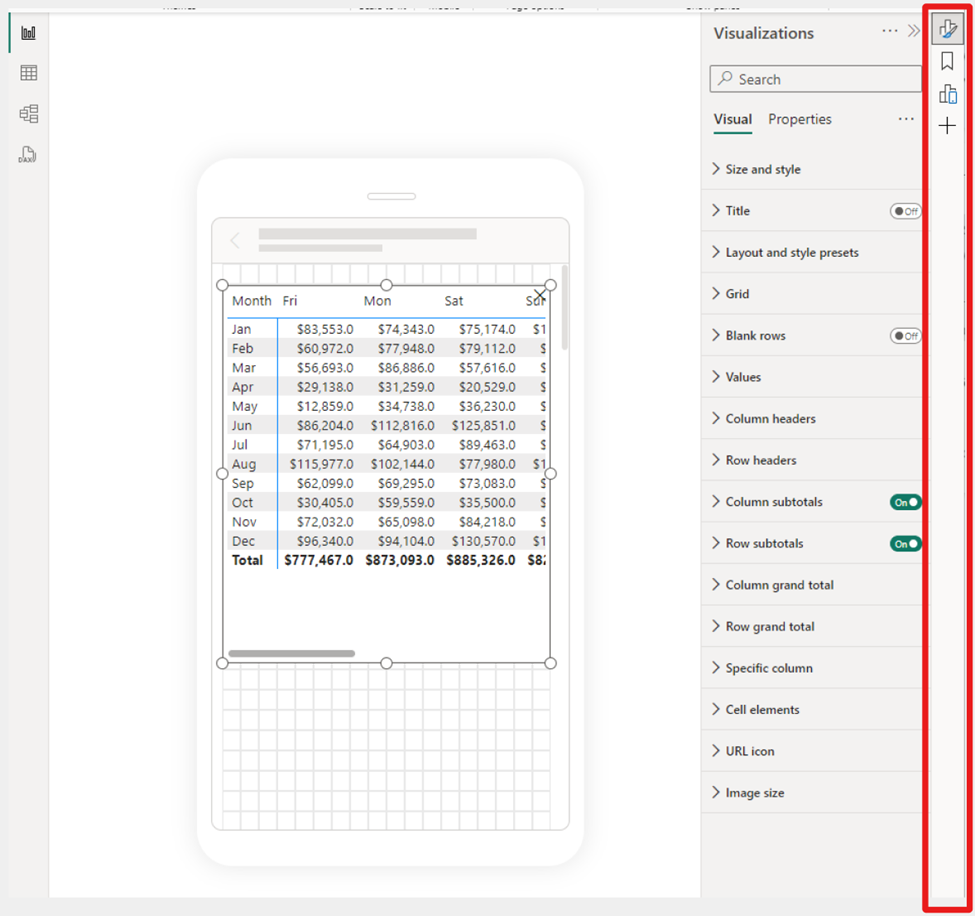
We recently announced a public preview for folders in workspaces, allowing you to create a hierarchical structure for organizing and managing your items. In the latest Desktop release, you can now publish your reports to specific folders in your workspace.
When you publish a report, you can choose the specific workspace and folder for your report. The interface is simplistic and easy to understand, making organizing your Power BI content from Desktop better than ever.
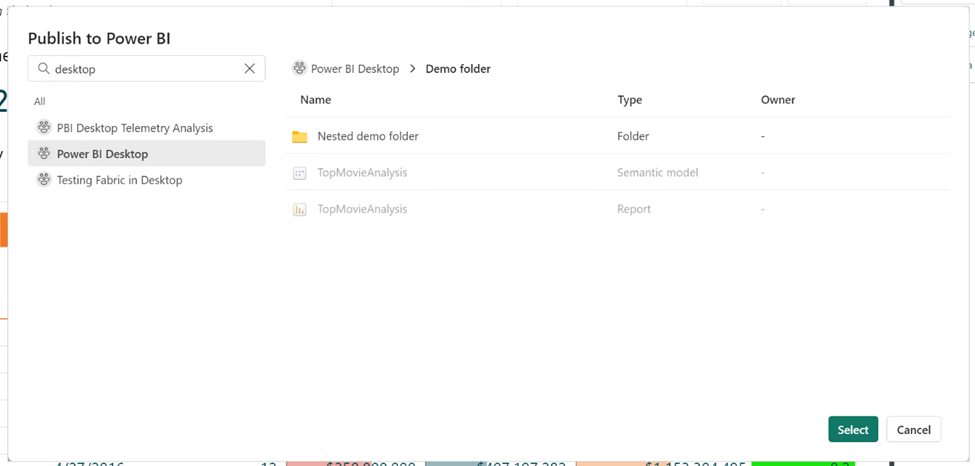
To publish reports to specific folders in the service, make sure the “Publish dialogs support folder selection” setting is enabled in the Preview features tab in the Options menu.
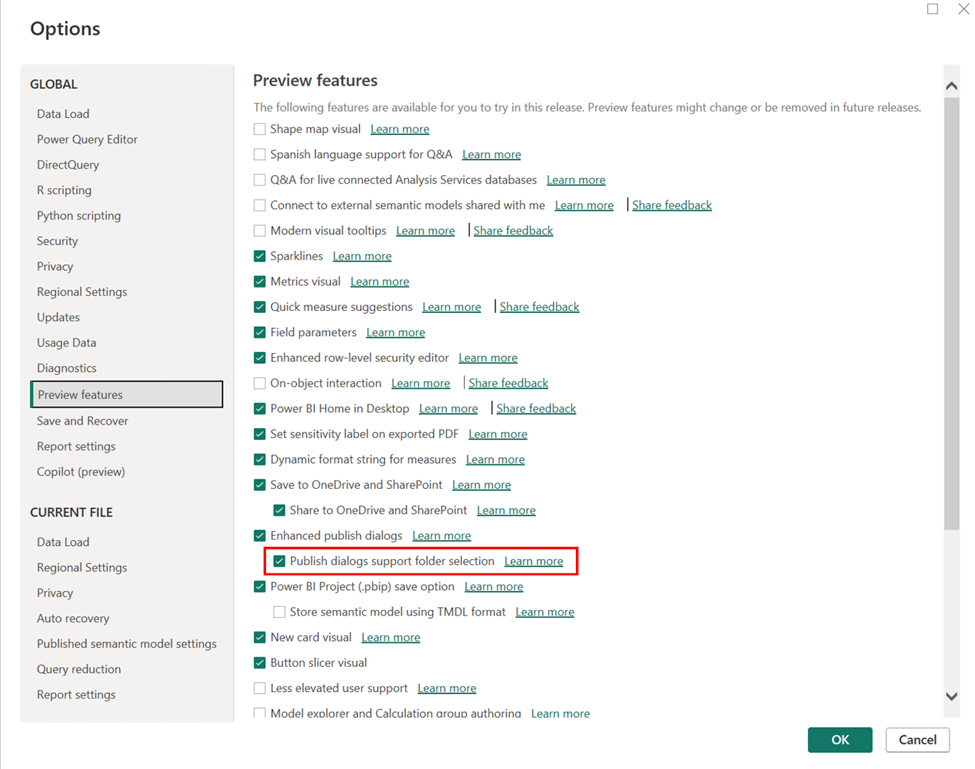
Learn more about folders in workspaces.
We’re excited to preview a new capability for Power BI Copilot allowing you to ask questions about the data in your model! You could already ask questions about the data present in the visuals on your report pages – and now you can go deeper by getting answers directly from the underlying model. Just ask questions about your data, and if the answer isn’t already on your report, Copilot will then query your model for the data instead and return the answer to your question in the form of a visual!
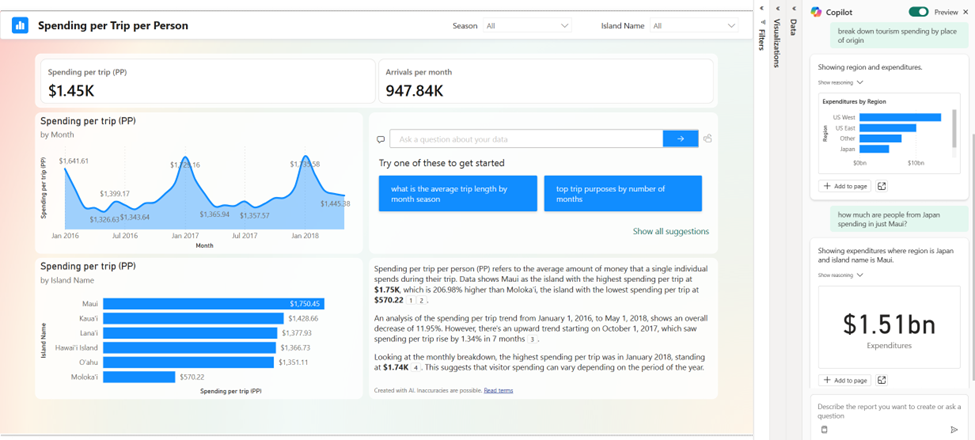
We’re starting this capability off in both Edit and View modes in Power BI Service. Because this is a preview feature, you’ll need to enable it via the preview toggle in the Copilot pane. You can learn more about all the details of the feature in our announcement post here! (will link to announcement post)
We are excited to announce the general availability of DAX query view. DAX query view is the fourth view in Power BI Desktop to run DAX queries on your semantic model.
DAX query view comes with several ways to help you be as productive as possible with DAX queries.
- Quick queries. Have the DAX query written for you from the context menu of tables, columns, or measures in the Data pane of DAX query view. Get the top 100 rows of a table, statistics of a column, or DAX formula of a measure to edit and validate in just a couple clicks!
- DirectQuery model authors can also use DAX query view. View the data in your tables whenever you want!
- Create and edit measures. Edit one or multiple measures at once. Make changes and see the change in action in a DA query. Then update the model when you are ready. All in DAX query view!
- See the DAX query of visuals. Investigate the visuals DAX query in DAX query view. Go to the Performance Analyzer pane and choose “Run in DAX query view”.
- Write DAX queries. You can create DAX queries with Intellisense, formatting, commenting/uncommenting, and syntax highlighting. And additional professional code editing experiences such as “Change all occurrences” and block folding to expand and collapse sections. Even expanded find and replace options with regex.
Learn more about DAX query view with these resources:
- Deep dive blog: https://powerbi.microsoft.com/blog/deep-dive-into-dax-query-view-and-writing-dax-queries/
- Learn more: https://learn.microsoft.com/power-bi/transform-model/dax-query-view
- Video: https://youtu.be/oPGGYLKhTOA?si=YKUp1j8GoHHsqdZo
DAX query view includes an inline Fabric Copilot to write and explain DAX queries, which remains in public preview. This month we have made the following updates.
- Run the DAX query before you keep it . Previously the Run button was disabled until the generated DAX query was accepted or Copilot was closed. Now you can Run the DAX query then decide to Keep or Discard the DAX query.
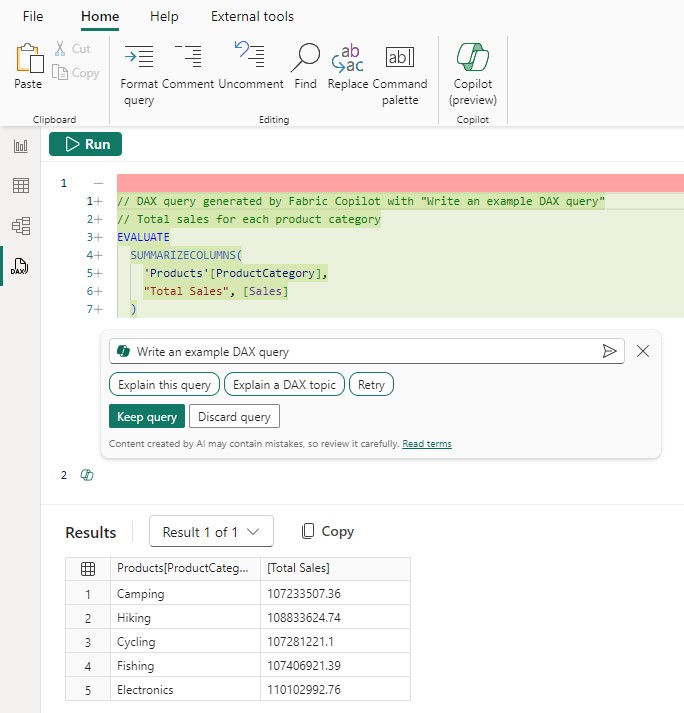
2. Conversationally build the DAX query. Previously the DAX query generated was not considered if you typed additional prompts and you had to keep the DAX query, select it again, then use Copilot again to adjust. Now you can simply adjust by typing in additional user prompts.
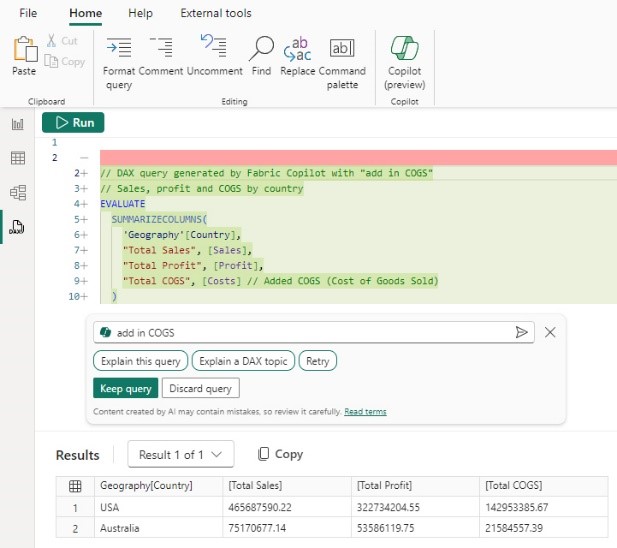
3. Syntax checks on the generated DAX query. Previously there was no syntax check before the generated DAX query was returned. Now the syntax is checked, and the prompt automatically retried once. If the retry is also invalid, the generated DAX query is returned with a note that there is an issue, giving you the option to rephrase your request or fix the generated DAX query.

4. Inspire buttons to get you started with Copilot. Previously nothing happened until a prompt was entered. Now click any of these buttons to quickly see what you can do with Copilot!
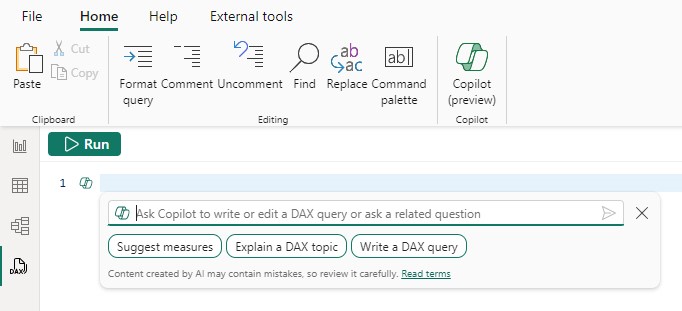
Learn more about DAX queries with Copilot with these resources:
- Deep dive blog: https://powerbi.microsoft.com/en-us/blog/deep-dive-into-dax-query-view-with-copilot/
- Learn more: https://learn.microsoft.com/en-us/dax/dax-copilot
- Video: https://www.youtube.com/watch?v=0kE3TE34oLM
We are excited to introduce you to the redesigned ‘Manage relationships’ dialog in Power BI Desktop! To open this dialog simply select the ‘Manage relationships’ button in the modeling ribbon.

Once opened, you’ll find a comprehensive view of all your relationships, along with their key properties, all in one convenient location. From here you can create new relationships or edit an existing one.
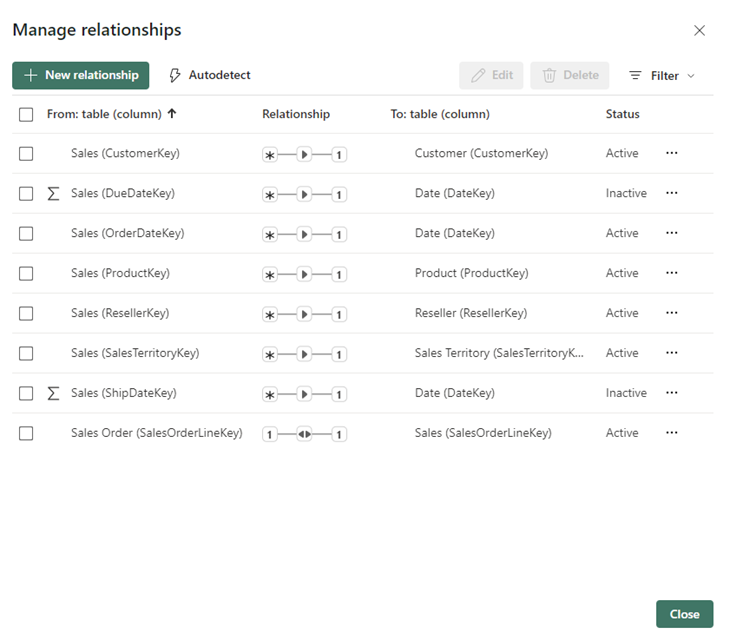
Additionally, you have the option to filter and focus on specific relationships in your model based on cardinality and cross filter direction.
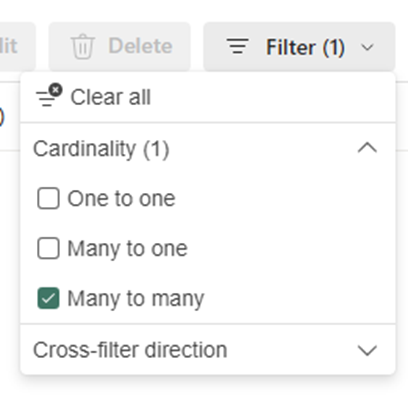
Learn more about creating and managing relationships in Power BI Desktop in our documentation .
Ever since we released composite models on Power BI semantic models and Analysis Services , you have been asking us to support the refresh of calculated columns and tables in the Service. This month, we have enabled the refresh of calculated columns and tables in Service for any DirectQuery source that uses single sign-on authentication. This includes the sources you use when working with composite models on Power BI semantic models and Analysis Services.
Previously, the refresh of a semantic model that uses a DirectQuery source with single-sign-on authentication failed with one of the following error messages: “Refresh is not supported for datasets with a calculated table or calculated column that depends on a table which references Analysis Services using DirectQuery.” or “Refresh over a dataset with a calculated table or a calculated column which references a Direct Query data source is not supported.”
Starting today, you can successfully refresh the calculated table and calculated columns in a semantic model in the Service using specific credentials as long as:
- You used a shareable cloud connection and assigned it and/or.
- Enabled granular access control for all data connection types.
Here’s how to do this:
- Create and publish your semantic model that uses a single sign-on DirectQuery source. This can be a composite model but doesn’t have to be.
- In the semantic model settings, under Gateway and cloud connections , map each single sign-on DirectQuery connection to a specific connection. If you don’t have a specific connection yet, select ‘Create a connection’ to create it:
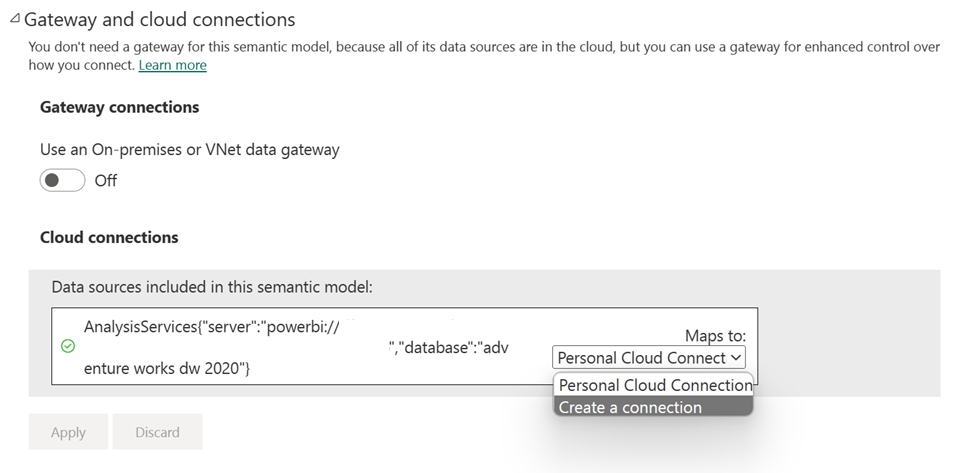
- If you are creating a new connection, fill out the connection details and click Create , making sure to select ‘Use SSO via Azure AD for DirectQuery queries:
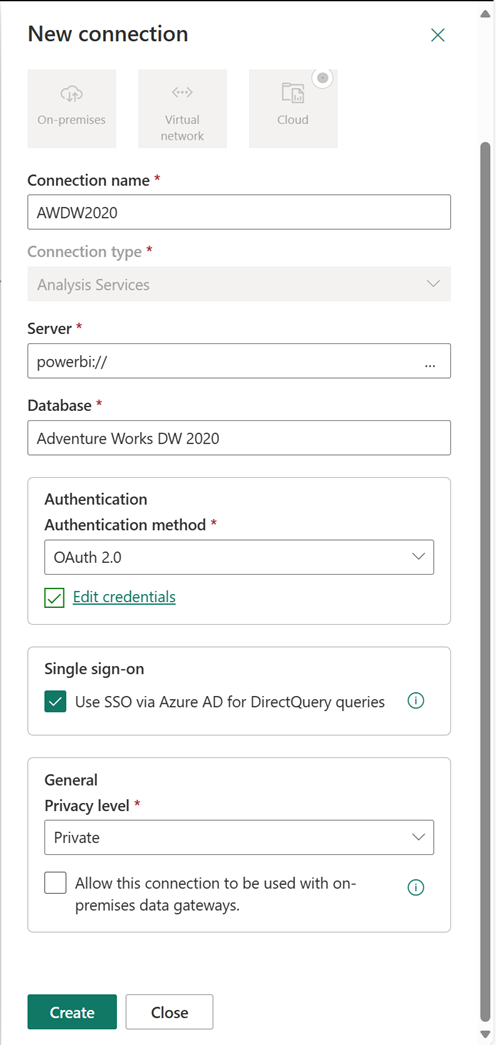
- Finally, select the connection for each single sign-on DirectQuery source and select Apply :
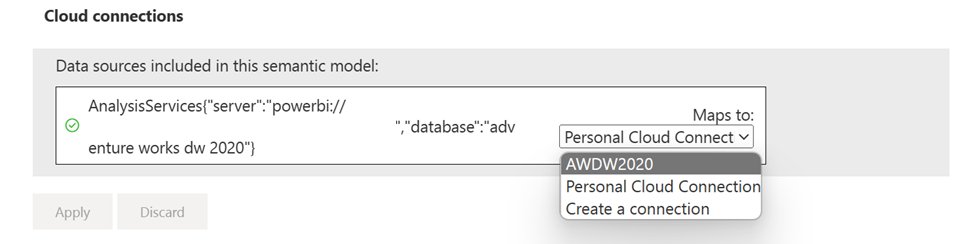
2. Either refresh the semantic model manually or plan a scheduled refresh to confirm the refresh now works successfully. Congratulations, you have successfully set up refresh for semantic models with a single sign-on DirectQuery connection that uses calculated columns or calculated tables!
We are excited to announce the general availability of Model Explorer in the Model view of Power BI, including the authoring of calculation groups. Semantic modeling is even easier with an at-a-glance tree view with item counts, search, and in context paths to edit the semantic model items with Model Explorer. Top level semantic model properties are also available as well as the option to quickly create relationships in the properties pane. Additionally, the styling for the Data pane is updated to Fluent UI also used in Office and Teams.
A popular community request from the Ideas forum, authoring calculation groups is also included in Model Explorer. Calculation groups significantly reduce the number of redundant measures by allowing you to define DAX formulas as calculation items that can be applied to existing measures. For example, define a year over year, prior month, conversion, or whatever your report needs in DAX formula once as a calculation item and reuse it with existing measures. This can reduce the number of measures you need to create and make the maintenance of the business logic simpler.
Available in both Power BI Desktop and when editing a semantic model in the workspace, take your semantic model authoring to the next level today!
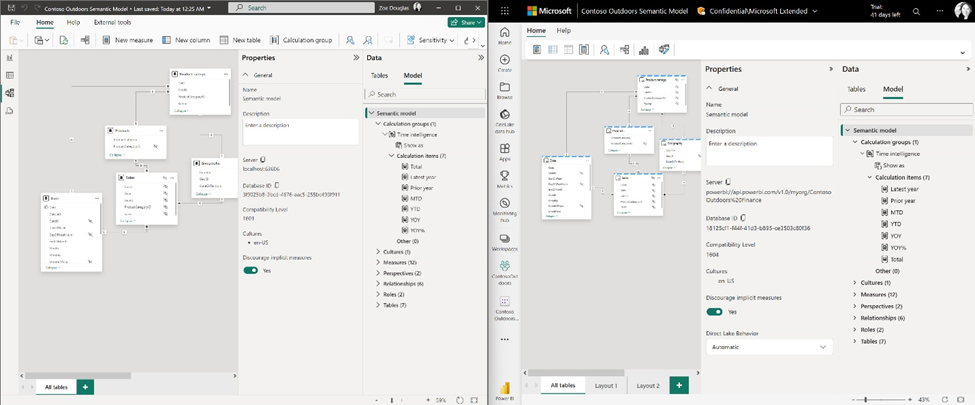
Learn more about Model Explorer and authoring calculation groups with these resources:
- Use Model explorer in Power BI (preview) – Power BI | Microsoft Learn
- Create calculation groups in Power BI (preview) – Power BI | Microsoft Learn
Data connectivity
We’re happy to announce that the Oracle database connector has been enhanced this month with the addition of Single Sign-On support in the Power BI service with Microsoft Entra ID authentication.
Microsoft Entra ID SSO enables single sign-on to access data sources that rely on Microsoft Entra ID based authentication. When you configure Microsoft Entra SSO for an applicable data source, queries run under the Microsoft Entra identity of the user that interacts with the Power BI report.
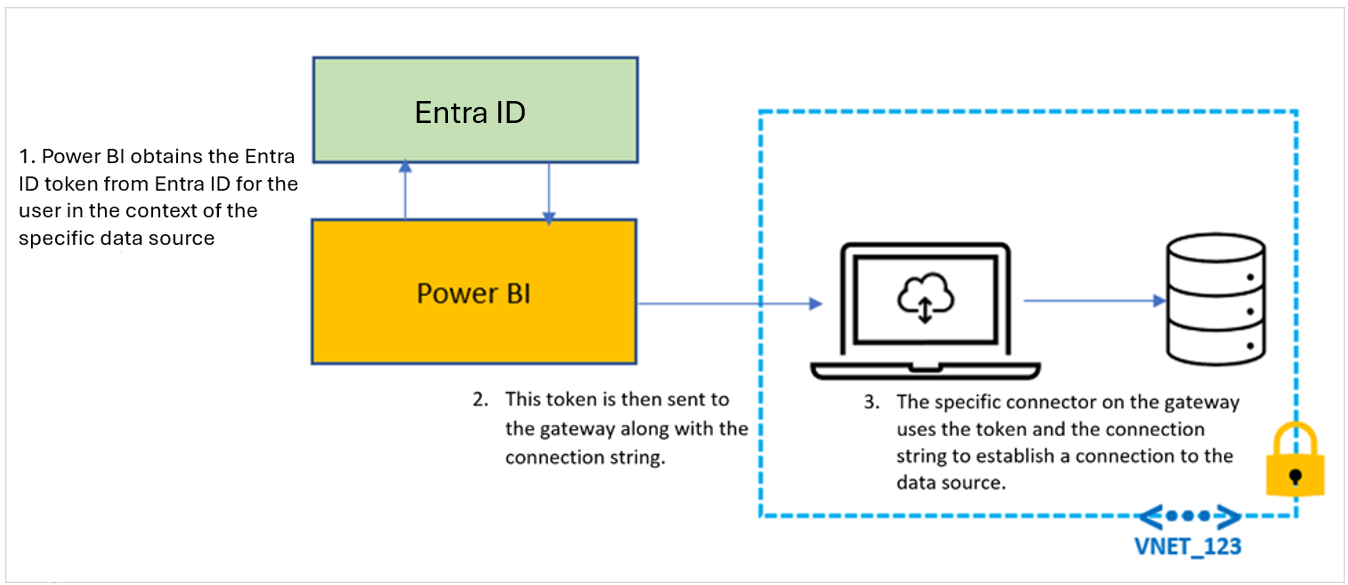
We’re pleased to announce the new and updated connectors in this release:
- [New] OneStream : The OneStream Power BI Connector enables you to seamlessly connect Power BI to your OneStream applications by simply logging in with your OneStream credentials. The connector uses your OneStream security, allowing you to access only the data you have based on your permissions within the OneStream application. Use the connector to pull cube and relational data along with metadata members, including all their properties. Visit OneStream Power BI Connector to learn more. Find this connector in the other category.
- [New] Zendesk Data : A new connector developed by the Zendesk team that aims to go beyond the functionality of the existing Zendesk legacy connector created by Microsoft. Learn more about what this new connector brings.
- [New] CCH Tagetik
- [Update] Azure Databricks
Are you interested in creating your own connector and publishing it for your customers? Learn more about the Power Query SDK and the Connector Certification program .
Last May, we announced the integration between Power BI and OneDrive and SharePoint. Previously, this capability was limited to only reports with data in import mode. We’re excited to announce that you can now seamlessly view Power BI reports with live connected data directly in OneDrive and SharePoint!
When working on Power BI Desktop with a report live connected to a semantic model in the service, you can easily share a link to collaborate with others on your team and allow them to quickly view the report in their browser. We’ve made it easier than ever to access the latest data updates without ever leaving your familiar OneDrive and SharePoint environments. This integration streamlines your workflows and allows you to access reports within the platforms you already use. With collaboration at the heart of this improvement, teams can work together more effectively to make informed decisions by leveraging live connected semantic models without being limited to data only in import mode.
Utilizing OneDrive and SharePoint allows you to take advantage of built-in version control, always have your files available in the cloud, and utilize familiar and simplistic sharing.
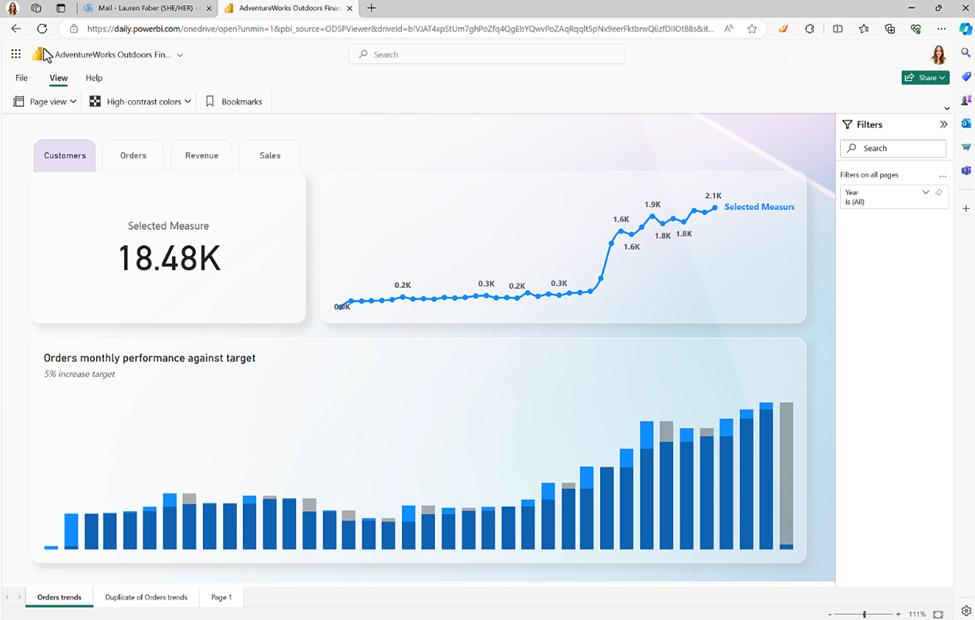
While you told us that you appreciate the ability to limit the image view to only those who have permission to view the report, you asked for changes for the “Public snapshot” mode.
To address some of the feedback we got from you, we have made a few more changes in this area.
- Add-ins that were saved as “Public snapshot” can be printed and will not require that you go over all the slides and load the add-ins for permission check before the public image is made visible.
- You can use the “Show as saved image” on add-ins that were saved as “Public snapshot”. This will replace the entire add-in with an image representation of it, so the load time might be faster when you are presenting your presentation.
Many of us keep presentations open for a long time, which might cause the data in the presentation to become outdated.
To make sure you have in your slides the data you need, we added a new notification that tells you if more up to date data exists in Power BI and offers you the option to refresh and get the latest data from Power BI.
Developers
Direct Lake semantic models are now supported in Fabric Git Integration , enabling streamlined version control, enhanced collaboration among developers, and the establishment of CI/CD pipelines for your semantic models using Direct Lake.
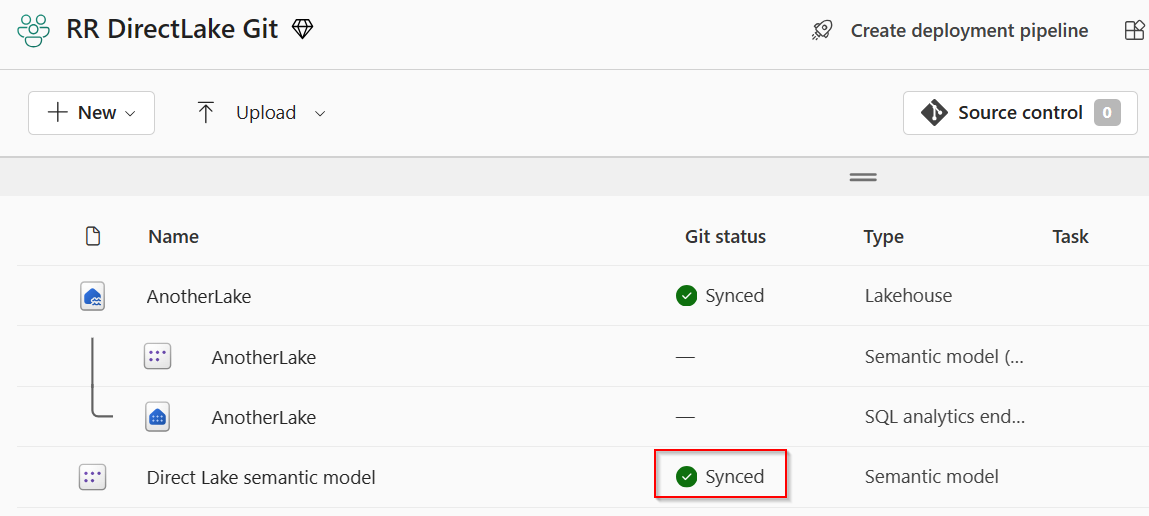
Learn more about version control, testing, and deployment of Power BI content in our Power BI implementation planning documentation: https://learn.microsoft.com/power-bi/guidance/powerbi-implementation-planning-content-lifecycle-management-overview
Visualizations
Editor’s pick of the quarter .
– Animator for Power BI Innofalls Charts SuperTables Sankey Diagram for Power BI by ChartExpo Dynamic KPI Card by Sereviso Shielded HTML Viewer Text search slicer
New visuals in AppSource
Mapa Polski – Województwa, Powiaty, Gminy Workstream Income Statement Table
Gas Detection Chart
Seasonality Chart PlanIn BI – Data Refresh Service
Chart Flare
PictoBar ProgBar
Counter Calendar Donut Chart image
Financial Reporting Matrix by Profitbase
Making financial statements with a proper layout has just become easier with the latest version of the Financial Reporting Matrix.
Users are now able to specify which rows should be classified as cost-rows, which will make it easier to get the conditional formatting of variances correctly:
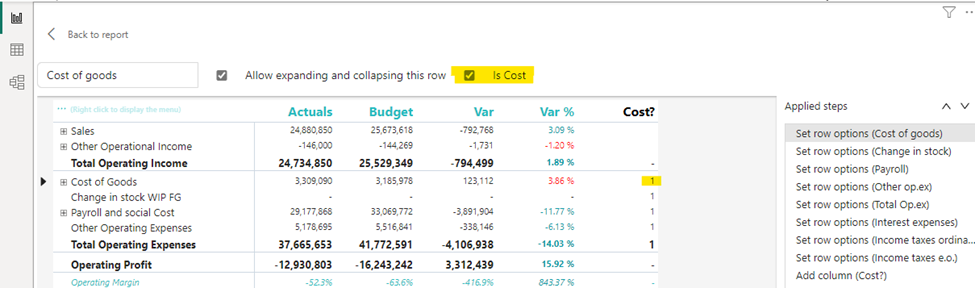
Selecting a row, and ticking “is cost” will tag the row as cost. This can be used in conditional formatting to make sure that positive variances on expenses are a bad for the result, while a positive variance on an income row is good for the result.
The new version also includes more flexibility in measuring placement and column subtotals.
Measures can be placed either:
- Default (below column headers)
- Above column headers
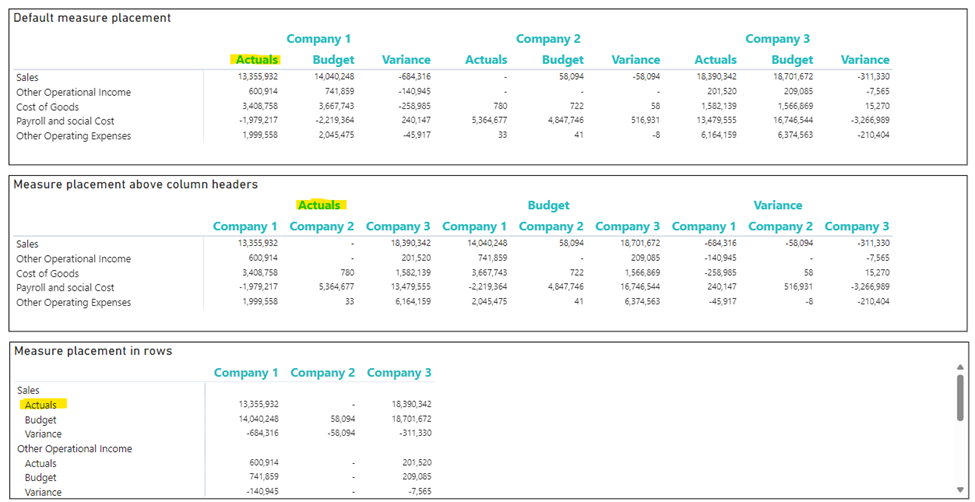
- Conditionally hide columns
- + much more
Highlighted new features:
- Measure placement – In rows
- Select Column Subtotals
- New Format Pane design
- Row Options
Get the visual from AppSource and find more videos here !
Horizon Chart by Powerviz
A Horizon Chart is an advanced visual, for time-series data, revealing trends and anomalies. It displays stacked data layers, allowing users to compare multiple categories while maintaining data clarity. Horizon Charts are particularly useful to monitor and analyze complex data over time, making this a valuable visual for data analysis and decision-making.
Key Features:
- Horizon Styles: Choose Natural, Linear, or Step with adjustable scaling.
- Layer: Layer data by range or custom criteria. Display positive and negative values together or separately on top.
- Reference Line : Highlight patterns with X-axis lines and labels.
- Colors: Apply 30+ color palettes and use FX rules for dynamic coloring.
- Ranking: Filter Top/Bottom N values, with “Others”.
- Gridline: Add gridlines to the X and Y axis.
- Custom Tooltip: Add highest, lowest, mean, and median points without additional DAX.
- Themes: Save designs and share seamlessly with JSON files.
Other features included are ranking, annotation, grid view, show condition, and accessibility support.
Business Use Cases: Time-Series Data Comparison, Environmental Monitoring, Anomaly Detection
🔗 Try Horizon Chart for FREE from AppSource
📊 Check out all features of the visual: Demo file
📃 Step-by-step instructions: Documentation
💡 YouTube Video: Video Link
📍 Learn more about visuals: https://powerviz.ai/
✅ Follow Powerviz : https://lnkd.in/gN_9Sa6U
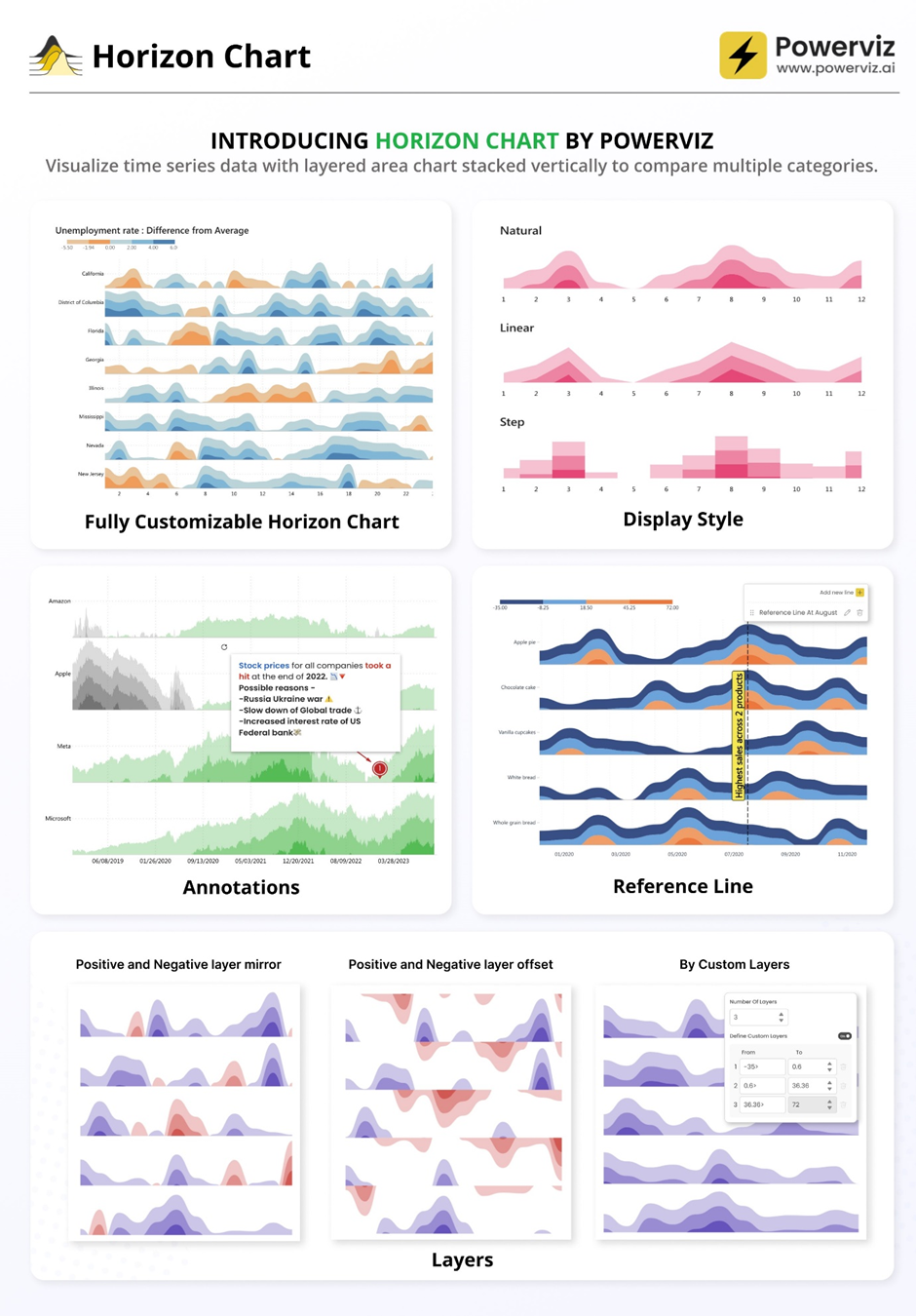
Exciting news! Thanks to your valuable feedback, we’ve enhanced our Milestone Trend Analysis Chart even further. We’re thrilled to announce that you can now switch between horizontal and vertical orientations, catering to your preferred visualization style.
The Milestone Trend Analysis (MTA) Chart remains your go-to tool for swiftly identifying deadline trends, empowering you to take timely corrective actions. With this update, we aim to enhance deadline awareness among project participants and stakeholders alike.
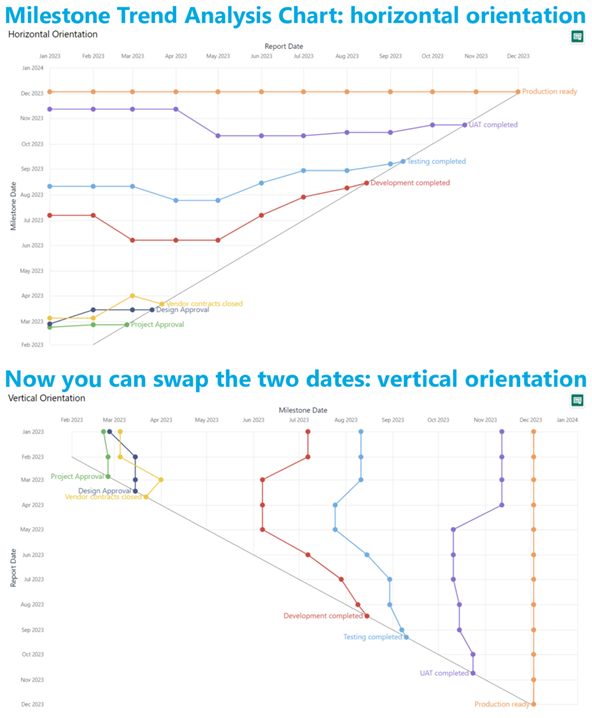
In our latest version, we seamlessly navigate between horizontal and vertical views within the familiar Power BI interface. No need to adapt to a new user interface – enjoy the same ease of use with added flexibility. Plus, it benefits from supported features like themes, interactive selection, and tooltips.
What’s more, ours is the only Microsoft Certified Milestone Trend Analysis Chart for Power BI, ensuring reliability and compatibility with the platform.
Ready to experience the enhanced Milestone Trend Analysis Chart? Download it from AppSource today and explore its capabilities with your own data – try for free!
We welcome any questions or feedback at our website: https://visuals.novasilva.com/ . Try it out and elevate your project management insights now!
Sunburst Chart by Powerviz
Powerviz’s Sunburst Chart is an interactive tool for hierarchical data visualization. With this chart, you can easily visualize multiple columns in a hierarchy and uncover valuable insights. The concentric circle design helps in displaying part-to-whole relationships.
- Arc Customization: Customize shapes and patterns.
- Color Scheme: Accessible palettes with 30+ options.
- Centre Circle: Design an inner circle with layers. Add text, measure, icons, and images.
- Conditional Formatting: Easily identify outliers based on measure or category rules.
- Labels: Smart data labels for readability.
- Image Labels: Add an image as an outer label.
- Interactivity: Zoom, drill down, cross-filtering, and tooltip features.
Other features included are annotation, grid view, show condition, and accessibility support.
Business Use Cases:
- Sales and Marketing: Market share analysis and customer segmentation.
- Finance : Department budgets and expenditures distribution.
- Operations : Supply chain management.
- Education : Course structure, curriculum creation.
- Human Resources : Organization structure, employee demographics.
🔗 Try Sunburst Chart for FREE from AppSource
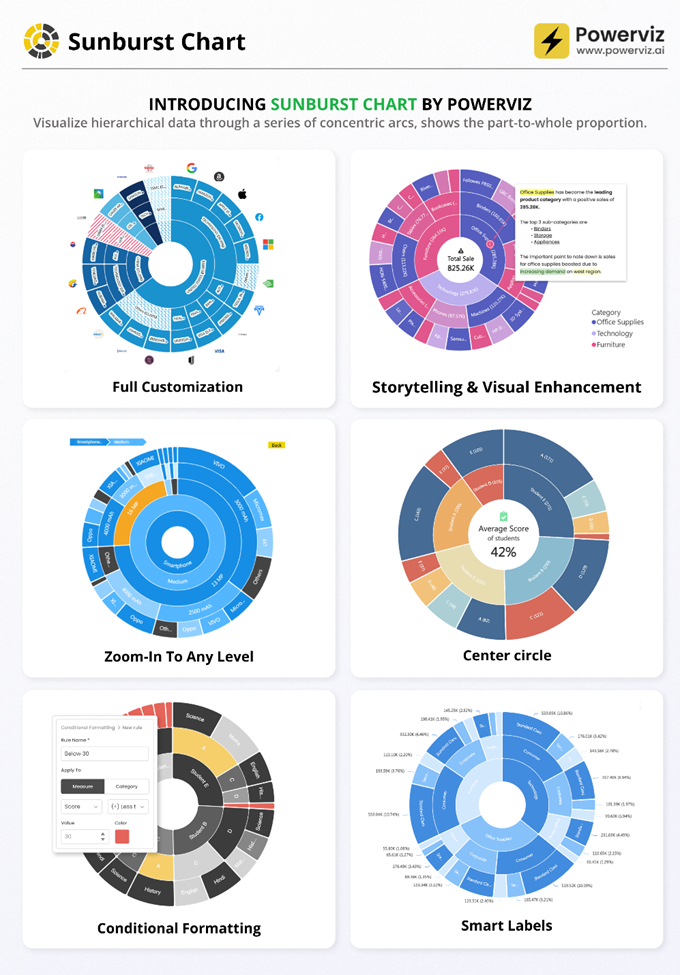
Stacked Bar Chart with Line by JTA
Clustered bar chart with the possibility to stack one of the bars
Stacked Bar Chart with Line by JTA seamlessly merges the simplicity of a traditional bar chart with the versatility of a stacked bar, revolutionizing the way you showcase multiple datasets in a single, cohesive display.
Unlocking a new dimension of insight, our visual features a dynamic line that provides a snapshot of data trends at a glance. Navigate through your data effortlessly with multiple configurations, gaining a swift and comprehensive understanding of your information.
Tailor your visual experience with an array of functionalities and customization options, enabling you to effortlessly compare a primary metric with the performance of an entire set. The flexibility to customize the visual according to your unique preferences empowers you to harness the full potential of your data.
Features of Stacked Bar Chart with Line:
- Stack the second bar
- Format the Axis and Gridlines
- Add a legend
- Format the colors and text
- Add a line chart
- Format the line
- Add marks to the line
- Format the labels for bars and line
If you liked what you saw, you can try it for yourself and find more information here . Also, if you want to download it, you can find the visual package on the AppSource .
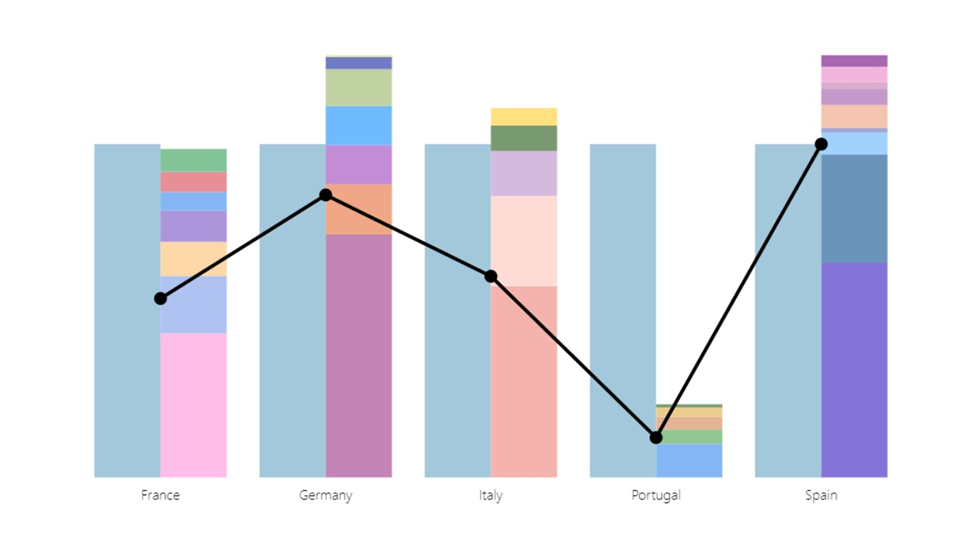
We have added an exciting new feature to our Combo PRO, Combo Bar PRO, and Timeline PRO visuals – Legend field support . The Legend field makes it easy to visually split series values into smaller segments, without the need to use measures or create separate series. Simply add a column with category names that are adjacent to the series values, and the visual will do the following:
- Display separate segments as a stack or cluster, showing how each segment contributed to the total Series value.
- Create legend items for each segment to quickly show/hide them without filtering.
- Apply custom fill colors to each segment.
- Show each segment value in the tooltip
Read more about the Legend field on our blog article
Drill Down Combo PRO is made for creators who want to build visually stunning and user-friendly reports. Cross-chart filtering and intuitive drill down interactions make data exploration easy and fun for any user. Furthermore, you can choose between three chart types – columns, lines, or areas; and feature up to 25 different series in the same visual and configure each series independently.
📊 Get Drill Down Combo PRO on AppSource
🌐 Visit Drill Down Combo PRO product page
Documentation | ZoomCharts Website | Follow ZoomCharts on LinkedIn
We are thrilled to announce that Fabric Core REST APIs are now generally available! This marks a significant milestone in the evolution of Microsoft Fabric, a platform that has been meticulously designed to empower developers and businesses alike with a comprehensive suite of tools and services.
The Core REST APIs are the backbone of Microsoft Fabric, providing the essential building blocks for a myriad of functionalities within the platform. They are designed to improve efficiency, reduce manual effort, increase accuracy, and lead to faster processing times. These APIs help with scale operations more easily and efficiently as the volume of work grows, automate repeatable processes with consistency, and enable integration with other systems and applications, providing a streamlined and efficient data pipeline.
The Microsoft Fabric Core APIs encompasses a range of functionalities, including:
- Workspace management: APIs to manage workspaces, including permissions.
- Item management: APIs for creating, reading, updating, and deleting items, with partial support for data source discovery and granular permissions management planned for the near future.
- Job and tenant management: APIs to manage jobs, tenants, and users within the platform.
These APIs adhere to industry standards and best practices, ensuring a unified developer experience that is both coherent and easy to use.
For developers looking to dive into the details of the Microsoft Fabric Core APIs, comprehensive documentation is available. This includes guidelines on API usage, examples, and articles managed in a centralized repository for ease of access and discoverability. The documentation is continuously updated to reflect the latest features and improvements, ensuring that developers have the most current information at their fingertips. See Microsoft Fabric REST API documentation
We’re excited to share an important update we made to the Fabric Admin APIs. This enhancement is designed to simplify your automation experience. Now, you can manage both Power BI and the new Fabric items (previously referred to as artifacts) using the same set of APIs. Before this enhancement, you had to navigate using two different APIs—one for Power BI items and another for new Fabric items. That’s no longer the case.
The APIs we’ve updated include GetItem , ListItems , GetItemAccessDetails , and GetAccessEntities . These enhancements mean you can now query and manage all your items through a single API call, regardless of whether they’re Fabric types or Power BI types. We hope this update makes your work more straightforward and helps you accomplish your tasks more efficiently.
We’re thrilled to announce the public preview of the Microsoft Fabric workload development kit. This feature now extends to additional workloads and offers a robust developer toolkit for designing, developing, and interoperating with Microsoft Fabric using frontend SDKs and backend REST APIs. Introducing the Microsoft Fabric Workload Development Kit .
The Microsoft Fabric platform now provides a mechanism for ISVs and developers to integrate their new and existing applications natively into Fabric’s workload hub. This integration provides the ability to add net new capabilities to Fabric in a consistent experience without leaving their Fabric workspace, thereby accelerating data driven outcomes from Microsoft Fabric.
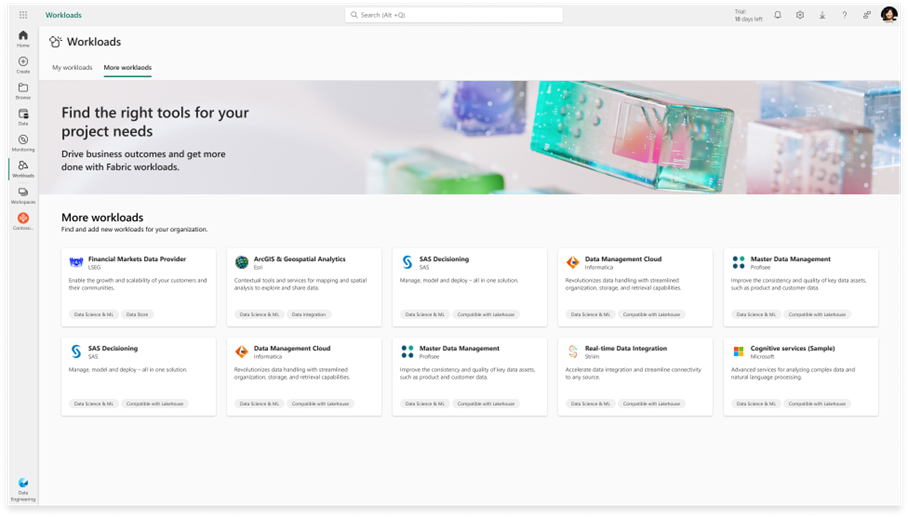
By downloading and leveraging the development kit , ISVs and software developers can build and scale existing and new applications on Microsoft Fabric and offer them via the Azure Marketplace without the need to ever leave the Fabric environment.
The development kit provides a comprehensive guide and sample code for creating custom item types that can be added to the Fabric workspace. These item types can leverage the Fabric frontend SDKs and backend REST APIs to interact with other Fabric capabilities, such as data ingestion, transformation, orchestration, visualization, and collaboration. You can also embed your own data application into the Fabric item editor using the Fabric native experience components, such as the header, toolbar, navigation pane, and status bar. This way, you can offer consistent and seamless user experience across different Fabric workloads.
This is a call to action for ISVs, software developers, and system integrators. Let’s leverage this opportunity to create more integrated and seamless experiences for our users.
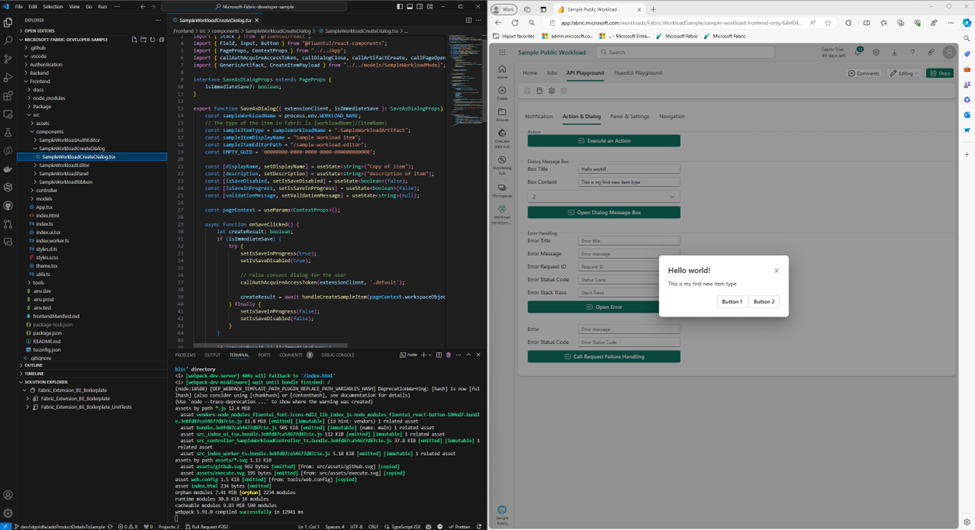
We’re excited about this journey and look forward to seeing the innovative workloads from our developer community.
We are proud to announce the public preview of external data sharing. Sharing data across organizations has become a standard part of day-to-day business for many of our customers. External data sharing, built on top of OneLake shortcuts, enables seamless, in-place sharing of data, allowing you to maintain a single copy of data even when sharing data across tenant boundaries. Whether you’re sharing data with customers, manufacturers, suppliers, consultants, or partners; the applications are endless.
How external data sharing works
Sharing data across tenants is as simple as any other share operation in Fabric. To share data, navigate to the item to be shared, click on the context menu, and then click on External data share . Select the folder or table you want to share and click Save and continue . Enter the email address and an optional message and then click Send .
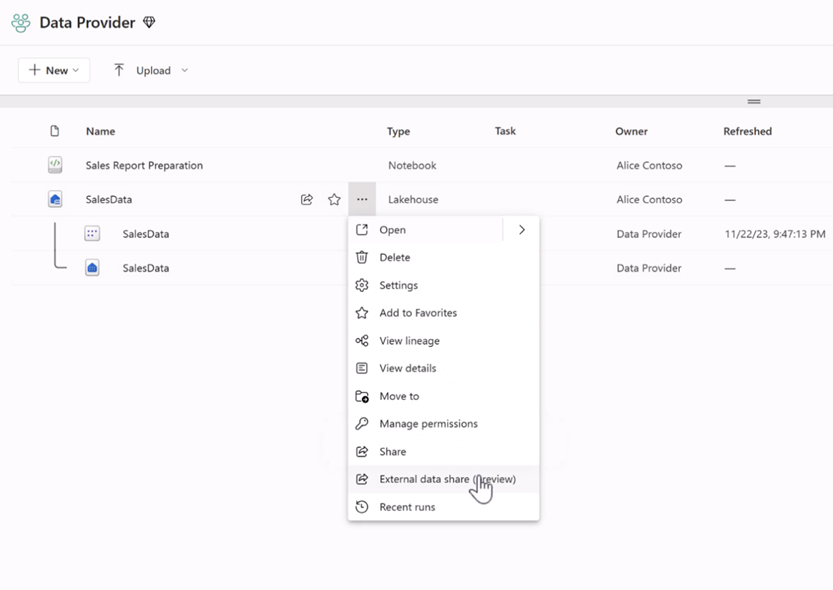
The data consumer will receive an email containing a share link. They can click on the link to accept the share and access the data within their own tenant.
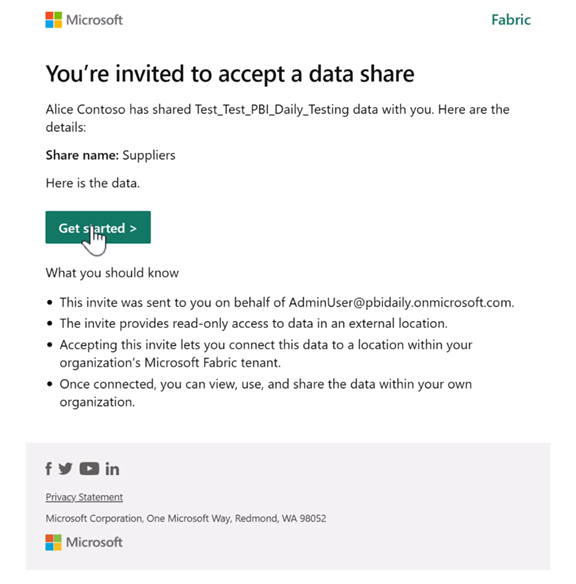
Click here for more details about external data sharing .
Following the release of OneLake data access roles in public preview, the OneLake team is excited to announce the availability of APIs for managing data access roles. These APIs can be used to programmatically manage granular data access for your lakehouses. Manage all aspects of role management such as creating new roles, editing existing ones, or changing memberships in a programmatic way.
Check out the API reference to learn more.
Do you have data stored on-premises or behind a firewall that you want to access and analyze with Microsoft Fabric? With OneLake shortcuts, you can bring on-premises or network-restricted data into OneLake, without any data movement or duplication. Simply install the Fabric on-premises data gateway and create a shortcut to your S3 compatible, Amazon S3, or Google Cloud Storage data source. Then use any of Fabric’s powerful analytics engines and OneLake open APIs to explore, transform, and visualize your data in the cloud.
Try it out today and unlock the full potential of your data with OneLake shortcuts!
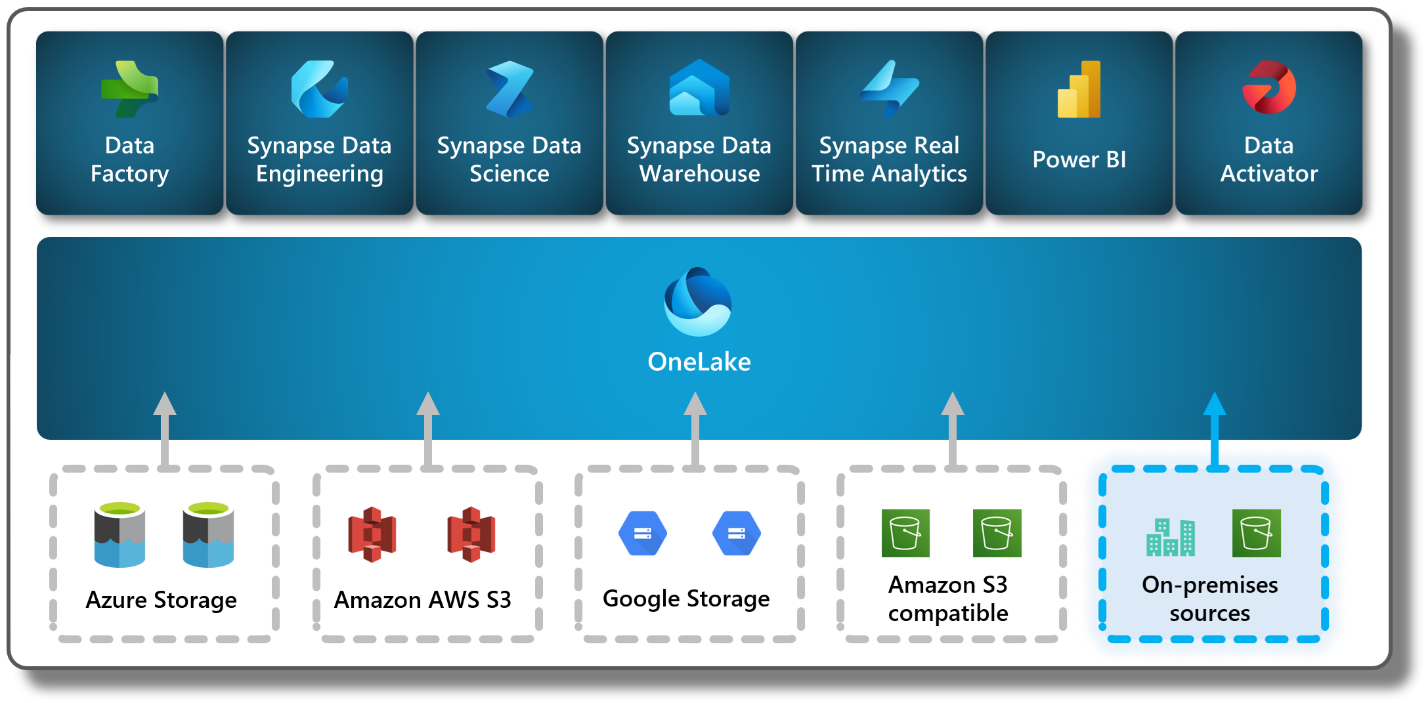
Data Warehouse
We are excited to announce Copilot for Data Warehouse in public preview! Copilot for Data Warehouse is an AI assistant that helps developers generate insights through T-SQL exploratory analysis. Copilot is contextualized your warehouse’s schema. With this feature, data engineers and data analysts can use Copilot to:
- Generate T-SQL queries for data analysis.
- Explain and add in-line code comments for existing T-SQL queries.
- Fix broken T-SQL code.
- Receive answers regarding general data warehousing tasks and operations.
There are 3 areas where Copilot is surfaced in the Data Warehouse SQL Query Editor:
- Code completions when writing a T-SQL query.
- Chat panel to interact with the Copilot in natural language.
- Quick action buttons to fix and explain T-SQL queries.
Learn more about Copilot for Data Warehouse: aka.ms/data-warehouse-copilot-docs. Copilot for Data Warehouse is currently only available in the Warehouse. Copilot in the SQL analytics endpoint is coming soon.
As data volumes continue to grow in today’s rapidly evolving world of Artificial Intelligence, it is crucial to reflect on historical data. It empowers businesses to derive valuable insights that aid in making well-informed decisions for the future. Preserving multiple historical data versions not only incurred significant costs but also presented challenges in upholding data integrity, resulting in a notable impact on query performance. So, we are thrilled to announce the ability to query the historical data through time travel at the T-SQL statement level which helps unlock the evolution of data over time.
The Fabric warehouse retains historical versions of tables for seven calendar days. This retention allows for querying the tables as if they existed at any point within the retention timeframe. Time travel clause can be included in any top level SELECT statement. For complex queries that involve multiple tables, joins, stored procedures, or views, the timestamp is applied just once for the entire query instead of specifying the same timestamp for each table within the same query. This ensures the entire query is executed with reference to the specified timestamp, maintaining the data’s uniformity and integrity throughout the query execution.
From historical trend analysis and forecasting to compliance management, stable reporting and real-time decision support, the benefits of time travel extend across multiple business operations. Embrace the capability of time travel to navigate the data-driven landscape and gain a competitive edge in today’s fast-paced world of Artificial Intelligence.
We are excited to announce not one but two new enhancements to the Copy Into feature for Fabric Warehouse: Copy Into with Entra ID Authentication and Copy Into for Firewall-Enabled Storage!
Entra ID Authentication
When authenticating storage accounts in your environment, the executing user’s Entra ID will now be used by default. This ensures that you can leverage A ccess C ontrol L ists and R ole – B ased a ccess c ontrol to authenticate to your storage accounts when using Copy Into. Currently, only organizational accounts are supported.
How to Use Entra ID Authentication
- Ensure your Entra ID organizational account has access to the underlying storage and can execute the Copy Into statement on your Fabric Warehouse.
- Run your Copy Into statement without specifying any credentials; the Entra ID organizational account will be used as the default authentication mechanism.
Copy into firewall-enabled storage
The Copy Into for firewall-enabled storage leverages the trusted workspace access functionality ( Trusted workspace access in Microsoft Fabric (preview) – Microsoft Fabric | Microsoft Learn ) to establish a secure and seamless connection between Fabric and your storage accounts. Secure access can be enabled for both blob and ADLS Gen2 storage accounts. Secure access with Copy Into is available for warehouses in workspaces with Fabric Capacities (F64 or higher).
To learn more about Copy into , please refer to COPY INTO (Transact-SQL) – Azure Synapse Analytics and Microsoft Fabric | Microsoft Learn
We are excited to announce the launch of our new feature, Just in Time Database Attachment, which will significantly enhance your first experience, such as when connecting to the Datawarehouse or SQL endpoint or simply opening an item. These actions trigger the workspace resource assignment process, where, among other actions, we attach all necessary metadata of your items, Data warehouses and SQL endpoints, which can be a long process, particularly for workspaces that have a high number of items.
This feature is designed to attach your desired database during the activation process of your workspace, allowing you to execute queries immediately and avoid unnecessary delays. However, all other databases will be attached asynchronously in the background while you are able to execute queries, ensuring a smooth and efficient experience.
Data Engineering
We are advancing Fabric Runtime 1.3 from an Experimental Public Preview to a full Public Preview. Our Apache Spark-based big data execution engine, optimized for both data engineering and science workflows, has been updated and fully integrated into the Fabric platform.
The enhancements in Fabric Runtime 1.3 include the incorporation of Delta Lake 3.1, compatibility with Python 3.11, support for Starter Pools, integration with Environment and library management capabilities. Additionally, Fabric Runtime now enriches the data science experience by supporting the R language and integrating Copilot.
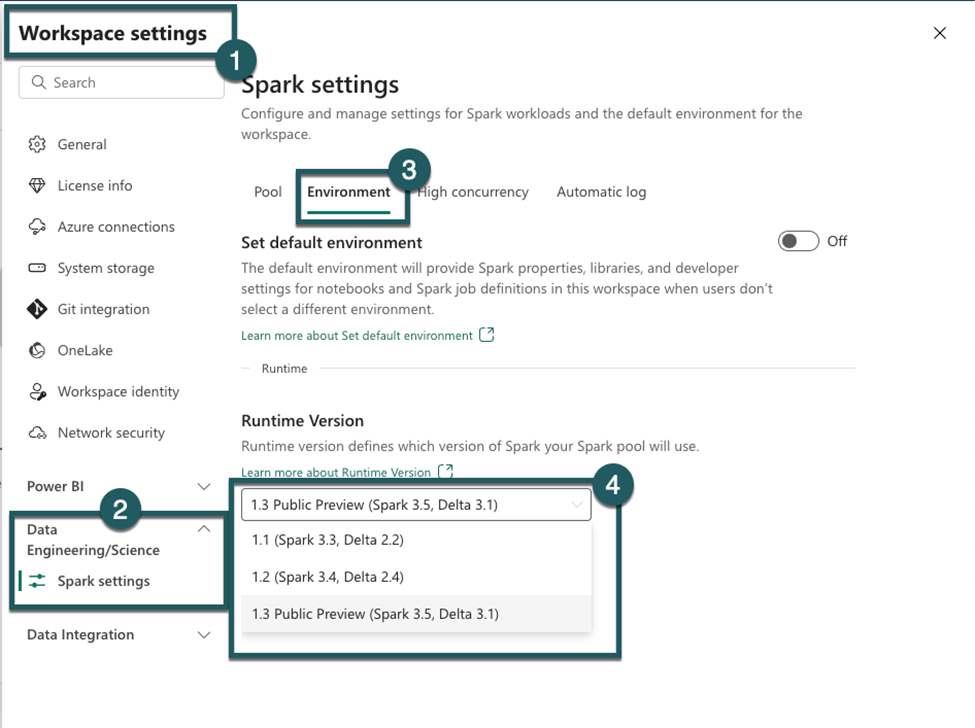
Native Execution Engine for Fabric Runtime 1.2 (Apache Spark 3.4) – Public Preview – eskot
We are pleased to share that the Native Execution Engine for Fabric Runtime 1.2 is currently available in public preview. The Native Execution Engine can greatly enhance the performance for your Spark jobs and queries. The engine has been rewritten in C++ and operates in columnar mode and uses vectorized processing. The Native Execution Engine offers superior query performance – encompassing data processing, ETL, data science, and interactive queries – all directly on your data lake. Overall, Fabric Saprk delivers a 4x speed-up on the sum of execution time of all 99 queries in the TPC-DS 1TB benchmark when compared against Apache Spark. This engine is fully compatible with Apache Spark™ APIs (including Spark SQL API).
It is seamless to use with no code changes – activate it and go. Enable it in your environment for your notebooks and your SJDs.
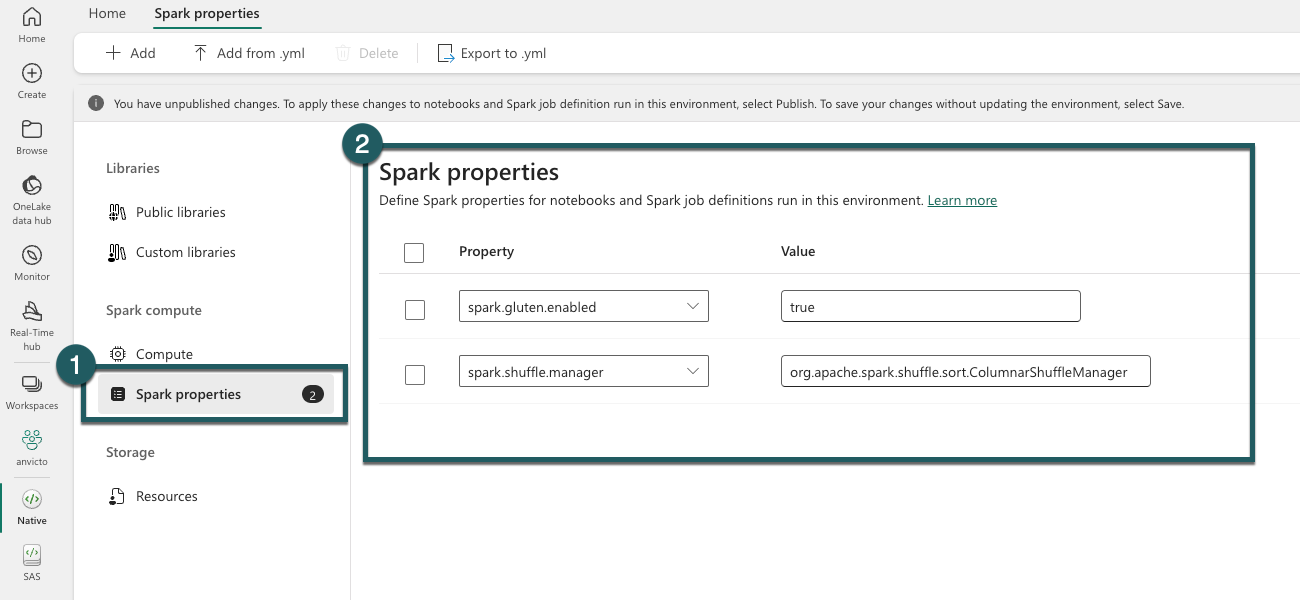
This feature is in the public preview, at this stage of the preview, there is no additional cost associated with using it.
We are excited to announce the Spark Monitoring Run Series Analysis features, which allow you to analyze the run duration trend and performance comparison for Pipeline Spark activity recurring run instances and repetitive Spark run activities from the same Notebook or Spark Job Definition.
- Run Series Comparison: Users can compare the duration of a Notebook run with that of previous runs and evaluate the input and output data to understand the reasons behind prolonged run durations.
- Outlier Detection and Analysis: The system can detect outliers in the run series and analyze them to pinpoint potential contributing factors.
- Detailed Run Instance Analysis: Clicking on a specific run instance provides detailed information on time distribution, which can be used to identify performance enhancement opportunities.
- Configuration Insights : Users can view the Spark configuration used for each run, including auto-tuned configurations for Spark SQL queries in auto-tune enabled Notebook runs.
You can access the new feature from the item’s recent runs panel and Spark application monitoring page.
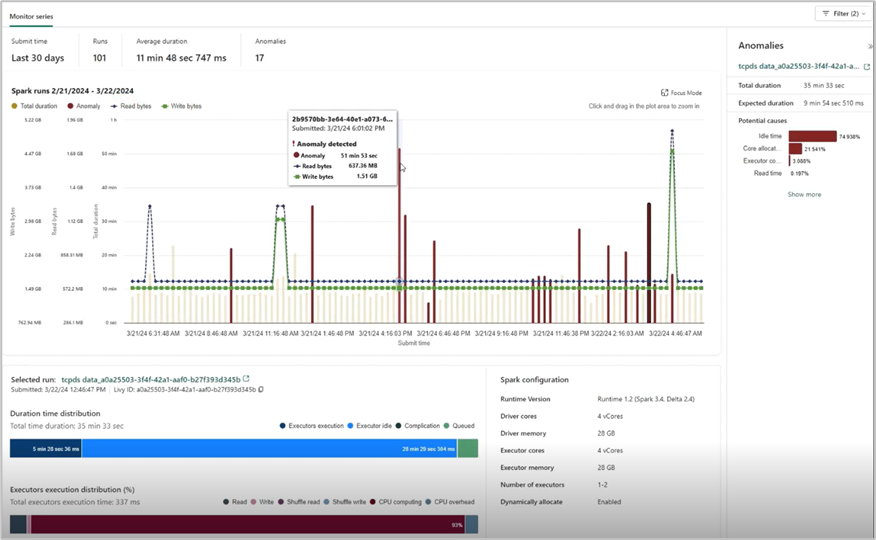
We are excited to announce that Notebook now supports the ability to tag others in comments, just like the familiar functionality of using Office products!
When you select a section of code in a cell, you can add a comment with your insights and tag one or more teammates to collaborate or brainstorm on the specifics. This intuitive enhancement is designed to amplify collaboration in your daily development work.
Moreover, you can easily configure the permissions when tagging someone who doesn’t have the permission, to make sure your code asset is well managed.
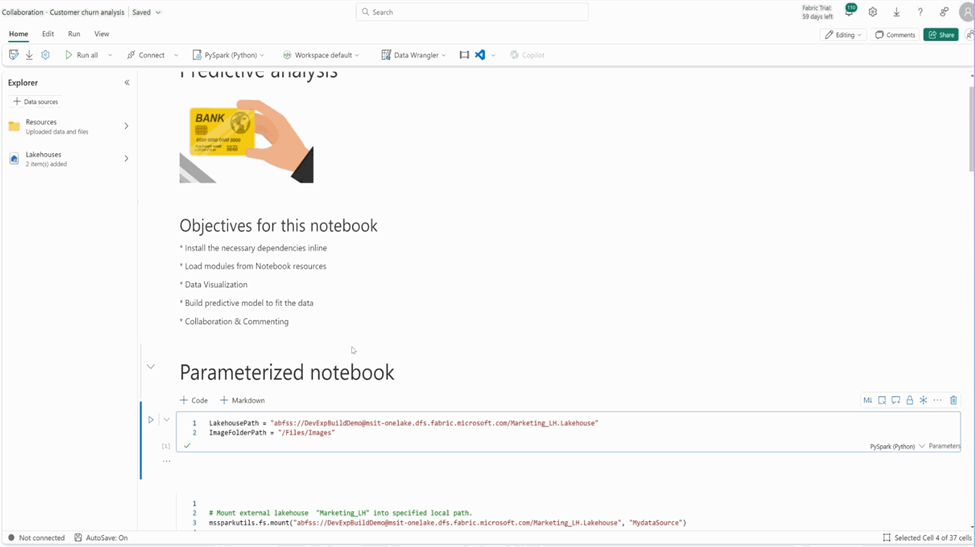
We are thrilled to unveil a significant enhancement to the Fabric notebook ribbon, designed to elevate your data science and engineering workflows.

In the new version, you will find the new Session connect control on the Home tab, and now you can start a standard session without needing to run a code cell.
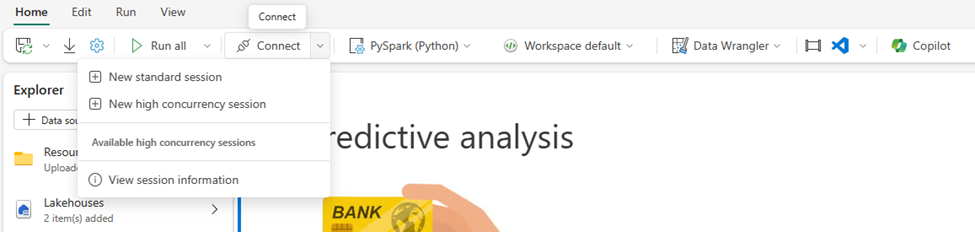
You can also easily spin up a High concurrency session and share the session across multiple notebooks to improve the compute resource utilization. And you can easily attach/leave a high concurrency session with a single click.
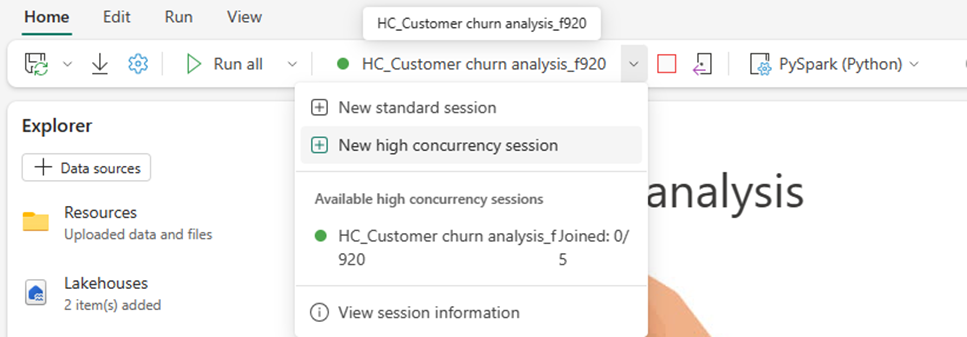
The “ View session information ” can navigate you to the session information dialog, where you can find a lot of useful detailed information, as well as configure the session timeout. The diagnostics info is essentially helpful when you need support for notebook issues.
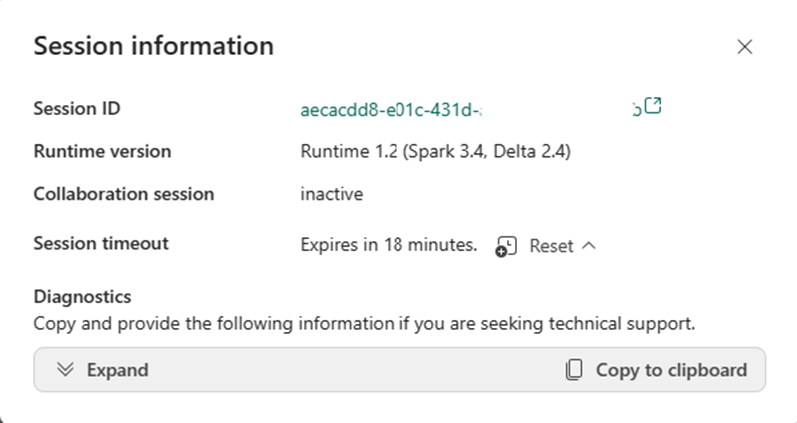
Now you can easily access the powerful “ Data Wrangler ” on Home tab with the new ribbon! You can explore your data with the fancy low-code experience of data wrangler, and the pandas DataFrames and Spark DataFrames are all supported.
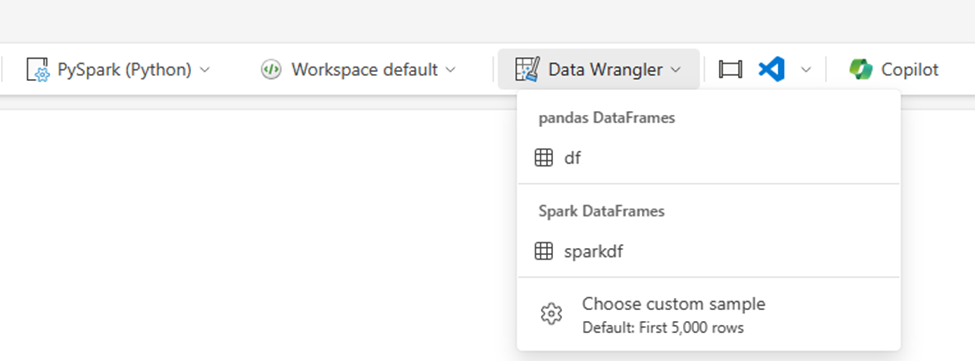
We recently made some changes to the Fabric notebook metadata to ensure compliance and consistency:
Notebook file content:
- The keyword “trident” has been replaced with “dependencies” in the notebook content. This adjustment ensures consistency and compliance.
- Notebook Git format:
- The preface of the notebook has been modified from “# Synapse Analytics notebook source” to “# Fabric notebook source”.
- Additionally, the keyword “synapse” has been updated to “dependencies” in the Git repo.
The above changes will be marked as ‘uncommitted’ for one time if your workspace is connected to Git. No action is needed in terms of these changes , and there won’t be any breaking scenario within the Fabric platform . If you have any further updates or questions, feel free to share with us.
We are thrilled to announce that the environment is now a generally available item in Microsoft Fabric. During this GA timeframe, we have shipped a few new features of Environment.
- Git support
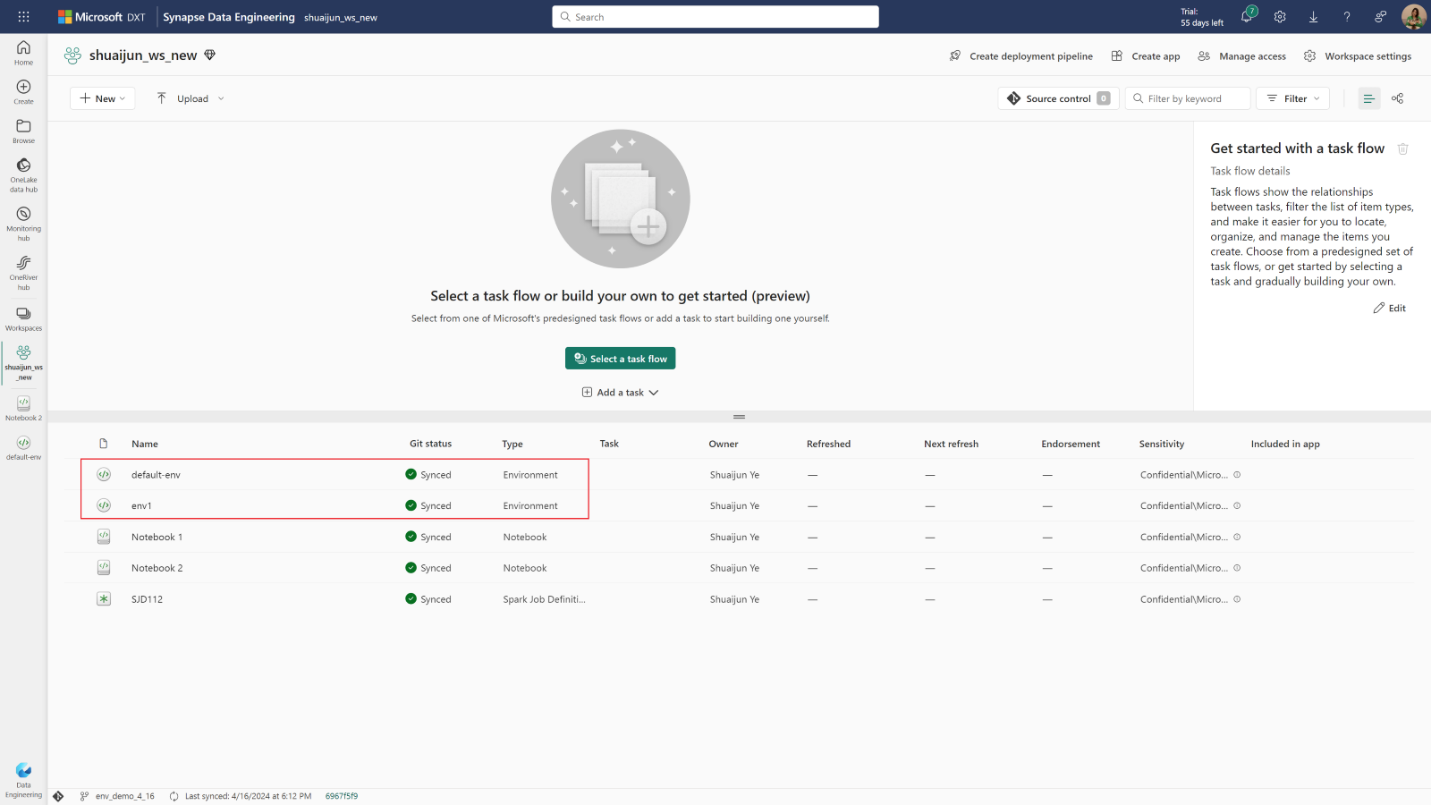
The environment is now Git supported. You can check-in the environment into your Git repo and manipulate the environment locally with its YAML representations and custom library files. After updating the changes from local to Fabric portal, you can publish them by manual action or through REST API.
- Deployment pipeline
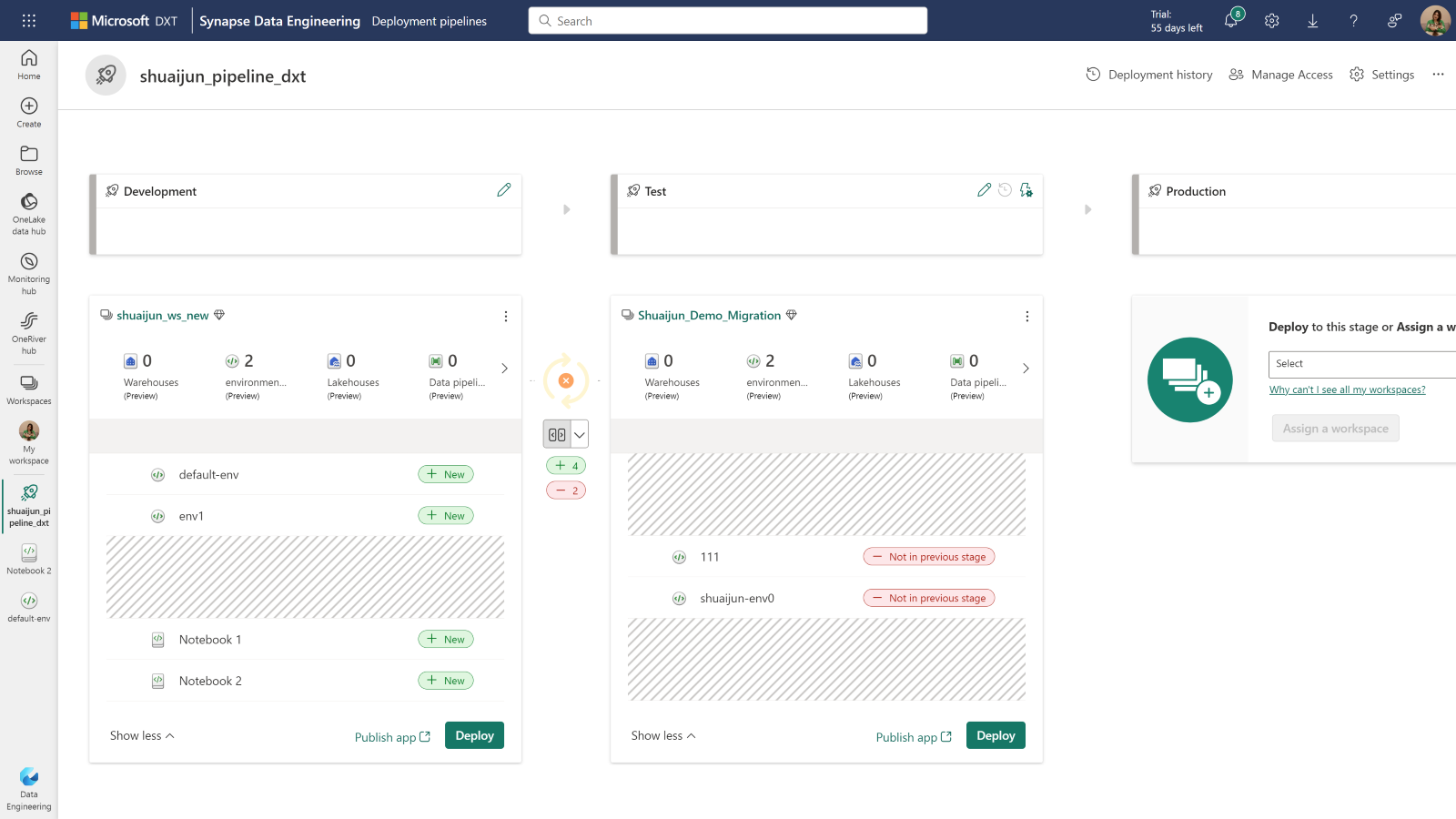
Deploying environments from one workspace to another is supported. Now, you can deploy the code items and their dependent environments together from development to test and even production.
With the REST APIs, you can have the code-first experience with the same abilities through Fabric portal. We provide a set of powerful APIs to ensure you the efficiency in managing your environment. You can create new environments, update libraries and Spark compute, publish the changes, delete an environment, attach the environment to a notebook, etc., all actions can be done locally in the tools of your choice. The article – Best practice of managing environments with REST API could help you get started with several real-world scenarios.
- Resources folder
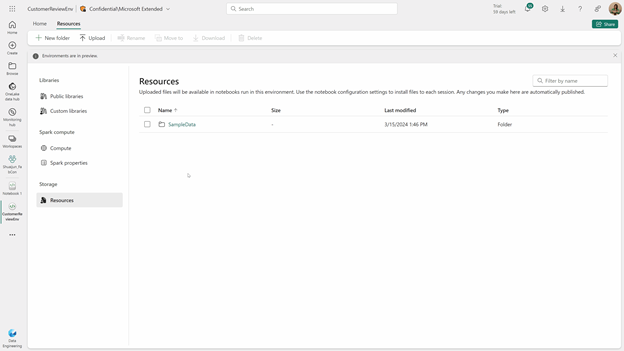
Resources folder enables managing small resources in the development cycle. The files uploaded in the environment can be accessed from notebooks once they’re attached to the same environment. The manipulation of the files and folders of resources happens in real-time. It could be super powerful, especially when you are collaborating with others.
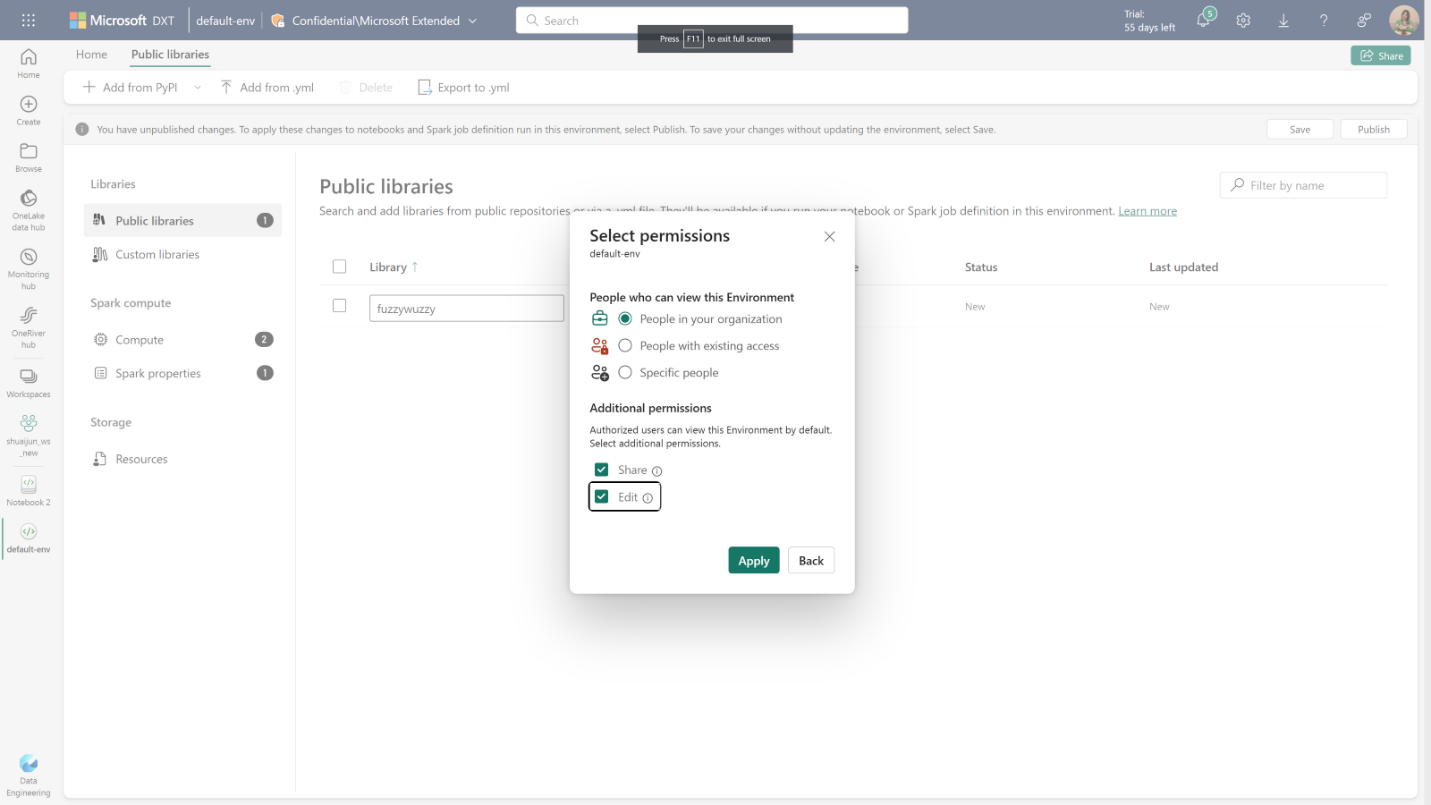
Sharing your environment with others is also available. We provide several sharing options. By default, the view permission is shared. If you want the recipient to have access to view and use the contents of the environment, sharing without permission customization is the best option. Furthermore, you can grant editing permission to allow recipients to update this environment or grant share permission to allow recipients to reshare this environment with their existing permissions.
We are excited to announce the REST api support for Fabric Data Engineering/Science workspace settings. Data Engineering/Science settings allows users to create/manage their Spark compute, select the default runtime/default environment, enable or disable high concurrency mode or ML autologging.
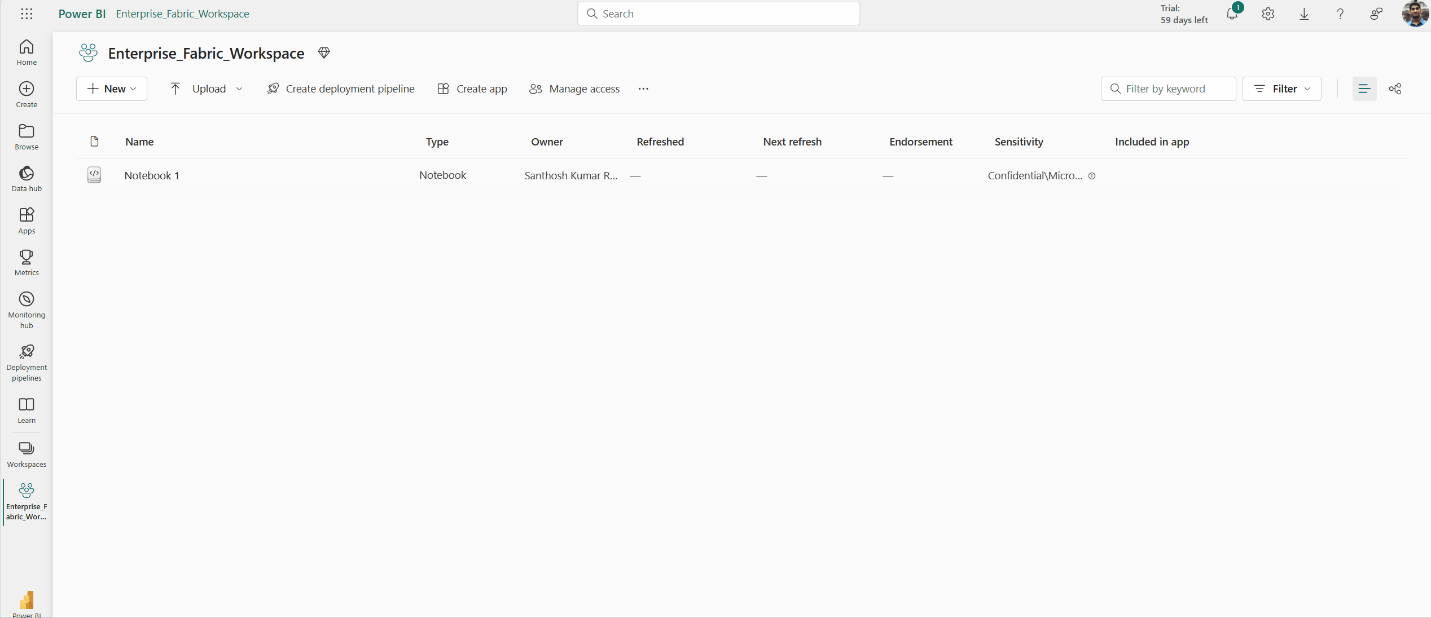
Now with the REST api support for the Data Engineering/Science settings, you would be able to
- Choose the default pool for a Fabric Workspace
- Configure the max nodes for Starter pools
- Create/Update/Delete the existing Custom Pools, Autoscale and Dynamic allocation properties
- Choose Workspace Default Runtime and Environment
- Select a default runtime
- Select the default environment for the Fabric workspace
- Enable or Disable High Concurrency Mode
- Enable or Disable ML Auto logging.
Learn more about the Workspace Spark Settings API in our API documentation Workspace Settings – REST API (Spark) | Microsoft Learn
We are excited to give you a sneak peek at the preview of User Data Functions in Microsoft Fabric. User Data Functions gives developers and data engineers the ability to easily write and run applications that integrate with resources in the Fabric Platform. Data engineering often presents challenges with data quality or complex data analytics processing in data pipelines, and using ETL tools may present limited flexibility and ability to customize to your needs. This is where User data functions can be used to run data transformation tasks and perform complex business logic by connecting to your data sources and other workloads in Fabric.
During preview, you will be able to use the following features:
- Use the Fabric portal to create new User Data Functions, view and test them.
- Write your functions using C#.
- Use the Visual Studio Code extension to create and edit your functions.
- Connect to the following Fabric-native data sources: Data Warehouse, Lakehouse and Mirrored Databases.
You can now create a fully managed GraphQL API in Fabric to interact with your data in a simple, flexible, and powerful way. We’re excited to announce the public preview of API for GraphQL, a data access layer that allows us to query multiple data sources quickly and efficiently in Fabric by leveraging a widely adopted and familiar API technology that returns more data with less client requests. With the new API for GraphQL in Fabric, data engineers and scientists can create data APIs to connect to different data sources, use the APIs in their workflows, or share the API endpoints with app development teams to speed up and streamline data analytics application development in your business.
You can get started with the API for GraphQL in Fabric by creating an API, attaching a supported data source, then selecting specific data sets you want to expose through the API. Fabric builds the GraphQL schema automatically based on your data, you can test and prototype queries directly in our graphical in-browser GraphQL development environment (API editor), and applications are ready to connect in minutes.
Currently, the following supported data sources can be exposed through the Fabric API for GraphQL:
- Microsoft Fabric Data Warehouse
- Microsoft Fabric Lakehouse via SQL Analytics Endpoint
- Microsoft Fabric Mirrored Databases via SQL Analytics Endpoint
Click here to learn more about how to get started.
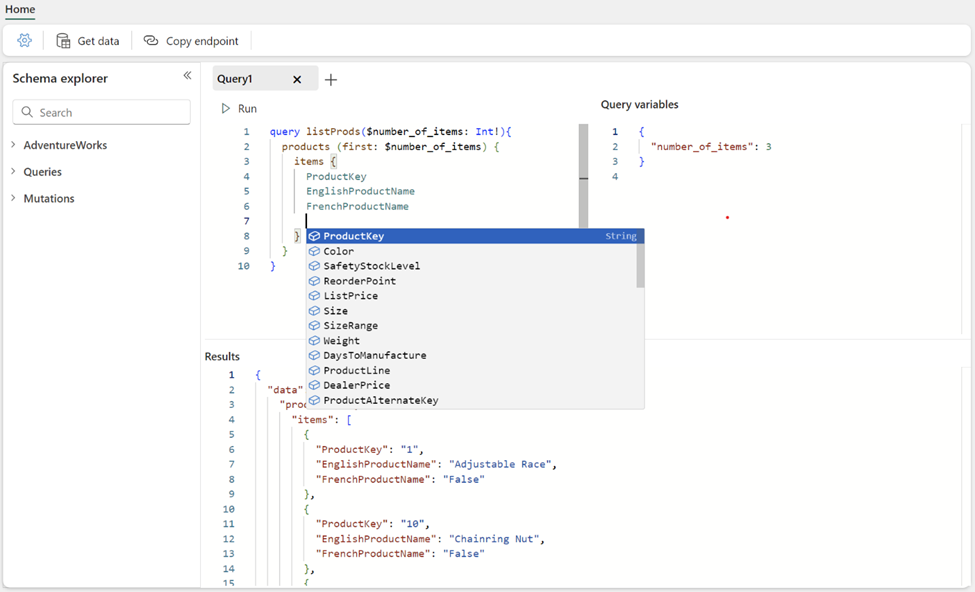
Data Science
As you may know, Copilot in Microsoft Fabric requires your tenant administrator to enable the feature from the admin portal. Starting May 20th, 2024, Copilot in Microsoft Fabric will be enabled by default for all tenants. This update is part of our continuous efforts to enhance user experience and productivity within Microsoft Fabric. This new default activation means that AI features like Copilot will be automatically enabled for tenants who have not yet enabled the setting.
We are introducing a new capability to enable Copilot on Capacity level in Fabric. A new option is being introduced in the tenant admin portal, to delegate the enablement of AI and Copilot features to Capacity administrators. This AI and Copilot setting will be automatically delegated to capacity administrators and tenant administrators won’t be able to turn off the delegation.
We also have a cross-geo setting for customers who want to use Copilot and AI features while their capacity is in a different geographic region than the EU data boundary or the US. By default, the cross-geo setting will stay off and will not be delegated to capacity administrators automatically. Tenant administrators can choose whether to delegate this to capacity administrators or not.
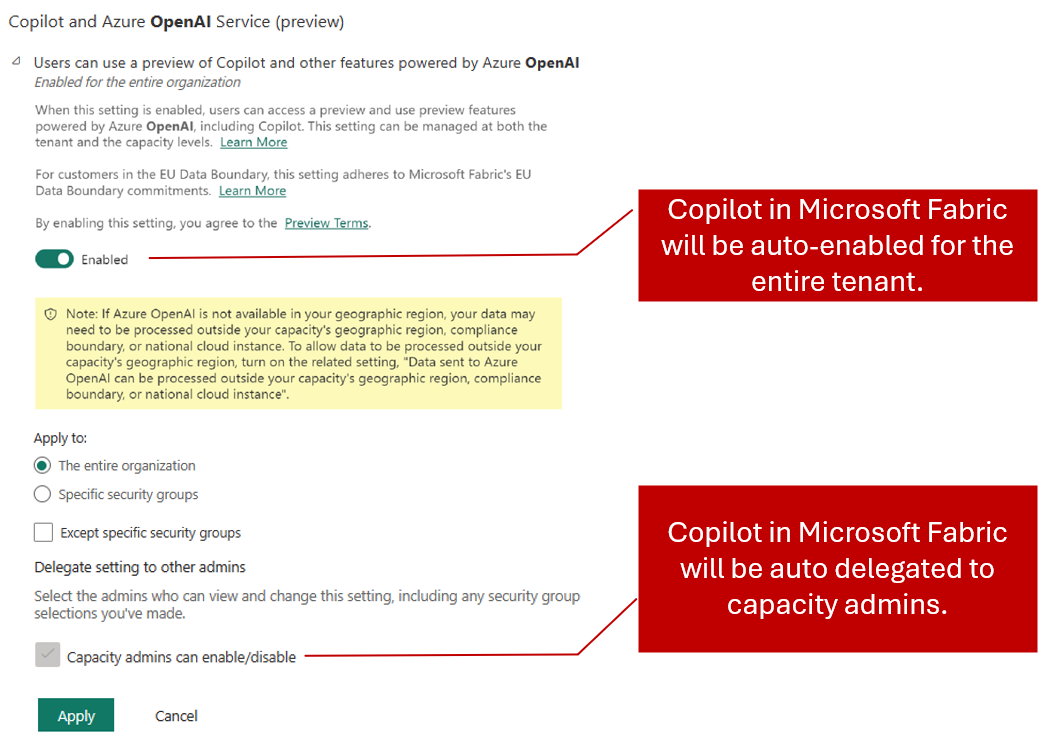
Figure 1. Copilot in Microsoft Fabric will be auto enabled and auto delegated to capacity administrators.
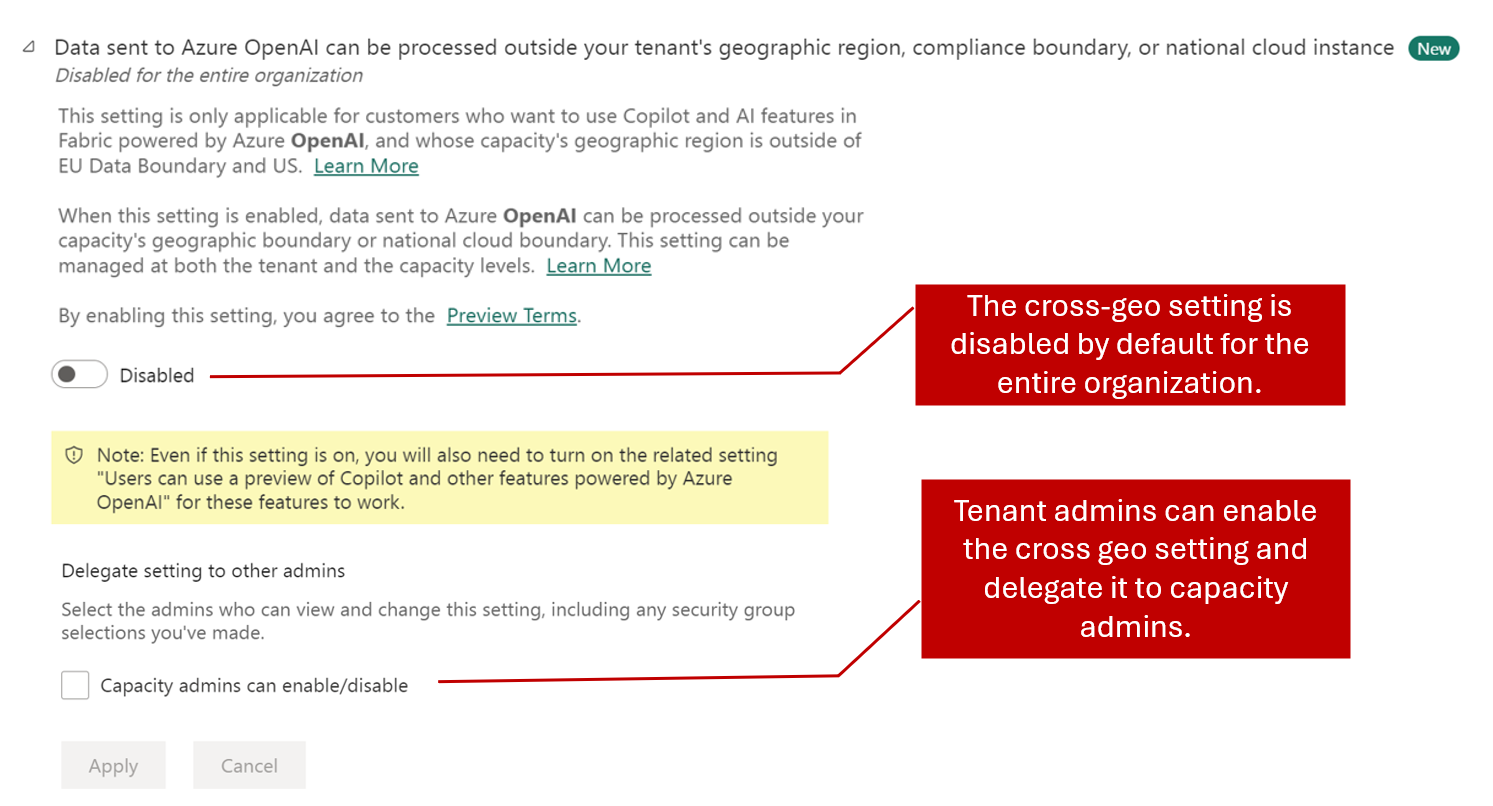
Capacity administrators will see the “Copilot and Azure OpenAI Service (preview)” settings under Capacity settings/ Fabric Capacity / <Capacity name> / Delegated tenant settings. By default, the capacity setting will inherit tenant level settings. Capacity administrators can decide whether to override the tenant administrator’s selection. This means that even if Copilot is not enabled on a tenant level, a capacity administrator can choose to enable Copilot for their capacity. With this level of control, we make it easier to control which Fabric workspaces can utilize AI features like Copilot in Microsoft Fabric.
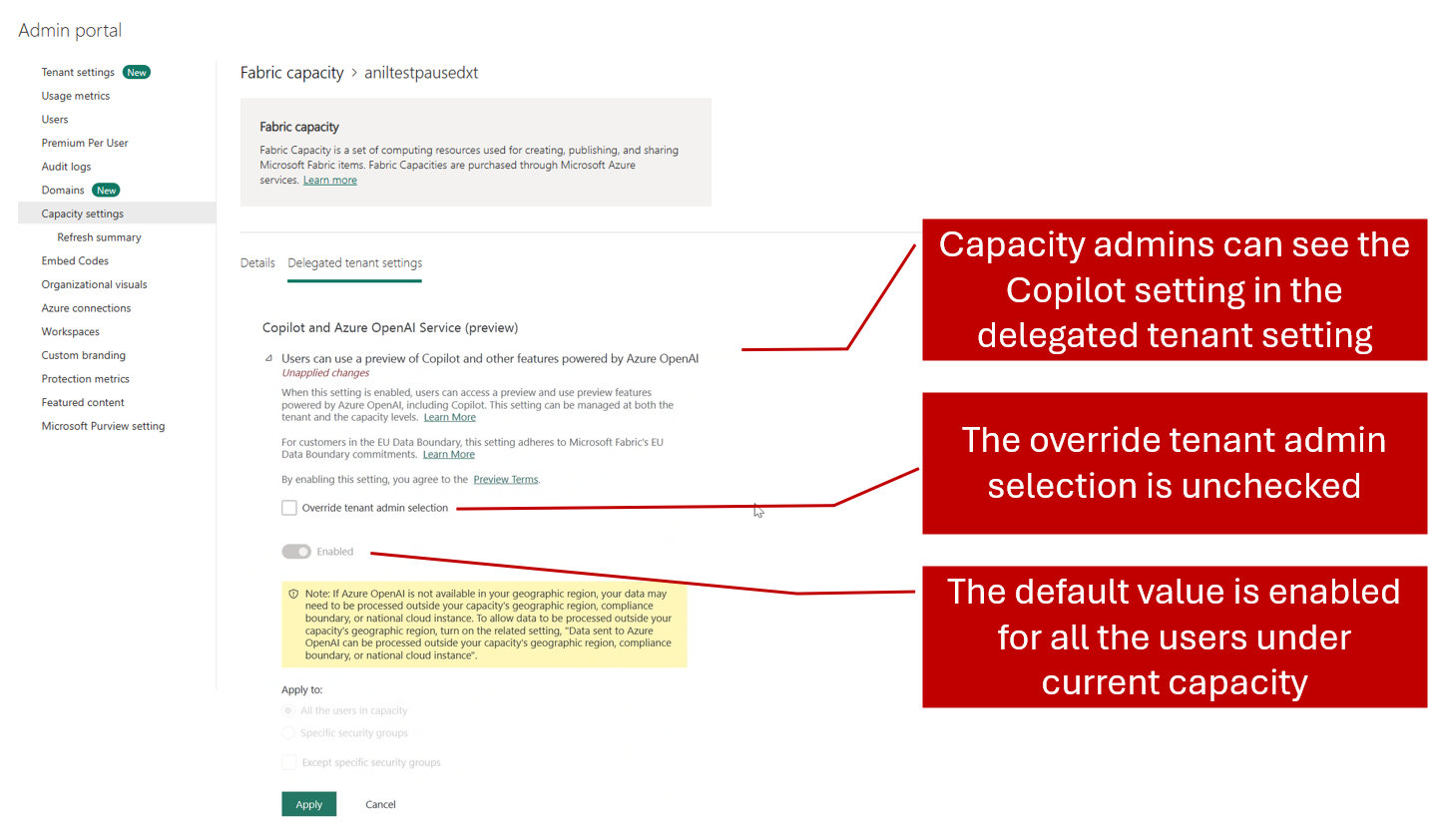
To enhance privacy and trust, we’ve updated our approach to abuse monitoring: previously, we retained data from Copilot in Fabric, including prompt inputs and outputs, for up to 30 days to check for misuse. Following customer feedback, we’ve eliminated this 30-day retention. Now, we no longer store prompt related data, demonstrating our unwavering commitment to your privacy and security. We value your input and take your concerns seriously.
Real-Time Intelligence
This month includes the announcement of Real-Time Intelligence, the next evolution of Real-Time Analytics and Data Activator. With Real-Time Intelligence, Fabric extends to the world of streaming and high granularity data, enabling all users in your organization to collect, analyze and act on this data in a timeline manner making faster and more informed business decisions. Read the full announcement from Build 2024.
Real-Time Intelligence includes a wide range of capabilities across ingestion, processing, analysis, transformation, visualization and taking action. All of this is supported by the Real-Time hub , the central place to discover and manage streaming data and start all related tasks.
Read on for more information on each capability and stay tuned for a series of blogs describing the features in more detail. All features are in Public Preview unless otherwise specified. Feedback on any of the features can be submitted at https://aka.ms/rtiidea
Ingest & Process
- Introducing the Real-Time hub
- Get Events with new sources of streaming and event data
- Source from Real-Time Hub in Enhanced Eventstream
- Use Real-Time hub to Get Data in KQL Database in Eventhouse
- Get data from Real-Time Hub within Reflexes
- Eventstream Edit and Live modes
- Default and derived streams
- Route data streams based on content
Analyze & Transform
- Eventhouse GA
- Eventhouse OneLake availability GA
- Create a database shortcut to another KQL Database
- Support for AI Anomaly Detector
- Copilot for Real-Time Intelligence
- Tenant-level private endpoints for Eventhouse
Visualize & Act
- Visualize data with Real-Time Dashboards
- New experience for data explorati on
- Create triggers from Real-Time Hub
- Set alert on Real-time Dashboards
- Taking action through Fabric Items
Ingest & Process
Real-Time hub is the single place for all data-in-motion across your entire organization. Several key features are offered in Real-Time hub:
- Single place for data-in-motion for the entire organization
Real-Time hub enables users to easily discover, ingest, manage, and consume data-in-motion from a wide variety of sources. It lists all the streams and KQL tables that customers can directly act on.
2. Real-Time hub is never empty
All data streams in Fabric automatically show up in the hub. Also, users can subscribe to events in Fabric gaining insights into the health and performance of their data ecosystem,
3. Numerous connectors to simplify data ingestion from anywhere to Real-Time hub
Real-Time hub makes it easy for you to ingest data into Fabric from a wide variety of sources like AWS Kinesis, Kafka clusters, Microsoft streaming sources, sample data and Fabric events using the Get Events experience.
There are 3 tabs in the Hub:
- Data streams : This tab contains all streams that are actively running in Fabric that user has access to. This includes all streams from Eventstreams and all tables from KQL Databases.
- Microsoft sources : This tab contains Microsoft sources (that user has access to) and can be connected to Fabric.
- Fabric events : Fabric now has event-driven capabilities to support real-time notifications and data processing. Users can monitor and react to events including Fabric Workspace Item events and Azure Blob Storage events. These events can be used to trigger other actions or workflows, such as invoking a data pipeline or sending a notification via email. Users can also send these events to other destinations via Event Streams.
Learn More
You can now connect to data from both inside and outside of Fabric in a mere few steps. Whether data is coming from new or existing sources, streams, or available events, the Get Events experience allows users to connect to a wide range of sources directly from Real-Time hub, Eventstreams, Eventhouse and Data Activator.
This enhanced capability allows you to easily connect external data streams into Fabric with out-of-box experience, giving you more options and helping you to get real-time insights from various sources. This includes Camel Kafka connectors powered by Kafka connect to access popular data platforms, as well as the Debezium connectors for fetching the Change Data Capture (CDC) streams.
Using Get Events, bring streaming data from Microsoft sources directly into Fabric with a first-class experience. Connectivity to notification sources and discrete events is also included, this enables access to notification events from Azure and other clouds solutions including AWS and GCP. The full set of sources which are currently supported are:
- Microsoft sources : Azure Event Hubs, Azure IoT hub
- External sources : Google Cloud Pub/Sub, Amazon Kinesis Data Streams, Confluent Cloud Kafka
- Change data capture databases : Azure SQL DB (CDC), PostgreSQL DB (CDC), Azure Cosmos DB (CDC), MySQL DB (CDC)
- Fabric events : Fabric Workspace Item events, Azure Blob Storage events
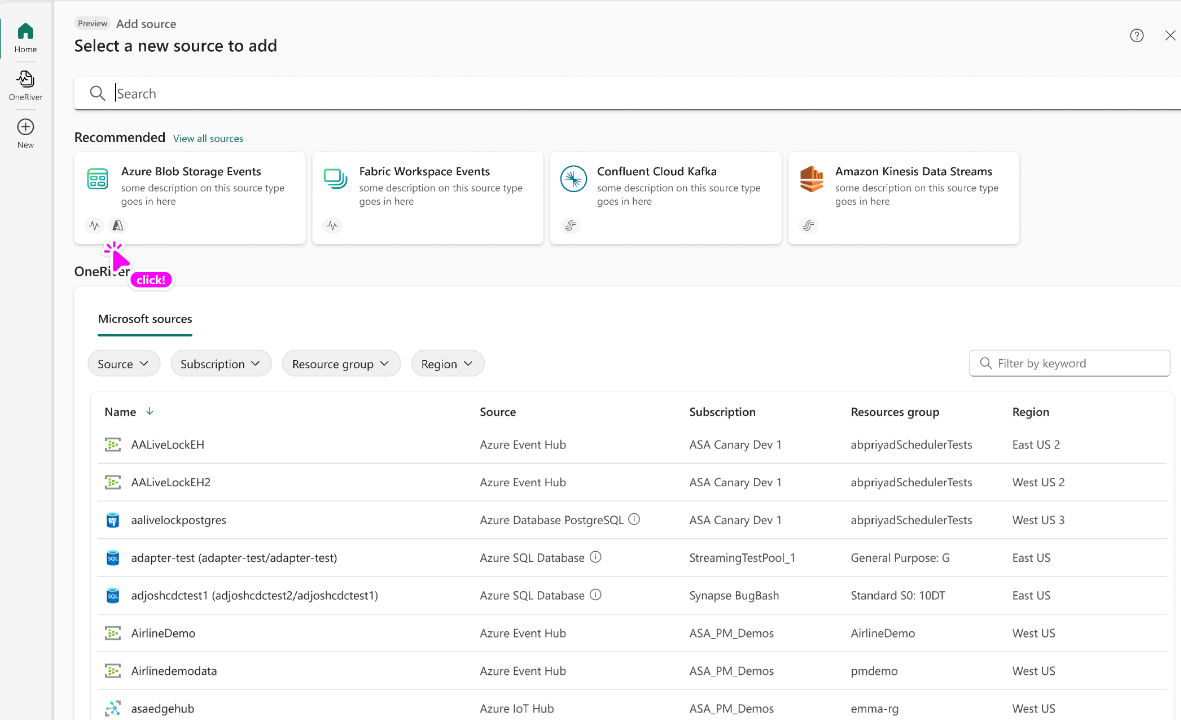
Learn More
With enhanced Eventstream, you can now stream data not only from Microsoft sources but also from other platforms like Google Cloud, Amazon Kinesis, Database change data capture streams, etc. using our new messaging connectors. The new Eventstream also lets you acquire and route real-time data not only from stream sources but also from discrete event sources, such as: Azure Blob Storage events, Fabric Workspace Item events.
To use these new sources in Eventstream, simply create an eventstream with choosing “Enhanced Capabilities (preview)”.
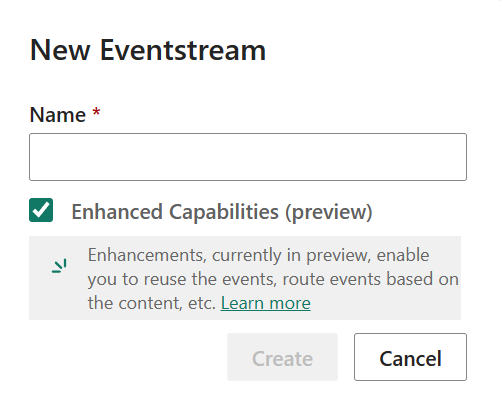
You will see the new Eventstream homepage that gives you some choices to begin with. By clicking on the “Add external source”, you will find these sources in the Get events wizard that helps you to set up the source in a few steps. After you add the source to your eventstream, you can publish it to stream the data into your eventstream.
Using Eventstream with discrete sources to turn events into streams for more analysis. You can send the streams to different Fabric data destinations, like Lakehouse and KQL Database. After the events are converted, a default stream will appear in Real-Time Hub. To turn them, click Edit on ribbon, select “Stream events” on the source node, and publish your eventstream.
To transform the stream data or route it to different Fabric destinations based on its content, you can click Edit in ribbon and enter the Edit mode. There you can add event processing operators and destinations.
With Real-Time hub embedded in KQL Database experience, each user in the tenant can view and add streams which they have access to and directly ingest it to a KQL Database table in Eventhouse.
This integration provides each user in the tenant with the ability to access and view data streams they are permitted to. They can now directly ingest these streams into a KQL Database table in Eventhouse. This simplifies the data discovery and ingestion process by allowing users to directly interact with the streams. Users can filter data based on the Owner, Parent and Location and provides additional information such as Endorsement and Sensitivity.
You can access this by clicking on the Get Data button from the Database ribbon in Eventhouse.
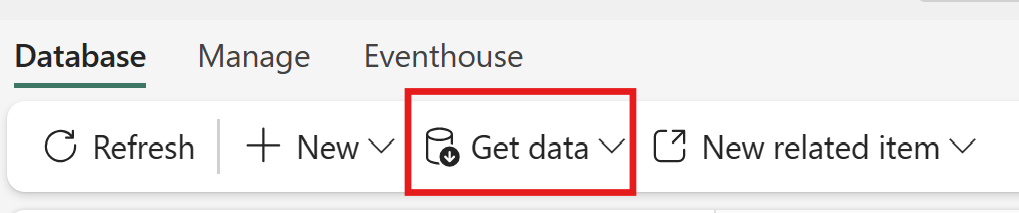
This will open the Get Data wizard with Real-Time hub embedded.
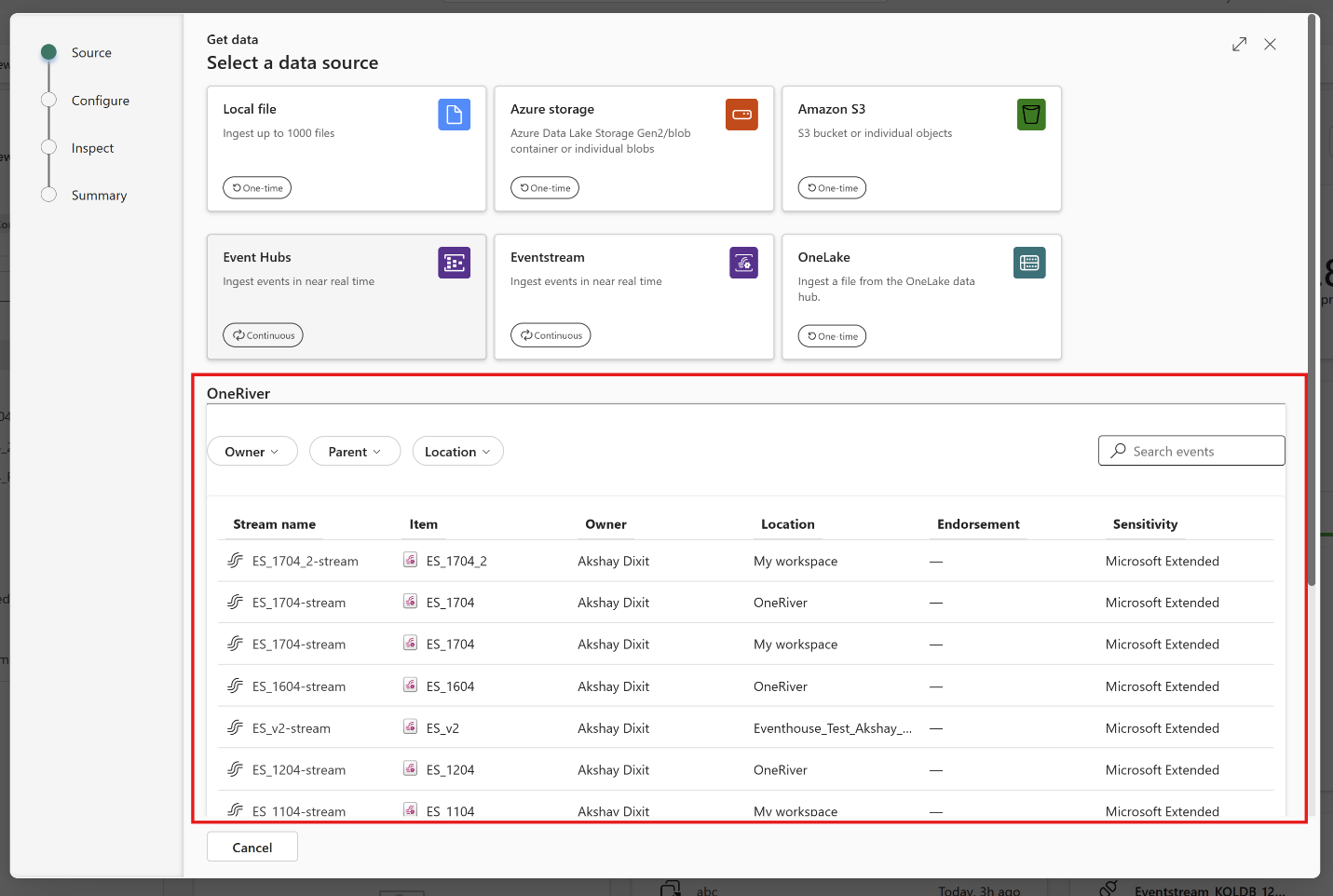
You can use events from Real-Time hub directly in reflex items as well. From within the main reflex UI, click ‘Get data’ in the toolbar:
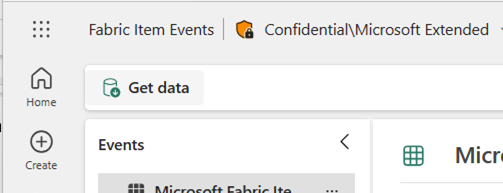
This will open a wizard that allows you to connect to new event sources or browse Real-Time Hub to use existing streams or system events.
Search new stream sources to connect to or select existing streams and tables to be ingested directly by Reflex.
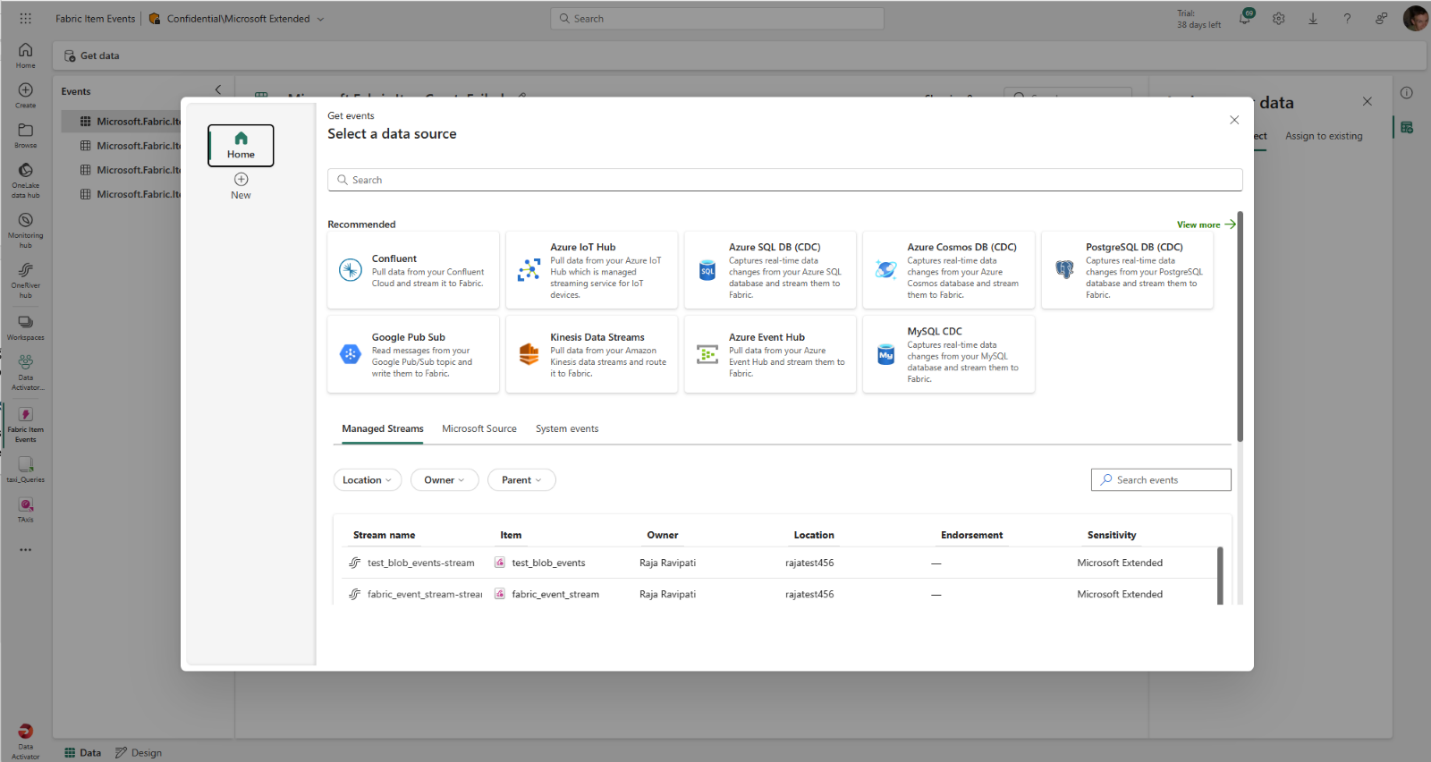
You then have access to the full reflex modeling experience to build properties and triggers over any events from Real-Time hub.
Eventstream offers two distinct modes, Edit and Live, to provide flexibility and control over the development process of your eventstream. If you create a new Eventstream with Enhanced Capabilities enabled, you can modify it in an Edit mode. Here, you can design stream processing operations for your data streams using a no-code editor. Once you complete the editing, you can publish your Eventstream and visualize how it starts streaming and processing data in Live mode .
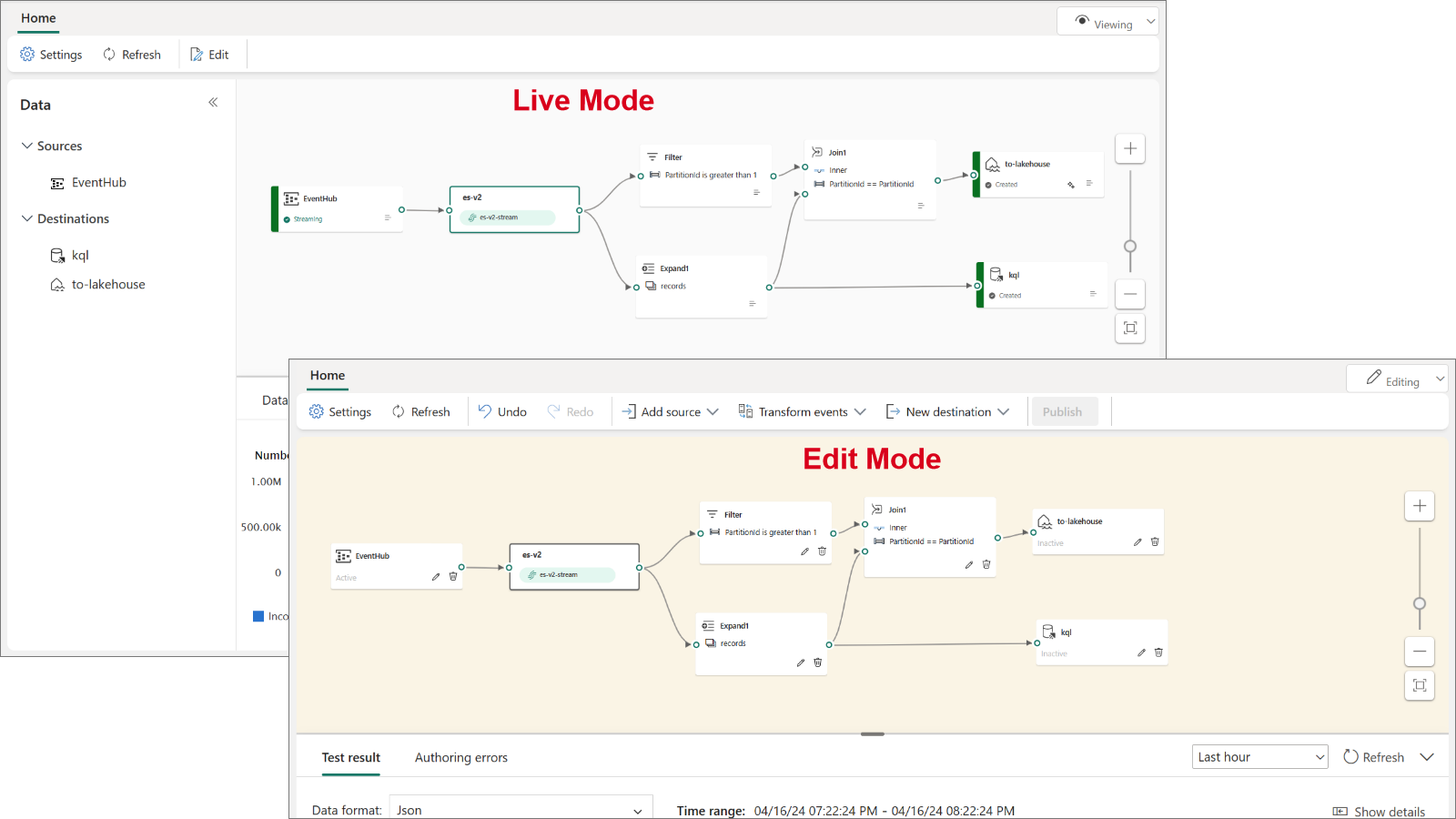
In Edit mode, you can:
- Make changes to an Eventstream without implementing them until you publish the Eventstream. This gives you full control over the development process.
- Avoid test data being streamed to your Eventstream. This mode is designed to provide a secure environment for testing without affecting your actual data streams.
For Live mode, you can :
- Visualize how your Eventstream streams, transforms, and routes your data streams to various destinations after publishing the changes.
- Pause the flow of data on selected sources and destinations, providing you with more control over your data streams being streamed into your Eventstream.
When you create a new Eventstream with Enhanced Capabilities enabled, you can now create and manage multiple data streams within Eventstream, which can then be displayed in the Real-Time hub for others to consume and perform further analysis.
There are two types of streams:
- Default stream : Automatically generated when a streaming source is added to Eventstream. Default stream captures raw event data directly from the source, ready for transformation or analysis.
- Derived stream : A specialized stream that users can create as a destination within Eventstream. Derived stream can be created after a series of operations such as filtering and aggregating, and then it’s ready for further consumption or analysis by other users in the organization through the Real-Time Hub.
The following example shows that when creating a new Eventstream a default stream alex-es1-stream is automatically generated. Subsequently, a derived stream dstream1 is added after an Aggregate operation within the Eventstream. Both default and derived streams can be found in the Real-Time hub.
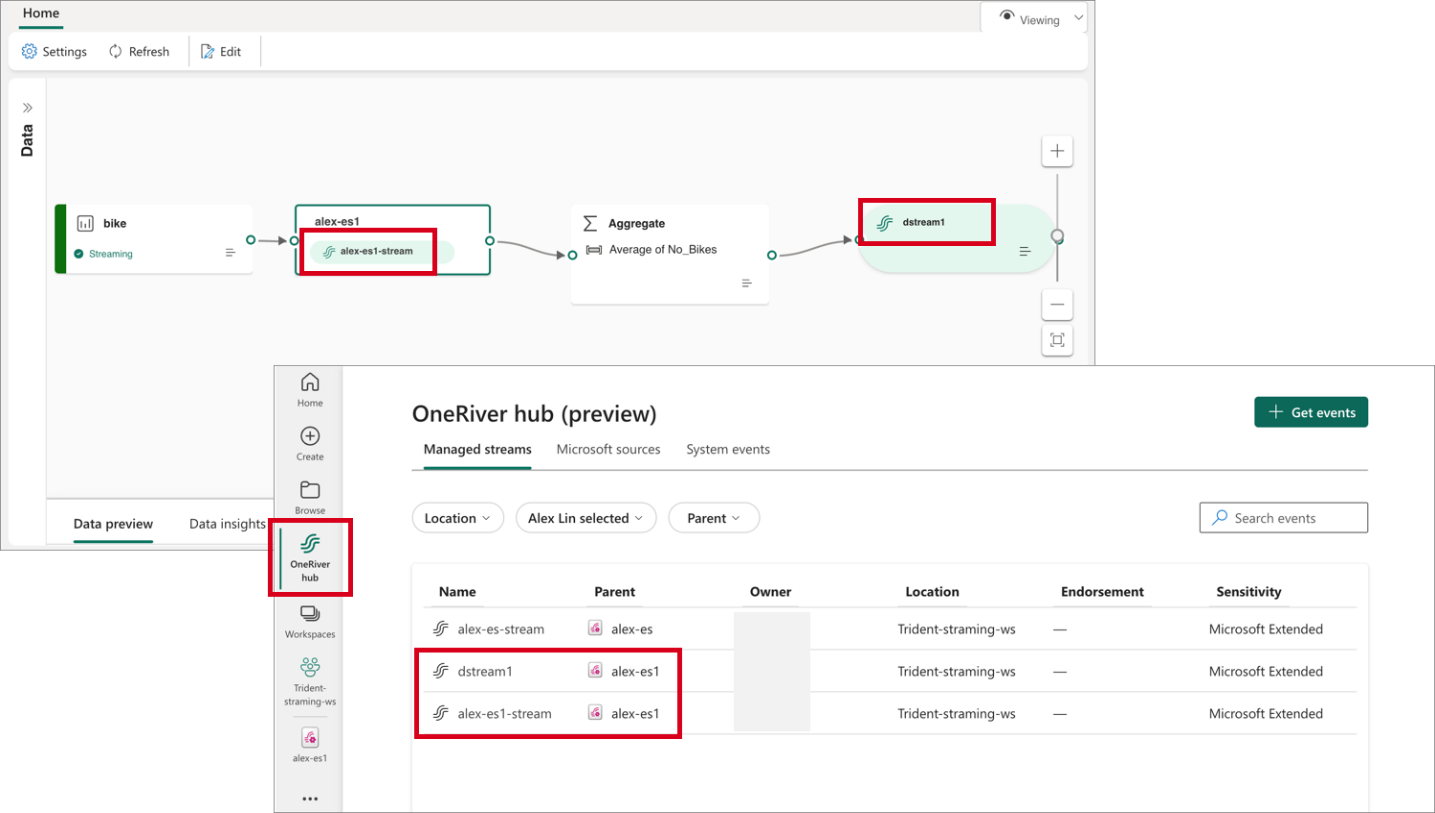
Customers can now perform stream operations directly within Eventstream’s Edit mode, instead of embedding in a destination. This enhancement allows you to design stream processing logics and route data streams in the top-level canvas. Custom processing and routing can be applied to individual destinations using built-in operations, allowing for routing to distinct destinations within the Eventstream based on different stream content.
These operations include:
- Aggregate : Perform calculations such as SUM, AVG, MIN, and MAX on a column of values and return a single result.
- Expand : Expand array values and create new rows for each element within the array.
- Filter : Select or filter specific rows from the data stream based on a condition.
- Group by : Aggregate event data within a certain time window, with the option to group one or more columns.
- Manage Fields : Customize your data streams by adding, removing, or changing data type of a column.
- Union : Merge two or more data streams with shared fields (same name and data type) into a unified data stream.
Analyze & Transform
Eventhouse, a cutting-edge database workspace meticulously crafted to manage and store event-based data, is now officially available for general use. Optimized for high granularity, velocity, and low latency streaming data, it incorporates indexing and partitioning for structured, semi-structured, and free text data. With Eventhouse, users can perform high-performance analysis of big data and real-time data querying, processing billions of events within seconds. The platform allows users to organize data into compartments (databases) within one logical item, facilitating efficient data management.
Additionally, Eventhouse enables the sharing of compute and cache resources across databases, maximizing resource utilization. It also supports high-performance queries across databases and allows users to apply common policies seamlessly. Eventhouse offers content-based routing to multiple databases, full view lineage, and high granularity permission control, ensuring data security and compliance. Moreover, it provides a simple migration path from Azure Synapse Data Explorer and Azure Data Explorer, making adoption seamless for existing users.
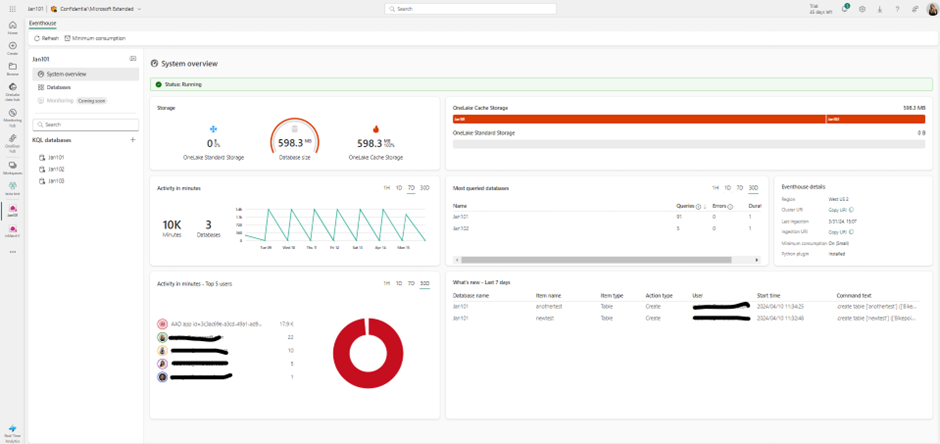
Engineered to handle data in motion, Eventhouse seamlessly integrates indexing and partitioning into its storing process, accommodating various data formats. This sophisticated design empowers high-performance analysis with minimal latency, facilitating lightning-fast ingestion and querying within seconds. Eventhouse is purpose-built to deliver exceptional performance and efficiency for managing event-based data across diverse applications and industries. Its intuitive features and seamless integration with existing Azure services make it an ideal choice for organizations looking to leverage real-time analytics for actionable insights. Whether it’s analyzing telemetry and log data, time series and IoT data, or financial records, Eventhouse provides the tools and capabilities needed to unlock the full potential of event-based data.
We’re excited to announce that OneLake availability of Eventhouse in Delta Lake format is Generally Available.
Delta Lake is the unified data lake table format chosen to achieve seamless data access across all compute engines in Microsoft Fabric.
The data streamed into Eventhouse is stored in an optimized columnar storage format with full text indexing and supports complex analytical queries at low latency on structured, semi-structured, and free text data.
Enabling data availability of Eventhouse in OneLake means that customers can enjoy the best of both worlds: they can query the data with high performance and low latency in their Eventhouse and query the same data in Delta Lake format via any other Fabric engines such as Power BI Direct Lake mode, Warehouse, Lakehouse, Notebooks, and more.
To learn more, please visit https://learn.microsoft.com/en-gb/fabric/real-time-analytics/one-logical-copy
A database shortcut in Eventhouse is an embedded reference to a source database. The source database can be one of the following:
- (Now Available) A KQL Database in Real-Time Intelligence
- An Azure Data Explorer database
The behavior exhibited by the database shortcut is similar to that of a follower database
The owner of the source database, the data provider, shares the database with the creator of the shortcut in Real-Time Intelligence, the data consumer. The owner and the creator can be the same person. The database shortcut is attached in read-only mode, making it possible to view and run queries on the data that was ingested into the source KQL Database without ingesting it.
This helps with data sharing scenarios where you can share data in-place either within teams, or even with external customers.
AI Anomaly Detector is an Azure service for high quality detection of multivariate and univariate anomalies in time series. While the standalone version is being retired October 2026, Microsoft open sourced the anomaly detection core algorithms and they are now supported in Microsoft Fabric. Users can leverage these capabilities in Data Science and Real-Time Intelligence workload. AI Anomaly Detector models can be trained in Spark Python notebooks in Data Science workload, while real time scoring can be done by KQL with inline Python in Real-Time Intelligence.
We are excited to announce the Public Preview of Copilot for Real-Time Intelligence. This initial version includes a new capability that translates your natural language questions about your data to KQL queries that you can run and get insights.
Your starting point is a KQL Queryset, that is connected to a KQL Database, or to a standalone Kusto database:
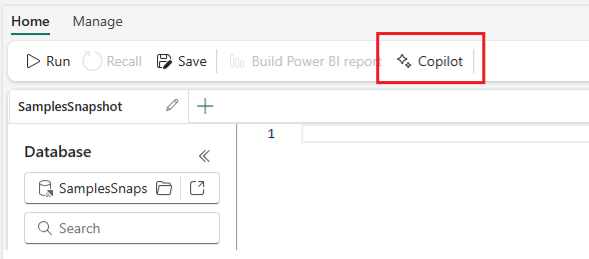
Simply type the natural language question about what you want to accomplish, and Copilot will automatically translate it to a KQL query you can execute. This is extremely powerful for users who may be less familiar with writing KQL queries but still want to get the most from their time-series data stored in Eventhouse.
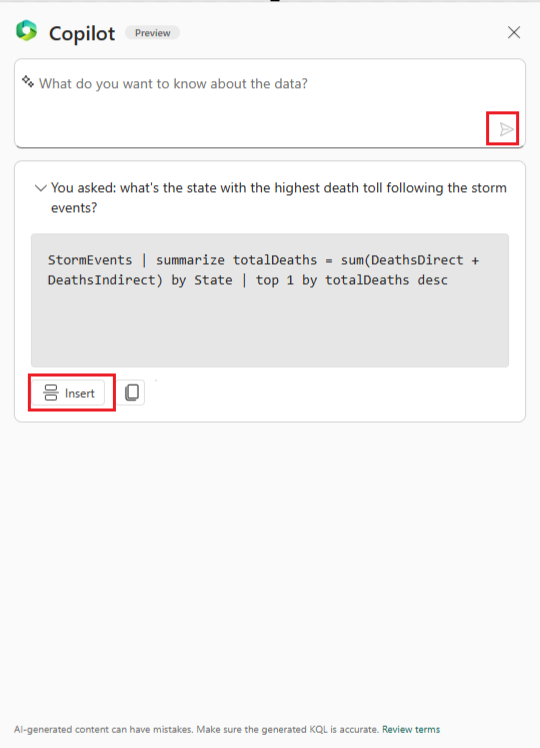
Stay tuned for more capabilities from Copilot for Real-Time Intelligence!
Customers can increase their network security by limiting access to Eventhouse at a tenant-level, from one or more virtual networks (VNets) via private links. This will prevent unauthorized access from public networks and only permit data plane operations from specific VNets.
Visualize & Act
Real-Time Dashboards have a user-friendly interface, allowing users to quickly explore and analyze their data without the need for extensive technical knowledge. They offer a high refresh frequency, support a range of customization options, and are designed to handle big data.
The following visual types are supported, and can be customized with the dashboard’s user-friendly interface:

You can also define conditional formatting rules to format the visual data points by their values using colors, tags, and icons. Conditional formatting can be applied to a specific set of cells in a predetermined column or to entire rows, and lets you easily identify interesting data points.
Beyond the support visual, Real-Time Dashboards provide several capabilities to allow you to interact with your data by performing slice and dice operations for deeper analysis and gaining different viewpoints.
- Parameters are used as building blocks for dashboard filters and can be added to queries to filter the data presented by visuals. Parameters can be used to slice and dice dashboard visuals either directly by selecting parameter values in the filter bar or by using cross-filters.
- Cross filters allow you to select a value in one visual and filter all other visuals on that dashboard based on the selected data point.
- Drillthrough capability allows you to select a value in a visual and use it to filter the visuals in a target page in the same dashboard. When the target page opens, the value is pushed to the relevant filters.
Real-Time Dashboards can be shared broadly and allow multiple stakeholders to view dynamic, real time, fresh data while easily interacting with it to gain desired insights.
Directly from a real-time dashboard, users can refine their exploration using a user-friendly, form-like interface. This intuitive and dynamic experience is tailored for insights explorers craving insights based on real-time data. Add filters, create aggregations, and switch visualization types without writing queries to easily uncover insights.
With this new feature, insights explorers are no longer bound by the limitations of pre-defined dashboards. As independent explorers, they have the freedom for ad-hoc exploration, leveraging existing tiles to kickstart their journey. Moreover, they can selectively remove query segments, and expand their view of the data landscape.
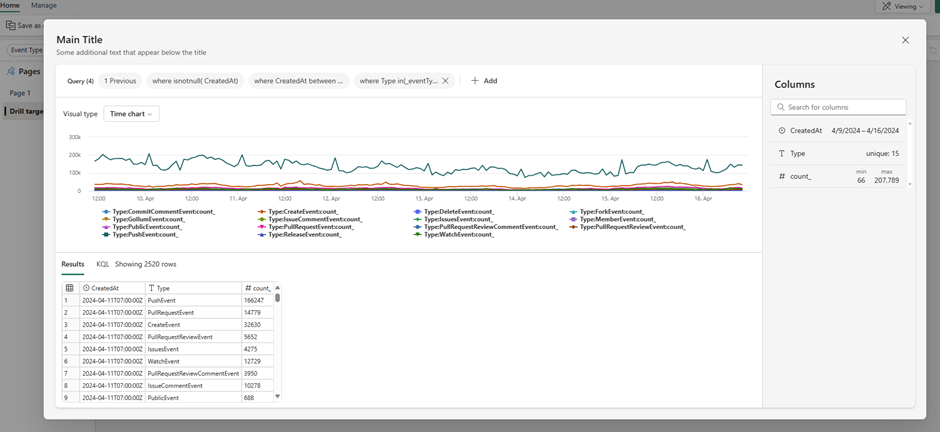
Dive deep, extract meaningful insights, and chart actionable paths forward, all with ease and efficiency, and without having to write complex KQL queries.
Data Activator allows you to monitor streams of data for various conditions and set up actions to be taken in response. These triggers are available directly within the Real-Time hub and in other workloads in Fabric. When the condition is detected, an action will automatically be kicked off such as sending alerts via email or Teams or starting jobs in Fabric items.
When you browse the Real-Time Hub, you’ll see options to set triggers in the detail pages for streams.

Selecting this will open a side panel where you can configure the events you want to monitor, the conditions you want to look for in the events, and the action you want to take while in the Real-Time hub experience.
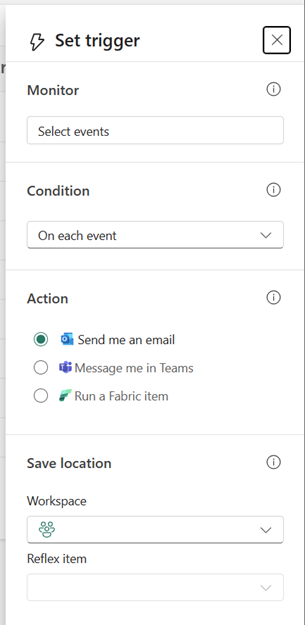
Completing this pane creates a new reflex item with a trigger that monitors the selected events and condition for you. Reflexes need to be created in a workspace supported by a Fabric or Power BI Premium capacity – this can be a trial capacity so you can get started with it today!
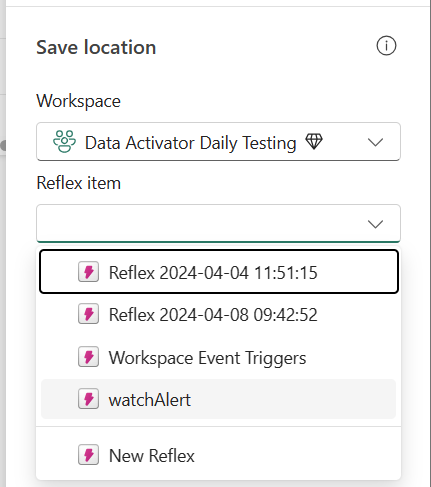
Data Activator has been able to monitor Power BI report data since it was launched, and we now support monitoring of Real-Time Dashboard visuals in the same way.
From real-time dashboard tiles you can click the ellipsis (…) button and select “Set alert”
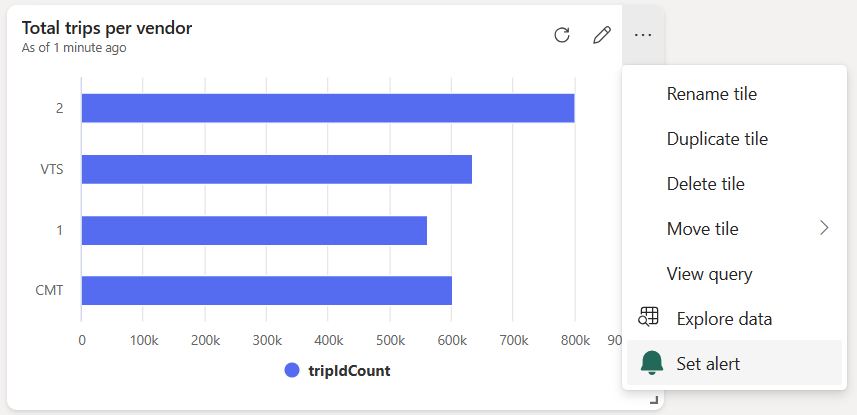
This opens the embedded trigger pane, where you can specify what conditions, you are looking for. You can choose whether to send email or Teams messages as the alert when these conditions are met.
When creating a new reflex trigger, from Real-time Hub or within the reflex item itself, you’ll notice a new ‘Run a Fabric item’ option in the Action section. This will create a trigger that starts a new Fabric job whenever its condition is met, kicking off a pipeline or notebook computation in response to Fabric events. A common scenario would be monitoring Azure Blob storage events via Real-Time Hub, and running data pipeline jobs when Blog Created events are detected.
This capability is extremely powerful and moves Fabric from a scheduled driven platform to an event driven platform.
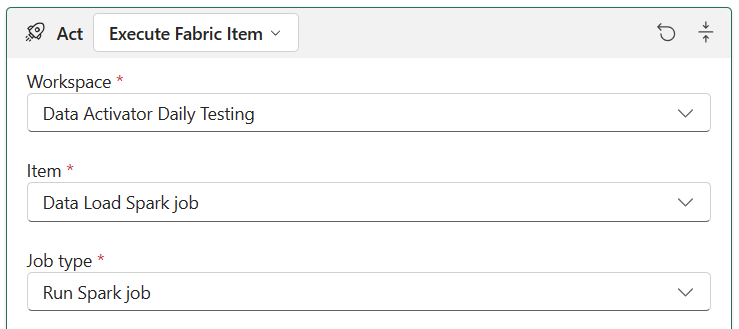
Pipelines, spark jobs, and notebooks are just the first Fabric items we’ll support here, and we’re keen to hear your feedback to help prioritize what else we support. Please leave ideas and votes on https://aka.ms/rtiidea and let us know!
Real-Time Intelligence, along with the Real-Time hub, revolutionizes what’s possible with real-time streaming and event data within Microsoft Fabric.
Learn more and try it today https://aka.ms/realtimeintelligence
Data Factory
Dataflow gen2 .
We are thrilled to announce that the Power Query SDK is now generally available in Visual Studio Code! This marks a significant milestone in our commitment to providing developers with powerful tools to enhance data connectivity and transformation.
The Power Query SDK is a set of tools that allow you as the developer to create new connectors for Power Query experiences available in products such as Power BI Desktop, Semantic Models, Power BI Datamarts, Power BI Dataflows, Fabric Dataflow Gen2 and more.
This new SDK has been in public preview since November of 2022, and we’ve been hard at work improving this experience which goes beyond what the previous Power Query SDK in Visual Studio had to offer.
The latest of these biggest improvements was the introduction of the Test Framework in March of 2024 that solidifies the developer experience that you can have within Visual Studio Code and the Power Query SDK for creating a Power Query connector.
The Power Query SDK extension for Visual Studio will be deprecated by June 30, 2024, so we encourage you to give this new Power Query SDK in Visual Studio Code today if you haven’t.
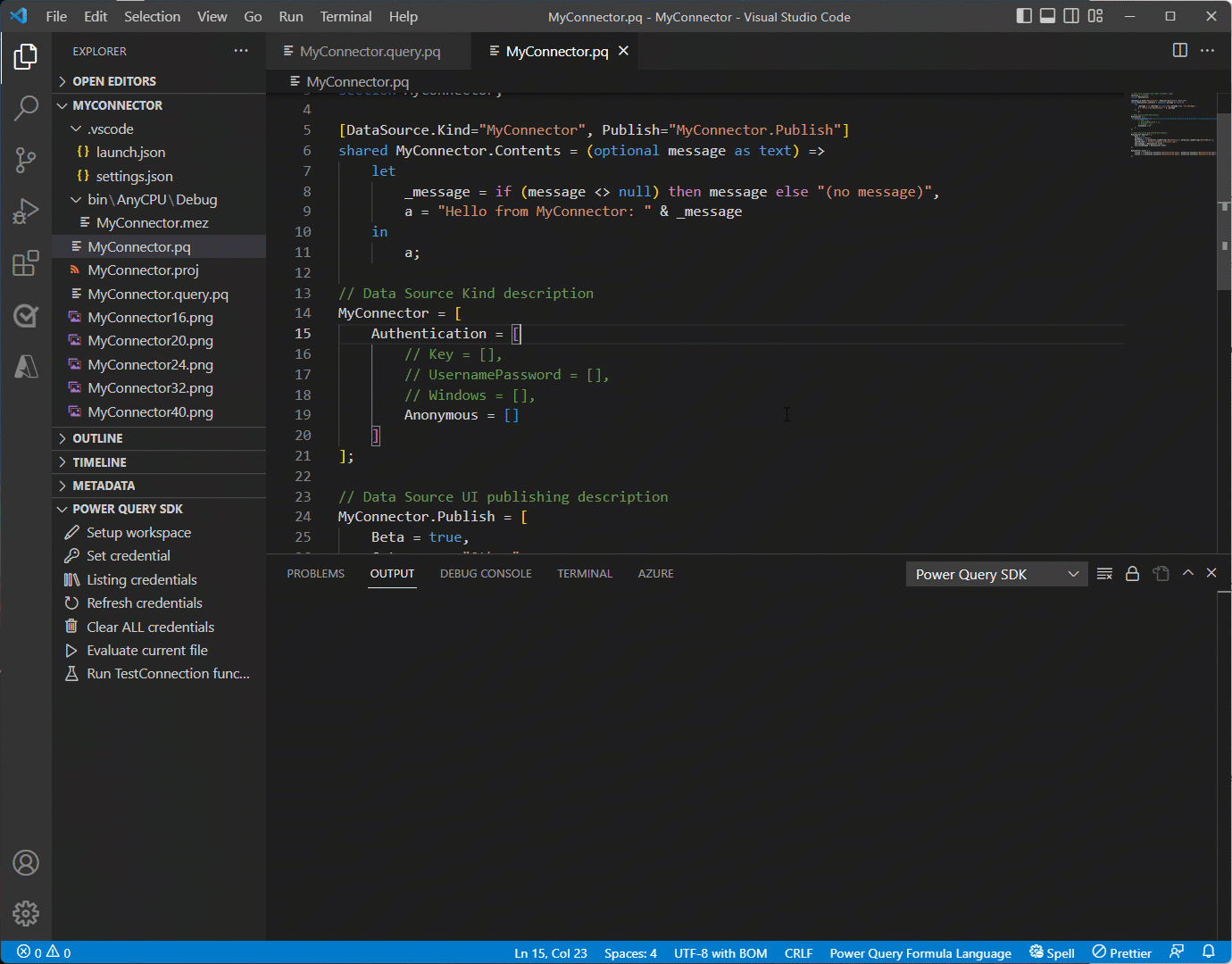
To get started with the Power Query SDK in Visual Studio Code, simply install it from the Visual Studio Code Marketplace . Our comprehensive documentation and tutorials are available to help you harness the full potential of your data.
Join our vibrant community of developers to share insights, ask questions, and collaborate on exciting projects. Our dedicated support team is always ready to assist you with any queries.
We look forward to seeing the innovative solutions you’ll create with the Power Query SDK in Visual Studio Code. Happy coding!
Introducing a convenient enhancement to the Dataflows Gen2 Refresh History experience! Now, alongside the familiar “X” button in the Refresh History screen, you’ll find a shiny new Refresh Button . This small but mighty addition empowers users to refresh the status of their dataflow refresh history status without the hassle of exiting the refresh history and reopening it. Simply click the Refresh Button , and voilà! Your dataflow’s refresh history status screen is updated, keeping you in the loop with minimal effort. Say goodbye to unnecessary clicks and hello to streamlined monitoring!
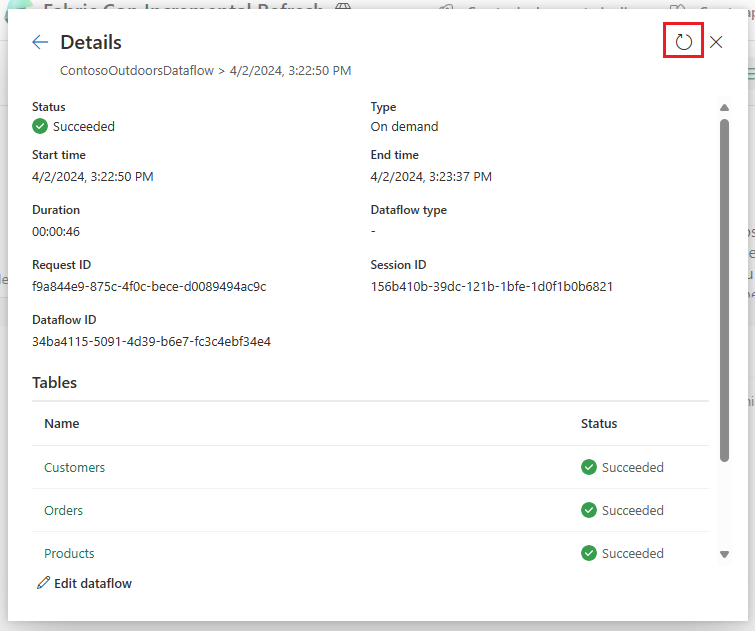
- [New] OneStream : The OneStream Power Query Connector enables you to seamlessly connect Data Factory to your OneStream applications by simply logging in with your OneStream credentials. The connector uses your OneStream security, allowing you to access only the data you have based on your permissions within the OneStream application. Use the connector to pull cube and relational data along with metadata members, including all their properties. Visit OneStream Power BI Connector to learn more. Find this connector in the other category.
Data workflows
We are excited to announce the preview of ‘Data workflows’, a new feature within the Data Factory that revolutionizes the way you build and manage your code-based data pipelines. Powered by Apache Airflow, Data workflows offer seamless authoring, scheduling, and monitoring experience for Python-based data processes defined as Directed Acyclic Graphs (DAGs). This feature brings a SaaS-like experience to running DAGs in a fully managed Apache Airflow environment, with support for autoscaling , auto-pause , and rapid cluster resumption to enhance cost-efficiency and performance.
It also includes native cloud-based authoring capabilities and comprehensive support for Apache Airflow plugins and libraries.
To begin using this feature:
- Access the Microsoft Fabric Admin Portal.
- Navigate to Tenant Settings.
Under Microsoft Fabric options, locate and expand the ‘Users can create and use Data workflows (preview)’ section. Note: This action is necessary only during the preview phase of Data workflows.
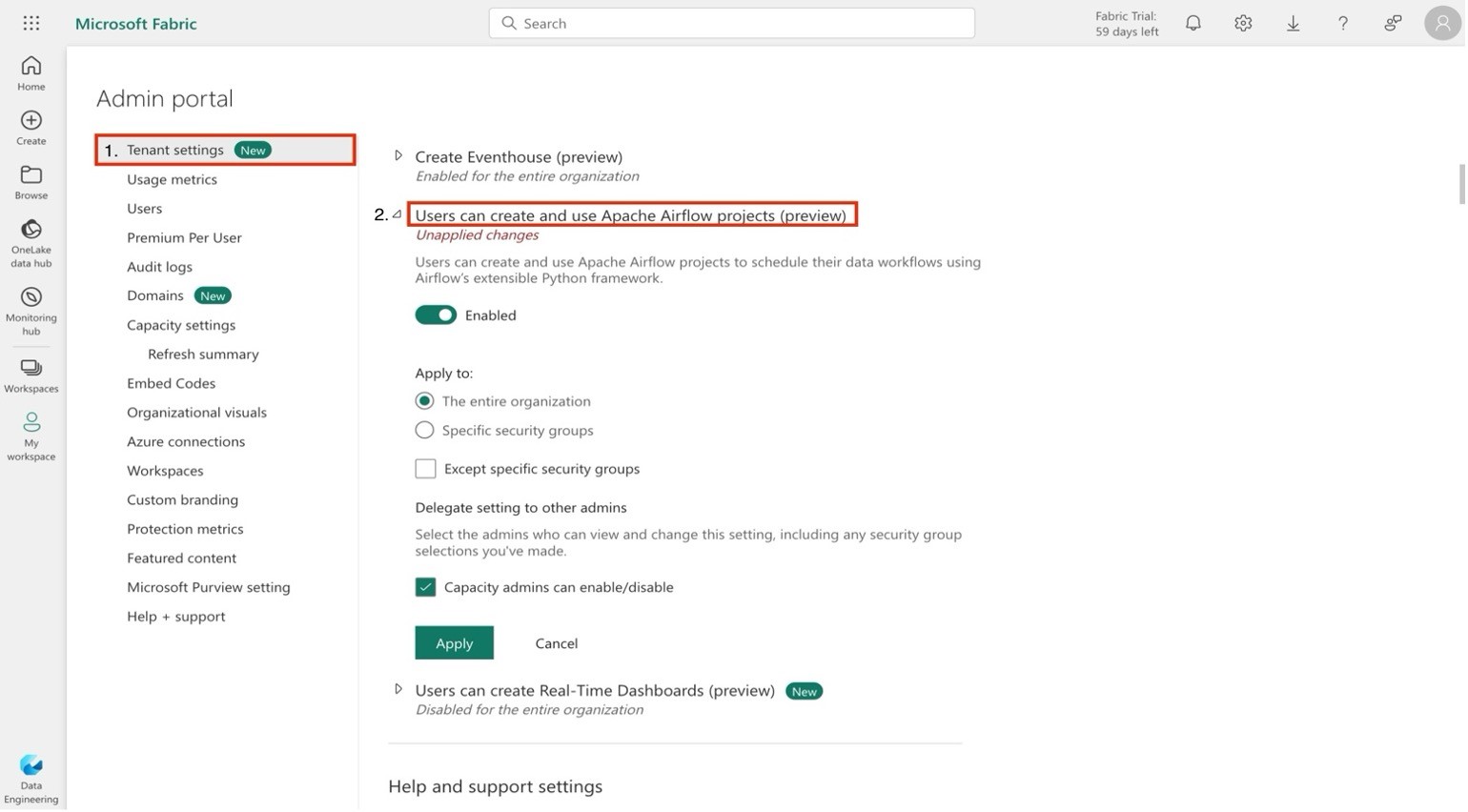
2. Create a new Data workflow within an existing or new workspace.
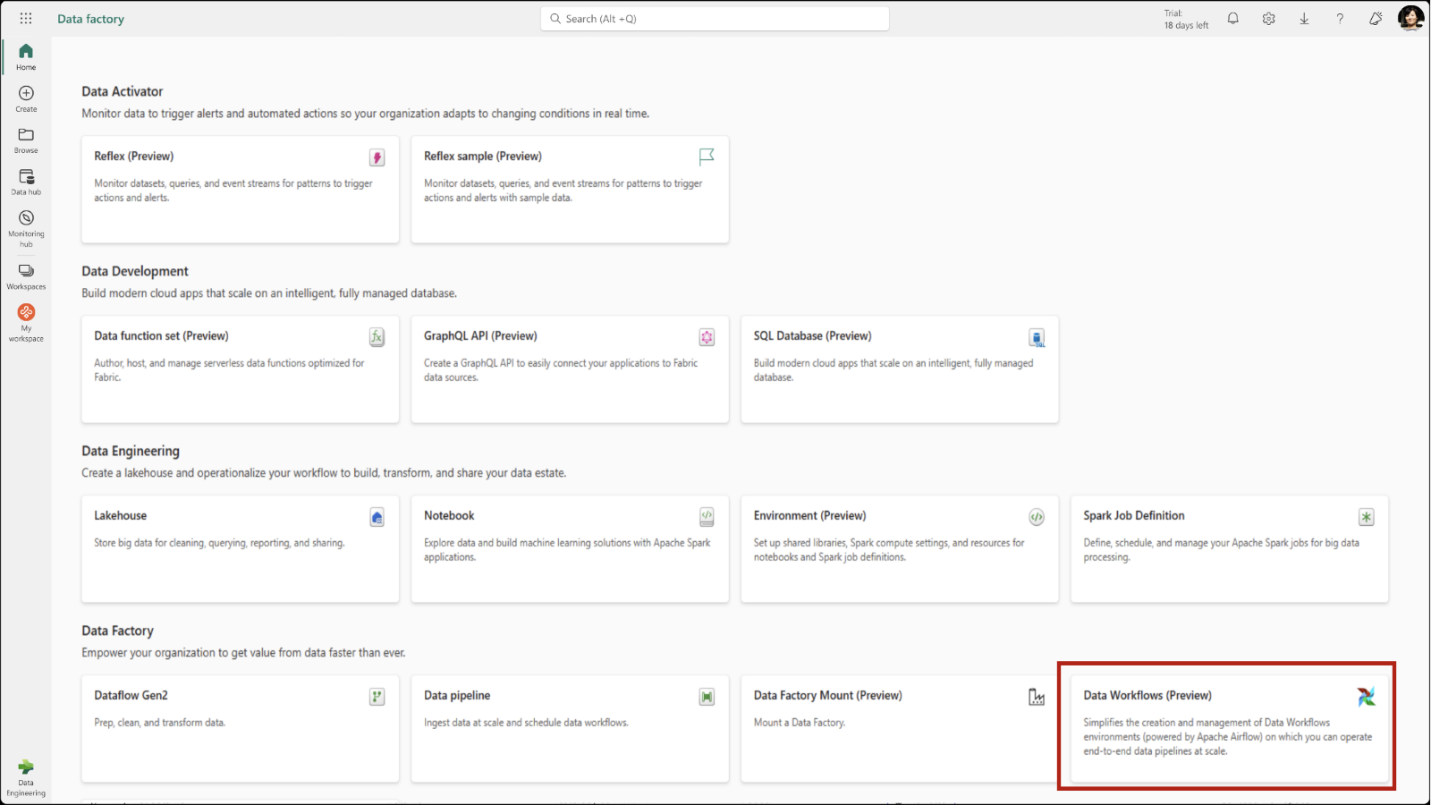
3. Add a new Directed Acyclic Graph (DAG) file via the user interface.
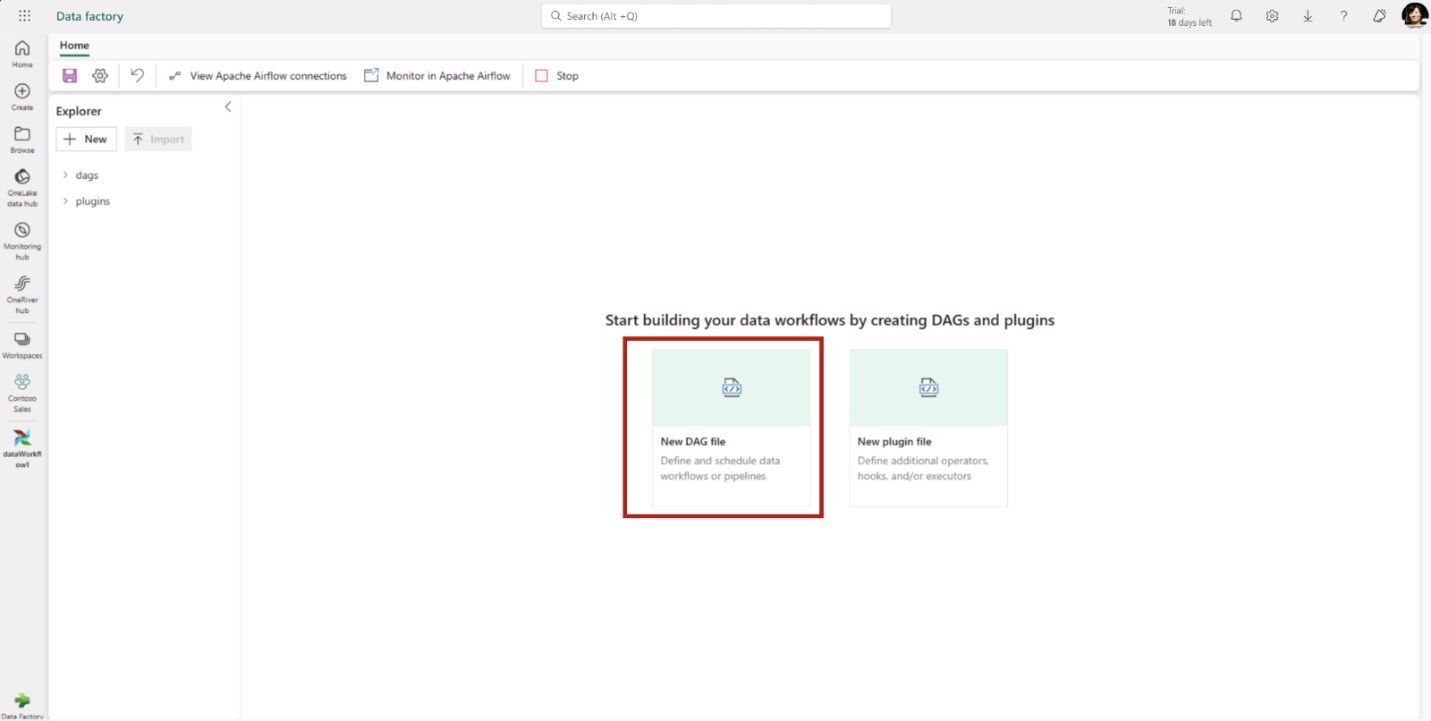
4. Save your DAG(s).
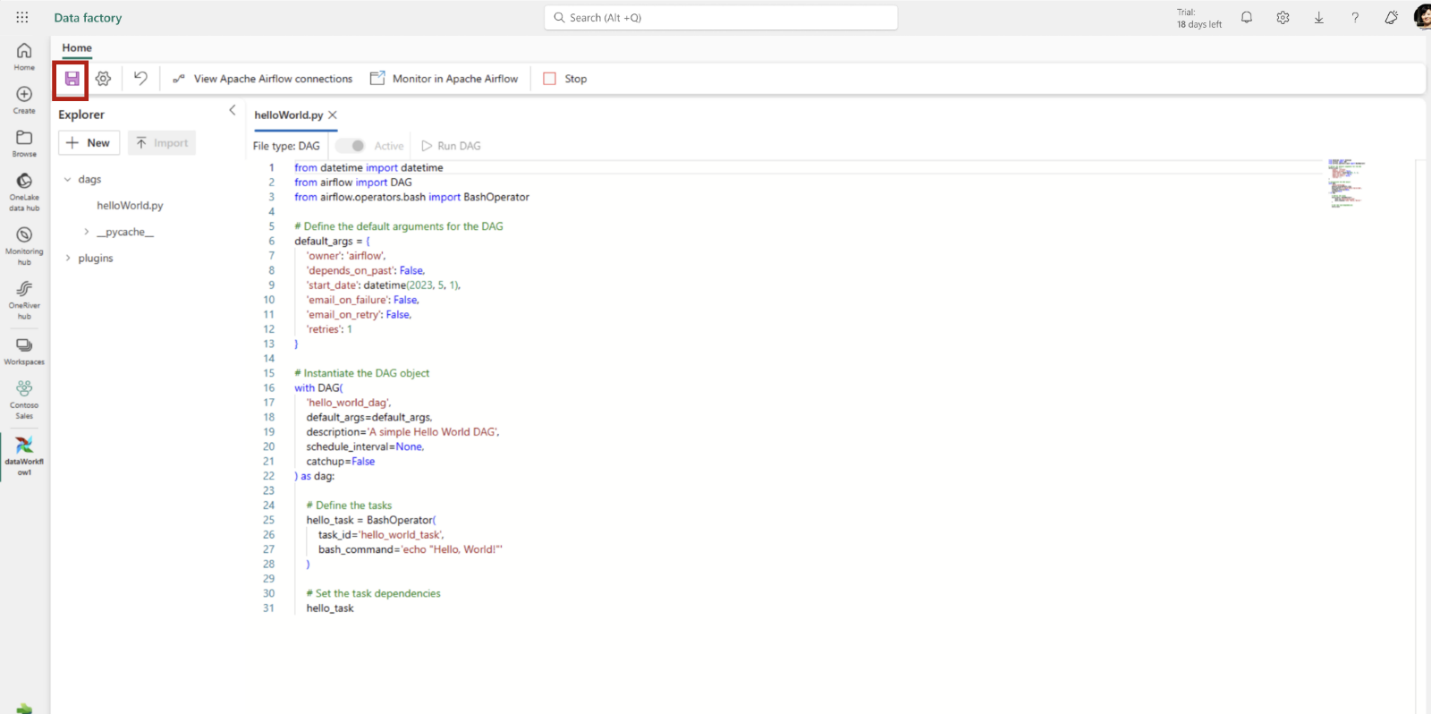
5. Use Apache Airflow monitoring tools to observe your DAG executions. In the ribbon, click on Monitor in Apache Airflow.
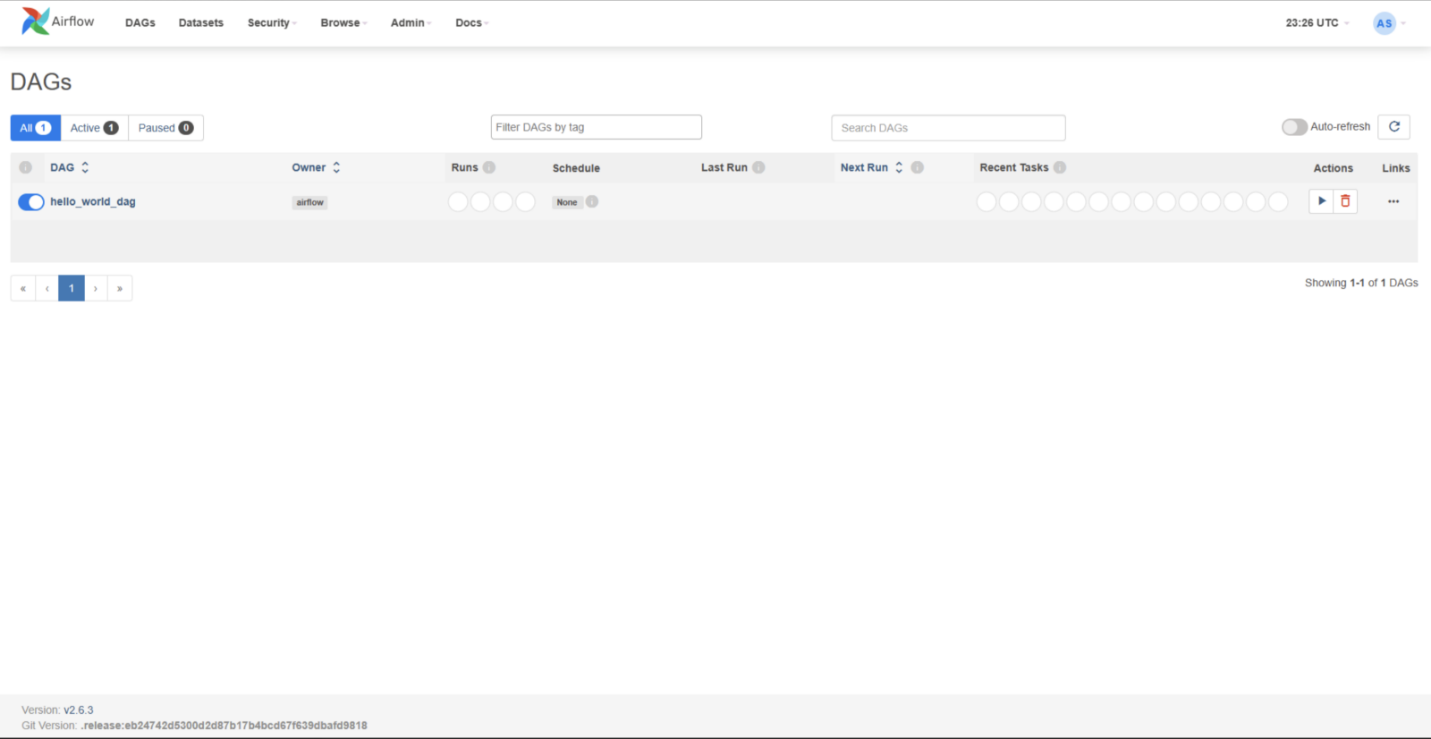
For additional information, please consult the product documentation . If you’re not already using Fabric capacity, consider signing up for the Microsoft Fabric free trial to evaluate this feature.
Data Pipelines
We are excited to announce a new feature in Fabric that enables you to create data pipelines to access your firewall-enabled Azure Data Lake Storage Gen2 (ADLS Gen2) accounts. This feature leverages the workspace identity to establish a secure and seamless connection between Fabric and your storage accounts.
With trusted workspace access, you can create data pipelines to your storage accounts with just a few clicks. Then you can copy data into Fabric Lakehouse and start analyzing your data with Spark, SQL, and Power BI. Trusted workspace access is available for workspaces in Fabric capacities (F64 or higher). It supports organizational accounts or service principal authentication for storage accounts.
How to use trusted workspace access in data pipelines
Create a workspace identity for your Fabric workspace. You can follow the guidelines provided in Workspace identity in Fabric .
Configure resource instance rules for the Storage account that you want to access from your Fabric workspace. Resource instance rules for Fabric workspaces can only be created through ARM templates. Follow the guidelines for configuring resource instance rules for Fabric workspaces here .
Create a data pipeline to copy data from the firewall enabled ADLS gen2 account to a Fabric Lakehouse.
To learn more about how to use trusted workspace access in data pipelines, please refer to Trusted workspace access in Fabric .
We hope you enjoy this new feature for your data integration and analytics scenarios. Please share your feedback and suggestions with us by leaving a comment here.
Introducing Blob Storage Event Triggers for Data Pipelines
A very common use case among data pipeline users in a cloud analytics solution is to trigger your pipeline when a file arrives or is deleted. We have introduced Azure Blob storage event triggers as a public preview feature in Fabric Data Factory Data Pipelines. This utilizes the Fabric Reflex alerts capability that also leverages Event Streams in Fabric to create event subscriptions to your Azure storage accounts.
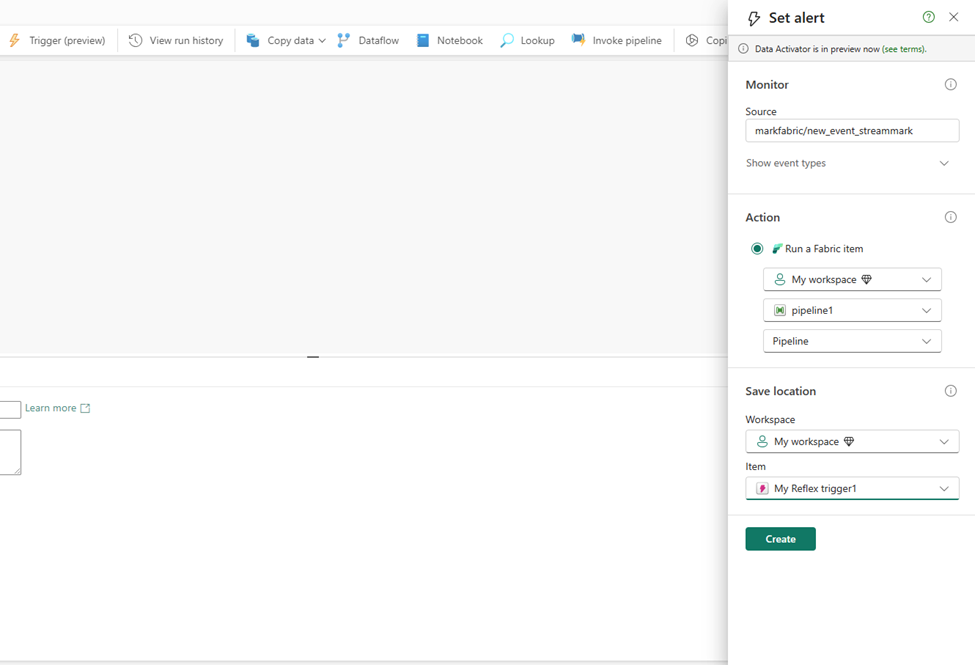
Parent/Child pipeline pattern monitoring improvements
Today, in Fabric Data Factory Data Pipelines, when you call another pipeline using the Invoke Pipeline activity, the child pipeline is not visible in the monitoring view. We have made updates to the Invoke Pipeline activity so that you can view your child pipeline runs. This requires an upgrade to any pipelines that you have in Fabric that already use the current Invoke Pipeline activity. You will be prompted to upgrade when you edit your pipeline and then provide a connection to your workspace to authenticate. Another additional new feature that will light up with this invoke pipeline activity update is the ability to invoke pipeline across workspaces in Fabric.
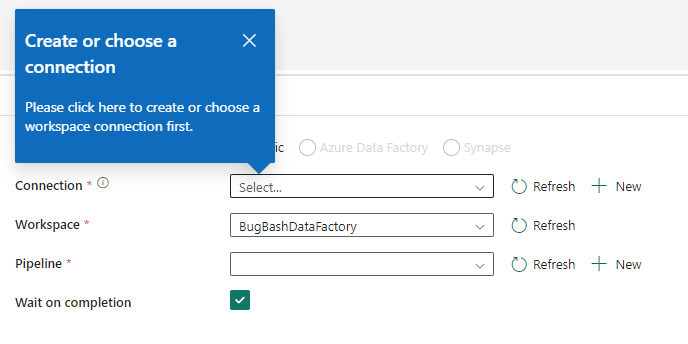
We are excited to announce the availability of the Fabric Spark job definition activity for data pipelines. With this new activity, you will be able to run a Fabric Spark Job definition directly in your pipeline. Detailed monitoring capabilities of your Spark Job definition will be coming soon!
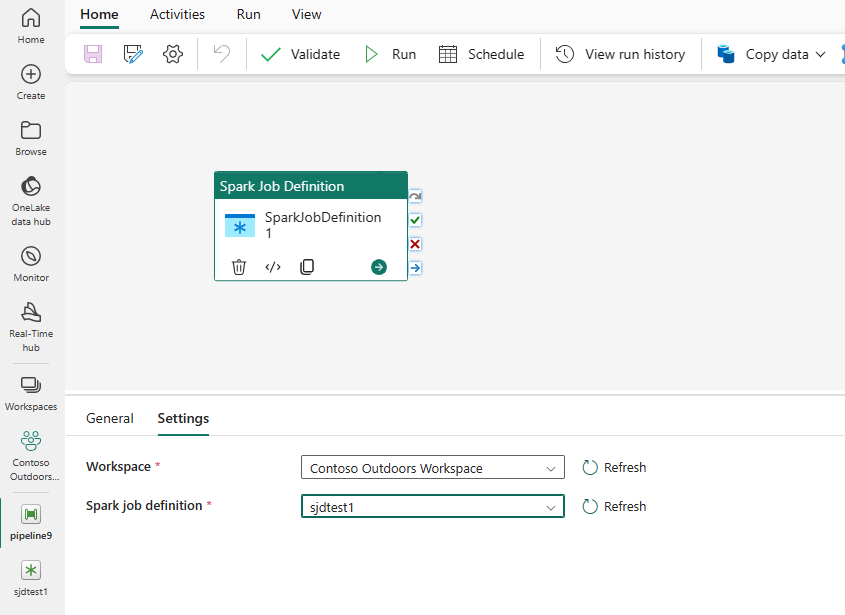
To learn more about this activity, read https://aka.ms/SparkJobDefinitionActivity
We are excited to announce the availability of the Azure HDInsight activity for data pipelines. The Azure HDInsight activity allows you to execute Hive queries, invoke a MapReduce program, execute Pig queries, execute a Spark program, or a Hadoop Stream program. Invoking either of the 5 activities can be done in a singular Azure HDInsight activity, and you can invoke this activity using your own or on-demand HDInsight cluster.
To learn more about this activity, read https://aka.ms/HDInsightsActivity
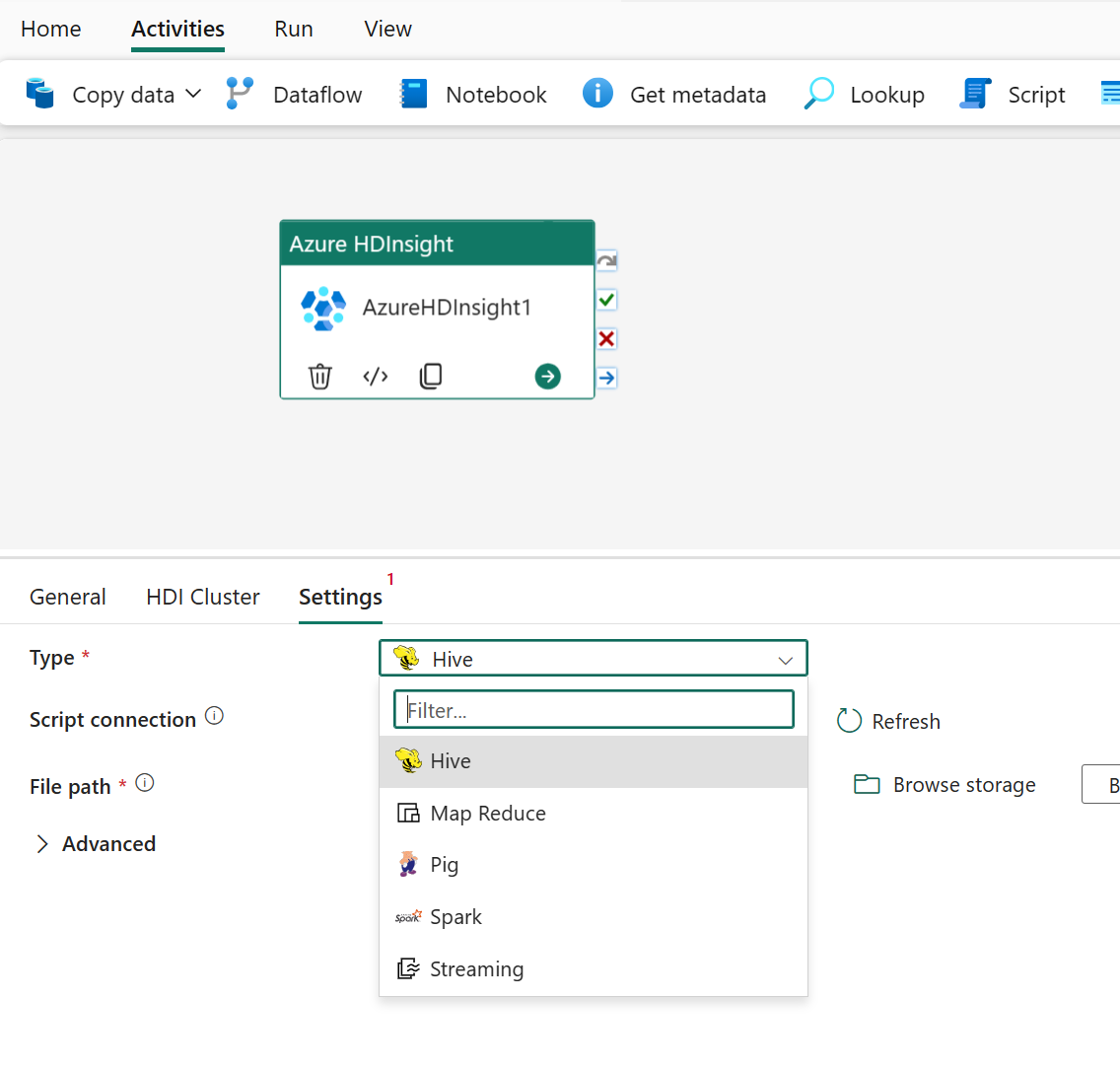
We are thrilled to share the new Modern Get Data experience in Data Pipeline to empower users intuitively and efficiently discover the right data, right connection info and credentials.
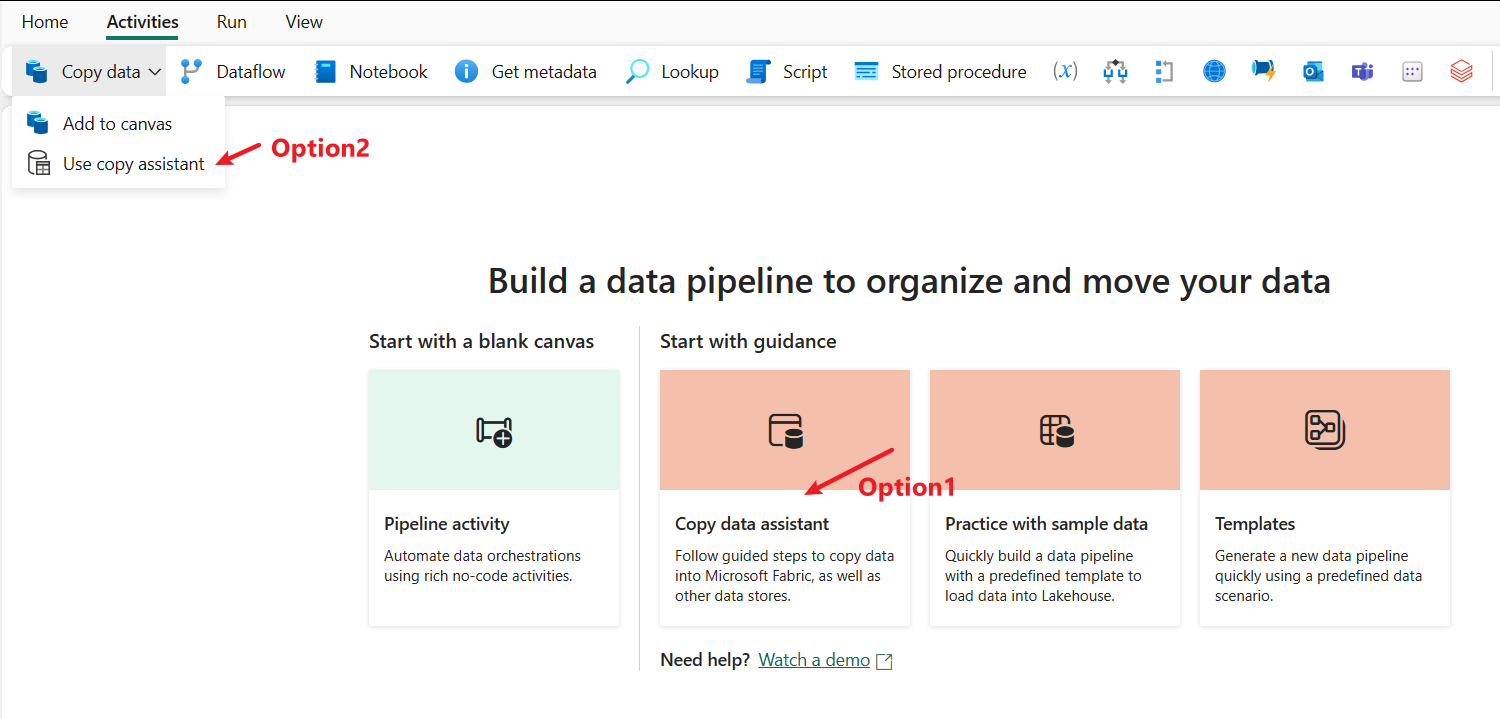
In the data destination, users can easily set destination by creating a new Fabric item or creating another destination or selecting existing Fabric item from OneLake data hub.
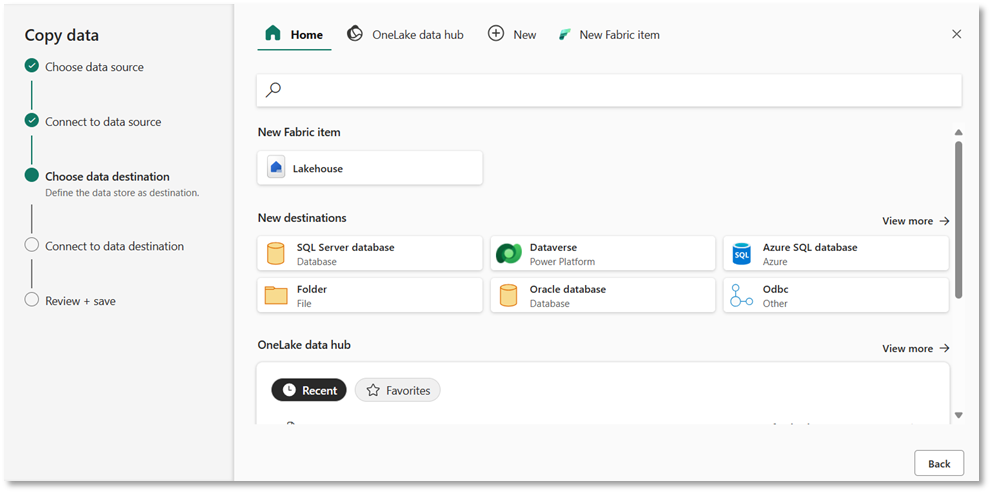
In the source tab of Copy activity, users can conveniently choose recent used connections from drop down or create a new connection using “More” option to interact with Modern Get Data experience.
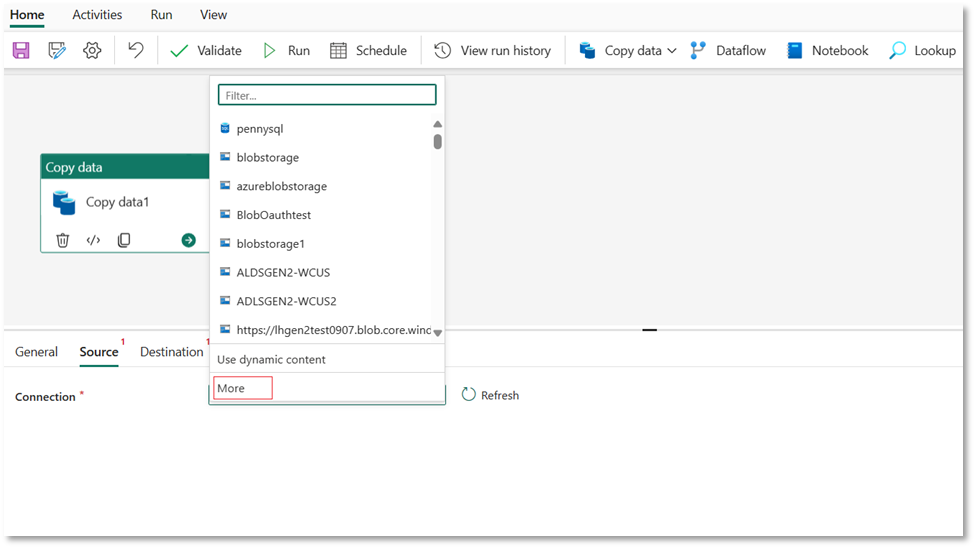
Related blog posts
Microsoft fabric april 2024 update.
Welcome to the April 2024 update! This month, you’ll find many great new updates, previews, and improvements. From Shortcuts to Google Cloud Storage and S3 compatible data sources in preview, Optimistic Job Admission for Fabric Spark, and New KQL Queryset Command Bar, that’s just a glimpse into this month’s update. There’s much more to explore! … Continue reading “Microsoft Fabric April 2024 Update”
Microsoft Fabric March 2024 Update
Welcome to the March 2024 update. We have a lot of great features this month including OneLake File Explorer, Autotune Query Tuning, Test Framework for Power Query SDK in VS Code, and many more! Earn a free Microsoft Fabric certification exam! We are thrilled to announce the general availability of Exam DP-600, which leads to … Continue reading “Microsoft Fabric March 2024 Update”
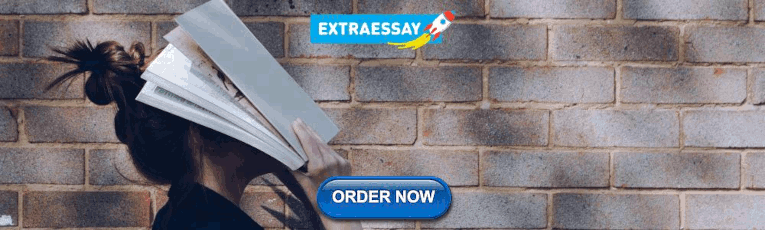
IMAGES
VIDEO
COMMENTS
TABULAR PRESENTATION OF DATA When to Use Tables • Written documents (reports, journal articles) typically present most results in tabular form. • Research Posters for conferences. • More concise format than graphs. • In oral presentations, only VERY simple tables should be presented.
As a result of this, it is simple to remember the statistical facts. Cost-effective: Tabular presentation is a very cost-effective way to convey data. It saves time and space. Provides Reference: As the data provided in a tabular presentation can be used for other studies and research, it acts as a source of reference.
The objectives of tabular data presentation are as follows. The tabular data presentation helps in simplifying the complex data. It also helps to compare different data sets thereby bringing out the important aspects. The tabular presentation provides the foundation for statistical analysis. The tabular data presentation further helps in the ...
The data is arranged in rows and columns. This is one of the most popularly used forms of presentation of data as data tables are simple to prepare and read. The most significant benefit of tabulation is that it coordinates data for additional statistical treatment and decision making. The analysis used in tabulation is of four types. They are:
Gary W. Oehlert. Tabular Display of Data. Or computer files. # Number of hawks responding to the "alarm" call # Variables are year (1999 or 2000), season (courtship, # nestling, fledgling), distance in meters between the # alarm call and the nest, number of hawks responding, # and number of. year season distance respond trials. 1 100 1 4.
In this method, we can arrange the data in tabular form in terms of frequency. For example, 3 students scored 50 marks. Hence, the frequency of 50 marks is 3. Now, let us construct the frequency distribution table for the given data. Therefore, the presentation of data is given as below:
👉Next Video : https://www.youtube.com/watch?v=MgGtxXeB9L0 ️📚👉 Watch Full Free Course:- https://www.magnetbrains.com ️📚👉 Get Notes Here: https://www ...
This page titled 7.3: Presenting Data in Tables is shared under a not declared license and was authored, remixed, and/or curated by John H. McDonald via source content that was edited to the style and standards of the LibreTexts platform; a detailed edit history is available upon request.
Sometime when you present you audience your research findings you need to do it with a table. Tables are difficult to be understood as they can show multiple dimensions together and often they use just captions to define a way to select data. When presenting records in a tabular way you risk your data isn't going to be recognized.
After collection of data (primary or secondary), it is necessary to summarize them suitably and present in such forms as can facilitate subsequent analysis and interpretation. There are two major tools/techniques for presentation of data as follows: Presentation in tabular form. Presentation in graphical form.
table is a perfectly valid presentation of the data, it's not a very efficient one. We need to summarize the values somehow, and present them using effective tabular and graphical methods, if we are to have any hope of seeing the patterns that are there. The form of such presentations is intimately connected to the type of data being examined.
In statistics, tabular data refers to data that is organized in a table with rows and columns. Within the table, the rows represent observations and the columns represent attributes for those observations. For example, the following table represents tabular data: This dataset has 9 rows and 5 columns. Each row represents one basketball player ...
To this end, large tables that we refer to as data inventories can be useful for compiling, in a tabular form, records of available data items (interviews, documents, images, videos, etc.), which can then be organized according to any number of useful criteria (such as time, people, roles, events, activities, issues, concepts—see Patton (2003 ...
The three main forms of presentation of data are: Textual presentation; Data tables; Diagrammatic presentation; Here we will be studying only the textual and tabular presentation, i.e. data tables in some detail. Textual Presentation. The discussion about the presentation of data starts off with it's most raw and vague form which is the ...
In this article, the techniques of data and information presentation in textual, tabular, and graphical forms are introduced. Text is the principal method for explaining findings, outlining trends, and providing contextual information. A table is best suited for representing individual information and represents both quantitative and ...
Steps for constructing a histogram is as follows. Step 1: Partition the data range into General guidelines are: classes. or. bins. Use between 6 and 15 bins. One suggested formula (Sturges) is: Number of Classes = 1 + 3.3 log(n) where n is the total number of observations. All bins should have the same width.
Abstract. The present paper aims to provide basic guidelines to present epidemiological data using tables and graphs in Dermatology. Although simple, the preparation of tables and graphs should follow basic recommendations, which make it much easier to understand the data under analysis and to promote accurate communication in science.
In Excel, type your frequencies into a column and then in the next column type in = (cell reference number)/35. Then copy and paste the formula in cell B2 down the page. If you used the cell reference number, Excel will automatically change the copied cells to the next row down.
Tabular Presentation of Data Tables present clear and organized data. A table must be clear and simple but complete. A good table should include the following parts. Table number and title -these are placed above the table. The title is usually written right after the table number.
Literature reviews offer a critical synthesis of empirical and theoretical literature to assess the strength of evidence, develop guidelines for practice and policymaking, and identify areas for future research.1 It is often essential and usually the first task in any research endeavour, particularly in masters or doctoral level education. For effective data extraction and rigorous synthesis ...
The textual presentation uses words to present the data.Tabular data is self-explanatory as there are segments that depict what the data wants to convey. The textual data need to be explained with words.The key difference thus is that the textual representation of data is subjective. In a tabular format, the data is mentioned in the form of ...
Related: 14 Data Modelling Tools For Data Analysis (With Features) Tabular Tabular presentation is using a table to share large amounts of information. When using this method, you organise data in rows and columns according to the characteristics of the data. Tabular presentation is useful in comparing data, and it helps visualise information.
Quick facts and stats. ACEs are common. About 64% of adults in the United States reported they had experienced at least one type of ACE before age 18. Nearly one in six (17.3%) adults reported they had experienced four or more types of ACEs. Preventing ACEs could potentially reduce many health conditions.
Learn the clinical signs and symptoms of West Nile virus disease. Clinical Testing and Diagnosis for West Nile Virus Disease. Transmission of West Nile Virus. West Nile Virus Resources. West Nile Virus Clinician Training - Free Continuing Medical Education (CME) Credit.
Activity Sheet-Organization and Presentation of Data in Textual, Tabular and Graphical Form Overview This resource can be used in providing real-life activity for students by conducting survey.
It displays stacked data layers, allowing users to compare multiple categories while maintaining data clarity. Horizon Charts are particularly useful to monitor and analyze complex data over time, making this a valuable visual for data analysis and decision-making. Key Features: Horizon Styles: Choose Natural, Linear, or Step with adjustable ...
Welcome to the May 2024 update. Here are a few, select highlights of the many we have for Fabric. You can now ask Copilot questions about data in your model, Model Explorer and authoring calculation groups in Power BI desktop is now generally available, and Real-Time Intelligence provides a complete end-to-end solution for ingesting, processing, analyzing, visualizing, monitoring, and acting ...