- COVID-19 Collection
- AI Collection
- Oxford Thesis Collection
- CC0 version of this metadata
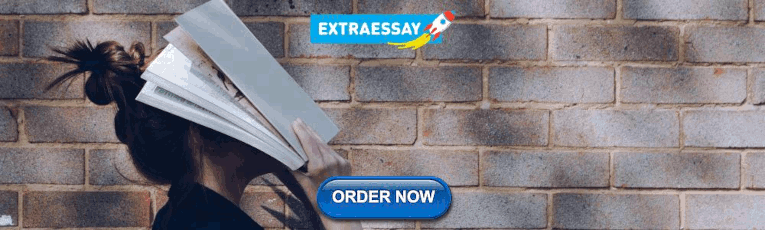
Machine learning approaches for computer aided drug discovery
Pharmaceutical drug discovery is expensive, time consuming and scientifically challenging. In order to increase efficiency of the pre-clinical drug discovery pathway, computational drug discovery methods and most recently, machine learning-based methods are increasingly used as powerful tools to aid early stage drug discovery.
In this thesis, I present three complementary computer-aided drug discovery methods, with a focus on aiding hit discovery and hit-to-lead optimization. In ad...
Email this record
Please enter the email address that the record information will be sent to.
Please add any additional information to be included within the email.
Cite this record
Chicago style, access document.
- PhD_Thesis_Marc_Moesser.pdf (Dissemination version, pdf, 73.2MB)
Why is the content I wish to access not available via ORA?
Content may be unavailable for the following four reasons.
- Version unsuitable We have not obtained a suitable full-text for a given research output. See the versions advice for more information.
- Recently completed Sometimes content is held in ORA but is unavailable for a fixed period of time to comply with the policies and wishes of rights holders.
- Permissions All content made available in ORA should comply with relevant rights, such as copyright. See the copyright guide for more information.
- Clearance Some thesis volumes scanned as part of the digitisation scheme funded by Dr Leonard Polonsky are currently unavailable due to sensitive material or uncleared third-party copyright content. We are attempting to contact authors whose theses are affected.
Alternative access to the full-text
Request a copy.
We require your email address in order to let you know the outcome of your request.
Provide a statement outlining the basis of your request for the information of the author.
Please note any files released to you as part of your request are subject to the terms and conditions of use for the Oxford University Research Archive unless explicitly stated otherwise by the author.
Contributors
Bibliographic details, item description, related items, terms of use, views and downloads.
If you are the owner of this record, you can report an update to it here: Report update to this record
Report an update
We require your email address in order to let you know the outcome of your enquiry.
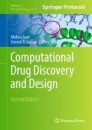
Computational Drug Discovery and Design pp 1–20 Cite as
Computer-Aided Drug Discovery and Design: Recent Advances and Future Prospects
- Alan Talevi 4 , 5
- First Online: 08 September 2023
1536 Accesses
2 Citations
1 Altmetric
Part of the book series: Methods in Molecular Biology ((MIMB,volume 2714))
Computer-aided drug discovery and design involve the use of information technologies to identify and develop, on a rational ground, chemical compounds that align a set of desired physicochemical and biological properties. In its most common form, it involves the identification and/or modification of an active scaffold (or the combination of known active scaffolds), although de novo drug design from scratch is also possible. Traditionally, the drug discovery and design processes have focused on the molecular determinants of the interactions between drug candidates and their known or intended pharmacological target(s). Nevertheless, in modern times, drug discovery and design are conceived as a particularly complex multiparameter optimization task, due to the complicated, often conflicting, property requirements.
This chapter provides an updated overview of in silico approaches for identifying active scaffolds and guiding the subsequent optimization process. Recent groundbreaking advances in the field have also analyzed the integration of state-of-the-art machine learning approaches in every step of the drug discovery process (from prediction of target structure to customized molecular docking scoring functions), integration of multilevel omics data, and the use of a diversity of computational approaches to assist target validation and assess plausible binding pockets.
This is a preview of subscription content, log in via an institution .
Buying options
- Available as PDF
- Read on any device
- Instant download
- Own it forever
- Available as EPUB and PDF
- Durable hardcover edition
- Dispatched in 3 to 5 business days
- Free shipping worldwide - see info
Tax calculation will be finalised at checkout
Purchases are for personal use only
Springer Nature is developing a new tool to find and evaluate Protocols. Learn more
Klabunde T, Everts A (2005) GPCR antitarget modeling: pharmacophore models for biogenic amine binding GPCRs to avoid GPCR-mediated side effects. Chembiochem 6:876–889
Article CAS PubMed Google Scholar
Raschi E, Vasina V, Poluzzi E et al (2008) The hERG K+ channel: target and antitarget strategies in drug development. Pharmacol Res 57:181–195
Crivori P (2008) Computational models for P-glycoprotein substrates and inhibitors. In: Vaz RJ, Klabunde T (eds) Anti-atrgets: prediction and prevention of drug side effects. Wiley-VCH, Weinheim
Google Scholar
Zamora I (2008) Site of metabolism predictions: facts and experiences. In: Vaz RJ, Klabunde T (eds) Anti-targets: prediction and prevention of drug side effects. Wiley-VCH, Weinheim
Fallico M, Alberca LN, Prada Gori DN et al (2022) Machine learning search of novel selective NaV1.2 and NaV1.6 inhibitors as potential treatment against Dravet syndrome. In: Ribeiro PRDA, Cota VR, Barone DAC, de Oliveira ACM (eds) Computational neuroscience. LAWCN 2021. Communications in computer and information science, vol 1519. Springer, Cham
Fatoba AJ, Okpeku M, Adeleke MA (2021) Subtractive genomics approach for identification of novel therapeutic drug targets in Mycoplasma genitalium . Pathogens 10:921
Article CAS PubMed Central PubMed Google Scholar
Süntar I (2020) Importance of ethnopharmacological studies in drug discovery: role of medicinal plants. Phytochem Rev 19:1199–1209
Article Google Scholar
Entzeroth M, Flotow H, Condron P (2009) Overview of high-throughput screening. Curr Protoc Pharmacol Chapter 9:Unit 9.4
Maia EHB, Assis LC, de Oliveira TA et al (2020) Structure-based virtual screening: from classical to artificial intelligence. Front Chem 8:343
Mouchlis VD, Afantitis A, Serra A et al (2021) Advances in de novo drug design: from conventional to machine learning methods. Int J Mol Sci 22:1676
Article PubMed Central PubMed Google Scholar
Kirsch P, Hartman AM, Hirsch AKH et al (2019) Concepts and core principles of fragment-based drug design. Molecules 24:4309
Romano P, Giugno R, Pulvirenti A (2011) Tools and collaborative environments for bioinformatics research. Brief Bioinform 12:549–561
Gorgulla C, Boeszoermenyi A, Wang ZF et al (2020) An open-source drug discovery platform enables ultra-large virtual screens. Nature 580:663–668
Cox PB, Gupta R (2022) Contemporary computational applications and tools in drug discovery. ACS Med Chem Lett 13:1016–1029
Prada Gori DN, Alberca LN, Rodriguez S et al (2022) LIDeB Tools: a Latin American resource of freely available, open-source cheminformatics apps. Artif Intell Life Sci 2:10049
Hartenfeller M, Schneider G (2011) De novo drug design. Methods Mol Biol 672:299–323
Kuttruff CA, Eastgate MD, Baran PS (2014) Natural product synthesis in the age of scalability. Nat Prod Rep 31:419–432
Nicolaou CA, Brown N (2013) Multi-objective optimization methods in drug design. Drug Discov Today Technol 10:e427–e435
Article PubMed Google Scholar
Talevi A (2016) Tailored multi-target agents. Applications and design considerations. Curr Pharm Des 22:3164–3170
Lipinski CA, Lombardo F, Dominy BW, Feeney PJ (1997) Experimental and computational approaches to estimate solubility and permeability in drug discovery and development settings. Adv Drug Deliv Rev 23:3–25
Article CAS Google Scholar
Pajouhesh H, Lenz GR (2005) Medicinal chemical properties of successful central nervous system drugs. NeuroRx 2:542–553
Gupta S, Kesarla R, Omri A (2013) Formulation strategies to improve the bioavailability of poorly absorbed drugs with special emphasis on self-emulsifying systems. ISRN Pharm 2013:848043
PubMed Central PubMed Google Scholar
Miller DC, Klute W, Calabrese A et al (2009) Optimising metabolic stability in lipophilic chemical space: the identification of a metabolic stable pyrazolopyrimidine CRF-1 receptor antagonist. Bioorg Med Chem Lett 19:6144–6147
Wager TT, Hou X, Verhoest PR et al (2016) Central nervous system multiparameter optimization desirability: application in drug discovery. ACS Chem Neurosci 7:767–775
Glen RC, Galloway WR, Spring DR et al (2016) Multiple-parameter optimization in drug discovery: example of the 5-HT1B GPCR. Mol Inform 35:599–605
Ghose AK, Ott GR, Hudkins RL (2017) Technically Extended MultiParameter Optimization (TEMPO): an advanced robust scoring scheme to calculate central nervous system druggability and monitor lead optimization. ACS Chem Neurosci 8:147–154
Winter R, Montanari F, Steffen A et al (2019) Efficient multi-objective molecular optimization in a continuous latent space. Chem Sci 10:8016–8024
Pennington LD, Muegge I (2021) Holistic drug design for multiparameter optimization in modern small molecule drug discovery. Bioorg Med Chem Lett 41:128003
He X (2009) Integration of physical, chemical, mechanical and biopharmaceutical properties in solid dosage oral form development. In: Solid dosage oral forms: pharmaceutical theory and practice. Academic Press, Burlington
Csermely P, Korcsmáros T, Kiss HJ et al (2013) Structure and dynamics of molecular networks: a novel paradigm of drug discovery: a comprehensive review. Pharmacol Ther 138:333–408
Wang J, Guo Z, Fu Y et al (2017) Weak-binding molecules are not drugs?-toward a systematic strategy for finding effective weak-binding drugs. Brief Bioinform 18:321–332
CAS PubMed Google Scholar
Talevi A (2022) Antiseizure medication discovery: recent and future paradigm shifts. Epilepsia Open 7(Suppl 1):S133–S141
Gashaw I, Ellinghaus P, Sommer A et al (2011) What makes a good drug target. Drug Discov Today 16:1037–1043
Knowles J, Gromo G (2003) Target selection in drug discovery. Nat Rev Drug Discov 2:63–69
Schmidtke P, Barril X (2010) Understanding and predicting druggability. A high-throughput method for detection of drug binding sites. J Med Chem 53:5858–5867
Yuan Y, Pei J, Lai L (2013) Binding site detection and druggability prediction of protein targets for structure-based drug design. Curr Pharm Des 19:2326–2333
Barril X (2013) Druggability predictions: methods, limitations and applications. Wires Comput Mol Sci 3:327–338
Talevi A, Carrillo C, Comini M (2019) The thiol-polyamine metabolism of Trypanosoma cruzi : molecular targets and drug repurposing strategies. Curr Med Chem 26:6614–6635
Tonge PJ (2018) Drug-target kinetics in drug discovery. ACS Chem Neurosci 9:29–39
Feng Y, Wang Q, Wang T (2017) Drug target protein-protein interaction networks: a systematic perspective. Biomed Res Int 2017:1289259
Viacava Follis A (2021) Centrality of drug targets in protein networks. BMC Bioinf 22:527
Sabetian S, Shamsir MS (2019) Computer aided analysis of disease linked protein networks. Bioinformation 15:513–522
Casas AI, Hassan AA, Larsen SJ et al (2019) From single drug targets to synergistic network pharmacology in ischemic stroke. Proc Natl Acad Sci U S A 116:7129–7136
Schidlitzki A, Bascuñana P, Srivastava PK et al (2020) Proof-of-concept that network pharmacology is effective to modify development of acquired temporal lobe epilepsy. Neurobiol Dis 134:104664
Kim B, Jo J, Han J et al (2017) In silico re-identification of properties of drug target proteins. BMC Bioinf 18:248
Dezső Z, Ceccarelli M (2020) Machine learning prediction of oncology drug targets based on protein and network properties. BMC Bioinf 21:104
Chen S, Jiang H, Cao Y et al (2016) Drug target identification using network analysis: taking active components in Sini decoction as an example. Sci Rep 6:24245
Ji X, Freudenberg JM, Agarwal P (2019) Integrating biological networks for drug target prediction and prioritization. Methods Mol Biol 1903:203–218
Capra JA, Singh M (2007) Predicting functionally important residues from sequence conservation. Bioinformatics 23:1875–1882
Yang J, Roy A, Zhang Y (2013) Protein-ligand binding site recognition using complementary binding-specific substructure comparison and sequence profile alignment. Bioinformatics 29:2588–2595
Han B, Salituro FG, Blanco MJ (2020) Impact of allosteric modulation in drug discovery: innovation in emerging chemical modalities. ACS Med Chem Lett 11:1810–1819
Liu T, Ish-Shalom S, Torng W et al (2018) Biological and functional relevance of CASP predictions. Proteins 86(Suppl 1):374–386
Clark JJ, Orban ZJ, Carlson HA (2020) Predicting binding sites from unbound versus bound protein structures. Sci Rep 10:15856
Kuzmanic A, Bowman GR, Juarez-Jimenez J et al (2020) Investigating cryptic binding sites by molecular dynamics simulations. Acc Chem Res 53:654–661
Smith RD, Carlson HA (2021) Identification of cryptic binding sites using MixMD with standard and accelerated molecular dynamics. J Chem Inf Model 61:1287–1299
Paul F, Weikl TR (2016) How to distinguish conformational selection and induced fit based on chemical relaxation rates. PLoS Comput Biol 12:e1005067
Vajda S, Beglov D, Wakefield AE et al (2018) Cryptic binding sites on proteins: definition, detection, and druggability. Curr Opin Chem Biol 44:1–8
Martinez-Rosell G, Lovera S, Sands ZA et al (2020) PlayMolecule CrypticScout: predicting protein cryptic sites using mixed-solvent molecular simulations. J Chem Inf Model 60:2314–2324
Zheng W (2021) Predicting cryptic ligand binding sites based on normal modes guided conformational sampling. Proteins 89:416–426
Aromolaran O, Aromolaran D, Isewon I et al (2021) Machine learning approach to gene essentiality prediction: a review. Brief Bioinform 22(5):bbab128
Basler G (2015) Computational prediction of essential metabolic genes using constraint-based approaches. Gene Essentiality 1279:183–204
Pushpakom S, Iorio F, Eyers PA et al (2019) Drug repurposing: progress, challenges and recommendations. Nat Rev Drug Discov 18:41–58
Talevi A, Bellera CL (2020) Challenges and opportunities with drug repurposing: finding strategies to find alternative uses of therapeutics. Expert Opin Drug Discov 15:397–401
Szymanski P, Markowicz M, Mikiciuk-Olasik E (2012) Adaptation of high-throughput screening in drug discovery – toxicological screening. Int J Mol Sci 13:427–452
Harris CJ, Hill RD, Sheppard DW, Slater MJ, Stouten PF (2011) The design and application of target-focused compound libraries. Comb Chem High Throughput Screen 14(6):521–531
Welsch ME, Snyder SA, Stockwell BR (2010) Privileged scaffolds for library design and drug discovery. Curr Opin Chem Biol 14:347–361
Jumper J, Evans R, Pritzel A et al (2021) Highly accurate protein structure prediction with AlphaFold. Nature 596:583–589
Baek M, DiMaio F, Anishchenko I et al (2021) Accurate prediction of protein structures and interactions using a three-track neural network. Science 373:871–876
Lin Z, Akin H, Rao R et al (2022) Language models of protein sequences at the scale of evolution enable accurate structure prediction. bioRxiv 2022.07.20.500902
Mirdita M, Schütze K, Moriwaki Y et al (2022) ColabFold: making protein folding accessible to all. Nat Methods 19:679–682
Varadi M, Anyango S, Deshpande M et al (2022) AlphaFold Protein Structure Database: massively expanding the structural coverage of protein-sequence space with high-accuracy models. Nucleic Acids Res 50:D439–D444
Procacci P (2016) Reformulating the entropic contribution of molecular docking scoring functions. J Comput Chem 37(19):1819–1827
Gilson MK, Zhou HX (2007) Calculation of protein-ligand binding affinities. Annu Rev Biophys Biomol Struct 36:21–42
Bello M, Martínez-Archundia M, Correa-Basurto J (2013) Automated docking for novel drug discovery. Expert Opin Drug Discov 8:821–834
Bodnarchuck MS (2016) Water, water, everywhere… It’s time to stop and think. Drug Discov Today 21:1139–1146
Mysinger MM, Schoichet BK (2010) Rapid context-dependent ligand desolvation in molecular docking. J Chem Inf Model 50:1561–1573
Li H, Sze KH, Lu G et al (2020) Machine-learning scoring functions for structure-based virtual screening. Wires Comput Mol Sci 11:e1478
Zhang X, Shen C, Guo X et al (2021) ASFP (Artificial Intelligence based Scoring Function Platform): a web server for the development of customized scoring functions. J Cheminform 13:6
Yang C, Chen EA, Zhang Y (2022) Protein-ligand docking in the machine-learning era. Molecules 27:4568
Ge H, Wang Y, Li C et al (2013) Molecular dynamics-based virtual screening: accelerating the drug discovery process by high-performance computing. J Chem Inf Model 53:2757–2764
Wang L, Wu Y, Deng Y et al (2015) Accurate and reliable prediction of relative ligand binding potency in prospective drug discovery by way of a modern free-energy calculation protocol and force field. J Am Chem Soc 137:2695–2703
Llanos MA, Alberca LN, Larrea SCV et al (2022) Homology modeling and molecular dynamics simulations of Trypanosoma cruzi phosphodiesterase b1. Chem Biodivers 19:e202100712
Lavechia A (2015) Machine-learning approaches in drug discovery: methods and applications. Drug Discov Today 20:318–331
Lemmen C, Zimmermann M, Lengauer T (2002) Multiple molecular superpositioning as an effective tool for virtual database screening. In: Virtual screening: an alternative or complement to high-throughput screening? 1st edn. Kluwer Academic Publishers, Marburg
Kristensen TG, Nielsen J, Pedersen CNS (2013) Methods for similarity-based virtual screening. Comput Struct Biotechnol J 5:e201302009
Talevi A, Bruno-Blanch LE (2016) Virtual screening applications in the search of novel antiepileptic drug candidates. In: Antiepileptic drug discovery. Novel approaches. Humana Press, New York
Chapter Google Scholar
Schneidman-Duhovny D, Dror O, Inbar Y et al (2008) Deterministic pharmacophore detection via multiple flexible alignment of drug-like molecules. J Comput Biol 15:737–754
Cottrell SJ, Gillet VJ, Taylor R et al (2004) Generation of multiple pharmacophore hypothesis using multiobjective optimization techniques. J Comput Aided Mol Des 18:665–682
Pirhadi S, Shiri F, Ghasemi JB (2013) Methods and applications of structure based pharmacophores in drug discovery. Curr Top Med Chem 13:1036–1047
Zhang Q, Muegge I (2006) Scaffold hopping through virtual screening using 2D and 3D similarity descriptors: ranking, voting, and consensus scoring. J Med Chem 9:1536–1548
Krüger DM, Evers A (2010) Comparison of structure- and ligand-based virtual screening protocols considering hit list complementarity and enrichment factors. ChemMedChem 5:148–158
Talevi A, Gavernet L, Bruno-Blanch LE (2009) Combined virtual screening strategies. Curr Comput Aided Drug Des 5:23–37
Pouliot M, Jeanmart S (2016) Pan Assay Interference Compounds (PAINS) and other promiscuous compounds in antifungal research. J Med Chem 59:497–503
Walters WP, Stahl MT, Murcko MA (1998) Virtual screening – an overview. Drug Discov Today 3:160–178
Zhu T, Cao S, Su PC et al (2013) Hit identification and optimization in virtual screening: practical recommendations based upon a critical literature analysis. J Med Chem 56:6560–6572
Ripphausen P, Nisius B, Pletason L et al (2010) Quo vadis, virtual screening? A comprehensive survey of prospective applications. J Med Chem 53:8461–8467
Neetoo-Isseliee Z, MacKenzie AE, Southern C et al (2013) High-throughput identification and characterization of novel, species-selective GPR35 agonists. J Pharmacol Exp Ther 344:568–578
Kola I, Landis J (2004) Can the pharmaceutical industry reduce attrition rates? Nat Rev Drug Discov 3:711–716
Schuster D, Laggner C, Langer T (2005) Why drugs fail – a study on side effects in new chemical entities. Curr Pharm Des 11:3545–3559
Talevi A (2016) Computational approaches for innovative antiepileptic drug discovery. Expert Opin Drug Discov 11:1001–1016
Wang S, Dong G, Sheng C (2019) Structural simplification of natural products. Chem Rev 119:4180–4220
Brown N, Lewis RA (2006) Exploiting QSAR methods in lead optimization. Curr Opin Drug Discov Devel 9:419–424
Wong WWL, Burkowski FJ (2009) A constructive approach for discovering new drug leads: using a kernel methodology for the inverse-QSAR problem. J Cheminform 1:4
Miyako T, Kaneko H, Funatsu K (2016) Inverse QSPR/QSAR analysis for chemical structure generation (from y to x). J Chem Inf Model 56:286–299
Waring MJ, Arrowsmith J, Leach AR et al (2015) An analysis of the attrition of drug candidates from four major pharmaceutical companies. Nat Rev Drug Discov 14:475–486
Cook D, Brown D, Alexander R et al (2014) Lessons learned from the fate of AstraZeneca’s drug pipeline: a five-dimensional framework. Nat Rev Drug Discov 13:419–431
Roberts RA, Kavanagh SL, Mellor HR et al (2014) Reducing attrition in drug development: smart loading preclinical safety assessment. Drug Discov Today 19:341–347
Veber DF, Johnson SR, Cheng HY et al (2002) Molecular properties that influence the oral bioavailability of drug candidates. J Med Chem 45:2615–2623
Price DA, Blagg J, Jones L et al (2009) Physicochemical drug properties associated with in vivo toxicological outcomes: a review. Expert Opin Drug Metab Toxicol 5:921–931
Sutherland JJ, Raymond JW, Stevens JL et al (2012) Relating molecular properties and in vitro assay results to in vivo drug disposition and toxicity outcomes. J Med Chem 55:6455–6466
Doak BC, Zheng J, Dobritzsch D et al (2016) How beyond rule of 5 drugs and clinical candidates bind to their targets. J Med Chem 59:2312–2327
Doak BC, Over B, Giordanetto F et al (2014) Oral druggable space beyond the rule of 5: insights from drugs and clinical candidates. Chem Biol 21:1115–1142
Lipinski CA (2016) Rule of five in 2015 and beyond: target and ligand structural limitations, ligand chemistry structure and drug discovery project decisions. Adv Drug Deliv Rev 101:34–41
Bergström CAS, Charman WN, Porter CJH (2016) Computational prediction of formulation strategies for beyond-rule-of-5 compounds. Adv Drug Deliv Rev 101:6–21
Download references
Acknowledgments
The author thanks CONICET and University of La Plata, where he holds permanent positions.
Author information
Authors and affiliations.
Laboratory of Bioactive Compound Research and Development (LIDeB), Faculty of Exact Sciences, National University of La Plata (UNLP), La Plata, Argentina
Alan Talevi
Argentinean National Council of Scientific and Technical Research (CONICET), La Plata, Argentina
You can also search for this author in PubMed Google Scholar
Corresponding author
Correspondence to Alan Talevi .
Editor information
Editors and affiliations.
Department of Basic and Applied Sciences, Dayananda Sagar University, Bangalore, Karnataka, India
Mohini Gore
Department of Botany, Rajaram College Kolhapur, Kolhapur, Maharashtra, India
Umesh B. Jagtap
Rights and permissions
Reprints and permissions
Copyright information
© 2024 The Author(s), under exclusive license to Springer Science+Business Media, LLC, part of Springer Nature
About this protocol
Cite this protocol.
Talevi, A. (2024). Computer-Aided Drug Discovery and Design: Recent Advances and Future Prospects. In: Gore, M., Jagtap, U.B. (eds) Computational Drug Discovery and Design. Methods in Molecular Biology, vol 2714. Humana, New York, NY. https://doi.org/10.1007/978-1-0716-3441-7_1
Download citation
DOI : https://doi.org/10.1007/978-1-0716-3441-7_1
Published : 08 September 2023
Publisher Name : Humana, New York, NY
Print ISBN : 978-1-0716-3440-0
Online ISBN : 978-1-0716-3441-7
eBook Packages : Springer Protocols
- Publish with us
Policies and ethics
- Find a journal
- Track your research
- Bibliography
- More Referencing guides Blog Automated transliteration Relevant bibliographies by topics
- Automated transliteration
- Relevant bibliographies by topics
- Referencing guides
Dissertations / Theses on the topic 'Computer-aided drug design'
Create a spot-on reference in apa, mla, chicago, harvard, and other styles.
Consult the top 50 dissertations / theses for your research on the topic 'Computer-aided drug design.'
Next to every source in the list of references, there is an 'Add to bibliography' button. Press on it, and we will generate automatically the bibliographic reference to the chosen work in the citation style you need: APA, MLA, Harvard, Chicago, Vancouver, etc.
You can also download the full text of the academic publication as pdf and read online its abstract whenever available in the metadata.
Browse dissertations / theses on a wide variety of disciplines and organise your bibliography correctly.
Kandil, Sahar. "Computer aided drug design." Thesis, Cardiff University, 2009. http://orca.cf.ac.uk/55845/.
Sun, Jian. "Computer-aided drug design for influenza A virus." Click to view the E-thesis via HKUTO, 2009. http://sunzi.lib.hku.hk/hkuto/record/B44205156.
Sun, Jian, and 孙健. "Computer-aided drug design for influenza A virus." Thesis, The University of Hong Kong (Pokfulam, Hong Kong), 2009. http://hub.hku.hk/bib/B44205156.
Klimenko, Kyrylo. "Computer-aided drug design of broad-spectrum antiviral compounds." Thesis, Strasbourg, 2017. http://www.theses.fr/2017STRAF008/document.
Tai, Hui-Chung. "Computer-aided drug design of photoactivated platinum anticancer complexes." Thesis, University of Warwick, 2011. http://wrap.warwick.ac.uk/55656/.
Khedr, Mohammed Abdou. "Computer-aided drug design and synthesis of novel antivirals." Thesis, Cardiff University, 2010. http://orca.cf.ac.uk/54378/.
Nomkoko, Thembelani Edmund. "Computer-aided chemical speciation in metal-based drug design." Doctoral thesis, University of Cape Town, 2002. http://hdl.handle.net/11427/21347.
Moorad, Razia. "Computer-aided drug design and the biological evaluation of anti-cancer drugs." Doctoral thesis, University of Cape Town, 2016. http://hdl.handle.net/11427/20715.
Ricketts, D. M. "Computer aided molecular design using a single user workstation." Thesis, University of Oxford, 1986. http://ethos.bl.uk/OrderDetails.do?uin=uk.bl.ethos.375319.
Du, Qishi. "Heuristic molecular lipophilicity potential for computer-aided rational drug design." Thesis, National Library of Canada = Bibliothèque nationale du Canada, 1998. http://www.collectionscanada.ca/obj/s4/f2/dsk2/ftp02/NQ27402.pdf.
Barakat, Maha Taysir. "Optimization of the atom assignment problem in computer-aided drug design." Thesis, University of Cambridge, 1993. http://ethos.bl.uk/OrderDetails.do?uin=uk.bl.ethos.309090.
Sturm, Noé. "Characterization of natural product biological imprints for computer-aided drug design applications." Thesis, Strasbourg, 2015. http://www.theses.fr/2015STRAF059/document.
Vlachakis, Dimitrios P. "Computer-aided drug design and biological evaluation of novel anti-viral agents." Thesis, Cardiff University, 2006. http://orca.cf.ac.uk/54324/.
Schumann, Marcel [Verfasser], and Oliver [Akademischer Betreuer] Kohlbacher. "New Approaches to Computer-Aided Drug Design / Marcel Schumann ; Betreuer: Oliver Kohlbacher." Tübingen : Universitätsbibliothek Tübingen, 2014. http://d-nb.info/1162897171/34.
Kumari, Vandana. "Structure-Based Computer Aided Drug Design and Analysis for Different Disease Targets." The Ohio State University, 2011. http://rave.ohiolink.edu/etdc/view?acc_num=osu1311612599.
Sturm, Noe Joseph. "Characterization of Natural Product Biological Imprints for Computer-aided Drug Design Applications." Thesis, Griffith University, 2016. http://hdl.handle.net/10072/367893.
Deanda, Felix. "Development and application of software tools for computer-assisted drug design /." Digital version accessible at:, 1999. http://wwwlib.umi.com/cr/utexas/main.
Athri, Prashanth. "Application of Computer-Aided Drug Discovery Methodologies Towards the Rational Design of Drugs Against Infectious Diseases." Digital Archive @ GSU, 2008. http://digitalarchive.gsu.edu/chemistry_diss/20.
Guasch, Pàmies Laura. "Identification of natural products as antidiabetic agents using computer-aided drug design methods." Doctoral thesis, Universitat Rovira i Virgili, 2011. http://hdl.handle.net/10803/111094.
Wassef, Abram. "Computer aided drug discovery: Design, synthesis and testing of novel anti-cancer agents." Thesis, The University of Sydney, 2015. http://hdl.handle.net/2123/14546.
HU, XIAO. "EXPLORING THE SPACE OF COMPUTER-AIDED DRUG DESIGN - MODULATOR DESIGNS TARGETING PFKFB3 AND COMPUTATIONAL WORKFLOW DEVELOPMENTS." Doctoral thesis, Università degli Studi di Milano, 2019. http://hdl.handle.net/2434/645883.
HU, XIAO. "Exploring the Space of Computer-Aided Drug Design - Modulator Designs Targeting PFKFB3 and Computational Workflow Developments." Doctoral thesis, Università degli Studi di Milano, 2019. http://hdl.handle.net/2434/645871.
Hu, Lihong, and 胡麗紅. "Application of neural networks in the first principles calculations and computer-aided drug design." Thesis, The University of Hong Kong (Pokfulam, Hong Kong), 2004. http://hub.hku.hk/bib/B45014796.
Hu, Lihong. "Application of neural networks in the first principles calculations and computer-aided drug design /." View the Table of Contents & Abstract, 2004. http://sunzi.lib.hku.hk/hkuto/record/B30575503.
García, Sosa Alfonso Tlatoani. "A new approach to the use of water molecules in computer-aided drug design." Thesis, University of Cambridge, 2004. https://www.repository.cam.ac.uk/handle/1810/275269.
CHHABRA, MONICA. "Modeling and Analysis of Ligand Docking to Norovirus Capsid Protein for the Computer-Aided Drug Design." University of Cincinnati / OhioLINK, 2008. http://rave.ohiolink.edu/etdc/view?acc_num=ucin1209001634.
Zarnecka, J. M. "Assessing and developing methods to explore the role of molecular shape in computer-aided drug design." Thesis, Liverpool John Moores University, 2018. http://researchonline.ljmu.ac.uk/8888/.
Gerogiokas, Georgios. "Quantitative models of biomolecular hydration thermodynamics." Thesis, University of Edinburgh, 2015. http://hdl.handle.net/1842/14181.
Kramar, S. "Computer-aided drug design of Keap1-Nrf2 inhibitors and the effect of physicochemical parameters on biomolecule interactions." Thesis, University College London (University of London), 2014. http://discovery.ucl.ac.uk/1455631/.
Tripathi, Ashutosh. "DEVELOPMENT OF HINT BASED COMPUTATIONAL TOOLS FOR DRUG DESIGN: APPLICATIONS IN THE DESIGN AND DEVELOPMENT OF NOVEL ANTI-CANCER AGENTS." VCU Scholars Compass, 2009. http://scholarscompass.vcu.edu/etd/1866.
Lundborg, Magnus. "Computer-Assisted Carbohydrate Structural Studies and Drug Discovery." Doctoral thesis, Stockholms universitet, Institutionen för organisk kemi, 2011. http://urn.kb.se/resolve?urn=urn:nbn:se:su:diva-56411.
Shi, Guqin. "Structure-based Computer-aided Drug Design and Analyses against Disease Target: Cytokine IL-6/IL-6R/GP130 Complex." The Ohio State University, 2017. http://rave.ohiolink.edu/etdc/view?acc_num=osu151197172881965.
Llorach, Parés Laura. "Computer-Aided Drug Design applied to marine drug discovery = Disseny de fàrmacs assistit per ordinador aplicat a la cerca de possibles fàrmacs marins." Doctoral thesis, Universitat de Barcelona, 2019. http://hdl.handle.net/10803/668298.
Thorsteinson, Nels. "Computational ligand discovery for the human and zebrafish sex hormone binding globulin." Thesis, University of British Columbia, 2008. http://hdl.handle.net/2429/943.
Mahasenan, Kiran V. "Discovery of novel small molecule enzyme inhibitors and receptor modulators through structure-based computational design." The Ohio State University, 2012. http://rave.ohiolink.edu/etdc/view?acc_num=osu1332367560.
Wacker, Sören [Verfasser], Bert de [Akademischer Betreuer] Groot, and Jörg [Akademischer Betreuer] Enderlein. "Computer-Aided Drug Design for Membrane Channel Proteins / Sören Wacker. Gutachter: Bert de Groot ; Jörg Enderlein. Betreuer: Bert de Groot." Göttingen : Niedersächsische Staats- und Universitätsbibliothek Göttingen, 2012. http://d-nb.info/1043611371/34.
Silva, Bárbara Athayde Vaz Galvão da. "Aplicação de metodologias do CADD (Computer-Aided Drug Design) a um conjunto de pirrolidina carboxamidas: mapeamento do farmacóforo e planejamento de novos protótipos tuberculostáticos potenciais." Universidade de São Paulo, 2012. http://www.teses.usp.br/teses/disponiveis/9/9138/tde-10032013-232818/.
Cockroft, Nicholas T. "Applications of Cheminformatics for the Analysis of Proteolysis Targeting Chimeras and the Development of Natural Product Computational Target Fishing Models." The Ohio State University, 2019. http://rave.ohiolink.edu/etdc/view?acc_num=osu156596730476322.
Boudon, Alain. "Drug design : techniques de modelisation moleculaire : application a l'etude des antagonistes du recepteur dopaminergique." Paris 7, 1988. http://www.theses.fr/1988PA077178.
Chang, Cheng. "In silico approaches for studying transporter and receptor structure-activity relationships." Connect to this title online, 2005. http://rave.ohiolink.edu/etdc/view?acc%5Fnum=osu1117553995.
Raymond, Justine. "Applications of Flow Chemistry Methods and Computer-Aided Approaches to Expedite the Development of HBV Inhibitors." Doctoral thesis, Universitat Rovira i Virgili, 2021. http://hdl.handle.net/10803/672255.
Rachman, Moira. "Fragment-to-Lead Optimization with Automated and Iterative Virtual Screening." Doctoral thesis, Universitat de Barcelona, 2020. http://hdl.handle.net/10803/671756.
Rodríguez, Torrecillas Ivan 1979. "Molecular basis to human P-glycoprotein reversion." Doctoral thesis, Universitat Pompeu Fabra, 2015. http://hdl.handle.net/10803/586099.
Gianella-Borradori, Matteo Luca. "The identification & optimisation of endogenous signalling pathway modulators." Thesis, University of Oxford, 2013. http://ora.ox.ac.uk/objects/uuid:4c87de5d-24a7-4998-8edb-917c3922aae1.
Swedberg, Joakim Erik. "Rational design of serine protease inhibitors." Thesis, Queensland University of Technology, 2011. https://eprints.qut.edu.au/48131/1/Joakim_Swedberg_Thesis.pdf.
Ward, D. J. "Further development of methods for the computer-aided design of neuropeptide-based drugs." Thesis, University of Manchester, 1990. http://ethos.bl.uk/OrderDetails.do?uin=uk.bl.ethos.280534.
GULOTTA, Maria Rita. "Computational methodologies applied to Protein-Protein Interactions for molecular insights in Medicinal Chemistry." Doctoral thesis, Università degli Studi di Palermo, 2021. http://hdl.handle.net/10447/479127.
Gutiérrez, de Terán Castañón Hugo. "Modelización molecular de los receptores de adenosina y sus ligandos en el marco de diseño de fármacos asistido por ordenador." Doctoral thesis, Universitat Pompeu Fabra, 2004. http://hdl.handle.net/10803/7075.
Lee, Tak-yee. "Part 1: Computer aided dosage form design: theory and applications. Part 2: Kinetics and mechanism of captopril oxidation in aqueous solutions under controlled oxygen partial pressure /." The Ohio State University, 1986. http://rave.ohiolink.edu/etdc/view?acc_num=osu1487266011224445.
Skone, Gwyn S. "Stratagems for effective function evaluation in computational chemistry." Thesis, University of Oxford, 2010. http://ora.ox.ac.uk/objects/uuid:8843465b-3e5f-45d9-a973-3b27949407ef.

Computer-Aided Drug Discovery and Design: Recent Advances and Future Prospects

Series: Methods In Molecular Biology > Book: Computational Drug Discovery and Design
Overview | DOI: 10.1007/978-1-0716-3441-7_1
- Laboratory of Bioactive Compound Research and Development (LIDeB), Faculty of Exact Sciences, National University of La Plata (UNLP), La Plata, Argentina
- Argentinean National Council of Scientific and Technical Research (CONICET), La Plata, Argentina

Computer-aided drug discovery and design involve the use of information technologies to identify and develop, on a rational ground, chemical compounds that align a set of desired physicochemical and biological properties. In its most common form, it
Computer-aided drug discovery and design involve the use of information technologies to identify and develop, on a rational ground, chemical compounds that align a set of desired physicochemical and biological properties. In its most common form, it involves the identification and/or modification of an active scaffold (or the combination of known active scaffolds), although de novo drug design from scratch is also possible. Traditionally, the drug discovery and design processes have focused on the molecular determinants of the interactions between drug candidates and their known or intended pharmacological target(s). Nevertheless, in modern times, drug discovery and design are conceived as a particularly complex multiparameter optimization task, due to the complicated, often conflicting, property requirements. This chapter provides an updated overview of in silico approaches for identifying active scaffolds and guiding the subsequent optimization process. Recent groundbreaking advances in the field have also analyzed the integration of state-of-the-art machine learning approaches in every step of the drug discovery process (from prediction of target structure to customized molecular docking scoring functions), integration of multilevel omics data, and the use of a diversity of computational approaches to assist target validation and assess plausible binding pockets.
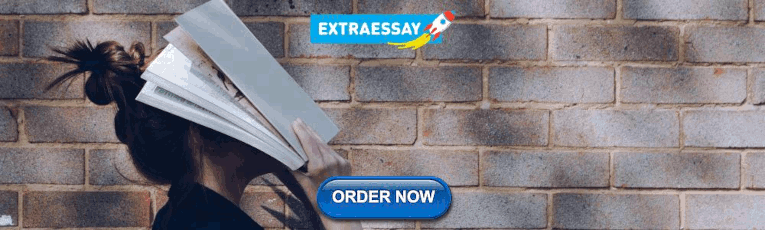
Figures ( 0 ) & Videos ( 0 )
Experimental specifications, other keywords.

Related articles
A practical guide to machine-learning scoring for structure-based virtual screening, artificial intelligence in compound design, artificial intelligence, machine learning, and deep learning in real-life drug design cases, artificial intelligence–enabled de novo design of novel compounds that are synthesizable, deep learning and computational chemistry, deep learning in structure-based drug design, learning strategies in protein directed evolution, ultrahigh throughput protein–ligand docking with deep learning, structural characterization of membrane protein dimers, computational modeling of multi-target-directed inhibitors against alzheimer’s disease.
- Klabunde T, Everts A (2005) GPCR antitarget modeling: pharmacophore models for biogenic amine binding GPCRs to avoid GPCR-mediated side effects. Chembiochem 6:876–889
- Raschi E, Vasina V, Poluzzi E et al (2008) The hERG K+ channel: target and antitarget strategies in drug development. Pharmacol Res 57:181–195
- Crivori P (2008) Computational models for P-glycoprotein substrates and inhibitors. In: Vaz RJ, Klabunde T (eds) Anti-atrgets: prediction and prevention of drug side effects. Wiley-VCH, Weinheim
- Zamora I (2008) Site of metabolism predictions: facts and experiences. In: Vaz RJ, Klabunde T (eds) Anti-targets: prediction and prevention of drug side effects. Wiley-VCH, Weinheim
- Fallico M, Alberca LN, Prada Gori DN et al (2022) Machine learning search of novel selective NaV1.2 and NaV1.6 inhibitors as potential treatment against Dravet syndrome. In: Ribeiro PRDA, Cota VR, Barone DAC, de Oliveira ACM (eds) Computational neuroscience. LAWCN 2021. Communications in computer and information science, vol 1519. Springer, Cham
- Fatoba AJ, Okpeku M, Adeleke MA (2021) Subtractive genomics approach for identification of novel therapeutic drug targets in Mycoplasma genitalium . Pathogens 10:921
- Süntar I (2020) Importance of ethnopharmacological studies in drug discovery: role of medicinal plants. Phytochem Rev 19:1199–1209
- Entzeroth M, Flotow H, Condron P (2009) Overview of high-throughput screening. Curr Protoc Pharmacol Chapter 9:Unit 9.4
- Maia EHB, Assis LC, de Oliveira TA et al (2020) Structure-based virtual screening: from classical to artificial intelligence. Front Chem 8:343
- Mouchlis VD, Afantitis A, Serra A et al (2021) Advances in de novo drug design: from conventional to machine learning methods. Int J Mol Sci 22:1676
- Kirsch P, Hartman AM, Hirsch AKH et al (2019) Concepts and core principles of fragment-based drug design. Molecules 24:4309
- Romano P, Giugno R, Pulvirenti A (2011) Tools and collaborative environments for bioinformatics research. Brief Bioinform 12:549–561
- Gorgulla C, Boeszoermenyi A, Wang ZF et al (2020) An open-source drug discovery platform enables ultra-large virtual screens. Nature 580:663–668
- Cox PB, Gupta R (2022) Contemporary computational applications and tools in drug discovery. ACS Med Chem Lett 13:1016–1029
- Prada Gori DN, Alberca LN, Rodriguez S et al (2022) LIDeB Tools: a Latin American resource of freely available, open-source cheminformatics apps. Artif Intell Life Sci 2:10049
- Hartenfeller M, Schneider G (2011) De novo drug design. Methods Mol Biol 672:299–323
- Kuttruff CA, Eastgate MD, Baran PS (2014) Natural product synthesis in the age of scalability. Nat Prod Rep 31:419–432
- Nicolaou CA, Brown N (2013) Multi-objective optimization methods in drug design. Drug Discov Today Technol 10:e427–e435
- Talevi A (2016) Tailored multi-target agents. Applications and design considerations. Curr Pharm Des 22:3164–3170
- Lipinski CA, Lombardo F, Dominy BW, Feeney PJ (1997) Experimental and computational approaches to estimate solubility and permeability in drug discovery and development settings. Adv Drug Deliv Rev 23:3–25
- Pajouhesh H, Lenz GR (2005) Medicinal chemical properties of successful central nervous system drugs. NeuroRx 2:542–553
- Gupta S, Kesarla R, Omri A (2013) Formulation strategies to improve the bioavailability of poorly absorbed drugs with special emphasis on self-emulsifying systems. ISRN Pharm 2013:848043
- Miller DC, Klute W, Calabrese A et al (2009) Optimising metabolic stability in lipophilic chemical space: the identification of a metabolic stable pyrazolopyrimidine CRF-1 receptor antagonist. Bioorg Med Chem Lett 19:6144–6147
- Wager TT, Hou X, Verhoest PR et al (2016) Central nervous system multiparameter optimization desirability: application in drug discovery. ACS Chem Neurosci 7:767–775
- Glen RC, Galloway WR, Spring DR et al (2016) Multiple-parameter optimization in drug discovery: example of the 5-HT1B GPCR. Mol Inform 35:599–605
- Ghose AK, Ott GR, Hudkins RL (2017) Technically Extended MultiParameter Optimization (TEMPO): an advanced robust scoring scheme to calculate central nervous system druggability and monitor lead optimization. ACS Chem Neurosci 8:147–154
- Winter R, Montanari F, Steffen A et al (2019) Efficient multi-objective molecular optimization in a continuous latent space. Chem Sci 10:8016–8024
- Pennington LD, Muegge I (2021) Holistic drug design for multiparameter optimization in modern small molecule drug discovery. Bioorg Med Chem Lett 41:128003
- He X (2009) Integration of physical, chemical, mechanical and biopharmaceutical properties in solid dosage oral form development. In: Solid dosage oral forms: pharmaceutical theory and practice. Academic Press, Burlington
- Csermely P, Korcsmáros T, Kiss HJ et al (2013) Structure and dynamics of molecular networks: a novel paradigm of drug discovery: a comprehensive review. Pharmacol Ther 138:333–408
- Wang J, Guo Z, Fu Y et al (2017) Weak-binding molecules are not drugs?-toward a systematic strategy for finding effective weak-binding drugs. Brief Bioinform 18:321–332
- Talevi A (2022) Antiseizure medication discovery: recent and future paradigm shifts. Epilepsia Open 7(Suppl 1):S133–S141
- Gashaw I, Ellinghaus P, Sommer A et al (2011) What makes a good drug target. Drug Discov Today 16:1037–1043
- Knowles J, Gromo G (2003) Target selection in drug discovery. Nat Rev Drug Discov 2:63–69
- Schmidtke P, Barril X (2010) Understanding and predicting druggability. A high-throughput method for detection of drug binding sites. J Med Chem 53:5858–5867
- Yuan Y, Pei J, Lai L (2013) Binding site detection and druggability prediction of protein targets for structure-based drug design. Curr Pharm Des 19:2326–2333
- Barril X (2013) Druggability predictions: methods, limitations and applications. Wires Comput Mol Sci 3:327–338
- Talevi A, Carrillo C, Comini M (2019) The thiol-polyamine metabolism of Trypanosoma cruzi : molecular targets and drug repurposing strategies. Curr Med Chem 26:6614–6635
- Tonge PJ (2018) Drug-target kinetics in drug discovery. ACS Chem Neurosci 9:29–39
- Feng Y, Wang Q, Wang T (2017) Drug target protein-protein interaction networks: a systematic perspective. Biomed Res Int 2017:1289259
- Viacava Follis A (2021) Centrality of drug targets in protein networks. BMC Bioinf 22:527
- Sabetian S, Shamsir MS (2019) Computer aided analysis of disease linked protein networks. Bioinformation 15:513–522
- Casas AI, Hassan AA, Larsen SJ et al (2019) From single drug targets to synergistic network pharmacology in ischemic stroke. Proc Natl Acad Sci U S A 116:7129–7136
- Schidlitzki A, Bascuñana P, Srivastava PK et al (2020) Proof-of-concept that network pharmacology is effective to modify development of acquired temporal lobe epilepsy. Neurobiol Dis 134:104664
- Kim B, Jo J, Han J et al (2017) In silico re-identification of properties of drug target proteins. BMC Bioinf 18:248
- Dezső Z, Ceccarelli M (2020) Machine learning prediction of oncology drug targets based on protein and network properties. BMC Bioinf 21:104
- Chen S, Jiang H, Cao Y et al (2016) Drug target identification using network analysis: taking active components in Sini decoction as an example. Sci Rep 6:24245
- Ji X, Freudenberg JM, Agarwal P (2019) Integrating biological networks for drug target prediction and prioritization. Methods Mol Biol 1903:203–218
- Capra JA, Singh M (2007) Predicting functionally important residues from sequence conservation. Bioinformatics 23:1875–1882
- Yang J, Roy A, Zhang Y (2013) Protein-ligand binding site recognition using complementary binding-specific substructure comparison and sequence profile alignment. Bioinformatics 29:2588–2595
- Han B, Salituro FG, Blanco MJ (2020) Impact of allosteric modulation in drug discovery: innovation in emerging chemical modalities. ACS Med Chem Lett 11:1810–1819
- Liu T, Ish-Shalom S, Torng W et al (2018) Biological and functional relevance of CASP predictions. Proteins 86(Suppl 1):374–386
- Clark JJ, Orban ZJ, Carlson HA (2020) Predicting binding sites from unbound versus bound protein structures. Sci Rep 10:15856
- Kuzmanic A, Bowman GR, Juarez-Jimenez J et al (2020) Investigating cryptic binding sites by molecular dynamics simulations. Acc Chem Res 53:654–661
- Smith RD, Carlson HA (2021) Identification of cryptic binding sites using MixMD with standard and accelerated molecular dynamics. J Chem Inf Model 61:1287–1299
- Paul F, Weikl TR (2016) How to distinguish conformational selection and induced fit based on chemical relaxation rates. PLoS Comput Biol 12:e1005067
- Vajda S, Beglov D, Wakefield AE et al (2018) Cryptic binding sites on proteins: definition, detection, and druggability. Curr Opin Chem Biol 44:1–8
- Martinez-Rosell G, Lovera S, Sands ZA et al (2020) PlayMolecule CrypticScout: predicting protein cryptic sites using mixed-solvent molecular simulations. J Chem Inf Model 60:2314–2324
- Zheng W (2021) Predicting cryptic ligand binding sites based on normal modes guided conformational sampling. Proteins 89:416–426
- Aromolaran O, Aromolaran D, Isewon I et al (2021) Machine learning approach to gene essentiality prediction: a review. Brief Bioinform 22(5):bbab128
- Basler G (2015) Computational prediction of essential metabolic genes using constraint-based approaches. Gene Essentiality 1279:183–204
- Pushpakom S, Iorio F, Eyers PA et al (2019) Drug repurposing: progress, challenges and recommendations. Nat Rev Drug Discov 18:41–58
- Talevi A, Bellera CL (2020) Challenges and opportunities with drug repurposing: finding strategies to find alternative uses of therapeutics. Expert Opin Drug Discov 15:397–401
- Szymanski P, Markowicz M, Mikiciuk-Olasik E (2012) Adaptation of high-throughput screening in drug discovery – toxicological screening. Int J Mol Sci 13:427–452
- Harris CJ, Hill RD, Sheppard DW, Slater MJ, Stouten PF (2011) The design and application of target-focused compound libraries. Comb Chem High Throughput Screen 14(6):521–531
- Welsch ME, Snyder SA, Stockwell BR (2010) Privileged scaffolds for library design and drug discovery. Curr Opin Chem Biol 14:347–361
- Jumper J, Evans R, Pritzel A et al (2021) Highly accurate protein structure prediction with AlphaFold. Nature 596:583–589
- Baek M, DiMaio F, Anishchenko I et al (2021) Accurate prediction of protein structures and interactions using a three-track neural network. Science 373:871–876
- Lin Z, Akin H, Rao R et al (2022) Language models of protein sequences at the scale of evolution enable accurate structure prediction. bioRxiv 2022.07.20.500902
- Mirdita M, Schütze K, Moriwaki Y et al (2022) ColabFold: making protein folding accessible to all. Nat Methods 19:679–682
- Varadi M, Anyango S, Deshpande M et al (2022) AlphaFold Protein Structure Database: massively expanding the structural coverage of protein-sequence space with high-accuracy models. Nucleic Acids Res 50:D439–D444
- Procacci P (2016) Reformulating the entropic contribution of molecular docking scoring functions. J Comput Chem 37(19):1819–1827
- Gilson MK, Zhou HX (2007) Calculation of protein-ligand binding affinities. Annu Rev Biophys Biomol Struct 36:21–42
- Bello M, Martínez-Archundia M, Correa-Basurto J (2013) Automated docking for novel drug discovery. Expert Opin Drug Discov 8:821–834
- Bodnarchuck MS (2016) Water, water, everywhere… It’s time to stop and think. Drug Discov Today 21:1139–1146
- Mysinger MM, Schoichet BK (2010) Rapid context-dependent ligand desolvation in molecular docking. J Chem Inf Model 50:1561–1573
- Li H, Sze KH, Lu G et al (2020) Machine-learning scoring functions for structure-based virtual screening. Wires Comput Mol Sci 11:e1478
- Zhang X, Shen C, Guo X et al (2021) ASFP (Artificial Intelligence based Scoring Function Platform): a web server for the development of customized scoring functions. J Cheminform 13:6
- Yang C, Chen EA, Zhang Y (2022) Protein-ligand docking in the machine-learning era. Molecules 27:4568
- Ge H, Wang Y, Li C et al (2013) Molecular dynamics-based virtual screening: accelerating the drug discovery process by high-performance computing. J Chem Inf Model 53:2757–2764
- Wang L, Wu Y, Deng Y et al (2015) Accurate and reliable prediction of relative ligand binding potency in prospective drug discovery by way of a modern free-energy calculation protocol and force field. J Am Chem Soc 137:2695–2703
- Llanos MA, Alberca LN, Larrea SCV et al (2022) Homology modeling and molecular dynamics simulations of Trypanosoma cruzi phosphodiesterase b1. Chem Biodivers 19:e202100712
- Lavechia A (2015) Machine-learning approaches in drug discovery: methods and applications. Drug Discov Today 20:318–331
- Lemmen C, Zimmermann M, Lengauer T (2002) Multiple molecular superpositioning as an effective tool for virtual database screening. In: Virtual screening: an alternative or complement to high-throughput screening? 1st edn. Kluwer Academic Publishers, Marburg
- Kristensen TG, Nielsen J, Pedersen CNS (2013) Methods for similarity-based virtual screening. Comput Struct Biotechnol J 5:e201302009
- Talevi A, Bruno-Blanch LE (2016) Virtual screening applications in the search of novel antiepileptic drug candidates. In: Antiepileptic drug discovery. Novel approaches. Humana Press, New York
- Schneidman-Duhovny D, Dror O, Inbar Y et al (2008) Deterministic pharmacophore detection via multiple flexible alignment of drug-like molecules. J Comput Biol 15:737–754
- Cottrell SJ, Gillet VJ, Taylor R et al (2004) Generation of multiple pharmacophore hypothesis using multiobjective optimization techniques. J Comput Aided Mol Des 18:665–682
- Pirhadi S, Shiri F, Ghasemi JB (2013) Methods and applications of structure based pharmacophores in drug discovery. Curr Top Med Chem 13:1036–1047
- Zhang Q, Muegge I (2006) Scaffold hopping through virtual screening using 2D and 3D similarity descriptors: ranking, voting, and consensus scoring. J Med Chem 9:1536–1548
- Krüger DM, Evers A (2010) Comparison of structure- and ligand-based virtual screening protocols considering hit list complementarity and enrichment factors. ChemMedChem 5:148–158
- Talevi A, Gavernet L, Bruno-Blanch LE (2009) Combined virtual screening strategies. Curr Comput Aided Drug Des 5:23–37
- Pouliot M, Jeanmart S (2016) Pan Assay Interference Compounds (PAINS) and other promiscuous compounds in antifungal research. J Med Chem 59:497–503
- Walters WP, Stahl MT, Murcko MA (1998) Virtual screening – an overview. Drug Discov Today 3:160–178
- Zhu T, Cao S, Su PC et al (2013) Hit identification and optimization in virtual screening: practical recommendations based upon a critical literature analysis. J Med Chem 56:6560–6572
- Ripphausen P, Nisius B, Pletason L et al (2010) Quo vadis, virtual screening? A comprehensive survey of prospective applications. J Med Chem 53:8461–8467
- Neetoo-Isseliee Z, MacKenzie AE, Southern C et al (2013) High-throughput identification and characterization of novel, species-selective GPR35 agonists. J Pharmacol Exp Ther 344:568–578
- Kola I, Landis J (2004) Can the pharmaceutical industry reduce attrition rates? Nat Rev Drug Discov 3:711–716
- Schuster D, Laggner C, Langer T (2005) Why drugs fail – a study on side effects in new chemical entities. Curr Pharm Des 11:3545–3559
- Talevi A (2016) Computational approaches for innovative antiepileptic drug discovery. Expert Opin Drug Discov 11:1001–1016
- Wang S, Dong G, Sheng C (2019) Structural simplification of natural products. Chem Rev 119:4180–4220
- Brown N, Lewis RA (2006) Exploiting QSAR methods in lead optimization. Curr Opin Drug Discov Devel 9:419–424
- Wong WWL, Burkowski FJ (2009) A constructive approach for discovering new drug leads: using a kernel methodology for the inverse-QSAR problem. J Cheminform 1:4
- Miyako T, Kaneko H, Funatsu K (2016) Inverse QSPR/QSAR analysis for chemical structure generation (from y to x). J Chem Inf Model 56:286–299
- Waring MJ, Arrowsmith J, Leach AR et al (2015) An analysis of the attrition of drug candidates from four major pharmaceutical companies. Nat Rev Drug Discov 14:475–486
- Cook D, Brown D, Alexander R et al (2014) Lessons learned from the fate of AstraZeneca’s drug pipeline: a five-dimensional framework. Nat Rev Drug Discov 13:419–431
- Roberts RA, Kavanagh SL, Mellor HR et al (2014) Reducing attrition in drug development: smart loading preclinical safety assessment. Drug Discov Today 19:341–347
- Veber DF, Johnson SR, Cheng HY et al (2002) Molecular properties that influence the oral bioavailability of drug candidates. J Med Chem 45:2615–2623
- Price DA, Blagg J, Jones L et al (2009) Physicochemical drug properties associated with in vivo toxicological outcomes: a review. Expert Opin Drug Metab Toxicol 5:921–931
- Sutherland JJ, Raymond JW, Stevens JL et al (2012) Relating molecular properties and in vitro assay results to in vivo drug disposition and toxicity outcomes. J Med Chem 55:6455–6466
- Doak BC, Zheng J, Dobritzsch D et al (2016) How beyond rule of 5 drugs and clinical candidates bind to their targets. J Med Chem 59:2312–2327
- Doak BC, Over B, Giordanetto F et al (2014) Oral druggable space beyond the rule of 5: insights from drugs and clinical candidates. Chem Biol 21:1115–1142
- Lipinski CA (2016) Rule of five in 2015 and beyond: target and ligand structural limitations, ligand chemistry structure and drug discovery project decisions. Adv Drug Deliv Rev 101:34–41
- Bergström CAS, Charman WN, Porter CJH (2016) Computational prediction of formulation strategies for beyond-rule-of-5 compounds. Adv Drug Deliv Rev 101:6–21
Advertisement

An official website of the United States government
The .gov means it’s official. Federal government websites often end in .gov or .mil. Before sharing sensitive information, make sure you’re on a federal government site.
The site is secure. The https:// ensures that you are connecting to the official website and that any information you provide is encrypted and transmitted securely.
- Publications
- Account settings
Preview improvements coming to the PMC website in October 2024. Learn More or Try it out now .
- Advanced Search
- Journal List
- Curr Neuropharmacol
- v.16(6); 2018 Jul
Computer Aided Drug Design and its Application to the Development of Potential Drugs for Neurodegenerative Disorders
Mohammad hassan baig, khurshid ahmad, gulam rabbani, mohd danishuddin.
Neurodegenerative disorders (NDs) are diverse group of disorders characterized by escalating loss of neurons (structural and functional). The development of potential therapeutics for NDs presents an important challenge, as traditional treatments are inefficient and usually are unable to stop or retard the process of neurodegeneration. Computer-Aided Drug Design (CADD) has emerged as an efficient means of developing candidate drugs for the treatment of many disease types. Applications of CADD approach to drug discovery are progressing day by day. The recent tendency in drug design is to rationally design potent therapeutics with multi-targeting effects, higher efficacies, and fewer side effects, especially in terms of toxicity.
A wide literature search was performed for writing this review. An updated view on different types of NDs, their effect on human population and a brief introduction to CADD, various approaches involved in this technique, ranging from structural-based to ligand-based drug design has been discussed. The successful application of CADD approaches for the treatment of neurodegenerative disorders is also included in this review.
In this review, we have briefly described about CADD and its use in the development of the therapeutic drug candidates against NDs. The successful applications, limitations and future prospects of this approach have also been discussed.
CADD can assist researchers studying interactions between drugs and receptors. We believe this review will be helpful for better understanding of CADD and its applications towards the discovery of new drug candidates against various fatal NDs.
1. BACKGROUND
The development of novel potential therapies for the treatment of NDs represents an important means of extending life span and quality of life in the elderly [ 1 ]. The development of potential therapeutics for NDs presents an important challenge, as traditional treatments are inefficient and usually are unable to stop or retard the process of neurodegeneration [ 2 ], which is highly complex and includes many neuropathological conditions and cognitive function losses, such as, memory and learning losses. NDs cause neuron loss and brain aging, which eventually lead to death. In fact, it has been estimated that more than 25% of global deaths and disabilities are caused by brain-associated disorders [ 3 ], such as, Alzheimer’s disease (AD), Parkinson’s disease (PD), Huntington’s disease (HD) and amyotrophic lateral sclerosis (ALS) [ 4 , 5 ]. Available treatments are limited, and no truly effective drug is available for many NDs. Furthermore, the number of drugs approved is limited by the high failure rates of lead compounds in clinical trials [ 6 ].
Identifying novel, potential drugs for NDs is difficult using traditional approaches of drug discovery [ 7 ]. However, during the last decade, computers have been used to aid and accelerate the process of drug discovery, and this process is now referred to as computer-aided drug design (CADD) or computer-assisted molecular design (CAMD).
Computer-Aided Drug Design (CADD) emerged as an efficient means of identifying potential lead compounds and for aiding the developments of possible drugs for a wide range of diseases [ 8 , 9 ]. Today, a number of computational approaches are being used to identify potential lead molecules from huge compound libraries.
Applications of CADD approach to drug discovery are progressing on a daily basis. The recent tendency in drug design is to rationally design potent therapeutics with
multi-targeting effects, higher efficacies, and fewer side effects, especially in terms of toxicity. In this review, we provide a brief introduction to CADD and include details of structure-based drug design (SBDD) and ligand-based drug design (LBDD), and their uses to identify potential drug candidates for NDs. In addition, we provide an up-to-date summary of the successes and limitations of CADD against NDs and discuss its future prospects.
2. COMPUTER AIDED DRUG DESIGN (CADD)
To introduce a new drug to the market is a costly affair that involves considerable time and money. The average time taken to discover/develop a drug is around 10-15 years and the cost stands at around US$ 800 million [ 10 - 12 ]. Not surprisingly, pharmaceutical companies focus on reducing development times and budgets without adversely affecting quality. In the 1990’s, a large number of developments were undertaken using combinatorial and high-throughput screening technologies, which accelerated drug discovery [ 13 - 15 ]. These technologies were widely adopted because they enabled the rapid synthesis and screening of large libraries, but unfortunately, no significant success was achieved and little progress toward the development of new molecular entities was made [ 16 , 17 ].
A combination of advanced computational techniques, biological science, and chemical synthesis was introduced to facilitate the discovery process, and this combinational approach enhanced the scale of discovery. Eventually, the term computer-aided drug design (CADD) was adopted for the use of computers in drug discovery [ 17 , 18 ]. Advanced computational applications have been shown to be effective tools and notable successes have been achieved using these techniques. CADD is a specialized discipline, whereby different computational methods are used to simulate interactions between receptors and drugs in order to determine binding affinities [ 19 ]. However, the technique is not limited to studies of chemical interactions and binding affinity predictions, as it has many more applications ranging from the design of compounds with desired physiochemical properties to the management of digital repositories of compounds. An overview of CADD is provided in Fig. ( 1 ) . CADD may be broadly categorized embracing both structure- and ligand-based drug design. Fig. ( 2 ) illustrates various approaches applied in CADD.

Overview of CADD process.

Various approaches applied in CADD.
Virtual screening (VS) is a computational technique used for screening large datasets of molecules, and has been successfully used to complement High Throughput Screening (HTS) for drug discovery [ 8 , 20 , 21 ]. The major aim of VS is to enable the rapid, cost-effective evaluation of huge virtual compound databases to screen for effective leads for synthesis and further study [ 22 ]. Virtual database screening can be applied to screen large libraries of compounds using various computational approaches to identify those entities likely to bind to a molecular target of interest [ 23 , 24 ]. To a large extent, VS mitigates the problem of drug synthesis because it utilizes large libraries of pre-synthesized compounds.
2.1. Structure-based Drug Design (SBDD)
Structure-based drug design utilizes protein three-dimensional (3D) structural information to design new biologically active molecules [ 25 ]. Thus, the identification of a target molecule and the determination of its structure is the main, initial step of SBDD [ 23 , 26 ]. The identified target may be an enzyme associated with a disease of interest. Based on binding affinity determinations, potential compounds are determined which attenuates the activity of target by its inhibition. Thus, SBDD utilizes information about a biological target and identifies potentially new medications. As such SBDD constitutes a marked advancement in the computational techniques used in the biophysics, medicinal chemistry, statistics, biochemistry, and other fields [ 27 ]. Scientific advancements have resulted in a large number of techniques for predicting protein structures. These state-of-the-art technologies enable the determination of the structures of large numbers of proteins by using cryo-electron microscopy (EM), nuclear magnetic resonance (NMR), X-ray crystallography and computational methods like homology modeling and molecular dynamic (MD) simulation [ 28 ].
2.1.1. Homology Modeling
Determining the structure of a target molecule follows the identification of a specific drug target [ 29 ]. Despite the availability of advanced techniques, the structures of a large number of proteins have not been identified [ 30 ]. Homology modelling helps in this situation because it can be used to generate the structures of proteins on information available for similar proteins [ 31 ].
Structural information about an identified target is a prerequisite for SBDD, but the structures of several identified neurodegenerative drug targets have yet not been determined [ 32 , 33 ]. A large number of studies have been conducted using the homology modelling approach to generate structures of identified target molecules. Structural information is also required to gain insights of protein activities. Dhanavade et al . generated the structure of cysteine protease, which degrades amyloid beta peptide, an important causative agent of Alzheimer’s disease [ 34 ]. Several in silico experiments have been conducted using the modeled structure of cysteine protease to investigate the nature of its binding site.
2.1.2. Molecular Docking
Molecular docking is a computational process widely used for rapidly predicting the binding modes and affinities of small molecules against their target molecules (usually proteins) [ 35 , 36 ]. This in silico process has achieved a position of great importance in the drug discovery field [ 21 , 36 - 38 ]. Molecular docking has emerged over the last two decades and is now considered an indispensable tool for CADD and in the structural biology field, and has been shown to be more efficient than traditional drug discovery methods. Molecular docking has been greatly facilitated by dramatic growth in computer power and the increasing availability of small molecule and protein databases. Fig. ( 3 ) illustrates the basic principle of molecular docking. Recent advancements in computer methods and access to 3D structural information of biological targets are set to increase the effectiveness of this technique and facilitate its large-scale application to studies of molecular interactions involved in ligand-protein binding. Generally, small molecules can be docked in three different ways, that is; (a) by rigid docking, where both target and ligands are treated as rigid entities; (b) by flexible docking, where both ligand and target are considered to be flexible; and (c) by flexible ligand docking, where the ligand is considered to be flexible and the target is considered rigid [ 39 ]. Many molecular docking programs have been developed during recent years, such as, AutoDock [ 40 ], Dock [ 41 ], FlexX [ 42 ], Glide [ 43 ], Gold [ 44 ], Surflex [ 45 ], ICM, and LigandFit [ 46 ], and been used successfully in many computer based drug discovery projects. Table 1 1 provides a list of major molecular docking tools in practice. Typically, the major goal of molecular docking is to identify ligands that bind most favorably within receptor binding sites and to determine its most energetically favored binding orientations (poses). The term “binding pose” is the orientation/confirmation of a ligand relative to its receptor. A binding pose either refers to a conformation of a ligand molecule within the binding site of its target protein which has been confirmed experimentally, or a computationally modelled hypothetical conformation. The search algorithm and the scoring function are two important components for determining protein-ligand interactions [ 47 ]. The search algorithm is responsible for searching different poses and conformations of a ligand within a given target protein and the scoring function estimates the binding affinities of generated poses, ranks them, and identifies the most favorable receptor/ligand binding modes [ 47 , 48 ]. An ideal search algorithm should be fast and effective, and the scoring function must be capable of determining the physicochemical properties of molecules and the thermodynamics of interactions.

Basic principle of molecular docking.
List of major available molecular docking tools.
A large number of trials are being conducted to identify binding modes of ligands and selection of the most energetically favored poses. In order to achieve this, molecular docking tools are used to generate a set of different ligand binding poses and a scoring function is used to estimate the binding affinities of generated poses to identify the best binding mode. The energy change caused by ligand/receptor complex formation, is given by the Gibbs free energy (ΔG) and the binding constant (Kd) [ 49 , 50 ]. The binding energy of a complex is predicted by evaluating physicochemical features involved in ligand-receptor binding, which include desolvation, intermolecular interactions, and entropic effects [ 51 ]. Sehgal et al . identified a number of compounds active against HSPB8 based on molecular docking results [ 52 ].
2.2. Ligand Based Drug Design (LBDD)
LBDD offers a general approach for elucidating relationships between the structural and physicochemical properties of compounds/ligands and their biological activities. This approach is applied when 3D structural information of a target protein is unavailable. In this process the available information of ligands and their biological activity is used for the development of new potential drug candidates. LBDD is widely used in pharmaceutical research, as more than 50% of approved drugs targeting membrane proteins (for which 3D structures are often not available, such as, GPCR). It is based on the assumption that compounds with similar structural features share common biological activities and interact/inhibit common target molecules [ 36 , 37 ].
The representation of molecules is the basis of LBDD approach. Molecular descriptors are numerical values used to represent the structural and physicochemical properties of molecules [ 53 , 54 ]. The molecular descriptor field is strikingly interdisciplinary and includes a number of different theories [ 55 ]. Active molecules are represented by the 0D-4D class of molecular descriptors [ 56 ]. Constitutional and count descriptors are 0D molecular descriptors, chemical fingerprints or lists of structural fragments, such as, SMILES and SLN, are 1D descriptors, graph invariants in which atoms are denoted as nodes and bonds as edges are 2D-descriptors, geometrical, WHIM and others are 3D descriptors, and those derived from CoMFA or DRID methods are classified as 4D descriptors [ 57 ]. Similarity searching is a key aspect of the LBDD method. This technique uses a known active compound as a query compound to find similar compounds and then rank compounds identified in a database. Based on this belief, structurally similar molecules exhibit similar biological activities and physicochemical properties. Numerical descriptors are applied and similarity coefficient is defined to quantify the degree of similarity (similarity/ dissimilarity). Fingerprint-based similarity or 2D similarity measures are widely used for similarity searching. A number of coefficients are applied in similarity searching with different fingerprint molecular databases (Cosine, Euclidian distance, Forbes, Tanimoto coefficients etc .).
The Tanimoto coefficient is most popular and widely accepted similarity index for binary variables, despite its well-documented size bias. It may be defined as:
where overlap ab , overlap aa , and overlap bb are the volume overlaps of molecule a with molecule b , of molecule a with itself, and of molecule b with itself, respectively. Values of T c range from 0 (no similarity) to 1 (a perfect match).
LBDD is generally categorized as Quantitative Structure Activity Relationship (QSAR) or pharmacophore modeling.
2.2.1. QSAR and its Role in Drug Discovery
The QSAR method and pharmacophore modeling are the most popular approaches to ligand-based drug design [ 58 , 59 ]. QSAR methods are based on the belief that molecular structures are directly associated with biological activities, and thus, that molecular or structural variations alter biological activities. QSAR is defined as a process involving the construction of computational or mathematical models using chemometric techniques to identify significant correlations between a series of structures and functions [ 60 ]. For QSAR, the primary hypothesis is that “compounds with similar structural or physiochemical properties show similar activities”. To identify potential leads, a library of lead compounds with the desired biological activities is produced. A model is then developed to predict the quantitative relation between the structural and physico-chemical features of these compounds and their biological activities. A statistical model generated using such relations is then used to mathematically optimize the biological properties of sets of compounds and maximize relevant biological activities. QSAR is used to modify existing compounds and improve their activities, and has been widely used in drug discovery to improve existing drugs for NDs. A study conducted by Dong et al . successfully designed a new series of PDE-4 inhibitors using the QSAR approach [ 61 ]. Bhadoriya et al . successfully implemented QSAR to the discovery of more potent anti-Alzheimer’s agents [ 62 ]. In latter, QSAR studies were carried out on a series of 34 fused 5,6-bicyclic heterocycles to identify the structural characteristics needed to inhibit Aβ42.
2.3. Pharmacophore Modelling
A pharmacophore is an assembly (3D arrangement) of 'steric' and 'electronic' features required for optimal supramolecular interaction with a specific biological target structure and to prompt/block its biological response [ 63 ]. Ligand-based pharmacophore model generation is based on available information on the biological activities of compounds/ligands. A pharmacophore does not symbolize an actual molecule/ligand or real connection between functional groups, but rather provides an abstract description of molecular features that are vital for molecular interactions between molecules and macromolecular ligands.
Pharmacophore modeling is widely used to identify potential lead molecules quickly. During the recent era of drug design, many therapeutically potent and well accepted drug targets with unknown active site geometries have been identified. Pharmacophore modeling provides an efficient means of rapidly screening huge databases of compounds. The elucidation of common pharmacophore features is conducted by aligning conformational models and active compounds three dimensionally. A superimposition algorithm assembles training set compounds (3D structure) in the same position/arrangement of their respective chemical properties/features. Pharmacophoric features are positioned such that all/maximum compounds share a common chemical functionality. To refine a shared pharmacophore feature, information regarding inactive compounds can be included in the model generation process. A number of tools and software have been developed for pharmacophore development, such as, Phase, Catalyst/Discovery Studio, MOE, and LigandScout [ 64 ].
3. SUCCESSFUL CADD APPROACHES TO THE TREATMENT OF NEURODEGENERATIVE DISORDERS
The success of CADD has resulted in its being recognized as an important technique in the research and pharmaceutical fields. There are many examples of the successful application of CADD, but here we describe its successes with respect to the design of drugs for the treatment of NDs. Amyloid-β is an important therapeutic target in Alzheimer’s disease [ 65 ]. Chen et al . used an in silico approach to study a series of peptides against the fibrillar form of Aβ, and reported two highly active compounds [ 66 ]. These peptides were subsequently found to inhibit the neurotoxic effects of Aβ on neuroblastoma cells.
BACE-1 is an enzyme that has been reported to be essential for β-amyloid generation [ 67 ]. Research suggests inhibition of this enzyme stops the production of β-amyloid, and thus, prevents NDs like Alzheimer's disease [ 68 ]. This finding has made BACE-1 an important therapeutic target for NDs. During the last few years, several computational approaches have been used to study the structural behavior of BACE-1 and to design their inhibitors [ 69 - 71 ].
ROCK-I and NOX2 are among the most attractive potential therapeutic targets for several NDs [ 72 - 75 ]. Inhibition of these two enzymes constitutes treatment for neurological diseases like autism spectral disorder, Alzheimer, and fragile X syndrome. Alokam et al . reported the successful use of CADD to design dual inhibitors for these enzymes [ 76 ], by employing a combination of pharmacophores and using a molecular docking approach to identify chemical entities. In vitro validation of selected chemical entities demonstrated their inhibitory potentials against ROCK-I and NOX2.
HDAC6 is a member of the class IIb Histone deacetylases (HDACs) family and is usually found in cytosol in association with non-histone proteins [ 77 , 78 ]. HDAC6 has been widely reported to be a crucial therapeutic drug target for several NDs [ 79 - 81 ]. The implementation of CADD has been reported to result in the design of a potential inhibitor of this enzyme. In one study conducted by Goracci et al ., a virtual screening approach was used to identify potential inhibitors for HDAC6, and these were then subjected to in vitro testing. The results obtained showed inhibitors had low cytotoxicities, suggesting potential for drug development [ 82 ]. Several other reports have described the successful use of CADD in the NDs.
4. Limitations
Despite a number of successful applications of CADD to modern drug design, it has its limitations. In particular, like any computer assisted hypothetical system results must be validated in actual systems, and many lead molecules identified using CADD have failed to exhibit desired activities in biological systems [ 83 , 84 ]. Several parameters must be met before potential compound to be approved as potent lead/drug, as it has to pass several pharmacological criteria. In fact, an average of only 40% of lead/drug candidates passes the different phases of clinical trials and obtains approval for clinical use.
Any computational tool based on pre-defined algorithms and scripts has its limitations, and the computational tools/methods used in CADD, such as, molecular docking, virtual screening, QSAR, pharmacophore modeling, and molecular dynamics, have their own limitations [ 49 , 85 - 88 ]. Furthermore, ADME and many toxicity prediction tools are not supported by solid experimental data, and many examples of the failure of these computational approaches can be found in the literature [ 89 , 90 ].
To overcome limitations and improve accuracy in terms of predicting potent leads, regular updates of tools and algorithms are needed. Database reliability and high quality validated experimental molecules is to be developed and updated because many pharmacophores do not pass biological activity process due to non-availability of good quality data sets. Databases should contain detail data on genomics and proteomics, high quality sequence information, physicochemical properties, and structures.
In the present era of drug discovery, the application of CADD counts up the most important accountability, and provides computational tools and algorithms that save time, costs, and reduce the risk of detecting non-viable developmental leads. The discovery of a new lead/drug using recent CADD paradigms requires a systematic understanding of the molecular and pathological conditions induced by diseases. Early diagnosis of NDs remains a huge challenge for researchers and clinicians. However, CADD can assist researchers studying interactions between drugs and receptors. The pharmacoinformatic approach is being applied to modern drug discovery and is providing much basic knowledge regarding drug-receptor interactions. Novel technologies and computational algorithms are required to move the CADD approach forward, as new developments are likely to lead to tools for disease identification and the screening of potential lead compounds. The emerging field of neurological studies, which includes neuroproteomics and neurogenomics, may aid understanding of the neuronal alterations associated with NDs. Furthermore, the application of technologies associated with neuroproteomics, neurogenomics, and next generation sequencing, and genome wide association studies may result in the identification of novel therapeutic targets and ultimately improve our ability to treat NDs.
Acknowledgements
This work was supported by the grant K16281 awarded to Korea Institute of Oriental Medicine (KIOM) from Ministry of Education, Science and Technology (MEST), Republic of Korea.
List OF Abbreviations
Consent for publication.
Not applicable.
Conflict of Interest
The authors declare no conflict of interest, financial or otherwise.
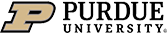
Applications of Deep Neural Networks in Computer-Aided Drug Design
Degree type.
- Doctor of Philosophy
- Medicinal Chemistry and Molecular Pharmacology
Campus location
- West Lafayette
Advisor/Supervisor/Committee Chair
Additional committee member 2, additional committee member 3, additional committee member 4, usage metrics.
- Computational chemistry
- Cheminformatics and quantitative structure-activity relationships
- Bioinformatics and computational biology not elsewhere classified
- Structural biology (incl. macromolecular modelling)

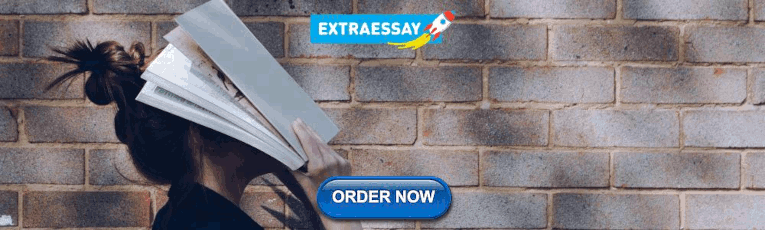
IMAGES
VIDEO
COMMENTS
The recent outbreak of the deadly coronavirus disease 19 (COVID-19) pandemic poses serious health concerns around the world. The lack of approved drugs or vaccines continues to be a challenge and further necessitates the discovery of new therapeutic molecules. Computer-aided drug design has helped to expedite the drug discovery and development ...
In the dynamic landscape of drug discovery, Computer-Aided Drug Design (CADD) emerges as a transformative force, bridging the realms of biology and technology. This paper overviews CADDs historical evolution, categorization into structure-based and ligand-based approaches, and its crucial role in rationalizing and expediting drug discovery. As CADD advances, incorporating diverse biological ...
Computer-aided drug design for choosing hit candidates in cancer therapy. In silico drug discovery has advanced in recent years. CADD have helped scientists simulate chemical systems, resolve 3D structures, optimize and develop new chemical compounds, and analyze the atomic processes of pharmaceuticals and naturally occurring molecules. ...
Computer-aided synthesis technology has laid the foundation for the automatic design, synthesis, testing and analysis of drug molecules. 3. Applications of computer-aided synthesis. AI, especially machine learning, plays an important role in the field of drug synthesis.
The thesis entitled "computer-aided drug design approaches in developing anti-cancer drug" is divided into a total of six chapters. In the first chapter, an overview of drug discovery and development are introduced. Nowadays, drug discovery and development has clearly changed
In order to increase efficiency of the pre-clinical drug discovery pathway, computational drug discovery methods and most recently, machine learning-based methods are increasingly used as powerful tools to aid early stage drug discovery. In this thesis, I present three complementary computer-aided drug discovery methods, with a focus on aiding ...
1 Introduction. Computer-aided drug discovery and design (CADDD) involve the use of information technologies to assist in the identification and/or development of novel chemical scaffolds with the desired alignment of relevant physicochemical and biological properties. Under the target-focused paradigm, computer-aided drug discovery and design ...
1 Introduction. Computer-aided drug discovery and design (CADDD) involve the use of information technologies to assist in the identification and/or development of novel chemical scaffolds with the desired alignment of relevant physicochemical and biological properties. Under the target-focused paradigm, computer-aided drug.
As a result, computer-aided drug design methods are now frequently utilized to improve the efficiency of the drug discovery and development process. Various CADD approaches are regarded as ...
Video (online) Consult the top 50 dissertations / theses for your research on the topic 'Computer-aided drug design.'. Next to every source in the list of references, there is an 'Add to bibliography' button. Press on it, and we will generate automatically the bibliographic reference to the chosen work in the citation style you need: APA, MLA ...
Computer-aided drug discovery (CADD) methods have substantially assisted in producing therapeutically important small compounds for over three decades. These techniques can be categorized as structure-based or ligand-based. In theory, high-throughput screening and structure-based approaches are similar. De novo ligand design, molecular docking ...
Computer-aided drug discovery and design involve the use of information technologies to identify and develop, on a rational ground, chemical compounds that align a set of desired physicochemical and biological properties. In its most common form, it involves the identification and/or modification of an active scaffold (or the combination of ...
The recent outbreak of the deadly coronavirus disease 19 (COVID-19) pandemic poses serious health concerns around the world. The lack of approved drugs or vaccines continues to be a challenge and further necessitates the discovery of new therapeutic molecules. Computer-aided drug design has helped to expedite the drug discovery and development process by minimizing the cost and time. In this ...
Computer-Aided Drug Design (CADD) has emerged as an efficient means of developing candidate drugs for the treatment of many disease types. Applications of CADD approach to drug discovery are progressing day by day. The recent tendency in drug design is to rationally design potent therapeutics with multi-targeting effects, higher efficacies, and ...
Computer-aided drug design (CADD) is an alternative in-silico tool in the field of drug development and discovery, which can quickly identify the most promising therapeutic candidate at a low cost ...
CADD is a modern computational technique used in the drug discovery process to identify and develop a potential lead [10, 14, 15]. Computer-aided drug design includes computational chemistry, molecular modeling, molecular design and rational drug design. CADD is being used to optimize identified leads. CADD techniques are gaining popularity and ...
A Bayesian network, also known as belief network, are graphical models, specifically a directed acyclic graph, in which the nodes are variables (in computer-aided drug design, these nodes might refer to molecular descriptors, activity data, or incidental information about an assay), and the edge weights are conditional dependencies. Bayesian ...
Here in this dissertation, three methods based on DNNs are discussed, tackling different problems in the field of computer-aided drug design.The first method described addresses the problem of prediction of hydration properties from 3D structures of proteins without requiring molecular dynamics simulations.
Computer-aided drug design is a pc era that designs a product and files the design's technique. CADD can also facilitate the manufacturing system through shifting unique diagrams of a products materials, methods, tolerances and dimensions with unique conventions for the product in query (2). It can be used to supply both two-dimensional or 3 ...
Introduction: Definition of CADD and systems pharmacology, the concept of "success stories". Computer-aided drug discovery (CADD) is a multidisciplinary field of scientific inquiry in the intersection of informatics and pharmacology. CADD employs computational methods to discover, develop, and analyze pharmaceutical agents and ...
Computer aided drug design is an efficient tool in the can find the most promising drug candidate in a very cost-effective way. It always provides a hope for betterment in drug discovery area. In the past years through Computer aided drug design many impressive researches are achieved so it will play a very much ...