
Want to create or adapt books like this? Learn more about how Pressbooks supports open publishing practices.
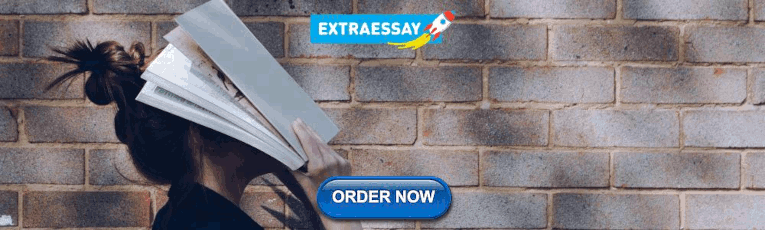
10.4 Matched or Paired Samples
DEPENDENT SAMPLES
If the choice of a sample member in one group automatically determines who’s going to be selected from the second group, then the samples are dependent. In these samples, there’s a distinct connection between members of the two samples. For example, when samples are taken from the same group at two different points in time (such as pre and post results) or under two different conditions (such as testing alone vs with a proctor), the two samples will be dependent. The group members can be different people and yet result in dependent samples if there is a natural connection between sample members from those two groups such as spouse/partner, twins, or some predefined criteria (gender, lifestyle, age, etc.) that can be used to match a member from one sample to another.
The requirements for hypothesis testing with dependent samples are:
- Samples taken are simple random samples with observations that are independent within each group. Sample sizes are often small.
- The two measurements (samples) are drawn from the same or same pair of individuals or objects (population variances should be the same)
- Either the matched pairs have differences that come from a population that is normal or the sampled number of differences is sufficiently large so that distribution of the sample mean of differences is approximately normal.
If the above conditions hold, then we can use the sampling distribution of the mean of the differences between matched pairs in the sample, [latex]\bar d[/latex], to conduct our hypothesis test. Since the differences form just one list of values, we’ll perform a t-test. The test statistic for the dependent t -test is \[t = \frac{\bar d}{s_{\bar d}}\]
where [latex]\bar d[/latex] is the mean difference and [latex]s_{\bar d}[/latex] is the standard error of the mean difference, which is
\[s_{\bar d} = {s_d\over\sqrt{n}}\]where [latex]s_d[/latex] is the standard deviation of the differences of the data values from [latex]n[/latex] matched pairs in the samples.
Symbols Used in Hypothesis Testing for Means
Here’s an overview of testing with dependent samples.
WORKED OUT EXAMPLE – Dependent Samples
A local group of economists wants to study the effects of the pandemic on the small businesses in their city. A survey was sent out to a random sample of small businesses to anonymously report their weekly earnings for January of 2020 and a year later in January 2021. Assume that the weekly earnings are normally distributed. Only 10 businesses provided enough data as shown below. Data values are in thousands.
Use [latex]\alpha = 0.01[/latex] to decide whether there is sufficient evidence to support that the pandemic reduced average weekly earnings for small businesses.
We might be tempted to compare the average earnings of all of the small businesses in January 2020 with the average of all small businesses in 2021, that is, to test if the difference of averages is [latex]\mu_1 on average these differences will amount to zero , which is to say that there’s really not a whole lot of difference between the two groups. The average of the differences from our samples is [latex]\bar d[/latex]. Let’s find that next by first finding the difference between January 2020 and January 2021 earnings for each pair:
The null hypothesis will be that on average these differences will amount to zero , which is to say that there’s really not a whole lot of difference between the two groups. The average of the differences from our samples is [latex]\bar d[/latex]. Let’s find that next by first finding the difference between January 2020 and January 2021 earnings for each pair:
For the differences we can calculate their average and standard deviation by using a calculator (One Variable Statistics calculator at SUBEDI Calcs, for example).
The average of the differences, [latex]\bar d=6.48[/latex]. This is our sample statistic.
The standard deviation of the differences, [latex]s_d = 13.977028773431545[/latex].
In order to understand how usual or unusual this sample is, we need to know the sampling distribution of [latex]\bar d[/latex]. This [latex]\bar d[/latex] will be an estimate for the actual difference between the two populations, [latex]d[/latex]. On average, if we expect the January 2021 earnings to be lower, then we’d expect the average of the differences to be positive (if we compute differences between 2020 and 2021 for the same month of January: 2020 Earnings – 2021 Earnings . So the claim we would like to test is if, on average, the difference of January 2020 and January 2021 earnings is positive . This claim in symbols is: [latex]\mu_d > 0[/latex]. The opposite of this claim is [latex]\mu_d\le 0[/latex].
Since the differences are just one data list, we will use one sample t -test with data option to perform a t -test with the differences between the samples data calculated earlier (last row in the table above). However, if we’re using one of the calculators below, we can actually skip the step to calculate the differences. We only have to enter the data from each samples into the appropriate input boxes and enter a few other details about the test so that calculator can give us the correct results.
Sample information Sample data is paired difference in the sample.
STEP 1: Write the claim (or what’s being tested) using mathematical symbols On average the differences are positive. That is, [latex]\mu_d > 0[/latex].
STEP 2: Write the opposite of the claim using mathematical symbols Opposite of the claim: [latex]\mu_d\le 0[/latex].
STEP 3: Write the null and alternative hypothesis The null hypothesis is the statement that contains the condition of equality which in this case is the opposite of the claim
STEP 4: Identify the tail-type of the test and the level of significance, [latex]\alpha[/latex]. If [latex]\alpha[/latex] is not given, assume it to be 5% or 0.05. This is a right tailed test. Why? Since the level of significance is given, use that value of [latex]\alpha=0.01[/latex] (or 1% level of significance)
STEP 5: Check if CLT conditions are valid. The number of paired sample is small, lower than 30, so we need the paired differences in the population to be normally distributed to meet CLT conditions. We’ll perform a t-test for dependent means since the population standard deviation of the paired differences in the population is not known. This is the stage where we assume the equality in the null hypothesis is true, that is, assume [latex]\mu_d=0[/latex]. We’re assuming that there’s no change between the weekly earnings for small businesses in January of 2020 and January of 2021. We know that the sampling distribution of the mean of the paired difference, [latex]\bar d[/latex], will be approximately normally distributed with mean of [latex]\mu_{\bar d} = 0[/latex] and a standard deviation of [latex]\sigma_{\bar d} = \dfrac{\sigma_d}{\sqrt n}[/latex]. Since the population standard deviation of [latex]\sigma_d[/latex] is unknown, the standard deviation of the sampling distribution of [latex]\bar d[/latex] can be approximated as [latex]\sigma_{\bar d} \approx s_{\bar d}=\dfrac{s_d}{\sqrt n}[/latex] [1] . This sampling distribution can be approximated by a t -distribution with [latex]n-1[/latex] degrees of freedom, where n is the number of matched pairs.
Results from STEP 6 can be obtained using a calculator. See below.
STEP 6: Plot t -score and find the p -value. Degrees of freedom for t -test: [latex]df = n - 1 = 10 - 1 = 9[/latex].
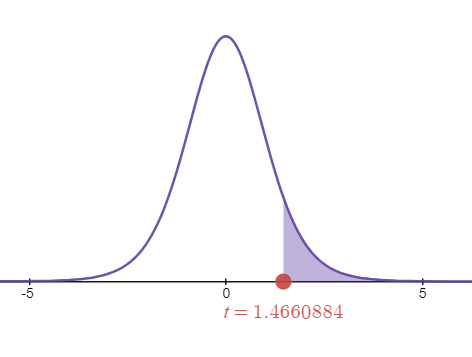
Use one or more of the calculators below to calculate p -value.
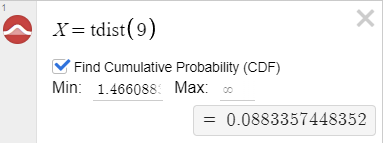
Go to Inference for the Mean @ rsubedi.com
Number of Samples
Independent or dependent samples?
Distribution Type for Test
Alternative Hypothesis
Confidence Interval? ← Optional
Enter Sample Data Enter your data for the two samples in the spreadsheet columns shown for data entry. You do not need to enter the difference data as the calculator will automatically calculate that to arrive at the test results. You can copy your data from the original source (comma or separated list, spreadsheet data, etc.) and paste that into the table on the calculator.
CALCULATE Results show in a panel to the right. Test statistic t , p -value, and df are displayed. The spreadsheet displays an additional column for the pair-wise difference of the sample data
Go to: Two Dependent Samples With Data from the list of online calculators
Enter the following values and press Calculate .
CALCULATE Results displayed are:
STEP 7: Make the decision by comparing p -value with α p-value is 0 . 0 8 8 3 3 5 7 4 4 8 3 5 8 which is more than [latex]\alpha[/latex] of 1%. Since p -value is more than [latex]\alpha[/latex], we fail to reject the null hypothesis ⇒ There’s not enough evidence to support Ha.
STEP 8: Interpret your decision in the context of original claim There is insufficient evidence at a significance level of 1% to support that the pandemic reduced average weekly earnings for small businesses.
- FSCJ Guides ↵
Statistics Study Guide Copyright © by Ram Subedi is licensed under a Creative Commons Attribution-ShareAlike 4.0 International License , except where otherwise noted.
Share This Book
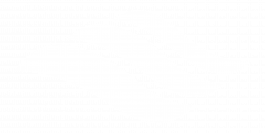
Want to create or adapt books like this? Learn more about how Pressbooks supports open publishing practices.
29 8.1 Inference for Two Dependent Samples (Matched Pairs)
[latexpage]
Learning Objectives
By the end of this chapter, the student should be able to:
- Classify hypothesis tests by type
- Conduct and interpret hypothesis tests for two population means, population standard deviations known
- Conduct and interpret hypothesis tests for two population means, population standard deviations unknown
- Conduct and interpret hypothesis tests for matched or paired samples
- Conduct and interpret hypothesis tests for two population proportions
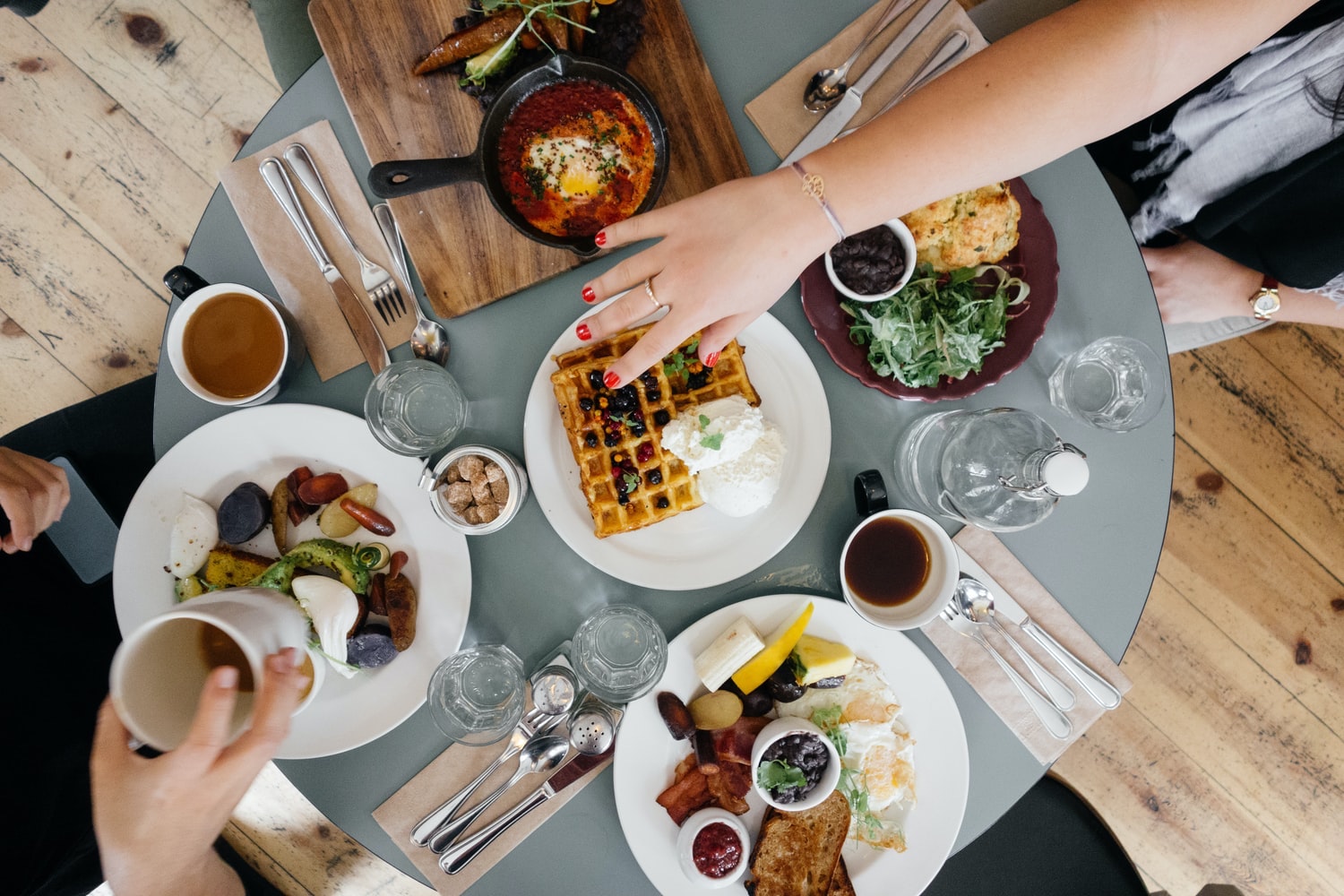
Studies often compare two groups. For example, maybe researchers are interested in the effect aspirin has in preventing heart attacks. One group is given aspirin and the other a placebo , and the heart attack rate is studied over several years. Other studies may compare various diet and exercise programs. Politicians compare the proportion of individuals from different income brackets who might vote for them. Students are interested in whether SAT or GRE preparatory courses really help raise their scores.
You have learned to conduct inference on single means and single proportions . We know that the first step is deciding what type of data we are working with. For quantitative data we are focused on means, while for categorical we are focused on proportions. In this chapter we will compare two means or two proportions to each other. The general procedure is still the same, just expanded. With two sample analysis it is good to know what the formulas look like and where they come from, however you will probably lean heavily on technology in preforming the calculations.
To compare two means we are obviously working with two groups, but first we need to think about the relationship between them. The groups are classified either as independent or dependent. I ndependent samples consist of two samples that have no relationship, that is, sample values selected from one population are not related in any way to sample values selected from the other population. Dependent samples consist of two groups that have some sort of identifiable relationship.
Two Dependent Samples (Matched Pairs)
Two samples that are dependent typically come from a matched pairs experimental design. The parameter tested using matched pairs is the population mean difference . When using inference techniques for matched or paired samples, the following characteristics should be present:
- Simple random sampling is used.
- Sample sizes are often small.
- Two measurements (samples) are drawn from the same pair of (or two extremely similar) individuals or objects.
- Differences are calculated from the matched or paired samples.
- The differences form the sample that is used for analysis.
To perform statistical inference techniques we first need to know about the sampling distribution of our parameter of interest. Remember although we start with two samples, the differences are the data we are interested in and our parameter of interest is μ d , the mean difference. Our point estimate is [latex]\overline{x}_d[/latex]. In a perfect world we could assume that both samples come from a normal distribution, therefore the difference in those normal distributions are also normal. However in order to use Z, we must know the population standard deviation which is near impossible for a difference distribution. Also it is very hard to find large numbers of matched pairs so the sampling distribution we typically use for [latex]\overline{x}_d[/latex] is a t distribution with n – 1 degrees of freedom, where n is the number of differences.
Confidence intervals may be calculated on their own for two samples but often, especially in the case of matched pairs, we first want to formally check to see if a difference exists with a hypothesis test. If we do find a statistically significant difference then we may estimate it with a CI after the fact.
Hypothesis Tests for the Mean difference
In a hypothesis test for matched or paired samples, subjects are matched in pairs and differences are calculated, and the population mean difference, μ d , is our parameter of interest. Although it is possible to test for a certain magnitude of effect, we are most often just looking for a general effect. Our hypothesis would then look like:
H o : μ d =0
H a : μ d (<, >, ≠) 0
The steps are the same as we are familiar with, but it is tested using a Student’s-t test for a single population mean with n – 1 degrees of freedom, with the test statistic:
\(t=\(\frac{{\overline{x}}_{d}-{\mu }_{d}}{\left(\frac{{s}_{d}}{\sqrt{n}}\right)}\)
A study was conducted to investigate the effectiveness of hypnotism in reducing pain. Results for randomly selected subjects are shown in the figure below. A lower score indicates less pain. The “before” value is matched to an “after” value and the differences are calculated. The differences have a normal distribution. Are the sensory measurements, on average, lower after hypnotism? Test at a 5% significance level.
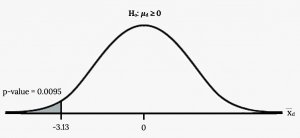
A study was conducted to investigate how effective a new diet was in lowering cholesterol. Results for the randomly selected subjects are shown in the table. The differences have a normal distribution. Are the subjects’ cholesterol levels lower on average after the diet? Test at the 5% level.
Confidence Intervals for the Mean difference
The general format of a confidence interval is: \((PE-MoE, PE+MoE)\)
The population parameter of interest is μ d , the mean difference. Our point estimate is [latex]\overline{x}_d[/latex]
If we are using the t distribution, the error bound for the population mean difference is:
- \(MoE=\left({t}_{\frac{\alpha }{2}}\right)\left(\frac{s_d}{\sqrt{n}}\right)\),
- \({t}_{\frac{\sigma }{2}}\) is the t critical value with area to the right equal to [latex]\frac{\alpha }{2}[/latex],
- use df = n – 1 degrees of freedom, where n is the number of pairs
- s d = standard deviation of the differences.
A college football coach was interested in whether the college’s strength development class increased his players’ maximum lift (in pounds) on the bench press exercise. He asked four of his players to participate in a study. The amount of weight they could each lift was recorded before they took the strength development class. After completing the class, the amount of weight they could each lift was again measured. The data are as follows:
The coach wants to know if the strength development class makes his players stronger, on average.
Using the differences data, calculate the sample mean and the sample standard deviation.
Using the difference data, this becomes a test of a single __________ (fill in the blank).
Define the random variable: \({\overline{X}}_{d}\) mean difference in the maximum lift per player.
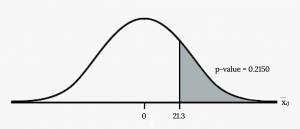
Calculate the p -value:
What is the conclusion?
A new prep class was designed to improve SAT test scores. Five students were selected at random. Their scores on two practice exams were recorded, one before the class and one after. The data recorded in the figure below. Are the scores, on average, higher after the class? Test at a 5% level.
Image Credits
Figure 8.1: Ali Inay (2015). “Brunching with Friends.” Public domain. Retrieved from https://unsplash.com/photos/y3aP9oo9Pjc
Figure 8.3: Kindred Grey via Virginia Tech (2020). “Figure 8.3” CC BY-SA 4.0. Retrieved from https://commons.wikimedia.org/wiki/File:Figure_8.3.png . Adaptation of Figure 5.39 from OpenStax Introductory Statistics (2013) (CC BY 4.0). Retrieved from https://openstax.org/books/statistics/pages/5-practice
Figure 8.6: Kindred Grey via Virginia Tech (2020). “Figure 8.6” CC BY-SA 4.0. Retrieved from https://commons.wikimedia.org/wiki/File:Figure_8.6.png . Adaptation of Figure 5.39 from OpenStax Introductory Statistics (2013) (CC BY 4.0). Retrieved from https://openstax.org/books/statistics/pages/5-practice
An inactive treatment that has no real effect on the explanatory variable
The facet of statistics dealing with using a sample to generalize (or infer) about the population
The arithmetic mean, or average of a population
The number of individuals that have a characteristic we are interested in divided by the total number in the population
Numerical data with a mathematical context
Data that describes qualities, or puts individuals into categories
The occurrence of one event has no effect on the probability of the occurrence of another event
Very similar individuals (or even the same individual) receive two different two treatments (or treatment vs. control) then the difference in results are compared
The mean of the differences in a matched pairs design
The probability distribution of a statistic at a given sample size
The value that is calculated from a sample used to estimate an unknown population parameter
Significant Statistics Copyright © 2020 by John Morgan Russell, OpenStaxCollege, OpenIntro is licensed under a Creative Commons Attribution-ShareAlike 4.0 International License , except where otherwise noted.
Share This Book
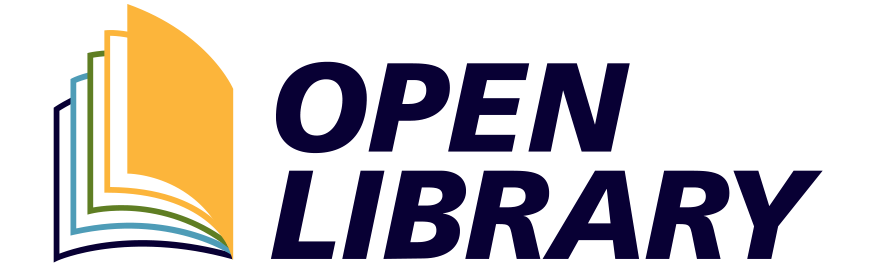
Want to create or adapt books like this? Learn more about how Pressbooks supports open publishing practices.
Chapter 10.5: Matched or Paired Samples
When using a hypothesis test for matched or paired samples, the following characteristics should be present:
- Simple random sampling is used.
- Sample sizes are often small.
- Two measurements (samples) are drawn from the same pair of individuals or objects.
- Differences are calculated from the matched or paired samples.
- The differences form the sample that is used for the hypothesis test.
- Either the matched pairs have differences that come from a population that is normal or the number of differences is sufficiently large so that distribution of the sample mean of differences is approximately normal.
In a hypothesis test for matched or paired samples, subjects are matched in pairs and differences are calculated. The differences are the data. The population mean for the differences, μ d , is then tested using a Student’s-t test for a single population mean with n – 1 degrees of freedom, where n is the number of differences.

A study was conducted to investigate the effectiveness of hypnotism in reducing pain. Results for randomly selected subjects are shown in (Figure) . A lower score indicates less pain. The “before” value is matched to an “after” value and the differences are calculated. The differences have a normal distribution. Are the sensory measurements, on average, lower after hypnotism? Test at a 5% significance level.
Corresponding “before” and “after” values form matched pairs. (Calculate “after” – “before.”)
The data for the test are the differences: {0.2, –4.1, –1.6, –1.8, –3.2, –2, –2.9, –9.6}

H 0 : μ d ≥ 0
The null hypothesis is zero or positive, meaning that there is the same or more pain felt after hypnotism. That means the subject shows no improvement. μ d is the population mean of the differences.)
H a : μ d < 0
The alternative hypothesis is negative, meaning there is less pain felt after hypnotism. That means the subject shows improvement. The score should be lower after hypnotism, so the difference ought to be negative to indicate improvement.
Distribution for the test: The distribution is a Student’s t with df = n – 1 = 8 – 1 = 7. Use t 7 . (Notice that the test is for a single population mean.)
Calculate the p -value using the Student’s-t distribution: p -value = 0.0095
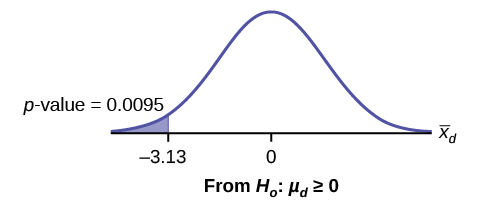
The sample mean and sample standard deviation of the differences are:

Compare α and the p -value: α = 0.05 and p -value = 0.0095. α > p -value.
Make a decision: Since α > p -value, reject H 0 . This means that μ d < 0 and there is improvement.
Conclusion: At a 5% level of significance, from the sample data, there is sufficient evidence to conclude that the sensory measurements, on average, are lower after hypnotism. Hypnotism appears to be effective in reducing pain.
For the TI-83+ and TI-84 calculators, you can either calculate the differences ahead of time ( after – before ) and put the differences into a list or you can put the after data into a first list and the before data into a second list. Then go to a third list and arrow up to the name. Enter 1 st list name – 2 nd list name. The calculator will do the subtraction, and you will have the differences in the third list.

A study was conducted to investigate how effective a new diet was in lowering cholesterol. Results for the randomly selected subjects are shown in the table. The differences have a normal distribution. Are the subjects’ cholesterol levels lower on average after the diet? Test at the 5% level.
A college football coach was interested in whether the college’s strength development class increased his players’ maximum lift (in pounds) on the bench press exercise. He asked four of his players to participate in a study. The amount of weight they could each lift was recorded before they took the strength development class. After completing the class, the amount of weight they could each lift was again measured. The data are as follows:
The coach wants to know if the strength development class makes his players stronger, on average. Record the differences data. Calculate the differences by subtracting the amount of weight lifted prior to the class from the weight lifted after completing the class. The data for the differences are: {90, 11, -8, -8}. Assume the differences have a normal distribution.
Using the differences data, calculate the sample mean and the sample standard deviation.
The data given here would indicate that the distribution is actually right-skewed. The difference 90 may be an extreme outlier? It is pulling the sample mean to be 21.3 (positive). The means of the other three data values are actually negative.
Using the difference data, this becomes a test of a single __________ (fill in the blank).
The distribution for the hypothesis test is t 3 .
H 0 : μ d ≤ 0, H a : μ d > 0
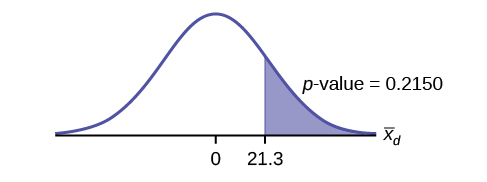
Calculate the p -value: The p -value is 0.2150
Decision: If the level of significance is 5%, the decision is not to reject the null hypothesis, because α < p -value.
What is the conclusion?
At a 5% level of significance, from the sample data, there is not sufficient evidence to conclude that the strength development class helped to make the players stronger, on average.
A new prep class was designed to improve SAT test scores. Five students were selected at random. Their scores on two practice exams were recorded, one before the class and one after. The data recorded in (Figure) . Are the scores, on average, higher after the class? Test at a 5% level.
Seven eighth graders at Kennedy Middle School measured how far they could push the shot-put with their dominant (writing) hand and their weaker (non-writing) hand. They thought that they could push equal distances with either hand. The data were collected and recorded in (Figure) .
<!–MAGTULOY DITO…–>
Conduct a hypothesis test to determine whether the mean difference in distances between the children’s dominant versus weaker hands is significant.
Record the differences data. Calculate the differences by subtracting the distances with the weaker hand from the distances with the dominant hand. The data for the differences are: {2, 12, 7, –1, 2, 0, 4}. The differences have a normal distribution.

Distribution for the hypothesis test: t 6
H 0 : μ d = 0 H a : μ d ≠ 0
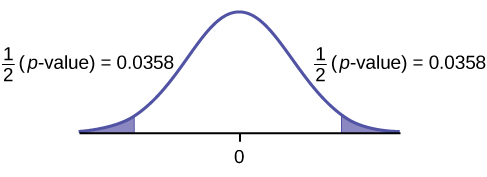
Calculate the p -value: The p -value is 0.0716 (using the data directly).

Decision: Assume α = 0.05. Since α < p -value, Do not reject H 0 .
Conclusion: At the 5% level of significance, from the sample data, there is not sufficient evidence to conclude that there is a difference in the children’s weaker and dominant hands to push the shot-put.
Five ball players think they can throw the same distance with their dominant hand (throwing) and off-hand (catching hand). The data were collected and recorded in (Figure) . Conduct a hypothesis test to determine whether the mean difference in distances between the dominant and off-hand is significant. Test at the 5% level.
Chapter Review
A hypothesis test for matched or paired samples (t-test) has these characteristics:
- Test the differences by subtracting one measurement from the other measurement
- Distribution: Student’s-t distribution with n – 1 degrees of freedom
- If the number of differences is small (less than 30), the differences must follow a normal distribution.
- Two samples are drawn from the same set of objects.
- Samples are dependent.
Formula Review

Use the following information to answer the next five exercises. A study was conducted to test the effectiveness of a software patch in reducing system failures over a six-month period. Results for randomly selected installations are shown in (Figure) . The “before” value is matched to an “after” value, and the differences are calculated. The differences have a normal distribution. Test at the 1% significance level.
What is the random variable?
the mean difference of the system failures
State the null and alternative hypotheses.
What is the p -value?
Draw the graph of the p -value.
What conclusion can you draw about the software patch?
With a p -value 0.0067, we can reject the null hypothesis. There is enough evidence to support that the software patch is effective in reducing the number of system failures.
Use the following information to answer next five exercises. A study was conducted to test the effectiveness of a juggling class. Before the class started, six subjects juggled as many balls as they could at once. After the class, the same six subjects juggled as many balls as they could. The differences in the number of balls are calculated. The differences have a normal distribution. Test at the 1% significance level.
What is the sample mean difference?
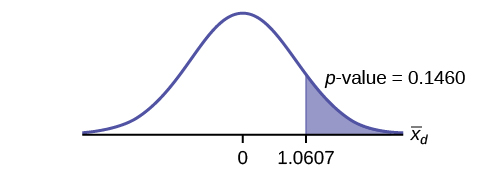
What conclusion can you draw about the juggling class?
Use the following information to answer the next five exercises. A doctor wants to know if a blood pressure medication is effective. Six subjects have their blood pressures recorded. After twelve weeks on the medication, the same six subjects have their blood pressure recorded again. For this test, only systolic pressure is of concern. Test at the 1% significance level.
What is the test statistic?
We decline to reject the null hypothesis. There is not sufficient evidence to support that the medication is effective.
DIRECTIONS: For each of the word problems, use a solution sheet to do the hypothesis test. The solution sheet is found in Appendix E . Please feel free to make copies of the solution sheets. For the online version of the book, it is suggested that you copy the .doc or the .pdf files.
If you are using a Student’s t -distribution for the homework problems, including for paired data, you may assume that the underlying population is normally distributed. (When using these tests in a real situation, you must first prove that assumption, however.)
1) Ten individuals went on a low–fat diet for 12 weeks to lower their cholesterol. The data are recorded in (Figure) . Do you think that their cholesterol levels were significantly lowered?
p -value = 0.1494
Use the following information to answer the next two exercises.
A new AIDS prevention drug was tried on a group of 224 HIV positive patients. Forty-five patients developed AIDS after four years. In a control group of 224 HIV positive patients, 68 developed AIDS after four years. We want to test whether the method of treatment reduces the proportion of patients that develop AIDS after four years or if the proportions of the treated group and the untreated group stay the same.
Let the subscript t = treated patient and ut = untreated patient.
2) The appropriate hypotheses are:
- H 0 : p t < p ut and H a : p t ≥ p ut
- H 0 : p t ≤ p ut and H a : p t > p ut
- H 0 : p t = p ut and H a : p t ≠ p ut
- H 0 : p t = p ut and H a : p t < p ut
3) If the p -value is 0.0062 what is the conclusion (use α = 0.05)?
- The method has no effect.
- There is sufficient evidence to conclude that the method reduces the proportion of HIV positive patients who develop AIDS after four years.
- There is sufficient evidence to conclude that the method increases the proportion of HIV positive patients who develop AIDS after four years.
- There is insufficient evidence to conclude that the method reduces the proportion of HIV positive patients who develop AIDS after four years.
4) The distribution for the test is:
- N (−10.2, 8.4)

5) If α = 0.05, the p -value and the conclusion are
- 0.0014; There is sufficient evidence to conclude that the blood pressure decreased after the training.
- 0.0014; There is sufficient evidence to conclude that the blood pressure increased after the training.
- 0.0155; There is sufficient evidence to conclude that the blood pressure decreased after the training.
- 0.0155; There is sufficient evidence to conclude that the blood pressure increased after the training.
6) A golf instructor is interested in determining if her new technique for improving players’ golf scores is effective. She takes four new students. She records their 18-hole scores before learning the technique and then after having taken her class. She conducts a hypothesis test. The data are as follows.
The correct decision is:
- Reject H 0 .
- Do not reject the H 0 .
7) A local cancer support group believes that the estimate for new female breast cancer cases in the south is higher in 2013 than in 2012. The group compared the estimates of new female breast cancer cases by southern state in 2012 and in 2013. The results are in (Figure) .
8) A traveler wanted to know if the prices of hotels are different in the ten cities that he visits the most often. The list of the cities with the corresponding hotel prices for his two favorite hotel chains is in (Figure) . Test at the 1% level of significance.
9) A politician asked his staff to determine whether the underemployment rate in the northeast decreased from 2011 to 2012. The results are in (Figure) .
Bringing It Together
Use the following information to answer the next ten exercises. indicate which of the following choices best identifies the hypothesis test.
- independent group means, population standard deviations and/or variances known
- independent group means, population standard deviations and/or variances unknown
- matched or paired samples
- single mean
- two proportions
- single proportion
10) A powder diet is tested on 49 people, and a liquid diet is tested on 36 different people. The population standard deviations are two pounds and three pounds, respectively. Of interest is whether the liquid diet yields a higher mean weight loss than the powder diet.
11) A new chocolate bar is taste-tested on consumers. Of interest is whether the proportion of children who like the new chocolate bar is greater than the proportion of adults who like it.
12) The mean number of English courses taken in a two–year time period by male and female college students is believed to be about the same. An experiment is conducted and data are collected from nine males and 16 females.
13) A football league reported that the mean number of touchdowns per game was five. A study is done to determine if the mean number of touchdowns has decreased.
14) A study is done to determine if students in the California state university system take longer to graduate than students enrolled in private universities. One hundred students from both the California state university system and private universities are surveyed. From years of research, it is known that the population standard deviations are 1.5811 years and one year, respectively.
15) According to a YWCA Rape Crisis Center newsletter, 75% of rape victims know their attackers. A study is done to verify this.
16) According to a recent study, U.S. companies have a mean maternity-leave of six weeks.
17) A recent drug survey showed an increase in use of drugs and alcohol among local high school students as compared to the national percent. Suppose that a survey of 100 local youths and 100 national youths is conducted to see if the proportion of drug and alcohol use is higher locally than nationally.
18) A new SAT study course is tested on 12 individuals. Pre-course and post-course scores are recorded. Of interest is the mean increase in SAT scores. The following data are collected:
19) University of Michigan researchers reported in the Journal of the National Cancer Institute that quitting smoking is especially beneficial for those under age 49. In this American Cancer Society study, the risk (probability) of dying of lung cancer was about the same as for those who had never smoked.
20) Lesley E. Tan investigated the relationship between left-handedness vs. right-handedness and motor competence in preschool children. Random samples of 41 left-handed preschool children and 41 right-handed preschool children were given several tests of motor skills to determine if there is evidence of a difference between the children based on this experiment. The experiment produced the means and standard deviations shown (Figure) . Determine the appropriate test and best distribution to use for that test.
- Two independent means, normal distribution
- Two independent means, Student’s-t distribution
- Matched or paired samples, Student’s-t distribution
- Two population proportions, normal distribution
21) A golf instructor is interested in determining if her new technique for improving players’ golf scores is effective. She takes four (4) new students. She records their 18-hole scores before learning the technique and then after having taken her class. She conducts a hypothesis test. The data are as (Figure) .
- a test of two independent means.
- a test of two proportions.
- a test of a single mean.
- a test of a single proportion.
Answers to odd questions
1) At the 5% significance level, there is insufficient evidence to conclude that the medication lowered cholesterol levels after 12 weeks.
Test: two matched pairs or paired samples ( t -test)
Distribution: t 12
H 0 : μ d = 0 H a : μ d > 0
The mean of the differences of new female breast cancer cases in the south between 2013 and 2012 is greater than zero. The estimate for new female breast cancer cases in the south is higher in 2013 than in 2012.
Graph: right-tailed
p -value: 0.0004
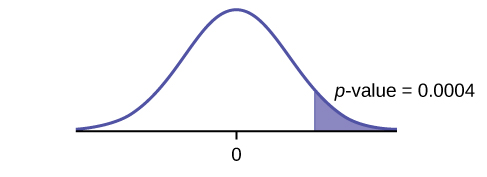
Decision: Reject H 0
Conclusion: At the 5% level of significance, from the sample data, there is sufficient evidence to conclude that there was a higher estimate of new female breast cancer cases in 2013 than in 2012.
Test: matched or paired samples ( t -test)
Difference data: {–0.9, –3.7, –3.2, –0.5, 0.6, –1.9, –0.5, 0.2, 0.6, 0.4, 1.7, –2.4, 1.8}
Distribution: H 0 : μ d = 0 H a : μ d < 0
The mean of the differences of the rate of underemployment in the northeastern states between 2012 and 2011 is less than zero. The underemployment rate went down from 2011 to 2012.
Graph: left-tailed.
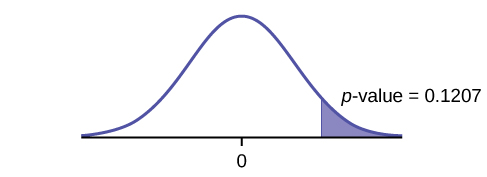
p -value: 0.1207
Decision: Do not reject H 0 .
Conclusion: At the 5% level of significance, from the sample data, there is not sufficient evidence to conclude that there was a decrease in the underemployment rates of the northeastern states from 2011 to 2012.
College Statistics Copyright © 2022 by St. Clair College is licensed under a Creative Commons Attribution-NonCommercial-ShareAlike 4.0 International License , except where otherwise noted.
Share This Book
BUS204: Business Statistics
Hypothesis Testing with Two Samples
Read this chapter, which discusses how to compare data from two similar groups. This is useful when, for example, you want to analyze things like how someone's income relates to another sample that you are interested in. Make sure you read the introduction as well as sections 10.1 through 10.6. Attempt the practice problems and homework at the end of the chapter.
Matched or Paired Samples
- Simple random sampling is used.
- Sample sizes are often small.
- Two measurements (samples) are drawn from the same pair of individuals or objects.
- Differences are calculated from the matched or paired samples.
- The differences form the sample that is used for the hypothesis test.
- Either the matched pairs have differences that come from a population that is normal or the number of differences is sufficiently large so that distribution of the sample mean of differences is approximately normal.
In a hypothesis test for matched or paired samples, subjects are matched in pairs and differences are calculated. The differences are the data. The population mean for the differences, μd, is then tested using a Student's-t test for a single population mean with n – 1 degrees of freedom, where n is the number of differences, that is, the number of pairs not the number of observations.
The null and alternative hypotheses for this test are:
The test statistic is:
Example 10.9
Problem A company has developed a training program for its entering employees because they have become concerned with the results of the six-month employee review. They hope that the training program can result in better six-month reviews. Each trainee constitutes a "pair", the entering score the employee received when first entering the firm and the score given at the six-month review. The difference in the two scores were calculated for each employee and the means for before and after the training program was calculated. The sample mean before the training program was 20.4 and the sample mean after the training program was 23.9. The standard deviation of the differences in the two scores across the 20 employees was 3.8 points. Test at the 10% significance level the null hypothesis that the two population means are equal against the alternative that the training program helps improve the employees' scores.
Solution 1 The first step is to identify this as a two sample case: before the training and after the training. This differentiates this problem from simple one sample issues. Second, we determine that the two samples are "paired". Each observation in the first sample has a paired observation in the second sample. This information tells us that the null and alternative hypotheses should be:
This form reflects the implied claim that the training course improves scores; the test is one-tailed and the claim is in the alternative hypothesis. Because the experiment was conducted as a matched paired sample rather than simply taking scores from people who took the training course those who didn't, we use the matched pair test statistic:
In order to solve this equation, the individual scores, pre-training course and post-training course need to be used to calculate the individual differences. These scores are then averaged and the average difference is calculated:
From these differences we can calculate the standard deviation across the individual differences:
We can now compare the calculated value of the test statistic, 4.12, with the critical value. The critical value is a Student's t with degrees of freedom equal to the number of pairs, not observations, minus 1. In this case 20 pairs and at 90% confidence level t a/2 = ±1.729 at df = 20 - 1 = 19. The calculated test statistic is most certainly in the tail of the distribution and thus we cannot accept the null hypothesis that there is no difference from the training program. Evidence seems indicate that the training aids employees in gaining higher scores.
Example 10.10
A study was conducted to investigate the effectiveness of hypnotism in reducing pain. Results for randomly selected subjects are shown in Table 10.5. A lower score indicates less pain. The "before" value is matched to an "after" value and the differences are calculated. Are the sensory measurements, on average, lower after hypnotism? Test at a 5% significance level.

Example 10.11


Want to create or adapt books like this? Learn more about how Pressbooks supports open publishing practices.
8.1 Inference for Two Dependent Samples (Matched Pairs)
Learning Objectives
By the end of this chapter, the student should be able to:
- Classify hypothesis tests by type
- Conduct and interpret hypothesis tests for two population means, population standard deviations known
- Conduct and interpret hypothesis tests for two population means, population standard deviations unknown
- Conduct and interpret hypothesis tests for matched or paired samples
- Conduct and interpret hypothesis tests for two population proportions
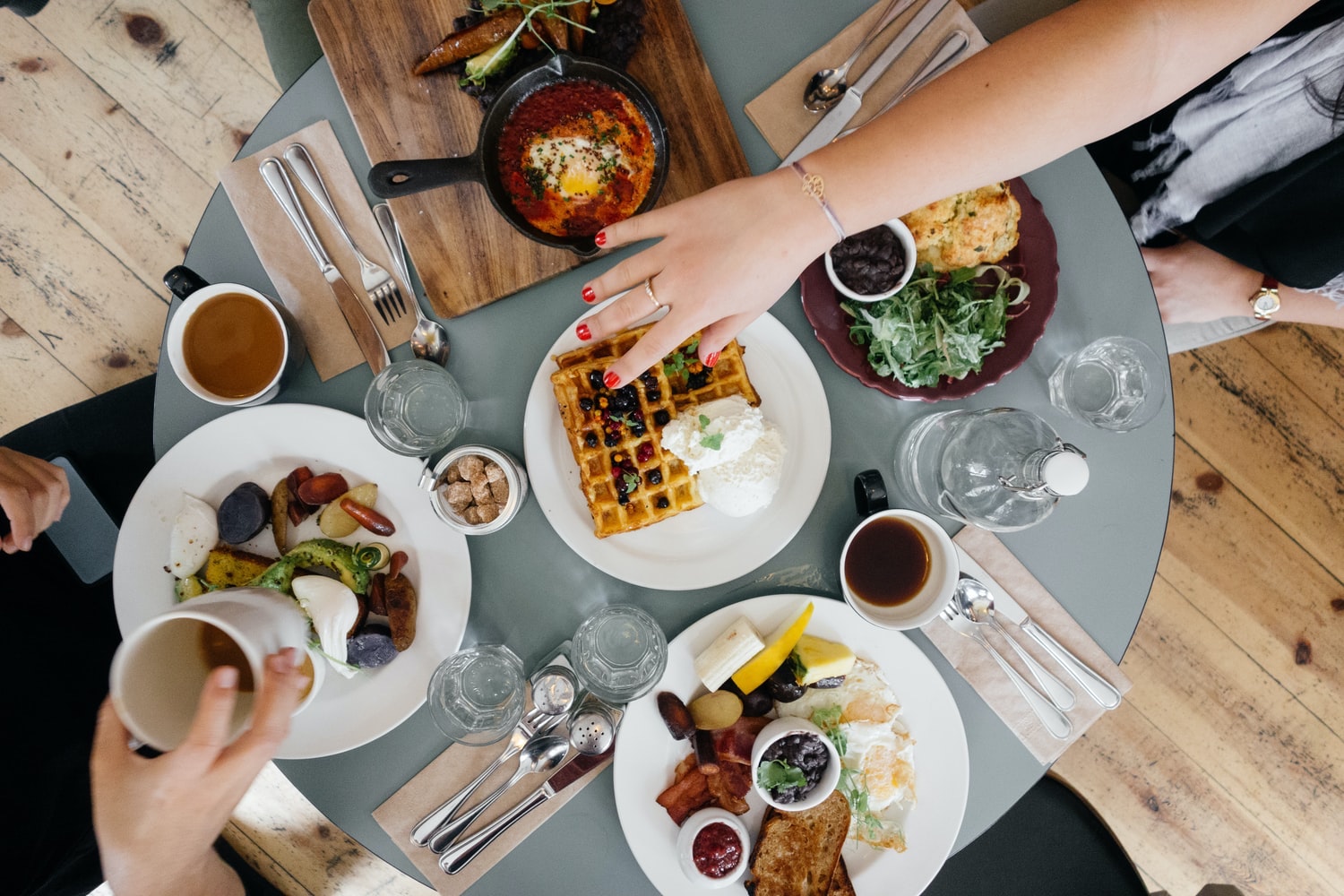
Studies often compare two groups. For example, maybe researchers are interested in the effect aspirin has in preventing heart attacks. One group is given aspirin and the other a placebo , and the heart attack rate is studied over several years. Other studies may compare various diet and exercise programs. Politicians compare the proportion of individuals from different income brackets who might vote for them. Students are interested in whether SAT or GRE preparatory courses really help raise their scores.
You have learned to conduct inference on single means and single proportions . We know that the first step is deciding what type of data we are working with. For quantitative data we are focused on means, while for categorical we are focused on proportions. In this chapter we will compare two means or two proportions to each other. The general procedure is still the same, just expanded. With two sample analysis it is good to know what the formulas look like and where they come from, however you will probably lean heavily on technology in preforming the calculations.
To compare two means we are obviously working with two groups, but first we need to think about the relationship between them. The groups are classified either as independent or dependent. I ndependent samples consist of two samples that have no relationship, that is, sample values selected from one population are not related in any way to sample values selected from the other population. Dependent samples consist of two groups that have some sort of identifiable relationship.
Two Dependent Samples (Matched Pairs)
Two samples that are dependent typically come from a matched pairs experimental design. The parameter tested using matched pairs is the population mean difference . When using inference techniques for matched or paired samples, the following characteristics should be present:
- Simple random sampling is used.
- Sample sizes are often small.
- Two measurements (samples) are drawn from the same pair of (or two extremely similar) individuals or objects.
- Differences are calculated from the matched or paired samples.
- The differences form the sample that is used for analysis.

Confidence intervals may be calculated on their own for two samples but often, especially in the case of matched pairs, we first want to formally check to see if a difference exists with a hypothesis test. If we do find a statistically significant difference then we may estimate it with a CI after the fact.
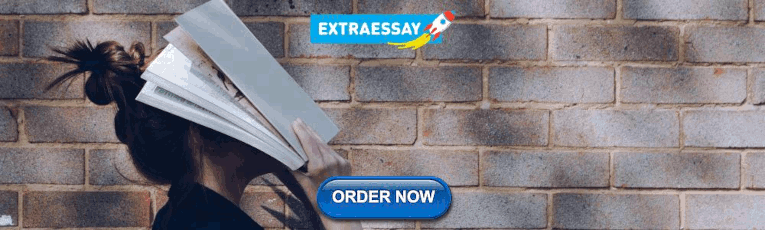
Hypothesis Tests for the Mean difference
In a hypothesis test for matched or paired samples, subjects are matched in pairs and differences are calculated, and the population mean difference, μ d , is our parameter of interest. Although it is possible to test for a certain magnitude of effect, we are most often just looking for a general effect. Our hypothesis would then look like:
H o : μ d =0
H a : μ d (<, >, ≠) 0
The steps are the same as we are familiar with, but it is tested using a Student’s-t test for a single population mean with n – 1 degrees of freedom, with the test statistic:

A study was conducted to investigate the effectiveness of hypnotism in reducing pain. Results for randomly selected subjects are shown in the figure below. A lower score indicates less pain. The “before” value is matched to an “after” value and the differences are calculated. The differences have a normal distribution. Are the sensory measurements, on average, lower after hypnotism? Test at a 5% significance level.
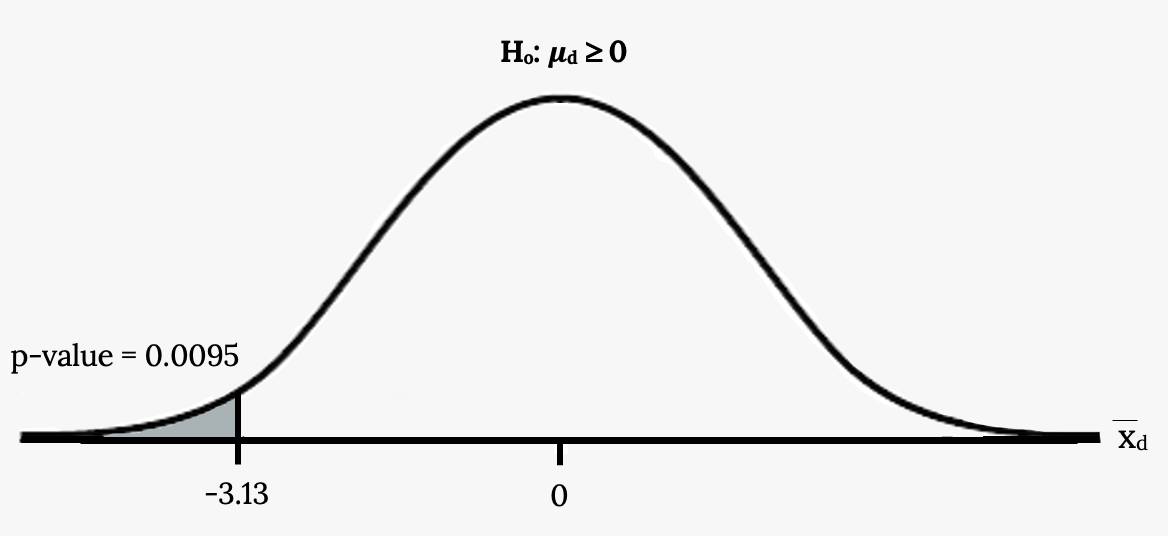
A study was conducted to investigate how effective a new diet was in lowering cholesterol. Results for the randomly selected subjects are shown in the table. The differences have a normal distribution. Are the subjects’ cholesterol levels lower on average after the diet? Test at the 5% level.
Confidence Intervals for the Mean difference

If we are using the t distribution, the error bound for the population mean difference is:
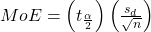
- use df = n – 1 degrees of freedom, where n is the number of pairs
- s d = standard deviation of the differences.
A college football coach was interested in whether the college’s strength development class increased his players’ maximum lift (in pounds) on the bench press exercise. He asked four of his players to participate in a study. The amount of weight they could each lift was recorded before they took the strength development class. After completing the class, the amount of weight they could each lift was again measured. The data are as follows:
The coach wants to know if the strength development class makes his players stronger, on average.
Using the differences data, calculate the sample mean and the sample standard deviation.
Using the difference data, this becomes a test of a single __________ (fill in the blank).

Calculate the p -value:
What is the conclusion?
A new prep class was designed to improve SAT test scores. Five students were selected at random. Their scores on two practice exams were recorded, one before the class and one after. The data recorded in the figure below. Are the scores, on average, higher after the class? Test at a 5% level.
Image Credits
Figure 8.1: Ali Inay (2015). “Brunching with Friends.” Public domain. Retrieved from https://unsplash.com/photos/y3aP9oo9Pjc
Figure 8.3: Kindred Grey via Virginia Tech (2020). “Figure 8.3” CC BY-SA 4.0. Retrieved from https://commons.wikimedia.org/wiki/File:Figure_8.3.png . Adaptation of Figure 5.39 from OpenStax Introductory Statistics (2013) (CC BY 4.0). Retrieved from https://openstax.org/books/statistics/pages/5-practice
Figure 8.6: Kindred Grey via Virginia Tech (2020). “Figure 8.6” CC BY-SA 4.0. Retrieved from https://commons.wikimedia.org/wiki/File:Figure_8.6.png . Adaptation of Figure 5.39 from OpenStax Introductory Statistics (2013) (CC BY 4.0). Retrieved from https://openstax.org/books/statistics/pages/5-practice
An inactive treatment that has no real effect on the explanatory variable
The facet of statistics dealing with using a sample to generalize (or infer) about the population
The arithmetic mean, or average of a population
The number of individuals that have a characteristic we are interested in divided by the total number in the population
Numerical data with a mathematical context
Data that describes qualities, or puts individuals into categories
The occurrence of one event has no effect on the probability of the occurrence of another event
Very similar individuals (or even the same individual) receive two different two treatments (or treatment vs. control) then the difference in results are compared
The mean of the differences in a matched pairs design
The probability distribution of a statistic at a given sample size
The value that is calculated from a sample used to estimate an unknown population parameter
Significant Statistics Copyright © 2020 by John Morgan Russell, OpenStaxCollege, OpenIntro is licensed under a Creative Commons Attribution-ShareAlike 4.0 International License , except where otherwise noted.
Share This Book
Module 10: Hypothesis Testing With Two Samples
Hypothesis testing with paired samples, learning outcomes.
- Conduct a hypothesis test for matched or paired data and interpret the conclusion in context
When using a hypothesis test for matched or paired samples, the following characteristics should be present:
- Simple random sampling is used.
- Sample sizes are often small.
- Two measurements (samples) are drawn from the same pair of individuals or objects.
- Differences are calculated from the matched or paired samples.
- The differences form the sample that is used for the hypothesis test.
- Either the matched pairs have differences that come from a population that is normal or the number of differences is sufficiently large so that distribution of the sample mean of differences is approximately normal.
In a hypothesis test for matched or paired samples, subjects are matched in pairs, and differences are calculated. The differences are the data. The population mean for the differences, μ d , is then tested using a Student’s t -test for a single population mean with n – 1 degrees of freedom, where n is the number of differences.
The test statistic ( t -score) is:
[latex]\displaystyle{t}=\dfrac{{\overline{{x}}_{{d}}-{\mu}_{{d}}}}{{{(\dfrac{{s}_{{d}}}{\sqrt{{n}}})}}}[/latex]
Recall: ORDER OF OPERATIONS
To calculate the test statistic ( t -score) follow these steps:.
First, find the numerator. Calculate the difference between the matched or paired sample mean and the population mean for the differences, [latex](\overline{x} _d - \mu _d)[/latex].
Second, find the denominator.
Step 1: Find the square root of the sample size (number of differences), [latex]\sqrt{n}[/latex].
Step 2: Take the population standard deviation for the differences and divide by the value you found in Step 1.
Third, take the numerator and divide by the denominator.
A study was conducted to investigate the effectiveness of hypnotism in reducing pain. Results for randomly selected subjects are shown in the table below. A lower score indicates less pain. The “before” value is matched to an “after” value and the differences are calculated. The differences have a normal distribution. Are the sensory measurements, on average, lower after hypnotism? Test at a 5% significance level.
Corresponding “before” and “after” values form matched pairs. (Calculate “after” – “before.”)
The data for the test are the differences: {0.2, –4.1, –1.6, –1.8, –3.2, –2, –2.9, –9.6}
The sample mean and sample standard deviation of the differences are: [latex]\displaystyle\overline{{x}}_{{d}}=-{3.13}{\quad\text{and}\quad}{s}_{{d}}={2.91}[/latex]. Verify these values.
Let [latex]{\mu}_{d}[/latex] be the population mean for the differences. We use the subscript to denote “differences.”
Random variable: [latex]\displaystyle\overline{{X}}_{{d}}[/latex] = the mean difference of the sensory measurements
H 0 : μ d ≥ 0
The null hypothesis is zero or positive, meaning that there is the same or more pain felt after hypnotism. That means the subject shows no improvement. μ d is the population mean of the differences.)
H a : μ d < 0
The alternative hypothesis is negative, meaning there is less pain felt after hypnotism. That means the subject shows improvement. The score should be lower after hypnotism, so the difference ought to be negative to indicate improvement.
Distribution for the test: The distribution is a Student’s t with df = n – 1 = 8 – 1 = 7. Use t 7 . (Notice that the test is for a single population mean.)
Calculate the p -value using the Student’s t -distribution: p -value = 0.0095
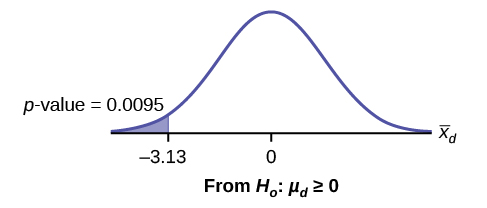
[latex]\displaystyle\overline{{X}}_{{d}}[/latex] is the random variable for the differences.
The sample mean and sample standard deviation of the differences are:
[latex]\displaystyle\overline{{x}}_{{d}}=-{3.13}[/latex]
[latex]\displaystyle{s}_{{d}}={2.91}[/latex]
Compare α and the p -value: α = 0.05 and p -value = 0.0095. α > p -value.
Make a decision: since α > p -value, reject H 0 . This means that μ d < 0 and there is improvement.
Conclusion: at a 5% level of significance, from the sample data, there is sufficient evidence to conclude that the sensory measurements, on average, are lower after hypnotism. Hypnotism appears to be effective in reducing pain.
For the TI-83+ and TI-84 calculators, you can either calculate the differences ahead of time (after – before) and put the differences into a list or you can put the after data into a first list and the before data into a second list. Then, go to a third list and arrow up to the name. Enter 1st list name – 2nd list name. The calculator will do the subtraction, and you will have the differences in the third list.
USING THE TI-83, 83+, 84, 84+ CALCULATOR
- Use your list of differences as the data.
- Press STAT and arrow over to TESTS .
- Press 2:T-Test .
- Arrow over to Data and press ENTER .
- Arrow down and enter 0 for the name of the list where you put the data, and 1 for Freq:.
- Arrow down to μ : and arrow over to < .
- Press ENTER .
- Arrow down to Calculate and press ENTER .
- The p -value is 0.0094, and the test statistic is –3.04.
- Do these instructions again except, arrow to Draw (instead of Calculate ). Press ENTER .
A study was conducted to investigate how effective a new diet was in lowering cholesterol. Results for the randomly selected subjects are shown in the table. The differences have a normal distribution. Are the subjects’ cholesterol levels lower on average after the diet? Test at the 5% level.
The p -value is 0.0130, so we can reject the null hypothesis. There is enough evidence to suggest that the diet lowers cholesterol.
A college football coach was interested in whether the college’s strength development class increased his players’ maximum lift (in pounds) on the bench press exercise. He asked four of his players to participate in a study. The amount of weight they could each lift was recorded before they took the strength development class. After completing the class, the amount of weight they could each lift was again measured. The data are as follows:
The coach wants to know if the strength development class makes his players stronger, on average.
Record the differences data. Calculate the differences by subtracting the amount of weight lifted prior to the class from the weight lifted after completing the class. The data for the differences are: {90, 11, -8, -8}. Assume the differences have a normal distribution.
Using the differences data, calculate the sample mean and the sample standard deviation.
[latex]\displaystyle\overline{{x}}_{{d}}={21.3},{s}_{{d}}={46.7}[/latex]
The data given here would indicate that the distribution is actually right-skewed. The difference 90 may be an extreme outlier? It is pulling the sample mean to be 21.3 (positive). The means of the other three data values are actually negative.
Using the difference data, this becomes a test of a single __________ (fill in the blank).
Define the random variable: [latex]\displaystyle\overline{{X}}_{{d}}[/latex] mean difference in the maximum lift per player.
The distribution for the hypothesis test is t 3 .
H 0 : μ d ≤ 0, H a : μ d > 0
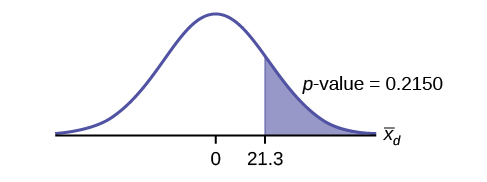
Calculate the p -value: the p -value is 0.2150
Decision: if the level of significance is 5%, the decision is not to reject the null hypothesis, because α < p -value.
Conclusion: a t a 5% level of significance, from the sample data, there is not sufficient evidence to conclude that the strength development class helped to make the players stronger, on average.
A new prep class was designed to improve SAT test scores. Five students were selected at random. Their scores on two practice exams were recorded, one before the class and one after. The data recorded in this table. Are the scores, on average, higher after the class? Test at a 5% level.
The p -value is 0.0874, so we decline to reject the null hypothesis. The data do not support that the class improves SAT scores significantly.
Seven eighth-graders at Kennedy Middle School measured how far they could push the shot-put with their dominant (writing) hand and their weaker (non-writing) hand. They thought that they could push equal distances with either hand. The data were collected and recorded in this table.
Conduct a hypothesis test to determine whether the mean difference in distances between the children’s dominant versus weaker hands is significant.
Record the differences data. Calculate the differences by subtracting the distances with the weaker hand from the distances with the dominant hand. The data for the differences are: {2, 12, 7, –1, 2, 0, 4}. The differences have a normal distribution.
Using the differences data, calculate the sample mean and the sample standard deviation. [latex]\displaystyle\overline{{x}}_{{d}}={3.71},{s}_{{d}}={4.5}[/latex].
Random variable: [latex]\displaystyle\overline{{X}}_{{d}}[/latex] = mean difference in the distances between the hands.
Distribution for the hypothesis test: t 6
H 0 : μ d = 0 H a : μ d ≠ 0
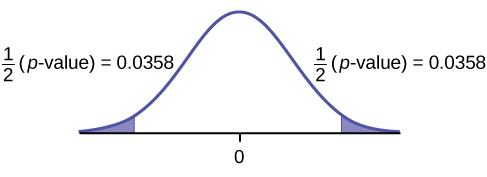
Calculate the p -value: the p -value is 0.0716 (using the data directly).
(test statistic = 2.18. p -value = 0.0719 using ([latex]{\overline x} = 3.71, {s_d} = 4.5[/latex].)
Decision: assume α = 0.05. Since α < p -value, Do not reject H 0 .
Conclusion: at the 5% level of significance, from the sample data, there is not sufficient evidence to conclude that there is a difference in the children’s weaker and dominant hands to push the shot-put.
Five ball players think they can throw the same distance with their dominant hand (throwing) and off-hand (catching hand). The data were collected and recorded in the table below. Conduct a hypothesis test to determine whether the mean difference in distances between the dominant and off-hand is significant. Test at the 5% level.
The p -level is 0.0230, so we can reject the null hypothesis. The data show that the players do not throw the same distance with their off-hands as they do with their dominant hands.
- Statistics, Matched or Paired Sample. Provided by : OpenStax. Located at : https://openstax.org/books/statistics/pages/10-4-matched-or-paired-samples-optional . License : CC BY: Attribution . License Terms : Access for free at https://openstax.org/books/statistics/pages/1-introduction
- Introductory Statistics. Authored by : Barbara Illowsky, Susan Dean. Provided by : OpenStax. Located at : https://openstax.org/books/introductory-statistics/pages/1-introduction . License : CC BY: Attribution . License Terms : Access for free at https://openstax.org/books/introductory-statistics/pages/1-introduction
- Z-statistics vs. T-statistics | Inferential statistics | Probability and Statistics | Khan Academy . Authored by : Khan Academy. Located at : https://www.youtube.com/embed/5ABpqVSx33I . License : All Rights Reserved . License Terms : Standard YouTube License
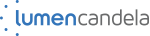
Privacy Policy
Teach yourself statistics
Hypothesis Test: Difference Between Paired Means
This lesson explains how to conduct a hypothesis test for the difference between paired means . The test procedure, called the matched-pairs t-test , is appropriate when the following conditions are met:
- The sampling method for each sample is simple random sampling .
- The test is conducted on paired data. (As a result, the data sets are not independent .)
- The population distribution is normal.
- The population data are symmetric , unimodal , without outliers , and the sample size is 15 or less.
- The population data are slightly skewed , unimodal, without outliers, and the sample size is 16 to 40.
- The sample size is greater than 40, without outliers.
This approach consists of four steps: (1) state the hypotheses, (2) formulate an analysis plan, (3) analyze sample data, and (4) interpret results.
State the Hypotheses
Every hypothesis test requires the analyst to state a null hypothesis and an alternative hypothesis . The hypotheses are stated in such a way that they are mutually exclusive. That is, if one is true, the other must be false; and vice versa.
The hypotheses concern a new variable d, which is based on the difference between paired values from two data sets.
d = x 1 - x 2
where x 1 is the value of variable x in the first data set, and x 2 is the value of the variable from the second data set that is paired with x 1 .
The table below shows three sets of null and alternative hypotheses. Each makes a statement about how the true difference in population values μ d is related to some hypothesized value D. (In the table, the symbol ≠ means " not equal to ".)
The first set of hypotheses (Set 1) is an example of a two-tailed test , since an extreme value on either side of the sampling distribution would cause a researcher to reject the null hypothesis. The other two sets of hypotheses (Sets 2 and 3) are one-tailed tests , since an extreme value on only one side of the sampling distribution would cause a researcher to reject the null hypothesis.
Formulate an Analysis Plan
The analysis plan describes how to use sample data to accept or reject the null hypothesis. It should specify the following elements.
- Significance level. Often, researchers choose significance levels equal to 0.01, 0.05, or 0.10; but any value between 0 and 1 can be used.
- Test method. Use the matched-pairs t-test to determine whether the difference between sample means for paired data is significantly different from the hypothesized difference between population means.
Analyze Sample Data
Using sample data, find the standard deviation, standard error, degrees of freedom, test statistic, and the P-value associated with the test statistic.
s d = sqrt [ (Σ(d i - d ) 2 / (n - 1) ]
SE = s d * sqrt{ ( 1/n ) * [ (N - n) / ( N - 1 ) ] }
SE = s d / sqrt( n )
- Degrees of freedom. The degrees of freedom (DF) is: DF = n - 1 .
t = [ ( x 1 - x 2 ) - D ] / SE = ( d - D) / SE
- P-value. The P-value is the probability of observing a sample statistic as extreme as the test statistic. Since the test statistic is a t statistic, use the t Distribution Calculator to assess the probability associated with the t statistic, having the degrees of freedom computed above. (See the sample problem at the end of this lesson for guidance on how this is done.)
Interpret Results
If the sample findings are unlikely, given the null hypothesis, the researcher rejects the null hypothesis. Typically, this involves comparing the P-value to the significance level , and rejecting the null hypothesis when the P-value is less than the significance level.
Test Your Understanding
Forty-four sixth graders were randomly selected from a school district. Then, they were divided into 22 matched pairs, each pair having equal IQ's. One member of each pair was randomly selected to receive special training. Then, all of the students were given an IQ test. Test results are summarized below.
Σ(d - d ) 2 = 270
Do these results provide evidence that the special training helped or hurt student performance? Use an 0.05 level of significance. Assume that the mean differences are approximately normally distributed.
The solution to this problem takes four steps: (1) state the hypotheses, (2) formulate an analysis plan, (3) analyze sample data, and (4) interpret results. We work through those steps below:
Null hypothesis: μ d = 0
Alternative hypothesis: μ d ≠ 0
- Formulate an analysis plan . For this analysis, the significance level is 0.05. Using sample data, we will conduct a matched-pairs t-test of the null hypothesis.
s = sqrt [ (Σ(d i - d ) 2 / (n - 1) ]
s = sqrt[ 270/(22-1) ] = sqrt(12.857) = 3.586
SE = s / sqrt(n) = 3.586 / [ sqrt(22) ]
SE = 3.586/4.69 = 0.765
DF = n - 1 = 22 -1 = 21
t = [ ( x 1 - x 2 ) - D ] / SE
t = ( d - D)/ SE = (1 - 0)/0.765 = 1.307
where d i is the observed difference for pair i , d is mean difference between sample pairs, D is the hypothesized mean difference between population pairs, and n is the number of pairs.
Since we have a two-tailed test , the P-value is the probability that the t statistic having 21 degrees of freedom is less than -1.307 or greater than 1.307. We use the t Distribution Calculator to find P(t < -1.307) is about 0.103.
- Then, we enter 1.307 as the sample mean in the t Distribution Calculator. We find the that the P(t < 1.307) is about 0.897. Therefore, P(t > 1.307) is 1 minus 0.897 or 0.103. Thus, the P-value = 0.103 + 0.103 = 0.206.
- Interpret results . Since the P-value (0.206) is greater than the significance level (0.05), we cannot reject the null hypothesis.
Note: If you use this approach on an exam, you may also want to mention why this approach is appropriate. Specifically, the approach is appropriate because the sampling method was simple random sampling, the samples consisted of paired data, and the mean differences were normally distributed. In addition, we used the approximation formula to compute the standard error, since the sample size was small relative to the population size.

Hypothesis Testing for Means & Proportions
- 1
- | 2
- | 3
- | 4
- | 5
- | 6
- | 7
- | 8
- | 9
- | 10

All Modules

Z score Table
t score Table
Tests with Matched Samples, Continuous Outcome
In the previous section we compared two groups with respect to their mean scores on a continuous outcome. An alternative study design is to compare matched or paired samples. The two comparison groups are said to be dependent, and the data can arise from a single sample of participants where each participant is measured twice (possibly before and after an intervention) or from two samples that are matched on specific characteristics (e.g., siblings). When the samples are dependent, we focus on difference scores in each participant or between members of a pair and the test of hypothesis is based on the mean difference, μ d . The null hypothesis again reflects "no difference" and is stated as H 0 : μ d =0 . Note that there are some instances where it is of interest to test whether there is a difference of a particular magnitude (e.g., μ d =5) but in most instances the null hypothesis reflects no difference (i.e., μ d =0).
The appropriate formula for the test of hypothesis depends on the sample size. The formulas are shown below and are identical to those we presented for estimating the mean of a single sample presented (e.g., when comparing against an external or historical control), except here we focus on difference scores.
Test Statistics for Testing H 0 : μ d =0
- if n > 30
- if n < 30
A new drug is proposed to lower total cholesterol and a study is designed to evaluate the efficacy of the drug in lowering cholesterol. Fifteen patients agree to participate in the study and each is asked to take the new drug for 6 weeks. However, before starting the treatment, each patient's total cholesterol level is measured. The initial measurement is a pre-treatment or baseline value. After taking the drug for 6 weeks, each patient's total cholesterol level is measured again and the data are shown below. The rightmost column contains difference scores for each patient, computed by subtracting the 6 week cholesterol level from the baseline level. The differences represent the reduction in total cholesterol over 4 weeks. (The differences could have been computed by subtracting the baseline total cholesterol level from the level measured at 6 weeks. The way in which the differences are computed does not affect the outcome of the analysis only the interpretation.)
Because the differences are computed by subtracting the cholesterols measured at 6 weeks from the baseline values, positive differences indicate reductions and negative differences indicate increases (e.g., participant 12 increases by 2 units over 6 weeks). The goal here is to test whether there is a statistically significant reduction in cholesterol. Because of the way in which we computed the differences, we want to look for an increase in the mean difference (i.e., a positive reduction). In order to conduct the test, we need to summarize the differences. In this sample, we have
The calculations are shown below.
Is there statistical evidence of a reduction in mean total cholesterol in patients after using the new medication for 6 weeks? We will run the test using the five-step approach.
- Step 1. Set up hypotheses and determine level of significance
H 0 : μ d = 0 H 1 : μ d > 0 α=0.05
NOTE: If we had computed differences by subtracting the baseline level from the level measured at 6 weeks then negative differences would have reflected reductions and the research hypothesis would have been H 1 : μ d < 0.
- Step 2 . Select the appropriate test statistic.
Because the sample size is small (n<30) the appropriate test statistic is
- Step 3. Set up decision rule.
This is an upper-tailed test, using a t statistic and a 5% level of significance. The appropriate critical value can be found in the t Table at the right, with df=15-1=14. The critical value for an upper-tailed test with df=14 and α=0.05 is 2.145 and the decision rule is Reject H 0 if t > 2.145.
- Step 4. Compute the test statistic.
We now substitute the sample data into the formula for the test statistic identified in Step 2.
- Step 5. Conclusion.
We reject H 0 because 4.61 > 2.145. We have statistically significant evidence at α=0.05 to show that there is a reduction in cholesterol levels over 6 weeks.
Here we illustrate the use of a matched design to test the efficacy of a new drug to lower total cholesterol. We also considered a parallel design (randomized clinical trial) and a study using a historical comparator. It is extremely important to design studies that are best suited to detect a meaningful difference when one exists. There are often several alternatives and investigators work with biostatisticians to determine the best design for each application. It is worth noting that the matched design used here can be problematic in that observed differences may only reflect a "placebo" effect . All participants took the assigned medication, but is the observed reduction attributable to the medication or a result of these participation in a study.
Video - Hypothesis Testing With a Matched Sample and a Continuous Outcome (3:11)
Link to transcript of the video

return to top | previous page | next page
Content ©2017. All Rights Reserved. Date last modified: November 6, 2017. Wayne W. LaMorte, MD, PhD, MPH
10.6 Matched or Paired Samples
In most cases of economic or business data we have little or no control over the process of how the data are gathered. In this sense the data are not the result of a planned controlled experiment. In some cases, however, we can develop data that are part of a controlled experiment. This situation occurs frequently in quality control situations. Imagine that the production rates of two machines built to the same design, but at different manufacturing plants, are being tested for differences in some production metric such as speed of output or meeting some production specification such as strength of the product. The test is the same in format to what we have been testing, but here we can have matched pairs for which we can test if differences exist. Each observation has its matched pair against which differences are calculated. First, the differences in the metric to be tested between the two lists of observations must be calculated, and this is typically labeled with the letter "d." Then, the average of these matched differences, X ¯ d X ¯ d is calculated as is its standard deviation, S d . We expect that the standard deviation of the differences of the matched pairs will be smaller than unmatched pairs because presumably fewer differences should exist because of the correlation between the two groups.
When using a hypothesis test for matched or paired samples, the following characteristics may be present:
- Simple random sampling is used.
- Sample sizes are often small.
- Two measurements (samples) are drawn from the same pair of individuals or objects.
- Differences are calculated from the matched or paired samples.
- The differences form the sample that is used for the hypothesis test.
- Either the matched pairs have differences that come from a population that is normal or the number of differences is sufficiently large so that distribution of the sample mean of differences is approximately normal.
In a hypothesis test for matched or paired samples, subjects are matched in pairs and differences are calculated. The differences are the data. The population mean for the differences, μ d , is then tested using a Student's-t test for a single population mean with n – 1 degrees of freedom, where n is the number of differences, that is, the number of pairs not the number of observations.
Example 10.9
A company has developed a training program for its entering employees because they have become concerned with the results of the six-month employee review. They hope that the training program can result in better six-month reviews. Each trainee constitutes a “pair”, the entering score the employee received when first entering the firm and the score given at the six-month review. The difference in the two scores were calculated for each employee and the means for before and after the training program was calculated. The sample mean before the training program was 20.4 and the sample mean after the training program was 23.9. The standard deviation of the differences in the two scores across the 20 employees was 3.8 points. Test at the 10% significance level the null hypothesis that the two population means are equal against the alternative that the training program helps improve the employees’ scores.
The first step is to identify this as a two sample case: before the training and after the training. This differentiates this problem from simple one sample issues. Second, we determine that the two samples are "paired." Each observation in the first sample has a paired observation in the second sample. This information tells us that the null and alternative hypotheses should be:
This form reflects the implied claim that the training course improves scores; the test is one-tailed and the claim is in the alternative hypothesis. Because the experiment was conducted as a matched paired sample rather than simply taking scores from people who took the training course those who didn't, we use the matched pair test statistic:
In order to solve this equation, the individual scores, pre-training course and post-training course need to be used to calculate the individual differences. These scores are then averaged and the average difference is calculated:
From these differences we can calculate the standard deviation across the individual differences:
We can now compare the calculated value of the test statistic, 4.12, with the critical value. The critical value is a Student's t with degrees of freedom equal to the number of pairs, not observations, minus 1. In this case 20 pairs and at 90% confidence level t a/2 = ±1.729 at df = 20 - 1 = 19. The calculated test statistic is most certainly in the tail of the distribution and thus we cannot accept the null hypothesis that there is no difference from the training program. Evidence seems indicate that the training aids employees in gaining higher scores.
Example 10.10
A study was conducted to investigate the effectiveness of hypnotism in reducing pain. Results for randomly selected subjects are shown in Table 10.5 . A lower score indicates less pain. The "before" value is matched to an "after" value and the differences are calculated. Are the sensory measurements, on average, lower after hypnotism? Test at a 5% significance level.
Corresponding "before" and "after" values form matched pairs. (Calculate "after" – "before.")
The data for the test are the differences: {0.2, –4.1, –1.6, –1.8, –3.2, –2, –2.9, –9.6}
The sample mean and sample standard deviation of the differences are: x – d = –3.13 x – d = –3.13 and s d = 2.91 s d = 2.91 Verify these values.
Let μ d μ d be the population mean for the differences. We use the subscript d d to denote "differences."
Random variable: X – d X – d = the mean difference of the sensory measurements
H 0 : μ d ≥ 0
The null hypothesis is zero or positive, meaning that there is the same or more pain felt after hypnotism. That means the subject shows no improvement. μ d is the population mean of the differences.)
H a : μ d < 0
The alternative hypothesis is negative, meaning there is less pain felt after hypnotism. That means the subject shows improvement. The score should be lower after hypnotism, so the difference ought to be negative to indicate improvement.
Distribution for the test: The distribution is a Student's t with df = n – 1 = 8 – 1 = 7. Use t 7 . (Notice that the test is for a single population mean.)
Calculate the test statistic and look up the critical value using the Student's-t distribution: The calculated value of the test statistic is 3.06 and the critical value of the t -distribution with 7 degrees of freedom at the 5% level of confidence is 1.895 with a one-tailed test.
X – d X – d is the random variable for the differences.
The sample mean and sample standard deviation of the differences are:
x – d x – d = –3.13
s – d s – d = 2.91
Compare the critical value for alpha against the calculated test statistic.
The conclusion from using the comparison of the calculated test statistic and the critical value will gives us the result. In this question the calculated test statistic is 3.06 and the critical value is 1.895. The test statistic is clearly in the tail and thus we cannot accept the null hypotheses that there is no difference between the two situations, hypnotized and not hypnotized.
Make a decision: Cannot accept the null hypothesis, H 0 . This means that μ d < 0 and there is a statistically significant improvement.
Conclusion: At a 5% level of significance, from the sample data, there is sufficient evidence to conclude that the sensory measurements, on average, are lower after hypnotism. Hypnotism appears to be effective in reducing pain.
Example 10.11
A college football coach was interested in whether the college's strength development class increased his players' maximum lift (in pounds) on the bench press exercise. He asked four of his players to participate in a study. The amount of weight they could each lift was recorded before they took the strength development class. After completing the class, the amount of weight they could each lift was again measured. The data are as follows:
The coach wants to know if the strength development class makes his players stronger, on average. Record the differences data. Calculate the differences by subtracting the amount of weight lifted prior to the class from the weight lifted after completing the class. The data for the differences are: {90, 11, -8, -8}.
x – d x – d = 21.3, s d = 46.7
Using the difference data, this becomes a test of a single mean.
Define the random variable: X – d X – d mean difference in the maximum lift per player.
The distribution for the hypothesis test is a student's t with 3 degrees of freedom.
H 0 : μ d ≤ 0, H a : μ d > 0
Calculate the test statistic look up the critical value: Critical value of the test statistic is 0.91. The critical value of the student's t at 5% level of significance and 3 degrees of freedom is 2.353.
Decision: If the level of significance is 5%, we cannot reject the null hypothesis, because the calculated value of the test statistic is not in the tail.
What is the conclusion?
At a 5% level of significance, from the sample data, there is not sufficient evidence to conclude that the strength development class helped to make the players stronger, on average.
As an Amazon Associate we earn from qualifying purchases.
This book may not be used in the training of large language models or otherwise be ingested into large language models or generative AI offerings without OpenStax's permission.
Want to cite, share, or modify this book? This book uses the Creative Commons Attribution License and you must attribute OpenStax.
Access for free at https://openstax.org/books/introductory-business-statistics/pages/1-introduction
- Authors: Alexander Holmes, Barbara Illowsky, Susan Dean
- Publisher/website: OpenStax
- Book title: Introductory Business Statistics
- Publication date: Nov 29, 2017
- Location: Houston, Texas
- Book URL: https://openstax.org/books/introductory-business-statistics/pages/1-introduction
- Section URL: https://openstax.org/books/introductory-business-statistics/pages/10-6-matched-or-paired-samples
© Jun 23, 2022 OpenStax. Textbook content produced by OpenStax is licensed under a Creative Commons Attribution License . The OpenStax name, OpenStax logo, OpenStax book covers, OpenStax CNX name, and OpenStax CNX logo are not subject to the Creative Commons license and may not be reproduced without the prior and express written consent of Rice University.
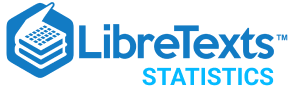
- school Campus Bookshelves
- menu_book Bookshelves
- perm_media Learning Objects
- login Login
- how_to_reg Request Instructor Account
- hub Instructor Commons
Margin Size
- Download Page (PDF)
- Download Full Book (PDF)
- Periodic Table
- Physics Constants
- Scientific Calculator
- Reference & Cite
- Tools expand_more
- Readability
selected template will load here
This action is not available.
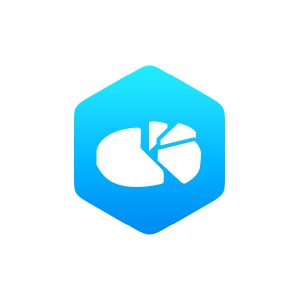
10: Hypothesis Testing for Paired and Unpaired Data
- Last updated
- Save as PDF
- Page ID 26111
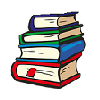
\( \newcommand{\vecs}[1]{\overset { \scriptstyle \rightharpoonup} {\mathbf{#1}} } \)
\( \newcommand{\vecd}[1]{\overset{-\!-\!\rightharpoonup}{\vphantom{a}\smash {#1}}} \)
\( \newcommand{\id}{\mathrm{id}}\) \( \newcommand{\Span}{\mathrm{span}}\)
( \newcommand{\kernel}{\mathrm{null}\,}\) \( \newcommand{\range}{\mathrm{range}\,}\)
\( \newcommand{\RealPart}{\mathrm{Re}}\) \( \newcommand{\ImaginaryPart}{\mathrm{Im}}\)
\( \newcommand{\Argument}{\mathrm{Arg}}\) \( \newcommand{\norm}[1]{\| #1 \|}\)
\( \newcommand{\inner}[2]{\langle #1, #2 \rangle}\)
\( \newcommand{\Span}{\mathrm{span}}\)
\( \newcommand{\id}{\mathrm{id}}\)
\( \newcommand{\kernel}{\mathrm{null}\,}\)
\( \newcommand{\range}{\mathrm{range}\,}\)
\( \newcommand{\RealPart}{\mathrm{Re}}\)
\( \newcommand{\ImaginaryPart}{\mathrm{Im}}\)
\( \newcommand{\Argument}{\mathrm{Arg}}\)
\( \newcommand{\norm}[1]{\| #1 \|}\)
\( \newcommand{\Span}{\mathrm{span}}\) \( \newcommand{\AA}{\unicode[.8,0]{x212B}}\)
\( \newcommand{\vectorA}[1]{\vec{#1}} % arrow\)
\( \newcommand{\vectorAt}[1]{\vec{\text{#1}}} % arrow\)
\( \newcommand{\vectorB}[1]{\overset { \scriptstyle \rightharpoonup} {\mathbf{#1}} } \)
\( \newcommand{\vectorC}[1]{\textbf{#1}} \)
\( \newcommand{\vectorD}[1]{\overrightarrow{#1}} \)
\( \newcommand{\vectorDt}[1]{\overrightarrow{\text{#1}}} \)
\( \newcommand{\vectE}[1]{\overset{-\!-\!\rightharpoonup}{\vphantom{a}\smash{\mathbf {#1}}}} \)
You have learned to conduct hypothesis tests on single means and single proportions. You will expand upon that in this chapter. You will compare two means or two proportions to each other. The general procedure is still the same, just expanded. To compare two means or two proportions, you work with two groups. The groups are classified either as independent or matched pairs. Independent groups consist of two samples that are independent, that is, sample values selected from one population are not related in any way to sample values selected from the other population. Matched pairs consist of two samples that are dependent. The parameter tested using matched pairs is the population mean. The parameters tested using independent groups are either population means or population proportions.
- 10.1: Two Population Means The comparison of two population means is very common. A difference between the two samples depends on both the means and the standard deviations. Very different means can occur by chance if there is great variation among the individual samples.
- 10.2: Two Independent Population Proportions Comparing two proportions, like comparing two means, is common. If two estimated proportions are different, it may be due to a difference in the populations or it may be due to chance. A hypothesis test can help determine if a difference in the estimated proportions reflects a difference in the population proportions.
- 10.3: Matched or Paired Samples When using a hypothesis test for matched or paired samples, the following characteristics should be present: Simple random sampling is used. Sample sizes are often small. Two measurements (samples) are drawn from the same pair of individuals or objects. Differences are calculated from the matched or paired samples. The differences form the sample that is used for the hypothesis test. Either the matched pairs have differences that come from a population that is normal or the number of difference
- 10.4: Two Population Means with Known Standard Deviations Even though this situation is not likely (knowing the population standard deviations is not likely), the following example illustrates hypothesis testing for independent means, known population standard deviations.
- 10.5: Difference of Two Means In this section we consider a difference in two population means, μ1−μ2, under the condition that the data are not paired. The methods are similar in theory but different in the details. Just as with a single sample, we identify conditions to ensure a point estimate of the difference is nearly normal. Next we introduce a formula for the standard error, which allows us to apply our general tools discussed previously.
Contributors and Attributions
Barbara Illowsky and Susan Dean (De Anza College) with many other contributing authors. Content produced by OpenStax College is licensed under a Creative Commons Attribution License 4.0 license. Download for free at http://cnx.org/contents/[email protected] .
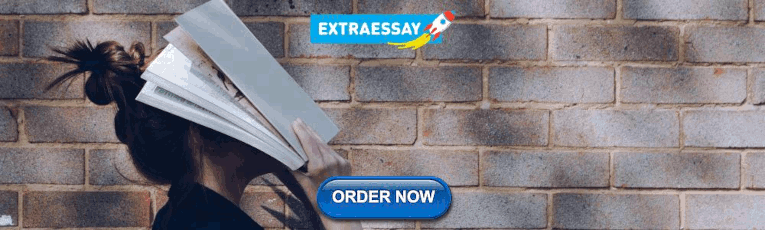
IMAGES
VIDEO
COMMENTS
In a hypothesis test for matched or paired samples, subjects are matched in pairs and differences are calculated. The differences are the data. The population mean for the differences, \(\mu_{d}\), is then tested using a Student's \(t\)-test for a single population mean with \(n - 1\) degrees of freedom, where \(n\) is the number of differences
In a hypothesis test for matched or paired samples, subjects are matched in pairs and differences are calculated. The differences are the data. The population mean for the differences, μ d, is then tested using a Student's-t test for a single population mean with n - 1 degrees of freedom, where n is the number of differences.
In a hypothesis test for matched or paired samples, subjects are matched in pairs and differences are calculated. The differences are the data. The population mean for the differences, μ d, is then tested using a Student's-t test for a single population mean with n - 1 degrees of freedom, where n is the number of differences.
The requirements for hypothesis testing with dependent samples are: Samples taken are simple random samples with observations that are independent within each group. Sample sizes are often small. ... If the above conditions hold, then we can use the sampling distribution of the mean of the differences between matched pairs in the sample, [latex ...
The Test Statistic for a Test of Matched Pairs (2 Means from Dependent Samples): t = ¯x − 0 s √n t = x ¯ − 0 s n. n n is the sample size, or the number of pairs of data. df = n −1 d f = n − 1 is the degrees of freedom. μd μ d is the mean value of the differences for the population of all matched pairs of data.
Hypothesis Tests for the Mean difference. In a hypothesis test for matched or paired samples, subjects are matched in pairs and differences are calculated, and the population mean difference, μ d, is our parameter of interest. Although it is possible to test for a certain magnitude of effect, we are most often just looking for a general effect.
The hypothesis test for matched samples is a well established process: Write down the null and alternative hypotheses in terms of the mean difference [latex]\mu_D[/latex]. Use the form of the alternative hypothesis to determine if the test is left-tailed, right-tailed, or two-tailed.
Hypothesis Tests for the Mean difference. In a hypothesis test for matched or paired samples, subjects are matched in pairs and differences are calculated, and the population mean difference, μ d, is our parameter of interest. Although it is possible to test for a certain magnitude of effect, we are most often just looking for a general effect.
In a hypothesis test for matched or paired samples, subjects are matched in pairs and differences are calculated. The differences are the data. The population mean for the differences, μ d, is then tested using a Student's-t test for a single population mean with n - 1 degrees of freedom, where n is the number of differences.
In a hypothesis test for matched or paired samples, subjects are matched in pairs and differences are calculated. The differences are the data. The population mean for the differences, μd, is then tested using a Student's-t test for a single population mean with n - 1 degrees of freedom, where n is the number of differences, that is, the ...
Hypothesis Tests for the Mean difference. In a hypothesis test for matched or paired samples, subjects are matched in pairs and differences are calculated, and the population mean difference, μ d, is our parameter of interest. Although it is possible to test for a certain magnitude of effect, we are most often just looking for a general effect.
In a hypothesis test for matched or paired samples, subjects are matched in pairs, and differences are calculated. The differences are the data. The population mean for the differences, μ d, is then tested using a Student's t-test for a single population mean with n - 1 degrees of freedom, where n is the number of differences.
Another case of a t-test is when we have two samples that are related to each other i.e. the matched pairs situation, where we will use a paired t-test. For a one sample t-test, the null hypothesis H₀ is "the mean is equal to θ₀" vs. the alternative hypothesis Ha that "the mean is not equal to/less than/greater θ₀".
Present the findings in your results and discussion section. Though the specific details might vary, the procedure you will use when testing a hypothesis will always follow some version of these steps. Table of contents. Step 1: State your null and alternate hypothesis. Step 2: Collect data. Step 3: Perform a statistical test.
Hypothesis Test: Difference Between Paired Means. This lesson explains how to conduct a hypothesis test for the difference between paired means.The test procedure, called the matched-pairs t-test, is appropriate when the following conditions are met: The sampling method for each sample is simple random sampling.
The critical value for an upper-tailed test with df=14 and α=0.05 is 2.145 and the decision rule is Reject H 0 if t > 2.145. Step 4. Compute the test statistic. We now substitute the sample data into the formula for the test statistic identified in Step 2. Step 5.
In a hypothesis test for matched or paired samples, subjects are matched in pairs and differences are calculated. The differences are the data. The population mean for the differences, \(\mu_{d}\), is then tested using a Student's \(t\)-test for a single population mean with \(n - 1\) degrees of freedom, where \(n\) is the number of differences
In a hypothesis test for matched or paired samples, subjects are matched in pairs and differences are calculated. The differences are the data. The population mean for the differences, μ d , is then tested using a Student's-t test for a single population mean with n - 1 degrees of freedom, where n is the number of differences, that is, the ...
A hypothesis test can help determine if a difference in the estimated proportions reflects a difference in the population proportions. 10.3: Matched or Paired Samples When using a hypothesis test for matched or paired samples, the following characteristics should be present: Simple random sampling is used. Sample sizes are often small.
In this article, I won't delve into how sample size is computed (I will probably do it in a follow-up). For now, let's simply use the Statmodel's function for testing the difference between sample means as a black box: ### input (hypothesis + confusion matrix) control_mean = 10 control_std = 8 treatment_mean = 10.5 treatment_std = 9 confidence = .975 power = .80 ### compute sample size ...