A review on face recognition systems: recent approaches and challenges
- Published: 30 July 2020
- Volume 79 , pages 27891–27922, ( 2020 )
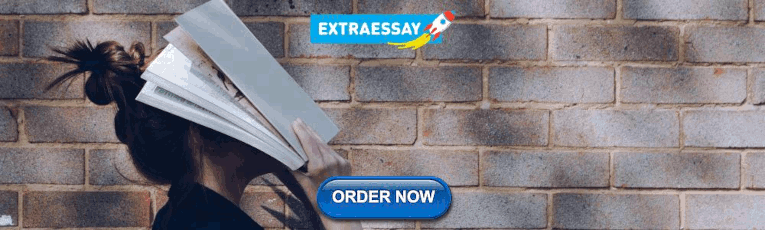
Cite this article
- Muhtahir O. Oloyede 1 , 2 ,
- Gerhard P. Hancke 2 &
- Hermanus C. Myburgh 2
4236 Accesses
56 Citations
Explore all metrics
Face recognition is an efficient technique and one of the most preferred biometric modalities for the identification and verification of individuals as compared to voice, fingerprint, iris, retina eye scan, gait, ear and hand geometry. This has over the years necessitated researchers in both the academia and industry to come up with several face recognition techniques making it one of the most studied research area in computer vision. A major reason why it remains a fast-growing research lies in its application in unconstrained environments, where most existing techniques do not perform optimally. Such conditions include pose, illumination, ageing, occlusion, expression, plastic surgery and low resolution. In this paper, a critical review on the different issues of face recognition systems are presented, and different approaches to solving these issues are analyzed by presenting existing techniques that have been proposed in the literature. Furthermore, the major and challenging face datasets that consist of the different facial constraints which depict real-life scenarios are also discussed stating the shortcomings associated with them. Also, recognition performance on the different datasets by researchers are also reported. The paper is concluded, and directions for future works are highlighted.
This is a preview of subscription content, log in via an institution to check access.
Access this article
Price excludes VAT (USA) Tax calculation will be finalised during checkout.
Instant access to the full article PDF.
Rent this article via DeepDyve
Institutional subscriptions
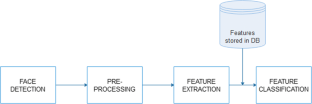
Similar content being viewed by others
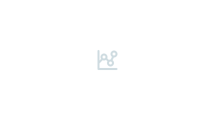
The ethical application of biometric facial recognition technology
Marcus Smith & Seumas Miller
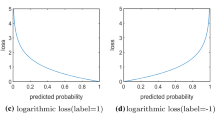
A Comprehensive Survey of Loss Functions in Machine Learning
Qi Wang, Yue Ma, … Yingjie Tian
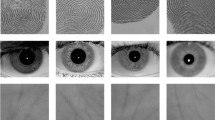
Biometrics recognition using deep learning: a survey
Shervin Minaee, Amirali Abdolrashidi, … David Zhang
Abate AF, Nappi M, Riccio D, Sabatino G (2007) 2D and 3D face recognition: a survey. Pattern Recogn Lett 28:1885–1906
Google Scholar
Ali ASO, Sagayan V, Malik A, Aziz A (2016) Proposed face recognition system after plastic surgery. IET Comput Vis 10:344–350
Alkkiomaki O, Kyrki V, Liu Y, Handroos H, and Kalviainen H (2009) Multi-modal force/vision sensor fusion in 6-DOF pose tracking," in Advanced Robotics. ICAR 2009. International conference on 2009,, pp. 1–8.
Angadi SA, Kagawade VC (2017) A robust face recognition approach through symbolic modeling of polar FFT features. Pattern Recogn 71:235–248
Bartlett MS, Movellan JR, Sejnowski TJ (2002) Face recognition by independent component analysis. IEEE Trans Neural Netw 13:1450–1464
Belahcene M, Chouchane A, and Ouamane H (2014) 3D face recognition in presence of expressions by fusion regions of interest," in 2014 22nd Signal Processing and Communications Applications Conference (SIU), pp. 2269–2274.
Bhat FA, Wani MA (2016) Elastic bunch graph matching based face recognition under varying lighting, pose, and expression conditions. IAES International Journal of Artificial Intelligence (IJ-AI) 3:177–182
Bolme DS (2003) Elastic bunch graph matching. Colorado State University
Bowyer KW, Chang K, Flynn P (2006) A survey of approaches and challenges in 3D and multi-modal 3D+ 2D face recognition. Comput Vis Image Underst 101:1–15
Breiman L (2001) Random forests. Mach Learn 45:5–32
MATH Google Scholar
Brunelli R, Poggio T (1993) Face recognition: features versus templates. IEEE Trans Pattern Anal Mach Intell 15:1042–1052
Cao X, Shen W, Yu L, Wang Y, Yang J, Zhang Z (2012) Illumination invariant extraction for face recognition using neighboring wavelet coefficients. Pattern Recogn 45:1299–1305
Chen L, Liang M, Song W, and Xiao K (2018) A multi-scale parallel convolutional neural network based intelligent human identification using face information. Journal of Information Processing Systems, vol. 14.
Cheng EJ, Chou KP, Jin S, Tanveer M, Lin CT, Young KY, Lin WC, Prasad M (2019) Deep sparse representation classifier for facial recognition and detection system. Pattern Recogn Lett 125:71–77
Chihaoui M, Elkefi A, Bellil W, Ben Amar C (2016) A survey of 2D face recognition techniques. Computers 5:21
Chu Y, Ahmad T, Bebis G, Zhao L (2017) Low-resolution face recognition with single sample per person. Signal Process 141:144–157
Chude-Olisah CC, Sulong G, Chude-Okonkwo UA, Hashim SZ (2014) Face recognition via edge-based Gabor feature representation for plastic surgery-altered images. EURASIP Journal on Advances in Signal Processing 2014:102
Delac K, Grgic M, Grgic S (2005) Independent comparative study of PCA, ICA, and LDA on the FERET data set. Int J Imaging Syst Technol 15:252–260
Deng W, Jiani H, Jun G (2017) Face recognition via collaborative representation: its discriminant nature and superposed representation. IEEE Transaction on pattern analysis and machine intelligence 40:2513–2521
Ding C, Tao D (2017) Pose-invariant face recognition with homography-based normalization. Pattern Recogn 66:144–152
Ding C, Hu Z, Karmoshi S, Zhu M (2017) A novel two-stage learning pipeline for deep neural networks. Neural processing letters
Drira H, Amor BB, Srivastava A, Daoudi M, Slama R (2013) 3D face recognition under expressions, occlusions, and pose variations. IEEE Trans Pattern Anal Mach Intell 35:2270–2283
Feng Z-H, Kittler J, Awais M, Huber P, and Wu X-J (2017) Face detection, bounding box aggregation and pose estimation for robust facial landmark localisation in the Wild, arXiv preprint arXiv:1705.02402.
Fu Y, Wu X, Wen Y, Xiang Y (2017) Efficient locality-constrained occlusion coding for face recognition. Neurocomputing 260:104–111
Gao G, Yang J, Jing X-Y, Shen F, Yang W, Yue D (2017) Learning robust and discriminative low-rank representations for face recognition with occlusion. Pattern Recogn 66:129–143
Gao C-z, Cheng Q, He P, Susilo W, Li J (2018) Privacy-preserving naive Bayes classifiers secure against the substitution-then-comparison attack. Inf Sci 444:72–88
MathSciNet MATH Google Scholar
Ghiass RS, Arandjelović O, Bendada A, Maldague X (2014) Infrared face recognition: a comprehensive review of methodologies and datasets. Pattern Recogn 47:2807–2824
Goyal SJ, Upadhyay AK, Jadon R, and Goyal R (2018) Real-life facial expression recognition systems: a review," in Smart Computing and Informatics, ed: Springer, pp. 311–331.
Guo Y, Zhang L, Hu Y, He X, and Gao J (2016) Ms-celeb-1m: A dataset and benchmark for large-scale face recognition, in European Conference on Computer Vision, pp. 87–102.
Hanmandlu M, Gupta D, and Vasikarla S (2013) Face recognition using Elastic bunch graph matching. in Applied Imagery Pattern Recognition Workshop (AIPR): Sensing for Control and Augmentation, 2013 IEEE, pp. 1–7.
Heo J, Marios S (2008) Face recognition across pose using view based active appearance models on CMU multi-PIE dataset. In Proceeding of International Conference on Computer Vision Systems, May, pp 527–535
Hijazi S, Kumar R, and Rowen C (2015) Using convolutional neural networks for image recognition, ed.
Ho C, Morgado P, Persekian A, Vasconcelos N (2019) "PIEs: pose invariant Embeddings," IEEE/CVF conference on computer vision and pattern recognition (CVPR). Long Beach, CA, USA, pp 12369–12378. https://doi.org/10.1109/CVPR.2019.01266
Book Google Scholar
Hsu G-SJ, Ambikapathi A, Chung S-L, Shie H-C (2018) Robust cross-pose face recognition using landmark oriented depth warping. J Vis Commun Image Represent 53:273–280
Hu H (2008) ICA-based neighborhood preserving analysis for face recognition. Comput Vis Image Underst 112:286–295
Huang D, Shan C, Ardabilian M, Wang Y, Chen L (2011) Local binary patterns and its application to facial image analysis: a survey. IEEE Trans Syst Man Cybern Part C Appl Rev 41:765–781
Jia S, Lansdall-Welfare T, and Cristianini N (2016) Gender classification by deep learning on millions of weakly labelled images, in Data Mining Workshops (ICDMW), 2016 IEEE 16th International Conference on, pp. 462–467.
Jiang L, Li C, Wang S, Zhang L (2016) Deep feature weighting for naive Bayes and its application to text classification. Eng Appl Artif Intell 52:26–39
Jin X, Tan X (2017) Face alignment in-the-wild: a survey. Comput Vis Image Underst 162:1–22
Jin T, Liu Z, Yu Z, Min X, Li L (2017) Locality preserving collaborative representation for face recognition. Neural Process Lett 45:967–979
Kakadiaris IA, Toderici G, Evangelopoulos G, Passalis G, Chu D, Zhao X, Shah SK, Theoharis T (2017) 3D-2D face recognition with pose and illumination normalization. Comput Vis Image Underst 154:137–151
Karamizadeh S, Abdullah SM, Zamani M, Shayan J, and Nooralishahi P (2017) Face recognition via taxonomy of illumination normalization," in Multimedia Forensics and Security, ed: Springer, pp. 139–160.
Kim P (2017) Convolutional Neural Network, in MATLAB Deep Learning, ed: Springer, pp. 121–147.
Kotropoulos C, Pitas I, Fischer S, and Duc B (1997) Face authentication using morphological dynamic link architecture," in Audio-and Video-based Biometric Person Authentication, pp. 169–176.
Lades M, Vorbruggen JC, Buhmann J, Lange J, von der Malsburg C, Wurtz RP, Konen W (1993) Distortion invariant object recognition in the dynamic link architecture. IEEE Trans Comput 42:300–311
Lahasan BM, Venkat I, Al-Betar MA, Lutfi SL, De Wilde P (2016) Recognizing faces prone to occlusions and common variations using optimal face subgraphs. Appl Math Comput 283:316–332
Le QV (2013) Building high-level features using large scale unsupervised learning, in Acoustics, Speech and Signal Processing (ICASSP), 2013 IEEE International Conference on, pp. 8595–8598.
Lei G, Li X-h, Zhou J-l, and Gong X-g (2009) Geometric feature based facial expression recognition using multiclass support vector machines," in Granular Computing, 2009, GRC'09. IEEE International Conference on, pp. 318–321.
Li L-y, Li D-r (2010) Research on particle swarm optimization in remote sensing image enhancement [J]. Journal of Geomatics Science and Technology 2:012
Li M, Yuan B (2005) 2D-LDA: a statistical linear discriminant analysis for image matrix. Pattern Recogn Lett 26:527–532
Li Z, Gong D, Li X, Tao D (2016) Aging face recognition: a hierarchical learning model based on local patterns selection. IEEE Trans Image Process 25:2146–2154
Li Y, Wang Y, Liu J, Hao W (2018) Expression-insensitive 3D face recognition by the fusion of multiple subject-specific curves. Neurocomputing 275:1295–1307
Liao S, Lei Z, Yi D, and Li SZ (2014) A benchmark study of large-scale unconstrained face recognition," in Biometrics (IJCB), 2014 IEEE International Joint Conference on, pp. 1–8.
Liu H-D, Yang M, Gao Y, Cui C (2014) Local histogram specification for face recognition under varying lighting conditions. Image Vis Comput 32:335–347
Long Y, Zhu F, Shao L, and Han J (2017) Face recognition with a small occluded training set using spatial and statistical pooling. Inf Sci.
Lopes AT, de Aguiar E, De Souza AF, Oliveira-Santos T (2017) Facial expression recognition with convolutional neural networks: coping with few data and the training sample order. Pattern Recogn 61:610–628
Luan X, Fang B, Liu L, Yang W, Qian J (2014) Extracting sparse error of robust PCA for face recognition in the presence of varying illumination and occlusion. Pattern Recogn 47:495–508
Ma X, Song H, Qian X (2015) Robust framework of single-frame face Superresolution across head pose, facial expression, and illumination variations. IEEE Transactions on Human-Machine Systems 45:238–250
Manjani I, Sumerkan H, Flynn PJ, and Bowyer KW (2016) Template aging in 3D and 2D face recognition," in Biometrics Theory, Applications and Systems (BTAS), 2016 IEEE 8th International Conference on, pp. 1–6.
Martinez AM (1998) The AR face dataset, CVC technical report, vol. 24.
Martins JA, Lam R, Rodrigues J, du Buf J (2018) Expression-invariant face recognition using a biological disparity energy model. Neurocomputing 297:82–93
Masi L, Rawls S, Medioni G, and Natarajan P (2016) Pose-aware face recognition in the wild. In Proceedings of the IEEE Conference on computer vision and pattern recognition, pp. 4838–4846.
Mi J-X, Liu T (2016) Multi-step linear representation-based classification for face recognition. IET Comput Vis 10:836–841
Nappi M, Ricciardi S, Tistarelli M (2016) Deceiving faces: when plastic surgery challenges face recognition. Image Vis Comput 54:71–82
Oloyede MO, Hancke GP (2016) Unimodal and multimodal biometric sensing systems: a review. IEEE Access 4:7532–7555
Oloyede MO, Hancke GP, and Kapileswar N (2017) Evaluating the effect of occlusion in face recognition systems, In Proceedings of IEEE Africon Conference, pp. 1547–1551.
Oloyede MO, Hancke GP, and Myburgh HC (2018) Improving face recognition systems using a new image enhancement technique, hybrid features and the convolutional neural network. IEEE Access, pp. 1–11.
Oloyede MO, Hancke GP, Myburgh HC, and Onumanyi AJ (2019) A new evaluation function for face image in unconstrained environments using metaheuristic algorithms. Eurasip Journal on Image and Video Processing, pp. 1–18.
Ouyang S, Hospedales T, Song Y-Z, Li X, Loy CC, Wang X (2016) A survey on heterogeneous face recognition: sketch, infra-red, 3d and low-resolution. Image Vis Comput 56:28–48
Patacchiola M, Cangelosi A (2017) Head pose estimation in the wild using convolutional neural networks and adaptive gradient methods. Pattern Recogn 71:132–143
Pereira JF, Barreto RM, Cavalcanti GD, and Tsang R (2011) A robust feature extraction algorithm based on class-modular image principal component analysis for face verification, in Acoustics, Speech and Signal Processing (ICASSP), 2011 IEEE International Conference on, pp. 1469–1472.
Petpairote C, Madarasmi S, Chamnongthai K (2017) A pose and expression face recognition method using transformation based on single face neutral reference. In Proceedings of IEEE Internationl Conference on Global Wireless Summit, October:123–126
Qi Z, Tian Y, Shi Y (2013) Robust twin support vector machine for pattern classification. Pattern Recogn 46:305–316
Qian Y, Deng W, and Hu J (2019) Unsupervised face normalization with extreme pose and expressionin the wild , In proceedings of the IEEE Conference on Computer Vision and Pattern Recognition, pp. 9851–9858.
Rakshit RD, Kisku DR (2020) Face identification via strategic combination of local features. In Proceedings of Computational Intelligencein Pattern Recognition:207–217
Rasti P, Uiboupin T, Escalera S, and Anbarjafari G (2016) Convolutional neural network super resolution for face recognition in surveillance monitoring, in International Conference on Articulated Motion and Deformable Objects, pp. 175–184.
Rehman A, Saba T (2014) Neural networks for document image preprocessing: state of the art. Artif Intell Rev 42:253–273
Revina IM, Emmanuel WS (2018) Face expression recognition using LDN and dominant gradient local ternary pattern descriptors. Journal of King Saud University-Computer and Information Sciences
Sabharwal T, Rashimi G (2019) Human identification after plastic surgery using region based score level fusion of local facial features. Journal of information security and application 48:102373
Sable AH, Talbar SN, Dhirbasi HA (2017) Recognition of plastic surgery faces and the surgery types: An approach with entropy based scale invariant features. Journal of King Saud University-Computer and Information Sciences
Sariyanidi E, Gunes H, Cavallaro A (2015) Automatic analysis of facial affect: a survey of registration, representation, and recognition. IEEE Trans Pattern Anal Mach Intell 37:1113–1133
Savran A, Sankur B (2017) Non-rigid registration based model-free 3D facial expression recognition. Comput Vis Image Underst 162:146–165
Suri S, Sankaran A, Vasta M, Singh R (2018) On matching faces with alterations due to plastic surgery and disguise. In Proceedings of IEEE Conference on Biometrics Theory, Applications and Systems, pp 1–7
Tan S, Xi S, Wenato C, Lei Q, Ling S (2017) Robust face recognition with kernalized locality-sensitive group sparsity representation. IEEE Transaction on image processing 26:4661–4668
Tefas A, Kotropoulos C, and Pitas I (1998) Variants of dynamic link architecture based on mathematical morphology for frontal face authentication, in Proceedings. 1998 IEEE Computer Society Conference on Computer Vision and Pattern Recognition (Cat. No.98CB36231), pp. 814–819.
Tong Z, Aihara K, and Tanaka G (2016) A hybrid pooling method for convolutional neural networks, in International Conference on Neural Information Processing, pp. 454–461.
Tsai H-H, Chang Y-C (2017) Facial expression recognition using a combination of multiple facial features and support vector machine. Soft Comput:1–17
Turk MA and Pentland AP (1991) Face recognition using eigenfaces, in Computer Vision and Pattern Recognition. Proceedings CVPR'91., IEEE Computer Society Conference on, 1991, pp. 586–591.
Wang K, Chen Z, Wu QJ, Liu C (2017) Illumination and pose variable face recognition via adaptively weighted ULBP_MHOG and WSRC. Signal Process Image Commun 58:175–186
Wang J-W, Le NT, Lee J-S, Wang C-C (2017) Illumination compensation for face recognition using adaptive singular value decomposition in the wavelet domain. Inf Sci
Xanthopoulos P, Pardalos PM, and Trafalis TB (2013) Linear discriminant analysis, in Robust data mining, ed: Springer, pp. 27–33.
Xu C, Liu Q, Ye M (2017) Age invariant face recognition and retrieval by coupled auto-encoder networks. Neurocomputing 222:62–71
Yang J, Luo L, Qian J, Tai Y, Zhang F, Xu Y (2017) Nuclear norm based matrix regression with applications to face recognition with occlusion and illumination changes. IEEE Trans Pattern Anal Mach Intell 39:156–171
Yang J, Ren P, Zhang D, Chen D, Wen F, Li H, and Hua G (2017) Neural aggregation network for video face recognition. In Proceedings of the IEEE conference on computer vision and pattern recognition, pp. 4362–4371.
Yu Y-F, Dai D-Q, Ren C-X, Huang K-K (2017) Discriminative multi-layer illumination-robust feature extraction for face recognition. Pattern Recogn 67:201–212
Zafeiriou S, Zhang C, Zhang Z (2015) A survey on face detection in the wild: past, present and future. Comput Vis Image Underst 138:1–24
Zeng S, Jianping G, Deng L (2017) An antinoise sparse representation method for robust face recognition via joint l 1 and l 2 regularization. Expert System with Application 82:1–9
Zhang P, Ben X, Jiang W, Yan R, Zhang Y (2015) Coupled marginal discriminant mappings for low-resolution face recognition. Optik-International Journal for Light and Electron Optics 126:4352–4357
Zhang Y, Lu Y, Wu H, Wen C, and Ge C (2016) Face occlusion detection using cascaded convolutional neural network, in Chinese Conference on Biometric Recognition, pp. 720–727.
Zhang D-x, An P, Zhang H-x (2018) Application of robust face recognition in video surveillance systems. Optoelectron Lett 14:152–155
Zhang MM, Shang K, Wu H (2019) Learning deep discriminative face features by customized weighted constraint. Nuerocomputing 332:71–79
Zhao S (2018) Pixel-level occlusion detection based on sparse representation for face recognition. Optik 168:920–930
Zhao K, Jingyl X, and Cheng MM (2019) Regukarface: Deep face recognition via exclusive regularization”, In Proceedings of the IEEE Conference on Computer Vision and Pattern Recognition, pp. 1136–1144.
Zhou H, Lam K-M (2018) Age-invariant face recognition based on identity inference from appearance age. Pattern Recogn 76:191–202
Zhou Z, Wagner A, Mobahi H, Wright J, and Ma Y (2009) Face recognition with contiguous occlusion using markov random fields, in Computer Vision, 2009 IEEE 12th International Conference on, pp. 1050–1057.
Zhou L-F, Du Y-W, Li W-S, Mi J-X, Luan X (2018) Pose-robust face recognition with Huffman-LBP enhanced by divide-and-rule strategy. Pattern Recogn
Zhou Q, Zhang C, Yu W, Fan Y, Zhu H, Xiaofu W (2018) Face recognition via fast dense correspondence. Multimed Tools Appl 77:10501–10519
Zhuang L, Chan T-H, Yang AY, Sastry SS, Ma Y (2015) Sparse illumination learning and transfer for single-sample face recognition with image corruption and misalignment. Int J Comput Vis 114:272–287
Download references
Acknowledgments
This work was supported by the Council for Scientific and Industrial Research (CSIR), South Africa.
[ICT: Meraka].
Author information
Authors and affiliations.
Department of Information and Communication Science, University of Ilorin, Ilorin, Nigeria
Muhtahir O. Oloyede
Department of Electrical, Electronic and Computer Engineering, University of Pretoria, Pretoria, South Africa
Muhtahir O. Oloyede, Gerhard P. Hancke & Hermanus C. Myburgh
You can also search for this author in PubMed Google Scholar
Corresponding author
Correspondence to Muhtahir O. Oloyede .
Additional information
Publisher’s note.
Springer Nature remains neutral with regard to jurisdictional claims in published maps and institutional affiliations.
Rights and permissions
Reprints and permissions
About this article
Oloyede, M.O., Hancke, G.P. & Myburgh, H.C. A review on face recognition systems: recent approaches and challenges. Multimed Tools Appl 79 , 27891–27922 (2020). https://doi.org/10.1007/s11042-020-09261-2
Download citation
Received : 08 August 2019
Revised : 16 April 2020
Accepted : 24 June 2020
Published : 30 July 2020
Issue Date : October 2020
DOI : https://doi.org/10.1007/s11042-020-09261-2
Share this article
Anyone you share the following link with will be able to read this content:
Sorry, a shareable link is not currently available for this article.
Provided by the Springer Nature SharedIt content-sharing initiative
- Face recognition
- Uncontrolled environment
- Face dataset
- Find a journal
- Publish with us
- Track your research

An official website of the United States government
The .gov means it’s official. Federal government websites often end in .gov or .mil. Before sharing sensitive information, make sure you’re on a federal government site.
The site is secure. The https:// ensures that you are connecting to the official website and that any information you provide is encrypted and transmitted securely.
- Publications
- Account settings
Preview improvements coming to the PMC website in October 2024. Learn More or Try it out now .
- Advanced Search
- Journal List
- Comput Intell Neurosci
- v.2021; 2021

Research on Face Image Digital Processing and Recognition Based on Data Dimensionality Reduction Algorithm
Institute of Design, Chongqing Industry Polytechnic College, Chongqing 401120, China
Associated Data
The labeled dataset used to support the findings of this study is available from the corresponding author upon request.
Because face recognition is greatly affected by external environmental factors and the partial lack of face information challenges the robustness of face recognition algorithm, while the existing methods have poor robustness and low accuracy in face image recognition, this paper proposes a face image digital processing and recognition based on data dimensionality reduction algorithm. Based on the analysis of the existing data dimensionality reduction and face recognition methods, according to the face image input, feature composition, and external environmental factors, the face recognition and processing technology flow is given, and the face feature extraction method is proposed based on nonparametric subspace analysis (NSA). Finally, different methods are used to carry out comparative experiments in different face databases. The results show that the method proposed in this paper has a higher correct recognition rate than the existing methods and has an obvious effect on the XM2VTS face database. This method not only improves the shortcomings of existing methods in dealing with complex face images but also provides a certain reference for face image feature extraction and recognition in complex environment.
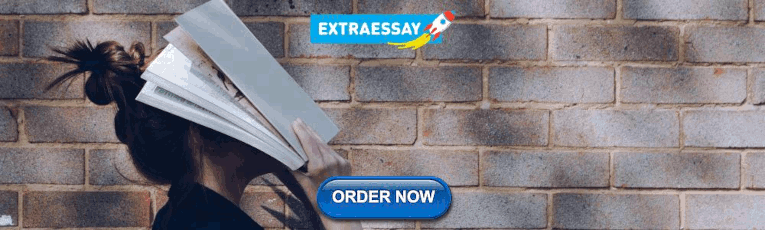
1. Introduction
As a common biometric recognition method, face recognition (FR) has been well applied in the fields of mobile phone unlocking, secure payment, intelligent door lock, and so on. Face recognition uses facial features to identify individuals. However, in these application fields, the information in the face image is lost due to the occlusion problem of the collected face image. Therefore, the lack of some face information will make the effect of face recognition worse. Face recognition is widely used in face image recognition and verification and has attracted extensive attention in recent years. Because FR algorithms are accurate and harmless, they are used in applications such as access control, smart card, information security, and law enforcement [ 1 – 3 ]. People mostly use shallow or deep face recognition learning. The traditional algorithm based on feature extraction uses local or nonlocal facial features to train the model and uses the model to recognize the invisible face image [ 4 , 5 ]. In addition, in face recognition, the deep learning method is to use the whole face image database to learn and predict the invisible face image without generating specific face features [ 6 ]. Most of the current FR approaches assume that multiple images of the same face are available for training their algorithms [ 7 , 8 ]. In fact, the number of available images of the same face is limited, and the more images used will increase the complexity and processing time of the algorithm. However, when only one sample image can be used for system training, it is difficult to recognize others' faces under different lighting conditions. Therefore, people study FR through local characteristics and achieve more results. Gao proposed a face description and similarity measurement technology for Visual Similarity Retrieval (VSR) in a single model face database, which does not use local operations on isolated points but uses Direction Corner Point (DCP) matching, in which the direction information of connectivity with adjacent points is displayed for similar points [ 9 ]. In these feature-based methods, most facial features are usually eyes, nose, and mouth. Because the position and number of facial feature points are not fixed, the number and position of feature vectors can be used to represent different facial features of different faces. The feature points obtained by this method are usually around the main facial features and the individual's special facial features.
In face recognition, the algorithm based on Sparse Representation Classification (SRC) has also been deeply studied [ 10 ]. The sparse representation method mainly uses the library image to construct the dictionary and uses the sparse linear combination reconstruction of the dictionary to query the image and complete the matching. However, sparse representation has some shortcomings. For example, sparse representation is applied to each input signal independently regardless of whether the image comes from the same category or not, so some structural information in the image cannot be used. Therefore, some people have also proposed a new facial image representation method, that is, the sequence of forehead, eyes, nose, mouth, and chin, which is similar to sparse representation, that is, based on the similarity between the features extracted from the gallery image area and the features extracted from the query image [ 11 ]. In face recognition methods, people use different methods to extract audio fingerprints, such as wavelet transform, Fourier transform, or entropy based for feature matching [ 12 – 15 ]. These algorithms have little interference to environmental noise and volume. The recognition of these local features is a method to create a database, which can not only reduce the information about signals but also retain unique elements, so it is easier to search and find matches in the database.
At the same time, multiple learning has developed rapidly in recent years [ 16 ]. Usually, the observation data are located on a low-dimensional submanifold embedded in a high-dimensional environment space [ 17 ]. Then, in order to further explore this structure, many manifold learning methods are proposed [ 18 – 21 ]. These algorithms are based on the local invariance principle, and the geometric and topological properties of submanifolds are estimated from the random points sampled from unknown submanifolds. For example, if two data points are very close in the inner manifold, it can be inferred that their representation in any space is also very close [ 22 , 23 ]. Although designing reliable FR systems requires a lot of work, these systems still face some difficulties, such as when the face looks different under different lighting conditions or facial expressions.
In recent years, in the field of face image recognition, traditional methods based on subspace learning, such as principal component analysis (PCA), linear discriminant analysis (LDA), and its extended versions, are widely used for low-dimensional feature extraction in face recognition [ 24 ]. Principal component analysis is an unsupervised method, which aims to obtain a subspace with the largest prediction global variance and maintain the diversity of data [ 25 ]. The typical feature face method developed from PCA applies K-L transform to face recognition [ 26 ]. The main feature vector of face image usually represents the shape of face, so this method can not only reduce the spatial dimension but also represent the features of face. However, LDA is a monitoring method. This method mainly uses the label information of face image to construct the interclass and intraclass dispersion matrix and makes the face data complete face image recognition in a suitable low-dimensional subspace. The classic fish face based on LDA is also the mainstream face recognition method, which can achieve better classification in face recognition [ 27 ].
Recently, a method based on low-rank representation (LRR) has attracted much attention because of its robustness and good performance [ 28 , 29 ]. This method considers that face data points are located in low-dimensional subspace, and the representation matrix of these data points is low rank. However, the application of LRR and its subsequences is limited because they cannot obtain low-dimensional subspace. The main problem faced by the method based on low-rank representation is how to solve the rank minimization problem. The general solution is to relax it to kernel specification minimization (NNM). Although the kernel norm is a typical convex approximation of the rank minimization problem, the kernel norm minimization makes the solution quite different from the actual value [ 30 ]. In view of these situations, this paper presents a face image feature extraction and recognition method based on data dimensionality reduction algorithm. The nonparametric subspace analysis method is used to block the recognition image matrix and preextract the features. After obtaining the low-dimensional new pattern instead of the original image, the face image is digitally processed and recognized. Finally, this method is verified on the AR face database, ORL face database, and XM2VTS face database, and its recognition performance is better than other typical face recognition methods.
2. Related Works
2.1. data dimensionality reduction.
Effective data dimensionality reduction technology can explore the internal structure and relationship of the original data. It can not only eliminate the redundancy between data and simplify data but also greatly improve the computational efficiency, the understandability of data, and the accuracy of the learning algorithm. The adoption of effective dimensionality reduction methods can first compress the data and reduce the amount of storage (eliminating data redundancy will be more effective, large data information is compressed to 50% of the original size, and some can even be compressed to about 20%, greatly saving storage resources). The simplification of data significantly improves the efficiency of calculation, and on this basis, the comprehensibility of data (Intelligence) can be expressed more conveniently. Secondly, the dimension reduction technology is used to make the extracted data features more accurate. It is easy to classify and project to low-dimensional visual space. This step enables us to see the distribution of data in a more intuitive way and internal structure, that is, data visualization.
Data dimensionality reduction can be divided into two types: feature selection and feature extraction [ 25 ]. The former works by finding a subset that can express the original data, while the latter mines new low-dimensional features of the original data. However, these features are not limited to the subset of the original features. Generally speaking, the dimensionality reduction methods used in face recognition are feature extraction. From the perspective of the processed data attributes, data dimensionality reduction can be divided into linear methods and nonlinear methods, as shown in Figure 1 .

Dimensionality reduction method based on different classification of data attributes to be processed.
From the perspective of category information of data to be processed, dimensionality reduction methods can be divided into unsupervised methods, semisupervised methods, and supervised methods. Semisupervised method is the latest research achievement in recent years. It is between nonsupervised and semisupervised, as shown in Figure 2 .

Data dimensionality reduction method classified according to whether the data information to be processed is used.
2.2. Face Recognition
Since the French scientist Francis Galton opened the prelude to human exploration and research on face recognition, after nearly half a century of development, the research field of face recognition has formed two mainstream directions: method based on feature analysis and holistic approach. The method based on feature analysis combines the relative ratio of face reference points with other shape parameters that can be used to describe face features to form the recognition feature vector. The advantage of this method is that it not only retains the topological relationship between face components but also retains a large amount of information about the components themselves. The improved integral projection method is used to extract the 35-dimensional feature vector represented by Euclidean distance, which is then used for pattern classification, including not only the normalized distance and ratio between points but also the two-dimensional topology composed of the corners of the eyes, nose, and mouth. In this way, the overall attributes of the pattern are fully considered. Many experts and scholars have also proposed various improved methods based on this method, such as Fisher face method, elastic graph matching method, RBF network recognition method, hidden Markov model method, and hidden Markov model method.
After years of continuous exploration and research, face recognition has made some achievements in the following directions: face recognition method based on relative difference space, face recognition method based on local, face recognition method based on global, and face recognition method based on subspace.
3.1. Face Recognition and Its Processing
Because faces contain the same features, such as eyes, mouth, and nose, how to effectively recognize different faces is one of the main problems studied by scholars in this field. These features in the face have subtle differences in position, shape, and color. Research shows that the difference between face detection and face recognition is that face detection generalizes face and distinguishes it from nonface. Face recognition must distinguish different faces. Therefore, if face detection is a basic problem, face recognition is a deep-seated problem based on face monitoring. In the design and development of a face recognition system, the problems to be solved include the speed and accuracy of the system from detection to recognition. The system is scalable; that is, it can add more recognition objects. A typical face recognition system is mainly composed of the following five modules, as shown in Figure 3 .

Schematic diagram of face recognition process.
Face image information can be obtained through a camera or other digital input devices. The face image information in this paper comes from the commonly used face database. Face image digitization and preprocessing module is an important part of face detection and recognition. In the process of face image acquisition, many factors will lead to the acquisition of images that are not standard enough, for example, the light intensity and brightness of the acquisition environment, the pixel resolution of the acquisition equipment; sometimes, there are phenomena such as insufficient contrast and noise interference in the acquisition. In order to ensure the consistency of faces in the image (face position, face size, image quality, etc.), it is generally necessary to carry out a series of preprocessing operations on the face image. This preprocessing mainly includes the rotation operation, cutting operation, scaling operation, image enhancement operation, geometric normalization processing, and gray normalization processing. In order to obtain the face image in the correct position, it is necessary to straighten the face in the image, and in order to improve the quality of the image, the image is enhanced. By adopting the enhanced image operation, it can not only produce a clearer image visually but also be more conducive to the processing and recognition of the computer, reduce the impact of light on the image, and strengthen the light. In addition, the normalization operation of the image is to obtain a standard face image with the same size and the same gray value.
In terms of feature extraction, firstly, the transformation of the original data is realized through the mapping from face image space to feature space so as to obtain the features that can best reflect the essence of classification. This step provides a reference basis for subsequent work (such as better realization of classification purpose). In the process of feature extraction, we should select appropriate features according to different recognition methods. In a complete face recognition system, feature extraction is the most critical and core part, and it is also one of the greatest difficulties. The effectiveness and stability of the extracted features directly determine the performance and success of the recognition effect.
The design of the classifier is mainly to extract the knowledge of training sequence in unsupervised or semisupervised and supervised ways and obtain the distribution law and class distribution characteristics of the training sequence, so as to provide a basis for subsequent classification decisions. Finally, the pattern classification is used to complete the tasks of face discrimination and classification, and the final discrimination results are obtained.
3.2. Feature Extraction Method
In face recognition and processing, the objects to be processed are generally high-dimensional facial makeup images. Due to the sparse distribution of these images in high-dimensional space, it is difficult to classify the images. Therefore, feature selection or extraction methods are often used in face recognition, which can not only improve the execution efficiency of the recognition algorithm but also achieve the purpose of space compression and denoising. The important information of the collected face samples is generally only concentrated in some attributes. The low-dimensional data can be obtained by transforming the high-dimensional data samples so that the most effective features can be extracted. Nonparametric subspace analysis (NSA) can extract these important features from face images [ 31 ].
Suppose that there is a training sample set containing n ( n =∑ i =1 m n i ) face images, each sample is represented as Y 1 , Y 2 ,…, Y n , they belong to class m ( m 1 , m 2 ,…, m k ), and each sample image is a h dimensional column vector. The number of samples of class im i is expressed as n i .
The total mean vector ν of all samples and the sample mean vector ν i within m i are expressed, respectively, as follows:
where Y k ∈ m i .
The intraclass scatter matrix of training samples is similar to the interclass scatter matrix T e in linear discriminant analysis, expressed as follows:
The interclass dispersion matrix T f n of the training sample is expressed as follows:
In equations ( 3 ) and ( 4 ), Y i ( j ) means the j -th image of class i ( i =1,2,…, m ).
In equation ( 4 ), the weight function ω ( i , j , k ) is defined as follows:
In equation ( 5 ), ϕ is a parameter that varies from zero to infinity. Its function is to control the change speed of distance ratio in weight. l ( v 1, v 2) is the Euclidean distance of vectors v 1 and v 2.
K nearest neighbor mean k i ( Y i ( j ) ) is defined as follows:
In equation ( 6 ), nn q ( Y i ( j ) , i ) means the nearest neighbor distance from the q -th face to the face vector Y i ( j ) in class i .
Three conclusions can be obtained from the selection of various parameters in comprehensive equations ( 3 ) to ( 6 ).
First, if the values of all ω ( i , j , k ) functions are set to integer 1 and h = n i , that is, h is equal to the number of samples of class i , the K nearest neighbor mean becomes ν j (i.e., the sample mean of class j ).
The interclass scatter matrix of NSA is constructed from the class center, not all training samples. In addition, according to the definition of K nearest neighbor mean k i ( Y i ( j ) ), the NSA method can make better use of different types of boundary information.
Substitute equations ( 3 ) and ( 4 ) into the best criterion function, which is expressed as follows:
In equation ( 7 ), w is the optimal identification projection space. w is transformed into a generalized eigenvalue problem.
When T w is reversible, the characteristic equation is solved, which is as follows:
The eigenvector corresponding to the first h maximum eigenvalues of equation ( 8 ) can be used as the optimal discrimination vector.
By analyzing equations ( 1 ) to ( 8 ), if the NSA algorithm wants to obtain a high recognition rate, the value of the nearest neighbor K is particularly important.
It is assumed that there are m categories, which are recorded as a 1 , a 2 ,…, a m . The number of class i samples is k i , B i ( j ) is the j th m of class i image matrix y × zi =1,2, ⋯, m , j =1,2, ⋯, k i , and the total number of samples is Y sum =∑ i =1 m k i . The idea of modular 2DPCA is to divide the matrix B i ( j ) into o × p block matrix, as follows:
where each image matrix ( B i ( j ) ) e , f ( i =1,2,…, m ; j =1,2,…, k i ; e =1,2,…, o ; f =1,2,…, p ) is matrix y x × z x ( y x × o = y , z x × p = z ).
The overall dispersion matrix of training samples is defined as follows:
where 1/ n ∑ i =1 m ∑ j =1 n i ∑ e =1 o ∑ f =1 p ( B i ( j ) ) e , f is the mean matrix of all training sample submatrixes and the number of all subimages n =Mop.
The criterion function is defined as follows:
Among them, the normalized orthogonal eigenvectors corresponding to the first n maximum eigenvalues of S n , b 1 , b 2 ,…, b n are the optimal projection vector group, and D (optimal projection matrix) is expressed as D =[ b 1 , b 2 ,…, b n ]. The characteristic matrix of the training sample projected on D is expressed as follows:
In the process of modular 2DPCA, when the image blocking mode is determined, the number of projection axes (i.e., n ) determines the dimension of the characteristic matrix (i.e., A i ( j ) ).
If the value of n is too small, the characteristic matrix will lose some favorable discrimination information for later classification. However, if the value of n is too large, there will be a lot of redundant information in the characteristic matrix, which will interfere with the dimensionality reduction.
3.3. Improved Algorithm
Based on the above algorithm, we could improve the face recognition procession. Let ( y i ( j ) ) e , f = V ( A i ( j ) ) e , f ; then ( y i ( j ) ) e , f ∈ E i k j k , e =1,2,…, o , f =1,2,…, p . The mean value of the subimage matrix of the j -th image sample of class i is calculated as follows:
Note that nn k ( y i ( j ) , r ) is the set of K nearest neighbors of classes r to y i ( j ) and n r ( y i ( j ) )=1/ k ∑ n =1 k nn k ( y i ( j ) , r ) is the mean of K nearest neighbors of classes r to y i ( j ) .
Define the interclass scatter matrix as follows:
In equation ( 14 ), the weight ω ( i , j , r ) is calculated as follows:
In equation ( 15 ), β is a positive parameter in h β ( y i ( j ) , nn k ( y i ( j ) , r )), which can control the weight change about the distance ratio velocity, and its distance is from y i ( j ) to set nn k ( y i ( j ) , r ).
Within class scatter matrix T w is calculated as follows:
In equation ( 16 ), η i =1/ n i ∑ j =1 n i y i ( j ) is the mean vector of class i samples. Therefore, the corresponding criterion function is expressed as follows:
The first T f n ν = T w ην maximum eigenvalues of the characteristic equation h and their corresponding eigenvectors can be used as the optimal discriminant vector.
4. Experiment and Analysis
We have carried out relevant experiments on AR database, ORL database, and XM2VTS database to verify that the valuable information of the image is not lost when using this method to extract the low-dimensional features of the image. At the same time, the experiment also compresses this method with other common dimensionality reduction (DR) methods, including LRE [ 32 ], LSDP [ 25 ], TNNL [ 33 ], LPRR [ 34 ], and Truncated-LRE [ 35 ].
4.1. Evaluation Method
The recognition rate and standard deviation are used as evaluation indexes [ 33 ]. Firstly, we use the projection matrix obtained by each method to reduce the dimension of the test data set so that the dimension of the test data set changes from 1 to 70 with an interval of 5. Therefore, the recognition rate (rr) of each method in different dimensions can be calculated by the following formula:
where pl ∈ R 1× n t is a set of data prediction labels obtained on the test data set using the DR method, al ∈ R 1× n t is the actual label of a set of data on the test dataset, n t is the number of samples in the test dataset, and sum() is a function that calculates the number of equal elements at the same position in pl and al .
In order to avoid randomness, the experiment was repeated five times, and the average recognition rate was calculated. In addition, the stability of the model is evaluated by calculating the standard deviation (SD). The standard deviation can be calculated according to the following formula:
where n represents the number of repetitions, x i represents the recognition rate of each experiment, and x ¯ is the average recognition rate of all experiments.
4.2. Experimental Design
In order to evaluate the performance of this method, we verify the effectiveness of this method by evaluating the recognition rate and standard deviation of various methods in different subspaces.
During the experiment, we randomly selected l images of a person from each face database for training, and the other images were tested. Moreover, the value of S is adjusted according to the number of people in different databases. S = 3, 4, and 5, respectively, represent the AR database, S = 4, 5, and 6, respectively, represent the ORL database, and S = 5, 6, and 7, respectively, represent the XM2VTS database. Details are shown in Table 1 .
Number of training and testing samples for different databases.
4.3. Sample Database Description
AR database is a large face database established by a computer vision center in Barcelona, Spain, in the 1990s. There are 120 volunteers in this library. Each volunteer has taken 26 images, 3120 in total, of which the proportion of men and women is half, and the age range is between 18 and 45 years. The size of the image is 100 × 80. Each image acquisition environment is different, the light intensity is different, the facial expression is different (open eyes, close eyes, open mouth, shut mouth, laugh, do not laugh, pain, happiness, etc.), and the wearing accessories are also different (scarf, earrings, sunglasses, eyes, etc.). In the experiment, the AR face database is divided into two parts: extracting the training set of human face subspace and a test set to test the performance of the algorithm. The first 10 sheets of each group were used as the training set, and the last 5 sheets were used as the test set. The following is a set of images taken from AR data, as shown in Figure 4 .

Face image in AR database.
ORL face database is often selected to test and evaluate the performance of the proposed face recognition algorithm in the world. The shooting time of this database is from 1992 to 1994. There are 400 face images in the ORL face database. All images in this database are 92 × 112 in size, with a gray level of 256. There are 40 volunteers in total. Each volunteer takes 10 images. All volunteers are students and teaching staff. The oldest of the volunteers is 81 years old, the youngest is 18 years old, and most of them are between 20 and 35 years old. There is a large difference in the number of men and women in the database, which is 9 : 1; that is, there are 36 male and 4 female volunteers. The time period of face image acquisition is different, and various details are also different, such as light intensity (strong light and weak light), facial ornaments (with or without glasses or sunglasses, beard, and hair change), and facial expression (smile, serious, open, close, and squint). In the experiment, the ORL face database is divided into two parts: extract the training set of human face subspace and use the first four images of each person as the training set. The last four pictures of each person were used as the test set. The following is a group of photos taken from the ORL face database, as shown in Figure 5 .

Face image in ORL database.
XM2VTS face database is also one of the commonly used databases in face recognition experiments. There are in total 295 × 8 images or video and audio clips in the library, of which the gray image resolution is 55 × 51; the differences include whether to wear glasses, makeup, expression, and posture. In this experiment, the first five sheets of each group were used as the training set, and the last five sheets were used as the test set. The following is a group of photos taken from the XM2VTS face database, as shown in Figure 6 .

Face image in XM2VTS database.
4.4. Experimental Results
In order to compare the experimental results of each method on the AR face database and objectively reflect the correct recognition rate of images obtained by different methods, the original image matrix is cut into image submatrix sizes 6 × 4 and 12 × 4. After the two blocks are divided, the experimental results of different methods are compared by the recognition rate. The comparison results are shown in Table 2 .
Experimental comparison of different methods on AR face database.
Compared with LRE, LSDP, TNNL, LPRR, and Truncated-LRE, the new method is better. Specifically, in the AR database, the results using LRE, LSDP, TNNL, LPRR, and Truncated-LRE methods are 80.46%, 78.69%, 81.07%, 79.63%, and 80.26%, respectively, and the highest recognition rate of the method proposed in this paper is 82.83%.
In order to compare the experimental results of each method on the ORL face database and objectively reflect the correct recognition rate of images obtained by different methods, the original image matrix is cut into image submatrix sizes 8 × 6 and 12 × 6. After the two blocks are divided, the experimental results of different methods are compared by the recognition rate. The comparison results are shown in Table 3 .
Experimental comparison of different methods on the ORL face database.
Compared with LRE, LSDP, TNNL, LPRR, and Truncated-LRE, the new method is better. Specifically, in the ORL database, the results using LRE, LSDP, TNNL, LPRR, and Truncated-LRE methods are 91.46%, 90.69%, 90.07%, 91.63%, and 90.26%, respectively, and the highest recognition rate of the method proposed in this paper is 93.53%.
In order to compare the experimental results of each method on the XM2VTS face database and objectively reflect the correct recognition rate of images obtained by different methods, the original image matrix is cut into image submatrix sizes 10 × 4 and 16 × 4. After the two blocks are divided, the experimental results of different methods are compared by the recognition rate. The comparison results are shown in Table 4 .
Experimental comparison of different methods on XM2VTS face database.
Compared with LRE, LSDP, TNNL, LPRR, and Truncated-LRE, the new method is better. Specifically, in the XM2VTS database, the results using LRE, LSDP, TNNL, LPRR, and Truncated-LRE methods are 92.43%, 92.59%, 92.17%, 91.35%, and 92.68%, respectively, and the highest recognition rate of the method proposed in this paper is 94.75%.
By comparing the experimental results of this method in different face databases, it can be concluded that this method has an obvious effect on the XM2VTS face database.
5. Discussion
According to the experimental statistical results from Tables Tables2 2 2 – 4 , by comparing the correct recognition rates obtained by different methods, it can be seen that this method is better than LRE, LSDP, TNNL, LPRR, and Truncated-LRE methods, but the effect is obvious on XM2VTS face database. In addition, if different methods are used on the same face database, the block method determines the value of the correct recognition rate. When the face databases are the same and the blocking methods are the same, the number of training samples and test samples has a significant impact on the correct recognition rate, but the more training samples, the better the recognition effect.
From the above results, compared with other methods, the methods proposed, LRE, TNNL, and Truncated-LRE, can effectively process the original image and damaged image. This undoubtedly proves the superiority of low-rank regularization in learning the optimal human face subspace. This is mainly because the low-rank representation can accurately capture the subspace structure of the original data. In addition, compared with LRE, the methods proposed, TNNL and Truncated-LRE, have better performance in processing damaged images, which verifies that the method proposed can reduce the negative impact of noise. This is mainly because the TNNL contained in the method proposed and Truncated-LRE replaces the rank function better than the kernel norm in LRE. However, the method proposed has more improvements than TNNL. The key reason is that the method proposed is supervised, and TNNL is not. By adding linear discriminant, the method proposed makes full use of label information. Therefore, the low-dimensional features learned by the method proposed are distinguishable. In conclusion, we can find that the method proposed is the best of these methods. In addition, it also shows the effectiveness of integrating label information and low-rank minimization into the model.
6. Conclusion
In the research process of face recognition, feature extraction is not only a key problem but also one of the main difficulties in the development of face recognition. How to effectively extract features directly affects the recognition rate of face image. Aiming at the image processing problem in the process of face recognition, this paper proposes a face image feature extraction and recognition method based on data dimensionality reduction algorithm. The nonparametric subspace analysis method is used to block the recognition image matrix and preextract the features. After obtaining the low-dimensional pattern instead of the original image, the face image is digitally processed and recognized. Finally, through experiments, the method is verified on the AR face database, ORL face database, and XM2VTS face database. According to different methods, its recognition performance is better than other typical face recognition methods.
For two-dimensional face images, the difficulties of face recognition technology still focus on the problems such as illumination intensity and brightness, face expression, and face changes caused by age changes. In recent years, experts and scholars have proposed a face recognition method based on a three-dimensional face model based on these urgent problems. Face recognition method based on 3D face model will be the focus of our research in the next few years [ 33 , 36 – 38 ].
Acknowledgments
This work was supported in part by the Humanities and Social Sciences General Planning Project of Chongqing Education Commission “Research on the Countermeasures of the Inheritance and Innovation Development of the Digital Protection of Bayo Witch Culture” (no. 20SKGH320), the Chongqing social science planning project “Research on Sustainable Development and Application of Digital Resources of Basho Witch Culture” (no. 2020YBYS192), and the key projects of Chongqing Industry Polytechnic College (no. GZY2020-KZ-03).
Data Availability
Conflicts of interest.
The author declares no conflicts of interest.
Announcing new Microsoft AI Hub in London
Apr 7, 2024 | Mustafa Suleyman, EVP and CEO of Microsoft AI
- Share on Facebook (opens new window)
- Share on Twitter (opens new window)
- Share on LinkedIn (opens new window)
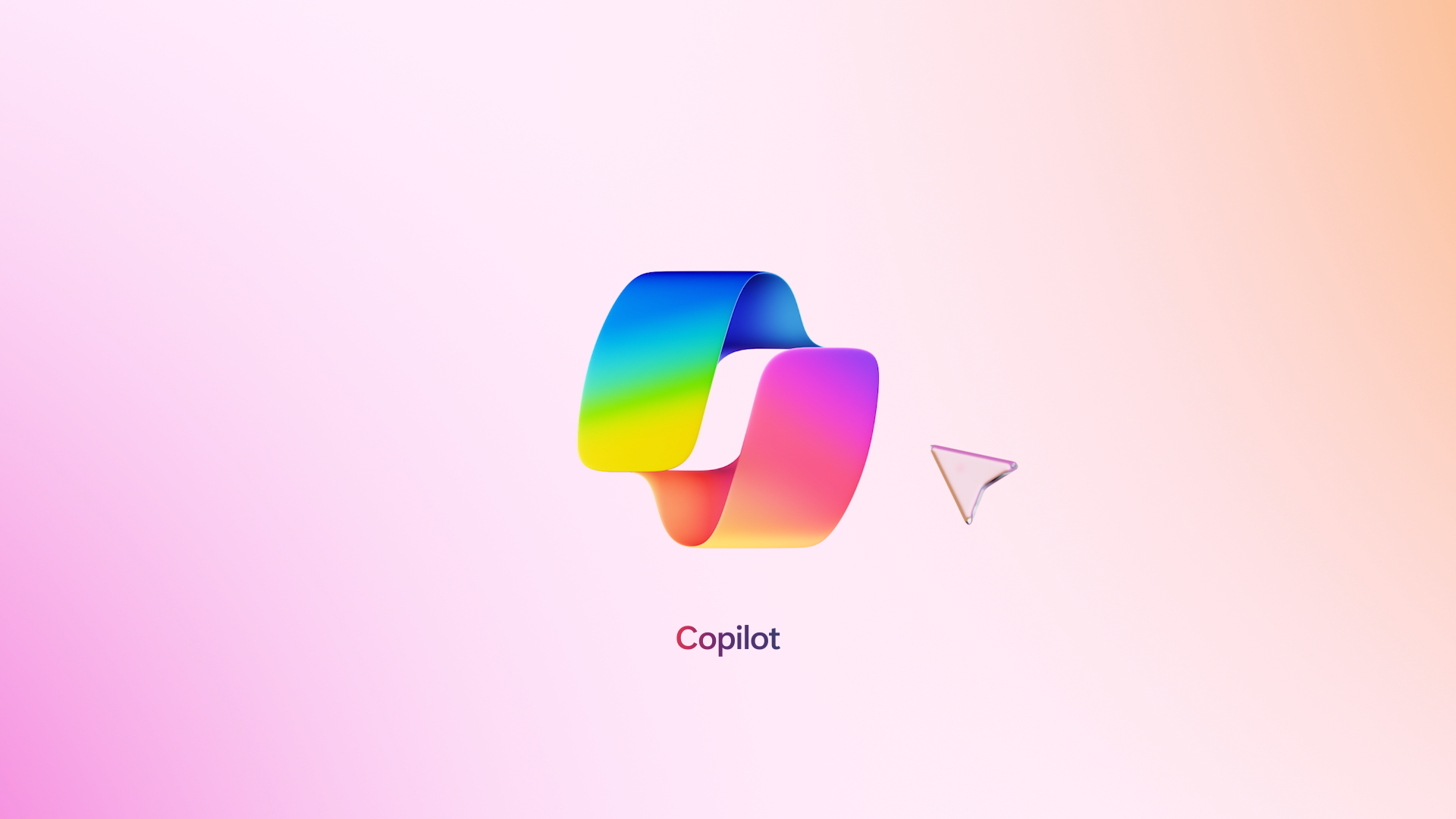
Microsoft recently announced the creation of Microsoft AI , a newly formed organization to help advance our consumer AI products and research, including Copilot. Building on that news, I’m thrilled to share that Microsoft AI is opening a new AI hub in the heart of London. Microsoft AI London will drive pioneering work to advance state-of-the-art language models and their supporting infrastructure, and to create world-class tooling for foundation models, collaborating closely with our AI teams across Microsoft and with our partners, including OpenAI.
The new AI hub will be led by Jordan Hoffmann, an exceptional AI scientist and engineer. Prior to joining Microsoft AI, Hoffmann distinguished himself as an AI pioneer at Inflection and DeepMind, based in London. Hoffmann will be joined by a talented group of Microsoft AI team members based in our London Paddington office.
There is an enormous pool of AI talent and expertise in the U.K., and Microsoft AI plans to make a significant, long-term investment in the region as we begin hiring the best AI scientists and engineers into this new AI hub.
In the coming weeks and months, we will be posting job openings and actively hiring exceptional individuals who want to work on the most interesting and challenging AI questions of our time. We’re looking for new team members who are driven by impact at scale, and who are passionate innovators eager to contribute to a team culture where continuous learning is the norm.
This is great news for Microsoft AI and for the U.K. As a British citizen, born and raised in London, I’m proud to have co-founded and built a cutting-edge AI business here. I’m deeply aware of the extraordinary talent pool and AI ecosystem in the U.K., and I’m excited to make this commitment to the U.K. on behalf of Microsoft AI. I know – through my close work with thought leaders in the U.K. government, business community and academia – that the country is committed to advancing AI responsibly and with a safety-first commitment to drive investment, innovation and economic growth. Our decision to open this hub in the U.K. reflects this ambition.
The Microsoft AI London hub adds to Microsoft’s existing presence in the U.K., including the Microsoft Research Cambridge lab, home to some of the foremost researchers in the areas of AI, cloud and productivity. At the same time, it builds off Microsoft’s recently announced £2.5 billion investment to upskill the U.K. workforce for the AI era and to build the infrastructure to power the AI economy, including our commitment to bring 20,000 of the most advanced GPUs to the country by 2026.
Stay tuned for more updates as we continue to push the boundaries of what’s possible with AI and extend the benefits to every person and organization across the U.K.
Tags: AI , Microsoft AI
- Check us out on RSS
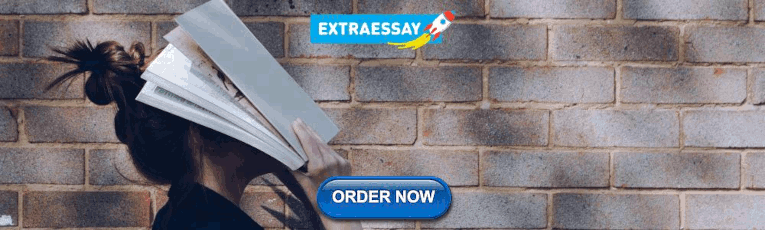
COMMENTS
Abstract and Figures. The task of face recognition has been actively researched in recent years. This paper provides an up-to-date review of major human face recognition research. We first present ...
This Thesis is brought to you for free and open access by the Master's Theses and Graduate Research at SJSU ScholarWorks. It has been accepted for inclusion in Master's Theses by an authorized administrator of SJSU ... The Designated Thesis Committee Approves the Thesis Titled FACE RECOGNITION: AN ENGINEERING APPROACH . by . Farshad Ghahramani ...
facial recognition is known as the Karhunen-Loeve method. It is the most thoroughly studied. method for face recognition, with its main usability being a reduction in the dimensionality of the image. This method was first applied for face recognition and then subsequently used for facial. reconstruction.
Based on the remarkably augmented face dataset, the face recognition can be effectively implemented via an ingenious CNN. Several experiments are carried out to verify the effectiveness of the augmented dataset, and the superiority of the new approach can also be confirmed in comparison with some of the frequently used face recognition methods.
face recognition relate to occlusion, illumination and pose invariance, which causes a notable decline in ... Current research in both face detection and recognition algorithms is focused on Deep Convolutional Neural Networks (DCNN), which have demonstrated impressive accuracy on highly challenging databases such as the WIDER FACE dataset [5 ...
Face recognition is one of the most active research fields of computer vision and pattern recognition, with many practical and commercial applications including identification, access control, forensics, and human-computer interactions. However, identifying a face in a crowd raises serious questions about individual freedoms and poses ethical issues. Significant methods, algorithms, approaches ...
rotation. Therefore, face recognition based on deep learning can greatly improve the recognition speed and compatible external interference. In this thesis, we use convolutional neural networks (ConvNets) for face recognition, the neural networks have the merits of end-to-end, sparse connection and weight sharing.
Face Recognition (FR) is a biometric application capable of uniquely identifying and verifying a person by analysing and comparing patterns based on the individual's face. ... He has published number of research papers and books in field of image processing, computer graphics and computer algorithms. He has served as committee member and ...
Fig. 2: Face recognition building blocks. face recognition research, as CNNs are being used to solve many other computer vision tasks, such as object detection and recognition, segmentation, optical character recognition, facial expression analysis, age estimation, etc. Face recognition systems are usually composed of the following building blocks:
Face recognition is an efficient technique and one of the most preferred biometric modalities for the identification and verification of individuals as compared to voice, fingerprint, iris, retina eye scan, gait, ear and hand geometry. This has over the years necessitated researchers in both the academia and industry to come up with several face recognition techniques making it one of the most ...
FaceNet [3] is a notable face-recognition technique, which uses a deep convolutional neural network with 22 layers trained via a triplet loss function to directly output a 128-dimensional embedding. VGGFace2 [4] is a dataset for training face recognition models taking into account pose and age. At the heart of the proposed system is the face ...
The advent of high speed processors and high resolution cameras has spearheaded the research towards design of face recognition systems for various applications. Face recognition systems use either offline data or real-time input, based on the application. In this paper, design and evaluation of a real-time face recognition system using ...
DISCRIMINATION IN FACIAL RECOGNITION TECHNOLOGY. A Thesis submitted to the Faculty of the Graduate School of Arts and Sciences of Georgetown University in partial fulfillment of the requirements for the degree of Masters of Arts In Communication, Culture, and Technology. By. Jieshu Wang, M. Eng.
In the research process of face recognition, feature extraction is not only a key problem but also one of the main difficulties in the development of face recognition. How to effectively extract features directly affects the recognition rate of face image. Aiming at the image processing problem in the process of face recognition, this paper ...
The work of this thesis aims at designing a robust Facial Expression Recognition (FER) system by combining various techniques from computer vision and pattern recognition. Expression recognition is closely related to face recognition where a lot of research has been done and a vast array of algorithms have been introduced. FER can also be
Face Recognition has been used for many applications concerning security, identification, and authentication. This research paper examined 60 years of face recognition history and highlighted the ...
A review of the face detection and face recognition literature as comprehensive as possible, leaving advanced issues, such as video face recognition or expression invariances, for the future work in the framework of a doctoral research. The goal of this "proyecto fin de carrera" was to produce a review of the face detection and face recognition literature as comprehensive as possible.
Face detectors are equipped with a photo of 2500 left or right eyes and the snapshots of the eyestrain terrible sets. Overall advantageous 94 percent and fake-fantastic thirteen percent are detected in facial detection. Eyes are detected at a fee of 88 percentages with the simplest 1 percent false nice outcome.
The project consists of building a facial recognition, electronics operation, and webpage design for the database. Firstly, machine learning and deep learning algorithms were used to recognize faces. In the second step, AI data is transmitted to the electronics components and sensors to make a smart lock system.
The thesis covers the whole process of face recognition, includ-ing preprocessing of images and face detection. The concept of each algorithm is ex-plained, and the description is given accordingly. Additionally, to assess the efficiency of each algorithm a test case with static images was conducted and evaluated.
Human emotion recognition is crucial in various technological domains, reflecting our growing reliance on technology. Facial expressions play a vital role in conveying and preserving human emotions. While deep learning has been successful in recognizing emotions in video sequences, it struggles to effectively model spatio-temporal interactions and identify salient features, limiting its accuracy.
Microsoft recently announced the creation of Microsoft AI, a newly formed organization to help advance our consumer AI products and research, including Copilot.Building on that news, I'm thrilled to share that Microsoft AI is opening a new AI hub in the heart of London. Microsoft AI London will drive pioneering work to advance state-of-the-art language models and their supporting ...