Change Password
Your password must have 8 characters or more and contain 3 of the following:.
- a lower case character,
- an upper case character,
- a special character
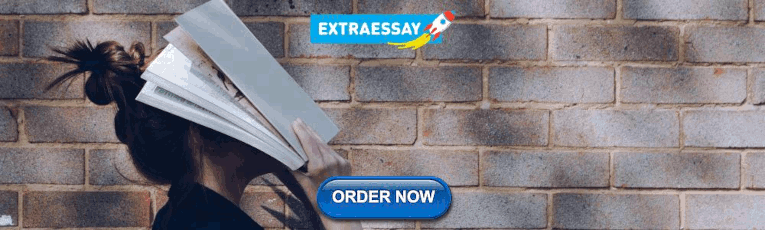
Password Changed Successfully
Your password has been changed
- Sign in / Register
Request Username
Can't sign in? Forgot your username?
Enter your email address below and we will send you your username
If the address matches an existing account you will receive an email with instructions to retrieve your username
Design-Based Research: A Methodology to Extend and Enrich Biology Education Research
- Emily E. Scott
- Mary Pat Wenderoth
- Jennifer H. Doherty
*Address correspondence to: Emily E. Scott ( E-mail Address: [email protected] ).
Department of Biology, University of Washington, Seattle, WA 98195
Search for more papers by this author
Recent calls in biology education research (BER) have recommended that researchers leverage learning theories and methodologies from other disciplines to investigate the mechanisms by which students to develop sophisticated ideas. We suggest design-based research from the learning sciences is a compelling methodology for achieving this aim. Design-based research investigates the “learning ecologies” that move student thinking toward mastery. These “learning ecologies” are grounded in theories of learning, produce measurable changes in student learning, generate design principles that guide the development of instructional tools, and are enacted using extended, iterative teaching experiments. In this essay, we introduce readers to the key elements of design-based research, using our own research into student learning in undergraduate physiology as an example of design-based research in BER. Then, we discuss how design-based research can extend work already done in BER and foster interdisciplinary collaborations among cognitive and learning scientists, biology education researchers, and instructors. We also explore some of the challenges associated with this methodological approach.
INTRODUCTION
There have been recent calls for biology education researchers to look toward other fields of educational inquiry for theories and methodologies to advance, and expand, our understanding of what helps students learn to think like biologists ( Coley and Tanner, 2012 ; Dolan, 2015 ; Peffer and Renken, 2016 ; Lo et al. , 2019 ). These calls include the recommendations that biology education researchers ground their work in learning theories from the cognitive and learning sciences ( Coley and Tanner, 2012 ) and begin investigating the underlying mechanisms by which students to develop sophisticated biology ideas ( Dolan, 2015 ; Lo et al. , 2019 ). Design-based research from the learning sciences is one methodology that seeks to do both by using theories of learning to investigate how “learning ecologies”—that is, complex systems of interactions among instructors, students, and environmental components—support the process of student learning ( Brown, 1992 ; Cobb et al. , 2003 ; Collins et al. , 2004 ; Peffer and Renken, 2016 ).
The purpose of this essay is twofold. First, we want to introduce readers to the key elements of design-based research, using our research into student learning in undergraduate physiology as an example of design-based research in biology education research (BER). Second, we will discuss how design-based research can extend work already done in BER and explore some of the challenges of its implementation. For a more in-depth review of design-based research, we direct readers to the following references: Brown (1992) , Barab and Squire (2004) , and Collins et al. (2004) , as well as commentaries by Anderson and Shattuck (2012) and McKenney and Reeves (2013) .
WHAT IS DESIGN-BASED RESEARCH?
Design-based research is a methodological approach that aligns with research methods from the fields of engineering or applied physics, where products are designed for specific purposes ( Brown, 1992 ; Joseph, 2004 ; Middleton et al. , 2008 ; Kelly, 2014 ). Consequently, investigators using design-based research approach educational inquiry much as an engineer develops a new product: First, the researchers identify a problem that needs to be addressed (e.g., a particular learning challenge that students face). Next, they design a potential “solution” to the problem in the form of instructional tools (e.g., reasoning strategies, worksheets; e.g., Reiser et al. , 2001 ) that theory and previous research suggest will address the problem. Then, the researchers test the instructional tools in a real-world setting (i.e., the classroom) to see if the tools positively impact student learning. As testing proceeds, researchers evaluate the instructional tools with emerging evidence of their effectiveness (or lack thereof) and progressively revise the tools— in real time —as necessary ( Collins et al. , 2004 ). Finally, the researchers reflect on the outcomes of the experiment, identifying the features of the instructional tools that were successful at addressing the initial learning problem, revising those aspects that were not helpful to learning, and determining how the research informed the theory underlying the experiment. This leads to another research cycle of designing, testing, evaluating, and reflecting to refine the instructional tools in support of student learning. We have characterized this iterative process in Figure 1 after Sandoval (2014) . Though we have portrayed four discrete phases to design-based research, there is often overlap of the phases as the research progresses (e.g., testing and evaluating can occur simultaneously).
FIGURE 1. The four phases of design-based research experienced in an iterative cycle (A). We also highlight the main features of each phase of our design-based research project investigating students’ use of flux in physiology (B).
Design-based research has no specific requirements for the form that instructional tools must take or the manner in which the tools are evaluated ( Bell, 2004 ; Anderson and Shattuck, 2012 ). Instead, design-based research has what Sandoval (2014) calls “epistemic commitments” 1 that inform the major goals of a design-based research project as well as how it is implemented. These epistemic commitments are: 1) Design based research should be grounded in theories of learning (e.g., constructivism, knowledge-in-pieces, conceptual change) that both inform the design of the instructional tools and are improved upon by the research ( Cobb et al. , 2003 ; Barab and Squire, 2004 ). This makes design-based research more than a method for testing whether or not an instructional tool works; it also investigates why the design worked and how it can be generalized to other learning environments ( Cobb et al. , 2003 ). 2) Design-based research should aim to produce measurable changes in student learning in classrooms around a particular learning problem ( Anderson and Shattuck, 2012 ; McKenney and Reeves, 2013 ). This requirement ensures that theoretical research into student learning is directly applicable, and impactful, to students and instructors in classroom settings ( Hoadley, 2004 ). 3) Design-based research should generate design principles that guide the development and implementation of future instructional tools ( Edelson, 2002 ). This commitment makes the research findings broadly applicable for use in a variety of classroom environments. 4) Design-based research should be enacted using extended, iterative teaching experiments in classrooms. By observing student learning over an extended period of time (e.g., throughout an entire term or across terms), researchers are more likely to observe the full effects of how the instructional tools impact student learning compared with short-term experiments ( Brown, 1992 ; Barab and Squire, 2004 ; Sandoval and Bell, 2004 ).
HOW IS DESIGN-BASED RESEARCH DIFFERENT FROM AN EXPERIMENTAL APPROACH?
Many BER studies employ experimental approaches that align with traditional scientific methods of experimentation, such as using treatment versus control groups, randomly assigning treatments to different groups, replicating interventions across multiple spatial or temporal periods, and using statistical methods to guide the kinds of inferences that arise from an experiment. While design-based research can similarly employ these strategies for educational inquiry, there are also some notable differences in its approach to experimentation ( Collins et al. , 2004 ; Hoadley, 2004 ). In this section, we contrast the differences between design-based research and what we call “experimental approaches,” although both paradigms represent a form of experimentation.
The first difference between an experimental approach and design-based research regards the role participants play in the experiment. In an experimental approach, the researcher is responsible for making all the decisions about how the experiment will be implemented and analyzed, while the instructor facilitates the experimental treatments. In design-based research, both researchers and instructors are engaged in all stages of the research from conception to reflection ( Collins et al. , 2004 ). In BER, a third condition frequently arises wherein the researcher is also the instructor. In this case, if the research questions being investigated produce generalizable results that have the potential to impact teaching broadly, then this is consistent with a design-based research approach ( Cobb et al. , 2003 ). However, when the research questions are self-reflective about how a researcher/instructor can improve his or her own classroom practices, this aligns more closely with “action research,” which is another methodology used in education research (see Stringer, 2013 ).
A second difference between experimental research and design-based research is the form that hypotheses take and the manner in which they are investigated ( Collins et al. , 2004 ; Sandoval, 2014 ). In experimental approaches, researchers develop a hypothesis about how a specific instructional intervention will impact student learning. The intervention is then tested in the classroom(s) while controlling for other variables that are not part of the study in order to isolate the effects of the intervention. Sometimes, researchers designate a “control” situation that serves as a comparison group that does not experience the intervention. For example, Jackson et al. (2018) were interested in comparing peer- and self-grading of weekly practice exams to if they were equally effective forms of deliberate practice for students in a large-enrollment class. To test this, the authors (including authors of this essay J.H.D., M.P.W.) designed an experiment in which lab sections of students in a large lecture course were randomly assigned to either a peer-grading or self-grading treatment so they could isolate the effects of each intervention. In design-based research, a hypothesis is conceptualized as the “design solution” rather than a specific intervention; that is, design-based researchers hypothesize that the designed instructional tools, when implemented in the classroom, will create a learning ecology that improves student learning around the identified learning problem ( Edelson, 2002 ; Bell, 2004 ). For example, Zagallo et al. (2016) developed a laboratory curriculum (i.e., the hypothesized “design solution”) for molecular and cellular biology majors to address the learning problem that students often struggle to connect scientific models and empirical data. This curriculum entailed: focusing instruction around a set of target biological models; developing small-group activities in which students interacted with the models by analyzing data from scientific papers; using formative assessment tools for student feedback; and providing students with a set of learning objectives they could use as study tools. They tested their curriculum in a novel, large-enrollment course of upper-division students over several years, making iterative changes to the curriculum as the study progressed.
By framing the research approach as an iterative endeavor of progressive refinement rather than a test of a particular intervention when all other variables are controlled, design-based researchers recognize that: 1) classrooms, and classroom experiences, are unique at any given time, making it difficult to truly “control” the environment in which an intervention occurs or establish a “control group” that differs only in the features of an intervention; and 2) many aspects of a classroom experience may influence the effectiveness of an intervention, often in unanticipated ways, which should be included in the research team’s analysis of an intervention’s success. Consequently, the research team is less concerned with controlling the research conditions—as in an experimental approach—and instead focuses on characterizing the learning environment ( Barab and Squire, 2004 ). This involves collecting data from multiple sources as the research progresses, including how the instructional tools were implemented, aspects of the implementation process that failed to go as planned, and how the instructional tools or implementation process was modified. These characterizations can provide important insights into what specific features of the instructional tools, or the learning environment, were most impactful to learning ( DBR Collective, 2003 ).
A third difference between experimental approaches and design-based research is when the instructional interventions can be modified. In experimental research, the intervention is fixed throughout the experimental period, with any revisions occurring only after the experiment has concluded. This is critical for ensuring that the results of the study provide evidence of the efficacy of a specific intervention. By contrast, design-based research takes a more flexible approach that allows instructional tools to be modified in situ as they are being implemented ( Hoadley, 2004 ; Barab, 2014 ). This flexibility allows the research team to modify instructional tools or strategies that prove inadequate for collecting the evidence necessary to evaluate the underlying theory and ensures a tight connection between interventions and a specific learning problem ( Collins et al. , 2004 ; Hoadley, 2004 ).
Finally, and importantly, experimental approaches and design-based research differ in the kinds of conclusions they draw from their data. Experimental research can “identify that something meaningful happened; but [it is] not able to articulate what about the intervention caused that story to unfold” ( Barab, 2014 , p. 162). In other words, experimental methods are robust for identifying where differences in learning occur, such as between groups of students experiencing peer- or self-grading of practice exams ( Jackson et al. , 2018 ) or receiving different curricula (e.g., Chi et al. , 2012 ). However, these methods are not able to characterize the underlying learning process or mechanism involved in the different learning outcomes. By contrast, design-based research has the potential to uncover mechanisms of learning, because it investigates how the nature of student thinking changes as students experience instructional interventions ( Shavelson et al. , 2003 ; Barab, 2014 ). According to Sandoval (2014) , “Design research, as a means of uncovering causal processes, is oriented not to finding effects but to finding functions , to understanding how desired (and undesired) effects arise through interactions in a designed environment” (p. 30). In Zagallo et al. (2016) , the authors found that their curriculum supported students’ data-interpretation skills, because it stimulated students’ spontaneous use of argumentation during which group members coconstructed evidence-based claims from the data provided. Students also worked collaboratively to decode figures and identify data patterns. These strategies were identified from the researchers’ qualitative data analysis of in-class recordings of small-group discussions, which allowed them to observe what students were doing to support their learning. Because design-based research is focused on characterizing how learning occurs in classrooms, it can begin to answer the kinds of mechanistic questions others have identified as central to advancing BER ( National Research Council [NRC], 2012 ; Dolan, 2015 ; Lo et al. , 2019 ).
DESIGN-BASED RESEARCH IN ACTION: AN EXAMPLE FROM UNDERGRADUATE PHYSIOLOGY
To illustrate how design-based research could be employed in BER, we draw on our own research that investigates how students learn physiology. We will characterize one iteration of our design-based research cycle ( Figure 1 ), emphasizing how our project uses Sandoval’s four epistemic commitments (i.e., theory driven, practically applied, generating design principles, implemented in an iterative manner) to guide our implementation.
Identifying the Learning Problem
Understanding physiological phenomena is challenging for students, given the wide variety of contexts (e.g., cardiovascular, neuromuscular, respiratory; animal vs. plant) and scales involved (e.g., using molecular-level interactions to explain organism functioning; Wang, 2004 ; Michael, 2007 ; Badenhorst et al. , 2016 ). To address these learning challenges, Modell (2000) identified seven “general models” that undergird most physiology phenomena (i.e., control systems, conservation of mass, mass and heat flow, elastic properties of tissues, transport across membranes, cell-to-cell communication, molecular interactions). Instructors can use these models as a “conceptual framework” to help students build intellectual coherence across phenomena and develop a deeper understanding of physiology ( Modell, 2000 ; Michael et al. , 2009 ). This approach aligns with theoretical work in the learning sciences that indicates that providing students with conceptual frameworks improves their ability to integrate and retrieve knowledge ( National Academies of Sciences, Engineering, and Medicine, 2018 ).
Before the start of our design-based project, we had been using Modell’s (2000) general models to guide our instruction. In this essay, we will focus on how we used the general models of mass and heat flow and transport across membranes in our instruction. These two models together describe how materials flow down gradients (e.g., pressure gradients, electrochemical gradients) against sources of resistance (e.g., tube diameter, channel frequency). We call this flux reasoning. We emphasized the fundamental nature and broad utility of flux reasoning in lecture and lab and frequently highlighted when it could be applied to explain a phenomenon. We also developed a conceptual scaffold (the Flux Reasoning Tool) that students could use to reason about physiological processes involving flux.
Although these instructional approaches had improved students’ understanding of flux phenomena, we found that students often demonstrated little commitment to using flux broadly across physiological contexts. Instead, they considered flux to be just another fact to memorize and applied it to narrow circumstances (e.g., they would use flux to reason about ions flowing across membranes—the context where flux was first introduced—but not the bulk flow of blood in a vessel). Students also struggled to integrate the various components of flux (e.g., balancing chemical and electrical gradients, accounting for variable resistance). We saw these issues reflected in students’ lower than hoped for exam scores on the cumulative final of the course. From these experiences, and from conversations with other physiology instructors, we identified a learning problem to address through design-based research: How do students learn to use flux reasoning to explain material flows in multiple physiology contexts?
The process of identifying a learning problem usually emerges from a researcher’s own experiences (in or outside a classroom) or from previous research that has been described in the literature ( Cobb et al. , 2003 ). To remain true to Sandoval’s first epistemic commitment, a learning problem must advance a theory of learning ( Edelson, 2002 ; McKenney and Reeves, 2013 ). In our work, we investigated how conceptual frameworks based on fundamental scientific concepts (i.e., Modell’s general models) could help students reason productively about physiology phenomena (National Academies of Sciences, Engineering, and Medicine, 2018; Modell, 2000 ). Our specific theoretical question was: Can we characterize how students’ conceptual frameworks around flux change as they work toward robust ideas? Sandoval’s second epistemic commitment stated that a learning problem must aim to improve student learning outcomes. The practical significance of our learning problem was: Does using the concept of flux as a foundational idea for instructional tools increase students’ learning of physiological phenomena?
We investigated our learning problem in an introductory biology course at a large R1 institution. The introductory course is the third in a biology sequence that focuses on plant and animal physiology. The course typically serves between 250 and 600 students in their sophomore or junior years each term. Classes have the following average demographics: 68% male, 21% from lower-income situations, 12% from an underrepresented minority, and 26% first-generation college students.
Design-Based Research Cycle 1, Phase 1: Designing Instructional Tools
The first phase of design-based research involves developing instructional tools that address both the theoretical and practical concerns of the learning problem ( Edelson, 2002 ; Wang and Hannafin, 2005 ). These instructional tools can take many forms, such as specific instructional strategies, classroom worksheets and practices, or technological software, as long as they embody the underlying learning theory being investigated. They must also produce classroom experiences or materials that can be evaluated to determine whether learning outcomes were met ( Sandoval, 2014 ). Indeed, this alignment between theory, the nature of the instructional tools, and the ways students are assessed is central to ensuring rigorous design-based research ( Hoadley, 2004 ; Sandoval, 2014 ). Taken together, the instructional tools instantiate a hypothesized learning environment that will advance both the theoretical and practical questions driving the research ( Barab, 2014 ).
In our work, the theoretical claim that instruction based on fundamental scientific concepts would support students’ flux reasoning was embodied in our instructional approach by being the central focus of all instructional materials, which included: a revised version of the Flux Reasoning Tool ( Figure 2 ); case study–based units in lecture that explicitly emphasized flux phenomena in real-world contexts ( Windschitl et al. , 2012 ; Scott et al. , 2018 ; Figure 3 ); classroom activities in which students practiced using flux to address physiological scenarios; links to online videos describing key flux-related concepts; constructed-response assessment items that cued students to use flux reasoning in their thinking; and pretest/posttest formative assessment questions that tracked student learning ( Figure 4 ).
FIGURE 2. The Flux Reasoning Tool given to students at the beginning of the quarter.
FIGURE 3. An example flux case study that is presented to students at the beginning of the neurophysiology unit. Throughout the unit, students learn how ion flows into and out of cells, as mediated by chemical and electrical gradients and various ion/molecular channels, sends signals throughout the body. They use this information to better understand why Jaime experiences persistent neuropathy. Images from: uz.wikipedia.org/wiki/Fayl:Blausen_0822_SpinalCord.png and commons.wikimedia.org/wiki/File:Figure_38_01_07.jpg.
FIGURE 4. An example flux assessment question about ion flows given in a pre-unit/post-unit formative assessment in the neurophysiology unit.
Phase 2: Testing the Instructional Tools
In the second phase of design-based research, the instructional tools are tested by implementing them in classrooms. During this phase, the instructional tools are placed “in harm’s way … in order to expose the details of the process to scrutiny” ( Cobb et al. , 2003 , p. 10). In this way, researchers and instructors test how the tools perform in real-world settings, which may differ considerably from the design team’s initial expectations ( Hoadley, 2004 ). During this phase, if necessary, the design team may make adjustments to the tools as they are being used to account for these unanticipated conditions ( Collins et al. , 2004 ).
We implemented the instructional tools during the Autumn and Spring quarters of the 2016–2017 academic year. Students were taught to use the Flux Reasoning Tool at the beginning of the term in the context of the first case study unit focused on neurophysiology. Each physiology unit throughout the term was associated with a new concept-based case study (usually about flux) that framed the context of the teaching. Embedded within the daily lectures were classroom activities in which students could practice using flux. Students were also assigned readings from the textbook and videos related to flux to watch during each unit. Throughout the term, students took five exams that each contained some flux questions as well as some pre- and post-unit formative assessment questions. During Winter quarter, we conducted clinical interviews with students who would take our course in the Spring term (i.e., “pre” data) as well as students who had just completed our course in Autumn (i.e., “post” data).
Phase 3: Evaluating the Instructional Tools
The third phase of a design-based research cycle involves evaluating the effectiveness of instructional tools using evidence of student learning ( Barab and Squire, 2004 ; Anderson and Shattuck, 2012 ). This can be done using products produced by students (e.g., homework, lab reports), attitudinal gains measured with surveys, participation rates in activities, interview testimonials, classroom discourse practices, and formative assessment or exam data (e.g., Reiser et al. , 2001 ; Cobb et al. , 2003 ; Barab and Squire, 2004 ; Mohan et al. , 2009 ). Regardless of the source, evidence must be in a form that supports a systematic analysis that could be scrutinized by other researchers ( Cobb et al. , 2003 ; Barab, 2014 ). Also, because design-based research often involves multiple data streams, researchers may need to use both quantitative and qualitative analytical methods to produce a rich picture of how the instructional tools affected student learning ( Collins et al. , 2004 ; Anderson and Shattuck, 2012 ).
In our work, we used the quality of students’ written responses on exams and formative assessment questions to determine whether students improved their understanding of physiological phenomena involving flux. For each assessment question, we analyzed a subset of student’s pretest answers to identify overarching patterns in students’ reasoning about flux, characterized these overarching patterns, then ordinated the patterns into different levels of sophistication. These became our scoring rubrics, which identified five different levels of student reasoning about flux. We used the rubrics to code the remainder of students’ responses, with a code designating the level of student reasoning associated with a particular reasoning pattern. We used this ordinal rubric format because it would later inform our theoretical understanding of how students build flux conceptual frameworks (see phase 4). This also allowed us to both characterize the ideas students held about flux phenomena and identify the frequency distribution of those ideas in a class.
By analyzing changes in the frequency distributions of students’ ideas across the rubric levels at different time points in the term (e.g., pre-unit vs. post-unit), we could track both the number of students who gained more sophisticated ideas about flux as the term progressed and the quality of those ideas. If the frequency of students reasoning at higher levels increased from pre-unit to post-unit assessments, we could conclude that our instructional tools as a whole were supporting students’ development of sophisticated flux ideas. For example, on one neuromuscular ion flux assessment question in the Spring of 2017, we found that relatively more students were reasoning at the highest levels of our rubric (i.e., levels 4 and 5) on the post-unit test compared with the pre-unit test. This meant that more students were beginning to integrate sophisticated ideas about flux (i.e., they were balancing concentration and electrical gradients) in their reasoning about ion movement.
To help validate this finding, we drew on three additional data streams: 1) from in-class group recordings of students working with flux items, we noted that students increasingly incorporated ideas about gradients and resistance when constructing their explanations as the term progressed; 2) from plant assessment items in the latter part of the term, we began to see students using flux ideas unprompted; and 3) from interviews, we observed that students who had already taken the course used flux ideas in their reasoning.
Through these analyses, we also noticed an interesting pattern in the pre-unit test data for Spring 2017 when compared with the frequency distribution of students’ responses with a previous term (Autumn 2016). In Spring 2017, 42% of students reasoned at level 4 or 5 on the pre-unit test, indicating these students already had sophisticated ideas about ion flux before they took the pre-unit assessment. This was surprising, considering only 2% of students reasoned at these levels for this item on the Autumn 2016 pre-unit test.
Phase 4: Reflecting on the Instructional Tools and Their Implementation
The final phase of a design-based research cycle involves a retrospective analysis that addresses the epistemic commitments of this methodology: How was the theory underpinning the research advanced by the research endeavor (theoretical outcome)? Did the instructional tools support student learning about the learning problem (practical outcome)? What were the critical features of the design solution that supported student learning (design principles)? ( Cobb et al. , 2003 ; Barab and Squire, 2004 ).
Theoretical Outcome (Epistemic Commitment 1).
Reflecting on how a design-based research experiment advances theory is critical to our understanding of how students learn in educational settings ( Barab and Squire, 2004 ; Mohan et al. , 2009 ). In our work, we aimed to characterize how students’ conceptual frameworks around flux change as they work toward robust ideas. To do this, we drew on learning progression research as our theoretical framing ( NRC, 2007 ; Corcoran et al. , 2009 ; Duschl et al. , 2011 ; Scott et al. , 2019 ). Learning progression frameworks describe empirically derived patterns in student thinking that are ordered into levels representing cognitive shifts in the ways students conceive a topic as they work toward mastery ( Gunckel et al. , 2012 ). We used our ion flux scoring rubrics to create a preliminary five-level learning progression framework ( Table 1 ). The framework describes how students’ ideas about flux often start with teleological-driven accounts at the lowest level (i.e., level 1), shift to focusing on driving forces (e.g., concentration gradients, electrical gradients) in the middle levels, and arrive at complex ideas that integrate multiple interacting forces at the higher levels. We further validated these reasoning patterns with our student interviews. However, our flux conceptual framework was largely based on student responses to our ion flux assessment items. Therefore, to further validate our learning progression framework, we needed a greater diversity of flux assessment items that investigated student thinking more broadly (i.e., about bulk flow, water movement) across physiological systems.
Practical Outcome (Epistemic Commitment 2).
In design-based research, learning theories must “do real work” by improving student learning in real-world settings ( DBR Collective, 2003 ). Therefore, design-based researchers must reflect on whether or not the data they collected show evidence that the instructional tools improved student learning ( Cobb et al. , 2003 ; Sharma and McShane, 2008 ). We determined whether our flux-based instructional approach aided student learning by analyzing the kinds of answers students provided to our assessment questions. Specifically, we considered students who reasoned at level 4 or above as demonstrating productive flux reasoning. Because almost half of students were reasoning at level 4 or 5 on the post-unit assessment after experiencing the instructional tools in the neurophysiology unit (in Spring 2017), we concluded that our tools supported student learning in physiology. Additionally, we noticed that students used language in their explanations that directly tied to the Flux Reasoning Tool ( Figure 2 ), which instructed them to use arrows to indicate the magnitude and direction of gradient-driving forces. For example, in a posttest response to our ion flux item ( Figure 4 ), one student wrote:
Ion movement is a function of concentration and electrical gradients . Which arrow is stronger determines the movement of K+. We can make the electrical arrow bigger and pointing in by making the membrane potential more negative than Ek [i.e., potassium’s equilibrium potential]. We can make the concentration arrow bigger and pointing in by making a very strong concentration gradient pointing in.
Given that almost half of students reasoned at level 4 or above, and that students used language from the Flux Reasoning Tool, we concluded that using fundamental concepts was a productive instructional approach for improving student learning in physiology and that our instructional tools aided student learning. However, some students in the 2016–2017 academic year continued to apply flux ideas more narrowly than intended (i.e., for ion and simple diffusion cases, but not water flux or bulk flow). This suggested that students had developed nascent flux conceptual frameworks after experiencing the instructional tools but could use more support to realize the broad applicability of this principle. Also, although our cross-sectional interview approach demonstrated how students’ ideas, overall, could change after experiencing the instructional tools, it did not provide information about how a student developed flux reasoning.
Reflecting on practical outcomes also means interpreting any learning gains in the context of the learning ecology. This reflection allowed us to identify whether there were particular aspects of the instructional tools that were better at supporting learning than others ( DBR Collective, 2003 ). Indeed, this was critical for our understanding why 42% of students scored at level 3 and above on the pre-unit ion assessment in the Spring of 2017, while only 2% of students scored level 3 and above in Autumn of 2016. When we reviewed notes of the Spring 2017 implementation scheme, we saw that the pretest was due at the end of the first day of class after students had been exposed to ion flux ideas in class and in a reading/video assignment about ion flow, which may be one reason for the students’ high performance on the pretest. Consequently, we could not tell whether students’ initial high performance was due to their learning from the activities in the first day of class or for other reasons we did not measure. It also indicated we needed to close pretests before the first day of class for a more accurate measure of students’ incoming ideas and the effectiveness of the instructional tools employed at the beginning of the unit.
Design Principles (Epistemic Commitment 3).
Although design-based research is enacted in local contexts (i.e., a particular classroom), its purpose is to inform learning ecologies that have broad applications to improve learning and teaching ( Edelson, 2002 ; Cobb et al. , 2003 ). Therefore, design-based research should produce design principles that describe characteristics of learning environments that researchers and instructors can use to develop instructional tools specific to their local contexts (e.g., Edelson, 2002 ; Subramaniam et al. , 2015 ). Consequently, the design principles must balance specificity with adaptability so they can be used broadly to inform instruction ( Collins et al. , 2004 ; Barab, 2014 ).
From our first cycle of design-based research, we developed the following design principles: 1) Key scientific concepts should provide an overarching framework for course organization. This way, the individual components that make up a course, like instructional units, activities, practice problems, and assessments, all reinforce the centrality of the key concept. 2) Instructional tools should explicitly articulate the principle of interest, with specific guidance on how that principle is applied in context. This stresses the applied nature of the principle and that it is more than a fact to be memorized. 3) Instructional tools need to show specific instances of how the principle is applied in multiple contexts to combat students’ narrow application of the principle to a limited number of contexts.
Design-Based Research Cycle 2, Phase 1: Redesign and Refine the Experiment
The last “epistemic commitment” Sandoval (2014) articulated was that design-based research be an iterative process with an eye toward continually refining the instructional tools, based on evidence of student learning, to produce more robust learning environments. By viewing educational inquiry as formative research, design-based researchers recognize the difficulty in accounting for all variables that could impact student learning, or the implementation of the instructional tools, a priori ( Collins et al. , 2004 ). Robust instructional designs are the products of trial and error, which are strengthened by a systematic analysis of how they perform in real-world settings.
To continue to advance our work investigating student thinking using the principle of flux, we began a second cycle of design-based research that continued to address the learning problem of helping students reason with fundamental scientific concepts. In this cycle, we largely focused on broadening the number of physiological systems that had accompanying formative assessment questions (i.e., beyond ion flux), collecting student reasoning from a more diverse population of students (e.g., upper division, allied heath, community college), and refining and validating the flux learning progression with both written and interview data in a student through time. We developed a suite of constructed-response flux assessment questions that spanned neuromuscular, cardiovascular, respiratory, renal, and plant physiological contexts and asked students about several kinds of flux: ion movement, diffusion, water movement, and bulk flow (29 total questions; available at beyondmultiplechoice.org). This would provide us with rich qualitative data that we could use to refine the learning progression. We decided to administer written assessments and conduct interviews in a pretest/posttest manner at the beginning and end of each unit both as a way to increase our data about student reasoning and to provide students with additional practice using flux reasoning across contexts.
From this second round of designing instructional tools (i.e., broader range of assessment items), testing them in the classroom (i.e., administering the assessment items to diverse student populations), evaluating the tools (i.e., developing learning progression–aligned rubrics across phenomena from student data, tracking changes in the frequency distribution of students across levels through time), and reflecting on the tools’ success, we would develop a more thorough and robust characterization of how students use flux across systems that could better inform our creation of new instructional tools to support student learning.
HOW CAN DESIGN-BASED RESEARCH EXTEND AND ENRICH BER?
While design-based research has primarily been used in educational inquiry at the K–12 level (see Reiser et al. , 2001 ; Mohan et al. , 2009 ; Jin and Anderson, 2012 ), other science disciplines at undergraduate institutions have begun to employ this methodology to create robust instructional approaches (e.g., Szteinberg et al. , 2014 in chemistry; Hake, 2007 , and Sharma and McShane, 2008 , in physics; Kelly, 2014 , in engineering). Our own work, as well as that by Zagallo et al. (2016) , provides two examples of how design-based research could be implemented in BER. Below, we articulate some of the ways incorporating design-based research into BER could extend and enrich this field of educational inquiry.
Design-Based Research Connects Theory with Practice
One critique of BER is that it does not draw heavily enough on learning theories from other disciplines like cognitive psychology or the learning sciences to inform its research ( Coley and Tanner, 2012 ; Dolan, 2015 ; Peffer and Renken, 2016 ; Davidesco and Milne, 2019 ). For example, there has been considerable work in BER developing concept inventories as formative assessment tools that identify concepts students often struggle to learn (e.g., Marbach-Ad et al. , 2009 ; McFarland et al. , 2017 ; Summers et al. , 2018 ). However, much of this work is detached from a theoretical understanding of why students hold misconceptions in the first place, what the nature of their thinking is, and the learning mechanisms that would move students to a more productive understanding of domain ideas ( Alonzo, 2011 ). Using design-based research to understand the basis of students’ misconceptions would ground these practical learning problems in a theoretical understanding of the nature of student thinking (e.g., see Coley and Tanner, 2012 , 2015 ; Gouvea and Simon, 2018 ) and the kinds of instructional tools that would best support the learning process.
Design-Based Research Fosters Collaborations across Disciplines
Recently, there have been multiple calls across science, technology, engineering, and mathematics education fields to increase collaborations between BER and other disciplines so as to increase the robustness of science education research at the collegiate level ( Coley and Tanner, 2012 ; NRC, 2012 ; Talanquer, 2014 ; Dolan, 2015 ; Peffer and Renken, 2016 ; Mestre et al. , 2018 ; Davidesco and Milne, 2019 ). Engaging in design-based research provides both a mechanism and a motivation for fostering interdisciplinary collaborations, as it requires the design team to have theoretical knowledge of how students learn, domain knowledge of practical learning problems, and instructional knowledge for how to implement instructional tools in the classroom ( Edelson, 2002 ; Hoadley, 2004 ; Wang and Hannafin, 2005 ; Anderson and Shattuck, 2012 ). For example, in our current work, our research team consists of two discipline-based education learning scientists from an R1 institution, two physiology education researchers/instructors (one from an R1 institution the other from a community college), several physiology disciplinary experts/instructors, and a K–12 science education expert.
Design-based research collaborations have several distinct benefits for BER: first, learning or cognitive scientists could provide theoretical and methodological expertise that may be unfamiliar to biology education researchers with traditional science backgrounds ( Lo et al. , 2019 ). This would both improve the rigor of the research project and provide biology education researchers with the opportunity to explore ideas and methods from other disciplines. Second, collaborations between researchers and instructors could help increase the implementation of evidence-based teaching practices by instructors/faculty who are not education researchers and would benefit from support while shifting their instructional approaches ( Eddy et al. , 2015 ). This may be especially true for community college and primarily undergraduate institution faculty who often do not have access to the same kinds of resources that researchers and instructors at research-intensive institutions do ( Schinske et al. , 2017 ). Third, making instructors an integral part of a design-based research project ensures they are well versed in the theory and learning objectives underlying the instructional tools they are implementing in the classroom. This can improve the fidelity of implementation of the instructional tools, because the instructors understand the tools’ theoretical and practical purposes, which has been cited as one reason there have been mixed results on the impact of active learning across biology classes ( Andrews et al. , 2011 ; Borrego et al. , 2013 ; Lee et al. , 2018 ; Offerdahl et al. , 2018 ). It also gives instructors agency to make informed adjustments to the instructional tools during implementation that improve their practical applications while remaining true to the goals of the research ( Hoadley, 2004 ).
Design-Based Research Invites Using Mixed Methods to Analyze Data
The diverse nature of the data that are often collected in design-based research can require both qualitative and quantitative methodologies to produce a rich picture of how the instructional tools and their implementation influenced student learning ( Anderson and Shattuck, 2012 ). Using mixed methods may be less familiar to biology education researchers who were primarily trained in quantitative methods as biologists ( Lo et al. , 2019 ). However, according to Warfa (2016 , p. 2), “Integration of research findings from quantitative and qualitative inquiries in the same study or across studies maximizes the affordances of each approach and can provide better understanding of biology teaching and learning than either approach alone.” Although the number of BER studies using mixed methods has increased over the past decade ( Lo et al. , 2019 ), engaging in design-based research could further this trend through its collaborative nature of bringing social scientists together with biology education researchers to share research methodologies from different fields. By leveraging qualitative and quantitative methods, design-based researchers unpack “mechanism and process” by characterizing the nature of student thinking rather than “simply reporting that differences did or did not occur” ( Barab, 2014 , p. 158), which is important for continuing to advance our understanding of student learning in BER ( Dolan, 2015 ; Lo et al. , 2019 ).
CHALLENGES TO IMPLEMENTING DESIGN-BASED RESEARCH IN BER
As with any methodological approach, there can be challenges to implementing design-based research. Here, we highlight three that may be relevant to BER.
Collaborations Can Be Difficult to Maintain
While collaborations between researchers and instructors offer many affordances (as discussed earlier), the reality of connecting researchers across departments and institutions can be challenging. For example, Peffer and Renken (2016) noted that different traditions of scholarship can present barriers to collaboration where there is not mutual respect for the methods and ideas that are part and parcel to each discipline. Additionally, Schinske et al. (2017) identified several constraints that community college faculty face for engaging in BER, such as limited time or support (e.g., infrastructural, administrative, and peer support), which could also impact their ability to form the kinds of collaborations inherent in design-based research. Moreover, the iterative nature of design-based research requires these collaborations to persist for an extended period of time. Attending to these challenges is an important part of forming the design team and identifying the different roles researchers and instructors will play in the research.
Design-Based Research Experiments Are Resource Intensive
The focus of design-based research on studying learning ecologies to uncover mechanisms of learning requires that researchers collect multiple data streams through time, which often necessitates significant temporal and financial resources ( Collins et al., 2004 ; O’Donnell, 2004 ). Consequently, researchers must weigh both practical as well as methodological considerations when formulating their experimental design. For example, investigating learning mechanisms requires that researchers collect data at a frequency that will capture changes in student thinking ( Siegler, 2006 ). However, researchers may be constrained in the number of data-collection events they can anticipate depending on: the instructor’s ability to facilitate in-class collection events or solicit student participation in extracurricular activities (e.g., interviews); the cost of technological devices to record student conversations; the time and logistical considerations needed to schedule and conduct student interviews; the financial resources available to compensate student participants; the financial and temporal costs associated with analyzing large amounts of data.
Identifying learning mechanisms also requires in-depth analyses of qualitative data as students experience various instructional tools (e.g., microgenetic methods; Flynn et al. , 2006 ; Siegler, 2006 ). The high intensity of these in-depth analyses often limits the number of students who can be evaluated in this way, which must be balanced with the kinds of generalizations researchers wish to make about the effectiveness of the instructional tools ( O’Donnell, 2004 ). Because of the large variety of data streams that could be collected in a design-based research experiment—and the resources required to collect and analyze them—it is critical that the research team identify a priori how specific data streams, and the methods of their analysis, will provide the evidence necessary to address the theoretical and practical objectives of the research (see the following section on experimental rigor; Sandoval, 2014 ). These are critical management decisions because of the need for a transparent, systematic analysis of the data that others can scrutinize to evaluate the validity of the claims being made ( Cobb et al. , 2003 ).
Concerns with Experimental Rigor
The nature of design-based research, with its use of narrative to characterize versus control experimental environments, has drawn concerns about the rigor of this methodological approach. Some have challenged its ability to produce evidence-based warrants to support its claims of learning that can be replicated and critiqued by others ( Shavelson et al. , 2003 ; Hoadley, 2004 ). This is a valid concern that design-based researchers, and indeed all education researchers, must address to ensure their research meets established standards for education research ( NRC, 2002 ).
One way design-based researchers address this concern is by “specifying theoretically salient features of a learning environment design and mapping out how they are predicted to work together to produce desired outcomes” ( Sandoval, 2014 , p. 19). Through this process, researchers explicitly show before they begin the work how their theory of learning is embodied in the instructional tools to be tested, the specific data the tools will produce for analysis, and what outcomes will be taken as evidence for success. Moreover, by allowing instructional tools to be modified during the testing phase as needed, design-based researchers acknowledge that it is impossible to anticipate all aspects of the classroom environment that might impact the implementation of instructional tools, “as dozens (if not millions) of factors interact to produce the measureable outcomes related to learning” ( Hoadley, 2004 , p. 204; DBR Collective, 2003 ). Consequently, modifying instructional tools midstream to account for these unanticipated factors can ensure they retain their methodological alignment with the underlying theory and predicted learning outcomes so that inferences drawn from the design experiment accurately reflect what was being tested ( Edelson, 2002 ; Hoadley, 2004 ). Indeed, Barab (2014) states, “the messiness of real-world practice must be recognized, understood, and integrated as part of the theoretical claims if the claims are to have real-world explanatory value” (p. 153).
CONCLUSIONS
providing a methodology that integrates theories of learning with practical experiences in classrooms,
using a range of analytical approaches that allow for researchers to uncover the underlying mechanisms of student thinking and learning,
fostering interdisciplinary collaborations among researchers and instructors, and
characterizing learning ecologies that account for the complexity involved in student learning
By employing this methodology from the learning sciences, biology education researchers can enrich our current understanding of what is required to help biology students achieve their personal and professional aims during their college experience. It can also stimulate new ideas for biology education that can be discussed and debated in our research community as we continue to explore and refine how best to serve the students who pass through our classroom doors.
1 “Epistemic commitment” is defined as engaging in certain practices that generate knowledge in an agreed-upon way.
ACKNOWLEDGMENTS
We thank the UW Biology Education Research Group’s (BERG) feedback on drafts of this essay as well as Dr. L. Jescovich for last-minute analyses. This work was supported by a National Science Foundation award (NSF DUE 1661263/1660643). Any opinions, findings, and conclusions or recommendations expressed in this material are those of the author(s) and do not necessarily reflect the views of the NSF. All procedures were conducted in accordance with approval from the Institutional Review Board at the University of Washington (52146) and the New England Independent Review Board (120160152).
- Alonzo, A. C. ( 2011 ). Learning progressions that support formative assessment practices . Measurement , 9 (2/3), 124–129. Google Scholar
- Anderson, T., & Shattuck, J. ( 2012 ). Design-based research: A decade of progress in education research? Educational Researcher , 41 (1), 16–25. Google Scholar
- Andrews, T. M., Leonard, M. J., Colgrove, C. A., & Kalinowski, S. T. ( 2011 ). Active learning not associated with student learning in a random sample of college biology courses . CBE—Life Sciences Education , 10 (4), 394–405. Link , Google Scholar
- Badenhorst, E., Hartman, N., & Mamede, S. ( 2016 ). How biomedical misconceptions may arise and affect medical students’ learning: A review of theoretical perspectives and empirical evidence . Health Professions Education , 2 (1), 10–17. Google Scholar
- Barab, S. ( 2014 ). Design-based research: A methodological toolkit for engineering change . In The Cambridge handbook of the learning sciences (2nd ed., pp. 151–170). Cambridge University Press. https://doi.org/10.1017/CBO9781139519526.011 Google Scholar
- Barab, S., & Squire, K. ( 2004 ). Design-based research: Putting a stake in the ground . Journal of the Learning Sciences , 13 (1), 1–14. Google Scholar
- Bell, P. ( 2004 ). On the theoretical breadth of design-based research in education . Educational Psychologist , 39 (4), 243–253. Google Scholar
- Borrego, M., Cutler, S., Prince, M., Henderson, C., & Froyd, J. E. ( 2013 ). Fidelity of implementation of research-based instructional strategies (RBIS) in engineering science courses . Journal of Engineering Education , 102 (3), 394–425. Google Scholar
- Brown, A. L. ( 1992 ). Design experiments: Theoretical and methodological challenges in creating complex interventions in classroom settings . Journal of the Learning Sciences , 2 (2), 141–178. Google Scholar
- Chi, M. T. H., Roscoe, R. D., Slotta, J. D., Roy, M., & Chase, C. C. ( 2012 ). Misconceived causal explanations for emergent processes . Cognitive Science , 36 (1), 1–61. Medline , Google Scholar
- Cobb, P., Confrey, J., diSessa, A., Lehrer, R., & Schauble, L. ( 2003 ). Design experiments in educational research . Educational Researcher , 32 (1), 9–13. Google Scholar
- Coley, J. D., & Tanner, K. D. ( 2012 ). Common origins of diverse misconceptions: Cognitive principles and the development of biology thinking . CBE—Life Sciences Education , 11 (3), 209–215. Link , Google Scholar
- Coley, J. D., & Tanner, K. ( 2015 ). Relations between intuitive biological thinking and biological misconceptions in biology majors and nonmajors . CBE—Life Sciences Education , 14 (1). https://doi.org/10.1187/cbe.14-06-0094 Medline , Google Scholar
- Collins, A., Joseph, D., & Bielaczyc, K. ( 2004 ). Design research: Theoretical and methodological issues . Journal of the Learning Sciences , 13 (1), 15–42. Google Scholar
- Corcoran, T., Mosher, F. A., & Rogat, A. D. ( 2009 ). Learning progressions in science: An evidence-based approach to reform (CPRE Research Report No. RR-63) . Philadelphia, PA: Consortium for Policy Research in Education. Google Scholar
- Davidesco, I., & Milne, C. ( 2019 ). Implementing cognitive science and discipline-based education research in the undergraduate science classroom . CBE—Life Sciences Education , 18 (3), es4. Link , Google Scholar
- Design-Based Research Collective . ( 2003 ). Design-based research: An emerging paradigm for educational inquiry . Educational Researcher , 32 (1), 5–8. Google Scholar
- Dolan, E. L. ( 2015 ). Biology education research 2.0 . CBE—Life Sciences Education , 14 (4), ed1. Link , Google Scholar
- Duschl, R., Maeng, S., & Sezen, A. ( 2011 ). Learning progressions and teaching sequences: A review and analysis . Studies in Science Education , 47 (2), 123–182. Google Scholar
- Eddy, S. L., Converse, M., & Wenderoth, M. P. ( 2015 ). PORTAAL: A classroom observation tool assessing evidence-based teaching practices for active learning in large science, technology, engineering, and mathematics classes . CBE—Life Sciences Education , 14 (2), ar23. Link , Google Scholar
- Edelson, D. C. ( 2002 ). Design research: What we learn when we engage in design . Journal of the Learning Sciences , 11 (1), 105–121. Google Scholar
- Flynn, E., Pine, K., & Lewis, C. ( 2006 ). The microgenetic method—Time for change? The Psychologist , 19 (3), 152–155. Google Scholar
- Gouvea, J. S., & Simon, M. R. ( 2018 ). Challenging cognitive construals: A dynamic alternative to stable misconceptions . CBE—Life Sciences Education , 17 (2), ar34. Link , Google Scholar
- Gunckel, K. L., Mohan, L., Covitt, B. A., & Anderson, C. W. ( 2012 ). Addressing challenges in developing learning progressions for environmental science literacy . In Alonzo, A. C.Gotwals, A. W. (Eds.), Learning progressions in science: Current challenges and future directions (pp. 39–75). Rotterdam: SensePublishers. https://doi.org/10.1007/978-94-6091-824-7_4 Google Scholar
- Hake, R. R. ( 2007 ). Design-based research in physics education research: A review . In Kelly, A. E.Lesh, R. A.Baek, J. Y. (Eds.), Handbook of design research methods in mathematics, science, and technology education (p. 24). New York: Routledge. Google Scholar
- Hoadley, C. M. ( 2004 ). Methodological alignment in design-based research . Educational Psychologist , 39 (4), 203–212. Google Scholar
- Jackson, M., Tran, A., Wenderoth, M. P., & Doherty, J. H. ( 2018 ). Peer vs. self-grading of practice exams: Which is better? CBE—Life Sciences Education , 17 (3), es44. https://doi.org/10.1187/cbe.18-04-0052 Link , Google Scholar
- Jin, H., & Anderson, C. W. ( 2012 ). A learning progression for energy in socio-ecological systems . Journal of Research in Science Teaching , 49 (9), 1149–1180. Google Scholar
- Joseph, D. ( 2004 ). The practice of design-based research: Uncovering the interplay between design, research, and the real-world context . Educational Psychologist , 39 (4), 235–242. Google Scholar
- Kelly, A. E. ( 2014 ). Design-based research in engineering education . In Cambridge handbook of engineering education research (pp. 497–518). New York, NY: Cambridge University Press. https://doi.org/10.1017/CBO9781139013451.032 Google Scholar
- Lee, C. J., Toven-Lindsey, B., Shapiro, C., Soh, M., Mazrouee, S., Levis-Fitzgerald, M., & Sanders, E. R. ( 2018 ). Error-discovery learning boosts student engagement and performance, while reducing student attrition in a bioinformatics course . CBE—Life Sciences Education , 17 (3), ar40. https://doi.org/10.1187/cbe.17-04-0061 Link , Google Scholar
- Lo, S. M., Gardner, G. E., Reid, J., Napoleon-Fanis, V., Carroll, P., Smith, E., & Sato, B. K. ( 2019 ). Prevailing questions and methodologies in biology education research: A longitudinal analysis of research in CBE — life sciences education and at the society for the advancement of biology education research . CBE—Life Sciences Education , 18 (1), ar9. Link , Google Scholar
- Marbach-Ad, G., Briken, V., El-Sayed, N. M., Frauwirth, K., Fredericksen, B., Hutcheson, S., … & Smith, A. C. ( 2009 ). Assessing student understanding of host pathogen interactions using a concept inventory . Journal of Microbiology & Biology Education , 10 (1), 43–50. Medline , Google Scholar
- McFarland, J. L., Price, R. M., Wenderoth, M. P., Martinková, P., Cliff, W., Michael, J. , … & Wright, A. ( 2017 ). Development and validation of the homeostasis concept inventory . CBE—Life Sciences Education , 16 (2), ar35. Link , Google Scholar
- McKenney, S., & Reeves, T. C. ( 2013 ). Systematic review of design-based research progress: Is a little knowledge a dangerous thing? Educational Researcher , 42 (2), 97–100. Google Scholar
- Mestre, J. P., Cheville, A., & Herman, G. L. ( 2018 ). Promoting DBER-cognitive psychology collaborations in STEM education . Journal of Engineering Education , 107 (1), 5–10. Google Scholar
- Michael, J. A. ( 2007 ). What makes physiology hard for students to learn? Results of a faculty survey . AJP: Advances in Physiology Education , 31 (1), 34–40. Medline , Google Scholar
- Michael, J. A., Modell, H., McFarland, J., & Cliff, W. ( 2009 ). The “core principles” of physiology: What should students understand? Advances in Physiology Education , 33 (1), 10–16. Medline , Google Scholar
- Middleton, J., Gorard, S., Taylor, C., & Bannan-Ritland, B. ( 2008 ). The “compleat” design experiment: From soup to nuts . In Kelly, A. E.Lesh, R. A.Baek, J. Y. (Eds.), Handbook of design research methods in education: Innovations in science, technology, engineering, and mathematics learning and teaching (pp. 21–46). New York, NY: Routledge. Google Scholar
- Modell, H. I. ( 2000 ). How to help students understand physiology? Emphasize general models . Advances in Physiology Education , 23 (1), S101–S107. Medline , Google Scholar
- Mohan, L., Chen, J., & Anderson, C. W. ( 2009 ). Developing a multi-year learning progression for carbon cycling in socio-ecological systems . Journal of Research in Science Teaching , 46 (6), 675–698. Google Scholar
- National Academies of Sciences, Engineering, and Medicine . ( 2018 ). How People Learn II: Learners, Contexts, and Cultures . Washington, DC: National Academies Press. Retrieved June 24, 2019, from https://doi.org/10.17226/24783 Google Scholar
- National Research Council (NRC) . ( 2002 ). Scientific research in education . Washington, DC: National Academies Press. Retrieved January 31, 2019, from https://doi.org/10.17226/10236 Google Scholar
- NRC . ( 2007 ). Taking science to school: Learning and teaching science in grades K–8 . Washington, DC: National Academies Press. Retrieved March 22, 2019, from www.nap.edu/catalog/11625/taking-science-to-school-learning-and-teaching-science-in-grades . https://doi.org/10.17226/11625 Google Scholar
- NRC . ( 2012 ). Discipline-based education research: Understanding and improving learning in undergraduate science and engineering . Washington, DC: National Academies Press. Retrieved from www.nap.edu/catalog/13362/discipline-based-education-research-understanding-and-improving-learning-in-undergraduate . https://doi.org/10.17226/13362 Google Scholar
- NRC . ( 2018 ). How people learn II: Learners, contexts, and cultures . Washington, DC: National Academies Press. Retrieved from www.nap.edu/read/24783/chapter/7 . https://doi.org/10.17226/24783 Google Scholar
- O’Donnell, A. M. ( 2004 ). A commentary on design research . Educational Psychologist , 39 (4), 255–260. Google Scholar
- Offerdahl, E. G., McConnell, M., & Boyer, J. ( 2018 ). Can I have your recipe? Using a fidelity of implementation (FOI) framework to identify the key ingredients of formative assessment for learning . CBE—Life Sciences Education , 17 (4), es16. Link , Google Scholar
- Peffer, M., & Renken, M. ( 2016 ). Practical strategies for collaboration across discipline-based education research and the learning sciences . CBE—Life Sciences Education , 15 (4), es11. Link , Google Scholar
- Reiser, B. J., Smith, B. K., Tabak, I., Steinmuller, F., Sandoval, W. A., & Leone, A. J. ( 2001 ). BGuILE: Strategic and conceptual scaffolds for scientific inquiry in biology classrooms . In Carver, S. M.Klahr, D. (Eds.), Cognition and instruction: Twenty-five years of progress (pp. 263–305). Mahwah, NJ: Lawrence Erlbaum Associates, Inc. Google Scholar
- Sandoval, W. ( 2014 ). Conjecture mapping: An approach to systematic educational design research . Journal of the Learning Sciences , 23 (1), 18–36. Google Scholar
- Sandoval, W. A., & Bell, P. ( 2004 ). Design-based research methods for studying learning in context: Introduction . Educational Psychologist , 39 (4), 199–201. Google Scholar
- Schinske, J. N., Balke, V. L., Bangera, M. G., Bonney, K. M., Brownell, S. E., Carter, R. S. , … & Corwin, L. A. ( 2017 ). Broadening participation in biology education research: Engaging community college students and faculty . CBE—Life Sciences Education , 16 (2), mr1. Link , Google Scholar
- Scott, E., Anderson, C. W., Mashood, K. K., Matz, R. L., Underwood, S. M., & Sawtelle, V. ( 2018 ). Developing an analytical framework to characterize student reasoning about complex processes . CBE—Life Sciences Education , 17 (3), ar49. https://doi.org/10.1187/cbe.17-10-0225 Link , Google Scholar
- Scott, E., Wenderoth, M. P., & Doherty, J. H. ( 2019 ). Learning progressions: An empirically grounded, learner-centered framework to guide biology instruction . CBE—Life Sciences Education , 18 (4), es5. https://doi.org/10.1187/cbe.19-03-0059 Link , Google Scholar
- Sharma, M. D., & McShane, K. ( 2008 ). A methodological framework for understanding and describing discipline-based scholarship of teaching in higher education through design-based research . Higher Education Research & Development , 27 (3), 257–270. Google Scholar
- Shavelson, R. J., Phillips, D. C., Towne, L., & Feuer, M. J. ( 2003 ). On the science of education design studies . Educational Researcher , 32 (1), 25–28. Google Scholar
- Siegler, R. S. ( 2006 ). Microgenetic analyses of learning . In Damon, W.Lerner, R. M. (Eds.), Handbook of child psychology (pp. 464–510). Hoboken, NJ: John Wiley & Sons, Inc. https://doi.org/10.1002/9780470147658.chpsy0211 Google Scholar
- Stringer, E. T. ( 2013 ). Action research . Thousand Oaks, CA: Sage Publications, Inc. Google Scholar
- Subramaniam, M., Jean, B. S., Taylor, N. G., Kodama, C., Follman, R., & Casciotti, D. ( 2015 ). Bit by bit: Using design-based research to improve the health literacy of adolescents . JMIR Research Protocols , 4 (2), e62. Medline , Google Scholar
- Summers, M. M., Couch, B. A., Knight, J. K., Brownell, S. E., Crowe, A. J., Semsar, K. , … & Batzli, J. ( 2018 ). EcoEvo-MAPS: An ecology and evolution assessment for introductory through advanced undergraduates . CBE—Life Sciences Education , 17 (2), ar18. Link , Google Scholar
- Szteinberg, G., Balicki, S., Banks, G., Clinchot, M., Cullipher, S., Huie, R. , … & Sevian, H. ( 2014 ). Collaborative professional development in chemistry education research: Bridging the gap between research and practice . Journal of Chemical Education , 91 (9), 1401–1408. Google Scholar
- Talanquer, V. ( 2014 ). DBER and STEM education reform: Are we up to the challenge? Journal of Research in Science Teaching , 51 (6), 809–819. Google Scholar
- Wang, F., & Hannafin, M. J. ( 2005 ). Design-based research and technology-enhanced learning environments . Educational Technology Research and Development , 53 (4), 5–23. Google Scholar
- Wang, J.-R. ( 2004 ). Development and validation of a Two-tier instrument to examine understanding of internal transport in plants and the human circulatory system . International Journal of Science and Mathematics Education , 2 (2), 131–157. Google Scholar
- Warfa, A.-R. M. ( 2016 ). Mixed-methods design in biology education research: Approach and uses . CBE—Life Sciences Education , 15 (4), rm5. Link , Google Scholar
- Windschitl, M., Thompson, J., Braaten, M., & Stroupe, D. ( 2012 ). Proposing a core set of instructional practices and tools for teachers of science . Science Education , 96 (5), 878–903. Google Scholar
- Zagallo, P., Meddleton, S., & Bolger, M. S. ( 2016 ). Teaching real data interpretation with models (TRIM): Analysis of student dialogue in a large-enrollment cell and developmental biology course . CBE—Life Sciences Education , 15 (2), ar17. Link , Google Scholar
- Codéveloppement d’un programme d’autogestion de la douleur chronique en ligne: un projet de recherche basé sur la conception et axé sur l’engagement des patients 12 March 2024 | Canadian Journal of Pain, Vol. 8, No. 1
- Enhancing undergraduates’ engagement in a learning community by including their voices in the technological and instructional design Computers & Education, Vol. 214
- Practice-Based Teacher Education Benefits Graduate Trainees and Their Students Through Inclusive and Active Teaching Methods 16 October 2023 | Journal for STEM Education Research, Vol. 7, No. 1
- Leveraging learning experience design: digital media approaches to influence motivational traits that support student learning behaviors in undergraduate online courses 11 October 2022 | Journal of Computing in Higher Education, Vol. 35, No. 3
- Investigating an Assessment Design that Prevents Students from Using ChatGPT as the Sole Basis to Pass Assessment at the Tertiary Level 30 November 2023 | E-Journal of Humanities, Arts and Social Sciences
- Spatial Variations in Aquatic Insect Community Structure in the Winam Gulf of Lake Victoria, Kenya International Journal of Ecology, Vol. 2023
- The Perceived Effectiveness of Various Forms of Feedback on the Acquisition of Technical Skills by Advanced Learners in Simulation-Based Health Professions Education Cureus, Vol. 44
- Occupational therapists' acceptance of 3D printing 22 August 2023 | South African Journal of Occupational Therapy, Vol. 53, No. 2
- An app by students for students – the DPaCK-model for a digital collaborative teamwork project to identify butterflies 4 August 2023 | Frontiers in Education, Vol. 8
- Applying DBR to design protocols for synchronous online Chinese learning: An activity theoretic perspective System, Vol. 116
- Defining the Nature of Augmented Feedback for Learning Intraosseous Access Skills in Simulation-Based Health Professions Education Cureus, Vol. 86
- Practice-based 21st-century teacher education: Design principles for adaptive expertise Teaching and Teacher Education, Vol. 128
- Undergraduate students’ neurophysiological reasoning: what we learn from the attractive distractors students select Advances in Physiology Education, Vol. 47, No. 2
- Oaks to arteries: the Physiology Core Concept of flow down gradients supports transfer of student reasoning Advances in Physiology Education, Vol. 47, No. 2
- Audrey Chen ,
- Kimberley A. Phillips ,
- Jennifer E. Schaefer , and
- Patrick M. Sonner
- Kyle Frantz,, Monitoring Editor
- Optimizing the Learner’s Role in Feedback: Development of a Feedback-Preparedness Online Application for Medical Students in the Clinical Setting Cureus, Vol. 42
- History, Status, and Development of AI-Based Learning Science 8 April 2023 | SN Computer Science, Vol. 4, No. 3
- An Analytical Dashboard of Collaborative Activities for the Knowledge Building 4 March 2023 | Technology, Knowledge and Learning, Vol. 29
- The Application of a Design-Based Research Framework for Simulation-Based Education Cureus, Vol. 22
- Erin Stanfield ,
- Corin D. Slown ,
- Quentin Sedlacek , and
- Suzanne E. Worcester
- James Hewlett, Monitoring Editor
- The effect of the e-mentoring-based education program on professional development of preschool teachers 3 July 2021 | Education and Information Technologies, Vol. 27, No. 1
- Education Sciences, Vol. 12, No. 8
- Training Digital Competences of Educators in Continuing Education: A Three-Level Approach 27 October 2022
- Design-based research as a framework for developing and deploying augmented reality applications and scenarios for intercultural exchange 13 December 2021
- Repetition Is Important to Students and Their Understanding during Laboratory Courses That Include Research Journal of Microbiology & Biology Education, Vol. 22, No. 2
- Another look at the core concepts of physiology: revisions and resources Advances in Physiology Education, Vol. 44, No. 4

An official website of the United States government
The .gov means it’s official. Federal government websites often end in .gov or .mil. Before sharing sensitive information, make sure you’re on a federal government site.
The site is secure. The https:// ensures that you are connecting to the official website and that any information you provide is encrypted and transmitted securely.
- Publications
- Account settings
Preview improvements coming to the PMC website in October 2024. Learn More or Try it out now .
- Advanced Search
- Journal List
- CBE Life Sci Educ
- v.19(3); Fall 2020
Design-Based Research: A Methodology to Extend and Enrich Biology Education Research
Emily e. scott.
† Department of Biology, University of Washington, Seattle, WA 98195
Mary Pat Wenderoth
Jennifer h. doherty.
Recent calls in biology education research (BER) have recommended that researchers leverage learning theories and methodologies from other disciplines to investigate the mechanisms by which students to develop sophisticated ideas. We suggest design-based research from the learning sciences is a compelling methodology for achieving this aim. Design-based research investigates the “learning ecologies” that move student thinking toward mastery. These “learning ecologies” are grounded in theories of learning, produce measurable changes in student learning, generate design principles that guide the development of instructional tools, and are enacted using extended, iterative teaching experiments. In this essay, we introduce readers to the key elements of design-based research, using our own research into student learning in undergraduate physiology as an example of design-based research in BER. Then, we discuss how design-based research can extend work already done in BER and foster interdisciplinary collaborations among cognitive and learning scientists, biology education researchers, and instructors. We also explore some of the challenges associated with this methodological approach.
INTRODUCTION
There have been recent calls for biology education researchers to look toward other fields of educational inquiry for theories and methodologies to advance, and expand, our understanding of what helps students learn to think like biologists ( Coley and Tanner, 2012 ; Dolan, 2015 ; Peffer and Renken, 2016 ; Lo et al. , 2019 ). These calls include the recommendations that biology education researchers ground their work in learning theories from the cognitive and learning sciences ( Coley and Tanner, 2012 ) and begin investigating the underlying mechanisms by which students to develop sophisticated biology ideas ( Dolan, 2015 ; Lo et al. , 2019 ). Design-based research from the learning sciences is one methodology that seeks to do both by using theories of learning to investigate how “learning ecologies”—that is, complex systems of interactions among instructors, students, and environmental components—support the process of student learning ( Brown, 1992 ; Cobb et al. , 2003 ; Collins et al. , 2004 ; Peffer and Renken, 2016 ).
The purpose of this essay is twofold. First, we want to introduce readers to the key elements of design-based research, using our research into student learning in undergraduate physiology as an example of design-based research in biology education research (BER). Second, we will discuss how design-based research can extend work already done in BER and explore some of the challenges of its implementation. For a more in-depth review of design-based research, we direct readers to the following references: Brown (1992) , Barab and Squire (2004) , and Collins et al. (2004) , as well as commentaries by Anderson and Shattuck (2012) and McKenney and Reeves (2013) .
WHAT IS DESIGN-BASED RESEARCH?
Design-based research is a methodological approach that aligns with research methods from the fields of engineering or applied physics, where products are designed for specific purposes ( Brown, 1992 ; Joseph, 2004 ; Middleton et al. , 2008 ; Kelly, 2014 ). Consequently, investigators using design-based research approach educational inquiry much as an engineer develops a new product: First, the researchers identify a problem that needs to be addressed (e.g., a particular learning challenge that students face). Next, they design a potential “solution” to the problem in the form of instructional tools (e.g., reasoning strategies, worksheets; e.g., Reiser et al. , 2001 ) that theory and previous research suggest will address the problem. Then, the researchers test the instructional tools in a real-world setting (i.e., the classroom) to see if the tools positively impact student learning. As testing proceeds, researchers evaluate the instructional tools with emerging evidence of their effectiveness (or lack thereof) and progressively revise the tools— in real time —as necessary ( Collins et al. , 2004 ). Finally, the researchers reflect on the outcomes of the experiment, identifying the features of the instructional tools that were successful at addressing the initial learning problem, revising those aspects that were not helpful to learning, and determining how the research informed the theory underlying the experiment. This leads to another research cycle of designing, testing, evaluating, and reflecting to refine the instructional tools in support of student learning. We have characterized this iterative process in Figure 1 after Sandoval (2014) . Though we have portrayed four discrete phases to design-based research, there is often overlap of the phases as the research progresses (e.g., testing and evaluating can occur simultaneously).

The four phases of design-based research experienced in an iterative cycle (A). We also highlight the main features of each phase of our design-based research project investigating students’ use of flux in physiology (B).
Design-based research has no specific requirements for the form that instructional tools must take or the manner in which the tools are evaluated ( Bell, 2004 ; Anderson and Shattuck, 2012 ). Instead, design-based research has what Sandoval (2014) calls “epistemic commitments” 1 that inform the major goals of a design-based research project as well as how it is implemented. These epistemic commitments are: 1) Design based research should be grounded in theories of learning (e.g., constructivism, knowledge-in-pieces, conceptual change) that both inform the design of the instructional tools and are improved upon by the research ( Cobb et al. , 2003 ; Barab and Squire, 2004 ). This makes design-based research more than a method for testing whether or not an instructional tool works; it also investigates why the design worked and how it can be generalized to other learning environments ( Cobb et al. , 2003 ). 2) Design-based research should aim to produce measurable changes in student learning in classrooms around a particular learning problem ( Anderson and Shattuck, 2012 ; McKenney and Reeves, 2013 ). This requirement ensures that theoretical research into student learning is directly applicable, and impactful, to students and instructors in classroom settings ( Hoadley, 2004 ). 3) Design-based research should generate design principles that guide the development and implementation of future instructional tools ( Edelson, 2002 ). This commitment makes the research findings broadly applicable for use in a variety of classroom environments. 4) Design-based research should be enacted using extended, iterative teaching experiments in classrooms. By observing student learning over an extended period of time (e.g., throughout an entire term or across terms), researchers are more likely to observe the full effects of how the instructional tools impact student learning compared with short-term experiments ( Brown, 1992 ; Barab and Squire, 2004 ; Sandoval and Bell, 2004 ).
HOW IS DESIGN-BASED RESEARCH DIFFERENT FROM AN EXPERIMENTAL APPROACH?
Many BER studies employ experimental approaches that align with traditional scientific methods of experimentation, such as using treatment versus control groups, randomly assigning treatments to different groups, replicating interventions across multiple spatial or temporal periods, and using statistical methods to guide the kinds of inferences that arise from an experiment. While design-based research can similarly employ these strategies for educational inquiry, there are also some notable differences in its approach to experimentation ( Collins et al. , 2004 ; Hoadley, 2004 ). In this section, we contrast the differences between design-based research and what we call “experimental approaches,” although both paradigms represent a form of experimentation.
The first difference between an experimental approach and design-based research regards the role participants play in the experiment. In an experimental approach, the researcher is responsible for making all the decisions about how the experiment will be implemented and analyzed, while the instructor facilitates the experimental treatments. In design-based research, both researchers and instructors are engaged in all stages of the research from conception to reflection ( Collins et al. , 2004 ). In BER, a third condition frequently arises wherein the researcher is also the instructor. In this case, if the research questions being investigated produce generalizable results that have the potential to impact teaching broadly, then this is consistent with a design-based research approach ( Cobb et al. , 2003 ). However, when the research questions are self-reflective about how a researcher/instructor can improve his or her own classroom practices, this aligns more closely with “action research,” which is another methodology used in education research (see Stringer, 2013 ).
A second difference between experimental research and design-based research is the form that hypotheses take and the manner in which they are investigated ( Collins et al. , 2004 ; Sandoval, 2014 ). In experimental approaches, researchers develop a hypothesis about how a specific instructional intervention will impact student learning. The intervention is then tested in the classroom(s) while controlling for other variables that are not part of the study in order to isolate the effects of the intervention. Sometimes, researchers designate a “control” situation that serves as a comparison group that does not experience the intervention. For example, Jackson et al. (2018) were interested in comparing peer- and self-grading of weekly practice exams to if they were equally effective forms of deliberate practice for students in a large-enrollment class. To test this, the authors (including authors of this essay J.H.D., M.P.W.) designed an experiment in which lab sections of students in a large lecture course were randomly assigned to either a peer-grading or self-grading treatment so they could isolate the effects of each intervention. In design-based research, a hypothesis is conceptualized as the “design solution” rather than a specific intervention; that is, design-based researchers hypothesize that the designed instructional tools, when implemented in the classroom, will create a learning ecology that improves student learning around the identified learning problem ( Edelson, 2002 ; Bell, 2004 ). For example, Zagallo et al. (2016) developed a laboratory curriculum (i.e., the hypothesized “design solution”) for molecular and cellular biology majors to address the learning problem that students often struggle to connect scientific models and empirical data. This curriculum entailed: focusing instruction around a set of target biological models; developing small-group activities in which students interacted with the models by analyzing data from scientific papers; using formative assessment tools for student feedback; and providing students with a set of learning objectives they could use as study tools. They tested their curriculum in a novel, large-enrollment course of upper-division students over several years, making iterative changes to the curriculum as the study progressed.
By framing the research approach as an iterative endeavor of progressive refinement rather than a test of a particular intervention when all other variables are controlled, design-based researchers recognize that: 1) classrooms, and classroom experiences, are unique at any given time, making it difficult to truly “control” the environment in which an intervention occurs or establish a “control group” that differs only in the features of an intervention; and 2) many aspects of a classroom experience may influence the effectiveness of an intervention, often in unanticipated ways, which should be included in the research team’s analysis of an intervention’s success. Consequently, the research team is less concerned with controlling the research conditions—as in an experimental approach—and instead focuses on characterizing the learning environment ( Barab and Squire, 2004 ). This involves collecting data from multiple sources as the research progresses, including how the instructional tools were implemented, aspects of the implementation process that failed to go as planned, and how the instructional tools or implementation process was modified. These characterizations can provide important insights into what specific features of the instructional tools, or the learning environment, were most impactful to learning ( DBR Collective, 2003 ).
A third difference between experimental approaches and design-based research is when the instructional interventions can be modified. In experimental research, the intervention is fixed throughout the experimental period, with any revisions occurring only after the experiment has concluded. This is critical for ensuring that the results of the study provide evidence of the efficacy of a specific intervention. By contrast, design-based research takes a more flexible approach that allows instructional tools to be modified in situ as they are being implemented ( Hoadley, 2004 ; Barab, 2014 ). This flexibility allows the research team to modify instructional tools or strategies that prove inadequate for collecting the evidence necessary to evaluate the underlying theory and ensures a tight connection between interventions and a specific learning problem ( Collins et al. , 2004 ; Hoadley, 2004 ).
Finally, and importantly, experimental approaches and design-based research differ in the kinds of conclusions they draw from their data. Experimental research can “identify that something meaningful happened; but [it is] not able to articulate what about the intervention caused that story to unfold” ( Barab, 2014 , p. 162). In other words, experimental methods are robust for identifying where differences in learning occur, such as between groups of students experiencing peer- or self-grading of practice exams ( Jackson et al. , 2018 ) or receiving different curricula (e.g., Chi et al. , 2012 ). However, these methods are not able to characterize the underlying learning process or mechanism involved in the different learning outcomes. By contrast, design-based research has the potential to uncover mechanisms of learning, because it investigates how the nature of student thinking changes as students experience instructional interventions ( Shavelson et al. , 2003 ; Barab, 2014 ). According to Sandoval (2014) , “Design research, as a means of uncovering causal processes, is oriented not to finding effects but to finding functions , to understanding how desired (and undesired) effects arise through interactions in a designed environment” (p. 30). In Zagallo et al. (2016) , the authors found that their curriculum supported students’ data-interpretation skills, because it stimulated students’ spontaneous use of argumentation during which group members coconstructed evidence-based claims from the data provided. Students also worked collaboratively to decode figures and identify data patterns. These strategies were identified from the researchers’ qualitative data analysis of in-class recordings of small-group discussions, which allowed them to observe what students were doing to support their learning. Because design-based research is focused on characterizing how learning occurs in classrooms, it can begin to answer the kinds of mechanistic questions others have identified as central to advancing BER ( National Research Council [NRC], 2012 ; Dolan, 2015 ; Lo et al. , 2019 ).
DESIGN-BASED RESEARCH IN ACTION: AN EXAMPLE FROM UNDERGRADUATE PHYSIOLOGY
To illustrate how design-based research could be employed in BER, we draw on our own research that investigates how students learn physiology. We will characterize one iteration of our design-based research cycle ( Figure 1 ), emphasizing how our project uses Sandoval’s four epistemic commitments (i.e., theory driven, practically applied, generating design principles, implemented in an iterative manner) to guide our implementation.
Identifying the Learning Problem
Understanding physiological phenomena is challenging for students, given the wide variety of contexts (e.g., cardiovascular, neuromuscular, respiratory; animal vs. plant) and scales involved (e.g., using molecular-level interactions to explain organism functioning; Wang, 2004 ; Michael, 2007 ; Badenhorst et al. , 2016 ). To address these learning challenges, Modell (2000) identified seven “general models” that undergird most physiology phenomena (i.e., control systems, conservation of mass, mass and heat flow, elastic properties of tissues, transport across membranes, cell-to-cell communication, molecular interactions). Instructors can use these models as a “conceptual framework” to help students build intellectual coherence across phenomena and develop a deeper understanding of physiology ( Modell, 2000 ; Michael et al. , 2009 ). This approach aligns with theoretical work in the learning sciences that indicates that providing students with conceptual frameworks improves their ability to integrate and retrieve knowledge ( National Academies of Sciences, Engineering, and Medicine, 2018 ).
Before the start of our design-based project, we had been using Modell’s (2000) general models to guide our instruction. In this essay, we will focus on how we used the general models of mass and heat flow and transport across membranes in our instruction. These two models together describe how materials flow down gradients (e.g., pressure gradients, electrochemical gradients) against sources of resistance (e.g., tube diameter, channel frequency). We call this flux reasoning. We emphasized the fundamental nature and broad utility of flux reasoning in lecture and lab and frequently highlighted when it could be applied to explain a phenomenon. We also developed a conceptual scaffold (the Flux Reasoning Tool) that students could use to reason about physiological processes involving flux.
Although these instructional approaches had improved students’ understanding of flux phenomena, we found that students often demonstrated little commitment to using flux broadly across physiological contexts. Instead, they considered flux to be just another fact to memorize and applied it to narrow circumstances (e.g., they would use flux to reason about ions flowing across membranes—the context where flux was first introduced—but not the bulk flow of blood in a vessel). Students also struggled to integrate the various components of flux (e.g., balancing chemical and electrical gradients, accounting for variable resistance). We saw these issues reflected in students’ lower than hoped for exam scores on the cumulative final of the course. From these experiences, and from conversations with other physiology instructors, we identified a learning problem to address through design-based research: How do students learn to use flux reasoning to explain material flows in multiple physiology contexts?
The process of identifying a learning problem usually emerges from a researcher’s own experiences (in or outside a classroom) or from previous research that has been described in the literature ( Cobb et al. , 2003 ). To remain true to Sandoval’s first epistemic commitment, a learning problem must advance a theory of learning ( Edelson, 2002 ; McKenney and Reeves, 2013 ). In our work, we investigated how conceptual frameworks based on fundamental scientific concepts (i.e., Modell’s general models) could help students reason productively about physiology phenomena (National Academies of Sciences, Engineering, and Medicine, 2018; Modell, 2000 ). Our specific theoretical question was: Can we characterize how students’ conceptual frameworks around flux change as they work toward robust ideas? Sandoval’s second epistemic commitment stated that a learning problem must aim to improve student learning outcomes. The practical significance of our learning problem was: Does using the concept of flux as a foundational idea for instructional tools increase students’ learning of physiological phenomena?
We investigated our learning problem in an introductory biology course at a large R1 institution. The introductory course is the third in a biology sequence that focuses on plant and animal physiology. The course typically serves between 250 and 600 students in their sophomore or junior years each term. Classes have the following average demographics: 68% male, 21% from lower-income situations, 12% from an underrepresented minority, and 26% first-generation college students.
Design-Based Research Cycle 1, Phase 1: Designing Instructional Tools
The first phase of design-based research involves developing instructional tools that address both the theoretical and practical concerns of the learning problem ( Edelson, 2002 ; Wang and Hannafin, 2005 ). These instructional tools can take many forms, such as specific instructional strategies, classroom worksheets and practices, or technological software, as long as they embody the underlying learning theory being investigated. They must also produce classroom experiences or materials that can be evaluated to determine whether learning outcomes were met ( Sandoval, 2014 ). Indeed, this alignment between theory, the nature of the instructional tools, and the ways students are assessed is central to ensuring rigorous design-based research ( Hoadley, 2004 ; Sandoval, 2014 ). Taken together, the instructional tools instantiate a hypothesized learning environment that will advance both the theoretical and practical questions driving the research ( Barab, 2014 ).
In our work, the theoretical claim that instruction based on fundamental scientific concepts would support students’ flux reasoning was embodied in our instructional approach by being the central focus of all instructional materials, which included: a revised version of the Flux Reasoning Tool ( Figure 2 ); case study–based units in lecture that explicitly emphasized flux phenomena in real-world contexts ( Windschitl et al. , 2012 ; Scott et al. , 2018 ; Figure 3 ); classroom activities in which students practiced using flux to address physiological scenarios; links to online videos describing key flux-related concepts; constructed-response assessment items that cued students to use flux reasoning in their thinking; and pretest/posttest formative assessment questions that tracked student learning ( Figure 4 ).

The Flux Reasoning Tool given to students at the beginning of the quarter.

An example flux case study that is presented to students at the beginning of the neurophysiology unit. Throughout the unit, students learn how ion flows into and out of cells, as mediated by chemical and electrical gradients and various ion/molecular channels, sends signals throughout the body. They use this information to better understand why Jaime experiences persistent neuropathy. Images from: uz.wikipedia.org/wiki/Fayl:Blausen_0822_SpinalCord.png and commons.wikimedia.org/wiki/File:Figure_38_01_07.jpg.

An example flux assessment question about ion flows given in a pre-unit/post-unit formative assessment in the neurophysiology unit.
Phase 2: Testing the Instructional Tools
In the second phase of design-based research, the instructional tools are tested by implementing them in classrooms. During this phase, the instructional tools are placed “in harm’s way … in order to expose the details of the process to scrutiny” ( Cobb et al. , 2003 , p. 10). In this way, researchers and instructors test how the tools perform in real-world settings, which may differ considerably from the design team’s initial expectations ( Hoadley, 2004 ). During this phase, if necessary, the design team may make adjustments to the tools as they are being used to account for these unanticipated conditions ( Collins et al. , 2004 ).
We implemented the instructional tools during the Autumn and Spring quarters of the 2016–2017 academic year. Students were taught to use the Flux Reasoning Tool at the beginning of the term in the context of the first case study unit focused on neurophysiology. Each physiology unit throughout the term was associated with a new concept-based case study (usually about flux) that framed the context of the teaching. Embedded within the daily lectures were classroom activities in which students could practice using flux. Students were also assigned readings from the textbook and videos related to flux to watch during each unit. Throughout the term, students took five exams that each contained some flux questions as well as some pre- and post-unit formative assessment questions. During Winter quarter, we conducted clinical interviews with students who would take our course in the Spring term (i.e., “pre” data) as well as students who had just completed our course in Autumn (i.e., “post” data).
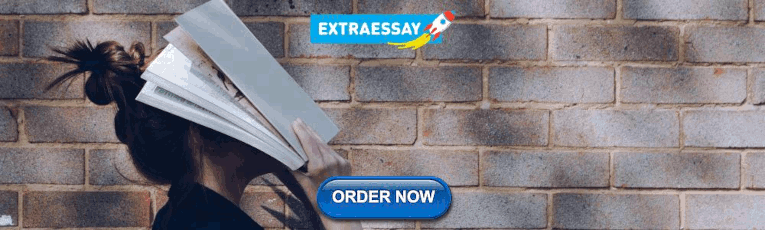
Phase 3: Evaluating the Instructional Tools
The third phase of a design-based research cycle involves evaluating the effectiveness of instructional tools using evidence of student learning ( Barab and Squire, 2004 ; Anderson and Shattuck, 2012 ). This can be done using products produced by students (e.g., homework, lab reports), attitudinal gains measured with surveys, participation rates in activities, interview testimonials, classroom discourse practices, and formative assessment or exam data (e.g., Reiser et al. , 2001 ; Cobb et al. , 2003 ; Barab and Squire, 2004 ; Mohan et al. , 2009 ). Regardless of the source, evidence must be in a form that supports a systematic analysis that could be scrutinized by other researchers ( Cobb et al. , 2003 ; Barab, 2014 ). Also, because design-based research often involves multiple data streams, researchers may need to use both quantitative and qualitative analytical methods to produce a rich picture of how the instructional tools affected student learning ( Collins et al. , 2004 ; Anderson and Shattuck, 2012 ).
In our work, we used the quality of students’ written responses on exams and formative assessment questions to determine whether students improved their understanding of physiological phenomena involving flux. For each assessment question, we analyzed a subset of student’s pretest answers to identify overarching patterns in students’ reasoning about flux, characterized these overarching patterns, then ordinated the patterns into different levels of sophistication. These became our scoring rubrics, which identified five different levels of student reasoning about flux. We used the rubrics to code the remainder of students’ responses, with a code designating the level of student reasoning associated with a particular reasoning pattern. We used this ordinal rubric format because it would later inform our theoretical understanding of how students build flux conceptual frameworks (see phase 4). This also allowed us to both characterize the ideas students held about flux phenomena and identify the frequency distribution of those ideas in a class.
By analyzing changes in the frequency distributions of students’ ideas across the rubric levels at different time points in the term (e.g., pre-unit vs. post-unit), we could track both the number of students who gained more sophisticated ideas about flux as the term progressed and the quality of those ideas. If the frequency of students reasoning at higher levels increased from pre-unit to post-unit assessments, we could conclude that our instructional tools as a whole were supporting students’ development of sophisticated flux ideas. For example, on one neuromuscular ion flux assessment question in the Spring of 2017, we found that relatively more students were reasoning at the highest levels of our rubric (i.e., levels 4 and 5) on the post-unit test compared with the pre-unit test. This meant that more students were beginning to integrate sophisticated ideas about flux (i.e., they were balancing concentration and electrical gradients) in their reasoning about ion movement.
To help validate this finding, we drew on three additional data streams: 1) from in-class group recordings of students working with flux items, we noted that students increasingly incorporated ideas about gradients and resistance when constructing their explanations as the term progressed; 2) from plant assessment items in the latter part of the term, we began to see students using flux ideas unprompted; and 3) from interviews, we observed that students who had already taken the course used flux ideas in their reasoning.
Through these analyses, we also noticed an interesting pattern in the pre-unit test data for Spring 2017 when compared with the frequency distribution of students’ responses with a previous term (Autumn 2016). In Spring 2017, 42% of students reasoned at level 4 or 5 on the pre-unit test, indicating these students already had sophisticated ideas about ion flux before they took the pre-unit assessment. This was surprising, considering only 2% of students reasoned at these levels for this item on the Autumn 2016 pre-unit test.
Phase 4: Reflecting on the Instructional Tools and Their Implementation
The final phase of a design-based research cycle involves a retrospective analysis that addresses the epistemic commitments of this methodology: How was the theory underpinning the research advanced by the research endeavor (theoretical outcome)? Did the instructional tools support student learning about the learning problem (practical outcome)? What were the critical features of the design solution that supported student learning (design principles)? ( Cobb et al. , 2003 ; Barab and Squire, 2004 ).
Theoretical Outcome (Epistemic Commitment 1).
Reflecting on how a design-based research experiment advances theory is critical to our understanding of how students learn in educational settings ( Barab and Squire, 2004 ; Mohan et al. , 2009 ). In our work, we aimed to characterize how students’ conceptual frameworks around flux change as they work toward robust ideas. To do this, we drew on learning progression research as our theoretical framing ( NRC, 2007 ; Corcoran et al. , 2009 ; Duschl et al. , 2011 ; Scott et al. , 2019 ). Learning progression frameworks describe empirically derived patterns in student thinking that are ordered into levels representing cognitive shifts in the ways students conceive a topic as they work toward mastery ( Gunckel et al. , 2012 ). We used our ion flux scoring rubrics to create a preliminary five-level learning progression framework ( Table 1 ). The framework describes how students’ ideas about flux often start with teleological-driven accounts at the lowest level (i.e., level 1), shift to focusing on driving forces (e.g., concentration gradients, electrical gradients) in the middle levels, and arrive at complex ideas that integrate multiple interacting forces at the higher levels. We further validated these reasoning patterns with our student interviews. However, our flux conceptual framework was largely based on student responses to our ion flux assessment items. Therefore, to further validate our learning progression framework, we needed a greater diversity of flux assessment items that investigated student thinking more broadly (i.e., about bulk flow, water movement) across physiological systems.
The preliminary flux learning progression framework characterizing the patterns of reasoning students may exhibit as they work toward mastery of flux reasoning. The student exemplars are from the ion flux formative assessment question presented in Figure 4 . The “/” divides a student’s answers to the first and second parts of the question. Level 5 represents the most sophisticated ideas about flux phenomena.
Practical Outcome (Epistemic Commitment 2).
In design-based research, learning theories must “do real work” by improving student learning in real-world settings ( DBR Collective, 2003 ). Therefore, design-based researchers must reflect on whether or not the data they collected show evidence that the instructional tools improved student learning ( Cobb et al. , 2003 ; Sharma and McShane, 2008 ). We determined whether our flux-based instructional approach aided student learning by analyzing the kinds of answers students provided to our assessment questions. Specifically, we considered students who reasoned at level 4 or above as demonstrating productive flux reasoning. Because almost half of students were reasoning at level 4 or 5 on the post-unit assessment after experiencing the instructional tools in the neurophysiology unit (in Spring 2017), we concluded that our tools supported student learning in physiology. Additionally, we noticed that students used language in their explanations that directly tied to the Flux Reasoning Tool ( Figure 2 ), which instructed them to use arrows to indicate the magnitude and direction of gradient-driving forces. For example, in a posttest response to our ion flux item ( Figure 4 ), one student wrote:
Ion movement is a function of concentration and electrical gradients . Which arrow is stronger determines the movement of K+. We can make the electrical arrow bigger and pointing in by making the membrane potential more negative than Ek [i.e., potassium’s equilibrium potential]. We can make the concentration arrow bigger and pointing in by making a very strong concentration gradient pointing in.
Given that almost half of students reasoned at level 4 or above, and that students used language from the Flux Reasoning Tool, we concluded that using fundamental concepts was a productive instructional approach for improving student learning in physiology and that our instructional tools aided student learning. However, some students in the 2016–2017 academic year continued to apply flux ideas more narrowly than intended (i.e., for ion and simple diffusion cases, but not water flux or bulk flow). This suggested that students had developed nascent flux conceptual frameworks after experiencing the instructional tools but could use more support to realize the broad applicability of this principle. Also, although our cross-sectional interview approach demonstrated how students’ ideas, overall, could change after experiencing the instructional tools, it did not provide information about how a student developed flux reasoning.
Reflecting on practical outcomes also means interpreting any learning gains in the context of the learning ecology. This reflection allowed us to identify whether there were particular aspects of the instructional tools that were better at supporting learning than others ( DBR Collective, 2003 ). Indeed, this was critical for our understanding why 42% of students scored at level 3 and above on the pre-unit ion assessment in the Spring of 2017, while only 2% of students scored level 3 and above in Autumn of 2016. When we reviewed notes of the Spring 2017 implementation scheme, we saw that the pretest was due at the end of the first day of class after students had been exposed to ion flux ideas in class and in a reading/video assignment about ion flow, which may be one reason for the students’ high performance on the pretest. Consequently, we could not tell whether students’ initial high performance was due to their learning from the activities in the first day of class or for other reasons we did not measure. It also indicated we needed to close pretests before the first day of class for a more accurate measure of students’ incoming ideas and the effectiveness of the instructional tools employed at the beginning of the unit.
Design Principles (Epistemic Commitment 3).
Although design-based research is enacted in local contexts (i.e., a particular classroom), its purpose is to inform learning ecologies that have broad applications to improve learning and teaching ( Edelson, 2002 ; Cobb et al. , 2003 ). Therefore, design-based research should produce design principles that describe characteristics of learning environments that researchers and instructors can use to develop instructional tools specific to their local contexts (e.g., Edelson, 2002 ; Subramaniam et al. , 2015 ). Consequently, the design principles must balance specificity with adaptability so they can be used broadly to inform instruction ( Collins et al. , 2004 ; Barab, 2014 ).
From our first cycle of design-based research, we developed the following design principles: 1) Key scientific concepts should provide an overarching framework for course organization. This way, the individual components that make up a course, like instructional units, activities, practice problems, and assessments, all reinforce the centrality of the key concept. 2) Instructional tools should explicitly articulate the principle of interest, with specific guidance on how that principle is applied in context. This stresses the applied nature of the principle and that it is more than a fact to be memorized. 3) Instructional tools need to show specific instances of how the principle is applied in multiple contexts to combat students’ narrow application of the principle to a limited number of contexts.
Design-Based Research Cycle 2, Phase 1: Redesign and Refine the Experiment
The last “epistemic commitment” Sandoval (2014) articulated was that design-based research be an iterative process with an eye toward continually refining the instructional tools, based on evidence of student learning, to produce more robust learning environments. By viewing educational inquiry as formative research, design-based researchers recognize the difficulty in accounting for all variables that could impact student learning, or the implementation of the instructional tools, a priori ( Collins et al. , 2004 ). Robust instructional designs are the products of trial and error, which are strengthened by a systematic analysis of how they perform in real-world settings.
To continue to advance our work investigating student thinking using the principle of flux, we began a second cycle of design-based research that continued to address the learning problem of helping students reason with fundamental scientific concepts. In this cycle, we largely focused on broadening the number of physiological systems that had accompanying formative assessment questions (i.e., beyond ion flux), collecting student reasoning from a more diverse population of students (e.g., upper division, allied heath, community college), and refining and validating the flux learning progression with both written and interview data in a student through time. We developed a suite of constructed-response flux assessment questions that spanned neuromuscular, cardiovascular, respiratory, renal, and plant physiological contexts and asked students about several kinds of flux: ion movement, diffusion, water movement, and bulk flow (29 total questions; available at beyondmultiplechoice.org). This would provide us with rich qualitative data that we could use to refine the learning progression. We decided to administer written assessments and conduct interviews in a pretest/posttest manner at the beginning and end of each unit both as a way to increase our data about student reasoning and to provide students with additional practice using flux reasoning across contexts.
From this second round of designing instructional tools (i.e., broader range of assessment items), testing them in the classroom (i.e., administering the assessment items to diverse student populations), evaluating the tools (i.e., developing learning progression–aligned rubrics across phenomena from student data, tracking changes in the frequency distribution of students across levels through time), and reflecting on the tools’ success, we would develop a more thorough and robust characterization of how students use flux across systems that could better inform our creation of new instructional tools to support student learning.
HOW CAN DESIGN-BASED RESEARCH EXTEND AND ENRICH BER?
While design-based research has primarily been used in educational inquiry at the K–12 level (see Reiser et al. , 2001 ; Mohan et al. , 2009 ; Jin and Anderson, 2012 ), other science disciplines at undergraduate institutions have begun to employ this methodology to create robust instructional approaches (e.g., Szteinberg et al. , 2014 in chemistry; Hake, 2007 , and Sharma and McShane, 2008 , in physics; Kelly, 2014 , in engineering). Our own work, as well as that by Zagallo et al. (2016) , provides two examples of how design-based research could be implemented in BER. Below, we articulate some of the ways incorporating design-based research into BER could extend and enrich this field of educational inquiry.
Design-Based Research Connects Theory with Practice
One critique of BER is that it does not draw heavily enough on learning theories from other disciplines like cognitive psychology or the learning sciences to inform its research ( Coley and Tanner, 2012 ; Dolan, 2015 ; Peffer and Renken, 2016 ; Davidesco and Milne, 2019 ). For example, there has been considerable work in BER developing concept inventories as formative assessment tools that identify concepts students often struggle to learn (e.g., Marbach-Ad et al. , 2009 ; McFarland et al. , 2017 ; Summers et al. , 2018 ). However, much of this work is detached from a theoretical understanding of why students hold misconceptions in the first place, what the nature of their thinking is, and the learning mechanisms that would move students to a more productive understanding of domain ideas ( Alonzo, 2011 ). Using design-based research to understand the basis of students’ misconceptions would ground these practical learning problems in a theoretical understanding of the nature of student thinking (e.g., see Coley and Tanner, 2012 , 2015 ; Gouvea and Simon, 2018 ) and the kinds of instructional tools that would best support the learning process.
Design-Based Research Fosters Collaborations across Disciplines
Recently, there have been multiple calls across science, technology, engineering, and mathematics education fields to increase collaborations between BER and other disciplines so as to increase the robustness of science education research at the collegiate level ( Coley and Tanner, 2012 ; NRC, 2012 ; Talanquer, 2014 ; Dolan, 2015 ; Peffer and Renken, 2016 ; Mestre et al. , 2018 ; Davidesco and Milne, 2019 ). Engaging in design-based research provides both a mechanism and a motivation for fostering interdisciplinary collaborations, as it requires the design team to have theoretical knowledge of how students learn, domain knowledge of practical learning problems, and instructional knowledge for how to implement instructional tools in the classroom ( Edelson, 2002 ; Hoadley, 2004 ; Wang and Hannafin, 2005 ; Anderson and Shattuck, 2012 ). For example, in our current work, our research team consists of two discipline-based education learning scientists from an R1 institution, two physiology education researchers/instructors (one from an R1 institution the other from a community college), several physiology disciplinary experts/instructors, and a K–12 science education expert.
Design-based research collaborations have several distinct benefits for BER: first, learning or cognitive scientists could provide theoretical and methodological expertise that may be unfamiliar to biology education researchers with traditional science backgrounds ( Lo et al. , 2019 ). This would both improve the rigor of the research project and provide biology education researchers with the opportunity to explore ideas and methods from other disciplines. Second, collaborations between researchers and instructors could help increase the implementation of evidence-based teaching practices by instructors/faculty who are not education researchers and would benefit from support while shifting their instructional approaches ( Eddy et al. , 2015 ). This may be especially true for community college and primarily undergraduate institution faculty who often do not have access to the same kinds of resources that researchers and instructors at research-intensive institutions do ( Schinske et al. , 2017 ). Third, making instructors an integral part of a design-based research project ensures they are well versed in the theory and learning objectives underlying the instructional tools they are implementing in the classroom. This can improve the fidelity of implementation of the instructional tools, because the instructors understand the tools’ theoretical and practical purposes, which has been cited as one reason there have been mixed results on the impact of active learning across biology classes ( Andrews et al. , 2011 ; Borrego et al. , 2013 ; Lee et al. , 2018 ; Offerdahl et al. , 2018 ). It also gives instructors agency to make informed adjustments to the instructional tools during implementation that improve their practical applications while remaining true to the goals of the research ( Hoadley, 2004 ).
Design-Based Research Invites Using Mixed Methods to Analyze Data
The diverse nature of the data that are often collected in design-based research can require both qualitative and quantitative methodologies to produce a rich picture of how the instructional tools and their implementation influenced student learning ( Anderson and Shattuck, 2012 ). Using mixed methods may be less familiar to biology education researchers who were primarily trained in quantitative methods as biologists ( Lo et al. , 2019 ). However, according to Warfa (2016 , p. 2), “Integration of research findings from quantitative and qualitative inquiries in the same study or across studies maximizes the affordances of each approach and can provide better understanding of biology teaching and learning than either approach alone.” Although the number of BER studies using mixed methods has increased over the past decade ( Lo et al. , 2019 ), engaging in design-based research could further this trend through its collaborative nature of bringing social scientists together with biology education researchers to share research methodologies from different fields. By leveraging qualitative and quantitative methods, design-based researchers unpack “mechanism and process” by characterizing the nature of student thinking rather than “simply reporting that differences did or did not occur” ( Barab, 2014 , p. 158), which is important for continuing to advance our understanding of student learning in BER ( Dolan, 2015 ; Lo et al. , 2019 ).
CHALLENGES TO IMPLEMENTING DESIGN-BASED RESEARCH IN BER
As with any methodological approach, there can be challenges to implementing design-based research. Here, we highlight three that may be relevant to BER.
Collaborations Can Be Difficult to Maintain
While collaborations between researchers and instructors offer many affordances (as discussed earlier), the reality of connecting researchers across departments and institutions can be challenging. For example, Peffer and Renken (2016) noted that different traditions of scholarship can present barriers to collaboration where there is not mutual respect for the methods and ideas that are part and parcel to each discipline. Additionally, Schinske et al. (2017) identified several constraints that community college faculty face for engaging in BER, such as limited time or support (e.g., infrastructural, administrative, and peer support), which could also impact their ability to form the kinds of collaborations inherent in design-based research. Moreover, the iterative nature of design-based research requires these collaborations to persist for an extended period of time. Attending to these challenges is an important part of forming the design team and identifying the different roles researchers and instructors will play in the research.
Design-Based Research Experiments Are Resource Intensive
The focus of design-based research on studying learning ecologies to uncover mechanisms of learning requires that researchers collect multiple data streams through time, which often necessitates significant temporal and financial resources ( Collins et al., 2004 ; O’Donnell, 2004 ). Consequently, researchers must weigh both practical as well as methodological considerations when formulating their experimental design. For example, investigating learning mechanisms requires that researchers collect data at a frequency that will capture changes in student thinking ( Siegler, 2006 ). However, researchers may be constrained in the number of data-collection events they can anticipate depending on: the instructor’s ability to facilitate in-class collection events or solicit student participation in extracurricular activities (e.g., interviews); the cost of technological devices to record student conversations; the time and logistical considerations needed to schedule and conduct student interviews; the financial resources available to compensate student participants; the financial and temporal costs associated with analyzing large amounts of data.
Identifying learning mechanisms also requires in-depth analyses of qualitative data as students experience various instructional tools (e.g., microgenetic methods; Flynn et al. , 2006 ; Siegler, 2006 ). The high intensity of these in-depth analyses often limits the number of students who can be evaluated in this way, which must be balanced with the kinds of generalizations researchers wish to make about the effectiveness of the instructional tools ( O’Donnell, 2004 ). Because of the large variety of data streams that could be collected in a design-based research experiment—and the resources required to collect and analyze them—it is critical that the research team identify a priori how specific data streams, and the methods of their analysis, will provide the evidence necessary to address the theoretical and practical objectives of the research (see the following section on experimental rigor; Sandoval, 2014 ). These are critical management decisions because of the need for a transparent, systematic analysis of the data that others can scrutinize to evaluate the validity of the claims being made ( Cobb et al. , 2003 ).
Concerns with Experimental Rigor
The nature of design-based research, with its use of narrative to characterize versus control experimental environments, has drawn concerns about the rigor of this methodological approach. Some have challenged its ability to produce evidence-based warrants to support its claims of learning that can be replicated and critiqued by others ( Shavelson et al. , 2003 ; Hoadley, 2004 ). This is a valid concern that design-based researchers, and indeed all education researchers, must address to ensure their research meets established standards for education research ( NRC, 2002 ).
One way design-based researchers address this concern is by “specifying theoretically salient features of a learning environment design and mapping out how they are predicted to work together to produce desired outcomes” ( Sandoval, 2014 , p. 19). Through this process, researchers explicitly show before they begin the work how their theory of learning is embodied in the instructional tools to be tested, the specific data the tools will produce for analysis, and what outcomes will be taken as evidence for success. Moreover, by allowing instructional tools to be modified during the testing phase as needed, design-based researchers acknowledge that it is impossible to anticipate all aspects of the classroom environment that might impact the implementation of instructional tools, “as dozens (if not millions) of factors interact to produce the measureable outcomes related to learning” ( Hoadley, 2004 , p. 204; DBR Collective, 2003 ). Consequently, modifying instructional tools midstream to account for these unanticipated factors can ensure they retain their methodological alignment with the underlying theory and predicted learning outcomes so that inferences drawn from the design experiment accurately reflect what was being tested ( Edelson, 2002 ; Hoadley, 2004 ). Indeed, Barab (2014) states, “the messiness of real-world practice must be recognized, understood, and integrated as part of the theoretical claims if the claims are to have real-world explanatory value” (p. 153).
CONCLUSIONS
In this essay, we have highlighted some of the ways design-based research can advance—and expand upon—research done in biology education. These ways include:
- providing a methodology that integrates theories of learning with practical experiences in classrooms,
- using a range of analytical approaches that allow for researchers to uncover the underlying mechanisms of student thinking and learning,
- fostering interdisciplinary collaborations among researchers and instructors, and
- characterizing learning ecologies that account for the complexity involved in student learning
By employing this methodology from the learning sciences, biology education researchers can enrich our current understanding of what is required to help biology students achieve their personal and professional aims during their college experience. It can also stimulate new ideas for biology education that can be discussed and debated in our research community as we continue to explore and refine how best to serve the students who pass through our classroom doors.
Acknowledgments
We thank the UW Biology Education Research Group’s (BERG) feedback on drafts of this essay as well as Dr. L. Jescovich for last-minute analyses. This work was supported by a National Science Foundation award (NSF DUE 1661263/1660643). Any opinions, findings, and conclusions or recommendations expressed in this material are those of the author(s) and do not necessarily reflect the views of the NSF. All procedures were conducted in accordance with approval from the Institutional Review Board at the University of Washington (52146) and the New England Independent Review Board (120160152).
1 “Epistemic commitment” is defined as engaging in certain practices that generate knowledge in an agreed-upon way.
- Alonzo, A. C. (2011). Learning progressions that support formative assessment practices . Measurement , 9 ( 2/3 ), 124–129. [ Google Scholar ]
- Anderson, T., Shattuck, J. (2012). Design-based research: A decade of progress in education research? Educational Researcher , 41 ( 1 ), 16–25. [ Google Scholar ]
- Andrews, T. M., Leonard, M. J., Colgrove, C. A., Kalinowski, S. T. (2011). Active learning not associated with student learning in a random sample of college biology courses . CBE—Life Sciences Education , 10 ( 4 ), 394–405. [ PMC free article ] [ PubMed ] [ Google Scholar ]
- Badenhorst, E., Hartman, N., Mamede, S. (2016). How biomedical misconceptions may arise and affect medical students’ learning: A review of theoretical perspectives and empirical evidence . Health Professions Education , 2 ( 1 ), 10–17. [ Google Scholar ]
- Barab, S. (2014). Design-based research: A methodological toolkit for engineering change . In The Cambridge handbook of the learning sciences (2nd ed., pp. 151–170). Cambridge University Press. 10.1017/CBO9781139519526.011 [ CrossRef ] [ Google Scholar ]
- Barab, S., Squire, K. (2004). Design-based research: Putting a stake in the ground . Journal of the Learning Sciences , 13 ( 1 ), 1–14. [ Google Scholar ]
- Bell, P. (2004). On the theoretical breadth of design-based research in education . Educational Psychologist , 39 ( 4 ), 243–253. [ Google Scholar ]
- Borrego, M., Cutler, S., Prince, M., Henderson, C., Froyd, J. E. (2013). Fidelity of implementation of research-based instructional strategies (RBIS) in engineering science courses . Journal of Engineering Education , 102 ( 3 ), 394–425. [ Google Scholar ]
- Brown, A. L. (1992). Design experiments: Theoretical and methodological challenges in creating complex interventions in classroom settings . Journal of the Learning Sciences , 2 ( 2 ), 141–178. [ Google Scholar ]
- Chi, M. T. H., Roscoe, R. D., Slotta, J. D., Roy, M., Chase, C. C. (2012). Misconceived causal explanations for emergent processes . Cognitive Science , 36 ( 1 ), 1–61. [ PubMed ] [ Google Scholar ]
- Cobb, P., Confrey, J., diSessa, A., Lehrer, R., Schauble, L. (2003). Design experiments in educational research . Educational Researcher , 32 ( 1 ), 9–13. [ Google Scholar ]
- Coley, J. D., Tanner, K. D. (2012). Common origins of diverse misconceptions: Cognitive principles and the development of biology thinking . CBE—Life Sciences Education , 11 ( 3 ), 209–215. [ PMC free article ] [ PubMed ] [ Google Scholar ]
- Coley, J. D., Tanner, K. (2015). Relations between intuitive biological thinking and biological misconceptions in biology majors and nonmajors . CBE—Life Sciences Education , 14 ( 1 ). 10.1187/cbe.14-06-0094 [ PMC free article ] [ PubMed ] [ CrossRef ] [ Google Scholar ]
- Collins, A., Joseph, D., Bielaczyc, K. (2004). Design research: Theoretical and methodological issues . Journal of the Learning Sciences , 13 ( 1 ), 15–42. [ Google Scholar ]
- Corcoran, T., Mosher, F. A., Rogat, A. D. (2009). Learning progressions in science: An evidence-based approach to reform (CPRE Research Report No. RR-63) . Philadelphia, PA: Consortium for Policy Research in Education. [ Google Scholar ]
- Davidesco, I., Milne, C. (2019). Implementing cognitive science and discipline-based education research in the undergraduate science classroom . CBE—Life Sciences Education , 18 ( 3 ), es4. [ PMC free article ] [ PubMed ] [ Google Scholar ]
- Design-Based Research Collective. (2003). Design-based research: An emerging paradigm for educational inquiry . Educational Researcher , 32 ( 1 ), 5–8. [ Google Scholar ]
- Dolan, E. L. (2015). Biology education research 2.0 . CBE—Life Sciences Education , 14 ( 4 ), ed1. [ PMC free article ] [ PubMed ] [ Google Scholar ]
- Duschl, R., Maeng, S., Sezen, A. (2011). Learning progressions and teaching sequences: A review and analysis . Studies in Science Education , 47 ( 2 ), 123–182. [ Google Scholar ]
- Eddy, S. L., Converse, M., Wenderoth, M. P. (2015). PORTAAL: A classroom observation tool assessing evidence-based teaching practices for active learning in large science, technology, engineering, and mathematics classes . CBE—Life Sciences Education , 14 ( 2 ), ar23. [ PMC free article ] [ PubMed ] [ Google Scholar ]
- Edelson, D. C. (2002). Design research: What we learn when we engage in design . Journal of the Learning Sciences , 11 ( 1 ), 105–121. [ Google Scholar ]
- Flynn, E., Pine, K., Lewis, C. (2006). The microgenetic method—Time for change? The Psychologist , 19 ( 3 ), 152–155. [ Google Scholar ]
- Gouvea, J. S., Simon, M. R. (2018). Challenging cognitive construals: A dynamic alternative to stable misconceptions . CBE—Life Sciences Education , 17 ( 2 ), ar34. [ PMC free article ] [ PubMed ] [ Google Scholar ]
- Gunckel, K. L., Mohan, L., Covitt, B. A., Anderson, C. W. (2012). Addressing challenges in developing learning progressions for environmental science literacy . In Alonzo, A. C., Gotwals, A. W. (Eds.), Learning progressions in science: Current challenges and future directions (pp. 39–75). Rotterdam: SensePublishers. 10.1007/978-94-6091-824-7_4 [ CrossRef ] [ Google Scholar ]
- Hake, R. R. (2007). Design-based research in physics education research: A review . In Kelly, A. E., Lesh, R. A., Baek, J. Y. (Eds.), Handbook of design research methods in mathematics, science, and technology education (p. 24). New York: Routledge. [ Google Scholar ]
- Hoadley, C. M. (2004). Methodological alignment in design-based research . Educational Psychologist , 39 ( 4 ), 203–212. [ Google Scholar ]
- Jackson, M., Tran, A., Wenderoth, M. P., Doherty, J. H. (2018). Peer vs. self-grading of practice exams: Which is better? CBE—Life Sciences Education , 17 ( 3 ), es44. 10.1187/cbe.18-04-0052 [ PMC free article ] [ PubMed ] [ CrossRef ] [ Google Scholar ]
- Jin, H., Anderson, C. W. (2012). A learning progression for energy in socio-ecological systems . Journal of Research in Science Teaching , 49 ( 9 ), 1149–1180. [ Google Scholar ]
- Joseph, D. (2004). The practice of design-based research: Uncovering the interplay between design, research, and the real-world context . Educational Psychologist , 39 ( 4 ), 235–242. [ Google Scholar ]
- Kelly, A. E. (2014). Design-based research in engineering education . In Cambridge handbook of engineering education research (pp. 497–518). New York, NY: Cambridge University Press. 10.1017/CBO9781139013451.032 [ CrossRef ] [ Google Scholar ]
- Lee, C. J., Toven-Lindsey, B., Shapiro, C., Soh, M., Mazrouee, S., Levis-Fitzgerald, M., Sanders, E. R. (2018). Error-discovery learning boosts student engagement and performance, while reducing student attrition in a bioinformatics course . CBE—Life Sciences Education , 17 ( 3 ), ar40. 10.1187/cbe.17-04-0061 [ PMC free article ] [ PubMed ] [ CrossRef ] [ Google Scholar ]
- Lo, S. M., Gardner, G. E., Reid, J., Napoleon-Fanis, V., Carroll, P., Smith, E., Sato, B. K. (2019). Prevailing questions and methodologies in biology education research: A longitudinal analysis of research in CBE — life sciences education and at the society for the advancement of biology education research . CBE—Life Sciences Education , 18 ( 1 ), ar9. [ PMC free article ] [ PubMed ] [ Google Scholar ]
- Marbach-Ad, G., Briken, V., El-Sayed, N. M., Frauwirth, K., Fredericksen, B., Hutcheson, S., Smith, A. C. (2009). Assessing student understanding of host pathogen interactions using a concept inventory . Journal of Microbiology & Biology Education , 10 ( 1 ), 43–50. [ PMC free article ] [ PubMed ] [ Google Scholar ]
- McFarland, J. L., Price, R. M., Wenderoth, M. P., Martinková, P., Cliff, W., Michael, J., … & Wright, A. (2017). Development and validation of the homeostasis concept inventory . CBE—Life Sciences Education , 16 ( 2 ), ar35. [ PMC free article ] [ PubMed ] [ Google Scholar ]
- McKenney, S., Reeves, T. C. (2013). Systematic review of design-based research progress: Is a little knowledge a dangerous thing? Educational Researcher , 42 ( 2 ), 97–100. [ Google Scholar ]
- Mestre, J. P., Cheville, A., Herman, G. L. (2018). Promoting DBER-cognitive psychology collaborations in STEM education . Journal of Engineering Education , 107 ( 1 ), 5–10. [ Google Scholar ]
- Michael, J. A. (2007). What makes physiology hard for students to learn? Results of a faculty survey . AJP: Advances in Physiology Education , 31 ( 1 ), 34–40. [ PubMed ] [ Google Scholar ]
- Michael, J. A., Modell, H., McFarland, J., Cliff, W. (2009). The “core principles” of physiology: What should students understand? Advances in Physiology Education , 33 ( 1 ), 10–16. [ PubMed ] [ Google Scholar ]
- Middleton, J., Gorard, S., Taylor, C., Bannan-Ritland, B. (2008). The “compleat” design experiment: From soup to nuts . In Kelly, A. E., Lesh, R. A., Baek, J. Y. (Eds.), Handbook of design research methods in education: Innovations in science, technology, engineering, and mathematics learning and teaching (pp. 21–46). New York, NY: Routledge. [ Google Scholar ]
- Modell, H. I. (2000). How to help students understand physiology? Emphasize general models . Advances in Physiology Education , 23 ( 1 ), S101–S107. [ PubMed ] [ Google Scholar ]
- Mohan, L., Chen, J., Anderson, C. W. (2009). Developing a multi-year learning progression for carbon cycling in socio-ecological systems . Journal of Research in Science Teaching , 46 ( 6 ), 675–698. [ Google Scholar ]
- National Academies of Sciences, Engineering, and Medicine. (2018). How People Learn II: Learners, Contexts, and Cultures . Washington, DC: National Academies Press. Retrieved June 24, 2019, from 10.17226/24783 [ CrossRef ] [ Google Scholar ]
- National Research Council (NRC). (2002). Scientific research in education . Washington, DC: National Academies Press. Retrieved January 31, 2019, from 10.17226/10236 [ CrossRef ] [ Google Scholar ]
- NRC. (2007). Taking science to school: Learning and teaching science in grades K–8 . Washington, DC: National Academies Press. Retrieved March 22, 2019, from www.nap.edu/catalog/11625/taking-science-to-school-learning-and-teaching-science-in-grades . 10.17226/11625 [ CrossRef ] [ Google Scholar ]
- NRC. (2012). Discipline-based education research: Understanding and improving learning in undergraduate science and engineering . Washington, DC: National Academies Press. Retrieved from www.nap.edu/catalog/13362/discipline-based-education-research-understanding-and-improving-learning-in-undergraduate . 10.17226/13362 [ CrossRef ] [ Google Scholar ]
- NRC. (2018). How people learn II: Learners, contexts, and cultures . Washington, DC: National Academies Press. Retrieved from www.nap.edu/read/24783/chapter/7 . 10.17226/24783 [ CrossRef ] [ Google Scholar ]
- O’Donnell, A. M. (2004). A commentary on design research . Educational Psychologist , 39 ( 4 ), 255–260. [ Google Scholar ]
- Offerdahl, E. G., McConnell, M., Boyer, J. (2018). Can I have your recipe? Using a fidelity of implementation (FOI) framework to identify the key ingredients of formative assessment for learning . CBE—Life Sciences Education , 17 ( 4 ), es16. [ PMC free article ] [ PubMed ] [ Google Scholar ]
- Peffer, M., Renken, M. (2016). Practical strategies for collaboration across discipline-based education research and the learning sciences . CBE—Life Sciences Education , 15 ( 4 ), es11. [ PMC free article ] [ PubMed ] [ Google Scholar ]
- Reiser, B. J., Smith, B. K., Tabak, I., Steinmuller, F., Sandoval, W. A., Leone, A. J. (2001). BGuILE: Strategic and conceptual scaffolds for scientific inquiry in biology classrooms . In Carver, S. M., Klahr, D. (Eds.), Cognition and instruction: Twenty-five years of progress (pp. 263–305). Mahwah, NJ: Lawrence Erlbaum Associates, Inc. [ Google Scholar ]
- Sandoval, W. (2014). Conjecture mapping: An approach to systematic educational design research . Journal of the Learning Sciences , 23 ( 1 ), 18–36. [ Google Scholar ]
- Sandoval, W. A., Bell, P. (2004). Design-based research methods for studying learning in context: Introduction . Educational Psychologist , 39 ( 4 ), 199–201. [ Google Scholar ]
- Schinske, J. N., Balke, V. L., Bangera, M. G., Bonney, K. M., Brownell, S. E., Carter, R. S., … & Corwin, L. A. (2017). Broadening participation in biology education research: Engaging community college students and faculty . CBE—Life Sciences Education , 16 ( 2 ), mr1. [ PMC free article ] [ PubMed ] [ Google Scholar ]
- Scott, E., Anderson, C. W., Mashood, K. K., Matz, R. L., Underwood, S. M., Sawtelle, V. (2018). Developing an analytical framework to characterize student reasoning about complex processes . CBE—Life Sciences Education , 17 ( 3 ), ar49. 10.1187/cbe.17-10-0225 [ PMC free article ] [ PubMed ] [ CrossRef ] [ Google Scholar ]
- Scott, E., Wenderoth, M. P., Doherty, J. H. (2019). Learning progressions: An empirically grounded, learner-centered framework to guide biology instruction . CBE—Life Sciences Education , 18 ( 4 ), es5. 10.1187/cbe.19-03-0059 [ PMC free article ] [ PubMed ] [ CrossRef ] [ Google Scholar ]
- Sharma, M. D., McShane, K. (2008). A methodological framework for understanding and describing discipline-based scholarship of teaching in higher education through design-based research . Higher Education Research & Development , 27 ( 3 ), 257–270. [ Google Scholar ]
- Shavelson, R. J., Phillips, D. C., Towne, L., Feuer, M. J. (2003). On the science of education design studies . Educational Researcher , 32 ( 1 ), 25–28. [ Google Scholar ]
- Siegler, R. S. (2006). Microgenetic analyses of learning . In Damon, W., Lerner, R. M. (Eds.), Handbook of child psychology (pp. 464–510). Hoboken, NJ: John Wiley & Sons, Inc. 10.1002/9780470147658.chpsy0211 [ CrossRef ] [ Google Scholar ]
- Stringer, E. T. (2013). Action research . Thousand Oaks, CA: Sage Publications, Inc. [ Google Scholar ]
- Subramaniam, M., Jean, B. S., Taylor, N. G., Kodama, C., Follman, R., Casciotti, D. (2015). Bit by bit: Using design-based research to improve the health literacy of adolescents . JMIR Research Protocols , 4 ( 2 ), e62. [ PMC free article ] [ PubMed ] [ Google Scholar ]
- Summers, M. M., Couch, B. A., Knight, J. K., Brownell, S. E., Crowe, A. J., Semsar, K., … & Batzli, J. (2018). EcoEvo-MAPS: An ecology and evolution assessment for introductory through advanced undergraduates . CBE—Life Sciences Education , 17 ( 2 ), ar18. [ PMC free article ] [ PubMed ] [ Google Scholar ]
- Szteinberg, G., Balicki, S., Banks, G., Clinchot, M., Cullipher, S., Huie, R., … & Sevian, H. (2014). Collaborative professional development in chemistry education research: Bridging the gap between research and practice . Journal of Chemical Education , 91 ( 9 ), 1401–1408. [ Google Scholar ]
- Talanquer, V. (2014). DBER and STEM education reform: Are we up to the challenge? Journal of Research in Science Teaching , 51 ( 6 ), 809–819. [ Google Scholar ]
- Wang, F., Hannafin, M. J. (2005). Design-based research and technology-enhanced learning environments . Educational Technology Research and Development , 53 ( 4 ), 5–23. [ Google Scholar ]
- Wang, J.-R. (2004). Development and validation of a Two-tier instrument to examine understanding of internal transport in plants and the human circulatory system . International Journal of Science and Mathematics Education , 2 ( 2 ), 131–157. [ Google Scholar ]
- Warfa, A.-R. M. (2016). Mixed-methods design in biology education research: Approach and uses . CBE—Life Sciences Education , 15 ( 4 ), rm5. [ PMC free article ] [ PubMed ] [ Google Scholar ]
- Windschitl, M., Thompson, J., Braaten, M., Stroupe, D. (2012). Proposing a core set of instructional practices and tools for teachers of science . Science Education , 96 ( 5 ), 878–903. [ Google Scholar ]
- Zagallo, P., Meddleton, S., Bolger, M. S. (2016). Teaching real data interpretation with models (TRIM): Analysis of student dialogue in a large-enrollment cell and developmental biology course . CBE—Life Sciences Education , 15 ( 2 ), ar17. [ PMC free article ] [ PubMed ] [ Google Scholar ]
Eurasia Journal of Mathematics, Science and Technology Education
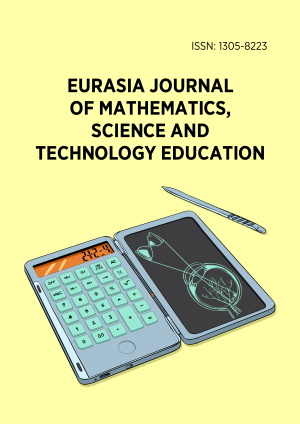
Researchers often develop teaching-learning solutions to improve the quality of instruction. Some of these solutions are developed in the paradigm of design-based research (DBR). The output of DBR projects goes beyond design products for practice and includes contributions to local theories about teaching-learning in specific subject areas and contexts as well as knowledge about how to design and implement these processes. Design knowledge and contributions to local theories are intended to construct a cumulative, content-specific body of knowledge about teaching and learning that is transferable to related subject areas or contexts. To make this process work, dimensions of DBR need to be systematically reported. However, DBR projects are sometimes criticized for focusing more on practical output than on reports about research output and the form of cooperation with practitioners. To empirically investigate these presumed voids, we examined DBR projects conducted by the German-speaking physics education research community during the past 20 years.
- design-based research
- theory-practice gap
- curriculum development
This is an open access article distributed under the Creative Commons Attribution License which permits unrestricted use, distribution, and reproduction in any medium, provided the original work is properly cited.
Article Type: Research Article
EURASIA J Math Sci Tech Ed, 2024, Volume 20, Issue 1, Article No: em2378
https://doi.org/10.29333/ejmste/13928
Publication date: 01 Jan 2024
Online publication date: 26 Nov 2023
Article Views: 942
Article Downloads: 545
- Bakker, A. (2018). Design research in education: A practical guide for early career researchers . Routledge. https://doi.org/10.4324/9780203701010
- Barab, S. (2014). Design-based research: A methodological toolkit for engineering change. In R. K. Sawyer (Ed.), Cambridge handbook of the learning sciences (pp. 151-170). Cambridge University Press. https://doi.org/10.1017/CBO9781139519526.011
- Behrens, D. (2018). Konzeption, Entwicklung und Evaluation von Lernmaterialien (KEEL) [ Conception, development and evaluation of learning materials (KEEL) ]. https://oops.uni-oldenburg.de/id/eprint/3691
- Bereiter, C. (2002). Design research for sustained innovation. Bulletin of the Japanese Cognitive Science Society, 9 (3), 321-327. https://doi.org/10.11225/jcss.9.321
- Bitzenbauer, P. (2020). Quantenoptik an Schulen. Studie im mixed-methods Design zur Evaluation des Erlanger Unterrichtskonzepts zur Quantenoptik [ Quantum optics in schools. Study in mixed-methods design to evaluate the Erlangen teaching concept on quantum optics ]. Logos Verlag Berlin. https://doi.org/10.30819/5123
- Bliesmer, K. (2020). Physik der Küste für außerschulische Lernorte: Eine didaktische Rekonstruktion [ Physics of the coast for extracurricular learning places: A didactic reconstruction ]. Logos Verlag Berlin. https://doi.org/10.30819/5190
- Brown, A. L. (1992). Design experiments: Theoretical and methodological challenges in creating complex interventions in classroom settings. Journal of the Learning Sciences, 2 (2), 141-178. https://doi.org/10.1207/s15327809jls0202_2
- Buhl, M., Dirckinck-Holmfeld, L., & Jensen, E. O. (2022). Expanding and orchestrating the problem identification phase of design-based research. Nordic Journal of Digital Literacy, 17 (4), 211-221. https://doi.org/10.18261/njdl.17.4.2
- Burde, J.-P. (2018). Konzeption und Evaluation eines Unterrichtskonzepts zu einfachen Stromkreisen auf Basis des Elektronengasmodells [ Conception and evaluation of a teaching concept for simple circuits based on the electron gas model ]. Logos Verlag Berlin. https://doi.org/10.30819/4726
- Cobb, P., Confrey, J., diSessa, A. A., Lehrer, R., & Schauble, L. (2003). Design experiments in educational research. Educational Researcher, 32 (1), 9-13. https://doi.org/10.3102/0013189X032001009
- Collins, A. (1992). Toward a design science of education. In E. Scanlon, & T. O’Shea (Eds.), New directions in educational technology (pp. 15-22). Springer. https://doi.org/10.1007/978-3-642-77750-9_2
- Confrey, J. (2005). The evolution of design studies as methodology. In K. R. Sawyer (Ed.), Cambridge handbook of the learning sciences (pp. 135-152). Cambridge University Press. https://doi.org/10.1017/cbo9780511816833.010
- Design-Based Research Collective. (2003). Design-based research: An emerging paradigm for educational inquiry. Educational Researcher, 32 (1), 5-8. https://doi.org/10.3102/0013189x032001005
- diSessa, A. A., & Cobb, P. (2004). Ontological innovation and the role of theory in design experiments. Journal of the Learning Sciences, 13 (1), 77-103. https://doi.org/10.1207/s15327809jls1301_4
- Duit, R., & Treagust, D. F. (2003). Conceptual change: A powerful framework for improving science teaching and learning. International Journal of Science Education, 25 (6), 671-688. https://doi.org/10.1080/09500690305016
- Duit, R., Treagust, D. F., & Widodo, A. (2013). Teaching science for conceptual change: Theory and practice. In S. Vosniadou (Ed.), International handbook of research on conceptual change (pp. 499-515). Routledge. https://doi.org/10.4324/9780203154472.ch25
- Edelson, D. C. (2002). Design research: What we learn when we engage in design. Journal of the Learning Sciences, 11 (1), 105-121. https://doi.org/10.1207/S15327809JLS1101_4
- Firestone, W. A. (1993). Alternative arguments for generalizing from data as applied to qualitative research. Educational Researcher, 22 (4), 16-23. https://doi.org/10.3102/0013189x022004016
- Haagen-Schützenhöfer, C. (2016). Lehr- und Lernprozesse im Anfangsoptikunterricht der Sekundarstufe I [ Teaching and learning processes in early secondary school optics lessons ] [Cumulative habilitation thesis, Universität Graz].
- Haagen-Schützenhöfer, C., & Hopf, M. (2020). Design-based research as a model for systematic curriculum development: The example of a curriculum for introductory optics. Physical Review Physics Education Research, 16 (2), 20152. https://doi.org/10.1103/PhysRevPhysEducRes.16.020152
- Haak, I. (2017). Maßnahmen zur Unterstützung kognitiver und metakognitiver Prozesse in der Studieneingangsphase: Eine Design-based-research-Studie zum universitären Lernzentrum Physiktreff [ Measures to support cognitive and metacognitive processes in the initial study phase: A design-based research study on the university learning center physics meeting ]. Logos Verlag Berlin.
- Heran-Dörr, E. (2006). Entwicklung und Evaluation einer Lehrerfortbildung zur Förderung der physikdidaktischen Kompetenz von Sachunterrichtslehrkräften [ Development and evaluation of teacher training to promote the physics didactic competence of subject matter teachers ]. Ludwig-Maximilians-Universität München. https://edoc.ub.uni-muenchen.de/00005878/
- Hußmann, S., Thiele, J., Hinz, R., Prediger, S., & Ralle, B. (2013). Gegenstandsorientierte Unterrichtsdesigns entwickeln und erforschen: Fachdidaktische Entwicklungsforschung im Dortmunder Modell [Developing and researching subject-oriented teaching designs: Didactic development research in Dortmund model]. In M. Komorek (Ed.), Fachdidaktische forschungen: Der lange Weg zum Unterrichtsdesign: Zur Begründung und Umsetzung fachdidaktischer Forschungs- und Entwicklungsprogramme [ Subject didactic research: The long path to teaching design: On the justification and implementation of subject didactic research and development programs ] (pp. 25-42). Waxmann.
- Jannack, V. (2017). Empirische Studie zum Einsatz von Problembasiertem Lernen (PBL) im interdisziplinären naturwissenschaftlichen Unterricht: Kompetenzentwicklung bei Schülerinnen und Schülern und Akzeptantz bei Lehrerinnen und Lehrern [ Empirical study on the use of problem-based learning (PBL) in interdisciplinary science teaching: Competence development in students and acceptance in teachers ]. https://core.ac.uk/download/pdf/212115186.pdf
- Jung, W. (1992). Probing acceptance, a technique for investigating learning difficulties. In R. Duit, F. Goldberg, & H. Niedderer (Eds.), Research in physics learning: Theoretical issues and empirical studies (pp. 278-295). IPN.
- Kelly, A. (2004). Design research in education: Yes, but is it methodological? Journal of the Learning Sciences, 13 (1), 115-128. https://doi.org/10.1207/s15327809jls1301_6
- Küpper, A. M. (2021). (Weiter-)entwicklung und Evaluation der Lernumgebung “Mit dem Licht durch unser Sonnensystem und darüber hinaus” zur dualen Förderung von Kompetenzen zum Umgang mit Fachwissen, der sozialen Integration, der Kooperationsfähigkeit und der Selbstständigkeit im inklusiven Physikunterricht der Orientierungsstufe: Ein design-based research-Projekt [ (Further) development and evaluation of the learning environment “with light through our solar system and beyond” for the dual promotion of skills in dealing with specialist knowledge, social integration, the ability to cooperate and independence in inclusive physics lessons at the orientation level: A design-based research-project ] [Doctoral thesis, University of Cologne].
- Laumann, D. (2017). Magnetismus hoch 4: Fachliche Strukturierung und Entwicklung multipler Repräsentationen zum Magnetismus für die Hochschule [ Magnetism to the power of 4: Professional structuring and development of multiple representations on magnetism for universities ]. Logos Verlag. https://doi.org/10.30819/4571
- Mayring, P. (2014). Qualitative content analysis: Theoretical foundation, basic procedures and software solution . Klagenfurt, 2014. Retrieved October 31, 2023, from https://www.ssoar.info/ssoar/handle/document/39517
- McKenney, S., & Reeves, T. C. (2018). Conducting educational design research . Routledge. https://doi.org/10.4324/9781315105642
- Mikelskis, H., & Mikelskis, H. F. (2010). Physikdidaktik: Praxishandbuch für die Sekundarstufe I und II [ Physics didactics: Practical handbook for secondary schools I and II ]. Cornelsen Scriptor.
- Mikelskis-Seifert, S., Ringelband, U., & Brückmann, M. (2008). Four decades of research in science education–From curriculum development to quality improvement . Waxmann.
- Newman, M., & Gough, D. (2020). Systematic reviews in educational research: Methodology, perspectives and application. In O. Zawacki-Richter, M. Kerres, S. M. Bedenlier, M. Bond, & K. Buntins (Eds.), Systematic reviews in educational research: Methodology, perspectives and application (pp. 3-22). Springer. https://doi.org/10.1007/978-3-658-27602-7_1
- Niedderer, H., & van Aufschnaiter, S. (2008). Curriculum development and related research yesterday and today–The example of the IPN curriculum physics. In S. Mikelskis-Seifert, U. Ringelband, & M. Brückmann (Eds.), Four decades of research in science education–From curriculum development to quality improvement (pp. 13-28). Waxmann.
- Page, M. J., McKenzie, J. E., Bossuyt, P. M., Boutron, I., Hoffmann, T. C., Mulrow, C. D., Shamseer, L., Tetzlaff, J. M., Akl, E. A., Brennan, S. E., Chou, R., Glanville, J., Grimshaw, J. M., Hróbjartsson, A., Lalu, M. M., Li, T., Loder, E. W., Mayo-Wilson, E., McDonald, S., . . . Moher, D. (2021). The PRISMA 2020 statement: An updated guideline for reporting systematic reviews. Systematic Reviews, 10 (1), 89. https://doi.org/10.1186/s13643-021-01626-4
- Polit, D. F., & Beck, C. T. (2010). Generalization in quantitative and qualitative research: Myths and strategies. International Journal of Nursing Studies, 47 (11), 1451-1458. https://doi.org/10.1016/j.ijnurstu.2010.06.004
- Prediger, S., & Link, M. (2012). Fachdidaktische Entwicklungsforschung–Ein lernprozessfokussierendes Forschungsprogramm mit Verschränkung fachdidaktischer Arbeitsbereiche [Subject-didactic development research–A research program that focuses on the learning process and interlinks subject-didactic work areas]. In H. Bayrhuber, U. Harms, & B. Muszynski (Eds.), Formate fachdidaktischer Forschung: Empirische Projekte – historische Analysen – theoretische Grundlegungen [ Formats of didactic research: Empirical projects–historical analyzes–theoretical foundations ] (pp. 29-45). Waxmann.
- Prediger, S., & Zwetzschler, L. (2013). Topic-specific design research with a focus on learning processes: The case of understanding algebraic equivalence in grade 8. In T. Plomp, & N. Nieveen (Eds.), Educational design research–Part B: Illustrative cases (pp. 407-424). SLO.
- Prediger, S., Gravemeijer, K., & Confrey, J. (2015). Design research with a focus on learning processes: An overview on achievements and challenges. ZDM, 47 (6), 877-891. https://doi.org/10.1007/s11858-015-0722-3
- Prediger, S., Komorek, M., Fischer, A., Hinz, R., Hußmann, S., Moschner, B., Ralle, B., & Thiele, J. (2013). Der lange Weg zum Unterrichtsdesign [The long road to instructional design]. In M. Komorek, & S. Prediger (Eds.), Der lange Weg zum Unterrichtsdesign. Zur Begründung und Umsetzung fachdidaktischer Forschungs-und Entwicklungsprogramme [ The long road to instructional design. On the justification and implementation of didactic research and development programs ] (pp. 9-23). Waxmann.
- Reinmann, G. (2005). Innovation ohne Forschung? Ein Plädoyer für den design-based research-Ansatz in der Lehr-lernforschung [Innovation without research? A plea for the design-based research approach in teaching-learning research]. Zeitschrift für Lernforschung [ Journal for Learning Research ] , 33 (1), 52-69. https://doi.org/10.15460/eder.6.2.1909
- Reinmann, G. (2022). Was macht design-based research zu Forschung? Die Debatte um Standards und die vernachlässigte Rolle des Designs [What makes design-based research? The debate about standards and the neglected role of design]. Educational Design Research, 6 (2), 48.
- Roggema, R. (2016). Research by design: Proposition for a methodological approach. Urban Science, 1 (1), 2. https://doi.org/10.3390/urbansci1010002
- Roskam, A. (2020). Kognitive Verarbeitungsprozesse in der Interaktion mit Strömungsexperimenten in einer Ausstellung [ Cognitive processing processes in interaction with flow experiments in an exhibition ]. Springer. https://doi.org/10.1007/978-3-658-30756-1
- Sajons, C. M. (2020). Kognitive und motivationale Dynamik in Schülerlaboren. Kontextualisierung, Problemorientierung und Autonomieunterstützung der didaktischen Struktur analysieren und weiterentwickeln [ Cognitive and motivational dynamics in student laboratories. Analyze and further develop the contextualization, problem orientation and autonomy support of the didactic structure ]. Logos Verlag Berlin. https://doi.org/10.30819/5155
- Schecker, H., & Hopf, M. (2021). Entwicklung von Unterrichtskonzeptionen [Development of teaching concepts]. In T. Wilhelm, H. Schecker, & M. Hopf (Eds.), Unterrichtskonzeptionen für den Physikunterricht: Ein Lehrbuch für Studium, Referendariat und Unterrichtspraxis [ Teaching concepts for physics lessons: A textbook for studies, traineeships and teaching practice ] (pp. 1-16). Springer. https://doi.org/10.1007/978-3-662-63053-2_1
- Shah, J. K., Ensminger, D. C., & Thier, K. (2015). The time for design-based research is right and right now. Mid-Western Educational Researcher, 27 (2), 152-171.
- Skorsetz, N. (2019). Empathisierer und Systematisierer im Vorschulalter: Eine Fragebogen- und Videostudie zur Motivation, sich mit Naturphänomenen zu beschäftigen [ Empathizers and systematizers in preschool age: A questionnaire and video study on the motivation to deal with natural phenomena ]. Logos Verlag Berlin. https://doi.org/10.30819/4825
- Smith, G. J., Schmidt, M. M., Edelen-Smith, P. J., & Cook, B. G. (2013). Pasteur’s quadrant as the bridge linking rigor with relevance. Exceptional Children, 79 (3), 147-161. https://doi.org/10.1177/001440291307900202
- Smoor, S. (2018). Lehr-lern-labore als Instrument der Professionalisierung im Lehramtsstudium Physik [ Teaching-learning laboratories as an instrument of professionalization in physics teacher training courses ]. https://oops.uni-oldenburg.de/id/eprint/3962
- Stokes, D. E. (1997). Pasteur’s quadrant: Basic science and technological innovation . Brookings Institution Press.
- Stokes, D. E. (2011). Pasteur’s quadrant: Basic science and technological innovation . Brookings Institution Press.
- Tobias, V. (2010). Newton’sche Mechanik im Anfangsunterricht: Die Wirksamkeit einer Einführung über die zweidimensionale Dynamik auf das Lehren und Lernen [ Newtonian mechanics in early teaching: The effectiveness of an introduction to two-dimensional dynamics on teaching and learning ]. Logos Berlin.
- van den Akker, J. J. H., Gravemeijer, K., McKenney, S., & Nieveen, N. (2006). Educational design research . Routledge. https://doi.org/10.4324/9780203088364
- Wiener, G. (2017). Elementary particle physics in early physics education. CERN . https://indico.cern.ch/event/657410/contributions/2904368/attachments/1614319/2565184/JW_ElementaryParticles.pdf
- Wilhelm, T., & Hopf, M. (2014). Design-Forschung [Design research]. In D. Krüger, I. Parchmann, & H. Schecker (Eds.), Methoden in der naturwissenschaftsdidaktischen Forschung [ Methods in scientific didactic research ] (pp. 31–42). Springer. https://doi.org/10.1007/978-3-642-37827-0_3
- Wilhelm, T., Tobias, V., Waltner, C., Hopf, M., & Wiesner, H. (2012). Design-based research am Beispiel der zweidimensional-dynamischen Mechanik [ Design-based research using the example of two-dimensional dynamic mechanics ]. Retrieved October 31, 2023, from https://www.thomas-wilhelm.net/veroeffentlichung/Plenarvortrag.pdf
How to cite this article
Haagen-Schützenhöfer, C., Obczovsky, M., & Kislinger, P. (2024). Design-based research–Tension between practical relevance and knowledge generation–What can we learn from projects?. Eurasia Journal of Mathematics, Science and Technology Education, 20 (1), em2378. https://doi.org/10.29333/ejmste/13928
Haagen-Schützenhöfer C, Obczovsky M, Kislinger P. Design-based research–Tension between practical relevance and knowledge generation–What can we learn from projects?. EURASIA J Math Sci Tech Ed. 2024;20(1):em2378. https://doi.org/10.29333/ejmste/13928
Haagen-Schützenhöfer C, Obczovsky M, Kislinger P. Design-based research–Tension between practical relevance and knowledge generation–What can we learn from projects?. EURASIA J Math Sci Tech Ed . 2024;20(1), em2378. https://doi.org/10.29333/ejmste/13928
Haagen-Schützenhöfer, Claudia, Markus Obczovsky, and Paula Kislinger. "Design-based research–Tension between practical relevance and knowledge generation–What can we learn from projects?". Eurasia Journal of Mathematics, Science and Technology Education 2024 20 no. 1 (2024): em2378. https://doi.org/10.29333/ejmste/13928
Haagen-Schützenhöfer, C., Obczovsky, M., and Kislinger, P. (2024). Design-based research–Tension between practical relevance and knowledge generation–What can we learn from projects?. Eurasia Journal of Mathematics, Science and Technology Education , 20(1), em2378. https://doi.org/10.29333/ejmste/13928
Haagen-Schützenhöfer, Claudia et al. "Design-based research–Tension between practical relevance and knowledge generation–What can we learn from projects?". Eurasia Journal of Mathematics, Science and Technology Education , vol. 20, no. 1, 2024, em2378. https://doi.org/10.29333/ejmste/13928

Maintenance work is planned for Wednesday 1st May 2024 from 9:00am to 11:00am (BST).
During this time, the performance of our website may be affected - searches may run slowly and some pages may be temporarily unavailable. If this happens, please try refreshing your web browser or try waiting two to three minutes before trying again.
We apologise for any inconvenience this might cause and thank you for your patience.
Chemical Society Reviews
The mechanism of water oxidation using transition metal-based heterogeneous electrocatalysts.

* Corresponding authors
a Key Laboratory of Applied Surface and Colloid Chemistry, Ministry of Education, School of Chemistry and Chemical Engineering, Shaanxi Normal University, Xi’an 710119, P. R. China E-mail: [email protected] , [email protected] , [email protected]
The water oxidation reaction, a crucial process for solar energy conversion, has garnered significant research attention. Achieving efficient energy conversion requires the development of cost-effective and durable water oxidation catalysts. To design effective catalysts, it is essential to have a fundamental understanding of the reaction mechanisms. This review presents a comprehensive overview of recent advancements in the understanding of the mechanisms of water oxidation using transition metal-based heterogeneous electrocatalysts, including Mn, Fe, Co, Ni, and Cu-based catalysts. It highlights the catalytic mechanisms of different transition metals and emphasizes the importance of monitoring of key intermediates to explore the reaction pathway. In addition, advanced techniques for physical characterization of water oxidation intermediates are also introduced, for the purpose of providing information for establishing reliable methodologies in water oxidation research. The study of transition metal-based water oxidation electrocatalysts is instrumental in providing novel insights into understanding both natural and artificial energy conversion processes.
Article information
Download citation, permissions.

S. Yang, X. Liu, S. Li, W. Yuan, L. Yang, T. Wang, H. Zheng, R. Cao and W. Zhang, Chem. Soc. Rev. , 2024, Advance Article , DOI: 10.1039/D3CS01031G
To request permission to reproduce material from this article, please go to the Copyright Clearance Center request page .
If you are an author contributing to an RSC publication, you do not need to request permission provided correct acknowledgement is given.
If you are the author of this article, you do not need to request permission to reproduce figures and diagrams provided correct acknowledgement is given. If you want to reproduce the whole article in a third-party publication (excluding your thesis/dissertation for which permission is not required) please go to the Copyright Clearance Center request page .
Read more about how to correctly acknowledge RSC content .
Social activity
Search articles by author.
This article has not yet been cited.
Advertisements
Fachdidaktischer Forschungsverbund FaBiT: Erforschung von Wandel im Fachunterricht mit dem Bremer Modell des Design-Based Research
- First Online: 11 August 2016
Cite this chapter
- Maria Peters 3 &
- Bàrbara Roviró 4
2463 Accesses
7 Citations
Zusammenfassung
Das Bremer Design-Based Research-Modell wird in seinen Dimensionen, Bedingungen und Erkenntnismöglichkeiten zur Erforschung von Wandel in fachbezogenen Lehr-Lernprozessen dargestellt. Eine Kontextualisierung des Modells durch unterschiedliche Ansätze von design research in ihrer Genese seit Ende des letzten Jahrhunderts zeigt Bezüge zu weiteren Varianten der Praxisforschung auf.
This is a preview of subscription content, log in via an institution to check access.
Access this chapter
- Available as PDF
- Read on any device
- Instant download
- Own it forever
- Compact, lightweight edition
- Dispatched in 3 to 5 business days
- Free shipping worldwide - see info
Tax calculation will be finalised at checkout
Purchases are for personal use only
Institutional subscriptions
Unable to display preview. Download preview PDF.
Bibliographie
Barab, S. & Squire, K. (2004): Design-Based Research. Putting a Stake in the Ground. In: The journal of the learning sciences, 13 (1), 1–14. Online: http://www.jstor.org/stable/1466930 . (23.01.2016)
Bereiter, C. (2002): Design Research for Sustained Innovation. In: Cognitive Studies, Bulletin of the Japanese Cognitive Science Society, 9 (3), 321–327. Online: https://www.jstage.jst.go.jp/browse/jcss/9/3/_contents . (23.01.2016)
Cobb, P., Confrey, J., diSessa, A., Lehrer, R. & Schauble, L. (2003): Design Experiments in Educational Research. In: Educational Researcher, 32 (1), 9–13. Online: http://edr.sagepub.com/content/32/1.toc . (06.03.2016)
Collins, A., Joseph, D. & Bielaczyc, K. (2004): Design Research: Theoretical and Methodological Issues. In: The journal of the learning sciences, 13 (1), 15–42. Online: http://www.jstor.org/stable/i263858 . (06.03.2016)
Design-Based Research Collective (2003): Design-Based Research. An Emerging Paradigm for Educational Inquiry. In: Educational Researcher, 32 (1), 5–8. Online: http://edr.sagepub.com/content/32/1.toc . (Online: 06.03.2016)
Hußmann, St., Thiele, J., Hinz, R., Prediger, S. & Ralle, B. (2013): Gegenstandsorientierte Unterrichtsdesigns entwickeln und erforschen. Fachdidaktische Entwicklungsforschung im Dortmunder Modell. In: Komorek, M. & Prediger, S. (Hrsg.): Der lange Weg zum Unterrichtsdesign - Zur Begründung und Umsetzung fachdidaktischer Forschungs- und Entwicklungsprogramme. Münster: Waxmann Verlag, 25–42
Google Scholar
Klep, J., Letschert, J. & Thijs, A. (Hrsg.) (2004): What are we going to learn? Choosing educational content. SLO, Enschede. Online: https://www.academia.edu/4947719/What_are_we_going_to_learn . (24.01.2016)
McKenney, S., Nieveen, N. & van den Akker, J. (2006): Design research from a curriculum perspektive. In: Van den Akker, J., Gravemeijer, K., McKenney, S. & Nieveen, N. (Hrsg.): Educational Design Research. London [u.a.]: Routledge, 67–90
Plomp, T. (2010): Educational Design Research: an Introduction. In: Plomp, T. & Nieveen, N. (Hrsg.): An introduction to educational design research. Proceedings of the seminar conducted at the East China Normal University, Shanghai (PR China), November 23-26, 2007. 3rd print. Enschede: SLO, 9–36
Prediger, S., Gravemeijer, K. & Confrey, J. (2015): Design research with a focus on learning processes. An overview on achievements and challenges. In: ZDM, 47 (6), 877–891
Prediger, S. & Link, M. (2012): Fachdidaktische Entwicklungsforschung. Ein lernprozessfokussierendes Forschungsprogramm mit Verschränkung fachdidaktischer Arbeitsbereiches. In: Bayrhuber, H., Harms, U., Muszynski, B., Ralle, B., Rothgangel, M., Schön, L.-H., Vollmer, H. J. & Weigand, H.-G. (Hrsg.): Formate fachdidaktischer Forschung. Empirische Projekte - historische Analysen - theoretische Grundlegungen. Münster [u.a.]: Waxmann Verlag, 29–45
Prediger, S., Link, M., Hinz, R., Hussmann, St., Ralle, B. & Thiele, J. (2012): Lehr-Lernprozesse initiieren und erforschen. Fachdidaktische Entwicklungsforschung im Dortmunder Modell. In: MNU - Der mathematische und naturwissenschaftliche Unterricht, 65 (8), 452–457. Online: http://www.mathematik.uni-dortmund.de/~prediger/veroeff/12Prediger_et_al_MNU_FUNKEN_Webversion.pdf . (08.10.2015)
Reinmann, G. (i. Dr.): Design-Based Research. In: Schemme, D. & Novak, H. (Hrsg.): Gestaltungsorientierte Forschung in Innovations- und Entwicklungsprogrammen. Potenzial für Praxisgestaltung und Theoriebildung. Bonn: Bundesinstitut für Berufsbildung
Reinmann, G. (2005): Innovation ohne Forschung? Ein Plädoyer für den Design-Based Research-Ansatz in der Lehr-Lernforschung. In: Unterrichtswissenschaft. Zeitschrift für Lernforschung, 33 (1), 52–69
Van den Akker, J., Gravemeijer, K., McKenney, S. & Nieveen, N. (Hrsg.): Educational Design Research. London [u.a.]: Routledge
Zwetzschler, L. & Prediger, S. (2013): Der lange Weg zum Herstellen von Beziehungen. Fachdidaktische Entwicklungsforschung zur Gleichwertigkeit algebraischer Terme. In: Komorek, M. & Prediger, S. (Hrsg.): Der lange Weg zum Unterrichtsdesign − Zur Begründung und Umsetzung fachdidaktischer Forschungs- und Entwicklungsprogramme. Münster: Waxmann Verlag, 141–156
Download references
Author information
Authors and affiliations.
Fachbereich 9, Universität Bremen, Bibliothekstr. 1, 28359, Bremen, Deutschland
Maria Peters
Fachbereich 10, Universität Bremen, Bibliothekstr. 1, 28359, Bremen, Deutschland
Bàrbara Roviró
You can also search for this author in PubMed Google Scholar
Corresponding author
Correspondence to Maria Peters .
Editor information
Editors and affiliations.
FB Sprach- u. Lit.wiss., Universität Bremen FB Sprach- u. Lit.wiss., Bremen, Germany
Sabine Doff
Zentrum für Lehrerbildung, Universität Bremen Zentrum für Lehrerbildung, Bremen, Germany
Regine Komoss
Rights and permissions
Reprints and permissions
Copyright information
© 2017 Springer Fachmedien Wiesbaden
About this chapter
Peters, M., Roviró, B. (2017). Fachdidaktischer Forschungsverbund FaBiT: Erforschung von Wandel im Fachunterricht mit dem Bremer Modell des Design-Based Research. In: Doff, S., Komoss, R. (eds) Making Change Happen. Springer VS, Wiesbaden. https://doi.org/10.1007/978-3-658-14979-6_2
Download citation
DOI : https://doi.org/10.1007/978-3-658-14979-6_2
Published : 11 August 2016
Publisher Name : Springer VS, Wiesbaden
Print ISBN : 978-3-658-14978-9
Online ISBN : 978-3-658-14979-6
eBook Packages : Social Science and Law (German Language)
Share this chapter
Anyone you share the following link with will be able to read this content:
Sorry, a shareable link is not currently available for this article.
Provided by the Springer Nature SharedIt content-sharing initiative
- Publish with us
Policies and ethics
- Find a journal
- Track your research
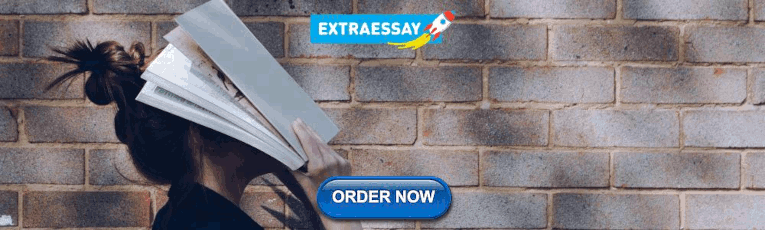
IMAGES
VIDEO
COMMENTS
Recent calls in biology education research (BER) have recommended that researchers leverage learning theories and methodologies from other disciplines to investigate the mechanisms by which students to develop sophisticated ideas. We suggest design-based research from the learning sciences is a compelling methodology for achieving this aim. Design-based research investigates the "learning ...
The first phase of design-based research is the analysis and exploration, which includes problem identification and diagnosis. As noted by Bannan-Ritland ( 2003 ): "The first phase of design-based research is rooted in essential research steps of problem identification, literature survey, and problem definition" (p. 22).
Design research became more widely known in 2003 and 2004 with special issues of the Educational Researcher(C obb, Confrey, diSessa, Lehrer, andSchauble 2003; De-sign-based research collective 2003) and the Journal of the Learning Sciences (Barab and Squire 2004). Especially the article by Cobb et al. (2003) became a reference point,
1.4 Four intertwined working areas for PD Design Research Figure 1 shows the four working areas that are iteratively connected in the design and research process, adapted from Prediger and Zwetzschler ) for PD design re- (2013 search. The four working areas comprise (a) specifying and structuring PD goals and
Design-based research methods are a thirty-year old tradition from the learning sciences that have been taken up in many domains as a way to study designed interventions that challenge the traditional relationship between research and design, as is the case with online learning. Key to the contribution and coherence of this method are different ...
Thus, modifications and tweaking were necessary and were carefully documented and carried out using a modified design based research cycle (Prediger & Gravemeijer, 2019), as will now be described. ...
This overview summarizes the origins and the current state of design research practices focusing on methodological requirements and processes of theorizing, with one being focused on curriculum innovations, and one focused on developing theories on the learning processes, which is the main focus of the thematic issue. Design research continues to gain prominence as a significant methodology in ...
Summary. Design-based research (DBR) is a methodology used to study learning in environments that are designed and systematically changed by the researcher. The goal of DBR is to engage the close study of learning as it unfolds within a particular context that contains one or more theoretically inspired innovations and then to develop new ...
Design-based research (Brown, 1992; Collins, 1992) is an emerging paradigm for the study of learning in context through the systematic design and study of instructional strategies and tools. We argue that design-based research can help create and extend knowledge about developing, enacting, and sustaining in-
The Design-Based Research Collective is a group of faculty and researchers founded to examine, improve, and practice design-based research methods in education. The group's members all blend research on learning and the design of educational interventions.
Prediger et al., 2013; Schecker & Hopf, 2021) within which different approaches have emerged. In recent years, particularly approaches with a strong focus on research- and evidence-based curriculum development have often been framed within the design-based research (DBR) paradigm (Mikelskis & Mikelskis, 2010;
This chapter provides an overview and selected insights for novice researchers who want to find out if didactical design research is suitable for their own projects, and what possible research outcomes can look like. Design research has become a powerful research methodology of increasing relevance in mathematics education research. This chapter provides an overview and selected insights for ...
Design-based research has no specific requirements for the form that instructional tools must take or the manner in which the tools are evaluated (Bell, 2004; Anderson and Shattuck, 2012).Instead, design-based research has what Sandoval (2014) calls "epistemic commitments" 1 that inform the major goals of a design-based research project as well as how it is implemented.
Die Begriffe „ Design-Based Research " (Design-Based Research Collective, 2003), „ Design Research ", „fachdidaktische Entwicklungsforschung" (Prediger und Link 2012) oder „Didaktik als Design Science " (Fischer et al. 2005) bezeichnen alle diese Grundidee. Der Ertrag entsprechender Forschung besteht in Entwicklungsprodukten, z ...
This chapter arose from the need to introduce researchers, including Master and PhD students, to design-based research (DBR). In Sect. 16.1 we address key features of DBR and differences from other research approaches. We also describe the meaning of validity and reliability in DBR and discuss how they can be improved. Section 16.2 illustrates DBR with an example from statistics education.
Schauble, and Putz 2000). Most design research with a focus on mathematics learning processes is informed by. grand learning theorists such as Piaget, Vygotsky and Dewey and their intellectual ...
Researchers often develop teaching-learning solutions to improve the quality of instruction. Some of these solutions are developed in the paradigm of design-based research (DBR). The output of DBR projects goes beyond design products for practice and includes contributions to local theories about teaching-learning in specific subject areas and contexts as well as knowledge about how to design ...
Design research is an evolving methodology with substantial variation among concrete approaches, and yet with common features. We briefly sketch the origins of design research (in Sect. 2.1) and identify dimensions of variability (Sect. 2.2).Distinguishing two archetypes, 'design research with a focus on curriculum products', and 'design research with a focus on learning processes' (in ...
3. Explikation des Forschungsansatzes Design-Based Research. In diesem Kapitel erfolgt die allgemeine Vorstellung des DBR-For-schungsansatzes. Dabei wird in Kapitel 3.1 zunächst die Entwicklung des DBR-Ansatzes angerissen. Danach wird eine allgemeine Begriffsklärung vorgenommen, bevor eine exemplarische Definition des DBR-Ansatzes aufgeführt ...
18-BzMU_Strukturen-DesignResearch-Webversion. Webversion des Beitrags Prediger, Susanne (2018). Design-Research als fachdidaktisches Forschungsformat: Am Beispiel Auffalten und Verdichten mathematischer Strukturen. In Fachgruppe für Didaktik der Mathematik der Universität Pader-born (Hrsg.), Beiträge zum Mathematikunterricht (S. 33-40).
Gene editing has the potential to solve fundamental challenges in agriculture, biotechnology, and human health. CRISPR-based gene editors derived from microbes, while powerful, often show significant functional tradeoffs when ported into non-native environments, such as human cells. Artificial intelligence (AI) enabled design provides a powerful alternative with potential to bypass ...
Design-based research (Brown, 1992; Collins, 1992) is an emerging paradigm for the study of learning in context through the systematic design and study of instructional strategies and tools. We argue that design-based research can help create and extend knowledge about developing, enacting, and sustaining in-
Design-based research is not so much an approach as it is a series of approaches, with the intent of producing new theories, artifacts, and practises that account for ... Der Design-Based Research-Ansatz leistet nach Prediger einen bedeutenden Beitrag zur fachdidaktischen Forschung, indem das Theoretisieren als ein konti-
To design effective catalysts, it is essential to have a fundamental understanding of the reaction mechanisms. This review presents a comprehensive overview of recent advancements in the understanding of the mechanisms of water oxidation using transition metal-based heterogeneous electrocatalysts, including Mn, Fe, Co, Ni, and Cu-based catalysts.
Das Bremer Design-Based Research-Modell wird in seinen Dimensionen, Bedingungen und Erkenntnismöglichkeiten zur Erforschung von Wandel in fachbezogenen Lehr-Lernprozessen dargestellt. Eine Kontextualisierung des Modells durch unterschiedliche Ansätze von design research in ihrer Genese seit Ende des letzten Jahrhunderts zeigt Bezüge zu ...