- Bipolar Disorder
- Therapy Center
- When To See a Therapist
- Types of Therapy
- Best Online Therapy
- Best Couples Therapy
- Best Family Therapy
- Managing Stress
- Sleep and Dreaming
- Understanding Emotions
- Self-Improvement
- Healthy Relationships
- Student Resources
- Personality Types
- Guided Meditations
- Verywell Mind Insights
- 2023 Verywell Mind 25
- Mental Health in the Classroom
- Editorial Process
- Meet Our Review Board
- Crisis Support
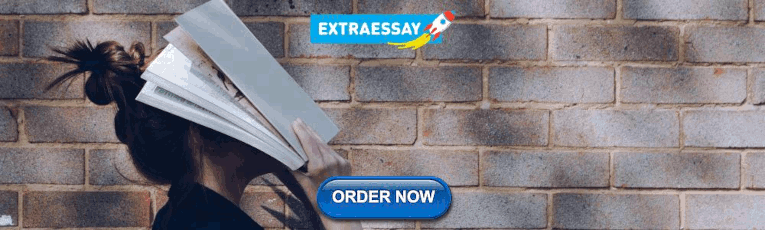
Correlation Studies in Psychology Research
Determining the relationship between two or more variables.
Kendra Cherry, MS, is a psychosocial rehabilitation specialist, psychology educator, and author of the "Everything Psychology Book."
:max_bytes(150000):strip_icc():format(webp)/IMG_9791-89504ab694d54b66bbd72cb84ffb860e.jpg)
Emily is a board-certified science editor who has worked with top digital publishing brands like Voices for Biodiversity, Study.com, GoodTherapy, Vox, and Verywell.
:max_bytes(150000):strip_icc():format(webp)/Emily-Swaim-1000-0f3197de18f74329aeffb690a177160c.jpg)
Verywell / Brianna Gilmartin
- Characteristics
Potential Pitfalls
Frequently asked questions.
A correlational study is a type of research design that looks at the relationships between two or more variables. Correlational studies are non-experimental, which means that the experimenter does not manipulate or control any of the variables.
A correlation refers to a relationship between two variables. Correlations can be strong or weak and positive or negative. Sometimes, there is no correlation.
There are three possible outcomes of a correlation study: a positive correlation, a negative correlation, or no correlation. Researchers can present the results using a numerical value called the correlation coefficient, a measure of the correlation strength. It can range from –1.00 (negative) to +1.00 (positive). A correlation coefficient of 0 indicates no correlation.
- Positive correlations : Both variables increase or decrease at the same time. A correlation coefficient close to +1.00 indicates a strong positive correlation.
- Negative correlations : As the amount of one variable increases, the other decreases (and vice versa). A correlation coefficient close to -1.00 indicates a strong negative correlation.
- No correlation : There is no relationship between the two variables. A correlation coefficient of 0 indicates no correlation.
Characteristics of a Correlational Study
Correlational studies are often used in psychology, as well as other fields like medicine. Correlational research is a preliminary way to gather information about a topic. The method is also useful if researchers are unable to perform an experiment.
Researchers use correlations to see if a relationship between two or more variables exists, but the variables themselves are not under the control of the researchers.
While correlational research can demonstrate a relationship between variables, it cannot prove that changing one variable will change another. In other words, correlational studies cannot prove cause-and-effect relationships.
When you encounter research that refers to a "link" or an "association" between two things, they are most likely talking about a correlational study.
Types of Correlational Research
There are three types of correlational research: naturalistic observation, the survey method, and archival research. Each type has its own purpose, as well as its pros and cons.
Naturalistic Observation
The naturalistic observation method involves observing and recording variables of interest in a natural setting without interference or manipulation.
Can inspire ideas for further research
Option if lab experiment not available
Variables are viewed in natural setting
Can be time-consuming and expensive
Extraneous variables can't be controlled
No scientific control of variables
Subjects might behave differently if aware of being observed
This method is well-suited to studies where researchers want to see how variables behave in their natural setting or state. Inspiration can then be drawn from the observations to inform future avenues of research.
In some cases, it might be the only method available to researchers; for example, if lab experimentation would be precluded by access, resources, or ethics. It might be preferable to not being able to conduct research at all, but the method can be costly and usually takes a lot of time.
Naturalistic observation presents several challenges for researchers. For one, it does not allow them to control or influence the variables in any way nor can they change any possible external variables.
However, this does not mean that researchers will get reliable data from watching the variables, or that the information they gather will be free from bias.
For example, study subjects might act differently if they know that they are being watched. The researchers might not be aware that the behavior that they are observing is not necessarily the subject's natural state (i.e., how they would act if they did not know they were being watched).
Researchers also need to be aware of their biases, which can affect the observation and interpretation of a subject's behavior.
Surveys and questionnaires are some of the most common methods used for psychological research. The survey method involves having a random sample of participants complete a survey, test, or questionnaire related to the variables of interest. Random sampling is vital to the generalizability of a survey's results.
Cheap, easy, and fast
Can collect large amounts of data in a short amount of time
Results can be affected by poor survey questions
Results can be affected by unrepresentative sample
Outcomes can be affected by participants
If researchers need to gather a large amount of data in a short period of time, a survey is likely to be the fastest, easiest, and cheapest option.
It's also a flexible method because it lets researchers create data-gathering tools that will help ensure they get the information they need (survey responses) from all the sources they want to use (a random sample of participants taking the survey).
Survey data might be cost-efficient and easy to get, but it has its downsides. For one, the data is not always reliable—particularly if the survey questions are poorly written or the overall design or delivery is weak. Data is also affected by specific faults, such as unrepresented or underrepresented samples .
The use of surveys relies on participants to provide useful data. Researchers need to be aware of the specific factors related to the people taking the survey that will affect its outcome.
For example, some people might struggle to understand the questions. A person might answer a particular way to try to please the researchers or to try to control how the researchers perceive them (such as trying to make themselves "look better").
Sometimes, respondents might not even realize that their answers are incorrect or misleading because of mistaken memories .
Archival Research
Many areas of psychological research benefit from analyzing studies that were conducted long ago by other researchers, as well as reviewing historical records and case studies.
For example, in an experiment known as "The Irritable Heart ," researchers used digitalized records containing information on American Civil War veterans to learn more about post-traumatic stress disorder (PTSD).
Large amount of data
Can be less expensive
Researchers cannot change participant behavior
Can be unreliable
Information might be missing
No control over data collection methods
Using records, databases, and libraries that are publicly accessible or accessible through their institution can help researchers who might not have a lot of money to support their research efforts.
Free and low-cost resources are available to researchers at all levels through academic institutions, museums, and data repositories around the world.
Another potential benefit is that these sources often provide an enormous amount of data that was collected over a very long period of time, which can give researchers a way to view trends, relationships, and outcomes related to their research.
While the inability to change variables can be a disadvantage of some methods, it can be a benefit of archival research. That said, using historical records or information that was collected a long time ago also presents challenges. For one, important information might be missing or incomplete and some aspects of older studies might not be useful to researchers in a modern context.
A primary issue with archival research is reliability. When reviewing old research, little information might be available about who conducted the research, how a study was designed, who participated in the research, as well as how data was collected and interpreted.
Researchers can also be presented with ethical quandaries—for example, should modern researchers use data from studies that were conducted unethically or with questionable ethics?
You've probably heard the phrase, "correlation does not equal causation." This means that while correlational research can suggest that there is a relationship between two variables, it cannot prove that one variable will change another.
For example, researchers might perform a correlational study that suggests there is a relationship between academic success and a person's self-esteem. However, the study cannot show that academic success changes a person's self-esteem.
To determine why the relationship exists, researchers would need to consider and experiment with other variables, such as the subject's social relationships, cognitive abilities, personality, and socioeconomic status.
The difference between a correlational study and an experimental study involves the manipulation of variables. Researchers do not manipulate variables in a correlational study, but they do control and systematically vary the independent variables in an experimental study. Correlational studies allow researchers to detect the presence and strength of a relationship between variables, while experimental studies allow researchers to look for cause and effect relationships.
If the study involves the systematic manipulation of the levels of a variable, it is an experimental study. If researchers are measuring what is already present without actually changing the variables, then is a correlational study.
The variables in a correlational study are what the researcher measures. Once measured, researchers can then use statistical analysis to determine the existence, strength, and direction of the relationship. However, while correlational studies can say that variable X and variable Y have a relationship, it does not mean that X causes Y.
The goal of correlational research is often to look for relationships, describe these relationships, and then make predictions. Such research can also often serve as a jumping off point for future experimental research.
Heath W. Psychology Research Methods . Cambridge University Press; 2018:134-156.
Schneider FW. Applied Social Psychology . 2nd ed. SAGE; 2012:50-53.
Curtis EA, Comiskey C, Dempsey O. Importance and use of correlational research . Nurse Researcher . 2016;23(6):20-25. doi:10.7748/nr.2016.e1382
Carpenter S. Visualizing Psychology . 3rd ed. John Wiley & Sons; 2012:14-30.
Pizarro J, Silver RC, Prause J. Physical and mental health costs of traumatic war experiences among civil war veterans . Arch Gen Psychiatry . 2006;63(2):193. doi:10.1001/archpsyc.63.2.193
Post SG. The echo of Nuremberg: Nazi data and ethics . J Med Ethics . 1991;17(1):42-44. doi:10.1136/jme.17.1.42
Lau F. Chapter 12 Methods for Correlational Studies . In: Lau F, Kuziemsky C, eds. Handbook of eHealth Evaluation: An Evidence-based Approach . University of Victoria.
Akoglu H. User's guide to correlation coefficients . Turk J Emerg Med . 2018;18(3):91-93. doi:10.1016/j.tjem.2018.08.001
Price PC. Research Methods in Psychology . California State University.
By Kendra Cherry, MSEd Kendra Cherry, MS, is a psychosocial rehabilitation specialist, psychology educator, and author of the "Everything Psychology Book."
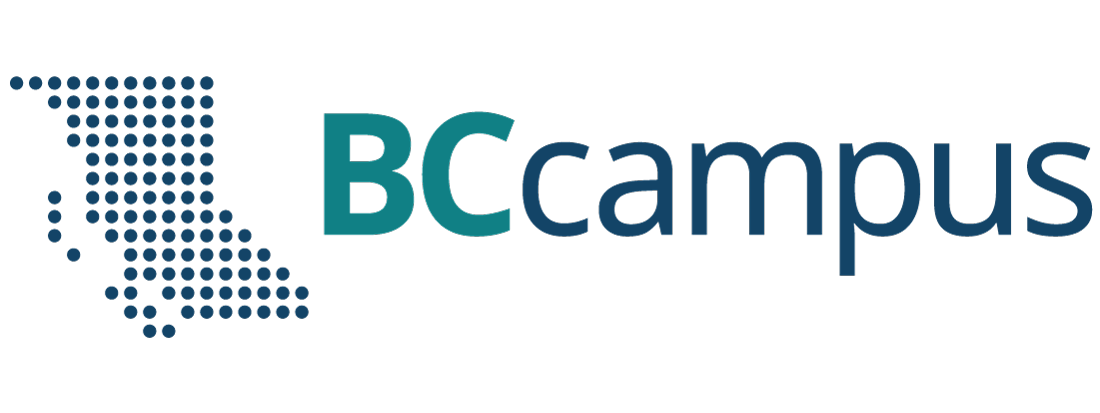
Want to create or adapt books like this? Learn more about how Pressbooks supports open publishing practices.
Chapter 3. Psychological Science
3.2 Psychologists Use Descriptive, Correlational, and Experimental Research Designs to Understand Behaviour
Learning objectives.
- Differentiate the goals of descriptive, correlational, and experimental research designs and explain the advantages and disadvantages of each.
- Explain the goals of descriptive research and the statistical techniques used to interpret it.
- Summarize the uses of correlational research and describe why correlational research cannot be used to infer causality.
- Review the procedures of experimental research and explain how it can be used to draw causal inferences.
Psychologists agree that if their ideas and theories about human behaviour are to be taken seriously, they must be backed up by data. However, the research of different psychologists is designed with different goals in mind, and the different goals require different approaches. These varying approaches, summarized in Table 3.2, are known as research designs . A research design is the specific method a researcher uses to collect, analyze, and interpret data . Psychologists use three major types of research designs in their research, and each provides an essential avenue for scientific investigation. Descriptive research is research designed to provide a snapshot of the current state of affairs . Correlational research is research designed to discover relationships among variables and to allow the prediction of future events from present knowledge . Experimental research is research in which initial equivalence among research participants in more than one group is created, followed by a manipulation of a given experience for these groups and a measurement of the influence of the manipulation . Each of the three research designs varies according to its strengths and limitations, and it is important to understand how each differs.
Descriptive Research: Assessing the Current State of Affairs
Descriptive research is designed to create a snapshot of the current thoughts, feelings, or behaviour of individuals. This section reviews three types of descriptive research : case studies , surveys , and naturalistic observation (Figure 3.4).
Sometimes the data in a descriptive research project are based on only a small set of individuals, often only one person or a single small group. These research designs are known as case studies — descriptive records of one or more individual’s experiences and behaviour . Sometimes case studies involve ordinary individuals, as when developmental psychologist Jean Piaget used his observation of his own children to develop his stage theory of cognitive development. More frequently, case studies are conducted on individuals who have unusual or abnormal experiences or characteristics or who find themselves in particularly difficult or stressful situations. The assumption is that by carefully studying individuals who are socially marginal, who are experiencing unusual situations, or who are going through a difficult phase in their lives, we can learn something about human nature.
Sigmund Freud was a master of using the psychological difficulties of individuals to draw conclusions about basic psychological processes. Freud wrote case studies of some of his most interesting patients and used these careful examinations to develop his important theories of personality. One classic example is Freud’s description of “Little Hans,” a child whose fear of horses the psychoanalyst interpreted in terms of repressed sexual impulses and the Oedipus complex (Freud, 1909/1964).
Another well-known case study is Phineas Gage, a man whose thoughts and emotions were extensively studied by cognitive psychologists after a railroad spike was blasted through his skull in an accident. Although there are questions about the interpretation of this case study (Kotowicz, 2007), it did provide early evidence that the brain’s frontal lobe is involved in emotion and morality (Damasio et al., 2005). An interesting example of a case study in clinical psychology is described by Rokeach (1964), who investigated in detail the beliefs of and interactions among three patients with schizophrenia, all of whom were convinced they were Jesus Christ.
In other cases the data from descriptive research projects come in the form of a survey — a measure administered through either an interview or a written questionnaire to get a picture of the beliefs or behaviours of a sample of people of interest . The people chosen to participate in the research (known as the sample) are selected to be representative of all the people that the researcher wishes to know about (the population). In election polls, for instance, a sample is taken from the population of all “likely voters” in the upcoming elections.
The results of surveys may sometimes be rather mundane, such as “Nine out of 10 doctors prefer Tymenocin” or “The median income in the city of Hamilton is $46,712.” Yet other times (particularly in discussions of social behaviour), the results can be shocking: “More than 40,000 people are killed by gunfire in the United States every year” or “More than 60% of women between the ages of 50 and 60 suffer from depression.” Descriptive research is frequently used by psychologists to get an estimate of the prevalence (or incidence ) of psychological disorders.
A final type of descriptive research — known as naturalistic observation — is research based on the observation of everyday events . For instance, a developmental psychologist who watches children on a playground and describes what they say to each other while they play is conducting descriptive research, as is a biopsychologist who observes animals in their natural habitats. One example of observational research involves a systematic procedure known as the strange situation , used to get a picture of how adults and young children interact. The data that are collected in the strange situation are systematically coded in a coding sheet such as that shown in Table 3.3.
The results of descriptive research projects are analyzed using descriptive statistics — numbers that summarize the distribution of scores on a measured variable . Most variables have distributions similar to that shown in Figure 3.5 where most of the scores are located near the centre of the distribution, and the distribution is symmetrical and bell-shaped. A data distribution that is shaped like a bell is known as a normal distribution .
A distribution can be described in terms of its central tendency — that is, the point in the distribution around which the data are centred — and its dispersion, or spread . The arithmetic average, or arithmetic mean , symbolized by the letter M , is the most commonly used measure of central tendency . It is computed by calculating the sum of all the scores of the variable and dividing this sum by the number of participants in the distribution (denoted by the letter N ). In the data presented in Figure 3.5 the mean height of the students is 67.12 inches (170.5 cm). The sample mean is usually indicated by the letter M .
In some cases, however, the data distribution is not symmetrical. This occurs when there are one or more extreme scores (known as outliers ) at one end of the distribution. Consider, for instance, the variable of family income (see Figure 3.6), which includes an outlier (a value of $3,800,000). In this case the mean is not a good measure of central tendency. Although it appears from Figure 3.6 that the central tendency of the family income variable should be around $70,000, the mean family income is actually $223,960. The single very extreme income has a disproportionate impact on the mean, resulting in a value that does not well represent the central tendency.
The median is used as an alternative measure of central tendency when distributions are not symmetrical. The median is the score in the center of the distribution, meaning that 50% of the scores are greater than the median and 50% of the scores are less than the median . In our case, the median household income ($73,000) is a much better indication of central tendency than is the mean household income ($223,960).
A final measure of central tendency, known as the mode , represents the value that occurs most frequently in the distribution . You can see from Figure 3.6 that the mode for the family income variable is $93,000 (it occurs four times).
In addition to summarizing the central tendency of a distribution, descriptive statistics convey information about how the scores of the variable are spread around the central tendency. Dispersion refers to the extent to which the scores are all tightly clustered around the central tendency , as seen in Figure 3.7.
Or they may be more spread out away from it, as seen in Figure 3.8.
One simple measure of dispersion is to find the largest (the maximum ) and the smallest (the minimum ) observed values of the variable and to compute the range of the variable as the maximum observed score minus the minimum observed score. You can check that the range of the height variable in Figure 3.5 is 72 – 62 = 10. The standard deviation , symbolized as s , is the most commonly used measure of dispersion . Distributions with a larger standard deviation have more spread. The standard deviation of the height variable is s = 2.74, and the standard deviation of the family income variable is s = $745,337.
An advantage of descriptive research is that it attempts to capture the complexity of everyday behaviour. Case studies provide detailed information about a single person or a small group of people, surveys capture the thoughts or reported behaviours of a large population of people, and naturalistic observation objectively records the behaviour of people or animals as it occurs naturally. Thus descriptive research is used to provide a relatively complete understanding of what is currently happening.
Despite these advantages, descriptive research has a distinct disadvantage in that, although it allows us to get an idea of what is currently happening, it is usually limited to static pictures. Although descriptions of particular experiences may be interesting, they are not always transferable to other individuals in other situations, nor do they tell us exactly why specific behaviours or events occurred. For instance, descriptions of individuals who have suffered a stressful event, such as a war or an earthquake, can be used to understand the individuals’ reactions to the event but cannot tell us anything about the long-term effects of the stress. And because there is no comparison group that did not experience the stressful situation, we cannot know what these individuals would be like if they hadn’t had the stressful experience.
Correlational Research: Seeking Relationships among Variables
In contrast to descriptive research, which is designed primarily to provide static pictures, correlational research involves the measurement of two or more relevant variables and an assessment of the relationship between or among those variables. For instance, the variables of height and weight are systematically related (correlated) because taller people generally weigh more than shorter people. In the same way, study time and memory errors are also related, because the more time a person is given to study a list of words, the fewer errors he or she will make. When there are two variables in the research design, one of them is called the predictor variable and the other the outcome variable . The research design can be visualized as shown in Figure 3.9, where the curved arrow represents the expected correlation between these two variables.
One way of organizing the data from a correlational study with two variables is to graph the values of each of the measured variables using a scatter plot . As you can see in Figure 3.10 a scatter plot is a visual image of the relationship between two variables . A point is plotted for each individual at the intersection of his or her scores for the two variables. When the association between the variables on the scatter plot can be easily approximated with a straight line , as in parts (a) and (b) of Figure 3.10 the variables are said to have a linear relationship .
When the straight line indicates that individuals who have above-average values for one variable also tend to have above-average values for the other variable , as in part (a), the relationship is said to be positive linear . Examples of positive linear relationships include those between height and weight, between education and income, and between age and mathematical abilities in children. In each case, people who score higher on one of the variables also tend to score higher on the other variable. Negative linear relationships , in contrast, as shown in part (b), occur when above-average values for one variable tend to be associated with below-average values for the other variable. Examples of negative linear relationships include those between the age of a child and the number of diapers the child uses, and between practice on and errors made on a learning task. In these cases, people who score higher on one of the variables tend to score lower on the other variable.
Relationships between variables that cannot be described with a straight line are known as nonlinear relationships . Part (c) of Figure 3.10 shows a common pattern in which the distribution of the points is essentially random. In this case there is no relationship at all between the two variables, and they are said to be independent . Parts (d) and (e) of Figure 3.10 show patterns of association in which, although there is an association, the points are not well described by a single straight line. For instance, part (d) shows the type of relationship that frequently occurs between anxiety and performance. Increases in anxiety from low to moderate levels are associated with performance increases, whereas increases in anxiety from moderate to high levels are associated with decreases in performance. Relationships that change in direction and thus are not described by a single straight line are called curvilinear relationships .
The most common statistical measure of the strength of linear relationships among variables is the Pearson correlation coefficient , which is symbolized by the letter r . The value of the correlation coefficient ranges from r = –1.00 to r = +1.00. The direction of the linear relationship is indicated by the sign of the correlation coefficient. Positive values of r (such as r = .54 or r = .67) indicate that the relationship is positive linear (i.e., the pattern of the dots on the scatter plot runs from the lower left to the upper right), whereas negative values of r (such as r = –.30 or r = –.72) indicate negative linear relationships (i.e., the dots run from the upper left to the lower right). The strength of the linear relationship is indexed by the distance of the correlation coefficient from zero (its absolute value). For instance, r = –.54 is a stronger relationship than r = .30, and r = .72 is a stronger relationship than r = –.57. Because the Pearson correlation coefficient only measures linear relationships, variables that have curvilinear relationships are not well described by r , and the observed correlation will be close to zero.
It is also possible to study relationships among more than two measures at the same time. A research design in which more than one predictor variable is used to predict a single outcome variable is analyzed through multiple regression (Aiken & West, 1991). Multiple regression is a statistical technique, based on correlation coefficients among variables, that allows predicting a single outcome variable from more than one predictor variable . For instance, Figure 3.11 shows a multiple regression analysis in which three predictor variables (Salary, job satisfaction, and years employed) are used to predict a single outcome (job performance). The use of multiple regression analysis shows an important advantage of correlational research designs — they can be used to make predictions about a person’s likely score on an outcome variable (e.g., job performance) based on knowledge of other variables.
An important limitation of correlational research designs is that they cannot be used to draw conclusions about the causal relationships among the measured variables. Consider, for instance, a researcher who has hypothesized that viewing violent behaviour will cause increased aggressive play in children. He has collected, from a sample of Grade 4 children, a measure of how many violent television shows each child views during the week, as well as a measure of how aggressively each child plays on the school playground. From his collected data, the researcher discovers a positive correlation between the two measured variables.
Although this positive correlation appears to support the researcher’s hypothesis, it cannot be taken to indicate that viewing violent television causes aggressive behaviour. Although the researcher is tempted to assume that viewing violent television causes aggressive play, there are other possibilities. One alternative possibility is that the causal direction is exactly opposite from what has been hypothesized. Perhaps children who have behaved aggressively at school develop residual excitement that leads them to want to watch violent television shows at home (Figure 3.13):
Although this possibility may seem less likely, there is no way to rule out the possibility of such reverse causation on the basis of this observed correlation. It is also possible that both causal directions are operating and that the two variables cause each other (Figure 3.14).
Still another possible explanation for the observed correlation is that it has been produced by the presence of a common-causal variable (also known as a third variable ). A common-causal variable is a variable that is not part of the research hypothesis but that causes both the predictor and the outcome variable and thus produces the observed correlation between them . In our example, a potential common-causal variable is the discipline style of the children’s parents. Parents who use a harsh and punitive discipline style may produce children who like to watch violent television and who also behave aggressively in comparison to children whose parents use less harsh discipline (Figure 3.15)
In this case, television viewing and aggressive play would be positively correlated (as indicated by the curved arrow between them), even though neither one caused the other but they were both caused by the discipline style of the parents (the straight arrows). When the predictor and outcome variables are both caused by a common-causal variable, the observed relationship between them is said to be spurious . A spurious relationship is a relationship between two variables in which a common-causal variable produces and “explains away” the relationship . If effects of the common-causal variable were taken away, or controlled for, the relationship between the predictor and outcome variables would disappear. In the example, the relationship between aggression and television viewing might be spurious because by controlling for the effect of the parents’ disciplining style, the relationship between television viewing and aggressive behaviour might go away.
Common-causal variables in correlational research designs can be thought of as mystery variables because, as they have not been measured, their presence and identity are usually unknown to the researcher. Since it is not possible to measure every variable that could cause both the predictor and outcome variables, the existence of an unknown common-causal variable is always a possibility. For this reason, we are left with the basic limitation of correlational research: correlation does not demonstrate causation. It is important that when you read about correlational research projects, you keep in mind the possibility of spurious relationships, and be sure to interpret the findings appropriately. Although correlational research is sometimes reported as demonstrating causality without any mention being made of the possibility of reverse causation or common-causal variables, informed consumers of research, like you, are aware of these interpretational problems.
In sum, correlational research designs have both strengths and limitations. One strength is that they can be used when experimental research is not possible because the predictor variables cannot be manipulated. Correlational designs also have the advantage of allowing the researcher to study behaviour as it occurs in everyday life. And we can also use correlational designs to make predictions — for instance, to predict from the scores on their battery of tests the success of job trainees during a training session. But we cannot use such correlational information to determine whether the training caused better job performance. For that, researchers rely on experiments.
Experimental Research: Understanding the Causes of Behaviour
The goal of experimental research design is to provide more definitive conclusions about the causal relationships among the variables in the research hypothesis than is available from correlational designs. In an experimental research design, the variables of interest are called the independent variable (or variables ) and the dependent variable . The independent variable in an experiment is the causing variable that is created (manipulated) by the experimenter . The dependent variable in an experiment is a measured variable that is expected to be influenced by the experimental manipulation . The research hypothesis suggests that the manipulated independent variable or variables will cause changes in the measured dependent variables. We can diagram the research hypothesis by using an arrow that points in one direction. This demonstrates the expected direction of causality (Figure 3.16):
Research Focus: Video Games and Aggression
Consider an experiment conducted by Anderson and Dill (2000). The study was designed to test the hypothesis that viewing violent video games would increase aggressive behaviour. In this research, male and female undergraduates from Iowa State University were given a chance to play with either a violent video game (Wolfenstein 3D) or a nonviolent video game (Myst). During the experimental session, the participants played their assigned video games for 15 minutes. Then, after the play, each participant played a competitive game with an opponent in which the participant could deliver blasts of white noise through the earphones of the opponent. The operational definition of the dependent variable (aggressive behaviour) was the level and duration of noise delivered to the opponent. The design of the experiment is shown in Figure 3.17
Two advantages of the experimental research design are (a) the assurance that the independent variable (also known as the experimental manipulation ) occurs prior to the measured dependent variable, and (b) the creation of initial equivalence between the conditions of the experiment (in this case by using random assignment to conditions).
Experimental designs have two very nice features. For one, they guarantee that the independent variable occurs prior to the measurement of the dependent variable. This eliminates the possibility of reverse causation. Second, the influence of common-causal variables is controlled, and thus eliminated, by creating initial equivalence among the participants in each of the experimental conditions before the manipulation occurs.
The most common method of creating equivalence among the experimental conditions is through random assignment to conditions, a procedure in which the condition that each participant is assigned to is determined through a random process, such as drawing numbers out of an envelope or using a random number table . Anderson and Dill first randomly assigned about 100 participants to each of their two groups (Group A and Group B). Because they used random assignment to conditions, they could be confident that, before the experimental manipulation occurred, the students in Group A were, on average, equivalent to the students in Group B on every possible variable, including variables that are likely to be related to aggression, such as parental discipline style, peer relationships, hormone levels, diet — and in fact everything else.
Then, after they had created initial equivalence, Anderson and Dill created the experimental manipulation — they had the participants in Group A play the violent game and the participants in Group B play the nonviolent game. Then they compared the dependent variable (the white noise blasts) between the two groups, finding that the students who had viewed the violent video game gave significantly longer noise blasts than did the students who had played the nonviolent game.
Anderson and Dill had from the outset created initial equivalence between the groups. This initial equivalence allowed them to observe differences in the white noise levels between the two groups after the experimental manipulation, leading to the conclusion that it was the independent variable (and not some other variable) that caused these differences. The idea is that the only thing that was different between the students in the two groups was the video game they had played.
Despite the advantage of determining causation, experiments do have limitations. One is that they are often conducted in laboratory situations rather than in the everyday lives of people. Therefore, we do not know whether results that we find in a laboratory setting will necessarily hold up in everyday life. Second, and more important, is that some of the most interesting and key social variables cannot be experimentally manipulated. If we want to study the influence of the size of a mob on the destructiveness of its behaviour, or to compare the personality characteristics of people who join suicide cults with those of people who do not join such cults, these relationships must be assessed using correlational designs, because it is simply not possible to experimentally manipulate these variables.
Key Takeaways
- Descriptive, correlational, and experimental research designs are used to collect and analyze data.
- Descriptive designs include case studies, surveys, and naturalistic observation. The goal of these designs is to get a picture of the current thoughts, feelings, or behaviours in a given group of people. Descriptive research is summarized using descriptive statistics.
- Correlational research designs measure two or more relevant variables and assess a relationship between or among them. The variables may be presented on a scatter plot to visually show the relationships. The Pearson Correlation Coefficient ( r ) is a measure of the strength of linear relationship between two variables.
- Common-causal variables may cause both the predictor and outcome variable in a correlational design, producing a spurious relationship. The possibility of common-causal variables makes it impossible to draw causal conclusions from correlational research designs.
- Experimental research involves the manipulation of an independent variable and the measurement of a dependent variable. Random assignment to conditions is normally used to create initial equivalence between the groups, allowing researchers to draw causal conclusions.
Exercises and Critical Thinking
- There is a negative correlation between the row that a student sits in in a large class (when the rows are numbered from front to back) and his or her final grade in the class. Do you think this represents a causal relationship or a spurious relationship, and why?
- Think of two variables (other than those mentioned in this book) that are likely to be correlated, but in which the correlation is probably spurious. What is the likely common-causal variable that is producing the relationship?
- Imagine a researcher wants to test the hypothesis that participating in psychotherapy will cause a decrease in reported anxiety. Describe the type of research design the investigator might use to draw this conclusion. What would be the independent and dependent variables in the research?
Image Attributions
Figure 3.4: “ Reading newspaper ” by Alaskan Dude (http://commons.wikimedia.org/wiki/File:Reading_newspaper.jpg) is licensed under CC BY 2.0
Aiken, L., & West, S. (1991). Multiple regression: Testing and interpreting interactions . Newbury Park, CA: Sage.
Ainsworth, M. S., Blehar, M. C., Waters, E., & Wall, S. (1978). Patterns of attachment: A psychological study of the strange situation . Hillsdale, NJ: Lawrence Erlbaum Associates.
Anderson, C. A., & Dill, K. E. (2000). Video games and aggressive thoughts, feelings, and behavior in the laboratory and in life. Journal of Personality and Social Psychology, 78 (4), 772–790.
Damasio, H., Grabowski, T., Frank, R., Galaburda, A. M., Damasio, A. R., Cacioppo, J. T., & Berntson, G. G. (2005). The return of Phineas Gage: Clues about the brain from the skull of a famous patient. In Social neuroscience: Key readings. (pp. 21–28). New York, NY: Psychology Press.
Freud, S. (1909/1964). Analysis of phobia in a five-year-old boy. In E. A. Southwell & M. Merbaum (Eds.), Personality: Readings in theory and research (pp. 3–32). Belmont, CA: Wadsworth. (Original work published 1909).
Kotowicz, Z. (2007). The strange case of Phineas Gage. History of the Human Sciences, 20 (1), 115–131.
Rokeach, M. (1964). The three Christs of Ypsilanti: A psychological study . New York, NY: Knopf.
Stangor, C. (2011). Research methods for the behavioural sciences (4th ed.). Mountain View, CA: Cengage.
Long Descriptions
Figure 3.6 long description: There are 25 families. 24 families have an income between $44,000 and $111,000 and one family has an income of $3,800,000. The mean income is $223,960 while the median income is $73,000. [Return to Figure 3.6]
Figure 3.10 long description: Types of scatter plots.
- Positive linear, r=positive .82. The plots on the graph form a rough line that runs from lower left to upper right.
- Negative linear, r=negative .70. The plots on the graph form a rough line that runs from upper left to lower right.
- Independent, r=0.00. The plots on the graph are spread out around the centre.
- Curvilinear, r=0.00. The plots of the graph form a rough line that goes up and then down like a hill.
- Curvilinear, r=0.00. The plots on the graph for a rough line that goes down and then up like a ditch.
[Return to Figure 3.10]
Introduction to Psychology - 1st Canadian Edition Copyright © 2014 by Jennifer Walinga and Charles Stangor is licensed under a Creative Commons Attribution-NonCommercial-ShareAlike 4.0 International License , except where otherwise noted.
Share This Book

Want to create or adapt books like this? Learn more about how Pressbooks supports open publishing practices.
7.2 Correlational Research
Learning objectives.
- Define correlational research and give several examples.
- Explain why a researcher might choose to conduct correlational research rather than experimental research or another type of nonexperimental research.
What Is Correlational Research?
Correlational research is a type of nonexperimental research in which the researcher measures two variables and assesses the statistical relationship (i.e., the correlation) between them with little or no effort to control extraneous variables. There are essentially two reasons that researchers interested in statistical relationships between variables would choose to conduct a correlational study rather than an experiment. The first is that they do not believe that the statistical relationship is a causal one. For example, a researcher might evaluate the validity of a brief extraversion test by administering it to a large group of participants along with a longer extraversion test that has already been shown to be valid. This researcher might then check to see whether participants’ scores on the brief test are strongly correlated with their scores on the longer one. Neither test score is thought to cause the other, so there is no independent variable to manipulate. In fact, the terms independent variable and dependent variable do not apply to this kind of research.
The other reason that researchers would choose to use a correlational study rather than an experiment is that the statistical relationship of interest is thought to be causal, but the researcher cannot manipulate the independent variable because it is impossible, impractical, or unethical. For example, Allen Kanner and his colleagues thought that the number of “daily hassles” (e.g., rude salespeople, heavy traffic) that people experience affects the number of physical and psychological symptoms they have (Kanner, Coyne, Schaefer, & Lazarus, 1981). But because they could not manipulate the number of daily hassles their participants experienced, they had to settle for measuring the number of daily hassles—along with the number of symptoms—using self-report questionnaires. Although the strong positive relationship they found between these two variables is consistent with their idea that hassles cause symptoms, it is also consistent with the idea that symptoms cause hassles or that some third variable (e.g., neuroticism) causes both.
A common misconception among beginning researchers is that correlational research must involve two quantitative variables, such as scores on two extraversion tests or the number of hassles and number of symptoms people have experienced. However, the defining feature of correlational research is that the two variables are measured—neither one is manipulated—and this is true regardless of whether the variables are quantitative or categorical. Imagine, for example, that a researcher administers the Rosenberg Self-Esteem Scale to 50 American college students and 50 Japanese college students. Although this “feels” like a between-subjects experiment, it is a correlational study because the researcher did not manipulate the students’ nationalities. The same is true of the study by Cacioppo and Petty comparing college faculty and factory workers in terms of their need for cognition. It is a correlational study because the researchers did not manipulate the participants’ occupations.
Figure 7.2 “Results of a Hypothetical Study on Whether People Who Make Daily To-Do Lists Experience Less Stress Than People Who Do Not Make Such Lists” shows data from a hypothetical study on the relationship between whether people make a daily list of things to do (a “to-do list”) and stress. Notice that it is unclear whether this is an experiment or a correlational study because it is unclear whether the independent variable was manipulated. If the researcher randomly assigned some participants to make daily to-do lists and others not to, then it is an experiment. If the researcher simply asked participants whether they made daily to-do lists, then it is a correlational study. The distinction is important because if the study was an experiment, then it could be concluded that making the daily to-do lists reduced participants’ stress. But if it was a correlational study, it could only be concluded that these variables are statistically related. Perhaps being stressed has a negative effect on people’s ability to plan ahead (the directionality problem). Or perhaps people who are more conscientious are more likely to make to-do lists and less likely to be stressed (the third-variable problem). The crucial point is that what defines a study as experimental or correlational is not the variables being studied, nor whether the variables are quantitative or categorical, nor the type of graph or statistics used to analyze the data. It is how the study is conducted.
Figure 7.2 Results of a Hypothetical Study on Whether People Who Make Daily To-Do Lists Experience Less Stress Than People Who Do Not Make Such Lists

Data Collection in Correlational Research
Again, the defining feature of correlational research is that neither variable is manipulated. It does not matter how or where the variables are measured. A researcher could have participants come to a laboratory to complete a computerized backward digit span task and a computerized risky decision-making task and then assess the relationship between participants’ scores on the two tasks. Or a researcher could go to a shopping mall to ask people about their attitudes toward the environment and their shopping habits and then assess the relationship between these two variables. Both of these studies would be correlational because no independent variable is manipulated. However, because some approaches to data collection are strongly associated with correlational research, it makes sense to discuss them here. The two we will focus on are naturalistic observation and archival data. A third, survey research, is discussed in its own chapter.
Naturalistic Observation
Naturalistic observation is an approach to data collection that involves observing people’s behavior in the environment in which it typically occurs. Thus naturalistic observation is a type of field research (as opposed to a type of laboratory research). It could involve observing shoppers in a grocery store, children on a school playground, or psychiatric inpatients in their wards. Researchers engaged in naturalistic observation usually make their observations as unobtrusively as possible so that participants are often not aware that they are being studied. Ethically, this is considered to be acceptable if the participants remain anonymous and the behavior occurs in a public setting where people would not normally have an expectation of privacy. Grocery shoppers putting items into their shopping carts, for example, are engaged in public behavior that is easily observable by store employees and other shoppers. For this reason, most researchers would consider it ethically acceptable to observe them for a study. On the other hand, one of the arguments against the ethicality of the naturalistic observation of “bathroom behavior” discussed earlier in the book is that people have a reasonable expectation of privacy even in a public restroom and that this expectation was violated.
Researchers Robert Levine and Ara Norenzayan used naturalistic observation to study differences in the “pace of life” across countries (Levine & Norenzayan, 1999). One of their measures involved observing pedestrians in a large city to see how long it took them to walk 60 feet. They found that people in some countries walked reliably faster than people in other countries. For example, people in the United States and Japan covered 60 feet in about 12 seconds on average, while people in Brazil and Romania took close to 17 seconds.
Because naturalistic observation takes place in the complex and even chaotic “real world,” there are two closely related issues that researchers must deal with before collecting data. The first is sampling. When, where, and under what conditions will the observations be made, and who exactly will be observed? Levine and Norenzayan described their sampling process as follows:
Male and female walking speed over a distance of 60 feet was measured in at least two locations in main downtown areas in each city. Measurements were taken during main business hours on clear summer days. All locations were flat, unobstructed, had broad sidewalks, and were sufficiently uncrowded to allow pedestrians to move at potentially maximum speeds. To control for the effects of socializing, only pedestrians walking alone were used. Children, individuals with obvious physical handicaps, and window-shoppers were not timed. Thirty-five men and 35 women were timed in most cities. (p. 186)
Precise specification of the sampling process in this way makes data collection manageable for the observers, and it also provides some control over important extraneous variables. For example, by making their observations on clear summer days in all countries, Levine and Norenzayan controlled for effects of the weather on people’s walking speeds.
The second issue is measurement. What specific behaviors will be observed? In Levine and Norenzayan’s study, measurement was relatively straightforward. They simply measured out a 60-foot distance along a city sidewalk and then used a stopwatch to time participants as they walked over that distance. Often, however, the behaviors of interest are not so obvious or objective. For example, researchers Robert Kraut and Robert Johnston wanted to study bowlers’ reactions to their shots, both when they were facing the pins and then when they turned toward their companions (Kraut & Johnston, 1979). But what “reactions” should they observe? Based on previous research and their own pilot testing, Kraut and Johnston created a list of reactions that included “closed smile,” “open smile,” “laugh,” “neutral face,” “look down,” “look away,” and “face cover” (covering one’s face with one’s hands). The observers committed this list to memory and then practiced by coding the reactions of bowlers who had been videotaped. During the actual study, the observers spoke into an audio recorder, describing the reactions they observed. Among the most interesting results of this study was that bowlers rarely smiled while they still faced the pins. They were much more likely to smile after they turned toward their companions, suggesting that smiling is not purely an expression of happiness but also a form of social communication.
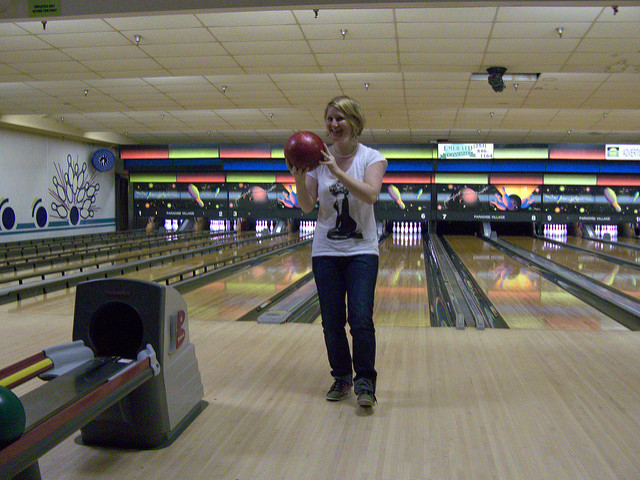
Naturalistic observation has revealed that bowlers tend to smile when they turn away from the pins and toward their companions, suggesting that smiling is not purely an expression of happiness but also a form of social communication.
sieneke toering – bowling big lebowski style – CC BY-NC-ND 2.0.
When the observations require a judgment on the part of the observers—as in Kraut and Johnston’s study—this process is often described as coding . Coding generally requires clearly defining a set of target behaviors. The observers then categorize participants individually in terms of which behavior they have engaged in and the number of times they engaged in each behavior. The observers might even record the duration of each behavior. The target behaviors must be defined in such a way that different observers code them in the same way. This is the issue of interrater reliability. Researchers are expected to demonstrate the interrater reliability of their coding procedure by having multiple raters code the same behaviors independently and then showing that the different observers are in close agreement. Kraut and Johnston, for example, video recorded a subset of their participants’ reactions and had two observers independently code them. The two observers showed that they agreed on the reactions that were exhibited 97% of the time, indicating good interrater reliability.
Archival Data
Another approach to correlational research is the use of archival data , which are data that have already been collected for some other purpose. An example is a study by Brett Pelham and his colleagues on “implicit egotism”—the tendency for people to prefer people, places, and things that are similar to themselves (Pelham, Carvallo, & Jones, 2005). In one study, they examined Social Security records to show that women with the names Virginia, Georgia, Louise, and Florence were especially likely to have moved to the states of Virginia, Georgia, Louisiana, and Florida, respectively.
As with naturalistic observation, measurement can be more or less straightforward when working with archival data. For example, counting the number of people named Virginia who live in various states based on Social Security records is relatively straightforward. But consider a study by Christopher Peterson and his colleagues on the relationship between optimism and health using data that had been collected many years before for a study on adult development (Peterson, Seligman, & Vaillant, 1988). In the 1940s, healthy male college students had completed an open-ended questionnaire about difficult wartime experiences. In the late 1980s, Peterson and his colleagues reviewed the men’s questionnaire responses to obtain a measure of explanatory style—their habitual ways of explaining bad events that happen to them. More pessimistic people tend to blame themselves and expect long-term negative consequences that affect many aspects of their lives, while more optimistic people tend to blame outside forces and expect limited negative consequences. To obtain a measure of explanatory style for each participant, the researchers used a procedure in which all negative events mentioned in the questionnaire responses, and any causal explanations for them, were identified and written on index cards. These were given to a separate group of raters who rated each explanation in terms of three separate dimensions of optimism-pessimism. These ratings were then averaged to produce an explanatory style score for each participant. The researchers then assessed the statistical relationship between the men’s explanatory style as college students and archival measures of their health at approximately 60 years of age. The primary result was that the more optimistic the men were as college students, the healthier they were as older men. Pearson’s r was +.25.
This is an example of content analysis —a family of systematic approaches to measurement using complex archival data. Just as naturalistic observation requires specifying the behaviors of interest and then noting them as they occur, content analysis requires specifying keywords, phrases, or ideas and then finding all occurrences of them in the data. These occurrences can then be counted, timed (e.g., the amount of time devoted to entertainment topics on the nightly news show), or analyzed in a variety of other ways.
Key Takeaways
- Correlational research involves measuring two variables and assessing the relationship between them, with no manipulation of an independent variable.
- Correlational research is not defined by where or how the data are collected. However, some approaches to data collection are strongly associated with correlational research. These include naturalistic observation (in which researchers observe people’s behavior in the context in which it normally occurs) and the use of archival data that were already collected for some other purpose.
Discussion: For each of the following, decide whether it is most likely that the study described is experimental or correlational and explain why.
- An educational researcher compares the academic performance of students from the “rich” side of town with that of students from the “poor” side of town.
- A cognitive psychologist compares the ability of people to recall words that they were instructed to “read” with their ability to recall words that they were instructed to “imagine.”
- A manager studies the correlation between new employees’ college grade point averages and their first-year performance reports.
- An automotive engineer installs different stick shifts in a new car prototype, each time asking several people to rate how comfortable the stick shift feels.
- A food scientist studies the relationship between the temperature inside people’s refrigerators and the amount of bacteria on their food.
- A social psychologist tells some research participants that they need to hurry over to the next building to complete a study. She tells others that they can take their time. Then she observes whether they stop to help a research assistant who is pretending to be hurt.
Kanner, A. D., Coyne, J. C., Schaefer, C., & Lazarus, R. S. (1981). Comparison of two modes of stress measurement: Daily hassles and uplifts versus major life events. Journal of Behavioral Medicine, 4 , 1–39.
Kraut, R. E., & Johnston, R. E. (1979). Social and emotional messages of smiling: An ethological approach. Journal of Personality and Social Psychology, 37 , 1539–1553.
Levine, R. V., & Norenzayan, A. (1999). The pace of life in 31 countries. Journal of Cross-Cultural Psychology, 30 , 178–205.
Pelham, B. W., Carvallo, M., & Jones, J. T. (2005). Implicit egotism. Current Directions in Psychological Science, 14 , 106–110.
Peterson, C., Seligman, M. E. P., & Vaillant, G. E. (1988). Pessimistic explanatory style is a risk factor for physical illness: A thirty-five year longitudinal study. Journal of Personality and Social Psychology, 55 , 23–27.
Research Methods in Psychology Copyright © 2016 by University of Minnesota is licensed under a Creative Commons Attribution-NonCommercial-ShareAlike 4.0 International License , except where otherwise noted.
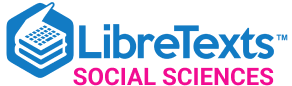
- school Campus Bookshelves
- menu_book Bookshelves
- perm_media Learning Objects
- login Login
- how_to_reg Request Instructor Account
- hub Instructor Commons
- Download Page (PDF)
- Download Full Book (PDF)
- Periodic Table
- Physics Constants
- Scientific Calculator
- Reference & Cite
- Tools expand_more
- Readability
selected template will load here
This action is not available.
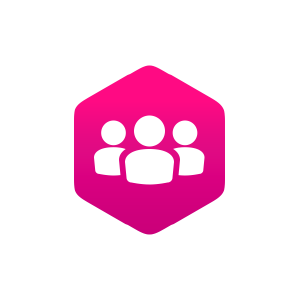
1.4: Psychologists Use Descriptive, Correlational, and Experimental Research Designs to Understand Behavior
- Last updated
- Save as PDF
- Page ID 40632

Learning Objectives
- Differentiate the goals of descriptive, correlational, and experimental research designs and explain the advantages and disadvantages of each.
- Explain the goals of descriptive research and the statistical techniques used to interpret it.
- Summarize the uses of correlational research and describe why correlational research cannot be used to infer causality.
- Review the procedures of experimental research and explain how it can be used to draw causal inferences.
Psychologists agree that if their ideas and theories about human behavior are to be taken seriously, they must be backed up by data. However, the research of different psychologists is designed with different goals in mind, and the different goals require different approaches. These varying approaches, summarized in Table \(\PageIndex{2}\), are known as research designs . A research design is the specific method a researcher uses to collect, analyze, and interpret data . Psychologists use three major types of research designs in their research, and each provides an essential avenue for scientific investigation. Descriptive research is research designed to provide a snapshot of the current state of affairs . Correlational research is research designed to discover relationships among variables and to allow the prediction of future events from present knowledge . Experimental research is research in which initial comparability among research participants in more than one group is created, followed by a manipulation of a given experience for these groups and a measurement of the influence of the manipulation . Each of the three research designs varies according to its strengths and limitations, and it is important to understand how each differs.
Descriptive Research: Assessing the Current State of Affairs
Descriptive research is designed to create a snapshot of the current thoughts, feelings, or behavior of individuals. This section reviews three types of descriptive research: case studies , surveys , and naturalistic observation .
Sometimes the data in a descriptive research project are based on only a small set of individuals, often only one person or a single small group. These research designs are known as case studies— descriptive records of one or more individual’s experiences and behavior . Sometimes case studies involve ordinary individuals, as when developmental psychologist Jean Piaget used his observation of his own children to develop his stage theory of cognitive development. More frequently, case studies are conducted on individuals who have unusual or abnormal experiences or characteristics or who find themselves in particularly difficult or stressful situations. The assumption is that by carefully studying individuals who are socially marginal, who are experiencing unusual situations, or who are going through a difficult phase in their lives, we can learn something about human nature.
Sigmund Freud was a master of using the psychological difficulties of individuals to draw conclusions about basic psychological processes. Freud wrote case studies of some of his most interesting patients and used these careful examinations to develop his important theories of personality. One classic example is Freud’s description of “Little Hans,” a child whose fear of horses the psychoanalyst interpreted in terms of repressed sexual impulses and the Oedipus complex (Freud (1909/1964).
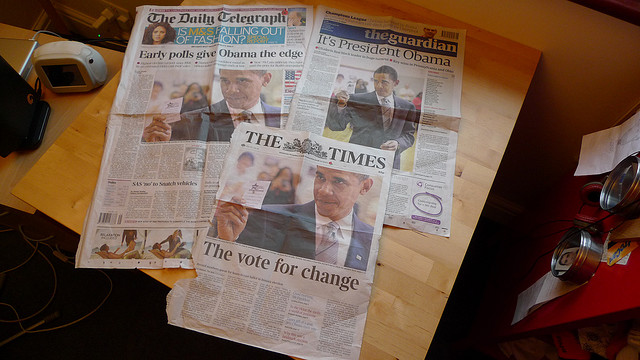
Another well-known case study is Phineas Gage, a man whose thoughts and emotions were extensively studied by cognitive psychologists after a railroad spike was blasted through his skull in an accident. Although there is question about the interpretation of this case study (Kotowicz, 2007), it did provide early evidence that the brain’s frontal lobe is involved in emotion and morality (Damasio et al., 2005). An interesting example of a case study in clinical psychology is described by Rokeach (1964), who investigated in detail the beliefs and interactions among three patients with schizophrenia, all of whom were convinced they were Jesus Christ.
In other cases the data from descriptive research projects come in the form of a survey— a measure administered through either an interview or a written questionnaire to get a picture of the beliefs or behaviors of a sample of people of interest . The people chosen to participate in the research (known as the sample) are selected to be representative of all the people that the researcher wishes to know about (the population). In election polls, for instance, a sample is taken from the population of all “likely voters” in the upcoming elections.
The results of surveys may sometimes be rather mundane, such as “Nine out of ten doctors prefer Tymenocin,” or “The median income in Montgomery County is $36,712.” Yet other times (particularly in discussions of social behavior), the results can be shocking: “More than 40,000 people are killed by gunfire in the United States every year,” or “More than 60% of women between the ages of 50 and 60 suffer from depression.” Descriptive research is frequently used by psychologists to get an estimate of the prevalence (or incidence ) of psychological disorders.
A final type of descriptive research—known as naturalistic observation—is research based on the observation of everyday events . For instance, a developmental psychologist who watches children on a playground and describes what they say to each other while they play is conducting descriptive research, as is a biopsychologist who observes animals in their natural habitats. One example of observational research involves a systematic procedure known as the strange situation , used to get a picture of how adults and young children interact. The data that are collected in the strange situation are systematically coded in a coding sheet such as that shown in Table \(\PageIndex{3}\).
The results of descriptive research projects are analyzed using descriptive statistics— numbers that summarize the distribution of scores on a measured variable . Most variables have distributions similar to that shown in Figure \(\PageIndex{5}\), where most of the scores are located near the center of the distribution, and the distribution is symmetrical and bell-shaped. A data distribution that is shaped like a bell is known as a normal distribution.
A distribution can be described in terms of its central tendency —that is, the point in the distribution around which the data are centered—and its dispersion , or spread. The arithmetic average, or arithmetic mean, is the most commonly used measure of central tendency . It is computed by calculating the sum of all the scores of the variable and dividing this sum by the number of participants in the distribution (denoted by the letter N ). In the data presented in Figure \(\PageIndex{5}\), the mean height of the students is 67.12 inches. The sample mean is usually indicated by the letter M .
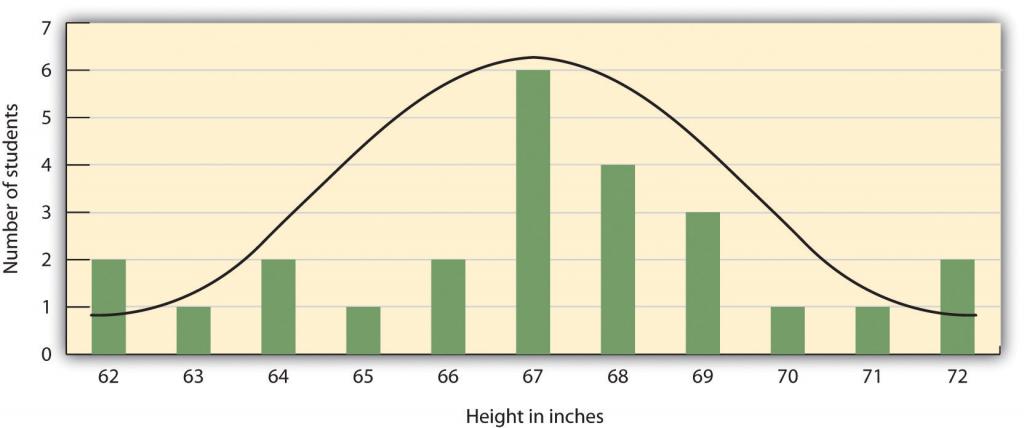
In some cases, however, the data distribution is not symmetrical. This occurs when there are one or more extreme scores (known as outliers ) at one end of the distribution. Consider, for instance, the variable of family income (Figure \(\PageIndex{6}\)), which includes an outlier (a value of $3,800,000). In this case the mean is not a good measure of central tendency. Although it appears from Figure \(\PageIndex{6}\) that the central tendency of the family income variable should be around $70,000, the mean family income is actually $223,960. The single very extreme income has a disproportionate impact on the mean, resulting in a value that does not well represent the central tendency.
The median is used as an alternative measure of central tendency when distributions are not symmetrical. The median is the score in the center of the distribution, meaning that 50% of the scores are greater than the median and 50% of the scores are less than the median . In our case, the median household income ($73,000) is a much better indication of central tendency than is the mean household income ($223,960).
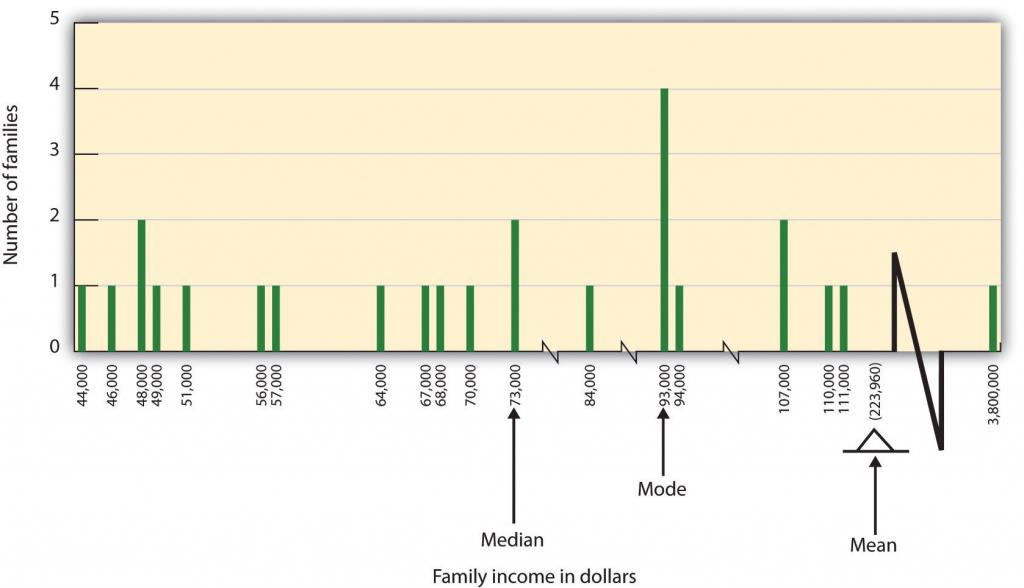
A final measure of central tendency, known as the mode, represents the value that occurs most frequently in the distribution . You can see from Figure \(\PageIndex{6}\) that the mode for the family income variable is $93,000 (it occurs four times).
In addition to summarizing the central tendency of a distribution, descriptive statistics convey information about how the scores of the variable are spread around the central tendency. Dispersion refers to the extent to which the scores are all tightly clustered around the central tendency, like this:
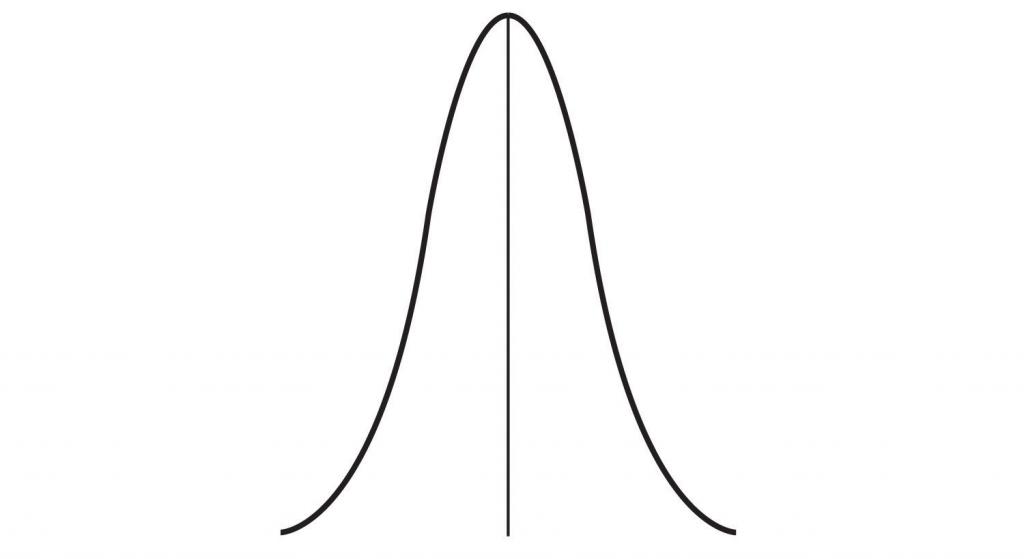
Or they may be more spread out away from it, like this:
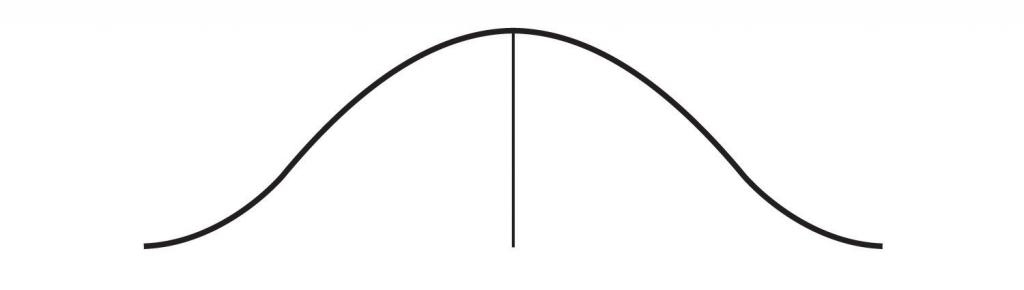
One simple measure of dispersion is to find the largest (the maximum ) and the smallest (the minimum ) observed values of the variable and to compute the range of the variable as the maximum observed score minus the minimum observed score. You can check that the range of the height variable in Figure \(\PageIndex{5}\) is 72 – 62 = 10. The standard deviation, symbolized as s , is the most commonly used measure of dispersion . Distributions with a larger standard deviation have more spread. The standard deviation of the height variable is s = 2.74, and the standard deviation of the family income variable is s = $745,337.
An advantage of descriptive research is that it attempts to capture the complexity of everyday behavior. Case studies provide detailed information about a single person or a small group of people, surveys capture the thoughts or reported behaviors of a large population of people, and naturalistic observation objectively records the behavior of people or animals as it occurs naturally. Thus descriptive research is used to provide a relatively complete understanding of what is currently happening.
Despite these advantages, descriptive research has a distinct disadvantage in that, although it allows us to get an idea of what is currently happening, it is usually limited to static pictures. Although descriptions of particular experiences may be interesting, they are not always transferable to other individuals in other situations, nor do they tell us exactly why specific behaviors or events occurred. For instance, descriptions of individuals who have suffered a stressful event, such as a war or an earthquake, can be used to understand the individuals’ reactions to the event but cannot tell us anything about the long-term effects of the stress. And because there is no comparison group that did not experience the stressful situation, we cannot know what these individuals would be like if they hadn’t had the stressful experience.
Correlational Research: Seeking Relationships Among Variables
In contrast to descriptive research, which is designed primarily to provide static pictures, correlational research involves the measurement of two or more relevant variables and an assessment of the relationship between or among those variables. For instance, the variables of height and weight are systematically related (correlated) because taller people generally weigh more than shorter people. In the same way, study time and memory errors are also related, because the more time a person is given to study a list of words, the fewer errors he or she will make.
One way of organizing the data from a correlational study with two variables is to graph the values of each of the measured variables using a scatter plot . As you can see in Figure \(\PageIndex{10}\), a scatter plot is a visual image of the relationship between two variables . A point is plotted for each individual at the intersection of his or her scores for the two variables. When the association between the variables on the scatter plot can be easily approximated with a straight line, as in parts (a) and (b) of Figure \(\PageIndex{10}\), the variables are said to have a linear relationship .
When the straight line indicates that individuals who have above-average values for one variable also tend to have above-average values for the other variable, as in part (a), the relationship is said to be positive linear . Examples of positive linear relationships include those between height and weight, between education and income, and between age and mathematical abilities in children. In each case people who score higher on one of the variables also tend to score higher on the other variable. Negative linear relationships , in contrast, as shown in part (b), occur when above-average values for one variable tend to be associated with below-average values for the other variable. Examples of negative linear relationships include those between the age of a child and the number of diapers the child uses, and between practice on and errors made on a learning task. In these cases people who score higher on one of the variables tend to score lower on the other variable.
Relationships between variables that cannot be described with a straight line are known as nonlinear relationships . Part (c) of Figure \(\PageIndex{10}\) shows a common pattern in which the distribution of the points is essentially random. In this case there is no relationship at all between the two variables, and they are said to be independent . Parts (d) and (e) of Figure \(\PageIndex{10}\) show patterns of association in which, although there is an association, the points are not well described by a single straight line. For instance, part (d) shows the type of relationship that frequently occurs between anxiety and performance. Increases in anxiety from low to moderate levels are associated with performance increases, whereas increases in anxiety from moderate to high levels are associated with decreases in performance. Relationships that change in direction and thus are not described by a single straight line are called curvilinear relationships .
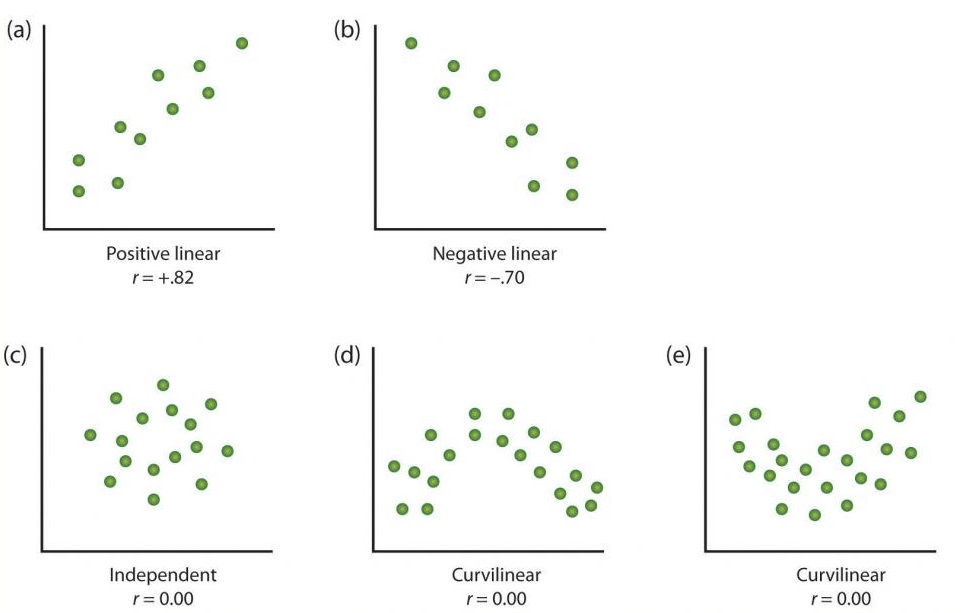
The most common statistical measure of the strength of linear relationships among variables is the Pearson correlation coefficient , which is symbolized by the letter r . The value of the correlation coefficient ranges from r = –1.00 to r = +1.00. The direction of the linear relationship is indicated by the sign of the correlation coefficient. Positive values of r (such as r = 0.54 or r = 0.67) indicate that the relationship is positive linear (i.e., the pattern of the dots on the scatter plot runs from the lower left to the upper right), whereas negative values of r (such as r = –0.30 or r = –0.72) indicate negative linear relationships (i.e., the dots run from the upper left to the lower right). The strength of the linear relationship is indexed by the distance of the correlation coefficient from zero (its absolute value). For instance, r = -0.54 is a stronger relationship than r = 0.30, and r = 0.72 is a stronger relationship than r = –0.57. Because the Pearson correlation coefficient only measures linear relationships, variables that have curvilinear relationships are not well described by r , and the observed correlation will be close to zero.
It is also possible to study relationships among more than two measures at the same time. A research design in which more than one variable is used to predict a single outcome variable is analyzed through multiple regression (Aiken & West, 1991). Multiple regression is a statistical technique, based on correlation coefficients among variables, that allows predicting a single outcome variable from more than one predictor variable . For instance, Figure \(\PageIndex{11}\) shows a multiple regression analysis in which three predictor variables are used to predict a single outcome. The use of multiple regression analysis shows an important advantage of correlational research designs—they can be used to make predictions about a person’s likely score on an outcome variable (e.g., job performance) based on knowledge of other variables. It is important, however, to keep in mind that using data to make a prediction is not the same thing as being able to say that one thing causes another. Height and weight, for example, are correlated - but you can't state that one "causes" the other.
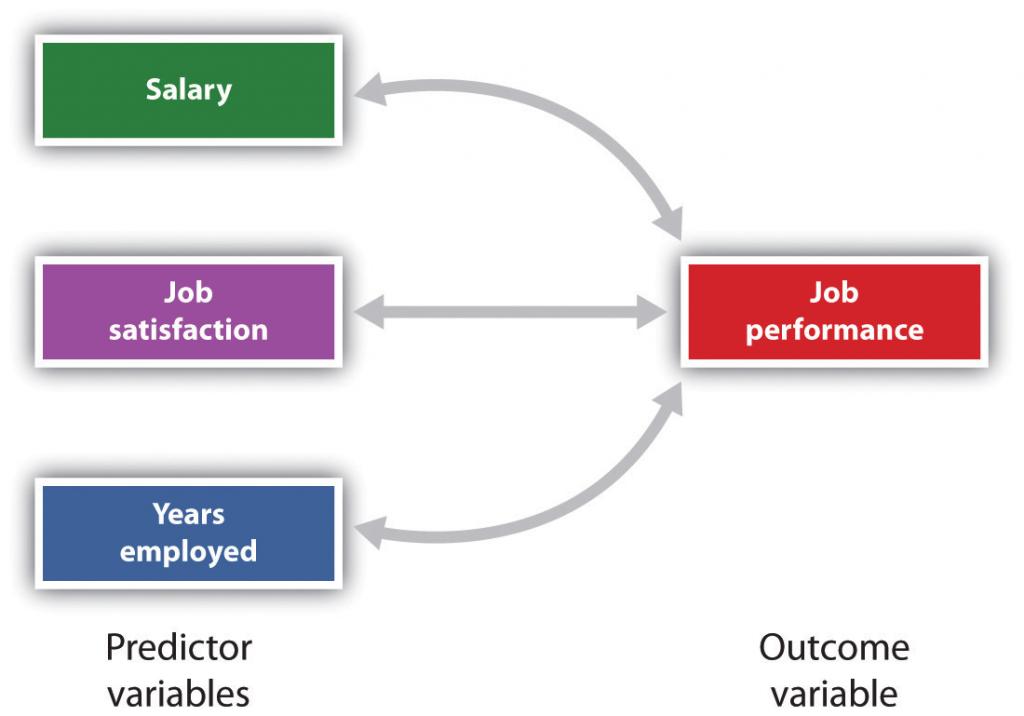
An important limitation of correlational research designs is that they cannot be used to draw conclusions about the causal relationships among the measured variables. Consider, for instance, a researcher who has hypothesized that viewing violent behavior will cause increased aggressive play in children. He has collected, from a sample of fourth-grade children, a measure of how many violent television shows each child views during the week, as well as a measure of how aggressively each child plays on the school playground. From his collected data, the researcher discovers a positive correlation between the two measured variables.
Although this positive correlation appears to support the researcher’s hypothesis, it cannot be taken to indicate that viewing violent television causes aggressive behavior. Although the researcher is tempted to assume that viewing violent television causes aggressive play,

there are other possibilities. One alternate possibility is that the causal direction is exactly opposite from what has been hypothesized. Perhaps children who have behaved aggressively at school develop residual excitement that leads them to want to watch violent television shows at home:

Although this possibility may seem less likely, there is no way to rule out the possibility of such reverse causation on the basis of this observed correlation. It is also possible that both causal directions are operating and that the two variables cause each other:

Still another possible explanation for the observed correlation is that it has been produced by the presence of a common-causal variable (also known as a third variable ). A common-causal variable is a variable that is not part of the research hypothesis but that impacts both of the measured variables and thus produces the observed correlation between them . In our example a potential common-causal variable is the discipline style of the children’s parents. Parents who use a harsh and punitive discipline style may produce children who both like to watch violent television and who behave aggressively in comparison to children whose parents use less harsh discipline:
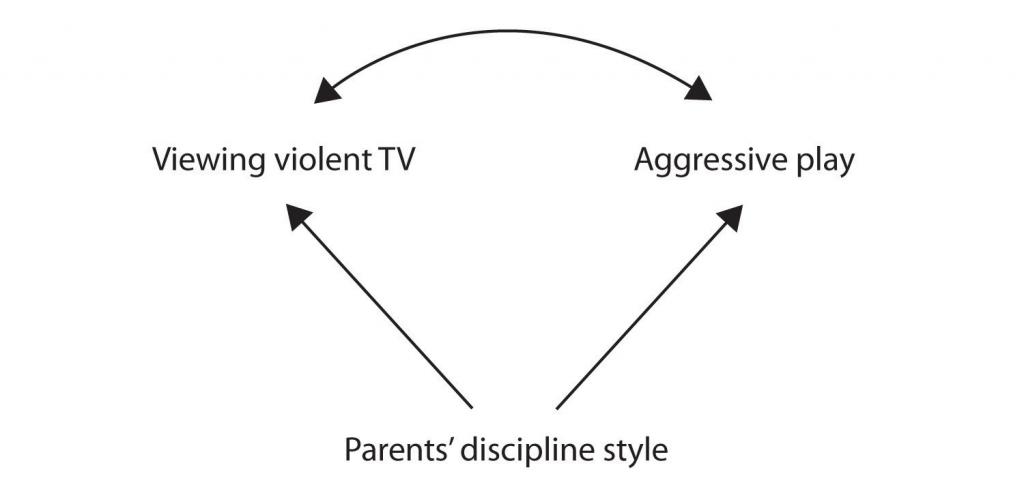
In this case, television viewing and aggressive play would be positively correlated (as indicated by the curved arrow between them), even though neither one caused the other but they were both caused by the discipline style of the parents (the straight arrows). When both of the measured variables are both impacted by a common-causal variable, the observed relationship between them is said to be spurious . A spurious relationship is a relationship between two variables in which a common-causal variable produces and “explains away” the relationship . If effects of the common-causal variable were taken away, or controlled for, the relationship between the predictor and outcome variables would disappear. In the example the relationship between aggression and television viewing might be spurious because by controlling for the effect of the parents’ disciplining style, the relationship between television viewing and aggressive behavior might go away.
Common-causal variables in correlational research designs can be thought of as “mystery” variables because, as they have not been measured, their presence and identity are usually unknown to the researcher. Since it is not possible to measure every variable that could impact your results, the existence of an unknown common-causal variable is always a possibility. For this reason, we are left with the basic limitation of correlational research: Correlation does not demonstrate causation. It is important that when you read about correlational research projects, you keep in mind the possibility of spurious relationships, and be sure to interpret the findings appropriately. Although correlational research is sometimes reported as demonstrating causality without any mention being made of the possibility of reverse causation or common-causal variables, informed consumers of research, like you, are aware of these interpretational problems. It should be noted that one is also unable to control - or measure - all variables that could impact your results in an experiment - but experiments can lead to conclusions about cause.
In sum, correlational research designs have both strengths and limitations. One strength is that they can be used when experimental research is not possible because the variables of interest cannot be manipulated. Correlational designs also have the advantage of allowing the researcher to study behavior as it occurs in everyday life. And we can also use correlational designs to make predictions—for instance, to predict from the scores on their battery of tests the success of job trainees during a training session. But we cannot use such correlational information to determine whether the training caused better job performance. For that, researchers rely on experiments.
Experimental Research: Understanding the Causes of Behavior
The goal of experimental research design is to provide more definitive conclusions about the causal relationships among the variables in the research hypothesis than is available from correlational designs. In an experimental research design, the variables of interest are called the independent variable (or variables ) and the dependent variable . The independent variable in an experiment is the causing variable that is created (manipulated) by the experimenter . The dependent variable in an experiment is a measured variable that is expected to be influenced by the experimental manipulation . The research hypothesis suggests that the manipulated independent variable or variables will cause changes in the measured dependent variables. We can diagram the research hypothesis by using an arrow that points in one direction. This demonstrates the expected direction of causality:

Research Focus: Video Games and Aggression
Consider an experiment conducted by Anderson and Dill (2000). The study was designed to test the hypothesis that viewing violent video games would increase aggressive behavior. In this research, male and female undergraduates from Iowa State University were given a chance to play with either a violent video game (Wolfenstein 3D) or a nonviolent video game (Myst). During the experimental session, the participants played their assigned video games for 15 minutes. Then, after the play, each participant played a competitive game with an opponent in which the participant could deliver blasts of white noise through the earphones of the opponent. The operational definition of the dependent variable (aggressive behavior) was the level and duration of noise delivered to the opponent. The design of the experiment is shown in Figure \(\PageIndex{17}\).
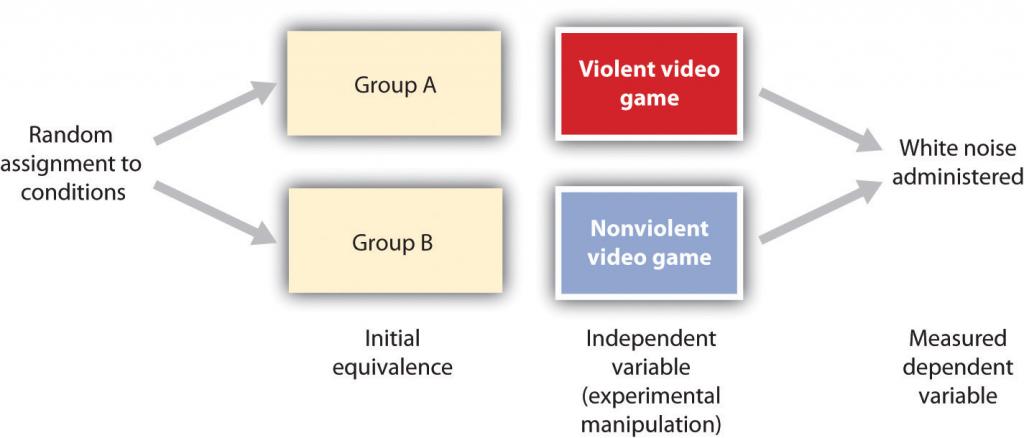
Two advantages of the experimental research design are (1) the assurance that the independent variable (also known as the experimental manipulation) occurs prior to the measured dependent variable, and (2) the creation of initial equivalence between the conditions of the experiment (in this case by using random assignment to conditions).
Experimental designs have two very nice features. For one, they guarantee that the independent variable occurs prior to the measurement of the dependent variable. This eliminates the possibility of reverse causation. Second, the influence of common-causal variables is controlled, and thus eliminated, by creating initial equivalence among the participants in each of the experimental conditions before the manipulation occurs.
The most common method of creating equivalence among the experimental conditions is through random assignment to conditions, a procedure in which the condition that each participant is assigned to is determined through a random process, such as drawing numbers out of an envelope or using a random number table . Anderson and Dill first randomly assigned about 100 participants to each of their two groups (Group A and Group B). Because they used random assignment to conditions, they could be confident that, before the experimental manipulation occurred, the students in Group A were, on average, equivalent to the students in Group B on every possible variable, including variables that are likely to be related to aggression, such as parental discipline style, peer relationships, hormone levels, diet—and in fact everything else.
Then, after they had created initial equivalence, Anderson and Dill created the experimental manipulation—they had the participants in Group A play the violent game and the participants in Group B play the nonviolent game. Then they compared the dependent variable (the white noise blasts) between the two groups, finding that the students who had viewed the violent video game gave significantly longer noise blasts than did the students who had played the nonviolent game.
Anderson and Dill had from the outset created initial equivalence between the groups. This initial equivalence allowed them to observe differences in the white noise levels between the two groups after the experimental manipulation, leading to the conclusion that it was the independent variable (and not some other variable) that caused these differences. The idea is that the only thing that was different between the students in the two groups was the video game they had played.
Despite the advantage of determining causation, experiments do have limitations. One is that they are often conducted in laboratory situations rather than in the everyday lives of people. Therefore, we do not know whether results that we find in a laboratory setting will necessarily hold up in everyday life. Second, and more important, is that some of the most interesting and key social variables cannot be experimentally manipulated. If we want to study the influence of the size of a mob on the destructiveness of its behavior, or to compare the personality characteristics of people who join suicide cults with those of people who do not join such cults, these relationships must be assessed using correlational designs, because it is simply not possible to experimentally manipulate these variables.
Key Takeaways
- Descriptive, correlational, and experimental research designs are used to collect and analyze data.
- Descriptive designs include case studies, surveys, and naturalistic observation. The goal of these designs is to get a picture of the current thoughts, feelings, or behaviors in a given group of people. Descriptive research is summarized using descriptive statistics.
- Correlational research designs measure two or more relevant variables and assess a relationship between or among them. The variables may be presented on a scatter plot to visually show the relationships. The Pearson Correlation Coefficient ( r ) is a measure of the strength of linear relationship between two variables.
- Common-causal variables may impact the measured variables in a correlational design, producing a spurious relationship. The possibility of common-causal variables makes it impossible to draw causal conclusions from correlational research designs.
- Experimental research involves the manipulation of an independent variable and the measurement of a dependent variable. Random assignment to conditions is normally used to create initial equivalence (comparability) between the groups, allowing researchers to draw causal conclusions.
Exercises and Critical Thinking
- There is a negative correlation between the row that a student sits in in a large class (when the rows are numbered from front to back) and his or her final grade in the class. Do you think this represents a causal relationship or a spurious relationship, and why?
- Think of two variables (other than those mentioned in this book) that are likely to be correlated, but in which the correlation is probably spurious. What is the likely common-causal variable that is producing the relationship?
- Imagine a researcher wants to test the hypothesis that participating in psychotherapy will cause a decrease in reported anxiety. Describe the type of research design the investigator might use to draw this conclusion. What would be the independent and dependent variables in the research?
Updates and Edits
This page was forked and modified on January 14, 2021. Slight language modifications were made and references to "predictor" and "outcome" variables with respect to correlational data were modified. While correlational data can be be used to make predictions, referring to the variables in a correlational study as predictors and outcomes may inappropriately imply a causal relationship.
Aiken, L., & West, S. (1991). Multiple regression: Testing and interpreting interactions . Newbury Park, CA: Sage.
Ainsworth, M. S., Blehar, M. C., Waters, E., & Wall, S. (1978). Patterns of attachment: A psychological study of the strange situation . Hillsdale, NJ: Lawrence Erlbaum Associates.
Anderson, C. A., & Dill, K. E. (2000). Video games and aggressive thoughts, feelings, and behavior in the laboratory and in life. Journal of Personality and Social Psychology, 78 (4), 772–790.
Damasio, H., Grabowski, T., Frank, R., Galaburda, A. M., Damasio, A. R., Cacioppo, J. T., & Berntson, G. G. (2005). The return of Phineas Gage: Clues about the brain from the skull of a famous patient. In Social neuroscience: Key readings. (pp. 21–28). New York, NY: Psychology Press.
Freud, S. (1964). Analysis of phobia in a five-year-old boy. In E. A. Southwell & M. Merbaum (Eds.), Personality: Readings in theory and research (pp. 3–32). Belmont, CA: Wadsworth. (Original work published 1909)
Kotowicz, Z. (2007). The strange case of Phineas Gage. History of the Human Sciences, 20 (1), 115–131.
Rokeach, M. (1964). The three Christs of Ypsilanti: A psychological study . New York, NY: Knopf.
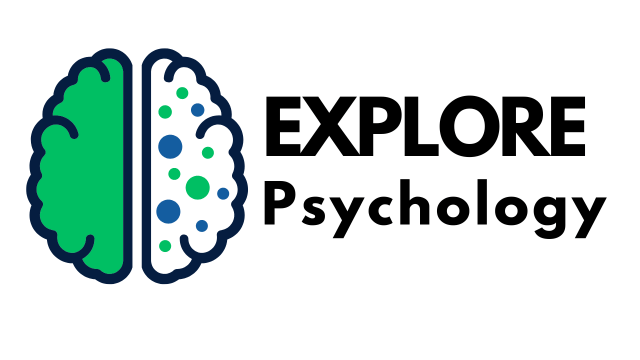
Correlational Research in Psychology: Definition and How It Works
Categories Research Methods
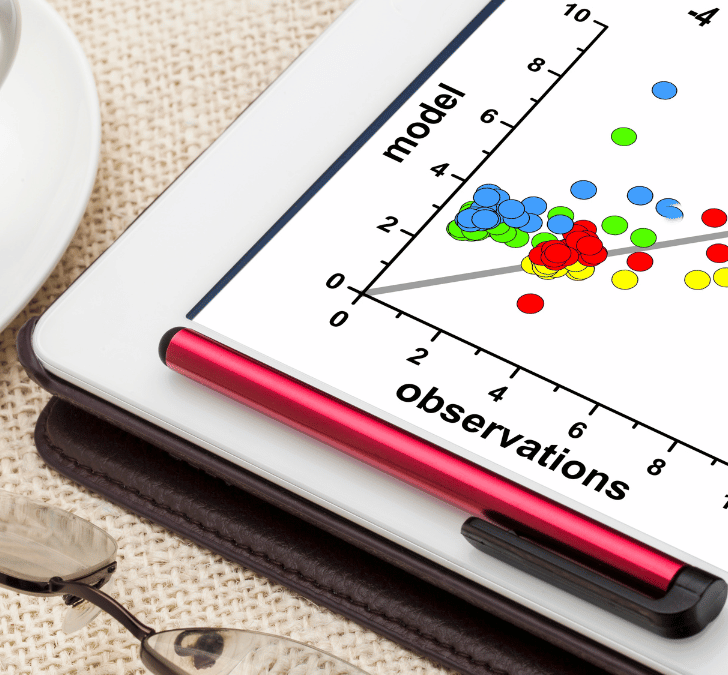
Sharing is caring!
Correlational research is a type of scientific investigation in which a researcher looks at the relationships between variables but does not vary, manipulate, or control them. It can be a useful research method for evaluating the direction and strength of the relationship between two or more different variables.
When examining how variables are related to one another, researchers may find that the relationship is positive or negative. Or they may also find that there is no relationship at all.
Table of Contents
How Does Correlational Research Work?
In correlational research, the researcher measures the values of the variables of interest and calculates a correlation coefficient, which quantifies the strength and direction of the relationship between the variables.
The correlation coefficient ranges from -1.0 to +1.0, where -1.0 represents a perfect negative correlation, 0 represents no correlation, and +1.0 represents a perfect positive correlation.
A negative correlation indicates that as the value of one variable increases, the value of the other variable decreases, while a positive correlation indicates that as the value of one variable increases, the value of the other variable also increases. A zero correlation indicates that there is no relationship between the variables.
Correlational Research vs. Experimental Research
Correlational research differs from experimental research in that it does not involve manipulating variables. Instead, it focuses on analyzing the relationship between two or more variables.
In other words, correlational research seeks to determine whether there is a relationship between two variables and, if so, the nature of that relationship.
Experimental research, on the other hand, involves manipulating one or more variables to determine the effect on another variable. Because of this manipulation and control of variables, experimental research allows for causal conclusions to be drawn, while correlational research does not.
Both types of research are important in understanding the world around us, but they serve different purposes and are used in different situations.
Types of Correlational Research
There are three main types of correlational studies:
Cohort Correlational Study
This type of study involves following a cohort of participants over a period of time. This type of research can be useful for understanding how certain events might influence outcomes.
For example, researchers might study how exposure to a traumatic natural disaster influences the mental health of a group of people over time.
By examining the data collected from these individuals, researchers can determine whether there is a correlation between the two variables under investigation. This information can be used to develop strategies for preventing or treating certain conditions or illnesses.
Cross-Sectional Correlational Study
A cross-sectional design is a research method that examines a group of individuals at a single time. This type of study collects information from a diverse group of people, usually from different backgrounds and age groups, to gain insight into a particular phenomenon or issue.
The data collected from this type of study is used to analyze relationships between variables and identify patterns and trends within the group.
Cross-sectional studies can help identify potential risk factors for certain conditions or illnesses, and can also be used to evaluate the prevalence of certain behaviors, attitudes, or beliefs within a population.
Case-Control Correlational Study
A case-control correlational study is a type of research design that investigates the relationship between exposure and health outcomes. In this study, researchers identify a group of individuals with the health outcome of interest (cases) and another group of individuals without the health outcome (controls).
The researchers then compare the exposure history of the cases and controls to determine whether the exposure and health outcome correlate.
This type of study design is often used in epidemiology and can provide valuable information about potential risk factors for a particular disease or condition.
When to Use Correlational Research
There are a number of situations where researchers might opt to use a correlational study instead of some other research design.
Correlational research can be used to investigate a wide range of psychological phenomena, including the relationship between personality traits and academic performance, the association between sleep duration and mental health, and the correlation between parental involvement and child outcomes.
To Generate Hypotheses
Correlational research can also be used to generate hypotheses for further research by identifying variables that are associated with each other.
To Investigate Variables Without Manipulating Them
Researchers should use correlational research when they want to investigate the relationship between two variables without manipulating them. This type of research is useful when the researcher cannot or should not manipulate one of the variables or when it is impossible to conduct an experiment due to ethical or practical concerns.
To Identify Patterns
Correlational research allows researchers to identify patterns and relationships between variables, which can inform future research and help to develop theories. However, it is important to note that correlational research does not prove that one variable causes changes in the other.
While correlational research has its limitations, it is still a valuable tool for researchers in many fields, including psychology, sociology, and education.
How to Collect Data in Correlational Research
Researchers can collect data for correlational research in a few different ways. To conduct correlational research, data can be collected using the following:
- Surveys : One method is through surveys, where participants are asked to self-report their behaviors or attitudes. This approach allows researchers to gather large amounts of data quickly and affordably.
- Naturalistic observation : Another method is through observation, where researchers observe and record behaviors in a natural or controlled setting. This method allows researchers to learn more about the behavior in question and better generalize the results to real-world settings.
- Archival, retrospective data : Additionally, researchers can collect data from archival sources, such as medical, school records, official records, or past polls.
The key is to collect data from a large and representative sample to measure the relationship between two variables accurately.
Pros and Cons of Correlational Research
There are some advantages of using correlational research, but there are also some downsides to consider.
- One of the strengths of correlational research is its ability to identify patterns and relationships between variables that may be difficult or unethical to manipulate in an experimental study.
- Correlational research can also be used to examine variables that are not under the control of the researcher , such as age, gender, or socioeconomic status.
- Correlational research can be used to make predictions about future behavior or outcomes, which can be valuable in a variety of fields.
- Correlational research can be conducted quickly and inexpensively , making it a practical option for researchers with limited resources.
- Correlational research is limited by its inability to establish causality between variables. Correlation does not imply causation, and it is possible that a third variable may be influencing both variables of interest, creating a spurious correlation. Therefore, it is important for researchers to use multiple methods of data collection and to be cautious when interpreting correlational findings.
- Correlational research relies heavily on self-reported data , which can be biased or inaccurate.
- Correlational research is limited in its ability to generalize findings to larger populations, as it only measures the relationship between two variables in a specific sample.
Frequently Asked Questions About Correlational Research
What are the main problems with correlational research.
Some of the main problems that can occur in correlational research include selection bias, confounding variables. and misclassification.
- Selecting participants based on their exposure to an event means that the sample might be biased since the selection was not randomized.
- Correlational studies may also be impacted by extraneous factors that researchers cannot control.
- Finally, there may be problems with how accurately data is recorded and classified, which can be particularly problematic in retrospective studies.
What are the variables in a correlational study?
In a correlational study, variables refer to any measurable factors being examined for their potential relationship or association with each other. These variables can be continuous (meaning they can take on a range of values) or categorical (meaning they fall into distinct categories or groups).
For example, in a study examining the correlation between exercise and mental health, the independent variable would be exercise frequency (measured in times per week), while the dependent variable would be mental health (measured using a standardized questionnaire).
What is the goal of correlational research?
The goal of correlational research is to examine the relationship between two or more variables. It involves analyzing data to determine if there is a statistically significant connection between the variables being studied.
Correlational research is useful for identifying patterns and making predictions but cannot establish causation. Instead, it helps researchers to better understand the nature of the relationship between variables and to generate hypotheses for further investigation.
How do you identify correlational research?
To identify correlational research, look for studies that measure two or more variables and analyze their relationship using statistical techniques. The results of correlational studies are typically presented in the form of correlation coefficients or scatterplots, which visually represent the degree of association between the variables being studied.
Correlational research can be useful for identifying potential causal relationships between variables but cannot establish causation on its own.
Curtis EA, Comiskey C, Dempsey O. Importance and use of correlational research . Nurse Researcher . 2016;23(6):20-25. doi10.7748/nr.2016.e1382
Lau F. Chapter 12 Methods for Correlational Studies . University of Victoria; 2017.
Mitchell TR. An evaluation of the validity of correlational research conducted in organizations . The Academy of Management Review . 1985;10(2):192. doi:10.5465/amr.1985.4277939
Seeram E. An overview of correlational research . Radiol Technol . 2019;91(2):176-179.
Have a language expert improve your writing
Run a free plagiarism check in 10 minutes, generate accurate citations for free.
- Knowledge Base
Methodology
- Correlational Research | When & How to Use
Correlational Research | When & How to Use
Published on July 7, 2021 by Pritha Bhandari . Revised on June 22, 2023.
A correlational research design investigates relationships between variables without the researcher controlling or manipulating any of them.
A correlation reflects the strength and/or direction of the relationship between two (or more) variables. The direction of a correlation can be either positive or negative.
Table of contents
Correlational vs. experimental research, when to use correlational research, how to collect correlational data, how to analyze correlational data, correlation and causation, other interesting articles, frequently asked questions about correlational research.
Correlational and experimental research both use quantitative methods to investigate relationships between variables. But there are important differences in data collection methods and the types of conclusions you can draw.
Receive feedback on language, structure, and formatting
Professional editors proofread and edit your paper by focusing on:
- Academic style
- Vague sentences
- Style consistency
See an example
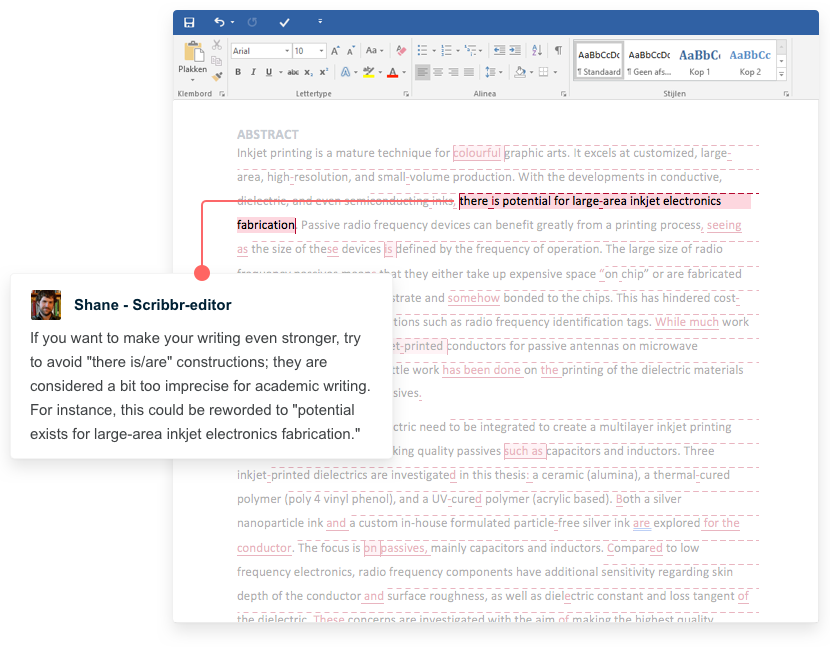
Correlational research is ideal for gathering data quickly from natural settings. That helps you generalize your findings to real-life situations in an externally valid way.
There are a few situations where correlational research is an appropriate choice.
To investigate non-causal relationships
You want to find out if there is an association between two variables, but you don’t expect to find a causal relationship between them.
Correlational research can provide insights into complex real-world relationships, helping researchers develop theories and make predictions.
To explore causal relationships between variables
You think there is a causal relationship between two variables, but it is impractical, unethical, or too costly to conduct experimental research that manipulates one of the variables.
Correlational research can provide initial indications or additional support for theories about causal relationships.
To test new measurement tools
You have developed a new instrument for measuring your variable, and you need to test its reliability or validity .
Correlational research can be used to assess whether a tool consistently or accurately captures the concept it aims to measure.
There are many different methods you can use in correlational research. In the social and behavioral sciences, the most common data collection methods for this type of research include surveys, observations , and secondary data.
It’s important to carefully choose and plan your methods to ensure the reliability and validity of your results. You should carefully select a representative sample so that your data reflects the population you’re interested in without research bias .
In survey research , you can use questionnaires to measure your variables of interest. You can conduct surveys online, by mail, by phone, or in person.
Surveys are a quick, flexible way to collect standardized data from many participants, but it’s important to ensure that your questions are worded in an unbiased way and capture relevant insights.
Naturalistic observation
Naturalistic observation is a type of field research where you gather data about a behavior or phenomenon in its natural environment.
This method often involves recording, counting, describing, and categorizing actions and events. Naturalistic observation can include both qualitative and quantitative elements, but to assess correlation, you collect data that can be analyzed quantitatively (e.g., frequencies, durations, scales, and amounts).
Naturalistic observation lets you easily generalize your results to real world contexts, and you can study experiences that aren’t replicable in lab settings. But data analysis can be time-consuming and unpredictable, and researcher bias may skew the interpretations.
Secondary data
Instead of collecting original data, you can also use data that has already been collected for a different purpose, such as official records, polls, or previous studies.
Using secondary data is inexpensive and fast, because data collection is complete. However, the data may be unreliable, incomplete or not entirely relevant, and you have no control over the reliability or validity of the data collection procedures.
After collecting data, you can statistically analyze the relationship between variables using correlation or regression analyses, or both. You can also visualize the relationships between variables with a scatterplot.
Different types of correlation coefficients and regression analyses are appropriate for your data based on their levels of measurement and distributions .
Correlation analysis
Using a correlation analysis, you can summarize the relationship between variables into a correlation coefficient : a single number that describes the strength and direction of the relationship between variables. With this number, you’ll quantify the degree of the relationship between variables.
The Pearson product-moment correlation coefficient , also known as Pearson’s r , is commonly used for assessing a linear relationship between two quantitative variables.
Correlation coefficients are usually found for two variables at a time, but you can use a multiple correlation coefficient for three or more variables.
Regression analysis
With a regression analysis , you can predict how much a change in one variable will be associated with a change in the other variable. The result is a regression equation that describes the line on a graph of your variables.
You can use this equation to predict the value of one variable based on the given value(s) of the other variable(s). It’s best to perform a regression analysis after testing for a correlation between your variables.
It’s important to remember that correlation does not imply causation . Just because you find a correlation between two things doesn’t mean you can conclude one of them causes the other for a few reasons.
Directionality problem
If two variables are correlated, it could be because one of them is a cause and the other is an effect. But the correlational research design doesn’t allow you to infer which is which. To err on the side of caution, researchers don’t conclude causality from correlational studies.
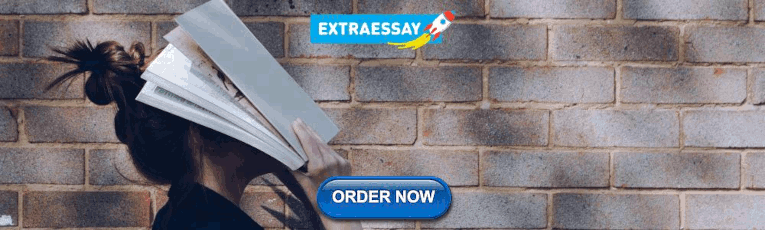
Third variable problem
A confounding variable is a third variable that influences other variables to make them seem causally related even though they are not. Instead, there are separate causal links between the confounder and each variable.
In correlational research, there’s limited or no researcher control over extraneous variables . Even if you statistically control for some potential confounders, there may still be other hidden variables that disguise the relationship between your study variables.
Although a correlational study can’t demonstrate causation on its own, it can help you develop a causal hypothesis that’s tested in controlled experiments.
If you want to know more about statistics , methodology , or research bias , make sure to check out some of our other articles with explanations and examples.
- Normal distribution
- Degrees of freedom
- Null hypothesis
- Discourse analysis
- Control groups
- Mixed methods research
- Non-probability sampling
- Quantitative research
- Ecological validity
Research bias
- Rosenthal effect
- Implicit bias
- Cognitive bias
- Selection bias
- Negativity bias
- Status quo bias
A correlation reflects the strength and/or direction of the association between two or more variables.
- A positive correlation means that both variables change in the same direction.
- A negative correlation means that the variables change in opposite directions.
- A zero correlation means there’s no relationship between the variables.
A correlational research design investigates relationships between two variables (or more) without the researcher controlling or manipulating any of them. It’s a non-experimental type of quantitative research .
Controlled experiments establish causality, whereas correlational studies only show associations between variables.
- In an experimental design , you manipulate an independent variable and measure its effect on a dependent variable. Other variables are controlled so they can’t impact the results.
- In a correlational design , you measure variables without manipulating any of them. You can test whether your variables change together, but you can’t be sure that one variable caused a change in another.
In general, correlational research is high in external validity while experimental research is high in internal validity .
A correlation is usually tested for two variables at a time, but you can test correlations between three or more variables.
A correlation coefficient is a single number that describes the strength and direction of the relationship between your variables.
Different types of correlation coefficients might be appropriate for your data based on their levels of measurement and distributions . The Pearson product-moment correlation coefficient (Pearson’s r ) is commonly used to assess a linear relationship between two quantitative variables.
Cite this Scribbr article
If you want to cite this source, you can copy and paste the citation or click the “Cite this Scribbr article” button to automatically add the citation to our free Citation Generator.
Bhandari, P. (2023, June 22). Correlational Research | When & How to Use. Scribbr. Retrieved April 13, 2024, from https://www.scribbr.com/methodology/correlational-research/
Is this article helpful?
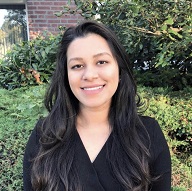
Pritha Bhandari
Other students also liked, what is quantitative research | definition, uses & methods, correlation vs. causation | difference, designs & examples, correlation coefficient | types, formulas & examples, unlimited academic ai-proofreading.
✔ Document error-free in 5minutes ✔ Unlimited document corrections ✔ Specialized in correcting academic texts

An official website of the United States government
The .gov means it’s official. Federal government websites often end in .gov or .mil. Before sharing sensitive information, make sure you’re on a federal government site.
The site is secure. The https:// ensures that you are connecting to the official website and that any information you provide is encrypted and transmitted securely.
- Publications
- Account settings
Preview improvements coming to the PMC website in October 2024. Learn More or Try it out now .
- Advanced Search
- Journal List
- HHS Author Manuscripts

Interpretation of correlations in clinical research
1 University of Utah Department of Orthopaedic Surgery Operations, Salt Lake City, Utah, USA
Jerry Bounsanga
Maren wright voss, background:.
Critically analyzing research is a key skill in evidence-based practice and requires knowledge of research methods, results interpretation, and applications, all of which rely on a foundation based in statistics. Evidence-based practice makes high demands on trained medical professionals to interpret an ever-expanding array of research evidence.
As clinical training emphasizes medical care rather than statistics, it is useful to review the basics of statistical methods and what they mean for interpreting clinical studies.
We reviewed the basic concepts of correlational associations, violations of normality, unobserved variable bias, sample size, and alpha inflation. The foundations of causal inference were discussed and sound statistical analyses were examined. We discuss four ways in which correlational analysis is misused, including causal inference overreach, over-reliance on significance, alpha inflation, and sample size bias.
Recent published studies in the medical field provide evidence of causal assertion overreach drawn from correlational findings. The findings present a primer on the assumptions and nature of correlational methods of analysis and urge clinicians to exercise appropriate caution as they critically analyze the evidence before them and evaluate evidence that supports practice.
Conclusion:
Critically analyzing new evidence requires statistical knowledge in addition to clinical knowledge. Studies can overstate relationships, expressing causal assertions when only correlational evidence is available. Failure to account for the effect of sample size in the analyses tends to overstate the importance of predictive variables. It is important not to overemphasize the statistical significance without consideration of effect size and whether differences could be considered clinically meaningful.
Introduction
It is common for physicians to underestimate the relevance and importance of their training in statistics and probability, at least until its relevance becomes clear in later clinical practice. 1 Evidence based practice is the standard of care, yet evaluating the quality of evidence can be a difficult process. The British Medical Journal established clear statistical guidelines for contributions to medical journals in the 1980’s. 2 A survey of medical residents showed near perfect agreement (95%) that understanding statistics they encounter in medical journals is important, but 75% said they lacked knowledge. 3 The average score on the basic biostatistics exam for the residents surveyed was 41% correct, showing both the objective and subjective need for a stronger statistical training foundation. An international survey of practicing doctors similarly found that doctors averaged scores of 40% when tested on the basics of statistical methods and epidemiology. 4
The efforts to improve the methods behind clinical science are ongoing. Consolidated Standards of Reporting Trials (CONSORT) have been established, 5 along with multiple other research and statistical method guidelines in recent years. 6 These guidelines are updated periodically 7 and endorsed or extended by specific clinical medical groups, 8 , 9 or medical journals. 10 – 13 Key to many of these guidelines are attempts to ensure that bias and error are minimized in research, ensuring interpretations are meaningful and accurate. It would not be practical to detail an exhaustive list of guidance and recommendations. However, with new information and research approaches constantly coming forward, physicians must have the ability to critically evaluate the quality of evidence presented. Critically analyzing research is a key skill in evidence based practice and requires knowledge of methods, results interpretation, and applicability—all three of which require an understanding of basic statistics. 14
The intention of this article is to serve as a basic primer regarding critical statistical concepts that appear in medical literature with a focus on the concept of correlation and how it is best utilized in clinical interpretation for understanding the relationships between health factors. At its foundation, correlational analysis quantifies the direction and strength of relationships between two variables. Understanding correlations can form the basis for interpreting applications of clinical research.
1. What Does Correlation Tell Us?
Correlation is concerned with association; it can look at any two measured concepts and compare their relationships. These measured concepts are often referred to as variables and are assigned letter labels (X, Y). Thus, the correlation is the measure of the relationship between X and Y, and it ranges from −1 to 1. Its value (or coefficient) is scaled within this range to assist in interpretation, with 0 indicating no relationship between variables X and Y, and −1 or 1 indicating the ability to perfectly predict X from Y or Y from X (see Figure 1 ). A correlation coefficient provides two pieces of information. First, it predicts where X (the measured value of interest) falls on a line given a known value of Y. Second, it expresses a reduction in variability associated with knowing Y, telling us something about the expected range of the X value. 15 Correlation takes into account the full range of scores, but as a statistical tool it is not very sensitive to scores on the very high or very low ends of our X value. The common Pearson correlation is best used to describe linear relationships. If the pattern of association between the two variables is, for example, a “U” shaped curve, the correlation results might be low, even though a defined relationship exists. 16

1) Perfect negative correlation between two variables; 2) No patterned relationship between 2 variables; 3) Perfect positive correlation between two variables
Correlation is not easily impacted by skewed or off-center data results. The nature of the data can be described by its parameters, like measures of central tendency, which inform us about the distribution of the data. Parametric tests make assumptions, such as the data are normally distributed, while non-parametric tests are called distribution-free tests because they make no assumptions about the distribution of the data. Yet even if the pattern of scores are not in a normal bell shaped curve or they don’t create a direct linear relationship, correlations can still be reliable. A number of correlation measures have been developed to handle different types of data (non-parametric tests like the kendall rank, spearman rank correlation, phi correlation, biserial correlation, point-biserial correlation and gamma correlation). Yet even the simple Pearson correlation handles extreme violations of normality (no bell shape to the pattern of scores) and scale. The Pearson correlation was tested by randomly drawing 5,000 small samples (n=5 to n=15) from a population of 10,000 to calculate the distribution of r values yielded (small samples might challenge parametric assumptions), and was still found to be a reliable indicator of the relationship between variables. 17 It is also possible to use transformations to normalize the distribution of data. Correlation can be a robust measure, in part from its ability to tolerate these violations of normally distributed data while staying sensitive to the individual case. The properties of correlation make the technique useful in interpreting the meaning derived from clinical data.
2. Use of Correlation
There are many concerns with the statistical techniques that are commonly utilized in the literature. Some concerns arise from a misunderstanding of the statistical measure and others from its misapplication. We discuss four ways in which correlational analysis is misused, including causal inference overreach, over-reliance on significance, alpha inflation, and sample size bias. Importantly, correlation is a measure of association, which is insufficient to infer causation. Correlation can only measure whether a relationship exists between two variables, but it does not indicate causal relationship. There must be a convincing body of evidence to take the next step on the path to inferring that one variable causes the other. Randomized controlled trials or more advanced statistical methods such as path analysis and structural equation modeling, coupled with proper research design, are needed in order to take the next step of inference in the causal chain, testing a causal hypothesis. While correlational analyses are by definition from non-experimental research, research without carefully controlled experimental conditions, it is nevertheless relevant to evidence-based practice. 18 Observational methods of study can be conducted using either a cross-sectional design (a snap-shot of prevalence at one time-point), a retrospective design (looking back to compare current with past attributes) or prospective design (documenting current occurrences and following up at a future time-point to make comparisons). Correlations drawn from cross-sectional studies cannot establish the temporal relationship that links cause with effect, yet adding a retrospective or prospective observational design provides additional strength to the association and helps support hypothesis generation to then later test the causal assertions with a different research design. 19
When non-experimental methods are used, it means the relationship seen between the two variables is vulnerable to bias from anything that was not measured (unobserved variables). Whether studying pain or function or treatment response, there are a host of possible factors that might be important to the observed correlational relationship between X and Y. Any given study has only measured and reported on a fraction of the potential variables of impact. These unobserved variables could potentially explain the observed relationship, so it would be premature to assume a treatment effect based on correlational data. The unobserved variables might be affecting the study variables, changing the relationship in a way that might alter the interpretation of the data. Thus interpretation of correlational findings must be quite cautious until further research is completed.
Another concern is the use (or abuse) of the term ‘statistically significant’ in correlational analysis. This concern is not new. The abuse of significance testing was noted in a 1987 review published in the New England Journal of Medicine. That article found that a number of components in clinical trials, such as having several measures of the outcome (i.e., multiple tests of function, health, or pain), repeated measures over time, including subgroup analyses, or multiple treatments in the same trial, can lead to a bias in reporting which exaggerates the size or importance of observed differences. 20 It is natural for researchers to want to thoroughly evaluate the potential difference between treatments conditions. This has been sometimes referred to as the kitchen sink approach and it presents a problem for using significance tests. Significance as a statistical procedure addresses the question of the probability of the hypothesized occurrence. If the probability ( p ) is less than say 5% or 1%, the researcher might feel comfortable making the assumption that the observed event was not due to chance. The 0.05 significance value was original proposed by Sir Ronald Fisher in 1925, but the 0.05 value was never intended as a hard and fast rule. 21 If researchers use a cut-off of p=0.05 to determine whether the effect they see in their research has occurred randomly by chance, running multiple tests can quickly move the needle from a rare to an expected event. Every analysis run with a p =0.05 alpha criteria yields a 5% chance that the “significant” finding is actually a chance occurrence, called a Type 1 error.
So we can see the difficulty that occurs when running 20 different analyses on the same data. This would produce a 64% chance that a significant p -value will show up erroneously, when there is no systematic relationship, and it is really just a chance occurrence (see Figure 2 ). This over analysis of the data can possibly create an interpretation that a test or treatment should be used when there was no actual treatment effect. This is the problem of alpha-inflation and it needs to be carefully considered both in conducting and interpreting correlational research. It can be corrected by planning ahead for the analyses that will be run and keeping them limited to key theoretical questions. 20 Additionally, alpha can be adjusted in the statistical calculation, for example with the Bonferroni correction, a procedure used to reduce alpha and statistically correct for the inflation created by multiple comparisons. It is performed by simply dividing the alpha value (α) by the number of hypotheses or measures ( m ) tested. If the study wanted to evaluate 5 different surgical placements, using the Bonferroni correction would adjust an original α=0.05 by applying the formula, α/ m or 0.05/5, yielding α=0.01, a stricter standard before a study finding would be considered significant. A Bonferroni correction is a conservative correction for multiple comparisons that reduces the Type 1 error, 22 though less conservative alternatives exist like the Tukey or the Holm-Sidak corrections. The main idea is that clinicians acknowledge the problem of multiple comparisons made in a single study and address the concern so that spurious relationships are not erroneously reported as significant. A lack of awareness about this issue can lead to naïve interpretations of study findings.

As the number of comparisons increases, the alpha error rate increases; running 20 comparisons with no correction factor and a significance level set at 0.05 will result in 64% chance of a relationship appearing to be significant (better than 50/50 odds).
Exaggeration of significance testing leads to a third point - are the findings clinically meaningful? A significant finding does not infer a meaningful finding. This is because factors other than variance in scores influence the p -value or significance in a correlational analysis. Sample size is an important element in whether a non-random effect will be found. Small sample sizes might produce unstable, but significant, correlation estimates, so sample sizes greater than 150 to 200 have been recommended. 23 Yet, it is not uncommon for published papers to report significant effects through correlational analysis of sample sizes of less than 150 patients. 24 – 26 While reporting and publishing both the significant and non-significant results are important, given the instability that comes from a small sample size, there should be caution taken with interpretation until replication studies can verify the findings.
Likewise, large samples can also be problematic. A large sample might reveal a statistically significant difference between groups, but its effect might be minimal. In a classic example, a sample of 22,000 subjects showed a highly significant ( p <.00001) reduction in myocardial infarctions that prompted a general recommendation to take aspirin for myocardial infarction prevention. 27 The effect size, however, was less than a 1% reduction in risk, such that the risk of taking aspirin exceeded the benefit. Effect size is the standardized mean difference between groups and is a measure of the magnitude of between group differences. A significant p -value indicates only that a difference exists with no indication of size of the effect. Additionally, a confidence interval (CI) can be constructed for the effect size. CIs present a lower and upper range where the true population value is most likely to lie. 28 If a zero value is not included within the CI of the effect size, we have added assurance that the effect exists, with the size of the CI helpful in estimating the size of the effect. 29 It has been recommended that effect sizes or confidence intervals be included in all reported medical research so that the clinical significance of findings can be assessed. 20 , 28 , 30
3. Proper Interpretation of Correlation
Correlational analyses have been reported as one of the most common analytic techniques in research at the beginning of the 21 st century, particularly in health and epidemiological research. 15 Thus effective and proper interpretation is critical to understanding the literature. Cautious interpretation is particularly important, not only due to the interpretive concerns just detailed (causal inference overreach, over-reliance on significance, alpha inflation, and sample size bias), but also given the publication bias of journals to accept and publish studies with positive findings. 31 If clinicians are less likely to be exposed to under-published contradictory reports, based on null findings that treatments actually had no effect, the interpretation of the positive results must necessarily be cautious until confirmed through strong evidence.
One recent clinical example of correlational findings is an inference that because Cobb angle and sagittal balance are related to symptom severity in back pain, treatments should be aimed to improve sagittal balance. Studies used to draw these conclusions were making an important first step in identifying potentially relationships, but were not conclusive as they did not establish causal relationships, did not report effect sizes, and did not include control groups in the analyses. 32 – 34 The Pearson correlation or the Spearman correlation are tools that predict X from Y or Y from X. The nature of the correlation is symmetric, so that if the variables are inferred from a reversed direction (pain predicting spine function rather than spine function predicting pain), the same prediction holds true. 15 If one is looking for cause and effect, the correlational statistics cannot help. The mathematics of correlation tells us that Y is just as likely to precede X as to come after, because the prediction is the same regardless of which variable is inputted first. Effects cannot be determined directly through correlational analysis and perhaps the reverse relationship is the true relationship.
Because of the possibility of a bidirectional relationship, causal inference will be premature if relying purely on correlational statistics, no matter how many studies report the correlational finding. Correlation can be interpreted as the association between two variables. It cannot be used to indicate causal relationship. In fact, statistical tests cannot prove causal relationships but can only be used to test causal hypotheses. Misinterpretation of correlation is generally related to a lack of understanding of what a statistical test can or cannot do, as well as lacking knowledge in proper research design. Rather than jumping to an assumption of causality, the correlations should prompt the next stage of clinical research through randomly controlled clinical trials or the application of more complex statistical methods such as causal and path analysis. Perhaps a part of the tendency to jump too quickly to causal assertion arises from the nature of the questions asked in clinical research and the desire to quickly move to enhance patient care. New frameworks are emerging in the health sciences that challenge the appeal to a single cause by considering potential outcomes in a more complex ways. 35 Until then, understanding the nature of correlational analysis allows clinicians to be more cautious in interpreting study results.
Advances in research have led to many significant findings that are shaping how we diagnose and treat patients. As these findings might guide surgeons and clinicians into new treatment directions, it is important to consider the strength and nature of the research. Critically analyzing new evidence requires understanding of research methods and relevant statistical applications, all of which require an understanding of the analytic methodologies that lie behind the study findings.14 Evidence based practice is demanding new skills of trained medical professionals as they are presented with an ever-expanding array of research evidence. This short primer on theassumptions and nature of correlational methods of analysis can assist emerging physicians in understanding and exercising the appropriate caution as they critically analyze the evidence before them.
Acknowledgments
This investigation was supported by the University of Utah Department of Orthopaedics Quality Outcomes Research and Assessment, Study Design and Biostatistics Center, with funding in part from the National Center for Research Resources and the National Center for Advancing Translational Sciences, National Institutes of Health, through Grant 5UL1TR001067–02.
Declaration of Interests
The authors have no relevant affiliations or financial involvement with any organization or entity with a financial interest in or financial conflict with the subject matter or materials discussed in the manuscript. This includes employment, consultancies, honoraria, stock ownership or options, expert testimony, grants or patents received or pending, or royalties.
- Primary research
- Open access
- Published: 28 August 2013
Correlational study: illness representations and coping styles in caregivers for individuals with schizophrenia
- Shyhrete Rexhaj 1 , 2 ,
- Nataly Viens Python 2 ,
- Diane Morin 3 ,
- Charles Bonsack 1 &
- Jérôme Favrod 1 , 2
Annals of General Psychiatry volume 12 , Article number: 27 ( 2013 ) Cite this article
30k Accesses
25 Citations
2 Altmetric
Metrics details
Caring for individuals with schizophrenia can create distress for caregivers which can, in turn, have a harmful impact on patient progress. There could be a better understanding of the connections between caregivers’ representations of schizophrenia and coping styles. This study aims at exploring those connections.
This correlational descriptive study was conducted with 92 caregivers of individuals suffering from schizophrenia. The participants completed three questionnaires translated and validated in French: (a) a socio-demographic questionnaire, (b) the Illness Perception Questionnaire for Schizophrenia and (c) the Family Coping Questionnaire.
Our results show that illness representations are slightly correlated with coping styles. More specifically, emotional representations are correlated to an emotion-focused coping style centred on coercion, avoidance and resignation.
Our results are coherent with the Commonsense Model of Self-Regulation of Health and Illness and should enable to develop new interventions for caregivers.
Problem statement
Between 30% and 91% of individuals with schizophrenia live in a family setting [ 1 – 3 ]. The decreased length of stay in hospital and restrictions on involuntary treatments mean that family-based caregivers provide an important support during periods of psychological instability. This support implies that caregivers rely on a variety of strategies to confront the consequences resulting from the psychological instability of the schizophrenia patient.
Burden, distress, illness representations and coping strategies
The literature indicates that there are interactions between the different concepts of family burden, distress, illness representations, the expressed emotion (EE) and coping strategies. It has been demonstrated that caregivers’ representations of negative consequences of the illness for the patient are positively correlated with their objective burden, while representations of the negative consequences of schizophrenia for the caregiver are positively correlated to a subjective burden [ 4 , 5 ]. The caregivers’ subjective burden is also associated with negative emotional responses to the illness [ 5 ]. Also, caregivers having an elevated and hostile type of EE usually consider the patient responsible for the causes of illness [ 6 , 7 ]. Furthermore, in comparison with caregivers having a low EE, those having a high, critical-type EE tend to underestimate their possibilities of controlling problems themselves and perceive the illness as unlikely to be controlled by treatment as well as attribute more negative consequences of the illness for the patient and themselves [ 4 ].
Distress experienced by caregivers is also correlated with illness representations such as the following: (1) illness outbreaks are chronic, (2) the feeling that treatment does not help control the illness, (3) the perception that the patient can have greater personal control over the illness, (4) the perception that the illness brings about negative consequences for the patient and (5) that the illness brings about negative consequences for the caregiver and (6) representations that the illness elicits painful emotions such as anxiety and fear [ 4 , 5 , 8 ].
Coping strategies by caregivers of patients with schizophrenia have been explored by several authors who mainly based their observations using models or frameworks derived from Lazarus and Folkman’s theory of stress coping [ 9 – 12 ]. A review of the literature showed that there are strategies that are more or less efficient for confronting stress. More specifically for caregivers, strategies like coercion, avoidance and resignation are associated with suffering and patient relapse [ 13 , 14 ].
Birchwood and Cochrane [ 14 ] explored coping strategies used by caregivers of schizophrenia patients. Their results detail eight essential coping categories: coercion, avoidance, ignorance/acceptance, constructive, resignation, reassurance, disorganization and collusion. They also showed that coercion is the first predictor in patient relapses. As concerns specificities of different coping styles, Magliano et al. [ 15 ] conducted a study which explored coping strategies in relation to physical and somatic symptoms of caregivers as well as the association between these two variables. The Family Coping Questionnaire (FCQ) was used [ 10 ]. Their results indicated that emotion-focused coping (coercion, avoidance and resignation) is positively correlated with participant anxiety and depression [ 15 ]. In another study, Knudson and Coyle came up with a procedure which incited certain caregivers to modify their coping strategies [ 11 ]. In their qualitative study, the caregivers of individuals with schizophrenia were invited to describe their coping strategy. At the onset of the illness, the caregivers tended to use a problem-focused coping strategy. However, if the symptoms became persistent, they progressively opted for emotion-focused strategies, which enabled them to attain a position of acceptance and, ultimately, of well-being. Nevertheless, the conceptualization of emotion-focused coping done according to factor analysis in the study of Magliano et al. only included strategies such as resignation, avoidance and coercion [ 10 ]. Acceptance of the illness has seldom been studied and could be a potential functional human response in some instances.
Magliano et al. reported that the high level of burden is associated with a reduction in social interests, a reduction of social support network as well as a resignation and an avoidance of contact with the family member suffering from schizophrenia. It also appears that in the absence of any specific intervention with these caregivers, this result can remain unchanged for as long as a year [ 15 ]. Specific to the subjective burden, it appears that emotional and cognitive reactions are associated with the use of coping strategies that are specifically emotional, such as avoidance and isolation [ 16 , 17 ]. These strategies have been identified as being linked to an increase in caregiver distress [ 18 ]. Increased distress can result in a deterioration of the family atmosphere through elevated EE levels [ 12 ]. These elements lead to a further increase in burden [ 19 ].
Theoretical framework
In terms of theory, in the 1960s, Leventhal and his colleagues developed the Commonsense Model of Self-Regulation of Health and Illness (SRM) [ 20 ]. The results from their initial research indicated that modifying the personal representations of health and the development of an action plan were the two determining factors for the creation of health-promoting actions. Their conclusions led to the development of a series of other studies meant to define the different characteristics of health and illness representations. According to the model, representations are cognitive and emotional constructions of a health problem. The SRM includes eight concepts or dimensions: (1) internal and external stimuli, (2) treatment system, (3) representation of the illness and the treatment, (4) coping procedures, (5) evaluation of the cognitive treatment of the information, (6) emotional representation, (7) coping responses and (8) evaluation of the emotional treatment of the information.
Coping involves procedures which enable an individual to collect information and control the problem, as well as different responses such as distraction or relaxation. Coping can be described as having two primary functions. The first is centred on problem solving and the second on the immediate regulation of the emotion elicited by the problem. According to the SRM, the choice of a coping strategy used by caregivers is associated with the kind of representation of the illness that the caregiver has developed. In this way, the caregiver’s explicative model seems to have an influence on their choice of coping strategy. This choice can then, in turn, lighten or increase caregiver burden [ 17 ]. Effectively, negative representations of the illness can lead to the use of unsuitable coping strategies [ 21 , 22 ].
Goal of the study
The goal of this study was to explore the associations between illness representations and three forms of coping styles—(1) problem-focused coping, (2) emotion-focused coping and (3) social support-focused coping—for caregivers of individuals with schizophrenia.
Design and recruitment
This correlational descriptive study was conducted with 92 caregivers of individuals with schizophrenia. Participants were members of French-speaking social support organizations, were recruited using a convenience sampling strategy and met the following criteria: (1) being 18 years or older, (2) living in Switzerland or France, (3) being able to speak French, (4) acting as carer for a family member or close friend who suffers from schizophrenia and (5) having had at least a 1-h contact with this person in the last month. As the first step of the recruiting strategy, social support organizations were met at the time of meeting with members in order to provide a complete presentation of the study. Then, the members who were present received an envelope containing an informed consent sheet, an information letter and the necessary questionnaires, as well as a pre-addressed and pre-stamped envelope for the return of all completed documents.
A second recruiting strategy was conducted using an electronic survey. The presidents of the social support organizations sent to all members of their respective groups the survey link leading to a dedicated website that included the same documentation as the paper version.
Instruments
Participants filled out three self-administered forms: (a) a socio-demographic questionnaire, (b) the Illness Perception Questionnaire for Schizophrenia : Relatives’ version and (c) the Family Coping Questionnaire. Authorization to translate these questionnaires into French using independent backward translation was granted by the authors.
The socio-demographic questionnaire
According to the literature, the most significant socio-demographic variables which influence illness representations and coping strategies are the following: (1) caregiver gender [ 23 ], (2) caregiver age, (3) caregiver education level [ 13 ], (4) the length of contact with the patient [ 19 , 24 ], (5) professional and social support [ 25 , 26 ] such as involvement in a Profamille program [ 27 ], (6) the nature of their connection with the patient [ 28 ], (7) the length of the illness [ 29 ], (8) whether the caregiver lives with the patient or not [ 30 ], (9) age of the patient, (10) patient gender and (11) the patient’s professional support. With the further goal of comparing our results with other studies already reported in the literature, we collected data on the number of people living in a household and the civil status of the caregivers.
The Illness Perception Questionnaire for Schizophrenia: Relatives’ version
Illness representations were measured using a self-administered questionnaire entitled ‘Illness Perception Questionnaire for Schizophrenia: Relatives’ version (IPQS: Relatives)’ [ 5 ]. It involves 13 sub-scales (150 items): identity, timeline (acute/chronic), timeline (cyclic), negative consequences for the individual, negative consequences for the caregiver, personal control (feeling of powerlessness—patient), personal control (feeling of powerlessness—caregiver), personal blame (patient responsibility), personal blame (caregiver responsibility), therapeutic control, mental health problem coherence, emotional representations and causes. For the current study, the identity and the causes of the illness were not considered. The responses provided by the caregivers regarding their perceptions of the illness were measured using a 5-level Likert scale: 0 = do not agree at all, 1 = do not agree, 2 = more or less agree = 3 = agree and 4 = completely agree. Results show a dimensional reliability situated between α = 0.63 and α = 0.83 [ 5 ].
The Family Coping Questionnaire
Coping styles were measured using a self-administered questionnaire entitled ‘Family Coping Questionnaire (FCQ)’. The version of the FCQ, used in this study, included 27 items measuring seven dimensions (information gathering, positive communication, social involvement, coercion, avoidance, resignation, the patient’s social involvement). A factor analysis enabled the authors to identify three main factors. Looking at these three factors and at the conceptual definition of coping, it is clear that the seven dimensions can be regrouped into three coping modes: (1) problem-focused coping (the patient’s social involvement, positive communication and information gathering are positively correlated with each other and negatively with avoidance, (2) emotion-focused coping (coercion, avoidance and resignation are positively correlated) and (3) social support-focused coping (social involvement is associated with avoidance) [ 10 ]. Our study catalogued these strategies. Caregiver responses to different situations were measured using a 5-level Likert scale: 1 = never, 2 = rarely, 3 = sometimes, 4 = very often and 5 = not applicable. Its validity was demonstrated during the BIOMED 1 study, conducted in five European countries [ 15 ]. Results showed a dimensional reliability between α = 0.68 and α = 0.83 [ 13 ].
Data analysis
Data were treated using the computing program ‘IBM SPSS Statistics® version 20’. Descriptive statistics were used to describe socio-demographic characteristics. To test associations between illness representations and coping styles, bivariate correlational analyses were used. The p value threshold used was set to <0.05.
Ethical considerations
All participants were required to sign an informed consent form for the paper version of the survey or confirm their consent in order to access the electronic version of the survey. The research protocol received full authorization by the Canton of Vaud’s Ethics Committee for human-based research.
Results and discussion
While Table 1 presents the socio-demographic characteristics of participants, Table 2 presents the main characteristics of the individuals with schizophrenia cared for. As showed, the final sample involved 92 middle-aged individuals (mean age = 56 years, standard deviation (SD) = 12.6) including 68% of women. Most participants were married or lived in a household as a married couple (70.3%). The majority of respondents were either mothers or fathers (66.3%) of the individual with schizophrenia. All participants had completed some level of education; vocational school, apprenticeship and university-level education were the most often cited. Most participants reported that they lived with one or several other individuals. Most of them (62%) participated to the Profamille program, a psycho-educational program for family members and friends of individuals with schizophrenia [ 31 ]. Among the 92 participants, 37 (40.2%) reported that they lived with the schizophrenia patient.
As concerns the individual with schizophrenia, results show that the duration of the illness is long (mean = 15.1 years, SD = 9.7). The mean age of the individual with schizophrenia taken care for by the participants of this study was 34 years and 77.2% were male. Individuals with schizophrenia call upon community services in a variety of ways. In 88% of all cases, a psychiatrist was involved in patient follow-up. In 34.8% of all cases, a general medical practitioner was involved in patient follow-up. In our sample, 33.7% of the schizophrenia patients received nursing care. Also, a significant number (33.7%) of the individuals with schizophrenia in our sample were involved in sheltered workshops. Other community services were also used at more than 10% (social worker, 20.7%; psychologist, 13%; day centre, 17.4%).
Results presented in Table 3 show that caregivers have a great conception of the illness as being chronic and as having recurring symptoms per cycle. They have a strong perception that the illness brings about negative consequences for the individual with schizophrenia. While at the same time, they perceive fewer negative consequences for themselves caused by the patient’s illness. They have a feeling of control over the illness and that the patient can also have control. They do not think that the patient is responsible for his or her illness. They do not see themselves as responsible for the appearance of the illness. Treatment is perceived as helpful for better controlling the illness. For these caregivers, the illness has meaning and coherence. They more or less agree that the illness brings about negative emotions like sorrow or anxiety, measured here under the category of emotional representations. Participants in this study mostly used problem-focused and social support-focused coping styles. Emotion-focused coping was used less compared to the two other styles (median = 15, min to max = 7.00–28.00).
Correlations between illness representations and coping styles are presented in Table 4 . It can be seen that all the statistically significant correlations are somewhat low ranging from r = 0.23 to r = 0.41. The statistically significant correlations are the following:
Representations that the illness brings about negative consequences for the caregiver have a moderate positive correlation with problem-focused coping ( r = 0.31, p = 0.006) and emotion-focused coping ( r = 0.35, p = 0.002). There was also a slight negative correlation between these representations and social support-focused coping ( r = −0.29, p = 0.012).
Representations that the illness brings about negative consequences for the patient have a slight positive correlation with emotion-focused coping ( r = 0.23, p = 0.037).
The presence of a feeling of control by the caregiver is moderately positively correlated with problem-focused coping ( r = 0.31, p = 0.006).
Representations that the caregiver might be responsible for the onset of the illness have a moderate positive correlation with emotion-focused coping ( r = 0.34, p = 0.001).
Representations that the patient is responsible for the onset of the illness have a slight positive correlation with emotion-focused coping ( r = 0.25, p = 0.020).
Representations that treatment helps to control the illness have a slight positive correlation with problem-focused coping ( r = 0.23, p = 0.040).
A lack of meaning attributed to the illness has a moderate positive correlation with emotion-focused coping ( r = 0.31, p = 0.005).
Representations that the illness brings about negative emotions have a moderate positive correlation with emotion-focused coping ( r = 0.41, p = 0.000) and a moderate negative correlation with social support-focused coping ( r = −0.39, p = 0.000).
It is timely to note that this study is the first known to use French-validated tools to measure correlations between representations of schizophrenia and coping styles within a caregiver sample. For most variables, our results align with several other studies in this field [ 8 , 9 , 13 , 15 , 32 ]. Nevertheless, the caregivers in this study were less likely to have the perception that the patient was at fault or that they themselves were responsible for the onset of the illness than compared to the results published by Lobban and collaborators [ 5 ]. Our results also show that emotion-focused coping is moderately correlated with (1) negative consequences for the caregivers, (2) the feeling of being at fault, (3) the feeling that the mental health problem is not coherent and (4) the overall score of the emotional representation scale. Problem-focused coping is itself moderately correlated with (1) the perception of negative consequences for caregivers and (2) the feeling of control. Social support-focused coping has a moderate negative correlation with the overall scores of the emotional representation scale. The other correlations are non-significant or less than 0.30 with a slight scaling effect [ 33 ].
The SRM predicts that illness representations influence coping procedures and that emotional representations influence coping responses. These different factors also influence each other. More specifically, when a health threat occurs, it is handled by the processing system , the second concept of SRM. This system consists of two types of threat management: (1) the representations of illness and treatment and (2) the emotional representations. Both types can influence each other. Each type of threat management includes three steps to process information: representation (illness and treatment), coping procedures and evaluation. Regarding the first type of threat management, the first stage of data processing is the development of a representation of the disease and the treatment. This concept determines the goals and coping procedures to achieve them [ 34 ]. Coping procedures are the second stage of information processing. They are a wide range of cognitive and behavioural measures undertaken in response to the cognitive representation, such as problem-focused coping [ 22 , 34 ]. The third step of information processing is the evaluation of the cognitive treatment of information. Regarding the second type of threat management, the first step of information processing is the formation of an emotional representation [ 22 ]. Based on this emotional representation, goals are set and coping responses are determined to achieve them. Coping responses are the second step of information processing. They are a wide range of strategies focused on managing emotions that are applied in response to the emotional representation. The last step of information processing for this second type is the evaluation of the emotional treatment of the information. The results of the evaluation step produce a feedback at the preceding steps .
Our results are quite coherent with this predictive model, especially for emotion-focused coping and the score of emotional representations, consequences for the caregiver and the feeling of being at fault. Consequences for the patient and the feeling of control are associated with problem-focused coping. The fact that the consequences for the caregiver are associated as much with problem-focused coping as with emotion-focused coping underscores the presence of interactions between the emotional and cognitive variables.
A study similar to ours [ 8 ] did not establish a positive correlation between the representations of the illness that bring about negative consequences for the caregiver and problem-focused coping. It is important to note that these authors did not conceptualize coping as we did, and therefore, comparing our results is a limited exercise. However, the abovementioned correlation leads one to think that the Profamille psycho-educational program followed by a significant portion of our participants had an impact on this coping style without actually modifying the representations of the illness as conceived by the participants.
Our sample pool was particular in that it was composed of individuals belonging to family-type assistance programs and that most of the participants had followed some kind of psycho-educational program. On average, our participants had been caring for their patient for 15 years. This could explain the weak level of reliance on emotion-focused coping. Nevertheless, the correlations observed suggest that the SRM model is still valid over time.
In terms of intervention, the psycho-educational programs available for the caregivers tend to focus on knowledge acquisition of the illness and treatment and positive communication skills training to reduce stress within the family. Our data suggest that it would be of interest to take better into account the emotional representations of the illness. In everyday practice, first-line health care professionals have to care for caregivers’ painful emotions such as anger, guilt, sadness and fear. However, the required skills to help caregivers to better manage their painful emotions are difficult to define precisely. The development and evaluation of the best professional strategies to help caregivers to better manage their painful emotions appear to be an interesting line of research for the future. Refining the best professionals’ skills to help caregivers to deal with negative emotions may influence more directly emotional representations and coping skills in caregivers.
Given the scarcity of studies to compare to, further studies could be useful to better identify coping styles that improve emotional regulation without negative consequences for the patient, like acceptance or changing value systems. In this way, a holistic understanding of care, as suggested by several authors [ 35 , 36 ], could be further promoted. Thus, interventions could be focused on the development of alternatives to less effective or even painful strategies like resignation, coercion and avoidance. Furthermore, the tools used to measure emotion-focused coping tend to highlight more negative emotional coping methods like coercion, avoidance or resignation. It is important to develop a measuring instrument that also takes into account the positive strategies focused on emotion.
Limitations of the study
This was the first study using the IPQS: Relatives and the FCQ in French. Participant recruitment took place within the context of social support organizations according to a convenience sampling method. Therefore, our results could be cautiously generalized to other programs having a similar context to ours, in terms of culture and health care systems.
In the empirical literature, as well as in the Commonsense Model of Self-Regulation of Health and Illness, problem-focused and social support-focused coping styles are recognized as efficient for decreasing caregiver loads and increasing a patient’s chances of better coping with schizophrenia. The results of this study also show that illness representations influence the choice of coping styles. The coping style approach is useful to develop further targeted and feasible interventions. Further studies in this field should focus on the evaluation of interventions based on illness representations in order to prevent the use of unsuitable coping styles. Also, it will be necessary to identify this phenomenon in a caregiver population of individuals who are not involved into any mutual support group in order to adapt appropriate care and support procedures.
Abbreviations
Expressed emotion
Family coping questionnaire
Relatives, illness perception questionnaire for schizophrenia: relatives’ version
Standard deviation
Commonsense model of self-regulation of health and illness.
van Wijngaarden B, Schene A, Koeter M, Becker T, Knapp M, Knudsen HC, Tansella M, Thornicroft G, Vazquez-Barquero JL, Lasalvia A, Leese M, EPSILON Study Group: People with schizophrenia in five countries: conceptual similarities and intercultural differences in family caregiving. Schizophrenia Bulletin. 2003, 29 (3): 573-586. 10.1093/oxfordjournals.schbul.a007029.
Article PubMed Google Scholar
Heider D, Angermeyer MC, Winkler I, Schomerus G, Bebbington PE, Brugha T, Azorin JM, Toumi M: A prospective study of quality of life in schizophrenia in three European countries. Schizophrenia Research. 2007, 93 (1–3): 194-202.
Lauber C, Eichenberger A, Luginbuhl P, Keller C, Rossler W: Determinants of burden in caregivers of patients with exacerbating schizophrenia. European Psychiatry: The Journal of the Association of European Psychiatrists. 2003, 18 (6): 285-289. 10.1016/j.eurpsy.2003.06.004.
Article Google Scholar
Barrowclough C, Lobban F, Hatton C, Quinn J: An investigation of models of illness in carers of schizophrenia patients using the illness perception questionnaire. The British Journal of Clinical Psychology/The British Psychological Society. 2001, 40 (Pt 4): 371-385.
Article CAS PubMed Google Scholar
Lobban F, Barrowclough C, Jones S: Assessing cognitive representations of mental health problems. II. The illness perception questionnaire for schizophrenia: relatives’ version. The British Journal of Clinical Psychology/The British Psychological Society. 2005, 44 (Pt 2): 163-179.
Barrowclough C, Tarrier N, Johnston M: Distress, expressed emotion, and attributions in relatives of schizophrenia patients. Schizophrenia Bulletin. 1996, 22 (4): 691-702. 10.1093/schbul/22.4.691.
Lobban F, Barrowclough C, Jones S: Does expressed emotion need to be understood within a more systemic framework? An examination of discrepancies in appraisals between patients diagnosed with schizophrenia and their relatives. Social Psychiatry and Psychiatric Epidemiology. 2006, 41 (1): 50-55. 10.1007/s00127-005-0993-z.
Fortune DG, Smith JV, Garvey K: Perceptions of psychosis, coping, appraisals, and psychological distress in the relatives of patients with schizophrenia: an exploration using self-regulation theory. The British Journal of Clinical Psychology/The British Psychological Society. 2005, 44 (Pt 3): 319-331.
Moller-Leimkuhler AM: Burden of relatives and predictors of burden. Baseline results from the Munich 5-year-follow-up study on relatives of first hospitalized patients with schizophrenia or depression. European Archives of Psychiatry and Clinical Neuroscience. 2005, 255 (4): 223-231. 10.1007/s00406-004-0550-x.
Magliano L, Guarneri M, Marasco C, Tosini P, Morosini PL, Maj M: A new questionnaire assessing coping strategies in relatives of patients with schizophrenia: development and factor analysis. Acta Psychiatrica Scandinavica. 1996, 94 (4): 224-228. 10.1111/j.1600-0447.1996.tb09853.x.
Knudson B, Coyle A: Parents’ experiences of caring for sons and daughters with schizophrenia: a qualitative analysis of coping. European Journal of Psychotherapy & Counselling. 2002, 5 (5): 169-183.
Barrowclough C, Parle M: Appraisal, psychological adjustment and expressed emotion in relatives of patients suffering from schizophrenia. The British Journal of Psychiatry: The Journal of Mental Science. 1997, 171: 26-30. 10.1192/bjp.171.1.26.
Article CAS Google Scholar
Magliano L, Fadden G, Economou M, Xavier M, Held T, Guarneri M, Marasco C, Tosini P, Maj M: Social and clinical factors influencing the choice of coping strategies in relatives of patients with schizophrenia: results of the BIOMED I study. Social Psychiatry and Psychiatric Epidemiology. 1998, 33 (9): 413-419. 10.1007/s001270050074.
Birchwood M, Cochrane R: Families coping with schizophrenia: coping styles, their origins and correlates. Psychological Medicine. 1990, 20 (4): 857-865. 10.1017/S0033291700036552.
Magliano L, Fadden G, Economou M, Held T, Xavier M, Guarneri M, Malangone C, Marasco C, Maj M: Family burden and coping strategies in schizophrenia: 1-year follow-up data from the BIOMED I study. Social Psychiatry and Psychiatric Epidemiology. 2000, 35 (3): 109-115. 10.1007/s001270050192.
Gonzalez-Torres MA, Oraa R, Aristegui M, Fernandez-Rivas A, Guimon J: Stigma and discrimination towards people with schizophrenia and their family members. A qualitative study with focus groups. Social Psychiatry and Psychiatric Epidemiology. 2007, 42 (1): 14-23. 10.1007/s00127-006-0126-3.
Magliano L, Fadden G, Madianos M, de Almeida JM, Held T, Guarneri M, Marasco C, Tosini P, Maj M: Burden on the families of patients with schizophrenia: results of the BIOMED I study. Social Psychiatry and Psychiatric Epidemiology. 1998, 33 (9): 405-412. 10.1007/s001270050073.
Magliano L, Veltro F, Guarneri M, Marasco C: Clinical and socio-demographic correlates of coping strategies in relatives of schizophrenic patients. European Psychiatry: The Journal of the Association of European Psychiatrists. 1995, 10 (3): 155-158. 10.1016/0767-399X(96)80104-0.
Madianos M, Economou M, Dafni O, Koukia E, Palli A, Rogakou E: Family disruption, economic hardship and psychological distress in schizophrenia: can they be measured?. European Psychiatry: The Journal of the Association of European Psychiatrists. 2004, 19 (7): 408-414. 10.1016/j.eurpsy.2004.06.028.
Leventhal H, Leventhal EA, Schafer P: Vigilant coping and health behavior: a life span problem. Aging, Health, and Behavior. Edited by: IMORA. 1991, Baltimore: Johns Hopkins, 109-140.
Google Scholar
Magliano L, Guarneri M, Fiorillo A, Marasco C, Malangone C, Maj M: A multicenter Italian study of patients’ relatives’ beliefs about schizophrenia. Psychiatric Services. 2001, 52 (11): 1528-1530. 10.1176/appi.ps.52.11.1528.
Leventhal H, Leventhal EA, Cameron LD: Representations, procedures, and affect in illness self-regulation: a perceptual-cognitive approach. Handbook of Health Psychology. Edited by: Baum AS, Revenson TA, Singer JE. 2001, New York: Erbaum, 19-48.
Mitsonis C, Voussoura E, Dimopoulos N, Psarra V, Kararizou E, Latzouraki E, Zervas I, Katsanou MN: Factors associated with caregiver psychological distress in chronic schizophrenia. Social Psychiatry and Psychiatric Epidemiology. 2010, 47 (2): 331-337.
Koukia E, Madianos MG: Is psychosocial rehabilitation of schizophrenic patients preventing family burden? A comparative study. Journal of Psychiatric and Mental Health Nursing. 2005, 12 (4): 415-422. 10.1111/j.1365-2850.2005.00852.x.
Magliano L, Fiorillo A, Malangone C, Marasco C, Guarneri M, Maj M, National Mental Health Project Working Group: The effect of social network on burden and pessimism in relatives of patients with schizophrenia. The American Journal of Orthopsychiatry. 2003, 73 (3): 302-309.
Magliano L, Fiorillo A, Malangone C, De Rosa C, Maj M, Family Intervention Working Group: Implementing psychoeducational interventions in Italy for patients with schizophrenia and their families. Psychiatric Services. 2006, 57 (2): 266-269. 10.1176/appi.ps.57.2.266.
Hodé Y, Krychowski R, Beck N, Vonthron R, Rouyèr N, Huentz M, Risacher P, Fattah S, Pasche M, Pasche P: Effet d’un programme psychoéducatif sur l’humeur des familles des malades soufrant de schizophrénie. Journal de thérapie comportementale et cognitive. 2008, 18: 104-107. 10.1016/j.jtcc.2008.06.003.
Stalberg G, Ekerwald H, Hultman CM: At issue: siblings of patients with schizophrenia: sibling bond, coping patterns, and fear of possible schizophrenia heredity. Schizophrenia Bulletin. 2004, 30 (2): 445-458. 10.1093/oxfordjournals.schbul.a007091.
Moller-Leimkuhler AM: Multivariate prediction of relatives’ stress outcome one year after first hospitalization of schizophrenic and depressed patients. European Archives of Psychiatry and Clinical Neuroscience. 2006, 256 (2): 122-130. 10.1007/s00406-005-0619-1.
Schene AH, van Wijngaarden B: A survey of an organization for families of patients with serious mental illness in The Netherlands. Psychiatric Services. 1995, 46 (8): 807-813.
Cromier H, Guimond G, Joncas J, Leblanc G, Morin R, Vaillancourt S: Profamille, programme d’intervention de groupe auprès des familles de personnes atteintes de schizophrénie. 1991, Québec: Unité de psychiatrie sociale et préventive du CHUL et Centre de recherche de l’Université Laval Robert Giffard
Kartalova-O’Doherty Y, Doherty DT: Coping strategies and styles of family carers of persons with enduring mental illness: a mixed methods analysis. Scandinavian Journal of Caring Sciences. 2008, 22 (1): 19-28. 10.1111/j.1471-6712.2007.00583.x.
Article PubMed Central PubMed Google Scholar
Field AP: Discovering Statistics Using SPSS: (and Sex and Drugs and Rock 'n' Roll). 2009, Thousand Oaks: Sage, 3
Leventhal H, Leventhal EA, Contrada RJ: Self regulation, health, and behavior: a perceptual-cognitive approach. Psychology and Health. 1998, 13: 717-733. 10.1080/08870449808407425.
Dallaire C, Toupin I: Les théories à spectre modéré, les théories propres aux situations et les interventions infirmières. Le savoir infirmier: au coeur de la discipline et de la profession infirmière. Edited by: Dallaire IC. 2008, Boucherville: Gaëtan Morin, 239-264.
Pépin J, Kérouac S, Ducharme F: La pensée infirmière. 2010, Montréal: Chenelière Éducation, 3
Download references
Acknowledgements
This work has been supported by a donation from Dr. Alexander Engelhorn.
Author information
Authors and affiliations.
Community Psychiatry Service, Department of Psychiatry, University Hospital Centre of Vaud, Site de Cery, Prilly, 1008, Switzerland
Shyhrete Rexhaj, Charles Bonsack & Jérôme Favrod
School of Nursing Sciences, La Source, University of Applied Sciences of Western Switzerland, Av. Vinet 30, Lausanne, 1004, Switzerland
Shyhrete Rexhaj, Nataly Viens Python & Jérôme Favrod
Institut Universitaire de Formation et de Recherche en Soins, University of Lausanne, Biopôle 2, Rue de la Corniche 10, Lausanne, 1010, Switzerland
Diane Morin
You can also search for this author in PubMed Google Scholar
Corresponding author
Correspondence to Shyhrete Rexhaj .
Additional information
Competing interests.
The authors declare that they have no competing interests.
Authors’ contributions
SR, DM and JF contributed to the conception and design of the study. SR contributed to the acquisition of data. SR and JF performed the statistical analysis and drafted the first manuscript. NVP and CB critically reviewed and revised the manuscript. All authors read and approved the final manuscript.
Authors’ original submitted files for images
Below are the links to the authors’ original submitted files for images.
Authors’ original file for figure 1
Authors’ original file for figure 2, authors’ original file for figure 3, authors’ original file for figure 4, rights and permissions.
This article is published under license to BioMed Central Ltd. This is an Open Access article distributed under the terms of the Creative Commons Attribution License ( http://creativecommons.org/licenses/by/2.0 ), which permits unrestricted use, distribution, and reproduction in any medium, provided the original work is properly cited.
Reprints and permissions
About this article
Cite this article.
Rexhaj, S., Python, N.V., Morin, D. et al. Correlational study: illness representations and coping styles in caregivers for individuals with schizophrenia. Ann Gen Psychiatry 12 , 27 (2013). https://doi.org/10.1186/1744-859X-12-27
Download citation
Received : 12 June 2013
Accepted : 20 August 2013
Published : 28 August 2013
DOI : https://doi.org/10.1186/1744-859X-12-27
Share this article
Anyone you share the following link with will be able to read this content:
Sorry, a shareable link is not currently available for this article.
Provided by the Springer Nature SharedIt content-sharing initiative
- Representations of schizophrenia
- Nursing care
Annals of General Psychiatry
ISSN: 1744-859X
- Submission enquiries: [email protected]
Social Sciences
© 2024 Inquiries Journal/Student Pulse LLC . All rights reserved. ISSN: 2153-5760.
Disclaimer: content on this website is for informational purposes only. It is not intended to provide medical or other professional advice. Moreover, the views expressed here do not necessarily represent the views of Inquiries Journal or Student Pulse, its owners, staff, contributors, or affiliates.
Home | Current Issue | Blog | Archives | About The Journal | Submissions Terms of Use :: Privacy Policy :: Contact
Need an Account?
Forgot password? Reset your password »
Thank you for visiting nature.com. You are using a browser version with limited support for CSS. To obtain the best experience, we recommend you use a more up to date browser (or turn off compatibility mode in Internet Explorer). In the meantime, to ensure continued support, we are displaying the site without styles and JavaScript.
- View all journals
- Explore content
- About the journal
- Publish with us
- Sign up for alerts
- Perspective
- Published: 26 November 2018
Quasi-experimental causality in neuroscience and behavioural research
- Ioana E. Marinescu 1 ,
- Patrick N. Lawlor 2 &
- Konrad P. Kording ORCID: orcid.org/0000-0001-8408-4499 3 , 4
Nature Human Behaviour volume 2 , pages 891–898 ( 2018 ) Cite this article
8222 Accesses
58 Citations
241 Altmetric
Metrics details
- Neuroscience
In many scientific domains, causality is the key question. For example, in neuroscience, we might ask whether a medication affects perception, cognition or action. Randomized controlled trials are the gold standard to establish causality, but they are not always practical. The field of empirical economics has developed rigorous methods to establish causality even when randomized controlled trials are not available. Here we review these quasi-experimental methods and highlight how neuroscience and behavioural researchers can use them to do research that can credibly demonstrate causal effects.
This is a preview of subscription content, access via your institution
Access options
Access Nature and 54 other Nature Portfolio journals
Get Nature+, our best-value online-access subscription
24,99 € / 30 days
cancel any time
Subscribe to this journal
Receive 12 digital issues and online access to articles
111,21 € per year
only 9,27 € per issue
Rent or buy this article
Prices vary by article type
Prices may be subject to local taxes which are calculated during checkout
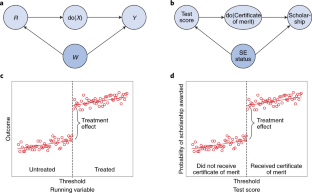
Similar content being viewed by others
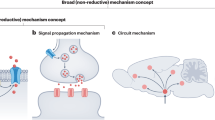
Causation in neuroscience: keeping mechanism meaningful
Lauren N. Ross & Dani S. Bassett
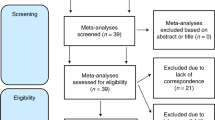
Comparing meta-analyses and preregistered multiple-laboratory replication projects
Amanda Kvarven, Eirik Strømland & Magnus Johannesson
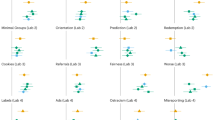
High replicability of newly discovered social-behavioural findings is achievable
John Protzko, Jon Krosnick, … Jonathan W. Schooler
Pearl, J. Causality (Cambridge Univ. Press, New York, 2009).
The Notorious B.I.G. Mo’ Money Mo’ Problems (Bad Boy Records, 1997).
Grodstein, F. et al. A prospective, observational study of postmenopausal hormone therapy and primary prevention of cardiovascular disease. Ann. Intern. Med. 133 , 933–941 (2000).
Article CAS Google Scholar
Manson, J. E. et al. Estrogen plus progestin and the risk of coronary heart disease. N. Engl. J. Med. 349 , 523–534 (2003).
Humphrey, L. L., Chan, B. K. & Sox, H. C. Postmenopausal hormone replacement therapy and the primary prevention of cardiovascular disease. Ann. Intern. Med. 137 , 273–284 (2002).
Greenland, S. Randomization, statistics, and causal inference. Epidemiology 1 , 421–429 (1990).
Ismail-Beigi, F. et al. Effect of intensive treatment of hyperglycaemia on microvascular outcomes in type 2 diabetes: an analysis of the ACCORD randomised trial. Lancet 376 , 419–430 (2010).
Article Google Scholar
Officers, T. A. Major outcomes in high-risk hypertensive patients randomized to or calcium channel blocker vs diuretic. J. Am. Med. Assoc. 288 , 2981–2997 (2002).
Group, S. R. A randomized trial of intensive versus standard blood-pressure control. N. Engl. J. Med. 373 , 2103–2116 (2015).
Granger, C. W. Investigating causal relations by econometric models and cross-spectral methods. Econometrica 37 , 424–438 (1969).
Angrist, J. D. & Pischke, J.-S. Mostly Harmless Econometrics: An Empiricist’s Companion (Princeton Univ. Press, Princeton, 2008).
Leamer, E. E. Let’s take the con out of econometrics. Am. Econ. Rev. 73 , 31–43 (1983).
Google Scholar
Thistlethwaite, D. L. & Campbell, D. T. Regression-discontinuity analysis: an alternative to the ex-post facto experiment. J. Educ. Psychol. 51 , 309–317 (1960).
Imbens, G. W. & Lemieux, T. Regression discontinuity designs: a guide to practice. J. Econom. 142 , 615–635 (2008).
Angrist, J., Azoulay, P., Ellison, G., Hill, R. & Lu, S. F. Economic research evolves: fields and styles. Am. Econ. Rev. 107 , 293–297 (2017).
Angrist, J., Azoulay, P., Ellison, G., Hill, R. & Lu, S. F. Inside Job or Deep Impact? Using Extramural Citations to Assess Economic Scholarship (National Bureau of Economic Research, 2017).
McCrary, J. Manipulation of the running variable in the regression discontinuity design: a density test. J. Econom. 142 , 698–714 (2008).
Trochim, W. M. Research Design for Program Evaluation: The Regression-Discontinuity Approach (Sage Publications, Beverly Hills, 1984).
Jacob, R., Zhu, P., Somers, M. A., & Bloom, H. A practical guide to regression discontinuity. MDRC https://www.mdrc.org/publication/practical-guide-regression-discontinuity (2012).
Lansdell, B. & Kording, K. Spiking allows neurons to estimate their causal effect. Preprint at bioRxiv https://doi.org/10.1101/253351 (2018).
Moscoe, E., Bor, J. & Bärnighausen, T. Regression discontinuity designs are underutilized in medicine, epidemiology, and public health: a review of current and best practice. J. Clin. Epidemiol. 68 , 122–133 (2015).
Pischke, J. S. The impact of length of the school year on student performance and earnings: evidence from the German short school years. Econ. J. 117 , 1216–1242 (2007).
Athey, S. & Imbens, G. W. Identification and inference in nonlinear difference-in-differences models. Econometrica 74 , 431–497 (2006).
Angrist, J. D. et al. Identification of causal effects using instrumental variables. J. Am. Stat. Assoc. 91 , 444–455 (2016).
Evans, W. N. & Ringel, J. S. Can higher cigarette taxes improve birth outcomes? J. Public. Econ. 72 , 135–154 (1999).
Stock, J. H. & Yogo, M. Testing for Weak Instruments in Linear IV Regression . (National Bureau of Economic Research, Cambridge, 2002).
Book Google Scholar
Li, X., Yamawaki, N., Barrett, J. M., Körding, K. P. & Shepherd, G. Scaling of optogenetically evoked signaling in a higher-order corticocortical pathway in the anesthetized mouse. Front. Syst. Neurosci . 12 , 16 (2018).
Koller, D. & Friedman, N. Probabilistic Graphical Models: Principles and Techniques (MIT Press, Cambridge, 2009).
Ullman, J. B., & Bentler, P. M. in Handbook of Psychology 2nd edn (eds Schinka, J. A. & Velicer, W. F.) Ch. 23 (John Wiley & Sons, Hoboken, 2012).
Dehejia, R. H. & Wahba, S. Propensity score-matching methods for nonexperimental causal studies. Rev. Econ. Stat. 84 , 151–161 (2002).
Rosenbaum, P. R. & Rubin, D. B. The central role of the propensity score in observational studies for causal effects. Biometrika 70 , 41–55 (1983).
Imbens, G. W. & Rubin, D. B. Causal Inference in Statistics, Social, and Biomedical Sciences (Cambridge Univ. Press, New York, 2015).
Hoyer, P. O., Janzing, D., Mooij, J. M., Peters, J. & Schölkopf, B. Nonlinear causal discovery with additive noise models. Adv. Neural Inf. Process. Syst. 21 , 689–696 (2009).
Abadie, A., Diamond, A. & Hainmueller, J. Synthetic control methods for comparative case studies: estimating the effect of California’s tobacco control program. J. Am. Stat. Assoc. 105 , 493–505 (2010).
Ramsey, J. D. et al. Six problems for causal inference from fMRI. NeuroImage 49 , 1545–1558 (2010).
Jonas, E. & Kording, K. P. Could a neuroscientist understand a microprocessor? PLoS Comput. Biol. 13 , e1005268 (2017).
Valdes-Sosa, P. A., Roebroeck, A., Daunizeau, J. & Friston, K. Effective connectivity: influence, causality and biophysical modeling. NeuroImage 58 , 339–361 (2011).
Smith, S. M., Miller, K. L., Salimi-Khorshidi, G., Webster, M. & Beckmann, C. F. et al. Network modelling methods for FMRI. NeuroImage 54 , 875–891 (2011).
Kwak, H., Lee, C., Park, H. & Moon, S. What is Twitter, a social network or a news media? In Proc. 19th International Conference on World Wide Web 591–600 (ACM, 2010).
Bem, J. Using match confidence to adjust a performance threshold. Google Patent 7346615 (2008).
Slemrod, J. Buenas notches: lines and notches in tax system design. EJ Tax Res. 11 , 259–283 (2013).
Angrist, J. D. & Pischke, J.-S. The credibility revolution in empirical economics: how better research design is taking the con out of econometrics. J. Econ. Perspect. 24 , 3–30 (2010).
Krakauer, J. W., Ghazanfar, A. A., Gomez-Marin, A., MacIver, M. A. & Poeppel, D. Neuroscience needs behavior: correcting a reductionist bias. Neuron (in the press).
Pillow, J. W. et al. Spatio-temporal correlations and visual signalling in a complete neuronal population. Nature 454 , 995–999 (2008).
Stevenson, I. H. & Körding, K. P. On the similarity of functional connectivity between neurons estimated across timescales. PLoS ONE 5 , e9206 (2010).
Sakkalis, V. Review of advanced techniques for the estimation of brain connectivity measured with EEG/MEG. Comput. Biol. Med. 41 , 1110–1117 (2011).
Bressler, S. L. & Seth, A. K. Wiener–Granger causality: a well established methodology. Neuroimage 58 , 323–329 (2011).
Ding, M., Chen, Y. & Bressler, S. L. in Handbook of Time Series Analysis: Recent Theoretical Developments and Applications (eds Schelter, B., Winterhalder, M. & Timmer, J.) 335–368 (Wiley-VCH, Weinheim, 2006).
Hiemstra, C. & Jones, J. D. Testing for linear and nonlinear Granger causality in the stock price — volume relation. J. Finance 49 , 1639–1664 (1994).
Chen, Z. Advanced State Space Methods for Neural and Clinical Data (Cambridge Univ. Press, Cambridge, 2015).
Shumway, R. H. & Stoffer, D. S. in Time Series Analysis and its Applications (Shumway, R. H. & Stoffer, D. S.) 319–404 (Springer, New York, 2011).
Friston, K. J., Harrison, L. & Penny, W. Dynamic causal modelling. NeuroImage 19 , 1273–1302 (2003).
Semedo, J., Zandvakili, A., Kohn, A., Machens, C. K. & Byron, M. Y. Extracting latent structure from multiple interacting neural populations. Adv. Neural Inf. Process. Syst. 27 , 2942–2950 (2014).
Daunizeau, J., David, O. & Stephan, K. E. Dynamic causal modelling: a critical review of the biophysical and statistical foundations. NeuroImage 58 , 312–322 (2009).
Latimer, K. W., Yates, J. L., Meister, M. L. R., Huk, A. C. & Pillow, J. W. Single-trial spike trains in parietal cortex reveal discrete steps during decision-making. Science 349 , 184–187 (2015).
Nevo, A. & Whinston, M. D. Taking the dogma out of econometrics: structural modeling and credible inference. J. Econ. Perspect. 24 , 69–82 (2010).
Song, L., Kolar, M. & Xing, E. P. Time-varying dynamic bayesian networks. Adv. Neural Inf. Process. Syst. 22 , 1732–1740 (2009).
Goodman, N. D., Ullman, T. D. & Tenenbaum, J. B. Learning a theory of causality. Psychol. Rev. 118 , 110–119 (2011).
Gopnik, A. et al. A Theory of causal learning in children: causal maps and Bayes nets. Psychol. Rev. 111 , 3–32 (2004).
Gopnik, A. & Tenenbaum, J. B. Bayesian networks, Bayesian learning and cognitive development. Dev. Sci. 10 , 281–287 (2007).
Körding, K. P. et al. Causal inference in multisensory perception. PLoS ONE 2 , e943 (2007).
Download references
Author information
Authors and affiliations.
Department of Social Policy and Practice, University of Pennsylvania, Philadelphia, PA, USA
Ioana E. Marinescu
Division of Neurology, Children’s Hospital of Philadelphia, Philadelphia, PA, USA
Patrick N. Lawlor
Departments of Neuroscience and Bioengineering, Leonard Davis Institute, Warren Center for Network Science, Wharton Neuroscience Initiative, University of Pennsylvania, Philadelphia, PA, USA
Konrad P. Kording
Canadian Institute For Advanced Research, Toronto, Ontario, Canada
You can also search for this author in PubMed Google Scholar
Corresponding author
Correspondence to Ioana E. Marinescu .
Ethics declarations
Competing interests.
The authors declare no competing interests.
Additional information
Publisher’s note: Springer Nature remains neutral with regard to jurisdictional claims in published maps and institutional affiliations.
Rights and permissions
Reprints and permissions
About this article
Cite this article.
Marinescu, I.E., Lawlor, P.N. & Kording, K.P. Quasi-experimental causality in neuroscience and behavioural research. Nat Hum Behav 2 , 891–898 (2018). https://doi.org/10.1038/s41562-018-0466-5
Download citation
Received : 18 March 2018
Accepted : 02 October 2018
Published : 26 November 2018
Issue Date : December 2018
DOI : https://doi.org/10.1038/s41562-018-0466-5
Share this article
Anyone you share the following link with will be able to read this content:
Sorry, a shareable link is not currently available for this article.
Provided by the Springer Nature SharedIt content-sharing initiative
This article is cited by
- Lauren N. Ross
- Dani S. Bassett
Nature Reviews Neuroscience (2024)
Workers’ whole day workload and next day cognitive performance
- Raymond Hernandez
- Haomiao Jin
- Stefan Schneider
Current Psychology (2024)
A transdiagnostic network for psychiatric illness derived from atrophy and lesions
- Joseph J. Taylor
- Christopher Lin
- Michael D. Fox
Nature Human Behaviour (2023)
Causal theory error in college students’ understanding of science studies
- Colleen M. Seifert
- Michael Harrington
Cognitive Research: Principles and Implications (2022)
Quantifying causal effects from observed data using quasi-intervention
- Jinghua Yang
- Lifang Zhou
BMC Medical Informatics and Decision Making (2022)
Quick links
- Explore articles by subject
- Guide to authors
- Editorial policies
Sign up for the Nature Briefing newsletter — what matters in science, free to your inbox daily.

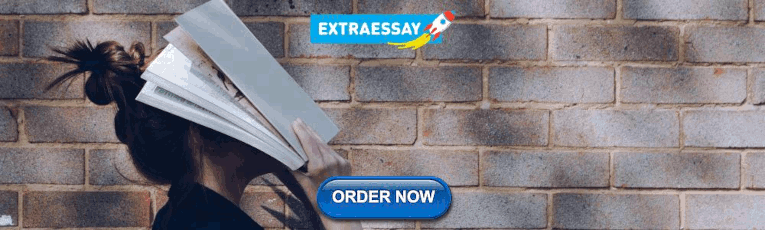
IMAGES
VIDEO
COMMENTS
A correlational study is a type of research design that looks at the relationships between two or more variables. Correlational studies are non-experimental, which means that the experimenter does not manipulate or control any of the variables. A correlation refers to a relationship between two variables. Correlations can be strong or weak and ...
The goal of experimental research design is to provide more definitive conclusions about the causal relationships among the variables in the research hypothesis than is available from correlational designs. In an experimental research design, the variables of interest are called the independent variable (or variables) and the dependent variable.
To explore the association between depression and anxiety symptoms among college students and the relationship between the two and physical activity. A cross-sectional study design was used to ...
Researchers who instead decide to rely on observational data often attempt to deal with its weaknesses by cautiously avoiding causal language: They refer to "associations," "relationships," or tentative "links" between variables instead of clear cause-effect relationships, and they usually add a general disclaimer ("Of course, as the data were only observational, future ...
Correlational research is a type of nonexperimental research in which the researcher measures two variables and assesses the statistical relationship (i.e., the correlation) between them with little or no effort to control extraneous variables. There are essentially two reasons that researchers interested in statistical relationships between ...
Correlational studies examining the influence of nature exposure on cognitive performance have found a positive association between green space around schools and cognitive development in children (Dadvand et al., 2015), as well as an association between green views at home and self-control behaviors in young girls (Taylor, Kuo, & Sullivan, 2002). ...
Descriptive, correlational, and experimental research designs are used to collect and analyze data. Descriptive designs include case studies, surveys, and naturalistic observation. The goal of these designs is to get a picture of the current thoughts, feelings, or behaviors in a given group of people.
1. Teacher provides sample psychological studies from recent research (see sample below). 2. Students are given instructions to decide whether the research is correlational or experimental. a. If correlational, they should predict a sample coefficient (r-value) or they should draw a sample scatter plot. b.
Between 2010-2012 and 2017-2019 effect sizes declined in both fields: 0.36 to 0.30 in developmental, and 0.29 to 0.23 in social psychology (Sample 2). The correlation effect sizes in Sample 2 were negatively correlated with associated sample size in the earliest publication years we studied.
First, the intervention emphasized generating alternative theories as a way to counter assumptions of causation. Second, the intervention included a diagram-drawing component, prompting creativity ...
Understanding the association between theoretical constructs is at the heart of quantitative research. Researchers use correlation to understand how two or more variables are associated. Note that correlation does not infer causality especially when it is applied to cross-sectional data (Alamer and Lee, 2021). Beyond this, in first-generation ...
Correlational research differs from experimental research in that it does not involve manipulating variables. Instead, it focuses on analyzing the relationship between two or more variables. In other words, correlational research seeks to determine whether there is a relationship between two variables and, if so, the nature of that relationship.
Revised on June 22, 2023. A correlational research design investigates relationships between variables without the researcher controlling or manipulating any of them. A correlation reflects the strength and/or direction of the relationship between two (or more) variables. The direction of a correlation can be either positive or negative.
Proper Interpretation of Correlation. Correlational analyses have been reported as one of the most common analytic techniques in research at the beginning of the 21 st century, particularly in health and epidemiological research. 15 Thus effective and proper interpretation is critical to understanding the literature.
Spending time in nature can act as a balm for our busy brains. Both correlational and experimental research have shown that interacting with nature has cognitive benefits—a topic University of Chicago psychologist Marc Berman, PhD, and his student Kathryn Schertz explored in a 2019 review.
Correlational surveys. The basic approach in correlational research is to obtain a measure of viewing violent programs and to relate this to a measure or battery of measures of aggressiveness. The ultimate goal is to demonstrate a causal relation, with the first step being to find out whether the possibility of a relation exists in the first ...
1: Journal Article Reporting Standards for Qualitative Research in Psychology. This American Psychologist open-access article lays out—for the first time—journal article reporting standards for qualitative research in psychology (Levitt, H.M., et al., Vol. 73, No. 1). The voluntary guidelines are designed to help authors communicate their work clearly, accurately and transparently.
This is the case of Paul Ekman's model 4. Along a series of studies, Ekman found high agreement across members of diverse Western and Eastern literate cultures on selecting emotional labels that ...
Background Caring for individuals with schizophrenia can create distress for caregivers which can, in turn, have a harmful impact on patient progress. There could be a better understanding of the connections between caregivers' representations of schizophrenia and coping styles. This study aims at exploring those connections. Methods This correlational descriptive study was conducted with 92 ...
Trust and self-esteem are vital to social functioning and social functioning has an impact on the mental health of all individuals. Research found by Williams, & Galliher (2006) display the relationship that social engagement and social functioning, as a whole, impact the individual's psychological well-being.
Abstract. In many scientific domains, causality is the key question. For example, in neuroscience, we might ask whether a medication affects perception, cognition or action. Randomized controlled ...
Although correlational studies of literacy were most common in the 1960s through the mid-1980s, this approach to inquiry continues. For instance, researchers of two such studies ( Allen et al., 2016; Allen et al., 2019) reported mixed results when studying whether or not writing flexibility in argumentative essays predicts reading comprehension.