
Statistics Made Easy
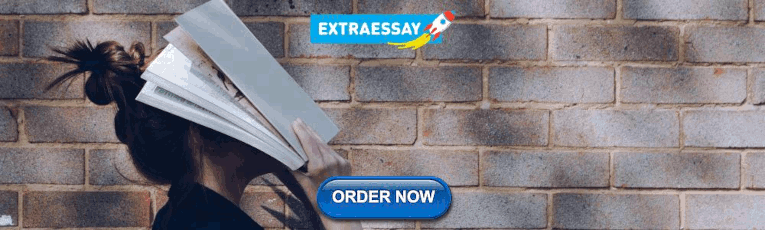
What is an Alternative Hypothesis in Statistics?
Often in statistics we want to test whether or not some assumption is true about a population parameter .
For example, we might assume that the mean weight of a certain population of turtle is 300 pounds.
To determine if this assumption is true, we’ll go out and collect a sample of turtles and weigh each of them. Using this sample data, we’ll conduct a hypothesis test .
The first step in a hypothesis test is to define the null and alternative hypotheses .
These two hypotheses need to be mutually exclusive, so if one is true then the other must be false.
These two hypotheses are defined as follows:
Null hypothesis (H 0 ): The sample data is consistent with the prevailing belief about the population parameter.
Alternative hypothesis (H A ): The sample data suggests that the assumption made in the null hypothesis is not true. In other words, there is some non-random cause influencing the data.
Types of Alternative Hypotheses
There are two types of alternative hypotheses:
A one-tailed hypothesis involves making a “greater than” or “less than ” statement. For example, suppose we assume the mean height of a male in the U.S. is greater than or equal to 70 inches.
The null and alternative hypotheses in this case would be:
- Null hypothesis: µ ≥ 70 inches
- Alternative hypothesis: µ < 70 inches
A two-tailed hypothesis involves making an “equal to” or “not equal to” statement. For example, suppose we assume the mean height of a male in the U.S. is equal to 70 inches.
- Null hypothesis: µ = 70 inches
- Alternative hypothesis: µ ≠ 70 inches
Note: The “equal” sign is always included in the null hypothesis, whether it is =, ≥, or ≤.
Examples of Alternative Hypotheses
The following examples illustrate how to define the null and alternative hypotheses for different research problems.
Example 1: A biologist wants to test if the mean weight of a certain population of turtle is different from the widely-accepted mean weight of 300 pounds.
The null and alternative hypothesis for this research study would be:
- Null hypothesis: µ = 300 pounds
- Alternative hypothesis: µ ≠ 300 pounds
If we reject the null hypothesis, this means we have sufficient evidence from the sample data to say that the true mean weight of this population of turtles is different from 300 pounds.
Example 2: An engineer wants to test whether a new battery can produce higher mean watts than the current industry standard of 50 watts.
- Null hypothesis: µ ≤ 50 watts
- Alternative hypothesis: µ > 50 watts
If we reject the null hypothesis, this means we have sufficient evidence from the sample data to say that the true mean watts produced by the new battery is greater than the current industry standard of 50 watts.
Example 3: A botanist wants to know if a new gardening method produces less waste than the standard gardening method that produces 20 pounds of waste.
- Null hypothesis: µ ≥ 20 pounds
- Alternative hypothesis: µ < 20 pounds
If we reject the null hypothesis, this means we have sufficient evidence from the sample data to say that the true mean weight produced by this new gardening method is less than 20 pounds.
When to Reject the Null Hypothesis
Whenever we conduct a hypothesis test, we use sample data to calculate a test-statistic and a corresponding p-value.
If the p-value is less than some significance level (common choices are 0.10, 0.05, and 0.01), then we reject the null hypothesis.
This means we have sufficient evidence from the sample data to say that the assumption made by the null hypothesis is not true.
If the p-value is not less than some significance level, then we fail to reject the null hypothesis.
This means our sample data did not provide us with evidence that the assumption made by the null hypothesis was not true.
Additional Resource: An Explanation of P-Values and Statistical Significance
Featured Posts

Hey there. My name is Zach Bobbitt. I have a Masters of Science degree in Applied Statistics and I’ve worked on machine learning algorithms for professional businesses in both healthcare and retail. I’m passionate about statistics, machine learning, and data visualization and I created Statology to be a resource for both students and teachers alike. My goal with this site is to help you learn statistics through using simple terms, plenty of real-world examples, and helpful illustrations.
Leave a Reply Cancel reply
Your email address will not be published. Required fields are marked *
- Math Article
Alternative Hypothesis
Alternative hypothesis defines there is a statistically important relationship between two variables. Whereas null hypothesis states there is no statistical relationship between the two variables. In statistics, we usually come across various kinds of hypotheses. A statistical hypothesis is supposed to be a working statement which is assumed to be logical with given data. It should be noticed that a hypothesis is neither considered true nor false.
The alternative hypothesis is a statement used in statistical inference experiment. It is contradictory to the null hypothesis and denoted by H a or H 1 . We can also say that it is simply an alternative to the null. In hypothesis testing, an alternative theory is a statement which a researcher is testing. This statement is true from the researcher’s point of view and ultimately proves to reject the null to replace it with an alternative assumption. In this hypothesis, the difference between two or more variables is predicted by the researchers, such that the pattern of data observed in the test is not due to chance.
To check the water quality of a river for one year, the researchers are doing the observation. As per the null hypothesis, there is no change in water quality in the first half of the year as compared to the second half. But in the alternative hypothesis, the quality of water is poor in the second half when observed.
Difference Between Null and Alternative Hypothesis
Basically, there are three types of the alternative hypothesis, they are;
Left-Tailed : Here, it is expected that the sample proportion (π) is less than a specified value which is denoted by π 0 , such that;
H 1 : π < π 0
Right-Tailed: It represents that the sample proportion (π) is greater than some value, denoted by π 0 .
H 1 : π > π 0
Two-Tailed: According to this hypothesis, the sample proportion (denoted by π) is not equal to a specific value which is represented by π 0 .
H 1 : π ≠ π 0
Note: The null hypothesis for all the three alternative hypotheses, would be H 1 : π = π 0 .

- Share Share
Register with BYJU'S & Download Free PDFs
Register with byju's & watch live videos.


Want to create or adapt books like this? Learn more about how Pressbooks supports open publishing practices.
1.3 The Economists’ Tool Kit
Learning objectives.
- Explain how economists test hypotheses, develop economic theories, and use models in their analyses.
- Explain how the all-other-things unchanged (ceteris paribus) problem and the fallacy of false cause affect the testing of economic hypotheses and how economists try to overcome these problems.
- Distinguish between normative and positive statements.
Economics differs from other social sciences because of its emphasis on opportunity cost, the assumption of maximization in terms of one’s own self-interest, and the analysis of choices at the margin. But certainly much of the basic methodology of economics and many of its difficulties are common to every social science—indeed, to every science. This section explores the application of the scientific method to economics.
Researchers often examine relationships between variables. A variable is something whose value can change. By contrast, a constant is something whose value does not change. The speed at which a car is traveling is an example of a variable. The number of minutes in an hour is an example of a constant.
Research is generally conducted within a framework called the scientific method , a systematic set of procedures through which knowledge is created. In the scientific method, hypotheses are suggested and then tested. A hypothesis is an assertion of a relationship between two or more variables that could be proven to be false. A statement is not a hypothesis if no conceivable test could show it to be false. The statement “Plants like sunshine” is not a hypothesis; there is no way to test whether plants like sunshine or not, so it is impossible to prove the statement false. The statement “Increased solar radiation increases the rate of plant growth” is a hypothesis; experiments could be done to show the relationship between solar radiation and plant growth. If solar radiation were shown to be unrelated to plant growth or to retard plant growth, then the hypothesis would be demonstrated to be false.
If a test reveals that a particular hypothesis is false, then the hypothesis is rejected or modified. In the case of the hypothesis about solar radiation and plant growth, we would probably find that more sunlight increases plant growth over some range but that too much can actually retard plant growth. Such results would lead us to modify our hypothesis about the relationship between solar radiation and plant growth.
If the tests of a hypothesis yield results consistent with it, then further tests are conducted. A hypothesis that has not been rejected after widespread testing and that wins general acceptance is commonly called a theory . A theory that has been subjected to even more testing and that has won virtually universal acceptance becomes a law . We will examine two economic laws in the next two chapters.
Even a hypothesis that has achieved the status of a law cannot be proven true. There is always a possibility that someone may find a case that invalidates the hypothesis. That possibility means that nothing in economics, or in any other social science, or in any science, can ever be proven true. We can have great confidence in a particular proposition, but it is always a mistake to assert that it is “proven.”
Models in Economics
All scientific thought involves simplifications of reality. The real world is far too complex for the human mind—or the most powerful computer—to consider. Scientists use models instead. A model is a set of simplifying assumptions about some aspect of the real world. Models are always based on assumed conditions that are simpler than those of the real world, assumptions that are necessarily false. A model of the real world cannot be the real world.
We will encounter our first economic model in Chapter 35 “Appendix A: Graphs in Economics” . For that model, we will assume that an economy can produce only two goods. Then we will explore the model of demand and supply. One of the assumptions we will make there is that all the goods produced by firms in a particular market are identical. Of course, real economies and real markets are not that simple. Reality is never as simple as a model; one point of a model is to simplify the world to improve our understanding of it.
Economists often use graphs to represent economic models. The appendix to this chapter provides a quick, refresher course, if you think you need one, on understanding, building, and using graphs.
Models in economics also help us to generate hypotheses about the real world. In the next section, we will examine some of the problems we encounter in testing those hypotheses.
Testing Hypotheses in Economics
Here is a hypothesis suggested by the model of demand and supply: an increase in the price of gasoline will reduce the quantity of gasoline consumers demand. How might we test such a hypothesis?
Economists try to test hypotheses such as this one by observing actual behavior and using empirical (that is, real-world) data. The average retail price of gasoline in the United States rose from an average of $2.12 per gallon on May 22, 2005 to $2.88 per gallon on May 22, 2006. The number of gallons of gasoline consumed by U.S. motorists rose 0.3% during that period.
The small increase in the quantity of gasoline consumed by motorists as its price rose is inconsistent with the hypothesis that an increased price will lead to an reduction in the quantity demanded. Does that mean that we should dismiss the original hypothesis? On the contrary, we must be cautious in assessing this evidence. Several problems exist in interpreting any set of economic data. One problem is that several things may be changing at once; another is that the initial event may be unrelated to the event that follows. The next two sections examine these problems in detail.
The All-Other-Things-Unchanged Problem
The hypothesis that an increase in the price of gasoline produces a reduction in the quantity demanded by consumers carries with it the assumption that there are no other changes that might also affect consumer demand. A better statement of the hypothesis would be: An increase in the price of gasoline will reduce the quantity consumers demand, ceteris paribus. Ceteris paribus is a Latin phrase that means “all other things unchanged.”
But things changed between May 2005 and May 2006. Economic activity and incomes rose both in the United States and in many other countries, particularly China, and people with higher incomes are likely to buy more gasoline. Employment rose as well, and people with jobs use more gasoline as they drive to work. Population in the United States grew during the period. In short, many things happened during the period, all of which tended to increase the quantity of gasoline people purchased.
Our observation of the gasoline market between May 2005 and May 2006 did not offer a conclusive test of the hypothesis that an increase in the price of gasoline would lead to a reduction in the quantity demanded by consumers. Other things changed and affected gasoline consumption. Such problems are likely to affect any analysis of economic events. We cannot ask the world to stand still while we conduct experiments in economic phenomena. Economists employ a variety of statistical methods to allow them to isolate the impact of single events such as price changes, but they can never be certain that they have accurately isolated the impact of a single event in a world in which virtually everything is changing all the time.
In laboratory sciences such as chemistry and biology, it is relatively easy to conduct experiments in which only selected things change and all other factors are held constant. The economists’ laboratory is the real world; thus, economists do not generally have the luxury of conducting controlled experiments.
The Fallacy of False Cause
Hypotheses in economics typically specify a relationship in which a change in one variable causes another to change. We call the variable that responds to the change the dependent variable ; the variable that induces a change is called the independent variable . Sometimes the fact that two variables move together can suggest the false conclusion that one of the variables has acted as an independent variable that has caused the change we observe in the dependent variable.
Consider the following hypothesis: People wearing shorts cause warm weather. Certainly, we observe that more people wear shorts when the weather is warm. Presumably, though, it is the warm weather that causes people to wear shorts rather than the wearing of shorts that causes warm weather; it would be incorrect to infer from this that people cause warm weather by wearing shorts.
Reaching the incorrect conclusion that one event causes another because the two events tend to occur together is called the fallacy of false cause . The accompanying essay on baldness and heart disease suggests an example of this fallacy.
Because of the danger of the fallacy of false cause, economists use special statistical tests that are designed to determine whether changes in one thing actually do cause changes observed in another. Given the inability to perform controlled experiments, however, these tests do not always offer convincing evidence that persuades all economists that one thing does, in fact, cause changes in another.
In the case of gasoline prices and consumption between May 2005 and May 2006, there is good theoretical reason to believe the price increase should lead to a reduction in the quantity consumers demand. And economists have tested the hypothesis about price and the quantity demanded quite extensively. They have developed elaborate statistical tests aimed at ruling out problems of the fallacy of false cause. While we cannot prove that an increase in price will, ceteris paribus, lead to a reduction in the quantity consumers demand, we can have considerable confidence in the proposition.
Normative and Positive Statements
Two kinds of assertions in economics can be subjected to testing. We have already examined one, the hypothesis. Another testable assertion is a statement of fact, such as “It is raining outside” or “Microsoft is the largest producer of operating systems for personal computers in the world.” Like hypotheses, such assertions can be demonstrated to be false. Unlike hypotheses, they can also be shown to be correct. A statement of fact or a hypothesis is a positive statement .
Although people often disagree about positive statements, such disagreements can ultimately be resolved through investigation. There is another category of assertions, however, for which investigation can never resolve differences. A normative statement is one that makes a value judgment. Such a judgment is the opinion of the speaker; no one can “prove” that the statement is or is not correct. Here are some examples of normative statements in economics: “We ought to do more to help the poor.” “People in the United States should save more.” “Corporate profits are too high.” The statements are based on the values of the person who makes them. They cannot be proven false.
Because people have different values, normative statements often provoke disagreement. An economist whose values lead him or her to conclude that we should provide more help for the poor will disagree with one whose values lead to a conclusion that we should not. Because no test exists for these values, these two economists will continue to disagree, unless one persuades the other to adopt a different set of values. Many of the disagreements among economists are based on such differences in values and therefore are unlikely to be resolved.
Key Takeaways
- Economists try to employ the scientific method in their research.
- Scientists cannot prove a hypothesis to be true; they can only fail to prove it false.
- Economists, like other social scientists and scientists, use models to assist them in their analyses.
- Two problems inherent in tests of hypotheses in economics are the all-other-things-unchanged problem and the fallacy of false cause.
- Positive statements are factual and can be tested. Normative statements are value judgments that cannot be tested. Many of the disagreements among economists stem from differences in values.
Look again at the data in Table 1.1 “LSAT Scores and Undergraduate Majors” . Now consider the hypothesis: “Majoring in economics will result in a higher LSAT score.” Are the data given consistent with this hypothesis? Do the data prove that this hypothesis is correct? What fallacy might be involved in accepting the hypothesis?
Case in Point: Does Baldness Cause Heart Disease?
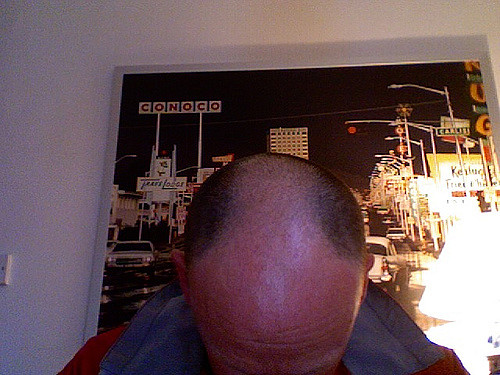
Mark Hunter – bald – CC BY-NC-ND 2.0.
A website called embarrassingproblems.com received the following email:
What did Dr. Margaret answer? Most importantly, she did not recommend that the questioner take drugs to treat his baldness, because doctors do not think that the baldness causes the heart disease. A more likely explanation for the association between baldness and heart disease is that both conditions are affected by an underlying factor. While noting that more research needs to be done, one hypothesis that Dr. Margaret offers is that higher testosterone levels might be triggering both the hair loss and the heart disease. The good news for people with early balding (which is really where the association with increased risk of heart disease has been observed) is that they have a signal that might lead them to be checked early on for heart disease.
Source: http://www.embarrassingproblems.com/problems/problempage230701.htm .
Answer to Try It! Problem
The data are consistent with the hypothesis, but it is never possible to prove that a hypothesis is correct. Accepting the hypothesis could involve the fallacy of false cause; students who major in economics may already have the analytical skills needed to do well on the exam.
Principles of Economics Copyright © 2016 by University of Minnesota is licensed under a Creative Commons Attribution-NonCommercial-ShareAlike 4.0 International License , except where otherwise noted.
- About the IMF
- Capacity Development
- Publications
Back to Basics
What is econometrics.
Finance & Development, December 2011, Vol. 48, No. 4
Sam Ouliaris
PDF version
Taking a theory and quantifying it
ECONOMISTS develop economic models to explain consistently recurring relationships. Their models link one or more economic variables to other economic variables (see “ What Are Economic Models ,” F&D , June 2011). For example, economists connect the amount individuals spend on consumer goods to disposable income and wealth, and expect consumption to increase as disposable income and wealth increase (that is, the relationship is positive).
There are often competing models capable of explaining the same recurring relationship, called an empirical regularity, but few models provide useful clues to the magnitude of the association. Yet this is what matters most to policymakers. When setting monetary policy, for example, central bankers need to know the likely impact of changes in official interest rates on inflation and the growth rate of the economy. It is in cases like this that economists turn to econometrics.
Econometrics uses economic theory, mathematics, and statistical inference to quantify economic phenomena. In other words, it turns theoretical economic models into useful tools for economic policymaking. The objective of econometrics is to convert qualitative statements (such as “the relationship between two or more variables is positive”) into quantitative statements (such as “consumption expenditure increases by 95 cents for every one dollar increase in disposable income”). Econometricians—practitioners of econometrics—transform models developed by economic theorists into versions that can be estimated. As Stock and Watson (2007) put it, “econometric methods are used in many branches of economics, including finance, labor economics, macroeconomics, microeconomics, and economic policy.” Economic policy decisions are rarely made without econometric analysis to assess their impact.
A daunting task
Certain features of economic data make it challenging for economists to quantify economic models. Unlike researchers in the physical sciences, econometricians are rarely able to conduct controlled experiments in which only one variable is changed and the response of the subject to that change is measured. Instead, econometricians estimate economic relationships using data generated by a complex system of related equations, in which all variables may change at the same time. That raises the question of whether there is even enough information in the data to identify the unknowns in the model.
Econometrics can be divided into theoretical and applied components.
Theoretical econometricians investigate the properties of existing statistical tests and procedures for estimating unknowns in the model. They also seek to develop new statistical procedures that are valid (or robust) despite the peculiarities of economic data—such as their tendency to change simultaneously. Theoretical econometrics relies heavily on mathematics, theoretical statistics, and numerical methods to prove that the new procedures have the ability to draw correct inferences.
Applied econometricians, by contrast, use econometric techniques developed by the theorists to translate qualitative economic statements into quantitative ones. Because applied econometricians are closer to the data, they often run into—and alert their theoretical counterparts to—data attributes that lead to problems with existing estimation techniques. For example, the econometrician might discover that the variance of the data (how much individual values in a series differ from the overall average) is changing over time.
The main tool of econometrics is the linear multiple regression model, which provides a formal approach to estimating how a change in one economic variable, the explanatory variable, affects the variable being explained, the dependent variable—taking into account the impact of all the other determinants of the dependent variable. This qualification is important because a regression seeks to estimate the marginal impact of a particular explanatory variable after taking into account the impact of the other explanatory variables in the model (see “ Regressions: Why Are Economists Obsessed with Them? ” F&D , March 2006). For example, the model may try to isolate the effect of a 1 percentage point increase in taxes on average household consumption expenditure, holding constant other determinants of consumption, such as pretax income, wealth, and interest rates.
Stages of development
The methodology of econometrics is fairly straightforward.
The first step is to suggest a theory or hypothesis to explain the data being examined. The explanatory variables in the model are specified, and the sign and/or magnitude of the relationship between each explanatory variable and the dependent variable are clearly stated. At this stage of the analysis, applied econometricians rely heavily on economic theory to formulate the hypothesis. For example, a tenet of international economics is that prices across open borders move together after allowing for nominal exchange rate movements (purchasing power parity). The empirical relationship between domestic prices and foreign prices (adjusted for nominal exchange rate movements) should be positive, and they should move together approximately one for one.
The second step is the specification of a statistical model that captures the essence of the theory the economist is testing. The model proposes a specific mathematical relationship between the dependent variable and the explanatory variables—on which, unfortunately, economic theory is usually silent. By far the most common approach is to assume linearity—meaning that any change in an explanatory variable will always produce the same change in the dependent variable (that is, a straight-line relationship).
Because it is impossible to account for every influence on the dependent variable, a catchall variable is added to the statistical model to complete its specification. The role of the catchall is to represent all the determinants of the dependent variable that cannot be accounted for—because of either the complexity of the data or its absence. Economists usually assume that this “error” term averages to zero and is unpredictable, simply to be consistent with the premise that the statistical model accounts for all the important explanatory variables.
The third step involves using an appropriate statistical procedure and an econometric software package to estimate the unknown parameters (coefficients) of the model using economic data. This is often the easiest part of the analysis thanks to readily available economic data and excellent econometric software. Still, the famous GIGO (garbage in, garbage out) principle of computing also applies to econometrics. Just because something can be computed doesn’t mean it makes economic sense to do so.
The fourth step is by far the most important: administering the smell test. Does the estimated model make economic sense—that is, yield meaningful economic predictions? For example, are the signs of the estimated parameters that connect the dependent variable to the explanatory variables consistent with the predictions of the underlying economic theory? (In the household consumption example, for instance, the validity of the statistical model would be in question if it predicted a decline in consumer spending when income increased). If the estimated parameters do not make sense, how should the econometrician change the statistical model to yield sensible estimates? And does a more sensible estimate imply an economically significant effect? This step, in particular, calls on and tests the applied econometrician’s skill and experience.
Testing the hypothesis
The main tool of the fourth stage is hypothesis testing, a formal statistical procedure during which the researcher makes a specific statement about the true value of an economic parameter, and a statistical test determines whether the estimated parameter is consistent with that hypothesis. If it is not, the researcher must either reject the hypothesis or make new specifications in the statistical model and start over.
If all four stages proceed well, the result is a tool that can be used to assess the empirical validity of an abstract economic model. The empirical model may also be used to construct a way to forecast the dependent variable, potentially helping policymakers make decisions about changes in monetary and/or fiscal policy to keep the economy on an even keel.
Students of econometrics are often fascinated by the ability of linear multiple regression to estimate economic relationships. Three fundamentals of econometrics are worth remembering.
• First, the quality of the parameter estimates depends on the validity of the underlying economic model.
• Second, if a relevant explanatory variable is excluded, the most likely outcome is poor parameter estimates.
• Third, even if the econometrician identifies the process that actually generated the data, the parameter estimates have only a slim chance of being equal to the actual parameter values that generated the data. Nevertheless, the estimates will be used because, statistically speaking, they will become precise as more data become available.
Econometrics, by design, can yield correct predictions on average, but only with the help of sound economics to guide the specification of the empirical model. Even though it is a science, with well-established rules and procedures for fitting models to economic data, in practice econometrics is an art that requires considerable judgment to obtain estimates useful for policymaking. ■
Sam Ouliaris is a Senior Economist in the IMF Institute.

- Archive of F&D Issues

Write to us
F&D welcomes comments and brief letters, a selection of which are posted under Letters to the Editor. Letters may be edited. Please send your letters to [email protected]
F&D Magazine
- About F&D
- Advertising Information
- Subscription Information
- Copyright Information
- Writing Guidelines
- Use the free Adobe Acrobat Reader to view pdf files
Free Email Notification
Receive emails when we post new items of interest to you. Subscribe or Modify your profile
- Country Info
- Data and Statistics
- Copyright and Usage
- Privacy Policy
- How to Contact Us
- Français
- Español
- Search Search Please fill out this field.
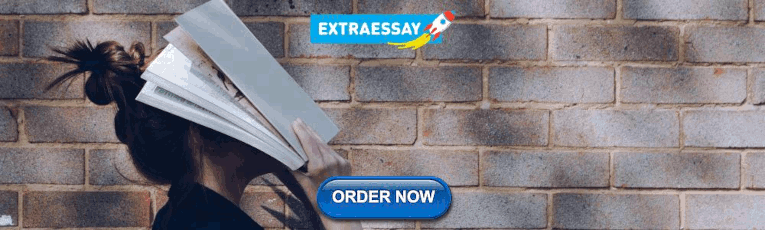
What Is Econometrics?
Understanding econometrics.
- Limitations
- Econometrics FAQs
The Bottom Line
- Corporate Finance
- Financial Analysis
Econometrics: Definition, Models, and Methods
Adam Hayes, Ph.D., CFA, is a financial writer with 15+ years Wall Street experience as a derivatives trader. Besides his extensive derivative trading expertise, Adam is an expert in economics and behavioral finance. Adam received his master's in economics from The New School for Social Research and his Ph.D. from the University of Wisconsin-Madison in sociology. He is a CFA charterholder as well as holding FINRA Series 7, 55 & 63 licenses. He currently researches and teaches economic sociology and the social studies of finance at the Hebrew University in Jerusalem.
:max_bytes(150000):strip_icc():format(webp)/adam_hayes-5bfc262a46e0fb005118b414.jpg)
Econometrics is the use of statistical and mathematical models to develop theories or test existing hypotheses in economics and to forecast future trends from historical data. It subjects real-world data to statistical trials and then compares the results against the theory being tested.
Depending on whether you are interested in testing an existing theory or in using existing data to develop a new hypothesis, econometrics can be subdivided into two major categories: theoretical and applied. Those who routinely engage in this practice are commonly known as econometricians.
Key Takeaways
- Econometrics is the use of statistical methods to develop theories or test existing hypotheses in economics or finance.
- Econometrics relies on techniques such as regression models and null hypothesis testing.
- Econometrics can also be used to try to forecast future economic or financial trends.
- As with other statistical tools, econometricians should be careful not to infer a causal relationship from statistical correlation.
- Some economists have criticized the field of econometrics for prioritizing statistical models over economic reasoning.
Investopedia / Michela Buttignol
Econometrics analyzes data using statistical methods in order to test or develop economic theory. These methods rely on statistical inferences to quantify and analyze economic theories by leveraging tools such as frequency distributions , probability, and probability distributions , statistical inference, correlation analysis, simple and multiple regression analysis, simultaneous equations models, and time series methods.
Econometrics was pioneered by Lawrence Klein , Ragnar Frisch, and Simon Kuznets . All three won the Nobel Prize in economics for their contributions. Today, it is used regularly among academics as well as practitioners such as Wall Street traders and analysts.
An example of the application of econometrics is to study the income effect using observable data. An economist may hypothesize that as a person increases their income, their spending will also increase.
If the data show that such an association is present, a regression analysis can then be conducted to understand the strength of the relationship between income and consumption and whether or not that relationship is statistically significant—that is, it appears to be unlikely that it is due to chance alone.
Methods of Econometrics
The first step to econometric methodology is to obtain and analyze a set of data and define a specific hypothesis that explains the nature and shape of the set. This data may be, for example, the historical prices for a stock index, observations collected from a survey of consumer finances, or unemployment and inflation rates in different countries.
If you are interested in the relationship between the annual price change of the S&P 500 and the unemployment rate, you'd collect both sets of data. Then, you might test the idea that higher unemployment leads to lower stock market prices. In this example, stock market price would be the dependent variable and the unemployment rate is the independent or explanatory variable.
The most common relationship is linear, meaning that any change in the explanatory variable will have a positive correlation with the dependent variable. This relationship could be explored with a simple regression model, which amounts to generating a best-fit line between the two sets of data and then testing to see how far each data point is, on average, from that line.
Note that you can have several explanatory variables in your analysis—for example, changes to GDP and inflation in addition to unemployment in explaining stock market prices. When more than one explanatory variable is used, it is referred to as multiple linear regression . This is the most commonly used tool in econometrics.
Some economists, including John Maynard Keynes , have criticized econometricians for their over-reliance on statistical correlations in lieu of economic thinking.
Different Regression Models
There are several different regression models that are optimized depending on the nature of the data being analyzed and the type of question being asked. The most common example is the ordinary least squares (OLS) regression, which can be conducted on several types of cross-sectional or time-series data. If you're interested in a binary (yes-no) outcome—for instance, how likely you are to be fired from a job based on your productivity—you might use a logistic regression or a probit model. Today, econometricians have hundreds of models at their disposal.
Econometrics is now conducted using statistical analysis software packages designed for these purposes, such as STATA, SPSS, or R. These software packages can also easily test for statistical significance to determine the likelihood that correlations might arise by chance. R-squared , t-tests , p-values , and null-hypothesis testing are all methods used by econometricians to evaluate the validity of their model results.
Limitations of Econometrics
Econometrics is sometimes criticized for relying too heavily on the interpretation of raw data without linking it to established economic theory or looking for causal mechanisms. It is crucial that the findings revealed in the data are able to be adequately explained by a theory, even if that means developing your own theory of the underlying processes.
Regression analysis also does not prove causation, and just because two data sets show an association, it may be spurious. For example, drowning deaths in swimming pools increase with GDP. Does a growing economy cause people to drown? This is unlikely, but perhaps more people buy pools when the economy is booming. Econometrics is largely concerned with correlation analysis, and it is important to remember that correlation does not equal causation.
What Are Estimators in Econometrics?
An estimator is a statistic that is used to estimate some fact or measurement about a larger population. Estimators are frequently used in situations where it is not practical to measure the entire population. For example, it is not possible to measure the exact employment rate at any specific time, but it is possible to estimate unemployment based on a randomly-chosen sample of the population.
What Is Autocorrelation in Econometrics?
Autocorrelation measures the relationships between a single variable at different time periods. For this reason, it is sometimes called lagged correlation or serial correlation, since it is used to measure how the past value of a certain variable might predict future values of the same variable. Autocorrelation is a useful tool for traders, especially in technical analysis.
What Is Endogeneity in Econometrics?
An endogenous variable is a variable that is influenced by changes in another variable. Due to the complexity of economic systems, it is difficult to determine all of the subtle relationships between different factors, and some variables may be partially endogenous and partially exogenous. In econometric studies, the researchers must be careful to account for the possibility that the error term may be partially correlated with other variables.
Econometrics is a popular discipline that integrates statistical tools and modeling for economic data, and it is frequently used by policymakers to forecast the result of policy changes. Like with other statistical tools, there are many possibilities for error when econometric tools are used carelessly. Econometricians must be careful to justify their conclusions with sound reasoning as well as statistical inferences.
The Nobel Prize. " Simon Kuznets ."
The Nobel Prize. " Ragnar Frisch ."
The Nobel Prize. " Lawrence R. Klein ."
Statistics How To. " Endogenous Variable and Exogenous Variable ."
:max_bytes(150000):strip_icc():format(webp)/regression-4190330-ab4b9c8673074b01985883d2aae8b9b3.jpg)
- Terms of Service
- Editorial Policy
- Privacy Policy
- Your Privacy Choices
Principles of Econometrics with \(R\)
Chapter 8 heteroskedasticity.
Reference for the package sandwich (Lumley and Zeileis 2015 ) .
One of the assumptions of the Gauss-Markov theorem is homoskedasticity , which requires that all observations of the response (dependent) variable come from distributions with the same variance \(\sigma^2\) . In many economic applications, however, the spread of \(y\) tends to depend on one or more of the regressors \(x\) . For example, in the food simple regression model (Equation \ref{eq:foodagain8}) expenditure on food stays closer to its mean (regression line) at lower incomes and to be more spread about its mean at higher incomes. Think just that people have more choices at higher income whether to spend their extra income on food or something else.
In the presence of heteroskedasticity, the coefficient estimators are still unbiased, but their variance is incorrectly calculated by the usual OLS method, which makes confidence intervals and hypothesis testing incorrect as well. Thus, new methods need to be applied to correct the variances.
8.1 Spotting Heteroskedasticity in Scatter Plots
When the variance of \(y\) , or of \(e\) , which is the same thing, is not constant, we say that the response or the residuals are heteroskedastic . Figure 8.1 shows, again, a scatter diagram of the food dataset with the regression line to show how the observations tend to be more spread at higher income.

Figure 8.1: Heteroskedasticity in the ‘food’ data
Another useful method to visualize possible heteroskedasticity is to plot the residuals against the regressors suspected of creating heteroskedasticity, or, more generally, against the fitted values of the regression. Figure 8.2 shows both these options for the simple food_exp model.

Figure 8.2: Residual plots in the ‘food’ model
8.2 Heteroskedasticity Tests
The test we are construction assumes that the variance of the errors is a function \(h\) of a number of regressors \(z_{s}\) , which may or may not be present in the initial regression model that we want to test. Equation \ref{eq:hetfctn8} shows the general form of the variance function.
Te relevant test statistic is \(\chi ^2\) , given by Equation \ref{eq:chisq8}, where \(R^2\) is the one resulted from Equation \ref{eq:hetres8}.
The Breusch-Pagan heteroskedasticiy test uses the method we have just described, where the regressors \(z_{s}\) are the variables \(x_{k}\) in the initial model. Let us apply this test to the food model. The function to determine a critical value of the \(\chi ^2\) distribution for a significance level \(\alpha\) and \(S-1\) degrees of freedom is qchisq(1-alpha, S-1 ).
Our test yields a value of the test statistic \(\chi ^2\) of \(7.38\) , which is to be compared to the critical \(\chi^{2}_{cr}\) having \(S-1=1\) degrees of freedom and \(\alpha = 0.05\) . This critical value is \(\chi ^{2}_{cr}=3.84\) . Since the calculated \(\chi ^2\) exceeds the critical value, we reject the null hypothesis of homoskedasticity, which means there is heteroskedasticity in our data and model. Alternatively, we can find the \(p\) -value corresponding to the calculated \(\chi^{2}\) , \(p=0.007\) .
Let us now do the same test, but using a White version of the residuals equation, in its quadratic form.
The calculated \(p\) -value in this version is \(p=0.023\) , which also implies rejection of the null hypothesis of homoskedasticity.
The function bptest() in package lmtest does (the robust version of) the Breusch-Pagan test in \(R\) . The following code applies this function to the basic food equation, showing the results in Table 8.1 , where ‘statistic’ is the calculated \(\chi^2\) .
The test statistic when the null hyppthesis is true, given in Equation \ref{eq:gqf8}, has an \(F\) distribution with its two degrees of freedom equal to the degrees of freedom of the two subsamples, respectively \(N_{1}-K\) and \(N_{0}-K\) .
Let us apply this test to a \(wage\) equation based on the dataset \(cps2\) , where \(metro\) is an indicator variable equal to \(1\) if the individual lives in a metropolitan area and \(0\) for rural area. I will split the dataset in two based on the indicator variable \(metro\) and apply the regression model (Equation \ref{eq:hetwage8}) separately to each group.
The results of these calculations are as follows: calculated \(F\) statistic \(F=2.09\) , the lower tail critical value \(F_{lc}=0.81\) , and the upper tail critical value \(F_{uc}=1.26\) . Since the calculated amount is greater than the upper critical value, we reject the hypothesis that the two variances are equal, facing, thus, a heteroskedasticity problem. If one expects the variance in the metropolitan area to be higher and wants to test the (alternative) hypothesis \(H_{0}:\sigma^{2}_{1}\leq \sigma^{2}_{0},\;\;\;\; H_{A}:\sigma^{2}_{1}>\sigma^{2}_{0}\) , one needs to re-calcuate the critical value for \(\alpha=0.05\) as follows:
The critical value for the right tail test is \(F_{c}=1.22\) , which still implies rejecting the null hypothesis.
The Goldfeld-Quant test can be used even when there is no indicator variable in the model or in the dataset. One can split the dataset in two using an arbitrary rule. Let us apply the method to the basic \(food\) equation, with the data split in low-income ( \(li\) ) and high-income ( \(hi\) ) halves. The cutoff point is, in this case, the median income, and the hypothesis to be tested \[H_{0}: \sigma^{2}_{hi}\le \sigma^{2}_{li},\;\;\;\;H_{A}:\sigma^{2}_{hi} > \sigma^{2}_{li}\]
The resulting \(F\) statistic in the \(food\) example is \(F=3.61\) , which is greater than the critical value \(F_{cr}=2.22\) , rejecting the null hypothesis in favour of the alternative hypothesis that variance is higher at higher incomes. The \(p\) -value of the test is \(p=0.0046\) .
In the package lmtest , \(R\) has a specialized function to perform Goldfeld-Quandt tests, the function gqtest which takes, among other arguments, the formula describing the model to be tested, a break point specifying how the data should be split (percentage of the number of observations), what is the alternative hypothesis (“greater”, “two.sided”, or “less”), how the data should be ordered ( order.by= ), and data= . Let us apply gqtest() to the \(food\) example with the same partition as we have just did before.
Please note that the results from applying gqtest() (Table 8.2 are the same as those we have already calculated.
8.3 Heteroskedasticity-Consistent Standard Errors
Since the presence of heteroskedasticity makes the lest-squares standard errors incorrect, there is a need for another method to calculate them. White robust standard errors is such a method.
The \(R\) function that does this job is hccm() , which is part of the car package and yields a heteroskedasticity-robust coefficient covariance matrix. This matrix can then be used with other functions, such as coeftest() (instead of summary ), waldtest() (instead of anova ), or linearHypothesis() to perform hypothesis testing. The function hccm() takes several arguments, among which is the model for which we want the robust standard errors and the type of standard errors we wish to calculate. type can be “constant” (the regular homoskedastic errors), “hc0”, “hc1”, “hc2”, “hc3”, or “hc4”; “hc1” is the default type in some statistical software packages. Let us compute robust standard errors for the basic \(food\) equation and compare them with the regular (incorrect) ones.
When comparing Tables 8.3 and 8.4 , it can be observed that the robust standard errors are smaller and, since the coefficients are the same, the \(t\) -statistics are higher and the \(p\) -values are smaller. Lower \(p\) -values with robust standard errors is, however, the exception rather than the rule.
Next is an example of using robust standard errors when performing a fictitious linear hypothesis test on the basic ‘andy’ model, to test the hypothesis \(H_{0}: \beta_{2}+\beta_{3}=0\)
This example demonstrates how to introduce robust standards errors in a linearHypothesis function. It also shows that, when heteroskedasticity is not significant ( bptst does not reject the homoskedasticity hypothesis) the robust and regular standard errors (and therefore the \(F\) statistics of the tests) are very similar.
Just for completeness, I should mention that a similar function, with similar uses is the function vcov , which can be found in the package sandwich .
8.4 GLS: Known Form of Variance
Let us consider the regression equation given in Equation \ref{eq:genheteq8}), where the errors are assumed heteroskedastic.
Heteroskedasticity implies different variances of the error term for each observation. Ideally, one should be able to estimate the \(N\) variances in order to obtain reliable standard errors, but this is not possible. The second best in the absence of such estimates is an assumption of how variance depends on one or several of the regressors. The estimator obtained when using such an assumption is called a generalized least squares estimator, gls , which may involve a structure of the errors as proposed in Equation \ref{eq:glsvardef8}, which assumes a linear relationship between variance and the regressor \(x_{i}\) with the unknown parameter \(\sigma^2\) as a proportionality factor.
One way to circumvent guessing a proportionality factor in Equation \ref{eq:glsvardef8} is to transform the initial model in Equation \ref{eq:genheteq8} such that the error variance in the new model has the structure proposed in Equation \ref{eq:glsvardef8}. This can be achieved if the initial model is divided through by \(\sqrt x_{i}\) and estimate the new model shown in Equation \ref{eq:glsstar8}. If Equation \ref{eq:glsstar8} is correct, then the resulting estimator is BLUE.
In general, if the initial variables are multiplied by quantities that are specific to each observation, the resulting estimator is called a weighted least squares estimator, wls . Unlike the robust standard errors method for heteroskedasticity correction, gls or wls methods change the estimates of regression coefficients.
The function lm() can do wls estimation if the argument weights is provided under the form of a vector of the same size as the other variables in the model. \(R\) takes the square roots of the weights provided to multiply the variables in the regression. Thus, if you wish to multiply the model by \(\frac{1}{\sqrt {x_{i}}}\) , the weights should be \(w_{i}=\frac{1}{x_{i}}\) .
Let us apply these ideas to re-estimate the \(food\) equation, which we have determined to be affected by heteroskedasticity.
Tables 8.7 , 8.8 , and 8.9 compare ordinary least square model to a weighted least squares model and to OLS with robust standard errors. The WLS model multiplies the variables by \(1 \, / \, \sqrt{income}\) , where the weights provided have to be \(w=1\,/\, income\) . The effect of introducing the weights is a slightly lower intercept and, more importantly, different standard errors. Please note that the WLS standard errors are closer to the robust (HC1) standard errors than to the OLS ones.
8.5 Grouped Data
We have seen already (Equation \ref{eq:gqnull8}) how a dichotomous indicator variable splits the data in two groups that may have different variances. The generalized least squares method can account for group heteroskedasticity, by choosing appropriate weights for each group; if the variables are transformed by multiplying them by \(1/\sigma_{j}\) , for group \(j\) , the resulting model is homoskedastic. Since \(\sigma_{j}\) is unknown, we replace it with its estimate \(\hat \sigma_{j}\) . This method is named feasible generalized least squares .
The table titled “OLS, vs. FGLS estimates for the ‘cps2’ data” helps comparing the coefficients and standard errors of four models: OLS for rural area, OLS for metro area, feasible GLS with the whole dataset but with two types of weights, one for each area, and, finally, OLS with heteroskedasticity-consistent (HC1) standard errors. Please be reminded that the regular OLS standard errors are not to be trusted in the presence of heteroskedasticity.
The previous code sequence needs some explanation. It runs two regression models, rural.lm and metro.lm just to estimate \(\hat \sigma_{R}\) and \(\hat \sigma_{M}\) needed to calculate the weights for each group. The subsets, this time, were selected directly in the lm() function through the argument subset= , which takes as argument some logical expression that may involve one or more variables in the dataset. Then, I create a new vector of a size equal to the number of observations in the dataset, a vector that will be populated over the next few code lines with weights. I choose to create this vector as a new column of the dataset cps2 , a column named wght . With this the hard part is done; I just need to run an lm() model with the option weights=wght and that gives my FGLS coefficients and standard errors.
The next lines make a for loop runing through each observation. If observation \(i\) is a rural area observation, it receives a weight equal to \(1/\sigma_{R}^2\) ; otherwise, it receives the weight \(1/\sigma_{M}^2\) . Why did I square those \(sigmas\) ? Because, remember, the argument weights in the lm() function requires the square of the factor multiplying the regression model in the WLS method.
The remaining part of the code repeats models we ran before and places them in one table for making comparison easier.
8.6 GLS: Unknown Form of Variance
Equation \ref{eq:varfuneq8} uses the residuals from Equation \ref{eq:genericeq8} as estimates of the variances of the error terms and serves at estimating the functional form of the variance. If the assumed functional form of the variance is the exponential function \(var(e_{i})=\sigma_{i}^{2}=\sigma ^2 x_{i}^{\gamma}\) , then the regressors \(z_{is}\) in Equation \ref{eq:varfuneq8} are the logs of the initial regressors \(x_{is}\) , \(z_{is}=log(x_{is})\) .
The variance estimates for each error term in Equation \ref{eq:genericeq8} are the fitted values, \(\hat \sigma_{i}^2\) of Equation \ref{eq:varfuneq8}, which can then be used to construct a vector of weights for the regression model in Equation \ref{eq:genericeq8}. Let us follow these steps on the \(food\) basic equation where we assume that the variance of error term \(i\) is an unknown exponential function of income. So, the purpose of the following code fragment is to determine the weights and to supply them to the lm() function. Remember, lm() multiplies each observation by the square root of the weight you supply. For instance, if you want to multiply the observations by \(1/\sigma_{i}\) , you should supply the weight \(w_{i}=1/\sigma_{i}^2\) .
The table titled “Comparing various ‘food’ models” shows that the FGLS with unknown variances model substantially lowers the standard errors of the coefficients, which in turn increases the \(t\) -ratios (since the point estimates of the coefficients remain about the same), making an important difference for hypothesis testing.
For a few classes of variance functions, the weights in a GLS model can be calculated in \(R\) using the varFunc() and varWeights() functions in the package nlme .
8.7 Heteroskedasticity in the Linear Probability Model
As we have already seen, the linear probability model is, by definition, heteroskedastic, with the variance of the error term given by its binomial distribution parameter \(p\) , the probability that \(y\) is equal to 1, \(var(y)=p(1-p)\) , where \(p\) is defined in Equation \ref{eq:binomialp8}.
Thus, the linear probability model provides a known variance to be used with GLS, taking care that none of the estimated variances is negative. One way to avoid negative or greater than one probabilities is to artificially limit them to the interval \((0,1)\) .
Let us revise the \(coke\) model in dataset coke using this structure of the variance.
Lumley, Thomas, and Achim Zeileis. 2015. Sandwich: Robust Covariance Matrix Estimators . https://CRAN.R-project.org/package=sandwich .
9.1 Null and Alternative Hypotheses
The actual test begins by considering two hypotheses . They are called the null hypothesis and the alternative hypothesis . These hypotheses contain opposing viewpoints.
H 0 : The null hypothesis: It is a statement of no difference between the variables—they are not related. This can often be considered the status quo and as a result if you cannot accept the null it requires some action.
H a : The alternative hypothesis: It is a claim about the population that is contradictory to H 0 and what we conclude when we reject H 0 . This is usually what the researcher is trying to prove.
Since the null and alternative hypotheses are contradictory, you must examine evidence to decide if you have enough evidence to reject the null hypothesis or not. The evidence is in the form of sample data.
After you have determined which hypothesis the sample supports, you make a decision. There are two options for a decision. They are "reject H 0 " if the sample information favors the alternative hypothesis or "do not reject H 0 " or "decline to reject H 0 " if the sample information is insufficient to reject the null hypothesis.
Mathematical Symbols Used in H 0 and H a :
H 0 always has a symbol with an equal in it. H a never has a symbol with an equal in it. The choice of symbol depends on the wording of the hypothesis test. However, be aware that many researchers (including one of the co-authors in research work) use = in the null hypothesis, even with > or < as the symbol in the alternative hypothesis. This practice is acceptable because we only make the decision to reject or not reject the null hypothesis.
Example 9.1
H 0 : No more than 30% of the registered voters in Santa Clara County voted in the primary election. p ≤ .30 H a : More than 30% of the registered voters in Santa Clara County voted in the primary election. p > 30
A medical trial is conducted to test whether or not a new medicine reduces cholesterol by 25%. State the null and alternative hypotheses.
Example 9.2
We want to test whether the mean GPA of students in American colleges is different from 2.0 (out of 4.0). The null and alternative hypotheses are: H 0 : μ = 2.0 H a : μ ≠ 2.0
We want to test whether the mean height of eighth graders is 66 inches. State the null and alternative hypotheses. Fill in the correct symbol (=, ≠, ≥, <, ≤, >) for the null and alternative hypotheses.
- H 0 : μ __ 66
- H a : μ __ 66
Example 9.3
We want to test if college students take less than five years to graduate from college, on the average. The null and alternative hypotheses are: H 0 : μ ≥ 5 H a : μ < 5
We want to test if it takes fewer than 45 minutes to teach a lesson plan. State the null and alternative hypotheses. Fill in the correct symbol ( =, ≠, ≥, <, ≤, >) for the null and alternative hypotheses.
- H 0 : μ __ 45
- H a : μ __ 45
Example 9.4
In an issue of U. S. News and World Report , an article on school standards stated that about half of all students in France, Germany, and Israel take advanced placement exams and a third pass. The same article stated that 6.6% of U.S. students take advanced placement exams and 4.4% pass. Test if the percentage of U.S. students who take advanced placement exams is more than 6.6%. State the null and alternative hypotheses. H 0 : p ≤ 0.066 H a : p > 0.066
On a state driver’s test, about 40% pass the test on the first try. We want to test if more than 40% pass on the first try. Fill in the correct symbol (=, ≠, ≥, <, ≤, >) for the null and alternative hypotheses.
- H 0 : p __ 0.40
- H a : p __ 0.40
Collaborative Exercise
Bring to class a newspaper, some news magazines, and some Internet articles . In groups, find articles from which your group can write null and alternative hypotheses. Discuss your hypotheses with the rest of the class.
This book may not be used in the training of large language models or otherwise be ingested into large language models or generative AI offerings without OpenStax's permission.
Want to cite, share, or modify this book? This book uses the Creative Commons Attribution License and you must attribute OpenStax.
Access for free at https://openstax.org/books/introductory-statistics-2e/pages/1-introduction
- Authors: Barbara Illowsky, Susan Dean
- Publisher/website: OpenStax
- Book title: Introductory Statistics 2e
- Publication date: Dec 13, 2023
- Location: Houston, Texas
- Book URL: https://openstax.org/books/introductory-statistics-2e/pages/1-introduction
- Section URL: https://openstax.org/books/introductory-statistics-2e/pages/9-1-null-and-alternative-hypotheses
© Dec 6, 2023 OpenStax. Textbook content produced by OpenStax is licensed under a Creative Commons Attribution License . The OpenStax name, OpenStax logo, OpenStax book covers, OpenStax CNX name, and OpenStax CNX logo are not subject to the Creative Commons license and may not be reproduced without the prior and express written consent of Rice University.
The Federal Register
The daily journal of the united states government, request access.
Due to aggressive automated scraping of FederalRegister.gov and eCFR.gov, programmatic access to these sites is limited to access to our extensive developer APIs.
If you are human user receiving this message, we can add your IP address to a set of IPs that can access FederalRegister.gov & eCFR.gov; complete the CAPTCHA (bot test) below and click "Request Access". This process will be necessary for each IP address you wish to access the site from, requests are valid for approximately one quarter (three months) after which the process may need to be repeated.
An official website of the United States government.
If you want to request a wider IP range, first request access for your current IP, and then use the "Site Feedback" button found in the lower left-hand side to make the request.
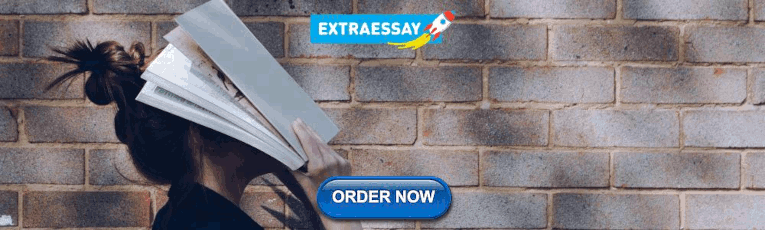
IMAGES
VIDEO
COMMENTS
The null and alternative hypotheses offer competing answers to your research question. When the research question asks "Does the independent variable affect the dependent variable?": The null hypothesis ( H0) answers "No, there's no effect in the population.". The alternative hypothesis ( Ha) answers "Yes, there is an effect in the ...
Null hypothesis: µ ≥ 70 inches. Alternative hypothesis: µ < 70 inches. A two-tailed hypothesis involves making an "equal to" or "not equal to" statement. For example, suppose we assume the mean height of a male in the U.S. is equal to 70 inches. The null and alternative hypotheses in this case would be: Null hypothesis: µ = 70 inches.
LECTURE 5 Introduction to Econometrics Hypothesis testing. October 18, 2016. 1/26. ON TODAY'S LECTURE. IWe are going to discuss how hypotheses about coefficients can be tested in regression models. IWe will explain what significance of coefficients means. IWe will learn how to read regression output.
The statement that is being tested against the null hypothesis is the alternative hypothesis. Alternative hypothesis is often denoted as H a or H 1. In statistical hypothesis testing, to prove the alternative hypothesis is true, it should be shown that the data is contradictory to the null hypothesis. Namely, there is sufficient evidence ...
The actual test begins by considering two hypotheses.They are called the null hypothesis and the alternative hypothesis.These hypotheses contain opposing viewpoints. H 0, the —null hypothesis: a statement of no difference between sample means or proportions or no difference between a sample mean or proportion and a population mean or proportion. In other words, the difference equals 0.
1. INTRODUCTION. This review highlights many current approaches to hypothesis testing in the econometrics literature. We consider the general problem of testing in the classical Neyman-Pearson framework, reviewing the key concepts in Section 2. As such, optimality is defined via the power function.
Wald = tμ=μ0 A∼ χ2(1) = χ. 1. The asymptotic t-test is different from the finite sample t-test in two re-spects: The actual size (significance level) of the asymptotic test is 5% (the nominal significance level) only as n → ∞. In finite samples, the actual size of the asymptotic test may be smaller or larger than 5%.
In this course, you will learn why it is rational to use the parameters recovered under the Classical Linear Regression Model for hypothesis testing in uncertain contexts. You will: - Develop your knowledge of the statistical properties of the OLS estimator as you see whether key assumptions work. - Learn that the OLS estimator has some ...
semiparametric or nonparametric model. A general hypothesis about the underlying model can be specified by a subset of Ω. In the classical Neyman-Pearson setup that we consider, the problem is to test the null hypothesis H0: θ ∈ Ω0 against the alternative hypothesis H1: θ ∈ Ω1. Here, Ω0 and Ω1 are disjoint subsets of Ω with union Ω.
Definition. The alternative hypothesis is a statement used in statistical inference experiment. It is contradictory to the null hypothesis and denoted by H a or H 1. We can also say that it is simply an alternative to the null. In hypothesis testing, an alternative theory is a statement which a researcher is testing.
The alternative hypothesis is a hypothesis used in significance testing which contains a strict inequality. A test of significance will result in either rejecting the null hypothesis (indicating ...
Hypothesis Testing in Econometrics. This article reviews important concepts and methods that are useful for hypothesis testing. First, we discuss the Neyman-Pearson framework. Various approaches to optimality are presented, including finite-sample and large-sample optimality. Then, we summarize some of the most important methods, as well as ...
Here are some examples of normative statements in economics: "We ought to do more to help the poor." "People in the United States should save more." "Corporate profits are too high.". The statements are based on the values of the person who makes them. They cannot be proven false.
Econometrics uses economic theory, mathematics, and statistical inference to quantify economic phenomena. In other words, it turns theoretical economic models into useful tools for economic policymaking. The objective of econometrics is to convert qualitative statements (such as "the relationship between two or more variables is positive ...
Econometrics is the application of statistical and mathematical theories in economics for the purpose of testing hypotheses and forecasting future trends. It takes economic models, tests them ...
i= g(x. ij ) where g( ) is just an arbitrary function and is some population parameter. 2.) Observe data from a sample of N observations of i = 1 ... N fy. i;x. 1i;x. 2igi= 1:::N 3.) Characterize parameters of model using some econometric method sampling estimating. 1.2 A rough taxonomy of econometric analyses 3.
the use of proxy variables to allow for uncertainties in the alternative specification. Our subsequent focus on the RESET test involves a procedure which really falls somewhere between these two categories, in that although a specific alternative hypothesis is formulated, it is largely a device to facilitate a test of a null specification.
This is a beginner's guide to applied econometrics using the free statistics software R. ... a break point specifying how the data should be split (percentage of the number of observations), what is the alternative hypothesis ("greater", "two.sided", or ... As we have already seen, the linear probability model is, by definition ...
econometric task is determining the most appropriate form of the trend in the data. For example, in ARMA modeling the data must be transformed ... against the alternative hypothesis that φ<1 (trend stationary). They are called unit root tests because under the null hypothesis the autoregressive polynomial of zt, φ(z)=(1−φz)=0,
The actual test begins by considering two hypotheses.They are called the null hypothesis and the alternative hypothesis.These hypotheses contain opposing viewpoints. H 0: The null hypothesis: It is a statement of no difference between the variables—they are not related. This can often be considered the status quo and as a result if you cannot accept the null it requires some action.
Econometric models (a.k.a. Controlled Regression) is a popular method for an Observational Study to estimate how the change of predictor variables (e.g., treatment X and other Covariates) relate to the change of response variable (Y). ... Alternative hypothesis (H1): ABC e-commerce site visitors who receive email coupons will have higher ...
The three statistics Exp-LR, Ch. 37." Empirical Process Methods in Econometrics 2261 Exp-LM, and Exp-W each have asymptotic optimality properties. Using empirical process results, each can be shown to have an asymptotic null distribution that is a function of the stochastic process X2 (z) discussed above. First, we introduce some notation.
Asymptotic theory (statistics) In statistics, asymptotic theory, or large sample theory, is a framework for assessing properties of estimators and statistical tests. Within this framework, it is often assumed that the sample size n may grow indefinitely; the properties of estimators and tests are then evaluated under the limit of n → ∞.
The National Academies of Science Engineering and Medicine (NASEM) report (Ref. 27), in their review of the Application of Systematic Review in TSCA Risk Evaluations (Ref. 28), highlights this need for alternative approaches, stating that "under some circumstances there may be reasonable alternatives to carrying out a de novo systematic ...