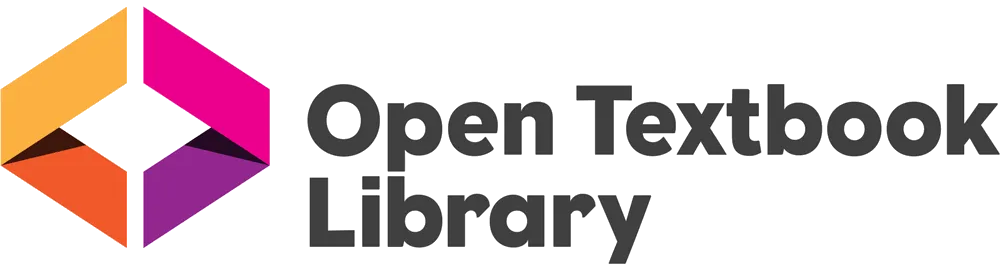
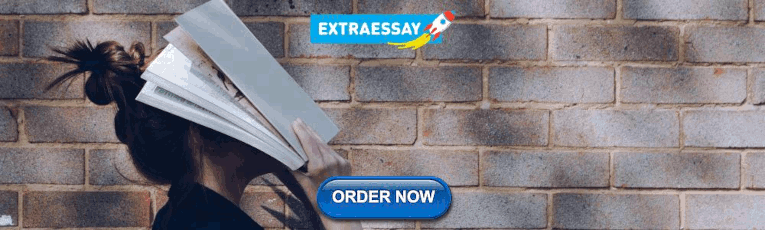
A Quick Guide to Quantitative Research in the Social Sciences
(12 reviews)

Christine Davies, Carmarthen, Wales
Copyright Year: 2020
Last Update: 2021
Publisher: University of Wales Trinity Saint David
Language: English
Formats Available
Conditions of use.

Learn more about reviews.

Reviewed by Jennifer Taylor, Assistant Professor, Texas A&M University-Corpus Christi on 4/18/24
This resource is a quick guide to quantitative research in the social sciences and not a comprehensive resource. It provides a VERY general overview of quantitative research but offers a good starting place for students new to research. It... read more
Comprehensiveness rating: 4 see less
This resource is a quick guide to quantitative research in the social sciences and not a comprehensive resource. It provides a VERY general overview of quantitative research but offers a good starting place for students new to research. It offers links and references to additional resources that are more comprehensive in nature.
Content Accuracy rating: 4
The content is relatively accurate. The measurement scale section is very sparse. Not all types of research designs or statistical methods are included, but it is a guide, so details are meant to be limited.
Relevance/Longevity rating: 4
The examples were interesting and appropriate. The content is up to date and will be useful for several years.
Clarity rating: 5
The text was clearly written. Tables and figures are not referenced in the text, which would have been nice.
Consistency rating: 5
The framework is consistent across chapters with terminology clearly highlighted and defined.
Modularity rating: 5
The chapters are subdivided into section that can be divided and assigned as reading in a course. Most chapters are brief and concise, unless elaboration is necessary, such as with the data analysis chapter. Again, this is a guide and not a comprehensive text, so sections are shorter and don't always include every subtopic that may be considered.
Organization/Structure/Flow rating: 5
The guide is well organized. I appreciate that the topics are presented in a logical and clear manner. The topics are provided in an order consistent with traditional research methods.
Interface rating: 5
The interface was easy to use and navigate. The images were clear and easy to read.
Grammatical Errors rating: 5
I did not notice any grammatical errors.
Cultural Relevance rating: 5
The materials are not culturally insensitive or offensive in any way.
I teach a Marketing Research course to undergraduates. I would consider using some of the chapters or topics included, especially the overview of the research designs and the analysis of data section.
Reviewed by Tiffany Kindratt, Assistant Professor, University of Texas at Arlington on 3/9/24
The text provides a brief overview of quantitative research topics that is geared towards research in the fields of education, sociology, business, and nursing. The author acknowledges that the textbook is not a comprehensive resource but offers... read more
Comprehensiveness rating: 3 see less
The text provides a brief overview of quantitative research topics that is geared towards research in the fields of education, sociology, business, and nursing. The author acknowledges that the textbook is not a comprehensive resource but offers references to other resources that can be used to deepen the knowledge. The text does not include a glossary or index. The references in the figures for each chapter are not included in the reference section. It would be helpful to include those.
Overall, the text is accurate. For example, Figure 1 on page 6 provides a clear overview of the research process. It includes general definitions of primary and secondary research. It would be helpful to include more details to explain some of the examples before they are presented. For instance, the example on page 5 was unclear how it pertains to the literature review section.
In general, the text is relevant and up-to-date. The text includes many inferences of moving from qualitative to quantitative analysis. This was surprising to me as a quantitative researcher. The author mentions that moving from a qualitative to quantitative approach should only be done when needed. As a predominantly quantitative researcher, I would not advice those interested in transitioning to using a qualitative approach that qualitative research would enhance their research—not something that should only be done if you have to.
Clarity rating: 4
The text is written in a clear manner. It would be helpful to the reader if there was a description of the tables and figures in the text before they are presented.
Consistency rating: 4
The framework for each chapter and terminology used are consistent.
Modularity rating: 4
The text is clearly divided into sections within each chapter. Overall, the chapters are a similar brief length except for the chapter on data analysis, which is much more comprehensive than others.
Organization/Structure/Flow rating: 4
The topics in the text are presented in a clear and logical order. The order of the text follows the conventional research methodology in social sciences.
I did not encounter any interface issues when reviewing this text. All links worked and there were no distortions of the images or charts that may confuse the reader.
Grammatical Errors rating: 3
There are some grammatical/typographical errors throughout. Of note, for Section 5 in the table of contents. “The” should be capitalized to start the title. In the title for Table 3, the “t” in typical should be capitalized.
Cultural Relevance rating: 4
The examples are culturally relevant. The text is geared towards learners in the UK, but examples are relevant for use in other countries (i.e., United States). I did not see any examples that may be considered culturally insensitive or offensive in any way.
I teach a course on research methods in a Bachelor of Science in Public Health program. I would consider using some of the text, particularly in the analysis chapter to supplement the current textbook in the future.
Reviewed by Finn Bell, Assistant Professor, University of Michigan, Dearborn on 1/3/24
For it being a quick guide and only 26 pages, it is very comprehensive, but it does not include an index or glossary. read more
For it being a quick guide and only 26 pages, it is very comprehensive, but it does not include an index or glossary.
Content Accuracy rating: 5
As far as I can tell, the text is accurate, error-free and unbiased.
Relevance/Longevity rating: 5
This text is up-to-date, and given the content, unlikely to become obsolete any time soon.
The text is very clear and accessible.
The text is internally consistent.
Given how short the text is, it seems unnecessary to divide it into smaller readings, nonetheless, it is clearly labelled such that an instructor could do so.
The text is well-organized and brings readers through basic quantitative methods in a logical, clear fashion.
Easy to navigate. Only one table that is split between pages, but not in a way that is confusing.
There were no noticeable grammatical errors.
The examples in this book don't give enough information to rate this effectively.
This text is truly a very quick guide at only 26 double-spaced pages. Nonetheless, Davies packs a lot of information on the basics of quantitative research methods into this text, in an engaging way with many examples of the concepts presented. This guide is more of a brief how-to that takes readers as far as how to select statistical tests. While it would be impossible to fully learn quantitative research from such a short text, of course, this resource provides a great introduction, overview, and refresher for program evaluation courses.
Reviewed by Shari Fedorowicz, Adjunct Professor, Bridgewater State University on 12/16/22
The text is indeed a quick guide for utilizing quantitative research. Appropriate and effective examples and diagrams were used throughout the text. The author clearly differentiates between use of quantitative and qualitative research providing... read more
Comprehensiveness rating: 5 see less
The text is indeed a quick guide for utilizing quantitative research. Appropriate and effective examples and diagrams were used throughout the text. The author clearly differentiates between use of quantitative and qualitative research providing the reader with the ability to distinguish two terms that frequently get confused. In addition, links and outside resources are provided to deepen the understanding as an option for the reader. The use of these links, coupled with diagrams and examples make this text comprehensive.
The content is mostly accurate. Given that it is a quick guide, the author chose a good selection of which types of research designs to include. However, some are not provided. For example, correlational or cross-correlational research is omitted and is not discussed in Section 3, but is used as a statistical example in the last section.
Examples utilized were appropriate and associated with terms adding value to the learning. The tables that included differentiation between types of statistical tests along with a parametric/nonparametric table were useful and relevant.
The purpose to the text and how to use this guide book is stated clearly and is established up front. The author is also very clear regarding the skill level of the user. Adding to the clarity are the tables with terms, definitions, and examples to help the reader unpack the concepts. The content related to the terms was succinct, direct, and clear. Many times examples or figures were used to supplement the narrative.
The text is consistent throughout from contents to references. Within each section of the text, the introductory paragraph under each section provides a clear understanding regarding what will be discussed in each section. The layout is consistent for each section and easy to follow.
The contents are visible and address each section of the text. A total of seven sections, including a reference section, is in the contents. Each section is outlined by what will be discussed in the contents. In addition, within each section, a heading is provided to direct the reader to the subtopic under each section.
The text is well-organized and segues appropriately. I would have liked to have seen an introductory section giving a narrative overview of what is in each section. This would provide the reader with the ability to get a preliminary glimpse into each upcoming sections and topics that are covered.
The book was easy to navigate and well-organized. Examples are presented in one color, links in another and last, figures and tables. The visuals supplemented the reading and placed appropriately. This provides an opportunity for the reader to unpack the reading by use of visuals and examples.
No significant grammatical errors.
The text is not offensive or culturally insensitive. Examples were inclusive of various races, ethnicities, and backgrounds.
This quick guide is a beneficial text to assist in unpacking the learning related to quantitative statistics. I would use this book to complement my instruction and lessons, or use this book as a main text with supplemental statistical problems and formulas. References to statistical programs were appropriate and were useful. The text did exactly what was stated up front in that it is a direct guide to quantitative statistics. It is well-written and to the point with content areas easy to locate by topic.
Reviewed by Sarah Capello, Assistant Professor, Radford University on 1/18/22
The text claims to provide "quick and simple advice on quantitative aspects of research in social sciences," which it does. There is no index or glossary, although vocabulary words are bolded and defined throughout the text. read more
The text claims to provide "quick and simple advice on quantitative aspects of research in social sciences," which it does. There is no index or glossary, although vocabulary words are bolded and defined throughout the text.
The content is mostly accurate. I would have preferred a few nuances to be hashed out a bit further to avoid potential reader confusion or misunderstanding of the concepts presented.
The content is current; however, some of the references cited in the text are outdated. Newer editions of those texts exist.
The text is very accessible and readable for a variety of audiences. Key terms are well-defined.
There are no content discrepancies within the text. The author even uses similarly shaped graphics for recurring purposes throughout the text (e.g., arrow call outs for further reading, rectangle call outs for examples).
The content is chunked nicely by topics and sections. If it were used for a course, it would be easy to assign different sections of the text for homework, etc. without confusing the reader if the instructor chose to present the content in a different order.
The author follows the structure of the research process. The organization of the text is easy to follow and comprehend.
All of the supplementary images (e.g., tables and figures) were beneficial to the reader and enhanced the text.
There are no significant grammatical errors.
I did not find any culturally offensive or insensitive references in the text.
This text does the difficult job of introducing the complicated concepts and processes of quantitative research in a quick and easy reference guide fairly well. I would not depend solely on this text to teach students about quantitative research, but it could be a good jumping off point for those who have no prior knowledge on this subject or those who need a gentle introduction before diving in to more advanced and complex readings of quantitative research methods.
Reviewed by J. Marlie Henry, Adjunct Faculty, University of Saint Francis on 12/9/21
Considering the length of this guide, this does a good job of addressing major areas that typically need to be addressed. There is a contents section. The guide does seem to be organized accordingly with appropriate alignment and logical flow of... read more
Considering the length of this guide, this does a good job of addressing major areas that typically need to be addressed. There is a contents section. The guide does seem to be organized accordingly with appropriate alignment and logical flow of thought. There is no glossary but, for a guide of this length, a glossary does not seem like it would enhance the guide significantly.
The content is relatively accurate. Expanding the content a bit more or explaining that the methods and designs presented are not entirely inclusive would help. As there are different schools of thought regarding what should/should not be included in terms of these designs and methods, simply bringing attention to that and explaining a bit more would help.
Relevance/Longevity rating: 3
This content needs to be updated. Most of the sources cited are seven or more years old. Even more, it would be helpful to see more currently relevant examples. Some of the source authors such as Andy Field provide very interesting and dynamic instruction in general, but they have much more current information available.
The language used is clear and appropriate. Unnecessary jargon is not used. The intent is clear- to communicate simply in a straightforward manner.
The guide seems to be internally consistent in terms of terminology and framework. There do not seem to be issues in this area. Terminology is internally consistent.
For a guide of this length, the author structured this logically into sections. This guide could be adopted in whole or by section with limited modifications. Courses with fewer than seven modules could also logically group some of the sections.
This guide does present with logical organization. The topics presented are conceptually sequenced in a manner that helps learners build logically on prior conceptualization. This also provides a simple conceptual framework for instructors to guide learners through the process.
Interface rating: 4
The visuals themselves are simple, but they are clear and understandable without distracting the learner. The purpose is clear- that of learning rather than visuals for the sake of visuals. Likewise, navigation is clear and without issues beyond a broken link (the last source noted in the references).
This guide seems to be free of grammatical errors.
It would be interesting to see more cultural integration in a guide of this nature, but the guide is not culturally insensitive or offensive in any way. The language used seems to be consistent with APA's guidelines for unbiased language.
Reviewed by Heng Yu-Ku, Professor, University of Northern Colorado on 5/13/21
The text covers all areas and ideas appropriately and provides practical tables, charts, and examples throughout the text. I would suggest the author also provides a complete research proposal at the end of Section 3 (page 10) and a comprehensive... read more
The text covers all areas and ideas appropriately and provides practical tables, charts, and examples throughout the text. I would suggest the author also provides a complete research proposal at the end of Section 3 (page 10) and a comprehensive research study as an Appendix after section 7 (page 26) to help readers comprehend information better.
For the most part, the content is accurate and unbiased. However, the author only includes four types of research designs used on the social sciences that contain quantitative elements: 1. Mixed method, 2) Case study, 3) Quasi-experiment, and 3) Action research. I wonder why the correlational research is not included as another type of quantitative research design as it has been introduced and emphasized in section 6 by the author.
I believe the content is up-to-date and that necessary updates will be relatively easy and straightforward to implement.
The text is easy to read and provides adequate context for any technical terminology used. However, the author could provide more detailed information about estimating the minimum sample size but not just refer the readers to use the online sample calculators at a different website.
The text is internally consistent in terms of terminology and framework. The author provides the right amount of information with additional information or resources for the readers.
The text includes seven sections. Therefore, it is easier for the instructor to allocate or divide the content into different weeks of instruction within the course.
Yes, the topics in the text are presented in a logical and clear fashion. The author provides clear and precise terminologies, summarizes important content in Table or Figure forms, and offers examples in each section for readers to check their understanding.
The interface of the book is consistent and clear, and all the images and charts provided in the book are appropriate. However, I did encounter some navigation problems as a couple of links are not working or requires permission to access those (pages 10 and 27).
No grammatical errors were found.
No culturally incentive or offensive in its language and the examples provided were found.
As the book title stated, this book provides “A Quick Guide to Quantitative Research in Social Science. It offers easy-to-read information and introduces the readers to the research process, such as research questions, research paradigms, research process, research designs, research methods, data collection, data analysis, and data discussion. However, some links are not working or need permissions to access them (pages 10 and 27).
Reviewed by Hsiao-Chin Kuo, Assistant Professor, Northeastern Illinois University on 4/26/21, updated 4/28/21
As a quick guide, it covers basic concepts related to quantitative research. It starts with WHY quantitative research with regard to asking research questions and considering research paradigms, then provides an overview of research design and... read more
As a quick guide, it covers basic concepts related to quantitative research. It starts with WHY quantitative research with regard to asking research questions and considering research paradigms, then provides an overview of research design and process, discusses methods, data collection and analysis, and ends with writing a research report. It also identifies its target readers/users as those begins to explore quantitative research. It would be helpful to include more examples for readers/users who are new to quantitative research.
Its content is mostly accurate and no bias given its nature as a quick guide. Yet, it is also quite simplified, such as its explanations of mixed methods, case study, quasi-experimental research, and action research. It provides resources for extended reading, yet more recent works will be helpful.
The book is relevant given its nature as a quick guide. It would be helpful to provide more recent works in its resources for extended reading, such as the section for Survey Research (p. 12). It would also be helpful to include more information to introduce common tools and software for statistical analysis.
The book is written with clear and understandable language. Important terms and concepts are presented with plain explanations and examples. Figures and tables are also presented to support its clarity. For example, Table 4 (p. 20) gives an easy-to-follow overview of different statistical tests.
The framework is very consistent with key points, further explanations, examples, and resources for extended reading. The sample studies are presented following the layout of the content, such as research questions, design and methods, and analysis. These examples help reinforce readers' understanding of these common research elements.
The book is divided into seven chapters. Each chapter clearly discusses an aspect of quantitative research. It can be easily divided into modules for a class or for a theme in a research method class. Chapters are short and provides additional resources for extended reading.
The topics in the chapters are presented in a logical and clear structure. It is easy to follow to a degree. Though, it would be also helpful to include the chapter number and title in the header next to its page number.
The text is easy to navigate. Most of the figures and tables are displayed clearly. Yet, there are several sections with empty space that is a bit confusing in the beginning. Again, it can be helpful to include the chapter number/title next to its page number.
Grammatical Errors rating: 4
No major grammatical errors were found.
There are no cultural insensitivities noted.
Given the nature and purpose of this book, as a quick guide, it provides readers a quick reference for important concepts and terms related to quantitative research. Because this book is quite short (27 pages), it can be used as an overview/preview about quantitative research. Teacher's facilitation/input and extended readings will be needed for a deeper learning and discussion about aspects of quantitative research.
Reviewed by Yang Cheng, Assistant Professor, North Carolina State University on 1/6/21
It covers the most important topics such as research progress, resources, measurement, and analysis of the data. read more
It covers the most important topics such as research progress, resources, measurement, and analysis of the data.
The book accurately describes the types of research methods such as mixed-method, quasi-experiment, and case study. It talks about the research proposal and key differences between statistical analyses as well.
The book pinpointed the significance of running a quantitative research method and its relevance to the field of social science.
The book clearly tells us the differences between types of quantitative methods and the steps of running quantitative research for students.
The book is consistent in terms of terminologies such as research methods or types of statistical analysis.
It addresses the headlines and subheadlines very well and each subheading should be necessary for readers.
The book was organized very well to illustrate the topic of quantitative methods in the field of social science.
The pictures within the book could be further developed to describe the key concepts vividly.
The textbook contains no grammatical errors.
It is not culturally offensive in any way.
Overall, this is a simple and quick guide for this important topic. It should be valuable for undergraduate students who would like to learn more about research methods.
Reviewed by Pierre Lu, Associate Professor, University of Texas Rio Grande Valley on 11/20/20
As a quick guide to quantitative research in social sciences, the text covers most ideas and areas. read more
As a quick guide to quantitative research in social sciences, the text covers most ideas and areas.
Mostly accurate content.
As a quick guide, content is highly relevant.
Succinct and clear.
Internally, the text is consistent in terms of terminology used.
The text is easily and readily divisible into smaller sections that can be used as assignments.
I like that there are examples throughout the book.
Easy to read. No interface/ navigation problems.
No grammatical errors detected.
I am not aware of the culturally insensitive description. After all, this is a methodology book.
I think the book has potential to be adopted as a foundation for quantitative research courses, or as a review in the first weeks in advanced quantitative course.
Reviewed by Sarah Fischer, Assistant Professor, Marymount University on 7/31/20
It is meant to be an overview, but it incredibly condensed and spends almost no time on key elements of statistics (such as what makes research generalizable, or what leads to research NOT being generalizable). read more
It is meant to be an overview, but it incredibly condensed and spends almost no time on key elements of statistics (such as what makes research generalizable, or what leads to research NOT being generalizable).
Content Accuracy rating: 1
Contains VERY significant errors, such as saying that one can "accept" a hypothesis. (One of the key aspect of hypothesis testing is that one either rejects or fails to reject a hypothesis, but NEVER accepts a hypothesis.)
Very relevant to those experiencing the research process for the first time. However, it is written by someone working in the natural sciences but is a text for social sciences. This does not explain the errors, but does explain why sometimes the author assumes things about the readers ("hail from more subjectivist territory") that are likely not true.
Clarity rating: 3
Some statistical terminology not explained clearly (or accurately), although the author has made attempts to do both.
Very consistently laid out.
Chapters are very short yet also point readers to outside texts for additional information. Easy to follow.
Generally logically organized.
Easy to navigate, images clear. The additional sources included need to linked to.
Minor grammatical and usage errors throughout the text.
Makes efforts to be inclusive.
The idea of this book is strong--short guides like this are needed. However, this book would likely be strengthened by a revision to reduce inaccuracies and improve the definitions and technical explanations of statistical concepts. Since the book is specifically aimed at the social sciences, it would also improve the text to have more examples that are based in the social sciences (rather than the health sciences or the arts).
Reviewed by Michelle Page, Assistant Professor, Worcester State University on 5/30/20
This text is exactly intended to be what it says: A quick guide. A basic outline of quantitative research processes, akin to cliff notes. The content provides only the essentials of a research process and contains key terms. A student or new... read more
This text is exactly intended to be what it says: A quick guide. A basic outline of quantitative research processes, akin to cliff notes. The content provides only the essentials of a research process and contains key terms. A student or new researcher would not be able to use this as a stand alone guide for quantitative pursuits without having a supplemental text that explains the steps in the process more comprehensively. The introduction does provide this caveat.
Content Accuracy rating: 3
There are no biases or errors that could be distinguished; however, it’s simplicity in content, although accurate for an outline of process, may lack a conveyance of the deeper meanings behind the specific processes explained about qualitative research.
The content is outlined in traditional format to highlight quantitative considerations for formatting research foundational pieces. The resources/references used to point the reader to literature sources can be easily updated with future editions.
The jargon in the text is simple to follow and provides adequate context for its purpose. It is simplified for its intention as a guide which is appropriate.
Each section of the text follows a consistent flow. Explanation of the research content or concept is defined and then a connection to literature is provided to expand the readers understanding of the section’s content. Terminology is consistent with the qualitative process.
As an “outline” and guide, this text can be used to quickly identify the critical parts of the quantitative process. Although each section does not provide deeper content for meaningful use as a stand alone text, it’s utility would be excellent as a reference for a course and can be used as an content guide for specific research courses.
The text’s outline and content are aligned and are in a logical flow in terms of the research considerations for quantitative research.
The only issue that the format was not able to provide was linkable articles. These would have to be cut and pasted into a browser. Functional clickable links in a text are very successful at leading the reader to the supplemental material.
No grammatical errors were noted.
This is a very good outline “guide” to help a new or student researcher to demystify the quantitative process. A successful outline of any process helps to guide work in a logical and systematic way. I think this simple guide is a great adjunct to more substantial research context.
Table of Contents
- Section 1: What will this resource do for you?
- Section 2: Why are you thinking about numbers? A discussion of the research question and paradigms.
- Section 3: An overview of the Research Process and Research Designs
- Section 4: Quantitative Research Methods
- Section 5: the data obtained from quantitative research
- Section 6: Analysis of data
- Section 7: Discussing your Results
Ancillary Material
About the book.
This resource is intended as an easy-to-use guide for anyone who needs some quick and simple advice on quantitative aspects of research in social sciences, covering subjects such as education, sociology, business, nursing. If you area qualitative researcher who needs to venture into the world of numbers, or a student instructed to undertake a quantitative research project despite a hatred for maths, then this booklet should be a real help.
The booklet was amended in 2022 to take into account previous review comments.
About the Contributors
Christine Davies , Ph.D
Contribute to this Page
- Subject List
- Take a Tour
- For Authors
- Subscriber Services
- Publications
- African American Studies
- African Studies
- American Literature
- Anthropology
- Architecture Planning and Preservation
- Art History
- Atlantic History
- Biblical Studies
- British and Irish Literature
- Childhood Studies
- Chinese Studies
- Cinema and Media Studies
- Communication
- Criminology
- Environmental Science
- Evolutionary Biology
- International Law
- International Relations
- Islamic Studies
- Jewish Studies
- Latin American Studies
- Latino Studies
- Linguistics
- Literary and Critical Theory
- Medieval Studies
- Military History
- Political Science
- Public Health
- Renaissance and Reformation
- Social Work
- Urban Studies
- Victorian Literature
- Browse All Subjects
How to Subscribe
- Free Trials
In This Article Expand or collapse the "in this article" section Quantitative Methods in Sociological Research
Introduction, professional associations.
- Data Sources
- Data Archives
- Statistical Software Packages
- Research Design
- Survey Research
- Categorical Data Analysis
- Longitudinal Data Analysis
- Structural Equation Modeling
- Multilevel Modeling
- Causal Inference
- Critical Reflections
- Mixed Methods
- Network Analysis
- Training and Other Resources
Related Articles Expand or collapse the "related articles" section about
About related articles close popup.
Lorem Ipsum Sit Dolor Amet
Vestibulum ante ipsum primis in faucibus orci luctus et ultrices posuere cubilia Curae; Aliquam ligula odio, euismod ut aliquam et, vestibulum nec risus. Nulla viverra, arcu et iaculis consequat, justo diam ornare tellus, semper ultrices tellus nunc eu tellus.
- Agent-Based Modeling
- Cohort Analysis
- Mathematical Sociology
- Multilevel Models
- Panel Studies
- Qualitative Comparative Analysis (QCA)
- Qualitative Methods in Sociological Research
- Social Indicators
- Social Networks
- Survey Methods
Other Subject Areas
Forthcoming articles expand or collapse the "forthcoming articles" section.
- Consumer Credit and Debt
- Economic Globalization
- Global Inequalities
- Find more forthcoming articles...
- Export Citations
- Share This Facebook LinkedIn Twitter
Quantitative Methods in Sociological Research by Erin Leahey LAST REVIEWED: 27 July 2011 LAST MODIFIED: 27 July 2011 DOI: 10.1093/obo/9780199756384-0044
Sociology develops, adopts, and adapts a wide variety of methods for understanding the social world. Realizing that this embarrassment of riches can bewilder the newcomer, this entry is intended to guide scholars through some of the main methods used by quantitative social scientists and some of the key resources for learning such methods. Because many sociologists in the United States receive foundational training in multivariate linear regression, this entry focuses on developments that go beyond this topic, including categorical data analysis, structural equation modeling, multilevel modeling, longitudinal data analysis, causal inference, and even network analysis. The recent wave of interest in mixed methods also merits inclusion. A section on critical reflections aims to encourage researchers to be reflective and thoughtful about the approach(es) they choose.
A number of professional associations are open to quantitative methodologists and researchers, including the two ASAs ( American Sociological Association and American Statistical Association ), the Population Association of American (PAA) , for demographers broadly defined, and the American Association for Public Opinion Research (AAPOR) for survey researchers and methodologists.
American Association of Public Opinion Research (AAPOR) .
Founded in 1947, AAPOR is an association of individuals who share an interest in survey research, qualitative and quantitative research methods, and public opinion data. Members come from academia, media, government, the nonprofit sector, and private industry. Meetings are held in even-numbered years.
American Sociological Association (ASA) .
The national professional association for sociologists, ASA serves as a reference for professional, ethical, and pedagogical topics; sponsors nine journals; and hosts an annual meeting.
American Statistical Association (ASA) .
ASA is the world’s largest community of statisticians and the second-oldest professional society in the United States. For 170 years, ASA has supported excellence in the development and dissemination of statistical science. Its members serve in industry, government, and academia, advancing research and promoting sound statistical practice to inform public policy and improve human welfare.
Population Association of America (PAA) .
PAA is a nonprofit organization that promotes research on population issues such as fertility, migration, health, and mortality. PAA sponsors the journal Demography .
back to top
Users without a subscription are not able to see the full content on this page. Please subscribe or login .
Oxford Bibliographies Online is available by subscription and perpetual access to institutions. For more information or to contact an Oxford Sales Representative click here .
- About Sociology »
- Meet the Editorial Board »
- Actor-Network Theory
- Adolescence
- African Americans
- African Societies
- Analysis, Spatial
- Analysis, World-Systems
- Anomie and Strain Theory
- Arab Spring, Mobilization, and Contentious Politics in the...
- Asian Americans
- Assimilation
- Authority and Work
- Bell, Daniel
- Biosociology
- Bourdieu, Pierre
- Catholicism
- Chicago School of Sociology
- Chinese Cultural Revolution
- Chinese Society
- Citizenship
- Civil Rights
- Civil Society
- Cognitive Sociology
- Collective Efficacy
- Collective Memory
- Comparative Historical Sociology
- Comte, Auguste
- Conflict Theory
- Conservatism
- Consumer Culture
- Consumption
- Contemporary Family Issues
- Contingent Work
- Conversation Analysis
- Corrections
- Cosmopolitanism
- Crime, Cities and
- Cultural Capital
- Cultural Classification and Codes
- Cultural Economy
- Cultural Omnivorousness
- Cultural Production and Circulation
- Culture and Networks
- Culture, Sociology of
- Development
- Discrimination
- Doing Gender
- Du Bois, W.E.B.
- Durkheim, Émile
- Economic Institutions and Institutional Change
- Economic Sociology
- Education and Health
- Education Policy in the United States
- Educational Policy and Race
- Empires and Colonialism
- Entrepreneurship
- Environmental Sociology
- Epistemology
- Ethnic Enclaves
- Ethnomethodology and Conversation Analysis
- Exchange Theory
- Families, Postmodern
- Family Policies
- Feminist Theory
- Field, Bourdieu's Concept of
- Forced Migration
- Foucault, Michel
- Frankfurt School
- Gender and Bodies
- Gender and Crime
- Gender and Education
- Gender and Health
- Gender and Incarceration
- Gender and Professions
- Gender and Social Movements
- Gender and Work
- Gender Pay Gap
- Gender, Sexuality, and Migration
- Gender Stratification
- Gender, Welfare Policy and
- Gendered Sexuality
- Gentrification
- Gerontology
- Globalization and Labor
- Goffman, Erving
- Historic Preservation
- Human Trafficking
- Immigration
- Indian Society, Contemporary
- Institutions
- Intellectuals
- Intersectionalities
- Interview Methodology
- Job Quality
- Knowledge, Critical Sociology of
- Labor Markets
- Latino/Latina Studies
- Law and Society
- Law, Sociology of
- LGBT Parenting and Family Formation
- LGBT Social Movements
- Life Course
- Lipset, S.M.
- Markets, Conventions and Categories in
- Marriage and Divorce
- Marxist Sociology
- Masculinity
- Mass Incarceration in the United States and its Collateral...
- Material Culture
- Medical Sociology
- Mental Illness
- Methodological Individualism
- Middle Classes
- Military Sociology
- Money and Credit
- Multiculturalism
- Multiracial, Mixed-Race, and Biracial Identities
- Nationalism
- Non-normative Sexuality Studies
- Occupations and Professions
- Organizations
- Parsons, Talcott
- Political Culture
- Political Economy
- Political Sociology
- Popular Culture
- Proletariat (Working Class)
- Protestantism
- Public Opinion
- Public Space
- Race and Sexuality
- Race and Violence
- Race and Youth
- Race in Global Perspective
- Race, Organizations, and Movements
- Rational Choice
- Relationships
- Religion and the Public Sphere
- Residential Segregation
- Revolutions
- Role Theory
- Rural Sociology
- Scientific Networks
- Secularization
- Sequence Analysis
- Sex versus Gender
- Sexual Identity
- Sexualities
- Sexuality Across the Life Course
- Simmel, Georg
- Single Parents in Context
- Small Cities
- Social Capital
- Social Change
- Social Closure
- Social Construction of Crime
- Social Control
- Social Darwinism
- Social Disorganization Theory
- Social Epidemiology
- Social History
- Social Mobility
- Social Movements
- Social Network Analysis
- Social Policy
- Social Problems
- Social Psychology
- Social Stratification
- Social Theory
- Socialization, Sociological Perspectives on
- Sociolinguistics
- Sociological Approaches to Character
- Sociological Research on the Chinese Society
- Sociological Research, Qualitative Methods in
- Sociological Research, Quantitative Methods in
- Sociology, History of
- Sociology of Manners
- Sociology of Music
- Sociology of War, The
- Suburbanism
- Symbolic Boundaries
- Symbolic Interactionism
- The Division of Labor after Durkheim
- Tilly, Charles
- Time Use and Childcare
- Time Use and Time Diary Research
- Tourism, Sociology of
- Transnational Adoption
- Unions and Inequality
- Urban Ethnography
- Urban Growth Machine
- Urban Inequality in the United States
- Veblen, Thorstein
- Visual Arts, Music, and Aesthetic Experience
- Wallerstein, Immanuel
- Welfare, Race, and the American Imagination
- Welfare States
- Women’s Employment and Economic Inequality Between Househo...
- Work and Employment, Sociology of
- Work/Life Balance
- Workplace Flexibility
- Privacy Policy
- Cookie Policy
- Legal Notice
- Accessibility
Powered by:
- [66.249.64.20|81.177.182.159]
- 81.177.182.159
- Utility Menu

Alexandra Killewald
Professor of sociology.

Soc 156: Quantitative Methods in Sociology
Semester: , offered: , related materials.
2.2 Research Methods
Learning objectives.
By the end of this section, you should be able to:
- Recall the 6 Steps of the Scientific Method
- Differentiate between four kinds of research methods: surveys, field research, experiments, and secondary data analysis.
- Explain the appropriateness of specific research approaches for specific topics.
Sociologists examine the social world, see a problem or interesting pattern, and set out to study it. They use research methods to design a study. Planning the research design is a key step in any sociological study. Sociologists generally choose from widely used methods of social investigation: primary source data collection such as survey, participant observation, ethnography, case study, unobtrusive observations, experiment, and secondary data analysis , or use of existing sources. Every research method comes with plusses and minuses, and the topic of study strongly influences which method or methods are put to use. When you are conducting research think about the best way to gather or obtain knowledge about your topic, think of yourself as an architect. An architect needs a blueprint to build a house, as a sociologist your blueprint is your research design including your data collection method.
When entering a particular social environment, a researcher must be careful. There are times to remain anonymous and times to be overt. There are times to conduct interviews and times to simply observe. Some participants need to be thoroughly informed; others should not know they are being observed. A researcher wouldn’t stroll into a crime-ridden neighborhood at midnight, calling out, “Any gang members around?”
Making sociologists’ presence invisible is not always realistic for other reasons. That option is not available to a researcher studying prison behaviors, early education, or the Ku Klux Klan. Researchers can’t just stroll into prisons, kindergarten classrooms, or Klan meetings and unobtrusively observe behaviors or attract attention. In situations like these, other methods are needed. Researchers choose methods that best suit their study topics, protect research participants or subjects, and that fit with their overall approaches to research.
As a research method, a survey collects data from subjects who respond to a series of questions about behaviors and opinions, often in the form of a questionnaire or an interview. The survey is one of the most widely used scientific research methods. The standard survey format allows individuals a level of anonymity in which they can express personal ideas.
At some point, most people in the United States respond to some type of survey. The 2020 U.S. Census is an excellent example of a large-scale survey intended to gather sociological data. Since 1790, United States has conducted a survey consisting of six questions to received demographical data pertaining to residents. The questions pertain to the demographics of the residents who live in the United States. Currently, the Census is received by residents in the United Stated and five territories and consists of 12 questions.
Not all surveys are considered sociological research, however, and many surveys people commonly encounter focus on identifying marketing needs and strategies rather than testing a hypothesis or contributing to social science knowledge. Questions such as, “How many hot dogs do you eat in a month?” or “Were the staff helpful?” are not usually designed as scientific research. The Nielsen Ratings determine the popularity of television programming through scientific market research. However, polls conducted by television programs such as American Idol or So You Think You Can Dance cannot be generalized, because they are administered to an unrepresentative population, a specific show’s audience. You might receive polls through your cell phones or emails, from grocery stores, restaurants, and retail stores. They often provide you incentives for completing the survey.
Sociologists conduct surveys under controlled conditions for specific purposes. Surveys gather different types of information from people. While surveys are not great at capturing the ways people really behave in social situations, they are a great method for discovering how people feel, think, and act—or at least how they say they feel, think, and act. Surveys can track preferences for presidential candidates or reported individual behaviors (such as sleeping, driving, or texting habits) or information such as employment status, income, and education levels.
A survey targets a specific population , people who are the focus of a study, such as college athletes, international students, or teenagers living with type 1 (juvenile-onset) diabetes. Most researchers choose to survey a small sector of the population, or a sample , a manageable number of subjects who represent a larger population. The success of a study depends on how well a population is represented by the sample. In a random sample , every person in a population has the same chance of being chosen for the study. As a result, a Gallup Poll, if conducted as a nationwide random sampling, should be able to provide an accurate estimate of public opinion whether it contacts 2,000 or 10,000 people.
After selecting subjects, the researcher develops a specific plan to ask questions and record responses. It is important to inform subjects of the nature and purpose of the survey up front. If they agree to participate, researchers thank subjects and offer them a chance to see the results of the study if they are interested. The researcher presents the subjects with an instrument, which is a means of gathering the information.
A common instrument is a questionnaire. Subjects often answer a series of closed-ended questions . The researcher might ask yes-or-no or multiple-choice questions, allowing subjects to choose possible responses to each question. This kind of questionnaire collects quantitative data —data in numerical form that can be counted and statistically analyzed. Just count up the number of “yes” and “no” responses or correct answers, and chart them into percentages.
Questionnaires can also ask more complex questions with more complex answers—beyond “yes,” “no,” or checkbox options. These types of inquiries use open-ended questions that require short essay responses. Participants willing to take the time to write those answers might convey personal religious beliefs, political views, goals, or morals. The answers are subjective and vary from person to person. How do you plan to use your college education?
Some topics that investigate internal thought processes are impossible to observe directly and are difficult to discuss honestly in a public forum. People are more likely to share honest answers if they can respond to questions anonymously. This type of personal explanation is qualitative data —conveyed through words. Qualitative information is harder to organize and tabulate. The researcher will end up with a wide range of responses, some of which may be surprising. The benefit of written opinions, though, is the wealth of in-depth material that they provide.
An interview is a one-on-one conversation between the researcher and the subject, and it is a way of conducting surveys on a topic. However, participants are free to respond as they wish, without being limited by predetermined choices. In the back-and-forth conversation of an interview, a researcher can ask for clarification, spend more time on a subtopic, or ask additional questions. In an interview, a subject will ideally feel free to open up and answer questions that are often complex. There are no right or wrong answers. The subject might not even know how to answer the questions honestly.
Questions such as “How does society’s view of alcohol consumption influence your decision whether or not to take your first sip of alcohol?” or “Did you feel that the divorce of your parents would put a social stigma on your family?” involve so many factors that the answers are difficult to categorize. A researcher needs to avoid steering or prompting the subject to respond in a specific way; otherwise, the results will prove to be unreliable. The researcher will also benefit from gaining a subject’s trust, from empathizing or commiserating with a subject, and from listening without judgment.
Surveys often collect both quantitative and qualitative data. For example, a researcher interviewing people who are incarcerated might receive quantitative data, such as demographics – race, age, sex, that can be analyzed statistically. For example, the researcher might discover that 20 percent of incarcerated people are above the age of 50. The researcher might also collect qualitative data, such as why people take advantage of educational opportunities during their sentence and other explanatory information.
The survey can be carried out online, over the phone, by mail, or face-to-face. When researchers collect data outside a laboratory, library, or workplace setting, they are conducting field research, which is our next topic.
Field Research
The work of sociology rarely happens in limited, confined spaces. Rather, sociologists go out into the world. They meet subjects where they live, work, and play. Field research refers to gathering primary data from a natural environment. To conduct field research, the sociologist must be willing to step into new environments and observe, participate, or experience those worlds. In field work, the sociologists, rather than the subjects, are the ones out of their element.
The researcher interacts with or observes people and gathers data along the way. The key point in field research is that it takes place in the subject’s natural environment, whether it’s a coffee shop or tribal village, a homeless shelter or the DMV, a hospital, airport, mall, or beach resort.
While field research often begins in a specific setting , the study’s purpose is to observe specific behaviors in that setting. Field work is optimal for observing how people think and behave. It seeks to understand why they behave that way. However, researchers may struggle to narrow down cause and effect when there are so many variables floating around in a natural environment. And while field research looks for correlation, its small sample size does not allow for establishing a causal relationship between two variables. Indeed, much of the data gathered in sociology do not identify a cause and effect but a correlation .
Sociology in the Real World
Beyoncé and lady gaga as sociological subjects.
Sociologists have studied Lady Gaga and Beyoncé and their impact on music, movies, social media, fan participation, and social equality. In their studies, researchers have used several research methods including secondary analysis, participant observation, and surveys from concert participants.
In their study, Click, Lee & Holiday (2013) interviewed 45 Lady Gaga fans who utilized social media to communicate with the artist. These fans viewed Lady Gaga as a mirror of themselves and a source of inspiration. Like her, they embrace not being a part of mainstream culture. Many of Lady Gaga’s fans are members of the LGBTQ community. They see the “song “Born This Way” as a rallying cry and answer her calls for “Paws Up” with a physical expression of solidarity—outstretched arms and fingers bent and curled to resemble monster claws.”
Sascha Buchanan (2019) made use of participant observation to study the relationship between two fan groups, that of Beyoncé and that of Rihanna. She observed award shows sponsored by iHeartRadio, MTV EMA, and BET that pit one group against another as they competed for Best Fan Army, Biggest Fans, and FANdemonium. Buchanan argues that the media thus sustains a myth of rivalry between the two most commercially successful Black women vocal artists.
Participant Observation
In 2000, a comic writer named Rodney Rothman wanted an insider’s view of white-collar work. He slipped into the sterile, high-rise offices of a New York “dot com” agency. Every day for two weeks, he pretended to work there. His main purpose was simply to see whether anyone would notice him or challenge his presence. No one did. The receptionist greeted him. The employees smiled and said good morning. Rothman was accepted as part of the team. He even went so far as to claim a desk, inform the receptionist of his whereabouts, and attend a meeting. He published an article about his experience in The New Yorker called “My Fake Job” (2000). Later, he was discredited for allegedly fabricating some details of the story and The New Yorker issued an apology. However, Rothman’s entertaining article still offered fascinating descriptions of the inside workings of a “dot com” company and exemplified the lengths to which a writer, or a sociologist, will go to uncover material.
Rothman had conducted a form of study called participant observation , in which researchers join people and participate in a group’s routine activities for the purpose of observing them within that context. This method lets researchers experience a specific aspect of social life. A researcher might go to great lengths to get a firsthand look into a trend, institution, or behavior. A researcher might work as a waitress in a diner, experience homelessness for several weeks, or ride along with police officers as they patrol their regular beat. Often, these researchers try to blend in seamlessly with the population they study, and they may not disclose their true identity or purpose if they feel it would compromise the results of their research.
At the beginning of a field study, researchers might have a question: “What really goes on in the kitchen of the most popular diner on campus?” or “What is it like to be homeless?” Participant observation is a useful method if the researcher wants to explore a certain environment from the inside.
Field researchers simply want to observe and learn. In such a setting, the researcher will be alert and open minded to whatever happens, recording all observations accurately. Soon, as patterns emerge, questions will become more specific, observations will lead to hypotheses, and hypotheses will guide the researcher in analyzing data and generating results.
In a study of small towns in the United States conducted by sociological researchers John S. Lynd and Helen Merrell Lynd, the team altered their purpose as they gathered data. They initially planned to focus their study on the role of religion in U.S. towns. As they gathered observations, they realized that the effect of industrialization and urbanization was the more relevant topic of this social group. The Lynds did not change their methods, but they revised the purpose of their study.
This shaped the structure of Middletown: A Study in Modern American Culture , their published results (Lynd & Lynd, 1929).
The Lynds were upfront about their mission. The townspeople of Muncie, Indiana, knew why the researchers were in their midst. But some sociologists prefer not to alert people to their presence. The main advantage of covert participant observation is that it allows the researcher access to authentic, natural behaviors of a group’s members. The challenge, however, is gaining access to a setting without disrupting the pattern of others’ behavior. Becoming an inside member of a group, organization, or subculture takes time and effort. Researchers must pretend to be something they are not. The process could involve role playing, making contacts, networking, or applying for a job.
Once inside a group, some researchers spend months or even years pretending to be one of the people they are observing. However, as observers, they cannot get too involved. They must keep their purpose in mind and apply the sociological perspective. That way, they illuminate social patterns that are often unrecognized. Because information gathered during participant observation is mostly qualitative, rather than quantitative, the end results are often descriptive or interpretive. The researcher might present findings in an article or book and describe what he or she witnessed and experienced.
This type of research is what journalist Barbara Ehrenreich conducted for her book Nickel and Dimed . One day over lunch with her editor, Ehrenreich mentioned an idea. How can people exist on minimum-wage work? How do low-income workers get by? she wondered. Someone should do a study . To her surprise, her editor responded, Why don’t you do it?
That’s how Ehrenreich found herself joining the ranks of the working class. For several months, she left her comfortable home and lived and worked among people who lacked, for the most part, higher education and marketable job skills. Undercover, she applied for and worked minimum wage jobs as a waitress, a cleaning woman, a nursing home aide, and a retail chain employee. During her participant observation, she used only her income from those jobs to pay for food, clothing, transportation, and shelter.
She discovered the obvious, that it’s almost impossible to get by on minimum wage work. She also experienced and observed attitudes many middle and upper-class people never think about. She witnessed firsthand the treatment of working class employees. She saw the extreme measures people take to make ends meet and to survive. She described fellow employees who held two or three jobs, worked seven days a week, lived in cars, could not pay to treat chronic health conditions, got randomly fired, submitted to drug tests, and moved in and out of homeless shelters. She brought aspects of that life to light, describing difficult working conditions and the poor treatment that low-wage workers suffer.
The book she wrote upon her return to her real life as a well-paid writer, has been widely read and used in many college classrooms.
Ethnography
Ethnography is the immersion of the researcher in the natural setting of an entire social community to observe and experience their everyday life and culture. The heart of an ethnographic study focuses on how subjects view their own social standing and how they understand themselves in relation to a social group.
An ethnographic study might observe, for example, a small U.S. fishing town, an Inuit community, a village in Thailand, a Buddhist monastery, a private boarding school, or an amusement park. These places all have borders. People live, work, study, or vacation within those borders. People are there for a certain reason and therefore behave in certain ways and respect certain cultural norms. An ethnographer would commit to spending a determined amount of time studying every aspect of the chosen place, taking in as much as possible.
A sociologist studying a tribe in the Amazon might watch the way villagers go about their daily lives and then write a paper about it. To observe a spiritual retreat center, an ethnographer might sign up for a retreat and attend as a guest for an extended stay, observe and record data, and collate the material into results.
Institutional Ethnography
Institutional ethnography is an extension of basic ethnographic research principles that focuses intentionally on everyday concrete social relationships. Developed by Canadian sociologist Dorothy E. Smith (1990), institutional ethnography is often considered a feminist-inspired approach to social analysis and primarily considers women’s experiences within male- dominated societies and power structures. Smith’s work is seen to challenge sociology’s exclusion of women, both academically and in the study of women’s lives (Fenstermaker, n.d.).
Historically, social science research tended to objectify women and ignore their experiences except as viewed from the male perspective. Modern feminists note that describing women, and other marginalized groups, as subordinates helps those in authority maintain their own dominant positions (Social Sciences and Humanities Research Council of Canada n.d.). Smith’s three major works explored what she called “the conceptual practices of power” and are still considered seminal works in feminist theory and ethnography (Fensternmaker n.d.).
Sociological Research
The making of middletown: a study in modern u.s. culture.
In 1924, a young married couple named Robert and Helen Lynd undertook an unprecedented ethnography: to apply sociological methods to the study of one U.S. city in order to discover what “ordinary” people in the United States did and believed. Choosing Muncie, Indiana (population about 30,000) as their subject, they moved to the small town and lived there for eighteen months.
Ethnographers had been examining other cultures for decades—groups considered minorities or outsiders—like gangs, immigrants, and the poor. But no one had studied the so-called average American.
Recording interviews and using surveys to gather data, the Lynds objectively described what they observed. Researching existing sources, they compared Muncie in 1890 to the Muncie they observed in 1924. Most Muncie adults, they found, had grown up on farms but now lived in homes inside the city. As a result, the Lynds focused their study on the impact of industrialization and urbanization.
They observed that Muncie was divided into business and working class groups. They defined business class as dealing with abstract concepts and symbols, while working class people used tools to create concrete objects. The two classes led different lives with different goals and hopes. However, the Lynds observed, mass production offered both classes the same amenities. Like wealthy families, the working class was now able to own radios, cars, washing machines, telephones, vacuum cleaners, and refrigerators. This was an emerging material reality of the 1920s.
As the Lynds worked, they divided their manuscript into six chapters: Getting a Living, Making a Home, Training the Young, Using Leisure, Engaging in Religious Practices, and Engaging in Community Activities.
When the study was completed, the Lynds encountered a big problem. The Rockefeller Foundation, which had commissioned the book, claimed it was useless and refused to publish it. The Lynds asked if they could seek a publisher themselves.
Middletown: A Study in Modern American Culture was not only published in 1929 but also became an instant bestseller, a status unheard of for a sociological study. The book sold out six printings in its first year of publication, and has never gone out of print (Caplow, Hicks, & Wattenberg. 2000).
Nothing like it had ever been done before. Middletown was reviewed on the front page of the New York Times. Readers in the 1920s and 1930s identified with the citizens of Muncie, Indiana, but they were equally fascinated by the sociological methods and the use of scientific data to define ordinary people in the United States. The book was proof that social data was important—and interesting—to the U.S. public.
Sometimes a researcher wants to study one specific person or event. A case study is an in-depth analysis of a single event, situation, or individual. To conduct a case study, a researcher examines existing sources like documents and archival records, conducts interviews, engages in direct observation and even participant observation, if possible.
Researchers might use this method to study a single case of a foster child, drug lord, cancer patient, criminal, or rape victim. However, a major criticism of the case study as a method is that while offering depth on a topic, it does not provide enough evidence to form a generalized conclusion. In other words, it is difficult to make universal claims based on just one person, since one person does not verify a pattern. This is why most sociologists do not use case studies as a primary research method.
However, case studies are useful when the single case is unique. In these instances, a single case study can contribute tremendous insight. For example, a feral child, also called “wild child,” is one who grows up isolated from human beings. Feral children grow up without social contact and language, which are elements crucial to a “civilized” child’s development. These children mimic the behaviors and movements of animals, and often invent their own language. There are only about one hundred cases of “feral children” in the world.
As you may imagine, a feral child is a subject of great interest to researchers. Feral children provide unique information about child development because they have grown up outside of the parameters of “normal” growth and nurturing. And since there are very few feral children, the case study is the most appropriate method for researchers to use in studying the subject.
At age three, a Ukranian girl named Oxana Malaya suffered severe parental neglect. She lived in a shed with dogs, and she ate raw meat and scraps. Five years later, a neighbor called authorities and reported seeing a girl who ran on all fours, barking. Officials brought Oxana into society, where she was cared for and taught some human behaviors, but she never became fully socialized. She has been designated as unable to support herself and now lives in a mental institution (Grice 2011). Case studies like this offer a way for sociologists to collect data that may not be obtained by any other method.
Experiments
You have probably tested some of your own personal social theories. “If I study at night and review in the morning, I’ll improve my retention skills.” Or, “If I stop drinking soda, I’ll feel better.” Cause and effect. If this, then that. When you test the theory, your results either prove or disprove your hypothesis.
One way researchers test social theories is by conducting an experiment , meaning they investigate relationships to test a hypothesis—a scientific approach.
There are two main types of experiments: lab-based experiments and natural or field experiments. In a lab setting, the research can be controlled so that more data can be recorded in a limited amount of time. In a natural or field- based experiment, the time it takes to gather the data cannot be controlled but the information might be considered more accurate since it was collected without interference or intervention by the researcher.
As a research method, either type of sociological experiment is useful for testing if-then statements: if a particular thing happens (cause), then another particular thing will result (effect). To set up a lab-based experiment, sociologists create artificial situations that allow them to manipulate variables.
Classically, the sociologist selects a set of people with similar characteristics, such as age, class, race, or education. Those people are divided into two groups. One is the experimental group and the other is the control group. The experimental group is exposed to the independent variable(s) and the control group is not. To test the benefits of tutoring, for example, the sociologist might provide tutoring to the experimental group of students but not to the control group. Then both groups would be tested for differences in performance to see if tutoring had an effect on the experimental group of students. As you can imagine, in a case like this, the researcher would not want to jeopardize the accomplishments of either group of students, so the setting would be somewhat artificial. The test would not be for a grade reflected on their permanent record of a student, for example.
And if a researcher told the students they would be observed as part of a study on measuring the effectiveness of tutoring, the students might not behave naturally. This is called the Hawthorne effect —which occurs when people change their behavior because they know they are being watched as part of a study. The Hawthorne effect is unavoidable in some research studies because sociologists have to make the purpose of the study known. Subjects must be aware that they are being observed, and a certain amount of artificiality may result (Sonnenfeld 1985).
A real-life example will help illustrate the process. In 1971, Frances Heussenstamm, a sociology professor at California State University at Los Angeles, had a theory about police prejudice. To test her theory, she conducted research. She chose fifteen students from three ethnic backgrounds: Black, White, and Hispanic. She chose students who routinely drove to and from campus along Los Angeles freeway routes, and who had had perfect driving records for longer than a year.
Next, she placed a Black Panther bumper sticker on each car. That sticker, a representation of a social value, was the independent variable. In the 1970s, the Black Panthers were a revolutionary group actively fighting racism. Heussenstamm asked the students to follow their normal driving patterns. She wanted to see whether seeming support for the Black Panthers would change how these good drivers were treated by the police patrolling the highways. The dependent variable would be the number of traffic stops/citations.
The first arrest, for an incorrect lane change, was made two hours after the experiment began. One participant was pulled over three times in three days. He quit the study. After seventeen days, the fifteen drivers had collected a total of thirty-three traffic citations. The research was halted. The funding to pay traffic fines had run out, and so had the enthusiasm of the participants (Heussenstamm, 1971).
Secondary Data Analysis
While sociologists often engage in original research studies, they also contribute knowledge to the discipline through secondary data analysis . Secondary data does not result from firsthand research collected from primary sources, but are the already completed work of other researchers or data collected by an agency or organization. Sociologists might study works written by historians, economists, teachers, or early sociologists. They might search through periodicals, newspapers, or magazines, or organizational data from any period in history.
Using available information not only saves time and money but can also add depth to a study. Sociologists often interpret findings in a new way, a way that was not part of an author’s original purpose or intention. To study how women were encouraged to act and behave in the 1960s, for example, a researcher might watch movies, televisions shows, and situation comedies from that period. Or to research changes in behavior and attitudes due to the emergence of television in the late 1950s and early 1960s, a sociologist would rely on new interpretations of secondary data. Decades from now, researchers will most likely conduct similar studies on the advent of mobile phones, the Internet, or social media.
Social scientists also learn by analyzing the research of a variety of agencies. Governmental departments and global groups, like the U.S. Bureau of Labor Statistics or the World Health Organization (WHO), publish studies with findings that are useful to sociologists. A public statistic like the foreclosure rate might be useful for studying the effects of a recession. A racial demographic profile might be compared with data on education funding to examine the resources accessible by different groups.
One of the advantages of secondary data like old movies or WHO statistics is that it is nonreactive research (or unobtrusive research), meaning that it does not involve direct contact with subjects and will not alter or influence people’s behaviors. Unlike studies requiring direct contact with people, using previously published data does not require entering a population and the investment and risks inherent in that research process.
Using available data does have its challenges. Public records are not always easy to access. A researcher will need to do some legwork to track them down and gain access to records. To guide the search through a vast library of materials and avoid wasting time reading unrelated sources, sociologists employ content analysis , applying a systematic approach to record and value information gleaned from secondary data as they relate to the study at hand.
Also, in some cases, there is no way to verify the accuracy of existing data. It is easy to count how many drunk drivers, for example, are pulled over by the police. But how many are not? While it’s possible to discover the percentage of teenage students who drop out of high school, it might be more challenging to determine the number who return to school or get their GED later.
Another problem arises when data are unavailable in the exact form needed or do not survey the topic from the precise angle the researcher seeks. For example, the average salaries paid to professors at a public school is public record. But these figures do not necessarily reveal how long it took each professor to reach the salary range, what their educational backgrounds are, or how long they’ve been teaching.
When conducting content analysis, it is important to consider the date of publication of an existing source and to take into account attitudes and common cultural ideals that may have influenced the research. For example, when Robert S. Lynd and Helen Merrell Lynd gathered research in the 1920s, attitudes and cultural norms were vastly different then than they are now. Beliefs about gender roles, race, education, and work have changed significantly since then. At the time, the study’s purpose was to reveal insights about small U.S. communities. Today, it is an illustration of 1920s attitudes and values.
As an Amazon Associate we earn from qualifying purchases.
This book may not be used in the training of large language models or otherwise be ingested into large language models or generative AI offerings without OpenStax's permission.
Want to cite, share, or modify this book? This book uses the Creative Commons Attribution License and you must attribute OpenStax.
Access for free at https://openstax.org/books/introduction-sociology-3e/pages/1-introduction
- Authors: Tonja R. Conerly, Kathleen Holmes, Asha Lal Tamang
- Publisher/website: OpenStax
- Book title: Introduction to Sociology 3e
- Publication date: Jun 3, 2021
- Location: Houston, Texas
- Book URL: https://openstax.org/books/introduction-sociology-3e/pages/1-introduction
- Section URL: https://openstax.org/books/introduction-sociology-3e/pages/2-2-research-methods
© Jan 18, 2024 OpenStax. Textbook content produced by OpenStax is licensed under a Creative Commons Attribution License . The OpenStax name, OpenStax logo, OpenStax book covers, OpenStax CNX name, and OpenStax CNX logo are not subject to the Creative Commons license and may not be reproduced without the prior and express written consent of Rice University.
ReviseSociology
A level sociology revision – education, families, research methods, crime and deviance and more!
Scientific Quantitative Methodology in Sociology
Table of Contents
Positivists prefer to the limit themselves the study of objective ‘social facts’ and use statistical data and the comparative method to find correlations, and multivariate analysis to uncover statistically significant ‘causal’ relationships between variables and thus derive the laws of human behaviour.
This post explores the Positivist approach to social research, defining and explaining all of the above key terms and using some examples from sociology to illustrate them.
Social Facts
The first rule of Positivist methodology is to consider social facts as things which means that the belief systems and customs of the social world should be considered as things in the same way as the objects and events of the natural world.
According to Durkheim, some of the key features of social facts are:
- they exist over and above individual consciousness
- they are not chosen by individuals and cannot be changed by will
- each person is limited (constrained) by social facts
According to Durkheim what effects do social facts make people act in certain ways, in the same way as door limits the means whereby you can enter a room or gravity limits how far you can jump.
Positivists believed that we should only study what can be observed and measured(objective facts), not subjective thoughts and feelings. The role of human consciousness is irrelevant to explaining human behaviour according to Positivists because humans have little or no choice over how they behave.
For a more in-depth account of social facts, have a look at this blog post: What are Social Facts?
Statistical data, Correlation, and Causation
Positivists believed it was possible to classify the social world in an objective way. Using these classifications it was then possible to count sets of observable facts and so produce statistics.
The point of identifying social facts was to look for correlations – a correlation is a tendency for two or more things to be found together, and it may refer to the strength of the relationship between them.
If there is a strong correlation between two ore more types of social phenomena then a positivist sociologist might suspect that one of these phenomena is causing the other to take place. However, this is not necessarily the case and it is important to analyse the data before any conclusion is reach.
Spurious Correlations
Spurious correlations pose a problem for Positivist research. A spurious correlation is when two or more phenomena are found together but have no direct connection to each other: one does not therefor cause the other. For example although more working class people commit crime, this may be because more men are found in the working classes – so the significant relationship might be between gender and crime, not between class and crime.
Multivariate Analysis
Positivists engage in multivariate analysis to overcome the problem of spurious correlations.
Multivariate Analysis involves isolating the effect of a particular independent variable upon a particular dependent variable. This can be done by holding one independent variable constant and changing the other. In the example above this might mean comparing the crime rates of men and women in the working class.
Positivists believe multivariate analysis can establish causal connections between two or more variables and once analysis is checked establish the laws of human behaviour.
Positivism – Establishing the Laws of Human Behaviour
A scientific law is a statement about the relationship between two or more phenomena which is true in all circumstances.
According to Positivists, the laws of human behaviour can be discovered by the collection of objective facts about the world in statistical form and uncovering correlations between them, checked for their significance by multivariate analysis.
Positivism and The Comparative Method
The comparative method involves the use of comparisons between different societies, or different points in time
The purpose of using the comparative method is to establish correlations, and ultimately causal connections, seek laws and test hypotheses.
The comparative method overcomes the following disadvantages of experiments:
- Moral problems are not as acute
- The research is less likely to affect the behaviour or those being studied because we are looking at natural settings
- The comparative method is superior to the experimental method because allows the sociologist to explore large scale social changes and changes over time
However, a fundamental problem with the comparative method is that the data you want may not be available, and you are limited to that data which already exists or which can be collected on a large scale via social surveys.
Related Posts
Positivism and Interpretivism in social research
Social Action Theory – criticises the positivist approach to social research, arguing that human consciousness is too complex to reduce to numbers.
Share this:
- Share on Tumblr
Leave a Reply Cancel reply
This site uses Akismet to reduce spam. Learn how your comment data is processed .
Discover more from ReviseSociology
Subscribe now to keep reading and get access to the full archive.
Continue reading
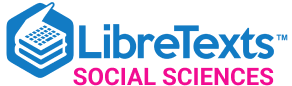
- school Campus Bookshelves
- menu_book Bookshelves
- perm_media Learning Objects
- login Login
- how_to_reg Request Instructor Account
- hub Instructor Commons
- Download Page (PDF)
- Download Full Book (PDF)
- Periodic Table
- Physics Constants
- Scientific Calculator
- Reference & Cite
- Tools expand_more
- Readability
selected template will load here
This action is not available.
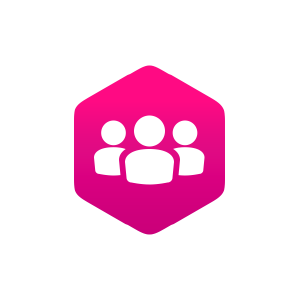
7.3: Sampling in Quantitative Research
- Last updated
- Save as PDF
- Page ID 12582

Learning Objectives
- Describe how probability sampling differs from nonprobability sampling.
- Define generalizability, and describe how it is achieved in probability samples.
- Identify the various types of probability samples, and provide a brief description of each.
Quantitative researchers are often interested in being able to make generalizations about groups larger than their study samples. While there are certainly instances when quantitative researchers rely on nonprobability samples (e.g., when doing exploratory or evaluation research), quantitative researchers tend to rely on probability sampling techniques. The goals and techniques associated with probability samples differ from those of nonprobability samples. We’ll explore those unique goals and techniques in this section.
Probability Sampling
Unlike nonprobability sampling, probability sampling refers to sampling techniques for which a person’s (or event’s) likelihood of being selected for membership in the sample is known. You might ask yourself why we should care about a study element’s likelihood of being selected for membership in a researcher’s sample. The reason is that, in most cases, researchers who use probability sampling techniques are aiming to identify a representative sample from which to collect data. A representative sample is one that resembles the population from which it was drawn in all the ways that are important for the research being conducted. If, for example, you wish to be able to say something about differences between men and women at the end of your study, you better make sure that your sample doesn’t contain only women. That’s a bit of an oversimplification, but the point with representativeness is that if your population varies in some way that is important to your study, your sample should contain the same sorts of variation.
Obtaining a representative sample is important in probability sampling because a key goal of studies that rely on probability samples is generalizability . In fact, generalizability is perhaps the key feature that distinguishes probability samples from nonprobability samples. Generalizability refers to the idea that a study’s results will tell us something about a group larger than the sample from which the findings were generated. In order to achieve generalizability, a core principle of probability sampling is that all elements in the researcher’s target population have an equal chance of being selected for inclusion in the study. In research, this is the principle of random selection . Random selection is a mathematical process that we won’t go into too much depth about here, but if you have taken or plan to take a statistics course, you’ll learn more about it there. The important thing to remember about random selection here is that, as previously noted, it is a core principal of probability sampling. If a researcher uses random selection techniques to draw a sample, he or she will be able to estimate how closely the sample represents the larger population from which it was drawn by estimating the sampling error. Sampling error is a statistical calculation of the difference between results from a sample and the actual parameters of a population.
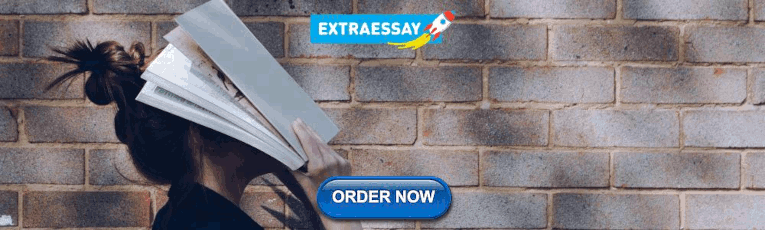
Types of Probability Samples
There are a variety of probability samples that researchers may use. These include simple random samples, systematic samples, stratified samples, and cluster samples.
Simple random samples are the most basic type of probability sample, but their use is not particularly common. Part of the reason for this may be the work involved in generating a simple random sample. To draw a simple random sample, a researcher starts with a list of every single member, or element, of his or her population of interest. This list is sometimes referred to as a sampling frame . Once that list has been created, the researcher numbers each element sequentially and then randomly selects the elements from which he or she will collect data. To randomly select elements, researchers use a table of numbers that have been generated randomly. There are several possible sources for obtaining a random number table. Some statistics and research methods textbooks offer such tables as appendices to the text. Perhaps a more accessible source is one of the many free random number generators available on the Internet. A good online source is the website Stat Trek, which contains a random number generator that you can use to create a random number table of whatever size you might need ( stattrek.com/Tables/Random.aspx ). Randomizer.org also offers a useful random number generator ( http://randomizer.org ).
As you might have guessed, drawing a simple random sample can be quite tedious. Systematic sampling techniques are somewhat less tedious but offer the benefits of a random sample. As with simple random samples, you must be able to produce a list of every one of your population elements. Once you’ve done that, to draw a systematic sample you’d simply select every k th element on your list. But what is k , and where on the list of population elements does one begin the selection process? k is your selection interval or the distance between the elements you select for inclusion in your study. To begin the selection process, you’ll need to figure out how many elements you wish to include in your sample. Let’s say you want to interview 25 fraternity members on your campus, and there are 100 men on campus who are members of fraternities. In this case, your selection interval, or k , is 4. To arrive at 4, simply divide the total number of population elements by your desired sample size. This process is represented in Figure 7.5.
Figure 7.5 Formula for Determining Selection Interval for Systematic Sample
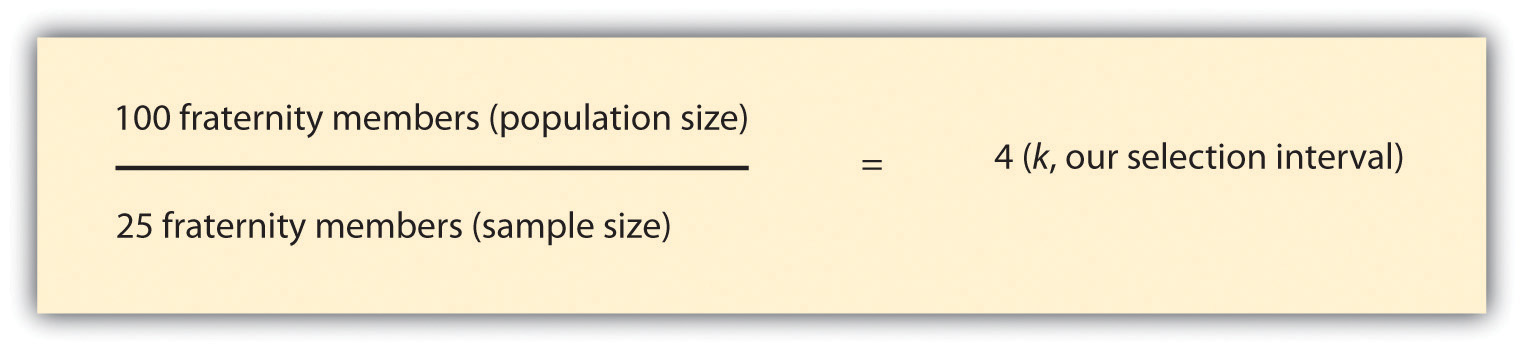
To determine where on your list of population elements to begin selecting the names of the 25 men you will interview, select a random number between 1 and k , and begin there. If we randomly select 3 as our starting point, we’d begin by selecting the third fraternity member on the list and then select every fourth member from there. This might be easier to understand if you can see it visually. Table 7.2 lists the names of our hypothetical 100 fraternity members on campus. You’ll see that the third name on the list has been selected for inclusion in our hypothetical study, as has every fourth name after that. A total of 25 names have been selected.
There is one clear instance in which systematic sampling should not be employed. If your sampling frame has any pattern to it, you could inadvertently introduce bias into your sample by using a systemic sampling strategy. This is sometimes referred to as the problem of periodicity . Periodicity refers to the tendency for a pattern to occur at regular intervals. Let’s say, for example, that you wanted to observe how people use the outdoor public spaces on your campus. Perhaps you need to have your observations completed within 28 days and you wish to conduct four observations on randomly chosen days. Table 7.3 shows a list of the population elements for this example. To determine which days we’ll conduct our observations, we’ll need to determine our selection interval. As you’ll recall from the preceding paragraphs, to do so we must divide our population size, in this case 28 days, by our desired sample size, in this case 4 days. This formula leads us to a selection interval of 7. If we randomly select 2 as our starting point and select every seventh day after that, we’ll wind up with a total of 4 days on which to conduct our observations. You’ll see how that works out in the following table.
Do you notice any problems with our selection of observation days? Apparently we’ll only be observing on Tuesdays. As you have probably figured out, that isn’t such a good plan if we really wish to understand how public spaces on campus are used. My guess is that weekend use probably differs from weekday use, and that use may even vary during the week, just as class schedules do. In cases such as this, where the sampling frame is cyclical, it would be better to use a stratified sampling technique . In stratified sampling, a researcher will divide the study population into relevant subgroups and then draw a sample from each subgroup. In this example, we might wish to first divide our sampling frame into two lists: weekend days and weekdays. Once we have our two lists, we can then apply either simple random or systematic sampling techniques to each subgroup.
Stratified sampling is a good technique to use when, as in our example, a subgroup of interest makes up a relatively small proportion of the overall sample. In our example of a study of use of public space on campus, we want to be sure to include weekdays and weekends in our sample, but because weekends make up less than a third of an entire week, there’s a chance that a simple random or systematic strategy would not yield sufficient weekend observation days. As you might imagine, stratified sampling is even more useful in cases where a subgroup makes up an even smaller proportion of the study population, say, for example, if we want to be sure to include both men’s and women’s perspectives in a study, but men make up only a small percentage of the population. There’s a chance simple random or systematic sampling strategy might not yield any male participants, but by using stratified sampling, we could ensure that our sample contained the proportion of men that is reflective of the larger population.
Up to this point in our discussion of probability samples, we’ve assumed that researchers will be able to access a list of population elements in order to create a sampling frame. This, as you might imagine, is not always the case. Let’s say, for example, that you wish to conduct a study of hairstyle preferences across the United States. Just imagine trying to create a list of every single person with (and without) hair in the country. Basically, we’re talking about a list of every person in the country. Even if you could find a way to generate such a list, attempting to do so might not be the most practical use of your time or resources. When this is the case, researchers turn to cluster sampling. Cluster sampling occurs when a researcher begins by sampling groups (or clusters) of population elements and then selects elements from within those groups.
Let’s take a look at a couple more examples. Perhaps you are interested in the workplace experiences of public librarians. Chances are good that obtaining a list of all librarians that work for public libraries would be rather difficult. But I’ll bet you could come up with a list of all public libraries without too much hassle. Thus you could draw a random sample of libraries (your cluster) and then draw another random sample of elements (in this case, librarians) from within the libraries you initially selected. Cluster sampling works in stages. In this example, we sampled in two stages. As you might have guessed, sampling in multiple stages does introduce the possibility of greater error (each stage is subject to its own sampling error), but it is nevertheless a highly efficient method.
Jessica Holt and Wayne Gillespie (2008)Holt, J. L., & Gillespie, W. (2008). Intergenerational transmission of violence, threatened egoism, and reciprocity: A test of multiple pychosocial factors affecting intimate partner violence. American Journal of Criminal Justice, 33 , 252–266. used cluster sampling in their study of students’ experiences with violence in intimate relationships. Specifically, the researchers randomly selected 14 classes on their campus and then drew a random subsample of students from those classes. But you probably know from your experience with college classes that not all classes are the same size. So if Holt and Gillespie had simply randomly selected 14 classes and then selected the same number of students from each class to complete their survey, then students in the smaller of those classes would have had a greater chance of being selected for the study than students in the larger classes. Keep in mind with random sampling the goal is to make sure that each element has the same chance of being selected. When clusters are of different sizes, as in the example of sampling college classes, researchers often use a method called probability proportionate to size (PPS). This means that they take into account that their clusters are of different sizes. They do this by giving clusters different chances of being selected based on their size so that each element within those clusters winds up having an equal chance of being selected.
KEY TAKEAWAYS
- In probability sampling, the aim is to identify a sample that resembles the population from which it was drawn.
- There are several types of probability samples including simple random samples, systematic samples, stratified samples, and cluster samples.
- Imagine that you are about to conduct a study of people’s use of public parks. Explain how you could employ each of the probability sampling techniques described earlier to recruit a sample for your study.
- Of the four probability sample types described, which seems strongest to you? Which seems weakest? Explain.
- Types of Research in Sociology
In this section, you will find an overview of different research methods in sociology. You will find links to tools and resources in the library related to the different types of research and writing.
Qualitative vs Quantitative Research
- What's the Difference?
- Qualitative Research
- Quantitative Research
Research in Psychology is categorized into two general methods:

Non-numerical evidence, usually examined in its raw form
Used when a researcher wants to understand people's opinions, idiosyncratic responses to an event, motivations, or underlying reasons for actions or decisions.
Learn about the Types of Qualitative Research Methods. link will open in a new window
Example: Interviewing the victims of a natural disaster to gather a range of emotional responses.

Numbers! Collected as numerical data or converted into numerical data and examined using statistical methods of analysis.
Used to examine trends and compare populations.
Learn about the Types of Quantitative Research Methods. link will open in a new window
Example: Asking victims of a natural disaster to rank their feelings of anxiety using a pre-determined scale.
When to use them
Psychological research is best when it uses complementary quantitative and qualitative approaches together in the same study, a method called triangulation.
Example: Observing parent-child interactions while watching tv then comparing those observations to measured rates of social and cognitive development in the children who participated in the study.
The Research Continuum

The researchers record data by studying participants at a distance. Researchers try not to influence the participants or their actions.
Types of observational studies include: Naturalistic Observation link will open in a new window , Participant Observation link will open in a new window , or Ethnography. link will open in a new window

The researcher will collect and write detailed accounts of individual lives. A case study can combine a few research approaches, including interviews, observational data, and archival data.
Examples of Case Studies include Freud's history of Anna O link will open in a new window ., and the stories related in Oliver Sacks's best selling book The Man Who Mistook his Wife for a Hat .
A researcher applies their own analytical model to data that has already been collected. They attempt to answer a new question or discover a new trend by looking at old data.
Typical sources of archival data include: census data link will open in a new window , court records will open in a new window , medical records, and even case files from other researchers.

Participants are asked a standard set of questions. These questions may be delivered in writing or through an interview format.
There are three main types of questionnaire methods: Random Sampling link will open in a new window , Stratified Sampling link will open in a new window , and Convenience Sampling link will open in a new window .

Researchers are trying to find a solution to an immediate, practical problem. Examples include reducing drug use or improving worker happiness.
Field research is a type of applied research that is undertaken in a non-laboratory setting. These settings may include a hospital or workplace.

Research conducted in a controlled environment. The results help scholars in the field to learn more about psychological processes such as cognition or emotional development.
Common Research Methods
Types of observational studies include: Non-Participant Observation window or Participant Observation .

A classic example of Case Studies in sociology is Erving Goffman's classic Asylums. link will open in a new window

Information is gathered one-on-one by asking questions orally. Structured Interviews can sometimes be used to gather quantitative data, because the structure allows the interview to function like a questionnaire.
More qualitative types of interviewing range from the Unstructured Interview which functions like a conversation based around a set list of topics, to the In-Depth Interview which may range widely with only a loose guide to direct it.

In-depth studies of groups in their natural setting. These studies utilize multiple type of research to create a multi-layered report. In addition to Participant Observation, a researcher may use interviews, questionnaires, or analysis of secondary research related to the group.
Some good examples of ethnographies available through the Library's online resources include: Recovery's Edge : An Ethnography of Mental Health Care and Moral Agency Link will open in a new window by Neely Laurenzo Meyers, Sex Work and the City Link will open in a new window by Ysmina Katsulis, and The breakup 2.0 : disconnecting over new media Link will open in a new window by Ilana Gershon.
Experimental Research Methods
What is Experimental Research?
Experimental Research is a sub-type of research in sociology. It may utilize the same methods as other research, but it differs in that it attempts measure variables as precisely as possible.
Experimental Research starts with a hypothesis and uses a variety of research methods to test that hypothesis. Ususally involves testing causal relationships.
Researchers study the cause and effect of variables in a natural setting , such as a classroom or workplace.
This allows the researcher to study participants or phenomena in their natural setting, so results might be more accurate . But they have less control over the variables, so results might not be as precise .
Research conducted in a controlled environment.
Participants' reactions may be influenced by the unnatural setting, but researchers have more control over variables.
- << Previous: Home
- Next: Next Steps >>
- Last Updated: Aug 23, 2023 12:40 PM
- URL: https://cccc.libguides.com/sociology
Open Education Sociology Dictionary
quantitative research
Table of Contents
Definition of Quantitative Research
( noun ) Using statistical analysis to quantify and measure social phenomenon, seeking to identify where possible causal relationships, then reporting the findings numerically.
Examples of Quantitative Research
- statistical analysis
- trend study
Quantitative Research Pronunciation
Pronunciation Usage Guide
Syllabification : quan·ti·ta·tive re·search
Audio Pronunciation
Phonetic Spelling
- American English – /kwAHn-tuh-tay-tiv rEE-suhrch/
- British English – /kwOn-ti-tuh-tiv ri-sUHRch/
International Phonetic Alphabet
- American English – /ˈkwɑntɪˌteɪtɪv riˈsɜrʧ/
- British English – /ˈkwɒntɪtətɪv rɪˈsɜːʧ/
Usage Notes
- Plural: quantitative researches
- Quantitative research is compared and contrasted to qualitative research.
- Quantitative research is often viewed as reductionistic , whereas qualitative research is viewed as holistic. However, quantitative and qualitative research are complementary, not contradictory.
- Reductively , quantitative research seeks to identify “how much” and “how often” and qualitative research seeks to explain the “how” and “why”.
- Variant spelling: quantititive
- quantitative analysis
- quantitative data analysis
- quantitative method
- quantitative research method
- quantitative sociology
- Researchers ( adverb ) quantitatively study topic’s ( noun ) quantitativeness to determine the appropriate research method.
Related Videos
Additional Information
- Quantitative Research Resources – Books, Journals, and Helpful Links
- Word origin of “quantitative” and “research” – Online Etymology Dictionary: etymonline.com
Related Terms
- reliability
Works Consulted
Andersen, Margaret L., and Howard Francis Taylor. 2011. Sociology: The Essentials . 6th ed. Belmont, CA: Wadsworth.
Carrabine, Eamonn, Pam Cox, Maggy Lee, Ken Plummer, and Nigel South. 2009. Criminology: A Sociological Introduction . 2nd ed. London: Routledge.
Ferris, Kerry, and Jill Stein. 2010. The Real World: An Introduction to Sociology . 2nd ed. New York: Norton.
Macionis, John, and Kenneth Plummer. 2012. Sociology: A Global Introduction . 4th ed. Harlow, England: Pearson Education.
Macmillan. (N.d.) Macmillan Dictionary . ( https://www.macmillandictionary.com/ ).
Marsh, Ian, and Mike Keating, eds. 2006. Sociology: Making Sense of Society . 3rd ed. Harlow, England: Pearson Education.
Merriam-Webster. (N.d.) Merriam-Webster Dictionary . ( http://www.merriam-webster.com/ ).
Oxford University Press. (N.d.) Oxford Dictionaries . ( https://www.oxforddictionaries.com/ ).
Schaefer, Richard. 2013. Sociology: A Brief Introduction . 10th ed. New York: McGraw-Hill.
Stolley, Kathy S. 2005. The Basics of Sociology . Westport, CT: Greenwood Press.
Wikipedia contributors. (N.d.) Wikipedia, The Free Encyclopedia . Wikimedia Foundation. ( https://en.wikipedia.org/ ).
Cite the Definition of Quantitative Research
ASA – American Sociological Association (5th edition)
Bell, Kenton, ed. 2013. “quantitative research.” In Open Education Sociology Dictionary . Retrieved April 20, 2024 ( https://sociologydictionary.org/quantitative-research/ ).
APA – American Psychological Association (6th edition)
quantitative research. (2013). In K. Bell (Ed.), Open education sociology dictionary . Retrieved from https://sociologydictionary.org/quantitative-research/
Chicago/Turabian: Author-Date – Chicago Manual of Style (16th edition)
Bell, Kenton, ed. 2013. “quantitative research.” In Open Education Sociology Dictionary . Accessed April 20, 2024. https://sociologydictionary.org/quantitative-research/ .
MLA – Modern Language Association (7th edition)
“quantitative research.” Open Education Sociology Dictionary . Ed. Kenton Bell. 2013. Web. 20 Apr. 2024. < https://sociologydictionary.org/quantitative-research/ >.
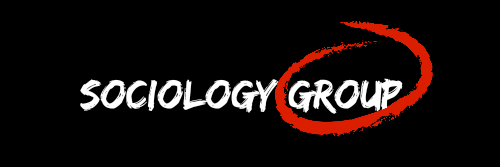
Sociological Research Methods: Qualitative and Quantitative Methods
Research methods and analysis of sociology dealt with techniques to obtain information in a vivid form.
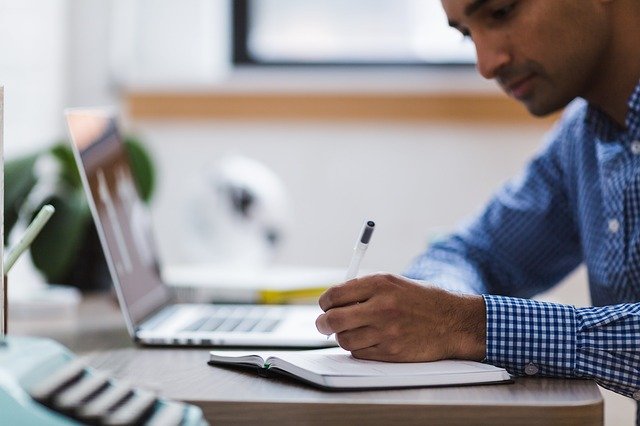
Research is carefully observing patterns for searching for new facts or terms in any kind of subject. For example, there are several research centers for obtaining new results for better performance, say Bhabha Atomic Research center which specializes in nuclear fission and fusion reactions.
Sociologists Redman and Mory explained research work as a systematic way to earn new knowledge or say angle towards anything. For example, after a research work, various developments can be seen.
Research methods are categorized into Qualitative and Quantitative methods .
Quantitative methods included data structures, mathematical formulas, postulates, analysis by pie charts, graphical representations, Co-relation, Regression, etc. The methods used in Quantitative research will be studied in detail below.
- Statistical data
Positivists majorly depend upon this method because they think it is the most convenient and efficient way to see society and its problems. For example, the rate of sex ratio or the number of rape happening in a particular area makes sociologists see the present scenario of the society.
- Comparative Method
It can be easily guessed from the name itself that the method includes comparing different values. For example, in the science laboratory, there are comparators which compare different values of resistance and thus a mean value is written. Same is the case with sociology, different societies are compared by sociologist and after observing each and every factor they develop some theories under their research work. Marx, Durkheim , and Weber are said to be the inventor of this method which profoundly deals with the logic. The three of them compared many societies with each other to give some of the wonderful research work. Marx studied the phenomenon of difference and thus agreed that societies transform via many changes.
Durkheim observed the basis of division of labour and Weber tried to link the relation between capitalist and exploited countries. This method is still used by many sociologists for letting the world know about differences. For example, Michael Mann compared how every country differs when it comes to power and dominance. Devine showed the condition of workers in different time periods.
- Field Methods
Science experiments are generally done in respective laboratories. But sociology experiments are performed in a natural arrangement outside the labs. For example, sociologists can carry out experiments in which they can observe people interaction ability thus categorizing them into introverts, ambivert, and extroverts. The advantage of this method is that it allows the expansion of areas where the experiment can be performed and better results are obtained as compared to other methods. But likewise, its biggest disadvantage is the variance can cause experiment results to differ unlike experiments performed in a science laboratory. This error is also termed as a Hawthorne effect. The experiments do not account for generalizing any theory as a particular amount of people can be tested.
Qualitative Methods are those methods which depend on the theories of Interactionism Theories. For example people way of talking under different circumstances studied by a researcher. The result will be completely based on the way the researcher perceives everything. The various methods of a Qualitative method are studied below.
- Participant Observation
It can be seen as a modification of Field methods as this method involves the researcher too. The researcher has to keep a mindset as an observant which will decrease the chances of a biased opinion as the perception will not be compressed. The field researchers, data or any theory is studied comprehensively as a researcher and participant point of view.
- Direct Observation
This method was one step up-gradation to field methods and Participant Observation. This observation also included a third party involvement whose perception cannot fall into the claws of a biased nature. For example, even if a researcher tries to complete experiment, he will not totally drench himself into the perception of the participant, thus a third person who will see the whole activity without any judgment will yield better results. For example in cricket matches, apart from umpires, a proper video is taken to see whether the player is out or not. This makes the judgment fair enough for everybody. In simple words, participant and researchers are not aware of the fact that they are being observed which accounts for natural reactions.
- Unstructured Interviewing
- These interviews are completely in contrast to conventionally structured interviews. They differ in various aspects. In unstructured interviewing, there are no set of standardized questions. The discussion can travel in any direction depending on the interviewer. Due to lack of patter, these interviews are hard to crack.
- Case Studies
Case studies do not go along with a single method. There are various methods which are being used for observing even the minute details. It can be called as the summation of the direct method, unstructured interviews etc. The quantitative and qualitative approaches a given situation in an entirely different way. For example, quantitative methods are based on mathematical numbers, graphs, and statistics. But because of this method, much information is lost accounting for little information as compared to the qualitative method. Quantitative analysis is fact-driven but the facts can change anytime but they are mostly copied from earlier records, whereas qualitative analysis is observation-driven, its data can be changed accordingly which is its biggest advantage over the other.
TECHNIQUES OF DATA COLLECTION
Data collection is mainly stored in two ways, primary resources , and secondary resources .
Primary Resources are the data which are obtained by researchers, for example through personal or telephonic interviews, participant behaviour by keenly observing them or asking them a set of questions.
Secondary resources are the data which are mainly records in any form. For example, any old book can provide much information about the time period comes under secondary resources. There is no direct information but mainly statistics, graphs, old research works, or historical books.
MORE METHODS OF QUALITATIVE AND QUANTITATIVE ANALYSIS:-
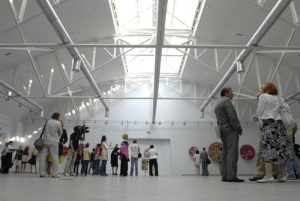
- Participant and Quasi-Participant Observation
It has been proved for a long time that observation helps in collecting data as well as result in accurate analysis. Observation contains two major functions viz. causes and effects. The observation is categorized in two ways viz. controlled and uncontrolled, active and passive.
Inactive observation, the researcher is also a part of an analysis. For example, he will take part in a game and will play fairly at his part.
In passive observation, the researcher observes everything from a distant place without getting noticed. For example mother-son duo small gestures can be easily noticed by him/her.
Controlled observations are those matter of solicitation in which things can be brought under control anytime. For example, knowing that someone is observing me I can easily change my reactions.
Uncontrolled observations are those observations in which neither researcher nor the people under observation stop the process of analysis. They are being adaptive to any situation no matter what results can be obtained.
There is another type called a Mixed Observation type. In these methods, extremities are found. Either the researcher is totally drenching in the activity or will be observing every bit in solitude. It is also known as Quasi Participant Observation.
This method involves a panel of interviewers and applicants. For example, in any placement drive, a panel is set up and they took a massive amount of information about the applicants by asking them many questions. Much information about their personality, IQ, confidence, abilities is judged in a matter of some minutes. The interviews can be of many types viz. formal, informal, solo or group.
Informal interviews are not much in trend but the other three are practised at a rapid rate.
- Questionnaire
A questionnaire is a set of questions designed in a format which can be solved by only those who can read and write. Thus the biggest disadvantage of this method is that it cannot be fulfilled by everybody. The sole purpose of this method is storing answers and due to same questions, best answers manage to secure the position.
The schedule is entirely based on the way an interviewer seek things. The questionnaire set is solved by a person in front of the researchers. Thus the question does not affect much, but the perspective of the researcher does. There are many types of schedule:-
- Rating Schedules – This kind of schedules generally come under the HR department. The opinions, ways of accepting or rejecting things, or habits are observed keenly.
- Document Schedules – As the name suggests, it generally involves the paperwork. For example in criminology, criminal’s history is studied. Case studies are also popular, for example how to transform a city into the smart city.
- Evaluation Schedules – Quantitative analysis for example data collection is a primary objective of this schedule. For example, if a company arrives at placement, the students collect every data, for example, the company position, job profile, CTC etc.
- Observation Schedules – The researcher will observe everybody’s intention, either by involving in any activity or by being aloof.
- Interview Schedules – The researcher freely asks respondents any question and after deciding their confidence, time to think, IQ etc is judged.
Continue Reading → Variable,Sampling,Hypothesis,Reliability & Validity
Sociology Group
We believe in sharing knowledge with everyone and making a positive change in society through our work and contributions. If you are interested in joining us, please check our 'About' page for more information
Quantitative Methodology Research Paper
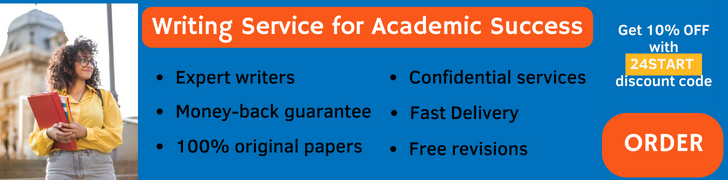
View sample sociology research paper on quantitative methodology. Browse other research paper examples for more inspiration. If you need a thorough research paper written according to all the academic standards, you can always turn to our experienced writers for help. This is how your paper can get an A! Feel free to contact our custom writing service for professional assistance. We offer high-quality assignments for reasonable rates.
History of Sociological Quantification
Quantitative reasoning is widely applied in the discipline of sociology and quantification aids sociologists in at least seven main research areas: quantitative modeling, measurement, sampling, computerization, data analysis, hypothesis testing, and data storage and retrieval. But sociologists differ widely in their views of the role of quantification in sociology. This has apparently always been true to some degree. While Durkheim was a proponent of quantification, Weber was less enthusiastic. However, while Weber advocated the nonquantitative method Verstehen, both Weber and Durkheim saw the importance of method as well as theory, as both authored books on method (Weber 1949; Durkheim [1938] 1964). Today, the situation is much different, as a wide gulf exits between theory and method in twenty-first-century sociology, with only a few authors such as Abell (1971, 2004) and Fararo (1989) simultaneously developing theory and quantitative methodology designed to test theoretical propositions.
Academic Writing, Editing, Proofreading, And Problem Solving Services
Get 10% off with 24start discount code.
The most vocal proponent of quantification in sociology may have been Lundberg (1939), who was known as the unabashed champion of strict operationalism. Operationalism, as originally defined in physics by Bridgman (1948), is the belief that “in general any concept is nothing more than a set of operations, the concept is synonymous with the corresponding set of operations ” (Bridgman 1948:5–6). George Lundberg (1939, 1947) took the application of operationalism in sociology to an extreme. In Lundberg’s view, one did not approach an already existing concept and then attempt to measure it. The correct procedure in Lundberg’s view is to use measurement as a way of defining concepts. Thus, if one is asked what is meant by the concept of authoritarianism, the correct answer would be that authoritarianism is what an authoritarianism scale measures.
When he encountered objections to his advocacy of the use of quantification in sociology, Lundberg (1939, 1947) replied that quantitative concepts are ubiquitous in sociology, and need not even be symbolized by numerals, but can be conveyed verbally as well. For example, words such as “many,” “few,” or “several” connote quantitative concepts. In Lundberg’s view, quantification is embedded in verbal social research as well as in everyday thought and is not just an artificial construct that must be added to the research process by quantitative researchers.
After Lundberg (1939, 1947) and others such as Goode and Hatt (1952) and Lazarsfeld (1954) laid the foundation for quantitative sociology in the 1930s, 1940s, and 1950s, the field surged in the 1960s and 1970s. The 1960s saw increased visibility for quantitative sociology with the publication of books and articles such as Blalock’s (1960) Social Statistics, Kemeny and Snell’s (1962) Mathematical Models in the Social Sciences; White’s (1963) An Anatomy of Kinship; Coleman’s (1964) Introduction to Mathematical Sociology, Foundations of Social Theory; Duncan’s (1966) “Path Analysis: Sociological Examples”; Land’s (1968) “Principles of Path Analysis”; Blalock’s (1969) Theory Construction: From Verbal to Mathematical Formulations; and White’s (1970) Chains of Opportunity.
Quantitative methods became even more visible in the 1970s and 1980s with the publication of a host of mathematical and statistical works, including Abell’s (1971) Model Building in Sociology; Blalock’s (1971) Causal Models in the Social Sciences; Fararo’s (1973) Mathematical Sociology; Fararo’s (1989) Meaning of General Theoretical Sociology; Bailey’s (1974b) “Cluster Analysis”; and Blalock’s (1982) Conceptualization and Measurement in the Social Sciences.
Quantitative Data Collection
Specific quantitative techniques make rigorous assumptions about the kind of data that is suitable for analysis with that technique. This requires careful attention to data collection. For data to meet the assumptions of a quantitative technique, the research process generally entails four distinct steps: hypothesis formulation, questionnaire construction, probability sampling, and data collection.
Hypothesis Formulation
A hypothesis is defined as a proposition designed to be tested in the research project. To achieve testability, all variables in the hypothesis must be clearly stated and must be capable of empirical measurement. Research hypotheses may be univariate, bivariate, or multivariate, and some may contain auxiliary information, such as information about control variables. The vast majority of hypotheses used by quantitative sociologists are bivariate. The classical sequence is to formulate the hypotheses first, before instrument construction, sample design, or data collection. Hypotheses may be inductively derived during prior research (Kemeny and Snell 1962) or may be deductively derived (Bailey 1973). Increasingly, however, quantitative sociologists are turning to the secondary analysis of existing data sets. In such a case, hypothesis formulation can be a somewhat ad hoc process of examining the available data in the data bank or data set and formulating a hypothesis that includes the existing available variables.
For example, Lee (2005) used an existing data set and so was constrained to formulate hypotheses using the available variables. He presented three hypotheses, one of which stated that democracy is not directly related to income inequality (Lee 2005:162). While many quantitative studies in contemporary sociology present lists of formal hypotheses (usually five or less), some studies either leave hypotheses implicit or do not present them at all. For example, Torche (2005) discusses the relationship between mobility and inequality but does not present any formal hypotheses (p. 124).
Questionnaire Construction
In the classical research sequence, the researcher designed a questionnaire that would collect the data necessary for hypotheses testing. Questionnaire construction, as a middle component of the research sequence, is subject to a number of constraints that are not always well recognized. First and foremost is the necessity for the questionnaire to faithfully measure the concepts in the hypotheses. But other constraints are also imposed after questionnaire construction, chiefly sampling constraints, data-collection constraints, and quantitative data-analysis constraints. The questionnaire constrains the sampling design. If the questionnaire is very short and easily administered, this facilitates the use of a complicated sample design.
However, if the questionnaire is complex, then sample size may need to be reduced. The construction of a large and complex questionnaire means that it is difficult and time-consuming to conduct a large number of interviews. It also means that money that could otherwise be spent on the sample design must now be used for interviewer training, interviewing, and codebook construction. In addition to such sampling and data-collection constraints, the chief constraint on instrument design is the type of quantitative technique to be used for data analysis.
That is, the questionnaire must be designed to collect data that meet the statistical assumptions of the quantitative techniques to be used. Questionnaires can quickly become long and complicated. Furthermore, there is a tendency to construct closed-ended questions with not more than seven answer categories. While such nominal or ordinal data are often used in regression analyses, they are marginally inappropriate for ordinary least squares (OLS) regression and other quantitative techniques that assume interval or ratio data. Clearly, one of the great advantages of conducting a secondary analysis of data that has already been collected is that it avoids dealing with the many constraints imposed on the construction of an original datacollection instrument.
Probability Sampling
Many extant quantitative techniques (particularly inductive statistics) can only be used on data collected with a rigorous and sufficiently large probability sample, generally a random sample of some sort. One of the questions most frequently asked of research consultants is, “What is the minimum sample size acceptable for my research project?” Based on the law of large numbers and other considerations, some researchers permit the use of samples as small as 30 cases (Monette, Sullivan, and DeJong 2005:141). There is clearly a trend in the sociological literature toward larger sample sizes, often achieved through the use of the secondary analysis of existing samples and the pooling of multiple samples.
Sociology had few if any research methods books of its own prior to the publication of the volume by Goode and Hatt (1952). Before 1952, sociological researchers relied primarily on psychology research books, such as Jahoda, Deutsch, and Cook (1951), which de-emphasized sampling by relegating it to the appendix. Psychology emphasized the experimental method, with a small number of research subjects (often 15 or less), and de-emphasized surveys. Furthermore, in the mid-twentieth century, it was common for both psychology and sociology to use a “captive audience” sample of students from the researcher’s classes.
The chief research models for sociology before 1952 were psychology and (to a lesser degree) medicine. While psychology routinely used a small sample of subjects in experiments, samples in medical research were often quite small as well. If a researcher is conducting medical research, such as a study of pediatric obsessive compulsive disorder, it may be difficult to obtain more than 8 or 10 cases, as the onset of this syndrome is usually later in life. With psychology and medicine as its chief models before 1952, sample sizes in sociology tended to be small.
Over time, sample sizes in sociology have grown dramatically. The present emphasis is on national samples and multinational comparisons, as sociology moves away from the psychological model and toward the economic model. For example, Hollister (2004:669, table 1) did not collect her own data, but used secondary data with an N of 443, 399 to study hourly wages.
Data Collection
During the period 1950 to 1980 when social psychology was dominant in sociology, data collection was often a matter of using Likert scales of 5–7 categories (see Bailey 1994b) to collect data on concepts such as authoritarianism or alienation from a relatively small sample of persons.
Now that economics is becoming the dominant model (see Davis 2001), there are at least two salient ramifications of this trend. One is that an individual researcher is unlikely to possess the resources (even with a large grant) to collect data on 3,000 or more cases and so must often rely on secondary data, as did Joyner and Kao (2005). Another ramification is that researchers wishing to use these large economic data sets that are relatively prevalent must obviously use a different kind of data, and different quantitative techniques, than researchers did in an earlier era when psychology predominated. The psychological orientation resulted in data collection more conducive to analysis of variance, analysis of covariance, and factor analysis, in addition to multiple regression (OLS). Today things have changed, and the technique of choice for the large economic data sets is logistic regression.
Mathematical Sociology
It is useful to divide the extant quantitative techniques in twenty-first-century sociology into inferential statistics (probability-based techniques with tests of significance) and mathematical models (techniques that lack significance tests and are often nonprobabilistic). Rudner (1966) makes a distinction between method and methodology. Although the two terms are often used interchangeably in sociology and elsewhere, there is an important difference between them. According to Rudner, methods are techniques for gathering data, such as survey research, observation, experimentation, and so on. In contrast, methodologies are criteria for acceptance or rejection of hypotheses. This is a crucial distinction. Some mathematical models lack quantitative techniques for testing hypotheses, as these are not built into the model.
In contrast, inductive statistics, in conjunction with statistical sampling theory, provides a valuable means for sociologists not only to test hypotheses for a given sample but also to judge the efficacy of their inferences to larger populations. Tests of significance used in sociology take many forms, from gamma to chi-square to t -tests, and so on. Whatever the form or level of measurement, significance tests yielding probability, or “ p, ” values provide not only a way to test hypotheses but also a common element for community with researchers in other disciplines that also use significance tests.
Mathematical sociology has traditionally used methods such as differential and integral calculus (Blalock 1969: 88–109). Differential equations are frequently used to construct dynamic models (e.g., Kemeny and Snell 1962; Blalock 1969). However, one of the problems with mathematical models in sociology (and a problem that is easily glossed over) is that they are sometimes very difficult to apply and test empirically. Kemeny and Snell (1962) state that mathematical models are used to deduce “consequences” from theory, and that these consequences “must be put to the test of experimental verification” (p. 3). Since experimental verification in the strictest sense is relatively rare in sociology, this seems to be an Achilles heel of mathematical sociology.
To verify the predictions by comparing them with the experimental data, Kemeny and Snell (1962) use the statistical test chi-square. That is, the mathematical model proves inadequate for hypothesis testing and must be augmented by a statistical test (p. 62). Kemeny and Snell (1962) then “improve” the model by stating that there may be some subjects to which the model does not apply and “adding the assumption that some 20 per cent of subjects are of this type” (p. 62). Unfortunately, such “model simplification,” achieved by simply excluding a proportion of the population from the analysis, is rather common in quantitative sociology. Yamaguchi (1983) explains his failure to include women in the analysis by writing, “In this paper, I limit my analysis to non-black men to simplify the model” (p. 218).
The dilemma is real. If the sociological phenomenon is too complex, then the mathematical sociologist will not be able to solve all the inherent computational problems, even with a large computer. Fortunately, the future technological advances in computer hardware and software, along with the continued development of new mathematical techniques such as blockmodeling (Doreian, Batagelj, and Ferligoj 2005), ensure a bright future for mathematical sociology. While the challenges of social complexity are real, the rewards for those who can successfully model this complexity with mathematics are great. For additional commentary and references on mathematical sociology in the twenty-first century, see Edling (2002), Iverson (2004), Lewis-Beck, Bryman, and Liao (2004), Meeker and Leik (2000), and Raftery (2005).
Statistical Sociology
While statistical methods extant in sociology can all be classified as probability based, they can be divided into tests of significance (such as gamma) and methods used for explanation (often in terms of the amount of variance explained), prediction, or the establishment of causality. Among these techniques, the most commonly used are multiple correlation, multiple regression, logistic regression, as well as analysis of variance (the dominant method in psychology) or analysis of covariance. Other methods used less frequently by sociologists include cluster analysis, factor analysis, multiple discriminant analysis, canonical correlation, and smallest space analysis (Bailey 1973, 1974a), and latent class analysis (Uggen and Blackstone 2004).
Which statistical technique is appropriate for a given analysis can depend on a number of factors, one of which is the so-called level of measurement of the quantitative data involved. S. S. Stevens (1951) divided data into four distinct levels—nominal, ordinal, interval, and ratio. It is important to stress consistent measurement at all four levels, as lack of attention to consistent measurement across studies in sociology is problematic for the field.
The reality is that nominal variables can be very important in both sociological theory and statistics, but unfortunately they have been badly neglected by sociologists and often are created and treated in a haphazard fashion. This is unfortunate because discussions of classification techniques are readily available to sociologists in the form of work on cluster analysis and various classification techniques for forming typologies and taxonomies (McKinney 1966; Bailey 1973, 1994a). Carefully constructed classification schemas can form the foundation for all “higher” levels of measurement. A sociological model lacking adequate nominal categories can be the proverbial house of cards, ready to collapse at any moment.
The nominal level of measurement deals with nonhierarchical categories. Many of the most theoretically important and frequently used sociological variables lie at this level of measurement, including religion, sex, political affiliation, region, and so on. Much of the statistical analyses at the nominal level consist of simple frequency, percentage, and rate analysis (Blalock 1979). However, the chi-square significance test can be used at the nominal level, as can a number of measures of association, such as Tschuprow’s T, V, C, Tau, and Lambda (Blalock 1979:299–325). Sociologists often dislike nominal categorical variables because it is felt that they are merely descriptive variables that do not possess the explanatory and predictive power of continuous variables, such as interval and ratio variables. But more important, nominal (and also ordinal) categorical variables are disliked because they generally do not fit into the classical multiple regression (OLS) models that (until the recent dominance of logistic regression) have been widely used in sociology.
In univariate cases with a large number of categories, or especially in multivariate cases with a large number of variables, and with each containing a large number of categories, the analysis can quickly become very complex, so that one is dealing with dozens if not hundreds of categories. As Blalock (1979) notes, there is often a tendency for researchers to simplify the analysis by dichotomizing variables (p. 327). Unfortunately, such attenuation results in both loss of information and bias.
Another problem with categorical data is that the printed page is limited to two dimensions. Thus, if one has as few as five categorical variables, and wishes to construct a contingency table showing their interrelations, this requires a five-dimensional table, but only two dimensions are available. The customary way to deal with this, even in computer printouts, is to print 10 bivariate tables, often leading to an unmanageable level of complexity.
Nominal and ordinal variables share some similarities and problems. Measures of association such as Spearman’s r s and tests of significance such as the Wilcoxon test are also available for ordinal variables (Blalock 1979). As with nominal variables, ordinal variables cannot be added, subtracted, multiplied, or divided (one cannot add rank 1 to rank 2 to obtain rank 3).
The ordinal level shares with the nominal level the problem of the desire to simplify. Sociologists often wish to reduce the number of ordered categories to simplify the research project, but unfortunately they often conduct this simplification in an ad hoc manner, without any statistical or theoretical guidelines for reducing the number of categories. Again, this leads to problems of attenuation and bias, as noted for the nominal level.
Interval and Ratio
A sea change has occurred in sociology in the last 40 years, as shown later in the review of American Sociological Review ( ASR ) . During the 1950s and 1960s, American sociologists relied primarily on percentage analysis, often using nominal and ordinal measurement. Later in the twentieth century, quantitative researchers stressed the use of interval and ratio variables to meet the assumptions of OLS multiple regression analysis. Now, as seen below, there has been a major shift back to the use of nominal and ordinal variables in logistic regression.
Interval variables are continuous, with “arbitrary” zero points, while ratio variables have absolute or “nonarbitrary” zero points. Theoretically, only ratio variables, and only those found in nonattenuated fashion with a wide range of continuous values, should be used in multiple regression models, either as independent or dependent variables. Although textbooks such as Blalock (1979) say that only interval measurement is needed, in my opinion ratio is preferred and should be used whenever possible (p. 382). In reality, continuous variables are routinely used in regression without testing to see whether they can be considered ratio or only interval.
Furthermore, while such continuous variables may theoretically or potentially have a wide range of values, they often are empirically attenuated, with extremely high and low values (or perhaps even midrange values) occurring infrequently or rarely. Also, attenuated variables that are essentially ordinal, and contain only five values or so, are often used in surveys (e.g., Likert scales). While these Likert variables do not meet the technical requirements of multiple regression, either as dependent or independent variables, they are often used in regression, not only as independent variables but also as dependent variables.
As noted earlier, sociologists have traditionally struggled to meet the requirements of OLS regression, especially when encountering so many nominal and ordinal variables in everyday theory and research. For example, Knoke and Hout (1974) described their dependent variable (party identification) by saying, “The set of final responses may be coded several ways, but we have selected a fivepoint scale with properties close to the interval scaling our analysis requires” (p. 702). While this dependent variable may indeed be “close” to interval, it remains severely attenuated, possessing only five “points” or values compared with the hundreds or even thousands of potential values in some interval variables. In addition to using attenuated ordinal scales in regression (even though they clearly do not meet the assumptions of regression), sociologists often use nominal variables in regression. These are often used as predictors (independent variables) through the technique of “dummy variable analysis” involving binary coding.
As shown later by my review of ASR, the most common statistical technique in contemporary sociology is multiple regression in some form, including OLS and logistic regression. However, many of the variables used in sociology are nominal or ordinal. Those that are interval or ratio are often recoded as ordinal variables during data collection. The result is that between the existence of “naturally occurring” nominal and ordinal variables and the (often unnecessary) attenuation of nominal, ordinal, interval, and ratio variables, the range of empirical variation is greatly attenuated.
A common example is when an income variable with potentially dozens or even hundreds of values is reduced to five or so income categories to make it more manageable during the survey research process (see Bailey 1994b).
While it is true that respondents are often reluctant to provide their exact income, other alternatives to severe category attenuation are available. These include the use of additional categories (up to 24) or even the application of techniques for dealing with missing data. In addition, some common dependent variables, when studied empirically, are found to have small empirical ranges, but the adequacy of correlation and regression is formally assessed in terms of the degree of variance explained. Considering the cumulative effect of variables that are empirically attenuated, added to those variables that are attenuated by sociologists during the course of research, it is not surprising that explained variance levels are often disappointing in sociology.
A generic multiple regression equation for two independent variables is shown in Equation 10.1.
Y = a + b 1 X 1 + b 2 X 2 [10.1]
The model in Equation 10.1 is quite robust and adaptable but should not be abused by using it with severely attenuated data. Although one cannot add additional dependent variables, additional independent variables are easily added. Also, the model can easily be made nonlinear by using multiplicative predictors such as X 1 X 2 or X n .
Assume that the dependent variable ( Y ) is annual income, and the predictors are, respectively, age and educational level. One could conduct an OLS regression analysis for a large data set and experience a fairly small degree of attenuation if the data were collected properly and the variables were not attenuated through unnecessary categorization. But now assume that a second regression analysis is computed on Equation 10.1, but this time the dependent variable is whether the person attends college or not, coded 1 or 0, and the independent variables are sex (coded 1 for female and 0 for male) and age (coded 1 for 20 or younger and 0 for 21 or older). Running OLS regression on this will yield very little in terms of explained variance. The analysis can be converted to logistic regression by computing the odds ratio and taking the natural log (logit) to make it linear. The limitations of this model are that little variance exists to be explained and the predictors are inadequate.
Implications
While many of the logistic regressions one sees in the sociological literature have many more predictors, many of these are often dummy variables (ordinal or ratio), and the wisdom of running regression on such data remains debatable. What accounts for the tremendous popularity of logistic regression, when many times the degree of variance explained remains decidedly unimpressive (see the discussion below)? Perhaps logistic regression is now a fad, or perhaps users do not see an adequate alternative. Why do they not just present correlation matrices? Why is regression needed? Perhaps because typologies using nominal variables are said to provide description, correlation is said to provide explanation, and regression is said to provide prediction, with prediction considered to be the highest form of analysis (Blalock 1979).
The implications of the analysis to this point are clear: Sociologists have long struggled to deal with the analytical problems posed by the different levels of measurement, and they continue to do so. While the recent widespread adoption of logistic regression has surely changed the way that sociologists deal with nominal (and to a lesser extent ordinal) variables, for example, it is not clear that the fit between theory and method, or between empirical data and method, has been drastically improved. Changes are still needed, and some recommendations are presented below.
Method and Theory
As previously noted, method and theory have become sharply bifurcated within sociology over the past 40 years. While the ASR once published methods articles, now these articles are routinely segregated into journals, such as Sociological Methodology, Sociological Methods and Research, or the Journal of Mathematical Sociology. Thus, quantitative methods are not only separated from qualitative sociology (which has its own journals such as Qualitative Sociology ) but also are separated from sociological theory (with its own American Sociological Association journal, Sociological Theory ).
Kemeny and Snell (1962) state that one first inductively derives a theory through observation and empirical research and then uses quantitative models to deduce testable hypotheses from the theory. The procedure suggested by Kemeny and Snell (1962) is a sound one. The obvious problem with successfully using such an integrated theory/method research process in contemporary sociology is that the theory and quantitative methods knowledge segments are so segregated and widely divided that it is increasingly difficult for the individual researcher to have access to all of this separated literature. By segregating sociology into largely verbal theory ( Sociological Theory) and quantitative sociology (the Journal of Mathematical Sociology ), the process of developing theories and testing them is made more difficult than it should be.
In spite of the wide degree of artificial separation of theory and method in sociology, the quantitative area has changed in a manner that makes it more consistent with the needs of theory. To meet the goal of operationalizing sociological theory, the quantitative method area should minimally provide three main services:
- Quantitative sociology must provide both diachronic (dynamic) models dealing with process and synchronic (cross-sectional) models dealing with structure. Until the last decade or so, statistical sociology provided mainly synchronic or cross-sectional models via OLS. Now many logistic regression models are longitudinal as in event history analysis (Allison 1984).
- The second service that quantitative method (including both statistical sociology and mathematical sociology) must provide is to talk increasingly in terms of actors rather than primarily in terms of equations or variables. While theory talks in terms of action by individuals or groups (agency), quantitative method talks in terms of change in variables (mathematics) or relationships among sets of variables (regression). A good example of the use of actor-oriented dependent variables in logistic regression is provided by Harknett and McLanahan (2004) who predict whether the baby’s mother will take a certain action or not (marry the baby’s father within 30 days).
- Quantitative sociology must do a better job of raising R 2 s as variance explained in many regression analyses in sociology (whether OLS or logistic regression) remains unacceptably low. A lot of this may be due to attenuation of variables, both dependent and independent. As seen above, some of the attenuation is avoidable, and some unavoidable. Until recently, the dominant regression model was OLS regression, which did a poor job of incorporating nominal and ordinal variables. Logistic regression includes nominal variables aggressively, thus making it more compatible with theory that is replete with such nominal variables and providing a welcome means of bridging the theory-method gap. However, it is unclear that the incorporation of nominal variables (both dependent and independent) in logistic regression has raised the variance explained by any meaningful degree. It is important that we pay more attention to this problem and that we focus on R 2 values, not just on p That is, it is likely that there is actually more variance that can be explained empirically, but the techniques in use are not picking it all up. Perhaps sociology has lost sight of whether sociological models fit the data well, which is the primary point of prediction. To say it another way, if logistic regression is used in virtually every analysis in the ASR, it seems obvious that this method will fit the data better in some cases than in others. In the cases where it can be determined that the fit is not good, perhaps an alternative method of analysis should be considered.
Historical Comparisons
Perhaps most sociologists are at least vaguely aware of changes in quantitative techniques that have appeared in the sociological literature in the last 40 years, particularly the shift toward logistic regression. I decided that it would be helpful to illustrate these changes by conducting a review of the ASR over the last 40 years. While a full review of all issues was impossible due to time constraints, it seemed that a partial review would be illuminating. I compared the last full volume of the ASR that was available (2004) with the volumes 40 years before (1964), and 30 years before (1974), as shown in Table 1.

Table 1 shows the presence or absence of quantitative analysis in every article of ASR in 1964 (Volume 29), 1974 (Volume 39), and 2004 (Volume 69). These volumes were not selected by scientific probability sampling but were arbitrarily chosen to reflect changes in quantitative methods. The first year (1964) shows the initial use of regression, 1974 shows the growth of OLS regression, and 2004 (the last full volume available) shows the dominance of regression, both the continuing presence of OLS and the predominance of logistic regression. Presidential addresses were omitted as they tended to be nonquantitative essays. I also omitted research notes, replies, and comments and included only the articles from the main research section of the journal.
The first row of Table 1 analyzes Volume 29 (1964) of ASR. It reveals that 70 percent of all articles (28 out of 40) were quantitative. The remaining 12 were verbal essays without any numbers. An article was counted as quantitative if it had raw scores or means. The predominant numerical method in 1964 was percentage analysis; however, there were two cases of regression analysis. These were OLS analyses with continuous dependent variables, although they were identified only as “regression analysis.” There were no instances of logistic regression. Although regression was soon to dominate sociological statistics, this trend was not yet evident in 1964.
However, by 1974, the trend toward the use of regression was clearly visible. The proportion of the articles that were quantitative in 1974 was 86 percent, up from 70 percent a decade earlier. Although there were still no logistic regression analyses in ASR in 1974 (regression with categorical dependent variables), fully 49 percent of all quantitative articles (and 42 percent of all articles in the entire volume) were OLS regressions showing clear evidence of its upcoming dominance in sociological analysis.
It should be noted that in 1974, many of the OLS regression analyses were presented in the form of “path analysis,” with the “path coefficients” presented in path diagrams. While 70 percent of all ASR articles were quantitative in 1964 and 86 percent in 1974, by 2004 the proportion of quantitative ASR articles had climbed to a startling 95 percent, with logistic regression in some form accounting for the majority of these. Out of a total of 37 articles in Volume 69, only two were entirely verbal, lacking any numerical analysis at all.
Even more startling was the fact that in 2004, out of the 35 quantitative articles in ASR, 32, or 86 percent of all articles in the volume, and 91 percent of all quantitative articles were regressions. Still more surprising, of the 32 articles with regressions, only three had OLS regression only. The remaining 29 had logistic regression, with 25 of these containing logistic regression only, and with four more articles presenting both OLS and logistic regression in the same article. Four additional articles (not shown in Table 1) contained “hybrid” models, which used various combinations of OLS and logged dependent variables, or presented models said to be “equivalent to OLS,” and so on. Of the three quantitative articles that contained no regression, one contained both analysis of variances and analysis of covariance, while the other two contained only percentage analysis.
When logistic regression occurs in 29 out of 35 (83 percent) of quantitative articles and 29 out of 37 total articles (78 percent), it obviously has an amazing degree of dominance for a single technique. In fact, in the last four issues of Volume 29 (Issues 3, 4, 5, and 6), 19 of the total of 20 articles contained logistic regression of some sort (the other article was entirely verbal, with no quantitative analysis of any kind). This means that fully 100 percent of the quantitative articles (and 95 percent of all articles) in the June through December issues of the 2004 ASR (Volume 69) contained at least one logistic regression analysis. This dominance prompts the rhetorical question of whether one can realistically hope to publish in ASR without conducting logistic regression. It appears possible, but the odds are against it. If one wishes to publish in ASR without logistic regression analysis, the article should include OLS regression.
What accounts for the fact that in 2004, 95 percent of all published ASR articles were quantitative, and of these, 83 percent contained at least one logistic regression analysis? Could it be that quantitative sociologists in general are taking over the field of sociology, and sociologists should expect a wave of mathematical sociology articles to be published in ASR? I did not see any publications in Volume 69 containing articles that I would classify as mathematical sociology. I did see two models in 1974 that I would classify as work in mathematical statistics (one stochastic model and one Poisson model), but none in 2004.
Comparing 1974 ASR articles with 2004 ASR articles, we see a sea change toward logistic regression. From the standpoint of quantitative methodology, I can certainly appreciate the heavy reliance that ASR currently has on logistic regression. While casual observers might say that “regression is regression” and that not much has changed in 30 years, in reality nothing could be farther from the truth. The 29 logistic regression analyses presented in Volume 69 of ASR differ from the 25 OLS regression analyses of Volume 39 in a number of important ways. The traditional OLS regression that was dominant in 1974 has the following features:
- It uses a continuous (internal or ratio) dependent variable.
- It uses predominantly continuous independent variables, perhaps with a few dummy variables.
- It uses R 2 to evaluate explanatory adequacy in terms of the amount of variance explained.
- It uses about 5 to 10 independent variables.
- It usually reports values of R 2 (explained variance) in the range of .20 to .80, with most values being in the intermediate lower part of this range.
In contrast, the logistic regression that dominates twenty-first-century sociology has these features:
- It uses categorical rather than continuous dependent variables (see Tubergen, Maas, and Flap 2004).
- It often uses rather ad hoc procedures for categorizing dependent and independent variables, apparently without knowledge of proper typological procedures (Bailey 1994a) and without regard to the loss of information that such categorization entails, as pointed out by Blalock (1979). Some of these decisions about how categories should be constructed may be theory driven, but many appear to be arbitrary and ad hoc categorizations designed to meet the specifications of a computerized model.
- It logs the dependent variable to “remove undesirable properties,” generally to achieve linearity, and to convert an unlogged skewed distribution to a logged normal distribution, more in keeping with the requirements of regression analysis (see Messner, Baumer, and Rosenfeld 2004).
- It uses more categorical or dummy variables as independent variables, on average, than does OLS regression.
- It uses larger samples.
- It uses more “pooled” data derived through combining different samples or past studies. This has the advantage of getting value from secondary data. While it is good to make use of data stored in data banks, in some cases this practice may raise the question of whether the data set is really the best one or is just used because it is available.
- It uses more models (often three or more) that can be compared in a single article.
- It uses more multilevel analysis.
- It uses more “corrections” of various sorts to correct for inadequacies in the data.
- It often does not report R 2 because it is generally recognized to have “undesirable properties” (see Bailey 2004), thereby providing no good way for evaluating the efficiency of the explication in terms of the amount of variance explained.
- It generally reports statistically significant relationships with p values less than .05, and often less than .01, or even .001.
- It presents more longitudinal analysis.
While the trends toward multilevel analysis, longitudinal analysis, and actor orientation are welcome, the plethora of categorical variables and the complexity of the presentations (often spilling over into online appendixes) are of concern. Also, while all computerized statistical programs are vulnerable to abuse, the probability that some of the “canned” logistic regression programs will be used incorrectly seems high due to their complexity. But the chief concern regarding the dominance of logistic regression is that while the recent logistic regressions appear more sophisticated than their traditional OLS counterparts, it is not clear that they have provided enhanced explanatory power in terms of variance explained. In fact, logistic regression in some cases may have lowered the explanatory efficacy of regression, at least when interpreted in terms of explained variance.
The binary coding of dependent and independent variables can obviously lead to extreme attenuation and loss of explanatory power, as noted by Blalock (1979). One of the most undesirable properties of R 2 for any dichotomous analysis is that the dichotomous dependent variable is so attenuated that little variance exists to be explained and so R 2 is necessarily low. If nothing else, the large number of cases when no R 2 of any sort is reported is certainly a matter of concern, as it makes it very difficult to compare the adequacy of OLS regressions with the adequacy of logistic regressions.
In lieu of R 2 , users of logistic regression generally follow one of three strategies: (1) They do not report any sort of R 2 (Hollister 2004:670), relying solely on p values. The p values of logistic regression often are significant due (at least in part) to large sample size, such as Hollister’s (2004:669, sample N of 443,399 in table 1). While large sample sizes may not guarantee significant p values, they make them easier to obtain than with the smaller sample sizes previously used in many traditional sociological studies; (2) they report a “pseudo R 2 ” (see Hagle 2004), such as those reported by McLeod and Kaiser (2004:646) for their table 3, ranging in value from .017 to .112 (the highest reported in the article is .245 in table 5, p. 648); or (3) they report some other R 2 term, such as the Nagelkerke R 2 , as reported by Griffin (2004:551), in his table 4, with values of .065 and .079.
In the middle of the twentieth century, sociology relied on careful percentage analysis as the backbone of its quantitative methodology, augmented by relatively rudimentary statistics, such as measures of central tendency, correlation coefficients, and tests of significance such as chi-square. Although sociologists were aware of multivariate statistics such as factor analysis and multiple discriminant analysis, the onerous computation that these methods required before computerization limited their use.
With the advent of mainframe computers in the 1960s and 1970s, sociologists could go to their universitycomputing center and run a variety of multivariate statistical analyses. Thus, by 1974, OLS regression became the dominant method. A major problem with OLS regression was that it could accommodate only a single intervaldependent variable, and the independent variables had to be intervally measured as well, except for “dummy” variables. Thus, many important theoretical variables, such as religion, race, gender, and so on, could not be properly accommodated in the dominant regression model.
But by 2004, all had changed. The sea change to logistic regression facilitated the use of multiple regression, as one no longer needed to limit the analysis to interval or ratio dependent variables. Also, the dependent variable could be logged. The advantages of logistic regression are great. These advantages include the facilitation of multilevel analysis (such as use of the individual and country levels) and the ease with which data can be pooled so that many surveys are used and sample sizes are large. Logistic regression makes good use of existing data sets and does a much better job of longitudinal analysis than OLS. Furthermore, the published logistic regressions are replete with categorical variables that were previously missing from OLS regression.
While the advantages of logistic regression are obvious, it may be debatable whether the dominance of this technique indicates that theory and method have merged in an ideal fashion in contemporary sociology. There are several reasons why. First, much sociological theory is not stated in terms of the binary-coded dichotomies favored in logistic regression. While the prediction of dichotomies is certainly theoretically significant in some cases, it would not seem to match the general significance of predicting the full range of values in an interval or ratio variable. That is, why limit the analysis to predicting 1 or 0, when it is possible to predict age from birth to death. Second, since sociological theory is generally not written in terms of logged variables, it is difficult to interpret statistical analysis where the dependent variables are logged to normalize them.
In summary, the logistic regression analyses now dominating provide a number of benefits. These include, among others, advances in longitudinal analysis, in multilevel analysis, in the use of pooled data, in the presentation of more comparative models in each analysis, and in the presentation of more interaction analyses. But logistic regression sometimes appears to relinquish these gains by losing theoretical power when it is unable to provide impressive R 2 values. This is due in part to the excessive attenuation resulting from the widespread use of binarycoded dependent variables (often dichotomies).
Prospects for the 21st Century
The future for quantitative sociology will include the continued use of logistic regression. There also will be further developments in blockmodeling and also in longitudinal methods, including event history analysis. There will also be continued interest in multilevel techniques (Guo and Zhao 2000) as well as in agent-based or actor modeling (Macy and Willer 2002). There will also be increased interest in nonlinear analysis (Meeker and Leik 2000; Macy and Willer 2002). In addition, there will be continued advances in regression analysis in such areas as fixed effects regression, including Cox regression (Allison 2005) and spline regression (Marsh and Cormier 2001).
Davis (2001) writes, “In sum, I believe the seeming technical progress of logistic regression (and its cousins) is actually regressive” (p. 111). In another analysis of the logistic regression model, Davis writes,
In short, despite the trappings of modeling, the analysts are not modeling or estimating anything; they are merely making glorified significance tests. Furthermore, these are usually merely wrong or deceptive significance tests because . . . they usually work with such large Ns that virtually anything is significant anyway. (P. 109)
Davis recommends a return to path analysis, in part because it is easier to measure the success or failure of path analysis (p. 110).
Sociologists rely on logistic regression because the variables used are conducive to this technique. Davis (2001) also notes the shift within sociology from using psychology as a model to the present reliance on economics. He writes that in the 1950s psychology was the “alpha animal,” but now economics is a “Colossus” (p. 105). Quantitative researchers have long favored economic variables because they are easier to quantify. Furthermore, inequality research has benefited from the wide availability of economic coefficients such as the Gini (Lee 2005). Nevertheless, sociologists are now more likely to be citing Econometrica or The World Bank Economic Review, and the future influence of economics on sociology seems clear.
While the advantages of logistic regression are clear, there are other methods that deserve consideration as well. It is clear that sociologists will increasingly employ the methods of epidemiology, such as hazard and survival models and Cox regression (Allison 2005), and the methods and data sets of economics. But in addition, sociologists will undoubtedly continue to collect their own data sets while employing the OLS regression and path analysis models. They will also use relatively neglected techniques such as factor analysis, analysis of variance, analysis of covariance, multiple discriminate analysis, canonical correlation, and smallest space analysis.
Bibliography:
- Abell, Peter. 1971. Model Building in Sociology. New York: Schocken Books.
- Abell, Peter. 2004. “Narrative Explanation: An Alternative to Variable Centered Explanation.” Annual Review of Sociology 30:287–310.
- Allison, Paul D. 1984. Event History Analysis: Regression for Longitudinal Event Data. Beverly Hills, CA: Sage.
- Allison, Paul D. 2005. Fixed Effects Regression Methods for Longitudinal Data Using SAS. Cary, NC: SAS.
- Bailey, Kenneth D. 1973. “Monothetic and Polythetic Typologies and Their Relationship to Conceptualization, Measurement, and Scaling.” American Sociological Review 38:18–33.
- Bailey, Kenneth D. 1974a. “Interpreting Smallest Space Analysis.” Sociological Methods and Research 3:3–29.
- Bailey, Kenneth D. 1974b. “Cluster Analysis.” Pp. 59–128 in Sociological Methodology 1975, edited by D. R. Heise. San Francisco, CA: Jossey-Bass.
- Bailey, Kenneth D. 1994a. Typologies and Taxonomies: An Introduction to Classification Techniques. Thousand Oaks, CA: Sage.
- Bailey, Kenneth D. 1994b. Methods of Social Research. 4th ed. New York: Free Press.
- Bailey, Stanley R. 2004. “Group Dominance and the Myth of Racial Democracy: Antiracism Attitudes in Brazil.” American Sociological Review 69:728–47.
- Blalock, Hubert M., Jr. 1960. Social Statistics. New York: McGraw-Hill.
- Blalock, Hubert M., Jr. 1969. Theory Construction: From Verbal to Mathematical Formulations. Englewood Cliffs, NJ: Prentice Hall.
- Blalock, Hubert M., Jr. 1971. Causal Models in the Social Sciences. Chicago, IL: Aldine.
- Blalock, Hubert M., Jr. 1979. Social Statistics. 2d ed. New York: McGraw-Hill.
- Blalock, Hubert M., Jr. 1982. Conceptualization and Measurement in the Social Sciences. Beverly Hills, CA: Sage.
- Bridgman, Percy W. 1948. The Logic of Modern Physics. New York: Macmillan.
- Cole, Stephen, ed. 2001. What’s Wrong with Sociology? New Brunswick, NJ: Transaction.
- Coleman, James S. 1964. Introduction to Mathematical Sociology. New York: Free Press.
- Davis, James A. 2001. “What’s Wrong with Sociology.” Pp. 99–119 in What’s Wrong with Sociology, edited by S. Cole. New Brunswick, NJ: Transaction.
- Doreian, Patrick, Vladimir Batagelj, and Anuska Ferligoj. Generalized Blockmodeling. Cambridge, England: Cambridge University Press.
- Duncan, Otis D. 1966. “Path Analysis: Sociological Examples.” American Journal of Sociology 72:1–16.
- Durkheim, Émile. [1938] 1964. Rules of the Sociological Method. New York: Free Press.
- Edling, Christofer R. 2002. “Mathematics in Sociology.” Annual Review of Sociology 28:197–220.
- Fararo, Thomas J. 1973. Mathematical Sociology: An Introduction to Fundamentals. New York: Wiley.
- Fararo, Thomas J. 1989. The Meaning of General Theoretical Sociology: Tradition and Formalization. Cambridge, England: Cambridge University Press.
- Goode, William J. and Paul K. Hatt. 1952. Methods in Social Research. New York: McGraw-Hill.
- Griffin, Larry J. 2004. “‘Generations and Collective Memory’ Revisited: Race, Region, and Memory of Civil Rights.” American Sociological Review 69:544–57.
- Guo, Guang and Hongxin Zhao. 2000. “Multilevel Modeling for Binary Data.” Annual Review of Sociology 26:441–62.
- Hagle, Timothy M. 2004. “Pseudo R-squared.” Pp. 878–79 in The Sage Encyclopedia of Social Science Research Methods, 3, edited by M. Lewis-Beck, A. E. Bryman, and T. F. Liao. Thousand Oaks, CA: Sage.
- Harknett, Kristen and Sara S. McLanahan. 2004. “Racial and Ethnic Differences in Marriage after the Birth of a Child.” American Sociological Review 69:790–811.
- Hollister, Matissa N. 2004. “Does Firm Size Matter Anymore? The New Economy and Firm Size Wage Effects.” American Sociological Review 69:659–76.
- Iverson, Gudmund R. 2004. “Quantitative Research.” Pp. 896–97 in The Sage Encyclopedia of Social Science Research Methods, 3, edited by M. Lewis-Beck, A. E. Bryman, and T. F Liao. Thousand Oaks, CA: Sage.
- Jahoda, Marie, Morton Deutsch, and Stuart W. Cook. 1951. Research Methods in Social Relations. New York: Holt, Rinehart & Winston.
- Joyner, Kara and Grace Kao. 2005. “Interracial Relationships and the Transition to Adulthood.” American Sociological Review 70:563–81.
- Kemeny, John G. and J. Laurie Snell. 1962. Mathematical Models in the Social Sciences. New York: Blaisdell.
- Knoke, David and Michael Hout. 1974. “Social and Demographic Factors in American Political Party Affiliation, 1952–72.” American Sociological Review 39:700–13.
- Land, Kenneth C. 1968. “Principles of Path Analysis.” Pp. 1–37 in Sociological Methodology 1969, edited by E. F. Borgatta. San Francisco, CA: Jossey-Bass.
- Lazarsfeld, Paul F., ed. 1954. Mathematical Thinking in the Social Sciences. Glencoe, IL: Free Press.
- Lee, Cheol-Sung. 2005. “Income Inequality, Democracy, and Public Sector Size.” American Sociological Review 70:158–81.
- Lewis-Beck, Michael, Alan E. Bryman, and Tim F. Liao. 2004. The Sage Encyclopedia of Social Science Research Methods. Thousand Oaks, CA: Sage.
- Lundberg, George A. 1939. Foundations of Sociology. New York: Macmillan.
- Lundberg, George A. 1947. Can Science Save Us? New York: Longmans, Green.
- Macy, Michael W. and Robert Willer. 2002. “From Factors to Actors: Computational Sociology and Agent-Based Modeling.” Annual Review of Sociology 28:143–66.
- McKinney, John C. 1966. Constructive Typology and Social Theory. New York: Appleton-Century-Crofts.
- McLeod, Jane D. and Karen Kaiser. 2004. “Childhood Emotional and Behavioral Problems and Educational Attainment.” American Sociological Review 69:636–58.
- Marsh, Lawrence C. and David R. Cormier. 2001. Spline Regression Models. Thousand Oaks, CA: Sage.
- Meeker, Barbara F. and Robert K. Leik. 2000. “Mathematical Sociology.” Pp. 1786–92 in Encyclopedia of Sociology, edited by E. F. Borgatta and R. J. V. Montgomery. 2d ed. New York: Macmillan.
- Messner, Steven F., Eric P. Baumer, and Richard Rosenfeld. 2004. “Dimensions of Social Capital and Rates of Criminal Homicide.” American Sociological Review 69:882–903.
- Monette, Duane R., Thomas J. Sullivan, and Cornell R. DeJong. 2005. Applied Social Research. 6th ed. Belmont, CA: Brooks/Cole.
- Raftery, Adrian E. 2005. “Quantitative Research Methods.” Pp. 15–39 in The Sage Handbook of Sociology, edited by C. Calhoun, C. Rojek, and B. Turner. Thousand Oaks, CA: Sage. Rudner, Richard. 1966. The Philosophy of the Social Sciences. Englewood Cliffs, NJ: Prentice Hall.
- Stevens, S. S. 1951. “Mathematics, Measurement, and Psychophysics.” Pp. 1–49 in Handbook of Experimental Psychology, edited by S. S. Stevens. New York: Wiley.
- Torche, Florencia. 2005. “Social Mobility in Chile.” American Sociological Review 70:422–49.
- Tubergen, Frank van, Ineke Maas, and Henk Flap. 2004. “The Economic Incorporation of Immigrants in 18 Western Societies: Origin, Destination, and Community Effects.” American Sociological Review 69:704–27.
- Uggen, Christopher and Amy Blackstone. 2004. “Sexual Harassment as a Gendered Expression of Power.” American Sociological Review 69:64–92.
- Weber, Max. 1949. The Methodology of the Social Sciences. Translated by E. A. Shils and H. A. Finch. Glencoe, IL: Free Press.
- White, Harrison C. 1963. An Anatomy of Kinship: Mathematical Models for Structures of Cumulated Roles. Englewood Cliffs, NJ: Prentice Hall.
- White, Harrison C. 1970. Chains of Opportunity: System Models of Mobility in Organizations. Cambridge, MA: Harvard University Press.
- Yamaguchi, Kazuo. 1983. “Structure of Intergenerational Occupational Mobility; Generality and Specificity in Resources, Channels, and Barriers.” American Journal of Sociology 88:718–45.
ORDER HIGH QUALITY CUSTOM PAPER

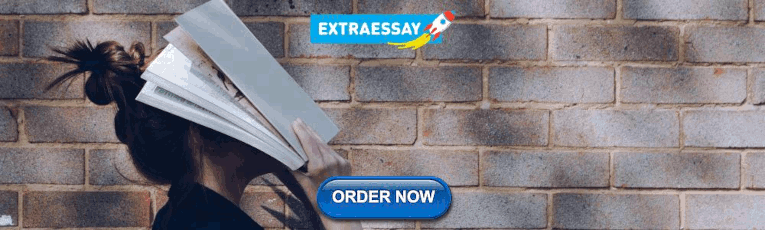
IMAGES
VIDEO
COMMENTS
In sociology, quantitative research methods can be used to study either inherently numeric things, such as population data, or data converted to numeric values, such as demographics (e.g ...
This resource is intended as an easy-to-use guide for anyone who needs some quick and simple advice on quantitative aspects of research in social sciences, covering subjects such as education, sociology, business, nursing. If you area qualitative researcher who needs to venture into the world of numbers, or a student instructed to undertake a quantitative research project despite a hatred for ...
Founded in 1947, AAPOR is an association of individuals who share an interest in survey research, qualitative and quantitative research methods, and public opinion data. Members come from academia, media, government, the nonprofit sector, and private industry. Meetings are held in even-numbered years. American Sociological Association (ASA).
One's theoretical starting point may give rise to research questions. For example, Marxist-leaning sociologists of education have been interested in researching the effects of cultural capital on educational achievement. Hypothesis Some quantitative research derives a hypothesis from the theoretical starting point to test. A hypothesis is a ...
Quantitative Methods in Sociology Spring 2019 Meetings: Mondays and Wednesdays, 10 ... Catalog Description Introduces quantitative analysis in social research, including principles of research design and the use of empirical evidence, particularly from social surveys. ... to the examples presented in lecture, the synthesis sessions, and prior ...
Example: Danni, a sociology Master's student. ... quantitative research advances universal epistemological principles that are nomothetically applied to reality (Howitt, 2019;Sarantakos, 2013). ...
Quantitative research is the opposite of qualitative research, which involves collecting and analyzing non-numerical data (e.g., text, video, or audio). Quantitative research is widely used in the natural and social sciences: biology, chemistry, psychology, economics, sociology, marketing, etc. Quantitative research question examples
Quantitative social science is an interdisciplinary field encompassing a large number of disciplines, including economics, education, political science, public policy, psy-chology, and sociology. In quantitative social science research, scholars analyze data to understand and solve problems about society and human behavior. For example,
SO5041: Quantitative Research Methods. Brendan Halpin, Sociology Autumn 2022/3. Contents. Contents Unit 0: Course Outline Unit 1: Introducing Quantitative Social Research Unit 2: Surveys, Questionnaires and Sampling Unit 3: Numbers as Information - Data Unit 4: Bivariate analyses Unit 5: Spread Unit 6: Sampling Unit 7: Distributions Unit 8 ...
Soc 156: Quantitative Methods in Sociology. Introduces quantitative analysis in social research, including principles of research design and the use of empirical evidence, particularly from social surveys. Descriptive and inferential statistics, contingency table analysis, and regression analysis. Emphasis on analysis of data and presentation ...
Concepts are the building blocks of theory, and are the points around which social research is conducted. Concepts are closely related to the main sociological perspectives, and some of the main concepts developed by different perspectives include: Functionalism - social integration and anomie. Marxism - social class and alienation.
Field Research. The work of sociology rarely happens in limited, confined spaces. Rather, sociologists go out into the world. They meet subjects where they live, work, and play. Field research refers to gathering primary data from a natural environment. To conduct field research, the sociologist must be willing to step into new environments and ...
Social Facts. The first rule of Positivist methodology is to consider social facts as things which means that the belief systems and customs of the social world should be considered as things in the same way as the objects and events of the natural world. According to Durkheim, some of the key features of social facts are: they exist over and ...
Probability Sampling. Unlike nonprobability sampling, probability sampling refers to sampling techniques for which a person's (or event's) likelihood of being selected for membership in the sample is known. You might ask yourself why we should care about a study element's likelihood of being selected for membership in a researcher's sample.
Example: Interviewing the victims of a natural disaster to gather a range of emotional responses. Quantitative Research. Numbers! Collected as numerical data or converted into numerical data and examined using statistical methods of analysis. ... Experimental Research is a sub-type of research in sociology. It may utilize the same methods as ...
The course focuses specifically on how to determine, apply, and interpret statistical methods appropriate for answering a sociological research question given a particular set of data. Basic probability theory is introduced as a building block of statistical reasoning, and a variety of commonly-used statistical methods are covered in the course ...
Abstract. Quantitative social science has long been dominated by self-consciously positivist approaches to the philosophy, rhetoric and methodology of research. This article outlines an alternative approach based on interpretive research methods. Interpretative approaches are usually associated with qualitative social science but are equally ...
1. Quantitativ e Anal ysis in Cultural Sociol ogy. Why It Should Be Done, How It Ca n Be Done. Dick Houtman and Peter Achterberg. Sociology and Culture: An Unhappy Marriage. Sociology took shape ...
Definition of Quantitative Research (noun) Using statistical analysis to quantify and measure social phenomenon, seeking to identify where possible causal relationships, then reporting the findings numerically.Examples of Quantitative Research. statistical analysis; surveys; trend study; Quantitative Research Pronunciation
Research methods and analysis of sociology dealt with techniques to obtain information in a vivid form. Research is carefully observing patterns for searching new facts or terms in any kind of subject. ... Evaluation Schedules- Quantitative analysis for example data collection is a primary objective of this schedule. For example, if a company ...
When collecting and analyzing data, quantitative research deals with numbers and statistics, while qualitative research deals with words and meanings. Both are important for gaining different kinds of knowledge. Quantitative research. Quantitative research is expressed in numbers and graphs. It is used to test or confirm theories and assumptions.
Sociology From a Quantitative Perspective. A bachelor of science in sociology often takes a quantitative approach to sociological study. According to Study.com, "Quantitative research involves the collecting and analysis of data that is quantifiable.". Focusing on analytics and statistics, a BS in sociology can prepare you for a career as a ...
History of Sociological Quantification. Quantitative reasoning is widely applied in the discipline of sociology and quantification aids sociologists in at least seven main research areas: quantitative modeling, measurement, sampling, computerization, data analysis, hypothesis testing, and data storage and retrieval.