Have a language expert improve your writing
Run a free plagiarism check in 10 minutes, generate accurate citations for free.
- Knowledge Base
- Starting the research process
- How to Write a Research Proposal | Examples & Templates
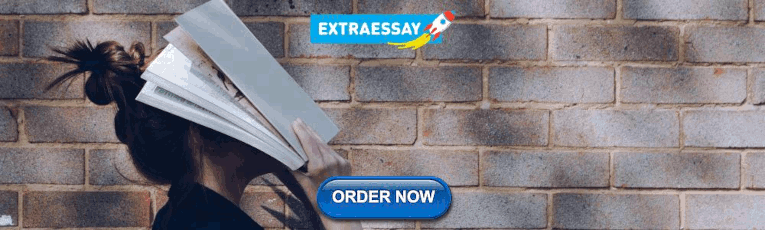
How to Write a Research Proposal | Examples & Templates
Published on October 12, 2022 by Shona McCombes and Tegan George. Revised on November 21, 2023.
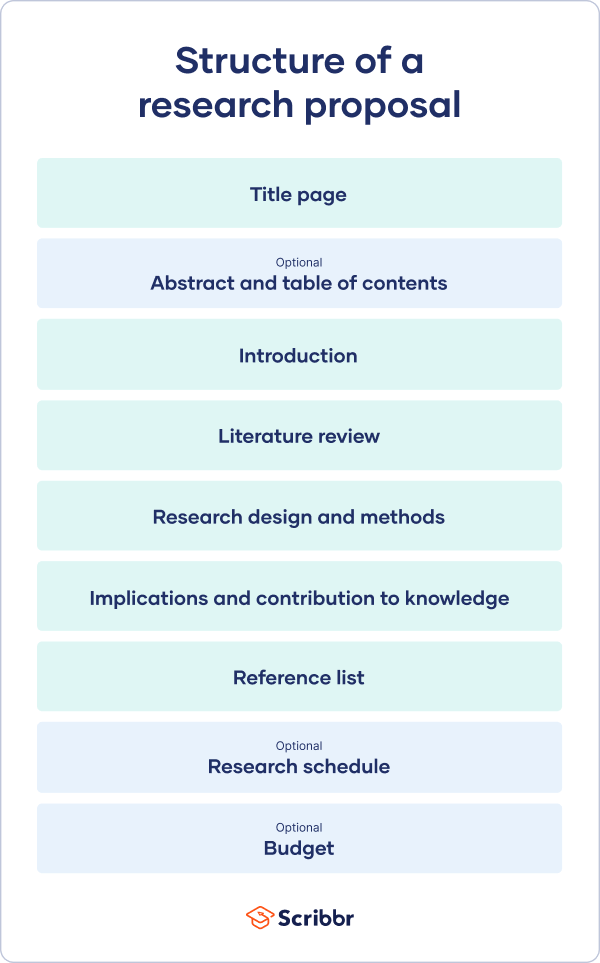
A research proposal describes what you will investigate, why it’s important, and how you will conduct your research.
The format of a research proposal varies between fields, but most proposals will contain at least these elements:
Introduction
Literature review.
- Research design
Reference list
While the sections may vary, the overall objective is always the same. A research proposal serves as a blueprint and guide for your research plan, helping you get organized and feel confident in the path forward you choose to take.
Table of contents
Research proposal purpose, research proposal examples, research design and methods, contribution to knowledge, research schedule, other interesting articles, frequently asked questions about research proposals.
Academics often have to write research proposals to get funding for their projects. As a student, you might have to write a research proposal as part of a grad school application , or prior to starting your thesis or dissertation .
In addition to helping you figure out what your research can look like, a proposal can also serve to demonstrate why your project is worth pursuing to a funder, educational institution, or supervisor.
Research proposal length
The length of a research proposal can vary quite a bit. A bachelor’s or master’s thesis proposal can be just a few pages, while proposals for PhD dissertations or research funding are usually much longer and more detailed. Your supervisor can help you determine the best length for your work.
One trick to get started is to think of your proposal’s structure as a shorter version of your thesis or dissertation , only without the results , conclusion and discussion sections.
Download our research proposal template
Receive feedback on language, structure, and formatting
Professional editors proofread and edit your paper by focusing on:
- Academic style
- Vague sentences
- Style consistency
See an example
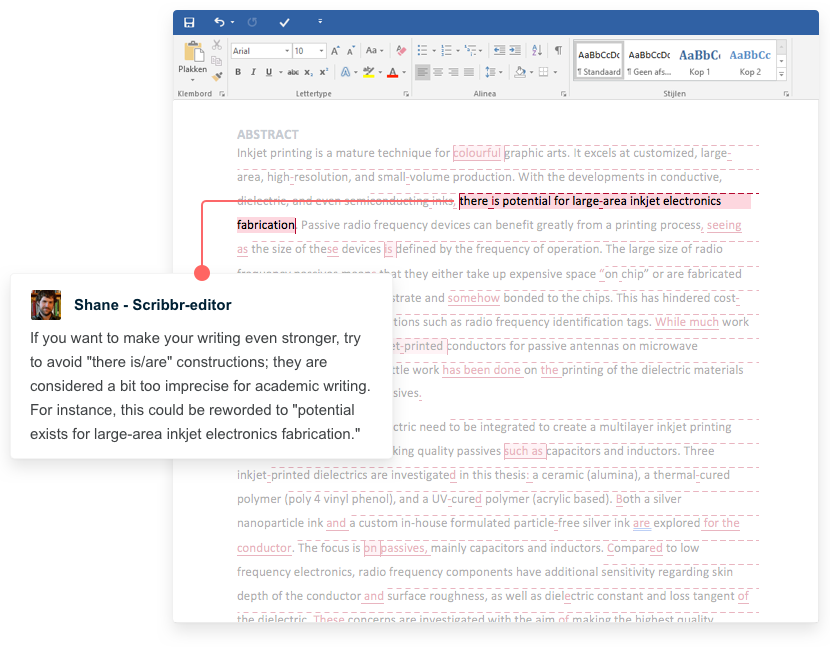
Writing a research proposal can be quite challenging, but a good starting point could be to look at some examples. We’ve included a few for you below.
- Example research proposal #1: “A Conceptual Framework for Scheduling Constraint Management”
- Example research proposal #2: “Medical Students as Mediators of Change in Tobacco Use”
Like your dissertation or thesis, the proposal will usually have a title page that includes:
- The proposed title of your project
- Your supervisor’s name
- Your institution and department
The first part of your proposal is the initial pitch for your project. Make sure it succinctly explains what you want to do and why.
Your introduction should:
- Introduce your topic
- Give necessary background and context
- Outline your problem statement and research questions
To guide your introduction , include information about:
- Who could have an interest in the topic (e.g., scientists, policymakers)
- How much is already known about the topic
- What is missing from this current knowledge
- What new insights your research will contribute
- Why you believe this research is worth doing
As you get started, it’s important to demonstrate that you’re familiar with the most important research on your topic. A strong literature review shows your reader that your project has a solid foundation in existing knowledge or theory. It also shows that you’re not simply repeating what other people have already done or said, but rather using existing research as a jumping-off point for your own.
In this section, share exactly how your project will contribute to ongoing conversations in the field by:
- Comparing and contrasting the main theories, methods, and debates
- Examining the strengths and weaknesses of different approaches
- Explaining how will you build on, challenge, or synthesize prior scholarship
Following the literature review, restate your main objectives . This brings the focus back to your own project. Next, your research design or methodology section will describe your overall approach, and the practical steps you will take to answer your research questions.
To finish your proposal on a strong note, explore the potential implications of your research for your field. Emphasize again what you aim to contribute and why it matters.
For example, your results might have implications for:
- Improving best practices
- Informing policymaking decisions
- Strengthening a theory or model
- Challenging popular or scientific beliefs
- Creating a basis for future research
Last but not least, your research proposal must include correct citations for every source you have used, compiled in a reference list . To create citations quickly and easily, you can use our free APA citation generator .
Some institutions or funders require a detailed timeline of the project, asking you to forecast what you will do at each stage and how long it may take. While not always required, be sure to check the requirements of your project.
Here’s an example schedule to help you get started. You can also download a template at the button below.
Download our research schedule template
If you are applying for research funding, chances are you will have to include a detailed budget. This shows your estimates of how much each part of your project will cost.
Make sure to check what type of costs the funding body will agree to cover. For each item, include:
- Cost : exactly how much money do you need?
- Justification : why is this cost necessary to complete the research?
- Source : how did you calculate the amount?
To determine your budget, think about:
- Travel costs : do you need to go somewhere to collect your data? How will you get there, and how much time will you need? What will you do there (e.g., interviews, archival research)?
- Materials : do you need access to any tools or technologies?
- Help : do you need to hire any research assistants for the project? What will they do, and how much will you pay them?
If you want to know more about the research process , methodology , research bias , or statistics , make sure to check out some of our other articles with explanations and examples.
Methodology
- Sampling methods
- Simple random sampling
- Stratified sampling
- Cluster sampling
- Likert scales
- Reproducibility
Statistics
- Null hypothesis
- Statistical power
- Probability distribution
- Effect size
- Poisson distribution
Research bias
- Optimism bias
- Cognitive bias
- Implicit bias
- Hawthorne effect
- Anchoring bias
- Explicit bias
Once you’ve decided on your research objectives , you need to explain them in your paper, at the end of your problem statement .
Keep your research objectives clear and concise, and use appropriate verbs to accurately convey the work that you will carry out for each one.
I will compare …
A research aim is a broad statement indicating the general purpose of your research project. It should appear in your introduction at the end of your problem statement , before your research objectives.
Research objectives are more specific than your research aim. They indicate the specific ways you’ll address the overarching aim.
A PhD, which is short for philosophiae doctor (doctor of philosophy in Latin), is the highest university degree that can be obtained. In a PhD, students spend 3–5 years writing a dissertation , which aims to make a significant, original contribution to current knowledge.
A PhD is intended to prepare students for a career as a researcher, whether that be in academia, the public sector, or the private sector.
A master’s is a 1- or 2-year graduate degree that can prepare you for a variety of careers.
All master’s involve graduate-level coursework. Some are research-intensive and intend to prepare students for further study in a PhD; these usually require their students to write a master’s thesis . Others focus on professional training for a specific career.
Critical thinking refers to the ability to evaluate information and to be aware of biases or assumptions, including your own.
Like information literacy , it involves evaluating arguments, identifying and solving problems in an objective and systematic way, and clearly communicating your ideas.
The best way to remember the difference between a research plan and a research proposal is that they have fundamentally different audiences. A research plan helps you, the researcher, organize your thoughts. On the other hand, a dissertation proposal or research proposal aims to convince others (e.g., a supervisor, a funding body, or a dissertation committee) that your research topic is relevant and worthy of being conducted.
Cite this Scribbr article
If you want to cite this source, you can copy and paste the citation or click the “Cite this Scribbr article” button to automatically add the citation to our free Citation Generator.
McCombes, S. & George, T. (2023, November 21). How to Write a Research Proposal | Examples & Templates. Scribbr. Retrieved April 15, 2024, from https://www.scribbr.com/research-process/research-proposal/
Is this article helpful?
Shona McCombes
Other students also liked, how to write a problem statement | guide & examples, writing strong research questions | criteria & examples, how to write a literature review | guide, examples, & templates, "i thought ai proofreading was useless but..".
I've been using Scribbr for years now and I know it's a service that won't disappoint. It does a good job spotting mistakes”

How to Create a Data Analysis Plan: A Detailed Guide
by Barche Blaise | Aug 12, 2020 | Writing

If a good research question equates to a story then, a roadmap will be very vita l for good storytelling. We advise every student/researcher to personally write his/her data analysis plan before seeking any advice. In this blog article, we will explore how to create a data analysis plan: the content and structure.
This data analysis plan serves as a roadmap to how data collected will be organised and analysed. It includes the following aspects:
- Clearly states the research objectives and hypothesis
- Identifies the dataset to be used
- Inclusion and exclusion criteria
- Clearly states the research variables
- States statistical test hypotheses and the software for statistical analysis
- Creating shell tables
1. Stating research question(s), objectives and hypotheses:
All research objectives or goals must be clearly stated. They must be Specific, Measurable, Attainable, Realistic and Time-bound (SMART). Hypotheses are theories obtained from personal experience or previous literature and they lay a foundation for the statistical methods that will be applied to extrapolate results to the entire population.
2. The dataset:
The dataset that will be used for statistical analysis must be described and important aspects of the dataset outlined. These include; owner of the dataset, how to get access to the dataset, how the dataset was checked for quality control and in what program is the dataset stored (Excel, Epi Info, SQL, Microsoft access etc.).
3. The inclusion and exclusion criteria :
They guide the aspects of the dataset that will be used for data analysis. These criteria will also guide the choice of variables included in the main analysis.
4. Variables:
Every variable collected in the study should be clearly stated. They should be presented based on the level of measurement (ordinal/nominal or ratio/interval levels), or the role the variable plays in the study (independent/predictors or dependent/outcome variables). The variable types should also be outlined. The variable type in conjunction with the research hypothesis forms the basis for selecting the appropriate statistical tests for inferential statistics. A good data analysis plan should summarize the variables as demonstrated in Figure 1 below.

5. Statistical software
There are tons of software packages for data analysis, some common examples are SPSS, Epi Info, SAS, STATA, Microsoft Excel. Include the version number, year of release and author/manufacturer. Beginners have the tendency to try different software and finally not master any. It is rather good to select one and master it because almost all statistical software have the same performance for basic and the majority of advance analysis needed for a student thesis. This is what we recommend to all our students at CRENC before they begin writing their results section .
6. Selecting the appropriate statistical method to test hypotheses
Depending on the research question, hypothesis and type of variable, several statistical methods can be used to answer the research question appropriately. This aspect of the data analysis plan outlines clearly why each statistical method will be used to test hypotheses. The level of statistical significance (p-value) which is often but not always <0.05 should also be written. Presented in figures 2a and 2b are decision trees for some common statistical tests based on the variable type and research question
A good analysis plan should clearly describe how missing data will be analysed.

7. Creating shell tables
Data analysis involves three levels of analysis; univariable, bivariable and multivariable analysis with increasing order of complexity. Shell tables should be created in anticipation for the results that will be obtained from these different levels of analysis. Read our blog article on how to present tables and figures for more details. Suppose you carry out a study to investigate the prevalence and associated factors of a certain disease “X” in a population, then the shell tables can be represented as in Tables 1, Table 2 and Table 3 below.
Table 1: Example of a shell table from univariate analysis

Table 2: Example of a shell table from bivariate analysis

Table 3: Example of a shell table from multivariate analysis

aOR = adjusted odds ratio
Now that you have learned how to create a data analysis plan, these are the takeaway points. It should clearly state the:
- Research question, objectives, and hypotheses
- Dataset to be used
- Variable types and their role
- Statistical software and statistical methods
- Shell tables for univariate, bivariate and multivariate analysis
Further readings
Creating a Data Analysis Plan: What to Consider When Choosing Statistics for a Study https://www.ncbi.nlm.nih.gov/pmc/articles/PMC4552232/pdf/cjhp-68-311.pdf
Creating an Analysis Plan: https://www.cdc.gov/globalhealth/healthprotection/fetp/training_modules/9/creating-analysis-plan_pw_final_09242013.pdf
Data Analysis Plan: https://www.statisticssolutions.com/dissertation-consulting-services/data-analysis-plan-2/
Photo created by freepik – www.freepik.com

Dr Barche is a physician and holds a Masters in Public Health. He is a senior fellow at CRENC with interests in Data Science and Data Analysis.
Post Navigation
16 comments.
Thanks. Quite informative.
Educative write-up. Thanks.
Easy to understand. Thanks Dr
Very explicit Dr. Thanks
I will always remember how you help me conceptualize and understand data science in a simple way. I can only hope that someday I’ll be in a position to repay you, my dear friend.
Plan d’analyse

This is interesting, Thanks
Very understandable and informative. Thank you..
love the figures.
Nice, and informative
This is so much educative and good for beginners, I would love to recommend that you create and share a video because some people are able to grasp when there is an instructor. Lots of love
Thank you Doctor very helpful.
Educative and clearly written. Thanks
Well said doctor,thank you.But when do you present in tables ,bars,pie chart etc?
Very informative guide!
Submit a Comment Cancel Reply
Your email address will not be published. Required fields are marked *
Notify me of follow-up comments by email.
Notify me of new posts by email.
Submit Comment
Receive updates on new courses and blog posts
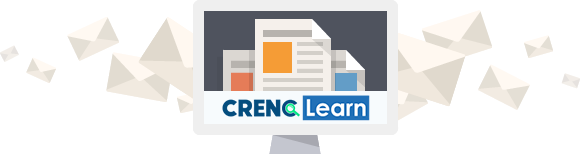
Never Miss a Thing!
Subscribe to our mailing list to receive the latest news and updates on our webinars, articles and courses.
You have Successfully Subscribed!
Have a language expert improve your writing
Run a free plagiarism check in 10 minutes, automatically generate references for free.
- Knowledge Base
- Research process
- How to Write a Research Proposal | Examples & Templates
How to Write a Research Proposal | Examples & Templates
Published on 30 October 2022 by Shona McCombes and Tegan George. Revised on 13 June 2023.
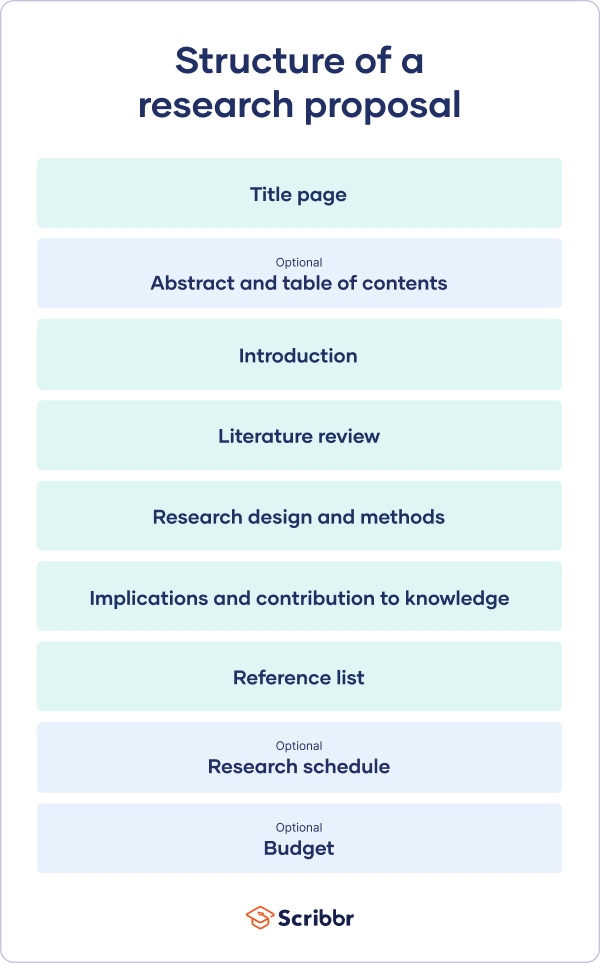
A research proposal describes what you will investigate, why it’s important, and how you will conduct your research.
The format of a research proposal varies between fields, but most proposals will contain at least these elements:
Introduction
Literature review.
- Research design
Reference list
While the sections may vary, the overall objective is always the same. A research proposal serves as a blueprint and guide for your research plan, helping you get organised and feel confident in the path forward you choose to take.
Table of contents
Research proposal purpose, research proposal examples, research design and methods, contribution to knowledge, research schedule, frequently asked questions.
Academics often have to write research proposals to get funding for their projects. As a student, you might have to write a research proposal as part of a grad school application , or prior to starting your thesis or dissertation .
In addition to helping you figure out what your research can look like, a proposal can also serve to demonstrate why your project is worth pursuing to a funder, educational institution, or supervisor.
Research proposal length
The length of a research proposal can vary quite a bit. A bachelor’s or master’s thesis proposal can be just a few pages, while proposals for PhD dissertations or research funding are usually much longer and more detailed. Your supervisor can help you determine the best length for your work.
One trick to get started is to think of your proposal’s structure as a shorter version of your thesis or dissertation , only without the results , conclusion and discussion sections.
Download our research proposal template
Prevent plagiarism, run a free check.
Writing a research proposal can be quite challenging, but a good starting point could be to look at some examples. We’ve included a few for you below.
- Example research proposal #1: ‘A Conceptual Framework for Scheduling Constraint Management’
- Example research proposal #2: ‘ Medical Students as Mediators of Change in Tobacco Use’
Like your dissertation or thesis, the proposal will usually have a title page that includes:
- The proposed title of your project
- Your supervisor’s name
- Your institution and department
The first part of your proposal is the initial pitch for your project. Make sure it succinctly explains what you want to do and why.
Your introduction should:
- Introduce your topic
- Give necessary background and context
- Outline your problem statement and research questions
To guide your introduction , include information about:
- Who could have an interest in the topic (e.g., scientists, policymakers)
- How much is already known about the topic
- What is missing from this current knowledge
- What new insights your research will contribute
- Why you believe this research is worth doing
As you get started, it’s important to demonstrate that you’re familiar with the most important research on your topic. A strong literature review shows your reader that your project has a solid foundation in existing knowledge or theory. It also shows that you’re not simply repeating what other people have already done or said, but rather using existing research as a jumping-off point for your own.
In this section, share exactly how your project will contribute to ongoing conversations in the field by:
- Comparing and contrasting the main theories, methods, and debates
- Examining the strengths and weaknesses of different approaches
- Explaining how will you build on, challenge, or synthesise prior scholarship
Following the literature review, restate your main objectives . This brings the focus back to your own project. Next, your research design or methodology section will describe your overall approach, and the practical steps you will take to answer your research questions.
To finish your proposal on a strong note, explore the potential implications of your research for your field. Emphasise again what you aim to contribute and why it matters.
For example, your results might have implications for:
- Improving best practices
- Informing policymaking decisions
- Strengthening a theory or model
- Challenging popular or scientific beliefs
- Creating a basis for future research
Last but not least, your research proposal must include correct citations for every source you have used, compiled in a reference list . To create citations quickly and easily, you can use our free APA citation generator .
Some institutions or funders require a detailed timeline of the project, asking you to forecast what you will do at each stage and how long it may take. While not always required, be sure to check the requirements of your project.
Here’s an example schedule to help you get started. You can also download a template at the button below.
Download our research schedule template
If you are applying for research funding, chances are you will have to include a detailed budget. This shows your estimates of how much each part of your project will cost.
Make sure to check what type of costs the funding body will agree to cover. For each item, include:
- Cost : exactly how much money do you need?
- Justification : why is this cost necessary to complete the research?
- Source : how did you calculate the amount?
To determine your budget, think about:
- Travel costs : do you need to go somewhere to collect your data? How will you get there, and how much time will you need? What will you do there (e.g., interviews, archival research)?
- Materials : do you need access to any tools or technologies?
- Help : do you need to hire any research assistants for the project? What will they do, and how much will you pay them?
Once you’ve decided on your research objectives , you need to explain them in your paper, at the end of your problem statement.
Keep your research objectives clear and concise, and use appropriate verbs to accurately convey the work that you will carry out for each one.
I will compare …
A research aim is a broad statement indicating the general purpose of your research project. It should appear in your introduction at the end of your problem statement , before your research objectives.
Research objectives are more specific than your research aim. They indicate the specific ways you’ll address the overarching aim.
A PhD, which is short for philosophiae doctor (doctor of philosophy in Latin), is the highest university degree that can be obtained. In a PhD, students spend 3–5 years writing a dissertation , which aims to make a significant, original contribution to current knowledge.
A PhD is intended to prepare students for a career as a researcher, whether that be in academia, the public sector, or the private sector.
A master’s is a 1- or 2-year graduate degree that can prepare you for a variety of careers.
All master’s involve graduate-level coursework. Some are research-intensive and intend to prepare students for further study in a PhD; these usually require their students to write a master’s thesis . Others focus on professional training for a specific career.
Critical thinking refers to the ability to evaluate information and to be aware of biases or assumptions, including your own.
Like information literacy , it involves evaluating arguments, identifying and solving problems in an objective and systematic way, and clearly communicating your ideas.
Cite this Scribbr article
If you want to cite this source, you can copy and paste the citation or click the ‘Cite this Scribbr article’ button to automatically add the citation to our free Reference Generator.
McCombes, S. & George, T. (2023, June 13). How to Write a Research Proposal | Examples & Templates. Scribbr. Retrieved 15 April 2024, from https://www.scribbr.co.uk/the-research-process/research-proposal-explained/
Is this article helpful?
Shona McCombes
Other students also liked, what is a research methodology | steps & tips, what is a literature review | guide, template, & examples, how to write a results section | tips & examples.

What (Exactly) Is A Research Proposal?
A simple explainer with examples + free template.
By: Derek Jansen (MBA) | Reviewed By: Dr Eunice Rautenbach | June 2020 (Updated April 2023)
Whether you’re nearing the end of your degree and your dissertation is on the horizon, or you’re planning to apply for a PhD program, chances are you’ll need to craft a convincing research proposal . If you’re on this page, you’re probably unsure exactly what the research proposal is all about. Well, you’ve come to the right place.
Overview: Research Proposal Basics
- What a research proposal is
- What a research proposal needs to cover
- How to structure your research proposal
- Example /sample proposals
- Proposal writing FAQs
- Key takeaways & additional resources
What is a research proposal?
Simply put, a research proposal is a structured, formal document that explains what you plan to research (your research topic), why it’s worth researching (your justification), and how you plan to investigate it (your methodology).
The purpose of the research proposal (its job, so to speak) is to convince your research supervisor, committee or university that your research is suitable (for the requirements of the degree program) and manageable (given the time and resource constraints you will face).
The most important word here is “ convince ” – in other words, your research proposal needs to sell your research idea (to whoever is going to approve it). If it doesn’t convince them (of its suitability and manageability), you’ll need to revise and resubmit . This will cost you valuable time, which will either delay the start of your research or eat into its time allowance (which is bad news).
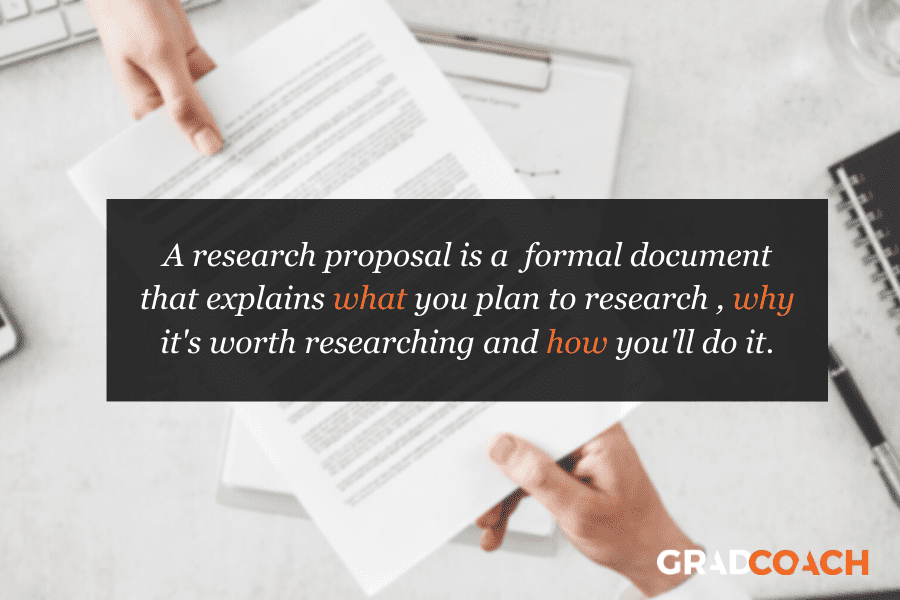
What goes into a research proposal?
A good dissertation or thesis proposal needs to cover the “ what “, “ why ” and” how ” of the proposed study. Let’s look at each of these attributes in a little more detail:
Your proposal needs to clearly articulate your research topic . This needs to be specific and unambiguous . Your research topic should make it clear exactly what you plan to research and in what context. Here’s an example of a well-articulated research topic:
An investigation into the factors which impact female Generation Y consumer’s likelihood to promote a specific makeup brand to their peers: a British context
As you can see, this topic is extremely clear. From this one line we can see exactly:
- What’s being investigated – factors that make people promote or advocate for a brand of a specific makeup brand
- Who it involves – female Gen-Y consumers
- In what context – the United Kingdom
So, make sure that your research proposal provides a detailed explanation of your research topic . If possible, also briefly outline your research aims and objectives , and perhaps even your research questions (although in some cases you’ll only develop these at a later stage). Needless to say, don’t start writing your proposal until you have a clear topic in mind , or you’ll end up waffling and your research proposal will suffer as a result of this.
Need a helping hand?
As we touched on earlier, it’s not good enough to simply propose a research topic – you need to justify why your topic is original . In other words, what makes it unique ? What gap in the current literature does it fill? If it’s simply a rehash of the existing research, it’s probably not going to get approval – it needs to be fresh.
But, originality alone is not enough. Once you’ve ticked that box, you also need to justify why your proposed topic is important . In other words, what value will it add to the world if you achieve your research aims?
As an example, let’s look at the sample research topic we mentioned earlier (factors impacting brand advocacy). In this case, if the research could uncover relevant factors, these findings would be very useful to marketers in the cosmetics industry, and would, therefore, have commercial value . That is a clear justification for the research.
So, when you’re crafting your research proposal, remember that it’s not enough for a topic to simply be unique. It needs to be useful and value-creating – and you need to convey that value in your proposal. If you’re struggling to find a research topic that makes the cut, watch our video covering how to find a research topic .
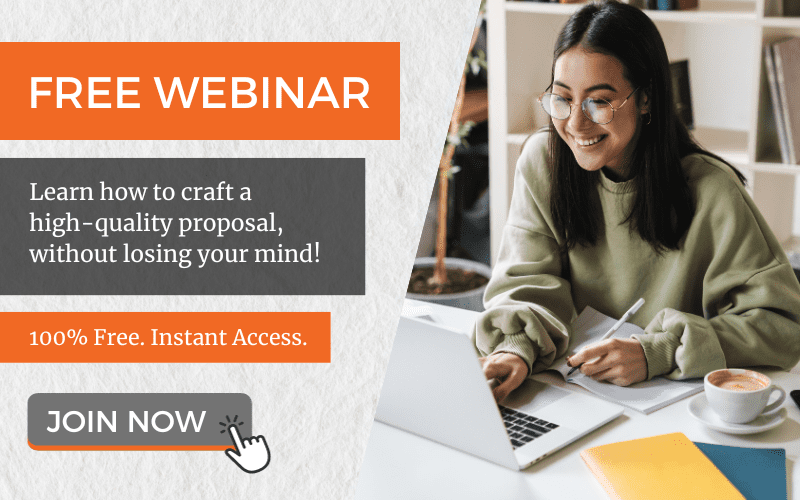
It’s all good and well to have a great topic that’s original and valuable, but you’re not going to convince anyone to approve it without discussing the practicalities – in other words:
- How will you actually undertake your research (i.e., your methodology)?
- Is your research methodology appropriate given your research aims?
- Is your approach manageable given your constraints (time, money, etc.)?
While it’s generally not expected that you’ll have a fully fleshed-out methodology at the proposal stage, you’ll likely still need to provide a high-level overview of your research methodology . Here are some important questions you’ll need to address in your research proposal:
- Will you take a qualitative , quantitative or mixed -method approach?
- What sampling strategy will you adopt?
- How will you collect your data (e.g., interviews, surveys, etc)?
- How will you analyse your data (e.g., descriptive and inferential statistics , content analysis, discourse analysis, etc, .)?
- What potential limitations will your methodology carry?
So, be sure to give some thought to the practicalities of your research and have at least a basic methodological plan before you start writing up your proposal. If this all sounds rather intimidating, the video below provides a good introduction to research methodology and the key choices you’ll need to make.
How To Structure A Research Proposal
Now that we’ve covered the key points that need to be addressed in a proposal, you may be wondering, “ But how is a research proposal structured? “.
While the exact structure and format required for a research proposal differs from university to university, there are four “essential ingredients” that commonly make up the structure of a research proposal:
- A rich introduction and background to the proposed research
- An initial literature review covering the existing research
- An overview of the proposed research methodology
- A discussion regarding the practicalities (project plans, timelines, etc.)
In the video below, we unpack each of these four sections, step by step.
Research Proposal Examples/Samples
In the video below, we provide a detailed walkthrough of two successful research proposals (Master’s and PhD-level), as well as our popular free proposal template.
Proposal Writing FAQs
How long should a research proposal be.
This varies tremendously, depending on the university, the field of study (e.g., social sciences vs natural sciences), and the level of the degree (e.g. undergraduate, Masters or PhD) – so it’s always best to check with your university what their specific requirements are before you start planning your proposal.
As a rough guide, a formal research proposal at Masters-level often ranges between 2000-3000 words, while a PhD-level proposal can be far more detailed, ranging from 5000-8000 words. In some cases, a rough outline of the topic is all that’s needed, while in other cases, universities expect a very detailed proposal that essentially forms the first three chapters of the dissertation or thesis.
The takeaway – be sure to check with your institution before you start writing.
How do I choose a topic for my research proposal?
Finding a good research topic is a process that involves multiple steps. We cover the topic ideation process in this video post.
How do I write a literature review for my proposal?
While you typically won’t need a comprehensive literature review at the proposal stage, you still need to demonstrate that you’re familiar with the key literature and are able to synthesise it. We explain the literature review process here.
How do I create a timeline and budget for my proposal?
We explain how to craft a project plan/timeline and budget in Research Proposal Bootcamp .
Which referencing format should I use in my research proposal?
The expectations and requirements regarding formatting and referencing vary from institution to institution. Therefore, you’ll need to check this information with your university.
What common proposal writing mistakes do I need to look out for?
We’ve create a video post about some of the most common mistakes students make when writing a proposal – you can access that here . If you’re short on time, here’s a quick summary:
- The research topic is too broad (or just poorly articulated).
- The research aims, objectives and questions don’t align.
- The research topic is not well justified.
- The study has a weak theoretical foundation.
- The research design is not well articulated well enough.
- Poor writing and sloppy presentation.
- Poor project planning and risk management.
- Not following the university’s specific criteria.
Key Takeaways & Additional Resources
As you write up your research proposal, remember the all-important core purpose: to convince . Your research proposal needs to sell your study in terms of suitability and viability. So, focus on crafting a convincing narrative to ensure a strong proposal.
At the same time, pay close attention to your university’s requirements. While we’ve covered the essentials here, every institution has its own set of expectations and it’s essential that you follow these to maximise your chances of approval.
By the way, we’ve got plenty more resources to help you fast-track your research proposal. Here are some of our most popular resources to get you started:
- Proposal Writing 101 : A Introductory Webinar
- Research Proposal Bootcamp : The Ultimate Online Course
- Template : A basic template to help you craft your proposal
If you’re looking for 1-on-1 support with your research proposal, be sure to check out our private coaching service , where we hold your hand through the proposal development process (and the entire research journey), step by step.
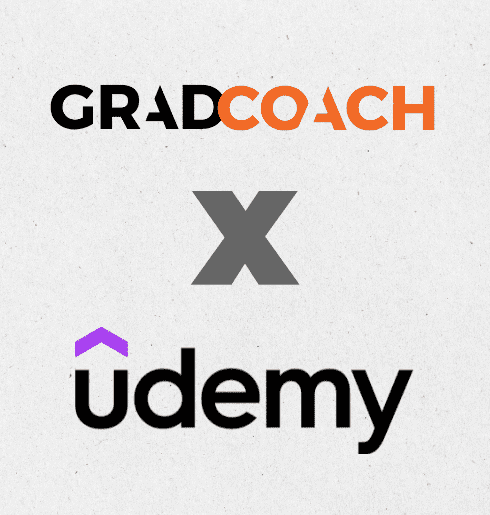
Psst… there’s more!
This post is an extract from our bestselling Udemy Course, Research Proposal Bootcamp . If you want to work smart, you don't want to miss this .
You Might Also Like:
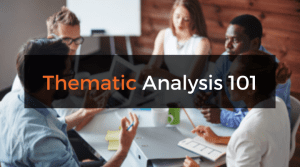
51 Comments
I truly enjoyed this video, as it was eye-opening to what I have to do in the preparation of preparing a Research proposal.
I would be interested in getting some coaching.
I real appreciate on your elaboration on how to develop research proposal,the video explains each steps clearly.
Thank you for the video. It really assisted me and my niece. I am a PhD candidate and she is an undergraduate student. It is at times, very difficult to guide a family member but with this video, my job is done.
In view of the above, I welcome more coaching.
Wonderful guidelines, thanks
This is very helpful. Would love to continue even as I prepare for starting my masters next year.
Thanks for the work done, the text was helpful to me
Bundle of thanks to you for the research proposal guide it was really good and useful if it is possible please send me the sample of research proposal
You’re most welcome. We don’t have any research proposals that we can share (the students own the intellectual property), but you might find our research proposal template useful: https://gradcoach.com/research-proposal-template/
Cheruiyot Moses Kipyegon
Thanks alot. It was an eye opener that came timely enough before my imminent proposal defense. Thanks, again
thank you very much your lesson is very interested may God be with you
I am an undergraduate student (First Degree) preparing to write my project,this video and explanation had shed more light to me thanks for your efforts keep it up.
Very useful. I am grateful.
this is a very a good guidance on research proposal, for sure i have learnt something
Wonderful guidelines for writing a research proposal, I am a student of m.phil( education), this guideline is suitable for me. Thanks
You’re welcome 🙂
Thank you, this was so helpful.
A really great and insightful video. It opened my eyes as to how to write a research paper. I would like to receive more guidance for writing my research paper from your esteemed faculty.
Thank you, great insights
Thank you, great insights, thank you so much, feeling edified
Wow thank you, great insights, thanks a lot
Thank you. This is a great insight. I am a student preparing for a PhD program. I am requested to write my Research Proposal as part of what I am required to submit before my unconditional admission. I am grateful having listened to this video which will go a long way in helping me to actually choose a topic of interest and not just any topic as well as to narrow down the topic and be specific about it. I indeed need more of this especially as am trying to choose a topic suitable for a DBA am about embarking on. Thank you once more. The video is indeed helpful.
Have learnt a lot just at the right time. Thank you so much.
thank you very much ,because have learn a lot things concerning research proposal and be blessed u for your time that you providing to help us
Hi. For my MSc medical education research, please evaluate this topic for me: Training Needs Assessment of Faculty in Medical Training Institutions in Kericho and Bomet Counties
I have really learnt a lot based on research proposal and it’s formulation
Thank you. I learn much from the proposal since it is applied
Your effort is much appreciated – you have good articulation.
You have good articulation.
I do applaud your simplified method of explaining the subject matter, which indeed has broaden my understanding of the subject matter. Definitely this would enable me writing a sellable research proposal.
This really helping
Great! I liked your tutoring on how to find a research topic and how to write a research proposal. Precise and concise. Thank you very much. Will certainly share this with my students. Research made simple indeed.
Thank you very much. I an now assist my students effectively.
Thank you very much. I can now assist my students effectively.
I need any research proposal
Thank you for these videos. I will need chapter by chapter assistance in writing my MSc dissertation
Very helpfull
the videos are very good and straight forward
thanks so much for this wonderful presentations, i really enjoyed it to the fullest wish to learn more from you
Thank you very much. I learned a lot from your lecture.
I really enjoy the in-depth knowledge on research proposal you have given. me. You have indeed broaden my understanding and skills. Thank you
interesting session this has equipped me with knowledge as i head for exams in an hour’s time, am sure i get A++
This article was most informative and easy to understand. I now have a good idea of how to write my research proposal.
Thank you very much.
Wow, this literature is very resourceful and interesting to read. I enjoyed it and I intend reading it every now then.
Thank you for the clarity
Thank you. Very helpful.
Thank you very much for this essential piece. I need 1o1 coaching, unfortunately, your service is not available in my country. Anyways, a very important eye-opener. I really enjoyed it. A thumb up to Gradcoach
What is JAM? Please explain.
Thank you so much for these videos. They are extremely helpful! God bless!
very very wonderful…
thank you for the video but i need a written example
Submit a Comment Cancel reply
Your email address will not be published. Required fields are marked *
Save my name, email, and website in this browser for the next time I comment.
- Print Friendly
How to write a research plan: Step-by-step guide
Last updated
30 January 2024
Reviewed by
Today’s businesses and institutions rely on data and analytics to inform their product and service decisions. These metrics influence how organizations stay competitive and inspire innovation. However, gathering data and insights requires carefully constructed research, and every research project needs a roadmap. This is where a research plan comes into play.
There’s general research planning; then there’s an official, well-executed research plan. Whatever data-driven research project you’re gearing up for, the research plan will be your framework for execution. The plan should also be detailed and thorough, with a diligent set of criteria to formulate your research efforts. Not including these key elements in your plan can be just as harmful as having no plan at all.
Read this step-by-step guide for writing a detailed research plan that can apply to any project, whether it’s scientific, educational, or business-related.
- What is a research plan?
A research plan is a documented overview of a project in its entirety, from end to end. It details the research efforts, participants, and methods needed, along with any anticipated results. It also outlines the project’s goals and mission, creating layers of steps to achieve those goals within a specified timeline.
Without a research plan, you and your team are flying blind, potentially wasting time and resources to pursue research without structured guidance.
The principal investigator, or PI, is responsible for facilitating the research oversight. They will create the research plan and inform team members and stakeholders of every detail relating to the project. The PI will also use the research plan to inform decision-making throughout the project.
- Why do you need a research plan?
Create a research plan before starting any official research to maximize every effort in pursuing and collecting the research data. Crucially, the plan will model the activities needed at each phase of the research project.
Like any roadmap, a research plan serves as a valuable tool providing direction for those involved in the project—both internally and externally. It will keep you and your immediate team organized and task-focused while also providing necessary definitions and timelines so you can execute your project initiatives with full understanding and transparency.
External stakeholders appreciate a working research plan because it’s a great communication tool, documenting progress and changing dynamics as they arise. Any participants of your planned research sessions will be informed about the purpose of your study, while the exercises will be based on the key messaging outlined in the official plan.
Here are some of the benefits of creating a research plan document for every project:
Project organization and structure
Well-informed participants
All stakeholders and teams align in support of the project
Clearly defined project definitions and purposes
Distractions are eliminated, prioritizing task focus
Timely management of individual task schedules and roles
Costly reworks are avoided
- What should a research plan include?
The different aspects of your research plan will depend on the nature of the project. However, most official research plan documents will include the core elements below. Each aims to define the problem statement, devising an official plan for seeking a solution.
Specific project goals and individual objectives
Ideal strategies or methods for reaching those goals
Required resources
Descriptions of the target audience, sample sizes, demographics, and scopes
Key performance indicators (KPIs)
Project background
Research and testing support
Preliminary studies and progress reporting mechanisms
Cost estimates and change order processes
Depending on the research project’s size and scope, your research plan could be brief—perhaps only a few pages of documented plans. Alternatively, it could be a fully comprehensive report. Either way, it’s an essential first step in dictating your project’s facilitation in the most efficient and effective way.
- How to write a research plan for your project
When you start writing your research plan, aim to be detailed about each step, requirement, and idea. The more time you spend curating your research plan, the more precise your research execution efforts will be.
Account for every potential scenario, and be sure to address each and every aspect of the research.
Consider following this flow to develop a great research plan for your project:
Define your project’s purpose
Start by defining your project’s purpose. Identify what your project aims to accomplish and what you are researching. Remember to use clear language.
Thinking about the project’s purpose will help you set realistic goals and inform how you divide tasks and assign responsibilities. These individual tasks will be your stepping stones to reach your overarching goal.
Additionally, you’ll want to identify the specific problem, the usability metrics needed, and the intended solutions.
Know the following three things about your project’s purpose before you outline anything else:
What you’re doing
Why you’re doing it
What you expect from it
Identify individual objectives
With your overarching project objectives in place, you can identify any individual goals or steps needed to reach those objectives. Break them down into phases or steps. You can work backward from the project goal and identify every process required to facilitate it.
Be mindful to identify each unique task so that you can assign responsibilities to various team members. At this point in your research plan development, you’ll also want to assign priority to those smaller, more manageable steps and phases that require more immediate or dedicated attention.
Select research methods
Research methods might include any of the following:
User interviews: this is a qualitative research method where researchers engage with participants in one-on-one or group conversations. The aim is to gather insights into their experiences, preferences, and opinions to uncover patterns, trends, and data.
Field studies: this approach allows for a contextual understanding of behaviors, interactions, and processes in real-world settings. It involves the researcher immersing themselves in the field, conducting observations, interviews, or experiments to gather in-depth insights.
Card sorting: participants categorize information by sorting content cards into groups based on their perceived similarities. You might use this process to gain insights into participants’ mental models and preferences when navigating or organizing information on websites, apps, or other systems.
Focus groups: use organized discussions among select groups of participants to provide relevant views and experiences about a particular topic.
Diary studies: ask participants to record their experiences, thoughts, and activities in a diary over a specified period. This method provides a deeper understanding of user experiences, uncovers patterns, and identifies areas for improvement.
Five-second testing: participants are shown a design, such as a web page or interface, for just five seconds. They then answer questions about their initial impressions and recall, allowing you to evaluate the design’s effectiveness.
Surveys: get feedback from participant groups with structured surveys. You can use online forms, telephone interviews, or paper questionnaires to reveal trends, patterns, and correlations.
Tree testing: tree testing involves researching web assets through the lens of findability and navigability. Participants are given a textual representation of the site’s hierarchy (the “tree”) and asked to locate specific information or complete tasks by selecting paths.
Usability testing: ask participants to interact with a product, website, or application to evaluate its ease of use. This method enables you to uncover areas for improvement in digital key feature functionality by observing participants using the product.
Live website testing: research and collect analytics that outlines the design, usability, and performance efficiencies of a website in real time.
There are no limits to the number of research methods you could use within your project. Just make sure your research methods help you determine the following:
What do you plan to do with the research findings?
What decisions will this research inform? How can your stakeholders leverage the research data and results?
Recruit participants and allocate tasks
Next, identify the participants needed to complete the research and the resources required to complete the tasks. Different people will be proficient at different tasks, and having a task allocation plan will allow everything to run smoothly.
Prepare a thorough project summary
Every well-designed research plan will feature a project summary. This official summary will guide your research alongside its communications or messaging. You’ll use the summary while recruiting participants and during stakeholder meetings. It can also be useful when conducting field studies.
Ensure this summary includes all the elements of your research project. Separate the steps into an easily explainable piece of text that includes the following:
An introduction: the message you’ll deliver to participants about the interview, pre-planned questioning, and testing tasks.
Interview questions: prepare questions you intend to ask participants as part of your research study, guiding the sessions from start to finish.
An exit message: draft messaging your teams will use to conclude testing or survey sessions. These should include the next steps and express gratitude for the participant’s time.
Create a realistic timeline
While your project might already have a deadline or a results timeline in place, you’ll need to consider the time needed to execute it effectively.
Realistically outline the time needed to properly execute each supporting phase of research and implementation. And, as you evaluate the necessary schedules, be sure to include additional time for achieving each milestone in case any changes or unexpected delays arise.
For this part of your research plan, you might find it helpful to create visuals to ensure your research team and stakeholders fully understand the information.
Determine how to present your results
A research plan must also describe how you intend to present your results. Depending on the nature of your project and its goals, you might dedicate one team member (the PI) or assume responsibility for communicating the findings yourself.
In this part of the research plan, you’ll articulate how you’ll share the results. Detail any materials you’ll use, such as:
Presentations and slides
A project report booklet
A project findings pamphlet
Documents with key takeaways and statistics
Graphic visuals to support your findings
- Format your research plan
As you create your research plan, you can enjoy a little creative freedom. A plan can assume many forms, so format it how you see fit. Determine the best layout based on your specific project, intended communications, and the preferences of your teams and stakeholders.
Find format inspiration among the following layouts:
Written outlines
Narrative storytelling
Visual mapping
Graphic timelines
Remember, the research plan format you choose will be subject to change and adaptation as your research and findings unfold. However, your final format should ideally outline questions, problems, opportunities, and expectations.
- Research plan example
Imagine you’ve been tasked with finding out how to get more customers to order takeout from an online food delivery platform. The goal is to improve satisfaction and retain existing customers. You set out to discover why more people aren’t ordering and what it is they do want to order or experience.
You identify the need for a research project that helps you understand what drives customer loyalty. But before you jump in and start calling past customers, you need to develop a research plan—the roadmap that provides focus, clarity, and realistic details to the project.
Here’s an example outline of a research plan you might put together:
Project title
Project members involved in the research plan
Purpose of the project (provide a summary of the research plan’s intent)
Objective 1 (provide a short description for each objective)
Objective 2
Objective 3
Proposed timeline
Audience (detail the group you want to research, such as customers or non-customers)
Budget (how much you think it might cost to do the research)
Risk factors/contingencies (any potential risk factors that may impact the project’s success)
Remember, your research plan doesn’t have to reinvent the wheel—it just needs to fit your project’s unique needs and aims.
Customizing a research plan template
Some companies offer research plan templates to help get you started. However, it may make more sense to develop your own customized plan template. Be sure to include the core elements of a great research plan with your template layout, including the following:
Introductions to participants and stakeholders
Background problems and needs statement
Significance, ethics, and purpose
Research methods, questions, and designs
Preliminary beliefs and expectations
Implications and intended outcomes
Realistic timelines for each phase
Conclusion and presentations
How many pages should a research plan be?
Generally, a research plan can vary in length between 500 to 1,500 words. This is roughly three pages of content. More substantial projects will be 2,000 to 3,500 words, taking up four to seven pages of planning documents.
What is the difference between a research plan and a research proposal?
A research plan is a roadmap to success for research teams. A research proposal, on the other hand, is a dissertation aimed at convincing or earning the support of others. Both are relevant in creating a guide to follow to complete a project goal.
What are the seven steps to developing a research plan?
While each research project is different, it’s best to follow these seven general steps to create your research plan:
Defining the problem
Identifying goals
Choosing research methods
Recruiting participants
Preparing the brief or summary
Establishing task timelines
Defining how you will present the findings
Get started today
Go from raw data to valuable insights with a flexible research platform
Editor’s picks
Last updated: 21 December 2023
Last updated: 16 December 2023
Last updated: 6 October 2023
Last updated: 5 March 2024
Last updated: 25 November 2023
Last updated: 15 February 2024
Last updated: 11 March 2024
Last updated: 12 December 2023
Last updated: 6 March 2024
Last updated: 10 April 2023
Last updated: 20 December 2023
Latest articles
Related topics, log in or sign up.
Get started for free
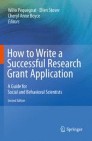
How to Write a Successful Research Grant Application pp 283–298 Cite as
Writing the Data Analysis Plan
- A. T. Panter 4
- First Online: 01 January 2010
5771 Accesses
3 Altmetric
You and your project statistician have one major goal for your data analysis plan: You need to convince all the reviewers reading your proposal that you would know what to do with your data once your project is funded and your data are in hand. The data analytic plan is a signal to the reviewers about your ability to score, describe, and thoughtfully synthesize a large number of variables into appropriately-selected quantitative models once the data are collected. Reviewers respond very well to plans with a clear elucidation of the data analysis steps – in an appropriate order, with an appropriate level of detail and reference to relevant literatures, and with statistical models and methods for that map well into your proposed aims. A successful data analysis plan produces reviews that either include no comments about the data analysis plan or better yet, compliments it for being comprehensive and logical given your aims. This chapter offers practical advice about developing and writing a compelling, “bullet-proof” data analytic plan for your grant application.
This is a preview of subscription content, log in via an institution .
Buying options
- Available as PDF
- Read on any device
- Instant download
- Own it forever
- Available as EPUB and PDF
- Compact, lightweight edition
- Dispatched in 3 to 5 business days
- Free shipping worldwide - see info
Tax calculation will be finalised at checkout
Purchases are for personal use only
Aiken, L. S. & West, S. G. (1991). Multiple regression: testing and interpreting interactions . Newbury Park, CA: Sage.
Google Scholar
Aiken, L. S., West, S. G., & Millsap, R. E. (2008). Doctoral training in statistics, measurement, and methodology in psychology: Replication and extension of Aiken, West, Sechrest and Reno’s (1990) survey of PhD programs in North America. American Psychologist , 63 , 32–50.
Article PubMed Google Scholar
Allison, P. D. (2003). Missing data techniques for structural equation modeling. Journal of Abnormal Psychology , 112 , 545–557.
American Psychological Association (APA) Task Force to Increase the Quantitative Pipeline (2009). Report of the task force to increase the quantitative pipeline . Washington, DC: American Psychological Association.
Bauer, D. & Curran, P. J. (2004). The integration of continuous and discrete latent variables: Potential problems and promising opportunities. Psychological Methods , 9 , 3–29.
Bollen, K. A. (1989). Structural equations with latent variables . New York: Wiley.
Bollen, K. A. & Curran, P. J. (2007). Latent curve models: A structural equation modeling approach . New York: Wiley.
Cohen, J., Cohen, P., West, S. G., & Aiken, L. S. (2003). Multiple correlation/regression for the behavioral sciences (3rd ed.). Mahwah, NJ: Erlbaum.
Curran, P. J., Bauer, D. J., & Willoughby, M. T. (2004). Testing main effects and interactions in hierarchical linear growth models. Psychological Methods , 9 , 220–237.
Embretson, S. E. & Reise, S. P. (2000). Item response theory for psychologists . Mahwah, NJ: Erlbaum.
Enders, C. K. (2006). Analyzing structural equation models with missing data. In G. R. Hancock & R. O. Mueller (Eds.), Structural equation modeling: A second course (pp. 313–342). Greenwich, CT: Information Age.
Hosmer, D. & Lemeshow, S. (1989). Applied logistic regression . New York: Wiley.
Hoyle, R. H. & Panter, A. T. (1995). Writing about structural equation models. In R. H. Hoyle (Ed.), Structural equation modeling: Concepts, issues, and applications (pp. 158–176). Thousand Oaks: Sage.
Kaplan, D. & Elliott, P. R. (1997). A didactic example of multilevel structural equation modeling applicable to the study of organizations. Structural Equation Modeling , 4 , 1–23.
Article Google Scholar
Lanza, S. T., Collins, L. M., Schafer, J. L., & Flaherty, B. P. (2005). Using data augmentation to obtain standard errors and conduct hypothesis tests in latent class and latent transition analysis. Psychological Methods , 10 , 84–100.
MacKinnon, D. P. (2008). Introduction to statistical mediation analysis . Mahwah, NJ: Erlbaum.
Maxwell, S. E. (2004). The persistence of underpowered studies in psychological research: Causes, consequences, and remedies. Psychological Methods , 9 , 147–163.
McCullagh, P. & Nelder, J. (1989). Generalized linear models . London: Chapman and Hall.
McDonald, R. P. & Ho, M. R. (2002). Principles and practices in reporting structural equation modeling analyses. Psychological Methods , 7 , 64–82.
Messick, S. (1989). Validity. In R. L. Linn (Ed.), Educational measurement (3rd ed., pp. 13–103). New York: Macmillan.
Muthén, B. O. (1994). Multilevel covariance structure analysis. Sociological Methods & Research , 22 , 376–398.
Muthén, B. (2008). Latent variable hybrids: overview of old and new models. In G. R. Hancock & K. M. Samuelsen (Eds.), Advances in latent variable mixture models (pp. 1–24). Charlotte, NC: Information Age.
Muthén, B. & Masyn, K. (2004). Discrete-time survival mixture analysis. Journal of Educational and Behavioral Statistics , 30 , 27–58.
Muthén, L. K. & Muthén, B. O. (2004). Mplus, statistical analysis with latent variables: User’s guide . Los Angeles, CA: Muthén &Muthén.
Peugh, J. L. & Enders, C. K. (2004). Missing data in educational research: a review of reporting practices and suggestions for improvement. Review of Educational Research , 74 , 525–556.
Preacher, K. J., Curran, P. J., & Bauer, D. J. (2006). Computational tools for probing interaction effects in multiple linear regression, multilevel modeling, and latent curve analysis. Journal of Educational and Behavioral Statistics , 31 , 437–448.
Preacher, K. J., Curran, P. J., & Bauer, D. J. (2003, September). Probing interactions in multiple linear regression, latent curve analysis, and hierarchical linear modeling: Interactive calculation tools for establishing simple intercepts, simple slopes, and regions of significance [Computer software]. Available from http://www.quantpsy.org .
Preacher, K. J., Rucker, D. D., & Hayes, A. F. (2007). Addressing moderated mediation hypotheses: Theory, methods, and prescriptions. Multivariate Behavioral Research , 42 , 185–227.
Raudenbush, S. W. & Bryk, A. S. (2002). Hierarchical linear models: Applications and data analysis methods (2nd ed.). Thousand Oaks, CA: Sage.
Radloff, L. (1977). The CES-D scale: A self-report depression scale for research in the general population. Applied Psychological Measurement , 1 , 385–401.
Rosenberg, M. (1965). Society and the adolescent self-image . Princeton, NJ: Princeton University Press.
Schafer. J. L. & Graham, J. W. (2002). Missing data: Our view of the state of the art. Psychological Methods , 7 , 147–177.
Schumacker, R. E. (2002). Latent variable interaction modeling. Structural Equation Modeling , 9 , 40–54.
Schumacker, R. E. & Lomax, R. G. (2004). A beginner’s guide to structural equation modeling . Mahwah, NJ: Erlbaum.
Selig, J. P. & Preacher, K. J. (2008, June). Monte Carlo method for assessing mediation: An interactive tool for creating confidence intervals for indirect effects [Computer software]. Available from http://www.quantpsy.org .
Singer, J. D. & Willett, J. B. (1991). Modeling the days of our lives: Using survival analysis when designing and analyzing longitudinal studies of duration and the timing of events. Psychological Bulletin , 110 , 268–290.
Singer, J. D. & Willett, J. B. (1993). It’s about time: Using discrete-time survival analysis to study duration and the timing of events. Journal of Educational Statistics , 18 , 155–195.
Singer, J. D. & Willett, J. B. (2003). Applied longitudinal data analysis: Modeling change and event occurrence . New York: Oxford University.
Book Google Scholar
Vandenberg, R. J. & Lance, C. E. (2000). A review and synthesis of the measurement invariance literature: Suggestions, practices, and recommendations for organizational research. Organizational Research Methods , 3 , 4–69.
Wirth, R. J. & Edwards, M. C. (2007). Item factor analysis: Current approaches and future directions. Psychological Methods , 12 , 58–79.
Article PubMed CAS Google Scholar
Download references
Author information
Authors and affiliations.
L. L. Thurstone Psychometric Laboratory, Department of Psychology, University of North Carolina, Chapel Hill, NC, USA
A. T. Panter
You can also search for this author in PubMed Google Scholar
Corresponding author
Correspondence to A. T. Panter .
Editor information
Editors and affiliations.
National Institute of Mental Health, Executive Blvd. 6001, Bethesda, 20892-9641, Maryland, USA
Willo Pequegnat
Ellen Stover
Delafield Place, N.W. 1413, Washington, 20011, District of Columbia, USA
Cheryl Anne Boyce
Rights and permissions
Reprints and permissions
Copyright information
© 2010 Springer Science+Business Media, LLC
About this chapter
Cite this chapter.
Panter, A.T. (2010). Writing the Data Analysis Plan. In: Pequegnat, W., Stover, E., Boyce, C. (eds) How to Write a Successful Research Grant Application. Springer, Boston, MA. https://doi.org/10.1007/978-1-4419-1454-5_22
Download citation
DOI : https://doi.org/10.1007/978-1-4419-1454-5_22
Published : 20 August 2010
Publisher Name : Springer, Boston, MA
Print ISBN : 978-1-4419-1453-8
Online ISBN : 978-1-4419-1454-5
eBook Packages : Medicine Medicine (R0)
Share this chapter
Anyone you share the following link with will be able to read this content:
Sorry, a shareable link is not currently available for this article.
Provided by the Springer Nature SharedIt content-sharing initiative
- Publish with us
Policies and ethics
- Find a journal
- Track your research
- Privacy Policy
Buy Me a Coffee

Home » How To Write A Research Proposal – Step-by-Step [Template]
How To Write A Research Proposal – Step-by-Step [Template]
Table of Contents

How To Write a Research Proposal
Writing a Research proposal involves several steps to ensure a well-structured and comprehensive document. Here is an explanation of each step:
1. Title and Abstract
- Choose a concise and descriptive title that reflects the essence of your research.
- Write an abstract summarizing your research question, objectives, methodology, and expected outcomes. It should provide a brief overview of your proposal.
2. Introduction:
- Provide an introduction to your research topic, highlighting its significance and relevance.
- Clearly state the research problem or question you aim to address.
- Discuss the background and context of the study, including previous research in the field.
3. Research Objectives
- Outline the specific objectives or aims of your research. These objectives should be clear, achievable, and aligned with the research problem.
4. Literature Review:
- Conduct a comprehensive review of relevant literature and studies related to your research topic.
- Summarize key findings, identify gaps, and highlight how your research will contribute to the existing knowledge.
5. Methodology:
- Describe the research design and methodology you plan to employ to address your research objectives.
- Explain the data collection methods, instruments, and analysis techniques you will use.
- Justify why the chosen methods are appropriate and suitable for your research.
6. Timeline:
- Create a timeline or schedule that outlines the major milestones and activities of your research project.
- Break down the research process into smaller tasks and estimate the time required for each task.
7. Resources:
- Identify the resources needed for your research, such as access to specific databases, equipment, or funding.
- Explain how you will acquire or utilize these resources to carry out your research effectively.
8. Ethical Considerations:
- Discuss any ethical issues that may arise during your research and explain how you plan to address them.
- If your research involves human subjects, explain how you will ensure their informed consent and privacy.
9. Expected Outcomes and Significance:
- Clearly state the expected outcomes or results of your research.
- Highlight the potential impact and significance of your research in advancing knowledge or addressing practical issues.
10. References:
- Provide a list of all the references cited in your proposal, following a consistent citation style (e.g., APA, MLA).
11. Appendices:
- Include any additional supporting materials, such as survey questionnaires, interview guides, or data analysis plans.
Research Proposal Format
The format of a research proposal may vary depending on the specific requirements of the institution or funding agency. However, the following is a commonly used format for a research proposal:
1. Title Page:
- Include the title of your research proposal, your name, your affiliation or institution, and the date.
2. Abstract:
- Provide a brief summary of your research proposal, highlighting the research problem, objectives, methodology, and expected outcomes.
3. Introduction:
- Introduce the research topic and provide background information.
- State the research problem or question you aim to address.
- Explain the significance and relevance of the research.
- Review relevant literature and studies related to your research topic.
- Summarize key findings and identify gaps in the existing knowledge.
- Explain how your research will contribute to filling those gaps.
5. Research Objectives:
- Clearly state the specific objectives or aims of your research.
- Ensure that the objectives are clear, focused, and aligned with the research problem.
6. Methodology:
- Describe the research design and methodology you plan to use.
- Explain the data collection methods, instruments, and analysis techniques.
- Justify why the chosen methods are appropriate for your research.
7. Timeline:
8. Resources:
- Explain how you will acquire or utilize these resources effectively.
9. Ethical Considerations:
- If applicable, explain how you will ensure informed consent and protect the privacy of research participants.
10. Expected Outcomes and Significance:
11. References:
12. Appendices:
Research Proposal Template
Here’s a template for a research proposal:
1. Introduction:
2. Literature Review:
3. Research Objectives:
4. Methodology:
5. Timeline:
6. Resources:
7. Ethical Considerations:
8. Expected Outcomes and Significance:
9. References:
10. Appendices:
Research Proposal Sample
Title: The Impact of Online Education on Student Learning Outcomes: A Comparative Study
1. Introduction
Online education has gained significant prominence in recent years, especially due to the COVID-19 pandemic. This research proposal aims to investigate the impact of online education on student learning outcomes by comparing them with traditional face-to-face instruction. The study will explore various aspects of online education, such as instructional methods, student engagement, and academic performance, to provide insights into the effectiveness of online learning.
2. Objectives
The main objectives of this research are as follows:
- To compare student learning outcomes between online and traditional face-to-face education.
- To examine the factors influencing student engagement in online learning environments.
- To assess the effectiveness of different instructional methods employed in online education.
- To identify challenges and opportunities associated with online education and suggest recommendations for improvement.
3. Methodology
3.1 Study Design
This research will utilize a mixed-methods approach to gather both quantitative and qualitative data. The study will include the following components:
3.2 Participants
The research will involve undergraduate students from two universities, one offering online education and the other providing face-to-face instruction. A total of 500 students (250 from each university) will be selected randomly to participate in the study.
3.3 Data Collection
The research will employ the following data collection methods:
- Quantitative: Pre- and post-assessments will be conducted to measure students’ learning outcomes. Data on student demographics and academic performance will also be collected from university records.
- Qualitative: Focus group discussions and individual interviews will be conducted with students to gather their perceptions and experiences regarding online education.
3.4 Data Analysis
Quantitative data will be analyzed using statistical software, employing descriptive statistics, t-tests, and regression analysis. Qualitative data will be transcribed, coded, and analyzed thematically to identify recurring patterns and themes.
4. Ethical Considerations
The study will adhere to ethical guidelines, ensuring the privacy and confidentiality of participants. Informed consent will be obtained, and participants will have the right to withdraw from the study at any time.
5. Significance and Expected Outcomes
This research will contribute to the existing literature by providing empirical evidence on the impact of online education on student learning outcomes. The findings will help educational institutions and policymakers make informed decisions about incorporating online learning methods and improving the quality of online education. Moreover, the study will identify potential challenges and opportunities related to online education and offer recommendations for enhancing student engagement and overall learning outcomes.
6. Timeline
The proposed research will be conducted over a period of 12 months, including data collection, analysis, and report writing.
The estimated budget for this research includes expenses related to data collection, software licenses, participant compensation, and research assistance. A detailed budget breakdown will be provided in the final research plan.
8. Conclusion
This research proposal aims to investigate the impact of online education on student learning outcomes through a comparative study with traditional face-to-face instruction. By exploring various dimensions of online education, this research will provide valuable insights into the effectiveness and challenges associated with online learning. The findings will contribute to the ongoing discourse on educational practices and help shape future strategies for maximizing student learning outcomes in online education settings.
About the author
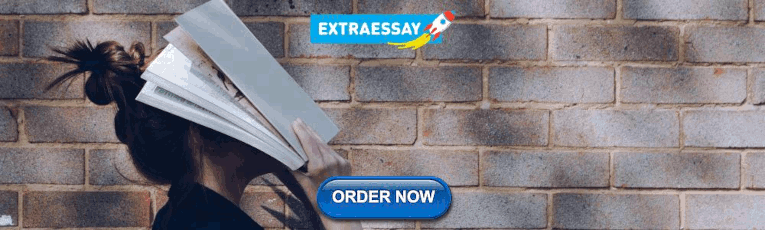
Muhammad Hassan
Researcher, Academic Writer, Web developer
You may also like

How To Write A Proposal – Step By Step Guide...

Grant Proposal – Example, Template and Guide

How To Write A Business Proposal – Step-by-Step...

Business Proposal – Templates, Examples and Guide

Proposal – Types, Examples, and Writing Guide

How to choose an Appropriate Method for Research?

- RIT Libraries
- Data Analytics Resources
- Writing a Research Proposal
- Electronic Books
- Print Books
- Data Science: Journals
- More Journals, Websites
- Alerts, IDS Express
- Readings on Data
- Sources with Data
- Google Scholar Library Links
- Zotero-Citation Management Tool
- Writing a Literature Review
- ProQuest Research Companion
- Thesis Submission Instructions
- Associations
Writing a Rsearch Proposal
A research proposal describes what you will investigate, why it’s important, and how you will conduct your research. Your paper should include the topic, research question and hypothesis, methods, predictions, and results (if not actual, then projected).
Research Proposal Aims
The format of a research proposal varies between fields, but most proposals will contain at least these elements:
- Introduction
Literature review
- Research design
Reference list
While the sections may vary, the overall objective is always the same. A research proposal serves as a blueprint and guide for your research plan, helping you get organized and feel confident in the path forward you choose to take.
Proposal Format
The proposal will usually have a title page that includes:
- The proposed title of your project
- Your supervisor’s name
- Your institution and department
Introduction The first part of your proposal is the initial pitch for your project. Make sure it succinctly explains what you want to do and why.. Your introduction should:
- Introduce your topic
- Give necessary background and context
- Outline your problem statement and research questions To guide your introduction , include information about:
- Who could have an interest in the topic (e.g., scientists, policymakers)
- How much is already known about the topic
- What is missing from this current knowledge
- What new insights will your research contribute
- Why you believe this research is worth doing
As you get started, it’s important to demonstrate that you’re familiar with the most important research on your topic. A strong literature review shows your reader that your project has a solid foundation in existing knowledge or theory. It also shows that you’re not simply repeating what other people have done or said, but rather using existing research as a jumping-off point for your own.
In this section, share exactly how your project will contribute to ongoing conversations in the field by:
- Comparing and contrasting the main theories, methods, and debates
- Examining the strengths and weaknesses of different approaches
- Explaining how will you build on, challenge, or synthesize prior scholarship
Research design and methods
Following the literature review, restate your main objectives . This brings the focus back to your project. Next, your research design or methodology section will describe your overall approach, and the practical steps you will take to answer your research questions. Write up your projected, if not actual, results.
Contribution to knowledge
To finish your proposal on a strong note, explore the potential implications of your research for your field. Emphasize again what you aim to contribute and why it matters.
For example, your results might have implications for:
- Improving best practices
- Informing policymaking decisions
- Strengthening a theory or model
- Challenging popular or scientific beliefs
- Creating a basis for future research
Lastly, your research proposal must include correct citations for every source you have used, compiled in a reference list . To create citations quickly and easily, you can use free APA citation generators like BibGuru. Databases have a citation button you can click on to see your citation. Sometimes you have to re-format it as the citations may have mistakes.
- << Previous: ProQuest Research Companion
- Next: DIR >>
Edit this Guide
Log into Dashboard
Use of RIT resources is reserved for current RIT students, faculty and staff for academic and teaching purposes only. Please contact your librarian with any questions.

Help is Available

Email a Librarian
A librarian is available by e-mail at [email protected]
Meet with a Librarian
Call reference desk voicemail.
A librarian is available by phone at (585) 475-2563 or on Skype at llll
Or, call (585) 475-2563 to leave a voicemail with the reference desk during normal business hours .
Chat with a Librarian
Data analytics resources infoguide url.
https://infoguides.rit.edu/DA
Use the box below to email yourself a link to this guide
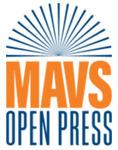
Want to create or adapt books like this? Learn more about how Pressbooks supports open publishing practices.
2.3 Data management and analysis
Learning objectives.
Learners will be able to…
- Define and construct a data analysis plan
- Define key quantitative data management terms—variable name, data dictionary, and observations/cases
- Differentiate between univariate and bivariate quantitative analysis
- Explain when we might use quantitative bivariate analysis in social work research
- Identify how your qualitative research question, research aim, and type of data may influence your choice of analytic methods
- Outline the steps you will take in preparation for conducting qualitative data analysis
After you have your raw data, whether this is secondary data or data you collected yourself, you will need to analyze it. While the specific steps to follow in quantitative or qualitative data analysis are beyond the scope of this chapter, we are going to address some basic concepts in this section to help you create a data analysis plan. A data analysis plan is an ordered outline that includes your research question, a description of the data you are going to use to answer it, and the exact step-by-step analyses that you plan to run to answer your research question. If you look back at Table 2.1, you will see that creating a data analysis plan is a part of the study design process. The data analysis plan flows from the research question, is integral to the study design, and should be well conceptualized prior to beginning data collection. In this section, we will walk through the basics of quantitative and qualitative data analysis to help you understand the fundamentals of creating a data analysis plan.
Quantitative Data: Management
When considering what data you might want to collect as part of your project, there are two important considerations that can create dilemmas for researchers. You might only get one chance to interact with your participants, so you must think comprehensively in your planning phase about what information you need and collect as much relevant data as possible. At the same time, though, especially when collecting sensitive information, you need to consider how onerous the data collection is for participants and whether you really need them to share that information. Just because something is interesting to us doesn’t mean it’s related enough to our research question to chase it down. Work with your research team and/or faculty early in your project to talk through these issues before you get to this point. And if you’re using secondary data, make sure you have access to all the information you need in that data before you use it.
Once you’ve collected your quantitative data, you need to make sure it is well-organized in a database in a way that’s actually usable. “Database” can be kind of a scary word, but really, it can be as simple as an Excel spreadsheet or a data file in whatever program you’re using to analyze your data. You may want to avoid Excel and use a formal database such as Microsoft Access or MySQL if you’ve got a large or complicated data set. But if your data set is smaller and you plan to keep your analyses simple, you can definitely get away with Excel. A typical data set is organized with variables as columns and observations/cases as rows. For example, let’s say we did a survey on ice cream preferences and collected the following information in Table 2.3:
There are a few key data management terms to understand:
- Variable name : Just what it sounds like—the name of your variable. Make sure this is something useful, short and, if you’re using something other than Excel, all one word. Most statistical programs will automatically rename variables for you if they aren’t one word, but the names can be a little ridiculous and long.
- Observations/cases : The rows in your data set. In social work, these are often your study participants (people), but can be anything from census tracts to black bears to trains. When we talk about sample size, we’re talking about the number of observations/cases. In our mini data set, each person is an observation/case.
- Data dictionary (also called a code book or metadata) : This is the document where you list your variable names, what the variables actually measure or represent, what each of the values of the variable mean if the meaning isn’t obvious (i.e., if there are numbers assigned to gender), the level of measurement and anything special to know about the variables (for instance, the source if you mashed two data sets together). If you’re using secondary data, the researchers sharing the data should make the data dictionary available.
Let’s take that mini data set we’ve got up above and we’ll show you what your data dictionary might look like in Table 2.4.
Quantitative Data: Univariate Analysis
As part of planning for your research, you should come up with a data analysis plan. Remember, a data analysis plan is an ordered outline that includes your research question, a description of the data you are going to use to answer it, and the exact step-by-step analyses that you plan to run to answer your research question. A basic data analysis plan might look something like what you see in Table 2.5. Don’t panic if you don’t yet understand some of the statistical terms in the plan; we’re going to delve into some of them in this section, and others will be covered in more depth in your statistics courses. Note here also that this is what operationalizing your variables and moving through your research with them looks like on a basic level. We will cover operationalization in more depth in Chapter 10.
An important point to remember is that you should never get stuck on using a particular statistical method because you or one of your co-researchers thinks it’s cool or it’s the hot thing in your field right now. You should certainly go into your data analysis plan with ideas, but in the end, you need to let your research question guide what statistical tests you plan to use. Be prepared to be flexible if your plan doesn’t pan out because the data is behaving in unexpected ways.
You’ll notice that the first step in the quantitative data analysis plan is univariate and descriptive statistics. Univariate data analysis is a quantitative method in which a variable is examined individually to determine its distribution , or the way the scores are distributed across the levels, or values, of that variable. When we talk about levels , what we are talking about are the possible values of the variable—like a participant’s age, income or gender. (Note that this is different from levels of measurement , which will be discussed in Chapter 11, but the level of measurement of your variables absolutely affects what kinds of analyses you can do with it.) Univariate analysis is non-relational , which just means that we’re not looking into how our variables relate to each other. Instead, we’re looking at variables in isolation to try to understand them better. For this reason, univariate analysis is used for descriptive research questions.
So when do you use univariate data analysis? Always! It should be the first thing you do with your quantitative data, whether you are planning to move on to more sophisticated statistical analyses or are conducting a study to describe a new phenomenon. You need to understand what the values of each variable look like—what if one of your variables has a lot of missing data because participants didn’t answer that question on your survey? What if there isn’t much variation in the gender of your sample? These are things you’ll learn through univariate analysis.
Quantitative Data: Bivariate Analysis
Did you know that ice cream causes shark attacks? It’s true! When ice cream sales go up in the summer, so does the rate of shark attacks. So you’d better put down that ice cream cone, unless you want to make yourself look more delicious to a shark.
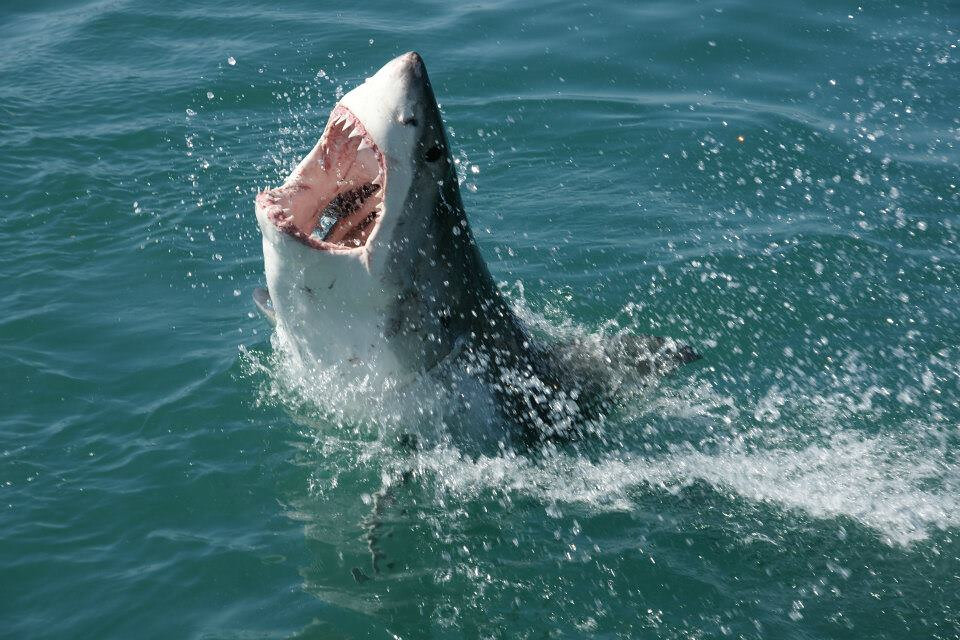
Ok, so it’s quite obviously not true that ice cream causes shark attacks. But if you looked at these two variables and how they’re related, you’d notice that during times of the year with high ice cream sales, there are also the most shark attacks. This is a classic example of the difference between correlation and causation. Despite the fact that the conclusion we drew about causation was wrong, it’s nonetheless true that these two variables appear related, and researchers figured that out through the use of bivariate analysis.
Bivariate analysis consists of a group of statistical techniques that examine the association between two variables. We could look at how anti-depressant medications and appetite are related, whether there is a relation between having a pet and emotional well-being, or if a policy-maker’s level of education is related to how they vote on bills related to environmental issues.
Bivariate analysis forms the foundation of multivariate analysis, which we don’t get to in this book. All you really need to know here is that there are steps beyond bivariate analysis, which you’ve undoubtedly seen in scholarly literature already! But before we can move forward with multivariate analysis, we need to understand the associations between the variables in our study.
Throughout your PhD program, you will learn much more about quantitative data analysis techniques, including more sophisticated multivariate analysis methods. Hopefully this section has provided you with some initial insights into how data is analyzed, and the importance of creating a data analysis plan prior to collecting data. Next, we will discuss some basic strategies for creating a qualitative data analysis plan.
Resources for Quantitative Data Analysis
While you are affiliated with a university, it is likely that you will have access to some kind of commercial statistics software. Examples in the previous section uses SPSS, the most common one our authoring team has seen in social work education. Like its competitors SAS and STATA, SPSS is expensive and your license to the software must be renewed every year (like a subscription). Even if you are able to install commercial statistics software on your computer, once your license expires, your program will no longer work. We believe that forcing students to learn software they will never use is wasteful and contributes to the (accurate, in many cases) perception from students that research class is unrelated to real-world practice. SPSS is more accessible due to its graphical user interface and does not require researchers to learn basic computer programming, but it is prohibitively costly if a student wanted to use it to measure practice data in their agency post-graduation.
Instead, we suggest getting familiar with JASP Statistics , a free and open-source alternative to SPSS developed and supported by the University of Amsterdam. It has a similar user interface as SPSS, and should be similarly easy to learn. Moreover, usability upgrades from SPSS like generating APA formatted tables make it a compelling option. While a great many of my students will rely on statistical analyses of their programs and practices in reports to funders, it is unlikely that any will use SPSS. Browse JASP’s how-to guide or consult this textbook Learning Statistics with JASP: A Tutorial for Psychology Students and Other Beginners , written by Danielle J. Navarro , David R. Foxcroft , and Thomas J. Faulkenberry .
Another open source statistics software package is R (a.k.a. The R Project for Statistical Computing ). R uses a command line interface, so you will need some coding knowledge in order to use it. Luckily, R is the most commonly used statistics software in the world, and the community of support and guides for using R are omnipresent online. For beginning researchers, consult the textbook Learning Statistics with R: A tutorial for psychology students and other beginners by Danielle J. Navarro .
While statistics software is sometimes needed to perform advanced statistical tests, most univariate and bivariate tests can be performed in spreadsheet software like Microsoft Excel, Google Sheets, or the free and open source LibreOffice Calc . Microsoft includes a ToolPak to perform complex data analysis as an add-on to Excel. For more information on using spreadsheet software to perform statistics, the open textbook Collaborative Statistics Using Spreadsheets by Susan Dean, Irene Mary Duranczyk, Barbara Illowsky, Suzanne Loch, and Janet Stottlemyer.
Statistical analysis is performed in just about every discipline, and as a result, there are a lot of openly licensed, free resources to assist you with your data analysis. We have endeavored to provide you the basics in the past few chapters, but ultimately, you will likely need additional support in completing quantitative data analysis from an instructor, textbook, or other resource. Browse the Open Textbook Library for statistics resources or look for video tutorials from reputable instructors like this video textbook on statistics by Bryan Koenig .
Qualitative Data: Management
Qualitative research often involves human participants and qualitative data can include of recordings or transcripts of their words, photographs or images, or diaries and documents. The personal nature of qualitative data poses the challenge of recognizability of sensitive information on individuals, communities, and places. If you choose this methodology for your research, you should familiarize yourself with policies, procedures, and rules to ensure safety and security of data in the documentation and dissemination process.
In any research involving primary data, a researcher is not only entrusted with the responsibility of upholding privacy of their participants but also accountable to them, making confidentiality and human subjects’ protection front and center of qualitative data management. Data such as audiotapes, videotapes, transcripts, notes, and other records should be stored and secured in locations where only authorized persons have access to them.
Sometimes in qualitative research, you will learn intimate details about people’s lives. Often, qualitative data contain personal identifiers. A helpful practice to ensure that participants confidentiality is to replace personal information in transcripts with pseudonyms or descriptive language (e.g., “[the participant’s sister]” instead of the sister’s name). Once audio and video recordings have been accurately transcribed with the de-identification of personal identifiers, the original recordings should be destroyed.
Qualitative Data: Analysis
There are many different types of qualitative data, including transcripts of interviews and focus groups, observational data, documents and other artifacts, and more. Your qualitative data analysis plan should be anchored in the type of data collected and the purpose of your study. Qualitative research can serve a range of purposes. Below is a brief list of general purposes we might consider when using a qualitative approach.
- Are you trying to understand how a particular group is affected by an issue?
- Are you trying to uncover how people arrive at a decision in a given situation?
- Are you trying to examine different points of view on the impact of a recent event?
- Are you trying to summarize how people understand or make sense of a condition?
- Are you trying to describe the needs of your target population?
If you don’t see the general aim of your research question reflected in one of these areas, don’t fret! This is only a small sampling of what you might be trying to accomplish with your qualitative study. Whatever your aim, you need to have a plan for what you will do once you have collected your data.
Iterative or Linear
Some qualitative research is linear , meaning it follows more of a traditionally quantitative process: create a plan, gather data, and analyze data; each step is completed before we proceed to the next. You can think of this like how information is presented in this book. We discuss each topic, one after another.
However, many times qualitative research is iterative , or evolving in cycles. An iterative approach means that once we begin collecting data, we also begin analyzing data as it is coming in. This early and ongoing analysis of our (incomplete) data then impacts our continued planning, data gathering and future analysis. Again, coming back to this book, while it may be written linear, we hope that you engage with it iteratively as you design and conduct your own research. By this we mean that you will revisit previous sections so you can understand how they fit together and you are in continuous process of building and revising how you think about the concepts you are learning about.
As you may have guessed, there are benefits and challenges to both linear and iterative approaches. A linear approach is much more straightforward, each step being fairly defined. However, linear research being more defined and rigid also presents certain challenges. A linear approach assumes that we know what we need to ask or look for at the very beginning of data collection, which often is not the case. Figure 2.1 contrasts the two approaches.
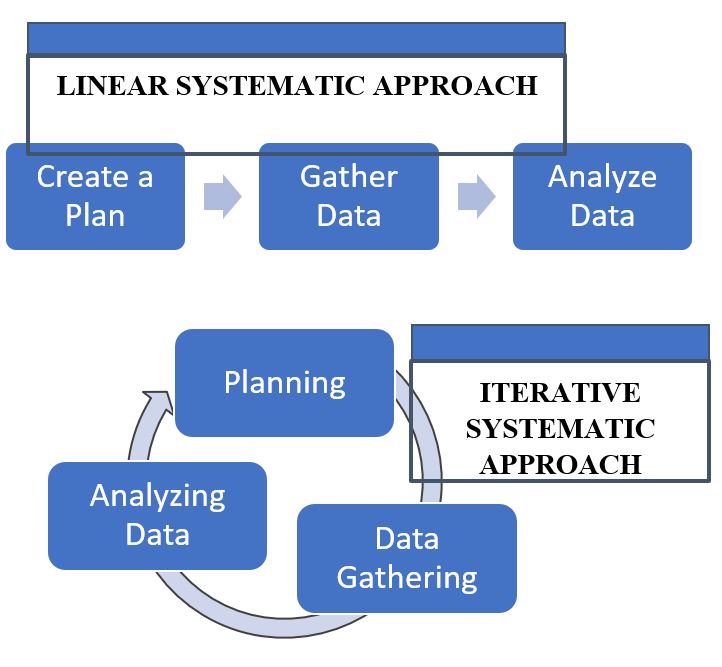
With iterative research, we have more flexibility to adapt our approach as we learn new things. We still need to keep our approach systematic and organized, however, so that our work doesn’t become a free-for-all. As we adapt, we do not want to stray too far from the original premise of our study. It’s also important to remember with an iterative approach that we may risk ethical concerns if our work extends beyond the original boundaries of our informed consent and institutional review board agreement (IRB; see Chapter 3 for more on IRBs). If you feel that you do need to modify your original research plan in a significant way as you learn more about the topic, you can submit an addendum to modify your original application that was submitted. Make sure to keep detailed notes of the decisions that you are making and what is informing these choices. This helps to support transparency and your credibility throughout the research process.
Acquainting yourself with your data
As you begin your analysis, you need to get to know your data. This often means reading through your data prior to any attempt at breaking it apart and labeling it. You might read through a couple of times, in fact. This helps give you a more comprehensive feel for each piece of data and the data as a whole, again, before you start to break it down into smaller units or deconstruct it. This is especially important if others assisted us in the data collection process. We often gather data as part of team and everyone involved in the analysis needs to be very familiar with all of the data.
Capturing your emerging understanding of the data
During your reviewing you will start to develop and evolve your understanding of what the data means. Coding is a part of the qualitative data analysis process where we begin to interpret and assign meaning to the data. It represents one of the first steps as we begin to filter the data through our own subjective lens as the researcher. This understanding of the data should be dynamic and flexible, but you want to have a way to capture this understanding as it evolves. You may include this as part of your qualitative codebook where you are tracking the main ideas that are emerging and what they mean. Table 2.6 is an example of how your thinking might change about a code and how you can go about capturing it.
There are a variety of different approaches to qualitative analysis, including thematic analysis, content analysis, grounded theory, phenomenology, photovoice, and more. The specific steps you will take to code your qualitative data, and to generate themes from these codes, will vary based on the analytic strategy you are employing. In designing your qualitative study, you would identify an analytical approach as you plan out your project. The one you select would depend on the type of data you have and what you want to accomplish with it. In Chapter 19, we will go into more detail about various types of qualitative data analysis. Each qualitative approach has specific techniques and methods that take substantial study and practice to master.
Key Takeaways
- Getting organized at the beginning of your project with a data analysis plan will help keep you on track. Data analysis plans should include your research question, a description of your data, and a step-by-step outline of what you’re going to do with it. [chapter 14.1]
- Be flexible with your data analysis plan—sometimes data surprises us and we have to adjust the statistical tests we are using. [chapter 14.1]
- Always make a data dictionary or, if using secondary data, get a copy of the data dictionary so you (or someone else) can understand the basics of your data. [chapter 14.1]
- Bivariate analysis is a group of statistical techniques that examine the relationship between two variables. [chapter 15.1]
- You need to conduct bivariate analyses before you can begin to draw conclusions from your data, including in future multivariate analyses. [chapter 15.1]
- There are a lot of high quality and free online resources to learn and perform statistical analysis.
- Qualitative research analysis requires preparation and careful planning. You will need to take time to familiarize yourself with the data in a general sense before you begin analyzing. [chapter 19.3]
- The specific steps you will take to code your qualitative data and generate final themes will depend on the qualitative analytic approach you select.
TRACK 1 (IF YOU ARE CREATING A RESEARCH PROPOSAL FOR THIS CLASS)
- Make a data analysis plan for your project. Remember this should include your research question, a description of the data you will use, and a step-by-step outline of what you’re going to do with your data once you have it, including statistical tests (non-relational and relational) that you plan to use. You can do this exercise whether you’re using quantitative or qualitative data! The same principles apply.
- Make a data dictionary for the data you are proposing to collect as part of your study. You can use the example above as a template.
TRACK 2 (IF YOU AREN’T CREATING A RESEARCH PROPOSAL FOR THIS CLASS)
You are researching the impact of your city’s recent harm reduction interventions for intravenous drug users (e.g., sterile injection kits, monitored use, overdose prevention, naloxone provision, etc.).
- Make a draft quantitative data analysis plan for your project. Remember this should include your research question, a description of the data you will use, and a step-by-step outline of what you’re going to do with your data once you have it, including statistical tests (non-relational and relational) that you plan to use. It’s okay if you don’t yet have a complete idea of the types of statistical analyses you might use.
An ordered outline that includes your research question, a description of the data you are going to use to answer it, and the exact analyses, step-by-step, that you plan to run to answer your research question.
The name of your variable.
The rows in your data set. In social work, these are often your study participants (people), but can be anything from census tracts to black bears to trains.
This is the document where you list your variable names, what the variables actually measure or represent, what each of the values of the variable mean if the meaning isn't obvious.
process by which researchers spell out precisely how a concept will be measured in their study
A group of statistical techniques that examines the relationship between at least three variables
Univariate data analysis is a quantitative method in which a variable is examined individually to determine its distribution.
the way the scores are distributed across the levels of that variable.
Chapter Outline
- Practical and ethical considerations ( 14 minute read)
- Raw data (10 minute read)
- Creating a data analysis plan (?? minute read)
- Critical considerations (3 minute read)
Content warning: Examples in this chapter discuss substance use disorders, mental health disorders and therapies, obesity, poverty, gun violence, gang violence, school discipline, racism and hate groups, domestic violence, trauma and triggers, incarceration, child neglect and abuse, bullying, self-harm and suicide, racial discrimination in housing, burnout in helping professions, and sex trafficking of indigenous women.
2.1 Practical and ethical considerations
Learners will be able to...
- Identify potential stakeholders and gatekeepers
- Differentiate between raw data and the results of scientific studies
- Evaluate whether you can feasibly complete your project
Pre-awareness check (Knowledge)
Similar to practice settings, research has ethical considerations that must be taken to ensure the safety of participants. What ethical considerations were relevant to your practice experience that may have impacted the delivery of services?
As a PhD student, you will have many opportunities to conduct research. You may be asked to be a part of a research team led by the faculty at your institution. You will also conduct your own research for your dissertation. As you will learn, research can take many forms. For example, you may want to focus qualitatively on individuals’ lived experiences, or perhaps you will quantitatively assess the impact of interventions on research subjects. You may work with large, already-existing datasets, or you may create your own data. Though social work research can vary widely from project to project, researchers typically follow the same general process, even if their specific research questions and methodologies differ. Table 2.1 outlines the major components of the research process covered in this textbook, and indicates the chapters where you will find more information on each subject. You will notice that your research paradigm is an organizing framework that guides each component of the research process.
Table 2.1 Components of the Research Process
Feasibility
Feasibility refers to whether you can practically conduct the study you plan to do, given the resources and ethical obligations you have. In this chapter, we will review some important practical and ethical considerations researchers should start thinking about from the beginning of a research project. These considerations apply to all research, but it is important to also consider the context of research and researchers when thinking about feasibility.
For example, as a doctoral student, you likely have a unique set of circumstances that inspire and constrain your research. Some students have the ability to engage in independent studies where they can gain skills and expertise in specialized research methods to prepare them for a research-intensive career. Others may have reasons, such as a limited amount of funding or family concerns, that encourage them to complete their dissertation research as quickly as possible. These circumstances relate to the feasibility of a research project. Regardless of the potential societal importance of a 10-year longitudinal study, it’s not feasible for a student to conduct it in time to graduate! Your dissertation chair, doctoral program director, and other faculty mentors can help you navigate the many decisions you will face as a doctoral student about conducting independent research or joining research projects.
The context and role of the researcher continue to affect feasibility even after a doctoral student graduates. Many will continue in their careers to become tenure track faculty with research expectations to obtain tenure. Some funders expect faculty members to have a track record of successful projects before trusting them to lead expensive or long-term studies. Realistically, these expectations will influence what research is feasible for a junior faculty member to conduct. Just like for doctoral students, mentorship is incredibly valuable for junior faculty to make informed decisions about what research to conduct. Senior faculty, associate deans of research, chairs, and deans can help junior faculty decide what projects to pursue to ensure they meet the expectations placed on them without losing sight of the reasons they became a researcher in the first place.
As you read about other feasibility considerations such as gaining access, consent, and collecting data, consider the ways in which context and roles also influence feasibility.
Access, consent, and ethical obligations
One of the most important feasibility issues is gaining access to your target population. For example, let’s say you wanted to better understand middle-school students who engaged in self-harm behaviors. That is a topic of social importance, but what challenges might you face in accessing this population? Let's say you proposed to identify students from a local middle school and interview them about self-harm. Methodologically, that sounds great since you are getting data from those with the most knowledge about the topic, the students themselves. But practically, that sounds challenging. Think about the ethical obligations a social work practitioner has to adolescents who are engaging in self-harm (e.g., competence, respect). In research, we are similarly concerned mostly with the benefits and harms of what you propose to do as well as the openness and honesty with which you share your project publicly.
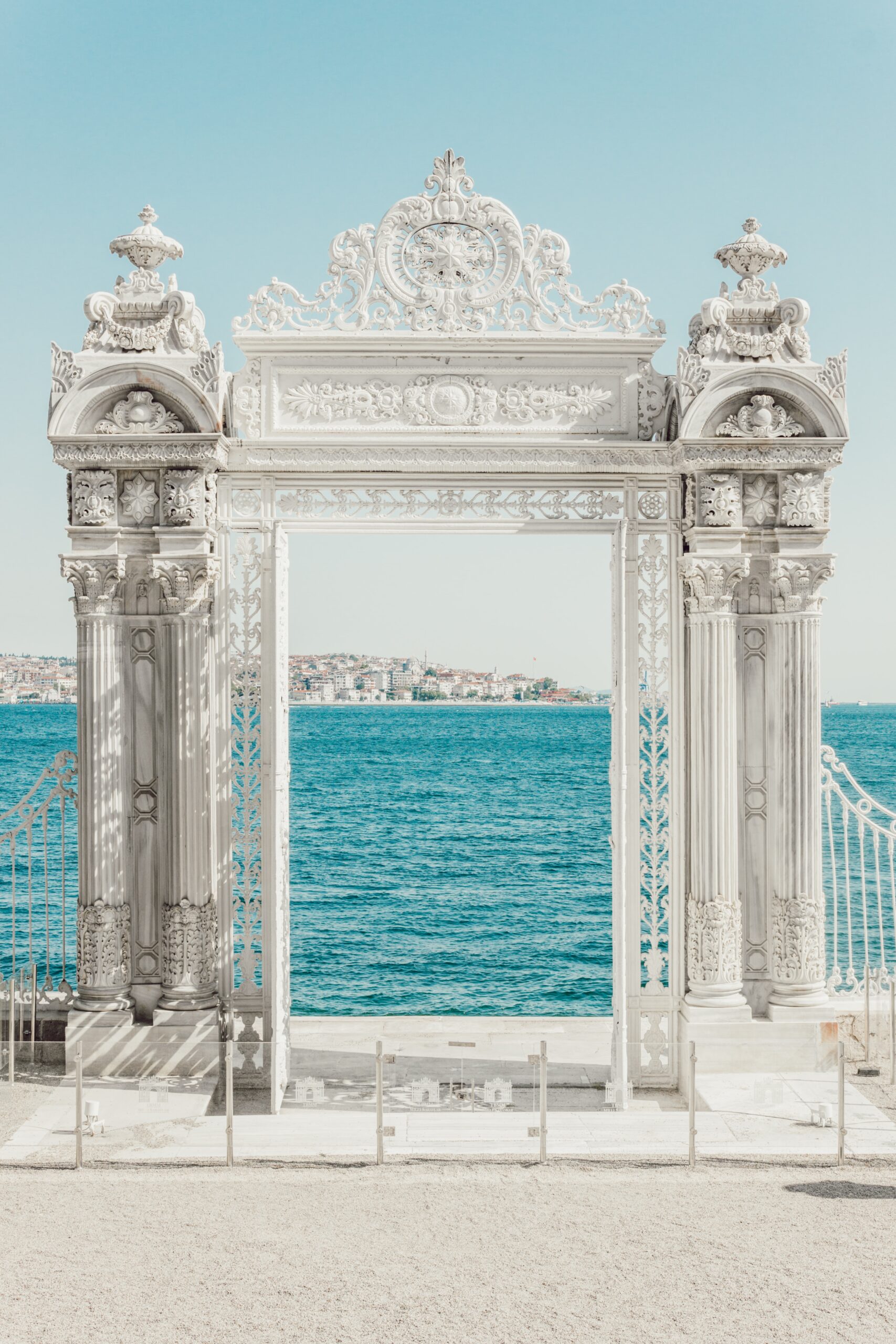
Gatekeepers
If you were the principal at your local middle school, would you allow researchers to interview kids in your schools about self-harm? What if the results of the study showed that self-harm was a big problem that your school was not addressing? What if the researcher's interviews themselves caused an increase in self-harming behaviors among the children? The principal in this situation is a gatekeeper . Gatekeepers are the individuals or organizations who control access to the population you want to study. The school board would also likely need to give consent for the research to take place at their institution. Gatekeepers must weigh their ethical questions because they have a responsibility to protect the safety of the people at their organization, just as you have an ethical obligation to protect the people in your research study.
For vulnerable populations, it can be a challenge to get consent from gatekeepers to conduct your research project. As a result, researchers often conduct research projects in places where they have established trust with gatekeepers. In the case where the population (children who self-harm) are too vulnerable, researchers may collect data from people who have secondary knowledge about the topic. For example, the principal may be more willing to let you talk to teachers or staff, rather than children.
Stakeholders
In some cases, researchers and gatekeepers partner on a research project. When this happens, the gatekeepers become stakeholders . Stakeholders are individuals or groups who have an interest in the outcome of the study you conduct. As you think about your project, consider whether there are formal advisory groups or boards (like a school board) or advocacy organizations who already serve or work with your target population. Approach them as experts and ask for their review of your study to see if there are any perspectives or details you missed that would make your project stronger.
There are many advantages to partnering with stakeholders to complete a research project together. Continuing with our example on self-harm in schools, in order to obtain access to interview children at a middle school, you will have to consider other stakeholders' goals. School administrators also want to help students struggling with self-harm, so they may want to use the results to form new programs. But they may also need to avoid scandal and panic if the results show high levels of self-harm. Most likely, they want to provide support to students without making the problem worse. By bringing in school administrators as stakeholders, you can better understand what the school is currently doing to address the issue and get an informed perspective on your project's questions. Negotiating the boundaries of a stakeholder relationship requires strong meso-level practice skills.
Of course, partnering with administrators probably sounds quite a bit easier than bringing on board the next group of stakeholders—parents. It's not ethical to ask children to participate in a study without their parents' consent. We will review the parameters of parental and child consent in Chapter 5 . Parents may be understandably skeptical of a researcher who wants to talk to their child about self-harm, and they may fear potential harm to the child and family from your study. Would you let a researcher you didn't know interview your children about a very sensitive issue?
Social work research must often satisfy multiple stakeholders. This is especially true if a researcher receives a grant to support the project, as the funder has goals it wants to accomplish by funding the research project. Your university is also a stakeholder in your project. When you conduct research, it reflects on your school. If you discover something of great importance, your school looks good. If you harm someone, they may be liable. Your university likely has opportunities for you to share your research with the campus community, and may have incentives or grant programs for researchers. Your school also provides you with support and access to resources like the library and data analysis software.
Target population
So far, we've talked about access in terms of gatekeepers and stakeholders. Let's assume all of those people agree that your study should proceed. But what about the people in the target population? They are the most important stakeholder of all! Think about the children in our proposed study on self-harm. How open do you think they would be to talking to you about such a sensitive issue? Would they consent to talk to you at all?
Maybe you are thinking about simply asking clients on your caseload. As we talked about before, leveraging existing relationships created through field work can help with accessing your target population. However, they introduce other ethical issues for researchers. Asking clients on your caseload or at your agency to participate in your project creates a dual relationship between you and your client. What if you learn something in the research project that you want to share with your clinical team? More importantly, would your client feel uncomfortable if they do not consent to your study? Social workers have power over clients, and any dual relationship would require strict supervision in the rare case it was allowed.
Resources and scope
Let's assume everyone consented to your project and you have adequately addressed any ethical issues with gatekeepers, stakeholders, and your target population. That means everything is ready to go, right? Not quite yet. As a researcher, you will need to carry out the study you propose to do. Depending on how big or how small your proposed project is, you’ll need a little or a lot of resources.
One thing that all projects need is raw data . Raw data can come in may forms. Very often in social science research, raw data includes the responses to a survey or transcripts of interviews and focus groups, but raw data can also include experimental results, diary entries, art, or other data points that social scientists use in analyzing the world. Primary data is data you have collected yourself. Sometimes, social work researchers do not collect raw data of their own, but instead use secondary data analysis to analyze raw data that has been shared by other researchers. Secondary data is data someone else has collected that you have permission to use in your research. For example, you could use data from a local probation program to determine if a shoplifting prevention group was reducing the rate at which people were re-offending. You would need data on who participated in the program and their criminal history six months after the end of their probation period. This is secondary data you could use to determine whether the shoplifting prevention group had any effect on an individual's likelihood of re-offending. Whether a researcher should use secondary data or collect their own raw data is an important choice which we will discuss in greater detail in section 2.2. Collecting raw data or obtaining secondary data can be time consuming or expensive, but without raw data there can be no research project.
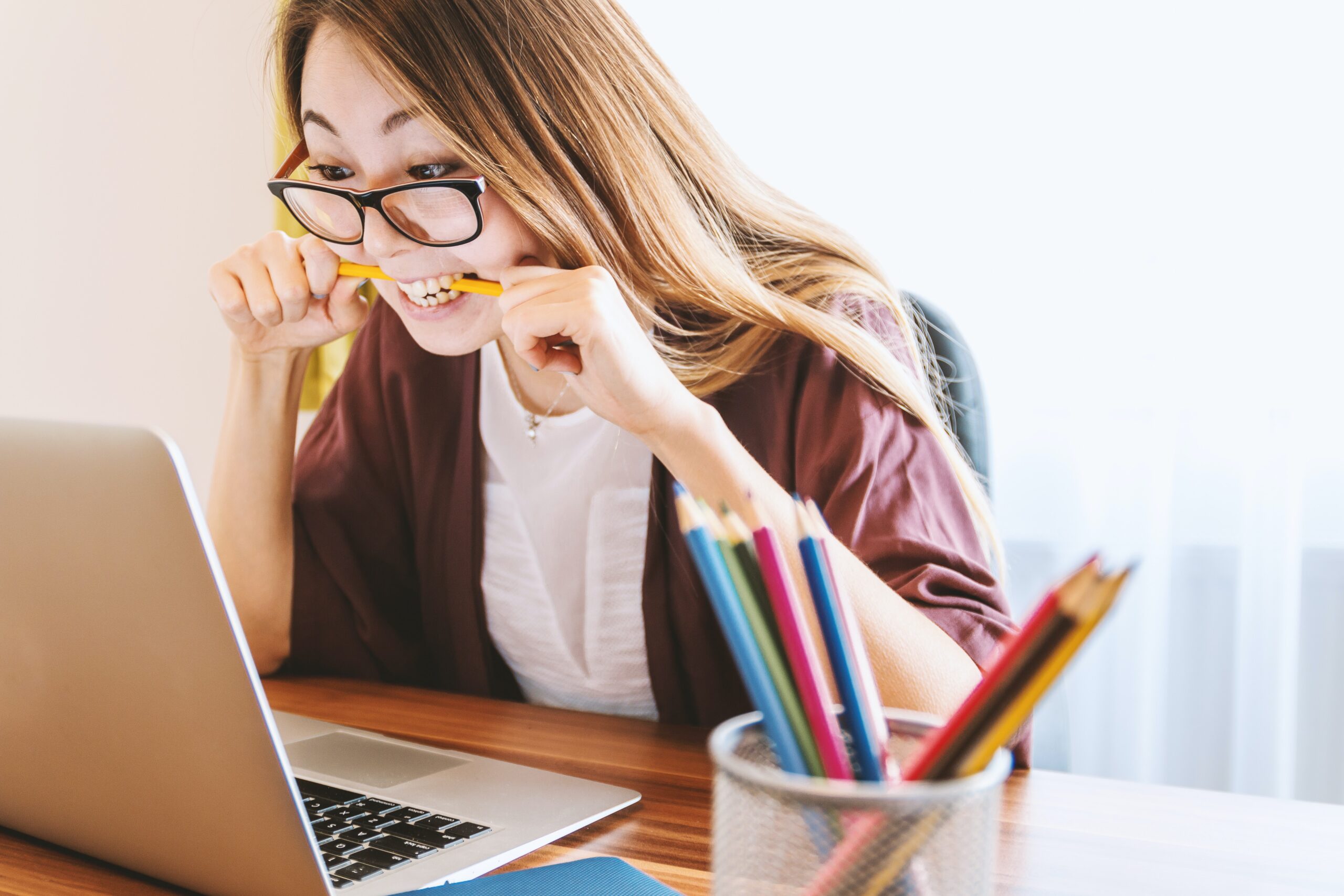
Time is an important resource to consider when designing research projects. Make sure that your proposal won't require you to spend more time than you have to collect and analyze data. Think realistically about the timeline for your research project. If you propose to interview fifty mental health professionals in their offices in your community about your topic, make sure you can dedicate fifty hours to conduct those interviews, account for travel time, and think about how long it will take to transcribe and analyze those interviews.
- What is reasonable for you to do in your timeframe?
- How many hours each week can the research team dedicate to this project?
One thing that can delay a research project is receiving approval from the institutional review board (IRB), the research ethics committee at your university. If your study involves human subjects , you may have to formally propose your study to the IRB and get their approval before gathering your data. A well-prepared study is likely to gain IRB approval with minimal revisions needed, but the process can take weeks to complete and must be done before data collection can begin. We will address the ethical obligations of researchers in greater detail in Chapter 5 .
Most research projects cost some amount of money. Potential expenses include wages for members of the research team, incentives for research participants, travel expenses, and licensing costs for standardized instruments. Most researchers seek grant funding to support the research. Grant applications can be time consuming to write and grant funding can be competitive to receive.
Knowledge, competence, and skills
For social work researchers, the social work value of competence is key in their research ethics.
Clearly, researchers need to be skilled in working with their target population in order to conduct ethical research. Some research addresses this challenge by collecting data from competent practitioners or administrators who have second-hand knowledge of target populations based on professional relationships. Members of the research team delivering an intervention also need to have training and skills in the intervention. For example, if a research study examines the effectiveness of dialectical behavioral therapy (DBT) in a particular context, the person delivering the DBT must be certified in DBT. Another idea to keep in mind is the level of data collection and analysis skills needed to complete the project. Some assessments require training to administer. Analyses may be complex or require statistical consultation or advanced training.
In summary, here are a few questions you should ask yourself about your project to make sure it's feasible. While we present them early on in the research process (we're only in Chapter 2), these are certainly questions you should ask yourself throughout the proposal writing process. We will revisit feasibility again in Chapter 9 when we work on finalizing your research question .
- Do you have access to the data you need or can you collect the data you need?
- Will you be able to get consent from stakeholders, gatekeepers, and your target population?
- Does your project pose risk to individuals through direct harm, dual relationships, or breaches in confidentiality?
- Are you competent enough to complete the study?
- Do you have the resources and time needed to carry out the project?
- People will have to say “yes” to your research project. Evaluate whether your project might have gatekeepers or potential stakeholders. They may control access to data or potential participants.
- Researchers need raw data such as survey responses, interview transcripts, or client charts. Your research project must involve more than looking at the analyses conducted by other researchers, as the literature review is only the first step of a research project.
- Make sure you have enough resources (time, money, and knowledge) to complete your research project.
Post-awareness check (Emotion)
What factors have created your passion toward assisting your target population? How can this connection enhance your ability to receive a “yes” from potential participants? What are the anticipated challenges to receiving a “yes” from potential participants?
Think about how you might answer your question by collecting your own data.
- Identify any gatekeepers and stakeholders you might need to contact.
- How can you increase the likelihood you will get access to the people or records you need for your study?
Describe the resources you will need for your project.
- Do you have concerns about feasibility?
TRACK 2 (IF YOU AREN'T CREATING A RESEARCH PROPOSAL FOR THIS CLASS)
You are researching the impact of your city's recent harm reduction interventions for intravenous drug users (e.g., sterile injection kits, monitored use, overdose prevention, naloxone provision, etc.).
- Thinking about the services related to this issue in your own city, identify any gatekeepers and stakeholders you might need to contact.
- How might you approach these gatekeepers and stakeholders? How would you explain your study?
2.2 Raw data
- Identify potential sources of available data
- Weigh the challenges and benefits of collecting your own data
In our previous section, we addressed some of the challenges researchers face in collecting and analyzing raw data. Just as a reminder, raw data are unprocessed, unanalyzed data that researchers analyze using social science research methods. It is not just the statistics or qualitative themes in journal articles. It is the actual data from which those statistical outputs or themes are derived (e.g., interview transcripts or survey responses).
There are two approaches to getting raw data. First, students can analyze data that are publicly available or from agency records. Using secondary data like this can make projects more feasible, but you may not find existing data that are useful for answering your working question. For that reason, many students gather their own raw data. As we discussed in the previous section, potential harms that come from addressing sensitive topics mean that surveys and interviews of practitioners or other less-vulnerable populations may be the most feasible and ethical way to approach data collection.
Using secondary data
Within the agency setting, there are two main sources of raw data. One option is to examine client charts. For example, if you wanted to know if substance use was related to parental reunification for youth in foster care, you could look at client files and compare how long it took for families with differing levels of substance use to be reunified. You will have to negotiate with the agency the degree to which your analysis can be public. Agencies may be okay with you using client files for a class project but less comfortable with you presenting your findings at a city council meeting. When analyzing data from your agency, you will have to manage a stakeholder relationship.
Another great example of agency-based raw data comes from program evaluations. If you are working with a grant funded agency, administrators and clinicians are likely producing data for grant reporting. The agency may consent to have you look at the raw data and run your own analysis. Larger agencies may also conduct internal research—for example, surveying employees or clients about new initiatives. These, too, can be good sources of available data. Generally, if the agency has already collected the data, you can ask to use them. Again, it is important to be clear on the boundaries and expectations of the agency. And don't be angry if they say no!
Some agencies, usually government agencies, publish their data in formal reports. You could take a look at some of the websites for county or state agencies to see if there are any publicly available data relevant to your research topic. As an example, perhaps there are annual reports from the state department of education that show how seclusion and restraint is disproportionately applied to Black children with disabilities , as students found in Virginia. In another example, one student matched public data from their city's map of criminal incidents with historically redlined neighborhoods. For this project, she is using publicly available data from Mapping Inequality , which digitized historical records of redlined housing communities and the Roanoke, VA crime mapping webpage . By matching historical data on housing redlining with current crime records, she is testing whether redlining still impacts crime to this day.
Not all public data are easily accessible, though. The student in the previous example was lucky that scholars had digitized the records of how Virginia cities were redlined by race. Sources of historical data are often located in physical archives, rather than digital archives. If your project uses historical data in an archive, it would require you to physically go to the archive in order to review the data. Unless you have a travel budget, you may be limited to the archival data in your local libraries and government offices. Similarly, government data may have to be requested from an agency, which can take time. If the data are particularly sensitive or if the department would have to dedicate a lot of time to your request, you may have to file a Freedom of Information Act request. This process can be time-consuming, and in some cases, it will add financial cost to your study.
Another source of secondary data is shared by researchers as part of the publication and review process. There is a growing trend in research to publicly share data so others can verify your results and attempt to replicate your study. In more recent articles, you may notice links to data provided by the researcher. Often, these have been de-identified by eliminating some information that could lead to violations of confidentiality. You can browse through the data repositories in Table 2.1 to find raw data to analyze. Make sure that you pick a data set with thorough and easy to understand documentation. You may also want to use Google's dataset search which indexes some of the websites below as well as others in a very intuitive and easy to use way.
Ultimately, you will have to weigh the strengths and limitations of using secondary data on your own. Engel and Schutt (2016, p. 327) [1] propose six questions to ask before using secondary data:
- What were the agency’s or researcher’s goals in collecting the data?
- What data were collected, and what were they intended to measure?
- When was the information collected?
- What methods were used for data collection? Who was responsible for data collection, and what were their qualifications? Are they available to answer questions about the data?
- How is the information organized (by date, individual, family, event, etc.)? Are identifiers used to indicate different types of data available?
- What is known about the success of the data collection effort? How are missing data indicated and treated? What kind of documentation is available? How consistent are the data with data available from other sources?
In this section, we've talked about data as though it is always collected by scientists and professionals. But that's definitely not the case! Think more broadly about sources of data that are already out there in the world. Perhaps you want to examine the different topics mentioned in the past 10 State of the Union addresses by the President. Or maybe you want to examine whether the websites and public information about local health and mental health agencies use gender-inclusive language. People share their experiences through blogs, social media posts, videos, performances, among countless other sources of data. When you think broadly about data, you'll be surprised how much you can answer with available data.
Collecting your own raw data
The primary benefit of collecting your own data is that it allows you to collect and analyze the specific data you are looking for, rather than relying on what other people have shared. You can make sure the right questions are asked to the right people. Your early research projects may be smaller in scope. This isn't necessarily a limitation. Early projects are often the first step in a long research trajectory in which the same topic is studied in increasing detail and sophistication over time.
Student researchers often propose to survey or interview practitioners. The focus of these projects should be about the practice of social work and the study will uncover how practitioners understand what they do. Surveys of practitioners often test whether responses to questions are related to each other. For example, you could propose to examine whether someone's length of time in practice was related to the type of therapy they use or their level of burnout. Interviews or focus groups can also illuminate areas of practice. One student proposed to conduct focus groups of individuals in different helping professions in order to understand how they viewed the process of leaving an abusive partner. She suspected that people from different disciplines would make unique assumptions about the survivor's choices.
It's worth remembering here that you need to have access to practitioners, as we discussed in the previous section. Resourceful researchers will look at publicly available databases of practitioners, draw from agency and personal contacts, or post in public forums like Facebook groups. Consent from gatekeepers is important, and as we described earlier, you and your agency may be interested in collaborating on a project. Bringing your agency on board as a stakeholder in your project may allow you access to company email lists or time at staff meetings as well as access to practitioners. One student partnered with her internship placement at a local hospital to measure the burnout that nurses experienced in their department. Her project helped the agency identify which departments may need additional support.
Another possible way you could collect data is by partnering with your agency on evaluating an existing program. Perhaps they want you to evaluate the early stage of a program to see if it's going as planned and if any changes need to be made. Maybe there is an aspect of the program they haven't measured but would like to, and you can fill that gap for them. Collaborating with agency partners in this way can be a challenge, as you must negotiate roles, get stakeholder buy-in, and manage the conflicting time schedules of field work and research work. At the same time, it allows you to make your work immediately relevant to your specific practice and client population.
In summary, many early projects fall into one of the following categories. These aren't your only options! But they may be helpful in thinking about what research projects can look like.
- Analyzing charts or program evaluations at an agency
- Analyzing existing data from an agency, government body, or other public source
- Analyzing popular media or cultural artifacts
- Surveying or interviewing practitioners, administrators, or other less-vulnerable groups
- Conducting a program evaluation in collaboration with an agency
- All research projects require analyzing raw data.
- Research projects often analyze available data from agencies, government, or public sources. Doing so allows researchers to avoid the process of recruiting people to participate in their study. This makes projects more feasible but limits what you can study to the data that are already available to you.
- Think through the potential harm of discussing sensitive topics when surveying or interviewing clients and other vulnerable populations. Since many social work topics are sensitive, researchers often collect data from less-vulnerable populations such as practitioners and administrators.
Post-awareness check (Environment)
In what environment are you most comfortable in data collection (phone calls, face to face recruitment, etc)? Consider your preferred method of data collection that may align with both your personality and your target population.
- Describe the difference between raw data and the results of research articles.
- Consider browsing around the data repositories in Table 2.1.
- Identify a common type of project (e.g., surveys of practitioners) and how conducting a similar project might help you answer your working question.
- What kind of raw data might you collect yourself for your study?
2.3 Creating a data analysis plan
- Define and construct a data analysis plan.
- Define key quantitative data management terms—variable name, data dictionary, primary and secondary data, observations/cases.
- Differentiate between univariate and bivariate quantitative analysis.
- Explain when we might use quantitative bivariate analysis in social work research.
- Identify how your qualitative research question, research aim, and type of data may influence your choice of analytic methods.
- Outline the steps you will take in preparation for conducting qualitative data analysis.
After you have your raw data , whether this is secondary data or data you collected yourself, you will need to analyze it. While the specific steps to follow in quantitative or qualitative data analysis are beyond the scope of this chapter, we are going to address some basic concepts in this section to help you create a data analysis plan. A data analysis plan is an ordered outline that includes your research question, a description of the data you are going to use to answer it, and the exact step-by-step analyses that you plan to run to answer your research question. If you look back at Table 2.1, you will see that creating a data analysis plan is a part of the study design process. The data analysis plan flows from the research question, is integral to the study desig n, and should be well conceptualized prior to beginning data collection. In this section, we will walk through the basics of quantitative and qualitative data analysis to help you understand the fundamentals of creating a data analysis plan.
When considering what data you might want to collect as part of your project, there are two important considerations that can create dilemmas for researchers. You might only get one chance to interact with your participants, so you must think comprehensively in your planning phase about what information you need and collect as much relevant data as possible. At the same time, though, especially when collecting sensitive information, you need to consider how onerous the data collection is for participants and whether you really need them to share that information. Just because something is interesting to us doesn't mean it's related enough to our research question to chase it down. Work with your research team and/or faculty early in your project to talk through these issues before you get to this point. And if you're using secondary data, make sure you have access to all the information you need in that data before you use it.
Once you've collected your quantitative data, you need to make sure it is well- organized in a database in a way that's actually usable. "Database" can be kind of a scary word, but really, it can be as simple as an Excel spreadsheet or a data file in whatever program you're using to analyze your data. You may want to avoid Excel and use a formal database such as Microsoft Access or MySQL if you've got a large or complicated data set. But if your data set is smaller and you plan to keep your analyses simple, you can definitely get away with Excel. A typical data set is organized with variables as columns and observations/cases as rows. For example, let's say we did a survey on ice cream preferences and collected the following information in Table 2.3:
- Variable name : Just what it sounds like—the name of your variable. Make sure this is something useful, short and, if you're using something other than Excel, all one word. Most statistical programs will automatically rename variables for you if they aren't one word, but the names can be a little ridiculous and long.
- Observations/cases : The rows in your data set. In social work, these are often your study participants (people), but can be anything from census tracts to black bears to trains. When we talk about sample size, we're talking about the number of observations/cases. In our mini data set, each person is an observation/case.
- Data dictionary (sometimes called a code book or metadata) : This is the document where you list your variable names, what the variables actually measure or represent, what each of the values of the variable mean if the meaning isn't obvious (i.e., if there are numbers assigned to gender), the level of measurement and anything special to know about the variables (for instance, the source if you mashed two data sets together). If you're using secondary data, the researchers sharing the data should make the data dictionary available .
Let's take that mini data set we've got up above and we'll show you what your data dictionary might look like in Table 2.4.
As part of planning for your research, you should come up with a data analysis plan. Remember, a data analysis plan is an ordered outline that includes your research question, a description of the data you are going to use to answer it, and the exact step-by-step analyses that you plan to run to answer your research question. A basic data analysis plan might look something like what you see in Table 2.5. Don't panic if you don't yet understand some of the statistical terms in the plan; we're going to delve into some of them in this section, and others will be covered in more depth in your statistics courses. Note here also that this is what operationalizing your variables and moving through your research with them looks like on a basic level. We will cover operationalization in more depth in Chapter 11.
An important point to remember is that you should never get stuck on using a particular statistical method because you or one of your co-researchers thinks it's cool or it's the hot thing in your field right now. You should certainly go into your data analysis plan with ideas, but in the end, you need to let your research question guide what statistical tests you plan to use. Be prepared to be flexible if your plan doesn't pan out because the data is behaving in unexpected ways.
You'll notice that the first step in the quantitative data analysis plan is univariate and descriptive statistics. Univariate data analysis is a quantitative method in which a variable is examined individually to determine its distribution , or the way the scores are distributed across the levels, or values, of that variable. When we talk about levels , what we are talking about are the possible values of the variable—like a participant's age, income or gender. (Note that this is different from levels of measurement , which will be discussed in Chapter 11, but the level of measurement of your variables absolutely affects what kinds of analyses you can do with it.) Univariate analysis is n on-relational , which just means that we're not looking into how our variables relate to each other. Instead, we're looking at variables in isolation to try to understand them better. For this reason, univariate analysis is used for descriptive research questions.
So when do you use univariate data analysis? Always! It should be the first thing you do with your quantitative data, whether you are planning to move on to more sophisticated statistical analyses or are conducting a study to describe a new phenomenon. You need to understand what the values of each variable look like—what if one of your variables has a lot of missing data because participants didn't answer that question on your survey? What if there isn't much variation in the gender of your sample? These are things you'll learn through univariate analysis.
Did you know that ice cream causes shark attacks? It's true! When ice cream sales go up in the summer, so does the rate of shark attacks. So you'd better put down that ice cream cone, unless you want to make yourself look more delicious to a shark.
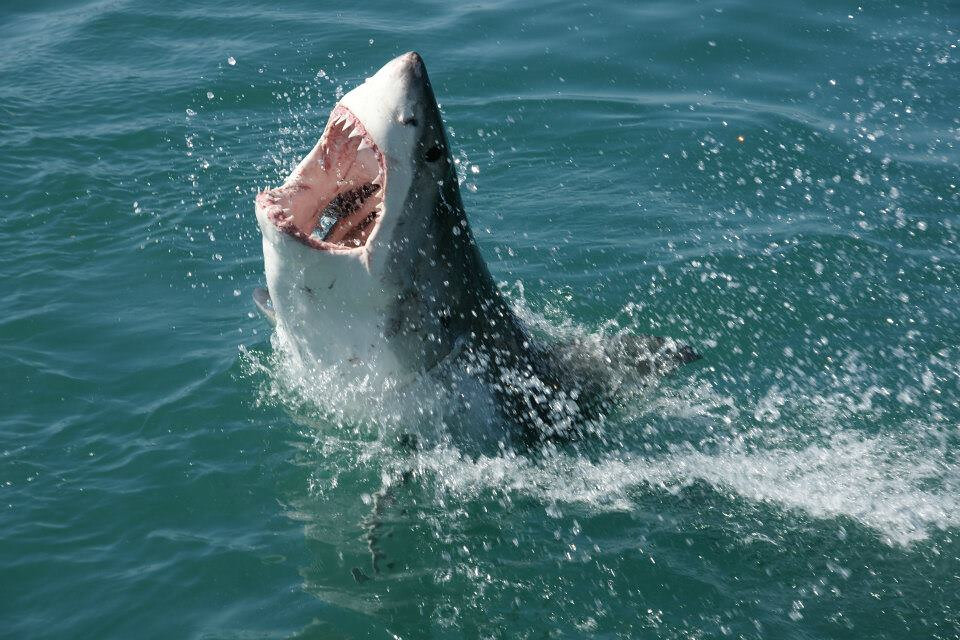
Ok, so it's quite obviously not true that ice cream causes shark attacks. But if you looked at these two variables and how they're related, you'd notice that during times of the year with high ice cream sales, there are also the most shark attacks. Despite the fact that the conclusion we drew about the relationship was wrong, it's nonetheless true that these two variables appear related, and researchers figured that out through the use of bivariate analysis. (You will learn about correlation versus causation in Chapter 8 .)
Bivariate analysis consists of a group of statistical techniques that examine the association between two variables. We could look at how anti-depressant medications and appetite are related, whether there is a relation between having a pet and emotional well-being, or if a policy-maker's level of education is related to how they vote on bills related to environmental issues.
Bivariate analysis forms the foundation of multivariate analysis, which we don't get to in this book. All you really need to know here is that there are steps beyond bivariate analysis, which you've undoubtedly seen in scholarly literature already! But before we can move forward with multivariate analysis, we need to understand the associations between the variables in our study .
[MADE THIS UP] Throughout your PhD program, you will learn more about quantitative data analysis techniques. Hopefully this section has provided you with some initial insights into how data is analyzed, and the importance of creating a data analysis plan prior to collecting data. Next, we will discuss some basic strategies for creating a qualitative data analysis plan.
If you don't see the general aim of your research question reflected in one of these areas, don't fret! This is only a small sampling of what you might be trying to accomplish with your qualitative study. Whatever your aim, you need to have a plan for what you will do once you have collected your data.
Iterative or linear
Some qualitative research is linear , meaning it follows more of a tra ditionally quantitative process: create a plan, gather data, and analyze data; each step is completed before we proceed to the next. You can think of this like how information is presented in this book. We discuss each topic, one after another.
However, many times qualitative research is iterative , or evolving in cycles. An iterative approach means that once we begin collecting data, we also begin analyzing data as it is coming in. This early and ongoing analysis of our (incomplete) data then impacts our continued planning, data gathering and future analysis. Again, coming back to this book, while it may be written linear, we hope that you engage with it iteratively as you design and conduct your own research. By this we mean that you will revisit previous sections so you can understand how they fit together and you are in continuous process of building and revising how you think about the concepts you are learning about.
As you may have guessed, there are benefits and challenges to both linear and iterative approaches. A linear approach is much more straightforward, each step being fairly defined. However, linear research being more defined and rigid also presents certain challenges. A linear approach assumes that we know what we need to ask or look for at the very beginning of data collection, which often is not the case.
With iterative research, we have more flexibility to adapt our approach as we learn new things. We still need to keep our approach systematic and organized, however, so that our work doesn't become a free-for-all. As we adapt, we do not want to stray too far from the original premise of our study. It's also important to remember with an iterative approach that we may risk ethical concerns if our work extends beyond the original boundaries of our informed consent and institutional review board agreement (IRB; see Chapter 6 for more on IRBs). If you feel that you do need to modify your original research plan in a significant way as you learn more about the topic, you can submit an addendum to modify your original application that was submitted. Make sure to keep detailed notes of the decisions that you are making and what is informing these choices. This helps to support transparency and your credibility throughout the research process.
As y ou begin your analysis, y ou need to get to know your data. This often means reading through your data prior to any attempt at breaking it apart and labeling it. You mig ht read through a couple of times, in fact. This helps give you a more comprehensive feel for each piece of data and the data as a whole, again, before you start to break it down into smaller units or deconstruct it. This is especially important if others assisted us in the data collection process. We often gather data as part of team and everyone involved in the analysis needs to be very familiar with all of the data.
During your reviewing you will start to develop and evolve your understanding of what the data means. Coding is a part of the qualitative data analysis process where we begin to interpret and assign meaning to the data. It represents one of the first steps as we begin to filter the data through our own subjective lens as the researcher. This understanding of the data should be dynamic and flexible, but you want to have a way to capture this understanding as it evolves. You may include this as part of your qualitative codebook where you are tracking the main ideas that are emerging and what they mean. Figure 2.2 is an example of how your thinking might change about a code and how you can go about capturing it.
There are a variety of different approaches to qualitative analysis, including thematic analysis, content analysis, grounded theory, phenomenology, photovoice, and more. The specific steps you will take to code your qualitative data, and to generate themes from these codes, will vary based on the analytic strategy you are employing. In designing your qualitative study, you would identify an analytical approach as you plan out your project. The one you select would depend on the type of data you have and what you want to accomplish with it.
- Getting organized at the beginning of your project with a data analysis plan will help keep you on track. Data analysis plans should include your research question, a description of your data, and a step-by-step outline of what you're going to do with it. [chapter 14.1]
Exercises [from chapter 14.1]
- Make a data analysis plan for your project. Remember this should include your research question, a description of the data you will use, and a step-by-step outline of what you're going to do with your data once you have it, including statistical tests (non-relational and relational) that you plan to use. You can do this exercise whether you're using quantitative or qualitative data! The same principles apply.
- Make a draft quantitative data analysis plan for your project. Remember this should include your research question, a description of the data you will use, and a step-by-step outline of what you're going to do with your data once you have it, including statistical tests (non-relational and relational) that you plan to use. It's okay if you don't yet have a complete idea of the types of statistical analyses you might use.
2.4 Critical considerations
- Critique the traditional role of researchers and identify how action research addresses these issues
So far in this chapter, we have presented the steps of research projects as follows:
- Find a topic that is important to you and read about it.
- Pose a question that is important to the literature and to your community.
- Propose to use specific research methods and data analysis techniques to answer your question.
- Carry out your project and report the results.
These were depicted in more detail in Table 2.1 earlier in this chapter. There are important limitations to this approach. This section examines those problems and how to address them.
Whose knowledge is privileged?
First, let's critically examine your role as the researcher. Following along with the steps in a research project, you start studying the literature on your topic, find a place where you can add to scientific knowledge, and conduct your study. But why are you the person who gets to decide what is important? Just as clients are the experts on their lives, members of your target population are the experts on their lives. What does it mean for a group of people to be researched on, rather than researched with? How can we better respect the knowledge and self-determination of community members?
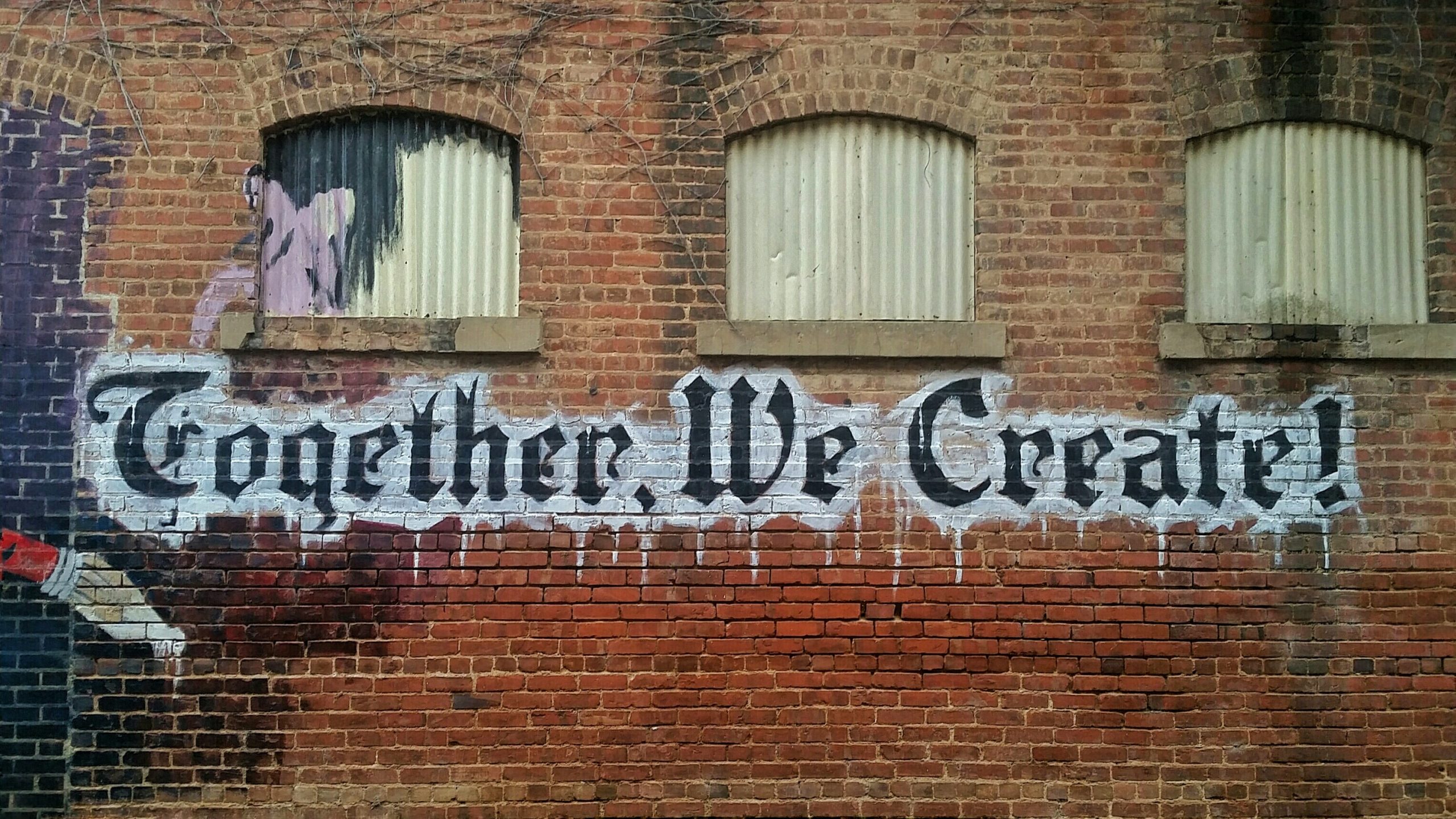
A different way of approaching your research project is to start by talking with members of the target population and those who are knowledgeable about that community. Perhaps there is a community-led organization you can partner with on a research project. The researcher's role in this case would be more similar to a consultant, someone with specialized knowledge about research who can help communities study problems they consider to be important. The social worker is a co-investigator, and community members are equal partners in the research project. Each has a type of knowledge—scientific expertise vs. lived experience—that should inform the research process.
The community focus highlights something important: they are localized. These projects can dedicate themselves to issues at a single agency or within a service area. With a local scope, researchers can bring about change in their community. This is the purpose behind action research.
Action research
Action research is research that is conducted for the purpose of creating social change. When engaging in action research, scholars collaborate with community stakeholders to conduct research that will be relevant to the community. Social workers who engage in action research don't just go it alone; instead, they collaborate with the people who are affected by the research at each stage in the process. Stakeholders, particularly those with the least power, should be consulted on the purpose of the research project, research questions, design, and reporting of results.
Action research also distinguishes itself from other research in that its purpose is to create change on an individual and community level. Kristin Esterberg puts it quite eloquently when she says, “At heart, all action researchers are concerned that research not simply contribute to knowledge but also lead to positive changes in people’s lives” (2002, p. 137). [2] Action research has multiple origins across the globe, including Kurt Lewin’s psychological experiments in the US and Paulo Friere’s literacy and education programs (Adelman, 1993; Reason, 1994). [3] Over the years, action research has become increasingly popular among scholars who wish for their work to have tangible outcomes that benefit the groups they study.
A traditional scientist might look at the literature or use their practice wisdom to formulate a question for quantitative or qualitative research, as we suggested earlier in this chapter. An action researcher, on the other hand, would consult with people in the target population and community to see what they believe the most pressing issues are and what their proposed solutions may be. In this way, action research flips traditional research on its head. Scientists are not the experts on the research topic. Instead, they are more like consultants who provide the tools and resources necessary for a target population to achieve their goals and to address social problems using social science research.
According to Healy (2001), [4] the assumptions of participatory-action research are that (a) oppression is caused by macro-level structures such as patriarchy and capitalism; (b) research should expose and confront the powerful; (c) researcher and participant relationships should be equal, with equitable distribution of research tasks and roles; and (d) research should result in consciousness-raising and collective action. Consistent with social work values, action research supports the self-determination of oppressed groups and privileges their voice and understanding through the conceptualization, design, data collection, data analysis, and dissemination processes of research. We will return to similar ideas in Part 4 of the textbook when we discuss qualitative research methods, though action research can certainly be used with quantitative research methods, as well.
- Traditionally, researchers did not consult target populations and communities prior to formulating a research question. Action research proposes a more community-engaged model in which researchers are consultants that help communities research topics of import to them.
Post- awareness check (Knowledge)
Based on what you know of your target population, what are a few ways to receive their “buy-in” to participate in your proposed research study?
- Apply the key concepts of action research to your project. How might you incorporate the perspectives and expertise of community members in your project?
The level that describes how data for variables are recorded. The level of measurement defines the type of operations can be conducted with your data. There are four levels: nominal, ordinal, interval, and ratio.
Referring to data analysis that doesn't examine how variables relate to each other.
a group of statistical techniques that examines the relationship between two variables
A research process where you create a plan, you gather your data, you analyze your data and each step is completed before you proceed to the next.
An iterative approach means that after planning and once we begin collecting data, we begin analyzing as data as it is coming in. This early analysis of our (incomplete) data, then impacts our planning, ongoing data gathering and future analysis as it progresses.
Part of the qualitative data analysis process where we begin to interpret and assign meaning to the data.
A document that we use to keep track of and define the codes that we have identified (or are using) in our qualitative data analysis.
Doctoral Research Methods in Social Work Copyright © by Mavs Open Press. All Rights Reserved.
Share This Book
- Skip to main content
- Skip to primary sidebar
- Skip to footer
- QuestionPro

- Solutions Industries Gaming Automotive Sports and events Education Government Travel & Hospitality Financial Services Healthcare Cannabis Technology Use Case NPS+ Communities Audience Contactless surveys Mobile LivePolls Member Experience GDPR Positive People Science 360 Feedback Surveys
- Resources Blog eBooks Survey Templates Case Studies Training Help center

Home Market Research
Data Analysis in Research: Types & Methods
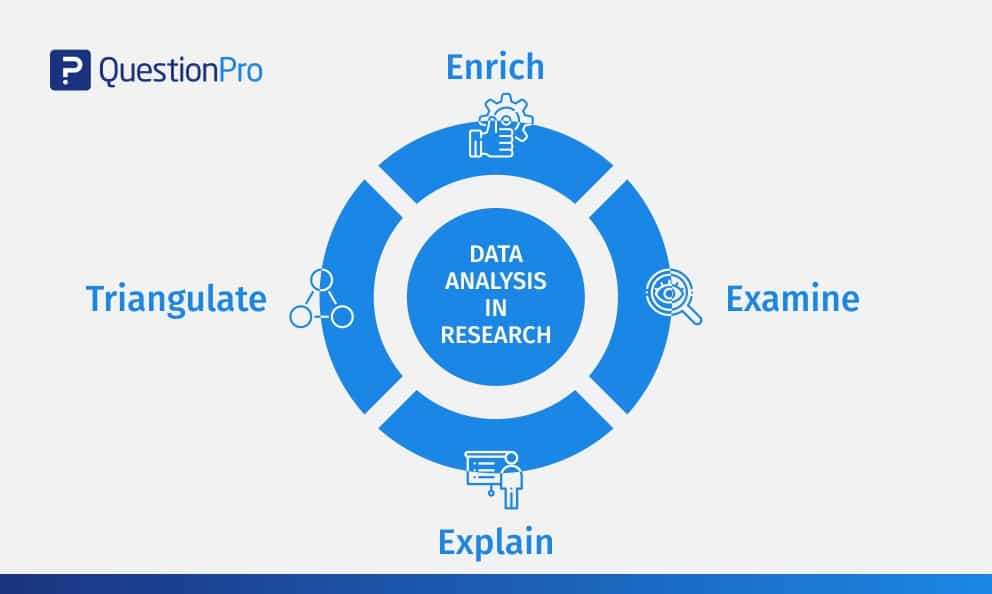
Content Index
Why analyze data in research?
Types of data in research, finding patterns in the qualitative data, methods used for data analysis in qualitative research, preparing data for analysis, methods used for data analysis in quantitative research, considerations in research data analysis, what is data analysis in research.
Definition of research in data analysis: According to LeCompte and Schensul, research data analysis is a process used by researchers to reduce data to a story and interpret it to derive insights. The data analysis process helps reduce a large chunk of data into smaller fragments, which makes sense.
Three essential things occur during the data analysis process — the first is data organization . Summarization and categorization together contribute to becoming the second known method used for data reduction. It helps find patterns and themes in the data for easy identification and linking. The third and last way is data analysis – researchers do it in both top-down and bottom-up fashion.
LEARN ABOUT: Research Process Steps
On the other hand, Marshall and Rossman describe data analysis as a messy, ambiguous, and time-consuming but creative and fascinating process through which a mass of collected data is brought to order, structure and meaning.
We can say that “the data analysis and data interpretation is a process representing the application of deductive and inductive logic to the research and data analysis.”
Researchers rely heavily on data as they have a story to tell or research problems to solve. It starts with a question, and data is nothing but an answer to that question. But, what if there is no question to ask? Well! It is possible to explore data even without a problem – we call it ‘Data Mining’, which often reveals some interesting patterns within the data that are worth exploring.
Irrelevant to the type of data researchers explore, their mission and audiences’ vision guide them to find the patterns to shape the story they want to tell. One of the essential things expected from researchers while analyzing data is to stay open and remain unbiased toward unexpected patterns, expressions, and results. Remember, sometimes, data analysis tells the most unforeseen yet exciting stories that were not expected when initiating data analysis. Therefore, rely on the data you have at hand and enjoy the journey of exploratory research.
Create a Free Account
Every kind of data has a rare quality of describing things after assigning a specific value to it. For analysis, you need to organize these values, processed and presented in a given context, to make it useful. Data can be in different forms; here are the primary data types.
- Qualitative data: When the data presented has words and descriptions, then we call it qualitative data . Although you can observe this data, it is subjective and harder to analyze data in research, especially for comparison. Example: Quality data represents everything describing taste, experience, texture, or an opinion that is considered quality data. This type of data is usually collected through focus groups, personal qualitative interviews , qualitative observation or using open-ended questions in surveys.
- Quantitative data: Any data expressed in numbers of numerical figures are called quantitative data . This type of data can be distinguished into categories, grouped, measured, calculated, or ranked. Example: questions such as age, rank, cost, length, weight, scores, etc. everything comes under this type of data. You can present such data in graphical format, charts, or apply statistical analysis methods to this data. The (Outcomes Measurement Systems) OMS questionnaires in surveys are a significant source of collecting numeric data.
- Categorical data: It is data presented in groups. However, an item included in the categorical data cannot belong to more than one group. Example: A person responding to a survey by telling his living style, marital status, smoking habit, or drinking habit comes under the categorical data. A chi-square test is a standard method used to analyze this data.
Learn More : Examples of Qualitative Data in Education
Data analysis in qualitative research
Data analysis and qualitative data research work a little differently from the numerical data as the quality data is made up of words, descriptions, images, objects, and sometimes symbols. Getting insight from such complicated information is a complicated process. Hence it is typically used for exploratory research and data analysis .
Although there are several ways to find patterns in the textual information, a word-based method is the most relied and widely used global technique for research and data analysis. Notably, the data analysis process in qualitative research is manual. Here the researchers usually read the available data and find repetitive or commonly used words.
For example, while studying data collected from African countries to understand the most pressing issues people face, researchers might find “food” and “hunger” are the most commonly used words and will highlight them for further analysis.
LEARN ABOUT: Level of Analysis
The keyword context is another widely used word-based technique. In this method, the researcher tries to understand the concept by analyzing the context in which the participants use a particular keyword.
For example , researchers conducting research and data analysis for studying the concept of ‘diabetes’ amongst respondents might analyze the context of when and how the respondent has used or referred to the word ‘diabetes.’
The scrutiny-based technique is also one of the highly recommended text analysis methods used to identify a quality data pattern. Compare and contrast is the widely used method under this technique to differentiate how a specific text is similar or different from each other.
For example: To find out the “importance of resident doctor in a company,” the collected data is divided into people who think it is necessary to hire a resident doctor and those who think it is unnecessary. Compare and contrast is the best method that can be used to analyze the polls having single-answer questions types .
Metaphors can be used to reduce the data pile and find patterns in it so that it becomes easier to connect data with theory.
Variable Partitioning is another technique used to split variables so that researchers can find more coherent descriptions and explanations from the enormous data.
LEARN ABOUT: Qualitative Research Questions and Questionnaires
There are several techniques to analyze the data in qualitative research, but here are some commonly used methods,
- Content Analysis: It is widely accepted and the most frequently employed technique for data analysis in research methodology. It can be used to analyze the documented information from text, images, and sometimes from the physical items. It depends on the research questions to predict when and where to use this method.
- Narrative Analysis: This method is used to analyze content gathered from various sources such as personal interviews, field observation, and surveys . The majority of times, stories, or opinions shared by people are focused on finding answers to the research questions.
- Discourse Analysis: Similar to narrative analysis, discourse analysis is used to analyze the interactions with people. Nevertheless, this particular method considers the social context under which or within which the communication between the researcher and respondent takes place. In addition to that, discourse analysis also focuses on the lifestyle and day-to-day environment while deriving any conclusion.
- Grounded Theory: When you want to explain why a particular phenomenon happened, then using grounded theory for analyzing quality data is the best resort. Grounded theory is applied to study data about the host of similar cases occurring in different settings. When researchers are using this method, they might alter explanations or produce new ones until they arrive at some conclusion.
LEARN ABOUT: 12 Best Tools for Researchers
Data analysis in quantitative research
The first stage in research and data analysis is to make it for the analysis so that the nominal data can be converted into something meaningful. Data preparation consists of the below phases.
Phase I: Data Validation
Data validation is done to understand if the collected data sample is per the pre-set standards, or it is a biased data sample again divided into four different stages
- Fraud: To ensure an actual human being records each response to the survey or the questionnaire
- Screening: To make sure each participant or respondent is selected or chosen in compliance with the research criteria
- Procedure: To ensure ethical standards were maintained while collecting the data sample
- Completeness: To ensure that the respondent has answered all the questions in an online survey. Else, the interviewer had asked all the questions devised in the questionnaire.
Phase II: Data Editing
More often, an extensive research data sample comes loaded with errors. Respondents sometimes fill in some fields incorrectly or sometimes skip them accidentally. Data editing is a process wherein the researchers have to confirm that the provided data is free of such errors. They need to conduct necessary checks and outlier checks to edit the raw edit and make it ready for analysis.
Phase III: Data Coding
Out of all three, this is the most critical phase of data preparation associated with grouping and assigning values to the survey responses . If a survey is completed with a 1000 sample size, the researcher will create an age bracket to distinguish the respondents based on their age. Thus, it becomes easier to analyze small data buckets rather than deal with the massive data pile.
LEARN ABOUT: Steps in Qualitative Research
After the data is prepared for analysis, researchers are open to using different research and data analysis methods to derive meaningful insights. For sure, statistical analysis plans are the most favored to analyze numerical data. In statistical analysis, distinguishing between categorical data and numerical data is essential, as categorical data involves distinct categories or labels, while numerical data consists of measurable quantities. The method is again classified into two groups. First, ‘Descriptive Statistics’ used to describe data. Second, ‘Inferential statistics’ that helps in comparing the data .
Descriptive statistics
This method is used to describe the basic features of versatile types of data in research. It presents the data in such a meaningful way that pattern in the data starts making sense. Nevertheless, the descriptive analysis does not go beyond making conclusions. The conclusions are again based on the hypothesis researchers have formulated so far. Here are a few major types of descriptive analysis methods.
Measures of Frequency
- Count, Percent, Frequency
- It is used to denote home often a particular event occurs.
- Researchers use it when they want to showcase how often a response is given.
Measures of Central Tendency
- Mean, Median, Mode
- The method is widely used to demonstrate distribution by various points.
- Researchers use this method when they want to showcase the most commonly or averagely indicated response.
Measures of Dispersion or Variation
- Range, Variance, Standard deviation
- Here the field equals high/low points.
- Variance standard deviation = difference between the observed score and mean
- It is used to identify the spread of scores by stating intervals.
- Researchers use this method to showcase data spread out. It helps them identify the depth until which the data is spread out that it directly affects the mean.
Measures of Position
- Percentile ranks, Quartile ranks
- It relies on standardized scores helping researchers to identify the relationship between different scores.
- It is often used when researchers want to compare scores with the average count.
For quantitative research use of descriptive analysis often give absolute numbers, but the in-depth analysis is never sufficient to demonstrate the rationale behind those numbers. Nevertheless, it is necessary to think of the best method for research and data analysis suiting your survey questionnaire and what story researchers want to tell. For example, the mean is the best way to demonstrate the students’ average scores in schools. It is better to rely on the descriptive statistics when the researchers intend to keep the research or outcome limited to the provided sample without generalizing it. For example, when you want to compare average voting done in two different cities, differential statistics are enough.
Descriptive analysis is also called a ‘univariate analysis’ since it is commonly used to analyze a single variable.
Inferential statistics
Inferential statistics are used to make predictions about a larger population after research and data analysis of the representing population’s collected sample. For example, you can ask some odd 100 audiences at a movie theater if they like the movie they are watching. Researchers then use inferential statistics on the collected sample to reason that about 80-90% of people like the movie.
Here are two significant areas of inferential statistics.
- Estimating parameters: It takes statistics from the sample research data and demonstrates something about the population parameter.
- Hypothesis test: I t’s about sampling research data to answer the survey research questions. For example, researchers might be interested to understand if the new shade of lipstick recently launched is good or not, or if the multivitamin capsules help children to perform better at games.
These are sophisticated analysis methods used to showcase the relationship between different variables instead of describing a single variable. It is often used when researchers want something beyond absolute numbers to understand the relationship between variables.
Here are some of the commonly used methods for data analysis in research.
- Correlation: When researchers are not conducting experimental research or quasi-experimental research wherein the researchers are interested to understand the relationship between two or more variables, they opt for correlational research methods.
- Cross-tabulation: Also called contingency tables, cross-tabulation is used to analyze the relationship between multiple variables. Suppose provided data has age and gender categories presented in rows and columns. A two-dimensional cross-tabulation helps for seamless data analysis and research by showing the number of males and females in each age category.
- Regression analysis: For understanding the strong relationship between two variables, researchers do not look beyond the primary and commonly used regression analysis method, which is also a type of predictive analysis used. In this method, you have an essential factor called the dependent variable. You also have multiple independent variables in regression analysis. You undertake efforts to find out the impact of independent variables on the dependent variable. The values of both independent and dependent variables are assumed as being ascertained in an error-free random manner.
- Frequency tables: The statistical procedure is used for testing the degree to which two or more vary or differ in an experiment. A considerable degree of variation means research findings were significant. In many contexts, ANOVA testing and variance analysis are similar.
- Analysis of variance: The statistical procedure is used for testing the degree to which two or more vary or differ in an experiment. A considerable degree of variation means research findings were significant. In many contexts, ANOVA testing and variance analysis are similar.
- Researchers must have the necessary research skills to analyze and manipulation the data , Getting trained to demonstrate a high standard of research practice. Ideally, researchers must possess more than a basic understanding of the rationale of selecting one statistical method over the other to obtain better data insights.
- Usually, research and data analytics projects differ by scientific discipline; therefore, getting statistical advice at the beginning of analysis helps design a survey questionnaire, select data collection methods , and choose samples.
LEARN ABOUT: Best Data Collection Tools
- The primary aim of data research and analysis is to derive ultimate insights that are unbiased. Any mistake in or keeping a biased mind to collect data, selecting an analysis method, or choosing audience sample il to draw a biased inference.
- Irrelevant to the sophistication used in research data and analysis is enough to rectify the poorly defined objective outcome measurements. It does not matter if the design is at fault or intentions are not clear, but lack of clarity might mislead readers, so avoid the practice.
- The motive behind data analysis in research is to present accurate and reliable data. As far as possible, avoid statistical errors, and find a way to deal with everyday challenges like outliers, missing data, data altering, data mining , or developing graphical representation.
LEARN MORE: Descriptive Research vs Correlational Research The sheer amount of data generated daily is frightening. Especially when data analysis has taken center stage. in 2018. In last year, the total data supply amounted to 2.8 trillion gigabytes. Hence, it is clear that the enterprises willing to survive in the hypercompetitive world must possess an excellent capability to analyze complex research data, derive actionable insights, and adapt to the new market needs.
LEARN ABOUT: Average Order Value
QuestionPro is an online survey platform that empowers organizations in data analysis and research and provides them a medium to collect data by creating appealing surveys.
MORE LIKE THIS
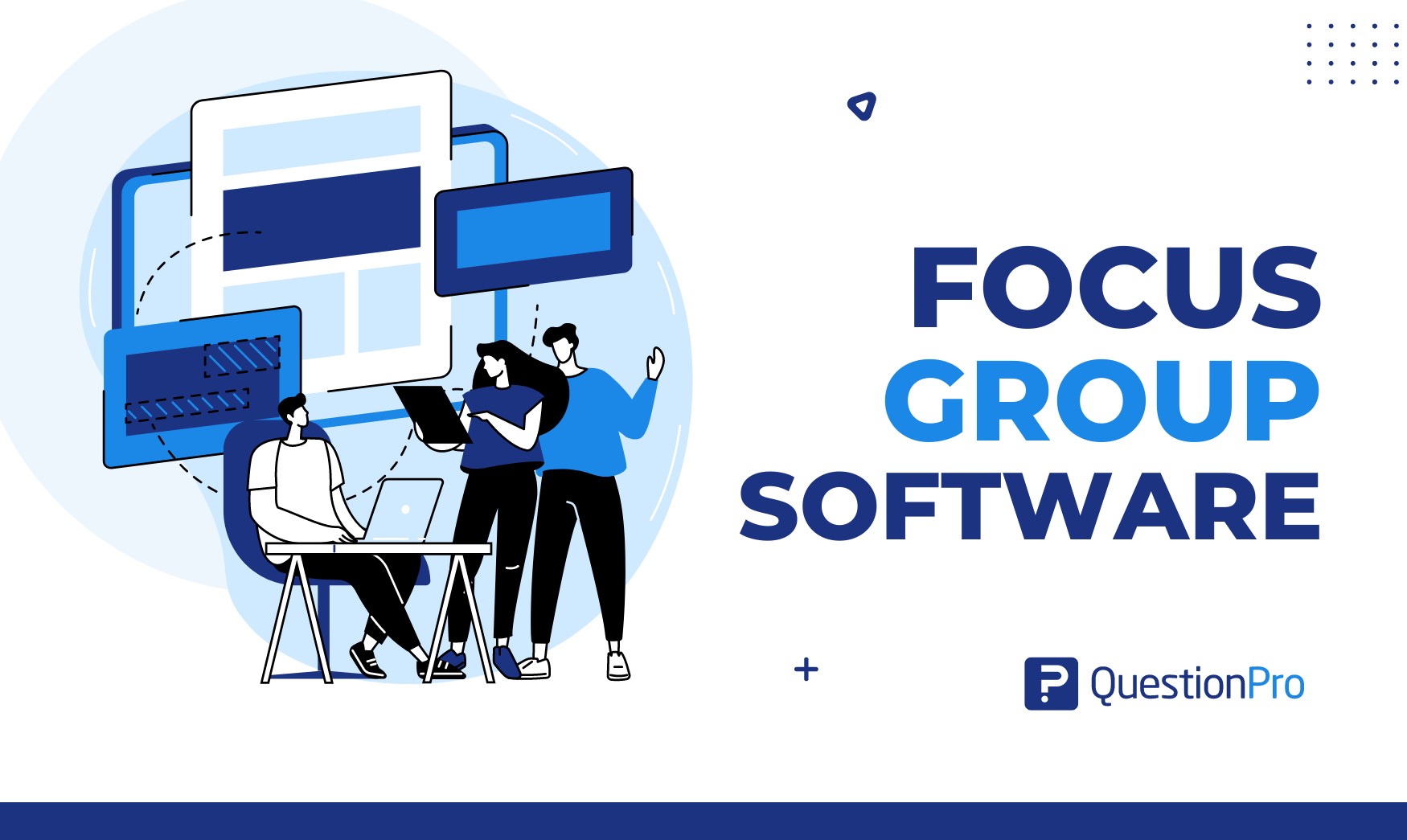
Top 7 Focus Group Software for Comprehensive Research
Apr 17, 2024
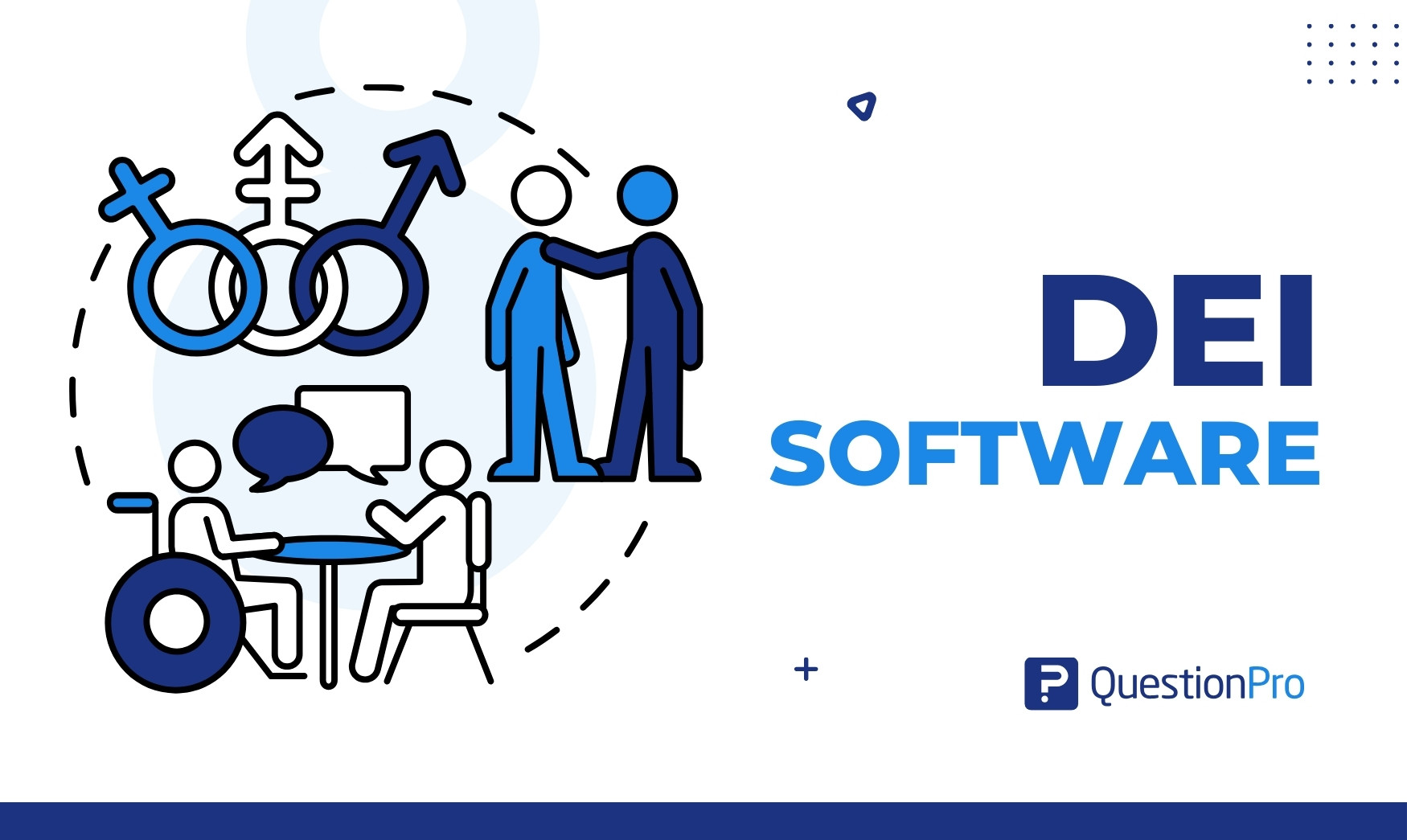
Top 7 DEI Software Solutions to Empower Your Workplace
Apr 16, 2024
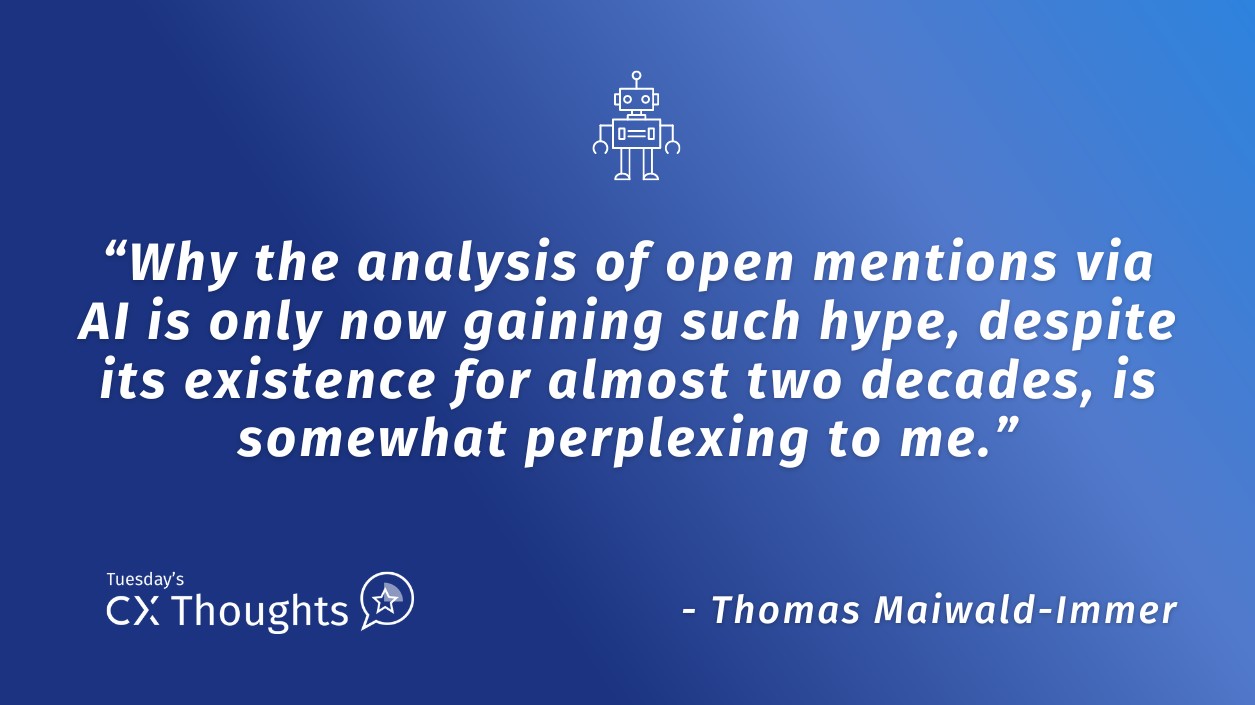
The Power of AI in Customer Experience — Tuesday CX Thoughts
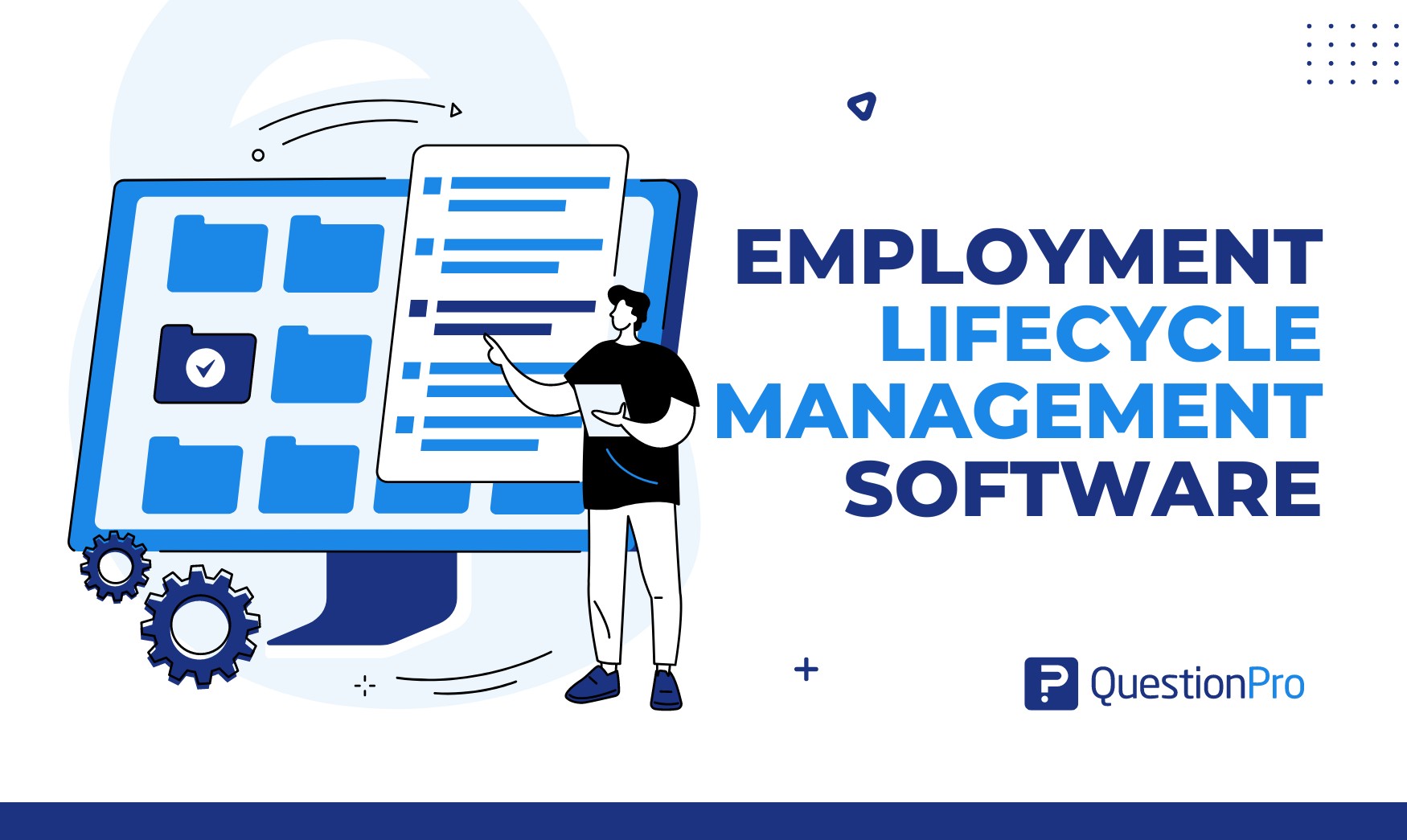
Employee Lifecycle Management Software: Top of 2024
Apr 15, 2024
Other categories
- Academic Research
- Artificial Intelligence
- Assessments
- Brand Awareness
- Case Studies
- Communities
- Consumer Insights
- Customer effort score
- Customer Engagement
- Customer Experience
- Customer Loyalty
- Customer Research
- Customer Satisfaction
- Employee Benefits
- Employee Engagement
- Employee Retention
- Friday Five
- General Data Protection Regulation
- Insights Hub
- Life@QuestionPro
- Market Research
- Mobile diaries
- Mobile Surveys
- New Features
- Online Communities
- Question Types
- Questionnaire
- QuestionPro Products
- Release Notes
- Research Tools and Apps
- Revenue at Risk
- Survey Templates
- Training Tips
- Uncategorized
- Video Learning Series
- What’s Coming Up
- Workforce Intelligence
- 1-icon/ui/arrow_right Amsterdam Public Health
- 1-icon/ui/arrow_right Home
- 1-icon/ui/arrow_right Research Lifecycle
More APH...
- 1-icon/ui/arrow_right About
- 1-icon/ui/arrow_right News
- 1-icon/ui/arrow_right Events
- 1-icon/ui/arrow_right Research information
- 1-icon/ui/arrow_right Our strenghts
- Amsterdam Public Health
- Research Lifecycle
- Research information
- Our strenghts
- Proposal Writing
- Study Preparation
- Methods & Data Collection
- Process & Analyze Data
- Writing & Publication
- Archiving & Open Data
- Knowledge Utilization
- Supervision
- Analysis plan
- Set-up & Conduct
- Quantitative research
Data analysis
- Initial data analysis
- Post-hoc & sensitivity analyses
- Data analysis documentation
- Handling missing data
To promote structured targeted data analysis.
Requirements
An analysis plan should be created and finalized prior to the data analyses.
Documentation
The analysis plan (Guidelines per study type are provided below)
Responsibilities
- Executing researcher: To create the analysis plan prior to the data analyses, containing a description of the research question and what the various steps in the analysis are going to be. This should also be signed and dated by the PI.
- Project leaders: To inform the executing researcher about setting up the analysis plan before analyses are undertaken.
- Research assistant: N.a.
An analysis plan should be created and finalized (signed and dated by PI) prior to the data analyses. The analysis plan contains a description of the research question and what the various steps in the analysis are going to be. It also contains an exploration of literature (what is already know? What will this study add?) to make sure your research question is relevant (see Glasziou et al. Lancet 2014 on avoiding research waste).The analysis plan is intended as a starting point for the analysis. It ensures that the analysis can be undertaken in a targeted manner, and promotes research integrity.
If you will perform an exploratory study you can adjust your analysis based on the data you find; this may be useful if not much is known about the research subject, but it is considered as relatively low level evidence and it should be clearly mentioned in your report that the presented study is exploratory. If you want to perform an hypothesis-testing study (be it interventional or using observational data) you need to pre-specify the analyses you intend to do prior to performing the analysis, including the population, subgroups, stratifications and statistical tests. If deviations from the analysis plan are made during the study this should be documented in the analysis plan and stated in the report (i.e. post-hoc tests). If you intend to do hypothesis-free research with multiple testing you should pre-specify your threshold for statistical significance according to the number of analyses you will perform. Lastly, if you intend to perform an RCT, the analysis plan is practically set in stone. (Also see ICH E9 - statistical principles for clinical trials )
If needed, an exploratory analysis may be part of the analysis plan, to inform the setting up of the final analysis (see initial data analysis ). For instance, you may want to know distributions of values in order to create meaningful categories, or determine whether data are normally distributed. The findings and decisions made during these preliminary exploratory analyses should be clearly documented, preferably in a version two of the analysis plan, and made reproducible by providing the data analysis syntax (in SPSS, SAS, STATA, R) (see guideline Documentation of data analysis ).
The concrete research question needs to be formulated firstly within the analysis plan following the literature review; this is the question intended to be answered by the analyses. Concrete research questions may be defined using the acronym PICO: Population, Intervention, Comparison, Outcomes. An example of a concrete question could be: “Does frequent bending at work lead to an elevated risk of lower back pain occurring in employees?” (Population = Employees; Intervention = Frequent bending; Comparison = Infrequent bending; Outcome = Occurrence of back pain). Concrete research questions are essential for determining the analyses required.
The analysis plan should then describe the primary and secondary outcomes, the determinants and data needed, and which statistical techniques are to be used to analyse the data. The following issues need to be considered in this process and described where applicable:
- In case of a trial: is the trial a superiority, non-inferiority or equivalence trial.
- Superiority: treatment A is better than the control.
- Non-inferiority: treatment A is not worse than treatment B.
- Equivalence: testing similarity using a tolerance range.
In other studies: what is the study design (case control, longitudinal cohort etc).
- Which (subgroup of the) population is to be included in the analyses? Which groups will you compare?;
- What are the primary and secondary endpoints? Which data from which endpoint (T1, T2, etc.) will be used?;
- Which (dependent and independent) variables are to be used in the analyses and how are the variables to be analysed (e.g. continuous or in categories)?;
- Which variables are to be investigated as potential confounders or effect modifiers (and why) and how are these variables to be analysed? There are different ways of dealing with confounders. We distinguish the following: 1) correct for all potential confounders (and do not concern about the question whether or not a variable is a ‘real’ confounder). Mostly, confounders are split up in little groups (demographic factors, clinical parameters, etc.). As a result you get corrected model 1, corrected model 2, etc. However, pay attention to collinearity and overcorrection if confounders coincide too much with primary determinants. 2) if the sample size is not big enough relative to the number of potential confounders, you may consider to only correct for those confounders that are relevant for the association between determinant and outcome. To select the relevant confounders, mostly a forward selection procedure is performed. In this case the confounders are added to the model one by one (the confounder that is associated strongest first). Subsequently, consider to what extent the effect of the variable of interest is changed. Then first choose the strongest confounder in the model. Subsequently, repeat this procedure untill no confounder has a relevant effect (<10% change in regression coefficient). Alternatively, you can select the confounders that univariately change the point estimate of the association with >10%. 3) Another option is to set up a Directed Acyclic Graph (DAG), to determine which confounders should be added to the model. Please see http://www.dagitty.net/ for more information.
- How to deal with missing values? (see chapter on handeling missing data );
- Which analyses are to be carried out in which order (e.g. univariable analyses, multivariable analyses, analysis of confounders, analysis of interaction effects, analysis of sub-populations, etc.)?; Which sensitivity analyses will be performed?
- Do the data meet the criteria for the specific statistical technique?
A statistician may need to be consulted regarding the choice of statistical techniques (also see this intanetpage on statistical analysis plan ).
It is recommended to already design the empty tables to be included in the article prior to the start of data analysis. This is often very helpful in deciding which analyses are exactly required in order to analyse the data in a targeted manner.
You may consider to make your study protocol including the (statistical) analysis plan public, either by placing in on a publicly accessible website (Concept Paper/Design paper) or by uploading it in an appropriate studies register (for human trials: NTR / EUDRACT / ClinicalTrials.gov , for non-/preclinicaltrials: preclinicaltrials.eu ).
Check the reporting guidelines when writing an analysis plan . These will help increase the quality of your research and guide you.

An official website of the United States government
The .gov means it’s official. Federal government websites often end in .gov or .mil. Before sharing sensitive information, make sure you’re on a federal government site.
The site is secure. The https:// ensures that you are connecting to the official website and that any information you provide is encrypted and transmitted securely.
- Publications
- Account settings
Preview improvements coming to the PMC website in October 2024. Learn More or Try it out now .
- Advanced Search
- Journal List
- Indian J Anaesth
- v.60(9); 2016 Sep
How to write a research proposal?
Department of Anaesthesiology, Bangalore Medical College and Research Institute, Bengaluru, Karnataka, India
Devika Rani Duggappa
Writing the proposal of a research work in the present era is a challenging task due to the constantly evolving trends in the qualitative research design and the need to incorporate medical advances into the methodology. The proposal is a detailed plan or ‘blueprint’ for the intended study, and once it is completed, the research project should flow smoothly. Even today, many of the proposals at post-graduate evaluation committees and application proposals for funding are substandard. A search was conducted with keywords such as research proposal, writing proposal and qualitative using search engines, namely, PubMed and Google Scholar, and an attempt has been made to provide broad guidelines for writing a scientifically appropriate research proposal.
INTRODUCTION
A clean, well-thought-out proposal forms the backbone for the research itself and hence becomes the most important step in the process of conduct of research.[ 1 ] The objective of preparing a research proposal would be to obtain approvals from various committees including ethics committee [details under ‘Research methodology II’ section [ Table 1 ] in this issue of IJA) and to request for grants. However, there are very few universally accepted guidelines for preparation of a good quality research proposal. A search was performed with keywords such as research proposal, funding, qualitative and writing proposals using search engines, namely, PubMed, Google Scholar and Scopus.
Five ‘C’s while writing a literature review

BASIC REQUIREMENTS OF A RESEARCH PROPOSAL
A proposal needs to show how your work fits into what is already known about the topic and what new paradigm will it add to the literature, while specifying the question that the research will answer, establishing its significance, and the implications of the answer.[ 2 ] The proposal must be capable of convincing the evaluation committee about the credibility, achievability, practicality and reproducibility (repeatability) of the research design.[ 3 ] Four categories of audience with different expectations may be present in the evaluation committees, namely academic colleagues, policy-makers, practitioners and lay audiences who evaluate the research proposal. Tips for preparation of a good research proposal include; ‘be practical, be persuasive, make broader links, aim for crystal clarity and plan before you write’. A researcher must be balanced, with a realistic understanding of what can be achieved. Being persuasive implies that researcher must be able to convince other researchers, research funding agencies, educational institutions and supervisors that the research is worth getting approval. The aim of the researcher should be clearly stated in simple language that describes the research in a way that non-specialists can comprehend, without use of jargons. The proposal must not only demonstrate that it is based on an intelligent understanding of the existing literature but also show that the writer has thought about the time needed to conduct each stage of the research.[ 4 , 5 ]
CONTENTS OF A RESEARCH PROPOSAL
The contents or formats of a research proposal vary depending on the requirements of evaluation committee and are generally provided by the evaluation committee or the institution.
In general, a cover page should contain the (i) title of the proposal, (ii) name and affiliation of the researcher (principal investigator) and co-investigators, (iii) institutional affiliation (degree of the investigator and the name of institution where the study will be performed), details of contact such as phone numbers, E-mail id's and lines for signatures of investigators.
The main contents of the proposal may be presented under the following headings: (i) introduction, (ii) review of literature, (iii) aims and objectives, (iv) research design and methods, (v) ethical considerations, (vi) budget, (vii) appendices and (viii) citations.[ 4 ]
Introduction
It is also sometimes termed as ‘need for study’ or ‘abstract’. Introduction is an initial pitch of an idea; it sets the scene and puts the research in context.[ 6 ] The introduction should be designed to create interest in the reader about the topic and proposal. It should convey to the reader, what you want to do, what necessitates the study and your passion for the topic.[ 7 ] Some questions that can be used to assess the significance of the study are: (i) Who has an interest in the domain of inquiry? (ii) What do we already know about the topic? (iii) What has not been answered adequately in previous research and practice? (iv) How will this research add to knowledge, practice and policy in this area? Some of the evaluation committees, expect the last two questions, elaborated under a separate heading of ‘background and significance’.[ 8 ] Introduction should also contain the hypothesis behind the research design. If hypothesis cannot be constructed, the line of inquiry to be used in the research must be indicated.
Review of literature
It refers to all sources of scientific evidence pertaining to the topic in interest. In the present era of digitalisation and easy accessibility, there is an enormous amount of relevant data available, making it a challenge for the researcher to include all of it in his/her review.[ 9 ] It is crucial to structure this section intelligently so that the reader can grasp the argument related to your study in relation to that of other researchers, while still demonstrating to your readers that your work is original and innovative. It is preferable to summarise each article in a paragraph, highlighting the details pertinent to the topic of interest. The progression of review can move from the more general to the more focused studies, or a historical progression can be used to develop the story, without making it exhaustive.[ 1 ] Literature should include supporting data, disagreements and controversies. Five ‘C's may be kept in mind while writing a literature review[ 10 ] [ Table 1 ].
Aims and objectives
The research purpose (or goal or aim) gives a broad indication of what the researcher wishes to achieve in the research. The hypothesis to be tested can be the aim of the study. The objectives related to parameters or tools used to achieve the aim are generally categorised as primary and secondary objectives.
Research design and method
The objective here is to convince the reader that the overall research design and methods of analysis will correctly address the research problem and to impress upon the reader that the methodology/sources chosen are appropriate for the specific topic. It should be unmistakably tied to the specific aims of your study.
In this section, the methods and sources used to conduct the research must be discussed, including specific references to sites, databases, key texts or authors that will be indispensable to the project. There should be specific mention about the methodological approaches to be undertaken to gather information, about the techniques to be used to analyse it and about the tests of external validity to which researcher is committed.[ 10 , 11 ]
The components of this section include the following:[ 4 ]
Population and sample
Population refers to all the elements (individuals, objects or substances) that meet certain criteria for inclusion in a given universe,[ 12 ] and sample refers to subset of population which meets the inclusion criteria for enrolment into the study. The inclusion and exclusion criteria should be clearly defined. The details pertaining to sample size are discussed in the article “Sample size calculation: Basic priniciples” published in this issue of IJA.
Data collection
The researcher is expected to give a detailed account of the methodology adopted for collection of data, which include the time frame required for the research. The methodology should be tested for its validity and ensure that, in pursuit of achieving the results, the participant's life is not jeopardised. The author should anticipate and acknowledge any potential barrier and pitfall in carrying out the research design and explain plans to address them, thereby avoiding lacunae due to incomplete data collection. If the researcher is planning to acquire data through interviews or questionnaires, copy of the questions used for the same should be attached as an annexure with the proposal.
Rigor (soundness of the research)
This addresses the strength of the research with respect to its neutrality, consistency and applicability. Rigor must be reflected throughout the proposal.
It refers to the robustness of a research method against bias. The author should convey the measures taken to avoid bias, viz. blinding and randomisation, in an elaborate way, thus ensuring that the result obtained from the adopted method is purely as chance and not influenced by other confounding variables.
Consistency
Consistency considers whether the findings will be consistent if the inquiry was replicated with the same participants and in a similar context. This can be achieved by adopting standard and universally accepted methods and scales.
Applicability
Applicability refers to the degree to which the findings can be applied to different contexts and groups.[ 13 ]
Data analysis
This section deals with the reduction and reconstruction of data and its analysis including sample size calculation. The researcher is expected to explain the steps adopted for coding and sorting the data obtained. Various tests to be used to analyse the data for its robustness, significance should be clearly stated. Author should also mention the names of statistician and suitable software which will be used in due course of data analysis and their contribution to data analysis and sample calculation.[ 9 ]
Ethical considerations
Medical research introduces special moral and ethical problems that are not usually encountered by other researchers during data collection, and hence, the researcher should take special care in ensuring that ethical standards are met. Ethical considerations refer to the protection of the participants' rights (right to self-determination, right to privacy, right to autonomy and confidentiality, right to fair treatment and right to protection from discomfort and harm), obtaining informed consent and the institutional review process (ethical approval). The researcher needs to provide adequate information on each of these aspects.
Informed consent needs to be obtained from the participants (details discussed in further chapters), as well as the research site and the relevant authorities.
When the researcher prepares a research budget, he/she should predict and cost all aspects of the research and then add an additional allowance for unpredictable disasters, delays and rising costs. All items in the budget should be justified.
Appendices are documents that support the proposal and application. The appendices will be specific for each proposal but documents that are usually required include informed consent form, supporting documents, questionnaires, measurement tools and patient information of the study in layman's language.
As with any scholarly research paper, you must cite the sources you used in composing your proposal. Although the words ‘references and bibliography’ are different, they are used interchangeably. It refers to all references cited in the research proposal.
Successful, qualitative research proposals should communicate the researcher's knowledge of the field and method and convey the emergent nature of the qualitative design. The proposal should follow a discernible logic from the introduction to presentation of the appendices.
Financial support and sponsorship
Conflicts of interest.
There are no conflicts of interest.
Mobile Menu Overlay
The White House 1600 Pennsylvania Ave NW Washington, DC 20500
The Economics of Administration Action on Student Debt
Higher education financing allows many Americans from lower- and middle-income backgrounds to invest in education. However, over the past 30 years, college tuition prices have increased faster than median incomes, leaving many Americans with large amounts of student debt that they struggle or are unable to, pay off.
Recognizing the burden of this debt, the Biden-Harris Administration has pursued two key strategies for debt reduction and cancellation. The first, student debt relief (SDR), aims to address the ill effects of flaws in the student debt system for borrowers. The second, the SAVE plan, reforms the federal student loan system, improving student loan affordability for future students and providing current graduates with breathing room during the beginning of a new career.
This issue brief examines the factors that precipitated the current student debt landscape, and details how both SDR and SAVE will enhance the economic status of millions of Americans with student debt: enabling them to allocate more funds towards basic necessities, take career risks, start businesses, and purchase homes. This brief highlights credible research, underscoring how the Administration’s student debt relief could boost consumption in the short-term by billions of dollars and could have important impacts on borrower mental health, financial security, and outcomes such as homeownership and entrepreneurship. This brief also details how the SAVE plan makes repaying college costs more affordable for current borrowers and future generations. CEA simulations show that, under SAVE, an average borrower with a bachelor’s degree could save $20,000 in loan payments, while a borrower with an associate degree could see nearly 90 percent savings compared to the standard loan repayment plan. These changes enable more people to pursue education and contribute to the broader economy.
Why do borrowers need relief?
Over the last 20 years especially, the sticker price of college has risen significantly. Despite recent minor declines, sticker prices at public universities (which over 70% of undergraduate students in the United States attend) are 56% higher today than two decades ago. [1] While there are many reasons for this trend, the most rapid increases in tuition often occur during economic downturns as tuitions grow to fill the budgetary holes that are left when states cut their support to public colleges ( Webber, 2017 ; Deming and Walters 2018 ). This is especially problematic given many people choose to return to school during economic downturns ( Betts and MacFarland 1995 ; Hillman and Orians 2013 ). Unfortunately, contracting state appropriations have played a role in shifting the responsibility of financing away from public subsidies and toward students and families ( Turner and Barr, 2013 ; Bound et al., 2019 )–leading many students to take on more debt.
At the same time that college sticker prices have risen, the wage premium (the earnings difference between college goers and high school graduates) has not seen analogous growth. While obtaining a college degree remains a reliable entry point to the middle class, the relative earning gains for degree holders began to stagnate in the early 2000s after increasing for several decades. As shown in Figure 1b, since 2000, the wage premium for both bachelor’s degree holders and those with “some college” education (which includes anyone who enrolled in college but didn’t earn a BA) saw declines around the 2001-02 and 2008-10 recessions and a slow, inconsistent recovery thereafter. The decline is particularly notable for students who didn’t complete a four-year degree, a group that includes two-year college enrollees who have among the highest student loan default rates. [2]
Traditional economic theory tells us that individuals choose to invest in post-secondary education based on the expected costs and wage returns associated with the investment. But rapid and unforeseeable rises in prices and declines in college wage premia have contributed to decades of “unlucky” college-entry cohorts affected by a form of recessionary scarring . For example, a student who entered college in 2006 would have expected a sticker price of roughly $8,800 per year for a four-year college, but actually faced tuition of over $10,000 in their final year of college, a roughly 15% difference. This same student, upon graduation if they worked full time, would have earned about $3,500 less, on average, than what they would have expected upon entering. This example illustrates that many borrowers made sound borrowing decisions with available information, but as a result of these trends ended up with more debt than they could afford to pay off. [3] Consistent with this notion, the default rate for “unlucky” college entry cohorts of the 2000s is much higher than those of other cohorts, with undergraduate default rates doubling between 2000 and 2010: in 2017, 21 percent of undergraduate loan holders and 6 percent of graduate loan holders defaulted within 3 years ( CBO, 2020 ).
It is important to note that sticker prices for public institutions have declined 7 percent since 2021, the same period over which college wage premiums have been rising. Declining tuition, for the first time in decades, coincided with increased investment in higher education through pandemic-era legislation such as the American Rescue Plan, which allocated $40 billion in 2021 to support institutes of higher education and their students. Despite these improvements, as well as significant advances in the return on college investments over the last three years, many current borrowers still need some relief. The Administration has taken significant action to protect future cohorts from similar risks.
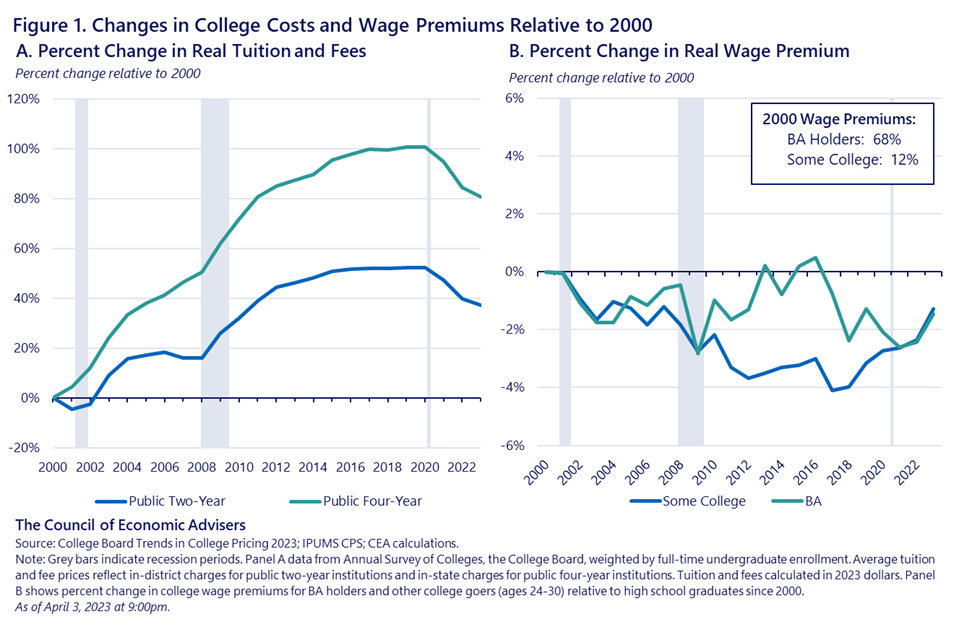
How the Administration is providing relief
Retrospective: Student Debt Relief Helps Existing Borrowers
In a commitment to help those who are overburdened with debt, the Administration has already approved Federal student debt cancellation for nearly 4 million Americans through various actions. Today , the Administration announced details of proposed rules that, if finalized as proposed, would provide relief to over 30 million borrowers when taken together with actions to date.
Importantly, much of this debt forgiveness comes from correcting program administration and improving regulations related to laws that were on the books before this Administration took office. This debt relief has affected borrowers from all walks of life, including nearly 900,000 Americans who have dedicated their lives to public service (such as teachers, social workers, nurses, firefighters, police officers, and others), borrowers who were misled and cheated by their institutions, and borrowers who are facing total or permanent disability, including many veterans. By relieving these borrowers of long-held, and in some cases very large burdens of debt, relief can have significant meaning and impact for borrowers, families, and their communities.
By reducing debtors’ liabilities, debt relief raises net worth (assets, including income less liabilities). Debt relief can also ease the financial burden of making payments—leading to greater disposable income for borrowers and their families, which enhances living standards and could positively influence decisions about employment, home buying, and mobility. While there are few direct estimates of the effect of debt cancelation in the literature, estimates based on the relationship between wealth and consumption suggest that this forgiveness could increase consumption by several billions of dollars each year in the next five to ten years.
Additionally, a recent study suggests that student debt cancellation can lead to increased earnings (due to greater geographic and career mobility), improved credit scores, and lower delinquency rates on other debts ( Di Maggio, Kalda, and Yao, 2019 ). This can facilitate access to capital for starting a business or buying a car or home. As home mortgages often require a certain debt-to-income ratio and depend heavily on credit scores, student debt cancellation could potentially increase home ownership. Indeed, based on the mechanical relationship between housing industry affordability standards and debt-to-income ratios, industry sources have suggested that those without student debt could afford to take out substantially larger mortgages ( Zillow, 2018 ). Other research also indicates a negative correlation between student loan debt and homeownership ( Mezza et al., 2020 ).
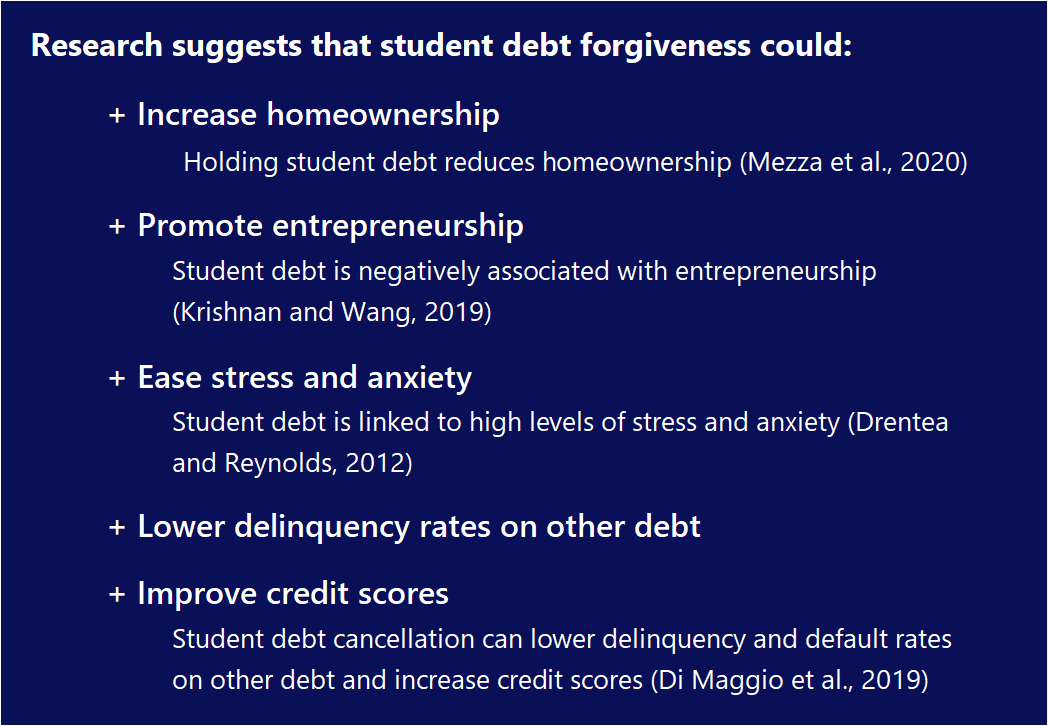
It is important to note that, while these pecuniary benefits are important, the benefits associated with debt relief are not merely financial. Experimental evidence has linked holding debt to heightened levels of stress and anxiety ( Drentea and Reynolds, 2012 ), worse self-reported physical health ( Sweet et al., 2013 ), and reduced cognitive capacity ( Robb et al., 2012 ; Ong et al., 2019 ). Studies also show that holding student debt can be a barrier to positive life cycle outcomes such as entrepreneurship ( Krishnan and Wang, 2019 ), and marriage ( Gicheva, 2016 ; Sieg and Wang, 2018 ). Student debt relief has the potential to improve these key outcomes for millions of borrowers.
Prospective: The SAVE Plan Helps Prevent Future Challenges
To address unaffordable education financing moving forward, the Administration has also introduced the Saving on a Valuable Education (SAVE) loan repayment program. The SAVE plan prospectively helps student borrowers by ensuring that once they graduate, they never have to pay more than they can afford towards their student loan debt. Importantly, the SAVE plan protects borrowers from being “unlucky” by ensuring that high tuition or low earnings do not result in loan payments that borrowers can’t afford. The CEA has detailed the real benefits of SAVE for borrowers in issue briefs and blogs , underscoring that SAVE is the most affordable student loan repayment program in U.S. history. By substantially reducing monthly payment amounts compared to previous income driven repayment (IDR) plans and reducing time to forgiveness to as little as 10 years for people who borrowed smaller amounts, the SAVE plan can mean tens of thousands of dollars in real savings for borrowers over the course of repayment.
Figure 2 gives the example of two representative borrowers. Take the first, a 4-year college graduate who has $31,000 in debt and earns about $40,500 per year. Under a standard repayment plan, this borrower would pay roughly $330 dollars each month for 10 years. Under SAVE, this borrower would pay about $50 per month for the first ten years, and on average about $130 per month for the next 10 years. Over a 20-year period, this borrower would make roughly $17,500 less in payments, not accounting for inflation over that period. This represents a 56 percent reduction in total payments compared to the standard repayment plan and includes considerable loan forgiveness. Similarly, the representative 2-year college graduate has $10,000 in debt and earns about $32,000 per year. Under a standard plan, this borrower would pay $110 dollars each month for 10 years. Under SAVE, this borrower would pay $0 per month for the first two years, and under $20 per month for the next eight years before their debt is forgiven at year 10. Overall, this borrower would be responsible for roughly $11,700 less in lifetime payments, not accounting for inflation. This borrower sees nearly 90 percent savings compared to the standard plan and receives considerable loan forgiveness.
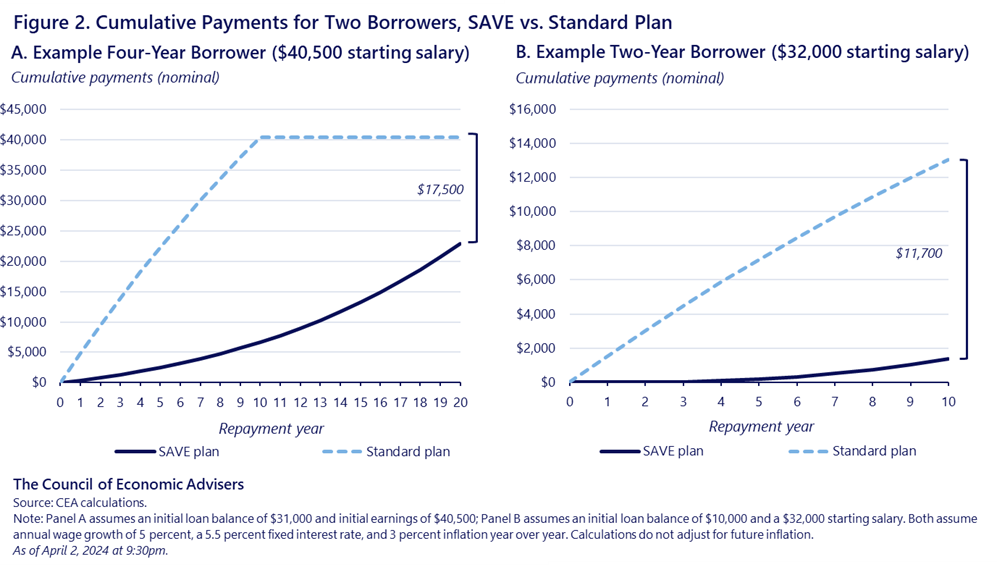
SAVE can also have benefits beyond the individual borrower. More money in borrowers’ pockets due to lower payment obligations under SAVE could boost consumption and give borrowers breathing room to make payments on other debt. This consumption effect is bolstered by a large literature documenting the benefits of easing liquidity constraints (see, for example, Aydin, 2022 ; Parker et al., 2022 ). Additionally, by shortening time to forgiveness for undergraduate borrowers, SAVE can lead to positive debt-relief outcomes (as discussed above) for many more borrowers.
Another key aspect of income-driven repayment plans like SAVE is that they protect borrowers from having to make large payments when incomes are low. Specifically, the required payments are not based on the initial loan balance, but on one’s income and household size so that those cohorts who need to borrow more to pay for college do not make larger payments unless they make more income. SAVE also protects more of a borrower’s income as discretionary and, when the full plan is implemented in Summer 2024, will limit monthly payments on undergraduate loans to 5 percent of discretionary income. In fact, for single borrowers who make less than $33,000 per year, the required monthly payments will be zero dollars. From a finance perspective, the SAVE plan provides a form of insurance against tuition spikes and economic downturns–taking some of the risk out of investing in one’s education while also bringing costs down.
A common concern, and one that could mute these benefits, is that increases in the generosity of education financing may encourage institutions to raise tuition and fees in response, a phenomenon commonly referred to as the Bennett Hypothesis (for an excellent overview of research, see Dynarski et al., 2022 ). Theoretically, in a market when sellers are maximizing profits, any policy that increases demand will also increase prices. However, this is less likely to impact the over 70% of U.S. undergraduates who attend public colleges, which are not profit-driven and often have statutorily set tuition. Consistent with this notion, the evidence in support of the Bennett Hypothesis primarily comes from for-profit colleges, which are highly reliant on students who receive federal financial aid ( Cellini and Goldin, 2014 ; Baird et al, 2022 ). [4] Importantly, although the for-profit sector enrolls some of the country’s most vulnerable students, enrollment in the sector in 2021 accounted for only 5 percent of total undergraduate enrollment, suggesting that aggregate tuition increases in response to changes in education financing may be modest. Furthermore, the Biden-Harris Administration has taken action to crack down on for-profit colleges that take advantage of, or mislead, their students. And, recent regulations, such as the Gainful Employment ( GE ) rule, add safeguards against unaffordable debt regardless of more generous education financing.
Although the SAVE plan stands to benefit borrowers of all backgrounds, the plan has important racial and socioeconomic equity implications because it is particularly beneficial for those borrowers with the lowest incomes. Centuries of inequities have led to Black, Hispanic, and Native households being more likely than their White peers to fall in the low end of the income distribution. This means that, mechanically, the SAVE plan’s benefits could accrue disproportionately to these groups. Indeed, using completion data from recent years, an Urban Institute analysis estimates that 59 percent of credentials earned by Black students and 53 percent of credentials earned by Hispanic students are likely to be eligible for some amount of loan forgiveness under SAVE, compared to 42 percent of credentials earned by White students ( Delisle and Cohn, 2023 ). Finally, the interest subsidy described in an August 2023 CEA blog , prevents ballooning balances when a borrower cannot cover their entire monthly interest payment, a phenomenon that has historically led to many borrowers in general, and Black borrowers in particular, to see loan balances that are higher than their original loan amount, even several years out from graduating with a bachelor’s degree ( NCES, 2023 ).
Broader economic impacts
The benefits associated with SDR and SAVE for millions of Americans are considerable. In the short run, under both SDR and SAVE, those who receive relief may be able to spend more in their communities and contribute to their local economies. Summing the likely consumption effects of the Administration’s student debt relief and SAVE programs results in billions of dollars in additional consumption annually. Despite the modest effect on the macroeconomy as a whole (note that the U.S. economy is roughly $28 trillion with a population of roughly 320 million), these consumption effects represent incredibly meaningful impacts on individual borrowers’ financial security and the economic wellbeing of their communities.
SAVE, because it brings down the cost of taking out loans to go to college, has the potential to lead to longer-term economic growth if it leads to greater educational attainment. This increased attainment can occur both through improved retention and completion of post-secondary education, and also the movement of students into college who would not have otherwise enrolled. There is a long macroeconomics literature linking educational attainment in a nation to GDP growth (see, for example, Lucas, 1988 ; Hanushek and Woesmann, 2008 ). While identifying the causal effect of schooling on GDP is challenging, researchers, using a variety of approaches, find that a one-year increase in average education (for the entire working population) would increase the real GDP level by between 5 and 12 percent ( Barro and Lee, 2013 ; Soto, 2002 ) —a result that is in line with the micro-founded relationship between years of education and earnings ( Lovenheim and Smith, 2022 ).
To put this relationship in perspective and highlight the growth potential of increasing educational attainment, the CEA simulated the hypothetical effect on GDP of increasing the college-going rate by 1, 3, and 5 percentage points, respectively. This range represents the kinds of changes in college going that have been observed over several years: the college enrollment rate for 18- to 24-year-olds declined 4 percentage points between 2011 and 2021 after increasing by 6 percentage points between 2000 and 2011 ( NCES 2023 ). CEA simulations show that by 2055, a policy that increased the college going rate by 1, 3, and 5 percentage points could increase the level of GDP in 2055 (thirty years from now) by 0.2, 0.6, and 1 percent respectively. This represents hundreds of billions of dollars of additional economic activity in the long run.
While increased growth is an exciting possibility, it would only occur insofar as SAVE leads to increased educational attainment, which is uncertain. The academic literature has found that student loans can promote academic performance ( Barr, et. al. 2021 ), and increase educational attainment by increasing transfers from 2-year to 4-year colleges and increasing college completion among enrollees ( Marx and Turner, 2019 ). At the same time, increases in college-going due to SAVE are by no means guaranteed. While, historically, policies that reduce the cost of college through direct means—such as providing students with generous grant aid, or reducing tuition—have succeeded at raising college enrollment levels ( Dynarski, 2003 ; Turner, 2011 ), a pair of recent studies show that prospective students may only respond to cost changes when they are salient, i.e., they are framed and marketed in the right way ( Dynarski et al., 2021 ), and relatively certain ( Burland et al., 2022 ). However, evidence suggests that there is demand for plans like SAVE ( Balakrishnan et al., 2024 ), particularly as SAVE can provide sizable benefits to borrowers in terms of reducing their long-term debt burden and keep monthly payments low (dependent on a borrower’s income) after they finish school.
This highlights the importance of communicating the benefits of the SAVE program to prospective students who otherwise would not enroll in college due to cost concerns, including potential barriers to paying off student loans in the future. Doing so could lead to meaningful increases in college enrollment, and the resulting improvements in productive capacity could increase the size of the U.S. economy for years to come.
Concluding remarks
The Biden-Harris Administration has taken bold action to address a student debt problem that has been decades in the making. This student debt cancellation will provide well-deserved relief for borrowers who have paid their fair share, many of whom had the proverbial rug pulled out from under them with concurrent rapidly rising tuition and declining returns to a college degree. The relief has and will improve economic health and wellbeing of those who have devoted years of their life to public service, those who were defrauded or misled by their institutions, and those who have been doing all they can to make payments, but have still seen their loan balances grow. Looking to future generations, the Administration implemented the SAVE plan to protect borrowers against tuition spikes and poorer than expected labor market outcomes that often plague students graduating into a period of economic downturn ( Rothstein, 2021 ; Schwandt and von Wachter, 2023 ).
Both student debt relief and SAVE will enhance the economic status of millions of Americans with student debt: enable them to allocate more funds towards basic necessities, take career risks, start businesses, and purchase homes with the understanding that they will never have to pay more than they can afford towards their student loans. Moreover, the SAVE plan makes repayment more affordable for future generations, which helps borrowers manage monthly payments, but also enables more people from all walks of life to explore their full potential and pursue higher education, enhancing the potential of the U.S. workforce and the economy more broadly.
[1] In 2021, 51% of total undergraduates attended public 4-year universities and 21% attended public 2-years in 2021.
[2] The BA group excludes those with a graduate degree, or any education beyond a bachelor’s degree.
[3] Recent research shows that, despite a positive return on investment (ROI) for many, including the average student, the distribution of ROI has widened over the last several decades such that the likelihood of negative ROI is higher than it has historically been, particularly so for underrepresented minority students ( Webber 2022 ).
[4] There is also some evidence in support of the Bennett Hypothesis at the graduate level ( Black et al. 2023 ).
Stay Connected
We'll be in touch with the latest information on how President Biden and his administration are working for the American people, as well as ways you can get involved and help our country build back better.
Opt in to send and receive text messages from President Biden.
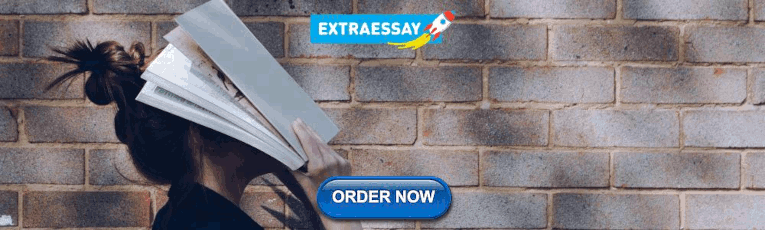
IMAGES
VIDEO
COMMENTS
Data analysis: Statistically analyze survey data; Conduct thematic analysis of interview transcripts; Draft results and discussion chapters; 22nd April: 5. Writing ... The best way to remember the difference between a research plan and a research proposal is that they have fundamentally different audiences. A research plan helps you, the ...
A good data analysis plan should summarize the variables as demonstrated in Figure 1 below. Figure 1. Presentation of variables in a data analysis plan. 5. Statistical software. There are tons of software packages for data analysis, some common examples are SPSS, Epi Info, SAS, STATA, Microsoft Excel.
DATA ANALYSIS PLAN 1. How it fits in 2. What it shows 3. Example 4. Tips and morals Henry Feldman Clinical Research Center Boston Children's Hospita l. analysis plan: bird's-eye view A good analysis plan is the ultimate demonstration that your whole proposal is well formulated. Specific Aims Hypotheses Study Design Data Collection
A Data Analysis Plan (DAP) is about putting thoughts into a plan of action. Research questions are often framed broadly and need to be clarified and funnelled down into testable hypotheses and action steps. The DAP provides an opportunity for input from collaborators and provides a platform for training. Having a clear plan of action is also ...
The first step in a data analysis plan is to describe the data collected in the study. This can be done using figures to give a visual presentation of the data and statistics to generate numeric descriptions of the data. Selection of an appropriate figure to represent a particular set of data depends on the measurement level of the variable.
1. Research Proposal Format Example. Following is a general outline of the material that should be included in your project proposal. I. Title Page II. Introduction and Literature Review (Chapters 2 and 3) A. Identification of specific problem area (e.g., what is it, why it is important). B. Prevalence, scope of problem.
Analysis Plan and Manage Data. The main tasks are as follows: 1. Create an analysis plan • Identify research questions and/or hypotheses. • Select and access a dataset. • List inclusion/exclusion criteria. • Review the data to determine the variables to be used in the main analysis. • Select the appropriate statistical methods and ...
Make sure you can ask the critical what, who, and how questions of your research before you put pen to paper. Your research proposal should include (at least) 5 essential components : Title - provides the first taste of your research, in broad terms. Introduction - explains what you'll be researching in more detail.
22.1 Writing the Data Analysis Plan. Congratulations! You have now arrived at one of the most creative and straightforward, sections of your grant proposal. You and your project statistician have one major goal for your data analysis plan: You need to convince all the reviewers reading your proposal that you would know what to do with your data ...
A research proposal describes what you will investigate, why it's important, ... A research proposal serves as a blueprint and guide for your research plan, helping you get organised and feel confident in the path forward you choose to take. ... Finalize sampling methods and data analysis methods; 13th February: 3. Data collection and ...
The purpose of the research proposal (its job, so to speak) is to convince your research supervisor, committee or university that your research is suitable (for the requirements of the degree program) and manageable (given the time and resource constraints you will face). The most important word here is "convince" - in other words, your ...
Start by defining your project's purpose. Identify what your project aims to accomplish and what you are researching. Remember to use clear language. Thinking about the project's purpose will help you set realistic goals and inform how you divide tasks and assign responsibilities.
22.1 Writing the Data Analysis Plan ... W. Pequegnat et al. (eds.), How to Write a Successful Research Grant Application: A Guide for Social and Behavioral Scientists, DOI 10.1007/978-1-4419-1454-5_22, ... All grant proposals include a conceptual model that drives the proposed study, and
An analysis plan is a description of the steps of the analyses that will be used to understand study objectives (Yuan et al., 2019). The analysis plan is a part of the collaborative process ...
Here is an explanation of each step: 1. Title and Abstract. Choose a concise and descriptive title that reflects the essence of your research. Write an abstract summarizing your research question, objectives, methodology, and expected outcomes. It should provide a brief overview of your proposal. 2.
Research design; Reference list; While the sections may vary, the overall objective is always the same. A research proposal serves as a blueprint and guide for your research plan, helping you get organized and feel confident in the path forward you choose to take.
A data analysis plan is a roadmap for how you're going to organize and analyze your survey data—and it should help you achieve three objectives that relate to the goal you set before you started your survey: Answer your top research questions. Use more specific survey questions to understand those answers. Segment survey respondents to ...
Quantitative Data: Univariate Analysis. As part of planning for your research, you should come up with a data analysis plan. Remember, a data analysis plan is an ordered outline that includes your research question, a description of the data you are going to use to answer it, and the exact step-by-step analyses that you plan to run to answer your research question.
Definition of research in data analysis: According to LeCompte and Schensul, research data analysis is a process used by researchers to reduce data to a story and interpret it to derive insights. The data analysis process helps reduce a large chunk of data into smaller fragments, which makes sense. Three essential things occur during the data ...
Concrete research questions are essential for determining the analyses required. The analysis plan should then describe the primary and secondary outcomes, the determinants and data needed, and which statistical techniques are to be used to analyse the data. The following issues need to be considered in this process and described where ...
The templates includes research questions stated in statistical language, analysis justification and assumptions of the analysis. Simply edit the blue text to reflect your research information and you will have the data analysis plan for your dissertation or research proposal.
Writing the proposal of a research work in the present era is a challenging task due to the constantly evolving trends in the qualitative research design and the need to incorporate medical advances into the methodology. The proposal is a detailed plan or 'blueprint' for the intended study, and once it is completed, the research project ...
The theory must inform or align with all phases of the research study, including the research question, the literature review, the design, the interview guide, and the analysis plan. More specifically, in qualitative proposals, theories may help develop the questions asked to populations so that they can answer the research questions adequately.
CEA simulations show that by 2055, a policy that increased the college going rate by 1, 3, and 5 percentage points could increase the level of GDP in 2055 (thirty years from now) by 0.2, 0.6, and ...