Free PDF: The 4 questions every SOP must answer → Master’s or PhD

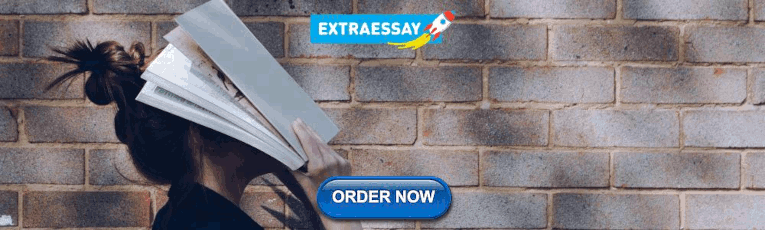
The Data Science Statement of Purpose: A Guide with Examples
- By Jordan Dotson
- Updated: April 28, 2023

Ready to start writing your Data Science statement of purpose? Well, it’s your lucky day. This article isn’t just a “how to” guide — it’s an object lesson in so many of the common anxieties grad applicants face every year:
- How do I write about overcoming obstacles (health, low grades, family death) as an undergraduate?
- How do I describe my career path (I’ve been away from school for awhile)?
- Should I mention MOOCs in my SOP?
- Can I get admitted with no research experience?
- Can I get admitted if I wasn’t a Data Science or CS major in undergrad?
The sample essay you’re about to read and model is a perfect answer to these questions. Why? Because the applicant, despite his circuitous background and previous academic struggles, earned admission to 6 of the best MSDS programs in the US .
To protect the author’s privacy, we won’t name the schools. But rest assured, it’s a “Who’s Who” list of sterling, fancy-pants universities that you’re definitely also considering. Thus, this isn’t just a brilliant data science statement of purpose — it’s a brilliant SOP in general. The author employed this framework successfully for both DS and CS programs, and honestly, ANY applicant in ANY field can use this essay as inspiration…
…and hopefully achieve the same wild success as my fascinating friend, Bennett.
The Student
As an applicant, Bennett ticked a lot of boxes:
- First-gen college student and child of immigrants;
- Undergrad Cognitive Science major at elite state university;
- Modest, less-than-perfect GPA;
- Multiple DS certifications with supplemental CS coursework (essentially self-taught);
- Online Executive MBA graduate;
- 4 years of post-undergrad work experience;
- Extensive work experience during undergrad;
- ZERO research experience
Some aspects of Bennett’s profile were fascinating. (He was an NSA analyst in undergrad!) Other parts were fairly normal. (No research, average GPA.)
What then made Bennett and his SOP so special? What made top MSDS programs excited to admit him?
The Structure of a Successful Data Science SOP
It goes without saying that Bennett used the SOP Starter Kit to outline his essay. That means he structured the paragraphs as follows:
- Introduction Frame Narrative – 1 paragraph (12% of word count)
- Why This Program – 2 paragraphs (23% of word count)
- Why I’m Qualified – 4 paragraphs (58% of word count…extremely long, but more on this later)
- Concluding Frame Narrative – 1 paragraph (7% of word count)
Before we read the actual essay, let’s examine these sections and see how you can mirror Bennett’s example in your own SOP.
1. Introduction Frame Narrative
In the intro, Bennett describes his work as a software engineer. He gets specific. He tells us exactly what he does, and the company he does it for. Most importantly, he describes a moment when he discovered a new intellectual purpose at work:
“Thus, for the first time, I was able to personalize parameters in the pipeline for unaccounted customers. Learning the importance of context for efficient yet equitable automation, I found myself incredibly curious about data-modeling methodologies that can truly represent real-world situations .”
You should do the same as Bennett. Your intro should have some color, some life. It should allow us to see a real human being in there. But it MUST also introduce the sub-niche intellectual problems you hope to tackle in grad school. Chances are, these problems and this sub-niche will define your professional career afterward. They’re the hinge of your whole candidacy.
Common Question #1: “What if I don’t know which sub-niche I want to specialize in?”
Find one. (The SOP Starter Kit has an exercise that will help you figure this out.) Otherwise, you won’t be as competitive as you could be.
Common Question #2: “What if I don’t have an interesting moment (or moments) to write about?”
Stop lying to yourself. No matter where you are, no matter what you’ve done, there was a moment when you decided you needed a graduate degree. There is absolutely a subfield of data science that’s most interesting to you. There are undoubtedly specific applications, in specific industries, you want to work on in the future. How did you discover them?
Bennett wants to study representation of data minorities in ML models for the healthcare industry. That’s the work he wants to do in the future. What kind of work do you want to do in the future? When did you realize this?
That’s the story you tell in your Introduction.
2. Why This Program
Either at the end of your introduction, or in the beginning of this new section, you’re going to include a Sentence of Purpose . It’s a thesis statement for your essay. Bennett’s looks like this:
“Through Gotham University’s Master’s program in Data Science, I hope to further explore how to enhance representation of data minorities in ML models, and thus ensure inclusive healthcare access for the customers I serve.”
The “Why This Program” section of your SOP provides all the evidence for how you’ll pursue this goal in grad school. It should take about 2 paragraphs. Which classes will you take? Which professors do you hope to work with? What will you study in your capstone project?
Let’s make this easy. Just complete the exercise in this article: How to Dominate Your SOP’s Why This Program Section . Trust me, it’s that easy! Then, you’re halfway done with your essay.
3. Why I’m Qualified
This section of your SOP is the easiest to write. It’s your “greatest hits” list – all the proof that you’re a smart student. Everything you write here should support the argument that you’re going to succeed in grad school: your GPA, advanced classes you’ve taken, research experience, etc. It doesn’t have to be long, and shouldn’t include every menial detail of every project you’ve ever done. (That’s what the CV is for .)
Yet, if it shouldn’t be long…why did Bennett write 4 paragraphs?!
Typically, I’d yell and scream at an applicant who spends half the SOP talking about his past credentials. That’s what most applicants do, and why most get rejected .
But Bennett had a unique situation.
His career was wild and fascinating. He’d never formally studied Data Science. He’d even done an MBA. But he had taken lots of MOOCS and online certification courses (seriously, like 10+), he did have amazing experience as a software engineer, and he also had one bad undergrad semester he felt he needed to explain. Thus, he’s a very atypical applicant, and his background required a lot of explaining.
Unless you too have an MBA, 10+ MOOCs, and a completely unrelated major, then I suggest you keep your “Why I’m Qualified” section much shorter – 2 paragraphs is enough.
4. Concluding Frame Narrative
If you have the previous sections in order, this final paragraph should write itself. Make sure to reemphasize the topical problem (your hopeful subfield) from the Intro Frame Narrative. Consider including a career goals statement . But in the end, this section should be easy to write.
That’s it. Four sections, tightly interwoven, all supporting the argument that you are going to be an A+ data science grad student. Now, let’s see how Bennett brought it altogether, so you can attempt to do the same.
A Brilliant Data Science Statement of Purpose
As a software engineer with WayneHealth Group, I maintain data pipelines and batch processing in the modernization team. In 2020, following a health check on existing infrastructure, I discovered that pipelines were delivering data too slowly to clients. After comparing our runtimes to industry standards, I pitched a project using open-source Apache Airflow to help automate pipelines and centralize patient data into a single workflow. However, when considering how to automate 35% of the data, I learned from the billing team how frequently bills are refinanced in our long-term elderly care programs. Thus, for the first time, I was able to personalize parameters in the pipeline for unaccounted customers. Learning the importance of context for efficient yet equitable automation, I found myself incredibly curious about data-modeling methodologies that can truly represent real-world situations.
Through Gotham University’s Master’s program in Data Science, I hope to further explore how to enhance representation of data minorities in ML models, and thus ensure inclusive healthcare access for the customers I serve. Earning my MBA at Metropolis University taught me how to coordinate the need for quantitative reasoning and human intuition through A/B testing, and I believe the MSDS program will build on that foundation. Mathematical Foundations in Computer Science , for example, will help me build real-time analytics dashboards that account for insurance claim data-entry errors through discrete probabilistic models. In the same vein, elective offerings such as Big Data Analytics and Artificial Intelligence will enable me to choose predictive models and evaluate their accuracy when applied to large data sets — particularly useful when predicting whether an insurance claim will necessitate revisions.
Resources like the IGNITE competition will also offer opportunities to collaborate on flexible models that solve real-world situations. Having worked on Apache Airflow implementation in WayneHealth, I understand how collaboration can play a key role in implementing a new idea. Having my IGNITE team’s project evaluated by MSDS professors, with their expertise in modular design and user experience, will only help me evaluate my own performance as I translate my education into functional healthcare applications. Thus, I am certain that Gotham’s MSDS program will prepare me to succeed in a team setting that balances many developer roles, while equipping me to better deliver sales pitches to investors.
Upon graduating, I endeavor to apply my education toward applied healthcare projects that focus on providing easy access to preventative care. Transparency is an integral part of healthcare access because it reduces the expenses and time necessary to find patient care. To help facilitate this transparency, I plan to transition into a Senior Data Scientist role in the Emerging Technologies Collaborative (ETC) at WayneHealth, hopefully working on projects that implement data-driven recommendations for our automated batch processes and servers. When storing vast amounts of patient data across different platforms, vulnerability patches and triage alerts often lead to reactive outcomes that can create downtime for end users. As a result, I seek to implement agentless server monitoring to, first, predict unscheduled outages for our billing and medical coverage systems, and second, recognize patterns in server behavior. Helping recognize outage patterns will not only help me identify problems beforehand, but also decipher the causes of live servers crashing. However, projects outside of WayneHealth excite me as well, including Amgen’s Crystal Bone algorithm which uses AI and machine-learning models to detect bones at risk for osteoporotic fractures. This project was the first tool I have ever seen that uses diagnostic codes sourced from WayneHealth electronic health records, and it inspired me to create my own model using EHR data. In the future, I hope to use EHR diagnostic codes to predict the cost of treatment for those prone to risk, as indicated by the algorithm.
Not only has my position as a software engineer equipped me with strong technical skills, but it has also given me the discipline to continuously learn what I do not yet know. On a project named Karra, an optical-character recognition engine which scans personal information from faxed hospital claim forms, I learned how to develop my own algorithms to calculate the coordinates of form fields to parse data. The technical skills I have gained, in tandem with the unwavering tenacity I developed in this position, will allow me to face any challenge that arises during the MSDS program.
To further prepare for the rigors of the MSDS program, I completed University of Pennsylvania Engineering MOOCs on Coursera, including the Introduction to Python and Java specialization taught by Brandon Krakowsky and the course Computational Thinking for Problem Solving by Susan Davidson. These MOOCs helped me comprehend important programming paradigms such as unit testing and debugging, which will help me test edge cases in MSDS course projects. Also, MOOCs from UC San Diego, such as Python for Data Science and Probability and Statistics in Data Science Using Python , enabled me to optimize data-cleansing techniques for better runtimes. The MCDS program’s Big Data Analytics course will culminate this self-learning effort, providing a solid theoretical understanding of the tools and techniques used to extract insights from large datasets.
While I have taken on a breadth of challenging problems in computer science and implemented solutions at WayneHealth, my prior undergraduate performance did not always reflect my best ability. Between Spring 2016 and Spring 2017, I experienced a personal health challenge that required substantial time away from the UC Coast City campus. I was further distracted by the realities of personally financing my education – working full-time for the National Security Agency (NSA) – while also suffering the loss of a close family member. Even as I struggled I knew the importance of higher education, and, advocating for my own success, I persisted. To strengthen my educational background, I enrolled in online courses and built coping mechanisms, such as managing my time between online courses and on-campus courses efficiently. In the end, these efforts helped me graduate early in the fall of 2018, and I plan to apply the same level of resilience throughout the rest of my academic and professional career.
As I grow increasingly aware of the intersection between ML and social computing, I am determined to study learning techniques such as principal component analysis, and to perform research in data organization/completeness. With my strong self-guided background in applied computer science, and my professional experience with ML and software development in the healthcare insurance industry, the practical knowledge I build at Gotham will help me make voices heard in the data we interact with in our daily lives.
What Makes This SOP Truly Special?
Some might argue that Bennett’s essay doesn’t fit the template described in the SOP Starter Kit. I disagree. The virtuosity of Bennett’s writing shows that the model is adaptable to all kinds of intellectual demands.
(In fact, he’s pointed out himself that the framework helped beautifully with his Computer Science SOPs, which should give confidence to anyone who may be still deciding between DS and CS.)
Personally, I love how Bennett began his Why I’m Qualified section with an expanded Career Goals Statement. It shows us, in painstaking detail, exactly what he’s going to achieve if the school admits him:
“Upon graduating, I endeavor to apply my education toward applied healthcare projects that focus on providing easy access to preventative care.”
There are real data problems in the healthcare insurance industry. Bennett is all-too-familiar with them. Few if any other applicants will ever be able to solve these problems the way he will. We know this because he tells us exactly what he’s going to do in his career afterward :
- Pursue a Senior Data Scientist role in his company;
- Automate batch processes and servers to predict unscheduled outages medical coverage systems;
- And use EHR diagnostic codes to predict treatment costs for high-risk patients.
In this way, Bennett’s expansive, thoughtful SOP makes certain that he isn’t just a boring applicant looking to acquire base knowledge in data science. He already has it! He got it for free from Coursera!
Instead, it shows that he’s deadly focused on his unique sub-niche — solving real data problems in the healthcare insurance industry — and will do everything it takes to succeed. Thus, when Bennett discusses the many obstacles he overcame in the past, we don’t worry about them. We have tremendous confidence in Bennett because he’s already succeeded. He’s already acquired great expertise. And he knows exactly what he needs to do to make an impact in the future.
Though the middle paragraphs are somewhat long, they never feel boring or clunky. They feel intelligent and interesting. Finally, when we get to the last paragraph, we can’t help feeling certain of one thing: “Wow, this guy is unstoppable.”
As you start planning your own data science statement of purpose, there’s one aspect of Bennett’s essay you should mimic. It’s not the MOOCs , his atypical background, or the obstacles he overcame. It’s this:
Bennett mapped out the intellectual problems he wants to study in grad school, and how he will address them pragmatically in his career afterward. It’s not a complex argument:
- In the last few years, I’ve grown fascinated with Problem X in Industry Z;
- At Gotham University, I plan to study Problem X in these specific ways;
- After graduating, I will be able to solve Problem X for companies in Industry Z;
- I know I’m capable of this because of my skills and record of success;
- Admission to Gotham is my immediate and necessary next step, so I hope we can begin solving these problems together.
I offer endless gratitude to Bennett for allowing me to share his story, his brilliant essay, and his resounding success. Data Science, Analytics, and Applied Statistics have become insanely competitive. But if you take the time to follow his example, you too can become a champion in the field, and start your journey toward solving unique problems that the world desperately needs you to solve.
Still need help structuring your own data science statement of purpose? The SOP Starter Kit will help, or, I’d love to hear from you !
Which data science problems do you plan to solve in grad school and beyond?
Need advice on other application essays? Check out our free guides!
- Structure is Magic: A Guide to the Graduate SOP
- Statement of Purpose for PhD Admission: A Universal Formula
- Diversity Statements 101: A Guide to All Personal Essays
Was this post helpful? Spread the love:
The sop starter kits.
These FREE (and highly insightful) guides will tell you exactly what to write, step-by-step, and leave you feeling super-confident and ready to hit “submit.”

© 2022 WriteIvy
[ninja_form id=3]
- Applying to Uni
- Apprenticeships
- Health & Relationships
- Money & Finance
Personal Statements
- Postgraduate
- U.S Universities
University Interviews
- Vocational Qualifications
- Accommodation
- Budgeting, Money & Finance
- Health & Relationships
- Jobs & Careers
- Socialising
Studying Abroad
- Studying & Revision
- Technology
- University & College Admissions
Guide to GCSE Results Day
Finding a job after school or college
Retaking GCSEs
In this section
Choosing GCSE Subjects
Post-GCSE Options
GCSE Work Experience
GCSE Revision Tips
Why take an Apprenticeship?
Applying for an Apprenticeship
Apprenticeships Interviews
Apprenticeship Wage
Engineering Apprenticeships
What is an Apprenticeship?
Choosing an Apprenticeship
Real Life Apprentices
Degree Apprenticeships
Higher Apprenticeships
A Level Results Day 2024
AS Levels 2024
Clearing Guide 2024
Applying to University
SQA Results Day Guide 2024
BTEC Results Day Guide
Vocational Qualifications Guide
Sixth Form or College
International Baccalaureate
Post 18 options
Finding a Job
Should I take a Gap Year?
Travel Planning
Volunteering
Gap Year Guide
Gap Year Blogs
Applying to Oxbridge
Applying to US Universities
Choosing a Degree
Choosing a University or College
Personal Statement Editing and Review Service
Guide to Freshers' Week
Student Guides
Student Cooking
Student Blogs
Top Rated Personal Statements
Personal Statement Examples
Writing Your Personal Statement
Postgraduate Personal Statements
International Student Personal Statements
Gap Year Personal Statements
Personal Statement Length Checker
Personal Statement Examples By University
Personal Statement Changes 2025
Personal Statement Template
Job Interviews
Types of Postgraduate Course
Writing a Postgraduate Personal Statement
Postgraduate Funding
Postgraduate Study
Internships
Choosing A College
Ivy League Universities
Common App Essay Examples
Universal College Application Guide
How To Write A College Admissions Essay
College Rankings
Admissions Tests
Fees & Funding
Scholarships
Budgeting For College
Online Degree
Platinum Express Editing and Review Service
Gold Editing and Review Service
Silver Express Editing and Review Service
UCAS Personal Statement Editing and Review Service
Oxbridge Personal Statement Editing and Review Service
Postgraduate Personal Statement Editing and Review Service
You are here
Data science personal statement example.
Ever since the commencement of high school studies, I was keen to know application of mathematics in factuality. Its abstract nature intrigued me to question my teachers about relevance and usefulness to mankind. I wanted to apply my learning and understanding invariably in real world circumstances.
It was until the introduction of Operations Research, while studying at college, which satiated my yearning to a certain extent. The problem solving and analytical techniques in Operations Research fostered the logic and decision making, which I believe, is one key component for developing models in real world or an approach towards mathematical modeling.
The process of creating mathematical representation of a real-world scenario to make predictions or provide insights captivated my interest. Although real world problems are often open ended and require iterative methods, but would also promote problem solving skills, creativity and innovation.
Besides Math, my interest in computing initiated right while coding the first program in C++ during bachelors. The backend scripting while using mobile phone, social media, scanning barcode at grocery or making online transactions, escalated my enthusiasm. I believed in the vigorous nature of programming and its effectiveness to engage world dynamically.
Also the symbiotic relation of computing and mathematics led me towards researching pathways blending the two disciplines, to evolve more powerful outcomes which would inherit the scientific and analytic approaches. While discussing it with a friend last year, I got introduced to the big data world and the interdisciplinary field of Data Science, combining aspects of statistics, mathematics, programming and domain expertise to address real problems. I was looking forward to traverse a similar realm and hence Data Science receives preference.
My previous studies helped to gain essential strategic and adaptive reasoning in the study of Algebra, Geometry, Statistics and Calculus. Integration of mathematics with computing widens the scopes and facilitates challenging yet interesting opportunities. This has instilled passion in me to explore computing specializations like Algorithms, Data structures and Programming fundamentals.
For the past six months I have been working diligently, enrolled in several courses to learn programming fundamentals with Python, SQL basics, Foundations of Data Science, k-Means clustering in Python and also Machine Learning concepts on self-learning platforms like Coursera, Udemy, Simplilearn and Youtube. Successful execution of programs like Conversion scales, time zones, board game and DNA processing using Python, exhilarated me and imparted encouragement. Although this helped me immensely to acquire foundational understanding of the subject. However, I needed more holistic education to gain mastery by pursuing formal studies and enrolling in university.
I started looking for reputed universities to pursue masters in Data science or related computer science streams. I figured that the role of data scientist is one of the most in-demand jobs currently in UK and US. Most likely its demand will originate in other regions of the world. My preference towards UK was obvious for couple of reasons. One year master’s program itself is an advantage from an international student perspective. Ever since inception, British universities are known for quality education and accreditation across the world. Many prominent world personalities from all walks of life, have graduated from English universities.
Joining on campus program at foreign land is much more than just universities. It is about the place, environment, people and also the facilities prevailing. As an expatriate living in Saudi Arabia, for about ten years, I have learnt this significantly. Cities like London, Birmingham, Manchester and Leeds provide residence to several expatriate communities exhibiting diverse vibrant cultures.
The world has reached a stage when every conceivable organization is becoming data-driven. Like the vast and ever-expanding universe, the big data fields are in a perpetual expansion mode, both fascinate me. Data is becoming more valuable in fast-paced life and this is creating a plethora of opportunities for data-centric roles in reputed organizations. It is no exaggeration to state that Data Science is making astonishing progress in the multiple domains of technology, economy, commerce and medicine.
After completing masters, I look forward to join established organization to acquire mastery and gain hands on experience in Data Science. However, time ahead, I would like to start a company where I can design advance models with the acquired knowledge and expertise from all the domains. For instance model like – ‘Accident free zones’ by collecting information from traffic flow, speed, regulations to make informed decisions regarding public safety or a ‘model school’ to be implemented by combining the best from several curriculums, adaptable for future generations.
For the past ten years I have been in the field of education, gaining expertise in teaching mathematics, designing curriculums and mentoring learning community. Although teaching-learning is my passion, and would definitely continue it as a hobby, however I am also a kind of person who believe in constant change and progress in life. This instinct supports me to accept demanding expeditions. My future endeavors would be to take slight detour from my current profession to establish myself in another exciting province, to leverage my knowledge, skills and career.
Profile info
There is no profile associated with this personal statement, as the writer has requested to remain anonymous.
This personal statement is unrated
Related Personal Statements
Add new comment.

- Conferences
- Last updated October 7, 2021
- In Innovation in AI
How To Write An Appealing Personal Statement For Masters Programme In Data Science
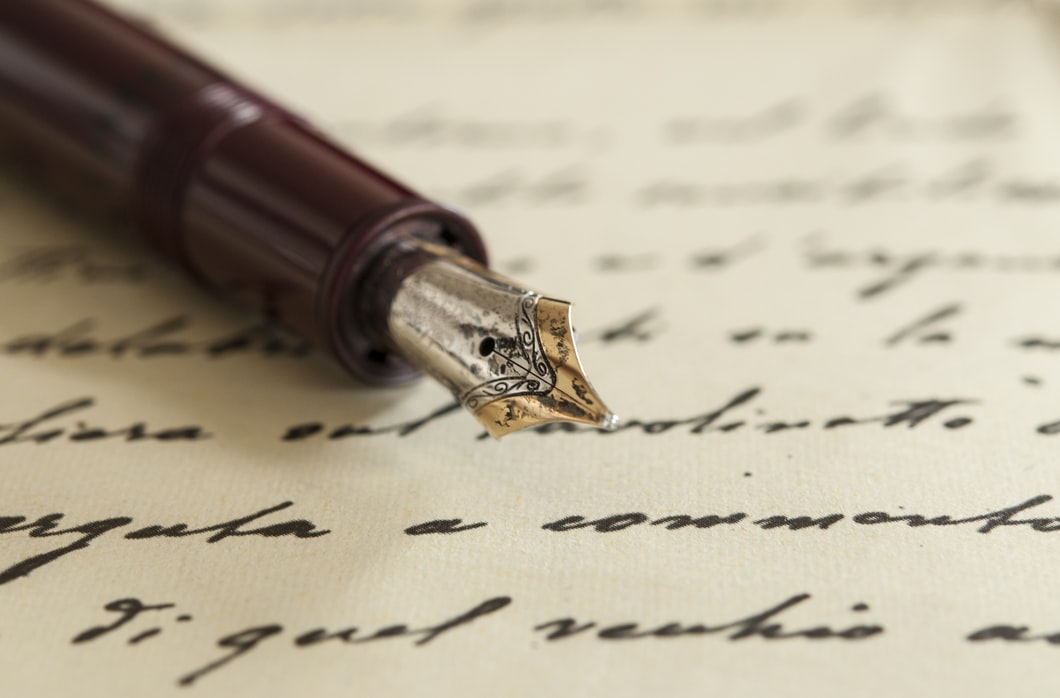
Illustration by How To Write An Appealing Personal Statement For Masters Programme In Data Science
- Published on July 31, 2020
- by Sejuti Das
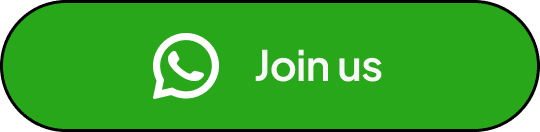
Besides submitting test scores, recommendation letters, and an undergraduate transcript, one essential requirement of applying to a data science masters programme is the application essay — aka personal statement. The personal statement is where applicants need to convince the professors their ability and worth of getting selected in the master programme .
In fact, many a time, a personal statement acts as a deciding factor for getting chosen for a prestigious masters programme in the field of data science . Thus, one needs to be extremely cautious while writing a personal statement for their master’s application. Not only does it help the university authorities determine the sincere interest of the applicant to enrol for the course, but also provide a chance to the students to stand out of the crowd highlighting their skills and relevancy.
Having said that, data scientists are experts in mathematics, but writing might not always be their expertise , and a personal statement is usually longer than you think and requires to be well crafted in order to grab the attention of professors and administrators. So, if decided to pursue higher studies in the data science field and have narrowed down universities to apply, this article can help you write a winning personal statement required to apply for the data science masters’ programme.
Also Read: The 10 Most Promising Data Science Masters Programs In US
Planning Is The Key: Highlight The Reason To Study Data Science Masters
Although it is stated as ‘personal,’ a personal statement doesn’t require applicants to share the intimate details of their life; instead, it needs to highlight the intention of the applicant for the particular master’s programme. To avoid any confusion or mistakes, the first step to write a personal statement for a data science masters programme is to brainstorm around it and plan it before actually starting to write. It is critical to make notes and use bullet points when planning, which can later be referred to while writing the personal statement. One should research thoroughly about the course requirements and the university, and prepare a list of their achievements and goals that can come handy while writing the essay.
Most universities expect their applicants to adhere to a specific word limit for the personal statement, and thus a good brainstorming will help applicants to keep their essay relevant and to the point. Planning will help in setting the context, creating a structure and forming a narrative of the piece that is critical for drafting a compelling statement.
Also Read: What Not To Include In Your Data Science Resume
Have A Killer Intro & A Concise Conclusion: Relevant To The Passion For The Field
An attention-grabbing intro and a hard-hitting conclusion are again critical for writing a compelling personal statement. The first paragraph can create the first impression of the applicant in front of the professors, and a sharp end will help them remember that candidate among the crowd. The readers of the personal statement are the experts from the data science industry and academics; thus, they expect the writeup to be extremely intriguing in terms of content.
Personal statements are usually lengthy but require to be extremely clear in sending out the message. Rather than starting the essay with some cliches, data science applicants should begin their personal statement highlighting their passion for the stream and their domain proficiency. And to have a definite ending, these data scientists must ensure to convey their genuine interest in pursuing the master’s programme, and how their skills are relevant to the stream.
Also Read: Tips And Templates For A Data Scientist Resume
Be A Good Story Teller: Highlight Experiences & Skill Sets
Thirdly, data science applicants must showcase their skill sets and experience in their personal statements without repeating the information that is already mentioned in their application form. And that’s why it is critical to be a good storyteller with their statement, where applicants can highlight their skills by talking about a particular data science research project that helped in solving real-life problems. One can also point out their experiences, knowledge, and quantify their expertise in the field that can help them in pursuing further studies.
The job of the personal statement is to let the administrators and professors know the abilities of the applicants to be qualified for the master’s programme. Data scientists can also mention their thesis, publications, journals or any relevant activities that can help them in getting selected. A well crafted personal statement avoids clichés, jargons, and too many details, and should be presented formally with a clear narrative.
Also Read: How To Create A Compelling Cover Letter To Land A Data Science Job
Focus On Your Domain & The Programme
Unlike undergraduate courses, masters programmes are more specific as well as require applicants to understand the domain they are pursuing. Consequently, while writing a personal statement, one needs to sync their interest according to the requirement of the programme and emphasise on the specific skills that match the area of expertise. One can also network with relevant faculty members and seniors to get a better understanding of the requirements of the program.
Many universities are also working on several ongoing data science projects, citing one of them corresponding to the interest, can also be a great addition to the personal statement. Furthermore, applicants can also write about what inspired them to pursue this particular domain and how their work will contribute to the field. One can also share their personal experiences and how that has helped in pursuing this course.
Also Read: What Data Science Graduates Need To Do To Get Hired During Covid-19
Don’t Be Generic: Customise The Write Up For The Course
Lastly, it is critical that the essay is unique and thus requires to be customised according to the university and its requirements. Applicants don’t have to start from scratch every time they are applying to a university, but they must ensure that they still provide a unique draft personal statement to each application. Professors and administrators read thousands of personal statements in a day, and therefore to be unique, applicants cannot pick up generic content to build their essay.
Applicants can also make the personal statement unique by adding up personal experiences relevant to the field, which will not only make the read interesting but also will allow the readers to empathise with the applicant. One can also add up a few of their failures to make it sound genuine as well as relatable. Usually, masters programmes don’t conduct face to face interviews; thus, personal statement plays a vital role in the applicants’ admission process.
Also Read: 10 Commonly Asked Puzzles In A Data Science Interview
Other Things To Keep In Mind
- Personal statements are not university applications, so don’t be repetitive.
- Highlight why this university is the right choice for the career you are planning to pursue.
- Although it’s the life experiences one shares in their personal statements, it indeed requires to be professional and to the point.
- Avoid grammar, spelling, and punctuation errors.
Access all our open Survey & Awards Nomination forms in one place
‘Diverse And Highly International’: North Carolina State University’s Masters In Data Science Program

IIT Roorkee Introduces 7 New Academic Programmes With Specialisation In AI & Data Science For 2021-22
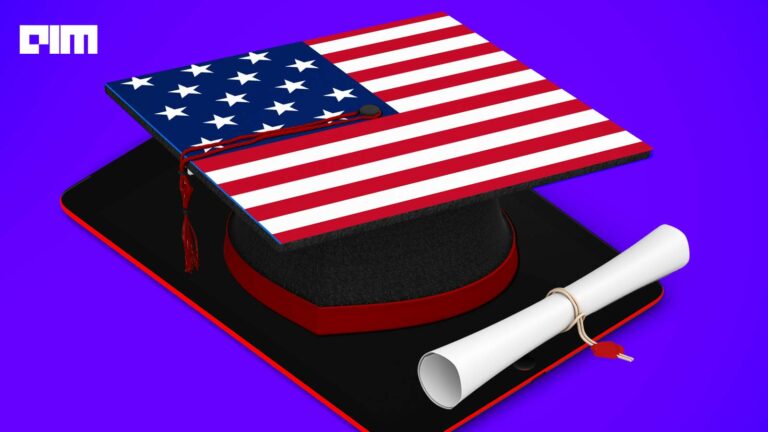
6 US Data Science Degrees That Can Be Obtained Online
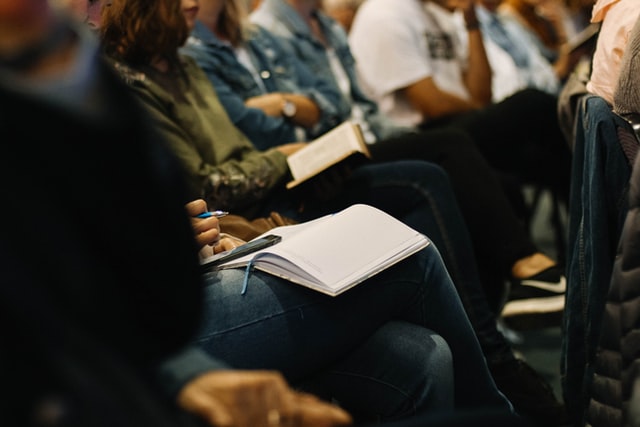
INSOFE Launches Dual Specialisation Master’s In Computer Science & AI
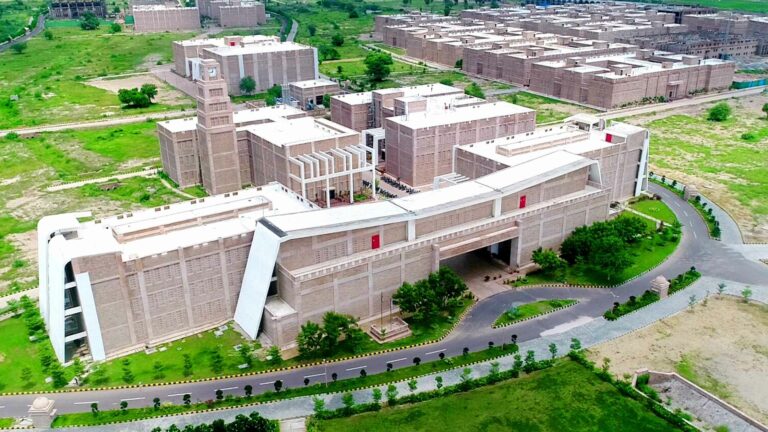
IIT Jodhpur To Offer B.Tech In AI & Data Science

Best Data Science Courses in India: Top Picks By AIM
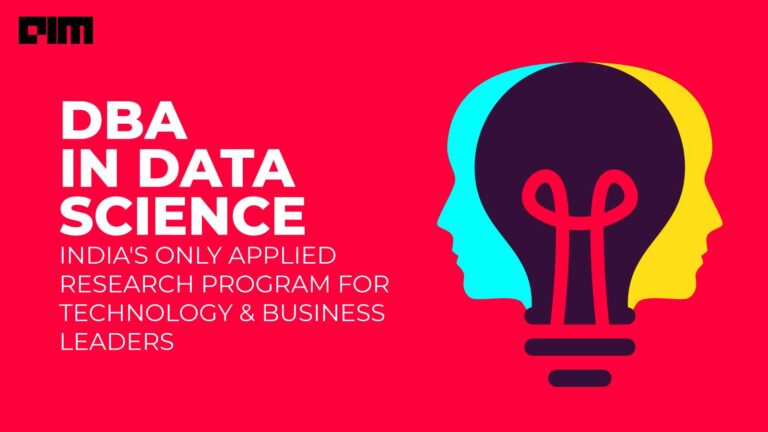
DBA In Data Science – India’s Only Applied Research Program For Technology And Business Leaders

Roadmap for Working Professionals to Pursue Data Science Education
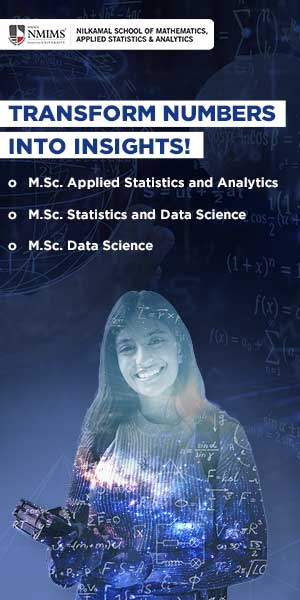
CORPORATE TRAINING PROGRAMS ON GENERATIVE AI
Generative ai skilling for enterprises, our customized corporate training program on generative ai provides a unique opportunity to empower, retain, and advance your talent., upcoming large format conference, data engineering summit 2024, may 30 and 31, 2024 | 📍 bangalore, india, download the easiest way to stay informed.

L&T Technology Services Trains 3,000 Engineers in GenAI
L&T Technology Services (LTTS) has so far conducted over 90 PoCs, and is working on nine use cases across segments.
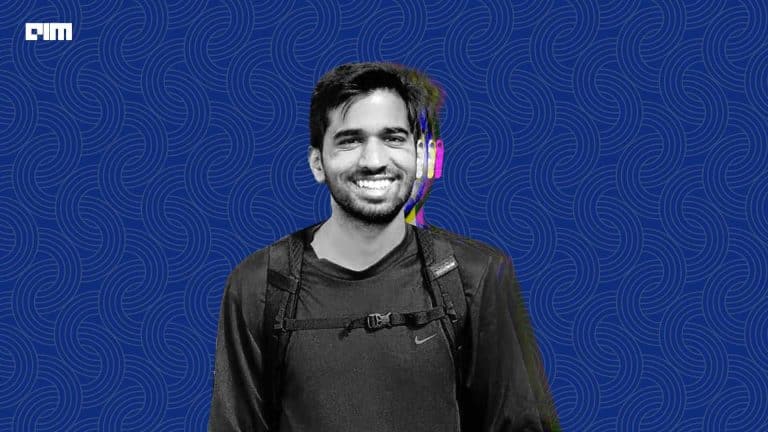
Meet the Child Prodigy from India Who Helped Build OpenAI’s GPT-4o

Intel is Bullish on India with its Xeon Processors
Top editorial picks, after stack overflow, openai partners with reddit , vmware makes workstation pro and fusion pro free for personal use, zoho ventures into chipmaking, plans $700m investment, openai hires google veteran to build ‘google search alternative’, subscribe to the belamy: our weekly newsletter, biggest ai stories, delivered to your inbox every week., also in news.
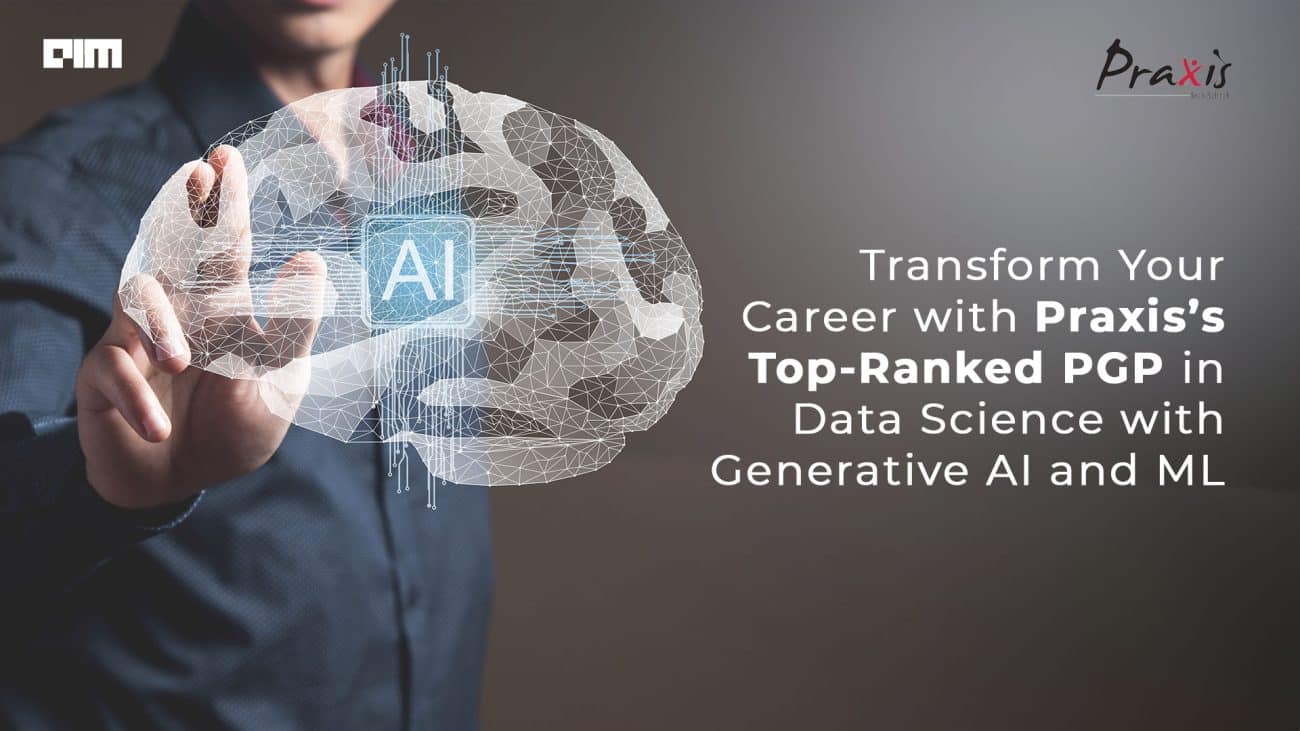
Transform Your Career with Praxis’s Top-Ranked PGP in Data Science with Generative AI and ML
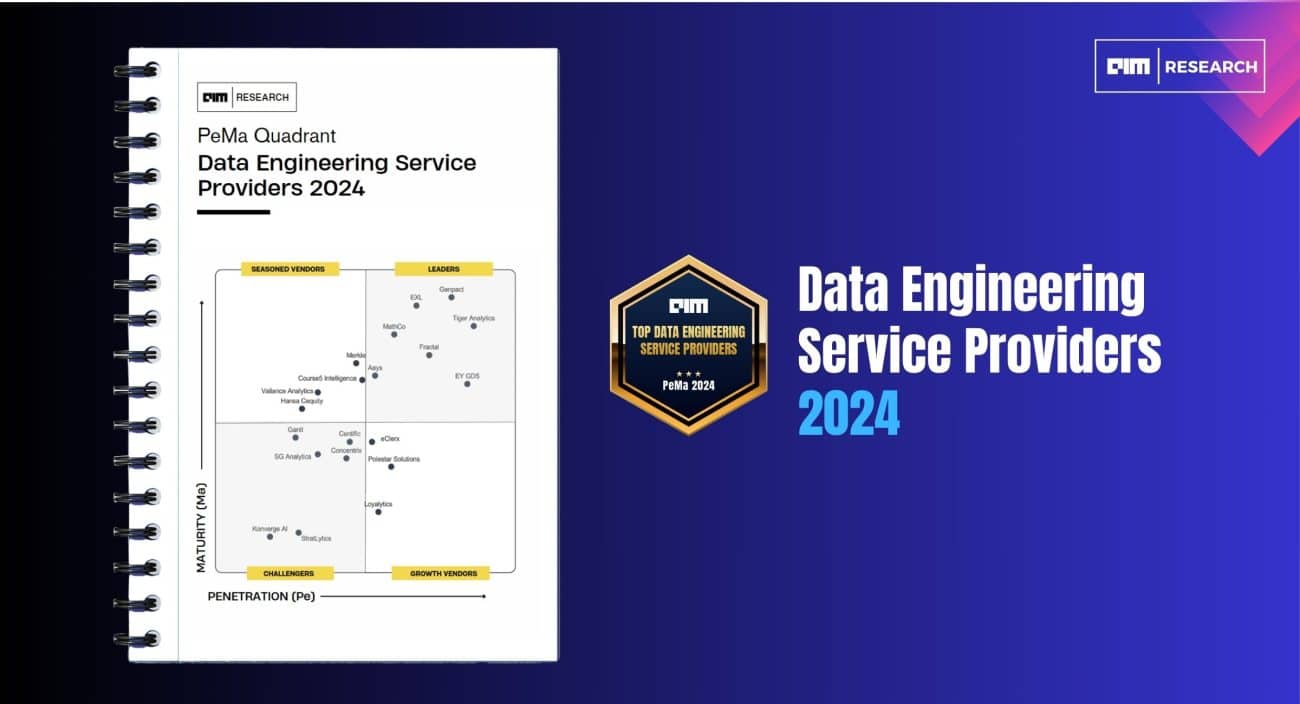
Top Data Engineering Service Providers – PeMa Quadrant 2024

OpenAI Needs Apple, Badly!
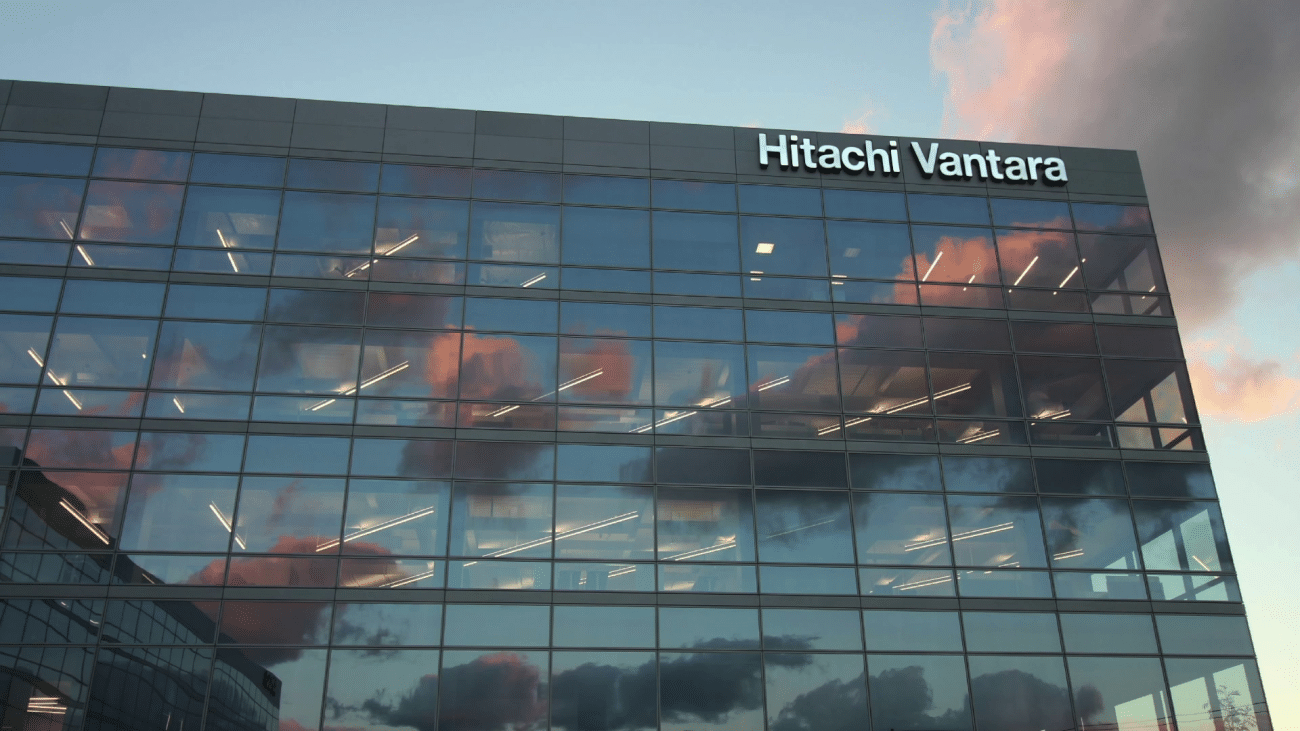
Hitachi Vantara & Veeam Partner to Provide Data Protection for Hybrid Cloud Environments
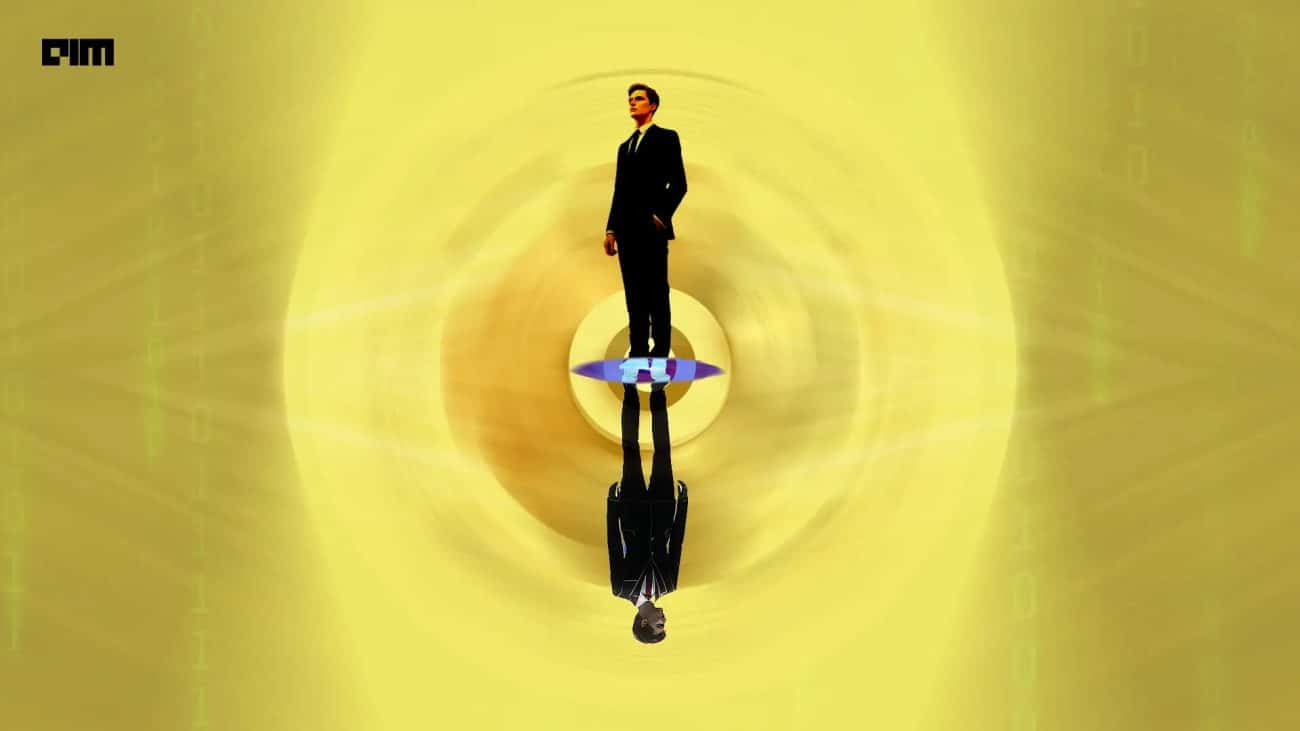
92% of Indian Knowledge Workers Embrace AI at Work: Microsoft & LinkedIn Report

Indian Government to Procure 10,000 GPUs Within Next 18 Months
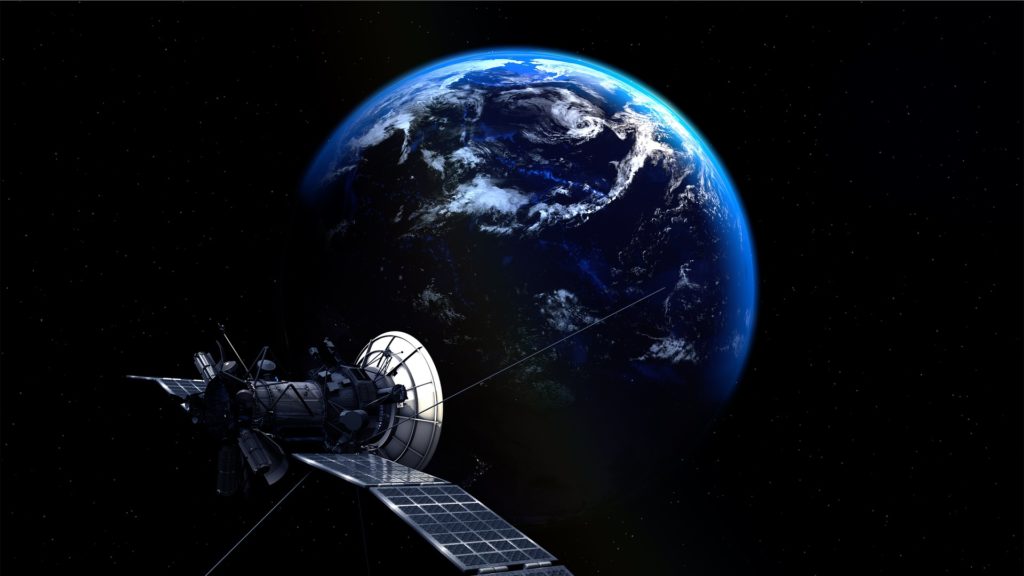
SkyServe’s STORM Ushers in “Smartphone Moment” for Satellite Imaging

Bengaluru is the San Francisco of the East
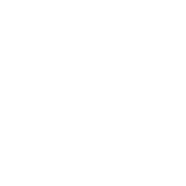
AI Forum for India
Our discord community for ai ecosystem, in collaboration with nvidia. , "> "> flagship events, rising 2024 | de&i in tech summit, april 4 and 5, 2024 | 📍 hilton convention center, manyata tech park, bangalore, machinecon gcc summit 2024, june 28 2024 | 📍bangalore, india, machinecon usa 2024, 26 july 2024 | 583 park avenue, new york, cypher india 2024, september 25-27, 2024 | 📍bangalore, india, cypher usa 2024, nov 21-22 2024 | 📍santa clara convention center, california, usa, genai corner.
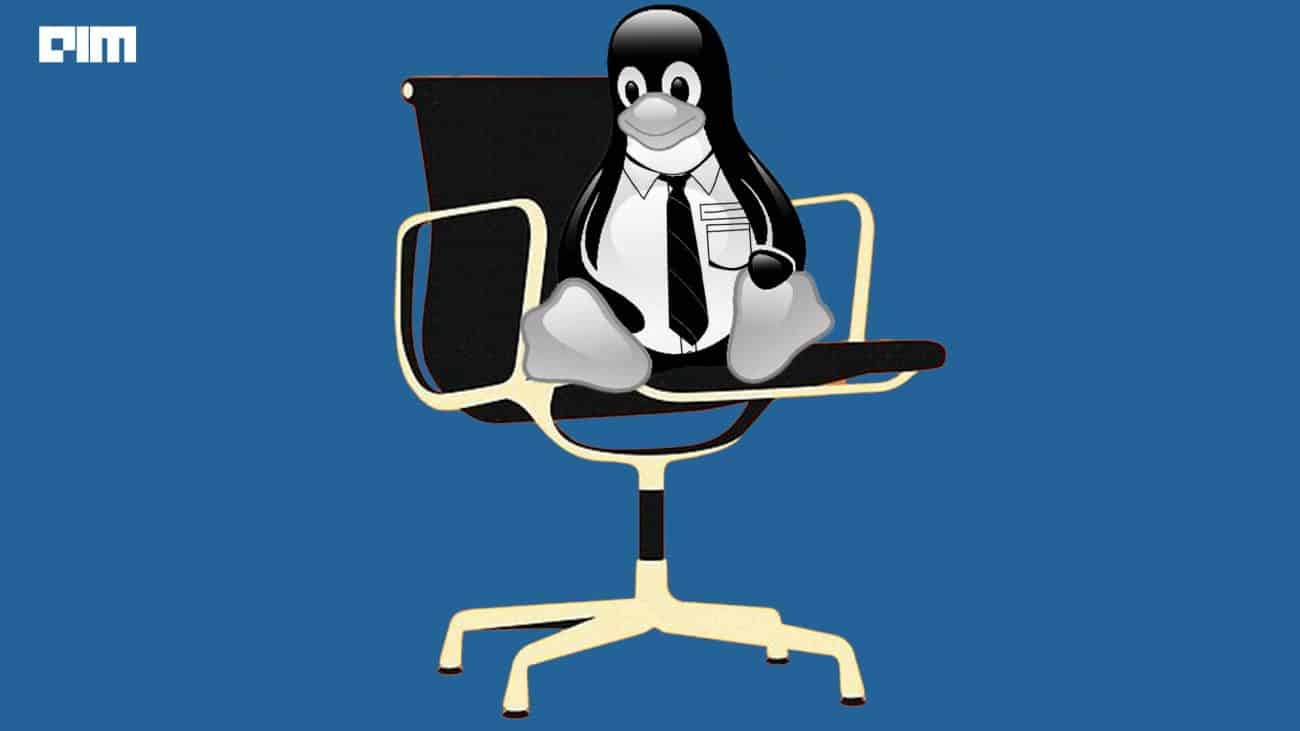
Will Linux Land You a Job?
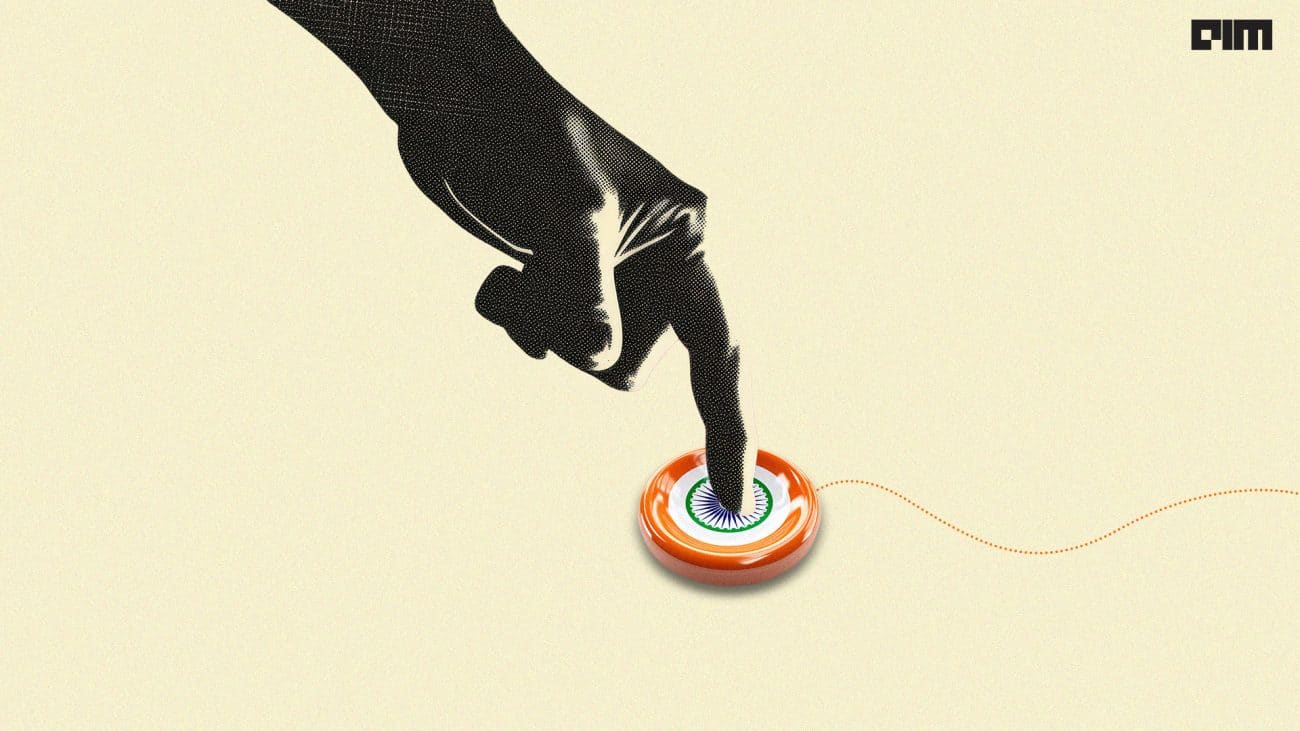
Soket AI Labs Partners with Google Cloud to Boost Pragna-1B Model
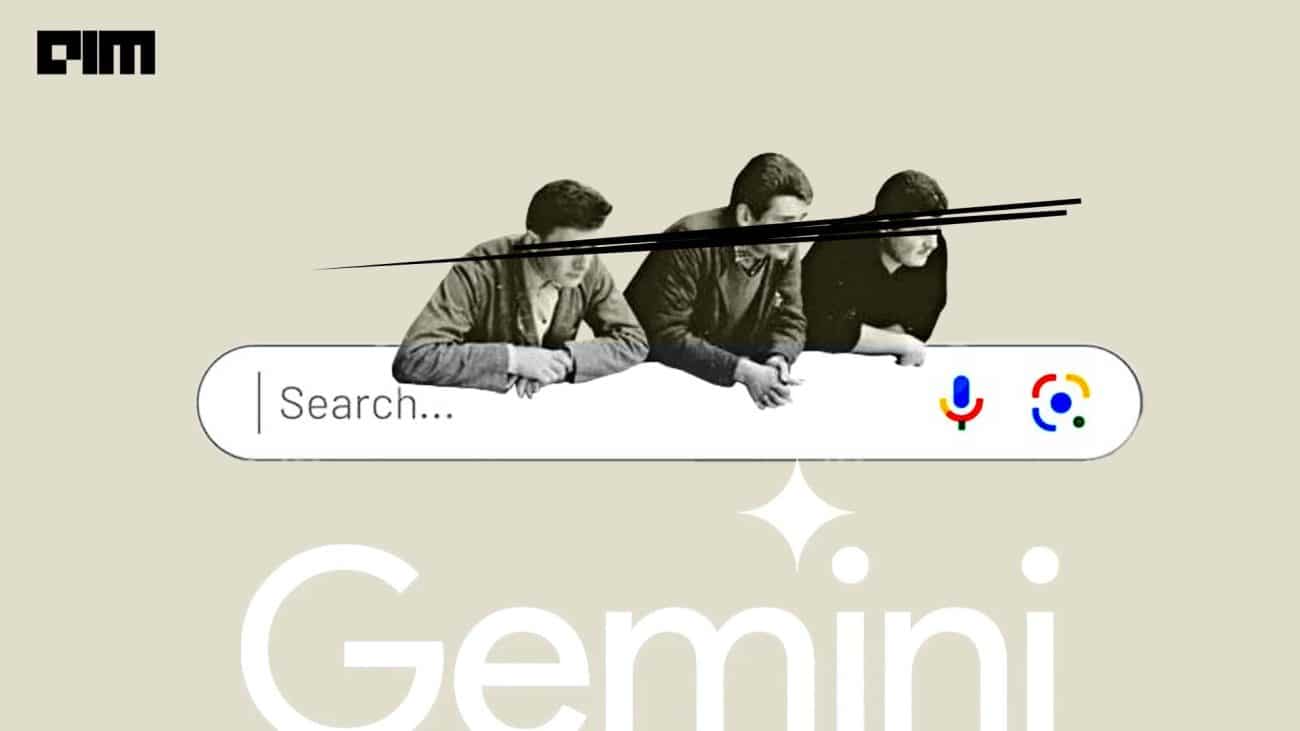
Google Search is Not Going Anywhere Anytime Soon; It’s Here to Stay!

Google’s ‘Astra’ Marks the Beginning of Autonomous AI Agents
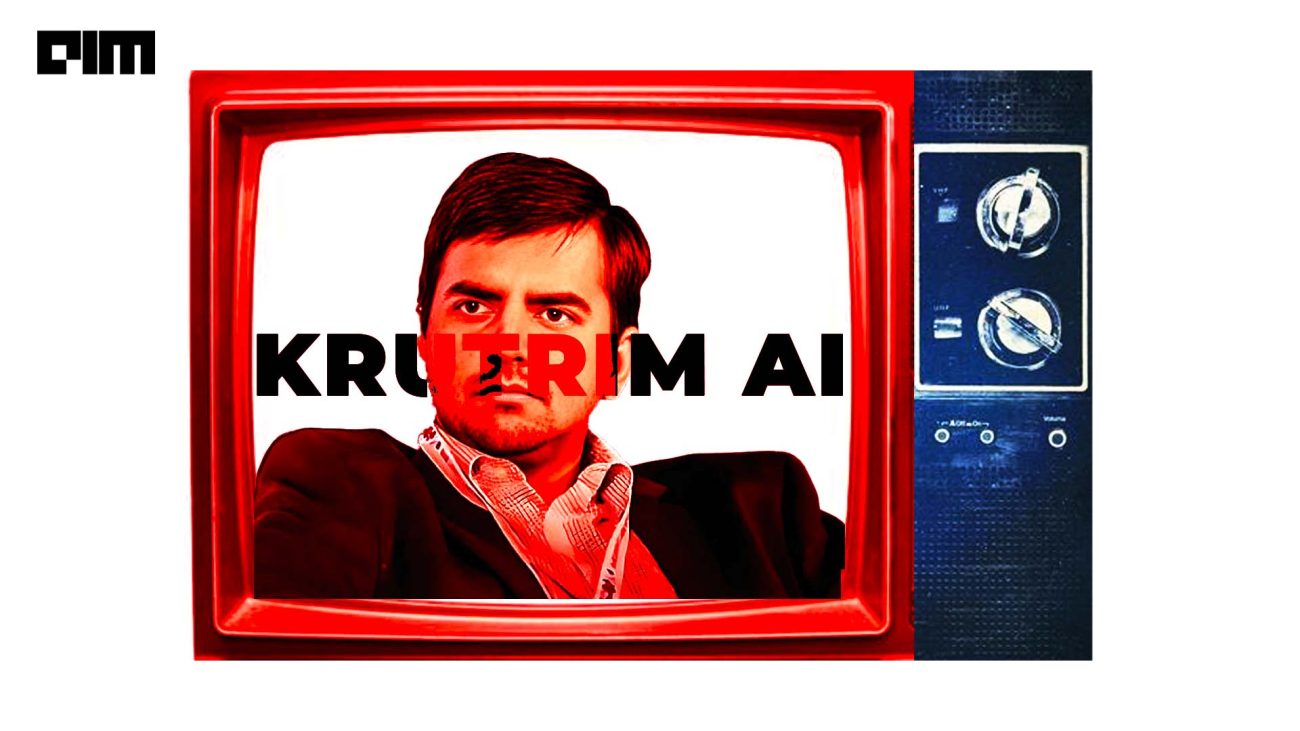
Bodhi Computing Acquisition Signals Ola’s Shift to RISC-V to Make Chips for Krutrim AI Cloud

AWS Launches Amazon Bedrock GenAI Service in APAC (Mumbai) Region
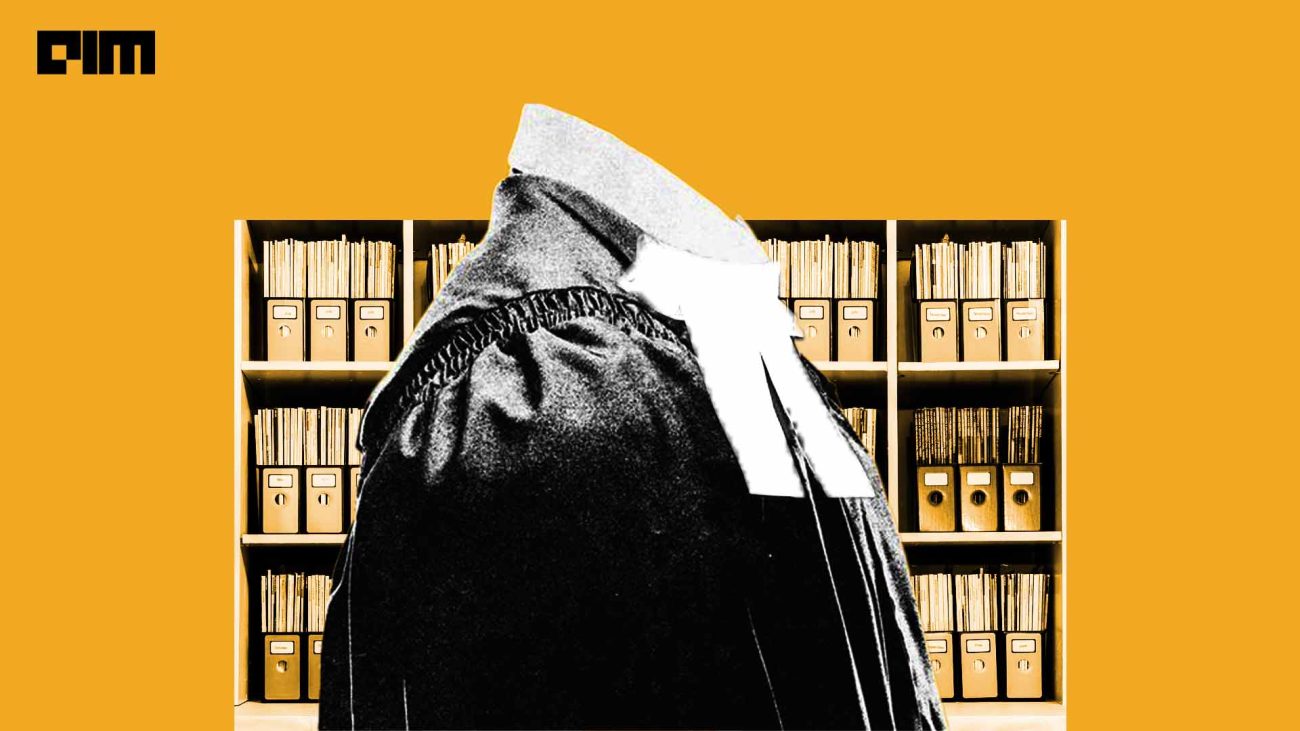
Can GenAI Solve Legal Troubles in India?

65% of Senior Executives Emphasise Human Competitive Advantage in the Age of AI: TCS Report
World's biggest media & analyst firm specializing in ai, advertise with us, aim publishes every day, and we believe in quality over quantity, honesty over spin. we offer a wide variety of branding and targeting options to make it easy for you to propagate your brand., branded content, aim brand solutions, a marketing division within aim, specializes in creating diverse content such as documentaries, public artworks, podcasts, videos, articles, and more to effectively tell compelling stories., corporate upskilling, adasci corporate training program on generative ai provides a unique opportunity to empower, retain and advance your talent, with machinehack you can not only find qualified developers with hiring challenges but can also engage the developer community and your internal workforce by hosting hackathons., talent assessment, conduct customized online assessments on our powerful cloud-based platform, secured with best-in-class proctoring, research & advisory, aim research produces a series of annual reports on ai & data science covering every aspect of the industry. request customised reports & aim surveys for a study on topics of your interest., conferences & events, immerse yourself in ai and business conferences tailored to your role, designed to elevate your performance and empower you to accomplish your organization’s vital objectives., aim launches the 3rd edition of data engineering summit. may 30-31, bengaluru.
Join the forefront of data innovation at the Data Engineering Summit 2024, where industry leaders redefine technology’s future.
© Analytics India Magazine Pvt Ltd & AIM Media House LLC 2024
- Terms of use
- Privacy Policy
Subscribe to Our Newsletter
The Belamy, our weekly Newsletter is a rage. Just enter your email below.
The Data Scientist
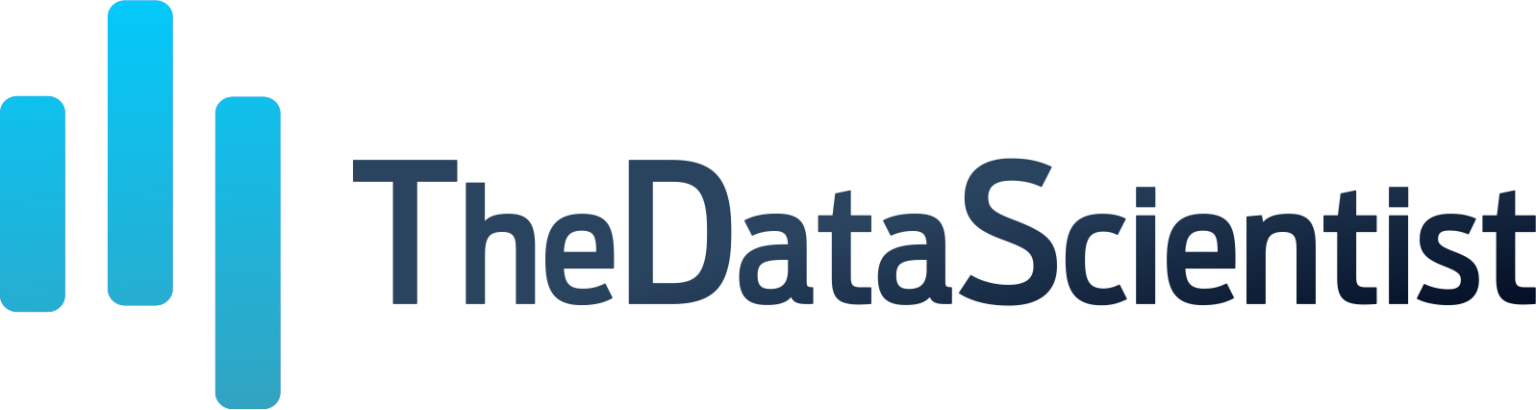
Optimal Structure for Data Science Essays
Writing a compelling data science essay requires a structured approach to convey complex information clearly. Aaron Henderson, a seasoned data science expert at StateOfWriting, offers strategic insights into structuring essays that are not only informative but also engaging. According to Henderson, a well-structured essay can distinguish between a reader grasping the essence of data findings and being overwhelmed by the technical details. Here’s how to structure your data science essays to enhance clarity and impact.
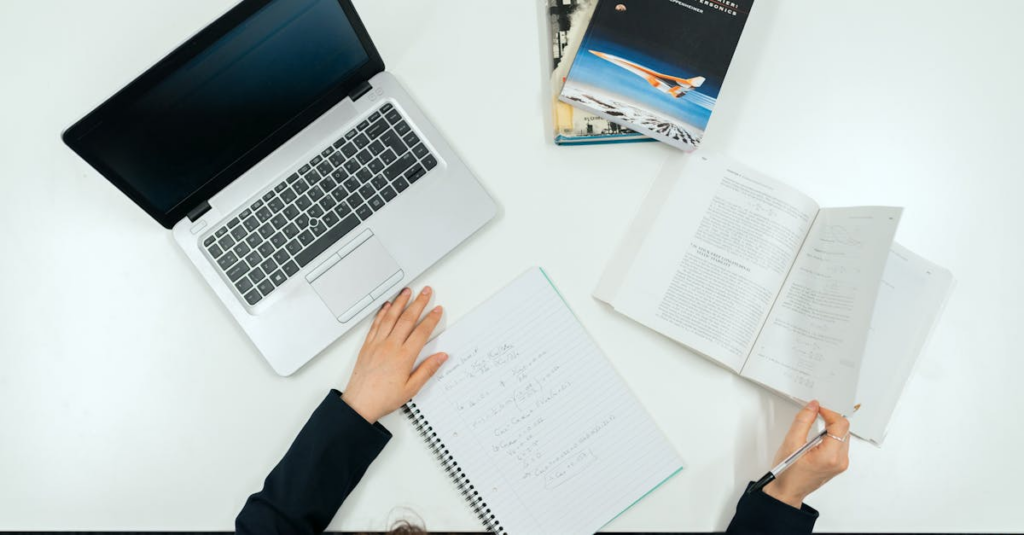
Introduction: Setting the Stage
The introduction of your data science essay should serve as the blueprint for your discussion, providing a clear outline of what the reader can expect. Henderson emphasizes the importance of starting with a hook that grabs the reader’s attention and then moving into a thesis statement that briefly presents the central argument or purpose of the essay.
- Start with a compelling fact or statistic highlighting your topic’s relevance.
- Introduce the central question or problem your essay will address.
- State your thesis clearly – this is your essay’s guiding light.
- Outline the structure of your essay briefly, letting the reader know how you will approach your topic.
This section sets the tone and direction for your essay, so it’s crucial to make it engaging and informative right from the start.
To further enhance the quality and effectiveness of your data science essays, Aaron Henderson advises considering professional writing services. The State Of Writing essay writing service supports organizing your thoughts, refining your arguments, and ensuring that your writing meets academic standards. Whether you’re struggling with the structure, need help with proofreading, or require feedback on your data interpretation, it can offer personalized assistance that complements your knowledge and boosts your essay’s clarity and impact. This additional support can be particularly beneficial for complex subjects like data science , where conveying information clearly and accurately is critical to the reader’s understanding.
Body: Discussing the Core Elements
The body of your essay is where the heavy lifting occurs. This section should be divided into clear, logical paragraphs, each focusing on a specific aspect of your thesis. Aaron Henderson suggests structuring each paragraph around a single main idea supported by data, examples, and analysis. This approach ensures that your essay remains coherent and focused.
- Begin each paragraph with a topic sentence that introduces the paragraph’s main idea.
- Include relevant data and figures to support your points, ensuring they are appropriately cited.
- Provide analysis and interpretation of the data, explaining how it supports your thesis.
- Conclude each paragraph by linking to the central thesis, showing how the information contributes to your argument.
This systematic approach helps maintain a clear narrative flow , making complex data more accessible and understandable to the reader.
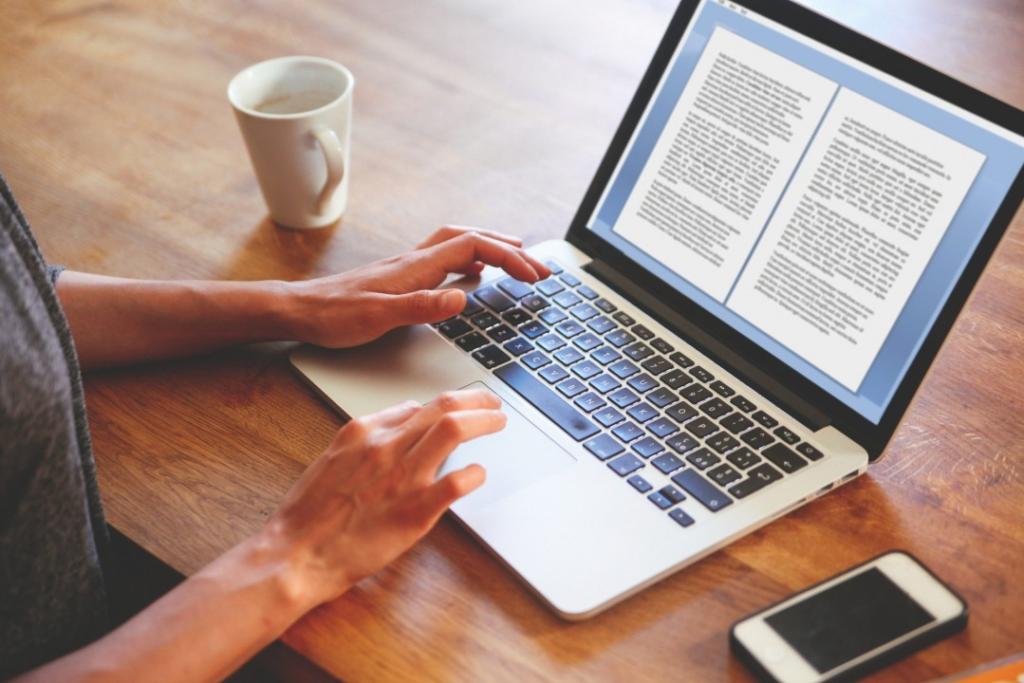
Conclusion: Wrapping It Up
The conclusion of your data science essay is your opportunity to summarize your findings and reinforce your thesis. Aaron Henderson advises against introducing new information in this section; instead, focus on distilling the main points you’ve covered and their implications. It is your chance to leave a lasting impression on your reader, so make it count.
- Restate your thesis in a new way, reflecting the insights gained from your essay.
- Summarize the key points you’ve discussed, emphasizing their relevance and impact.
- Discuss the implications of your findings, especially any future research or applications.
- End with a strong closing statement that highlights the significance of your analysis.
A well-crafted conclusion not only neatly wraps up your essay but also emphasizes the importance of your research and findings.
Additional Tips for Effective Data Science Writing
Beyond structure, Aaron Henderson highlights several vital elements that can enhance the quality and readability of data science essays:
- Clarity and Simplicity: Use simple language to explain complex concepts. Avoid jargon unless necessary, and constantly define terms that may not be familiar to your audience.
- Logical Flow: Ensure that each section of your essay flows logically into the next. Use transition words and phrases to help guide the reader through your arguments.
- Visuals and Diagrams: When appropriate, include charts, graphs, or diagrams to help illustrate your points. Visual aids can make data more digestible and engaging.
- Revision and Feedback: Don’t underestimate the importance of revising your essay and seeking feedback from others. Fresh eyes can catch inconsistencies and areas that need more clarification.
By embracing these additional tips from Aaron Henderson, you can elevate the precision and appeal of your data science essays, ensuring they are insightful and a pleasure to read.
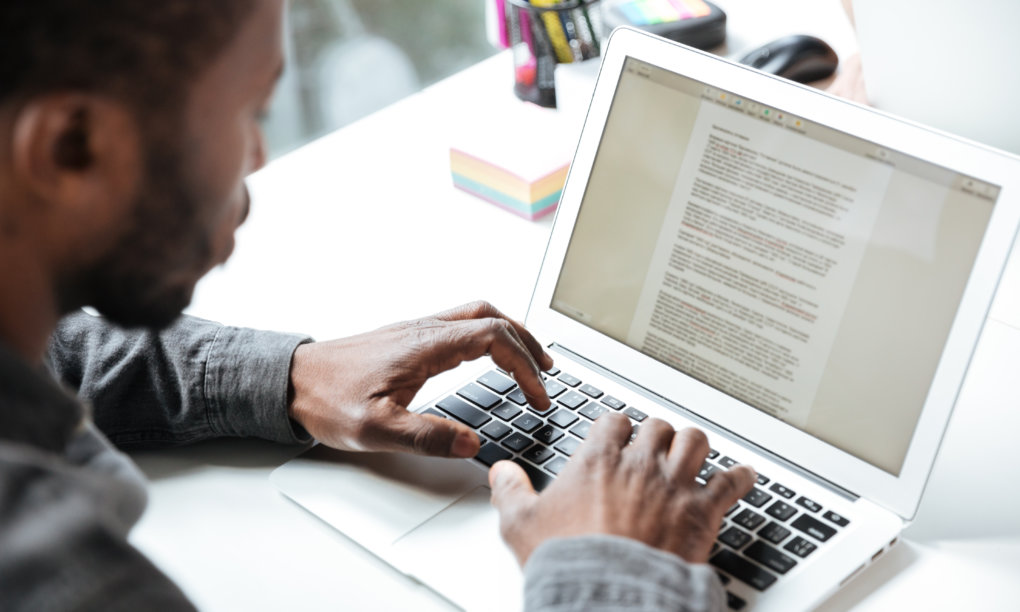
Following these guidelines from Aaron Henderson will help you craft data science essays that are informative, engaging, and accessible. Whether you’re a student aiming for academic excellence or a professional sharing insights in the field, a well-structured essay is the key to effectively communicating complex data-driven topics. By adhering to a clear, logical structure and integrating advanced writing techniques, your essays can stand out in academic or professional settings. The ability to present data and analyses enhances comprehension and demonstrates mastery of the subject matter, paving the way for further discussion and exploration within the data science community.
Guidance from Aaron Henderson at StateOfWriting
- Podcast: Data tactics vs Data Strategy – an interview with Ruben Sardaryan
- How to beat GDPR in research: synthetic data
- Best languages for machine learning and data analytics?
- 5 Tips For Developing Bespoke Software Solutions
- AI Content Shield
- AI KW Research
- AI Assistant
- SEO Optimizer
- AI KW Clustering
- Customer reviews
- The NLO Revolution
- Press Center
- Help Center
- Content Resources
- Facebook Group
Guide to Writing Data Science Personal Statements
Table of Contents
A data science personal statement is an integral part of the application process for aspiring data scientists. It provides recruiters and admissions board representatives insight into a candidate’s motivations, skills, and abilities related to their chosen field of study.
The statement should demonstrate a clear understanding of the concepts and practices that make up the data science discipline. It showcases an applicant’s technical aptitude and professional experience. A successful personal statement will convey passion for the profession through emotionally resonant language and examples.
Personal statements are everyday encounters in job applications as well as applications to special programs and postgraduate studies.
While personal statements and resumes both demonstrate an applicant’s qualifications, the former does so in paragraph form. This is crucial because it allows applicants a reasonable degree of creativity to create vivid depictions and powerful messages.
This allows them to not only create a good impression on readers but also to evoke emotions.
The Importance of a Personal Statement
The primary function of a personal statement is to give insight into the type of person you are. It provides recruiters and admissions board officers a glimpse into your qualifications .
The actual value of a personal statement lies in its exposition. While resumes and summaries give readers the information they need pertaining to your qualifications, personal statements have a more intimate feel.
They read like stories. They take readers on a journey that helps them fully appreciate an applicant’s skills, experience, and character. Personal statements are particularly beneficial because they encourage recruiters and admissions board members to see candidates as more than just their qualifications. They are a way to show evaluators the person behind the application.
But, as good as all these sound, you can reap these benefits only through a compelling personal statement. If you’re unsure of how to write your data science personal statement, heed the following tips.
Tips for Writing a Data Science Personal Statement
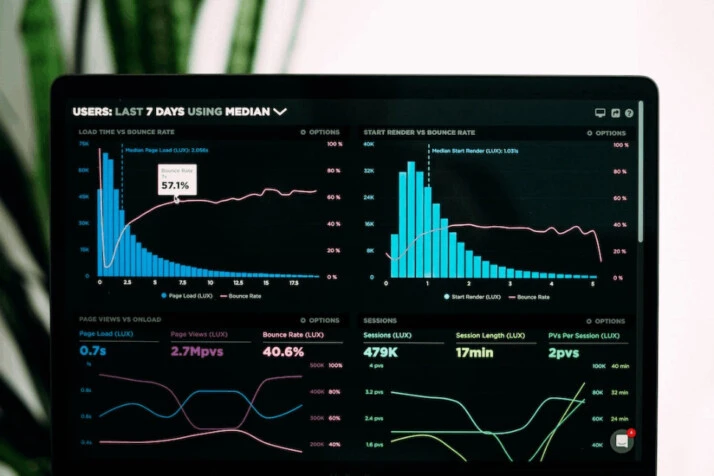
Highlight Your Most Relevant Experience
Demonstrate your skills and accomplishments in data science by including concrete examples. Include experiences such as projects you have worked on or organizations/industries in which you have experience. Doing so will help to demonstrate that you possess the necessary qualifications for a successful career in data science.
Showcase Personal Passion
Showcasing your passion for data science. You can do this by highlighting the personal challenges, successes, and motivations which led to your interest in the field. Explain what inspired you and how this has driven you to pursue further education and, ultimately, a career in data science.
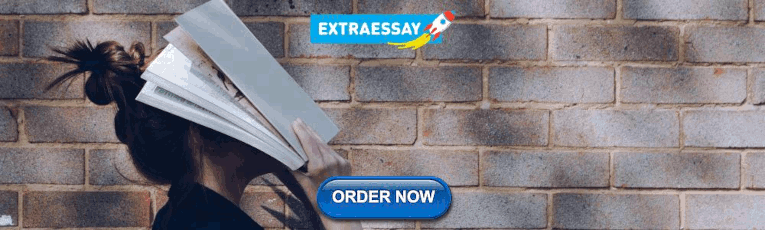
Be Specific
Make sure that when describing both experiences and achievements, they are as specific as possible.
Doing so will allow an admissions panel to better understand the nature of your work and its relevance to data science. Providing evidence to support statements (e.g., screenshots, code snippets, sample analyses) is also beneficial.
Use Clear Language
Being clear and concise is essential when writing about complex topics like data science.
Aim to use language which conveys your points without overcomplicating them with jargon or technical terms. This will make it easier for an admissions panel to understand your application, increasing the chances of being accepted onto their program.
Leverage Emotional Writing
The tone of your statement should reflect a human quality, using emotions and speaking authentically about why data science excites you. If appropriate, include colloquial language throughout; while ensuring it does not detract from the overall clarity of your essay.
Data Science Personal Statement Example
I have been deeply invested in the burgeoning field of data science for almost a decade. My expertise has allowed me to explore its nuances and applications with avid enthusiasm. I utilized my specialized knowledge to contribute significantly to many successful projects. As a result, I have accrued an immense portfolio of experience. My experience ranges from predictive analytics to natural language processing. This sets me apart as a leader in a rapidly-evolving industry.
From analyzing complex datasets to constructing scalable machine learning systems, my tenaciousness drives me to continually seek out dynamic challenges. Although I am thoroughly versed in all theoretical aspects of data science, I thrive on uncovering new possibilities through experimentation and creative problem-solving. I pride myself on being able to translate technical jargon into actionable solutions.
A personal statement is a short paragraph that outlines a candidate’s skills, experiences, and motivation . It is an essential document because it allows applicants to connect with readers and establish a good impression. Remember our simple tips. While they won’t make you an expert overnight, they will help you cement good writing habits that will serve you well in the future.
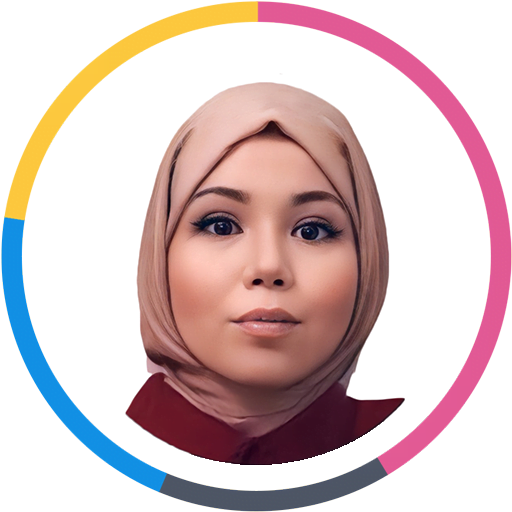
Abir Ghenaiet
Abir is a data analyst and researcher. Among her interests are artificial intelligence, machine learning, and natural language processing. As a humanitarian and educator, she actively supports women in tech and promotes diversity.
Explore All Write Personal Statement Articles
How to draft meaningful length of law school personal statement.
Are you confused on how to write a law school personal statement? One of the essential elements of your application…
- Write Personal Statement
Effective History and International Relations Personal Statement to Try
Are you considering studying history and international relations? Or you may be curious about what a degree in this field…
Guide to Quality Global Management Personal Statement
Are you applying for a global management program and want to stand out from the crowd? A well-written personal statement…
How to Draft Better Examples of Personal Statements for Residency
Achieving a residency can be a massive accomplishment for any aspiring medical professional. To secure your spot in one of…
Tips for Drafting a Free Example of Personal History Statement
A personal history statement can be crucial to many applications, from university admissions to job search processes. This blog will…
Writing Compelling Dietetic Internship Personal Statement
Applying for a dietetic internship is a rigorous process and requires submitting a personal statement, which is an essential part…
October 29, 2018
Application Advice Part 4: The Essays
Most graduate school applicants will agree – writing application essays is the most difficult and stressful part. While writing your essays may seem daunting, this is your chance to differentiate yourself from other applicants with comparable qualifications. It is likely that dozens of applicants, if not more, have academic records and professional backgrounds that are similar to yours, but strong essays may put you ahead of the competition.
Before you write:
- Read the questions. Before you begin writing, take the time to carefully read the questions in each essay prompt. Make you sure you understand everything that is being asked of you.
- Reflect on the purpose. Ask yourself: What purpose does this essay serve? For example, Essay 1: Why UW MSDS? should give the admissions committee insight into your short-term and long-term goals, why you want to attend our program specifically, and what kind of career you envision after graduation.
- Create an outline. Drafting an outline will help you organize your thoughts and identify the building blocks of your essay. An outline will also help you better understand what you are trying to communicate to the admissions committee.
Writing your essays:
- Write a compelling introduction . Do not start with “My name is…” or “I am applying because…”. Your name or the fact that you are applying are not the most interesting things about you! You should choose to begin your essay, for example, with an anecdote, a question, or an attention-grabbing statement, but make sure it is directly relevant to your essay.
- Don’t repeat your resume. If you do talk about your work experience, do so briefly and only to make a larger point.
- Show, don’t tell. Offer examples, stories, or descriptions when writing your essays. For example, rather than just stating that you want to work as a data scientist in the healthcare sector after graduation, describe what you find the most compelling about the industry or job role.
Revising your essays:
- Edit, edit, edit! Give yourself enough time to write multiple drafts of your essays. One of the most common mistakes applicants make is to leave too little time for writing their essays.
- Proofread. Proofread your essays carefully and try reading your essays out loud to help catch any awkward phrasing. Ask your classmates, instructors, or colleagues to proofread your essays. Make sure your work is free of spelling mistakes and grammatical errors.
- Stick to the word limit. It might be tempting to ignore the word limit, but we want to see that you can get your point across clearly and concisely.
Last, but not least, make sure you do not duplicate the content of your essays. Each essay has its own purpose. There is no reason to repeat the same ideas in your writings.
Admissions Timelines
Applications for Autumn 2024 admissions are now closed.
Information about Autumn 2025 applications will be available in October.
Admissions Updates
Be boundless, connect with us:.
© 2024 University of Washington | Seattle, WA
What are your chances of acceptance?
Calculate for all schools, your chance of acceptance.
Your chancing factors
Extracurriculars.
How to Write the “Why Computer Science?” Essay
What’s covered:, what is the purpose of the “why computer science” essay, elements of a good computer science essay, computer science essay example, where to get your essay edited.
You will encounter many essay prompts as you start applying to schools, but if you are intent on majoring in computer science or a related field, you will come across the “ Why Computer Science? ” essay archetype. It’s important that you know the importance behind this prompt and what constitutes a good response in order to make your essay stand out.
For more information on writing essays, check out CollegeVine’s extensive essay guides that include everything from general tips, to essay examples, to essay breakdowns that will help you write the essays for over 100 schools.
Colleges ask you to write a “ Why Computer Science? ” essay so you may communicate your passion for computer science, and demonstrate how it aligns with your personal and professional goals. Admissions committees want to see that you have a deep interest and commitment to the field, and that you have a vision for how a degree in computer science will propel your future aspirations.
The essay provides an opportunity to distinguish yourself from other applicants. It’s your chance to showcase your understanding of the discipline, your experiences that sparked or deepened your interest in the field, and your ambitions for future study and career. You can detail how a computer science degree will equip you with the skills and knowledge you need to make a meaningful contribution in this rapidly evolving field.
A well-crafted “ Why Computer Science? ” essay not only convinces the admissions committee of your enthusiasm and commitment to computer science, but also provides a glimpse of your ability to think critically, solve problems, and communicate effectively—essential skills for a computer scientist.
The essay also gives you an opportunity to demonstrate your understanding of the specific computer science program at the college or university you are applying to. You can discuss how the program’s resources, faculty, curriculum, and culture align with your academic interests and career goals. A strong “ Why Computer Science? ” essay shows that you have done your research, and that you are applying to the program not just because you want to study computer science, but because you believe that this particular program is the best fit for you.
Writing an effective “ Why Computer Science ?” essay often requires a blend of two popular college essay archetypes: “ Why This Major? ” and “ Why This College? “.
Explain “Why This Major?”
The “ Why This Major? ” essay is an opportunity for you to dig deep into your motivations and passions for studying Computer Science. It’s about sharing your ‘origin story’ of how your interest in Computer Science took root and blossomed. This part of your essay could recount an early experience with coding, a compelling Computer Science class you took, or a personal project that sparked your fascination.
What was the journey that led you to this major? Was it a particular incident, or did your interest evolve over time? Did you participate in related activities, like coding clubs, online courses, hackathons, or internships?
Importantly, this essay should also shed light on your future aspirations. How does your interest in Computer Science connect to your career goals? What kind of problems do you hope to solve with your degree?
The key for a strong “ Why This Major? ” essay is to make the reader understand your connection to the subject. This is done through explaining your fascination and love for computer science. What emotions do you feel when you are coding? How does it make you feel when you figure out the solution after hours of trying? What aspects of your personality shine when you are coding?
By addressing these questions, you can effectively demonstrate a deep, personal, and genuine connection with the major.
Emphasize “Why This College?”
The “ Why This College? ” component of the essay demonstrates your understanding of the specific university and its Computer Science program. This is where you show that you’ve done your homework about the college, and you know what resources it has to support your academic journey.
What unique opportunities does the university offer for Computer Science students? Are there particular courses, professors, research opportunities, or clubs that align with your interests? Perhaps there’s a study abroad program or an industry partnership that could give you a unique learning experience. Maybe the university has a particular teaching methodology that resonates with you.
Also, think about the larger university community. What aspects of the campus culture, community, location, or extracurricular opportunities enhance your interest in this college? Remember, this is not about general praises but about specific features that align with your goals. How will these resources and opportunities help you explore your interests further and achieve your career goals? How does the university’s vision and mission resonate with your own values and career aspirations?
It’s important when discussing the school’s resources that you always draw a connection between the opportunity and yourself. For example, don’t tell us you want to work with X professor because of their work pioneering regenerative AI. Go a step further and say because of your goal to develop AI surgeons for remote communities, learning how to strengthen AI feedback loops from X professor would bring you one step closer to achieving your dream.
By articulating your thoughts on these aspects, you demonstrate a strong alignment between the college and your academic goals, enhancing your appeal as a prospective student.
Demonstrate a Deep Understanding of Computer Science
As with a traditional “ Why This Major? ” essay, you must exhibit a deep and clear understanding of computer science. Discuss specific areas within the field that pique your interest and why. This could range from artificial intelligence to software development, or from data science to cybersecurity.
What’s important is to not just boast and say “ I have a strong grasp on cybersecurity ”, but instead use your knowledge to show your readers your passion: “ After being bombarded with cyber attack after cyber attack, I explained to my grandparents the concept of end-to-end encryption and how phishing was not the same as a peaceful afternoon on a lake. ”
Make it Fun!
Students make the mistake of thinking their college essays have to be serious and hyper-professional. While you don’t want to be throwing around slang and want to present yourself in a positive light, you shouldn’t feel like you’re not allowed to have fun with your essay. Let your personality shine and crack a few jokes.
You can, and should, also get creative with your essay. A great way to do this in a computer science essay is to incorporate lines of code or write the essay like you are writing out code.
Now we will go over a real “ Why Computer Science? ” essay a student submitted and explore what the essay did well, and where there is room for improvement.
Please note: Looking at examples of real essays students have submitted to colleges can be very beneficial to get inspiration for your essays. You should never copy or plagiarize from these examples when writing your own essays. Colleges can tell when an essay isn’t genuine and will not view students favorably if they plagiarized.
I held my breath and hit RUN. Yes! A plump white cat jumped out and began to catch the falling pizzas. Although my Fat Cat project seems simple now, it was the beginning of an enthusiastic passion for computer science. Four years and thousands of hours of programming later, that passion has grown into an intense desire to explore how computer science can serve society. Every day, surrounded by technology that can recognize my face and recommend scarily-specific ads, I’m reminded of Uncle Ben’s advice to a young Spiderman: “with great power comes great responsibility”. Likewise, the need to ensure digital equality has skyrocketed with AI’s far-reaching presence in society; and I believe that digital fairness starts with equality in education.
The unique use of threads at the College of Computing perfectly matches my interests in AI and its potential use in education; the path of combined threads on Intelligence and People gives me the rare opportunity to delve deep into both areas. I’m particularly intrigued by the rich sets of both knowledge-based and data-driven intelligence courses, as I believe AI should not only show correlation of events, but also provide insight for why they occur.
In my four years as an enthusiastic online English tutor, I’ve worked hard to help students overcome both financial and technological obstacles in hopes of bringing quality education to people from diverse backgrounds. For this reason, I’m extremely excited by the many courses in the People thread that focus on education and human-centered technology. I’d love to explore how to integrate AI technology into the teaching process to make education more available, affordable, and effective for people everywhere. And with the innumerable opportunities that Georgia Tech has to offer, I know that I will be able to go further here than anywhere else.
What the Essay Did Well
This essay perfectly accomplishes the two key parts of a “ Why Computer Science? ” essay: answering “ Why This Major? ” and “ Why This College? ”. Not to mention, we get a lot of insight into this student and what they care about beyond computer science, and a fun hook at the beginning.
Starting with the “ Why This Major? ” aspect of the response, this essay demonstrates what got the student into computer science, why they are passionate about the subject, and what their goals are. They show us their introduction to the world of CS with an engaging hook: “I held my breath and hit RUN. Yes! A plump white cat jumped out and began to catch the falling pizzas. ” We then see this is a core passion because they spent “ Four years and thousands of hours ,” coding.
The student shows us why they care about AI with the sentence, “ Every day, surrounded by technology that can recognize my face and recommend scarily-specific ads ,” which makes the topic personal by demonstrating their fear at AI’s capabilities. But, rather than let panic overwhelm them, the student calls upon Spiderman and tells us their goal of establishing digital equality through education. This provides a great basis for the rest of the essay, as it thoroughly explains the students motivations and goals, and demonstrates their appreciation for interdisciplinary topics.
Then, the essay shifts into answering “ Why This College? ”, which it does very well by honing in on a unique facet of Georgia Tech’s College of Computing: threads. This is a great example of how to provide depth to the school resources you mention. The student describes the two threads and not only why the combination is important to them, but how their previous experiences (i.e. online English tutor) correlate to the values of the thread: “ For this reason, I’m extremely excited by the many courses in the People thread that focus on education and human-centered technology. ”
What Could Be Improved
This essay does a good job covering the basics of the prompt, but it could be elevated with more nuance and detail. The biggest thing missing from this essay is a strong core to tie everything together. What do we mean by that? We want to see a common theme, anecdote, or motivation that is weaved throughout the entire essay to connect everything. Take the Spiderman quote for example. If this was expanded, it could have been the perfect core for this essay.
Underlying this student’s interest in AI is a passion for social justice, so they could have used the quote about power and responsibility to talk about existing injustices with AI and how once they have the power to create AI they will act responsibly and help affected communities. They are clearly passionate about equality of education, but there is a disconnect between education and AI that comes from a lack of detail. To strengthen the core of the essay, this student needs to include real-world examples of how AI is fostering inequities in education. This takes their essay from theoretical to practical.
Whether you’re a seasoned writer or a novice trying your hand at college application essays, the review and editing process is crucial. A fresh set of eyes can provide valuable insights into the clarity, coherence, and impact of your writing. Our free Peer Essay Review tool offers a unique platform to get your essay reviewed by another student. Peer reviews can often uncover gaps, provide new insights or enhance the clarity of your essay, making your arguments more compelling. The best part? You can return the favor by reviewing other students’ essays, which is a great way to hone your own writing and critical thinking skills.
For a more professional touch, consider getting your essay reviewed by a college admissions expert . CollegeVine advisors have years of experience helping students refine their writing and successfully apply to top-tier schools. They can provide specific advice on how to showcase your strengths, address any weaknesses, and generally present yourself in the best possible light.
Related CollegeVine Blog Posts
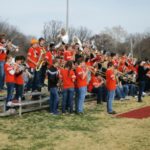

Data Science for Undergraduates: Opportunities and Options (2018)
Chapter: 6 conclusions, 6 conclusions.
Data science education is well into its formative stages of development; it is evolving into a self-supporting discipline and producing professionals with distinct and complementary skills relative to professionals in the computer, information, and statistical sciences. However, regardless of its potential eventual disciplinary status, the evidence points to robust growth of data science education that will indelibly shape the undergraduate students of the future. In fact, fueled by growing student interest and industry demand, data science education will likely become a staple of the undergraduate experience. There will be an increase in the number of students majoring, minoring, earning certificates, or just taking courses in data science as the value of data skills becomes even more widely recognized. The adoption of a general education requirement in data science for all undergraduates will endow future generations of students with the basic understanding of data science that they need to become responsible citizens. Continuing education programs such as data science boot camps, career accelerators, summer schools, and incubators will provide another stream of talent. This constitutes the emerging watershed of data science education that feeds multiple streams of generalists and specialists in society; citizens are empowered by their basic skills to examine, interpret, and draw value from data.
Today, the nation is in the formative phase of data science education, where educational organizations are pioneering their own programs, each with different approaches to depth, breadth, and curricular emphasis (e.g., business, computer science, engineering, information science, math-
ematics, social science, or statistics). It is too early to expect consensus to emerge on certain best practices of data science education. However, it is not too early to envision the possible forms that such practices might take. Nor is it too early to make recommendations that can help the data science education community develop strategic vision and practices. The following is a summary of the findings and recommendations discussed in the preceding four chapters of this report.
Finding 2.1: Data scientists today draw largely from extensions of the “analyst” of years past trained in traditional disciplines. As data science becomes an integral part of many industries and enriches research and development, there will be an increased demand for more holistic and more nuanced data science roles.
Finding 2.2: Data science programs that strive to meet the needs of their students will likely evolve to emphasize certain skills and capabilities. This will result in programs that prepare different types of data scientists.
Recommendation 2.1: Academic institutions should embrace data science as a vital new field that requires specifically tailored instruction delivered through majors and minors in data science as well as the development of a cadre of faculty equipped to teach in this new field.
Recommendation 2.2: Academic institutions should provide and evolve a range of educational pathways to prepare students for an array of data science roles in the workplace.
Finding 2.3: A critical task in the education of future data scientists is to instill data acumen. This requires exposure to key concepts in data science, real-world data and problems that can reinforce the limitations of tools, and ethical considerations that permeate many applications. Key concepts involved in developing data acumen include the following:
- Mathematical foundations,
- Computational foundations,
- Statistical foundations,
- Data management and curation,
- Data description and visualization,
- Data modeling and assessment,
- Workflow and reproducibility,
- Communication and teamwork,
- Domain-specific considerations, and
- Ethical problem solving.
Recommendation 2.3: To prepare their graduates for this new data-driven era, academic institutions should encourage the development of a basic understanding of data science in all undergraduates.
Recommendation 2.4: Ethics is a topic that, given the nature of data science, students should learn and practice throughout their education. Academic institutions should ensure that ethics is woven into the data science curriculum from the beginning and throughout.
Recommendation 2.5: The data science community should adopt a code of ethics; such a code should be affirmed by members of professional societies, included in professional development programs and curricula, and conveyed through educational programs. The code should be reevaluated often in light of new developments.
Finding 3.1: Undergraduate education in data science can be experienced in many forms. These include the following:
- Integrated introductory courses that can satisfy a general education requirement;
- A major in data science, including advanced skills, as the primary field of study;
- A minor or track in data science, where intermediate skills are connected to the major field of study;
- Two-year degrees and certificates;
- Other certificates, often requiring fewer courses than a major but more than a minor;
- Massive open online courses, which can engage large numbers of students at a variety of levels; and
- Summer programs and boot camps, which can serve to supplement academic or on-the-job training.
Recommendation 3.1: Four-year and two-year institutions should establish a forum for dialogue across institutions on all aspects of data science education, training, and workforce development.
Finding 4.1: The nature of data science is such that it offers multiple pathways for students of different backgrounds to engage at levels ranging from basic to expert.
Finding 4.2: Data science would particularly benefit from broad participation by underrepresented minorities because of the many applications to problems of interest to diverse populations.
Recommendation 4.1: As data science programs develop, they should focus on attracting students with varied backgrounds and degrees of preparation and preparing them for success in a variety of careers.
Finding 4.3: Institutional flexibility will involve the development of curricula that take advantage of current course availability and will potentially be constrained by the availability of teaching expertise. Whatever organizational or infrastructure model is adopted, incentives are needed to encourage faculty participation and to overcome barriers.
Finding 4.4: The economics of developing programs has recently changed with the shift to cloud-based approaches and platforms.
Finding 5.1: The evolution of data science programs at a particular institution will depend on the particular institution’s pedagogical style and the students’ backgrounds and goals, as well as the requirements of the job market and graduate schools.
Recommendation 5.1: Because these are early days for undergraduate data science education, academic institutions should be prepared to evolve programs over time. They should create and maintain the flexibility and incentives to facilitate the sharing of courses, materials, and faculty among departments and programs.
Finding 5.2: There is a need for broadening the perspective of faculty who are trained in particular areas of data science to be knowledgeable of the breadth of approaches to data science so that they can more effectively educate students at all levels.
Recommendation 5.2: During the development of data science programs, institutions should provide support so that the faculty can become more cognizant of the varied aspects of data science through discussion, co-teaching, sharing of materials, short courses, and other forms of training.
Finding 5.3: The data science community would benefit from the creation of websites and journals that document and make available best
practices, curricula, education research findings, and other materials related to undergraduate data science education.
Finding 5.4: The evolution of undergraduate education in data science can be driven by data science. Exploiting administrative records, in conjunction with other data sources such as economic information and survey data, can enable effective transformation of programs to better serve their students.
Finding 5.5: Data science methods applied both to individual programs and comparatively across programs can be used for both evaluation and evolution of data science program components. It is essential that both processes are sustained as new pathways emerge at institutions.
Recommendation 5.3: Academic institutions should ensure that programs are continuously evaluated and should work together to develop professional approaches to evaluation. This should include developing and sharing measurement and evaluation frameworks, data sets, and a culture of evolution guided by high-quality evaluation. Efforts should be made to establish relationships with sector-specific professional societies to help align education evaluation with market impacts.
Finding 5.6: As professional societies adapt to data science, improved coordination could offer new opportunities for additional collaboration and cross-pollination. A group or conference with bridging capabilities would be helpful. Professional societies may find it useful to collaborate to offer such training and networking opportunities to their joint communities.
Recommendation 5.4: Existing professional societies should coordinate to enable regular convening sessions on data science among their members. Peer review and discussion are essential to share ideas, best practices, and data.
This page intentionally left blank.
Data science is emerging as a field that is revolutionizing science and industries alike. Work across nearly all domains is becoming more data driven, affecting both the jobs that are available and the skills that are required. As more data and ways of analyzing them become available, more aspects of the economy, society, and daily life will become dependent on data. It is imperative that educators, administrators, and students begin today to consider how to best prepare for and keep pace with this data-driven era of tomorrow. Undergraduate teaching, in particular, offers a critical link in offering more data science exposure to students and expanding the supply of data science talent.
Data Science for Undergraduates: Opportunities and Options offers a vision for the emerging discipline of data science at the undergraduate level. This report outlines some considerations and approaches for academic institutions and others in the broader data science communities to help guide the ongoing transformation of this field.
READ FREE ONLINE
Welcome to OpenBook!
You're looking at OpenBook, NAP.edu's online reading room since 1999. Based on feedback from you, our users, we've made some improvements that make it easier than ever to read thousands of publications on our website.
Do you want to take a quick tour of the OpenBook's features?
Show this book's table of contents , where you can jump to any chapter by name.
...or use these buttons to go back to the previous chapter or skip to the next one.
Jump up to the previous page or down to the next one. Also, you can type in a page number and press Enter to go directly to that page in the book.
Switch between the Original Pages , where you can read the report as it appeared in print, and Text Pages for the web version, where you can highlight and search the text.
To search the entire text of this book, type in your search term here and press Enter .
Share a link to this book page on your preferred social network or via email.
View our suggested citation for this chapter.
Ready to take your reading offline? Click here to buy this book in print or download it as a free PDF, if available.
Get Email Updates
Do you enjoy reading reports from the Academies online for free ? Sign up for email notifications and we'll let you know about new publications in your areas of interest when they're released.
For enquiries call:
+1-469-442-0620

- Data Science
Data Science Personal Statement Sample and Examples
Home Blog Data Science Data Science Personal Statement Sample and Examples

Data science is one of the most popular career options for students, especially those pursuing a Bachelor's degree. It is also one of the most sought-after courses in universities today. If you just want some ideas on how to write a personal statement for data science, then this article is for you. Also, if you’re someone who is willing to secure a career in the field of data science, then it is recommended that you pursue Data Science Courses that will enable you to learn all aspe that will enable you to learn all aspects and principles of data science.
What is a Data Science Personal Statement?
In a nutshell, the personal statement for data science is a document that you write to explain why you are interested in pursuing the subject and what you can bring to the table. It should be written in a way that shows your interest in the subject and why you want to study it. You may want to include information about the following in your data science personal statement.
- What led up to your decision to pursue this field?
- Why do data scientists matter? What problems need solving by them? What value do they provide society as well as individuals?
- How will studying this specific field help prepare you for future careers or additional educational opportunities (e-learning programs, etc.)?
You can also include the following:
- What are your goals for this degree?
- How will it benefit you?
- What do you hope to achieve from studying data science?
- Why is this field important in today’s society?
- What are the challenges that you see in this field?
- How will you address those challenges?
- What do you think the future of data science is?
- How do you plan on staying relevant as technologies and trends change?
The Importance of Creating a Data Science Personal Statement
Data science personal statement is a formal document that will be used by the company to evaluate your skills. If you are applying for a Data Science job and want to impress the hiring manager, then you must write a strong data science personal statement.
A good personal statement for a master's in data science must be unique, creative, informative and interesting to read. It should describe not only your skills and experience but also showcase your ability to think critically and creatively.
A well-written data science personal statement will help you stand out from other applicants and make yourself an ideal candidate for the job that you want. Here are some useful tips for writing a strong data science personal statement:
- Be honest and straightforward in your personal statement.
- Don’t exaggerate or lie about your skills, experience and achievements. If you don’t have any relevant work experience, then focus on other areas where you can showcase your skills, such as volunteering or community projects.
- Know the company that you are applying to and tailor your personal statement accordingly. A generic resume won’t help if you are applying for a specific job position. Instead, write a customized letter that shows how well-suited you are for this role.
- Keep it short and sweet. The best personal statements are between a few hundred to a few thousand words long. Don’t try to cram everything in one big paragraph; break it up into smaller sections that will make it easier for readers to digest.
So now you might have understood how important data analytics personal statements are. To learn how to create a personal statement, it is recommended that you enroll in the Best Data Science Bootcamps .
Data Science Personal Statement Sample
I am writing this Data Science Personal Statement for the MS in Data Science program at UC Berkeley. My goal is to explain why I want to pursue a career in data science and how my experience as an undergraduate student has prepared me for graduate school. As you can see from my resume, I have had many opportunities to work with large amounts of data through internships and research projects over the course of my academic career. These experiences have given me valuable insight into how large-scale computational problems can be tackled by applying statistical methods under tight deadlines while still maintaining quality control over your results.
In addition, I have taken classes such as AI/ML Systems Design & Implementation and Machine Learning Algorithms. These classes have helped me develop new ways of approaching problems while also providing an understanding of why certain algorithms work better than others when applied in specific situations.
I am a Data Science Major at UC Berkeley and have been for two years. In order to graduate with a major in Data Science, you must complete four required classes, one of which is an independent study project.
I have chosen to take this independent study project in order to gain hands-on experience with a data science problem of my choosing and to learn how to effectively apply machine learning algorithms in the real world. My goal is to create an application that can accurately predict where students need tutoring based on their past grades. This project will require me to use various classes of statistical models, such as regression, decision trees, and neural networks.
How to Write a Personal Statement for Masters Programme in Data Science?
If you are looking for the best way to write a sample personal statement for a master in data science, you should follow these steps:
- Step 1: The first step is to find out what courses are available in your area and how long it takes to complete them. You can find this information on websites online.
- Step 2: Once you have this information, you need to think about how much time you will have available each day. It is important that you do not leave your studies until you finish all of your courses because once you finish your degree program, there will be no more work available for you. Your ability to continue working will depend upon how well your personal statement for data science courses was received by universities and whether or not they offer scholarships for those who want to study abroad or online.
- Step 3: In order to write a good personal statement for M.Sc data science, you will need to think about why you want to continue your education after completing your bachelor's degree program. This could be because of what happened during college or because of something else entirely (such as family obligations). If it is something that happened during college, then you will need to explain what that event was and how it has affected your desire to continue your education. If it is something that happened outside of college, then you should talk about how that event impacted your academic performance and why you want to continue studying.
Data Science Personal Statement Example
Following is a data science personal statement example. You can refer to this data science statement of purpose example and keep in mind the necessary points.

Source: personal-statement-examples.com
Tips to Write an Effective Data Science Personal Statement
The following tips will help you write an effective personal statement for a master in data science:
1. Use a Template
It's best to use a template that has been created by experienced admissions officers and other professionals in the field. This means you can skip the writing process entirely since they've already done most of it for you.
2. Keep Your Sentences Short and Simple
Your goal should be no more than one or two paragraphs per section (including your application summary), which means keeping your sentences as short as possible without compromising clarity or coherence. If there are too many adjectives or numbers used in an otherwise simple sentence, try replacing them with action verbs like "ran" instead of "ran fast."
3. Avoid Clichés
In your data science personal statement sample, instead of saying things like "I am dynamic," try saying something more descriptive such as “I am highly dynamic” instead because this shows off how creative your mind works while also showing off how well-rounded personas are important traits needed by anyone working at companies when writing an M.Sc data science personal statement.
Do’s and Don’ts While Writing Personal Statement
Data science is a booming field with a lot of opportunities. You can work anywhere and make a good salary with this skill. If you think that it’s not for you, then it’s time to think again. The world has changed and so have our needs as individuals. Data science professionals will be needed in the future because of their role in shaping our lives as we know them today. In order to pursue a career in this broad field of data science, it is recommended that you pursue KnowledgeHut to learn its principal aspects and gain in-depth knowledge about data science. Data Science Courses to learn its principal aspects and gain in-depth knowledge about data science.
Frequently Asked Questions (FAQs)
Find out what diploma courses are available in your area and how long it takes to complete them. Once you have this information, you need to think about how much time you will have available each day. After evaluating all these things, start writing your personal statement using templates.
- The reason(s) why you selected this subject(s)
- Your chosen area of study and how it relates to the current studies
- Your experiences in relation to your chosen subject(s)
- What are your interests and responsibilities in relation to the subject you are studying?
- After university, what's next?
- A summary of why you will make an excellent student
- Don’t use quotes
- Don’t let spelling and grammatical errors spoil your statement.
- Don’t copy and paste
During the writing of the letter of intent for the MS in Data Science course, it is important to take into account the basic questions asked by the institution, including what kind of ambitions the prospective candidate has and the inspiration behind those ambitions. If the students do not want to sound conversational in their essays, then they should keep in mind that the tone should be formal instead of informal.

Ashish Gulati
Ashish is a techology consultant with 13+ years of experience and specializes in Data Science, the Python ecosystem and Django, DevOps and automation. He specializes in the design and delivery of key, impactful programs.
Avail your free 1:1 mentorship session.
Something went wrong
Upcoming Data Science Batches & Dates

M.S. in Data Science
The m.s. in data science allows students to apply data science techniques to their field of interest..
Ours is one of the most highly-rated and sought-after advanced data science programs in the world.
Program Highlights
Columbia data science students have the opportunity to conduct original research, produce a capstone project , and interact with our industry partners and world-class faculty.
This program is jointly offered in collaboration with the Graduate School of Arts and Sciences’ Department of Statistics, and The Fu Foundation School of Engineering and Applied Science’s Department of Computer Science and Department of Industrial Engineering and Operations Research.
Some students are primarily concerned about data ethics, others are excited about data science as a new evolution in knowledge, but all are interested in how data science is changing our everyday lives.
Where are columbia data science graduates now*.
*A partial list as of May 2022
Computer Science
Prerequisites: Students are expected to have solid programming experience in Python or with an equivalent programming language. This class is intended to be accessible for students who do not necessarily have a background in databases, operating systems or distributed systems. The goal of this class is to provide data scientists and engineers that work with big data a better understanding of the foundations of how the systems they will be using are built. It will also give them a better understanding of the real-world performance, availability and scalability challenges when using and deploying these systems at scale. In the course we will cover foundational ideas in designing these systems, while focusing on specific popular systems that students are likely to encounter at work or when doing research.
Spring Semester: 3 credits
COMS 4721 is a graduate-level introduction to machine learning. The course covers basic statistical principles of supervised machine learning, as well as some common algorithmic paradigms. Additional topics, such as representation learning and online learning, may be covered if time permits.
Prerequisites: Background in linear algebra and probability and statistics.
Methods for organizing data, e.g. hashing, trees, queues, lists, priority queues. Streaming algorithms for computing statistics on the data. Sorting and searching. Basic graph models and algorithms for searching, shortest paths, and matching. Dynamic programming. Linear and convex programming. Floating point arithmetic, stability of numerical algorithms, Eigenvalues, singular values, PCA, gradient descent, stochastic gradient descent, and block coordinate descent. Conjugate gradient, Newton and quasi-Newton methods. Large scale applications from signal processing, collaborative filtering, recommendations systems, etc.
Prerequisites: basic knowledge in programming (e.g., at the level of COMS W1007), a basic grounding in calculus and linear algebra.
Engineering
Prerequisites: CSOR W4246 Algorithms for Data Science, STAT W4105 Probability, COMS W4121 Computer Systems for Data Science, or equivalent as approved by faculty advisor. Co-requisites: to be completed alongside or after: STAT W4702 Statistical Inference and Modeling, COMS W4721 Machine Learning for Data Science, STAT W4701 Exploratory Data Analysis and Visualization, or equivalent as approved by faculty advisor.
This course provides a unique opportunity for students in the M.S. in Data Science program to apply their knowledge of the foundations, theory and methods of data science to address data science problems in industry, government and the non-profit sector. The course activities focus on a semester-length data science project sponsored by a faculty member or local organization. The project synthesizes the statistical, computational, engineering challenges and social issues involved in solving complex real-world problems.
Fall and Spring Semesters: 3 credits
This course covers the following topics: Fundamentals of probability theory and statistical inference used in data science; Probabilistic models, random variables, useful distributions, expectations, law of large numbers, central limit theorem; Statistical inference; point and confidence interval estimation, hypothesis tests, linear regression.
Prerequisite: Calculus.
Prerequisite: Programming, fundamentals of data visualization, layered grammar of graphics, perception of discrete and continuous variables, introduction to Mondran, mosaic pots, parallel coordinate plots, introduction to ggobi, linked pots, brushing, dynamic graphics, model visualization, clustering and classification.
Course covers fundamentals of statistical inference and testing, and gives an introduction to statistical modeling. The first half of the course will be focused on inference and testing, covering topics such as maximum likelihood estimates, hypothesis testing, likelihood ratio test, Bayesian inference, etc. The second half of the course will provide introduction to statistical modeling via introductory lectures on linear regression models, generalized linear regression models, nonparametric regression, and statistical computing. Throughout the course, real-data examples will be used in lecture discussion and homework problems.
Prerequisites: Working knowledge of calculus and linear algebra (vectors and matrices) and STAT GR5203 or equivalent.
In addition to the 21 credits of core classes, M.S. in Data Science students are required to complete a minimum of nine (9) credits of electives. In addition to the DSI elective courses, MS students are encouraged to explore courses offered across the university and take advantage of the expertise in a wide range of disciplines at Columbia. Prior to registration, students receive advisement to determine if a course of interest is relevant and meets the criteria of a 4000-level or higher, technical course completed for a letter grade. You are welcome to explore the Columbia Directory of Classes for possible courses.
The following courses are examples of classes that MS students have used for elective credit. Elective courses and schedules are dependent on faculty availability and may vary each semester. Past course offerings are not guaranteed to be offered in the future.
Please note that many departments, including DSI, give registration priority to their students. Space permitting, courses are then opened up to students outside the department.
This class offers a hands-on approach to machine learning and data science. The class discusses the application of machine learning methods like SVMs, Random Forests, Gradient Boosting and neural networks on real world dataset, including data preparation, model selection and evaluation. This class complements COMS W4721 in that it relies entirely on available open source implementations in scikit-learn and tensor flow for all implementations. Apart from applying models, we will also discuss software development tools and practices relevant to productionizing machine learning models.
This course provides a practical, hands-on introduction to Deep Learning. We aim to help students understand the fundamentals of neural networks (DNNs, CNNs, and RNNs), and prepare students to successfully apply them in practice. This course will be taught using open-source software, including TensorFlow 2.0. In addition to covering the fundamental methods, we will discuss the rapidly developing space of frameworks and applications, including deep learning on the web. This course includes an emphasis on fairness and testing, and teaches best practices with these in mind.
Data scientists often have to answer questions that will lead to decisions about actions a company might take. Often, they will be able to run an experiment, and see the effect the decision might have by testing it first. Other times, they will only have observational data at their disposal. In both cases, they need to infer the causal effect of an action on some outcomes of interest. Causal inference is an essential skill for a data scientist. Without a proper understanding, potential biases as large as 1000% have been observed in practice! This course will cover the basics of the potential outcomes framework, the Pearlian framework, and a collection of methods for observational and experimental causal inference. We’ll use examples from industry applications throughout the course, especially focused on web applications.
“Data analytics pipeline” focuses on the intersection between data science, data engineering, and agile product development. In this course you’ll learn some common data generating processes, how the data is transported to be stored, how analytics and compute capabilities are built on top of that storage, and how production machine learning and modeling platforms can be built in that context. There is a strong focus on good architecture design patterns, and practical implementation considerations that focus on delivering results over building perfect systems
This course is designed as an introduction to elements that constitutes the skill set of a data scientist. The course will focus on the utility of these elements in common tasks of a data scientist, rather than their theoretical formulation and properties. The course provides a foundation of basic theory and methodology with applied examples to analyze large engineering, business, and social data for data science problems. Hands-on experiments with R or Python will be emphasized.
The world is full of noise and uncertainty. To make sense of it, we collect data and ask questions. Is there a tumor in this x-ray scan? What affects the quality of my manufacturing plant? How old is this planet I see through the telescope? Does this drug actually work? To pose and answer such questions, data scientists must iterate through a cycle: probabilistically model a system, infer hidden patterns from data, and evaluate how well our model describes reality. By the end of this course, you will learn how to use probabilistic programming to effectively iterate through this cycle. Specifically, you will master modeling real-world phenomena using probability models, using advanced algorithms to infer hidden patterns from data, and evaluating the effectiveness of your analysis. You will learn to use (and perhaps even contribute to) Edward throughout this course.
This applied Natural Language Processing course will focus on computational methods for extracting social and interactional meaning from large volumes of text and speech (both traditional media and social media). Topics will include:
- Sentiment Analysis: automatic detection of people’s sentiment towards a topic, event, product, or persons. Practical applications in various domains will be discussed (e.g., predicting stock market prices, or presidential elections)
- Emotion and Mood Analysis: automatic detection of people’s emotions (angry, sad, happy) by analyzing various media such as books, emails, lyrics, online discussion forums. Practical applications in various domains (such as predicting depression, categorization of songs)
- Belief Analysis and Hedging: automatic detection of people’s beliefs (committed belief and non-committed beliefs) from social media. Analysis of the use of hedging as a communicative device in various media: online discussions, scientific writing or legal discussions.
- Deception Detection (e.g., detecting fake reviews online, or deceptive speech in court proceedings)
- Argumentation Mining: automatic detection of arguments from text, such as online discussion or persuasive essays. Practical application for various domains (e.g., political, legal or education (e.g., improving students’ skills in writing persuasive essays)
- Social Power: automatic detection of power structure in organizations by analyzing people’s communications such as emails.
- Extracting Social Networks from text, such as networks of characters from novels, or networks from social media (e.g., people holding particular opinions, or network of friends).
- Personality and Interpersonal Stance
Contact DSI at [email protected] for more information about this course.
Personalization is a key tool for enhancing customer experience across industries, thereby driving user loyalty and customer value. It is therefore no surprise that creating and enhancing personalization systems is also increasingly one of the core responsibilities of data science teams, and a key focus for many of the machine learning algorithms in the sector. This course will focus on common personalization algorithms and theory, including behavior-based and content-based recommendation, commonly encountered issues in scaling and cold-starts, and state of the art research. It will also look at how businesses use, and misuse, these techniques in real world applications.
The vast proliferation of data and increasing technological complexities continue to transform the way industries operate and compete. Over the last two years, 90 percent of the data in the world has been created as a result of the creation of 2.5 quintillion bytes of data on a daily basis. Commonly referred to as big data, this rapid growth and storage creates opportunities for collection, processing and analysis of structured and unstructured data. Financial services, in particular, have widely adopted big data analytics to inform better investment decisions with consistent returns. In conjunction with big data, algorithmic trading uses vast historical data with complex mathematical models to maximize portfolio returns. The continued adoption of big data will inevitably transform the landscape of financial services. However, along with its apparent benefits, significant challenges remain in regards to big data’s ability to capture the mounting volume of data. The increasing volume of market data poses a big challenge for financial institutions. Along with vast historical data, banking and capital markets need to actively manage ticker data. Likewise, investment banks and asset management firms use voluminous data to make sound investment decisions. Insurance and retirement firms can access past policy and claims information for active risk management. The course will be a mix of Theory and practice with real big data cases in finance. We will invite guest lecturers mostly for real Big Data Finance Applications. We will give MATLAB, R, or Python examples.
The course focuses on translating technical expertise into work-place solutions by teaching students to: (1) identify relevant shortfalls in traditional processes; (2) precisely match datasets and machine learning features to overcome these shortfalls; (3) narrowly define value to fit work place processes, analytical framework, and bottom line. Each class will be structured as an actual end-to-end work-place project and use concrete examples to teach students to design, build and deliver solutions that integrate these considerations. A combination of assignments, presentation, and research paper will be sued to evaluation students’ progress in bridging technical and applied solutions with evaluation criteria matching those of a work-place project.
Images are everywhere. How to deal with image data, especially with big data, is an urgent problem for data analysts. Machine learning has proven to be a powerful technology to process and analyze such big data. The course will discuss how machine learning methods are use in the field of image analysis, including biometrics (iris and face recognition), natural images (object identification/recognition), brain images (encoding and decoding), and handwritten digit recognition. Students will learn how to sue traditional machine learning methods in image data processing and analysis, and develop techniques to improve these methods. The aim of this course is to prepare students with basis knowledge and skills to explore opportunities using machine learning in the field of image analysis.
Cross-Registration
- Instructions for Non-Data Science Students Please note that Data Science students have priority registration, so enrollment will be dependent on the space available after our student registration. Non-Data Science students will be able to register/join a waitlist via SSOL starting August 29th for Fall 2023 . Please be sure to obtain your program advisor approval before enrolling. The Fall 2023 Change of Program Period is Tuesday, September 5, 2023 through Friday, September 15, 2023
I wanted to be at an institution that would truly challange me and put me at the forefront of growing areas of research in data science. Columbia promised the most rigorous and innovative curriculum on the planet...I knew the educational experience here would be like no other.
Kevin Womack
Class of 2021
Explore More from Master of Science in Data Science
Are you ready to apply to the M.S. in Data Science program? Check out our FAQ down below or click the Learn More button.
Course Inventory
The number of data science-related courses available to Columbia students is growing. Here's an updated list.
Capstone Projects
All of our students complete capstone projects with industry affiliates and thought leaders.
Introduction to Data Science
Introduction to Data Science: A Comprehensive Overview
In today's data-driven world, the field of data science has emerged as a transformative force, reshaping industries and revolutionizing decision-making processes. This essay provides a comprehensive overview of data science, exploring its key concepts, applications, and impact on various domains.
Data science is an interdisciplinary field that combines elements of statistics, computer science, and domain expertise to extract valuable insights and knowledge from vast amounts of structured and unstructured data. It encompasses a wide range of techniques, including data collection, cleaning, analysis, visualization, and predictive modeling. The primary goal of data science is to uncover patterns, trends, and correlations hidden within data, enabling organizations to make data-driven decisions.
One of the essential aspects of data science is data preprocessing, where raw data is transformed into a usable format. This step involves handling missing values, outlier detection, and data normalization. Once the data is prepared, various statistical and machine learning algorithms are applied to gain meaningful insights. These algorithms range from simple linear regression to complex deep learning models, depending on the complexity of the problem at hand.
Data science finds application in diverse industries. In finance, it aids in fraud detection and risk assessment. In healthcare, it contributes to disease diagnosis and personalized treatment plans. In marketing, it optimizes advertising campaigns and customer segmentation. Moreover, data science has been instrumental in transforming fields like education, transportation, and entertainment.
The impact of data science is evident in the rise of artificial intelligence and machine learning. These technologies rely heavily on data to train models and make predictions. Natural language processing and computer vision are some of the exciting subdomains that have flourished due to data science advancements.
However, the widespread adoption of data science also poses challenges. Ensuring data privacy and security is a significant concern, especially when dealing with sensitive information. Moreover, the interpretability of complex machine learning models remains a subject of ongoing research, as decisions made by these models can have significant consequences.
In conclusion, data science is a powerful discipline that has reshaped the way we understand and leverage data. Its applications are vast and span across numerous industries, driving innovation and efficiency. As technology continues to advance, data science will play a crucial role in shaping our future. Embracing data science's potential while addressing its challenges will pave the way for a data-driven and informed society.
Get started for free

Please enter a valid Name
Please enter a valid email address
Please enter a valid Phone Number
Please enter a valid Password
By creating your account, you agree to the Terms of Service and Privacy Policy .
Already have an account? Sign In here.
Please enter your email address
Show Password
Forgot Password?
Don’t have an account? Sign Up
Verify Your Account
Enter the verification codes to confirm your identity.
Hi, Continue Logging In
Continue Logging In
Verify Your Email
Please check your inbox for a verification code to confirm your identity.
Code sent to [email protected]
Send again in seconds
Code sent to +1 302 385 6690
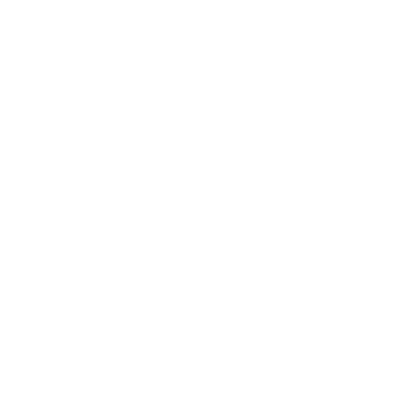
- Equity & Inclusion
2020 Undergraduate HCE Essay Prize
The division of computing, data science, and society held a competition for uc berkeley undergraduates doing independent, original research on social, cultural, historical, policy, or ethical issues at the intersection of data science, technology and society..
This year the prize committee received 37 submissions from students from a wide range of majors, who wrote as part of a diverse group of classes, including DATA 104 (formerly HIST C184D), COLWRIT R4B, CP 101, DEMOG 160, GSPP 117AC, ISF 100J, and RHETORIC R1B. We congratulate all of the entrants on their work and are pleased to announce the prize winners!
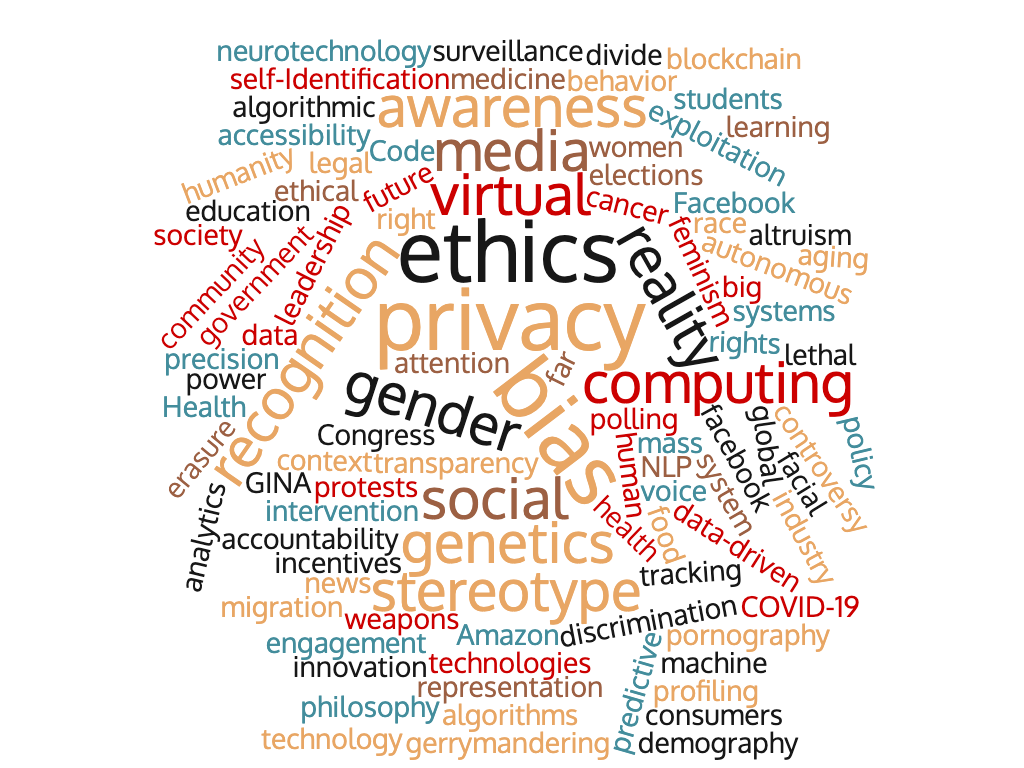
The winners of the inaugural 2020 Undergraduate HCE Essay Prize are:
Honorable mention lex nasser, "a challenging reality".
In his essay, "A Challenging Reality," Lex Nasser considers how values of "open source" are challenged in a context where private companies mediate between open source code contributors and where financial interests are at play. Written in a conversational and personal voice, the essay unpacks a developer's experience of their own agency and the responsibilities that the companies who seek to promote creation and collaboration on-line have to the communities they gather.
Runner Up Razi Mahmood for an untitled essay about the making of movie reviews
In HCE we frequently talk about the life-transforming consequences of representing people with data. We also talk about the significance of the public sphere as a space of expression and deliberation of serious ideas about our common world.
Therefore on first glance, an essay about aggregated scores for movies and the shaping of public opinion through on-line movie reviews , might seem inconsequential. What's the big deal about movie reviews?
Through his careful attention to both the subjective elements of movie reviews (who the reviewers are, the way in which their personal experience with the movie plays into the numerical score of the review and how the score speaks to the viewer) and the social structures (such as marketing dimensions, movie review platform infrastructure, and data-collection process) that inform them, Razi Mahmood makes a seemingly banal topic of the everyday movie choice into a microcosm for exploring questions of representation, opinion and influence in the datafied world.
Not only does the reader learn a lot about the process by which movie reviews are made, but Razi also invites them to consider the significance of the ways in which reviews reflect and shape cultural patterns and tastes, informing the images and narratives that people see and look up to. After reading this essay, you will not be able to look upon the movie review in the same way, and that, after all, is the spirit of HCE: to reframe how we see the daily datafied world around us!
Runner Up Catey Vera, "GINA Should be Amended to Provide Greater Protections"
Over the last decades, personal genetic information has become one of the most ethically challenging and contested domains in the datafied world, putting increased strain on pre-existing regulatory frameworks.
In her essay, "GINA Should be Amended to Provide Greater Protections," Catey Vera takes her readers through the many intricacies of one of the major existing legal frameworks for regulating this area--the Genetic Information Nondiscrimination Act of 2008 and proposes its expansion. In prose that is highly readable and engaging, Catey adeptly explores the real and potential discriminatory impacts of expanded forms of genetic testing on race, education, immigration, and clearly outlines how GINA in its contemporary form fails to adequately prevent it. She concludes by arguing that several key expansions would enable GINA to effectively prevent some of the more egregious consequences of genetic testing. Catey’s essay is a mature, well-researched, and sophisticated exploration of a major issue; it is sensitive to the vast stakes of this problem and makes a powerful and practical attempt to address them. For these reasons, Catey Vera is our second runner-up.
First Place Winner Spring Ma, "Our Broken Society: How Ethics in Tech Can Originate from College Initiatives"
This year’s prize winner exemplified many of the traits and values we associate with the Human Contexts and Ethics of Data. Not only does this essay bring a sharp analytical perspective, a clear call to action, and identify pressing ethical stakes, in clear and direct prose, it also focuses on an issue close to home, appealing to fellow students and to our own institutions here at Berkeley to change for the better.
In her prize winning essay, “Our Broken Society: How Ethics in Tech Can Originate from College Initiatives,” Spring Ma challenges both faculty and fellow students to confront their own ethical responsibility for the companies they choose to bring to campus to recruit students.
In particular, Spring’s essay responds to the student protest that erupted in the Fall of 2019 over Palantir’s participation in EECS’ Corporate Access Program (CAP), owing to the data analytics company’s contracts with Immigration and Customs Enforcement (ICE) to build tools to track and deport undocumented immigrants.
Spring’s essay affirms that this issue does not simply stem from a singular lapse in judgment on the part of individuals, but is a structural issue with how we design our institutions. Appealing to UC Berkeley’s own values, as well as broader shifts taking place within industry in response to the recent “techlash,” Spring thus proposes several concrete and structural reforms designed to help CAP, EECS, and student groups make decisions more informed by the human contexts and ethics of data. In doing so, she positions herself as a responsible and active member of a living community, calling out others to make real change, and thus elevates what began as a merely academic assignment into something much more.
Congratulations to the winners and all of this year's contestants!

ESSAY SAUCE
FOR STUDENTS : ALL THE INGREDIENTS OF A GOOD ESSAY
Essay: Data science and its applications
Essay details and download:.
- Subject area(s): Information technology essays
- Reading time: 8 minutes
- Price: Free download
- Published: 1 February 2016*
- File format: Text
- Words: 2,127 (approx)
- Number of pages: 9 (approx)
Text preview of this essay:
This page of the essay has 2,127 words. Download the full version above.
With vast amounts of data now available, organizations in almost every industry are focused on exploiting data for competitive advantage. On the other hand broad availability of data has led to increasing interest in new methods for extracting beneficial information and knowledge from data. The new discipline called data science has arose as a new paradigm to tackle these vast accumulation of data. Today it applies all most every fields in the world for different aspects. Mainly in security, health care, business, agriculture, transport, education, prediction, telecommunication, etc. Each area will also garner a different amount of return on their data science investment. This review aims to provide an overview of data science and mainly how some of these fields are currently using data science and how they could leverage it in their favor in the future. What is Data Science? According to Dhar, V. (2012), the fact that we now have vast amounts of data should not in and of itself justify the need for a new term âData scienceâ. It is a known fact that the extraction of information from data has been done by the use of statistics over decades. Nevertheless there are many reasons to consider Data science as a new field. First, the raw material, the âdataâ part of Data Science, is increasingly diverse and unstructured â” text, images, and video, frequently arising from networks with complex relationships among its entities. Further he reveals that the relative expected volumes of unstructured and structured data between 2008 and 2015, projecting a difference of almost 200 petabytes in 2015 compared to a difference of 50 petabytes in 2012. Secondly, the creation of markup languages, tags, etc. are mainly designed to let computers interpret data automatically, making them active agents in the manner of decision making (Dhar, V. (2012)). i.e. computers are increasingly doing the background work for each other. In the proceedings paper by Zhu, Y. & Xiong, Y. (2015), they have mentioned that the Data science research objects, goals, and techniques are essentially different from those of computer science, information science or knowledge science. Throughout the paper they always use a comparative method to discuss how data science differs from existing technologies and established sciences. According to them data science supports natural science and social science and dealing with data is one of the driving forces behind data science. Hence, they referred data science as a data-intensive science. It is evident that data science should be considered as a new science and new techniques and methods should be introduced in order to deal with its vast amount of data. Dhar, V. (2012) nicely explains how traditional data base methods are not suited for knowledge discovery. He explains that traditional data base methods are optimized for summarization of data given what the user wants to ask but not discovery of patterns in massive amount of data when the user does not have a well formulated query. i.e. Unlike database querying which asks âwhat data satisfy this patternâ discovery is about asking âwhat patterns satisfy the given data?â. Specifically ultimate goal of Data science is to finding interesting and robust patterns that satisfy the data. When the new technologies emerged it lead to research on the data themselves. Lot of fields such as health care, business got the advantage of that and they were able to discover growth patterns of data and predict the scale of data in cyberspace ten years into the future(Zhu, Y. & Xiong, Y. (2015)).This causes to discover lot of new theories, inventions, that havenât been uncovered for years. Many health related issues have been identified and solved using big data analytics. Applications of Data Science 1) Health care A key contemporary trend emerging in big data science is the quantified self (QS) -individuals engaged in the self-tracking of any kind of biological, physical, behavioral, or environmental information as n = 1 individuals or in groups(Swan, M. (2013)). In this article writer emphasis that Quantified self-projects are becoming an interesting data management and manipulation challenge for big data science in the areas of data collection, integration, and analysis. But at the same time he reveals, when as much larger QS data sets are being generated the quantified self, and health and biology more generally, are becoming full-fledged big data problems in many ways. Variety of self-tracking projects were conducted recently including food visualization, investigation of media consumption and reading habits, multilayer investigation in to diabetes and heart disease risk, idea tracking process, etc. These projects demonstrate the range of topics, depth of problem solving, and variety of methodologies of QS projects. Big health data streams are the main data stream in QS and most difficult task is to integrating big health data streams, especially blending genomic and environmental data. It was found that genetics has a one third of contribution of outcome to diseases like cancer and heart disease. Projects such as DIY genomics studies, 4P medicine personalized, Crohnâs disease tracking microbiomic sequencing, lactoferrin analysis project and Thyroid Hormone testing project are famous examples for applications of QS in genomics. These findings couldnât be found if data science doesnât exist or the newly tools which can handle large volume of data were not invented. Further Swan, M. (2013) suggests QS data streams need to be linked to healthy population longitudinal self-tracking more generally as they are the corresponding healthy cohorts to patient cohorts in clinical trials and he predicts eye tracking and emotion measurement could be coming in the future. X. Shi and S. Wang (2015) have written an article to provide an overview of theoretical background of applying the cyberGIS (Geographical information science) approach to spatial analysis for health studies. (cyberGIS is defined as geographic information systems and science based on advanced cyberinfrastructure.) As spatial analysis is a tool for analyze big data it has a high usage in medical fields. According to them many review of literature find that a majority of the methods use only geographically local information and generate non-parametric measurements. It can be found multiple related cases where computational and data sciences are central to solving challenging problems in the framework of health-GIS. Disease mapping is one of the major areas and it is basically used to measure the intensity of a disease in a particular area. Data aggregation is a method which was developed to deal with cancer registry data bases and birth defect data bases. These applications were not only limited for the disease base assessments but it also assess the environmental facts which associate with health such as disparities in geographic access to health care. X. Shi and S. Wang (2015) mentioned a study which estimated the distances or travel times from patientsâ locations represented by polygon level data. In conclusion, the above mentioned two articles review that health care is a field with lot of untapped potentials and the use of big data or data science is not only limited for find remedies for diseases but also it assess the other factors such as which causes the efficiency of health care. 2) Social media and networks Swan, M. (2013) points out having large data quantities continues to allow for new methods and discovery. As Google has proved, finally having large enough data sets was the key moment for progress in many venues, where simple machine-learning algorithms could then be run over large data amounts to produce significant results. She explains this through googlesâ spelling correction and language translation, image recognition and cultural anthropology via word searches on a database of 5 million digitally scanned books. Dhar, V. (2012) also address this manner in his article and further he explains that Googleâs language translator doesnât âunderstandâ language, nor do its algorithms know the contents on webpages. So such efficient and accurate systems were invented using machine learning algorithms not by tackle this problem through an extensive enumeration of possibilities but rather, âtrainâ a computer to interpret questions correctly based on large numbers of examples. In addition, he emphasis knowledge of text processing or âtext miningâ is becoming essential in light of the explosion of text and other unstructured data in healthcare systems, social networks, and other sectors. 3) Education Data science has been attracting a great deal of attention nowadays in academia and environments which dealt with theories and formulas. It improves the current research methods for scientific research in order to form new methods and improve specific theories, methods, and technologies in various fields (Zhu, Y. & Xiong, Y. (2015)). Vast accumulation of data provides the opportunity to filter considerably large portion which is useful to a particular object. It provides a great platform to research rare and important matters in any field. At the same time they argued data science itself requires more fundamental theories and new methods and techniques; for example, the existence of data, the measurement of data, time in cyberspace, data algebra, data similarities and the theory of clusters, data classification etc. New action plans, conferences, workshops, Data science journals, institutes specifically for data science, study materials in universities to study data science as a subject, etc. will increase the awareness and understanding on this new science. 4) Business Business field is one of the major sectors which gain benefits from data science principals and data mining techniques. Data mining techniques are widely used in marketing for tasks such as targeted marketing, online advertising, and recommendations for cross-selling (Provost, F. & Fawcett, T. (2013)). According to them data science is mainly used in business fields with the objective of improving decision making. They have mentioned two types of decisions; (1) decisions for which âdiscoveriesâ need to be made within data, and (2) decisions that repeat, especially at massive scale. In this article Provost, F. & Fawcett, T. (2013) nicely explain how the companies trying to increase their customers by using data science approach. They give an example using company called âTargetâ who sells baby related products. In order to increase the no of customers they were interested in whether they could predict that people are expecting a baby in advance. If so, they could make offers to them before their competitors. Usually most birth records are public, so retailers obtain those information and aware the new parents about their new offers. If the information could get before the baby was born then the ones who got that information first would gain an advantage on their marketing campaign. By using data science techniques, they analyzed historical data on customers and identified group of customers who later revealed to have been pregnant. This can be predicted by using change in motherâs diet, vitamin regimens, etc. According to Provost, F. & Fawcett, T. (2013), banking sector is also gain advantage of using Data science, and they were able to do more sophisticated predictive modeling on pricing, credit limits, low-initial-rate balance transfers, cash back, loyalty points, etc. Specially credit card system is also a outcome of big data analytics. Further they claim that the banks with bigger data assets may have an important strategic advantage over their smaller competitors.so the net result will be either increased acceptance of the bankâs products, decreased cost of customer acquirement, or both. 5) Telecommunication Customer churn is the most critical problem that service providers face. Customers switching from one company to another is called churn (Provost, F. & Fawcett, T. (2013)). They state that attracting new customers is much more expensive than retaining existing customers. So each and every service provider is trying to prevent customer chain by giving them a new retention offer. Data mining techniques are majorly used to identify customers who tend to churn. Challenges and barriers In this process lot of personal data were stored in each individual from any sector. With these vast amount of personal data there are certain boundaries and issues which the researchers or data scientists should be considered. Mainly in health data lot of patients are not comfortable with sharing their data in public (Swan, M. (2013)). In her opinion it is necessary to think about personal data privacy rights and neural data privacy rights proactively to facilitate humanityâs future directions in a mature, comfortable, and empowering way. Dhar, V. (2012) also address this matter. He explains with the vast technology development computer has become the decision maker, unaided by the humans and it raises multitude issues such as cost of incorrect decisions and ethical issues. Conclusion It is evident Data science is a newly emerged science that requires overall knowledge mainly in computational science, statistics and mathematics. New technologies are emerged to dealing with massive amount of data in any field and benefits are many and they are ranging from health care to telecommunication. At the same time they should be handled cautiously to ensure that the respondentsâ information are not exploited. In the near future data science will uncover many discoveries that support humans to improve their life style in every aspect.
...(download the rest of the essay above)
About this essay:
If you use part of this page in your own work, you need to provide a citation, as follows:
Essay Sauce, Data science and its applications . Available from:<https://www.essaysauce.com/information-technology-essays/data-science-and-its-applications/> [Accessed 16-05-24].
These Information technology essays have been submitted to us by students in order to help you with your studies.
* This essay may have been previously published on Essay.uk.com at an earlier date.
Essay Categories:
- Accounting essays
- Architecture essays
- Business essays
- Computer science essays
- Criminology essays
- Economics essays
- Education essays
- Engineering essays
- English language essays
- Environmental studies essays
- Essay examples
- Finance essays
- Geography essays
- Health essays
- History essays
- Hospitality and tourism essays
- Human rights essays
- Information technology essays
- International relations
- Leadership essays
- Linguistics essays
- Literature essays
- Management essays
- Marketing essays
- Mathematics essays
- Media essays
- Medicine essays
- Military essays
- Miscellaneous essays
- Music Essays
- Nursing essays
- Philosophy essays
- Photography and arts essays
- Politics essays
- Project management essays
- Psychology essays
- Religious studies and theology essays
- Sample essays
- Science essays
- Social work essays
- Sociology essays
- Sports essays
- Types of essay
- Zoology essays
- Share full article
Advertisement
Supported by
Letter of Recommendation
What I’ve Learned From My Students’ College Essays
The genre is often maligned for being formulaic and melodramatic, but it’s more important than you think.

By Nell Freudenberger
Most high school seniors approach the college essay with dread. Either their upbringing hasn’t supplied them with several hundred words of adversity, or worse, they’re afraid that packaging the genuine trauma they’ve experienced is the only way to secure their future. The college counselor at the Brooklyn high school where I’m a writing tutor advises against trauma porn. “Keep it brief , ” she says, “and show how you rose above it.”
I started volunteering in New York City schools in my 20s, before I had kids of my own. At the time, I liked hanging out with teenagers, whom I sometimes had more interesting conversations with than I did my peers. Often I worked with students who spoke English as a second language or who used slang in their writing, and at first I was hung up on grammar. Should I correct any deviation from “standard English” to appeal to some Wizard of Oz behind the curtains of a college admissions office? Or should I encourage students to write the way they speak, in pursuit of an authentic voice, that most elusive of literary qualities?
In fact, I was missing the point. One of many lessons the students have taught me is to let the story dictate the voice of the essay. A few years ago, I worked with a boy who claimed to have nothing to write about. His life had been ordinary, he said; nothing had happened to him. I asked if he wanted to try writing about a family member, his favorite school subject, a summer job? He glanced at his phone, his posture and expression suggesting that he’d rather be anywhere but in front of a computer with me. “Hobbies?” I suggested, without much hope. He gave me a shy glance. “I like to box,” he said.
I’ve had this experience with reluctant writers again and again — when a topic clicks with a student, an essay can unfurl spontaneously. Of course the primary goal of a college essay is to help its author get an education that leads to a career. Changes in testing policies and financial aid have made applying to college more confusing than ever, but essays have remained basically the same. I would argue that they’re much more than an onerous task or rote exercise, and that unlike standardized tests they are infinitely variable and sometimes beautiful. College essays also provide an opportunity to learn precision, clarity and the process of working toward the truth through multiple revisions.
When a topic clicks with a student, an essay can unfurl spontaneously.
Even if writing doesn’t end up being fundamental to their future professions, students learn to choose language carefully and to be suspicious of the first words that come to mind. Especially now, as college students shoulder so much of the country’s ethical responsibility for war with their protest movement, essay writing teaches prospective students an increasingly urgent lesson: that choosing their own words over ready-made phrases is the only reliable way to ensure they’re thinking for themselves.
Teenagers are ideal writers for several reasons. They’re usually free of preconceptions about writing, and they tend not to use self-consciously ‘‘literary’’ language. They’re allergic to hypocrisy and are generally unfiltered: They overshare, ask personal questions and call you out for microaggressions as well as less egregious (but still mortifying) verbal errors, such as referring to weed as ‘‘pot.’’ Most important, they have yet to put down their best stories in a finished form.
I can imagine an essay taking a risk and distinguishing itself formally — a poem or a one-act play — but most kids use a more straightforward model: a hook followed by a narrative built around “small moments” that lead to a concluding lesson or aspiration for the future. I never get tired of working with students on these essays because each one is different, and the short, rigid form sometimes makes an emotional story even more powerful. Before I read Javier Zamora’s wrenching “Solito,” I worked with a student who had been transported by a coyote into the U.S. and was reunited with his mother in the parking lot of a big-box store. I don’t remember whether this essay focused on specific skills or coping mechanisms that he gained from his ordeal. I remember only the bliss of the parent-and-child reunion in that uninspiring setting. If I were making a case to an admissions officer, I would suggest that simply being able to convey that experience demonstrates the kind of resilience that any college should admire.
The essays that have stayed with me over the years don’t follow a pattern. There are some narratives on very predictable topics — living up to the expectations of immigrant parents, or suffering from depression in 2020 — that are moving because of the attention with which the student describes the experience. One girl determined to become an engineer while watching her father build furniture from scraps after work; a boy, grieving for his mother during lockdown, began taking pictures of the sky.
If, as Lorrie Moore said, “a short story is a love affair; a novel is a marriage,” what is a college essay? Every once in a while I sit down next to a student and start reading, and I have to suppress my excitement, because there on the Google Doc in front of me is a real writer’s voice. One of the first students I ever worked with wrote about falling in love with another girl in dance class, the absolute magic of watching her move and the terror in the conflict between her feelings and the instruction of her religious middle school. She made me think that college essays are less like love than limerence: one-sided, obsessive, idiosyncratic but profound, the first draft of the most personal story their writers will ever tell.
Nell Freudenberger’s novel “The Limits” was published by Knopf last month. She volunteers through the PEN America Writers in the Schools program.
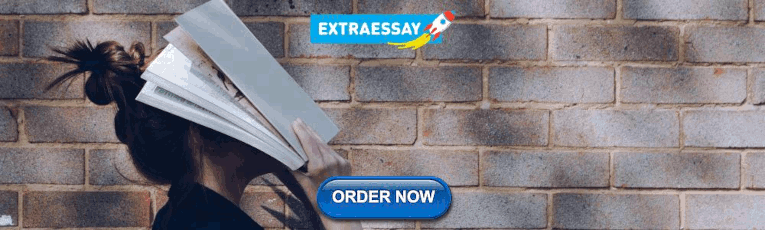
IMAGES
COMMENTS
The Structure of a Successful Data Science SOP. It goes without saying that Bennett used the SOP Starter Kit to outline his essay. That means he structured the paragraphs as follows: Introduction Frame Narrative - 1 paragraph (12% of word count) Why This Program - 2 paragraphs (23% of word count)
Data Science Personal Statement Example. Ever since the commencement of high school studies, I was keen to know application of mathematics in factuality. Its abstract nature intrigued me to question my teachers about relevance and usefulness to mankind. I wanted to apply my learning and understanding invariably in real world circumstances.
This is an example personal statement for a Masters degree application in Data Science. See our guide for advice on writing your own postgraduate personal statement. The emergence of big data over the past decade as a power for good - and, dare I say it, evil - has convinced me of the importance of developing and honing my skills in this arena.
Here are a few tips that will help you get started: The first step is to start early, as early as possible (because there are going to be numerous revisions). If you are reading this story on ...
An attention-grabbing intro and a hard-hitting conclusion are again critical for writing a compelling personal statement. The first paragraph can create the first impression of the applicant in front of the professors, and a sharp end will help them remember that candidate among the crowd. The readers of the personal statement are the experts ...
These short essays are an opportunity to articulate your candidacy for the Master of Science in Data Science program at the University of Washington. The best essays are clear, succinct, thoughtful, well-written, and engaging. Your essays play an important role in our holistic admissions process, and we expect that they are your own original work.
This approach ensures that your essay remains coherent and focused. Begin each paragraph with a topic sentence that introduces the paragraph's main idea. Include relevant data and figures to support your points, ensuring they are appropriately cited. Provide analysis and interpretation of the data, explaining how it supports your thesis.
Insert a quote from a well-known person. Challenge the reader with a common misconception. Use an anecdote, which is a short story that can be true or imaginary. Credibility is crucial when writing a personal statement as part of your college application process. If you choose a statistic, quote, or misconception for your hook, make sure it ...
A data science personal statement is an integral part of the application process for aspiring data scientists. It provides recruiters and admissions board representatives insight into a candidate's motivations, skills, and abilities related to their chosen field of study. The statement should demonstrate a clear understanding of the concepts ...
Application Advice Part 4: The Essays. Most graduate school applicants will agree - writing application essays is the most difficult and stressful part. While writing your essays may seem daunting, this is your chance to differentiate yourself from other applicants with comparable qualifications. It is likely that dozens of applicants, if not ...
1. Introduction. Statistics and data science are more popular than ever in this era of data explosion and technological advances. Decades ago, John Tukey (Brillinger, 2014) said, "The best thing about being a statistician is that you get to play in everyone's backyard."More recently, Xiao-Li Meng (2009) said, "We no longer simply enjoy the privilege of playing in or cleaning up everyone ...
1. Photo by Freddy Castro on Unsplash. The data science blogging ecosystem is rich and growing. TDS alone has an archive of more than 20,000 posts across numerous topics. Many experts have launched Substacks, newsletters, or personal blogs. If you're looking for great new reads to add to your roster, check out Vicky Boykis, Randy Au, or start ...
They will stress the importance of structure, substance and style. They will urge you to write down your methodology and results first, then progress to the literature review, introduction and conclusions and to write the summary or abstract last. To write clearly and directly with the reader's expectations always in mind.
Weaknesses: I got a C- in my first college math class (Calc 1). I didn't know how to code. Like, at all. What's a portfolio? Positives: I had taken up to Calc 4 and linear algebra (because I'm a sucker for punishment). My grades had gotten better over time. 1st place in enthusiasm. MS in Data Science/Analytics Programs: Applied: 12 ...
The essay also gives you an opportunity to demonstrate your understanding of the specific computer science program at the college or university you are applying to. You can discuss how the program's resources, faculty, curriculum, and culture align with your academic interests and career goals.
Definition, Examples, Tools & More. Data science is an interdisciplinary field that uses scientific methods, processes, algorithms, and systems to extract knowledge and insights from structured and unstructured data. Data science has been hailed as the 'sexiest job of the 21st century', and this is not just a hyperbolic claim.
Recommendation 2.3: To prepare their graduates for this new data-driven era, academic institutions should encourage the development of a basic understanding of data science in all undergraduates. Recommendation 2.4: Ethics is a topic that, given the nature of data science, students should learn and practice throughout their education.
Step 3: In order to write a good personal statement for M.Sc data science, you will need to think about why you want to continue your education after completing your bachelor's degree program. This could be because of what happened during college or because of something else entirely (such as family obligations).
Program Highlights. Columbia data science students have the opportunity to conduct original research, produce a capstone project, and interact with our industry partners and world-class faculty.. This program is jointly offered in collaboration with the Graduate School of Arts and Sciences' Department of Statistics, and The Fu Foundation School of Engineering and Applied Science's ...
This essay provides a comprehensive overview of data science, exploring its key concepts, applications, and impact on various domains. Data science is an interdisciplinary field that combines elements of statistics, computer science, and domain expertise to extract valuable insights and knowledge from vast amounts of structured and unstructured ...
The Division of Computing, Data Science, and Society held a competition for UC Berkeley undergraduates doing independent, original research on social, cultural, historical, policy, or ethical issues at the intersection of data science, technology and society. This year the prize committee received 37 submissions from students from a wide range of majors, who wrote as part of a diverse group of ...
Applications of Data Science. 1) Health care. A key contemporary trend emerging in big data science is the quantified self (QS) -individuals engaged in the self-tracking of any kind of biological, physical, behavioral, or environmental information as n = 1 individuals or in groups (Swan, M. (2013)). In this article writer emphasis that ...
Taken in Beijing, PRC, circa 2018 (AKA before covid); I'm the blonde in front! Grad school SOPs: They can feel like such a beast. I wasn't a perfect student when I was applying to M.S. in Data Science programs; in fact, I was far from it.I had never touched Python, never ran a machine learning algorithm, and hadn't had a sexy internship at Google or Facebook to speak of.
Andreas Kuster, a PhD student from Nanyang Technological University's College of Computing and Data Science (CCDS), has been named one of the three winners of the 53 rd St. Gallen Symposium's essay award. His essay, titled "Beyond the Noise: Innovating Information Verification in the Digital Age", stood out among over 700 submissions from all ...
By Nell Freudenberger. May 14, 2024. Most high school seniors approach the college essay with dread. Either their upbringing hasn't supplied them with several hundred words of adversity, or ...
Maricopa Community Colleges' bachelor's degrees are one-third the cost of Arizona's in-state universities, saving students $7,000 to $10,000 annually. The current bachelor's degree programs available include: Behavioral Science (BS) at SMCC. Data Analytics and Programming (BAS) at MCC.