
- school Campus Bookshelves
- menu_book Bookshelves
- perm_media Learning Objects
- login Login
- how_to_reg Request Instructor Account
- hub Instructor Commons
- Download Page (PDF)
- Download Full Book (PDF)
- Periodic Table
- Physics Constants
- Scientific Calculator
- Reference & Cite
- Tools expand_more
- Readability
selected template will load here
This action is not available.
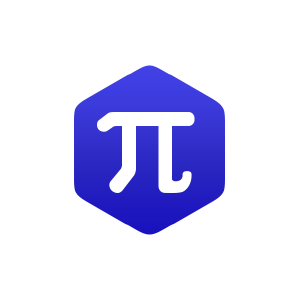
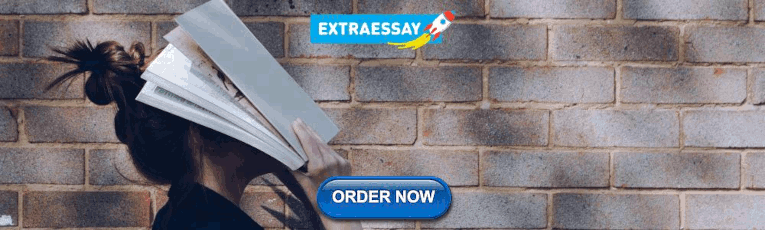
8.1.6: Estimating Probabilities Using Simulation
- Last updated
- Save as PDF
- Page ID 39015
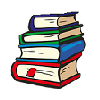
- Illustrative Mathematics
- OpenUp Resources
Let's simulate real-world situations.
Exercise \(\PageIndex{1}\): Which One Doesn't Belong: Spinners
Which spinner doesn't belong?
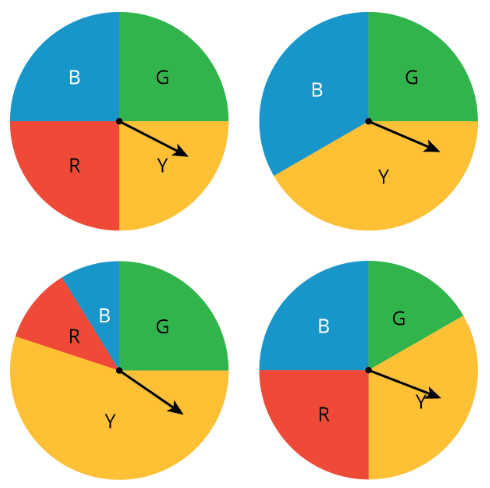
Exercise \(\PageIndex{2}\): Deigo's Walk
Your teacher will give your group the supplies for one of the three different simulations. Follow these instructions to simulate 15 days of Diego’s walk. The first 3 days have been done for you.
- If your group gets a bag of papers, reach into the bag, and select one paper without looking inside.
- If your group gets a spinner, spin the spinner, and see where it stops.
- If your group gets two number cubes, roll both cubes, and add the numbers that land face up. A sum of 2–8 means Diego has to wait.
- Record in the table whether or not Diego had to wait more than 1 minute.
- Calculate the total number of days and the cumulative fraction of days that Diego has had to wait so far.
- On the graph, plot the number of days and the fraction that Diego has had to wait. Connect each point by a line.
- If your group has the bag of papers, put the paper back into the bag, and shake the bag to mix up the papers.
- Pass the supplies to the next person in the group.

- Based on the data you have collected, do you think the fraction of days Diego has to wait after the 16th day will be closer to 0.9 or 0.7? Explain or show your reasoning.
- What do you notice about the graph?
- Based on the graph, estimate the probability that Diego will have to wait more than 1 minute to cross the crosswalk.
Are you ready for more?
Let's look at why the values tend to not change much after doing the simulation many times.
- If this group does the simulation 1 more time, what are the two possible outcomes for the fifth simulation?
- For each possibility, estimate the probability Diego has to wait.
- What are the differences between the possible estimates after 5 simulations and the estimate after 4 simulations?
- If this group does the simulation 1 more time, what are the two possible outcomes for the twenty-first simulation?
- What are the differences between the possible estimates after 21 simulations and the estimate after 20 simulations?
- Use these results to explain why a single result after many simulations does not affect the estimate as much as a single result after only a few simulations.
Exercise \(\PageIndex{3}\): Designing Experiments
For each situation, describe a chance experiment that would fairly represent it.
- Six people are going out to lunch together. One of them will be selected at random to choose which restaurant to go to. Who gets to choose?
- After a robot stands up, it is equally likely to step forward with its left foot or its right foot. Which foot will it use for its first step?
- In a computer game, there are three tunnels. Each time the level loads, the computer randomly selects one of the tunnels to lead to the castle. Which tunnel is it?
- Your school is taking 4 buses of students on a field trip. Will you be assigned to the same bus that your math teacher is riding on?
Sometimes it is easier to estimate a probability by doing a simulation . A simulation is an experiment that approximates a situation in the real world. Simulations are useful when it is hard or time-consuming to gather enough information to estimate the probability of some event.
For example, imagine Andre has to transfer from one bus to another on the way to his music lesson. Most of the time he makes the transfer just fine, but sometimes the first bus is late and he misses the second bus. We could set up a simulation with slips of paper in a bag. Each paper is marked with a time when the first bus arrives at the transfer point. We select slips at random from the bag. After many trials, we calculate the fraction of the times that he missed the bus to estimate the probability that he will miss the bus on a given day.
Glossary Entries
Definition: Chance Experiment
A chance experiment is something you can do over and over again, and you don’t know what will happen each time.
For example, each time you spin the spinner, it could land on red, yellow, blue, or green.
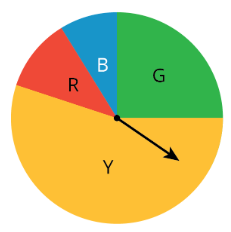
Definition: Event
An event is a set of one or more outcomes in a chance experiment. For example, if we roll a number cube, there are six possible outcomes.

Examples of events are “rolling a number less than 3,” “rolling an even number,” or “rolling a 5.”
Definition: Outcome
An outcome of a chance experiment is one of the things that can happen when you do the experiment. For example, the possible outcomes of tossing a coin are heads and tails.
Definition: Probability
The probability of an event is a number that tells how likely it is to happen. A probability of 1 means the event will always happen. A probability of 0 means the event will never happen.
For example, the probability of selecting a moon block at random from this bag is \(\frac{4}{5}\).
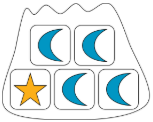
Definition: Random
Outcomes of a chance experiment are random if they are all equally likely to happen.
Definition: Sample Space
The sample space is the list of every possible outcome for a chance experiment.
For example, the sample space for tossing two coins is:
Definition: Simulation
A simulation is an experiment that is used to estimate the probability of a real-world event.
For example, suppose the weather forecast says there is a 25% chance of rain. We can simulate this situation with a spinner with four equal sections. If the spinner stops on red, it represents rain. If the spinner stops on any other color, it represents no rain.

Exercise \(\PageIndex{4}\)
The weather forecast says there is a 75% chance it will rain later today.
- Draw a spinner you could use to simulate this probability.
- Describe another way you could simulate this probability.
Exercise \(\PageIndex{5}\)
An experiment will produce one of ten different outcomes with equal probability for each. Why would using a standard number cube to simulate the experiment be a bad choice?
Exercise \(\PageIndex{6}\)
An ice cream shop offers 40 different flavors. To simulate the most commonly chosen flavor, you could write the name of each flavor on a piece of paper and put it in a bag. Draw from the bag 100 times, and see which flavor is chosen the most. This simulation is not a good way to figure out the most-commonly chosen flavor. Explain why.
Exercise \(\PageIndex{7}\)
Each set of three numbers represents the lengths, in units, of the sides of a triangle. Which set can not be used to make a triangle?
- \(7, 6, 14\)
- \(4, 4, 4\)
- \(6, 6, 2\)
- \(7, 8,13\)
(From Unit 7.2.2)
Exercise \(\PageIndex{8}\)
There is a proportional relationship between a volume measured in cups and the same volume measured in tablespoons. 48 tablespoons is equivalent to 3 cups, as shown in the graph.
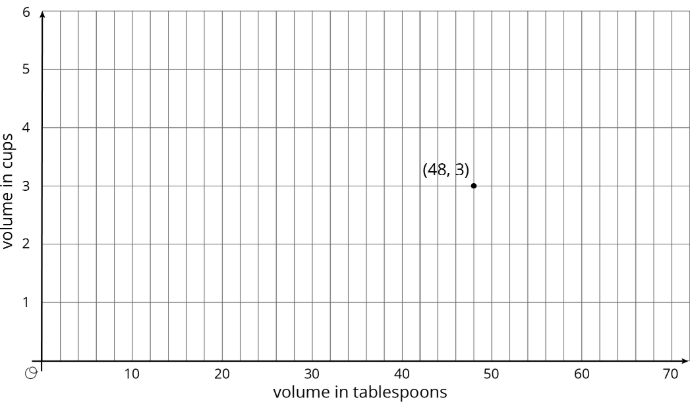
- Plot and label some more points that represent the relationship.
- Use a straightedge to draw a line that represents this proportional relationship.
- For which value \(y\) is (\(1,y\)) on the line you just drew?
- What is the constant of proportionality for this relationship?
- Write an equation representing this relationship. Use \(c\) for cups and \(t\) for tablespoons.
(From Unit 2.5.1)
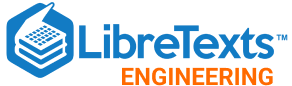
- school Campus Bookshelves
- menu_book Bookshelves
- perm_media Learning Objects
- login Login
- how_to_reg Request Instructor Account
- hub Instructor Commons
- Download Page (PDF)
- Download Full Book (PDF)
- Periodic Table
- Physics Constants
- Scientific Calculator
- Reference & Cite
- Tools expand_more
- Readability
selected template will load here
This action is not available.
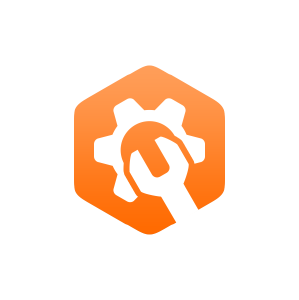
8.1: Statistical Estimation and Simulation
- Last updated
- Save as PDF
- Page ID 55665
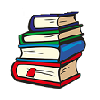
- Masayuki Yano, James Douglass Penn, George Konidaris, & Anthony T Patera
- Massachusetts Institute of Technology via MIT OpenCourseWare
Random Models and Phenomena
In science and engineering environments, we often encounter experiments whose outcome cannot be determined with certainty in practice and is better described as random. An example of such a random experiment is a coin flip. The outcome of flipping a (fair) coin is either heads (H) or tails (T), with each outcome having equal probability. Here, we define the probability of a given outcome as the frequency of its occurrence if the experiment is repeated a large number of times. \({ }^{1}{ }^{-}\) In other words, when we say that there is equal probability of heads and tails, we mean that there would be an equal number of heads and tails if a fair coin is flipped a large (technically infinite) number of times. In addition, we expect the outcome of a random experiment to be unpredictable in some sense; a coin that consistently produces a sequence HTHTHTHT or HHHTTTHHHTTT can be hardly called random. In the case of a coin flip, we may associate this notion of unpredictability or randomness with the inability to predict with certainty the outcome of the next flip by knowing the outcomes of all preceding flips. In other words, the outcome of any given flip is independent of or unrelated to the outcome of other flips.
While the event of heads or tails is random, the distribution of the outcome over a large number of repeated experiments (i.e. the probability density) is determined by non-random parameters. In the case of a coin flip, the sole parameter that dictates the probability density is the probability of heads, which is \(1 / 2\) for a fair coin; for a non-fair coin, the probability of heads is (by definition) different from \(1 / 2\) but is still some fixed number between 0 and 1 .
Now let us briefly consider why the outcome of each coin flip may be considered random. The outcome of each flip is actually governed by a deterministic process. In fact, given a full description of the experiment - the mass and moment of inertia of the coin, initial launch velocity, initial angular momentum, elasticity of the landing surface, density of the air, etc - we can, in principle, predict the outcome of our coin flip by solving a set of deterministic governing equations - Euler’s equations for rigid body dynamics, the Navier-Stokes equations for aerodynamics, etc. However, even for something as simple as flipping a coin, the number of variables that describe the state of the system is very large. Moreover, the equations that relate the state to the final outcome (i.e.
\({ }^{1}\) We adhere to the frequentistic view of probability throughout this unit. We note that the Baysian view is an alternative, popular interpretation of probability. heads or tails) are complicated and the outcome is very sensitive to the conditions that govern the experiments. This renders detailed prediction very difficult, but also suggests that a random model - which considers just the outcome and not the myriad "uncontrolled" ways in which we can observe the outcome - may suffice.
Although we will use a coin flip to illustrate various concepts of probability throughout this unit due to its simplicity and our familiarity with the process, we note that random experiments are ubiquitous in science and engineering. For example, in studying gas dynamics, the motion of the individual molecules is best described using probability distributions. Recalling that 1 mole of gas contains approximately \(6 \times 10^{23}\) particles, we can easily see that deterministic characterization of their motion is impractical. Thus, scientists describe their motion in probabilistic terms; in fact, the macroscale velocity and temperature are parameters that describe the probability distribution of the particle motion, just as the fairness of a given coin may be characterized by the probability of a head. In another instance, an engineer studying the effect of gust on an airplane may use probability distributions to describe the change in the velocity field affected by the gust. Again, even though the air motion is well-described by the Navier-Stokes equations, the highly sensitive nature of turbulence flows renders deterministic prediction of the gust behavior impractical. More importantly, as the engineer is most likely not interested in the detailed mechanics that governs the formation and propagation of the gust and is only interested in its effect on the airplane (e.g., stresses), the gust velocity is best described in terms of a probability distribution.
Statistical Estimation of Parameters/Properties of Probability Distributions
Statistical estimation is a process through which we deduce parameters that characterize the behavior of a random experiment based on a sample - a set of typically large but in any event finite number of outcomes of repeated random experiments. \({ }^{2}{ }^{2}\) In most cases, we postulate a probability distribution - based on some plausible assumptions or based on some descriptive observations such as crude histogram - with several parameters; we then wish to estimate these parameters. Alternatively, we may wish to deduce certain properties - for example, the mean - of the distribution; these properties may not completely characterize the distribution, but may suffice for our predictive purposes. (In other cases, we may need to estimate the full distribution through an empirical cumulative distribution function; We shall not consider this more advanced case in this text.) In Chapter \(\underline{9}\) , we will introduce a variety of useful distributions, more precisely parametrized discrete probability mass functions and continuous probability densities, as well as various properties and techniques which facilitate the interpretation of these distributions.
Let us illustrate the statistical estimation process in the context of a coin flip. We can flip a coin (say) 100 times, record each observed outcome, and take the mean of the sample - the fraction which are heads - to estimate the probability of heads. We expect from our frequentist interpretation that the sample mean will well approximate the probability of heads. Note that, we can only estimate - rather than evaluate - the probability of heads because evaluating the probability of heads would require, by definition, an infinite number of experiments. We expect that we can estimate the probability of heads - the sole parameter dictating the distribution of our outcome - with more confidence as the sample size increases. For instance, if we wish to verify the fairness of a given coin, our intuition tells us that we are more likely to deduce its fairness (i.e. the probability of heads equal to \(0.5\) ) correctly if we perform 10 flips than 3 flips. The probability of landing HHH using a fair coin in three flips - from which we might incorrectly conclude the coin as unfair - is \(1 / 8\) , which is not so unlikely, but that of landing HHHHHHHHHH in 10 flips is less than 1 in 1000 trials, which is very unlikely.
\({ }^{2}\) We will provide a precise mathematical definition of sample in Chapter \(10 .\) In Chapters 10 and 11 , we will introduce a mathematical framework that not only allows us to estimate the parameters that characterize a random experiment but also quantify the confidence we should have in such characterization; the latter, in turn, allows us to make claims - such as the fairness of a coin - with a given level of confidence. We consider two ubiquitous cases: a Bernoulli discrete mass density (relevant to our coin flipping model, for example) in Chapter 10 ; and the normal density in Chapter \(11 .\)
Of course, the ultimate goal of estimation is inference - prediction. In some cases the parameters or quantities estimated, or corresponding "hypothesis tests," in fact suffice for our purposes. In other cases, we can apply the rules of probability to make additional conclusions about how a system will behave and in particular the probability of certain events. In some sense, we go from a finite sample of a population to a probability "law" and then back to inferences about particular events relevant to finite samples from the population.
Monte Carlo Simulation
So far, we have argued that a probability distribution may be effectively used to characterize the outcome of experiments whose deterministic characterization is impractical due to a large number of variables governing its state and/or complicated functional dependencies of the outcome on the state. Another instance in which a probabilistic description is favored over a deterministic description is when their use is computationally advantageous even if the problem is deterministic.
One example of such a problem is determination of the area (or volume) of a region whose boundary is described implicitly. For example, what is the area of a unit-radius circle? Of course, we know the answer is \(\pi\) , but how might we compute the area if we did not know that \(A=\pi r^{2}\) ? One way to compute the area may be to tessellate (or discretize) the region into small pieces and employ the deterministic integration techniques discussed in Chapter 7 . However, application of the deterministic techniques becomes increasingly difficult as the region of interest becomes more complex. For instance, tessellating a volume intersected by multiple spheres is not a trivial task. More generally, deterministic techniques can be increasingly inefficient as the dimension of the integration domain increases.
Monte Carlo methods are better suited for integrating over such a complicated region. Broadly, Monte Carlo methods are a class of computational techniques based on synthetically generating random variables to deduce the implication of the probability distribution. Let us illustrate the idea more precisely for the area determination problem. We first note that if our region of interest is immersed in a unit square, then the area of the region is equal to the probability of a point drawn randomly from the unit square residing in the region. Thus, if we assign a value of 0 (tail) and 1 (head) to the event of drawing a point outside and inside of the region, respectively, approximating the area is equivalent to estimating the probability we land inside (a head). Effectively, we have turned our area determination problem into an statistical estimation problem; the problem is now no different from the coin flip experiment, except the outcome of each "flip" is determined by performing a (simple) check that determines if the point drawn is inside or outside of the region. In other words, we synthetically generate a random variable (by performing the in/out check on uniformly drawn samples) and deduce the implication on the distribution (in this case the area, which is the mean of the distribution). We will study Monte-Carlo-based area integration techniques in details in Chapter 12 .
There are several advantages to using Monte Carlo methods compared to deterministic integration approaches. First, Monte Carlo methods are simple to implement: in our case, we do not need to know the domain, we only need to know whether we are in the domain. Second, Monte Carlo methods do not rely on smoothness for convergence - if we think of our integrand as 0 and 1 (depending on outside or inside), our problem here is quite non-smooth. Third, although Monte Carlo methods do not converge particularly quickly, the convergence rate does not degrade in higher dimensions - for example, if we wished to estimate the volume of a region in a threedimensional space. Fourth, Monte Carlo methods provide a result, along with a simple built-in error estimator, "gradually" - useful, if not particularly accurate, answers are obtained early on in the process and hence inexpensively and quickly. Note for relatively smooth problems in smooth domains Monte Carlo techniques are not a particularly good idea. Different methods work better in different contexts.
Monte Carlo methods - and the idea of synthetically generating a distribution to deduce its implication - apply to a wide range of engineering problems. One such example is failure analysis. In the example of an airplane flying through a gust, we might be interested in the stress on the spar and wish to verify that the maximum stress anywhere in the spar does not exceed the yield strength of the material - and certainly not the fracture strength so that we may prevent a catastrophic failure. Directly drawing from the distribution of the gust-induced stress would be impractical; the process entails subjecting the wing to various gust and directly measuring the stress at various points. A more practical approach is to instead model the gust as random variables (based on empirical data), propagate its effect through an aeroelastic model of the wing, and synthetically generate the random distribution of the stress. To estimate the properties of the distribution such as the mean stress or the probability of the maximum stress exceeding the yield stress - we simply need to use a large enough set of realizations of our synthetically generated distribution. We will study the use of Monte Carlo methods for failure analysis in Chapter 14 .
Let us conclude this chapter with a practical example of area determination problem in which the use of Monte Carlo methods may be advantageous.

Provide details on what you need help with along with a budget and time limit. Questions are posted anonymously and can be made 100% private.

Studypool matches you to the best tutor to help you with your question. Our tutors are highly qualified and vetted.

Your matched tutor provides personalized help according to your question details. Payment is made only after you have completed your 1-on-1 session and are satisfied with your session.

- Homework Q&A
- Become a Tutor

All Subjects
Mathematics
Programming
Health & Medical
Engineering
Computer Science
Foreign Languages

Access over 20 million homework & study documents
Pdf lab 15 answers: probability, simulation, estimation, and assessing models.

Sign up to view the full document!

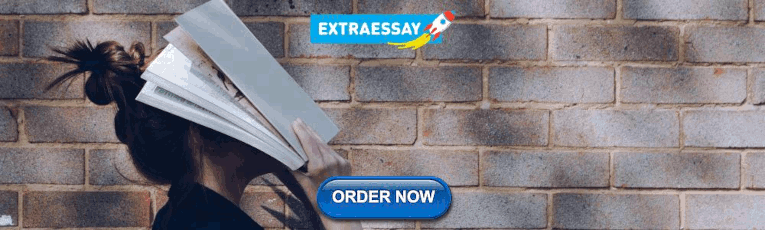
24/7 Homework Help
Stuck on a homework question? Our verified tutors can answer all questions, from basic math to advanced rocket science !

Similar Documents

working on a homework question?

Studypool is powered by Microtutoring TM
Copyright © 2024. Studypool Inc.
Studypool is not sponsored or endorsed by any college or university.
Ongoing Conversations

Access over 20 million homework documents through the notebank

Get on-demand Q&A homework help from verified tutors

Read 1000s of rich book guides covering popular titles

Sign up with Google

Sign up with Facebook
Already have an account? Login
Login with Google
Login with Facebook
Don't have an account? Sign Up
Estimating Probabilities by Collecting Data
Related Topics: Lesson Plans and Worksheets for Grade 7 Lesson Plans and Worksheets for all Grades More Lessons for Grade 7 Common Core For Grade 7
Examples, videos, and solutions to help Grade 7 students learn how to estimate probabilities by collecting data on an outcome of a chance experiment.
New York State Common Core Math Grade 7, Module 5, Lesson 2
Worksheets for Grade 7
Lesson 2 Student Outcomes
- Students learn how to estimate probabilities by collecting data on an outcome of a chance experiment. bull; Students use given data to estimate probabilities.
Lesson 2 Summary
- An estimate for finding the probability of an event occurring is
- P(event occurring) = (Number of observed occurrences of the event)/(Total number of observations)
Lesson 2 Classwork Example 1: Carnival Game At the school carnival, there is a game in which students spin a large spinner. The spinner has four equal sections numbered 1–4 as shown below. To play the game, a student spins the spinner twice and adds the two numbers that the spinner lands on. If the sum is greater than or equal to , the student wins a prize.
Exercises 1–8 You and your partner will play this game 15 times. Record the outcome of each spin in the table below.
- Out of the 15 turns how many times was the sum greater than or equal to 5?
- What sum occurred most often?
- What sum occurred least often?
- If students played a lot of games, what proportion of the games played will they win? Explain your answer.
- Name a sum that would be impossible to get while playing the game.
- What event is certain to occur while playing the game?
- Based on your experiment of playing the game, what is your estimate for the probability of getting a sum of 5 or more?
- Based on your experiment of playing the game, what is your estimate for the probability of getting a sum of exactly 5?
Example 2 A student brought a very large jar of animal crackers to share with students in class. Rather than count and sort all the different types of crackers, the student randomly chose 20 crackers and found the following counts for the different types of animal crackers. The student can now use that data to find an estimate for the probability of choosing a zebra from the jar by dividing the observed number of zebras by the total number of crackers selected.
Exercises 9–15 If a student were to randomly select a cracker from the large jar: 9. What is your estimate for the probability of selecting a lion? 10. What is the estimate for the probability of selecting a monkey? 11. What is the estimate for the probability of selecting a penguin or a camel? 12. What is the estimate for the probability of selecting a rabbit? 13. Is there the same number of each animal cracker in the large jar? Explain your answer. 14. If the student were to randomly select another 20 animal crackers, would the same results occur? Why or why not? 15. If there are animal crackers in the jar, how many elephants are in the jar? Explain your answer.
Sample Questions Example 1: Carnival Game At the school carnival, there is a game in which students spin a large spinner. The spinner has four equal sections numbered 1–4 as shown below. To play the game, a student spins the spinner twice and adds the two numbers that the spinner lands on. If the sum is greater than or equal to , the student wins a prize.
- Based on your experiment what is the estimate for the probability of getting a sum exactly equal to 5?
- Out of 15 turns, how many times was the sum greater than or equal to 5?
- Based on this experiment, what is the estimated probability that the sum would be greater than or equal to 5?
- If you played this game 300 times, how many times would the sum be greater than or equal to 5?
- A seventh grade student surveyed students at her school. She asked them how many hours a week they spend doing homework. The results are listed in the table below. a. Draw a dot plot of the results. Suppose a student will be randomly selected. b. What is your estimate for the probability of that student spending 7 hours per week doing homework? c. What is your estimate for the probability of that student spending more than 7 hours per week doing homework? d. If another 25 students were surveyed, do you think they will give the exact same results? Explain your answer. e. If there are 600 students at the school, what is your estimate for the number of students who would say they do 5 hours of homework per week?
A student played a game using one of the spinners below. The table shows the results of spins. Which spinner did the student use? Give a reason for your answer.
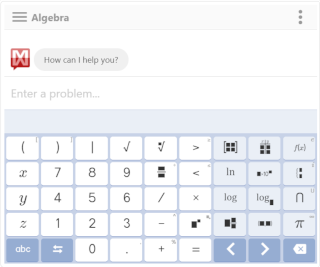
We welcome your feedback, comments and questions about this site or page. Please submit your feedback or enquiries via our Feedback page.
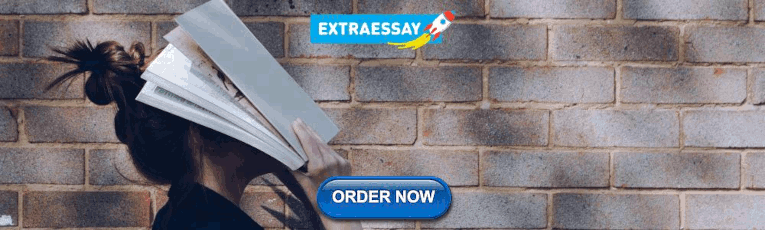
IMAGES
VIDEO
COMMENTS
1 Homework 6: Probability, Simulation, Estimation, and Assessing Models. Reading: Textbook chapters 9 , 10 , and 11. Please complete this notebook by filling in the cells provided. Before you begin, execute the following cell to load the provided tests. Each time you start your server, you will need to execute this cell again to load the tests.
1 Homework 6: Probability, Simulation, Estimation, and Assessing Models. Reading: Textbook chapters 9 , 10 , and 11. Please complete this notebook by filling in the cells provided. Before you begin, execute the following cell to load the provided tests. Each time you start your server, you will need to execute this cell again to load the tests.
View hw06_answers.pdf from MATHEMATIC 8 at O'bryant Sch Math/science. 3/18/2021 hw06 Homework 6: Probability, Simulation, Estimation, and Assessing Models Reading: Randomness
View Homework 6- Probability, Simulation, Estimation, and Assessing Models.pdf from STOR 120 at University of North Carolina, Chapel Hill. 11/19/21, 1:07 PM 2021 STOR 120 -
View Homework Help - hw06.pdf from DATA MISC at University of California, Berkeley. Homework 6: Probability, Simulation, Estimation, and Assessing Models Reading: Randomness
and the domain of the objective function is defined as. D = {(a, b) ∈ Rm+1 × Rn | q(t) > 0, α ≤ t ≤ β}. In this problem we fit a rational function p(t)/q(t) to given data, while constraining the denominator polynomial to be positive on the interval [α, β]. The optimization variables are the numerator and denominator coefficients ai, bi.
Definition: Simulation. A simulation is an experiment that is used to estimate the probability of a real-world event. For example, suppose the weather forecast says there is a 25% chance of rain. We can simulate this situation with a spinner with four equal sections. If the spinner stops on red, it represents rain.
hw06 March 3, 2023 1 Homework 6: Probability, Simulation, Estimation, and Assess- ing Models Reading: * Randomness * Sampling and Empirical Distributions * Testing Hypotheses Please complete this notebook by filling in the cells provided. Before you begin, execute the following cell to load the provided tests. Each time you start your server, you will need to execute this cell again to load ...
Dec 6, 2023. Type. pdf. Pages. 16. Uploaded by ElderValorGuanaco36 on coursehero.com. VIEW FULL DOCUMENT. Homework 6: Probability, Simulation, Estimation, and Assessing Models Reading : • Randomness • Sampling and Empirical Distributions • Testing Hypotheses Please complete this notebook by filling in the cells provided.
In [24]: # Initialize Otter import otter grader = otter.Notebook("hw06.ipynb") Homework 6: Probability, Simulation, Estimation, and Assessing Models ¶ Please complete this notebook by filling in the cells provided. Before you begin, execute the previous cell to load the provided tests. Helpful Resource: • Python Reference: Cheat sheet of helpful array & table methods used in Data 8!
Question 2.1. Suppose the monkey hits the keyboard 11 times. Compute the chance that the monkey types the sequence datascience. (Call this datascience_chance.)Use algebra and type in an arithmetic equation that Python can evalute. In [ ]: Question 2.2. Write a function called simulate_key_strike.It should take no arguments, and it should return a random one-character string that is equally ...
HW6 cive 6378 homework probabilistic models akaike information criterion assigned due 11:59 pm the goal of this homework is to compare which probability. Skip to document. University; High School ... Perform these additional analyses: a. By visually assessing the plots for the four model fits compared to the experimental cdf, which model ...
Computer-science document from University of California, Berkeley, 17 pages, MStokesHW6 March 23, 2023 [1]: import otter grader = otter.Notebook() 1 Homework 6: Probability, Simulation, Estimation, and Assessing Models Reading: * Randomness * Sampling and Empirical Distributions * Testing Hypotheses Please complete this notebook b
Instead, a number cube could be used by specifying that the numbers 1, 2, or 3 represent a hit, the number 4 represents a miss, and the numbers 5 and 6 would be ignored. Based on the following 50 trials of rolling a fair number cube, find an estimate of the probability that she makes five or six of the six foul shots she takes.
Homework 6: Probability, Simulation, Estimation, and Assessing Models Reading: Randomness Sampling and Empirical Distributions Testing Hypotheses Please complete this notebook by filling in the cells provided. Before you begin, execute the following cell to load the provided tests. Each time you start your server, you will need to execute this cell again to load the tests.
8.1: Statistical Estimation and Simulation Expand/collapse global location 8.1: Statistical Estimation and Simulation ... (fair) coin is either heads (H) or tails (T), with each outcome having equal probability. Here, we define the probability of a given outcome as the frequency of its occurrence if the experiment is repeated a large number of ...
Get Quality Help. Your matched tutor provides personalized help according to your question details. Payment is made only after you have completed your 1-on-1 session and are satisfied with your session.
Thompson begins with an exposition of the generation of randomnumbers and then delves into a variety of special topics, including models for stocks and derivatives, optimization and estimation in a noisy world, Monte-Carlo solutions to differential equations, simulation assessment of multivariate and robust procedures in statistical process ...
Unset In [1]: # Initialize Otter import otter grader = otter.Notebook("hw06.ipynb") Homework 6: Probability, Simulation, Estimation, and Assessing Models Please complete this notebook by filling in the cells provided. Before you begin, execute the previous cell to load the provided tests. Helpful Resource: Python Reference: Cheat sheet of helpful array & table methods used in Data 8!
Python Notebook Viewer Homework 6: Probability, Simulation, Estimation, and Assessing Models Reading: • Randomness • Sampling and Empirical Distributions • Testing Hypotheses Directly sharing answers is not okay, but discussing problems with the course staff or with other students is encouraged. Refer to the policies page to learn more about how to learn cooperatively.
Lesson 2 Summary. An estimate for finding the probability of an event occurring is. P (event occurring) = (Number of observed occurrences of the event)/ (Total number of observations) Lesson 2 Classwork. Example 1: Carnival Game. At the school carnival, there is a game in which students spin a large spinner.
Submitted Files for HW 06 Autograder hw06.ipynb Download In [1]: # Initialize Otter import otter grader = otter.Notebook("hw06.ipynb") Homework 6: Probability, Simulation, Estimation, and Assessing Models Please complete this notebook by filling in the cells provided. Before you begin, execute the previous cell to load the provided tests. Helpful Resource: Python Reference: Cheat sheet of ...