Sciencing_Icons_Science SCIENCE
Sciencing_icons_biology biology, sciencing_icons_cells cells, sciencing_icons_molecular molecular, sciencing_icons_microorganisms microorganisms, sciencing_icons_genetics genetics, sciencing_icons_human body human body, sciencing_icons_ecology ecology, sciencing_icons_chemistry chemistry, sciencing_icons_atomic & molecular structure atomic & molecular structure, sciencing_icons_bonds bonds, sciencing_icons_reactions reactions, sciencing_icons_stoichiometry stoichiometry, sciencing_icons_solutions solutions, sciencing_icons_acids & bases acids & bases, sciencing_icons_thermodynamics thermodynamics, sciencing_icons_organic chemistry organic chemistry, sciencing_icons_physics physics, sciencing_icons_fundamentals-physics fundamentals, sciencing_icons_electronics electronics, sciencing_icons_waves waves, sciencing_icons_energy energy, sciencing_icons_fluid fluid, sciencing_icons_astronomy astronomy, sciencing_icons_geology geology, sciencing_icons_fundamentals-geology fundamentals, sciencing_icons_minerals & rocks minerals & rocks, sciencing_icons_earth scructure earth structure, sciencing_icons_fossils fossils, sciencing_icons_natural disasters natural disasters, sciencing_icons_nature nature, sciencing_icons_ecosystems ecosystems, sciencing_icons_environment environment, sciencing_icons_insects insects, sciencing_icons_plants & mushrooms plants & mushrooms, sciencing_icons_animals animals, sciencing_icons_math math, sciencing_icons_arithmetic arithmetic, sciencing_icons_addition & subtraction addition & subtraction, sciencing_icons_multiplication & division multiplication & division, sciencing_icons_decimals decimals, sciencing_icons_fractions fractions, sciencing_icons_conversions conversions, sciencing_icons_algebra algebra, sciencing_icons_working with units working with units, sciencing_icons_equations & expressions equations & expressions, sciencing_icons_ratios & proportions ratios & proportions, sciencing_icons_inequalities inequalities, sciencing_icons_exponents & logarithms exponents & logarithms, sciencing_icons_factorization factorization, sciencing_icons_functions functions, sciencing_icons_linear equations linear equations, sciencing_icons_graphs graphs, sciencing_icons_quadratics quadratics, sciencing_icons_polynomials polynomials, sciencing_icons_geometry geometry, sciencing_icons_fundamentals-geometry fundamentals, sciencing_icons_cartesian cartesian, sciencing_icons_circles circles, sciencing_icons_solids solids, sciencing_icons_trigonometry trigonometry, sciencing_icons_probability-statistics probability & statistics, sciencing_icons_mean-median-mode mean/median/mode, sciencing_icons_independent-dependent variables independent/dependent variables, sciencing_icons_deviation deviation, sciencing_icons_correlation correlation, sciencing_icons_sampling sampling, sciencing_icons_distributions distributions, sciencing_icons_probability probability, sciencing_icons_calculus calculus, sciencing_icons_differentiation-integration differentiation/integration, sciencing_icons_application application, sciencing_icons_projects projects, sciencing_icons_news news.
- Share Tweet Email Print
- Home ⋅
- Science Fair Project Ideas for Kids, Middle & High School Students ⋅
- Probability & Statistics
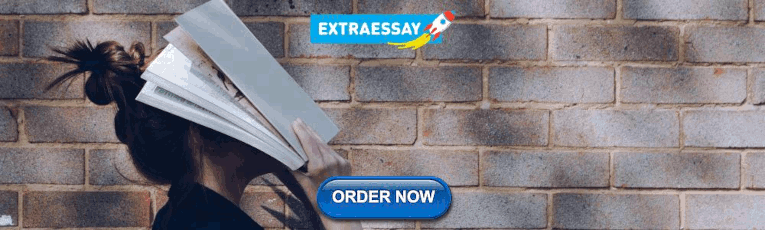
Distinguishing Between Descriptive & Causal Studies
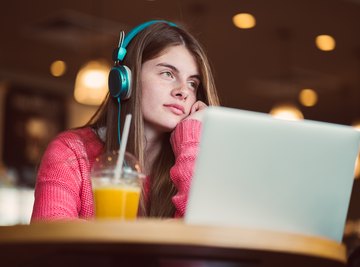
Internal & External Control in Experiments
Descriptive and causal studies answer fundamentally different kinds of questions. Descriptive studies are designed primarily to describe what is going on or what exists. Causal studies, which are also known as “experimental studies,” are designed to determine whether one or more variables causes or affects the value of other variables.
Directionality of Hypothesis
A causal study’s hypothesis is directional -- it does not simply claim that two or more variables are related, but predicts that one variable or set of variables, called “independent variables,” will affect another variable or set of variables, known as “dependent variables,” in a certain way. An example of a directional hypothesis would be, “I predict that increased levels of exercise will lead to weight loss.” A non-directional hypothesis, which would be suitable for a descriptive study, would simply predict that there exists some relationship between the variables “amount of exercise” and “weight loss.”
Variable Manipulation and Controls
In a causal study, researchers manipulate the set of independent variables to determine their effect, if any, on dependent variables. Researchers in causal studies also typically make use of a “control” -- a case in which the independent variables have not been manipulated, to allow researchers to compare the effects of manipulating the independent variables to the effects of leaving them the same. A descriptive study does not typically involve variable manipulation or a control.
Data Collection Methods: Descriptive Studies
Descriptive studies make use of two primary sorts of data collection: cross-sectional studies and longitudinal studies. The cross-sectional study attempts to give a snapshot of data at a certain moment in time -- variables in a cross-sectional study are measured only once. The longitudinal study, on the other hand, involves a fixed, relatively stable sample measured repeatedly over time. In both cases, methods used might include mail, online or in-person surveys or interviews.
Data Collection Methods: Causal Studies
Case studies likewise make use of two primary sorts of data collection: laboratory experiments and field experiments. Laboratory experiments are conducted in artificial environments which allow researchers to carefully control exactly which variables are manipulated while keeping other factors constant. Field experiments are conducted “in the field,” in a natural or realistic environment. Field experiments allow researchers to test how their hypotheses apply to the “real world.” However, it is often impossible for researchers to control for all possible variables in field experiments, making it harder for researchers to say with confidence exactly what produced a given effect.
Related Articles
How to determine the sample size in a quantitative..., research methods in science, difference between proposition & hypothesis, steps & procedures for conducting scientific research, what are parametric and nonparametric tests, advantages and disadvantages of quadrat use, how to write a hypothesis for correlation, five characteristics of the scientific method, definitions of control, constant, independent and dependent..., what is the difference between a control & a controlled..., the effects of a small sample size limitation, similarities of univariate & multivariate statistical..., how to use the pearson correlation coefficient, what are the 8 steps in scientific research, differences between conceptual independent variables..., how to calculate significance, how to determine sample size.
- Monroe College: Exploratory, Descriptive, and Causal Research Designs
About the Author
Based in Chicago, Adam Jefferys has been writing since 2007. He teaches college writing and literature, and has tutored students in ESL. He holds a Masters of Fine Arts in creative writing, and is currently completing a PhD in English Studies.
Find Your Next Great Science Fair Project! GO
Root out friction in every digital experience, super-charge conversion rates, and optimize digital self-service
Uncover insights from any interaction, deliver AI-powered agent coaching, and reduce cost to serve
Increase revenue and loyalty with real-time insights and recommendations delivered to teams on the ground
Know how your people feel and empower managers to improve employee engagement, productivity, and retention
Take action in the moments that matter most along the employee journey and drive bottom line growth
Whatever they’re are saying, wherever they’re saying it, know exactly what’s going on with your people
Get faster, richer insights with qual and quant tools that make powerful market research available to everyone
Run concept tests, pricing studies, prototyping + more with fast, powerful studies designed by UX research experts
Track your brand performance 24/7 and act quickly to respond to opportunities and challenges in your market
Explore the platform powering Experience Management
- Free Account
- For Digital
- For Customer Care
- For Human Resources
- For Researchers
- Financial Services
- All Industries
Popular Use Cases
- Customer Experience
- Employee Experience
- Employee Exit Interviews
- Net Promoter Score
- Voice of Customer
- Customer Success Hub
- Product Documentation
- Training & Certification
- XM Institute
- Popular Resources
- Customer Stories
Market Research
- Artificial Intelligence
- Partnerships
- Marketplace
The annual gathering of the experience leaders at the world’s iconic brands building breakthrough business results, live in Salt Lake City.
- English/AU & NZ
- Español/Europa
- Español/América Latina
- Português Brasileiro
- REQUEST DEMO
- Experience Management
- Causal Research
Try Qualtrics for free
Causal research: definition, examples and how to use it.
16 min read Causal research enables market researchers to predict hypothetical occurrences & outcomes while improving existing strategies. Discover how this research can decrease employee retention & increase customer success for your business.
What is causal research?
Causal research, also known as explanatory research or causal-comparative research, identifies the extent and nature of cause-and-effect relationships between two or more variables.
It’s often used by companies to determine the impact of changes in products, features, or services process on critical company metrics. Some examples:
- How does rebranding of a product influence intent to purchase?
- How would expansion to a new market segment affect projected sales?
- What would be the impact of a price increase or decrease on customer loyalty?
To maintain the accuracy of causal research, ‘confounding variables’ or influences — e.g. those that could distort the results — are controlled. This is done either by keeping them constant in the creation of data, or by using statistical methods. These variables are identified before the start of the research experiment.
As well as the above, research teams will outline several other variables and principles in causal research:
- Independent variables
The variables that may cause direct changes in another variable. For example, the effect of truancy on a student’s grade point average. The independent variable is therefore class attendance.
- Control variables
These are the components that remain unchanged during the experiment so researchers can better understand what conditions create a cause-and-effect relationship.
This describes the cause-and-effect relationship. When researchers find causation (or the cause), they’ve conducted all the processes necessary to prove it exists.
- Correlation
Any relationship between two variables in the experiment. It’s important to note that correlation doesn’t automatically mean causation. Researchers will typically establish correlation before proving cause-and-effect.
- Experimental design
Researchers use experimental design to define the parameters of the experiment — e.g. categorizing participants into different groups.
- Dependent variables
These are measurable variables that may change or are influenced by the independent variable. For example, in an experiment about whether or not terrain influences running speed, your dependent variable is the terrain.
Why is causal research useful?
It’s useful because it enables market researchers to predict hypothetical occurrences and outcomes while improving existing strategies. This allows businesses to create plans that benefit the company. It’s also a great research method because researchers can immediately see how variables affect each other and under what circumstances.
Also, once the first experiment has been completed, researchers can use the learnings from the analysis to repeat the experiment or apply the findings to other scenarios. Because of this, it’s widely used to help understand the impact of changes in internal or commercial strategy to the business bottom line.
Some examples include:
- Understanding how overall training levels are improved by introducing new courses
- Examining which variations in wording make potential customers more interested in buying a product
- Testing a market’s response to a brand-new line of products and/or services
So, how does causal research compare and differ from other research types?
Well, there are a few research types that are used to find answers to some of the examples above:
1. Exploratory research
As its name suggests, exploratory research involves assessing a situation (or situations) where the problem isn’t clear. Through this approach, researchers can test different avenues and ideas to establish facts and gain a better understanding.
Researchers can also use it to first navigate a topic and identify which variables are important. Because no area is off-limits, the research is flexible and adapts to the investigations as it progresses.
Finally, this approach is unstructured and often involves gathering qualitative data, giving the researcher freedom to progress the research according to their thoughts and assessment. However, this may make results susceptible to researcher bias and may limit the extent to which a topic is explored.
2. Descriptive research
Descriptive research is all about describing the characteristics of the population, phenomenon or scenario studied. It focuses more on the “what” of the research subject than the “why”.
For example, a clothing brand wants to understand the fashion purchasing trends amongst buyers in California — so they conduct a demographic survey of the region, gather population data and then run descriptive research. The study will help them to uncover purchasing patterns amongst fashion buyers in California, but not necessarily why those patterns exist.
As the research happens in a natural setting, variables can cross-contaminate other variables, making it harder to isolate cause and effect relationships. Therefore, further research will be required if more causal information is needed.
Get started on your market research journey with CoreXM
How is causal research different from the other two methods above?
Well, causal research looks at what variables are involved in a problem and ‘why’ they act a certain way. As the experiment takes place in a controlled setting (thanks to controlled variables) it’s easier to identify cause-and-effect amongst variables.
Furthermore, researchers can carry out causal research at any stage in the process, though it’s usually carried out in the later stages once more is known about a particular topic or situation.
Finally, compared to the other two methods, causal research is more structured, and researchers can combine it with exploratory and descriptive research to assist with research goals.
Summary of three research types
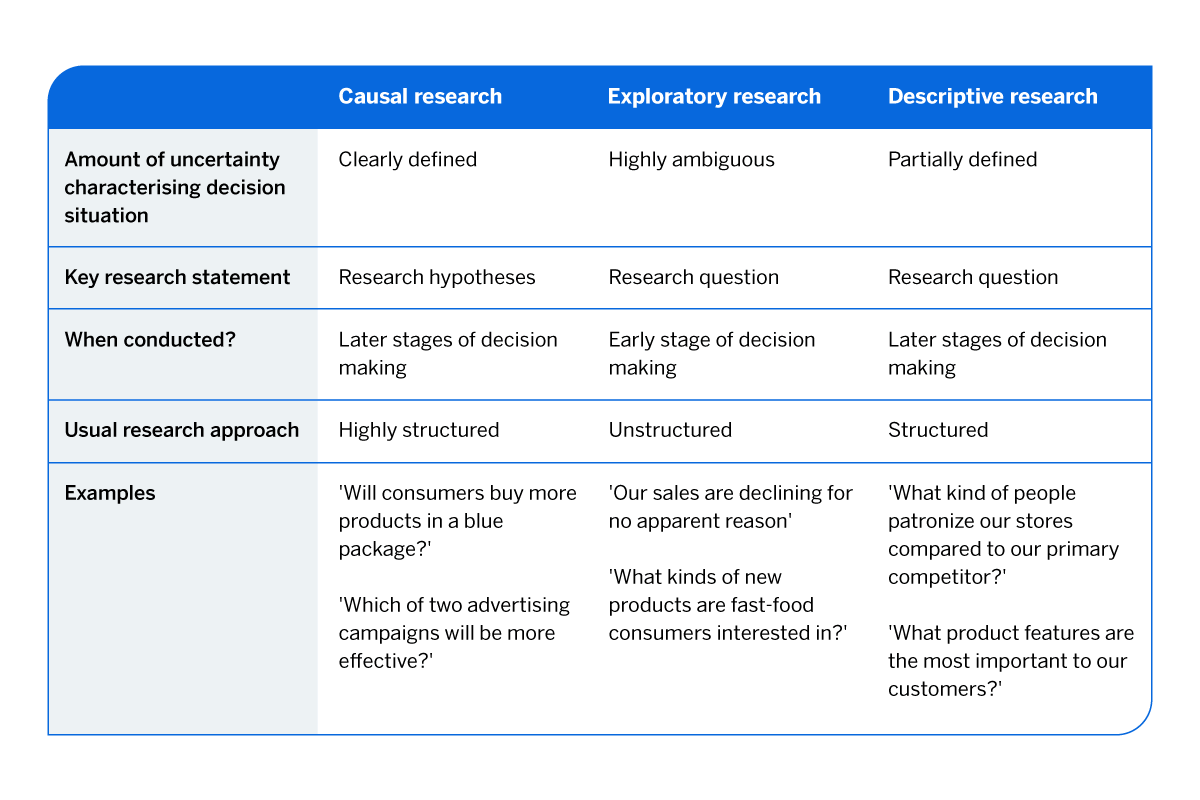
What are the advantages of causal research?
- Improve experiences
By understanding which variables have positive impacts on target variables (like sales revenue or customer loyalty), businesses can improve their processes, return on investment, and the experiences they offer customers and employees.
- Help companies improve internally
By conducting causal research, management can make informed decisions about improving their employee experience and internal operations. For example, understanding which variables led to an increase in staff turnover.
- Repeat experiments to enhance reliability and accuracy of results
When variables are identified, researchers can replicate cause-and-effect with ease, providing them with reliable data and results to draw insights from.
- Test out new theories or ideas
If causal research is able to pinpoint the exact outcome of mixing together different variables, research teams have the ability to test out ideas in the same way to create viable proof of concepts.
- Fix issues quickly
Once an undesirable effect’s cause is identified, researchers and management can take action to reduce the impact of it or remove it entirely, resulting in better outcomes.
What are the disadvantages of causal research?
- Provides information to competitors
If you plan to publish your research, it provides information about your plans to your competitors. For example, they might use your research outcomes to identify what you are up to and enter the market before you.
- Difficult to administer
Causal research is often difficult to administer because it’s not possible to control the effects of extraneous variables.
- Time and money constraints
Budgetary and time constraints can make this type of research expensive to conduct and repeat. Also, if an initial attempt doesn’t provide a cause and effect relationship, the ROI is wasted and could impact the appetite for future repeat experiments.
- Requires additional research to ensure validity
You can’t rely on just the outcomes of causal research as it’s inaccurate. It’s best to conduct other types of research alongside it to confirm its output.
- Trouble establishing cause and effect
Researchers might identify that two variables are connected, but struggle to determine which is the cause and which variable is the effect.
- Risk of contamination
There’s always the risk that people outside your market or area of study could affect the results of your research. For example, if you’re conducting a retail store study, shoppers outside your ‘test parameters’ shop at your store and skew the results.
How can you use causal research effectively?
To better highlight how you can use causal research across functions or markets, here are a few examples:
Market and advertising research
A company might want to know if their new advertising campaign or marketing campaign is having a positive impact. So, their research team can carry out a causal research project to see which variables cause a positive or negative effect on the campaign.
For example, a cold-weather apparel company in a winter ski-resort town may see an increase in sales generated after a targeted campaign to skiers. To see if one caused the other, the research team could set up a duplicate experiment to see if the same campaign would generate sales from non-skiers. If the results reduce or change, then it’s likely that the campaign had a direct effect on skiers to encourage them to purchase products.
Improving customer experiences and loyalty levels
Customers enjoy shopping with brands that align with their own values, and they’re more likely to buy and present the brand positively to other potential shoppers as a result. So, it’s in your best interest to deliver great experiences and retain your customers.
For example, the Harvard Business Review found that an increase in customer retention rates by 5% increased profits by 25% to 95%. But let’s say you want to increase your own, how can you identify which variables contribute to it?Using causal research, you can test hypotheses about which processes, strategies or changes influence customer retention. For example, is it the streamlined checkout? What about the personalized product suggestions? Or maybe it was a new solution that solved their problem? Causal research will help you find out.
Discover how to use analytics to improve customer retention.
Improving problematic employee turnover rates
If your company has a high attrition rate, causal research can help you narrow down the variables or reasons which have the greatest impact on people leaving. This allows you to prioritize your efforts on tackling the issues in the right order, for the best positive outcomes.
For example, through causal research, you might find that employee dissatisfaction due to a lack of communication and transparency from upper management leads to poor morale, which in turn influences employee retention.
To rectify the problem, you could implement a routine feedback loop or session that enables your people to talk to your company’s C-level executives so that they feel heard and understood.
How to conduct causal research first steps to getting started are:
1. Define the purpose of your research
What questions do you have? What do you expect to come out of your research? Think about which variables you need to test out the theory.
2. Pick a random sampling if participants are needed
Using a technology solution to support your sampling, like a database, can help you define who you want your target audience to be, and how random or representative they should be.
3. Set up the controlled experiment
Once you’ve defined which variables you’d like to measure to see if they interact, think about how best to set up the experiment. This could be in-person or in-house via interviews, or it could be done remotely using online surveys.
4. Carry out the experiment
Make sure to keep all irrelevant variables the same, and only change the causal variable (the one that causes the effect) to gather the correct data. Depending on your method, you could be collecting qualitative or quantitative data, so make sure you note your findings across each regularly.
5. Analyze your findings
Either manually or using technology, analyze your data to see if any trends, patterns or correlations emerge. By looking at the data, you’ll be able to see what changes you might need to do next time, or if there are questions that require further research.
6. Verify your findings
Your first attempt gives you the baseline figures to compare the new results to. You can then run another experiment to verify your findings.
7. Do follow-up or supplemental research
You can supplement your original findings by carrying out research that goes deeper into causes or explores the topic in more detail. One of the best ways to do this is to use a survey. See ‘Use surveys to help your experiment’.
Identifying causal relationships between variables
To verify if a causal relationship exists, you have to satisfy the following criteria:
- Nonspurious association
A clear correlation exists between one cause and the effect. In other words, no ‘third’ that relates to both (cause and effect) should exist.
- Temporal sequence
The cause occurs before the effect. For example, increased ad spend on product marketing would contribute to higher product sales.
- Concomitant variation
The variation between the two variables is systematic. For example, if a company doesn’t change its IT policies and technology stack, then changes in employee productivity were not caused by IT policies or technology.
How surveys help your causal research experiments?
There are some surveys that are perfect for assisting researchers with understanding cause and effect. These include:
- Employee Satisfaction Survey – An introductory employee satisfaction survey that provides you with an overview of your current employee experience.
- Manager Feedback Survey – An introductory manager feedback survey geared toward improving your skills as a leader with valuable feedback from your team.
- Net Promoter Score (NPS) Survey – Measure customer loyalty and understand how your customers feel about your product or service using one of the world’s best-recognized metrics.
- Employee Engagement Survey – An entry-level employee engagement survey that provides you with an overview of your current employee experience.
- Customer Satisfaction Survey – Evaluate how satisfied your customers are with your company, including the products and services you provide and how they are treated when they buy from you.
- Employee Exit Interview Survey – Understand why your employees are leaving and how they’ll speak about your company once they’re gone.
- Product Research Survey – Evaluate your consumers’ reaction to a new product or product feature across every stage of the product development journey.
- Brand Awareness Survey – Track the level of brand awareness in your target market, including current and potential future customers.
- Online Purchase Feedback Survey – Find out how well your online shopping experience performs against customer needs and expectations.
That covers the fundamentals of causal research and should give you a foundation for ongoing studies to assess opportunities, problems, and risks across your market, product, customer, and employee segments.
If you want to transform your research, empower your teams and get insights on tap to get ahead of the competition, maybe it’s time to leverage Qualtrics CoreXM.
Qualtrics CoreXM provides a single platform for data collection and analysis across every part of your business — from customer feedback to product concept testing. What’s more, you can integrate it with your existing tools and services thanks to a flexible API.
Qualtrics CoreXM offers you as much or as little power and complexity as you need, so whether you’re running simple surveys or more advanced forms of research, it can deliver every time.
Related resources
Market intelligence 10 min read, marketing insights 11 min read, ethnographic research 11 min read, qualitative vs quantitative research 13 min read, qualitative research questions 11 min read, qualitative research design 12 min read, primary vs secondary research 14 min read, request demo.
Ready to learn more about Qualtrics?
- Survey Software The world’s leading omnichannel survey software
- Online Survey Tools Create sophisticated surveys with ease.
- Mobile Offline Conduct efficient field surveys.
- Text Analysis
- Close The Loop
- Automated Translations
- NPS Dashboard
- CATI Manage high volume phone surveys efficiently
- Cloud/On-premise Dialer TCPA compliant Cloud on-premise dialer
- IVR Survey Software Boost productivity with automated call workflows.
- Analytics Analyze survey data with visual dashboards
- Panel Manager Nurture a loyal community of respondents.
- Survey Portal Best-in-class user friendly survey portal.
- Voxco Audience Conduct targeted sample research in hours.
- Predictive Analytics
- Customer 360
- Customer Loyalty
- Fraud & Risk Management
- AI/ML Enablement Services
- Credit Underwriting

Find the best survey software for you! (Along with a checklist to compare platforms)
Get Buyer’s Guide
- 100+ question types
- Drag-and-drop interface
- Skip logic and branching
- Multi-lingual survey
- Text piping
- Question library
- CSS customization
- White-label surveys
- Customizable ‘Thank You’ page
- Customizable survey theme
- Reminder send-outs
- Survey rewards
- Social media
- SMS surveys
- Website surveys
- Correlation analysis
- Cross-tabulation analysis
- Trend analysis
- Real-time dashboard
- Customizable report
- Email address validation
- Recaptcha validation
- SSL security
Take a peek at our powerful survey features to design surveys that scale discoveries.
Download feature sheet.
- Hospitality
- Financial Services
- Academic Research
- Customer Experience
- Employee Experience
- Product Experience
- Market Research
- Social Research
- Data Analysis
- Banking & Financial Services
- Retail Solution
- Risk Management
- Customer Lifecycle Solutions
- Net Promoter Score
- Customer Behaviour Analytics
- Customer Segmentation
- Data Unification
Explore Voxco
Need to map Voxco’s features & offerings? We can help!
Watch a Demo
Download Brochures
Get a Quote
- NPS Calculator
- CES Calculator
- A/B Testing Calculator
- Margin of Error Calculator
- Sample Size Calculator
- CX Strategy & Management Hub
- Market Research Hub
- Patient Experience Hub
- Employee Experience Hub
- Market Research Guide
- Customer Experience Guide
- The Voxco Guide to Customer Experience
- NPS Knowledge Hub
- Survey Research Guides
- Survey Template Library
- Webinars and Events
- Feature Sheets
- Try a sample survey
- Professional services
- Blogs & White papers
- Case Studies
Find the best customer experience platform
Uncover customer pain points, analyze feedback and run successful CX programs with the best CX platform for your team.
Get the Guide Now

We’ve been avid users of the Voxco platform now for over 20 years. It gives us the flexibility to routinely enhance our survey toolkit and provides our clients with a more robust dataset and story to tell their clients.
VP Innovation & Strategic Partnerships, The Logit Group
- Client Stories
- Voxco Reviews
- Why Voxco Research?
- Why Voxco Intelligence?
- Careers at Voxco
- Vulnerabilities and Ethical Hacking
Explore Regional Offices
- Cloud/On-premise Dialer TCPA compliant Cloud & on-premise dialer
- Fraud & Risk Management
Get Buyer’s Guide
- Banking & Financial Services
Explore Voxco
Watch a Demo
Download Brochures
- CX Strategy & Management Hub
- Blogs & White papers
VP Innovation & Strategic Partnerships, The Logit Group
- Our clients
- Client stories
- Featuresheets
Beyond the Basics: Exploring Research Approaches
SHARE THE ARTICLE ON
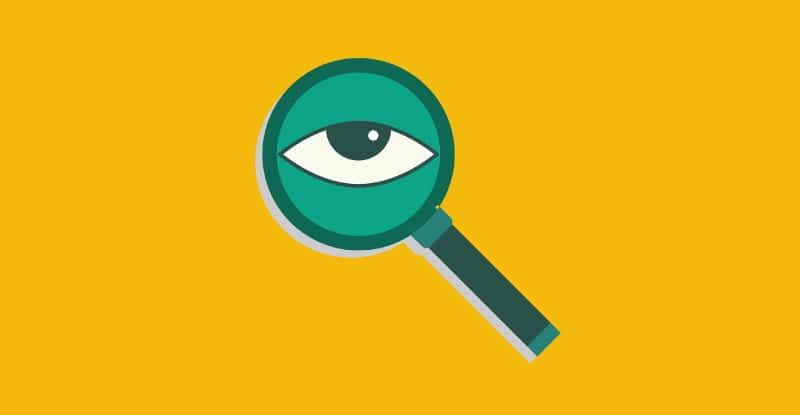
What is Exploratory Research?
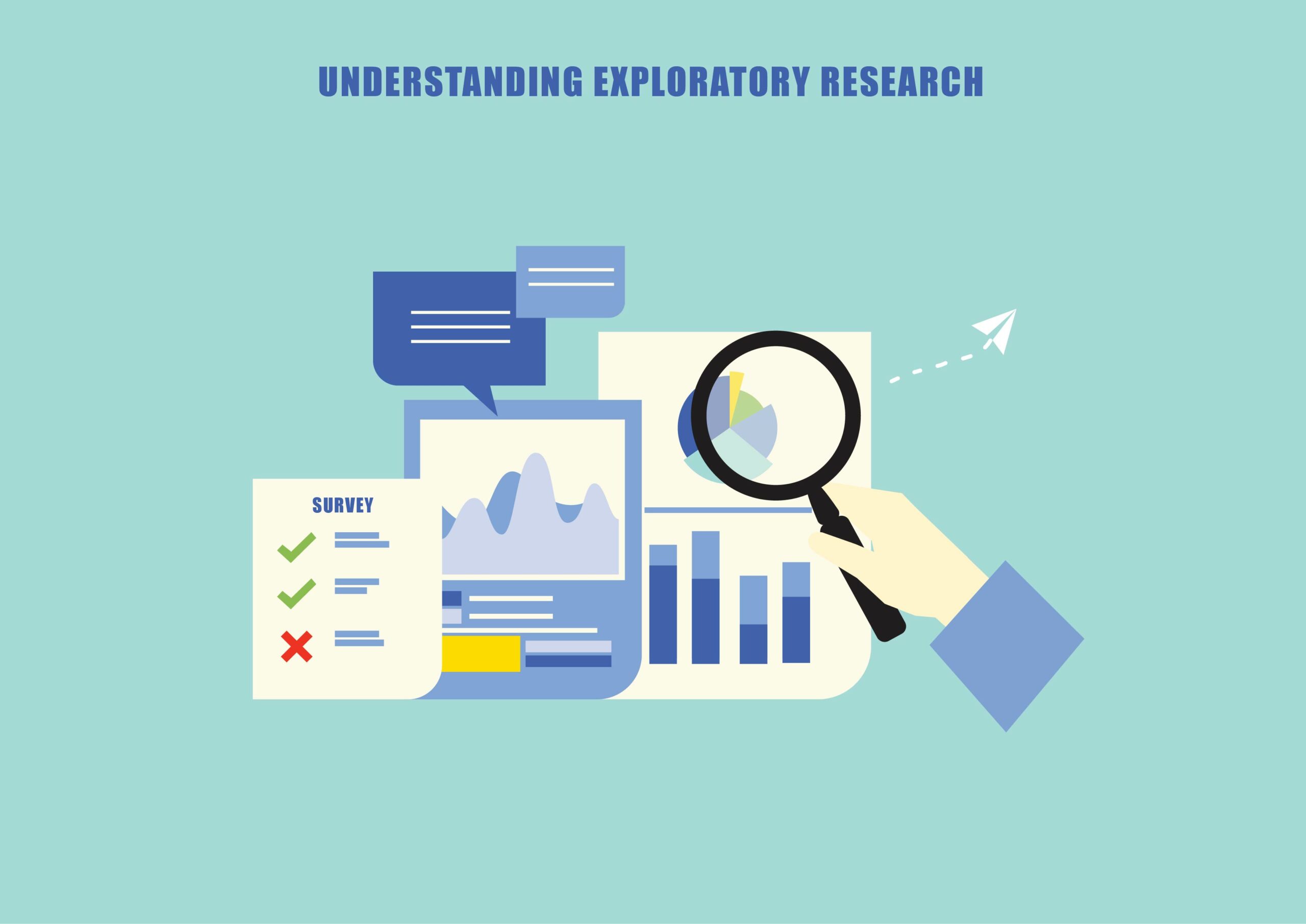
Exploratory research is a research design that is used to investigate a research problem that is not clearly defined or understood. It provides researchers with a deeper understanding of a research problem and its context before further research can be carried out.
Therefore, exploratory research acts as a groundwork to further research and is a useful tool when dealing with research problems that have not been properly investigated in the past.
This research design is also referred to as interpretive research, and helps answer questions like “what”, “where”, and “how”. A key feature of the exploratory research design is that it is unstructured and therefore very flexible in nature.

What are the characteristics of exploratory research?
Some characteristics of exploratory research are:
- It provides the groundwork for further research.
- It is used to investigate issues that aren’t fully defined.
- It is the very first form of research in the research process and therefore takes place before descriptive research.
- It is unstructured in nature.
- It generally involves the use of qualitative research.
Now let’s look at an example of exploratory research to understand it better.
Example of an exploratory research design
Let’s assume a researcher wants to study the effects of social media on a teenager’s attention span. Before going forth with the investigation itself, the researcher may choose to conduct surveys or interviews using open-ended questions.
The responses will be collected from the target audience, which, in this case, comprises those who fall between the ages of 13 to 19. The data collected will provide the researcher with meaningful insights that will help them frame a more specific and realistic research question that can be investigated effectively.
What is Descriptive Research?
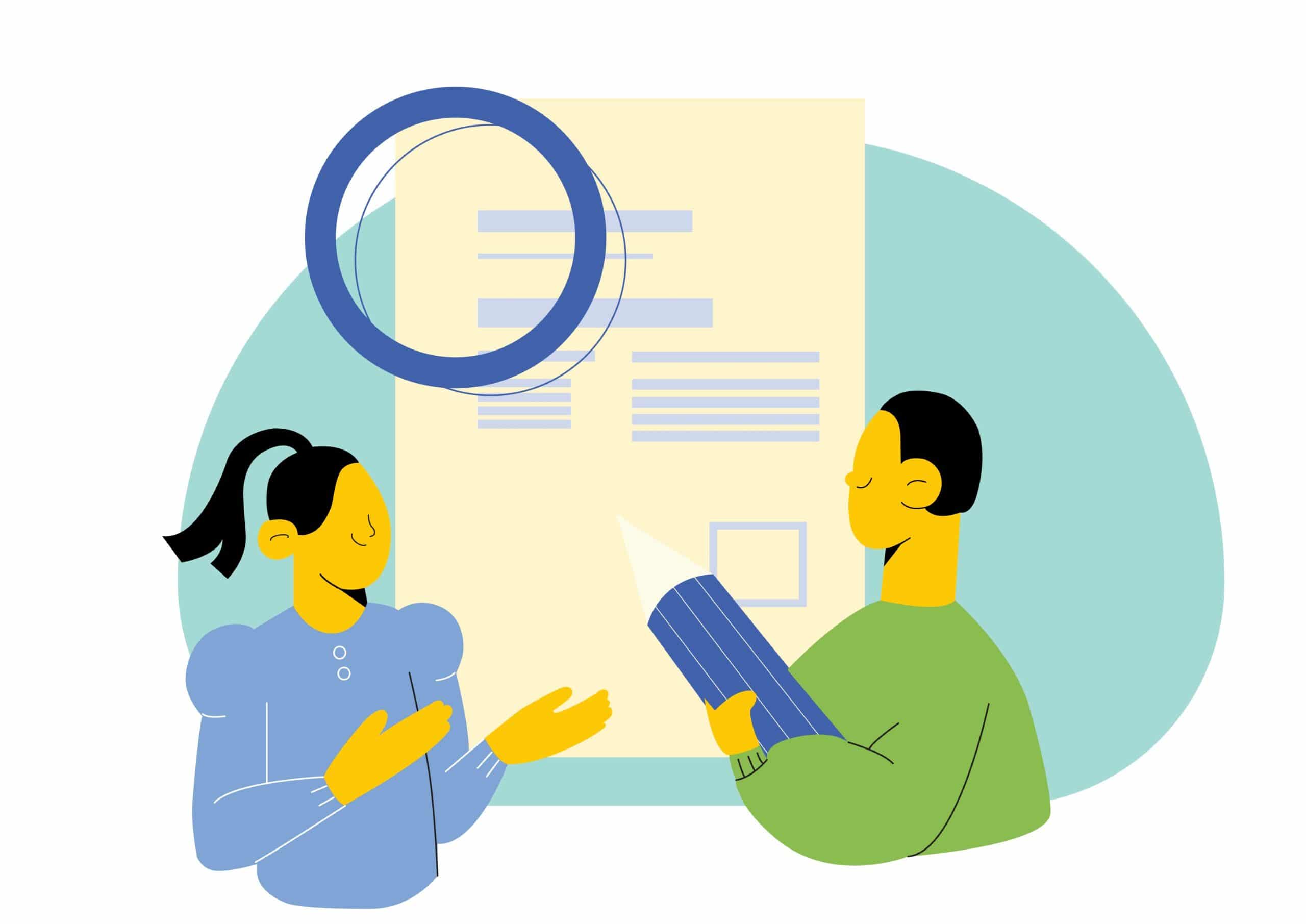
The descriptive research design is used to describe a phenomenon and its different characteristics. It is concerned with gaining a deeper understanding of what the phenomenon is rather than why or how it takes place. It, therefore, describes the subject of the research without addressing why it happens.
Without a thorough understanding of a research problem, researchers cannot effectively answer it. This enforces the importance of descriptive research as it gives researchers a proper understanding of a research problem before they begin investigating it.
When using descriptive research, researchers do not manipulate any variables. Instead, the observational method is used to observe and measure different variables and identify any changes and correlations depicted in the data collected.
What are the characteristics of descriptive research?
These are some of the characteristics of descriptive research:
- Variables aren’t controlled in descriptive research, rather, observational methods are used to conduct the research.
- Descriptive research generally takes the form of a cross-sectional study where multiple sections belonging to the same group are being investigated.
- It provides a base for further research.
Example of a Descriptive Research Design
Let’s take an example of a shoe company that is trying to conduct market research to understand the shoe purchasing trends in the city of Toronto. Before delving into the investigation itself, they may want first to conduct descriptive research to understand which variables and statistics are relevant to their company and, therefore, which variables and statistics need to be investigated.
The descriptive research conducted will provide the company with a deeper understanding of the research topic before the investigation can be commenced.
What is Causal Research?
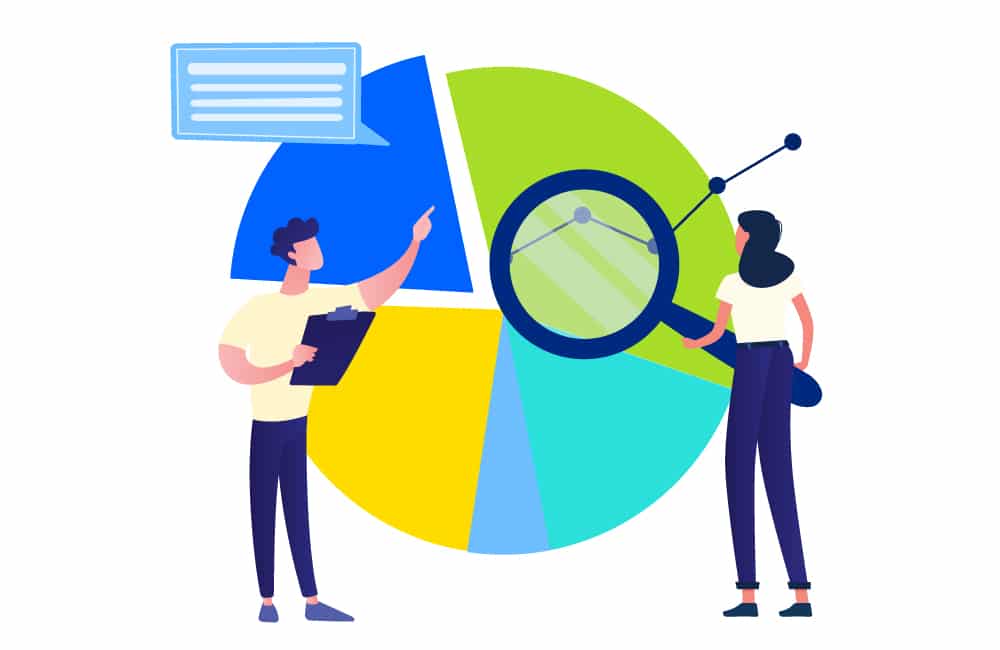
Causal Research is a type of conclusive research that attempts to establish a cause-and-effect relationship between two or more variables.
Several companies widely employ Causal Research. It assists in determining the impact
of a change in process and existing methods. It is easy to narrow down the cause-and-effect relationship by making sure that both variables are not affected by any force other than each other.
In order to maintain accuracy, other variables are assumed to be constant. It can help determine the exact impact an individual variable has on another. This type of research does not only reveal the existence of a cause-and-effect relationship but also explores the link between the two.
Many companies conduct causal research, for example, to find the connection between their customers and the changing prices of their goods. Thus, this method of research can be used by companies to help craft favorable outcomes for themselves.
Such assessment can help businesses navigate their future with fewer interruptions and also help them plan better for various situations.
What are the characteristics of causal research?
Some characteristics of causal research are:
- It follows a temporal sequence, and therefore the “cause” must take place before the “effect.”
- The variation must be systematic between the variables. This is non as concomitant variation.
- The association should be nonspurious, and therefore any covariation between a cause and effect must not be due to a ‘third’ factor.
Example of a Causal Research Design
A researcher is trying to study the effects of alcohol consumption on health. They select a sample group consisting of people who consume different amounts of alcohol and then also observe different metrics that are indicators of health.
This is an example of a causal research design as the researcher is investigating the cause-and-effect relationship between alcohol consumption and a person’s health.
What is the difference between exploratory, descriptive, and causal research?
Now that we’ve developed an understanding of these three research designs, we can take a look at their differences.
One of the key differences between these three designs is their research approach. Causal research has a highly structured and rigid research design and is generally conducted in the later stages of decision-making.
In contrast, exploratory research is highly unstructured. It provides a lot of flexibility as it is generally the first step in any research process and is, therefore, in the early stages of decision-making.
Descriptive research is conducted after explorative research, and its research design has more structure than the exploratory design but less structure than the causal design. In both exploratory and descriptive research, the key research statement is the research question itself. However, in causal research, the key research statement is generally the research hypothesis.
Exploratory research is sometimes confused with descriptive research, as both are conducted in the early stages of a research process. However, there are a few key differences between the two.
Exploratory research provides somewhat of a foundation or a hypothesis about the research problem. It is, therefore, the first form of research that must be conducted when studying an unknown topic.
This is in contrast to descriptive research, which is used to describe a phenomenon that’s already been established, discovered, or suspected in exploratory research. Therefore, descriptive research takes place after exploratory research in the overall research process.
Additionally, the research design in exploratory research is not as rigid as the research design in descriptive research.
Voxco powers 1B surveys annually and helps 500+ global brands gather data, measure sentiment, uncover insights, and act on them.
Get a personalized demo to see how Voxco can help enhance your research efficiency.
The following is an example of exploratory research:
A focus group where a researcher explores the different attributes of cars that matter most to a younger target audience. By gaining an understanding of which attributes are most important to consumers, the researcher can conduct further research on the different price points this target audience would be willing to pay for a car.
Causal research is used to identify the cause-and-effect relationship between variables and provides conclusive results that can answer the research problem. Descriptive research and exploratory research don’t answer a research problem and are instead used to gain a deeper understanding of the problem itself.
The purpose of exploratory research is to give researchers a deeper understanding of a research problem so that it can be investigated effectively. It can be used to formulate research problems, clarify concepts, and form hypotheses.
The causal research design is used when researchers are trying to identify the cause-and-effect relationship between two variables.
The four main types of research design are;
- Descriptive researc h: Seeks to gain a deeper understanding of a research problem and the relationship between the variables
- Correlational Research : Is used to determine the extent to which two variables are related
- Causal-Comparative/Quasi-Experimental Research : Has key differences when compared to true experiments, but has the same aim; to find the cause-and-effect relationship between variables
- Experimental Research : Used the scientific method to establish the cause-and-effect relationship between variables
Explore Voxco Survey Software
+ Omnichannel Survey Software
+ Online Survey Software
+ CATI Survey Software
+ IVR Survey Software
+ Market Research Tool
+ Customer Experience Tool
+ Product Experience Software
+ Enterprise Survey Software

Text Analytics Vs Sentiment Analysis
Text Analytics Vs Sentiment Analysis SHARE THE ARTICLE ON Table of Contents What is Text Analytics with Sentiment Analysis? The bulk of information generated nowadays
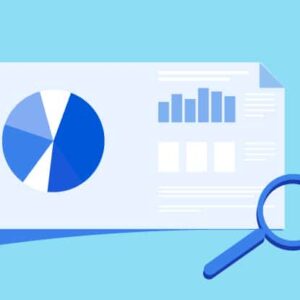
Causation Definition
Dive Deep: Understanding Causation in Modern Research SHARE THE ARTICLE ON Table of Contents What is Causation? Causation is an important and widely used term
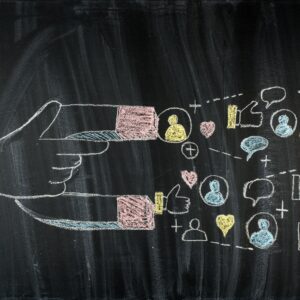
Improving Customer Loyalty: How an impactful Customer Experience Strategy can help?
Improving Customer Loyalty : How an impactful Customer Experience Strategy can help? Try a free Voxco Online sample survey! Unlock your Sample Survey SHARE THE ARTICLE
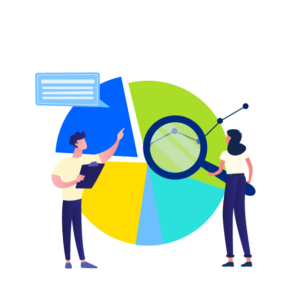
How to Deal With Outliers?
Outliers: Definition, Steps To Recognize, Eliminate Outliers, and Steps To Deal SHARE THE ARTICLE ON Share on facebook Share on twitter Share on linkedin Table

Are surveys qualitative or quantitative research?
Are surveys qualitative or quantitative research? Transform your insight generation process Use our in-depth online survey guide to create an actionable feedback collection survey process.

Role of metadata management
METADATA MANAGEMENT SHARE THE ARTICLE ON Table of Contents Metadata management is the proactive utilization of metadata in an organization to control data in order
We use cookies in our website to give you the best browsing experience and to tailor advertising. By continuing to use our website, you give us consent to the use of cookies. Read More
Looking for the best research tools?
Voxco offers the best online & offline survey research tools!
Have a language expert improve your writing
Run a free plagiarism check in 10 minutes, generate accurate citations for free.
- Knowledge Base
Methodology
- Descriptive Research | Definition, Types, Methods & Examples
Descriptive Research | Definition, Types, Methods & Examples
Published on May 15, 2019 by Shona McCombes . Revised on June 22, 2023.
Descriptive research aims to accurately and systematically describe a population, situation or phenomenon. It can answer what , where , when and how questions , but not why questions.
A descriptive research design can use a wide variety of research methods to investigate one or more variables . Unlike in experimental research , the researcher does not control or manipulate any of the variables, but only observes and measures them.
Table of contents
When to use a descriptive research design, descriptive research methods, other interesting articles.
Descriptive research is an appropriate choice when the research aim is to identify characteristics, frequencies, trends, and categories.
It is useful when not much is known yet about the topic or problem. Before you can research why something happens, you need to understand how, when and where it happens.
Descriptive research question examples
- How has the Amsterdam housing market changed over the past 20 years?
- Do customers of company X prefer product X or product Y?
- What are the main genetic, behavioural and morphological differences between European wildcats and domestic cats?
- What are the most popular online news sources among under-18s?
- How prevalent is disease A in population B?
Here's why students love Scribbr's proofreading services
Discover proofreading & editing
Descriptive research is usually defined as a type of quantitative research , though qualitative research can also be used for descriptive purposes. The research design should be carefully developed to ensure that the results are valid and reliable .
Survey research allows you to gather large volumes of data that can be analyzed for frequencies, averages and patterns. Common uses of surveys include:
- Describing the demographics of a country or region
- Gauging public opinion on political and social topics
- Evaluating satisfaction with a company’s products or an organization’s services
Observations
Observations allow you to gather data on behaviours and phenomena without having to rely on the honesty and accuracy of respondents. This method is often used by psychological, social and market researchers to understand how people act in real-life situations.
Observation of physical entities and phenomena is also an important part of research in the natural sciences. Before you can develop testable hypotheses , models or theories, it’s necessary to observe and systematically describe the subject under investigation.
Case studies
A case study can be used to describe the characteristics of a specific subject (such as a person, group, event or organization). Instead of gathering a large volume of data to identify patterns across time or location, case studies gather detailed data to identify the characteristics of a narrowly defined subject.
Rather than aiming to describe generalizable facts, case studies often focus on unusual or interesting cases that challenge assumptions, add complexity, or reveal something new about a research problem .
If you want to know more about statistics , methodology , or research bias , make sure to check out some of our other articles with explanations and examples.
- Normal distribution
- Degrees of freedom
- Null hypothesis
- Discourse analysis
- Control groups
- Mixed methods research
- Non-probability sampling
- Quantitative research
- Ecological validity
Research bias
- Rosenthal effect
- Implicit bias
- Cognitive bias
- Selection bias
- Negativity bias
- Status quo bias
Cite this Scribbr article
If you want to cite this source, you can copy and paste the citation or click the “Cite this Scribbr article” button to automatically add the citation to our free Citation Generator.
McCombes, S. (2023, June 22). Descriptive Research | Definition, Types, Methods & Examples. Scribbr. Retrieved April 17, 2024, from https://www.scribbr.com/methodology/descriptive-research/
Is this article helpful?
Shona McCombes
Other students also liked, what is quantitative research | definition, uses & methods, correlational research | when & how to use, descriptive statistics | definitions, types, examples, "i thought ai proofreading was useless but..".
I've been using Scribbr for years now and I know it's a service that won't disappoint. It does a good job spotting mistakes”
- Descriptive Research Designs: Types, Examples & Methods

One of the components of research is getting enough information about the research problem—the what, how, when and where answers, which is why descriptive research is an important type of research. It is very useful when conducting research whose aim is to identify characteristics, frequencies, trends, correlations, and categories.
This research method takes a problem with little to no relevant information and gives it a befitting description using qualitative and quantitative research method s. Descriptive research aims to accurately describe a research problem.
In the subsequent sections, we will be explaining what descriptive research means, its types, examples, and data collection methods.
What is Descriptive Research?
Descriptive research is a type of research that describes a population, situation, or phenomenon that is being studied. It focuses on answering the how, what, when, and where questions If a research problem, rather than the why.
This is mainly because it is important to have a proper understanding of what a research problem is about before investigating why it exists in the first place.
For example, an investor considering an investment in the ever-changing Amsterdam housing market needs to understand what the current state of the market is, how it changes (increasing or decreasing), and when it changes (time of the year) before asking for the why. This is where descriptive research comes in.
What Are The Types of Descriptive Research?
Descriptive research is classified into different types according to the kind of approach that is used in conducting descriptive research. The different types of descriptive research are highlighted below:
- Descriptive-survey
Descriptive survey research uses surveys to gather data about varying subjects. This data aims to know the extent to which different conditions can be obtained among these subjects.
For example, a researcher wants to determine the qualification of employed professionals in Maryland. He uses a survey as his research instrument , and each item on the survey related to qualifications is subjected to a Yes/No answer.
This way, the researcher can describe the qualifications possessed by the employed demographics of this community.
- Descriptive-normative survey
This is an extension of the descriptive survey, with the addition being the normative element. In the descriptive-normative survey, the results of the study should be compared with the norm.
For example, an organization that wishes to test the skills of its employees by a team may have them take a skills test. The skills tests are the evaluation tool in this case, and the result of this test is compared with the norm of each role.
If the score of the team is one standard deviation above the mean, it is very satisfactory, if within the mean, satisfactory, and one standard deviation below the mean is unsatisfactory.
- Descriptive-status
This is a quantitative description technique that seeks to answer questions about real-life situations. For example, a researcher researching the income of the employees in a company, and the relationship with their performance.
A survey will be carried out to gather enough data about the income of the employees, then their performance will be evaluated and compared to their income. This will help determine whether a higher income means better performance and low income means lower performance or vice versa.
- Descriptive-analysis
The descriptive-analysis method of research describes a subject by further analyzing it, which in this case involves dividing it into 2 parts. For example, the HR personnel of a company that wishes to analyze the job role of each employee of the company may divide the employees into the people that work at the Headquarters in the US and those that work from Oslo, Norway office.
A questionnaire is devised to analyze the job role of employees with similar salaries and who work in similar positions.
- Descriptive classification
This method is employed in biological sciences for the classification of plants and animals. A researcher who wishes to classify the sea animals into different species will collect samples from various search stations, then classify them accordingly.
- Descriptive-comparative
In descriptive-comparative research, the researcher considers 2 variables that are not manipulated, and establish a formal procedure to conclude that one is better than the other. For example, an examination body wants to determine the better method of conducting tests between paper-based and computer-based tests.
A random sample of potential participants of the test may be asked to use the 2 different methods, and factors like failure rates, time factors, and others will be evaluated to arrive at the best method.
- Correlative Survey
Correlative surveys are used to determine whether the relationship between 2 variables is positive, negative, or neutral. That is, if 2 variables say X and Y are directly proportional, inversely proportional or are not related to each other.
Examples of Descriptive Research
There are different examples of descriptive research, that may be highlighted from its types, uses, and applications. However, we will be restricting ourselves to only 3 distinct examples in this article.
- Comparing Student Performance:
An academic institution may wish 2 compare the performance of its junior high school students in English language and Mathematics. This may be used to classify students based on 2 major groups, with one group going ahead to study while courses, while the other study courses in the Arts & Humanities field.
Students who are more proficient in mathematics will be encouraged to go into STEM and vice versa. Institutions may also use this data to identify students’ weak points and work on ways to assist them.
- Scientific Classification
During the major scientific classification of plants, animals, and periodic table elements, the characteristics and components of each subject are evaluated and used to determine how they are classified.
For example, living things may be classified into kingdom Plantae or kingdom animal is depending on their nature. Further classification may group animals into mammals, pieces, vertebrae, invertebrae, etc.
All these classifications are made a result of descriptive research which describes what they are.
- Human Behavior
When studying human behaviour based on a factor or event, the researcher observes the characteristics, behaviour, and reaction, then use it to conclude. A company willing to sell to its target market needs to first study the behaviour of the market.
This may be done by observing how its target reacts to a competitor’s product, then use it to determine their behaviour.
What are the Characteristics of Descriptive Research?
The characteristics of descriptive research can be highlighted from its definition, applications, data collection methods, and examples. Some characteristics of descriptive research are:
- Quantitativeness
Descriptive research uses a quantitative research method by collecting quantifiable information to be used for statistical analysis of the population sample. This is very common when dealing with research in the physical sciences.
- Qualitativeness
It can also be carried out using the qualitative research method, to properly describe the research problem. This is because descriptive research is more explanatory than exploratory or experimental.
- Uncontrolled variables
In descriptive research, researchers cannot control the variables like they do in experimental research.
- The basis for further research
The results of descriptive research can be further analyzed and used in other research methods. It can also inform the next line of research, including the research method that should be used.
This is because it provides basic information about the research problem, which may give birth to other questions like why a particular thing is the way it is.
Why Use Descriptive Research Design?
Descriptive research can be used to investigate the background of a research problem and get the required information needed to carry out further research. It is used in multiple ways by different organizations, and especially when getting the required information about their target audience.
- Define subject characteristics :
It is used to determine the characteristics of the subjects, including their traits, behaviour, opinion, etc. This information may be gathered with the use of surveys, which are shared with the respondents who in this case, are the research subjects.
For example, a survey evaluating the number of hours millennials in a community spends on the internet weekly, will help a service provider make informed business decisions regarding the market potential of the community.
- Measure Data Trends
It helps to measure the changes in data over some time through statistical methods. Consider the case of individuals who want to invest in stock markets, so they evaluate the changes in prices of the available stocks to make a decision investment decision.
Brokerage companies are however the ones who carry out the descriptive research process, while individuals can view the data trends and make decisions.
Descriptive research is also used to compare how different demographics respond to certain variables. For example, an organization may study how people with different income levels react to the launch of a new Apple phone.
This kind of research may take a survey that will help determine which group of individuals are purchasing the new Apple phone. Do the low-income earners also purchase the phone, or only the high-income earners do?
Further research using another technique will explain why low-income earners are purchasing the phone even though they can barely afford it. This will help inform strategies that will lure other low-income earners and increase company sales.
- Validate existing conditions
When you are not sure about the validity of an existing condition, you can use descriptive research to ascertain the underlying patterns of the research object. This is because descriptive research methods make an in-depth analysis of each variable before making conclusions.
- Conducted Overtime
Descriptive research is conducted over some time to ascertain the changes observed at each point in time. The higher the number of times it is conducted, the more authentic the conclusion will be.
What are the Disadvantages of Descriptive Research?
- Response and Non-response Bias
Respondents may either decide not to respond to questions or give incorrect responses if they feel the questions are too confidential. When researchers use observational methods, respondents may also decide to behave in a particular manner because they feel they are being watched.
- The researcher may decide to influence the result of the research due to personal opinion or bias towards a particular subject. For example, a stockbroker who also has a business of his own may try to lure investors into investing in his own company by manipulating results.
- A case-study or sample taken from a large population is not representative of the whole population.
- Limited scope:The scope of descriptive research is limited to the what of research, with no information on why thereby limiting the scope of the research.
What are the Data Collection Methods in Descriptive Research?
There are 3 main data collection methods in descriptive research, namely; observational method, case study method, and survey research.
1. Observational Method
The observational method allows researchers to collect data based on their view of the behaviour and characteristics of the respondent, with the respondents themselves not directly having an input. It is often used in market research, psychology, and some other social science research to understand human behaviour.
It is also an important aspect of physical scientific research, with it being one of the most effective methods of conducting descriptive research . This process can be said to be either quantitative or qualitative.
Quantitative observation involved the objective collection of numerical data , whose results can be analyzed using numerical and statistical methods.
Qualitative observation, on the other hand, involves the monitoring of characteristics and not the measurement of numbers. The researcher makes his observation from a distance, records it, and is used to inform conclusions.
2. Case Study Method
A case study is a sample group (an individual, a group of people, organizations, events, etc.) whose characteristics are used to describe the characteristics of a larger group in which the case study is a subgroup. The information gathered from investigating a case study may be generalized to serve the larger group.
This generalization, may, however, be risky because case studies are not sufficient to make accurate predictions about larger groups. Case studies are a poor case of generalization.
3. Survey Research
This is a very popular data collection method in research designs. In survey research, researchers create a survey or questionnaire and distribute it to respondents who give answers.
Generally, it is used to obtain quick information directly from the primary source and also conducting rigorous quantitative and qualitative research. In some cases, survey research uses a blend of both qualitative and quantitative strategies.
Survey research can be carried out both online and offline using the following methods
- Online Surveys: This is a cheap method of carrying out surveys and getting enough responses. It can be carried out using Formplus, an online survey builder. Formplus has amazing tools and features that will help increase response rates.
- Offline Surveys: This includes paper forms, mobile offline forms , and SMS-based forms.
What Are The Differences Between Descriptive and Correlational Research?
Before going into the differences between descriptive and correlation research, we need to have a proper understanding of what correlation research is about. Therefore, we will be giving a summary of the correlation research below.
Correlational research is a type of descriptive research, which is used to measure the relationship between 2 variables, with the researcher having no control over them. It aims to find whether there is; positive correlation (both variables change in the same direction), negative correlation (the variables change in the opposite direction), or zero correlation (there is no relationship between the variables).
Correlational research may be used in 2 situations;
(i) when trying to find out if there is a relationship between two variables, and
(ii) when a causal relationship is suspected between two variables, but it is impractical or unethical to conduct experimental research that manipulates one of the variables.
Below are some of the differences between correlational and descriptive research:
- Definitions :
Descriptive research aims is a type of research that provides an in-depth understanding of the study population, while correlational research is the type of research that measures the relationship between 2 variables.
- Characteristics :
Descriptive research provides descriptive data explaining what the research subject is about, while correlation research explores the relationship between data and not their description.
- Predictions :
Predictions cannot be made in descriptive research while correlation research accommodates the possibility of making predictions.
Descriptive Research vs. Causal Research
Descriptive research and causal research are both research methodologies, however, one focuses on a subject’s behaviors while the latter focuses on a relationship’s cause-and-effect. To buttress the above point, descriptive research aims to describe and document the characteristics, behaviors, or phenomena of a particular or specific population or situation.
It focuses on providing an accurate and detailed account of an already existing state of affairs between variables. Descriptive research answers the questions of “what,” “where,” “when,” and “how” without attempting to establish any causal relationships or explain any underlying factors that might have caused the behavior.
Causal research, on the other hand, seeks to determine cause-and-effect relationships between variables. It aims to point out the factors that influence or cause a particular result or behavior. Causal research involves manipulating variables, controlling conditions or a subgroup, and observing the resulting effects. The primary objective of causal research is to establish a cause-effect relationship and provide insights into why certain phenomena happen the way they do.
Descriptive Research vs. Analytical Research
Descriptive research provides a detailed and comprehensive account of a specific situation or phenomenon. It focuses on describing and summarizing data without making inferences or attempting to explain underlying factors or the cause of the factor.
It is primarily concerned with providing an accurate and objective representation of the subject of research. While analytical research goes beyond the description of the phenomena and seeks to analyze and interpret data to discover if there are patterns, relationships, or any underlying factors.
It examines the data critically, applies statistical techniques or other analytical methods, and draws conclusions based on the discovery. Analytical research also aims to explore the relationships between variables and understand the underlying mechanisms or processes involved.
Descriptive Research vs. Exploratory Research
Descriptive research is a research method that focuses on providing a detailed and accurate account of a specific situation, group, or phenomenon. This type of research describes the characteristics, behaviors, or relationships within the given context without looking for an underlying cause.
Descriptive research typically involves collecting and analyzing quantitative or qualitative data to generate descriptive statistics or narratives. Exploratory research differs from descriptive research because it aims to explore and gain firsthand insights or knowledge into a relatively unexplored or poorly understood topic.
It focuses on generating ideas, hypotheses, or theories rather than providing definitive answers. Exploratory research is often conducted at the early stages of a research project to gather preliminary information and identify key variables or factors for further investigation. It involves open-ended interviews, observations, or small-scale surveys to gather qualitative data.
Read More – Exploratory Research: What are its Method & Examples?
Descriptive Research vs. Experimental Research
Descriptive research aims to describe and document the characteristics, behaviors, or phenomena of a particular population or situation. It focuses on providing an accurate and detailed account of the existing state of affairs.
Descriptive research typically involves collecting data through surveys, observations, or existing records and analyzing the data to generate descriptive statistics or narratives. It does not involve manipulating variables or establishing cause-and-effect relationships.
Experimental research, on the other hand, involves manipulating variables and controlling conditions to investigate cause-and-effect relationships. It aims to establish causal relationships by introducing an intervention or treatment and observing the resulting effects.
Experimental research typically involves randomly assigning participants to different groups, such as control and experimental groups, and measuring the outcomes. It allows researchers to control for confounding variables and draw causal conclusions.
Related – Experimental vs Non-Experimental Research: 15 Key Differences
Descriptive Research vs. Explanatory Research
Descriptive research focuses on providing a detailed and accurate account of a specific situation, group, or phenomenon. It aims to describe the characteristics, behaviors, or relationships within the given context.
Descriptive research is primarily concerned with providing an objective representation of the subject of study without explaining underlying causes or mechanisms. Explanatory research seeks to explain the relationships between variables and uncover the underlying causes or mechanisms.
It goes beyond description and aims to understand the reasons or factors that influence a particular outcome or behavior. Explanatory research involves analyzing data, conducting statistical analyses, and developing theories or models to explain the observed relationships.
Descriptive Research vs. Inferential Research
Descriptive research focuses on describing and summarizing data without making inferences or generalizations beyond the specific sample or population being studied. It aims to provide an accurate and objective representation of the subject of study.
Descriptive research typically involves analyzing data to generate descriptive statistics, such as means, frequencies, or percentages, to describe the characteristics or behaviors observed.
Inferential research, however, involves making inferences or generalizations about a larger population based on a smaller sample.
It aims to draw conclusions about the population characteristics or relationships by analyzing the sample data. Inferential research uses statistical techniques to estimate population parameters, test hypotheses, and determine the level of confidence or significance in the findings.
Related – Inferential Statistics: Definition, Types + Examples
Conclusion
The uniqueness of descriptive research partly lies in its ability to explore both quantitative and qualitative research methods. Therefore, when conducting descriptive research, researchers have the opportunity to use a wide variety of techniques that aids the research process.
Descriptive research explores research problems in-depth, beyond the surface level thereby giving a detailed description of the research subject. That way, it can aid further research in the field, including other research methods .
It is also very useful in solving real-life problems in various fields of social science, physical science, and education.
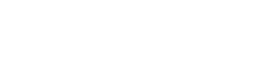
Connect to Formplus, Get Started Now - It's Free!
- descriptive research
- descriptive research method
- example of descriptive research
- types of descriptive research
- busayo.longe
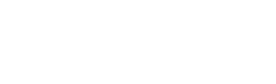
You may also like:
Acceptance Sampling: Meaning, Examples, When to Use
In this post, we will discuss extensively what acceptance sampling is and when it is applied.

Extrapolation in Statistical Research: Definition, Examples, Types, Applications
In this article we’ll look at the different types and characteristics of extrapolation, plus how it contrasts to interpolation.
Type I vs Type II Errors: Causes, Examples & Prevention
This article will discuss the two different types of errors in hypothesis testing and how you can prevent them from occurring in your research
Cross-Sectional Studies: Types, Pros, Cons & Uses
In this article, we’ll look at what cross-sectional studies are, how it applies to your research and how to use Formplus to collect...
Formplus - For Seamless Data Collection
Collect data the right way with a versatile data collection tool. try formplus and transform your work productivity today..
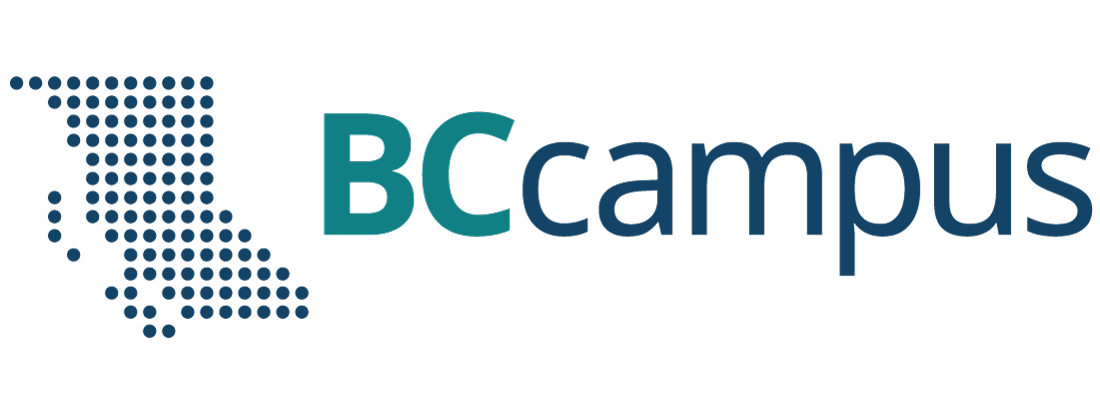
Want to create or adapt books like this? Learn more about how Pressbooks supports open publishing practices.
Chapter 3: Developing a Research Question
3.2 Exploration, Description, Explanation
As you can see, there is much to think about and many decisions to be made as you begin to define your research question and your research project. Something else you will need to consider in the early stages is whether your research will be exploratory, descriptive, or explanatory. Each of these types of research has a different aim or purpose, consequently, how you design your research project will be determined in part by this decision. In the following paragraphs we will look at these three types of research.
Exploratory research
Researchers conducting exploratory research are typically at the early stages of examining their topics. These sorts of projects are usually conducted when a researcher wants to test the feasibility of conducting a more extensive study; he or she wants to figure out the lay of the land with respect to the particular topic. Perhaps very little prior research has been conducted on this subject. If this is the case, a researcher may wish to do some exploratory work to learn what method to use in collecting data, how best to approach research participants, or even what sorts of questions are reasonable to ask. A researcher wanting to simply satisfy his or her own curiosity about a topic could also conduct exploratory research. Conducting exploratory research on a topic is often a necessary first step, both to satisfy researcher curiosity about the subject and to better understand the phenomenon and the research participants in order to design a larger, subsequent study. See Table 2.1 for examples.
Descriptive research
Sometimes the goal of research is to describe or define a particular phenomenon. In this case, descriptive research would be an appropriate strategy. A descriptive may, for example, aim to describe a pattern. For example, researchers often collect information to describe something for the benefit of the general public. Market researchers rely on descriptive research to tell them what consumers think of their products. In fact, descriptive research has many useful applications, and you probably rely on findings from descriptive research without even being aware that that is what you are doing. See Table 3.1 for examples.
Explanatory research
The third type of research, explanatory research, seeks to answer “why” questions. In this case, the researcher is trying to identify the causes and effects of whatever phenomenon is being studied. An explanatory study of college students’ addictions to their electronic gadgets, for example, might aim to understand why students become addicted. Does it have anything to do with their family histories? Does it have anything to do with their other extracurricular hobbies and activities? Does it have anything to do with the people with whom they spend their time? An explanatory study could answer these kinds of questions. See Table 3.1 for examples.
Table 3.1 Exploratory, descriptive and explanatory research differences (Adapted from Adjei, n.d.).
Research Methods for the Social Sciences: An Introduction Copyright © 2020 by Valerie Sheppard is licensed under a Creative Commons Attribution-NonCommercial-ShareAlike 4.0 International License , except where otherwise noted.
Share This Book
Causal-Comparative vs. Descriptive
What's the difference.
Causal-comparative and descriptive research are both types of quantitative research methods used in social science research. Causal-comparative research aims to determine the cause of a particular phenomenon by comparing two or more groups that differ in one key variable. On the other hand, descriptive research focuses on describing the characteristics of a population or phenomenon without manipulating any variables. While causal-comparative research allows researchers to make inferences about cause and effect relationships, descriptive research provides a detailed snapshot of a particular situation or group. Both methods have their own strengths and limitations, and the choice between the two depends on the research question and objectives.
Further Detail
Introduction.
Research is a crucial aspect of any field, as it helps in understanding phenomena, making decisions, and solving problems. Two common types of research methods used in various disciplines are causal-comparative and descriptive research. While both methods aim to gather information and analyze data, they have distinct attributes that set them apart. In this article, we will compare the attributes of causal-comparative and descriptive research to understand their differences and similarities.
Causal-Comparative Research
Causal-comparative research, also known as ex post facto research, involves studying the relationship between variables without manipulating them. This type of research aims to determine the cause of a particular phenomenon by comparing groups that differ in one key variable. Researchers use this method when it is not possible or ethical to conduct an experiment. Causal-comparative research is often used in educational and social sciences to explore the effects of certain variables on outcomes.
One of the key attributes of causal-comparative research is its retrospective nature. Researchers analyze existing data to draw conclusions about the relationship between variables. This method allows researchers to study phenomena that have already occurred and cannot be manipulated. By comparing groups that differ in a specific variable, researchers can infer causality and make predictions about future outcomes.
Causal-comparative research is also characterized by its focus on identifying causal relationships between variables. Researchers aim to determine whether a particular variable causes changes in another variable. By comparing groups with different levels of the independent variable, researchers can assess the impact of that variable on the dependent variable. This method helps in understanding the underlying mechanisms of a phenomenon and predicting its future behavior.
Another attribute of causal-comparative research is its ability to control for extraneous variables. Researchers carefully select groups that differ in one key variable while keeping other variables constant. This allows researchers to isolate the effect of the independent variable on the dependent variable. By controlling for extraneous variables, researchers can establish a causal relationship between variables and make valid conclusions about the phenomenon under study.
In summary, causal-comparative research is a retrospective method that aims to determine causal relationships between variables by comparing groups that differ in one key variable. This method allows researchers to control for extraneous variables and make predictions about future outcomes based on existing data.
Descriptive Research
Descriptive research, on the other hand, focuses on describing and analyzing a phenomenon without manipulating variables. This type of research aims to provide a detailed account of a particular phenomenon, behavior, or group. Descriptive research is often used in social sciences, psychology, and market research to understand the characteristics of a population, group, or event.
One of the key attributes of descriptive research is its observational nature. Researchers observe and record data without intervening or manipulating variables. This method allows researchers to gather information about a phenomenon as it naturally occurs in its environment. By collecting descriptive data, researchers can identify patterns, trends, and relationships within the data set.
Descriptive research is also characterized by its emphasis on objectivity and accuracy. Researchers strive to collect data that is reliable, valid, and representative of the phenomenon under study. By using standardized measurement tools and techniques, researchers can ensure the quality of the data collected. This method helps in producing accurate and unbiased descriptions of the phenomenon.
Another attribute of descriptive research is its focus on generalizability. Researchers aim to draw conclusions that can be applied to a larger population or group. By collecting data from a representative sample, researchers can make inferences about the characteristics of the population as a whole. This method helps in understanding the broader implications of the phenomenon and making informed decisions based on the data collected.
In summary, descriptive research is an observational method that aims to describe and analyze a phenomenon without manipulating variables. This method focuses on objectivity, accuracy, and generalizability to provide a detailed account of the phenomenon under study.
While causal-comparative and descriptive research have distinct attributes, they also share some similarities. Both methods aim to gather information, analyze data, and draw conclusions about a phenomenon. However, they differ in their approach to studying variables and the level of control over the research process.
- Causal-comparative research focuses on determining causal relationships between variables by comparing groups that differ in one key variable.
- Descriptive research aims to provide a detailed account of a phenomenon without manipulating variables, focusing on objectivity and generalizability.
- Causal-comparative research is retrospective and aims to predict future outcomes based on existing data.
- Descriptive research is observational and aims to describe patterns, trends, and relationships within a data set.
- Both methods contribute to the advancement of knowledge in various disciplines by providing valuable insights into complex phenomena.
In conclusion, causal-comparative and descriptive research are two important methods used in various fields to study phenomena, analyze data, and draw conclusions. While they have distinct attributes, both methods play a crucial role in advancing knowledge and understanding complex phenomena. Researchers can choose the method that best suits their research questions and objectives to conduct meaningful and impactful studies.
Comparisons may contain inaccurate information about people, places, or facts. Please report any issues.
What is causal research design?
Last updated
14 May 2023
Reviewed by
Examining these relationships gives researchers valuable insights into the mechanisms that drive the phenomena they are investigating.
Organizations primarily use causal research design to identify, determine, and explore the impact of changes within an organization and the market. You can use a causal research design to evaluate the effects of certain changes on existing procedures, norms, and more.
This article explores causal research design, including its elements, advantages, and disadvantages.
Analyze your causal research
Dovetail streamlines causal research analysis to help you uncover and share actionable insights
- Components of causal research
You can demonstrate the existence of cause-and-effect relationships between two factors or variables using specific causal information, allowing you to produce more meaningful results and research implications.
These are the key inputs for causal research:
The timeline of events
Ideally, the cause must occur before the effect. You should review the timeline of two or more separate events to determine the independent variables (cause) from the dependent variables (effect) before developing a hypothesis.
If the cause occurs before the effect, you can link cause and effect and develop a hypothesis .
For instance, an organization may notice a sales increase. Determining the cause would help them reproduce these results.
Upon review, the business realizes that the sales boost occurred right after an advertising campaign. The business can leverage this time-based data to determine whether the advertising campaign is the independent variable that caused a change in sales.
Evaluation of confounding variables
In most cases, you need to pinpoint the variables that comprise a cause-and-effect relationship when using a causal research design. This uncovers a more accurate conclusion.
Co-variations between a cause and effect must be accurate, and a third factor shouldn’t relate to cause and effect.
Observing changes
Variation links between two variables must be clear. A quantitative change in effect must happen solely due to a quantitative change in the cause.
You can test whether the independent variable changes the dependent variable to evaluate the validity of a cause-and-effect relationship. A steady change between the two variables must occur to back up your hypothesis of a genuine causal effect.
- Why is causal research useful?
Causal research allows market researchers to predict hypothetical occurrences and outcomes while enhancing existing strategies. Organizations can use this concept to develop beneficial plans.
Causal research is also useful as market researchers can immediately deduce the effect of the variables on each other under real-world conditions.
Once researchers complete their first experiment, they can use their findings. Applying them to alternative scenarios or repeating the experiment to confirm its validity can produce further insights.
Businesses widely use causal research to identify and comprehend the effect of strategic changes on their profits.
- How does causal research compare and differ from other research types?
Other research types that identify relationships between variables include exploratory and descriptive research .
Here’s how they compare and differ from causal research designs:
Exploratory research
An exploratory research design evaluates situations where a problem or opportunity's boundaries are unclear. You can use this research type to test various hypotheses and assumptions to establish facts and understand a situation more clearly.
You can also use exploratory research design to navigate a topic and discover the relevant variables. This research type allows flexibility and adaptability as the experiment progresses, particularly since no area is off-limits.
It’s worth noting that exploratory research is unstructured and typically involves collecting qualitative data . This provides the freedom to tweak and amend the research approach according to your ongoing thoughts and assessments.
Unfortunately, this exposes the findings to the risk of bias and may limit the extent to which a researcher can explore a topic.
This table compares the key characteristics of causal and exploratory research:
Descriptive research
This research design involves capturing and describing the traits of a population, situation, or phenomenon. Descriptive research focuses more on the " what " of the research subject and less on the " why ."
Since descriptive research typically happens in a real-world setting, variables can cross-contaminate others. This increases the challenge of isolating cause-and-effect relationships.
You may require further research if you need more causal links.
This table compares the key characteristics of causal and descriptive research.
Causal research examines a research question’s variables and how they interact. It’s easier to pinpoint cause and effect since the experiment often happens in a controlled setting.
Researchers can conduct causal research at any stage, but they typically use it once they know more about the topic.
In contrast, causal research tends to be more structured and can be combined with exploratory and descriptive research to help you attain your research goals.
- How can you use causal research effectively?
Here are common ways that market researchers leverage causal research effectively:
Market and advertising research
Do you want to know if your new marketing campaign is affecting your organization positively? You can use causal research to determine the variables causing negative or positive impacts on your campaign.
Improving customer experiences and loyalty levels
Consumers generally enjoy purchasing from brands aligned with their values. They’re more likely to purchase from such brands and positively represent them to others.
You can use causal research to identify the variables contributing to increased or reduced customer acquisition and retention rates.
Could the cause of increased customer retention rates be streamlined checkout?
Perhaps you introduced a new solution geared towards directly solving their immediate problem.
Whatever the reason, causal research can help you identify the cause-and-effect relationship. You can use this to enhance your customer experiences and loyalty levels.
Improving problematic employee turnover rates
Is your organization experiencing skyrocketing attrition rates?
You can leverage the features and benefits of causal research to narrow down the possible explanations or variables with significant effects on employees quitting.
This way, you can prioritize interventions, focusing on the highest priority causal influences, and begin to tackle high employee turnover rates.
- Advantages of causal research
The main benefits of causal research include the following:
Effectively test new ideas
If causal research can pinpoint the precise outcome through combinations of different variables, researchers can test ideas in the same manner to form viable proof of concepts.
Achieve more objective results
Market researchers typically use random sampling techniques to choose experiment participants or subjects in causal research. This reduces the possibility of exterior, sample, or demography-based influences, generating more objective results.
Improved business processes
Causal research helps businesses understand which variables positively impact target variables, such as customer loyalty or sales revenues. This helps them improve their processes, ROI, and customer and employee experiences.
Guarantee reliable and accurate results
Upon identifying the correct variables, researchers can replicate cause and effect effortlessly. This creates reliable data and results to draw insights from.
Internal organization improvements
Businesses that conduct causal research can make informed decisions about improving their internal operations and enhancing employee experiences.
- Disadvantages of causal research
Like any other research method, casual research has its set of drawbacks that include:
Extra research to ensure validity
Researchers can't simply rely on the outcomes of causal research since it isn't always accurate. There may be a need to conduct other research types alongside it to ensure accurate output.
Coincidence
Coincidence tends to be the most significant error in causal research. Researchers often misinterpret a coincidental link between a cause and effect as a direct causal link.
Administration challenges
Causal research can be challenging to administer since it's impossible to control the impact of extraneous variables .
Giving away your competitive advantage
If you intend to publish your research, it exposes your information to the competition.
Competitors may use your research outcomes to identify your plans and strategies to enter the market before you.
- Causal research examples
Multiple fields can use causal research, so it serves different purposes, such as.
Customer loyalty research
Organizations and employees can use causal research to determine the best customer attraction and retention approaches.
They monitor interactions between customers and employees to identify cause-and-effect patterns. That could be a product demonstration technique resulting in higher or lower sales from the same customers.
Example: Business X introduces a new individual marketing strategy for a small customer group and notices a measurable increase in monthly subscriptions.
Upon getting identical results from different groups, the business concludes that the individual marketing strategy resulted in the intended causal relationship.
Advertising research
Businesses can also use causal research to implement and assess advertising campaigns.
Example: Business X notices a 7% increase in sales revenue a few months after a business introduces a new advertisement in a certain region. The business can run the same ad in random regions to compare sales data over the same period.
This will help the company determine whether the ad caused the sales increase. If sales increase in these randomly selected regions, the business could conclude that advertising campaigns and sales share a cause-and-effect relationship.
Educational research
Academics, teachers, and learners can use causal research to explore the impact of politics on learners and pinpoint learner behavior trends.
Example: College X notices that more IT students drop out of their program in their second year, which is 8% higher than any other year.
The college administration can interview a random group of IT students to identify factors leading to this situation, including personal factors and influences.
With the help of in-depth statistical analysis, the institution's researchers can uncover the main factors causing dropout. They can create immediate solutions to address the problem.
Is a causal variable dependent or independent?
When two variables have a cause-and-effect relationship, the cause is often called the independent variable. As such, the effect variable is dependent, i.e., it depends on the independent causal variable. An independent variable is only causal under experimental conditions.
What are the three criteria for causality?
The three conditions for causality are:
Temporality/temporal precedence: The cause must precede the effect.
Rationality: One event predicts the other with an explanation, and the effect must vary in proportion to changes in the cause.
Control for extraneous variables: The covariables must not result from other variables.
Is causal research experimental?
Causal research is mostly explanatory. Causal studies focus on analyzing a situation to explore and explain the patterns of relationships between variables.
Further, experiments are the primary data collection methods in studies with causal research design. However, as a research design, causal research isn't entirely experimental.
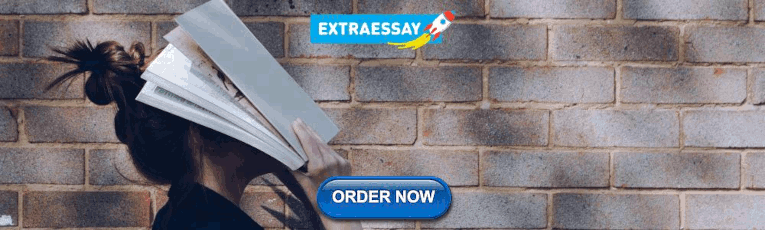
What is the difference between experimental and causal research design?
One of the main differences between causal and experimental research is that in causal research, the research subjects are already in groups since the event has already happened.
On the other hand, researchers randomly choose subjects in experimental research before manipulating the variables.
Get started today
Go from raw data to valuable insights with a flexible research platform
Editor’s picks
Last updated: 21 December 2023
Last updated: 16 December 2023
Last updated: 6 October 2023
Last updated: 5 March 2024
Last updated: 25 November 2023
Last updated: 15 February 2024
Last updated: 11 March 2024
Last updated: 12 December 2023
Last updated: 6 March 2024
Last updated: 10 April 2023
Last updated: 20 December 2023
Latest articles
Related topics, log in or sign up.
Get started for free
Causal Research: Definition, Design, Tips, Examples
Appinio Research · 21.02.2024 · 33min read
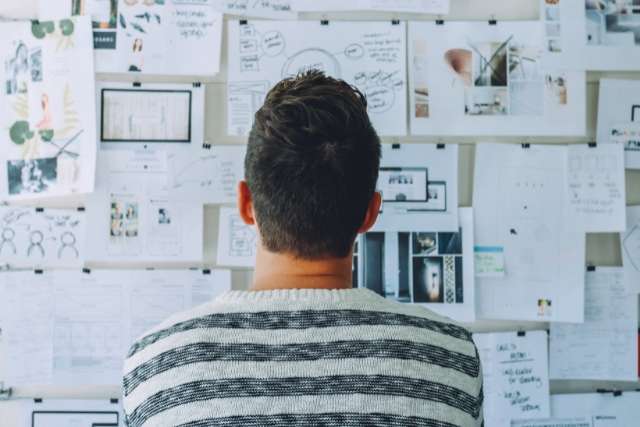
Ever wondered why certain events lead to specific outcomes? Understanding causality—the relationship between cause and effect—is crucial for unraveling the mysteries of the world around us. In this guide on causal research, we delve into the methods, techniques, and principles behind identifying and establishing cause-and-effect relationships between variables. Whether you're a seasoned researcher or new to the field, this guide will equip you with the knowledge and tools to conduct rigorous causal research and draw meaningful conclusions that can inform decision-making and drive positive change.
What is Causal Research?
Causal research is a methodological approach used in scientific inquiry to investigate cause-and-effect relationships between variables. Unlike correlational or descriptive research, which merely examine associations or describe phenomena, causal research aims to determine whether changes in one variable cause changes in another variable.
Importance of Causal Research
Understanding the importance of causal research is crucial for appreciating its role in advancing knowledge and informing decision-making across various fields. Here are key reasons why causal research is significant:
- Establishing Causality: Causal research enables researchers to determine whether changes in one variable directly cause changes in another variable. This helps identify effective interventions, predict outcomes, and inform evidence-based practices.
- Guiding Policy and Practice: By identifying causal relationships, causal research provides empirical evidence to support policy decisions, program interventions, and business strategies. Decision-makers can use causal findings to allocate resources effectively and address societal challenges.
- Informing Predictive Modeling: Causal research contributes to the development of predictive models by elucidating causal mechanisms underlying observed phenomena. Predictive models based on causal relationships can accurately forecast future outcomes and trends.
- Advancing Scientific Knowledge: Causal research contributes to the cumulative body of scientific knowledge by testing hypotheses, refining theories, and uncovering underlying mechanisms of phenomena. It fosters a deeper understanding of complex systems and phenomena.
- Mitigating Confounding Factors: Understanding causal relationships allows researchers to control for confounding variables and reduce bias in their studies. By isolating the effects of specific variables, researchers can draw more valid and reliable conclusions.
Causal Research Distinction from Other Research
Understanding the distinctions between causal research and other types of research methodologies is essential for researchers to choose the most appropriate approach for their study objectives. Let's explore the differences and similarities between causal research and descriptive, exploratory, and correlational research methodologies .
Descriptive vs. Causal Research
Descriptive research focuses on describing characteristics, behaviors, or phenomena without manipulating variables or establishing causal relationships. It provides a snapshot of the current state of affairs but does not attempt to explain why certain phenomena occur.
Causal research , on the other hand, seeks to identify cause-and-effect relationships between variables by systematically manipulating independent variables and observing their effects on dependent variables. Unlike descriptive research, causal research aims to determine whether changes in one variable directly cause changes in another variable.
Similarities:
- Both descriptive and causal research involve empirical observation and data collection.
- Both types of research contribute to the scientific understanding of phenomena, albeit through different approaches.
Differences:
- Descriptive research focuses on describing phenomena, while causal research aims to explain why phenomena occur by identifying causal relationships.
- Descriptive research typically uses observational methods, while causal research often involves experimental designs or causal inference techniques to establish causality.
Exploratory vs. Causal Research
Exploratory research aims to explore new topics, generate hypotheses, or gain initial insights into phenomena. It is often conducted when little is known about a subject and seeks to generate ideas for further investigation.
Causal research , on the other hand, is concerned with testing hypotheses and establishing cause-and-effect relationships between variables. It builds on existing knowledge and seeks to confirm or refute causal hypotheses through systematic investigation.
- Both exploratory and causal research contribute to the generation of knowledge and theory development.
- Both types of research involve systematic inquiry and data analysis to answer research questions.
- Exploratory research focuses on generating hypotheses and exploring new areas of inquiry, while causal research aims to test hypotheses and establish causal relationships.
- Exploratory research is more flexible and open-ended, while causal research follows a more structured and hypothesis-driven approach.
Correlational vs. Causal Research
Correlational research examines the relationship between variables without implying causation. It identifies patterns of association or co-occurrence between variables but does not establish the direction or causality of the relationship.
Causal research , on the other hand, seeks to establish cause-and-effect relationships between variables by systematically manipulating independent variables and observing their effects on dependent variables. It goes beyond mere association to determine whether changes in one variable directly cause changes in another variable.
- Both correlational and causal research involve analyzing relationships between variables.
- Both types of research contribute to understanding the nature of associations between variables.
- Correlational research focuses on identifying patterns of association, while causal research aims to establish causal relationships.
- Correlational research does not manipulate variables, while causal research involves systematically manipulating independent variables to observe their effects on dependent variables.
How to Formulate Causal Research Hypotheses?
Crafting research questions and hypotheses is the foundational step in any research endeavor. Defining your variables clearly and articulating the causal relationship you aim to investigate is essential. Let's explore this process further.
1. Identify Variables
Identifying variables involves recognizing the key factors you will manipulate or measure in your study. These variables can be classified into independent, dependent, and confounding variables.
- Independent Variable (IV): This is the variable you manipulate or control in your study. It is the presumed cause that you want to test.
- Dependent Variable (DV): The dependent variable is the outcome or response you measure. It is affected by changes in the independent variable.
- Confounding Variables: These are extraneous factors that may influence the relationship between the independent and dependent variables, leading to spurious correlations or erroneous causal inferences. Identifying and controlling for confounding variables is crucial for establishing valid causal relationships.
2. Establish Causality
Establishing causality requires meeting specific criteria outlined by scientific methodology. While correlation between variables may suggest a relationship, it does not imply causation. To establish causality, researchers must demonstrate the following:
- Temporal Precedence: The cause must precede the effect in time. In other words, changes in the independent variable must occur before changes in the dependent variable.
- Covariation of Cause and Effect: Changes in the independent variable should be accompanied by corresponding changes in the dependent variable. This demonstrates a consistent pattern of association between the two variables.
- Elimination of Alternative Explanations: Researchers must rule out other possible explanations for the observed relationship between variables. This involves controlling for confounding variables and conducting rigorous experimental designs to isolate the effects of the independent variable.
3. Write Clear and Testable Hypotheses
Hypotheses serve as tentative explanations for the relationship between variables and provide a framework for empirical testing. A well-formulated hypothesis should be:
- Specific: Clearly state the expected relationship between the independent and dependent variables.
- Testable: The hypothesis should be capable of being empirically tested through observation or experimentation.
- Falsifiable: There should be a possibility of proving the hypothesis false through empirical evidence.
For example, a hypothesis in a study examining the effect of exercise on weight loss could be: "Increasing levels of physical activity (IV) will lead to greater weight loss (DV) among participants (compared to those with lower levels of physical activity)."
By formulating clear hypotheses and operationalizing variables, researchers can systematically investigate causal relationships and contribute to the advancement of scientific knowledge.
Causal Research Design
Designing your research study involves making critical decisions about how you will collect and analyze data to investigate causal relationships.
Experimental vs. Observational Designs
One of the first decisions you'll make when designing a study is whether to employ an experimental or observational design. Each approach has its strengths and limitations, and the choice depends on factors such as the research question, feasibility , and ethical considerations.
- Experimental Design: In experimental designs, researchers manipulate the independent variable and observe its effects on the dependent variable while controlling for confounding variables. Random assignment to experimental conditions allows for causal inferences to be drawn. Example: A study testing the effectiveness of a new teaching method on student performance by randomly assigning students to either the experimental group (receiving the new teaching method) or the control group (receiving the traditional method).
- Observational Design: Observational designs involve observing and measuring variables without intervention. Researchers may still examine relationships between variables but cannot establish causality as definitively as in experimental designs. Example: A study observing the association between socioeconomic status and health outcomes by collecting data on income, education level, and health indicators from a sample of participants.
Control and Randomization
Control and randomization are crucial aspects of experimental design that help ensure the validity of causal inferences.
- Control: Controlling for extraneous variables involves holding constant factors that could influence the dependent variable, except for the independent variable under investigation. This helps isolate the effects of the independent variable. Example: In a medication trial, controlling for factors such as age, gender, and pre-existing health conditions ensures that any observed differences in outcomes can be attributed to the medication rather than other variables.
- Randomization: Random assignment of participants to experimental conditions helps distribute potential confounders evenly across groups, reducing the likelihood of systematic biases and allowing for causal conclusions. Example: Randomly assigning patients to treatment and control groups in a clinical trial ensures that both groups are comparable in terms of baseline characteristics, minimizing the influence of extraneous variables on treatment outcomes.
Internal and External Validity
Two key concepts in research design are internal validity and external validity, which relate to the credibility and generalizability of study findings, respectively.
- Internal Validity: Internal validity refers to the extent to which the observed effects can be attributed to the manipulation of the independent variable rather than confounding factors. Experimental designs typically have higher internal validity due to their control over extraneous variables. Example: A study examining the impact of a training program on employee productivity would have high internal validity if it could confidently attribute changes in productivity to the training intervention.
- External Validity: External validity concerns the extent to which study findings can be generalized to other populations, settings, or contexts. While experimental designs prioritize internal validity, they may sacrifice external validity by using highly controlled conditions that do not reflect real-world scenarios. Example: Findings from a laboratory study on memory retention may have limited external validity if the experimental tasks and conditions differ significantly from real-life learning environments.
Types of Experimental Designs
Several types of experimental designs are commonly used in causal research, each with its own strengths and applications.
- Randomized Control Trials (RCTs): RCTs are considered the gold standard for assessing causality in research. Participants are randomly assigned to experimental and control groups, allowing researchers to make causal inferences. Example: A pharmaceutical company testing a new drug's efficacy would use an RCT to compare outcomes between participants receiving the drug and those receiving a placebo.
- Quasi-Experimental Designs: Quasi-experimental designs lack random assignment but still attempt to establish causality by controlling for confounding variables through design or statistical analysis . Example: A study evaluating the effectiveness of a smoking cessation program might compare outcomes between participants who voluntarily enroll in the program and a matched control group of non-enrollees.
By carefully selecting an appropriate research design and addressing considerations such as control, randomization, and validity, researchers can conduct studies that yield credible evidence of causal relationships and contribute valuable insights to their field of inquiry.
Causal Research Data Collection
Collecting data is a critical step in any research study, and the quality of the data directly impacts the validity and reliability of your findings.
Choosing Measurement Instruments
Selecting appropriate measurement instruments is essential for accurately capturing the variables of interest in your study. The choice of measurement instrument depends on factors such as the nature of the variables, the target population , and the research objectives.
- Surveys : Surveys are commonly used to collect self-reported data on attitudes, opinions, behaviors, and demographics . They can be administered through various methods, including paper-and-pencil surveys, online surveys, and telephone interviews.
- Observations: Observational methods involve systematically recording behaviors, events, or phenomena as they occur in natural settings. Observations can be structured (following a predetermined checklist) or unstructured (allowing for flexible data collection).
- Psychological Tests: Psychological tests are standardized instruments designed to measure specific psychological constructs, such as intelligence, personality traits, or emotional functioning. These tests often have established reliability and validity.
- Physiological Measures: Physiological measures, such as heart rate, blood pressure, or brain activity, provide objective data on bodily processes. They are commonly used in health-related research but require specialized equipment and expertise.
- Existing Databases: Researchers may also utilize existing datasets, such as government surveys, public health records, or organizational databases, to answer research questions. Secondary data analysis can be cost-effective and time-saving but may be limited by the availability and quality of data.
Ensuring accurate data collection is the cornerstone of any successful research endeavor. With the right tools in place, you can unlock invaluable insights to drive your causal research forward. From surveys to tests, each instrument offers a unique lens through which to explore your variables of interest.
At Appinio , we understand the importance of robust data collection methods in informing impactful decisions. Let us empower your research journey with our intuitive platform, where you can effortlessly gather real-time consumer insights to fuel your next breakthrough. Ready to take your research to the next level? Book a demo today and see how Appinio can revolutionize your approach to data collection!
Book a Demo
Sampling Techniques
Sampling involves selecting a subset of individuals or units from a larger population to participate in the study. The goal of sampling is to obtain a representative sample that accurately reflects the characteristics of the population of interest.
- Probability Sampling: Probability sampling methods involve randomly selecting participants from the population, ensuring that each member of the population has an equal chance of being included in the sample. Common probability sampling techniques include simple random sampling , stratified sampling, and cluster sampling .
- Non-Probability Sampling: Non-probability sampling methods do not involve random selection and may introduce biases into the sample. Examples of non-probability sampling techniques include convenience sampling, purposive sampling, and snowball sampling.
The choice of sampling technique depends on factors such as the research objectives, population characteristics, resources available, and practical constraints. Researchers should strive to minimize sampling bias and maximize the representativeness of the sample to enhance the generalizability of their findings.
Ethical Considerations
Ethical considerations are paramount in research and involve ensuring the rights, dignity, and well-being of research participants. Researchers must adhere to ethical principles and guidelines established by professional associations and institutional review boards (IRBs).
- Informed Consent: Participants should be fully informed about the nature and purpose of the study, potential risks and benefits, their rights as participants, and any confidentiality measures in place. Informed consent should be obtained voluntarily and without coercion.
- Privacy and Confidentiality: Researchers should take steps to protect the privacy and confidentiality of participants' personal information. This may involve anonymizing data, securing data storage, and limiting access to identifiable information.
- Minimizing Harm: Researchers should mitigate any potential physical, psychological, or social harm to participants. This may involve conducting risk assessments, providing appropriate support services, and debriefing participants after the study.
- Respect for Participants: Researchers should respect participants' autonomy, diversity, and cultural values. They should seek to foster a trusting and respectful relationship with participants throughout the research process.
- Publication and Dissemination: Researchers have a responsibility to accurately report their findings and acknowledge contributions from participants and collaborators. They should adhere to principles of academic integrity and transparency in disseminating research results.
By addressing ethical considerations in research design and conduct, researchers can uphold the integrity of their work, maintain trust with participants and the broader community, and contribute to the responsible advancement of knowledge in their field.
Causal Research Data Analysis
Once data is collected, it must be analyzed to draw meaningful conclusions and assess causal relationships.
Causal Inference Methods
Causal inference methods are statistical techniques used to identify and quantify causal relationships between variables in observational data. While experimental designs provide the most robust evidence for causality, observational studies often require more sophisticated methods to account for confounding factors.
- Difference-in-Differences (DiD): DiD compares changes in outcomes before and after an intervention between a treatment group and a control group, controlling for pre-existing trends. It estimates the average treatment effect by differencing the changes in outcomes between the two groups over time.
- Instrumental Variables (IV): IV analysis relies on instrumental variables—variables that affect the treatment variable but not the outcome—to estimate causal effects in the presence of endogeneity. IVs should be correlated with the treatment but uncorrelated with the error term in the outcome equation.
- Regression Discontinuity (RD): RD designs exploit naturally occurring thresholds or cutoff points to estimate causal effects near the threshold. Participants just above and below the threshold are compared, assuming that they are similar except for their proximity to the threshold.
- Propensity Score Matching (PSM): PSM matches individuals or units based on their propensity scores—the likelihood of receiving the treatment—creating comparable groups with similar observed characteristics. Matching reduces selection bias and allows for causal inference in observational studies.
Assessing Causality Strength
Assessing the strength of causality involves determining the magnitude and direction of causal effects between variables. While statistical significance indicates whether an observed relationship is unlikely to occur by chance, it does not necessarily imply a strong or meaningful effect.
- Effect Size: Effect size measures the magnitude of the relationship between variables, providing information about the practical significance of the results. Standard effect size measures include Cohen's d for mean differences and odds ratios for categorical outcomes.
- Confidence Intervals: Confidence intervals provide a range of values within which the actual effect size is likely to lie with a certain degree of certainty. Narrow confidence intervals indicate greater precision in estimating the true effect size.
- Practical Significance: Practical significance considers whether the observed effect is meaningful or relevant in real-world terms. Researchers should interpret results in the context of their field and the implications for stakeholders.
Handling Confounding Variables
Confounding variables are extraneous factors that may distort the observed relationship between the independent and dependent variables, leading to spurious or biased conclusions. Addressing confounding variables is essential for establishing valid causal inferences.
- Statistical Control: Statistical control involves including confounding variables as covariates in regression models to partially out their effects on the outcome variable. Controlling for confounders reduces bias and strengthens the validity of causal inferences.
- Matching: Matching participants or units based on observed characteristics helps create comparable groups with similar distributions of confounding variables. Matching reduces selection bias and mimics the randomization process in experimental designs.
- Sensitivity Analysis: Sensitivity analysis assesses the robustness of study findings to changes in model specifications or assumptions. By varying analytical choices and examining their impact on results, researchers can identify potential sources of bias and evaluate the stability of causal estimates.
- Subgroup Analysis: Subgroup analysis explores whether the relationship between variables differs across subgroups defined by specific characteristics. Identifying effect modifiers helps understand the conditions under which causal effects may vary.
By employing rigorous causal inference methods, assessing the strength of causality, and addressing confounding variables, researchers can confidently draw valid conclusions about causal relationships in their studies, advancing scientific knowledge and informing evidence-based decision-making.
Causal Research Examples
Examples play a crucial role in understanding the application of causal research methods and their impact across various domains. Let's explore some detailed examples to illustrate how causal research is conducted and its real-world implications:
Example 1: Software as a Service (SaaS) User Retention Analysis
Suppose a SaaS company wants to understand the factors influencing user retention and engagement with their platform. The company conducts a longitudinal observational study, collecting data on user interactions, feature usage, and demographic information over several months.
- Design: The company employs an observational cohort study design, tracking cohorts of users over time to observe changes in retention and engagement metrics. They use analytics tools to collect data on user behavior , such as logins, feature usage, session duration, and customer support interactions.
- Data Collection: Data is collected from the company's platform logs, customer relationship management (CRM) system, and user surveys. Key metrics include user churn rates, active user counts, feature adoption rates, and Net Promoter Scores ( NPS ).
- Analysis: Using statistical techniques like survival analysis and regression modeling, the company identifies factors associated with user retention, such as feature usage patterns, onboarding experiences, customer support interactions, and subscription plan types.
- Findings: The analysis reveals that users who engage with specific features early in their lifecycle have higher retention rates, while those who encounter usability issues or lack personalized onboarding experiences are more likely to churn. The company uses these insights to optimize product features, improve onboarding processes, and enhance customer support strategies to increase user retention and satisfaction.
Example 2: Business Impact of Digital Marketing Campaign
Consider a technology startup launching a digital marketing campaign to promote its new product offering. The company conducts an experimental study to evaluate the effectiveness of different marketing channels in driving website traffic, lead generation, and sales conversions.
- Design: The company implements an A/B testing design, randomly assigning website visitors to different marketing treatment conditions, such as Google Ads, social media ads, email campaigns, or content marketing efforts. They track user interactions and conversion events using web analytics tools and marketing automation platforms.
- Data Collection: Data is collected on website traffic, click-through rates, conversion rates, lead generation, and sales revenue. The company also gathers demographic information and user feedback through surveys and customer interviews to understand the impact of marketing messages and campaign creatives .
- Analysis: Utilizing statistical methods like hypothesis testing and multivariate analysis, the company compares key performance metrics across different marketing channels to assess their effectiveness in driving user engagement and conversion outcomes. They calculate return on investment (ROI) metrics to evaluate the cost-effectiveness of each marketing channel.
- Findings: The analysis reveals that social media ads outperform other marketing channels in generating website traffic and lead conversions, while email campaigns are more effective in nurturing leads and driving sales conversions. Armed with these insights, the company allocates marketing budgets strategically, focusing on channels that yield the highest ROI and adjusting messaging and targeting strategies to optimize campaign performance.
These examples demonstrate the diverse applications of causal research methods in addressing important questions, informing policy decisions, and improving outcomes in various fields. By carefully designing studies, collecting relevant data, employing appropriate analysis techniques, and interpreting findings rigorously, researchers can generate valuable insights into causal relationships and contribute to positive social change.
How to Interpret Causal Research Results?
Interpreting and reporting research findings is a crucial step in the scientific process, ensuring that results are accurately communicated and understood by stakeholders.
Interpreting Statistical Significance
Statistical significance indicates whether the observed results are unlikely to occur by chance alone, but it does not necessarily imply practical or substantive importance. Interpreting statistical significance involves understanding the meaning of p-values and confidence intervals and considering their implications for the research findings.
- P-values: A p-value represents the probability of obtaining the observed results (or more extreme results) if the null hypothesis is true. A p-value below a predetermined threshold (typically 0.05) suggests that the observed results are statistically significant, indicating that the null hypothesis can be rejected in favor of the alternative hypothesis.
- Confidence Intervals: Confidence intervals provide a range of values within which the true population parameter is likely to lie with a certain degree of confidence (e.g., 95%). If the confidence interval does not include the null value, it suggests that the observed effect is statistically significant at the specified confidence level.
Interpreting statistical significance requires considering factors such as sample size, effect size, and the practical relevance of the results rather than relying solely on p-values to draw conclusions.
Discussing Practical Significance
While statistical significance indicates whether an effect exists, practical significance evaluates the magnitude and meaningfulness of the effect in real-world terms. Discussing practical significance involves considering the relevance of the results to stakeholders and assessing their impact on decision-making and practice.
- Effect Size: Effect size measures the magnitude of the observed effect, providing information about its practical importance. Researchers should interpret effect sizes in the context of their field and the scale of measurement (e.g., small, medium, or large effect sizes).
- Contextual Relevance: Consider the implications of the results for stakeholders, policymakers, and practitioners. Are the observed effects meaningful in the context of existing knowledge, theory, or practical applications? How do the findings contribute to addressing real-world problems or informing decision-making?
Discussing practical significance helps contextualize research findings and guide their interpretation and application in practice, beyond statistical significance alone.
Addressing Limitations and Assumptions
No study is without limitations, and researchers should transparently acknowledge and address potential biases, constraints, and uncertainties in their research design and findings.
- Methodological Limitations: Identify any limitations in study design, data collection, or analysis that may affect the validity or generalizability of the results. For example, sampling biases , measurement errors, or confounding variables.
- Assumptions: Discuss any assumptions made in the research process and their implications for the interpretation of results. Assumptions may relate to statistical models, causal inference methods, or theoretical frameworks underlying the study.
- Alternative Explanations: Consider alternative explanations for the observed results and discuss their potential impact on the validity of causal inferences. How robust are the findings to different interpretations or competing hypotheses?
Addressing limitations and assumptions demonstrates transparency and rigor in the research process, allowing readers to critically evaluate the validity and reliability of the findings.
Communicating Findings Clearly
Effectively communicating research findings is essential for disseminating knowledge, informing decision-making, and fostering collaboration and dialogue within the scientific community.
- Clarity and Accessibility: Present findings in a clear, concise, and accessible manner, using plain language and avoiding jargon or technical terminology. Organize information logically and use visual aids (e.g., tables, charts, graphs) to enhance understanding.
- Contextualization: Provide context for the results by summarizing key findings, highlighting their significance, and relating them to existing literature or theoretical frameworks. Discuss the implications of the findings for theory, practice, and future research directions.
- Transparency: Be transparent about the research process, including data collection procedures, analytical methods, and any limitations or uncertainties associated with the findings. Clearly state any conflicts of interest or funding sources that may influence interpretation.
By communicating findings clearly and transparently, researchers can facilitate knowledge exchange, foster trust and credibility, and contribute to evidence-based decision-making.
Causal Research Tips
When conducting causal research, it's essential to approach your study with careful planning, attention to detail, and methodological rigor. Here are some tips to help you navigate the complexities of causal research effectively:
- Define Clear Research Questions: Start by clearly defining your research questions and hypotheses. Articulate the causal relationship you aim to investigate and identify the variables involved.
- Consider Alternative Explanations: Be mindful of potential confounding variables and alternative explanations for the observed relationships. Take steps to control for confounders and address alternative hypotheses in your analysis.
- Prioritize Internal Validity: While external validity is important for generalizability, prioritize internal validity in your study design to ensure that observed effects can be attributed to the manipulation of the independent variable.
- Use Randomization When Possible: If feasible, employ randomization in experimental designs to distribute potential confounders evenly across experimental conditions and enhance the validity of causal inferences.
- Be Transparent About Methods: Provide detailed descriptions of your research methods, including data collection procedures, analytical techniques, and any assumptions or limitations associated with your study.
- Utilize Multiple Methods: Consider using a combination of experimental and observational methods to triangulate findings and strengthen the validity of causal inferences.
- Be Mindful of Sample Size: Ensure that your sample size is adequate to detect meaningful effects and minimize the risk of Type I and Type II errors. Conduct power analyses to determine the sample size needed to achieve sufficient statistical power.
- Validate Measurement Instruments: Validate your measurement instruments to ensure that they are reliable and valid for assessing the variables of interest in your study. Pilot test your instruments if necessary.
- Seek Feedback from Peers: Collaborate with colleagues or seek feedback from peer reviewers to solicit constructive criticism and improve the quality of your research design and analysis.
Conclusion for Causal Research
Mastering causal research empowers researchers to unlock the secrets of cause and effect, shedding light on the intricate relationships between variables in diverse fields. By employing rigorous methods such as experimental designs, causal inference techniques, and careful data analysis, you can uncover causal mechanisms, predict outcomes, and inform evidence-based practices. Through the lens of causal research, complex phenomena become more understandable, and interventions become more effective in addressing societal challenges and driving progress. In a world where understanding the reasons behind events is paramount, causal research serves as a beacon of clarity and insight. Armed with the knowledge and techniques outlined in this guide, you can navigate the complexities of causality with confidence, advancing scientific knowledge, guiding policy decisions, and ultimately making meaningful contributions to our understanding of the world.
How to Conduct Causal Research in Minutes?
Introducing Appinio , your gateway to lightning-fast causal research. As a real-time market research platform, we're revolutionizing how companies gain consumer insights to drive data-driven decisions. With Appinio, conducting your own market research is not only easy but also thrilling. Experience the excitement of market research with Appinio, where fast, intuitive, and impactful insights are just a click away.
Here's why you'll love Appinio:
- Instant Insights: Say goodbye to waiting days for research results. With our platform, you'll go from questions to insights in minutes, empowering you to make decisions at the speed of business.
- User-Friendly Interface: No need for a research degree here! Our intuitive platform is designed for anyone to use, making complex research tasks simple and accessible.
- Global Reach: Reach your target audience wherever they are. With access to over 90 countries and the ability to define precise target groups from 1200+ characteristics, you'll gather comprehensive data to inform your decisions.
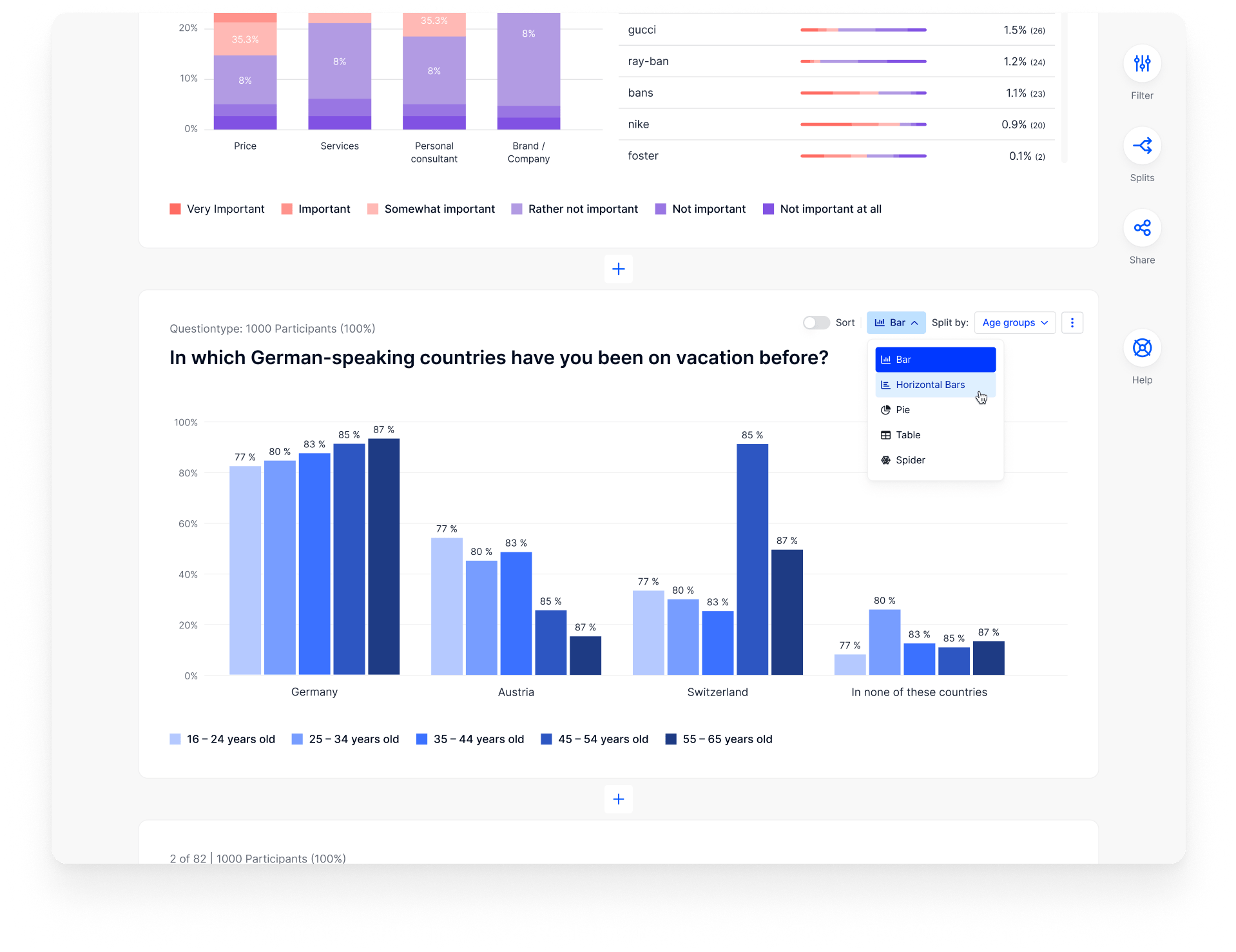
Get free access to the platform!
Join the loop 💌
Be the first to hear about new updates, product news, and data insights. We'll send it all straight to your inbox.
Get the latest market research news straight to your inbox! 💌
Wait, there's more
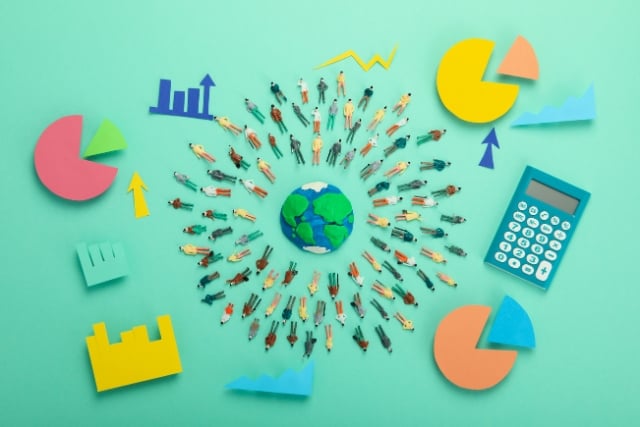
17.04.2024 | 25min read
Quota Sampling: Definition, Types, Methods, Examples
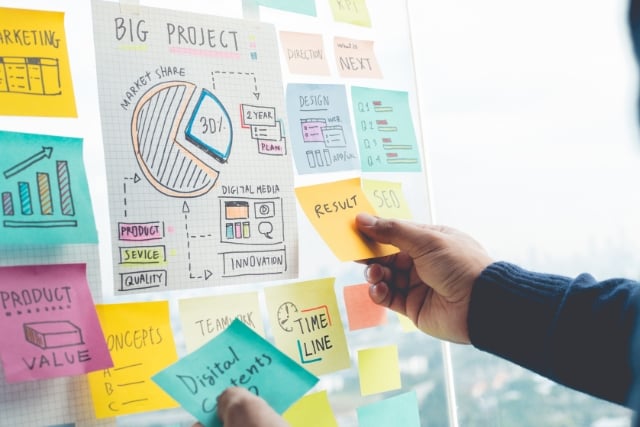
15.04.2024 | 34min read
What is Market Share? Definition, Formula, Examples
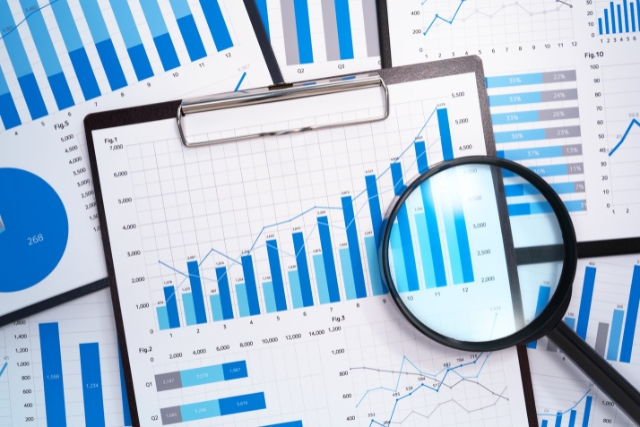
11.04.2024 | 34min read
What is Data Analysis? Definition, Tools, Examples
- Privacy Policy
Buy Me a Coffee

Home » Descriptive Research Design – Types, Methods and Examples
Descriptive Research Design – Types, Methods and Examples
Table of Contents

Descriptive Research Design
Definition:
Descriptive research design is a type of research methodology that aims to describe or document the characteristics, behaviors, attitudes, opinions, or perceptions of a group or population being studied.
Descriptive research design does not attempt to establish cause-and-effect relationships between variables or make predictions about future outcomes. Instead, it focuses on providing a detailed and accurate representation of the data collected, which can be useful for generating hypotheses, exploring trends, and identifying patterns in the data.
Types of Descriptive Research Design
Types of Descriptive Research Design are as follows:
Cross-sectional Study
This involves collecting data at a single point in time from a sample or population to describe their characteristics or behaviors. For example, a researcher may conduct a cross-sectional study to investigate the prevalence of certain health conditions among a population, or to describe the attitudes and beliefs of a particular group.
Longitudinal Study
This involves collecting data over an extended period of time, often through repeated observations or surveys of the same group or population. Longitudinal studies can be used to track changes in attitudes, behaviors, or outcomes over time, or to investigate the effects of interventions or treatments.
This involves an in-depth examination of a single individual, group, or situation to gain a detailed understanding of its characteristics or dynamics. Case studies are often used in psychology, sociology, and business to explore complex phenomena or to generate hypotheses for further research.
Survey Research
This involves collecting data from a sample or population through standardized questionnaires or interviews. Surveys can be used to describe attitudes, opinions, behaviors, or demographic characteristics of a group, and can be conducted in person, by phone, or online.
Observational Research
This involves observing and documenting the behavior or interactions of individuals or groups in a natural or controlled setting. Observational studies can be used to describe social, cultural, or environmental phenomena, or to investigate the effects of interventions or treatments.
Correlational Research
This involves examining the relationships between two or more variables to describe their patterns or associations. Correlational studies can be used to identify potential causal relationships or to explore the strength and direction of relationships between variables.
Data Analysis Methods
Descriptive research design data analysis methods depend on the type of data collected and the research question being addressed. Here are some common methods of data analysis for descriptive research:
Descriptive Statistics
This method involves analyzing data to summarize and describe the key features of a sample or population. Descriptive statistics can include measures of central tendency (e.g., mean, median, mode) and measures of variability (e.g., range, standard deviation).
Cross-tabulation
This method involves analyzing data by creating a table that shows the frequency of two or more variables together. Cross-tabulation can help identify patterns or relationships between variables.
Content Analysis
This method involves analyzing qualitative data (e.g., text, images, audio) to identify themes, patterns, or trends. Content analysis can be used to describe the characteristics of a sample or population, or to identify factors that influence attitudes or behaviors.
Qualitative Coding
This method involves analyzing qualitative data by assigning codes to segments of data based on their meaning or content. Qualitative coding can be used to identify common themes, patterns, or categories within the data.
Visualization
This method involves creating graphs or charts to represent data visually. Visualization can help identify patterns or relationships between variables and make it easier to communicate findings to others.
Comparative Analysis
This method involves comparing data across different groups or time periods to identify similarities and differences. Comparative analysis can help describe changes in attitudes or behaviors over time or differences between subgroups within a population.
Applications of Descriptive Research Design
Descriptive research design has numerous applications in various fields. Some of the common applications of descriptive research design are:
- Market research: Descriptive research design is widely used in market research to understand consumer preferences, behavior, and attitudes. This helps companies to develop new products and services, improve marketing strategies, and increase customer satisfaction.
- Health research: Descriptive research design is used in health research to describe the prevalence and distribution of a disease or health condition in a population. This helps healthcare providers to develop prevention and treatment strategies.
- Educational research: Descriptive research design is used in educational research to describe the performance of students, schools, or educational programs. This helps educators to improve teaching methods and develop effective educational programs.
- Social science research: Descriptive research design is used in social science research to describe social phenomena such as cultural norms, values, and beliefs. This helps researchers to understand social behavior and develop effective policies.
- Public opinion research: Descriptive research design is used in public opinion research to understand the opinions and attitudes of the general public on various issues. This helps policymakers to develop effective policies that are aligned with public opinion.
- Environmental research: Descriptive research design is used in environmental research to describe the environmental conditions of a particular region or ecosystem. This helps policymakers and environmentalists to develop effective conservation and preservation strategies.
Descriptive Research Design Examples
Here are some real-time examples of descriptive research designs:
- A restaurant chain wants to understand the demographics and attitudes of its customers. They conduct a survey asking customers about their age, gender, income, frequency of visits, favorite menu items, and overall satisfaction. The survey data is analyzed using descriptive statistics and cross-tabulation to describe the characteristics of their customer base.
- A medical researcher wants to describe the prevalence and risk factors of a particular disease in a population. They conduct a cross-sectional study in which they collect data from a sample of individuals using a standardized questionnaire. The data is analyzed using descriptive statistics and cross-tabulation to identify patterns in the prevalence and risk factors of the disease.
- An education researcher wants to describe the learning outcomes of students in a particular school district. They collect test scores from a representative sample of students in the district and use descriptive statistics to calculate the mean, median, and standard deviation of the scores. They also create visualizations such as histograms and box plots to show the distribution of scores.
- A marketing team wants to understand the attitudes and behaviors of consumers towards a new product. They conduct a series of focus groups and use qualitative coding to identify common themes and patterns in the data. They also create visualizations such as word clouds to show the most frequently mentioned topics.
- An environmental scientist wants to describe the biodiversity of a particular ecosystem. They conduct an observational study in which they collect data on the species and abundance of plants and animals in the ecosystem. The data is analyzed using descriptive statistics to describe the diversity and richness of the ecosystem.
How to Conduct Descriptive Research Design
To conduct a descriptive research design, you can follow these general steps:
- Define your research question: Clearly define the research question or problem that you want to address. Your research question should be specific and focused to guide your data collection and analysis.
- Choose your research method: Select the most appropriate research method for your research question. As discussed earlier, common research methods for descriptive research include surveys, case studies, observational studies, cross-sectional studies, and longitudinal studies.
- Design your study: Plan the details of your study, including the sampling strategy, data collection methods, and data analysis plan. Determine the sample size and sampling method, decide on the data collection tools (such as questionnaires, interviews, or observations), and outline your data analysis plan.
- Collect data: Collect data from your sample or population using the data collection tools you have chosen. Ensure that you follow ethical guidelines for research and obtain informed consent from participants.
- Analyze data: Use appropriate statistical or qualitative analysis methods to analyze your data. As discussed earlier, common data analysis methods for descriptive research include descriptive statistics, cross-tabulation, content analysis, qualitative coding, visualization, and comparative analysis.
- I nterpret results: Interpret your findings in light of your research question and objectives. Identify patterns, trends, and relationships in the data, and describe the characteristics of your sample or population.
- Draw conclusions and report results: Draw conclusions based on your analysis and interpretation of the data. Report your results in a clear and concise manner, using appropriate tables, graphs, or figures to present your findings. Ensure that your report follows accepted research standards and guidelines.
When to Use Descriptive Research Design
Descriptive research design is used in situations where the researcher wants to describe a population or phenomenon in detail. It is used to gather information about the current status or condition of a group or phenomenon without making any causal inferences. Descriptive research design is useful in the following situations:
- Exploratory research: Descriptive research design is often used in exploratory research to gain an initial understanding of a phenomenon or population.
- Identifying trends: Descriptive research design can be used to identify trends or patterns in a population, such as changes in consumer behavior or attitudes over time.
- Market research: Descriptive research design is commonly used in market research to understand consumer preferences, behavior, and attitudes.
- Health research: Descriptive research design is useful in health research to describe the prevalence and distribution of a disease or health condition in a population.
- Social science research: Descriptive research design is used in social science research to describe social phenomena such as cultural norms, values, and beliefs.
- Educational research: Descriptive research design is used in educational research to describe the performance of students, schools, or educational programs.
Purpose of Descriptive Research Design
The main purpose of descriptive research design is to describe and measure the characteristics of a population or phenomenon in a systematic and objective manner. It involves collecting data that describe the current status or condition of the population or phenomenon of interest, without manipulating or altering any variables.
The purpose of descriptive research design can be summarized as follows:
- To provide an accurate description of a population or phenomenon: Descriptive research design aims to provide a comprehensive and accurate description of a population or phenomenon of interest. This can help researchers to develop a better understanding of the characteristics of the population or phenomenon.
- To identify trends and patterns: Descriptive research design can help researchers to identify trends and patterns in the data, such as changes in behavior or attitudes over time. This can be useful for making predictions and developing strategies.
- To generate hypotheses: Descriptive research design can be used to generate hypotheses or research questions that can be tested in future studies. For example, if a descriptive study finds a correlation between two variables, this could lead to the development of a hypothesis about the causal relationship between the variables.
- To establish a baseline: Descriptive research design can establish a baseline or starting point for future research. This can be useful for comparing data from different time periods or populations.
Characteristics of Descriptive Research Design
Descriptive research design has several key characteristics that distinguish it from other research designs. Some of the main characteristics of descriptive research design are:
- Objective : Descriptive research design is objective in nature, which means that it focuses on collecting factual and accurate data without any personal bias. The researcher aims to report the data objectively without any personal interpretation.
- Non-experimental: Descriptive research design is non-experimental, which means that the researcher does not manipulate any variables. The researcher simply observes and records the behavior or characteristics of the population or phenomenon of interest.
- Quantitative : Descriptive research design is quantitative in nature, which means that it involves collecting numerical data that can be analyzed using statistical techniques. This helps to provide a more precise and accurate description of the population or phenomenon.
- Cross-sectional: Descriptive research design is often cross-sectional, which means that the data is collected at a single point in time. This can be useful for understanding the current state of the population or phenomenon, but it may not provide information about changes over time.
- Large sample size: Descriptive research design typically involves a large sample size, which helps to ensure that the data is representative of the population of interest. A large sample size also helps to increase the reliability and validity of the data.
- Systematic and structured: Descriptive research design involves a systematic and structured approach to data collection, which helps to ensure that the data is accurate and reliable. This involves using standardized procedures for data collection, such as surveys, questionnaires, or observation checklists.
Advantages of Descriptive Research Design
Descriptive research design has several advantages that make it a popular choice for researchers. Some of the main advantages of descriptive research design are:
- Provides an accurate description: Descriptive research design is focused on accurately describing the characteristics of a population or phenomenon. This can help researchers to develop a better understanding of the subject of interest.
- Easy to conduct: Descriptive research design is relatively easy to conduct and requires minimal resources compared to other research designs. It can be conducted quickly and efficiently, and data can be collected through surveys, questionnaires, or observations.
- Useful for generating hypotheses: Descriptive research design can be used to generate hypotheses or research questions that can be tested in future studies. For example, if a descriptive study finds a correlation between two variables, this could lead to the development of a hypothesis about the causal relationship between the variables.
- Large sample size : Descriptive research design typically involves a large sample size, which helps to ensure that the data is representative of the population of interest. A large sample size also helps to increase the reliability and validity of the data.
- Can be used to monitor changes : Descriptive research design can be used to monitor changes over time in a population or phenomenon. This can be useful for identifying trends and patterns, and for making predictions about future behavior or attitudes.
- Can be used in a variety of fields : Descriptive research design can be used in a variety of fields, including social sciences, healthcare, business, and education.
Limitation of Descriptive Research Design
Descriptive research design also has some limitations that researchers should consider before using this design. Some of the main limitations of descriptive research design are:
- Cannot establish cause and effect: Descriptive research design cannot establish cause and effect relationships between variables. It only provides a description of the characteristics of the population or phenomenon of interest.
- Limited generalizability: The results of a descriptive study may not be generalizable to other populations or situations. This is because descriptive research design often involves a specific sample or situation, which may not be representative of the broader population.
- Potential for bias: Descriptive research design can be subject to bias, particularly if the researcher is not objective in their data collection or interpretation. This can lead to inaccurate or incomplete descriptions of the population or phenomenon of interest.
- Limited depth: Descriptive research design may provide a superficial description of the population or phenomenon of interest. It does not delve into the underlying causes or mechanisms behind the observed behavior or characteristics.
- Limited utility for theory development: Descriptive research design may not be useful for developing theories about the relationship between variables. It only provides a description of the variables themselves.
- Relies on self-report data: Descriptive research design often relies on self-report data, such as surveys or questionnaires. This type of data may be subject to biases, such as social desirability bias or recall bias.
About the author
Muhammad Hassan
Researcher, Academic Writer, Web developer
You may also like

Questionnaire – Definition, Types, and Examples

Case Study – Methods, Examples and Guide

Observational Research – Methods and Guide

Quantitative Research – Methods, Types and...

Qualitative Research Methods

Explanatory Research – Types, Methods, Guide
Exploratory, Descriptive, and Causal Research Designs – Compare & Contrast
Design is a vital part of creating any product: websites, apps, home appliances, electronics, and more. Every item requires thorough research. If you are eager to learn the difference between exploratory descriptive and causal research approaches, this article is for you.
In the essay sample below, we will explain the criterion used to distinguish between exploratory, descriptive, and causal research with examples and discuss their role in marketing.
🖊️ Descriptive research design
🔍 exploratory research design, 🔥 causal research design, ⚖️ comparison of the three research designs, 🔗 references list.
Research design is a tool that is used in carrying out marketing researches. The design is supposed to give in detail the procedures that are supposed to be followed to solve problems that marketing researches present. The major approaches used in researches include exploratory, causal, and exploratory research designs.
Information requirements, measures, and scales have to be spelled out clearly. The sampling process, sample size, and data analysis plans also feature prominently as components of the three research designs (Collins, Sutton, and Onwuegbuzie 2006)
Descriptive research design is mainly used to describe a behavior or type of subject. It is not intended to look for specific relationships between variables, nor does it correlate with variables. Its major setback is that it cannot identify the cause because its setting is completely natural.
Moreover, it has all the variables present. Descriptive research design is an asset to a researcher because a lot of information can be acquired through description. For purposes of identification of variables and hypothetical constructs which can be subjected to further investigations using other means, descriptive research design comes in handy. Some of its advantages include the ability of its descriptions to be used to indirectly test theory or model behaviors that cannot be studied using any other means (Creswell 1998).
Categories of descriptive design include surveys and observational studies. Field surveys feature prominently under surveys. Under this, the participants are expected to fill in the questionnaires or be subjected to interviews in natural settings.
Much attention should be devoted to the construction and content validity of the questionnaires; the scores must be reliable; the questions should be clear and precise hence mutually exclusive answers; the interviewer must be consistent and controlled in his or her behavior; and the order of questions, as they appear in the questionnaire, have to be counterbalanced. This helps in identifying and controlling one-sided responses. Finally, field surveyors have to pay attention to concrete behaviors that naïve respondents describe accurately.
Surveys are quite often mailed or facilitated through phone calls. Surveys conducted through mail involve questionnaires that are relatively long. It takes some time before these emails are returned. However, not all of them can be returned, but a 50% return rate is normally okay for the survey.
The inclusion of a cover letter, giving the survey face validity, asking brief questions, guaranteeing rewards, and sending a reminder will only ensure that the return rate of the mail is enhanced. Telephones are credited for having a higher response rate.
Telephone interviewers normally use structured and closely-ended questions (Fraenkel and Wallen 2003). The introduction of telephone interviews has to be straight to the point and friendly. It is usually assumed that participants who fail to participate in phone interviews may be busy or guarded.
When it comes to identifying the population and samples to participate in surveys, all subgroups have to be identified without any element of bias. Random or stratified random sampling techniques are preferred. However, other sapling techniques like cluster sampling, convenience sampling, quota sampling, and snowball sampling can be used.
Potential biases that are encountered in field surveys include:
- By the time the interviewer is calling, the respondent may not be in the mood to talk,
- There may be biasness on the side of those who have accepted to take part in the view (such volunteers may be opinionated or bored),
- There may be the silent majority,
- The way one feels today may not be the same as the way he will feel tomorrow.
Survey results are normally analyzed using descriptive statistics. This helps in checking significant correlations in responses obtained from different questions.
In observational studies, descriptions normally rely on observations made on the subjects. The general techniques used here are naturalistic observation, systematic naturalistic observation, and participant observation. Naturalistic observation is unstructured and unsystematic.
It entails observation of a wide range of behaviors. Systematic naturalistic observation is systematic and looks into specific behaviors. Participant observation requires that the investigator infiltrate the group he or she is observing. The observer acts like a spy agent whose true identity and purpose cannot be known (Creswell 2005).
The major advantage of observation studies is that it is absolutely natural. It does not, therefore, demand characteristics. Its disadvantages are the unavailability of informed consent. It relies on convenience samples, and the data used is mostly qualitative, as there are much of verbal descriptions and observations made visually.
Observation studies are supposed to help in identifying variables that are valuable in indirect tests of models and predictions. In order to decrease bias in observation studies, well-defined scoring criteria have to be developed, a time sampling technique must be used, there have to be multiple observers, double-blind procedures have to be used, and for the purpose of increasing external validity, it is imperative that random samples are obtained.
Exploratory research design is normally used in research whose purpose involves inquiry into new products that should be developed, how the product appeal will enhance its advertising, and how the existing services can be improved.
Research questions in exploratory research design border on the alternative ways that exist that can be used, for example, to provide lunch for school children, the kind of benefits people stand to get from the product, and the nature of dissatisfaction the customer may be getting from the product in the case of marketing research. The hypothesis here has unknown constructs. The hypothesis also suspects that the major problem in the study could be impersonalization.
The purpose of this research design from a marketing research perspective is to ascertain whether an increase in the service staff can be profitable, which advertising program for public transit is suitable, and whether a new budget should be contemplated. The research questions here touch on the correlation between the size of staff offering services and the revenue that is accrued. What do people stand to get from cars and public transport?
The major objective of exploratory research design is to discover ideas and insights, whereas descriptive research design involves describing market aspects and functions. Nevertheless, causal research design tries to determine the cause-effect relationships in the research one is conducting.
A major feature of exploratory design is its flexibility, its adaptability, and the front end of total research design. However, descriptive research design is marked by past formulation of specific hypotheses from observations and studies. This design is pre-planned and structured. A causal research approach can proceed through one or more independent variables. Other mediating variables that fall under this design can also be controlled.
An exploratory research approach entails the use of surveys, case studies, information from other studies, and qualitative analyses. In contrast, a descriptive research approach uses information from other studies, panels, analyses, and observations. Causal research design strictly uses experiments.
Collins, K. M. T., Onwuegbuzie, A. J., & Sutton, I. L., 2006, A model incorporating the rationale and purpose for conducting mixed methods research in special education and beyond, Learning Disabilities: A Contemporary Journal, vol.4, pp. 67-100.
Creswell, J. W., 1998, Qualitative inquiry and research design: Choosing among the five traditions. Thousand Oaks, CA: Sage.
Creswell, J. W., 2005, Educational research: Planning, conducting, and evaluating quantitative and qualitative research (2nd ed.). Upper Saddle River, NJ: Pearson Education.
Fraenkel, J. R., & Wallen, N. E., 2003, How to design and evaluate research in education (5th ed.). Boston: McGraw-Hill.
Is causal research and explanatory research the same? Despite causal research can sometimes be referred to as explanatory research, there is a difference. Causal research is an evaluation of whether two different aspects have cause-and-effect relationships. The explanatory research goal is to determine the exact nature of the issue to be solved.
What is exploratory descriptive and causal research? An exploratory research method uses case studies, surveys, qualitative analyses, and information from other studies. A descriptive research approach entails using information from other studies, analyses, panels, and observations. In contrast, causal research design uses experiments.
What are the 3 types of research? Most research approaches can be divided into 3 types: descriptive, causal, and exploratory. Depending on the end purpose, a researcher should use the exact methods and instruments.
- Chicago (A-D)
- Chicago (N-B)
IvyPanda. (2023, October 28). Exploratory, Descriptive, and Causal Research Designs - Compare & Contrast. https://ivypanda.com/essays/comparison-and-contrast-of-exploratory-descriptive-and-causal-research-designs-giving-examples/
"Exploratory, Descriptive, and Causal Research Designs - Compare & Contrast." IvyPanda , 28 Oct. 2023, ivypanda.com/essays/comparison-and-contrast-of-exploratory-descriptive-and-causal-research-designs-giving-examples/.
IvyPanda . (2023) 'Exploratory, Descriptive, and Causal Research Designs - Compare & Contrast'. 28 October.
IvyPanda . 2023. "Exploratory, Descriptive, and Causal Research Designs - Compare & Contrast." October 28, 2023. https://ivypanda.com/essays/comparison-and-contrast-of-exploratory-descriptive-and-causal-research-designs-giving-examples/.
1. IvyPanda . "Exploratory, Descriptive, and Causal Research Designs - Compare & Contrast." October 28, 2023. https://ivypanda.com/essays/comparison-and-contrast-of-exploratory-descriptive-and-causal-research-designs-giving-examples/.
Bibliography
IvyPanda . "Exploratory, Descriptive, and Causal Research Designs - Compare & Contrast." October 28, 2023. https://ivypanda.com/essays/comparison-and-contrast-of-exploratory-descriptive-and-causal-research-designs-giving-examples/.
- Exploratory Research in Organizational Leadership
- Differences Between Conceptual Model and Explanatory Model
- Correlational Research: Explanatory and Predictive Designs
- Visual Display of Data: Exploratory Data Analysis
- Methods of Conducting Exploratory Marketing Research
- Naturalistic Art Versus Abstract Art
- Research Methods by Alwabel, Zairi, and Ahmed
- Principal Components and Exploratory Factor Analysis
- Qualitative and Quantitative Research Principles Comparison
- Non- vs. Experimental Designs in Different Studies
- Analysis of Variance: Limitations and Assumptions
- Assumptions when Using a Chi-Square Test
- Prenatal and Post Natal Motherhood
- Sampling and Sampling Distributions
- Sampling Techniques in Education
Applied Causal Analysis (with R)
3.1 descriptive vs. causal questions, 3.1.1 descriptive questions.
- e.g. How are observations distributed across trust categories in Table 3.1 ?
- How are observations distributed across trust and gender values?
- How are observations distributed across values of trust ( Y ), gender ( X 1 ) and time ( X 2 )?
- Are trust values higher (on average) among females than males?
As the name suggests descriptive research questions are about describing the data. For instance, we could measure trust within the German population using the question ‘ Would you say that most people can be trusted or that you can’t be too careful in dealing with people, if 0 means “Can’t be too careful” and 10 means “Most people can be trusted”? ’ Consequently, a descriptive question would be to ask are there more individuals with a high level of trust (define as those with a value above 8) or more with a low level of trust (defined as those with a value below 2) . In other words, descriptive questions are concerned with the distribution of observations (e.g. individuals) across values of a variable (or several variables), e.g., the variable trust (Y). Importantly, descriptive questions may involve as many variables as you like. We could add a second variable, gender ( X 1 , male vs. female), and ask whether females have a higher level of trust - on average - than males. This already points to how we deal with the underlying distibutions. Normally, we summarize them using statistics such as the mean (or other statistics). And we can also develop hypotheses for our descriptive questions, e.g., we could hypothesize that females have a higher level of trust than males and subsequently test this hypotheses using we collect. Potentially, it makes sense to call hypotheses that simply concern the distribution of data across one more dimensions descriptive hypotheses . Importantly, time (which will become important later on) is just another variable and a corresponding descriptive question would be: was trust in politicians higher in January 2019 than in January 2020?
3.1.2 Causal questions
- Is there a causal link between the distribution across values of Y and values of D ?
- Continuous variables: Compare means
- Categorical variables (several): Compare probabilites for categories
- Group level: Does victimization cause individuals to have a lower level of trust on average (then if they were not victimized)?
- Individual level: Does (non-)victimization cause individual i to have a (lower)higher trust level?
Causal research questions are of a different kind. From a distributional perspective we could ask whether the distribution of a first variable D is somehow causally related to the distribution of a second variable Y . Again we tend to summarize the corresponding distributions, e.g., we could take the mean of trust. In Table 3.2 we tabulate victimization (D), measured with the question Have you been insulted or threatened verbally since (month, year)? against trust (Y). Take note that the vicimization variable D is dichotomous (0,1) whereas the outcome variable Y has 11 values (0-10). The corresponding causal question would be: Does victimization cause individuals to have lower levels of trust (on average that is comparing the means)? Ultimately, this question resides on the group level but is strongly related to the corresponding question on the individual level: Does (non-)victimization cause individual i to have a (lower)higher trust level? One important aspect that we will encounter later on: In asking our causal question we may focus on certain subsets in our sample once we have collected some data. For instance, we could ask whether the people that have actually been victimized would have had a higher level of trust if they had not been victimized. This question focuses on the subest in our sample that has been victimized.
See Gerring ( 2012 ) for a discussion of “What?” and “Why?” questions. ↩

Exploratory, Descriptive & Causal Research: Why Are They Important
Every piece of research should have a purpose, and it’s important to understand the various types of research that can be used to get the most out of your project.
In this article, we’ll look at Exploratory , Descriptive , and Causal Research and why they are important for gathering the data needed to make informed decisions or discover insights.
Introduction
Overview of exploratory, descriptive & causal research, benefits of exploratory research, benefits of descriptive research, benefits of causal research, which part of research is the most important, what are the 3 main purposes of research, why is exploratory research so important, why is descriptive research so important, why is causal research so important, what are some ethical considerations that should be taken into account when conducting research.
Exploratory , descriptive and causal research are all important methods for uncovering information and understanding relationships.
Each has its own strengths and weaknesses, so it’s important to choose the right method for your particular research question.
Exploratory research is used when researchers are looking for insights into a problem or issue without having a specific hypothesis in mind. It allows the researcher to explore different angles and see what emerges.
Descriptive research focuses on understanding the characteristics of a specific population or phenomenon. The goal is to gain an accurate and complete picture of the subject.
Causal research is used when researchers want to understand how two variables interact with each other and how changes in one variable cause changes in another.
These three types of research have their own unique advantages and disadvantages, but they all play an important role in helping us answer questions about our world.
All research can be classified as either basic or applied. Basic research is also known as pure research because its main goal is to increase scientific knowledge. Applied research, on the other hand, is geared towards solving real-world problems.
There are three main types of research: exploratory , descriptive , and causal . Each has a different purpose and uses different methods.
Exploratory research is used to gain a better understanding of a topic by exploring it in detail. It is typically conducted before any other type of research, such as descriptive or causal research. It is often used to generate ideas and formulate hypotheses. This type of research can take many forms, such as interviews, surveys, focus groups, and observation .
Descriptive research is used to describe a phenomenon in detail. It involves collecting and analyzing data about the population of interest. Descriptive research can include surveys, interviews, case studies, and experiments . The goal of this type of research is to gain an understanding of how something works or what factors are associated with a particular outcome.
Causal research seeks to identify cause-and-effect relationships between two or more variables. This type of research usually involves experiments where different conditions are tested in order to determine which causes the observed outcomes . Causal research allows researchers to make predictions about how changes in one variable will affect another variable.
There are many benefits of exploratory research , which can be broadly categorized into understanding a problem or phenomena better, developing new hypotheses or ideas for future research, and refining existing theories or models.
In terms of understanding a problem or phenomena better
- Exploratory research can help uncover new insights and perspectives that may have been hidden or not obvious before.
- It can also help to generate new ideas or hypotheses about how something works or why something happens, which can then be tested in future research.
- Exploratory research can also be used to refine existing theories or models by exploring new areas that have not been studied before or by testing existing assumptions in new ways.
In terms of developing new hypotheses or ideas for future research
- Exploratory research is often the first step in the scientific process. By generating new ideas and perspectives, it provides a starting point for further investigation.
- Exploratory research allows for gathering information without making any assumptions about what the outcome may be, providing researchers with invaluable insights into their subject matter.
- As exploratory research is typically less formal and more flexible than other types of research, it allows researchers to explore creative and novel approaches to solving problems.
Finally, in terms of refining existing theories or models
- Exploratory research can provide important insights that may challenge existing assumptions. By testing these assumptions in new ways or by exploring new areas that have not been studied before, researchers can develop a deeper and more comprehensive understanding of a theory or model.
- Additionally, it also help identify gaps in current knowledge so that future research can be focused on filling these gaps.
Descriptive research is a type of research that is used to describe a population or phenomenon. This type of research is often used in the social sciences and can be used to describe things like behavior, attitudes, or beliefs. Descriptive research can be used to answer questions about who, what, where, when, and how.
- One of the benefits of descriptive research is that it can provide detailed information about a population or phenomenon. This type of research can be used to generate hypotheses or to test theories.
- It can also be used to compare different groups of people, helping researchers gain insights which would otherwise not be possible.
- Descriptive research can be used to understand complex phenomena.
- Descriptive research can be used to study rare events.
- Descriptive research can be used to inform public policy.
There are many benefits to conducting causal research .
- Causal research can help identify the causes of things, and can also help determine how to prevent them from happening.
- It can be used to develop new treatments for diseases or disorders.
- It can also be utilized to inform policy decisions, and can provide new insights into how social systems work.
- Causal research is often used to identify the most effective solutions for a problem. By understanding the causes behind an issue, researchers can create solutions that are more likely to deliver lasting results.
Each types of research has its own importance.
Exploratory research is important because it allows marketers to get a better understanding of the problem or issue they are trying to solve.
Descriptive research is important because it allows marketers to understand the target audience better and identify any trends that may be occurring.
Causal research is important because it allows marketers to understand the cause of the certain increase or decrease in demand and what effect can be in market if certain variables are changed.
Hence, each has its own importance depending what research objectives and goals, marketers are trying to achieve.
Exploratory , descriptive and causal research are three integral methods of conducting research.
Each form provides unique insights that can be used to improve decision-making processes and strategies for a variety of purposes.
When applied correctly, these forms of research provide valuable information about people, businesses or organizations that can be used to make better decisions and increase efficiency.
Ultimately, the importance of exploratory, descriptive and causal research cannot be understated as it is vital in helping us understand the world around us.
1. To gain knowledge and understanding of a given subject or topic. 2. To generate new ideas and insights into existing topics and issues. 3. To develop theories, models, and methods for solving problems or improving decision making processes.
Exploratory research is important as it allows researchers to gain a better understanding of the problem they are trying to solve or explore. It is also useful for discovering new topics and ideas which may not have been considered previously.
Descriptive research is important as it allows researchers to collect data and analyze it in order to describe the characteristics of a population or phenomena.
Causal research is important as it allows researchers to determine the cause and effect relationships between variables. This helps them to understand how different factors influence each other and can help in developing strategies for improving decision making processes or solving problems.
Some ethical considerations to take into account when conducting research include: obtaining informed consent from participants, protecting the privacy and confidentiality, avoiding potential harm to participants, and ensuring accuracy and validity in data collection and analysis.
Related Posts

Understanding ANOVA: What It Is, How To Use It, and What It Does?
- February 17, 2023

Value Analysis: Understanding Its Benefits and Why It Matters
- February 16, 2023

A Guide To Various Forms Of Transportation In India And Their Advantages
- February 14, 2023
Leave a Reply Cancel Reply
Your email address will not be published. Required fields are marked *
Name *
Email *
Add Comment *
Save my name, email, and website in this browser for the next time I comment.
I accept the Privacy Policy
Post Comment
Descriptive, Predictive, and Causal Research
A few years ago, I wrote an open letter to the NASSM community. Here is what I wrote:
Dear Sport Management Colleagues,
In this email, I would defend the value of descriptive studies to sport management research, and differentiate explanatory and predictive research.
I would argue even purely descriptive research can be very much needed and useful. It becomes a cliché that good descriptive research provokes why questions and good descriptive research builds the foundation for further causal studies. However, because the favors given to causal studies and theory testing, we often take on a causal study too hastily (I consider SEM as a causal technique). Without a solid description of phenomenon, causal studies, even informed by strong theory, can be on shaky ground. All theories can be wrong, and wrong theories can be supported by the means of poor science (such as problematic data generation process, highly flexible modelling methodology, p-hiking, and over-fitting). For instance, all theories can be potentially supported with SEM when there exist common method variances.
Instead, I would argue sport management field is in great need of good descriptive studies for further growth. I recently ask a question relating the two fundamental pillars of sport management - spectatorship and participation. What is the relationship between them? Whereas many studies examined the causal effect of spectatorship on participation, the covariation of these two behaviors are not fully established. And my work is readily rejected based on the theory that descriptive research has no value. In research methodology class, we teach students that the three conditions of establishing causality are temporal order, covariation of the cause and the consequence, and no alternative explanations. Sadly, many presumably causal studies simply ignore those conditions.
Explanatory research and predictive research are different. Predictive research is relational in nature. Theory is not a necessary condition for prediction. More often than not, prediction does not need theory. My four-year old can predict rain is coming when he sees dark clouds; my two-year old can predict train is coming when he hears whistle; the size of a person's right foot can be well predicted by the size of her left foot; the Pavlovian dog would predict food is coming when the bell rings. Accurate prediction can be made without taking recourse to a theory. Prediction only requires the learning of covariation. Prediction does not require an understanding of what cause the covariation. Yet, prediction is not of less value because it does not have a theoretical framework. Rather, prediction is of central interest to many sports management practitioners. Team managers want to predict the potential of a player based on her past performance; merchandise managers want to predict what products would be in vogue; sport entrepreneurs want to predict when their new products would take off; racing pundits and tipsters want to predict the winner of a race. Yet all those scholars could care less.
Will theory facilitate accurate prediction? First, good theory facilitates the learning of covariation as theory can direct observations to the relevant variables; second, theory allows reliable (defined as consistent, not necessarily accurate) prediction to minimize the impact of haphazard or wishful thinking. Third, a good theory is inherently very powerful in prediction.
Whereas, prediction is an attribute of a good theory, not all theories are predicative. Theories involving all unobservable are poor in prediction. Sports identification is not a good predictor of sport consumption because identification is unobservable. And if identification is measured based on consumption, then it becomes tautological to claim that identification predicts consumption. A theory that can explain everything is poor in prediction. A good theory must have its boundary conditions to allow accurate predictions. In theory testing studies, unfortunately, those boundary conditions often got neglected, and a theory was proven correct when it was not supposed to be correct. A great example of such is the theory of reasoned action. This theory is only supposed to explain a certain type of behaviors, namely reasoned action. However, we found that this theory has been used to predict all different kinds of behaviors, reasoned or not.
Theoretical testing becomes a trivial scholarly endeavor because of the ignorance of the boundary conditions, compounded with extremely powerful statistical methods. Alas, now all is good, all theories are supported! It is a pity, based on my own experience, that the reviewers often favor powerful statistical methods. My own work has often been criticized for using conservative statistical techniques. I would sometime use multivariate regression instead of SEM; I would use a simple correlation analysis instead of other more complicated zero-inflated models. If a study can find a significant relationship by using a more conservative method, the relationship must be detected by a more powerful technique.
In summary, I argued:
Descriptive study is very useful and needed to sport management field;
Predictive study is relational in nature which does not require theory;
Good theory can help accurate prediction;
Theory testing is trivial when boundary conditions are neglected and extremely powerful techniques are used.
Conservative technique should not be disregarded based on the belief/perception that they are too simple to be scientific.
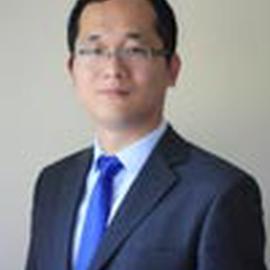
Luke L. Mao
Associate professor of sport administration.
- A Vosviewer Analysis of the Three Leading Sport Management Journals

An official website of the United States government
The .gov means it’s official. Federal government websites often end in .gov or .mil. Before sharing sensitive information, make sure you’re on a federal government site.
The site is secure. The https:// ensures that you are connecting to the official website and that any information you provide is encrypted and transmitted securely.
- Publications
- Account settings
Preview improvements coming to the PMC website in October 2024. Learn More or Try it out now .
- Advanced Search
- Journal List

Prediction or causality? A scoping review of their conflation within current observational research
Chava l. ramspek.
1 Department of Clinical Epidemiology, Leiden University Medical Center, Leiden, The Netherlands
Ewout W. Steyerberg
2 Department of Biomedical Data Sciences, Leiden University Medical Center, Leiden, The Netherlands
Richard D. Riley
3 Centre for Prognosis Research, School of Medicine, Keele University, Keele, UK
Frits R. Rosendaal
Olaf m. dekkers, friedo w. dekker, merel van diepen, associated data.
Data will be made available upon reasonable request to the corresponding author (C. L. Ramspek).
Not applicable.
Etiological research aims to uncover causal effects, whilst prediction research aims to forecast an outcome with the best accuracy. Causal and prediction research usually require different methods, and yet their findings may get conflated when reported and interpreted. The aim of the current study is to quantify the frequency of conflation between etiological and prediction research, to discuss common underlying mistakes and provide recommendations on how to avoid these. Observational cohort studies published in January 2018 in the top-ranked journals of six distinct medical fields (Cardiology, Clinical Epidemiology, Clinical Neurology, General and Internal Medicine, Nephrology and Surgery) were included for the current scoping review. Data on conflation was extracted through signaling questions. In total, 180 studies were included. Overall, 26% (n = 46) contained conflation between etiology and prediction. The frequency of conflation varied across medical field and journal impact factor. From the causal studies 22% was conflated, mainly due to the selection of covariates based on their ability to predict without taking the causal structure into account. Within prediction studies 38% was conflated, the most frequent reason was a causal interpretation of covariates included in a prediction model. Conflation of etiology and prediction is a common methodological error in observational medical research and more frequent in prediction studies. As this may lead to biased estimations and erroneous conclusions, researchers must be careful when designing, interpreting and disseminating their research to ensure this conflation is avoided.
Supplementary Information
The online version contains supplementary material available at 10.1007/s10654-021-00794-w.
Introduction
From an epidemiological perspective, clinical studies are often classified as having either a descriptive, etiological or predictive aim. In the current study we focus on etiology and prediction research. In etiological research the typical aim is to uncover the causal effect of a specific exposure (factor) on an outcome. The results generally help us answer ‘what if’ questions about treatment or management and are imperative in furthering our understanding of the mechanisms of disease. The gold standard to do so is traditionally a randomized experiment. However, this is often not feasible and advancements have been made towards undertaking causal inference from observational data [ 1 ]. A crucial part of observational causal studies is correction for confounding (variables which influence both the exposure and outcome and muddle the causal relationship). The data itself cannot tell us which variables give confounding, knowledge and assumptions on the underlying causal structure is necessary.
In prediction research, the goal is a model that utilizes multiple factors (‘predictors’) in combination to accurately predict an outcome in individuals to assist in diagnosis or prognosis. This is usually irrespective of whether included predictors are causal or not. The main focus of prediction model studies is the overall predictive or diagnostic performance of the model which should also be assessed in new patients (validation) [ 2 ]. A prior step to model development may be to identify novel predictors that have added predictive value when added to known predictors [ 3 ]. For a more in depth explanation on etiology and prediction research see the supplemental material. In the case of counterfactual prediction modelling (which answers ‘what if’ questions on prognosis related to interventions) prediction and etiology intentionally collide [ 4 , 5 ]. Though an important advancement, such studies are rare, require specialist techniques and are not the focus of this article [ 6 ].
Both etiological and prediction studies may be performed on the same observational data, but the underlying research question, methods and interpretation of results are usually different [ 7 ]. Unfortunately, when reading and reviewing the medical literature, we have found that these aims, methods, results and interpretations are often confused, leaving us with studies that no longer answer a clear etiological or prediction question and may be misinterpreted. In consultations with researchers, we’ve noticed that the distinction between prediction and etiology is often not clearly stipulated and therefore not considered in the research question or proposed statistical approach. The century-old decree that causal inference cannot be made from observational data has resulted in many causal observational studies using vague terminology and an apprehension to tackle causal questions explicitly [ 8 ]. The conflation between causal research and prediction research is also common within statistics and data science [ 9 , 10 ]. Although conflation of etiology and prediction appears anecdotally to be frequent in medical research, the extent of this is unknown. If the frequency and consequences of this conflation are better understood, this may promote change and awareness in medical research practice and scientific education.
Therefore, our research aim is to quantify the frequency of conflation between etiological and prediction goals, analysis approach and interpretation in observational studies from various medical research fields. Furthermore, we aim to discuss common mistakes underlying this conflation, elaborate on the hazards that lie in these mistakes, and provide recommendations on how to recognize and avoid them.
Data sources and study selection
In order to include a wide range of clinical observational studies, journals from the following six medical fields were included: Cardiology, Clinical Epidemiology, Clinical Neurology, General and Internal Medicine, Nephrology and Surgery. The top-ranked journals from these medical fields, according to the 2018 Clarivate Journal Citation Reports (JCR), were screened. To include Clinical Epidemiology journals we used the JCR category ‘Public, environmental & occupational health’ and for Nephrology the JCR category ‘Urology & Nephrology’, for the other medical fields the identically named JCR categories were referenced. Eligible studies were identified by examining the table of content from the January 2018 issue(s), starting with the journal with the highest impact factor per medical field. A total of 30 studies were included per medical field, and journals from each field were added until this number was reached.
The titles, abstracts and full-text studies in the original article section of the various journals were screened by CLR. Original clinical observational studies (etiological, prognostic and diagnostic) conducted on humans were included. We excluded the following types of research: trials, intervention studies, experiments, descriptive studies, fundamental research, genome wide association studies, methodological studies, systematic reviews, meta-analyses, qualitative studies, case-series, impact assessment studies, simulation studies, counterfactual prediction and cost-effectiveness studies.
Data extraction
General study characteristics were extracted and each study was classified as being conflated or not, using developed signaling questions (see below). Data-extraction of included studies was performed by CLR. When there was uncertainty on how to score a study, MvD assessed this study independently and the study was discussed by CLR and MvD, if necessary a third assessor was consulted (FWD).
Assessing conflation of etiology and prediction
To identify conflation, a list of unique study characteristics for both etiology and prediction was developed by CLR, MvD and FWD in an iterative fashion. Conflated studies from personal libraries were discussed and classified into themes and domains of conflation. Previous work on this topic was used to help identify key etiological and prediction characteristics and the STROBE (Strengthening the Reporting of Observational studies in Epidemiology) and TRIPOD (Transparent Reporting of a Multivariable Prediction Model for Individual Prognosis or Diagnosis) guidelines were consulted [ 7 , 11 – 13 ]. The developed list of characteristics of etiological and prediction research is shown in Box Box1. 1 . This list is not exhaustive, but contains key characteristics that belong to either etiological or prediction research (but not to both), are unrelated to the research topic and are relatively easy to assess.
: Etiology versus prediction key characteristics
Based on the key characteristics, signaling questions were developed to help identify conflation in various domains. The final signaling questions are shown in supplemental table S1. They were designed so that if any question in both the etiology and prediction column is answered by ‘yes’, this flags the potential for conflation. A single study might have both an etiological and prediction aim and therefore contain characteristics from both study types, and, if correctly performed, such studies were classified as containing both etiology and prediction without conflation.
The use of data-driven methods for confounder selection in etiological studies was considered conflation, unless stated that these covariates were pre-selected based on the causal structure (so as to only include potential confounders). If a study reported adjustment for a list of variables (without further clarification on what these variables were or how they were selected), the statistical approach was labelled as unclear; it didn’t contribute to the classification of etiology or prediction. Causal interpretation of predictors from a prognostic model (in which causal structure was not considered) was deemed conflation. Finally, the reporting of hazard ratios or odds ratios in the results section of a prediction model study was not considered conflation, unless stated that these were effect estimates in which bias was minimized by correcting for confounders or by accounting for confounding through the study design.
Statistical analysis
Descriptive statistics were used to summarize findings. Depending on their distribution, continuous characteristics are presented as mean with standard deviation (SD) or median with interquartile range (IQR). Dichotomous and categorical variables are summarized by reporting proportions. When appropriate a standard error (SE) or 95% confidence interval (CI) was added. The association between journal characteristics and conflation was quantified in univariate binomial regression analysis and presented as an odds ratio (OR) with CI. Statistical analyses and graphs were computed in R version 3.6.1.
Included studies
A total of 421 studies were screened for inclusion based on their title, abstract or full-text. Finally, 180 observational studies in humans were included for the current review; 30 studies were included from each of the 6 considered medical fields (Cardiology, Clinical Epidemiology, Clinical Neurology, General & Internal Medicine, Nephrology and Surgery). See supplemental figure S1 and table S2 for a flowchart of the study selection and list of journals from which studies were included. Each included study was classified as etiology, prediction or both. Subsequently using the signaling questions, it was determined whether each study contained conflation between etiology and prediction. The second assessor was consulted on 15 studies. In Table Table1, 1 , quotes from three included studies that were conflated are shown as an example of study assessment. These quotes exemplify how conflation may arise in observational studies. Table S3 shows the classification for each included study.
Three examples of observational studies included in this review are shown
Each of these studies contains conflation and quotes from each domain of the article are given that exemplify this. The table should be read vertically
Some quotes are paraphrased slightly to shorten them and abbreviations are written full-out
Frequency of conflation and study characteristics
Out of 180 studies, 46 were classed as conflated (26%, 95% CI 19–33%). In total, 127 studies (71%) were classified as etiological, 47 (26%) as prediction and 6 (4%) as both prediction and etiology. From the etiological studies 28 (22%) contained conflation and from the prediction studies 18 (38%) contained conflation. In Fig. 1 the classification of studies is shown per medical field, the proportion of conflation ranges from 0 to 40%. In our sample from General & Internal Medicine journals there was no article with conflation between etiology and prediction (30 in total), Clinical Epidemiology journals showed the second-least amount of conflation with 4 conflated articles (13%). In Fig. 2 each included journal is plotted according to its impact factor and proportion of conflated articles. In univariate regression impact factor and conflation were significantly associated; with every point increase in impact factor the odds of conflation decreased (OR 0.95, 95% CI 0.90–1.00).

Number of studies included by medical field and assessment; etiological, prediction, both (dual) or conflated

conflation proportion by journal impact factor and medical field. Each bubble represents 1 included journal, the size of the bubble corresponds to the number of articles included from this journal. Spearman’s correlation coefficient between conflation and impact factor is − 0.13 ( p value 0.08)
General characteristics of the included studies are shown in Table Table2. 2 . An epidemiology department was less frequently listed in the author affiliations of conflated articles (22% vs. 43%; OR 0.36, 95% CI 0.17–0.79). In total only 11 studies reported adherence to a reporting guideline and this reporting was less frequent in conflated articles (2% vs. 7%), odds ratio 0.28 (95% CI 0.03–2.21). The referenced reporting guidelines were STROBE (n = 8), RECORD (n = 2), STARD (n = 1) and PRISMA (n = 1).
Characteristics of included studies
Types of conflation identified
In Fig. 3 , different types of conflation are shown. We identified six main forms of conflation, of which multiple may be present in the same study. The most frequent form of conflation was the inclusion of covariates based on their ability to predict the outcome without taking the causal structure into account, in an otherwise etiological study (type A). This was observed in 25 out of 127 etiological studies (20%) and always entailed data-driven selection of confounders, based either on univariate associations or stepwise selection procedures. Another frequent mistake (n = 8) in causal research, was the presentation of predictive performance results, such as an AUC or calibration, for the adjusted model (type B). Additionally, two etiological studies proposed risk group stratification based on their multivariable causal model (type C).

Types of conflation. Type A = 25 studies, type B = 8 studies, type C = 2 studies, type D = 14 studies, type E = 5 studies, type F = 3 studies
In studies that were predictive in aim, the most frequent mistake was a causal interpretation of identified predictors and their estimate of effect (type D). This occurred in 14 studies out of 47 prediction studies (30%). These studies often described which predictors were modifiable and then suggested changing these predictors to improve prognosis. Furthermore, residual confounding was frequently (n = 5, 11%) recognized as limitation in prediction research (type E). Finally, three prediction studies selected predictors by restricting to causal factors or confounders, without having a counterfactual prediction goal.
In this scoping review which sampled observational studies from top journals in 6 medical fields, we found that conflation between etiology and prediction occurred in 46 out of 180 included studies (26%) and was more common in prediction studies. The frequency of conflation varied per medical field and journal impact factor, with less conflation in general & internal medicine and epidemiology journals. In causal research, the most frequent type of conflation was selecting adjustment covariates based on their ability to predict the outcome, rather than based on causal reasoning or pathways. In prediction studies, the most frequent form of conflation was a causal interpretation of predictor effects, instead of referring to their added value for risk prediction or overall model performance.
The identified conflation between etiology and prediction could easily lead to incorrect estimations and erroneous conclusions. If confounders are not accounted for in etiological research, effect estimates are most likely incorrect [ 17 ]. Specifically, in medicine it is important to determine causal factors correctly, as they might go on to be the target of novel pharmacological studies, be incentive for randomized controlled trials, or be incentive to change clinical patient care. If incorrect causal claims are made based on prediction models, this could have similarly detrimental effects on patients and further research efforts [ 9 ]. In our scoping review, 30% of prediction studies interpreted included predictors causally for instance by suggesting modification of a predictor to improve a patient’s prognosis. This is a dangerous misinterpretation; since predictors in no way need to be causally associated with the outcome, these studies cannot conclude that an individual’s prognosis would change if these predictors were to be modified. Imagine a prediction model of mortality, in which medication use is a predictor associated with a higher risk of death. Though medication use is modifiable, this does not mean that individuals should discontinue their medication in order to live a longer life. Though this example is rather obvious, similar mistakes frequently occur. For instance, an included study presenting a dementia risk score, concludes that a high BMI is protective. These conclusions may mislead readers into thinking obesity has health benefits [ 16 ]. Other identified conflation types, such as the presentation of performance measures for causal models or the recognition of residual confounding as limitation in prediction studies, probably have fewer directly harmful effects but constitute poor research methodology. Performance measures have a very limited role in etiological research and the concept of confounders or residual confounding is not appropriate in prediction studies that are not designed or aimed at uncovering a causal effect. We believe reducing conflation between etiology and prediction will improve the quality of observational research and lead to better and more efficient science.
Many excellent papers and books have been published on how to conduct both etiological and prediction research on observational data [ 18 – 23 ]. If these guidelines are followed precisely, conflation between etiology and prediction is unlikely. However, few studies explicitly tackle the differences between etiology and prediction. A study from Zalpuri et al. from 2012 surveyed 435 attendees of an international transfusion conference on the interpretation of a multivariable stepwise logistic regression model. In total, 40% of attendees thought that a stepwise model was a valid method to adjust for confounding and 60% of attendees agreed with a causal interpretation of a stepwise prediction model [ 24 ]. An important paper by Shmueli does discuss explaining versus prediction from a statistical viewpoint and concluded that the statistical literature lacks a thorough discussion of differences between prediction and causality [ 10 ]. A recent paper by Hernan et al. entitled ‘a second chance to get causal inference right’ contains a plea to data scientists to integrate causal and prediction research questions (and their differences) in their curricula and analysis framework [ 9 ]. In previous work we also addressed differences between prediction and etiology and discussed related common pitfalls that arise [ 7 ]. The current study has empirically confirmed these pitfalls, quantified how frequent they are, and identified additional types of conflation.
There are various factors that may contribute to conflation between etiology and prediction. We have formulated some general recommendations for researchers, research-institutes, journals and policy-makers (Table (Table3) 3 ) to ensure a clear distinction. For researchers, one of the most important recommendations is to clearly define the research question (including whether it is causal, predictive or descriptive). If the research question is unclear this ambiguity frequently continues throughout the rest of the article [ 25 ]. Once the aim is clear we suggest consulting appropriate reporting and methodological guidelines as well as methodological experts. For observational causal studies we recommend the STROBE guideline and the accompanying explanation & elaboration paper [ 18 ]. For diagnostic and prognostic prediction studies TRIPOD is recommended [ 19 , 20 ]. Terms such as ‘predictor’ are frequently used in both causal and predictive studies. Though this in itself does not constitute conflation, it may cause uncertainty on the aim of the study. We would suggest reserving the terms ‘risk factor’, ‘causal factor’, ‘exposure’ and ‘confounder’ for causal research and the terms ‘predictor’, ‘prognostic factor’, and ‘prediction’ for prediction studies. The word causation is often avoided in observational studies, and sometimes even forbidden by journals [ 8 ]. Though no study can definitively prove causation, lack of clarity regarding the research goals has negative effects on the quality of observational research [ 8 ]. Finally, it would be beneficial for journals to include methodological experts in the peer review and editorial process. Our signaling questions can be used by reviewers or in systematic reviews to quantify the risk of conflation between etiology and prediction in observational studies.
Recommendations on how to avoid conflation
Machine learning techniques are methodological advancements that have large potential in prediction research to analyze complex data structures [ 26 ]. However, the lack of transparency and black box nature of these methods complicates independent validation, updating and implementation. Therefore, it is imperative that such studies adhere to the same methodological guidelines as regression based prediction studies [ 26 ]. The more recent proposed use of machine learning in causal inference is not straightforward and can easily introduce conflation. Similar to data-driven selection methods, machine learning algorithms cannot distinguish mediators from confounders or recognize bias; the researchers’ knowledge and input on the causal structure remains crucial [ 27 ].
Though we have argued that variables in a prediction model should not be interpreted causally, many prediction models will benefit from including (previously identified) causal factors as predictors. This may help improve transportability of the model to new settings or different populations and can improve credibility and uptake of the model. Some might even go so far as to say that the ultimate prediction model—though unattainable—would contain all and only causal pathways of a condition [ 28 ]. On a less philosophical note, there are research questions for which prediction and etiological methods should be combined. The rather new approach termed counterfactual prediction per definition intertwines prediction and causation. Such studies aim to predict an individual’s prognosis for multiple scenario’s, while only one of these scenario’s is observed in the data (per individual). In particular, development datasets will typically observe outcomes in the context of current care, which includes current treatment and monitoring procedures. Thus, counterfactual prediction is then needed to make risk predictions in the hypothetical scenario where individuals receive a different treatment or care. This allows the comparison of multiple predicted risks for various treatment pathways within one individual.[ 4 – 6 , 29 ] Such research questions require causal inference methods such as inversed probability weighting when constructing a prediction model. It is worth mentioning that such counterfactual prediction studies are currently rare, and indeed were not encountered in our review, but they should not be seen as undesirable conflation.
The current study has a number of limitations. First of all, we included a limited sample of observational studies from 2018 which might not be representative of other years, journals or medical fields. It is worth noting that all recommended reporting guidelines were published before 2018. The median impact factor of our sample was relatively high (9.5) and it is likely that the amount of conflation is higher in journals with lower impact factors, which was a trend we also observed within our sample. Secondly, the data-extraction was performed by a single researcher and a second assessor was only consulted when the first assessor was unsure. The assessment will invariably contain some subjectivity, particularly whether heavily conflated articles are mainly etiological or predictive in aim. Furthermore, we could not assess whether relevant confounders were included or excluded for all included etiology studies, as this requires subject specific expertise. Importantly, our list of signaling questions is not exhaustive and may not pick up on all conflation. Conflation may not always be reflected in used terminology and studies labelled unclear may actually have been conflated. Additionally, conflation between prediction and etiology is only a small part of assessing a study’s methodological quality. A study which does not confuse prediction and etiology may still be sloppy or incorrect and some elements that we termed conflation, others might call poor etiology or prediction practices. Similarly, not all conflation leads to wrong conclusions. Finally, not all observational clinical research can be classified as either etiology or prediction and we appreciate the broad spectrum of forms that may lie between these two or completely outside of these realms.
In conclusion, undesirable conflation between prediction and etiology is common in medical observational studies and may present an obstacle to scientific progress. Researchers and readers should be mindful of the differences between etiology and prediction, to prevent biased estimates, erroneous conclusions and steering research in an inefficient or wrong direction.
Below is the link to the electronic supplementary material.
The work on this study by MvD was supported by a grant from the Dutch Kidney Foundation (16OKG12).
Availability of data and material
Code availability, declarations.
All authors declare no conflict of interest.
Publisher's Note
Springer Nature remains neutral with regard to jurisdictional claims in published maps and institutional affiliations.
Research design and its types: Exploratory, descriptive and causal
Research designs in marketing research and their types: exploratory, descriptive and causal.
A common definition of research is gathering and analysing information systematically. After having set the objectives of research, next step is to decide the research design which best suits the research objectives. It is on the basis of the research design that the research methodology and sampling procedure are selected. Then lay out the structure and sequence of the overall research project. Finally, the research design is implemented and this implementation begins with the design of the data gathering instruments. After that follows, data collection, manipulation and analysis and at last the data is interpreted to know the results.
A research design is an important part of research that serves as a road map letting you know your position and where you will stand once you have completed the research. Researchers must keep their ultimate objectives in mind even if they have to take detours and change direction during the research process. The road map helps them reach their destination. Based on the research objectives a researcher can decide which is the best way to reach the destination and there are always more than one to go. There is not one research design which you can consider the best but you can select from several. The choice of research design is affected by several factors, small and large. From research objectives to search budget and time frame, there are several factors that can help you determine which research design suits your research the best. Much of the choice is still based on the objectives of the research which may be one of the following or something similar:
– Gain insight into a specific phenomenon and conduct a broad exploration of a topic to gain better knowledge in that field.
– Describing a phenomenon, population or event where we can establish numerical relationships or determine the extent to which two variables covary.
– Establish cause and effect relationships between two variables to understand how one changes when we vary the other.
These were the three broadly defined types of objectives on which names of types of research designs have been obtained respectively.
The three types of research designs are:
– Exploratory research
– Descriptive research
– Causal research.
Exploratory research
It is a lot like exploration or detective work fuelled by curiosity. Researchers should use their instincts to find clues and venture into new territories in search of information. Flexibility is important in exploratory research and it is bound to result in new ideas, revelations and insights.
These are some of the objectives that warrant the need for exploratory research:
– To define an ambiguous problem more precisely like why sales of a specific product are declining.
– To gain a better understanding of an issue.
– to generate new ideas like what one can do to improve customer relationships.
– develop hypotheses that can explain the occurrence of specific phenomena.
– for providing insights like what political changes in the international environmental are going to affect our business.
– to determine if some research would be practical and to set priorities for future like we must focus on these two product categories because as per research interest in other categories has waned and they are not profitable nay more.
Some tools used to conduct exploratory research:
1. Secondary information:
Most often the best point to start research from is to study the previous research conducted in this area. If you study what others have found out, it will help you generate ideas, hypotheses and gain insights. For example if you are trying to conduct research to create an instrument that helps you measure customer satisfaction from your product or services then studying others’ research will help you reach your objectives quicker. Literature reviews most often are a great tool to conduct exploratory research.
2. Personal interviews:
Personal interviews are a great tool of exploratory research and talking to people with expertise in the respective areas can help you achieve your objectives more easily. Moreover, it is important to be flexible because the sources from which you can gain the most valuable insights are always not obvious. Sometimes you may find the right information from your managers, sometimes from your customers and sometimes talking to your supply chain partners could help understand the issue better. However, while personal interviews are also used in descriptive research, the level of flexibility differs. In descriptive research you are somewhat bound by your questions and in exploratory research you have to be flexible and rather than sticking to your original questions, you must ask questions related to what you are hearing from people you are talking to.
3. Focus groups:
Focus group is also a highly popular technique used to conduct exploratory research. A group of 8 to 12 people works on addressing a topic introduced by the moderator and the researcher. Focus groups have proved to be of special importance in :
– Letting marketing managers see how consumers respond to the company’s efforts.
– Generating hypothesis that can be tested through the use of descriptive and causal research.
– Introducing a new product to the respondents to know their impression.
– Suggest the current market trends.
– Deriving real results from abstract data or finding how a survey response translates into real life reactions.
The popularity of focus groups is because of their effectiveness and efficiency as well as because researchers or decision makers can themselves attend them and observe the response of the participants live. However, a major disadvantage is that a group of just 8 to 12 people cannot be believed to be representative of the entire consumer group. Moreover one cannot conduct extra focus groups to convert findings of exploratory research into descriptive data.
4. Case Analysis:
Analysing select cases also helps achieve the objectives of exploratory research. By doing an in-depth analysis of elect cases related to the topic one can reach his objectives. This approach is suitable when there are complex variables at work and to understand these complexities one may need to conduct intensive study. For example you want to know the reason or traits related to his level performance among your sales managers. You compare the top performers and bottom performers to check out which traits are common to the best performing salespeople.
5. Projective techniques:
Projective techniques are useful where the researchers are exploring a topic on which the respondents do not speak directly and clearly. Sensitive topics involving people’s personal lives fall obviously in this category. Projective techniques can be used to find out these deep hidden psychological motives which people would otherwise not reveal. Using a variety of communication and observable methods researchers explore these deep psychological motivations which otherwise do not reveal themselves at the surface.
These methods include (types of projective techniques):
– Word association –
In word association, a series of words are used to associate responses and find out the relationship. The respondents are provided with a series of words and they select the word that comes to mind first. The response, its frequency as well as the time taken to make the response helps establish the underlying motivations towards the topic. No response means a high level of emotional involvement blocking the response.
– Sentence completion –
Sentence completion is like a game of filling the gaps and allows responders to complete the sentence by inserting the right word or phrases. The responses are then analysed to establish underlying motivations towards the topic.
– Story telling –
Respondents are provided with a scene or drawing related to the topic and asked to knit a story around it. This allows the researcher to see there respondent’s association with the topic and his psychological motivations. Suppose a workplace scene is provided to the respondents and the stories help understand how these people view a workplace. The picture works as a visual aid or stimulus to bring out the inner thoughts or deep motivations of the respondent.
– Role playing or the third person technique –
Respondents are asked to play particular roles or enter a third person’s shoes to explain why a person might act in a certain way in a given situation. Like asking people to play the role of a retail customer arriving at a retain store..
While these techniques can provide intriguing insights, it is best to leave the tasks to the experts. Apart from being skilled at structuring these approaches, it is essential that one is also experienced at interpreting these results. If properly applied, these techniques can help you generate hypotheses, clarify results and generate ideas. Often it is good to start a multistage research project with exploratory research. Then based upon the results of exploratory research one can frame a descriptive research questionnaire or set a causal research experiment. However, exploratory results do not have value alone and cannot be used till the real research has taken place. It is because the preliminary research alone is not sufficient to support your claims and therefore exploratory research results cannot be used alone. Descriptive research is important to reach your objectives and test the hypotheses generated by exploratory research and still exploratory research is like a stepping stone to start the process.
Descriptive research:
Descriptive research as the name suggests is used for description or to describe phenomenon or idea. It is generally used in the following conditions:
1. to describe the traits of specific groups like our largest customers who account for more than 60 percent of our sales and based upon the results design future marketing efforts.
2. Covariance of two variables – like does consumption of our services vary by income range.
3. to estimate the size of consumer groups in a population that act in a specific manner. How often do newly married like to shop from our brand?
4. For specific predictions. Forecasting the number of companies switching to new CRM software.
Compared to exploratory research, descriptive research follows a very rigid approach. Its data collection methods are highly rigid as compared to the unstructured and flexible approach used in exploratory research. Exploratory research often forms the basis for descriptive research and the knowledge acquires through exploratory research is used to select respondents, setting priority issues, framing and asking questions as well as setting the time and place for the respondents like when and where to ask questions. While exploratory research can provide the hypotheses, you need to conduct descriptive research to prove the hypotheses. Exploratory research will answer the basic questions related to who, where, what when, why and how if descriptive research but then descriptive research will answer the final questions related to the market. The main difference between exploratory and descriptive research is that the first does not follow standardized methods but the second does.
So, descriptive research does the task of putting the picture created by exploratory research into the frame. Two basic types of descriptive research studies for collecting data are cross sectional and longitudinal studies.
Cross sectional studies:
Cross sectional designs are the most used and popular descriptive research design. It involves sampling the population at a given point of time. also referred to as sample surveys, it follows a high degree of structure in both data collection process and instrument.
Longitudinal studies:
While cross sectional studies sample the population at a point of time, longitudinal studies sample it over a period of time. The difference is just like that between a still photo and video film. The main objective of longitudinal studies is to observe behavioural changes occurring over period of time. Also known as true panels, these studies provide the same information at various specific points of time. This information can be combined with other information to know if some specific behaviour change was triggered by a particular act like brand switching was caused due to exposure to a particular advertisement.
Causal research:
Casual research is effective in terms of identifying covariation between variables but when it comes to identifying causality. Causal research helps identify if there is a causal relationship between two or more variables. It is highly structured like descriptive research and is also known for use of control procedures used during experimental designs related to tests of causal relationships. In most of such cases the researcher is concerned with knowing the impact the independent variable has on the dependent variable. Following are the main things that we are concerned with when using causal research:
Manipulation:
manipulation of the independent variable like price
Measurement:
Measuring the dependent variable like profit.
Controlling the variable that affects the dependent variable
Good marketing decisions and great strategy are always based upon useful market intelligence and such information can be obtained through research. Organisational problems can be carefully translated into research problems to find a solution. R esearch design is an essential and important component of research that helps you reach your destination and arrive at great decisions by getting actionable information. Research design includes one or more of the above categories. It is the research objectives that help us establish that whether one or more of the research designs must be used.
The Marketing Research Guide By Robert E. Stevens
- Key Differences
Know the Differences & Comparisons
Difference Between Exploratory and Descriptive Research
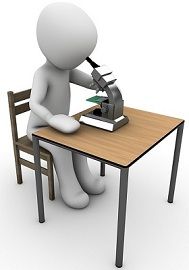
The research design is defined as a framework for carrying out research activities in different fields of study. The research design is classified into two important categories i.e. exploratory and conclusive research. Conclusive research is further subdivided into descriptive and casual research. The people often juxtapose exploratory research and descriptive research, but the fact is that they are different.
Take a read of this article to understand the differences between exploratory and descriptive research.
Content: Exploratory Research Vs Descriptive Research
Comparison chart, definition of exploratory research.
As the name implies, the primary objective of exploratory research is to explore a problem to provide insights into and comprehension for more precise investigation. It focuses on the discovery of ideas and thoughts. The exploratory research design is suitable for studies which are flexible enough to provide an opportunity for considering all the aspects of the problem.
At this point, the required information is loosely defined, and the research process is flexible and unstructured. It is used in the situation when you must define the problem correctly, identify alternative courses of actions, develop a hypothesis, gain additional insights before the development of an approach, set priorities for further examination. The following methods are used for conducting exploratory research
- Survey of concerning literature
- Experience survey
- Analysis of insights stimulating
Definition of Descriptive Research
By the term descriptive research, we mean a type of conclusive research study which is concerned with describing the characteristics of a particular individual or group. It includes research related to specific predictions, features or functions of person or group, the narration of facts, etc.
The descriptive research aims at obtaining complete and accurate information for the study, the method adopted must be carefully planned. The researcher should precisely define what he wants to measure? How does he want to measure? He should clearly define the population under study. It uses methods like quantitative analysis of secondary data, surveys, panels, observations, interviews, questionnaires, etc.
Descriptive Research concentrates on formulating the research objective, designing methods for the collection of data, selection of the sample, data collection, processing, and analysis, reporting the results.
Key Differences Between Exploratory and Descriptive Research
The difference between exploratory and descriptive research can be drawn clearly on the following grounds:
- Research conducted for formulating a problem for more clear investigation is called exploratory research. Research that explore and explains an individual, group or a situation, is called descriptive research.
- The exploratory research aims at the discovery of ideas and thoughts whereas the primary purpose of descriptive research is to describe the characteristics and functions.
- The overall design of the exploratory research should be flexible enough so that it provides an opportunity to consider various aspects of the problem. On the contrary, in descriptive research, the overall design should be rigid which protects against bias and also maximise reliability.
- The research process is unstructured in exploratory research. However, it is structured in the case of descriptive research.
- Non-probability sampling i.e. judgment or purposive sampling design is used in exploratory research. As opposed to descriptive research where probability (random) sampling design is used.
- When it comes to statistical design, exploratory research has no pre-planned design for analysis. Unlike, descriptive research that has the pre-planned design for analysis.
Therefore exploratory research results in insights or hypothesis, regardless of the method adopted, the most important thing is that it should remain flexible so that all the facets of the problem can be studied, as and when they arise. Conversely, descriptive research is a comparative design which is prepared according to the study and resources available. Such study minimises bias and maximises reliability.
You Might Also Like:
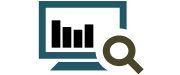
adrian says
November 16, 2017 at 6:22 pm
thank you so much for this incredible idea!
privanus simon says
June 20, 2018 at 11:42 am
The material you sent have been so useful in my study,Keep it up To me as as a Masters scholar I appriciate
Prasanna says
December 9, 2018 at 12:48 am
Overall website is superb,well explained.thanks a lot
ALBERT KOPES says
May 8, 2019 at 8:26 am
Thank you so much for your brilliant ideas. I really appreciated your hard work by putting your knowledge.. THANKS
Abinet A. Wanore says
October 11, 2019 at 10:11 pm
Thankyou so much. It is so helpful
Mkhulisi says
August 6, 2020 at 5:21 pm
this content has been very useful to me
Abdul Wahid Nuristani says
March 15, 2021 at 4:49 pm
great note.
Tina Sales says
January 25, 2022 at 9:02 pm
Thank you very much All information herein are very helpful for my current undertakings.
Sandile says
February 12, 2022 at 1:42 pm
I would like to reference your work, kindly assist
Leave a Reply Cancel reply
Your email address will not be published. Required fields are marked *
Save my name, email, and website in this browser for the next time I comment.
Religious Worship Attendance in America: Evidence from Cellphone Data
Religious worship is integral to the lives of millions of Americans. In this paper, I provide a descriptive analysis of religious worship attendance using geodata from smartphones for over 2 million Americans in 2019. I establish several key findings. First, 73% of people step into a religious place of worship at least once during the year on the primary day of worship (e.g. Sundays for most Christian churches). However, only 5% of Americans attend services “weekly”, far fewer than the ~22% who report to do so in surveys. The number of occasional vs. frequent attenders varies substantially by religion. I estimate that approximately 45M Americans attend worship services in a typical week of the year, but with large changes around Holidays (e.g. Easter). I document how start times, duration of attendance, and average household income all differ meaningfully across religious traditions. The intensity of religious observance correlates with a host of other activities. For example, relative to non-attenders and infrequent attenders, frequent religious attenders are less likely to go to strip clubs, liquor stores, and casinos. While cell phone data has limitations, this paper provides a unique way of understanding worship attendance and its correlates.
I am grateful to Carla Colina, Carolina Cannon, and Rory Lawson for incredible research assistance on this project. I also thank Josh Davis, Josh Dean, Stefano DellaVigna, Kareem Haggag, Alex Imas, Zack Nelson, David Ridge, Scott Shurtliff, Avner Strulov-Shlain, Justin Sydnor, and seminar participants at various universities for helpful comments and suggestions. The views expressed herein are those of the author and do not necessarily reflect the views of the National Bureau of Economic Research.
MARC RIS BibTeΧ
Download Citation Data
Mentioned in the News
More from nber.
In addition to working papers , the NBER disseminates affiliates’ latest findings through a range of free periodicals — the NBER Reporter , the NBER Digest , the Bulletin on Retirement and Disability , the Bulletin on Health , and the Bulletin on Entrepreneurship — as well as online conference reports , video lectures , and interviews .
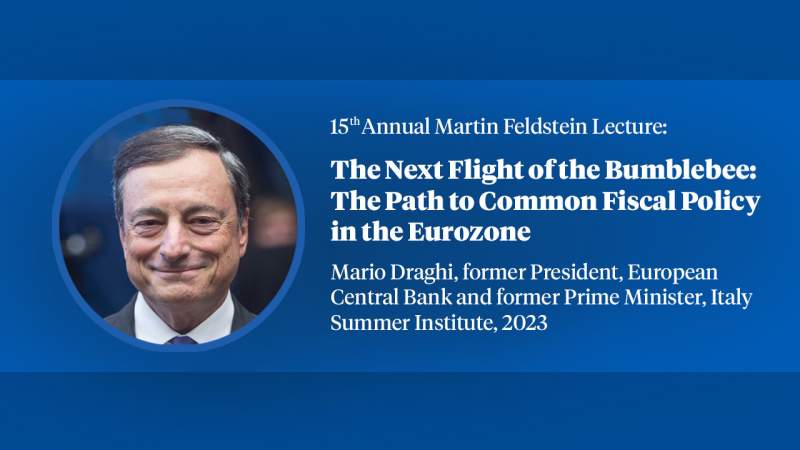
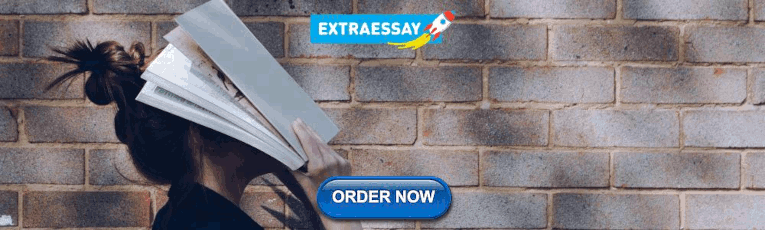
IMAGES
VIDEO
COMMENTS
In a causal study, researchers manipulate the set of independent variables to determine their effect, if any, on dependent variables. Researchers in causal studies also typically make use of a "control" -- a case in which the independent variables have not been manipulated, to allow researchers to compare the effects of manipulating the independent variables to the effects of leaving them ...
A previous Evidence in Practice article explained why a specific and answerable research question is important for clinicians and researchers. Determining whether a study aims to answer a descriptive, predictive, or causal question should be one of the first things a reader does when reading an article. Any type of question can be relevant and useful to support evidence-based practice, but ...
Descriptive research vs experimental research: Descriptive research gathers data without controlling any variables, while experimental research manipulates and controls variables to determine cause and effect. Do you want to identify characteristics, patterns and correlations or test causal relationships between variables?
Causal research, also known as explanatory research or causal-comparative research, identifies the extent and nature of cause-and-effect relationships between two or more variables. ... Descriptive research. Descriptive research is all about describing the characteristics of the population, phenomenon or scenario studied. It focuses more on the ...
While descriptive research observes and quantifies phenomena, causal research actively manipulates variables through experimentation to test hypotheses about causal effects. For example, a causal survey might compare control and test groups to evaluate the impact of different interventions. The objectives of causal research are two-fold:
Causal research has a highly structured and rigid research design and is generally conducted in the later stages of decision-making. In contrast, exploratory research is highly unstructured. It provides a lot of flexibility as it is generally the first step in any research process and is, therefore, in the early stages of decision-making.
for the study methods. Good-quality, clinically useful research begins. question. Research questions fall into 1 of 3 mutu-ally exclusive types: descriptive, predic-tive, or causal. Imagine you are seeking information about whiplash injuries. You might find studies that address the fol-lowing questions. 1.
Revised on June 22, 2023. Descriptive research aims to accurately and systematically describe a population, situation or phenomenon. It can answer what, where, when and how questions, but not why questions. A descriptive research design can use a wide variety of research methods to investigate one or more variables.
Descriptive Research vs. Causal Research. Descriptive research and causal research are both research methodologies, however, one focuses on a subject's behaviors while the latter focuses on a relationship's cause-and-effect. To buttress the above point, descriptive research aims to describe and document the characteristics, behaviors, or ...
Scientific research can be categorized into: a) descriptive research, with the main goal to summarize characteristics of a group (or person); b) predictive research, with the main goal to forecast future outcomes that can be used for screening, selection, or monitoring; and c) explanatory research, with the main goal to understand the underlying causal mechanism, which can then be used to ...
INTRODUCTION. In our previous article in this series, [ 1] we introduced the concept of "study designs"- as "the set of methods and procedures used to collect and analyze data on variables specified in a particular research question.". Study designs are primarily of two types - observational and interventional, with the former being ...
In fact, descriptive research has many useful applications, and you probably rely on findings from descriptive research without even being aware that that is what you are doing. See Table 3.1 for examples. Explanatory research. The third type of research, explanatory research, seeks to answer "why" questions. In this case, the researcher is ...
Causal-comparative research is retrospective and aims to predict future outcomes based on existing data. Descriptive research is observational and aims to describe patterns, trends, and relationships within a data set. Both methods contribute to the advancement of knowledge in various disciplines by providing valuable insights into complex ...
Descriptive research focuses more on the "what" of the research subject and less on the "why." Since descriptive research typically happens in a real-world setting, variables can cross-contaminate others. This increases the challenge of isolating cause-and-effect relationships. You may require further research if you need more causal links.
Descriptive vs. Causal Research. Descriptive research focuses on describing characteristics, behaviors, or phenomena without manipulating variables or establishing causal relationships. It provides a snapshot of the current state of affairs but does not attempt to explain why certain phenomena occur. Causal research, on the other hand, seeks to ...
Descriptive research design is used in situations where the researcher wants to describe a population or phenomenon in detail. It is used to gather information about the current status or condition of a group or phenomenon without making any causal inferences. Descriptive research design is useful in the following situations:
The major objective of exploratory research design is to discover ideas and insights, whereas descriptive research design involves describing market aspects and functions. Nevertheless, causal research design tries to determine the cause-effect relationships in the research one is conducting. We will write.
Importantly, descriptive questions may involve as many variables as you like. We could add a second variable, gender ( X1, male vs. female), and ask whether females have a higher level of trust - on average - than males. This already points to how we deal with the underlying distibutions. Normally, we summarize them using statistics such as the ...
Descriptive research focuses on understanding the characteristics of a specific population or phenomenon. The goal is to gain an accurate and complete picture of the subject. Causal research is used when researchers want to understand how two variables interact with each other and how changes in one variable cause changes in another.. These three types of research have their own unique ...
I would argue even purely descriptive research can be very much needed and useful. It becomes a cliché that good descriptive research provokes why questions and good descriptive research builds the foundation for further causal studies. However, because the favors given to causal studies and theory testing, we often take on a causal study too ...
For researchers, one of the most important recommendations is to clearly define the research question (including whether it is causal, predictive or descriptive). If the research question is unclear this ambiguity frequently continues throughout the rest of the article . Once the aim is clear we suggest consulting appropriate reporting and ...
Research designs in marketing research and their types: Exploratory, descriptive and causal. A common definition of research is gathering and analysing information systematically. After having set the objectives of research, next step is to decide the research design which best suits the research objectives. It is on the basis of the research ...
Exploratory research is one which aims at providing insights into and an understanding of the problem faced by the researcher. Descriptive research, on the other hand, aims at describing something, mainly functions and characteristics. The research design is defined as a framework for carrying out research activities in different fields of study.
In this paper, I provide a descriptive analysis of religious worship attendance using geodata from smartphones for over 2 million Americans in 2019. I establish several key findings. First, 73% of people step into a religious place of worship at least once during the year on the primary day of worship (e.g. Sundays for most Christian churches).