Featured Topics
Featured series.
A series of random questions answered by Harvard experts.
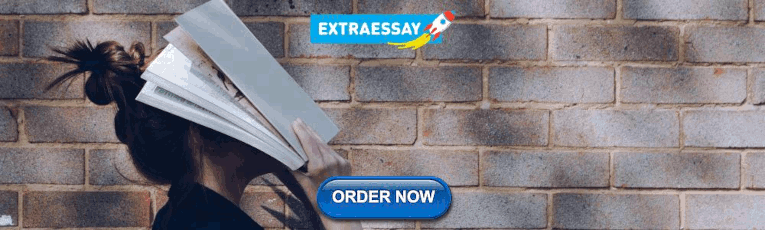
Explore the Gazette
Read the latest.
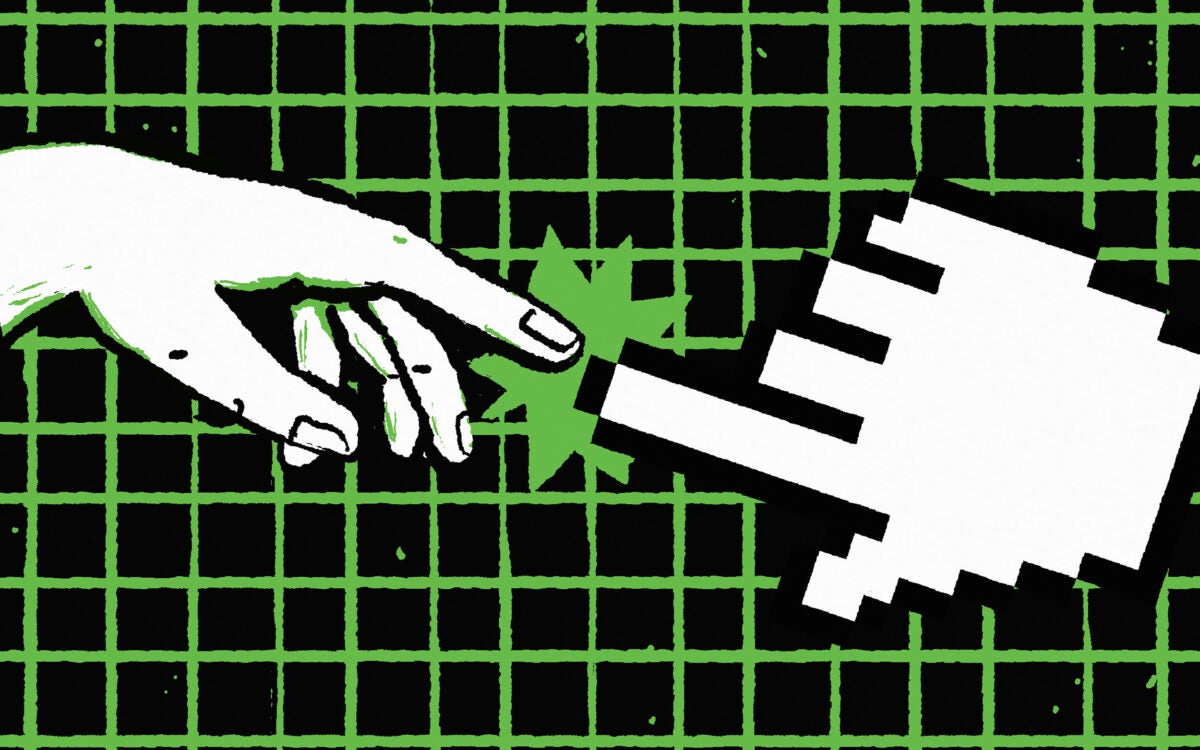
‘Harvard Thinking’: Is AI friend or foe? Wrong question.
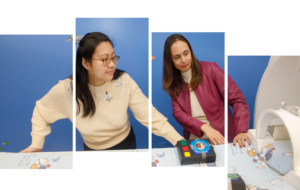
Getting ahead of dyslexia
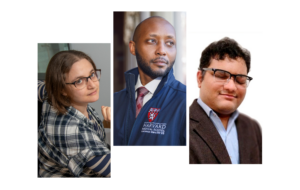
Why AI fairness conversations must include disabled people
Lessons in learning.
Sean Finamore ’22 (left) and Xaviera Zime ’22 study during a lecture in the Science Center.
Photos by Kris Snibbe/Harvard Staff Photographer
Peter Reuell
Harvard Staff Writer
Study shows students in ‘active learning’ classrooms learn more than they think
For decades, there has been evidence that classroom techniques designed to get students to participate in the learning process produces better educational outcomes at virtually all levels.
And a new Harvard study suggests it may be important to let students know it.
The study , published Sept. 4 in the Proceedings of the National Academy of Sciences, shows that, though students felt as if they learned more through traditional lectures, they actually learned more when taking part in classrooms that employed so-called active-learning strategies.
Lead author Louis Deslauriers , the director of science teaching and learning and senior physics preceptor, knew that students would learn more from active learning. He published a key study in Science in 2011 that showed just that. But many students and faculty remained hesitant to switch to it.
“Often, students seemed genuinely to prefer smooth-as-silk traditional lectures,” Deslauriers said. “We wanted to take them at their word. Perhaps they actually felt like they learned more from lectures than they did from active learning.”
In addition to Deslauriers, the study is authored by director of sciences education and physics lecturer Logan McCarty , senior preceptor in applied physics Kelly Miller, preceptor in physics Greg Kestin , and Kristina Callaghan, now a physics lecturer at the University of California, Merced.
The question of whether students’ perceptions of their learning matches with how well they’re actually learning is particularly important, Deslauriers said, because while students eventually see the value of active learning, initially it can feel frustrating.
“Deep learning is hard work. The effort involved in active learning can be misinterpreted as a sign of poor learning,” he said. “On the other hand, a superstar lecturer can explain things in such a way as to make students feel like they are learning more than they actually are.”
To understand that dichotomy, Deslauriers and his co-authors designed an experiment that would expose students in an introductory physics class to both traditional lectures and active learning.
For the first 11 weeks of the 15-week class, students were taught using standard methods by an experienced instructor. In the 12th week, half the class was randomly assigned to a classroom that used active learning, while the other half attended highly polished lectures. In a subsequent class, the two groups were reversed. Notably, both groups used identical class content and only active engagement with the material was toggled on and off.
Following each class, students were surveyed on how much they agreed or disagreed with statements such as “I feel like I learned a lot from this lecture” and “I wish all my physics courses were taught this way.” Students were also tested on how much they learned in the class with 12 multiple-choice questions.
When the results were tallied, the authors found that students felt as if they learned more from the lectures, but in fact scored higher on tests following the active learning sessions. “Actual learning and feeling of learning were strongly anticorrelated,” Deslauriers said, “as shown through the robust statistical analysis by co-author Kelly Miller, who is an expert in educational statistics and active learning.”
Those results, the study authors are quick to point out, shouldn’t be interpreted as suggesting students dislike active learning. In fact, many studies have shown students quickly warm to the idea, once they begin to see the results. “In all the courses at Harvard that we’ve transformed to active learning,” Deslauriers said, “the overall course evaluations went up.”
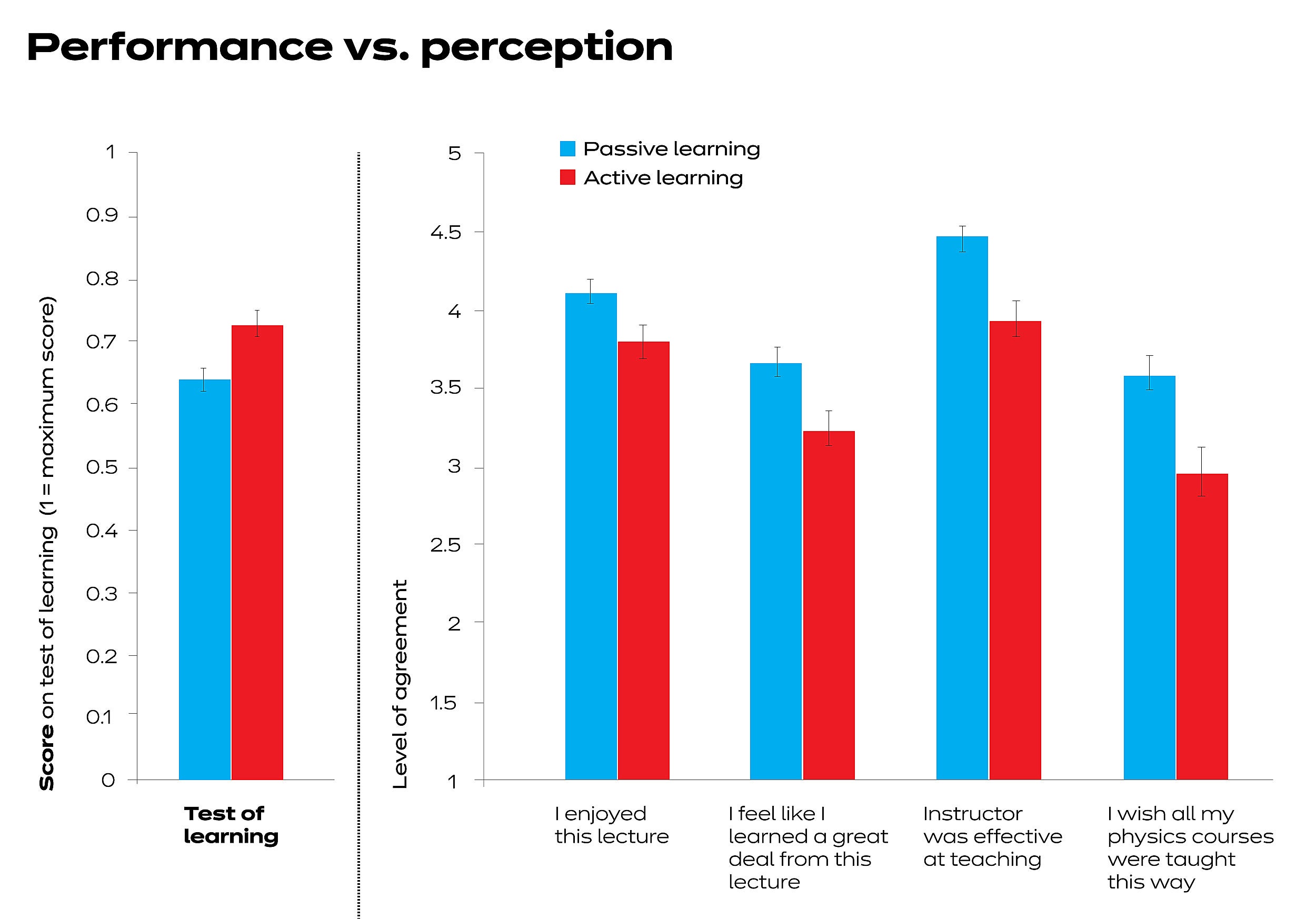
Co-author Kestin, who in addition to being a physicist is a video producer with PBS’ NOVA, said, “It can be tempting to engage the class simply by folding lectures into a compelling ‘story,’ especially when that’s what students seem to like. I show my students the data from this study on the first day of class to help them appreciate the importance of their own involvement in active learning.”
McCarty, who oversees curricular efforts across the sciences, hopes this study will encourage more of his colleagues to embrace active learning.
“We want to make sure that other instructors are thinking hard about the way they’re teaching,” he said. “In our classes, we start each topic by asking students to gather in small groups to solve some problems. While they work, we walk around the room to observe them and answer questions. Then we come together and give a short lecture targeted specifically at the misconceptions and struggles we saw during the problem-solving activity. So far we’ve transformed over a dozen classes to use this kind of active-learning approach. It’s extremely efficient — we can cover just as much material as we would using lectures.”
A pioneer in work on active learning, Balkanski Professor of Physics and Applied Physics Eric Mazur hailed the study as debunking long-held beliefs about how students learn.
“This work unambiguously debunks the illusion of learning from lectures,” he said. “It also explains why instructors and students cling to the belief that listening to lectures constitutes learning. I recommend every lecturer reads this article.”
Dean of Science Christopher Stubbs , Samuel C. Moncher Professor of Physics and of Astronomy, was an early convert. “When I first switched to teaching using active learning, some students resisted that change. This research confirms that faculty should persist and encourage active learning. Active engagement in every classroom, led by our incredible science faculty, should be the hallmark of residential undergraduate education at Harvard.”
Ultimately, Deslauriers said, the study shows that it’s important to ensure that neither instructors nor students are fooled into thinking that lectures are the best learning option. “Students might give fabulous evaluations to an amazing lecturer based on this feeling of learning, even though their actual learning isn’t optimal,” he said. “This could help to explain why study after study shows that student evaluations seem to be completely uncorrelated with actual learning.”
This research was supported with funding from the Harvard FAS Division of Science.
Share this article
You might like.
In podcast, a lawyer, computer scientist, and statistician debate ethics of artificial intelligence
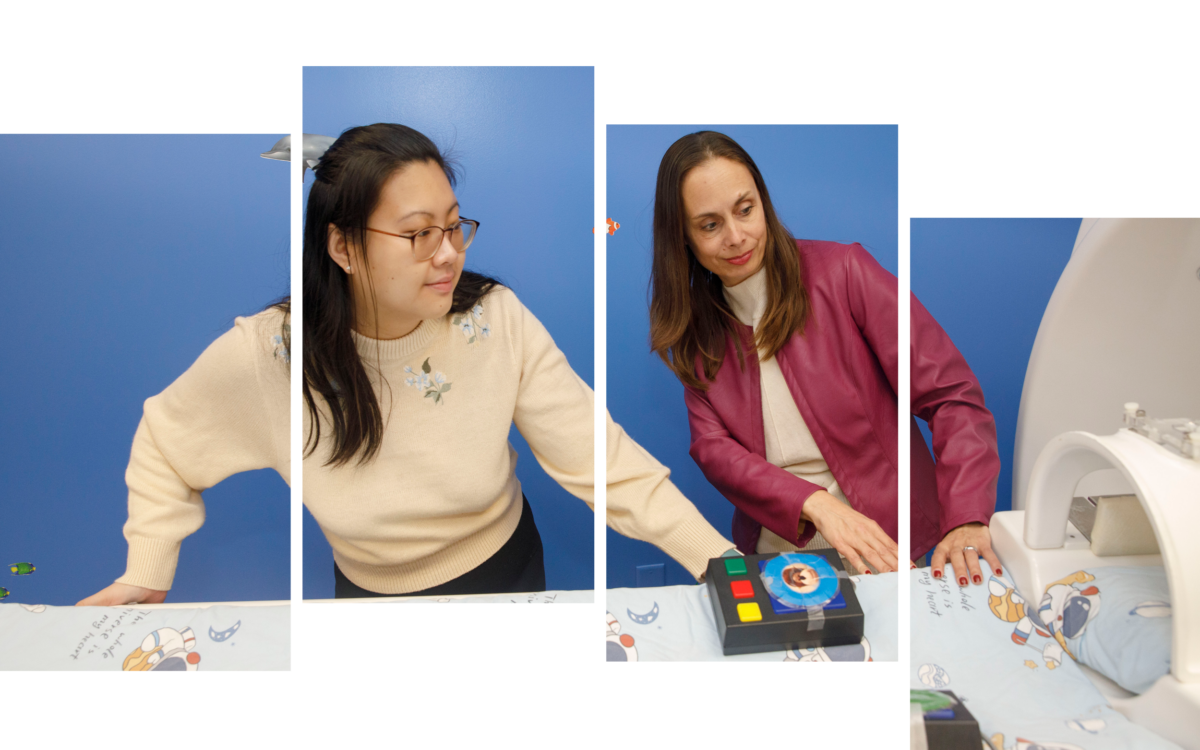
Harvard lab’s research suggests at-risk kids can be identified before they ever struggle in school
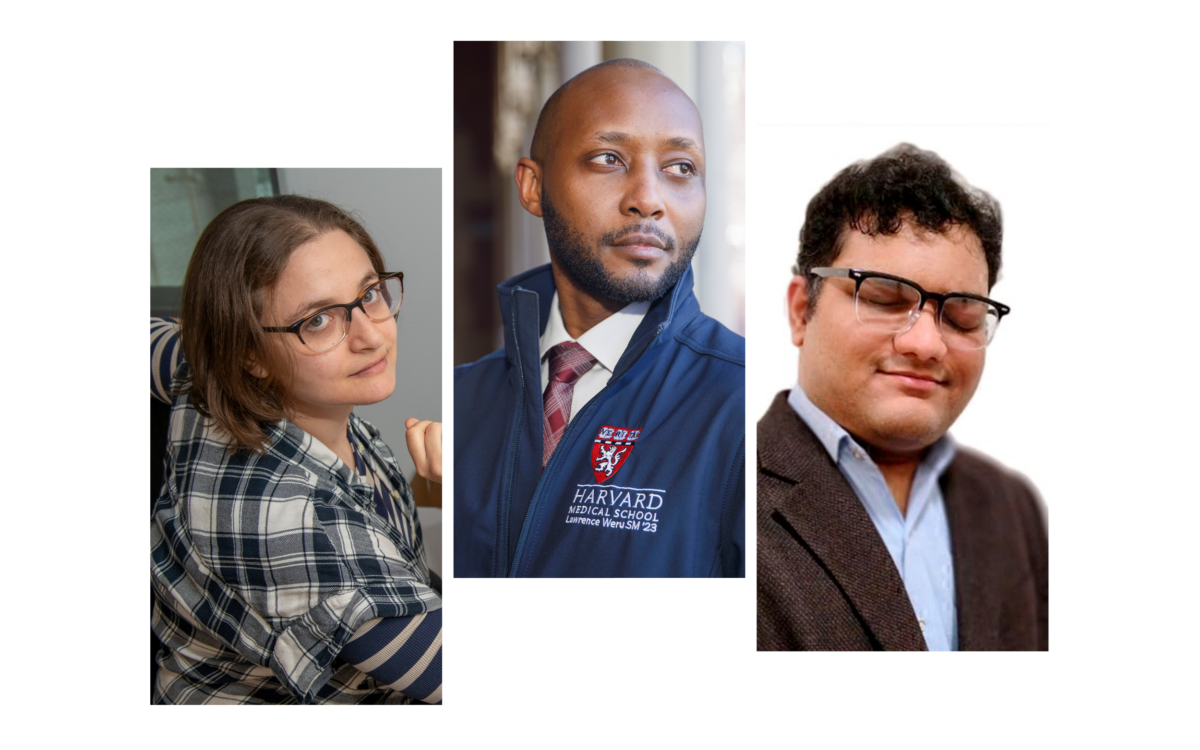
Tech offers promise to help yet too often perpetuates ableism, say researchers. It doesn’t have to be this way.
Harvard announces return to required testing
Leading researchers cite strong evidence that testing expands opportunity
For all the other Willie Jacks
‘Reservation Dogs’ star Paulina Alexis offers behind-the-scenes glimpse of hit show, details value of Native representation
When will patients see personalized cancer vaccines?
Sooner than you may think, says researcher who recently won Sjöberg Prize for pioneering work in field
Thank you for visiting nature.com. You are using a browser version with limited support for CSS. To obtain the best experience, we recommend you use a more up to date browser (or turn off compatibility mode in Internet Explorer). In the meantime, to ensure continued support, we are displaying the site without styles and JavaScript.
- View all journals
- My Account Login
- Explore content
- About the journal
- Publish with us
- Sign up for alerts
- Open access
- Published: 07 April 2023
Using machine learning to predict student retention from socio-demographic characteristics and app-based engagement metrics
- Sandra C. Matz 1 ,
- Christina S. Bukow 2 ,
- Heinrich Peters 1 ,
- Christine Deacons 3 ,
- Alice Dinu 5 na1 &
- Clemens Stachl 4
Scientific Reports volume 13 , Article number: 5705 ( 2023 ) Cite this article
7812 Accesses
2 Citations
35 Altmetric
Metrics details
- Human behaviour
An Author Correction to this article was published on 21 June 2023
This article has been updated
Student attrition poses a major challenge to academic institutions, funding bodies and students. With the rise of Big Data and predictive analytics, a growing body of work in higher education research has demonstrated the feasibility of predicting student dropout from readily available macro-level (e.g., socio-demographics or early performance metrics) and micro-level data (e.g., logins to learning management systems). Yet, the existing work has largely overlooked a critical meso-level element of student success known to drive retention: students’ experience at university and their social embeddedness within their cohort. In partnership with a mobile application that facilitates communication between students and universities, we collected both (1) institutional macro-level data and (2) behavioral micro and meso-level engagement data (e.g., the quantity and quality of interactions with university services and events as well as with other students) to predict dropout after the first semester. Analyzing the records of 50,095 students from four US universities and community colleges, we demonstrate that the combined macro and meso-level data can predict dropout with high levels of predictive performance (average AUC across linear and non-linear models = 78%; max AUC = 88%). Behavioral engagement variables representing students’ experience at university (e.g., network centrality, app engagement, event ratings) were found to add incremental predictive power beyond institutional variables (e.g., GPA or ethnicity). Finally, we highlight the generalizability of our results by showing that models trained on one university can predict retention at another university with reasonably high levels of predictive performance.
Similar content being viewed by others
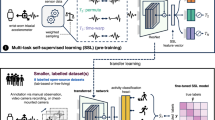
Self-supervised learning for human activity recognition using 700,000 person-days of wearable data
Hang Yuan, Shing Chan, … Aiden Doherty
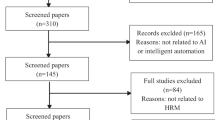
Ethics and discrimination in artificial intelligence-enabled recruitment practices
Zhisheng Chen
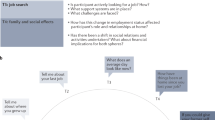
Interviews in the social sciences
Eleanor Knott, Aliya Hamid Rao, … Chana Teeger
Introduction
In the US, only about 60% of full-time students graduate from their program 1 , 2 with the majority of those who discontinue their studies dropping out during their first year 3 These high attrition rates pose major challenges to students, universities, and funding bodies alike 4 , 5 .
Dropping out of university without a degree negatively impacts students’ finances and mental health. Over 65% of US undergraduate students receive student loans to help pay for college, causing them to incur heavy debts over the course of their studies 6 . According to the U.S. Department of Education, students who take out a loan but never graduate are three times more likely to default on loan repayment than students who graduate 7 . This is hardly surprising, given that students who drop out of university without a degree, earn 66% less than university graduates with a bachelor's degree and are far more likely to be unemployed 2 . In addition to financial losses, the feeling of failure often adversely impacts students’ well-being and mental health 8 .
At the same time, student attrition negatively impacts universities and federal funding bodies. For universities, student attrition results in an average annual revenue reduction of approximately $16.5 billion per year through the loss of tuition fees 9 , 10 . Similarly, student attrition wastes valuable resources provided by states and federal governments. For example, the US Department of Education Integrated Postsecondary Education Data System (IPEDS) shows that between 2003 and 2008, state and federal governments together provided more than $9 billion in grants and subsidies to students who did not return to the institution where they were enrolled for a second year 11 .
Given the high costs of attrition, the ability to predict at-risk students – and to provide them with additional support – is critical 12 , 13 . As most dropouts occur during the first year 14 , such predictions are most valuable if they can identify at-risk students as early as possible 13 , 15 , 16 . The earlier one can identify students who might struggle, the better the chances that interventions aimed at protecting them from gradually falling behind – and eventually discontinuing their studies – will be effective 17 , 18 .
Indicators of student retention
Previous research has identified various predictors of student retention, including previous academic performance, demographic and socio-economic factors, and the social embeddedness of a student in their home institution 19 , 20 , 21 , 22 , 23 .
Prior academic performance (e.g., high school GPA, SAT and ACT scores or college GPA) has been identified as one of the most consistent predictors of student retention: Students who are more successful academically are less likely to drop out 17 , 21 , 24 , 25 , 26 , 27 , 28 , 29 . Similarly, research has highlighted the role of demographic and socio-economic variables, including age, gender, and ethnicity 12 , 19 , 25 , 27 , 30 as well as socio-economic status 31 in predicting a students’ likelihood of persisting. For example, women are more likely to continue their studies than men 12 , 30 , 32 , 33 while White and Asian students are more likely to persist than students from other ethnic groups 19 , 27 , 30 . Moreover, a student’s socio-economic status and immediate financial situation have been shown to impact retention. Students are more likely to discontinue their studies if they are first generation students 34 , 35 , 36 or experience high levels of financial distress, (e.g., due to student loans or working nearly full time to cover college expenses) 37 , 38 . In contrast, students who receive financial support that does not have to be repaid post-graduation are more likely to complete their degree 39 , 40 .
While most of the outlined predictors of student retention are relatively stable intrapersonal characteristics and oftentimes difficult or costly to change, research also points to a more malleable pillar of retention: the students’ experience at university. In particular, the extent to which they are successfully integrated and socialized into the institution 16 , 22 , 41 , 42 . As Bean (2005) notes, “few would deny that the social lives of students in college and their exchanges with others inside and outside the institution are important in retention decisions” (p. 227) 41 . The extent to which a student is socially integrated and embedded into their institution has been studied in a number of ways, relating retention to the development of friendships with fellow students 43 , the student’s position in the social networks 16 , 29 , the experience of social connectedness 44 and a sense of belonging 42 , 45 , 46 . Taken together, these studies suggest that interactions with peers as well as faculty and staff – for example through participation in campus activities, membership of organizations, and the pursuit of extracurricular activities – help students better integrate into university life 44 , 47 . In contrast, a lack of social integration resulting from commuting (i.e., not living on campus with other students) has shown to negatively impact a student’s chances of completing their degree 48 , 49 , 50 , 51 . In short, the stronger a student is embedded and feels integrated into the university community – particularly in their first year – the less likely the student will drop out 42 , 52 .
Predicting retention using machine learning
A large proportion of research on student attrition has focused on understanding and explaining drivers of student retention. However, alongside the rise of computational methods and predictive modeling in the social sciences 53 , 54 , 55 , educational researchers and practitioners have started exploring the feasibility and value of data-driven approaches in supporting institutional decision making and educational effectiveness (for excellent overviews of the growing field see 56 , 57 ). In line with this broader trend, a growing body of work has shown the potential of predicting student dropout with the help of machine learning. In contrast to traditional inferential approaches, machine learning approaches are predominantly concerned with predictive performance (i.e., the ability to accurately forecast behavior that has not yet occurred) 54 . In the context of student retention this means: How accurately can we predict whether a student is going to complete or discontinue their studies (in the future) by analyzing their demographic and socio-economic characteristics, their past and current academic performance, as well as their current embeddedness in the university system and culture?
Echoing the National Academy of Education’s statement (2017) that “in the educational context, big data typically take the form of administrative data and learning process data, with each offering their own promise for educational research” (p.4) 58 , the vast majority of existing studies have focused on the prediction of student retention from demographic and socio-economic characteristics as well as students’ academic history and current performance 13 , 59 , 60 , 61 , 62 , 63 , 64 , 65 , 66 . In a recent study, Aulck and colleagues trained a model on the administrative data of over 66,000 first-year students enrolled in a public US university (e.g., race, gender, highschool GPA, entrance exam scores and early college performance/transcript data) to predict whether they would re-enroll in the second year and eventually graduate 59 . Specifically, they used a range of linear and non-linear machine learning models (e.g., regularized logistic regression, k-nearest neighbor, random forest, support vector machine, and gradient boosted trees) to predict retention out-of-sample using a standard cross-validation procedures. Their model was able to predict dropouts with an accuracy of 88% and graduation with an accuracy of 81% (where 50% is chance).
While the existing body of work provides robust evidence for the potential of predictive models for identifying at-risk students, it is based on similar sets of macro-level data (e.g., institutional data, academic performance) or micro-level data (e.g., click-stream data). Almost entirely absent from this research is data on students’ daily experience and engagement with both other students and the university itself (meso-level). Although there have been a small number of studies trying to capture part of this experience by inferring social networks from smart card transactions that were made by students in the same time and place 16 or engagement metrics with an open online course 67 , none of the existing work has offered a more holistic and comprehensive view on students’ daily experience. One potential explanation for this gap is that information about students’ social interactions with classmates or their day-to-day engagement with university services and events is difficult to track. While universities often have access to demographic or socio-economic variables through their Student Information Systems (SISs), and can easily track their academic performance, most universities do not have an easy way of capturing student’s deeper engagement with the system.
Research overview
In this research, we partner with an educational software company – READY Education – that offers a virtual one-stop interaction platform in the form of a smartphone application to facilitate communication between students, faculty, and staff. Students receive relevant information and announcements, can manage their university activities, and interact with fellow students in various ways. For example, the app offers a social media experience like Facebook, including private messaging, groups, public walls, and friendships. In addition, it captures students’ engagement with the university asking them to check into events (e.g., orientation, campus events, and student services) using QR code functionality and prompting them to rate their experience afterwards (see Methods for more details on the features we extracted from this data). As a result, the READY Education app allows us to observe a comprehensive set of information about students that include both (i) institutional data (i.e., demographic, and socio-economic characteristics as well as academic performance), and (ii) their idiosyncratic experience at university captured by their daily interactions with other students and the university services/events. Combining the two data sources captures a student’s profile more holistically and makes it possible to consider potential interactions between the variable sets. For example, being tightly embedded in a social support network of friends might be more important for retention among first-generation students who might not receive the same level of academic support or learn about implicit academic norms and rules from their parents.
Building on this unique dataset, we use machine learning models to predict student retention (i.e., dropout) from both institutional and behavioral engagement data. Given the desire to identify at-risk students as early as possible, we only use information gathered in the students’ first semester to predict whether the student dropped out at any point in time during their program. To thoroughly validate and scrutinize our analytical approach, generate insights for potential interventions, and probe the generalizability of our predictive models across different universities, we investigate the following three research questions:
How accurately can we predict a student's likelihood of discontinuing their studies using information from the first term of their studies (i.e., institutional data, behavioral engagement data, and a combination of both)?
Which features are the most predictive of student retention?
How well do the predictive models generalize across universities (i.e., how well can we predict student retention of students from one university if we use the model trained on data from another university and vice versa)?
Participants
We analyze de-identified data from four institutions with a total of 50,095 students (min = 476, max = 45,062). All students provided informed consent to the use of the anonymized data by READY Education and research partners. All experimental protocols were approved by the Columbia University Ethics Board, and all methods carried out were in accordance with the Board’s guidelines and regulations. The data stem from two sources: (a) Institutional data and (b) behavioral engagement data. The institutional data collected by the universities contain socio-demographics (e.g., gender, ethnicity), general study information (e.g., term of admission, study program), financial information (e.g., pell eligibility), students’ academic achievement scores (e.g., GPA, ACT) as well as the retention status. The latter indicated whether students continued or dropped out and serves as the outcome variable. As different universities collect different information about their students, the scope of institutional data varied between universities. Table 1 provides a descriptive overview of the most important sociodemographic characteristics for each of the four universities. In addition, it provides a descriptive overview of the app usage, including the average number of logs per student, the total number of sessions and logs, as well as the percentage of students in a cohort using the app (i.e., coverage). The broad coverage of students using the app, ranging between 70 and 98%, results in a largely representative sample of the student populations at the respective universities.
Notably, Universities 1–3 are traditional university campuses, while University 4 is a combination of 16 different community colleges. Given that there is considerable heterogeneity across campuses, the predictive accuracies for University 4 are a-priori expected to be lower than those observed for universities 1–3 (and partly speak to the generalizability of findings already). The decision to include University 4 as a single entity was based on the fact that separating out the 16 colleges would have resulted in an over-representation of community colleges that all share similar characteristics thereby artificially inflating the observed cross-university accuracies. Given these limitations (and the fact that the University itself collapsed the college campuses for many of their internal reports), we decided to analyze it as a single unit, acknowledging that this approach brings its own limitations.
The behavioral engagement data were generated through the app (see Table 1 for the specific data collection windows at each University). Behavioral engagement data were available in the form of time-stamped event-logs (i.e., each row in the raw data represented a registered event such as a tab clicked, a comment posted, a message sent). Each log could be assigned to a particular student via an anonymized, unique identifier. Across all four universities, the engagement data contained 7,477,630 sessions (Mean = 1,869,408, SD = 3,329,852) and 17,032,633 logs (Mean = 4,258,158, SD = 6,963,613) across all universities. For complete overview of all behavioral engagement metrics including a description see Table S1 in the Supplementary Materials.
Pre-processing and feature extraction
As a first step, we cleaned both the institutional and app data. For the institutional data, we excluded students who did not use the app and therefore could not be assigned a unique identifier. In addition, we excluded students without a term of admission to guarantee that we are only observing the students’ first semester. Lastly, we removed duplicate entries resulting from dual enrollment in different programs. For the app usage data, we visually inspected the variables in our data set for outliers that might stem from technical issues. We pre-processed data that reflected clicking through the app, named “clicked_[…]” and “viewed_[…]” (see Table S1 in the Supplementary Materials). A small number of observations showed unrealistically high numbers of clicks on the same tab in a very short period, which is likely a reflection of a student repeatedly clicking on a tab due to long loading time or other technical issues. To avoid oversampling these behaviors, we removed all clicks of the same type which were made by the same person less than one minute apart.
We extracted up to 462 features for each university across two broad categories: (i) institutional features and (ii) engagement features, using evidence from previous research as a reference point (see Table S2 in the Supplementary Materials for a comprehensive overview of all features and their availability for each of the universities). Institutional features contain students’ demographic, socio-economic and academic information. The engagement features represent the students’ behavior during their first term of studies. They can be further divided into app engagement and community engagement. The app engagement features represent the students’ behavior related to app usage, such as whether the students used the app before the start of the semester, how often they clicked on notifications or the community tabs, or whether their app use increased over the course of the semester. The community involvement features reflect social behavior and interaction with others, e.g., the number of messages sent, posts and comments made, events visited or a student’s position in the network as inferred from friendships and direct messages. Importantly, many of the features in our dataset will be inter-correlated. For example, living in college accommodation could signal higher levels of socio-economic status, but also make it more likely that students attend campus events and connect with other students living on campus. While intercorrelations among predictors is a challenge with standard inferential statistical techniques such as regression analyses, the methods we apply in this paper can account for a large number of correlated predictors.
Institutional features were directly derived from the data recorded by the institutions. As noted above, not all features were available for all universities, resulting in slightly different feature sets across universities. The engagement features were extracted from the app usage data. As we focused on an early prediction of drop-out, we restricted the data to event-logs that were recorded in the respective students' first term. Notably, the data captures students’ engagement as a time-stamped series of events, offering fine-grained insights into their daily experience. For reasons of simplicity and interpretability (see research question 2), we collapse the data into a single entry for each student. Specifically, we describe a student’s overall experience during the first semester, by calculating distribution measures for each student such as the arithmetic mean, standard deviation, kurtosis, skewness, and sum values. For example, we calculate how many daily messages a particular student sent or received during their first semester, or how many campus events they attended in total. However, we also account for changes in a student’s behavior over time by calculating more complex features such as entropy (e.g., the extent to which a person has frequent contact with few people or the same degree of contact with many people) and the development of specific behaviors over time measured by the slope of regression analyses, as well as features representing the regularity of behavior (e.g., the deviation of time between sending messages). Overall, the feature set was aimed at describing a student’s overall engagement with campus resources and other students during the first semester as well as changed in engagement over time. Finally, we extracted some of the features separately for weekdays and weekends to account for differences and similarities in students’ activities during the week and the weekend. For example, little social interaction on weekdays might predict retention differently than little social interaction on the weekend.
We further cleaned the data by discarding participants for whom the retention status was missing and those in which 95% or more of the values were zero or missing. Furthermore, features were removed if they showed little or no variance across participants, which makes them essentially meaningless in a prediction task. Specifically, we excluded numerical features which showed the same values for more than 90% of observations and categorical features which showed the same value for all observations.
In addition to these general pre-processing procedures, we integrated additional pre-processing steps into the resampling prior to training the models to avoid an overestimation of model performance 68 . To prevent problems with categorical features that occur when there are fewer levels in the test than in the training data, we first removed categories that did not occur in the training data. Second, we removed constant categorical features containing a single value only (and therefore no variation). Third, we imputed missing values using the following procedures: Categorical features were imputed with the mode. Following commonly used approaches to dealing with missing data, the imputation of numeric features varied between the learners. For the elastic net, we imputed those features with the median. For the random forest, we used twice the maximum to give missing values a distinct meaning that would allow the model to leverage this information. Lastly, we used the "Synthetic Minority Oversampling Technique" (SMOTE) to create artificial examples for the minority class in the training data 69 . The only exception was University 4 which followed a different procedure due to the large sample size and estimated computing power for implementing SMOTE. Instead of oversampling minority cases, we downsampled majority cases such that the positive and negative class were balanced. This was done to address the class imbalance caused by most students continuing their studies rather than dropping out 12 .
Predictive modeling approach
We predicted the retention status (1 = dropped out, 0 = continued) in a binary prediction task, with three sets of features: (1) institutional features (2) engagement features, and (3) a combined set of all features. To ensure the robustness of our predictions and to identify the model which is best suited for the current prediction context 54 , we compared a linear classifier ( elastic net; implemented in glmnet 4.1–4) 70 , 71 and a nonlinear classifier ( random forest ; implemented in randomForest 4.7–1) 72 , 73 . Both models are particularly well suited for our prediction context and are common choices in computational social science. That is, simple linear or logistic regression models are not suitable to work with datasets that have many inter-correlated predictors (in our case, a total of 462 predictors many of which are highly correlated) due to a high risk of overfitting. Both the elastic net and the random forest algorithm can effectively utilize large feature sets while reducing the risk of overfitting. We evaluate the performance of our six models for each school (2 algorithms and 3 feature sets), using out-of-sample benchmark experiments that estimate predictive performance and compare it against a common non-informative baseline model. The baseline represents a null-model that does not include any features, but instead always predicts the majority class, which in our samples means “continued.” 74 Below, we provide more details about the specific algorithms (i.e., elastic net and random forest), the cross-validation procedure, and the performance metrics we used for model evaluation.
Elastic net model
The elastic net is a regularized regression approach that combines advantages of ridge regression 75 with those of the LASSO 76 and is motivated by the need to handle large feature sets. The elastic net shrinks the beta-coefficients of features that add little predictive value (e.g., intercorrelated, little variance). Additionally, the elastic net can effectively remove variables from the model by reducing the respective beta coefficients to zero 70 . Unlike classical regression models, the elastic net does not aim to optimize the sum of least squares, but includes two penalty terms (L1, L2) that incentivize the model to reduce the estimated beta value of features that do not add information to the model. Combining the L1 (the sum of absolute values of the coefficients) and L2 (the sum of the squared values of the coefficients) penalties, elastic net addresses the limitations of alternative linear models such as LASSO regression (not capable of handling multi-collinearity) and Ridge Regression (may not produce sparse-enough solutions) 70 .
Formally, following Hastie & Qian (2016) the model equation of elastic net for binary classification problems can be written as follows 77 . Suppose the response variable takes values in G = {0,1}, y i denoted as I(g i = 1), the model formula is written as
After applying the log-odds transformation, the model formula can be written as
The objective function for logistic regression is the penalized negative binomial log-likelihood
where λ is the regularization parameter that controls the overall strength of the regularization, α is the mixing parameter that controls the balance between L1 and L2 regularization with α values closer to zero to result in sparser models (lasso regression α = 1, ridge regression α = 0). β represents coefficients of the regression model, ||β|| 1 is the is the L1 norm of the coefficients (the sum of absolute values of the coefficients), ||β|| 2 is the L2 norm of the coefficients (the sum of the squared values of the coefficients).
The regularized regression approach is especially relevant for our model because many of the app-based engagement features are highly correlated (e.g., the number of clicks is related to the number of activities registered in the app). In addition, we favored the elastic net algorithm over more complex alternatives, because the regularized beta coefficients can be interpreted as feature importance, allowing insights into which predictors are most informative of college dropout 78 , 79 .
Random forest model
Random forest models are a widely used ensemble learning method that grows many bagged and decorrelated decision trees to come up with a “collective” prediction of the outcome (i.e., the outcome that is chosen by most trees in a classification problem) 72 . Individual decision trees recursively split the feature space (rules to distinguish classes) with the goal to separate the different classes of the criterion (drop out vs. remain in our case). For a detailed description of how individual decision trees operate and translate to a random forest see Pargent, Schoedel & Stachl 80 .
Unlike the elastic net, random forest models can account for nonlinear associations between features and criterion and automatically include multi-dimensional interactions between features. Each decision tree in a random forest considers a random subset of bootstrapped cases and features, thereby increasing the variance of predictions across trees and the robustness of the overall prediction. For the splitting in each node of each tree, a random subset of features (mtry hyperparameter that we optimize in our models) are used by randomly drawing from the total set. For each split, all combinations of split variables and split points are compared, with the model choosing the splits that optimize the separation between classes 72 .
The random forest algorithm can be formally described as follows (verbatim from Hastie et al., 2016, p. 588):
For b = 1 to B:
Draw a bootstrap sample of size N from the training data.
Grow a decision tree to the bootstrapped data, by recursively repeating the following steps for each terminal node of the tree, until the minimum node size is reached.
Select m variables at random from the p variables.
Pick the best variable/split-point among the m according to the loss function (in our case Gini-impurity decrease)
Split the node into two daughter nodes.
Output the ensemble of trees
New predictions can then be made by generating a prediction for each tree and aggregating the results using majority vote.
The aggregation of predictions across trees in random forests improves the prediction performance compared to individual decision trees, as it can benefit from the trees’ variance and greatly reduces it to arrive at a single prediction 72 , 81 .
(Nested) Cross-validation: Out-of-sample model evaluation
We evaluate the performance of our predictive models using an out-of-sample validation approach. The idea behind out-of-sample validation is to increase the likelihood that a model will accurately predict student dropout on new data (e.g. new students) by using different datasets when training and evaluating the model. A commonly used, efficient technique for out-of-sample validation is to repeatedly fit (cf. training) and evaluate (cf. testing) models on non-overlapping parts of the same datasets and to combine the individual estimates across multiple iterations. This procedure – known as cross-validation – can also be used for model optimization (e.g., hyperparameter-tuning, pre-processing, variable selection), by repeatedly evaluating different settings for optimal predictive performance. When both approaches are combined, evaluation and optimization steps need to be performed in a nested fashion to ensure a strict separation of training and test data for a realistic out-of-sample performance estimation. The general idea is to emulate all modeling steps in each fold of the resampling as if it were a single in-sample model. Here, we use nested cross-validation to estimate the predictive performance of our models, to optimize model hyperparameters, and to pre-process data. We illustrate the procedure in Fig. 1 .
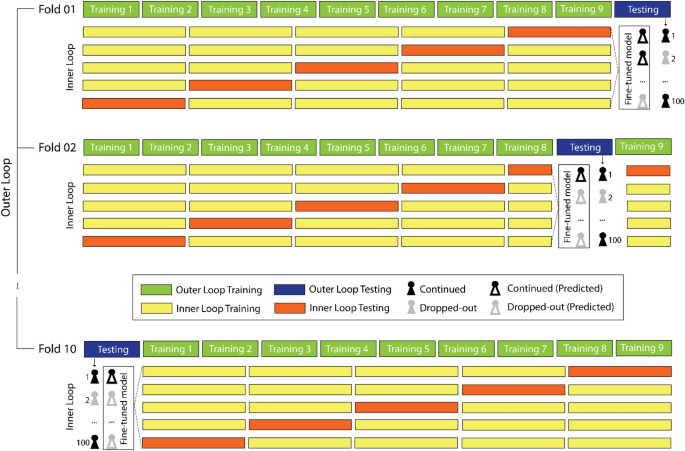
Schematic cross-validation procedure for out-of-sample predictions. The figure shows a tenfold cross-validation in the outer loop which is used to estimate the overall performance of the model by comparing the predicted outcomes for each student in the previously unseen test set with their actual outcomes. Within each of the 10 outer loops, a fivefold cross-validation in the inner loop is used to finetune model hyperparameters by evaluating different model settings.
The cross-validation procedure works as follows: Say we have a dataset with 1,000 students. In a first step, the dataset is split into ten different subsamples, each containing data from 100 students. In the first round, nine of these subsamples are used for training (i.e., fitting the model to estimate parameters, green boxes). That means, the data from the first 900 students will be included in training the model to relate the different features to the retention outcome. Once training is completed, the model’s performance can be evaluated on the data of the remaining 100 students (i.e., test dataset, blue boxes). For each student, the actual outcome (retained or discontinued, grey and black figures) is compared to the predicted outcome (retained or discontinued, grey and black figures). This comparison allows for the calculation of various performance metrics (see “ Performance metrics ” section below for more details). In contrast to the application of traditional inferential statistics, the evaluation process in predictive models separates the data used to train a model from the data used to evaluate these associations. Hence any overfitting that occurs at the training stage (e.g., using researcher degrees of freedom or due to the model learning relationships that are unique to the training data), hurts the predictive performance in the testing stage. To further increase the robustness of findings and leverage the entire dataset, this process is repeated for all 10 subsamples, such that each subsample is used nine times for training and once for testing. Finally, the obtained estimates from those ten iterations are aggregated to arrive at a cross-validated estimate of model performance. This tenfold cross validation procedure is referred to as the “outer loop”.
In addition to the outer loop, our models also contain an “inner loop”. The inner loop consists of an additional cross-validation procedure that is used to identify ideal hyperparameter settings (see “ Hyperparameter tuning ” section below). That is, in each of the ten iterations of the outer loop, the training sample is further divided into a training and test set to identify the best parameter constellations before model evaluation in the outer loop. We used fivefold cross-validation in the inner loop. All analyses scripts for the pre-processing and modeling steps are available on OSF ( https://osf.io/bhaqp/?view_only=629696d6b2854aa9834d5745425cdbbc ).
Performance metrics
We evaluate model performance based on four different metrics. Our main metric for model performance is AUC (area under the received operating characteristics curve). AUC is commonly used to assess the performance of a model over a 50%-chance baseline, and can range anywhere between 0 and 1. The AUC metric captures the area under the receiver operating characteristic (ROC) curve, which plots the true positive rate (TPR or recall; i.e. the percentage of correctly classified dropouts among all students who actually dropped out), against the false positive rate (FPR; i.e. the percentage of students erroneously classified as dropouts among all the students who actually continued). When the AUC is 0.5, the model’s predictive performance is equal to chance or a coin flip. The closer to 1, the higher the model’s predictive performance in distinguishing between students who continued and those who dropped out.
In addition, we report the F1 score, which ranges between 0 and 1 82 . The F1 score is based on the model’s positive predictive value (or precision, i.e., the percentage of correctly classified dropouts among all students predicted to have dropped out) as well as the model's TPR. A high F1 score hence indicates that there are both few false positives and few false negatives.
Given the specific context, we also report the TPR and the true negative rates (TNR, i.e. the percentage of students predicted to continue among all students who actually continued). Depending on their objective, universities might place a stronger emphasis on optimizing the TPR to make sure no student who is at risk of dropping out gets overlooked or on optimizing the TNR to save resources and assure that students do not get overly burdened. Notably, in most cases, universities are likely to strive for a balance between the two, which is reflected in our main AUC measure. All reported performance metrics represent the mean predictive performance across the 10 cross-validation folds of the outer loop 54 .
Hyperparameter tuning
We used a randomized search with 50 iterations and fivefold cross-validation for hyperparameter tuning in the inner loop of our cross-validation. The randomized search algorithm fits models with hyperparameter configurations randomly selected from a previously defined hyperparameter space and then picks the model that shows the best generalized performance averaged over the five cross-validation folds. The best hyperparamter configuration is used for training in the outer resampling loop to evaluate model performance.
For the elastic net classifier, we tuned the regularization parameter lambda, the decision rule used to choose lambda, and the L1-ratio parameter. The search space for lambda encompassed the 100 glmnet default values 71 . The space of decision rules for lambda included lambda.min which chooses the value of lambda that results in the minimum mean cross-validation error, and lambda.1se which chooses the value of lambda that results in the most regularized model such that the cross-validation error remains within one standard error of the minimum. The search space for the L1-ratio parameter included the range of values between 0 (ridge) to 1 (lasso). For the random forest classifier, we tuned the number of features selected for each split within a decision tree (mtry) and the minimum node size (i.e., how many cases are required to be left in the resulting end-nodes of the tree). The search space for the number of input features per decision tree was set to a range of 1 to p, where p represents the dimensionality of the feature space. The search space for minimum node size was set to a range of 1 to 5. Additionally, for both models, we tuned the oversampling rate and the number or neighbors used to generate new samples utilized by the SMOTE algorithm. The oversampling rate was set to a range of 2 to 15 and the number of nearest neighbors was set to a range of 1 to 10.
RQ1: How accurately can we predict a student's likelihood of discontinuing their studies using information from the first term of their studies?
Figure 2 displays AUC scores (Y-axis) across the different universities (rows), separated by the different feature sets (colors) and predictive algorithms (X-axis labels). The figure displays the distribution of AUC accuracies across the 10 cross-validation folds, alongside their mean and standard deviation. Independent t-tests using Holm corrections for multiple comparisons indicate statistical differences in the predictive performance across the different models and feature sets within each university. Table 2 provides the predictive performance across all four metrics.

AUC performance across the four universities for different feature sets and model. Inst. = Institutional data. Engag. = Engagement data. (EN) = Elastic Net. (RF) = Random Forest.
Overall, our models showed high levels of predictive accuracies across universities, models, feature sets and performance metrics, significantly outperforming the baseline in all instances. The main performance metric AUC reached an average of 73% (where 50% is chance), with a maximum of 88% for the random forest model and the full feature set in University 1. Both institutional features and engagement features significantly contributed to predictive performance, highlighting the fact that a student’s likelihood to drop out is both a function of their more stable socio-demographic characteristics as well as their experience of campus life. In most cases, the joint model (i.e., the combination of institutional and engagement features) performed better than each of the individual models alone. Finally, the random forest models produced higher levels of predictive performance than the elastic net in most cases (average AUC elastic net = 70%, AUC random forest = 75%), suggesting that the features are likely to interact with one another in predicting student retention and might not always be linearly related to the outcome.
RQ2: Which features are the most predictive of student retention?
To provide insights into the underlying relationships between student retention and socio-demographic as well as behavioral features, we examined two indicators of feature importance that both offer unique insights. First, we calculated the zero-order correlations between the features and the outcome for each of the four universities. We chose zero-order correlations over elastic net coefficients as they represent the relationships unaltered by the model’s regularization procedure (i.e., the relationship between a feature and the outcome is shown independently of the importance of the other features in the model). To improve the robustness of our findings, we only included the variables that passed the threshold for data inclusion in our models and had less than 50% of the data imputed. The top third of Table 3 displays the 10 most important features (i.e., highest absolute correlation with retention). The sign in brackets indicates the direction of the effects with ( +) indicating a protective factor and (−) indicating a risk factor. Features that showed up in the top 10 for more than 1 university are highlighted in bold.
Second, we calculated permutation variable importance scores for the elastic net and random forest models. For the elastic net model, feature importance is reported as the model coefficient after shrinking the coefficients according to their incremental predictive power. Compared to the zero-order correlation, the elastic net coefficients hence identify the features that have the strongest unique variance. For the random forest models, feature importance is reported as a model-agnostic metric that estimates the importance of a feature by observing the drop in model predictive performance when the actual association between the feature and the outcome is broken by randomly shuffling observations 72 , 83 . A feature is considered important if shuffling its values increases the model error (and therefore decreases the model’s predictive performance). In contrast to the coefficients from the elastic net model, the permutation feature importance scores are undirected and do not provide insights into the specific nature of the relationship between the feature and the outcome. However, they account for the fact that some features might not be predictive themselves but could still prove valuable in the overall model performance because they moderate the impact of other features. For example, minority or first-generation students might benefit more from being embedded in a strong social network than majority students who do not face the same barriers and are likely to have a stronger external support network. The bottom of Table 3 displays the 10 most important features in the elastic net and random forest models (i.e., highest permutation variable importance).
Supporting the findings reported in RQ1, the zero-order correlations confirm that both institutional and behavioral engagement features play an important role in predicting student retention. Aligned with prior work, students’ performance (measured by GPA or ACT) repeatedly appeared as one of the most important predictors across universities and models. In addition, many of the engagement features (e.g., services attended, chat messages network centrality) are related to social activities or network features, supporting the notion that a student’s social connections and support play a critical role in student retention. In addition, the extent to which students are positively engaged with their institutions (e.g., by attending events and rating them highly) appears to play a critical role in preventing dropout.
RQ3: How well do the predictive models generalize across universities?
To test the generalizability of our models across universities, we used the predictive model trained on one university (e.g., University 1) to predict retention of the remaining three universities (e.g., Universities 2–4). Figures 3 A,B display the AUCs across all possible pairs, indicating which university was used for training (X-axis) and which was used for testing (Y-axis, see Figure S1 in the SI for graphs illustrating the findings for F1, TNR and TPR).
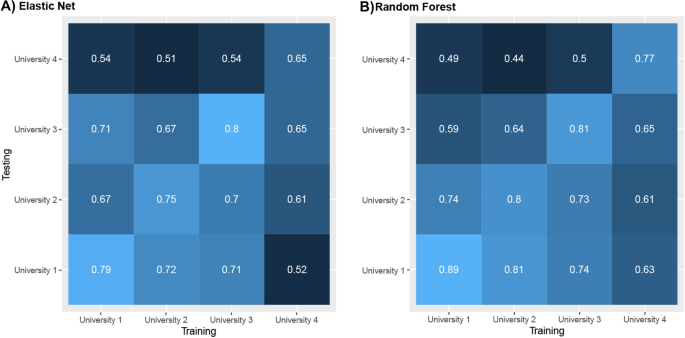
Performance (average AUC) of cross-university predictions.
Overall, we observed reasonably high levels of predictive performance when applying a model trained on one university to the data of another. The average AUC observed was 63% (for both the elastic net and the random forest), with the highest predictive performance reaching 74% (trained on University 1, predicting University 2), just 1%-point short of the predictive performance observed for the prediction from the universities own model (trained on University 2, predicting University 2). Contrary to the findings in RQ1, the random forest models did not perform better than the elastic net when making predictions for other universities. This suggests that the benefits afforded by the random forest models capture complex interaction patterns that are somewhat unique to each university but might not generalize well to new contexts. The main outlier in generalizability was University 4, where none of the other models reached accuracies much better than chance, and whose model produced relatively low levels of accuracies when predicting student retention across universities 1–2. This is likely a result of the fact that University 4 was qualitatively different from the other universities in several ways, including the fact that University 4 was a community college and consisted of 16 different campuses that were merged for the purpose of this analysis (see Methods for more details).
We show that student retention can be predicted from institutional data, behavioral engagement data, and their combination. Using data from over 50,000 students across four Universities, our predictive models achieve out-of-sample accuracies of up to 88% (where 50% is chance). Notably, while both institutional data and behavioral engagement data significantly predict retention, the combination of the two performs best in most instances. This finding is further supported by our feature importance analyses which suggest that both institutional and behavioral engagement features are among the most important predictors of student retention. Specifically, academic performance as measured by GPA and behavioral metrics associated with campus engagement (e.g., event attendances or ratings) or a student’s position in the network (e.g., closeness or centrality) were shown to consistently act as protective factors. Finally, we highlight the generalizability of our models across universities. Models trained on one university were able to predict student retention at another with reasonably high levels of predictive performance. As one might expect, the generalizability across universities heavily depends on the extent to which the universities are similar on important structural dimensions, with prediction accuracies dropping radically in cases where similarity is low (see low cross-generalization for University 4).
Contributions to the scientific literature
Our findings contribute to the existing literature in several ways. First, they respond to recent calls for more predictive research in psychology 54 , 55 as well as the use of Big Data analytics in education research 56 , 57 . Not only do our models consider socio-demographic characteristics that are collected by universities, but they also capture students’ daily experience and university engagement by tracking behaviors via the READY Education app. Our findings suggest, these more psychological predictors of student retention can improve the performance of predictive models above and beyond socio-demographic variables. This is consistent with previous findings suggesting that the inclusion of engagement metrics improves the performance of predictive models 16 , 84 , 85 . Overall, our models showed superior accuracies to models of former studies that were trained only on demographics and transcript records 15 , 25 or less comprehensive behavioral features 16 and provided results comparable to those reported in studies that additionally included a wide range of socio-economic variables 12 . Given that the READY Education app captures only a fraction of the students' actual experience, the high predictive accuracies make an even stronger case for the importance of student engagement in college retention.
Second, our findings provide insights into the features that are most important in predicting whether a student is going to drop out or not. By doing so they complement our predictive approach with layers of understanding that are conducive to not only validating our models but also generating insights into potential protective and risk factors. Most importantly, our findings highlight the relevance of the behavioral engagement metrics for predicting student retention. Most features identified as being important in the prediction were related to app and community engagement. In line with previous research, features indicative of early and deep social integration, such as interactions with peers and faculty or the development of friendships and social networks, were found to be highly predictive 16 , 41 . For example, it is reasonable to assume that a short time between app registration and the first visit of a campus event (one of the features identified as important) has a positive impact on retention, because campus events offer ideal opportunities for students to socialize 86 . Early participation in a campus event implies early integration and networking with others, protecting students from perceived stress 87 and providing better social and emotional support 88 . In contrast, a student who never attends an event or does so very late in the semester may be less connected to the campus life and the student community which in turn increases the likelihood of dropping out. This interpretation is strengthened by the fact that a high proportion of positive event ratings was identified as an important predictor of a student continuing their studies. Students who enjoy an event are likely to feel more comfortable, be embedded in the university life, make more connections, and build stronger connections. This might result in a virtuous cycle in which students continue attending events and over time create a strong social connection to their peers. As in most previous work, a high GPA score was consistently related to a higher likelihood of continuing one’s studies 21 , 24 . Although their importance varied across universities, ethnicity was also found to play a major role for retention, with consistent inequalities replicating in our predictive models 12 , 19 , 47 . For example, Black students were on average more likely to drop-out, suggesting that universities should dedicate additional resources to protect this group. Importantly, all qualitative interpretations are post-hoc. While many of the findings are intuitive and align with previous research on the topic, future studies should validate our results and investigate the causality underlying the effects in experimental or longitudinal within-person designs 54 , 78 .
Finally, our findings are the first to explore the extent to which the relationships between certain socio-demographic and behavioral characteristics might be idiosyncratic and unique to a specific university. By being able to compare the models across four different universities, we were able to show that many of the insights gained from one university can be leveraged to predict student retention at another. However, our findings also point to important boundary conditions: The more dissimilar universities are in their organizational structures and student experience, the more idiosyncratic the patterns between certain socio-demographic and behavioral features with student retention will be and the harder it is to merely translate general insights to the specific university campus.
Practical contributions
Our findings also have important practical implications. In the US, student attrition results in an average annual revenue loss of approximately $16.5 billion per year 9 , 10 and over $9 billion wasted in federal and state grants and subsidies that are awarded to students who do not finish their degree 11 . Hence, it is critical to predict potential dropouts as early and as accurately as possible to be able to offer dedicated support and allocate resources where they are needed the most. Our models rely exclusively on data collected in the first semester at university and are therefore an ideal “early warning” system for universities who want to predict whether their students will likely continue their studies or drop out at some point. Depending on the university’s resources and goals, the predictive models can be optimized for different performance measures. Indeed, a university might decide to focus on the true positive rate to capture as many dropouts as possible. While this would mean erroneously classifying “healthy “ students as potential dropouts, universities might decide that the burden of providing “unnecessary “ support to these healthy students is worth the reduced risk of missing a dropout. Importantly, our models go beyond mere socio-demographic variables and allow for a more nuanced, personal model that considers not just “who someone is” but also what their experience on campus looks like. As such, our models make it possible to acknowledge individuality rather than using over-generalized assessments of entire socio-demographic segments.
Importantly, however, it is critical to subject these models to continuous quality assurance. While predictive models could allow universities to flag at-risk students early, they could also perpetuate biases that get calcified in the predictive models themselves. For example, students who are traditionally less likely to discontinue their studies might have to pass a much higher level of dysfunctional engagement behavior before their file gets flagged as “at-risk”. Similarly, a person from a traditionally underrepresented group might receive an unnecessarily high volume of additional check-ins even though they are generally flourishing in their day-to-day experience. Given that being labeled as “at-risk” can be associated with stigma that could reinforce stigmas around historically marginalized groups, it will be critical to monitor both the performance of the model over time as well as the perception of its helpfulness among administrators, faculty, and students.
Limitations and future research
Our study has several limitations and highlights avenues for future research. First, our sample consisted of four US universities. Thus, our results are not necessarily generalizable to countries with more collectivistic cultures and other education systems such as Asia, where the reasons for dropping out might be different 89 , 90 , or Europe where most students work part-time jobs and live off-campus. Future research should investigate the extent to which our models can generalize to other cultural contexts and identify the features of student retention that are universally valid across contexts.
Second, our predictive models relied on app usage data. Therefore, our predictive approach could only be applied to students who decided to use the app. This selection, in and by itself, is likely to introduce a sampling bias, as students who decide to use the app might be more likely to retain in the first place, restricting the variance in observations, and excluding students for whom app usage data was not available. However, as our findings suggest, the institutional data alone provide predictive performance independent of the app features, making this a viable alternative for students who do not use the app.
Third, our predictive models rely on cross-sectional predictions. That is, we observe a students’ behavior over the course of an entire semester and based on the patterns observed in other students we predict whether that student is likely to drop out or not. Future research could try to improve both the predictive performance of the model and its usefulness for applied contexts by modeling within-person trends dynamically. Given enough data, the model could observe a person’s baseline behavior and identify changes from that baseline as potentially problematic. In fact, more social contact with other students might be considered a protective factor in our cross-sectional model. However, there are substantial individual differences in how much social contact individuals seek out and enjoy 91 . Hence, sending 10 chat messages a week might be considered a lot for one person, but very little for another. Future research should hence investigate whether the behavioral engagement features allow for a more dynamic within-person model that makes it possible to take base rates into account and provide a dynamic, momentary assessment of a student’s likelihood to drop out.
Fourth, although the engagement data was captured as a longitudinal time series with time-stamped events, we collapsed the data into a single set of cross-sectional features for each student. Although some of these features captures variation in behaviors over time (e.g., entropy and linear trends), future research should try to implement more advanced machine learning models to account for this time series data directly. For example, long short-term memory models (LSTMs) 92 – a type of recurrent neural network – are capable of learning patterns in longitudinal, sequential data like ours.
Fifth, even though the current research provides initial insights into the workings of the models by highlighting the importance of certain features, the conclusions that can be drawn from these analyses are limited as the importance metrics are calculated for the overall population. Future research could aim to calculate the importance of certain features at the individual level to test whether their importance varies across certain socio-demographic features. Estimating the importance of a person’s position in the social network on an individual level, for example, would make it possible to see whether the importance is correlated with institutional data such as minority or first-generation status.
Finally, our results lay the foundation for developing interventions that foster retention through shaping students’ experience at university 93 . Interventions which have been shown to have a positive effect on retention, include orientation programs and academic advising 94 , student support services like mentoring and coaching as well as need-based grants 95 . However, to date, the first-year experience programs meant to strengthen social integration of first year students, do not seem to have yielded positive results 96 , 97 . Our findings could support the development of interventions aimed at improving and maintaining student integration on campus. On a high level, the insights into the most important features provide an empirical path for developing relevant interventions that target the most important levers of student retention. For example, the fact that the time between registration and the first event attendance has such a big impact on student retention means that universities should do everything they can to get students to attend events as early as possible. Similarly, they could develop interventions that lead to more cohesive networks among cohorts and make sure that all students connect to their community. On a deeper, more sophisticated level, new approaches to model explainability could allow universities to tailor their intervention to each student 98 , 99 . For example, explainable AI makes it possible to derive decision rules for each student, indicating which features were critical in predicting the students’ outcome. While student A might be predicted to drop out because they are disconnected from the network, student B might be predicted to drop out because they don’t access the right information on the app. Given this information, universities would be able to personalize their offerings to the specific needs of the student. While student A might be encouraged to spend more time socializing with other students, student B might be reminded to check out important course information. Hence, predictive models could not only be used to identify students at risk but also provide an automated path to offering personalized guidance and support.
For every study that is discontinued, an educational dream shatters. And every shattered dream has a negative long-term impact both on the student and the university the student attended. In this study we introduce an approach to accurately predicting student retention after the first term. Our results show that student retention can be predicted with relatively high levels of predictive performance when considering institutional data, behavioral engagement data, or a combination of the two. By combining socio-demographic characteristics with passively observed behavioral traces reflecting a student’s daily activities, our models offer a holistic picture of students' university experiences and its relation to retention. Overall, such predictive models have great potential both for the early identification of at-risk students and for enabling timely, evidence-based interventions.
Data availability
Raw data are not publicly available due to their proprietary nature and the risks associated with de-anonymization, but they are available from the corresponding author on reasonable request. The pre-processed data and all analyses codes are available on OSF ( https://osf.io/bhaqp/ ) to facilitate reproducibility of our work. Data were analyzed using R, version 4.0.0 (R Core Team, 2020; see subsections for specific packages and versions used). The study’s design relies on secondary data and the analyses were not preregistered.
Change history
21 june 2023.
A Correction to this paper has been published: https://doi.org/10.1038/s41598-023-36579-2
Ginder, S. A., Kelly-Reid, J. E. & Mann, F. B. Graduation Rates for Selected Cohorts, 2009–14; Outcome Measures for Cohort Year 2009–10; Student Financial Aid, Academic Year 2016–17; and Admissions in Postsecondary Institutions, Fall 2017. First Look (Provisional Data). NCES 2018–151. National Center for Education Statistics (2018).
Snyder, T. D., de Brey, C. & Dillow, S. A. Digest of Education Statistics 2017 NCES 2018-070. Natl. Cent. Educ. Stat. (2019).
NSC Research Center. Persistence & Retention – 2019. NSC Research Center https://nscresearchcenter.org/snapshotreport35-first-year-persistence-and-retention/ (2019).
Bound, J., Lovenheim, M. F. & Turner, S. Why have college completion rates declined? An analysis of changing student preparation and collegiate resources. Am. Econ. J. Appl. Econ. 2 , 129–157 (2010).
Article PubMed PubMed Central Google Scholar
Bowen, W. G., Chingos, M. M. & McPherson, M. S. Crossing the finish line. in Crossing the Finish Line (Princeton University Press, 2009).
McFarland, J. et al. The Condition of Education 2019. NCES 2019-144. Natl. Cent. Educ. Stat. (2019).
Education, U. S. D. of. Fact sheet: Focusing higher education on student success. [Fact Sheet] (2015).
Freudenberg, N. & Ruglis, J. Peer reviewed: Reframing school dropout as a public health issue. Prev. Chronic Dis. 4 , 4 (2007).
Google Scholar
Raisman, N. The cost of college attrition at four-year colleges & universities-an analysis of 1669 US institutions. Policy Perspect. (2013).
Wellman, J., Johnson, N. & Steele, P. Measuring (and Managing) the Invisible Costs of Postsecondary Attrition. Policy brief. Delta Cost Proj. Am. Instit. Res. (2012).
Schneider, M. Finishing the first lap: The cost of first year student attrition in America’s four year colleges and universities (American Institutes for Research, 2010).
Delen, D. A comparative analysis of machine learning techniques for student retention management. Decis. Support Syst. 49 , 498–506 (2010).
Article Google Scholar
Yu, R., Lee, H. & Kizilcec, R. F. Should College Dropout Prediction Models Include Protected Attributes? in Proceedings of the Eighth ACM Conference on Learning@ Scale 91–100 (2021).
Tinto, V. Reconstructing the first year of college. Plan. High. Educ. 25 , 1–6 (1996).
Ortiz-Lozano, J. M., Rua-Vieites, A., Bilbao-Calabuig, P. & Casadesús-Fa, M. University student retention: Best time and data to identify undergraduate students at risk of dropout. Innov. Educ. Teach. Int. 57 , 74–85 (2020).
Ram, S., Wang, Y., Currim, F. & Currim, S. Using big data for predicting freshmen retention. in 2015 international conference on information systems: Exploring the information frontier, ICIS 2015 (Association for Information Systems, 2015).
Levitz, R. S., Noel, L. & Richter, B. J. Strategic moves for retention success. N. Dir. High. Educ. 1999 , 31–49 (1999).
Veenstra, C. P. A strategy for improving freshman college retention. J. Qual. Particip. 31 , 19–23 (2009).
Astin, A. W. How, “good” is your institution’s retention rate?. Res. High. Educ. 38 , 647–658 (1997).
Coleman, J. S. Social capital in the creation of human capital. Am. J. Sociol. 94 , S95–S120 (1988).
Reason, R. D. Student variables that predict retention: Recent research and new developments. J. Stud. Aff. Res. Pract. 40 , 704–723 (2003).
Tinto, V. Dropout from higher education: A theoretical synthesis of recent research. Rev Educ Res 45 , 89–125 (1975).
Tinto, V. Completing college: Rethinking institutional action (University of Chicago Press, 2012).
Book Google Scholar
Astin, A. Retaining and Satisfying Students. Educ. Rec. 68 , 36–42 (1987).
Aulck, L., Velagapudi, N., Blumenstock, J. & West, J. Predicting student dropout in higher education. arXiv preprint arXiv:1606.06364 (2016).
Bogard, M., Helbig, T., Huff, G. & James, C. A comparison of empirical models for predicting student retention (Western Kentucky University, 2011).
Murtaugh, P. A., Burns, L. D. & Schuster, J. Predicting the retention of university students. Res. High. Educ. 40 , 355–371 (1999).
Porter, K. B. Current trends in student retention: A literature review. Teach. Learn. Nurs. 3 , 3–5 (2008).
Thomas, S. L. Ties that bind: A social network approach to understanding student integration and persistence. J. High. Educ. 71 , 591–615 (2000).
Peltier, G. L., Laden, R. & Matranga, M. Student persistence in college: A review of research. J. Coll. Stud. Ret. 1 , 357–375 (2000).
Nandeshwar, A., Menzies, T. & Nelson, A. Learning patterns of university student retention. Expert Syst. Appl. 38 , 14984–14996 (2011).
Boero, G., Laureti, T. & Naylor, R. An econometric analysis of student withdrawal and progression in post-reform Italian universities. (2005).
Tinto, V. Leaving college: Rethinking the causes and cures of student attrition (ERIC, 1987).
Choy, S. Students whose parents did not go to college: Postsecondary access, persistence, and attainment. Findings from the condition of education, 2001. (2001).
Ishitani, T. T. Studying attrition and degree completion behavior among first-generation college students in the United States. J. High. Educ. 77 , 861–885 (2006).
Thayer, P. B. Retention of students from first generation and low income backgrounds. (2000).
Britt, S. L., Ammerman, D. A., Barrett, S. F. & Jones, S. Student loans, financial stress, and college student retention. J. Stud. Financ. Aid 47 , 3 (2017).
McKinney, L. & Burridge, A. B. Helping or hindering? The effects of loans on community college student persistence. Res. High Educ. 56 , 299–324 (2015).
Hochstein, S. K. & Butler, R. R. The effects of the composition of a financial aids package on student retention. J. Stud. Financ. Aid 13 , 21–26 (1983).
Singell, L. D. Jr. Come and stay a while: Does financial aid effect retention conditioned on enrollment at a large public university?. Econ. Educ. Rev. 23 , 459–471 (2004).
Bean, J. P. Nine themes of college student. Coll. Stud. Retent. Formula Stud. Success 215 , 243 (2005).
Tinto, V. Through the eyes of students. J. Coll. Stud. Ret. 19 , 254–269 (2017).
Cabrera, A. F., Nora, A. & Castaneda, M. B. College persistence: Structural equations modeling test of an integrated model of student retention. J. High. Educ. 64 , 123–139 (1993).
Roberts, J. & Styron, R. Student satisfaction and persistence: Factors vital to student retention. Res. High. Educ. J. 6 , 1 (2010).
Gopalan, M. & Brady, S. T. College students’ sense of belonging: A national perspective. Educ. Res. 49 , 134–137 (2020).
Hoffman, M., Richmond, J., Morrow, J. & Salomone, K. Investigating, “sense of belonging” in first-year college students. J. Coll. Stud. Ret. 4 , 227–256 (2002).
Terenzini, P. T. & Pascarella, E. T. Toward the validation of Tinto’s model of college student attrition: A review of recent studies. Res. High Educ. 12 , 271–282 (1980).
Astin, A. W. The impact of dormitory living on students. Educational record (1973).
Astin, A. W. Student involvement: A developmental theory for higher education. J. Coll. Stud. Pers. 25 , 297–308 (1984).
Terenzini, P. T. & Pascarella, E. T. Studying college students in the 21st century: Meeting new challenges. Rev. High Ed. 21 , 151–165 (1998).
Thompson, J., Samiratedu, V. & Rafter, J. The effects of on-campus residence on first-time college students. NASPA J. 31 , 41–47 (1993).
Tinto, V. Research and practice of student retention: What next?. J. Coll. Stud. Ret. 8 , 1–19 (2006).
Lazer, D. et al. Computational social science. Science 1979 (323), 721–723 (2009).
Yarkoni, T. & Westfall, J. Choosing prediction over explanation in psychology: Lessons from machine learning. Perspect. Psychol. Sci. 12 , 1100–1122 (2017).
Peters, H., Marrero, Z. & Gosling, S. D. The Big Data toolkit for psychologists: Data sources and methodologies. in The psychology of technology: Social science research in the age of Big Data. 87–124 (American Psychological Association, 2022). doi: https://doi.org/10.1037/0000290-004 .
Fischer, C. et al. Mining big data in education: Affordances and challenges. Rev. Res. Educ. 44 , 130–160 (2020).
Hilbert, S. et al. Machine learning for the educational sciences. Rev. Educ. 9 , e3310 (2021).
National Academy of Education. Big data in education: Balancing the benefits of educational research and student privacy . (2017).
Aulck, L., Nambi, D., Velagapudi, N., Blumenstock, J. & West, J. Mining university registrar records to predict first-year undergraduate attrition. Int. Educ. Data Min. Soc. (2019).
Beaulac, C. & Rosenthal, J. S. Predicting university students’ academic success and major using random forests. Res. High Educ. 60 , 1048–1064 (2019).
Berens, J., Schneider, K., Görtz, S., Oster, S. & Burghoff, J. Early detection of students at risk–predicting student dropouts using administrative student data and machine learning methods. Available at SSRN 3275433 (2018).
Dawson, S., Jovanovic, J., Gašević, D. & Pardo, A. From prediction to impact: Evaluation of a learning analytics retention program. in Proceedings of the seventh international learning analytics & knowledge conference 474–478 (2017).
Dekker, G. W., Pechenizkiy, M. & Vleeshouwers, J. M. Predicting students drop Out: A case study. Int. Work. Group Educ. Data Min. (2009).
del Bonifro, F., Gabbrielli, M., Lisanti, G. & Zingaro, S. P. Student dropout prediction. in International Conference on Artificial Intelligence in Education 129–140 (Springer, 2020).
Hutt, S., Gardner, M., Duckworth, A. L. & D’Mello, S. K. Evaluating fairness and generalizability in models predicting on-time graduation from college applications. Int. Educ. Data Min. Soc. (2019).
Jayaprakash, S. M., Moody, E. W., Lauría, E. J. M., Regan, J. R. & Baron, J. D. Early alert of academically at-risk students: An open source analytics initiative. J. Learn. Anal. 1 , 6–47 (2014).
Balakrishnan, G. & Coetzee, D. Predicting student retention in massive open online courses using hidden markov models. Elect. Eng. Comput. Sci. Univ. Calif. Berkeley 53 , 57–58 (2013).
Hastie, T., Tibshirani, R. & Friedman, J. The elements of statistical learning (Springer series in statistics, New York, NY, USA, 2001).
Book MATH Google Scholar
Chawla, N. V., Bowyer, K. W., Hall, L. O. & Kegelmeyer, W. P. SMOTE: Synthetic minority over-sampling technique. J. Artif. Intell. Res. 16 , 321–357 (2002).
Article MATH Google Scholar
Zou, H. & Hastie, T. Regularization and variable selection via the elastic net. J. R. Stat. Soc. Seri. B Stat. Methodol. 67 , 301–320 (2005).
Article MathSciNet MATH Google Scholar
Friedman, J., Hastie, T. & Tibshirani, R. Regularization paths for generalized linear models via coordinate descent. J. Stat. Softw. 33 , 1 (2010).
Breiman, L. Random forests. Mach. Learn. 45 , 5–32 (2001).
Liaw, A. & Wiener, M. Classification and regression by randomForest. R News 2 , 18–22 (2002).
Pargent, F., Schoedel, R. & Stachl, C. An introduction to machine learning for psychologists in R. Psyarxiv (2022).
Hoerl, A. E. & Kennard, R. W. Ridge Regression. in Encyclopedia of Statistical Sciences vol. 8 129–136 (John Wiley & Sons, Inc., 2004).
Tibshirani, R. Regression shrinkage and selection via the Lasso. J. R. Stat. Soc. Ser. B (Methodol.) 58 , 267–288 (1996).
MathSciNet MATH Google Scholar
Hastie, T. & Qian, J. Glmnet vignette. vol. 9 1–42 https://hastie.su.domains/Papers/Glmnet_Vignette.pdf (2016).
Orrù, G., Monaro, M., Conversano, C., Gemignani, A. & Sartori, G. Machine learning in psychometrics and psychological research. Front. Psychol. 10 , 2970 (2020).
Pargent, F. & Albert-von der Gönna, J. Predictive modeling with psychological panel data. Z Psychol (2019).
Pargent, F., Schoedel, R. & Stachl, C. Best practices in supervised machine learning: A tutorial for psychologists. Doi: https://doi.org/10.31234/osf.io/89snd (2023).
Friedman, J., Hastie, T. & Tibshirani, R. The elements of statistical learning Vol. 1 (Springer series in statistics, 2001).
MATH Google Scholar
Rijsbergen, V. & Joost, C. K. Information Retrieval Butterworths London. Google Scholar Google Scholar Digital Library Digital Library (1979).
Molnar, C. Interpretable machine learning . (Lulu. com, 2020).
Aguiar, E., Ambrose, G. A., Chawla, N. v, Goodrich, V. & Brockman, J. Engagement vs Performance: Using Electronic Portfolios to Predict First Semester Engineering Student Persistence . Journal of Learning Analytics vol. 1 (2014).
Chai, K. E. K. & Gibson, D. Predicting the risk of attrition for undergraduate students with time based modelling. Int. Assoc. Dev. Inf. Soc. (2015).
Saenz, T., Marcoulides, G. A., Junn, E. & Young, R. The relationship between college experience and academic performance among minority students. Int. J. Educ. Manag (1999).
Pidgeon, A. M., Coast, G., Coast, G. & Coast, G. Psychosocial moderators of perceived stress, anxiety and depression in university students: An international study. Open J. Soc. Sci. 2 , 23 (2014).
Wilcox, P., Winn, S. & Fyvie-Gauld, M. ‘It was nothing to do with the university, it was just the people’: The role of social support in the first-year experience of higher education. Stud. High. Educ. 30 , 707–722 (2005).
Guiffrida, D. A. Toward a cultural advancement of Tinto’s theory. Rev. High Ed. 29 , 451–472 (2006).
Triandis, H. C., McCusker, C. & Hui, C. H. Multimethod probes of individualism and collectivism. J. Pers. Soc. Psychol. 59 , 1006 (1990).
Watson, D. & Clark, L. A. Extraversion and its positive emotional core. in Handbook of personality psychology 767–793 (Elsevier, 1997).
Greff, K., Srivastava, R. K., Koutník, J., Steunebrink, B. R. & Schmidhuber, J. LSTM: A search space odyssey. IEEE Trans. Neural Netw. Learn. Syst. 28 , 2222–2232 (2017).
Article MathSciNet PubMed Google Scholar
Arnold, K. E. & Pistilli, M. D. Course signals at Purdue: Using learning analytics to increase student success. in Proceedings of the 2nd international conference on learning analytics and knowledge 267–270 (2012).
Braxton, J. M. & McClendon, S. A. The fostering of social integration and retention through institutional practice. J. Coll. Stud. Ret. 3 , 57–71 (2001).
Sneyers, E. & de Witte, K. Interventions in higher education and their effect on student success: A meta-analysis. Educ. Rev. (Birm) 70 , 208–228 (2018).
Jamelske, E. Measuring the impact of a university first-year experience program on student GPA and retention. High Educ. (Dordr) 57 , 373–391 (2009).
Purdie, J. R. & Rosser, V. J. Examining the academic performance and retention of first-year students in living-learning communities and first-year experience courses. Coll. Stud. Aff. J. 29 , 95 (2011).
Lundberg, S. M. et al. From local explanations to global understanding with explainable AI for trees. Nat. Mach. Intell. 2 , 56–67 (2020).
Ramon, Y., Farrokhnia, R. A., Matz, S. C. & Martens, D. Explainable AI for psychological profiling from behavioral data: An application to big five personality predictions from financial transaction records. Information 12 , 518 (2021).
Download references
Author information
Alice Dinu is an Independent Researcher.
Authors and Affiliations
Columbia University, New York, USA
Sandra C. Matz & Heinrich Peters
Ludwig Maximilian University of Munich, Munich, Germany
Christina S. Bukow
Ready Education, Montreal, Canada
Christine Deacons
University of St. Gallen, St. Gallen, Switzerland
Clemens Stachl
Montreal, Canada
You can also search for this author in PubMed Google Scholar
Contributions
S.C.M., C.B, A.D., H.P., and C.S. designed the research. C.D. and A.D. provided the data. S.C.M, C.B. and H.P. analyzed the data. S.C.M and C.B. wrote the manuscript. All authors reviewed the manuscript. Earlier versions of thi research were part of the C.B.’s masters thesis which was supervised by S.C.M. and C.S.
Corresponding author
Correspondence to Sandra C. Matz .
Ethics declarations
Competing interests.
C.D. is a former employee of Ready Education. None of the other authors have conflict of interests related to this submission.
Additional information
Publisher's note.
Springer Nature remains neutral with regard to jurisdictional claims in published maps and institutional affiliations.
The original online version of this Article was revised: Alice Dinu was omitted from the author list in the original version of this Article. The Author Contributions section now reads: “S.C.M., C.B, A.D., H.P., and C.S. designed the research. C.D. and A.D. provided the data. S.C.M, C.B. and H.P. analyzed the data. S.C.M and C.B. wrote the manuscript. All authors reviewed the manuscript. Earlier versions of this research were part of the C.B.’s masters thesis which was supervised by S.C.M. and C.S.” Additionally, the Article contained an error in Data Availability section and the legend of Figure 2 was incomplete.
Supplementary Information
Supplementary information., rights and permissions.
Open Access This article is licensed under a Creative Commons Attribution 4.0 International License, which permits use, sharing, adaptation, distribution and reproduction in any medium or format, as long as you give appropriate credit to the original author(s) and the source, provide a link to the Creative Commons licence, and indicate if changes were made. The images or other third party material in this article are included in the article's Creative Commons licence, unless indicated otherwise in a credit line to the material. If material is not included in the article's Creative Commons licence and your intended use is not permitted by statutory regulation or exceeds the permitted use, you will need to obtain permission directly from the copyright holder. To view a copy of this licence, visit http://creativecommons.org/licenses/by/4.0/ .
Reprints and permissions
About this article
Cite this article.
Matz, S.C., Bukow, C.S., Peters, H. et al. Using machine learning to predict student retention from socio-demographic characteristics and app-based engagement metrics. Sci Rep 13 , 5705 (2023). https://doi.org/10.1038/s41598-023-32484-w
Download citation
Received : 09 August 2022
Accepted : 28 March 2023
Published : 07 April 2023
DOI : https://doi.org/10.1038/s41598-023-32484-w
Share this article
Anyone you share the following link with will be able to read this content:
Sorry, a shareable link is not currently available for this article.
Provided by the Springer Nature SharedIt content-sharing initiative
By submitting a comment you agree to abide by our Terms and Community Guidelines . If you find something abusive or that does not comply with our terms or guidelines please flag it as inappropriate.
Quick links
- Explore articles by subject
- Guide to authors
- Editorial policies
Sign up for the Nature Briefing newsletter — what matters in science, free to your inbox daily.

- Follow us on Facebook
- Follow us on Twitter
- Criminal Justice
- Environment
- Politics & Government
- Race & Gender
Expert Commentary
Improving college student mental health: Research on promising campus interventions
Hiring more counselors isn’t enough to improve college student mental health, scholars warn. We look at research on programs and policies schools have tried, with varying results.
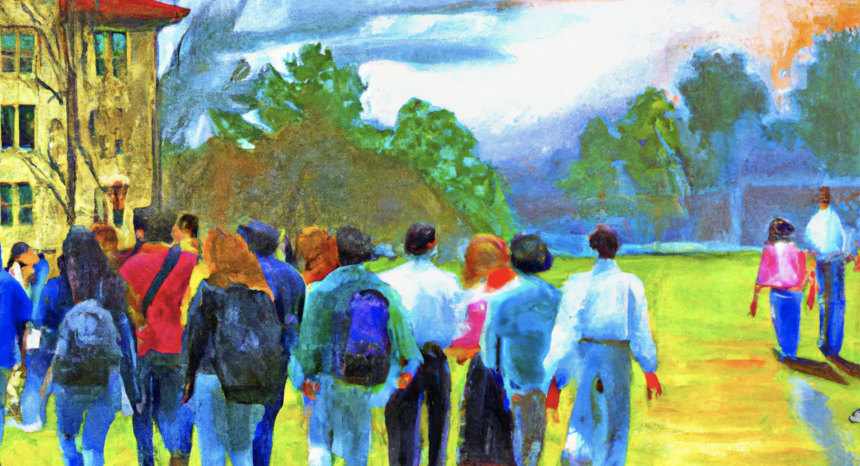
Republish this article

This work is licensed under a Creative Commons Attribution-NoDerivatives 4.0 International License .
by Denise-Marie Ordway, The Journalist's Resource September 13, 2023
This <a target="_blank" href="https://journalistsresource.org/education/college-student-mental-health-research-interventions/">article</a> first appeared on <a target="_blank" href="https://journalistsresource.org">The Journalist's Resource</a> and is republished here under a Creative Commons license.<img src="https://journalistsresource.org/wp-content/uploads/2020/11/cropped-jr-favicon-150x150.png" style="width:1em;height:1em;margin-left:10px;">
If you’re a journalist covering higher education in the U.S., you’ll likely be reporting this fall on what many healthcare professionals and researchers are calling a college student mental health crisis.
An estimated 49% of college students have symptoms of depression or anxiety disorder and 14% seriously considered committing suicide during the past year, according to a national survey of college students conducted during the 2022-23 school year. Nearly one-third of the 76,406 students who participated said they had intentionally injured themselves in recent months.
In December, U.S. Surgeon General Vivek Murthy issued a rare public health advisory calling attention to the rising number of youth attempting suicide , noting the COVID-19 pandemic has “exacerbated the unprecedented stresses young people already faced.”
Meanwhile, colleges and universities of all sizes are struggling to meet the need for mental health care among undergraduate and graduate students. Many schools have hired more counselors and expanded services but continue to fall short.
Hundreds of University of Houston students held a protest earlier this year , demanding the administration increase the number of counselors and make other changes after two students died by suicide during the spring semester, the online publication Chron reported.
In an essay in the student-run newspaper , The Cougar, last week, student journalist Malachi Key blasts the university for having one mental health counselor for every 2,122 students, a ratio higher than recommended by the International Accreditation of Counseling Services , which accredits higher education counseling services.
But adding staff to a campus counseling center won’t be enough to improve college student mental health and well-being, scholars and health care practitioners warn.
“Counseling centers cannot and should not be expected to solve these problems alone, given that the factors and forces affecting student well-being go well beyond the purview and resources that counseling centers can bring to bear,” a committee of the National Academies of Sciences, Engineering, and Medicine writes in a 2021 report examining the issue.
Advice from prominent scholars
The report is the culmination of an 18-month investigation the National Academies launched in 2019, at the request of the federal government, to better understand how campus culture affects college student mental health and well-being. Committee members examined data, studied research articles and met with higher education leaders, mental health practitioners, researchers and students.
The committee’s key recommendation: that schools take a more comprehensive approach to student mental health, implementing a wide range of policies and programs aimed at preventing mental health problems and improving the well-being of all students — in addition to providing services and treatment for students in distress and those with diagnosed mental illnesses.
Everyone on campus, including faculty and staff across departments, needs to pitch in to establish a new campus culture, the committee asserts.
“An ‘all hands’ approach, one that emphasizes shared responsibility and a holistic understanding of what it means in practice to support students, is needed if institutions of higher education are to intervene from anything more than a reactive standpoint,” committee members write. “Creating this systemic change requires that institutions examine the entire culture and environment of the institution and accept more responsibility for creating learning environments where a changing student population can thrive.”
In a more recent analysis , three leading scholars in the field also stress the need for a broader plan of action.
Sara Abelson , a research assistant professor at Temple University’s medical school; Sarah Lipson , an associate professor at the Boston University School of Public Health; and Daniel Eisenberg , a professor of health policy and management at the University of California, Los Angeles’ School of Public Health, have been studying college student mental health for years.
Lipson and Eisenberg also are principal investigators for the Healthy Minds Network , which administers the Healthy Minds Study , a national survey of U.S college students conducted annually to gather information about their mental health, whether and how they receive mental health care and related issues.
Abelson, Lipson and Eisenberg review the research to date on mental health interventions for college students in the 2022 edition of Higher Education: Handbook of Theory and Research . They note that while the evidence indicates a multi-pronged approach is best, it’s unclear which specific strategies are most effective.
Much more research needed
Abelson, Lipson and Eisenberg stress the need for more research. Many interventions in place at colleges and universities today — for instance, schoolwide initiatives aimed at reducing mental health stigma and encouraging students to seek help when in duress – should be evaluated to gauge their effectiveness, they write in their chapter, “ Mental Health in College Populations: A Multidisciplinary Review of What Works, Evidence Gaps, and Paths Forward .”
They add that researchers and higher education leaders also need to look at how campus operations, including hiring practices and budgetary decisions, affect college student mental health. It would be helpful to know, for example, how students are impacted by limits on the number of campus counseling sessions they can have during a given period, Abelson, Lipson and Eisenberg suggest.
Likewise, it would be useful to know whether students are more likely to seek counseling when they must pay for their sessions or when their school charges every member of the student body a mandatory health fee that provides free counseling for all students.
“These financially-based considerations likely influence help-seeking and treatment receipt, but they have not been evaluated within higher education,” they write.
Interventions that show promise
The report from the National Academies of Sciences, Engineering, and Medicine and the chapter by Abelson, Lipson and Eisenberg both spotlight programs and policies shown to prevent mental health problems or improve the mental health and well-being of young people. However, many intervention studies focus on high school students, specific groups of college students or specific institutions. Because of this, it can be tough to predict how well they would work across the higher education landscape.
Scientific evaluations of these types of interventions indicate they are effective:
- Building students’ behavior management skills and having them practice new skills under expert supervision . An example: A class that teaches students how to use mindfulness to improve their mental and physical health that includes instructor-led meditation exercises.
- Training some students to offer support to others , including sharing information and organizing peer counseling groups. “Peers may be ‘the single most potent source of influence’ on student affective and cognitive growth and development during college,” Abelson, Lipson and Eisenberg write.
- Reducing students’ access to things they can use to harm themselves , including guns and lethal doses of over-the-counter medication.
- Creating feelings of belonging through activities that connect students with similar interests or backgrounds.
- Making campuses more inclusive for racial and ethnic minorities, LGBTQ+ students and students who are the first in their families to go to college. One way to do that is by hiring mental health professionals trained to recognize, support and treat students from different backgrounds. “Research has shown that the presentation of [mental health] symptoms can differ based on racial and ethnic backgrounds, as can engaging in help-seeking behaviors that differ from those of cisgender, heteronormative white men,” explain members of National Academies of Sciences, Engineering, and Medicine committee.
Helping journalists sift through the evidence
We encourage journalists to read the full committee report and aforementioned chapter in Higher Education: Handbook of Theory and Research . We realize, though, that many journalists won’t have time to pour over the combined 304 pages of text to better understand this issue and the wide array of interventions colleges and universities have tried, with varying success.
To help, we’ve gathered and summarized meta-analyses that investigate some of the more common interventions. Researchers conduct meta-analyses — a top-tier form of scientific evidence — to systematically analyze all the numerical data that appear in academic studies on a given topic. The findings of a meta-analysis are statistically stronger than those reached in a single study, partly because pooling data from multiple, similar studies creates a larger sample to examine.
Keep reading to learn more. And please check back here occasionally because we’ll add to this list as new research on college student mental health is published.
Peer-led programs
Stigma and Peer-Led Interventions: A Systematic Review and Meta-Analysis Jing Sun; et al. Frontiers in Psychiatry, July 2022.
When people diagnosed with a mental illness received social or emotional support from peers with similar mental health conditions, they experienced less stress about the public stigma of mental illness, this analysis suggests.
The intervention worked for people from various age groups, including college students and middle-aged adults, researchers learned after analyzing seven studies on peer-led mental health programs written or published between 1975 and 2021.
Researchers found that participants also became less likely to identify with negative stereotypes associated with mental illness.
All seven studies they examined are randomized controlled trials conducted in the U.S., Germany or Switzerland. Together, the findings represent the experiences of a total of 763 people, 193 of whom were students at universities in the U.S.
Researchers focused on interventions designed for small groups of people, with the goal of reducing self-stigma and stress associated with the public stigma of mental illness. One or two trained peer counselors led each group for activities spanning three to 10 weeks.
Five of the seven studies tested the Honest, Open, Proud program, which features role-playing exercises, self-reflection and group discussion. It encourages participants to consider disclosing their mental health issues, instead of keeping them a secret, in hopes that will help them feel more confident and empowered. The two other programs studied are PhotoVoice , based in the United Kingdom, and
“By sharing their own experiences or recovery stories, peer moderators may bring a closer relationship, reduce stereotypes, and form a positive sense of identity and group identity, thereby reducing self-stigma,” the authors of the analysis write.
Expert-led instruction
The Effects of Meditation, Yoga, and Mindfulness on Depression, Anxiety, and Stress in Tertiary Education Students: A Meta-Analysis Josefien Breedvelt; et al. Frontiers in Psychiatry, April 2019.
Meditation-based programs help reduce symptoms of depression, anxiety and stress among college students, researchers find after analyzing the results of 24 research studies conducted in various parts of North America, Asia and Europe.
Reductions were “moderate,” researchers write. They warn, however, that the results of their meta-analysis should be interpreted with caution considering studies varied in quality.
A total of 1,373 college students participated in the 24 studies. Students practiced meditation, yoga or mindfulness an average of 153 minutes a week for about seven weeks. Most programs were provided in a group setting.
Although the researchers do not specify which types of mindfulness, yoga or meditation training students received, they note that the most commonly offered mindfulness program is Mindfulness-Based Stress Reduction and that a frequently practiced form of yoga is Hatha Yoga .
Meta-Analytic Evaluation of Stress Reduction Interventions for Undergraduate and Graduate Students Miryam Yusufov; et al. International Journal of Stress Management, May 2019.
After examining six types of stress-reduction programs common on college campuses, researchers determined all were effective at reducing stress or anxiety among students — and some helped with both stress and anxiety.
Programs focusing on cognitive-behavioral therapy , coping skills and building social support networks were more effective in reducing stress. Meanwhile, relaxation training, mindfulness-based stress reduction and psychoeducation were more effective in reducing anxiety.
The authors find that all six program types were equally effective for undergraduate and graduate students.
The findings are based on an analysis of 43 studies dated from 1980 to 2015, 30 of which were conducted in the U.S. The rest were conducted in Australia, China, India, Iran, Japan, Jordan, Kora, Malaysia or Thailand. A total of 4,400 students participated.
Building an inclusive environment
Cultural Adaptations and Therapist Multicultural Competence: Two Meta-Analytic Reviews Alberto Soto; et al. Journal of Clinical Psychology, August 2018.
If racial and ethnic minorities believe their therapist understands their background and culture, their treatment tends to be more successful, this analysis suggests.
“The more a treatment is tailored to match the precise characteristics of a client, the more likely that client will engage in treatment, remain in treatment, and experience improvement as a result of treatment,” the authors write.
Researchers analyzed the results of 15 journal articles and doctoral dissertations that examine therapists’ cultural competence . Nearly three-fourths of those studies were written or published in 2010 or later. Together, the findings represent the experiences of 2,640 therapy clients, many of whom were college students. Just over 40% of participants were African American and 32% were Hispanic or Latino.
The researchers note that they find no link between therapists’ ratings of their own level of cultural competence and client outcomes.
Internet-based interventions
Internet Interventions for Mental Health in University Students: A Systematic Review and Meta-Analysis Mathias Harrer; et al. International Journal of Methods in Psychiatric Research, June 2019.
Internet-based mental health programs can help reduce stress and symptoms of anxiety, depression and eating disorders among college students, according to an analysis of 48 research studies published or written before April 30, 2018 on the topic.
All 48 studies were randomized, controlled trials of mental health interventions that used the internet to engage with students across various platforms and devices, including mobile phones and apps. In total, 10,583 students participated in the trials.
“We found small effects on depression, anxiety, and stress symptoms, as well as moderate‐sized effects on eating disorder symptoms and students’ social and academic functioning,” write the authors, who conducted the meta-analysis as part of the World Mental Health International College Student Initiative .
The analysis indicates programs that focus on cognitive behavioral therapy “were superior to other types of interventions.” Also, programs “of moderate length” — one to two months – were more effective.
The researchers note that studies of programs targeting depression showed better results when students were not compensated for their participation, compared to studies in which no compensation was provided. The researchers do not offer possible explanations for the difference in results or details about the types of compensation offered to students.
About The Author
Denise-Marie Ordway
Greater Good Science Center • Magazine • In Action • In Education
Parenting & Family Articles & More
Our best education articles of 2020, readers and editors pick the most interesting and insightful articles from the past year about teaching, learning, and the keys to well-being at school..
In February of 2020, we launched the new website Greater Good in Education , a collection of free, research-based and -informed strategies and practices for the social, emotional, and ethical development of students, for the well-being of the adults who work with them, and for cultivating positive school cultures. Little did we know how much more crucial these resources would become over the course of the year during the COVID-19 pandemic.
Now, as we head back to school in 2021, things are looking a lot different than in past years. Our most popular education articles of 2020 can help you manage difficult emotions and other challenges at school in the pandemic, all while supporting the social-emotional well-being of your students.
In addition to these articles, you can also find tips, tools, and recommended readings in two resource guides we created in 2020: Supporting Learning and Well-Being During the Coronavirus Crisis and Resources to Support Anti-Racist Learning , which helps educators take action to undo the racism within themselves, encourage their colleagues to do the same, and teach and support their students in forming anti-racist identities.

Here are the 10 best education articles of 2020, based on a composite ranking of pageviews and editors’ picks.
Can the Lockdown Push Schools in a Positive Direction? , by Patrick Cook-Deegan: Here are five ways that COVID-19 could change education for the better.
How Teachers Can Navigate Difficult Emotions During School Closures , by Amy L. Eva: Here are some tools for staying calm and centered amid the coronavirus crisis.
Six Online Activities to Help Students Cope With COVID-19 , by Lea Waters: These well-being practices can help students feel connected and resilient during the pandemic.
Help Students Process COVID-19 Emotions With This Lesson Plan , by Maurice Elias: Music and the arts can help students transition back to school this year.
How to Teach Online So All Students Feel Like They Belong , by Becki Cohn-Vargas and Kathe Gogolewski: Educators can foster belonging and inclusion for all students, even online.
How Teachers Can Help Students With Special Needs Navigate Distance Learning , by Rebecca Branstetter: Kids with disabilities are often shortchanged by pandemic classroom conditions. Here are three tips for educators to boost their engagement and connection.
How to Reduce the Stress of Homeschooling on Everyone , by Rebecca Branstetter: A school psychologist offers advice to parents on how to support their child during school closures.
Three Ways to Help Your Kids Succeed at Distance Learning , by Christine Carter: How can parents support their children at the start of an uncertain school year?
How Schools Are Meeting Social-Emotional Needs During the Pandemic , by Frances Messano, Jason Atwood, and Stacey Childress: A new report looks at how schools have been grappling with the challenges imposed by COVID-19.
Six Ways to Help Your Students Make Sense of a Divisive Election , by Julie Halterman: The election is over, but many young people will need help understanding what just happened.
Train Your Brain to Be Kinder (video), by Jane Park: Boost your kindness by sending kind thoughts to someone you love—and to someone you don’t get along with—with a little guidance from these students.
From Othering to Belonging (podcast): We speak with john a. powell, director of the Othering & Belonging Institute, about racial justice, well-being, and widening our circles of human connection and concern.
About the Author
Greater good editors, you may also enjoy.

This article — and everything on this site — is funded by readers like you.
Become a subscribing member today. Help us continue to bring “the science of a meaningful life” to you and to millions around the globe.

Journal of Student Research
Journal of Student Research (JSR) is an Academic, Multidisciplinary, and Faculty-reviewed Journal (Houston, Texas) devoted to the Rapid Dissemination of Current Research Published by High School Edition , Undergraduate and Graduate students.
Articles Indexed in Scholarly Databases
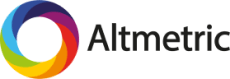
The journal seeks articles that are novel, integrative, and accessible to a broad audience, including an array of disciplines. The content of the journal ranges from Applied research to Theoretical research. In general, papers on all topics are welcome to submit. The journal uses an automated process from manuscript submission to publication. Manuscript submission, peer review, and publication are all handled online, and the journal automates all clerical steps during peer review.
Trusted By Student Authors Globally
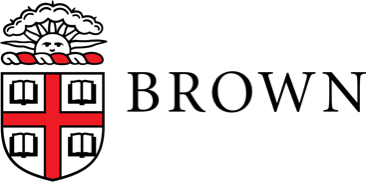
Focus and Scope
Students strive to be successful at publications, and with JSR, authors aspiring to publish will receive scholarly feedback after the reviews of their submissions are received. This feedback will help authors identify areas of improvement to their submission and help them better understand the process to be successful at publication. Once published, we strive to provide a global platform for our authors to showcase their work.
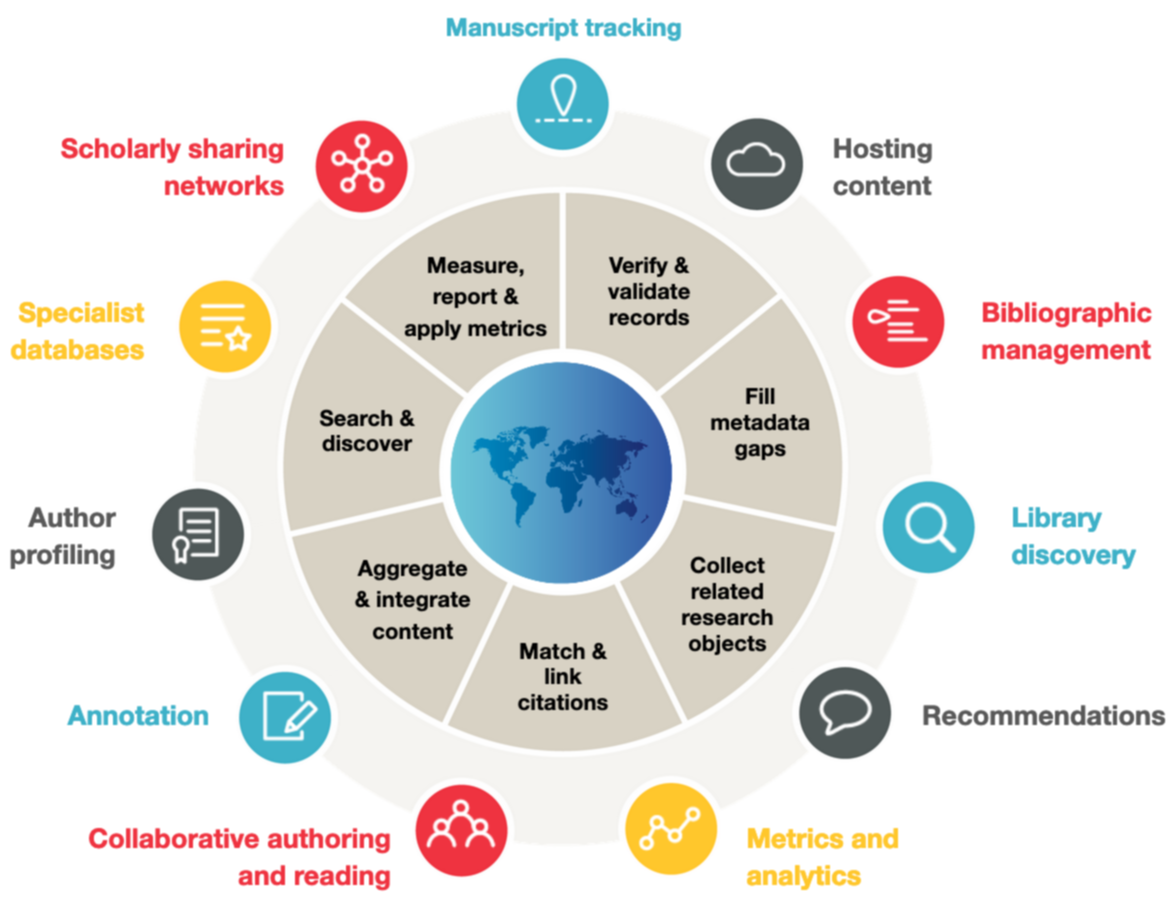
Faculty-Refereed Review Process
This journal uses a double-blind review, which means that both the reviewer and author identities are concealed from the reviewers, and vice versa, throughout the review process. Authors need to ensure that their manuscripts do not give away their identity to facilitate this. To find out more about the review process, please visit the Author Guidelines page. We invite teachers and faculty interested in reviewing articles for this journal; please visit our Reviewers page for more information.
Open Access Policy
This journal provides access to its published content on the principle that making research freely available to the public supports a greater global exchange of knowledge. Learn more about Open Access .
Authors Retain Copyright
Articles published in this journal are under a Creative Commons License , and the authors retain the copyright to their work.
Announcements
Call for papers: volume 13 issue 3.
If you are an undergraduate or graduate student at a college or university aspiring to publish, we are accepting submissions. Submit Your Article Now!
Deadline: 11:59 p.m. May 31, 2024
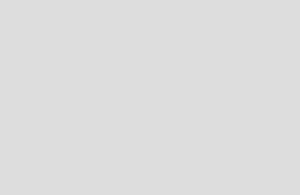
- Share full article
Advertisement
Supported by
How to Reduce Student Absenteeism
Readers discuss the reasons for the spike since the pandemic and how to lure students back.

Why School Absences Have ‘Exploded’ Almost Everywhere
The pandemic changed families’ lives and the culture of education: “Our relationship with school became optional.”
To the Editor:
“ Pandemic Effect: Absence From Schools Is Soaring ” (front page, March 30) highlights the persistent challenge of chronic absenteeism in U.S. schools. If pandemic-related “cultural shifts” are among the factors keeping students away from school, bringing them back may require us to rethink the culture of education itself.
Despite the efforts of many visionary educators, too many schools still offer a deskbound, test- and compliance-driven experience that leaves students passive, uninspired and flat-out bored.
Over the last two years, a pilot program in Salem, Mass. , has succeeded in cutting chronic absenteeism among middle schoolers in half by listening to students and designing learning with their interests in mind, including regular field trips, hands-on projects and mentoring with college students. Today, the chronic absenteeism rate among the pilot cohort of seventh and eighth graders hovers at 8 percent, in no small part because students don’t want to miss what’s on offer at school.
Educators can reset school culture by being adaptive, believing in teacher leadership and recognizing that powerful learning can happen outside classroom walls. Unlike the use of Band-Aids and gimmickry that do not result in long-term change, valuing a philosophy of “education everywhere,” as Salem has embraced, will result in improved attendance and academic growth.
Stephen Hinds Laura Tavares Stephen Zrike Chelsea Banks Mr. Hinds is president and Ms. Tavares is executive director of the WPS Institute, an education nonprofit. Dr. Zrike is superintendent and Ms. Banks is dean of innovation for Salem Public Schools.
This article brings light to an issue plaguing school districts across the country. As a former classroom teacher, I remember talking with students who returned to school after being absent. They would ask for the work they missed. While I could share the assignment, I could not possibly share the rest of what they missed, including the social and academic interaction with their peers, the instruction provided, the opportunity to ask questions while working through material and being part of a community.
When done right, school is more than a collection of assignments. It is a vibrant social fabric that provides a culture of belonging, and opportunities to grow and explore with trusted adults guiding the way.
The solution to the absenteeism problem is not easy. As the chief education officer at Mikva Challenge, a group that works to engage young people in the civic process and have their voices part of critical decision making affecting their lives, I know that for any solution to be successful, it must involve youth in the process.
Our default as adults is to make decisions in the best interest of children, without asking them what they think and whether an idea will work. Young people are not apathetic; they are uninvited. They care deeply about the issues that affect them. And when they are engaged in decision making, policy is better.
Jill Bass Chicago
We had mixed feelings when reading “Pandemic Effect: Absence From Schools Is Soaring.” On the one hand, it’s important for the public to understand that chronic absenteeism in America is no small problem. On the other hand, the article unwittingly minimized the deep struggle so many families experience, particularly those from underresourced backgrounds.
You quote a researcher who stated, “The problem got worse for everybody in the same proportional way,” but we question whether this accurately reflects the reality in America today. Based on our own and others’ research, we believe that families who struggled before the pandemic were much more vulnerable to its effects.
We cannot ignore just how much deeper Covid affected communities of color, communities with risk factors, communities in poverty and communities in rural areas. This does not negate anyone’s struggles; yet the struggle has been disproportionate. This cannot be ignored.
Zahava L. Friedman Keri Giordano Hillside, N.J. Dr. Friedman is an assistant professor and Dr. Giordano is an associate professor at the College of Health Professions and Human Services, Kean University.
My 12-year-old son has been absent from school most of this year and is a part of the chronic absenteeism statistics cited in the article. His attendance was excellent until he caught Covid twice in one year from school. He was 9 years old and has been chronically ill ever since.
It is shocking to me that the article never suggests that some absenteeism might be due to chronic illness from Covid.
One recent study suggested that as many as 5.8 million kids in the United States have had their health affected by long Covid. These statistics are highly contested, but given how reluctant our doctors have been to diagnose or treat our child for something that they cannot measure with any blood test, it is not surprising that we do not really know the full extent of this disease.
My child, and many other children like him, cannot go to school because they are struggling with the persistent life-altering symptoms of chronic illness. These children want to go back to school. Don’t leave them out of the story.
Sarah Mathis Pleasanton, Calif.
The root causes of chronic absenteeism in American public schools are as varied as the solutions needed to combat it. One often overlooked and underfunded strategy with the potential to re-engage students in learning is arts education.
A 2021 study on the benefits of arts instruction in the Boston Public Schools showed that increased access to arts education reduced student absenteeism, with a greater impact on students who had been chronically absent.
BPS Arts Expansion is a public-private partnership that has dramatically expanded access to quality arts education throughout the school district and enabled longitudinal research on its impact.
No one strategy will be the panacea for chronic absenteeism. But as districts across the country grapple with this issue, expanding access to in-school arts instruction warrants attention.
Marinell Rousmaniere Boston The writer is the president and C.E.O. of EdVestors, a nonprofit school improvement organization.
MINI REVIEW article
The impact of virtual reality on student engagement in the classroom–a critical review of the literature.

- 1 Faculty of Education, Silpakorn University, Nakhon Pathom, Thailand
- 2 Melbourne Graduate School of Education, The University of Melbourne, Melbourne, VIC, Australia
- 3 Graduate Department, Xi’an Physical Education University, Xi’an, China
- 4 College of Commerce and Tourism, Hunan Vocational College for Nationalities, Yueyang, China
- 5 Graduate Department, Sehan University, Yeongam County, Republic of Korea
Objective: The purpose of this review is to identify the impact of virtual reality (VR) technology on student engagement, specifically cognitive engagement, behavioral engagement, and affective engagement.
Methods: A comprehensive search of databases such as Google, Scopus, and Elsevier was conducted to identify English-language articles related to VR and classroom engagement for the period from 2014 to 2023. After systematic screening, 33 articles were finally reviewed.
Results: The use of VR in the classroom is expected to improve student engagement and learning outcomes, and is particularly effective for students with learning disabilities. However, introducing VR into middle school education poses several challenges, including difficulties in the education system to keep up with VR developments, increased demands on students’ digital literacy, and insufficient proficiency of teachers in using VR.
Conclusion: To effectively utilize VR to increase student engagement, we advocate for educational policymakers to provide training and technical support to teachers to ensure that they can fully master and integrate VR to increase student engagement and instructional effectiveness.
Introduction
In recent years, virtual reality (VR) has emerged as a transformative technology in education, providing new avenues for immersive and interactive learning experiences ( Pottle, 2019 ). At its core, VR offers a departure from the tangible, allowing users to delve into an environment transcending conventional reality ( Brooks, 1999 ; Jeong et al., 2019 ). VR’s essence is captured in three pillars: presence, interactivity, and immersion ( Lee et al., 2017 ). Presence grants users access to previously unreachable 3D landscapes, facilitating a unique, experiential insight ( Poux et al., 2020 ). Interactivity kindles user curiosity, enabling dynamic engagements within the virtual milieu ( Steuer et al. 1995 ; Huvila, 2013 ; Song et al., 2023 ). Immersion pushes the boundaries of conventional experiences, reviving or manifesting phenomena outside the realm of everyday life ( Sanchez-Vives and Slater, 2005 ; Poux et al., 2020 ).
The introduction of VR in education might increase student engagement, which is closely related to the cognitive, behavioral, and affective dimensions of the engagement model ( Wang and Degol, 2014 ). Cognitive engagement underscores the depth of students’ attention, comprehension, and retention ( Wang and Degol, 2014 ). Behavioral engagement is observable, characterized by consistent attendance and active classroom participation ( Wang and Degol, 2014 ). Affective engagement delves into the emotional realm, encompassing motivation, passion, and learning efficacy ( Wang and Degol, 2014 ).
Existing literature emphasizes the importance of virtual reality technology in promoting full student engagement in cognitive, behavioral, and affective dimensions, and states that the application of virtual reality technology in education has become a trend ( Mystakidis et al., 2021 ). Some literature shows that higher education institutions are increasingly adopting VR, with adoption rates as high as 46% at US universities and 96% at United Kingdom universities ( United Kingdom Authority, 2019 ; Agbo et al., 2021 ). In addition, the establishment of dedicated VR laboratories at leading universities such as Harvard University and Colorado State University underscores the commitment to using VR for educational innovation and advancement ( Reid, 1987 ; Leidner and Jarvenpaa, 1995 ). This literature shows that the widespread use of VR in education has attracted the attention of a growing number of researchers and educators, with a particular interest in the impact of VR in the classroom in terms of students’ cognitive, behavioral, and affective engagement.
It is worth noting that although existing literature begins to discuss the impact of VR on student engagement, there are still shortcomings in determining the impact of VR on various dimensions of student engagement, which may limit our overall understanding of the topic. Therefore, further discussion is needed to more specifically identify the impact of VR on the various dimensions of student engagement to gain a more comprehensive and concrete understanding. To accomplish this, this review is guided by the following three questions: (1) What are the positive impacts of VR in education? (2) What are the challenges of VR in education? (3) What interventions can address these challenges? With this in mind, the article will first discuss the positive impact of VR on students’ cognitive, behavioral, and affective engagement to help readers understand its potential in education. It will then discuss the challenges facing VR to make constructive recommendations to address the problems in education.
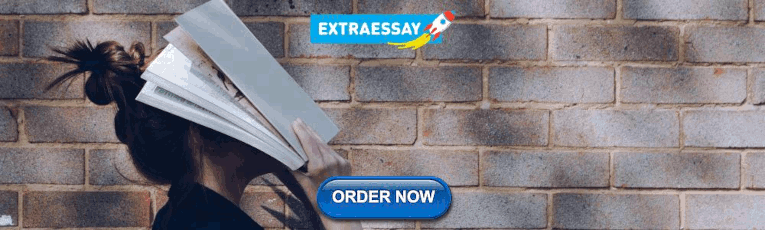
Searching strategy
In our methods, we used critical review. According to Grant and Booth (2009) “an effective critical review presents, analyses and synthesizes material from diverse sources”(p.93). Critical perspectives were used to assess the potential of VR in reforming educational practices and improving teaching and learning outcomes. The purpose of this article was to collect literature on the impact of VR on student engagement. Therefore, this article summarizes the previous studies as follows. First, information was obtained from Google, Scopus, and Elsevier databases: “virtual reality,” “cognitive engagement,” “affective engagement,” “behavioral engagement” and “learning outcomes.” The search was limited to articles published between January 2014 and December 2023 in English. The first search used all combinations of the above keywords and, after an initial review, produced 97 potentially relevant articles (Google: 92, Scopus: 3, Elsevier: 2).
In the second phase, secondary terms such as “affect,” “challenge,” and “education” were added, reducing the number of studies to 63 (Google:60, Scopus:1, Elsevier:2). Of these, 34 did not meet the criteria and were excluded. They were excluded because their target audience was teachers and did not discuss the impact of VR on student engagement from the student’s perspective. In the final stage, another 53 articles were excluded because they were repetitive and their purpose was to discuss either technology or engagement, or both. Finally, their full texts were reviewed to determine if their work fits the focus of this article 20 articles (Google: 17, Scopus: 1, Elsevier: 2) qualified for final review, covered a sample on the impact of VR on student engagement, and were included in the analysis.
Inclusion and exclusion criteria
To ensure the quality of the literature, we selected only peer-reviewed journal articles published in English in the last decade. The main purpose of this article was to review the impact of VR on student engagement. Therefore, we selected only review articles on the impact of VR on student engagement in educational settings. Articles that were not written in English did not discuss the impact on engagement from a student perspective, and were published beyond the previously established time and language were excluded. In addition, a selection of articles was identified and assessed by manually searching the references of articles related to the topic, of which 13 met the eligibility criteria. Therefore, 13 additional articles were added to the 20 identified. In total, 33 articles that met these eligibility criteria were included and reviewed here. Full-text versions of the articles were obtained, with each article being reviewed and confirmed as appropriate by the authors. Finally, to maximize transparency and traceability, we list the rationale and relevant evidence for all articles included (see Table 1 ). The process of article selection followed the Preferred Reporting of Items for Systematic Reviews and Meta-Analyses (PRISMA) Statement ( Moher et al., 2009 ; see Figure 1 ). Figure 1 illustrates the process of article selection.
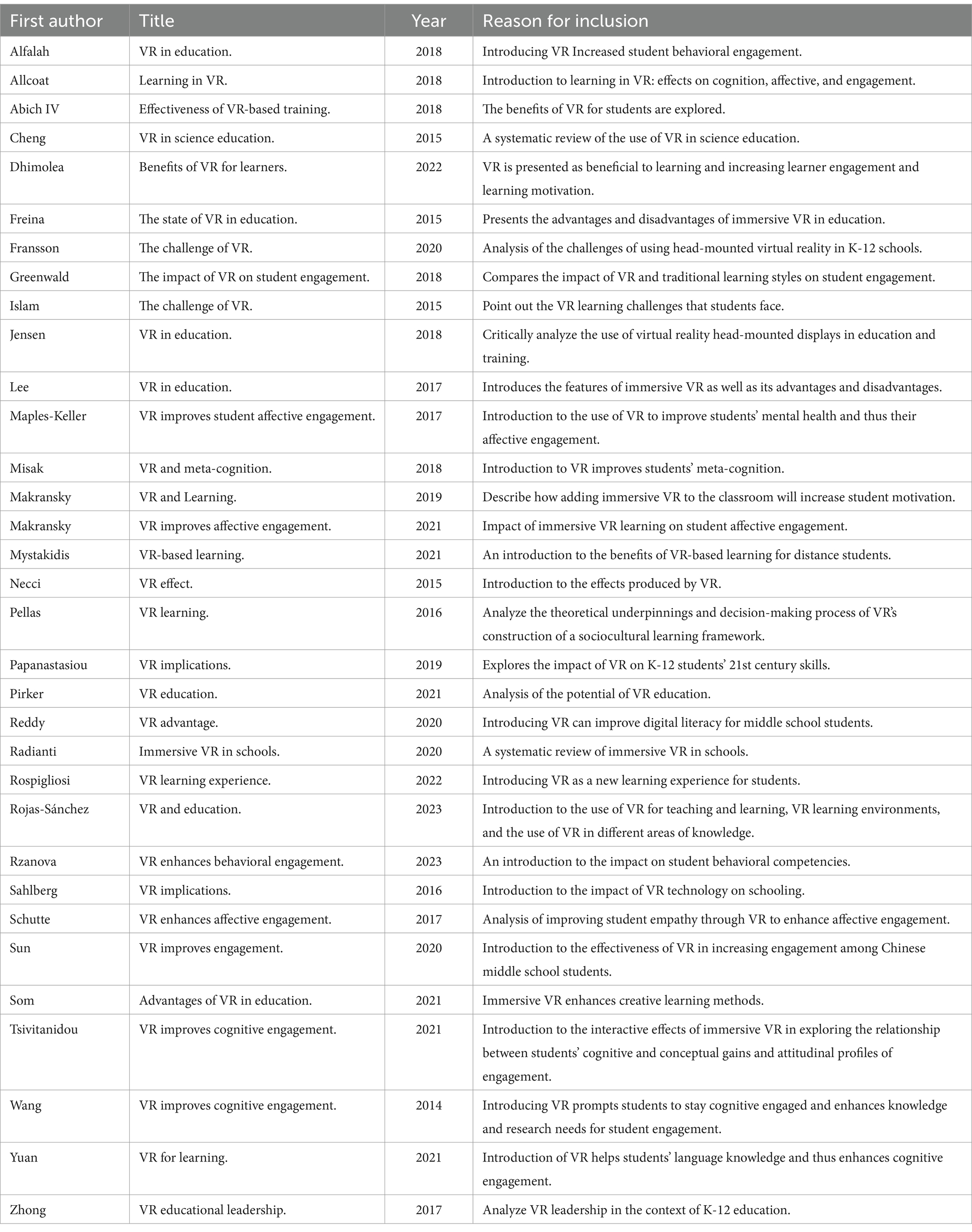
Table 1 . Publications reviewed in full text with reasons for inclusion or exclusion.
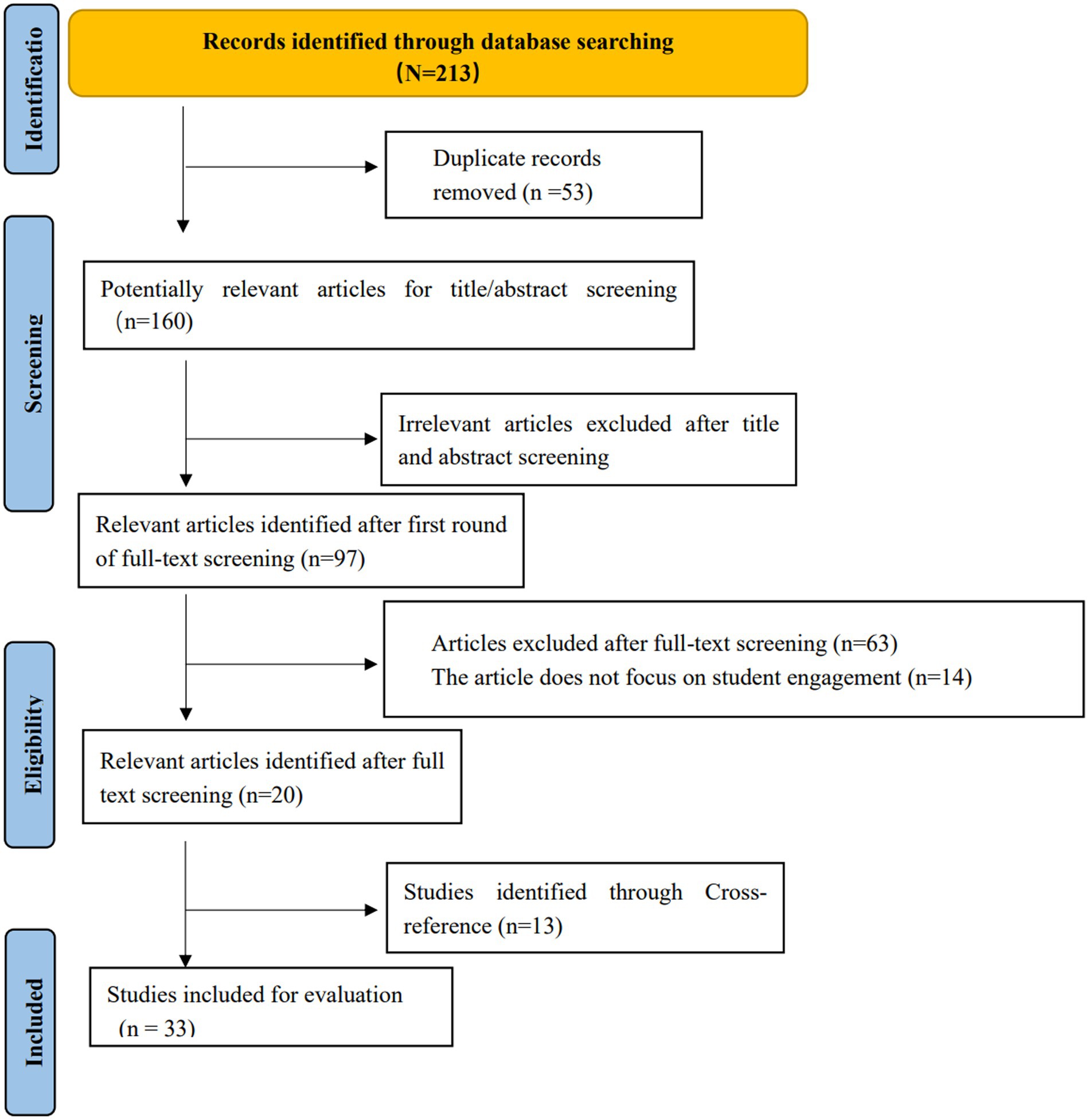
Figure 1 . PRISMA flow diagram for article selection.
The review found that the number of publications increased each year from 2014 to 2023, indicating the continued interest of researchers in exploring the impact of VR on student engagement. When reviewing the impact of VR on student engagement, Wang and Degol’s (2014) article had the most citations at 450, suggesting that the article had a strong impact in the area of student use of VR in the classroom. The majority of articles had only 10 or fewer citations, which may have indicated that these articles were relatively new or had less impact in the field. It was worth noting that more recently published articles, such as Rzanova et al. (2023) , did not have enough time to accumulate citations, so their impact on the field may not have been fully reflected in current citations.
To summarize, the differences in the number of citations for these articles highlighted their different levels of influence in the area of VR’s impact on student engagement. However, there were some limitations to the review methods. For example, some articles might not have fully reflected their impact on the field in the current citations due to their short time frames, which might have resulted in less comprehensive findings. Furthermore, the literature included was small, and in the future consideration would be given to expanding the search of literature and databases, such as PubMed and Web of Science databases, as well as expanding the search with keywords, such as “students’ attitudes toward VR.” In addition, the inclusion and exclusion criteria might have limited the generalizability of the results of the review, and therefore more caution was needed when generalizing the results of the review.
The positive impact of VR on education
This section will discuss the impact of VR on students’ cognitive, behavioral, and affective engagement participation. It is important in the field of education. Radianti et al. (2020) noted that student engagement in educational settings was critical to learning outcomes and classroom climate. Yuan and Wang (2021) further noted that the combined effects of cognitive, behavioral, and affective engagement could directly impact student learning outcomes and classroom contextual experiences. Therefore, a deeper understanding of the impact of VR on these three dimensions of engagement can provide valuable insights into educational practices and help educators better optimize classroom environments and teaching methods.
First, Papanastasiou et al. (2019) noted that VR immersive learning experiences promoted students’ cognitive engagement and aided in understanding complex and abstract knowledge. That is, through immersive learning, students can understand and remember what they have learned in greater depth and increase cognitive engagement. Pellas (2016) also found that VR encouraged students to learn through self-directed inquiry and move away from traditional teacher-centered instruction. Pellas (2016) further explained that, through VR scenario reenactments and simulations, students could engage in real-world unavailable learning experiences such as exploring historical sites and visiting distant planets. This means that such learning experiences enable students to explore knowledge in deeper and more varied ways, thus increasing cognitive engagement. Similarly, Maples-Keller et al. (2017) showed that VR was beneficial in engaging different types of students in learning, particularly for at-risk students, including those with learning difficulties, anxiety disorders, and other mental illnesses. VR provided personalized and adaptive learning environments that helped students improve cognitive engagement and achievement ( Maples-Keller et al., 2017 ). In summary, VR facilitates understanding of complex knowledge and promotes cognitive engagement for different types of students through immersive learning experiences and self-directed inquiry learning.
Secondly, Pirker and Dengel (2021) demonstrated that VR could promote student behavioral engagement. They discussed the potential of immersive VR in education through an in-depth analysis of 64 articles. They showed that “learning tasks in 3-D VLEs can foster intrinsic motivation for and engagement with the learning content” (p.77). Sun and Peng (2020) also suggested that by combining classical educational concepts with VR, such as Confucianism’s promotion of teaching for fun, students were better able to engage in learning activities. For example, Rzanova et al. (2023) found that the use of VR in the teaching of poetry to create the scenarios depicted in the verses enabled students to actively participate in classroom activities. Similarly, Freina and Ott (2015) also found that by simulating real school escape scenarios in VR, students could take on different roles to perform escape drills, and this sense of behavioral engagement can help students better master escape techniques and enhance safety awareness. These articles seem to echo that VR helps to enhance student behavioral engagement.
It is worth noting that there is debate about whether VR has a positive impact on student behavioral engagement. Proponents noted that students’ hands-on experience and exploration in virtual environments stimulated interest and behavioral engagement ( Wong et al., 2010 ; Allcoat and Von Mühlenen, 2018 ). This view suggests that VR provides an immersive learning experience that enhances students’ motivation and promotes deeper engagement in classroom activities. However, contrary findings exist, suggesting that the use of VR may have some negative effects. For example, students might have become addicted to the virtual world and neglected their real-life tasks and responsibilities, thus affecting their behavior in the classroom ( Cheng et al., 2015 ; Greenwald et al., 2018 ; Makransky et al., 2019 ). In addition, some other scholars noted that there might have been a gap between learning experiences in virtual environments and real-world learning experiences, which might have affected students’ ability to acquire and apply knowledge ( Makransky and Petersen, 2021 ). These conflicting results remind us that these complexities and diversities need to be taken into account when evaluating the role of VR technology in improving student engagement in the classroom.
Finally, scholars such as Wu et al. (2013) , Schutte and Stilinović (2017) , and Yuen et al. (2011) found that VR helped to promote student affective engagement. For example, Schutte and Stilinović (2017) found that contexts provided by VR for children with emotional impairments or disabilities taught them skills in communicating with people and managing their emotions, thus fostering empathy. This implies that VR may stimulate affective engagement. Wu et al. (2013) and Yuen et al. (2011) also found that VR provided opportunities for affective interaction, enabling students to interact with characters in the virtual environment. In language learning, for example, practicing through conversations with virtual characters could help students improve their oral expression ( Dhimolea et al., 2022 ). This means that affective interactions may increase students’ affective engagement with the learning content. Similarly, Misak (2018) noted that VR allowed students to role-play in virtual literature and experience the affective portrayed in the story. In other words, affective experiences may deepen students’ understanding of literary works and increase affective engagement. This literature seems to reflect that VR can promote student affective engagement.
In general, VR positively impacts students’ cognitive, behavioral, and affective engagement. In terms of cognitive engagement, VR can facilitate students’ cognitive engagement with learning materials and better understanding of abstract and complex knowledge by creating immersive situations. In terms of behavioral engagement, VR stimulates active student engagement and action through interactive learning. Although there is debate about whether VR has a positive impact on student behavioral engagement, literature has demonstrated the positive impact of VR on student behavioral engagement. In terms of affective engagement, VR promotes students’ emotional engagement by triggering affective resonance through affective experience and affective interaction. This full engagement helps students improve their learning and develop empathy.
The following section discusses the challenges faced when introducing VR in education. Through understanding these challenges, we can better understand the problems in the education system and make some constructive suggestions to help address them.
The challenge of VR in education
Despite the positive impact of VR on students’ cognitive, behavioral, and affective engagement, there are still two challenges to introducing VR into middle education, namely the difficulty of the educational system in keeping up with VR developments and the lack of teacher proficiency in VR use ( Islam et al., 2015 ; Zhong, 2017 ; Abich et al., 2021 ). For example, Islam et al. (2015) observed that the pace of technological advancement, including VR, outpaced the ability of the education system to adapt. This phenomenon is due to the slow reform of the education system, which takes time for the acceptance and adoption of emerging technologies ( Islam et al., 2015 ). To this end, the education sector may take longer to standardize the syllabus, resulting in students not having immediate access to VR ( Zhong, 2017 ). In other words, students may not have the opportunity to experience VR in the classroom until the education department completes the standardization process. Sahlberg (2016) further stated that while reform and standardization in the education sector took time, once VR and the education system evolved in tandem, students benefited from an education that matched the VR of the day.
Other scholars observed that VR education faced several challenges in developing digital literacy in students ( Aviram and Eshet-Alkalai, 2006 ; Sahlberg, 2016 ). According to Reddy et al. (2020) , “digital literacy is a set of skills required by 21st Century individuals to use digital tools to support the achievement of goals in their life situations” (p. 66). Digital literacy encompasses the assessment of digital technologies, critical thinking, and the ability to create and express oneself digitally ( Reddy et al., 2020 ). For example, Tsivitanidou et al. (2021) and Necci et al. (2015) emphasized the need for students to identify the differences between the results of simulation experiments and real experiments and to assess the reliability and accuracy of simulation experiments. In other words, students need to judge the plausibility of the results of simulation experiments and interpret and evaluate those results in real-world situations.
Similarly, Farmer and Farmer (2023) found that digital literacy required students to master VR painting and sculpting tools to create art. This involved learning to select appropriate colors and textures and creating three-dimensional effects with VR tools ( Skulmowski et al., 2021 ). Meanwhile, Andone et al. (2018) further noted that students also needed to learn to share and present their work to others in virtual reality. This observation seems to reflect the high demand for students’ creativity, technical skills, and expressive abilities when introducing VR into education. In sum, while the development of VR education benefits students’ learning in conjunction with VR, there are challenges to students’ digital literacy and the technological adaptability of the education system.
In addition, teachers’ lack of proficiency in the use of VR is another major challenge in introducing VR into middle education. For example, Abich et al. (2021) found that teachers might lack proficiency in the operation and application of VR, which might result in teachers not being able to fully utilize VR to supplement instruction. Jensen and Konradsen (2018) claimed that “for HMDs to become a relevant tool for instructors they must have the ability to produce and edit their content” (p.1525). This means that teachers need to spend time familiarizing themselves with HMDs and related software to create, edit, and customize content to meet their specific instructional needs. Similarly, Fransson et al. (2020) discussed the challenges of teachers operating VR equipment and software. They interviewed 28 teachers to understand teachers’ challenges with implementing helmet display VR in educational settings. Fransson et al. (2020) indicated that there might be a technological threshold and learning curve for teachers in controlling and operating VR devices, which might affect the effective use of VR for teaching and learning.
While teachers may lack familiarity with VR, there are solutions to this challenge. For example, Alfalah (2018) noted that proper training and support could help teachers make the most of VR to supplement instruction. That is, teacher training can provide teachers with the technical knowledge and operational skills they need to familiarize themselves with how VR equipment and software work. To this end, Alfalah (2018) found the impact of providing teachers with VR training in schools. They used a quantitative approach by distributing a questionnaire online to 30 IT teachers. Alfalah (2018) indicated that “technology training may be maximized for the integration of VR technology” (P.2634). This finding seems to reflect that proper teacher training and support can be effective in helping teachers overcome the operational and application of VR technology’s difficulties.
In sum, prior literature has shown that introducing VR into middle school education faces several challenges. First, the rapid development of technology makes the educational system keep up with VR, resulting in a disconnect between the educational curriculum and VR. Second, there may be a lack of proficiency in students’ digital literacy and teachers’ handling and application of VR. However, these challenges are not insurmountable. With proper training and support, teachers can make full use of VR to supplement their teaching and learning to realize the potential of VR in education. It is worth noting that through the literature we have found that in practice, due to the rapid development of technology and the limitations of the educational system, achieving a complete balance may take some time and effort. Therefore, considering how to address the gap between the speed of VR development and the education system to better integrate and apply VR in education makes sense.
This article describes the impact of VR on student cognitive, behavioral, and affective engagement and the challenges posed by VR education. The literature review finds that using VR in the classroom can positively impact student engagement and learning outcomes. An interesting finding is that VR can be a promising tool for providing education to students with learning disabilities. For example, the previous literature review section describes how for students with learning difficulties, anxiety disorders, and other mental illnesses, VR can provide personalized and adaptive learning environments that can help students improve cognitive engagement and academic performance. And, for children with emotional disorders or disabilities, VR provides contexts that can teach them skills for communicating with others and managing their emotions, thereby developing empathy and stimulating affective engagement.
However, the potential problems with incorporating VR in middle education are the difficulty of the education system in keeping up with VR developments, the higher demands of student digital literacy, and the lack of teacher proficiency in the use of VR. These challenges require educational policymakers to provide training and technical support to teachers to ensure that they can fully master and integrate VR to improve student engagement and teaching effectiveness.
Author contributions
XL: Writing – original draft, Writing – review & editing. BL: Conceptualization, Writing – original draft, Writing – review & editing. ZNY: Writing – original draft, Writing – review & editing. ZY: Funding acquisition, Supervision, Writing – original draft, Writing – review & editing. MZ: Funding acquisition, Writing – original draft, Writing – review & editing, Supervision.
The author(s) declare financial support was received for the research, authorship, and/or publication of this article. This work was supported by the General Topics of China’s Hunan Province Social Science Achievement Evaluation Committee Fund [Grant no. XSP2023JYC123].
Acknowledgments
We are deeply appreciative of the editors and reviewers of this journal for their unwavering dedication and contributions that have shaped the publication of this article. Their constructive feedback and invaluable insights were instrumental in bringing this piece to fruition. We extend our heartfelt thanks to the readers with a keen interest in virtual reality technology. It is our sincere hope that this article will inspire enriched discussions within the academic community about the potential and nuances of using virtual reality in educational contexts.
Conflict of interest
The authors declare that the research was conducted in the absence of any commercial or financial relationships that could be construed as a potential conflict of interest.
Publisher’s note
All claims expressed in this article are solely those of the authors and do not necessarily represent those of their affiliated organizations, or those of the publisher, the editors and the reviewers. Any product that may be evaluated in this article, or claim that may be made by its manufacturer, is not guaranteed or endorsed by the publisher.
Abich, J., Parker, J., Murphy, J. S., and Eudy, M. (2021). A review of the evidence for training effectiveness with virtual reality technology. Virtual Reality 25, 919–933. doi: 10.1007/s10055-020-00498-8
Crossref Full Text | Google Scholar
Agbo, F. J., Sanusi, I. T., Oyelere, S. S., and Suhonen, J. (2021). Application of virtual reality in computer science education: a systemic review based on bibliometric and content analysis methods. Educ. Sci. 11, 1–23. doi: 10.3390/educsci11030142
Alfalah, S. F. (2018). Perceptions toward adopting virtual reality as a teaching aid in information technology. Educ. Inf. Technol. 23, 2633–2653. doi: 10.1007/s10639-018-9734-2
Allcoat, D., and Von Mühlenen, A. (2018). Learning in virtual reality: effects on performance, emotion, and engagement. Res. Learn. Technol. 26, 1–13. doi: 10.25304/rlt.v26.2140
Andone, D., Vert, S., Frydenberg, M., and Vasiu, R. (2018). Open virtual reality project to improve students’ skills. In 2018 IEEE 18th International Conference on Advanced Learning Technologies (ICALT) , 6–10. doi: 10.1109/ICALT.2018.00008
Aviram, A., and Eshet-Alkalai, Y. (2006). Towards a theory of digital literacy: three scenarios for the next steps. Eur. J. Open Distance E Learn 9, 1–11.
Google Scholar
Brooks, F. P. (1999). What's real about virtual reality? Institute of Electrical and Electronic Engineers (IEEE). Comput. Graph. Appl. 19, 16–27. doi: 10.1109/38.799723
Cheng, M.-T., Chen, J.-H., Chu, S.-J., and Chen, S.-Y. (2015). The use of serious games in science education: a review of selected empirical research from 2002 to 2013. J. Comput. Educ. 2, 353–375. doi: 10.1007/s40692-015-0039-9
Dhimolea, T. K., Kaplan-Rakowski, R., and Lin, L. (2022). A systematic review of research on high-immersion virtual reality for language learning. TechTrends 66, 810–824. doi: 10.1007/s11528-022-00717-w
Fransson, G., Holmberg, J., and Westelius, C. (2020). The challenges of using head mounted virtual reality in K-12 schools from a teacher perspective. Educ. Inf. Technol. 25, 3383–3404. doi: 10.1007/s10639-020-10119-1
Freina, L., and Ott, M. (2015). A literature review on immersive virtual reality in education: state of the art and perspectives. Int. Sci. Conf. E Learn. Softw. Educ. 1, 10–1007. doi: 10.12753/2066-026x-15-020
Grant, M. J., and Booth, A. (2009). A typology of reviews: an analysis of 14 review types and associated methodologies. Health Inf. Libr. J. 26, 91–108. doi: 10.1111/j.1471-1842.2009.00848.x
PubMed Abstract | Crossref Full Text | Google Scholar
Greenwald, S. W., Corning, W., Funk, M., and Maes, P. (2018). Comparing learning in virtual reality with learning on a 2D screen using electrostatics activities. J. Comput. Sci. 24, 220–245. doi: 10.3217/jucs-024-02-0220
Huvila, I. (2013). Sorting out the metaverse and how the metaverse is sorting us out . London: Palgrave Macmillan.
Islam, N., Beer, M., and Slack, F. (2015). E-learning challenges faced by academics in higher education. J. Educ. Train. Stud. 3, 102–112. doi: 10.11114/jets.v3i5.947
Jensen, L., and Konradsen, F. (2018). A review of the use of virtual reality head-mounted displays in education and training. Educ. Inf. Technol. 23, 1515–1529. doi: 10.1007/s10639-017-9676-0
Jeong, K., Kim, J., Kim, M., Lee, J., and Kim, C. (2019). Asymmetric interface: user interface of asymmetric virtual reality for new presence and experience. Symmetry 12, 1–25. doi: 10.3390/sym12010053
Lee, J., Kim, M., and Kim, J. (2017). A study on immersion and VR sickness in walking interaction for immersive virtual reality applications. Symmetry 9, 1–17. doi: 10.3390/sym9050078
Leidner, D. E., and Jarvenpaa, S. L. (1995). The use of information technology to enhance management school education: a theoretical view. Manag. Inf. Serv. Q. 19, 265–291. doi: 10.2307/249596
Makransky, G., and Petersen, G. B. (2021). The cognitive-affective model of immersive learning: a theoretical research-based model of learning in immersive virtual reality. Educ. Psychol. Rev. 33, 937–958. doi: 10.1007/s10648-020-09586-2
Makransky, G., Terkildsen, T. S., and Mayer, R. E. (2019). Adding immersive virtual reality to a science lab simulation causes more presence but less learning. Learn. Instr. 60, 225–236. doi: 10.1016/j.learninstruc.2017.12.007
Maples-Keller, J. L., Bunnell, B. E., Kim, S.-J., and Rothbaum, B. O. (2017). The use of virtual reality technology in the treatment of anxiety and other psychiatric disorders. Harv. Rev. Psychiatry 25, 103–113. doi: 10.1097/HRP.0000000000000138
Misak, J. (2018). A (virtual) bridge not too far: teaching narrative sense of place with virtual reality. Comput. Compos. 50, 39–52. doi: 10.1016/j.compcom.2018.07.007
Moher, D., Liberati, A., Tetzlaff, J., and Altman, D. G.PRISMA Group* (2009). Preferred reporting items for systematic reviews and meta-analyses: the PRISMA statement. Ann. Intern. Med. 151, 264–269. doi: 10.7326/0003-4819-151-4-200908180-00135
Mystakidis, S., Berki, E., and Valtanen, J. P. (2021). Deep and meaningful e-learning with social virtual reality environments in higher education: a systematic literature review. Appl. Sci. 11, 1–25. doi: 10.3390/app11052412
Necci, A., Cozzani, V., Spadoni, G., and Khan, F. (2015). Assessment of domino effect: state of the art and research needs. Reliab. Eng. Syst. Saf. 143, 3–18. doi: 10.1016/j.ress.2015.05.017
Papanastasiou, G., Drigas, A., Skianis, C., Lytras, M., and Papanastasiou, E. (2019). Virtual and augmented reality effects on K-12, higher, and tertiary education students’twenty-first-century skills. Virtual Reality 23, 425–436. doi: 10.1007/s10055-018-0363-2
Pellas, N. (2016). “Unraveling a progressive inquiry script in persistent virtual worlds: theoretical foundations and decision processes for constructing a socio-cultural learning framework” in Web design and development: Concepts, methodologies, tools, and applications (Pennsylvania, US: IGI Global), 610–647.
Pirker, J., and Dengel, A. (2021). The potential of 360 virtual reality videos and real VR for education—a literature review. IEEE Comput. Graph. Appl. 41, 76–89. doi: 10.1109/MCG.2021.3067999
Pottle, J. (2019). Virtual reality and the transformation of medical education. Future Healthcare J. 6, 181–185. doi: 10.7861/fhj.2019-0036
Poux, F., Valembois, Q., Mattes, C., Kobbelt, L., and Billen, R. (2020). Initial user-centered design of a virtual reality heritage system: applications for digital tourism. Remote Sens. 12, 1–25. doi: 10.3390/rs12162583
Radianti, J., Majchrzak, T. A., Fromm, J., and Wohlgenannt, I. (2020). A systematic review of immersive virtual reality applications for higher education: design elements, lessons learned, and research agenda. Comput. Educ. 147, 103778–103729. doi: 10.1016/j.compedu.2019.103778
Reddy, P., Sharma, B., and Chaudhary, K. (2020). Digital literacy: a review of literature. Int. J. Technoethics (IJT) 11, 65–94. doi: 10.4018/IJT.20200701.oa1
Reid, J. M. (1987). The learning style preferences of English as a second language (ESL) students. Teach. Engl. Speakers Other Lang. Q. 21, 87–111. doi: 10.2307/3586356
Rzanova, S., Yushchik, E., Markova, S., and Sergeeva, A. (2023). Impact of virtual reality technologies in the context of the case method on engineering students’ competencies. Educ. Inf. Technol. 7, 1–19. doi: 10.56028/aetr.7.1.7.2023
Sahlberg, P. (2016). The global educational reform movement and its impact on schooling. In: K. Mundy, A. Green, B. Lingard, and A. Verger The Handbook of Global Education Policy . Hoboken, NJ: John Wiley and Sons, 128–144.
Sanchez-Vives, M. V., and Slater, M. (2005). From presence to consciousness through virtual reality. Nat. Rev. Neurosci. 6, 332–339. doi: 10.1038/nrn1651
Schutte, N. S., and Stilinović, E. J. (2017). Facilitating empathy through virtual reality. Motiv. Emot. 41, 708–712. doi: 10.1007/s11031-017-9641-7
Skulmowski, A., Nebel, S., Remmele, M., and Rey, G. D. (2021). Is a preference for realism really naive after all? A cognitive model of learning with realistic visualizations. Educ. Psychol. Rev. 34, 1–27. doi: 10.1007/s10648-021-09638-1
Song, C., Shin, S. Y., and Shin, K. S. (2023). Optimizing foreign language learning in virtual reality: a comprehensive theoretical framework based on constructivism and cognitive load theory. Appl. Sci. 13, 1–31. doi: 10.3390/app132312557
Steuer, J., Biocca, F., and Levy, M. R. (1995). Communication in the age of virtual reality , New York: Routledge
Sun, S. Y., and Peng, L. H. (2020). Study of the virtual reality education and digitalization in China. J. Physics 1456, 012042–012047. doi: 10.1088/1742-6596/1456/1/012042
Tsivitanidou, O. E., Georgiou, Y., and Ioannou, A. (2021). A learning experience in inquiry-based physics with immersive virtual reality: student perceptions and an interaction effect between conceptual gains and attitudinal profiles. J. Sci. Educ. Technol. 30, 841–861. doi: 10.1007/s10956-021-09924-1
United Kingdom Authority. (2019). VR and AR attract education sector interest . Available at: https://www.ukauthority.com/articles/vr-and-ar-attract-education-sector-interest/ .
Wang, M. T., and Degol, J. (2014). Staying engaged: knowledge and research need in student engagement. Child Dev. Perspect. 8, 137–143. doi: 10.1111/cdep.12073
Wong, B. M., Etchells, E. E., Kuper, A., Levinson, W., and Shojania, K. G. (2010). Teaching quality improvement and patient safety to trainees: a systematic review. Acad. Med. 85, 1425–1439. doi: 10.1097/ACM.0b013e3181e2d0c6
Wu, H.-K., Lee, S. W.-Y., Chang, H.-Y., and Liang, J.-C. (2013). Current status, opportunities and challenges of augmented reality in education. Comput. Educ. 62, 41–49. doi: 10.1016/j.compedu.2012.10.024
Yuan, H., and Wang, Z. (2021). A review of research on technology enhancing Chinese learning . 2021 international conference on internet, education and information technology (IEIT), pp. 462–467.
Yuen, S. C.-Y., Yaoyuneyong, G., and Johnson, E. (2011). Augmented reality: an overview and five directions for augmented reality (AR) in education. J. Educ. Technol. Dev. Exchange 4, 119–140. doi: 10.18785/jetde.0401.10
Zhong, L. (2017). Indicators of digital leadership in the context of K-12 education. J. Educ. Technol. Dev. Exchange 10, 27–40. doi: 10.18785/jetde.1001.03
Keywords: virtual reality technology, cognitive engagement, affective engagement, behavioral engagement, learning outcomes
Citation: Lin XP, Li BB, Yao ZN, Yang Z and Zhang M (2024) The impact of virtual reality on student engagement in the classroom–a critical review of the literature. Front. Psychol . 15:1360574. doi: 10.3389/fpsyg.2024.1360574
Received: 23 December 2023; Accepted: 22 March 2024; Published: 10 April 2024.
Reviewed by:
Copyright © 2024 Lin, Li, Yao, Yang and Zhang. This is an open-access article distributed under the terms of the Creative Commons Attribution License (CC BY) . The use, distribution or reproduction in other forums is permitted, provided the original author(s) and the copyright owner(s) are credited and that the original publication in this journal is cited, in accordance with accepted academic practice. No use, distribution or reproduction is permitted which does not comply with these terms.
*Correspondence: Zhi Yang, [email protected] ; Mingshu Zhang, [email protected]
† These authors have contributed equally to this work and share first authorship
Disclaimer: All claims expressed in this article are solely those of the authors and do not necessarily represent those of their affiliated organizations, or those of the publisher, the editors and the reviewers. Any product that may be evaluated in this article or claim that may be made by its manufacturer is not guaranteed or endorsed by the publisher.
Staying Engaged: Knowledge and Research Needs in Student Engagement
Ming-te wang.
School of Education, Learning Research and Development Center, Department of Psychology, University of Pittsburgh
Jessica Degol
School of Education, University of Pittsburgh
In this article, we review knowledge about student engagement and look ahead to the future of study in this area. We begin by describing how researchers in the field define and study student engagement. In particular, we describe the levels, contexts, and dimensions that constitute the measurement of engagement, summarize the contexts that shape engagement and the outcomes that result from it, and articulate person-centered approaches for analyzing engagement. We conclude by addressing limitations to the research and providing recommendations for study. Specifically, we point to the importance of incorporating more work on how learning-related emotions, personality characteristics, prior learning experiences, shared values across contexts, and engagement in nonacademic activities influence individual differences in student engagement. We also stress the need to improve our understanding of the nuances involved in developing engagement over time by incorporating more extensive longitudinal analyses, intervention trials, research on affective neuroscience, and interactions among levels and dimensions of engagement.
Over the past 25 years, student engagement has become prominent in psychology and education because of its potential for addressing problems of student boredom, low achievement, and high dropout rates. When students are engaged with learning, they can focus attention and energy on mastering the task, persist when difficulties arise, build supportive relationships with adults and peers, and connect to their school ( Wang & Eccles, 2012a , 2012b ). Therefore, student engagement is critical for successful learning ( Appleton, Christenson, & Furlong, 2008 ). In this article, we review research on student engagement in school and articulate the key features of student engagement. In addition, we provide recommendations for research on student engagement to address limits to our understanding, apply what we have learned to practice, and focus on aspects that warrant further investigation.
KEY FEATURES OF STUDENT ENGAGEMENT
Engagement is distinct from motivation.
Engagement is a broadly defined construct encompassing a variety of goal-directed behaviors, thoughts, or affective states ( Fredricks, Blumenfeld, & Paris, 2004 ). Although definitions of engagement vary across studies ( Reschly & Christenson, 2012 ), engagement is distinguished from motivation. A common conceptualization, though not universally established, is that engagement is the effort directed toward completing a task, or the action or energy component of motivation ( Appleton et al., 2008 ). For example, motivation has been defined as the psychological processes that underlie the energy, purpose, and durability of activities, while engagement is defined as the outward manifestation of motivation ( Skinner, Kindermann, Connell, & Wellborn, 2009 ). Engagement can take the form of observable behavior (e.g., participation in the learning activity, on-task behavior), or manifest as internal affective (e.g., interest, positive feelings about the task) and cognitive (e.g., metacognition, self-regulated learning) states ( Christenson et al., 2008 ). Therefore, when motivation to pursue a goal or succeed at an academic task is put into action deliberately, the energized result is engagement.
Engagement Is Multilevel
Engagement is a multilevel construct, embedded within several different levels of increasing hierarchy ( Eccles & Wang, 2012 ). Researchers have focused on at least three levels in relation to student engagement ( Skinner & Pitzer, 2012 ). The first level represents student involvement within the school community (e.g., involvement in school activities). The second level narrows the focus to the classroom or subject domain (e.g., how students interact with math teachers and curriculum). The third level examines student engagement in specific learning activities within the classroom, emphasizing the moment-to-moment or situation-to-situation variations in activity and experience.
Engagement Is Multidimensional
Although most researchers agree that student engagement is multidimensional, consensus is lacking over the dimensions that should be distinguished ( Fredricks et al., 2004 ). Most models contain both a behavioral (e.g., active participation within the school) and an emotional (e.g., affective responses to school experiences) component ( Finn, 1989 ). Other researchers have identified cognitive engagement as a third factor that incorporates mental efforts that strengthen learning and performance, such as self-regulated planning and preference for challenge ( Connell & Wellborn, 1991 ; Wang, Willett, & Eccles, 2011 ). Although not as widely recognized, a fourth dimension, agentic engagement , reflects a student’s direct and intentional attempts to enrich the learning process by actively influencing teacher instruction, whereas behavioral, emotional, and cognitive engagement typically represent student reactions to classroom experiences ( Reeve & Tseng, 2011 ). Given the variety of definitions of engagement throughout the field, researchers must specify their dimensions and ensure that their measures align properly with these descriptions of engagement.
Engagement Is Malleable
Student engagement is shaped by context, so it holds potential as a locus for interventions ( Wang & Holcombe, 2010 ). When students have positive learning experiences, supportive relationships with adults and peers, and reaffirmations of their developmental needs in learning contexts, they are more likely to remain actively engaged in school ( Wang & Eccles, 2013 ). Structural features of schools (e.g., class size, school location) have also been attributed to creating an educational atmosphere that influences student engagement and achievement. However, structural characteristics may not directly alter student engagement, but may in fact alter classroom processes, which in turn affect engagement ( Benner, Graham, & Mistry, 2008 ).
Several aspects of classroom processes are central to student engagement. For example, engagement is greater in classrooms where tasks are hands-on, challenging, and authentic ( Marks, 2000 ). Teachers who provide clear expectations and instructions, strong guidance during lessons, and constructive feedback have students who are more behaviorally and cognitively engaged ( Jang, Reeve, & Deci, 2010 ). Researchers have also linked high parental expectations to persistence and interest in school ( Spera, 2005 ), and linked high parental involvement to academic success and mental health both directly and indirectly through behavioral and emotional engagement ( Wang & Sheikh-Khalil, 2014 ). Conceptualizing student engagement as a malleable construct enables researchers to identify features of the environment that can be altered to increase student engagement and learning.
Engagement Predicts Student Outcomes
Student engagement is a strong predictor of educational outcomes. Students with higher behavioral and cognitive engagement have higher grades and aspire to higher education ( Wang & Eccles, 2012a ). Emotional engagement is also correlated positively with academic performance ( Stewart, 2008 ). Student engagement also operates as a mediator between supportive school contexts and academic achievement and school completion ( Wang & Holcombe, 2010 ). Therefore, increasing student engagement is a critical aspect of many intervention efforts aimed at reducing school dropout rates ( Archambault, Janosz, Morizot, & Pagani, 2009 ; Christenson & Reschly, 2010 ; Wang & Fredricks, 2014 ). Moreover, engagement is linked to other facets of child development. Youth with more positive trajectories of behavioral and emotional engagement are less depressed and less likely to be involved in delinquency and substance abuse ( Li & Lerner, 2011 ). School disengagement has been linked to negative indicators of youth development, including higher rates of substance use, problem behaviors, and delinquency ( Henry, Knight, & Thornberry, 2012 ). Some of these associations may actually be reciprocal, so that high engagement may lead to greater academic success, and greater academic success may then lead to even greater academic engagement ( Hughes, Luo, Kwok, & Loyd, 2008 ).
Engagement Comes in Qualitatively Different Patterns
Using person-centered approaches to study engagement advances our understanding of student variation in multivariate engagement profiles and the differential impact of these profiles on child development. One study ( Wang & Peck, 2013 ) used latent profile analysis to classify students into five groups of varying patterns of behavioral, emotional, and cognitive engagement, which were associated differentially with educational and psychological functioning. For example, a group of emotionally disengaged youth was identified (high behavioral and cognitive engagement, but low emotional engagement) with grade point averages and dropout rates comparable to those of the highly engaged group of youth (high on all three dimensions). However, despite their academic success, the emotionally disengaged students had a greater risk of poor mental health, reporting higher rates of symptoms of depression than any other group. Furthermore, growth mixture modeling analysis with a combined measure of behavioral, cognitive, and emotional engagement showed that unlike most individuals who experienced high to moderately stable trajectories of engagement throughout adolescence, many students experienced linear or nonlinear growth or declines ( Janosz, Archambault, Morizot, & Pagani, 2008 ). Students with unstable patterns of engagement were more likely to drop out. These developmental patterns and profiles cannot be detected by variable-centered approaches that focus on population means and overlook heterogeneity across groups. As person-centered research becomes more common, targeted intervention programs should be more effective at serving unique subgroups of students with specific developmental needs.
Disengagement Is More Than the Lack of Engagement
One of the inconsistencies found in the research is whether we should distinguish engagement from disengagement and measure these constructs on the same continuum or as separate continua. Most studies consider engagement as the opposite of disengagement with lower levels of engagement indicating more disengagement. However, some researchers have begun to view disengagement as a separate and distinct psychological process that makes unique contributions to academic outcomes, not simply as the absence of engagement ( Jimerson, Campos, & Greif, 2003 ). For example, behavioral and emotional indicators of engagement (e.g., effort, interest, persistence) and disaffection (e.g., withdrawal, boredom, frustration) can be treated as separate constructs, indicating that although similar, engagement and disaffection do not overlap completely ( Skinner, Furrer, Marchand, & Kindermann, 2008 ). Researchers should incorporate separate measures of engagement and disengagement into their work to determine the unique contributions of each construct to academic, behavioral, and psychological outcomes.
LOOKING AHEAD
Although we know much from research on student engagement, a number of areas require clarification and expansion.
Affective Arousal and Engagement
Emotions in educational contexts can enhance or impede learning by shaping the motivational and cognitive strategies that individuals use when faced with a new challenge. Negative emotions such as anxiety may interfere with performing a task by reducing the working memory, energy, and attention directed at completing the task, whereas positive emotions such as enjoyment, hope, and pride may increase performance by focusing attention on the task and promoting adaptive coping strategies ( Pekrun, Goetz, Titz, & Perry, 2002 ; Reschly, Huebner, Appleton, & Antaramian, 2008 ). However, much of the work on emotions and engagement focuses on general dispositions toward the learning environment, such as measuring interest in or valuing of school ( Stewart, 2008 ). Far less is known about how students’ actual emotions or affective states during specific learning activities influence their academic engagement and achievement ( Linnenbrink-Garcia & Pekrun, 2011 ). Researchers rarely measure how emotions relate to subsequent engagement, relying predominantly on retrospective student self-reports to measure affective states. Useful supplements to students’ reports would be psychophysiological indicators of emotional distress (e.g., facial expression, heart rate) and experience sampling methods to assess situational emotional states during classroom activities.
With the advancement of brain imaging technology, neuroimaging studies show that affective states during learning are important in determining how efficiently the brain processes new information ( Schwabe & Wolf, 2012 ). Although neuroimaging cannot be used to measure classroom engagement in real time, neuroscience techniques are valuable tools that may advance our understanding of how emotional experiences shape neural processing of information and affect engagement during a task. For example, do prolonged states of boredom in the classroom actually alter the shape and functionality of the brain over time, and can we intervene in these processes to reverse the negative effects of boredom or apathy? We also need a more thorough understanding of how genetic predispositions and environmental conditions interact to alter brain chemistry. Studies should identify precursors to or triggers for negative affective experiences, and identify environmental supports that can eliminate these negative emotions, foster adaptive coping strategies, and increase learning engagement and performance.
Interactions Among Levels
Engagement is represented at many hierarchical levels in the educational environment (e.g., school, classroom, momentary level). However, researchers rarely frame their conceptualizations and assessments of engagement in terms of a hierarchical system or process, so we lack understanding about how student engagement at these various levels interacts to influence performance. Learning is a continuous developmental process, not an instantaneous event, and engagement is the energy that directs mental, behavioral, and psychological faculties to the learning process. By focusing on only one level of engagement, we understand little about the process through which engagement is formed and leads ultimately to academic achievement.
Are there reciprocal interrelations between more immediate states of engagement and broader representations, such that moment-to-moment engagement within the classroom informs feelings and behaviors toward the school as a whole, which then trickle down to influence momentary classroom engagement through a continuous feedback loop? Are these levels additive or multiplicative, such that higher engagement across the board is associated with better academic outcomes than high engagement at only one or two levels? Or does engagement at one level compensate for lower engagement at another level, demonstrating that high engagement across all levels is not necessary for optimal functioning? Broadening the focus of research to incorporate engagement at many micro and macro levels of the educational context would advance our understanding of how different levels develop and interact to shape student engagement, and the differential pathways that lead to academic success.
Development of Many Dimensions
Despite the consensus over the multidimensionality of student engagement, the role that each dimension plays in shaping academic outcomes remains unclear ( Skinner et al., 2008 ). Three avenues warrant exploration: (a) independent relations, (b) emotional engagement (which drives behavioral and cognitive engagement), and (c) reciprocal relations.
Independent relations suggest that each dimension of engagement makes unique contributions to student functioning. In other words, high behavioral engagement cannot compensate for the effects of low emotional engagement, given that both shape student outcomes independently.
The second avenue posits that emotional engagement could be a prerequisite for behavioral and cognitive engagement. According to this viewpoint, students who enjoy learning should participate in classroom activities more often and take more ownership over their learning. Emotional engagement sets the stage for developing cognitive and behavioral processes of student engagement.
The third possibility suggests bidirectional relations among the organizational constructs of engagement, with each dimension influencing the others cyclically. For example, enjoyment of learning or high emotional engagement may lead to greater use of self-regulated learning strategies or cognitive engagement and greater behavioral engagement within the classroom. This increased behavioral participation and use of cognitive strategies to improve performance may elicit positive feedback from classmates and teachers, further increasing enjoyment of learning, and so on. With reciprocal relations, each process reinforces and feeds into the others. For researchers to understand the developmental progression of engagement over time, they should tease apart the unique versus compounded effects of each dimension of engagement.
Longitudinal Research Across Developmental Periods
Some research on how student engagement unfolds and changes over time has shown average declines in various indicators of engagement throughout adolescence and in the transition to secondary school ( Wang & Eccles, 2012a , 2012b ), but other studies have shown heterogeneity in engagement patterns across subgroups of individuals ( Archambault et al., 2009 ; Janosz et al., 2008 ; Li & Lerner, 2011 ). However, we know little about developmental trajectories of engagement spanning early childhood to late adolescence. Many studies track engagement only in early adolescence across a span of 3 or 4 years. Because the ability to become a self-regulated learner, set goals, and monitor progress advances as children mature and become active agents in their own learning, student engagement may take different forms in elementary school than it does in subsequent years ( Fredricks et al., 2004 ). Researchers should investigate how younger versus older students think of engagement, how engagement changes across developmental periods, and whether sociocultural and psychological factors differentially shape engagement at the elementary and secondary levels.
Students’ Prior Learning Experiences
Researchers should also explore the role of students’ previous learning experiences in shaping engagement. When students are confronted with new academic challenges, the emotions and cognitions attached to previous experiences should influence how they adjust or cope with these challenges. In particular, engagement and academic achievement decline during school transitions (e.g., elementary to middle school, middle school to high school), which can be stressful experiences for many students ( Eccles et al., 1993 ; Pekrun, 2006 ). Students with prior experiences of failure in school may be especially vulnerable to the alienating effects of school transitions. How do we discontinue students’ negative feelings about schoolwork and reengage them in their education? How do we maintain positive and engaging experiences for students through every grade level and every transition? Using students’ prior learning experiences to break the cycle of disengagement and strengthen the cycle of continuous interest and engagement could inform interventions, particularly during crucial transitory periods when students are most vulnerable to feelings of isolation, boredom, or alienation.
Intervention
Despite the malleability of student engagement and the connection between developmental contexts and engagement, very few theory- and evidence-based preventative programs have been developed, implemented, and tested on a large scale. A few interventions have increased student engagement. For example, Check & Connect, an evidence-based intervention program, has reduced rates of dropout and truancy, particularly for students at high risk of school failure ( Reschly & Christenson, 2012 ). Randomized control trials of schoolwide positive behavioral support programs have also improved student engagement and achievement, reducing discipline referrals and suspensions ( Horner et al., 2009 ; Ward & Gersten, 2013 ). However, many programs are small, intensive interventions that have not been implemented on a larger scale, raising concerns about implementation fidelity and reduced effectiveness. Many interventions also rely on one dose of services and track developmental changes over a short period, making it difficult to infer long-term benefits.
We need to develop comprehensive programs that adapt to the unique needs of individuals receiving services. Preventative programs often rely on one-size-fits-all models, so subgroups of students may not be served properly. Although universal interventions are beneficial for students in general, targeted programs might be more effective for students at greater risk of academic or psychological problems. Therefore, interventions should be implemented at many levels, incorporating a universal program for students in general and more selected services for at-risk students.
Engagement Across Contexts
We should also explore the relative alignment of educational messages, values, and goals across contexts and how this compatibility influences student engagement. Teachers, parents, and peers are not always in tune with each other over educational values, and these conflicting messages may impair how students engage fully with school. For example, parents might endorse educational excellence as a priority, whereas peers may endorse academic apathy. In these situations, students may have to set aside their personal values and pursue or coordinate the values of others, or try to integrate their personal values with the values of the other group. Students’ ability to coordinate the messages, goals, and values from different agents in their social circles will also determine how they see themselves as learners.
We lack studies on how students reconcile inconsistencies in these messages across groups and how it affects their engagement. If peer groups promote antiachievement goals that are directly in conflict with the educational ideals transmitted by parents, will students conform to peer norms or seek out friends with achievement values that are more aligned with the values endorsed by their families? Is misalignment of educational goals across social contexts a risk factor for school dropout, particularly among students from disadvantaged backgrounds? Researchers need to address this area to help students cope with the inconsistent messages about education in their social circles and to consolidate a stronger academic identity.
Student Character and Engagement
Although researchers have examined how contextual, sociocultural, and motivational factors influence student engagement, the influence of student character or personality factors is less well understood. Research on the Big Five personality traits has found conscientiousness, an indicator of perseverance, to be the most consistent predictor of academic achievement ( Poropat, 2009 ).
Persistence has been examined through grit , a characteristic that entails working passionately and laboriously to achieve a long-term goal, and persisting despite challenges, setbacks, or failures ( Duckworth, Peterson, Matthews, & Kelly, 2007 ). Individuals with grit are more likely to exert effort to prepare and practice to achieve their goals, leading them to be more successful than individuals who use less effortful strategies ( Duckworth, Kirby, Tsukayama, Berstein, & Ericsson, 2011 ).
Nevertheless, we know little about how personality traits might interact with environmental contexts to shape student engagement. Additionally, researchers have yet to examine how profiles of personality traits might interact with each other to influence student engagement. More nuanced research in these areas will aid in the development of learning strategies and educational contexts that may yield the most successful outcomes for various personality types.
Beyond Academic Engagement
Research on student engagement has focused on academic engagement or academic-related activities. Although academic experiences are critical determinants of educational success, school is also a place where students socialize with their friends and engage in nonacademic activities. Focusing exclusively on academic engagement neglects the school’s role as a developmental context in which students engage in a wide range of academic, social, and extracurricular activities that shape their identities as academically capable, socially integrated individuals who are committed to learning. For example, students who struggle with academic learning but are athletic may experience more engagement on the football field than in the classroom. Through participating in these types of nonacademic social activities, students build skills and learn life lessons such as collaborating as a team and becoming a leader. Thus, students’ schooling experiences should involve many forms of engagement, including academic, social, and extracurricular engagement. More research is needed to integrate these forms of engagement in school and examine how they interact to influence students’ academic and socioemotional well-being collectively.
Since its conception more than two decades ago, research on student engagement has permeated the fields of psychology and education. Over this period, we have learned much about engagement. We know that engagement can be measured as a multidimensional construct, including both observable and unobservable phenomena. We have come to appreciate the importance of engagement in preventing dropout and promoting academic success. We also understand that engagement is responsive to variations in classroom and family characteristics.
But in spite of the accrued knowledge on engagement, we have barely scratched the surface in understanding how engagement and disengagement can affect academic development, and how engagement unfolds over time by tracking interactions across contexts, dimensions, and levels. We also cannot dismiss the personal traits and affective states that students bring to the classroom, which may influence engagement regardless of the supportive nature of the environment. We lack knowledge about the extent to which large-scale interventions can produce long-term improvements in engagement across diverse groups. As we move forward with engagement research, we must apply what we have learned and focus on aspects that warrant further exploration. The insight this research provides will allow educators to create supportive learning environments in which diverse groups of students not only stay engaged but also experience the academic learning and success that is a byproduct of continuous engagement.
Acknowledgments
This project was supported by Grant DRL1315943 from the National Science Foundation and Grant DA034151-02 from the National Institute on Drug Abuse at the National Institute of Health to Ming-Te Wang.
Contributor Information
Ming-Te Wang, School of Education, Learning Research and Development Center, Department of Psychology, University of Pittsburgh.
Jessica Degol, School of Education, University of Pittsburgh.
- Appleton JJ, Christenson SL, Furlong MJ. Student engagement with school: Critical conceptual and methodological issues of the construct. Psychology in the Schools. 2008; 45 :369–386. [ Google Scholar ]
- Archambault I, Janosz M, Morizot J, Pagani L. Adolescent behavioral, affective, and cognitive engagement in school: Relationship to dropout. Journal of School Health. 2009; 79 :408–415. [ PubMed ] [ Google Scholar ]
- Benner AD, Graham S, Mistry RS. Discerning direct and mediated effects of ecological structures and processes on adolescents’ educational outcomes. Developmental Psychology. 2008; 44 :840–854. [ PubMed ] [ Google Scholar ]
- Christenson SL, Reschly AL. Check & Connect: Enhancing school completion through student engagement. In: Doll E, Charvat J, editors. Handbook of prevention science. New York, NY: Routledge; 2010. pp. 327–348. [ Google Scholar ]
- Christenson SL, Reschly AL, Appleton JJ, Berman-Young S, Spaniers DM, Varro P. Best practices in fostering student engagement. In: Thomas A, Grimes J, editors. Best practices in school psychology. 5th. Bethesda, MD: National Association of School Psychologists; 2008. pp. 1099–1119. [ Google Scholar ]
- Connell JP, Wellborn JG. Competence, autonomy, and relatedness: A motivational analysis of self-system processes. In: Gunnar MR, Sroufe LA, editors. Self processes in development: Minnesota symposium on child psychology. Vol. 23. Chicago, IL: University of Chicago Press; 1991. pp. 43–77. [ Google Scholar ]
- Duckworth AL, Kirby T, Tsukayama E, Berstein H, Ericsson KA. Deliberate practice spells success: Why grittier competitors triumph at the National Spelling Bee. Social Psychological and Personality Science. 2011; 2 :174–181. [ Google Scholar ]
- Duckworth AL, Peterson C, Matthews MD, Kelly DR. Grit: Perseverance and passion for long-term goals. Journal of Personality and Social Psychology. 2007; 92 :1087–1101. [ PubMed ] [ Google Scholar ]
- Eccles JS, Midgley C, Wigfield A, Buchanan CM, Reuman D, Flanagan C, Mac Iver D. Development during adolescence: The impact of stage-environment fit on young adolescents’ experiences in schools and in families. American Psychologist. 1993; 48 :90–101. [ PubMed ] [ Google Scholar ]
- Eccles JS, Wang M-T. Part I Commentary: So what is student engagement anyway? In: Christenson SL, Reschly AL, Wylie C, editors. Handbook of research on student engagement. New York, NY: Springer; 2012. pp. 133–148. [ Google Scholar ]
- Finn JD. Withdrawing from school. Review of Educational Research. 1989; 59 :117–142. [ Google Scholar ]
- Fredricks JA, Blumenfeld PC, Paris AH. School engagement: Potential of the concept, state of the evidence. Review of Educational Research. 2004; 74 :59–109. [ Google Scholar ]
- Henry KL, Knight KE, Thornberry TP. School disengagement as a predictor of dropout, delinquency, and problem substance use during adolescence and early adulthood. Journal of Youth and Adolescence. 2012; 41 :156–166. [ PMC free article ] [ PubMed ] [ Google Scholar ]
- Horner RH, Sugai G, Smolkowski K, Eber L, Nakasato J, Todd AW, Esperanza J. A randomized, wait-list controlled effectiveness trial assessing school-wide positive behavior support in elementary schools. Journal of Positive Behavior Interventions. 2009; 11 :133–144. [ Google Scholar ]
- Hughes JN, Luo W, Kwok OM, Loyd LK. Teacher-student support, effortful engagement, and achievement: A 3-year longitudinal study. Journal of Educational Psychology. 2008; 100 :1–14. [ PMC free article ] [ PubMed ] [ Google Scholar ]
- Jang H, Reeve J, Deci EL. Engaging students in learning activities: It is not autonomy support or structure but autonomy support and structure. Journal of Educational Psychology. 2010; 102 :588–600. [ Google Scholar ]
- Janosz M, Archambault I, Morizot J, Pagani LS. School engagement trajectories and their differential predictive relations to dropout. Journal of Social Issues. 2008; 64 :21–40. [ Google Scholar ]
- Jimerson SR, Campos E, Greif JL. Toward an understanding of definitions and measures of school engagement and related terms. The California School Psychologist. 2003; 8 :7–27. [ Google Scholar ]
- Li Y, Lerner RM. Trajectories of school engagement during adolescence: Implications for grades, depression, delinquency, and substance use. Developmental Psychology. 2011; 47 :233–247. [ PubMed ] [ Google Scholar ]
- Linnenbrink-Garcia L, Pekrun R. Students’ emotions and academic engagement: Introduction to the special issue. Contemporary Educational Psychology. 2011; 36 :1–3. [ Google Scholar ]
- Marks HM. Student engagement in instructional activity: Patterns in the elementary, middle, and high school years. American Educational Research Journal. 2000; 37 :153–184. [ Google Scholar ]
- Pekrun R. The control-value theory of achievement emotions: Assumptions, corollaries, and implications for educational research and practice. Educational Psychology Review. 2006; 18 :315–341. [ Google Scholar ]
- Pekrun R, Goetz T, Titz W, Perry RP. Academic emotions in students’ self-regulated learning and achievement: A program of qualitative and quantitative research. Educational Psychologist. 2002; 37 :91–105. [ Google Scholar ]
- Poropat AE. A meta-analysis of the five-factor model of personality and academic performance. Psychological Bulletin. 2009; 135 :322–338. [ PubMed ] [ Google Scholar ]
- Reeve J, Tseng CM. Agency as a fourth aspect of students’ engagement during learning activities. Contemporary Educational Psychology. 2011; 36 :257–267. [ Google Scholar ]
- Reschly AL, Christenson SL. Jingle, jangle, and conceptual haziness: Evolution and future directions of the engagement construct. In: Christenson SL, Reschly AL, Wylie C, editors. Handbook of research on student engagement. New York, NY: Springer; 2012. pp. 3–20. [ Google Scholar ]
- Reschly AL, Huebner ES, Appleton JJ, Antaramian S. Engagement as flourishing: The contribution of positive emotions and coping to adolescents’ engagement at school and with learning. Psychology in the Schools. 2008; 45 :419–431. [ Google Scholar ]
- Schwabe L, Wolf OT. Stress modulates the engagement of multiple memory systems in classification learning. Journal of Neuroscience. 2012; 32 :11042–11049. [ PMC free article ] [ PubMed ] [ Google Scholar ]
- Skinner EA, Furrer C, Marchand G, Kindermann T. Engagement and disaffection in the classroom: Part of a larger motivational dynamic? Journal of Educational Psychology. 2008; 100 :765–781. [ Google Scholar ]
- Skinner EA, Kindermann TA, Connell JP, Wellborn JG. Engagement and disaffection as organizational constructs in the dynamics of motivational development. In: Wentzel K, Wigfield A, editors. Handbook of motivation at school. Mahwah, NJ: Erlbaum; 2009. pp. 223–245. [ Google Scholar ]
- Skinner EA, Pitzer JR. Developmental dynamics of student engagement, coping, and everyday resilience. In: Christenson SL, Reschly AL, Wylie C, editors. Handbook of research on student engagement. New York, NY: Springer; 2012. pp. 21–44. [ Google Scholar ]
- Spera C. A review of the relationship among parenting practices, parenting styles, and adolescent school achievement. Educational Psychology Review. 2005; 17 :125–146. [ Google Scholar ]
- Stewart EB. School structural characteristics, student effort, peer associations, and parental involvement: The influence of school- and individual-level factors on academic achievement. Education and Urban Society. 2008; 40 :179–204. [ Google Scholar ]
- Wang M-T, Eccles JS. Adolescent behavioral, emotional, and cognitive engagement trajectories in school and their differential relations to educational success. Journal of Research on Adolescence. 2012a; 22 :31–39. [ Google Scholar ]
- Wang M-T, Eccles JS. Social support matters: Longitudinal effects of social support on three dimensions of school engagement from middle to high school. Child Development. 2012b; 83 :877–895. [ PubMed ] [ Google Scholar ]
- Wang M-T, Eccles JS. School context, achievement motivation, and academic engagement: A longitudinal study of school engagement using a multidimensional perspective. Learning and Instruction. 2013; 28 :12–23. [ Google Scholar ]
- Wang M-T, Fredricks JA. The reciprocal links between school engagement, youth problem behaviors, and school dropout during adolescence. Child Development. 2014; 85 :722–737. [ PMC free article ] [ PubMed ] [ Google Scholar ]
- Wang M-T, Holcombe R. Adolescents’ perceptions of school environment, engagement, and academic achievement in middle school. American Educational Research Journal. 2010; 47 :633–662. [ Google Scholar ]
- Wang M-T, Peck SC. Adolescent educational success and mental health vary across school engagement profiles. Developmental Psychology. 2013; 49 :1266–1276. [ PubMed ] [ Google Scholar ]
- Wang M-T, Sheikh-Khalil S. Does parental involvement matter for student achievement and mental health in high school? Child Development. 2014; 85 :610–625. [ PubMed ] [ Google Scholar ]
- Wang M-T, Willett JB, Eccles JS. The assessment of school engagement: Examining dimensionality and measurement invariance by gender and race/ethnicity. Journal of School Psychology. 2011; 49 :465–480. [ PubMed ] [ Google Scholar ]
- Ward B, Gersten R. A randomized evaluation of the Safe and Civil Schools model for positive behavioral interventions and supports at elementary schools in a large urban school district. School Psychology Review. 2013; 42 :317–333. [ Google Scholar ]

Translate this page
Hundreds of scientific articles. written for kids. approved by scientists. free., search or filter, search articles, reading level.
- Elementary school (29)
- Lower high school (246)
- Middle school (183)
- Upper high school (95)
Scientific Topic
- Biodiversity and Conservation (85)
- Biology (69)
- Chemistry (13)
- Energy and Climate (57)
- Food and Agriculture (26)
- Health and Medicine (80)
- Paleoscience (18)
- Physical Science (18)
- Pollution (30)
- Social Science (55)
- Technology (22)
- Water Resources (48)
- All NGSS standards 2-LS4 (2) 3-5-ETS1 (3) 3-LS2 (1) 3-LS3 (2) 3-LS4 (3) 4-ESS3 (3) 4-LS1 (3) 4-PS3 (1) 4-PS4 (1) 5-ESS1 (1) 5-ESS3 (2) 5-PS1 (1) HS-ESS1 (3) HS-ESS2 (19) HS-ESS3 (81) HS-ESS4 (1) HS-ETS1 (12) HS-LS1 (72) HS-LS2 (84) HS-LS3 (27) HS-LS4 (48) HS-PS1 (5) HS-PS2 (2) HS-PS3 (5) HS-PS4 (3) MS-ESS1 (5) MS-ESS2 (14) MS-ESS3 (77) MS-ETS1 (6) MS-LS1 (66) MS-LS2 (74) MS-LS3 (14) MS-LS4 (23) MS-PS1 (3) MS-PS2 (1) MS-PS3 (4) MS-PS4 (3)
- All AP Environmental Science topics 1. The Living World: Ecosystems (68) 2. The Living World: Biodiversity (70) 3. Populations (142) 4. Earth Systems and Resources (30) 5. Land and Water Use (67) 6. Energy Resources and Consumption (18) 7. Atmospheric Pollution (11) 8. Aquatic and Terrestrial Pollution (45) 9. Global Change (97)
- All IB Biology topics 01. Cell biology (2) 02. Molecular biology (38) 03. Genetics (41) 04. Ecology (28) 05. Evolution and biodiversity (32) 06. Human physiology (67) 07. Nucleic acids (5) 08. Metabolism, cell respiration and photosynthesis (9) 09. Plant biology (7) 10. Genetics and evolution (12) 11. Animal physiology (57) A. Neurobiology and behaviour (42) B. Biotechnology and bioinformatics (59) C. Ecology and conservation (6) D. Human physiology (12)
- All Scientific Methods Agricultural yield data (6) Bacterial culture (1) Case study (47) Cell culture (5) Chromatography (2) Climate scenarios (25) Community jury (1) Controlled blinded study (2) Correlational study (1) CRISPR (1) CT (1) Data extrapolation (44) Data reconstruction (19) Data validation (35) DNA sequencing (36) ELISA (6) Experiment (135) Expert committee (1) Field study (40) Fluorescence imaging (11) Gene editing (11) GIS (3) GPS tracking (12) High resolution filming (1) Life cycle assessment (2) Mark-release-recapture (2) Mass spectrometry (11) Meta-analysis (4) Microscopy (22) Observation (47) Onsite study (1) PCR (Polymerase chain reaction) (16) Policy analysis (23) Proxy data (22) Radiocarbon dating (2) Remote sensing (1) Representative sampling (84) Risk analysis (9) Scientific modeling (115) Spectroscopy (1) Survey research (28) Systematic review (20) Tomography scans (2) Tree core sampling (4) X-ray scanner (2)
- All Scientific Figures Bar graph (101) Box and whisker plot (7) Data table (28) Dendrogram (1) Flow chart (4) Histogram (8) Line graph (26) Map (103) Microscopy image (31) Pictograph (85) Pie chart (11) Scatter plot (26) Time series graph (36) Venn diagram (4)
- Research Location Africa (40) Antarctica (2) Asia (34) Australia (15) Europe (75) North America (149) Ocean (38) South America (18) Space (5)
- All Scientist Affiliations Aarhus University (1) Adam Mickiewicz University (1) Aix-Marseille University (1) Amherst College (1) Arizona State University (4) Baylor University (1) Boston University (1) Brown University (1) California Institute of Technology (3) California State University (1) Cardiff University (2) CDC (3) Cedars-Sinai Medical Center Los Angeles (1) Centre for Communicable Diseases Bangladesh (1) China Agricultural University (1) Chinese Academy of Sciences (1) City University of New York (1) Clemson University (2) Colorado State University (4) Columbia University (1) Complutense University (1) Cornell University (7) CSIRO (1) Duke University (6) Emory University (1) ETH Zurich (2) Five Gyres Institute (1) Forest Research Institute of Baden-Wuerttemberg (1) George Washington University (2) Georgetown University (2) Georgia Institute of Technology (1) Harvard University (10) Hebrew University of Jerusalem (1) Hokkaido University (1) IFPRI (1) Imperial College London (8) Indian Institute of Technology (1) INRS (3) Iowa State University (3) IRSTEA (1) ISAC (1) Johannes Kepler University (2) Johns Hopkins University (4) Katholieke Universiteit Leuven (1) King's College London (1) Kyoto University (1) Lancaster University (2) Lawrence Berkeley National Laboratory (2) Lehigh University (1) Leibniz Institute for Zoo and Wildlife Research (2) Leipzig University (1) London School of Economics (1) LSHTM (5) LSTM (1) Marine Biological Association UK (1) Max Planck Institute (4) McGill University (3) Merrimack College (1) Met Office UK (1) Missouri University of Science and Technology (1) MIT (4) Monash University (1) Murdoch Children's Research Institute (2) Museo Paleontológico Egidio Feruglio (1) Myongji University (1) Nanjing University (1) NASA (6) National Autonomous University of Mexico (1) National Clinical Research Center for Respiratory Diseases (1) National University of Ireland (1) Natural Resources Canada (2) New York University Abu Dhabi (1) NOAA (8) North Carolina State University (1) Northeastern University (2) Norwegian Institute for Nature Research (1) Norwegian University of Science and Technology (1) Oregon State University (1) Oswaldo Cruz Foundation (1) PATH (1) Peking University School of Public Health (1) Pennsylvania State University (4) Pepperdine University (1) Princeton University (2) Purdue University (1) Queen Mary University of London (2) Queensland University of Technology (1) RMIT University (1) Royal Netherlands Institute for Sea Research (1) RPI (1) RTI International (1) Rutgers University (1) San Diego State University (1) Seattle Pacific University (1) Stanford University (8) Stockholm University (2) Swedish University of Agricultural Sciences (1) Tampere University (1) Technical University of Denmark (1) Texas A&M University (3) The Francis Crick Institute (1) Trent University (1) Tulane University (2) UC Berkeley (10) UC Davis (4) UC Irvine (1) UC Los Angeles (6) UC Riverside (2) UC San Diego (2) UC Santa Barbara (1) UC Santa Cruz (2) UKCEH (1) United Nations Children’s Fund (1) Universidad Nacional de Colombia (1) Universit of Minnessotta (1) Universität of Osnabrück (1) University of Alaska (1) University of Alberta (1) University of Arizona (1) University of Arkansas (2) University of Auckland (1) University of Bern (1) University of Birmingham (2) University of Bologna (1) University of Bristol (1) University of British Columbia (4) University of Cambridge (3) University of Cape Town (1) University of Central Florida (1) University of Chicago (2) University of Cincinnati (1) University of Connecticut (1) University of Copenhagen (1) University of Dar es Salaam (1) University of East Anglia (1) University of Edinburgh (1) University of Exeter (1) University of Florida (5) University of Georgia (1) University of Groningen (1) University of Hawaii (1) University of Helsinki (2) University of Houston (2) University of Illinois (1) University of Konstanz (1) University of Lausanne (1) University of Leeds (1) University of Liverpool (1) University of London (2) University of Maine (1) University of Manitoba (1) University of Maryland (1) University of Miami (1) University of Michigan (2) University of Minnesota (2) University of Missouri (1) University of Montreal (2) University of Nebraska (1) University of New England Australia (2) University of North Carolina (2) University of Northern Colorado (1) University of Oklahoma (1) University of Otago (1) University of Oviedo (1) University of Oxford (3) University of Patras (1) University of Pennsylvania (2) University of Pittsburgh (1) University of Plymouth (5) University of Potsdam (1) University of Queensland (1) University of Reading (1) University of Rochester (1) University of Sassari (1) University of South Carolina (1) University of South Florida (1) University of Southern California (2) University of Southern Denmark (1) University of Southern Mississippi (1) University of Strathclyde (1) University of Texas (9) University of the Witwatersrand (2) University of Tokyo (1) University of Toulouse (3) University of Tübingen (1) University of Vienna (1) University of Virginia (2) University of Warwick (1) University of Washington (8) University of Western Australia (3) University of Wisconsin-Milwaukee (1) University of York (1) University of Zurich (3) Uppsala University (1) USDA (1) USDA-ARS (2) UT Austin (1) Utah State University (1) Utrecht University (1) Virginia Commonwealth University (1) Virginia Tech (1) Wageningen University (2) Washington University (1) Weill Cornell Medicine (1) West Virginia University (1) Wildlife Conservation Society (1) Woods Hole Oceanographic Institution (1) World Bank (1) World Health Organization (1) Yale University (5)
- All Additional Languages Afrikaans (1) Arabic (5) Bengali (1) Bulgarian (37) Burmese (1) Chinese (4) Danish (1) Dutch (3) French (17) German (4) Greek (1) Italian (1) Korean (1) Lao (1) Mongolian (1) Portuguese (2) Russian (2) Spanish (51) Swedish (1)
Articles by Publication Date
Where was the research.

How can doctors treat endometriosis?
+ Audio version of article + Links to activities about reproductive health and macrophages

How do bumble bees play?
+ Audio version of article + Links to lessons on bees and animal behavior + Blackboard version of article with bee footage

How can we tell whether we are talking to a computer or a person?
+ Audio version of article + Links to activities about AI and machine learning + Blackboard video version of article

How have the life choices of women in India changed over time?
+ Audio version of article + Links to lessons on demographics and empowering girls + Lesson Idea video

How do animals behave during a solar eclipse?
+ Audio version of article + Get involved: Link to citizen science projects about eclipses + Links to activities about solar eclipses

How do dolphin mothers speak with their babies?
+ Audio version of article + Links to lessons on dolphins and echolocation

What do parenting and brain size have to do with each other?
+ Audio version of article + Two reading levels available + Links to lessons on birds and brains

How does fear speech spread on social media?
+ Audio version of article + Links to lessons on identifying hate speech and misinformation + Link to lessons on how kids can navigate social media
Popular Articles

Why do measles survivors get sicker?

What can we learn from carbon on Mars?

What can we learn about dinosaur skin using a laser?

How well can a computer think?
Article collections.

Socially Important Scientific Research in 2024 Election Year

Gross and Disgusting Science Articles Collection

8 Research Articles on Women and Girls

High School Intro Biology Collection
Popular lesson ideas.

Data Graphing Activity for 4th-7th Graders

Articles Translated in Multiple Languages

SJK Recommends: The World’s Best STEM Resources. Free.

Ask-A-Scientist Podcast E2: Dr. Allison Master, social psychologist
Learn more about us, funding support by:.

Disclaimer: Funders play no role in article selection and writing.
Featured by:

DPT research pairs students with faculty

Article By: Clark Leonard
Students in the University of North Georgia's (UNG) Doctor of Physical Therapy (DPT) program are gaining valuable experience thanks to the research and innovation track within the DPT.
After researching alongside Dr. Stanislaw Solnik , associate professor of physical therapy, Dr. Adrien Buttram, '23, presented at two conferences and published an article in the Journal of Biomechanics, a top-tier journal in the field of rehabilitation and orthopedics. Other students who helped with the research and article were Ben Thomas, JT Hagar and Stephanie Gibson, and students who served as data collectors were Damon Knighton, Karlie Abernathy and José Canelon.

The group examined the impact of certain activities on movement variability. Buttram said the research helps her as a physical therapist when she is testing patients to rule in or rule out a certain problem.
"Now I know how these tests were formulated and put into practice," Buttram said. "I didn't appreciate how much went into every test we do until I did this research."
Buttram took part in every step of the research, from finding subjects, giving tests, collecting and analyzing the data to making the poster.
"Each year, several faculty incorporate students into their ongoing scholarly agenda to pique student interest in contributing to the body of PT evidence in the future. Each patient can be thought of as a single-subject research study deserving of a similar careful gathering of data and analysis of results as would be included in a formal study," Dr. Kimberly Castle , professor and department head of physical therapy, said. "We also encourage students to consider further training in the future to perform independent research studies by pursuing PT residencies or terminal academic degrees."
Solnik said the research students are able to conduct within the DPT program helps them in their future careers.
"These projects we do with students, we try to make sure they are clinically relevant," Solnik said. "The field of physical therapy is changing. There's more and more pressure on physical therapy to become precision-based and evidence-based."
Maddison Holder, a third-year DPT student from Swainsboro, Georgia, conducted survey research along with Dr. Brian Maloney , assistant professor of physical therapy; Dr. Myra Lisa Barnes-Foster, professor of physical therapy; Dr. Don Walsh , associate professor of physical therapy; and fellow students David Park and Rebekah Cox to see how physical therapy and PT assistant programs were teaching aquatic physical therapy and the impact of COVID-19 on their delivery of that instruction.
The group's research was accepted to the Combined Sections Meeting, and Maloney presented it at the national conference. Their scholarly work filled a gap as research on the topic of aquatic physical therapy in academic curricula was limited in the past few decades. It was a new subject for Holder too, but now she feels better equipped for what comes next.
"I can advocate for the importance of aquatic physical therapy," Holder said.
Dr. Andrzej Przyzbyla , professor of physical therapy, has also had multiple recent research projects involving his students.
"I always want to offer the opportunity for students to be part of the research process when they are able to," Przyzbyla said. "On top of presenting, students have an excellent opportunity to network."
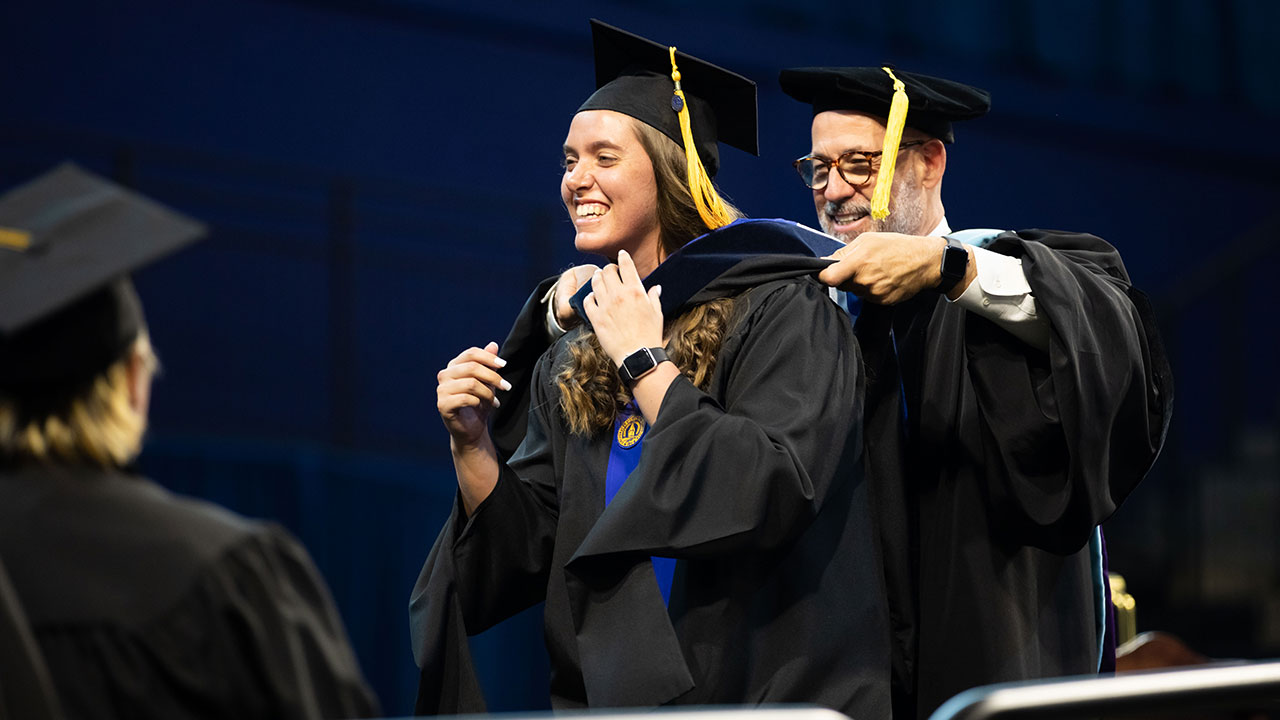
Commencement ceremonies scheduled for May 2-3
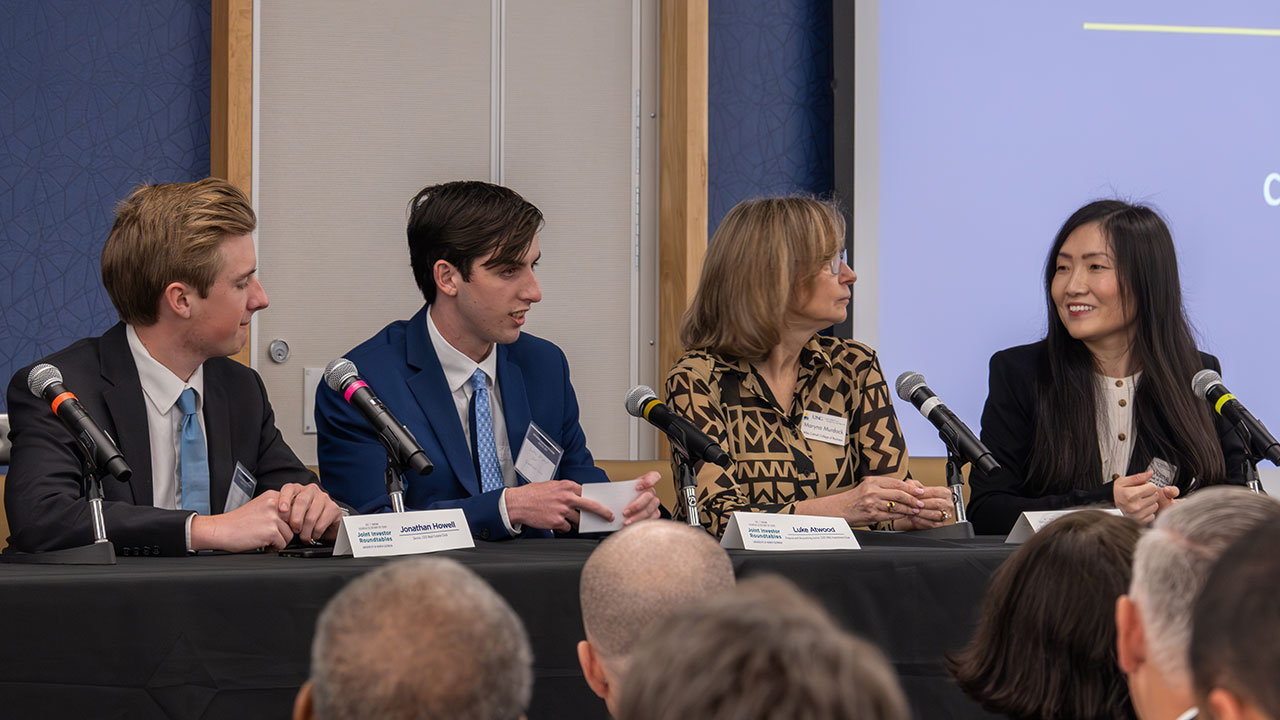
Students, faculty speak at investor roundtables
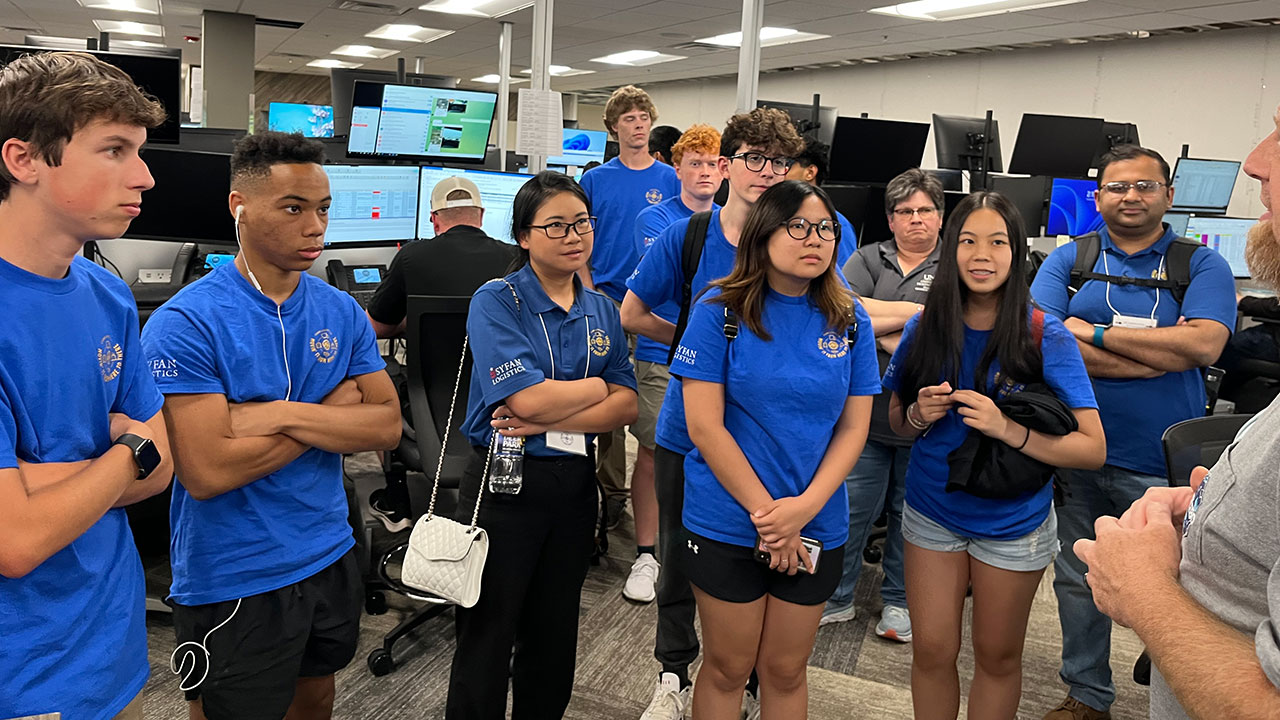
Camp Logistics is set for June 24-28
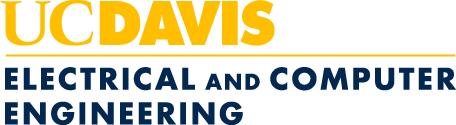
UC Davis ECExpo 2024: Uniting Industry and Academia through Innovation
- by Katherine Panaligan
- April 15, 2024
On April 5, Kemper Hall buzzed with the exchange of new ideas as the Department of Electrical and Computer Engineering at the University of California, Davis, hosted ECExpo 2024. The event was a vibrant meeting ground for over 150 industry partners, students, alumni and faculty, dedicated to showcasing the department's latest research and technological strides.
Stephan Schell, one of the department's two distinguished alumni of 2023 , opened the event with his keynote, "From Academia to Apple: A Journey of Innovation and Discovery." Schell discussed the career path that led him from being a student in the department to 10 years at Apple, where he helped develop devices like the iPhone and AirPods. He retired from Apple in 2015 as a senior director of wireless systems architecture and with the prestigious, director-like title of Distinguished Engineer, Scientist, Technologist, or DEST.
"Schell was a really remarkable speaker," said Billy Putnam , assistant professor of electrical and computer engineering and chair of the ECExpo planning committee. "He could really connect with everyone in our audience: undergraduates, graduate students, industry folks and faculty."
Following Schell's keynote, Assistant Professor Yubei Chen , a recent addition to the ECE faculty, delivered a presentation on "Self-Supervised Learning: The Principles and the Frontier," igniting conversations on the future of artificial intelligence. Chen's talk focused on the current state of supervised learning — a process for training AI systems using labeled datasets — and how this method may be improved with self-supervised learning principles, which mimic natural learning by training the AI systems to build upon inputted data.
Department Chair André Knoesen followed Chen to provide an update on the ECE department, which included enrollment figures, faculty, alumni and student awards for 2023.
Distinguished Professor S. J. Ben Yoo and Professor Saif Islam were next. They discussed the department's participation in the CHIPS and Science Act of 2022 and related workforce development programs launched by federal agencies. The Department of Defense Microelectronics Commons has designated UC Davis as part of the California-Pacific-Northwest AI-Hardware Hub . The hub, led by Stanford University and the UC Berkeley, is poised to propel AI hardware advancements while fostering workforce development and streamlining the journey from laboratory research to fabrication.
On the topic of workforce development, Islam spoke about the Center for Information Technology in the Interest of Society, or CITRIS. As its director, he mentioned how the center is pursuing innovative seed project proposals that integrate community colleges and K-12 institutions into the broader CHIPS and Science initiatives. Projects like this will play a pivotal role in crafting a skilled and ready workforce for the technological challenges of tomorrow.
Closing the afternoon's presentations, Professor Jeremy Munday provided a brief overview of the Center for Nano- and Micro-Manufacturing , or CNM2 — a 24/7 cleanroom that received a $20 million renovation in 2018.
At the event, students also had the opportunity to showcase their research during the student poster session. About 40 students presented and discussed their research with fellow undergraduates and graduate students within the College of Engineering, which ranged from robotic arms to gesture recognition technology. The poster session was followed by an ECExpo Industry Immersion networking session and social mixer hosted by the IEEE Student Club and ECE Graduate Student Association.
Watch Stephan Schell's full keynote
The cornerstone of ECExpo is the involvement of industry partners who actively engage with students, offering insights and demonstrations on emerging technologies.
"Several companies set up tables and showed off really exciting demonstrations of some of their products, and a handful of our excellent senior design project teams showcased really neat projects they're building," Putnam said. "We always have new and exciting things happening at ECExpo."
ECExpo 2024 was sponsored by Chevron, Anritsu, Digikey, Dell, Keysight, Texas Instruments and Silvaco.
View all photos from ECExpo
Primary Category
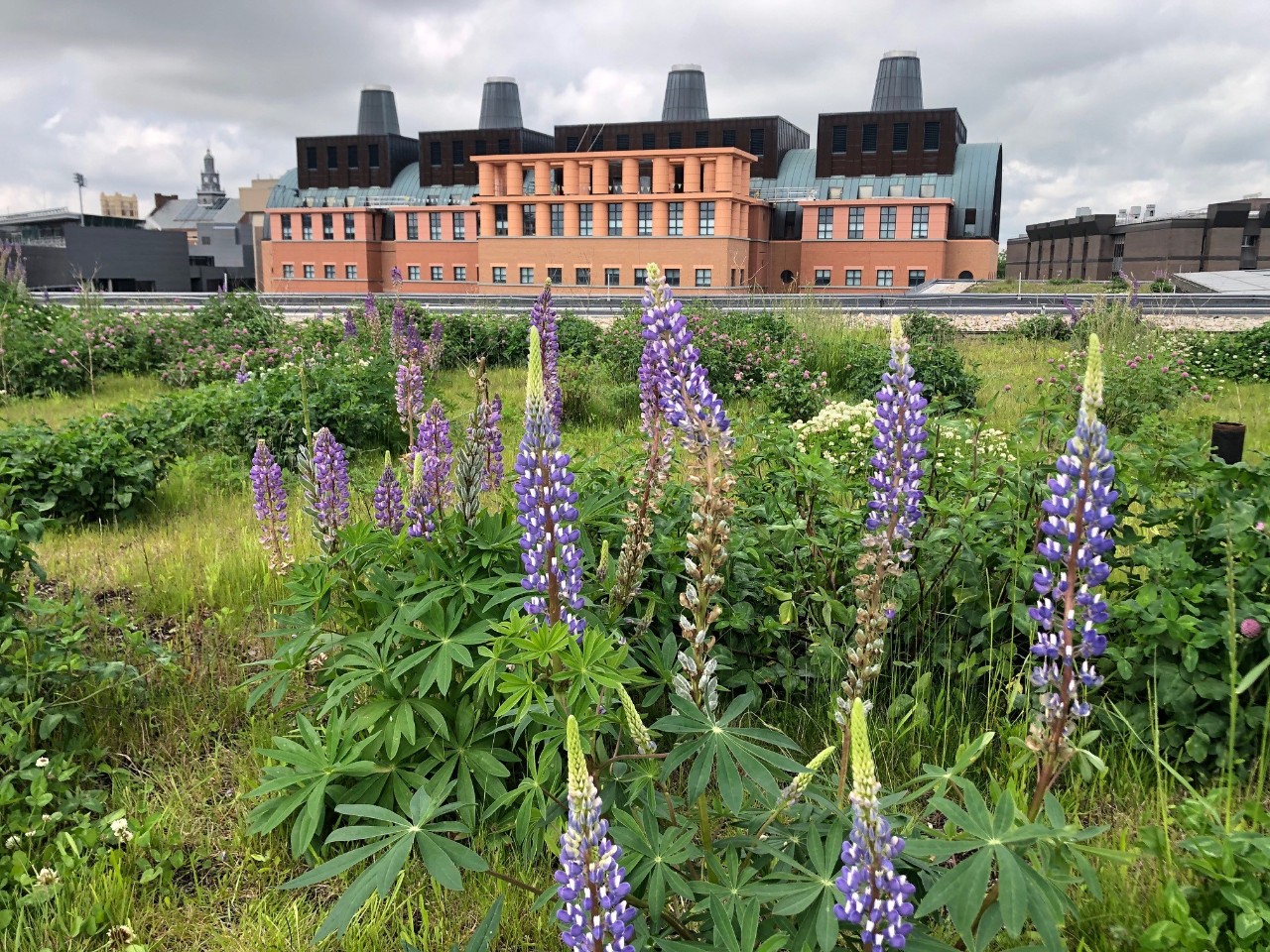
UC engineering co-op medalist excels through 6 co-op experiences
Jaclyn bashore was awarded the herman schneider medal for co-op excellence.
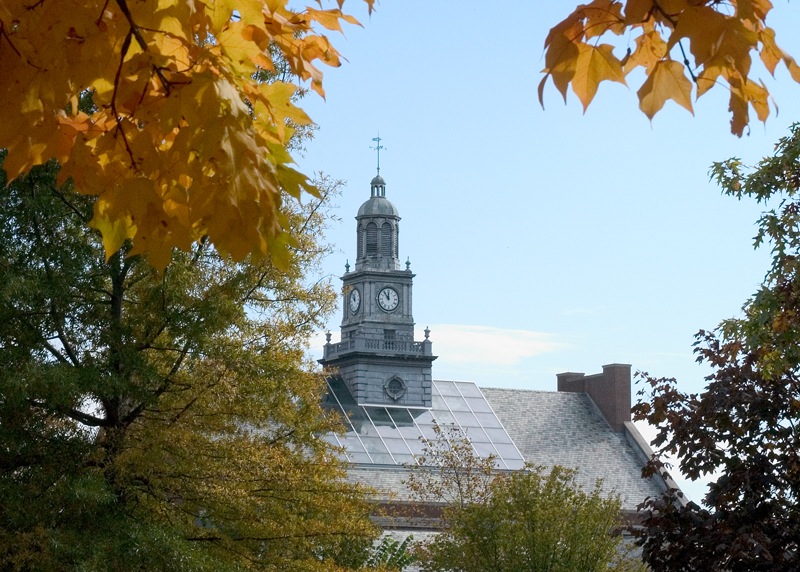
2024 Herman Schneider award recipient Jaclyn Bashore.
Ever since she garnered an appreciation for chemistry in high school, fifth-year University of Cincinnati student Jaclyn Bashore has not looked back.
When she graduates with a chemical engineering degree and a minor in chemistry this spring, Bashore will have had six co-op experiences — along with a new full-time job at AbbVie, a biopharmaceutical research company with whom she did two co-op rotations. To commemorate her exceptional co-op work, the UC College of Engineering and Applied Science awarded Bashore the 2024 Herman Schneider Medal, an annual award given to a graduating senior who demonstrates outstanding success as a co-op student.
Jaclyn Bashore poses with the Herman Schneider statue outside of UC's Baldwin Hall.
When the time came to make a college decision, Bashore didn’t have to look far from her home in Tipp City, Ohio. In addition to enjoying the vibrant atmosphere of campus, Bashore was particularly drawn to UC because of the top-ranking cooperative education (co-op) program. The program, which enables students to gain practical real-world experience as they rotate semesters in the classroom with semesters of full-time, paid, co-op work, entrusts students with important and meaningful work from the get-go. Though College of Engineering and Applied Science (CEAS) students typically do five co-op rotations, Bashore completed six. She was eager to learn as much as she could, so she took the first opportunity she had to accelerate her career readiness.
“I did my first co-op with P&G as a consumer data analyst in the summer of freshman year, even though that is the one summer we have off here as engineering students,” Bashore said. “It was during the initial onset of COVID and a lot of it was remote, but I was eager to get my first look into the professional world, and I ended up learning a lot about professional communication in an industry setting.”
After acquiring skills in data analysis, coding tools and instructional media production during her time at P&G, Bashore transitioned into a more hands-on role as a process engineer with KraussMaffei, a plastics engineering extrusion company in Florence, Kentucky. Though she recognized that putting together and taking apart machines was a whole new ball game compared to coding, Bashore was able to quickly see tangible results from her work. During her two co-op rotations with KraussMaffei, she led research trials on customers and reported on extrusion trends to inform department strategy. She even prevented six-figure product damage costs to the organization by ensuring the correct alignment of screw elements and equipment pieces for water supply lines.
Jaclyn Bashore at AbbVie's Waco, Texas, campus.
Armed with three full-time co-op experiences in just two years, Bashore next found herself in Chicago as a global packaging engineer for AbbVie, the first of her two rotations with the company. From the start, Bashore knew her experience would be rewarding.
“My first exposure to AbbVie was when I visited their campus in Chicago. I thought the giant campus of 60 buildings was crazy cool — it was the first time I’d ever been on a campus that big, because every other place was just one building,” Bashore said. “The capacity alone was inspiring, but when I saw their impact on patient lives directly, I was even more excited. Seeing firsthand how medicine can positively affect people was really inspiring.”
During her first stint with AbbVie, Bashore worked on labelling technology for thermochromic ink labels that indicate temperature and time for vaccine syringes that have been exposed to various elements. Her investigative and design work resulted in AbbVie saving over $9 million per year in potential costs. Similar to her previous co-ops, Bashore further cemented her research and communication skills by partaking in several shipping studies for drug products and authoring supplemental documentation.
For her next co-op rotation, Bashore joined the Jacobs Engineering Group, an engineering consulting firm in Cincinnati. At Jacobs, she contributed to a large-scale biopharmaceutical plant design project that was built in Limerick, Ireland, for Eli Lilly. During construction of the plant, which was created to manufacture new medication to treat Alzheimer’s disease, Bashore collaborated cross-functionally to manage Leadership in Energy and Environmental Design (LEED) certifications for seven project sites, in addition to ensuring minimal environmental impact for the construction project.
Jaclyn Bashore visiting Baylor University in Waco, Texas.
For her final co-op rotation, Bashore returned to AbbVie to work at its Waco, Texas, location as a process engineer. She notably created a skills matrix, or the system outlining operational standards, and increased its product output by over 3.6 million units.
“My second stint was a lot more hands-on, because I was directly in the manufacturing plant, whereas initially I was splitting my time between labs and my desk to help out with documentation,” Bashore said. “Going from occasionally seeing and interacting with plants to being on the fast-paced plant floor was a totally different experience, but it was a lot of fun. The people and the passion of the organization made it so easy to be genuinely involved.”
In addition to her class and co-op work, Bashore served as an undergraduate research assistant for chemical engineering professor Jonathan Nickels , with her research focusing on molecular energy transfer and supporting the Cincinnati Children’s Hospital Medical Center. Balancing out the many responsibilities of her co-ops with the demands of the classroom and beyond was often difficult for Bashore. However, she was able to find motivation and further her sense of community and purpose by extending her involvement outside of school and work.
It’s always really rewarding to see the direct impact of your actions. I want to inspire others in the same ways that I was inspired.
Jaclyn Bashore, CEAS '24
“During my freshman year, I was required to volunteer because of my Cincinnatus scholarship,” Bashore said. “As I continued to dabble throughout volunteering opportunities, I noticed that I would go past the required hours. Volunteering introduced me to some amazing experiences and some of my best friends.”
Jaclyn Bashore poses with the Bearcat.
Some of Bashore’s favorite volunteering experiences include her time with the student-led Clean Up Cincy and with Sweet Cheeks Diaper Bank, an organization that provides women’s wellness and family assistance. She also has served her community as a member of Tau Beta Pi, the Society of Women Engineers and the Omega Chi Epsilon Honor Society, among other organizations. As a result of her widespread community involvement, she was selected to be a constituent on the American Institute of Chemical Engineers Philanthropy Committee. She was named to the Senior 100, which celebrates graduating UC students for their service and leadership.
“It’s always really rewarding to see the direct impact of your actions. I want to inspire others in the same ways that I was inspired,” Bashore said. “It’s not always easy to balance it all, but it’s definitely worth it. Along with journaling and my faith, being a catalyst to help others keeps me going.”
Upon graduating this spring, Bashore will return to AbbVie’s Waco plant as a full-time employee in its departmental rotation program. As she looks toward her future, she is filled with excitement and gratitude.
Jaclyn Bashore with her parents.
“When I think about where I was freshman year compared to where I am now, it’s a little hard to believe,” Bashore said. “I never even thought I’d co-op outside of Cincinnati, since I used to be so adamant that I’d never leave. It’s hard to find the words to sum up so many feelings, but without any piece of it, I wouldn’t have the whole puzzle. I wouldn’t be here without the people around me, and I’m excited to continue learning and growing.”
Featured image at top: UC's Mantei Center. Photo/John Martini
Become a Bearcat
Whether you’re a first-generation student or from a family of Bearcats, UC is proud to support you at every step along your journey. We want to make sure you succeed — and feel right at home.
- Student Experience
- Experience-based Learning
- College of Engineering and Applied Science
- Chemical and Environmental Engineering
Related Stories
Undergraduate research co-ops inspire environmental engineer.
April 8, 2021
Megan Naber hopes to put her engineering degree to good use by finding new ways to reduce the environmental problems that arise from the agriculture industry. Naber, an environmental engineering student at the University of Cincinnati, is motivated by her passion for the natural world and her experiences as an undergraduate researcher. Naber is simultaneously working on two degrees as part of the UC College of Engineering and Applied Science ACCEND accelerated degree program.
Engineering student shifts from the stage to the lab
May 14, 2021
Garek Bushnell came to the University of Cincinnati with a singular goal: to study acting. He graduated in April 2021 with his bachelor’s degree in chemical engineering instead. Bushnell’s path between these two seemingly dissimilar fields is rooted in his mindset that following your passion will drive your determination to succeed – even if that passion comes from an unexpected source.
UC student converts waste grease to biofuel at city sewer district
September 28, 2021
Andres Mata’s research for his University of Cincinnati master’s degree in chemical engineering could result in a greener fuel source. Mata, who was awarded Graduate Student Engineer of the Month from UC’s College of Engineering and Applied Science, was the lead graduate student working on a pilot project in Professor Mingming Lu’s lab to extract lipids from grease at wastewater treatment plants for biodiesel production.
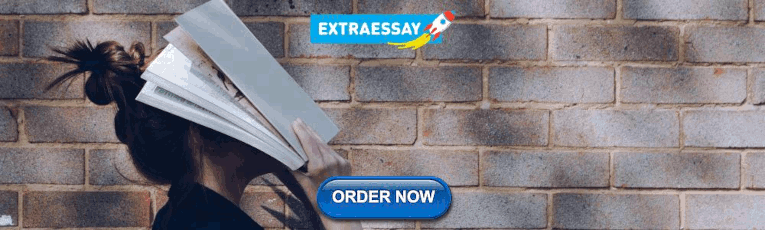
IMAGES
VIDEO
COMMENTS
I recommend every lecturer reads this article." Dean of Science Christopher Stubbs, Samuel C. Moncher Professor of Physics and of Astronomy, was an early convert. "When I first switched to teaching using active learning, some students resisted that change. This research confirms that faculty should persist and encourage active learning.
The number of students seeking help at campus counseling centers increased almost 40% between 2009 and 2015 and continued to rise until the pandemic began, according to data from Penn State University's Center for Collegiate Mental Health (CCMH), a research-practice network of more than 700 college and university counseling centers (CCMH Annual Report, 2015).
In this study, we describe the prevalence of stress, anxiety, and depression for undergraduate students in a public research university during the six weeks after the COVID-19 outbreak alongside their usage of mental health services. Using a self-administered online survey, we measured stress, anxiety, and depression levels with well ...
Introduction. Research shows that student engagement constitutes a crucial precondition for optimal and deep-level learning (Barkoukis et al. Citation 2014; Skinner Citation 2016; Skinner, Zimmer-Gembeck, and Connell Citation 1998).In addition, student engagement is associated with students' motivation to learn (Aelterman et al. Citation 2012), and their persistence to complete school ...
Nevertheless, previous meta-analyses focus mainly on all students with any kind of SEN. Instead, it can be assumed that the effects of inclusive education differ depending on the type and extent of a student's SEN (see Cooc, 2019).For example, Carlberg and Kavale (1980) showed in their older meta-analysis that both students with IQs from 50 to 75 and those with IQs from 75 to 90 in inclusive ...
Theoretical Relations Between Achievement Motivation and Academic Achievement. We take a social-cognitive approach to motivation (see also Pintrich et al., 1993; Elliot and Church, 1997; Wigfield and Cambria, 2010).This approach emphasizes the important role of students' beliefs and their interpretations of actual events, as well as the role of the achievement context for motivational ...
Previous research shows that the experience of positive and negative emotions are directly related to levels of student engagement (Reschly, Huebner, Appleton, & Antaramian, Citation 2008). In 293 students in Grades 7-10 from the United States, the frequency of positive emotions during classes was associated with higher student engagement.
Using our methodological approach of student-driven research and a mix of quantitative and qualitative analysis, we find that work has four main impacts on students' lives: it limits flexibility in students' schedules, leaving them vulnerable in emergencies; it requires students to make difficult financial calculations; it can cause ...
honors students, suggesting that, a priori, most engaged students strongly prefer in-person classes. As expected, the COVID-19 outbreak also had large negative e ects on students' current labor market participation and expectations about post-college labor outcomes. Working students su ered a 31% decrease
The report used data from about 5.5 million public school students in third through eighth grade who took the NWEA's tests during the 2020-21 school year, and compared their performance to ...
Student attrition poses a major challenge to academic institutions, funding bodies and students. With the rise of Big Data and predictive analytics, a growing body of work in higher education ...
Introduction. Student voice has become a popular research area and much of the literature reports student voice being used at the classroom level in positive and well received ways (Fielding and Bragg Citation 2003; McIntyre, Pedder, and Rudduck Citation 2005; Flutter Citation 2007; Graham et al. Citation 2018).In particular, many researchers have reported teachers being surprised and ...
Abstract. The purpose of this study is to map the literature on mental health and well-being of university students using metadata extracted from 5,561 journal articles indexed in the Web of Science database for the period 1975-2020. More specifically, this study uses bibliometric procedures to describe and visually represent the available ...
The intervention worked for people from various age groups, including college students and middle-aged adults, researchers learned after analyzing seven studies on peer-led mental health programs written or published between 1975 and 2021. Researchers found that participants also became less likely to identify with negative stereotypes ...
In February of 2020, we launched the new website Greater Good in Education, a collection of free, research-based and -informed strategies and practices for the social, emotional, and ethical development of students, for the well-being of the adults who work with them, and for cultivating positive school cultures.Little did we know how much more crucial these resources would become over the ...
Journal of Student Research (JSR) is an Academic, Multidisciplinary, and Faculty-reviewed Journal (Houston, Texas) devoted to the Rapid Dissemination of Current Research Published by High School Edition, Undergraduate and Graduate students. The journal seeks articles that are novel, integrative, and accessible to a broad audience, including an ...
To the Editor: " Pandemic Effect: Absence From Schools Is Soaring " (front page, March 30) highlights the persistent challenge of chronic absenteeism in U.S. schools. If pandemic-related ...
This article was published in Journal of College Student Retention: Research, Theory & Practice. VIEW ALL JOURNAL METRICS. Article usage * Total views and downloads: 3998 * Article usage tracking started in December 2016. Articles citing this one. Receive email alerts when this article is cited.
original definition of stress formulated by Selye (1983) was, "Stress is a non-specific response of the body". Stress is an. unavoidable phenomenon in all aspects of human life. Stress. is an ...
Over half (62%) of the articles included defined student voice. Of those that defined student voice, most noted that student voice can encompass a host of activities, ranging from student feedback to students' inclusion in school-level decision-making to student-initiated and led research into school conditions and students' experiences.
Articles that were not written in English did not discuss the impact on engagement from a student perspective, and were published beyond the previously established time and language were excluded. In addition, a selection of articles was identified and assessed by manually searching the references of articles related to the topic, of which 13 ...
Google Scholar provides a simple way to broadly search for scholarly literature. Search across a wide variety of disciplines and sources: articles, theses, books, abstracts and court opinions.
Researchers have focused on at least three levels in relation to student engagement ( Skinner & Pitzer, 2012 ). The first level represents student involvement within the school community (e.g., involvement in school activities). The second level narrows the focus to the classroom or subject domain (e.g., how students interact with math teachers ...
Only 60 percent of students who enrolled in college earned a degree or credential within eight years of graduating high school. That's one of the biggest takeaways from a new report the National Center for Education Statistics released Monday that analyzed the enrollment, completion and financial aid outcomes of students.. The researchers tracked the postsecondary educational outcomes of ...
Among US college athletes, suicide is now the second leading cause of death after accidents — and rates have doubled from 7.6% to 15.3% over the past 20 years, according to a study published ...
8 Research Articles on Women and Girls High School Intro Biology Collection Popular Lesson Ideas. Data Graphing Activity for 4th-7th Graders Articles Translated in Multiple Languages SJK Recommends: The World's Best STEM Resources. Free. Ask-A-Scientist Podcast E2: Dr. Allison Master, social psychologist ...
Students in the University of North Georgia's (UNG) Doctor of Physical Therapy (DPT) program are gaining valuable experience thanks to the research and innovation track within the DPT. After researching alongside Dr. Stanislaw Solnik, associate professor of physical therapy, Dr. Adrien Buttram, '23, presented at two conferences and published an article in the Journal of Biomechanics, a top ...
This review article analyzed 175 research studies on the challenges of international students published between 2002 and 2022 using content analysis. The results revealed that the US is the biggest producer of research articles, followed by Australia, the UK, and Canada.
At the event, students also had the opportunity to showcase their research during the student poster session. About 40 students presented and discussed their research with fellow undergraduates and graduate students within the College of Engineering, which ranged from robotic arms to gesture recognition technology.
Ever since she garnered an appreciation for chemistry in high school, fifth-year University of Cincinnati student Jaclyn Bashore has not looked back. When she graduates with a chemical engineering degree and a minor in chemistry this spring, Bashore will have had six co-op experiences — along with a new full-time job at AbbVie, a biopharmaceutical research company with whom she did two co-op ...